- Open access
- Published: 14 October 2022
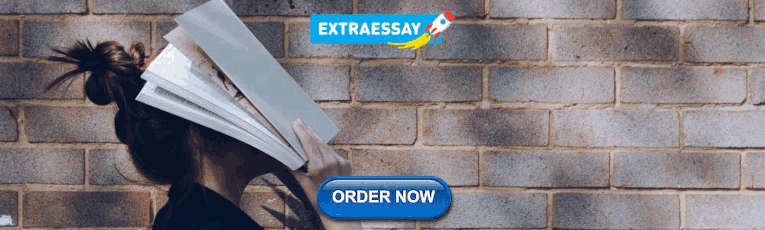
The effectiveness of case management for cancer patients: an umbrella review
- Nina Wang 1 , 2 ,
- Jia Chen 3 ,
- Wenjun Chen ORCID: orcid.org/0000-0001-5398-8508 4 , 5 ,
- Zhengkun Shi 1 ,
- Huaping Yang 1 ,
- Peng Liu 6 ,
- Xiao Wei 7 ,
- Xiangling Dong 6 ,
- Chen Wang 3 ,
- Ling Mao 8 &
- Xianhong Li 3
BMC Health Services Research volume 22 , Article number: 1247 ( 2022 ) Cite this article
2437 Accesses
24 Citations
1 Altmetric
Metrics details
Case management (CM) is widely utilized to improve health outcomes of cancer patients, enhance their experience of health care, and reduce the cost of care. While numbers of systematic reviews are available on the effectiveness of CM for cancer patients, they often arrive at discordant conclusions that may confuse or mislead the future case management development for cancer patients and relevant policy making. We aimed to summarize the existing systematic reviews on the effectiveness of CM in health-related outcomes and health care utilization outcomes for cancer patient care, and highlight the consistent and contradictory findings.
An umbrella review was conducted followed the Joanna Briggs Institute (JBI) Umbrella Review methodology. We searched MEDLINE (Ovid), EMBASE (Ovid), PsycINFO, CINAHL, and Scopus for reviews published up to July 8th, 2022. Quality of each review was appraised with the JBI Critical Appraisal Checklist for Systematic Reviews and Research Syntheses. A narrative synthesis was performed, the corrected covered area was calculated as a measure of overlap for the primary studies in each review. The results were reported followed the Preferred reporting items for overviews of systematic reviews checklist.
Eight systematic reviews were included. Average quality of the reviews was high. Overall, primary studies had a slight overlap across the eight reviews (corrected covered area = 4.5%). No universal tools were used to measure the effect of CM on each outcome. Summarized results revealed that CM were more likely to improve symptom management, cognitive function, hospital (re)admission, treatment received compliance, and provision of timely treatment for cancer patients. Overall equivocal effect was reported on cancer patients’ quality of life, self-efficacy, survivor status, and satisfaction. Rare significant effect was reported on cost and length of stay.
Conclusions
CM showed mixed effects in cancer patient care. Future research should use standard guidelines to clearly describe details of CM intervention and its implementation. More primary studies are needed using high-quality well-powered designs to provide solid evidence on the effectiveness of CM. Case managers should consider applying validated and reliable tools to evaluate effect of CM in multifaced outcomes of cancer patient care.
Peer Review reports
Cancer ranks as one of the leading causes of premature death among population around 30–69 years old across 134 countries [ 1 ], and the global incidence of cancer is about to reach 30.2 million new cases and 25.7 million deaths by 2040 [ 2 ]. Earlier detection and diagnosis, and development of diverse cancer treatments have increased the survival rate of cancer patients. According to Quaresma et al. [ 3 ], the cancer survival in the UK has doubled over the last 40 years alongside the advancement in cancer diagnosis and treatment. However, number of challenges exist in the current cancer care all over the world. Many cancer patients oftentimes receive a series of long-running and exhausting multi-modal treatments and experience descent in psychological, physical and social functioning, which have a significant negative impact on their quality of life (QoL) [ 4 , 5 ]. In addition, the significant healthcare spending and productivity losses of cancer patients lead to a heavy patient economic burden, which is another substantial issue with cancer care [ 6 ]. A systematic approach is needed to mobilize and deliver appropriate resources, provide accessible, safe, and well-coordinated care for cancer patients received stressful treatments and shouldered heavy economic burden [ 7 ].
Case management (CM) is defined by the Case Management Society of America (CMSA) as “a collaborative process of assessment, planning, facilitation, care coordination, evaluation, and advocacy for options and services to meet an individual’s and family’s comprehensive health needs through communication and available resources to promote quality, cost-effective outcomes” (P. 11) [ 8 ]. According to the definition, CM is designed to use resources effectively to improve the quality of treatments, patient care services, and QoL of patients while reducing the relevant healthcare costs.
With the worldwide utilization of CM in cancer patient care, studies examining the effect of CM in improving patient-related outcomes or healthcare service use outcomes have been skyrocketing. Numbers of systematic reviews and meta-analyses have been published to synthesis the effectiveness of CM in recent years and often arrive at discordant conclusions. For example, Joo et al. [ 9 ] retrieved and synthesised results from nine experimental studies and found that CM effectively improved patients’ QoL and symptom management. While Aubin et al. [ 10 ] reported equivocal effect on both QoL and symptom management. Chan et al. [ 11 ] reported that four of the five randomized controlled trials showed insignificant impact of CM on patients’ QoL. The inconsistent evidence on the impact of CM may confuse or mislead the future case management development and relevant policy making. Considering the exist of several systematic reviews and research synthesis available to inform the application of case management for cancer patient care improvement, umbrella review could now be undertaken to compare and contrast published reviews and to highlight the consistent or contradictory findings around the effect of CM on manifold aspects of cancer patient care [ 12 ]. Thus, the current review was conducted to 1) synthesis systematic reviews that assess the effects of CM on cancer patient outcomes (e.g., QoL, functioning status, symptom management, satisfaction, etc.) and health care utilization outcomes (e.g., cost, hospital admissions, length of stay, treatment received compliance, etc.), 2) summarize measurement used in evaluating patient outcomes and health care utilization outcomes.
This umbrella review followed the Joanna Briggs Institute (JBI) Umbrella Review (UR) methodology [ 12 ] and adhered to the Preferred Reporting Items for Overviews of systematic reviews (PRIO) checklist (see Additional file 1 ) [ 13 ]. This review has been registered with the Open Science Framework ( https://doi.org/10.17605/OSF.IO/7YQAP ).
Study searching methods
We performed literature search in five databases including MEDLINE (Ovid), EMBASE (Ovid), PsycINFO, CINAHL, and Scopus from inception to July 2022. Ethical approval and patient consent were not necessary since all analyses were based on previously published articles. The searching strategies in all five databases were developed with the help of a health science librarian. See Additional file 2 for the searching strategy and results in MEDLINE (Ovid). The studies were selected using the following inclusion and exclusion criteria.
Inclusion and exclusion criteria
Individuals diagnosed with any type of cancer at any cancer stages (early to advanced). Reviews targeted on people with no specified cancer diagnose were excluded.
Intervention
Case management interventions targeted on cancer patients. Case management is defined as a “collaborative process of assessment, planning, facilitation, care coordination, evaluation, and advocacy for options and services to meet an individual’s and family’s comprehensive health needs through communication and available resources to promote quality, cost-effective outcomes” [ 8 ]. Only reviews in which the effectiveness of CM as defined above was analyzed separately from other interventions were considered.
Individuals in comparison groups received “treatment as usual” (TAU). TAU may include various interventions called “standard of care,” “usual care,” or “standard treatment,” but generally refers to treatment as it is commonly provided. Only studies that compared case management with “TAU” were selected.
Patient outcomes (e.g., quality of life, symptom management, functioning status), health care utilization outcomes (e.g., cost, hospital admissions, length of stay), etc.
Acute care hospitals and primary care settings (e.g., long-term care, nursing homes, community care services). Hospital was defined as any department of internal medicine or surgery as well as unspecified hospital settings.
Study design
Systematic review/meta-analysis that only included quantitative studies. We excluded studies full-texts unavailable online.
Study selection
All retrieved studies were imported into Covidence systematic review software [ 14 ] and the duplicates were removed. Then, titles and abstracts were independently assessed by two researchers (XW and XD) according to the inclusion criteria. After that, the full texts of the selected abstracts were obtained and reviewed by the same two researchers (XW and XD) independently. The reference list of included studies was reviewed and searched for additional studies. Any disagreement between the two researchers were resolved through consultation with a senior researcher (PL).
Quality appraisal for included reviews
Two reviewers (NW and LM) independently assessed the methodological quality of the individual studies using the JBI Critical Appraisal Checklist for Systematic Reviews and Research Syntheses [ 15 ]. The tool aims to determine the extent to which the review has addressed the possibility of bias in its design, conduct and analysis [ 15 ]. It consists of 11 criteria scored as yes, no, unclear, or not applicable. We adopted a scoring system used in previously published systematic reviews [ 16 , 17 ]. For each article, a rating score was derived by taking the number obtained in the quality rating and dividing it by the total number of possible points allowed, giving each manuscript a total quality rating between 0 and 1. Studies were then classified as low (0–0.25), low-moderate (0.26–0.50), moderate (0.51–0.75), or high (0.76–1.0).
Data extraction
We developed the data extraction form based on the research questions, and extracted following information: characteristics of included reviews such as publication year range, whether conducted meta-analysis or not, type of cancer patients, age of population, type and number of primary studies included; intervention names, components, and duration; outcomes and evaluation tools used; author’s conclusions and interpretations. Two researchers (NW and LM) extracted data independently from all included articles into an Excel spreadsheet and another researcher (XL) verified it for accuracy.
Data synthesis
We were unable to statistically pool outcomes due to the heterogeneity of outcomes of the included reviews. Therefore, we conducted a narrative synthesis [ 18 ] of the numerical data of individual studies outcomes. The studies were summarized and synthesised by two reviewers (NW and ZS) independently and double checked by a third author (HY). Following the JBI UR methodology [ 12 ], we used a summary table to present clear, specific, and structured results from the selected reviews, and then synthesised these results to identify broad conclusions. To summarized information about the interventions we coded data into features, components and delivery strategies, and inductively developed themes within each domain as they emerged from the studies. As suggested by Li and colleagues [ 19 ], we grouped outcomes into: global QoL of patients, functional status (i.e. physical, cognitive, emotional, role, social), symptom management, cost, hospital (re)admission, length of stay, treatment received compliance, provision of timely treatment.
For clarity the term ‘primary studies’ refers to the articles found within the included reviews. As several primary studies are included in more than one review, the overall results and conclusions of an overview can be biased. To assess this bias, the degree of overlap between reviews was calculated with the Corrected Covered Area (CCA) method. The details of the CCA calculation have been described by Pieper and colleagues [ 20 ] elsewhere. A CCA score of less than 5% is regarded as a slight overlap, 5–9.9% as moderate overlap, 10–14.9% as high overlap and over 15% as a very high level of overlap. This measure has been validated in which the number of overlapped primary publications has a strong correlation with the CCA [ 21 ].
Search outcome
As shown in Fig. 1 , our search strategy generated 804 potentially relevant records. Upon removing the duplicates, 582 studies screened by title and abstract, 16 were identified for full text screening. We excluded eight of the 16 studies for the following reasons: no independent analysis on the effect of case management ( n = 6), or conference abstract ( n = 2). The eight remaining systematic reviews were selected and assessed for methodological quality. In total, all the eight reviews included 57 primary studies, among which 12 were duplicated included in two or three reviews. Forty-one of the 57 primary studies were randomized controlled trials (see Additional file 3 for included primary studies).
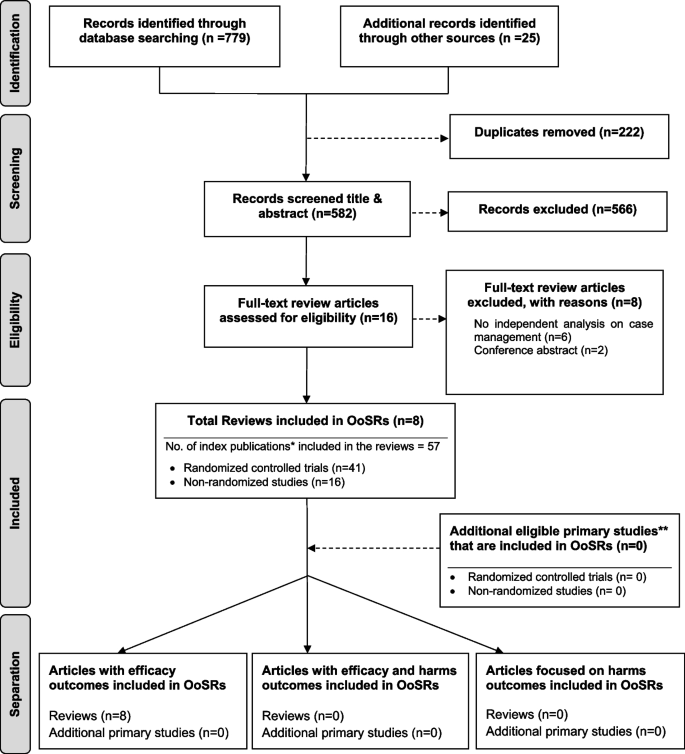
Flow chart for umbrella review. *Index publication is the first occurrence of a primary publication in the included reviews. **Additional eligible primary studies that had not been initially indentified by the search of the relevant reviews or obtained by updating the search of the included reviews
Methodological quality assessment
The quality assessment scores are presented in Table 1 . Only one review was rated as moderate because not clarify whether two or more reviewers independently assessed the quality of included primary studies, and did not report the methods to minimize errors in data extraction or publication bias. The other seven reviews were rated as high quality. Despite rated as strong, the seven reviews still companied with one or two issues on the assessment of heterogeneity, search strategy, and recommendations for policy and/or practice.
Characteristics of included studies
Table 2 presents a descriptive summary of characteristics of the eight systematic reviews [ 9 , 10 , 11 , 19 , 23 , 24 , 25 , 26 ]. The eight reviews aimed to identify evidence of the effectiveness of CM on cancer patients. Three of the studies were a systematic review with meta-analysis [ 10 , 25 , 26 ]. Five of the eight reviews adhered to the PRISMA statement [ 11 , 19 , 24 , 25 , 26 ], two adopted Cochrane systematic review methodology [ 9 , 10 ].
The eight reviews were published between 2008 and 2021, the primary studies in the reviews were published between 1983 and 2018. The number of primary studies regarding to CM included in each review ranged from three to 20. Five of the eight reviews included only randomized controlled trials (RCTs), the remaining reviews included a combination of study designs that involved RCTs, quasi-experimental and non-experimental studies (e.g., cohort study). The age of review participants ranged from 7 to 97 years and mean ages range from 48.63 to 66.31 years, which covers populations from children to elders. The total number of participants in each review ranged from 327 to 9601. Seven of the eight reviews included primary studies targeted on multiple types of cancer including breast, lung, colorectal, cervical, ovarian, prostate, gastric, hepatocellular, etc. Most of the primary studies included in the eight reviews were conducted in the United States, and there were also studies conducted in Canada, Australia, Europe (i.e., Germany, UK, Turkey, Switzerland, Denmark, Switzerland, Sweden, Norway, Netherlands) and East Asia (i.e., Hong Kong, Taiwan, South Korea, and Malaysia).
CM interventions
As shown in Table 2 , three studies reviewed trials of nurse-led CM interventions [ 9 , 25 , 26 ], two reviewed CM-like interventions that not termed as ‘CM’ while meet the CM definition by the CMSA [ 8 , 23 , 24 ]. Only one study reviewed CM focus solely on skill-training or symptom management [ 19 ]. All studies reviewed trials that facilitated the CM in a multidisciplinary collaboration approach. The duration of CM ranged from 4 days to 5 years. We presented the feature, components and delivery strategies of CM interventions for cancer patients in Fig. 2 by summarizing descriptions in each review. Congruent with the components defined by CMSA [ 8 ], all CM interventions included patient assessment, supportive services such as information and emotion support, care coordination by conducting education, consultation, and in-person, telephone or online coaching for regular follow-up. One critical component of CM interventions for cancer patients is the provision of palliative care. Control groups (CGs) of all studies reviewed in the reviews received usual treatment of care.
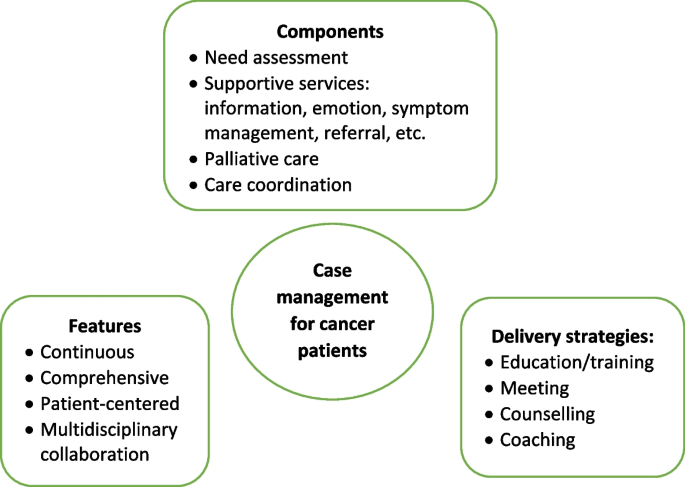
Features, components, and delivery strategies of case management for cancer patient care
Corrected Covered Area (CCA)
Table 3 presents the CCA for each outcome and as a whole. Overall, primary studies had a slight overlap across the eight reviews (CCA = 4.5%). In addition, no overlapping of primary studies was found for six of the 16 outcomes, including self-efficacy, psychological function, hospital (re)admissions, length of stay, and provision of timely treatment. Only one outcome (i.e., symptom management) showed slight overlap (0.7%). The CCA for other five outcomes (i.e., global QoL, physical function, role function, patient satisfaction, cost) evaluated by more than 2 reviews were between 5 to 9.9%, indicated a moderate overlap. The CCA for survivor status, cognitive function, emotional function, and treatment received compliance were over 10%.
Measurement used
Table 4 presents the quantitative measurement used in primary studies. As shown in Table 4 , studies investigated global QoL using different QoL-related scales, among which Functional Assessment of Cancer Therapy (FACT) (used in 15 primary studies) were most frequently applied, followed by the European Organisation for Research and Treatment of Cancer Core Quality of Life Questionnaire 30 (EORTC QLQ-C30) (used in 11 primary studies), and short form health survey (i.e., SF-8, SF-12, SF-36) (used in 10 primary studies). Different types of FACT tool were used according to the cancer types. For example, FACT-G was used for general cancer patients assessment, and FACT-B was used to evaluate breast cancer-related QoL. For the assessment of overall symptom management, SF-36 and Symptom Distress Scale (SDS) were used most frequently (used in four primary studies each). Different dimensions of SF-36 were also applied to evaluate other outcomes such as physical, emotional, and social function. Hospital Anxiety and Depression Scale (HADS) was the top employed tool in measuring the psychological function of patients. Patients’ sick leave days and the number of patients return to work were top employed metrics to evaluate the role function of patients. No unified tools were utilized to assess patient satisfaction towards the CM and majority of the primary studies used self-developed questionnaires.
Effect of CM on patient and health care utilization outcomes
The main outcomes from the seven systematic reviews are presented and summarized in Table 5 . Seven of the eight reviews reported the effects of case management on patients’ global QoL and showed mixed findings. Around half (49%, 19/39) of the primary studies included in the seven reviews reported significant positive impact of CM on global QoL. As for the functional status, there was a strong concordance among primary studies regarding the effectiveness of CM in improving cognitive function (e.g., uncertainty, health perceptions) (89%, 8/9); Equivocal effects were reported on psychological (e.g., patient anxiety, depression), physical (e.g., arm function), role function (e.g., sick leave days, patients returning to work), emotional (e.g., mood) and social function (e.g., social support) [ 9 , 11 , 26 ]. The findings regard to symptom management were more positive, with 75% (18/24) primary studies included in seven reviews revealed significant positive impact of CM on symptom severity and symptom distress decrease of pain, nausea, fatigue, discomfort, etc. Three of the four primary studies in two reviews [ 9 , 11 ] showed no significant influence of CM on patients’ self-efficacy. Wulff et al. [ 23 ] and Aubin et al. [ 10 ] reported mixed findings on the impact of CM on survivor status, with four of the six primary studies reported significant positive impact. The effect of CM on patient satisfaction was reported in five reviews and showed mixed results.
Of the eleven primary studies reported cost, only one controlled before-and-after study in Joo et al.’s [ 9 ] review reported significant impact on monthly cancer-related medical costs. The evidence concerning patients’ length of stay yielded no significant findings. Overall significant positive effect was reported on hospital (re)admission (e.g., inpatient and ICU admission rate), treatment received compliance (e.g., therapy acceptance or completion rate), and provision of timely treatment.
This umbrella review is the first to summarize the results of systematic reviews that synthesised the evidence on the effectiveness of CM on cancer patient outcomes and relevant health care utilization. Most reviews (7/8) showed a high methodological quality. Different tools were used to measure the effect of CM on the same outcome. The evidence regards to the effectiveness of CM is mixed. The summarized results revealed that CM was more likely to improve symptom management, cognitive function, hospital (re)admission, treatment received compliance, and provision of timely treatment for cancer patients. Overall equivocal effect was reported on cancer patients’ global QoL, psychological, physical, role, emotional and social function, self-efficacy, survivor status, and patient satisfaction.
No universal tools were used to measure improvement of each outcome in the CM group compared with the control group, making it challenging to conduct a meta-analysis of studies results [ 22 , 27 ]. This is a common issue faced the included reviews. Five of the eight reviews failed to conduct meta-analysis due to the heterogeneity [ 9 , 11 , 19 , 23 , 24 ]. Joo and Huber [ 22 ] conducted a review of reviews on the effect of CM on health care utilization outcome of chronic illness patients, they recognized the same problem and suggested using valid and standardized tools to minimize the differences in measurements. Despite various tools used, our review showed that FACT, EORTC QLQ-C30, and short form health survey (i.e., SF 36, SF 12, and SF 8) were most frequently applied to measure the effect of CM on the global QoL of cancer patients. These tools were also used in evaluating specific dimensions of QoL such as psychological, physical, emotional, and social function. This aligned with previous reviews [ 28 , 29 ] that found FACT and EORTC QLQ-C30 were the most common and well developed QoL instruments in cancer patients. FACT-G is considered appropriate for use with any types of cancer patients [ 30 ]. It is a 27-item tool that includes four primary QoL domains: physical well-being, social/family well-being, emotional well-being, and functional well-being [ 31 ]. Other versions of FACT (FACT-B [ 32 ], FACT-L [ 33 ] and FACT-E [ 34 ]) for specific type of cancer patients were developed by incorporating the four dimensions of FACT-G with additional cancer type-specific questions. EORTC QLQ-C30 was another type of QoL assessment tools for cancer patients specifically. It was developed by Aaronson et al. [ 35 ] and contains four domains: physical, emotional, cognitive and social functions, and a higher score indicates better QoL. The Short Form Health Survey is the most commonly used measure in evaluating QoL domains of patients suffering from a wide range of medical conditions [ 36 ]. Research found it provides reliable and valid indication of general health among cancer patients [ 37 , 38 ].
QoL is the most frequently evaluated outcome in our review with 39 primary studies in seven reviews reported the global QoL of cancer patients. Joo et al. [ 9 ] found that CM interventions improved QoL of cancer patients. Yin and colleagues [ 24 ] revealed that cancer patients achieved better physical and psychological condition through symptom management, needs assessment, direct referrals, and other services in CM. However, summarized results in our review show that the CM had equivocal effect on cancer patients’ global QoL and dimensions including psychological, physical, role, emotional and social function. Cognitive function is the only dimension showed positive change. Despite CM interventions share similar definitions and principles [ 8 ]. It is hard to foresee which aspect(s) of CM interventions contribute to certain effects due to their comprehensiveness [ 24 ]. Yin et al. [ 24 ] argued that the control group may receive a higher quality treatment than planned usual care since all the participants were not blinded and they have been informed about the aim of the study. Indicating a more rigorous design and evaluation is needed to avoid this information bias.
In the meantime, included reviews claimed that few primary studies reported enough details about CM interventions, including model used [ 10 , 11 ], dose and intensity [ 9 , 19 , 24 ], interventionist qualifications [ 11 ], protocol or manual used [ 9 , 23 ], and fidelity [ 23 ]. Particularly, the COVID-19 pandemic has considerable influence on the care delivery for cancer patients. For example, the more frequently utilization of remote patient monitoring technologies that incorporate community resources, primary care and allied health disciplines, as well as clinics to keep cancer patients away from acute care hospitals as much as possible [ 39 ]. Many of these changes have been integrated within routine case management for cancer care during the pandemic [ 39 ]. It is well-needed to report how those CM intervention were conducted follow standard reporting guidelines, in order to provide recommendation for future research.
Our review showed that CM is likely to improve the symptom management. Eighteen of the 24 included primary studies reported positive effect of CM on symptom management, including decrease symptom distress or severity of fatigue, pain, nausea, and vomiting. The same positive effect on symptom management was also revealed in other types of patients. Joo and colleagues [ 40 ] found that CM reduced substance use and significantly influenced abstinence rates among populations experienced substance disorders. Reviews by Stokes et al. [ 27 ] and Welch et al. [ 41 ] revealed positive effect on symptom release among people with long-term conditions and diabetes patients, respectively. The multidisciplinary collaboration approach adopted [ 10 ], and availability of professional support post-hospitalization [ 9 , 41 ] in CM might contribute to the improvement of symptom management. Specifically, multidisciplinary team involves physicians, nurses, and aligned healthcare professionals provides throughout and multifaced symptom assessment and management [ 10 ]. In addition, CM programs continuously follow up and advocate for patients’ concerns [ 8 ]. Specifically, case managers are available to patients 24 hours a day by phone call even after discharged, providing opportunity for immediate professional guidance on symptom management [ 9 ].
As for other patient outcomes, there is insufficient evidence of effect on self-efficacy and survivor status of cancer patients. Only three and four primary studies in total reported these two outcomes, respectively. Eleven primary studies in five reviews reported patient satisfaction and showed mixed results. Inconsistent results were found in a review of reviews by Buja et al. [ 7 ] which concluded strong evidence of CM improving satisfaction of patients with long term condition. In agreement with Joo and Huber’s [ 25 ] review, we found that CM favorably affect healthcare utilization outcomes such as treatment received compliance, hospital (re)admission, and provision of timely treatment. While the strength of the evidence was limited either by the high level of primary studies overlapping (CCA) (i.e., treatment received compliance, CCA = 13.3%) or the small number of studies reported certain outcomes (i.e., hospital admission, provision of timely treatment). Notably, the summarized results from included reviews conclude that despite theoretical benefits [ 8 ], in practice there is only slight evidence of benefits on reduction in the cost of care for cancer patients participated in CM interventions.
We provide some recommendations for future research based on the summarized results: 1) Future research should clearly describe details of CM intervention and its implementation, including theoretical underpinnings, dose and intensity, interventionist qualifications, protocol or manual used, fidelity, etc. In that way these details can be included in future systematic reviews, and effectiveness of individual elements of the intervention can be examined [ 27 ]. We recommend use standard guidelines to help organize the CM intervention reporting. For example, the Template for Intervention Description and Replication (TIDeiR) is one of the most popular guidelines that could be used to report the full breadth of CM interventions: from intervention rationale to assessments of treatment adherence and fidelity [ 42 ]. 2) More rigorous trials are needed to evaluate the effectiveness of CM. 3) Studies should also explore the barriers to and facilitators of CM implementation across various types of cancer patients at different stages, providing evidence for conducting successful CM implementation in the future.
Strengths and limitations
We conducted an umbrella review instead of a meta-analysis due to the heterogeneity of review outcomes. Although an umbrella review can only show the tendency or direction of the effect of CM rather than providing the magnitude or significance level of influence [ 12 ], the current evidence on the effect of CM in cancer patients was comprehensively summarized. There were some challenges when conducting the review. First, the quality of the umbrella reviews was greatly affected by the quality of the original reviews [ 12 ]. In this study, we confirmed that the quality of the original reviews were mostly high as assessed by the JBI Critical Appraisal Checklist [ 15 ]. Second, if the primary studies were included in several reviews, they may produce bias related to overlapping effects [ 20 ]. By calculating the CCA, we showed that 75% (12/16) of the individual outcomes had no to moderate overlapping of primary studies between included reviews, revealing that these results from each review were relatively independent. Cautious are needed on the summarized evidence regards to the effect of CM on survivor status, cognitive function, emotional function, and treatment received compliance because of the high overlapping (CCA > 10) between the reviews reported those outcomes.
There are limitations in our review. The first limitation concerns that the searching was limited to English-language articles and did not access unpublished papers. Second, as suggested by the JBI UR methodology [ 12 ], we did not assess the quality of evidence from included reviews, it increased the uncertainty of the review findings.
Effective CM aims to influence the health care delivery system in improving the health outcomes of cancer patients, enhancing their experience of health care, and reducing the cost of care. Our review found mixed effects of CM reported in cancer patient care. The summarized results revealed that CM was likely to improve symptom management for cancer patients. We also found CM has the tendency to enhance cancer patients’ experience of health care such as reducing hospital (re)admission rates, improving treatment received compliance and provision of timely treatment. Only slight evidence of benefits was reported on reducing the cost of care for cancer patients. Overall, more rigorous designed primary studies are needed to demonstrate the effects of CM on cancer patients and explore the elements of effective CM interventions.
Availability of data and materials
All data generated or analysed during this study are included in this published article and its supplementary information files.
Abbreviations
Corrected Covered Area
Control groups
- Case management
Case Management Society of America
European Organization for Research and Treatment of Cancer Core Quality of Life Questionnaire 30
Functional Assessment of Cancer Therapy - Breast Cancer
Functional Assessment of Cancer Therapy- Esophagus
Functional Assessment of Cancer Therapy- General
Functional Assessment of Cancer Therapy Scale-Lung
- Quality of life
Hospital Anxiety and Depression Scale
Joanna Briggs Institute
Preferred Reporting Items for Systematic Reviews and Meta-Analyses
Randomized controlled trials
Symptom Distress Scale
Medical Outcomes Study 8-item short form health survey
Medical Outcomes Study 12-item short form health survey
Medical Outcomes Study 36-item short form health survey
Treatment as usual
Ferlay J, Ervik M, Lam F, Colombet M, Mery L, Piñeros M, et al. Global cancer observatory: Cancer today: International Agency for Research on Cancer; 2020. https://gco.iarc.fr/today .
Ferlay J, Ervik M, Lam F, Colombet M, Mery L, Piñeros M, et al. Global Cancer observatory: Cancer tomorrow: International Agency for Research on Cancer; 2020. https://gco.iarc.fr/tomorrow .
Quaresma M, Coleman MP, Rachet B. 40-year trends in an index of survival for all cancers combined and survival adjusted for age and sex for each cancer in England and Wales, 1971-2011: a population-based study. Lancet. 2015;385:1206–18. https://doi.org/10.1016/S0140-6736(14)61396-9 .
Article PubMed Google Scholar
Yabroff KR, Dowling EC, Guy GP, Banegas MP, Davidoff A, Han X, et al. Financial hardship associated with cancer in the United States: findings from a population-based sample of adult cancer survivors. J Clin Oncol. 2016;34:259–67. https://doi.org/10.1200/JCO.2015.62.0468 .
Yan AF, Stevens P, Holt C, Walker A, Ng A, McManus P, et al. Culture, identity, strength and spirituality: a qualitative study to understand experiences of African American women breast cancer survivors and recommendations for intervention development. Eur J Cancer Care (Engl). 2019;28:e13013. https://doi.org/10.1111/ecc.13013 .
Article Google Scholar
Yabroff K, Mariotto A, Tangka F. Annual report to the nation on the status of Cancer, part II: patient economic burden associated with Cancer care. J Natl Cancer Inst. 2021;113:1670–82. https://doi.org/10.1093/jnci/djab192 .
Buja A, Francesconi P, Bellini I, Barletta V, Girardi G, Braga M, et al. Health and health service usage outcomes of case management for patients with long-term conditions: a review of reviews. Prim Heal Care Res Dev. 2020;21:1–21. https://doi.org/10.1017/S1463423620000080 .
Case Management Society of America. CMSA’s standards of practice for case management, Revised 2016. 2016. http://www.naylornetwork.com/cmsatoday/articles/index-v3.asp?aid=400028&issueID=53653 .
Google Scholar
Joo JY, Liu MF. Effectiveness of nurse-led case Management in Cancer Care: systematic review. Clin Nurs Res. 2019;28:968–91. https://doi.org/10.1177/1054773818773285 .
Aubin M, Giguère A, Martin M, Verreault R, Fitch MI, Kazanjian A, et al. Interventions to improve continuity of care in the follow-up of patients with cancer. Cochrane Database Syst Rev. 2012:1–193. https://doi.org/10.1002/14651858.CD007672.pub2 .
Chan RJ, Teleni L, McDonald S, Kelly J, Mahony J, Ernst K, et al. Breast cancer nursing interventions and clinical effectiveness: a systematic review. BMJ Support Palliat Care. 2020;10:276–86. https://doi.org/10.1136/bmjspcare-2019-002120 .
Aromataris E, Fernandez R, Godfrey C, Holly C, Khalil H, Tungpunkom P. JBI manual for evidence synthesis. In: Aromataris E, Munn Z, editors. JBI Manual for Evidence Synthesis. JBI; 2020. https://doi.org/10.46658/JBIMES-20-11 .
Chapter Google Scholar
Bougioukas KI, Liakos A, Tsapas A, Ntzani E, Haidich AB. Preferred reporting items for overviews of systematic reviews including harms checklist: a pilot tool to be used for balanced reporting of benefits and harms. J Clin Epidemiol. 2018;93:9–24. https://doi.org/10.1016/j.jclinepi.2017.10.002 .
Veritas Health Innovation. Covidence systematic review sofware. Covidence. 2016. https://get.covidence.org/systematic-review-software?campaignid=15030045989&adgroupid=130408703002&gclid=EAIaIQobChMIvc7KuJDO-QIVh97ICh1BeQKnEAAYASAAEgI4APD_BwE .
Aromataris E, Fernandez R, Godfrey C, Holly C, Kahlil H, Tungpunkom P. Summarizing systematic reviews: methodological development, conduct and reporting of an umbrella review approach. Int J Evid Based Heal. 2015;13:132–40 http://joannabriggs.org/research/critical-appraisal-tools.html .
Gifford W, Rowan M, Dick P, Modanloo S, Benoit M, Al AZ, et al. Interventions to improve cancer survivorship among indigenous peoples and communities : a systematic review with a narrative synthesis. Support Care Cancer. 2021;29:7029–48. https://doi.org/10.1007/s00520-021-06216-7 .
Article PubMed PubMed Central Google Scholar
Gifford W, Squires J, Angus D, Ashley L, Brosseau L, Craik J, et al. Managerial leadership for research use in nursing and allied health care professions : a systematic review. Implement Sci. 2018;13:1–23. https://doi.org/10.1186/s13012-018-0817-7 .
Popay J, Roberts H, Sowden A, Petticrew M, Arai L, Rodgers M, et al. Guidance on the conduct of narrative synthesis in systematic reviews: a product from the ESRC methods Programme. Lancaster: Lancaster University; 2006. https://www.lancaster.ac.uk/media/lancaster-university/content-assets/documents/fhm/dhr/chir/NSsynthesisguidanceVersion1-April2006.pdf .
Li Q, Lin Y, Liu X, Xu Y. A systematic review on patient-reported outcomes in cancer survivors of randomised clinical trials: direction for future research. Psychooncology. 2014;23:721–30. https://doi.org/10.1002/pon.3504 .
Article CAS PubMed Google Scholar
Pieper D, Antoine SL, Mathes T, Neugebauer EAM, Eikermann M. Systematic review finds overlapping reviews were not mentioned in every other overview. J Clin Epidemiol. 2014;67:368–75. https://doi.org/10.1016/j.jclinepi.2013.11.007 .
Choi J, Lee M, Lee JK, Kang D, Choi JY. Correlates associated with participation in physical activity among adults: a systematic review of reviews and update. BMC Public Health. 2017;17:1–13. https://doi.org/10.1186/s12889-017-4255-2 .
Joo JY, Huber DL. Case management effectiveness on health care utilization outcomes: a systematic review of reviews. West J Nurs Res. 2019;41:111–33. https://doi.org/10.1177/0193945918762135 .
Wulff CN, Thygesen M, Søndergaard J, Vedsted P. Case management used to optimize cancer care pathways: a systematic review. BMC Health Serv Res. 2008;8:1–7 http://www.biomedcentral.com/1472-6963/8/227 .
Yin YN, Wang Y, Jiang NJ, Long DR. Can case management improve cancer patients quality of life?: a systematic review following PRISMA. Medicine (Baltimore). 2020;99:1–7. https://doi.org/10.1097/MD.0000000000022448 .
Wu YL, Padmalatha KMS, Yu T, Lin YH, Ku HC, Tsai YT, et al. Is nurse-led case management effective in improving treatment outcomes for cancer patients? A systematic review and meta-analysis. J Adv Nurs. 2021;00:1–11. https://doi.org/10.1111/jan.14874 .
McQueen J, McFeely G. Case management for return to work for individuals living with cancer: a systematic review. Int J Ther Rehabil. 2017;24:203–10. https://doi.org/10.12968/ijtr.2017.24.5.203 .
Stokes J, Panagioti M, Alam R, Checkland K, Cheraghi-Sohi S, Bower P. Effectiveness of case management for “at risk” patients in primary care: a systematic review and meta-analysis. PLoS One. 2015;10:e0132340. https://doi.org/10.1371/journal.pone.0132340 .
Article CAS PubMed PubMed Central Google Scholar
Lemieux J, Goodwin PJ, Bordeleau LJ, Lauzier S, Théberge V. Quality-of-life measurement in randomized clinical trials in breast cancer: an updated systematic review (2001-2009). J Natl Cancer Inst. 2011;103:178–231. https://doi.org/10.1093/jnci/djq508 .
Montazeri A. Health-related quality of life in breast cancer patients: a bibliographic review of the literature from 1974 to 2007. J Exp Clin Cancer Res. 2008;27:1–31 https://jeccr.biomedcentral.com/articles/10.1186/1756-9966-27-32 .
Cella DF, Tulsky DS, Gray G, Sarafian B, Linn E, Bonomi A, et al. The functional assessment of cancer therapy scale: development and validation of the general measure. J Clin Oncol. 1993;11:570–9. https://doi.org/10.1200/JCO.1993.11.3.570 .
Webster K, Cella D, Yost K. The F unctional a ssessment of C hronic I llness T herapy (FACIT) measurement system: properties, applications, and interpretation. Health Qual Life Outcomes. 2003;1:79. https://doi.org/10.1186/1477-7525-1-79 .
Brady MJ, Cella DF, Mo F, Bonomi AE, Tulsky DS, Lloyd SR, et al. Reliability and validity of the functional assessment of cancer therapy- breast quality-of-life instrument. J Clin Oncol. 1997;15:974–86. https://doi.org/10.1200/jco.1997.15.3.974 .
Cella DF, Bonomi AE, Lloyd SR, Tulsky DS, Kaplan E, Bonomi P. Reliability and validity of the functional assessment of cancer therapy-lung (FACT-L) quality of life instrument. Lung Cancer. 1995;12:199–220. https://doi.org/10.1016/0169-5002(95)00450-F .
Darling G, Eton DT, Sulman J, Casson AG, Cella D. Validation of the functional assessment of cancer therapy esophageal cancer subscale. Cancer. 2006;107:854–63. https://doi.org/10.1002/cncr.22055 .
Aaronson NK, Ahmedzai S, Bergman B, Bullinger M, Cull A, Duez NJ, et al. The European organization for research and treatment of cancer QLQ-C30: a quality-of-life instrument for use in international clinical trials in oncology. J Natl Cancer Inst. 1993;85:365–76. https://doi.org/10.1093/jnci/85.5.365 .
Ware J, Kosinski M, Dewey J. How to score version two of the SF-36® health survey. Lincoln: QualityMetric Incorporated; 2000.
Treanor C, Donnelly M. A methodological review of the short form health survey 36 (SF-36) and its derivatives among breast cancer survivors. Qual Life Res. 2015;24:339–62. https://doi.org/10.1007/s11136-014-0785-6 .
Lins L, Carvalho FM. SF-36 total score as a single measure of health-related quality of life: scoping review. SAGE Open Med. 2016;4:1–12. https://doi.org/10.1177/2050312116671725 .
Chan RJ, Crawford-Williams F, Crichton M, Joseph R, Hart NH, Milley K, et al. Effectiveness and implementation of models of cancer survivorship care: an overview of systematic reviews. J Cancer Surviv. 2021:1–25. https://doi.org/10.1007/s11764-021-01128-1 .
Joo J, Huber D. Community-based case management effectiveness in populations that abuse substances. Int Nurs Rev. 2015;62:536–46. https://doi.org/10.1111/inr.12201 .
Welch G, Garb J, Zagarins S, Lendel I, Gabbay RA. Nurse diabetes case management interventions and blood glucose control: results of a meta-analysis. Diabetes Res Clin Pract. 2010;88:1–6. https://doi.org/10.1016/j.diabres.2009.12.026 .
Hoffmann TC, Glasziou PP, Boutron I, Milne R, Perera R, Moher D, et al. Better reporting of interventions: template for intervention description and replication (TIDieR) checklist and guide. BMJ. 2014;348:g1687. https://doi.org/10.1136/bmj.g1687 .
Download references
Acknowledgements
Not applicable.
Dual (co-)authorship
We declared that no author has authored one or more of the included systematic reviews.
This study was supported by 1) Hunan Provincial Key Laboratory of Nursing (2017TP1004, PI: Jia Chen), Hunan Provincial Science and Technology Department, 2) Changsha Natural Science Foundation (kq2202365, PI: Nina Wang) Changsha Science and Technology Department, and 3) Management research foundation of Xiangya Hospital (2021GL12, PI: Nina Wang).
Author information
Authors and affiliations.
Department of Respiratory Medicine, Xiangya Hospital, Central South University, Changsha, China
Nina Wang, Zhengkun Shi & Huaping Yang
National Clinical Research Center for Geriatric Disorders, Xiangya Hospital, Changsha, China
Xiangya School of Nursing, Central South University, Changsha, China
Jia Chen, Chen Wang & Xianhong Li
School of Nursing, University of Ottawa, Ottawa, Canada
Wenjun Chen
Center for Research on Health and Nursing, University of Ottawa, Ottawa, Canada
Intensive Care Unit of Cardiovascular Surgery Department, Xiangya Hospital, Central South University, Changsha, China
Peng Liu & Xiangling Dong
The 956th Army Hospital, Linzhi, China
School of Nursing, Changsha Medical University, Changsha, China
You can also search for this author in PubMed Google Scholar
Contributions
All authors have contributed to the production of this review. NW and WC conceptualized and designed the study and are the guarantor of the paper. JC and ZS conducted the literature search. PL, XW and XD were involved in the study screening. NW, LM and XL participated in the quality appraisal and data extraction. NW, ZS and HY conducted the data analysis. NW drafted the manuscript. WC and XL revised the manuscript. All authors participated in the review of the manuscript and approved the final manuscript.
Corresponding author
Correspondence to Wenjun Chen .
Ethics declarations
Ethics approval and consent to participate, consent for publication, competing interests.
The authors declare no competing interests.
Additional information
Publisher’s note.
Springer Nature remains neutral with regard to jurisdictional claims in published maps and institutional affiliations.
Supplementary Information
Additional file 1., additional file 2..
Searching strategies.
Additional file 3.
Rights and permissions.
Open Access This article is licensed under a Creative Commons Attribution 4.0 International License, which permits use, sharing, adaptation, distribution and reproduction in any medium or format, as long as you give appropriate credit to the original author(s) and the source, provide a link to the Creative Commons licence, and indicate if changes were made. The images or other third party material in this article are included in the article's Creative Commons licence, unless indicated otherwise in a credit line to the material. If material is not included in the article's Creative Commons licence and your intended use is not permitted by statutory regulation or exceeds the permitted use, you will need to obtain permission directly from the copyright holder. To view a copy of this licence, visit http://creativecommons.org/licenses/by/4.0/ . The Creative Commons Public Domain Dedication waiver ( http://creativecommons.org/publicdomain/zero/1.0/ ) applies to the data made available in this article, unless otherwise stated in a credit line to the data.
Reprints and permissions
About this article
Cite this article.
Wang, N., Chen, J., Chen, W. et al. The effectiveness of case management for cancer patients: an umbrella review. BMC Health Serv Res 22 , 1247 (2022). https://doi.org/10.1186/s12913-022-08610-1
Download citation
Received : 04 April 2022
Accepted : 21 September 2022
Published : 14 October 2022
DOI : https://doi.org/10.1186/s12913-022-08610-1
Share this article
Anyone you share the following link with will be able to read this content:
Sorry, a shareable link is not currently available for this article.
Provided by the Springer Nature SharedIt content-sharing initiative
- Cancer patients
- Umbrella review
- Health care
- Outcome assessment
BMC Health Services Research
ISSN: 1472-6963
- General enquiries: [email protected]
- Biomarker-Driven Lung Cancer
- HER2-Positive Breast Cancer
- Chronic Lymphocytic Leukemia
- Small Cell Lung Cancer
- Renal Cell Carcinoma
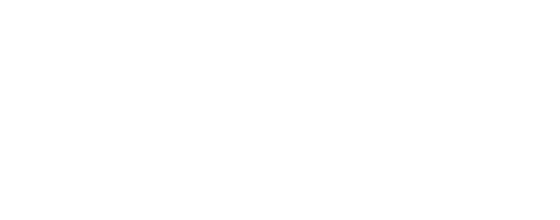
- CONFERENCES
- PUBLICATIONS
Case 1: 72-Year-Old Woman With Small Cell Lung Cancer
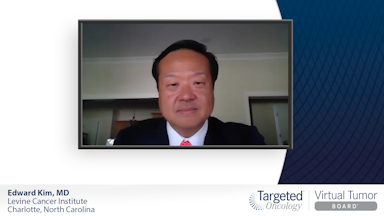
EP: 1 . Case 1: 72-Year-Old Woman With Small Cell Lung Cancer
Ep: 2 . case 1: extensive-stage small cell lung cancer background, ep: 3 . case 1: impower133 trial in small cell lung cancer, ep: 4 . case 1: caspian trial in extensive-stage small cell lung cancer, ep: 5 . case 1: biomarkers in small cell lung cancer, ep: 6 . case 1: small cell lung cancer in the era of immunotherapy.
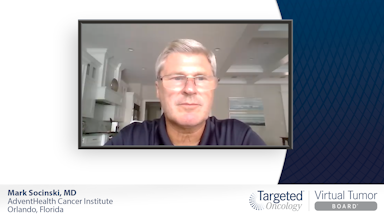
EP: 7 . Case 2: 67-Year-Old Woman With EGFR+ Non–Small Cell Lung Cancer
Ep: 8 . case 2: biomarker testing for non–small cell lung cancer, ep: 9 . case 2: egfr-positive non–small cell lung cancer, ep: 10 . case 2: flaura study for egfr+ metastatic nsclc, ep: 11 . case 2: egfr+ nsclc combination therapies.
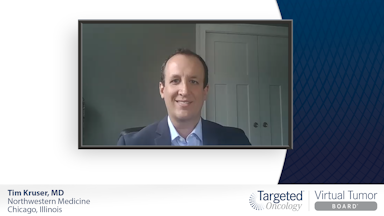
EP: 12 . Case 2: Treatment After Progression of EGFR+ NSCLC
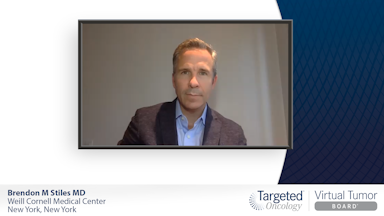
EP: 13 . Case 3: 63-Year-Old Man With Unresectable Stage IIIA NSCLC
Ep: 14 . case 3: molecular testing in stage iii nsclc, ep: 15 . case 3: chemoradiation for stage iii nsclc, ep: 16 . case 3: pacific trial in unresectable stage iii nsclc, ep: 17 . case 3: standard of care in unresectable stage iii nsclc, ep: 18 . case 3: management of immune-related toxicities in stage iii nsclc.
Mark Socinski, MD: Thank you for joining us for this Targeted Oncology ™ Virtual Tumor Board ® focused on advanced lung cancer. In today’s presentations my colleagues and I will review three clinical cases. We will discuss an individualized approach to treatment for each patient, and we’ll review key clinical trial data that impact our decisions. I’m Dr. Mark Socinski from the AdventHealth cancer institute in Orlando, Florida. Today I’m joined by Dr Ed Kim, a medical oncologist from the Levine Cancer Institute in Charlotte, North Carolina; Dr Brendon Stiles, who is a thoracic surgeon from the Weill Cornell Medical Center in New York ; and Dr Tim Kruser, radiation oncologist from Northwestern Medicine Feinberg School of Medicine in Chicago. Thank you all for joining me today. We’re going to move to the first case, which is a case of small cell lung cancer. I’m going to ask Dr Kim to do the presentation.
Edward Kim, MD: Thanks, Mark. It’s my pleasure to walk us through the first case, which is small cell lung cancer. This is a case with a 72-year-old woman who presents with shortness of breath, a productive cough, chest pain, some fatigue, anorexia, a recent 18-pound weight loss, and a history of hypertension. She is a schoolteacher and has a 45-pack-a-year smoking history; she is currently a smoker. She is married, has 2 kids, and has a grandchild on the way. On physical exam she had some dullness to percussion with some decreased-breath sounds, and the chest x-ray shows a left hilar mass and a 5.4-cm left upper-lobe mass. CT scan reveals a hilar mass with a bilateral mediastinal extension. Negative for distant metastatic disease. PET scan shows activity in the left upper-lobe mass with supraclavicular nodal areas and liver lesions, and there are no metastases in the brain on MRI. The interventional radiographic test biopsy for liver reveals small cell, and her PS is 1. Right now we do have a patient who has extensive-stage small cell lung cancer. Unfortunately, it’s what we found. It’s very common to see this with liver metastases.
Transcript edited for clarity.
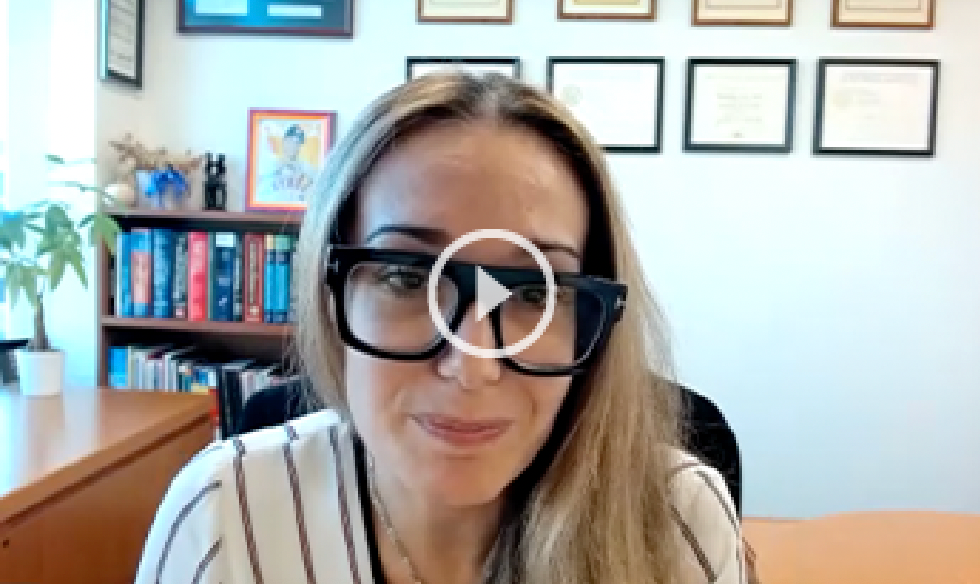
FDA Approval Marks Amivantamab's Milestone in EGFR+ NSCLC
In this episode, Joshua K. Sabari, MD, discusses the FDA approval of amivantamab plus chemotherapy as a first-line treatment for patients with EGFR exon 20 insertion mutation-positive non-small cell lung cancer.
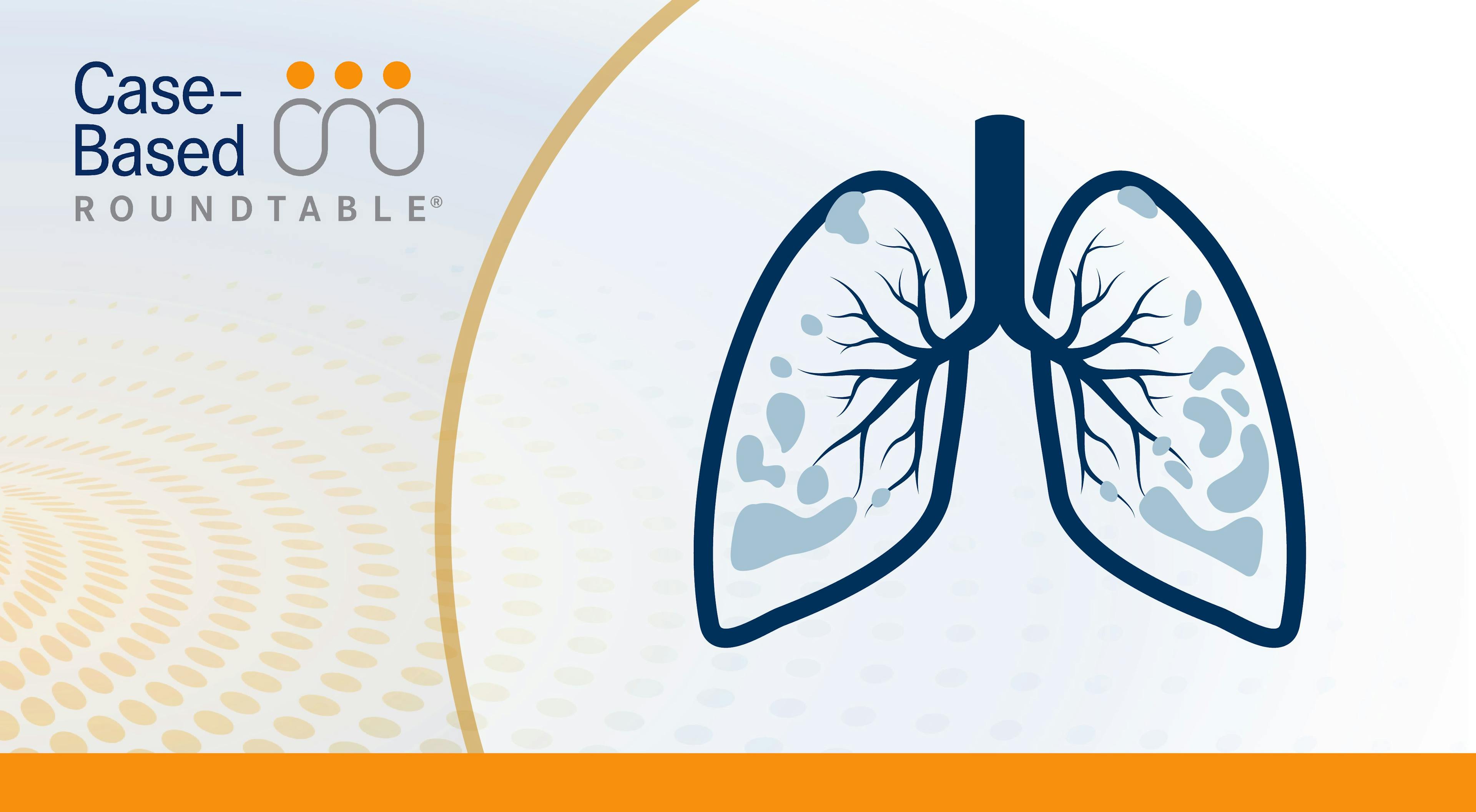
Emphasizing the Need for NGS in Advanced NSCLC to Determine Treatment
During a Case-Based Roundtable® event, Joshua Sabari, MD, led a discussion on the need for next-generation sequencing to determine treatment in patients with EGFR-positive advanced non–small cell lung cancer in the first article of a 2-part series.
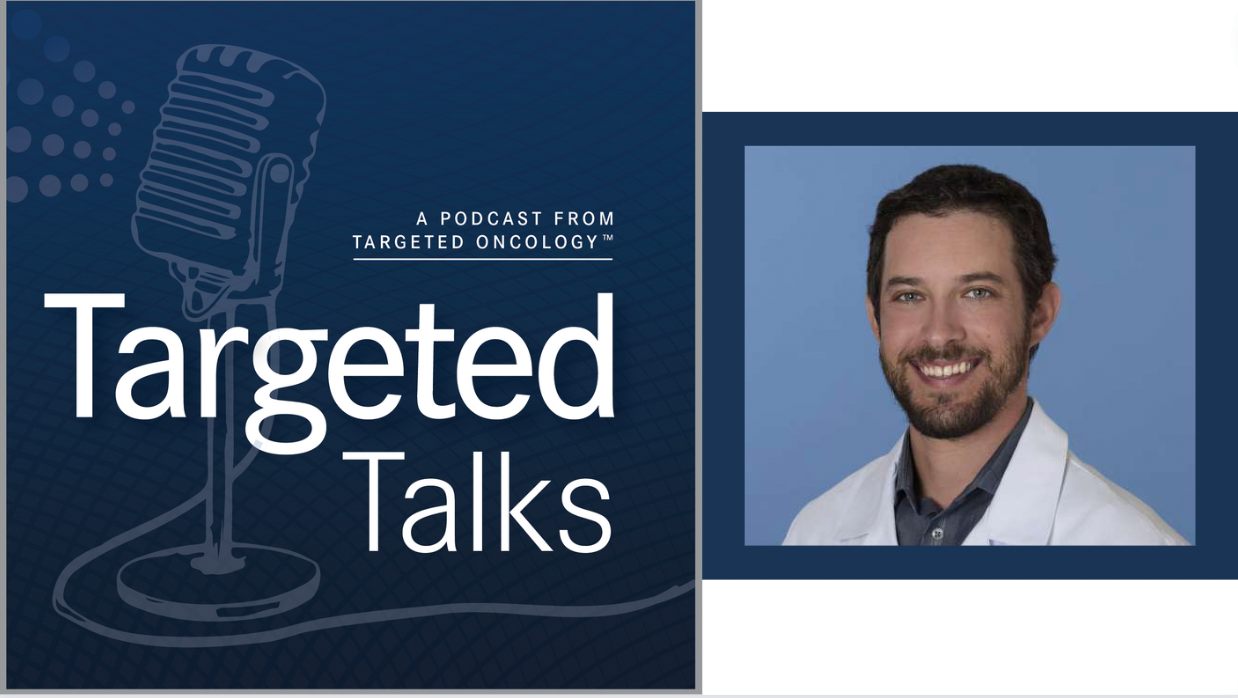
Lisberg Discusses Dato-DXd's Role in Advanced Lung Cancer Care
In this episode of Targeted Talks, Aaron Lisberg, MD, discusses results from the phase 3 TROPION-Lung01 study of datopotamab in advanced or metastatic non–small cell lung cancer.
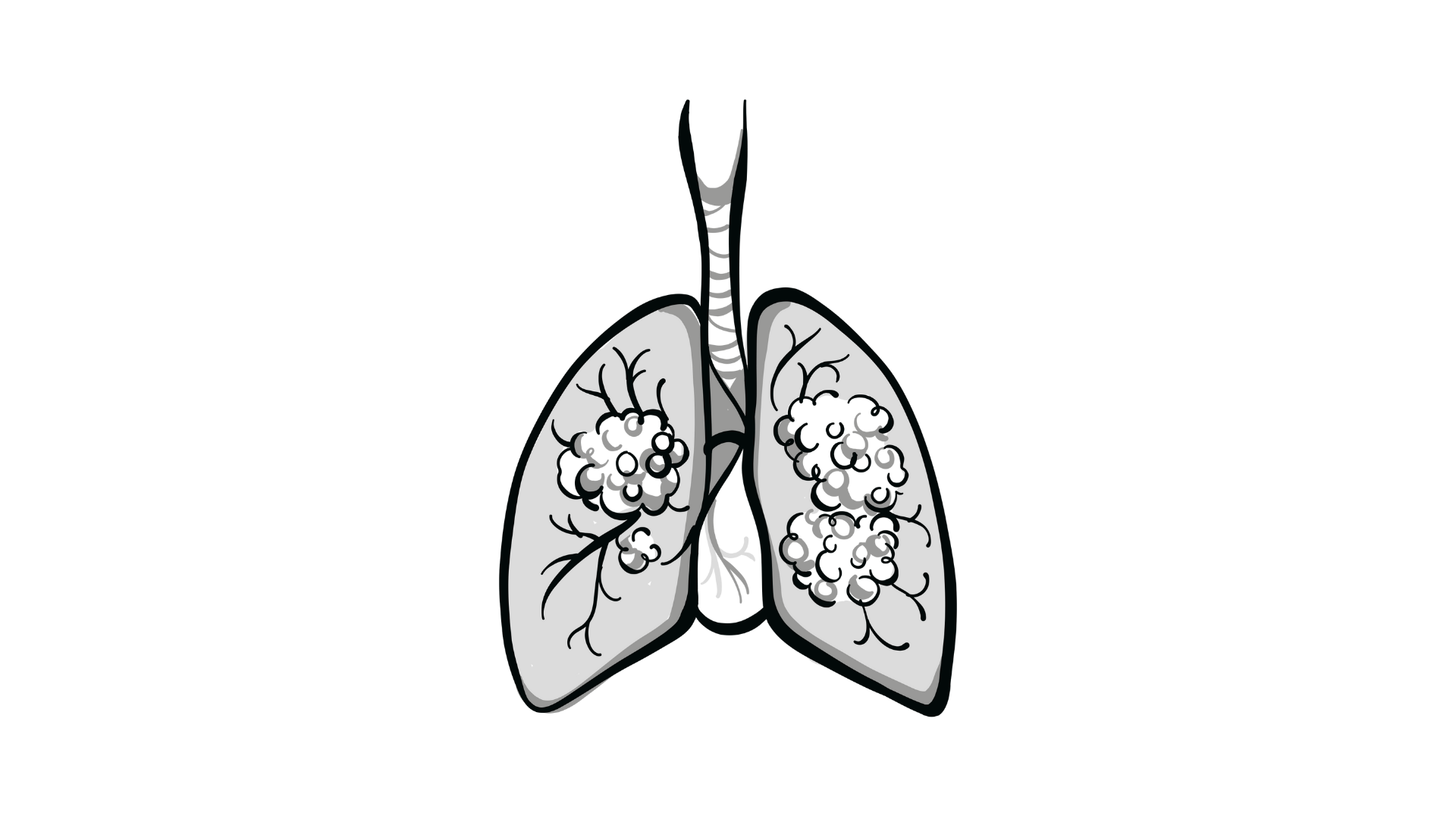
Dato-DXd Outperforms Docetaxel in Nonsquamous Lung Cancer
Aaron Lisberg, MD, discussed the phase 3 TROPION-Lung01 study which evaluated datopotamab deruxtecan for patients with advanced or metastatic non-small cell lung cancer.
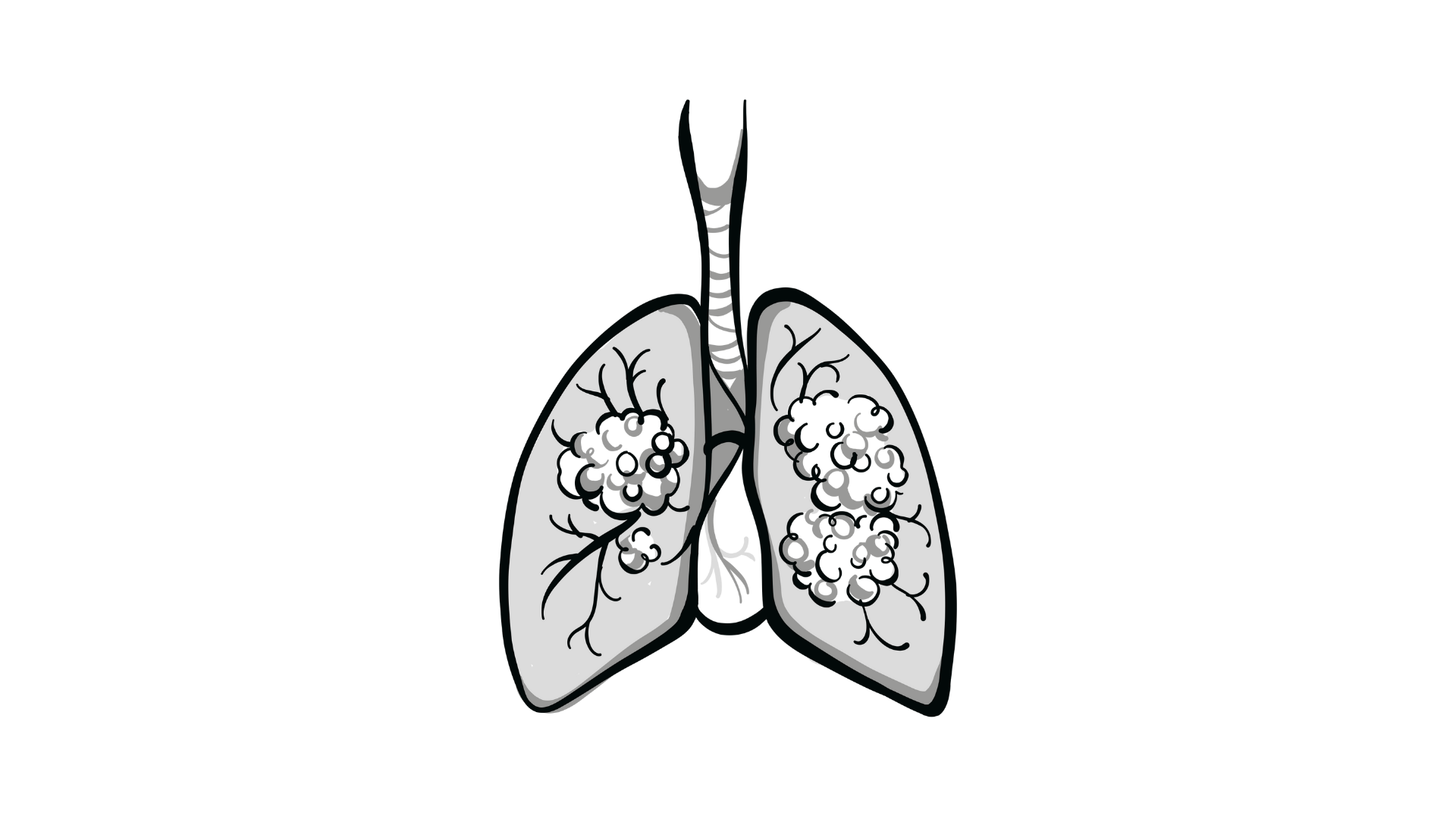
Evolving Treatment Paradigms with Amivantamab in Wild-Type NSCLC
Natasha Leighl, MD, MMSc, BSc, discussed the analysis of the CHRYSALIS study that was presented at the 2024 American Association for Cancer Research Annual Meeting.
2 Commerce Drive Cranbury, NJ 08512
609-716-7777

Ohio State nav bar
The Ohio State University
- BuckeyeLink
- Find People
- Search Ohio State
Patient Case Presentation
Patient Mrs. B.C. is a 56 year old female who is presenting to her WHNP for her annual exam. She had to cancel her appointment two months ago and didn’t reschedule until now. Her last pap smear and mammogram were normal. Today, while performing her breast exam, her nurse practitioner notices dimpling in the left breast as the patient raises her arms over her head. When the NP mentions it to Mrs. B.C. she is surprised and denies noticing it before today. A firm, non-tender, immobile nodule is palpated in the upper quadrant of her breast . The NP then asks Mrs. B.C. how frequently she is performing breast self-exams, she admits to only doing them randomly when she remembers, which is about every few months. She reports no recent or abnormal drainage from her breast. Further examination reveals palpable axillary lymph nodes.
Mrs. B.C. is about 30 pounds overweight and walks her dog around her neighborhood every morning before work and every evening when she gets home. She reports drinking a glass of white wine before bed each night. She denies any history of tobacco use. She reports use of a combination birth control pill on and off for 25 years until she reached menopause. She is not currently taking any prescription medications.
Past Medical History
- Menarche (Age 10)
- Post-menopausal (Age 53)
- No other pertinent medical history
Family History:
- Father George- deceased from stroke (75 years old), history of hypertension, CAD, HLD
- Mother Maryanne alive- 76 years old, history of dementia, osteoporosis
- Brother Michael- alive, 57 years old, history of hypertension, CAD and cardiac stent placement (54 years old)
- Sister, Michelle- alive 53 years old, history of GERD, Asthma
- Brother- Jimmy- alive 50 years old, no past medical history
Social History:
Mrs. B.C. works Monday-Friday 8am-5pm at the local dentist’s office at the front desk as a schedule coordinator. She is planning to retire in a few years. In her spare time, she is involved in various community efforts to feed the homeless and helps to prepare dinners at her local church one night a week. She also enjoys cooking and baking at home, gardening, and nature photography.
Mrs. B.C. has two children. Her oldest son, Patrick, is 21 years old and is in his final year of pre-med. He is attending a public university about 2 hours away from home where he lives year-round. As an infant, Patrick was breastfed until 18 months when he self-weaned. Her daughter, Veronica, is 19 years old and lives at home while attending the local branch campus of a state university. She is in her second year of a business degree and then plans to transfer to the main campus next year. When Veronica was an infant she had difficulty latching onto the breast due to an undiagnosed tongue and lip ties resulting in Mrs. BC exclusively pumping and bottle feeding for six months. After six months, Mrs. B.C. was having a hard time keeping up while working and her found her supply diminished. Veronica had begun eating solid foods so Mrs. B.C. switched to supplemental formula, which was a big relief.
Mrs. B.C. was married to her now ex-husband Kent for 26 years. They divorced two years ago when Veronica was a senior in high school. They have remained friends and Kent lives 25 minutes away in a condo with his girlfriend. She also has two brothers who live nearby and a sister who lives out of state. Her 7 nieces and nephews range in age from 9 years old to 26 years old. Her father, George, passed away from a sudden stroke 4 years ago. Her mother, Maryanne, has dementia and is living in a nearby memory care facility. She also has many close friends.
- Around the Practice
- Between the Lines
- Contemporary Concepts
- Readout 360
- Insights from Experts at Mayo Clinic on Translating Evidence to Clinical Practice
- Optimizing Outcomes in Patients with HER2+ Metastatic Breast Cancer

- Conferences
- Publications
Case 1: 48-Year-Old Patient With HER2+ Metastatic Breast Cancer
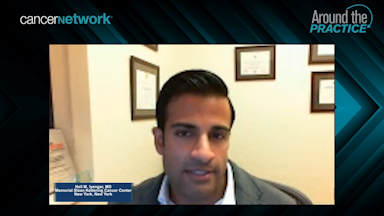
EP: 1 . Best Practices: HER2+ MBC With Brain Mets
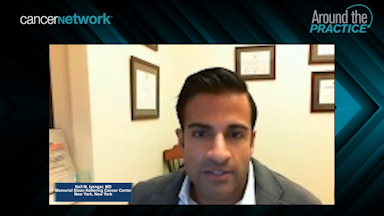
EP: 2 . Frontline Standards of Care for HER2+ MBC
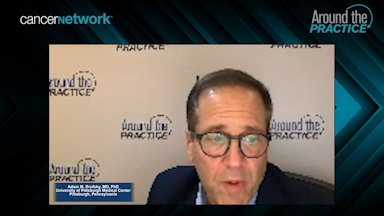
EP: 3 . Case 1: 48-Year-Old Patient With HER2+ Metastatic Breast Cancer
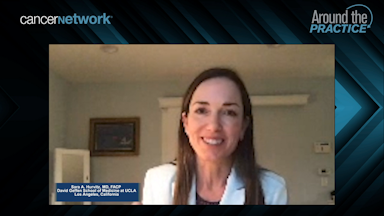
EP: 4 . Treatment Strategies for Relapsed/Refractory HER2+ MBC
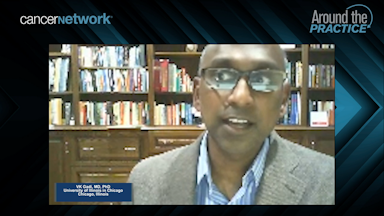
EP: 5 . Case 2: 61-Year-Old Patient With R/R HER2+ MBC
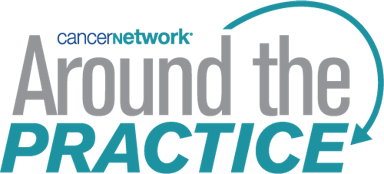
EP: 6 . Cancer Network Around the Practice: Relapsed/Refractory HER2+ Metastatic Breast Cancer
Adam M. Brufsky, MD, PhD: Let’s talk about this case. This is a 48-year-old woman who presented to her primary care physician a number of years ago with a lump in her breast. She had a 4.4-cm left breast mass and 3 palpable axillary lymph nodes. Her ultrasound and mammogram confirmed these physical findings.
She was referred to a medical oncologist and had a core needle biopsy that showed ER- [estrogen receptor-negative]/PR- [progesterone receptor-negative], HER2 [human epidermal growth factor receptor 2]-positive by IHC [immunohistochemistry score] that was 3+. A CT scan of the chest, abdomen, and pelvis showed 3 liver lesions, the largest being 3.1 cm. This is the de novo patient we always talk about. She had an MRI of the brain and it was negative for metastasis. She received 6 cycles of THP [docetaxel, trastuzumab, pertuzumab], followed by HP [trastuzumab, pertuzumab] for another 12 months. That’s 18 months of therapy.
She had a partial response in her breast mass, and her liver lesions fully responded. Later, she suddenly began to have rapid unexplained weight loss. The CT scan only showed 2 new liver lesions, so not quite the symptom I would imagine. She then got a brain MRI that showed about 30 widely scattered lesions, the largest being about 0.5 or 0.6 [cm]. They have all these little punctate ones; you’ve all seen those.
The question is: what treatment would you give this person? Let’s say the brain MRI shows 3 lesions, all in the frontal cortex, with the largest being 1.5 cm. That makes it a little bit of a different question because if there are widely scattered lesions, we’re not going to want to do SRS [stereotactic radiosurgery]. We are probably going to want to do whole brain radiation. Let’s say she’s asymptomatic with no edema. The polling question is: what treatment would you recommend? T-DM1 [trastuzumab emtansine], tucatinib/trastuzumab/capecitabine, SRS to the brain metastases, clinical trial, or other.
You guys could answer that question. Let me start with Sara. How would you approach this?
Sara A. Hurvitz, MD, FACP: They’re not totally mutually exclusive, right? You could do SRS and switch systemic therapy. She is progressing systemically in the liver, so I think switching systemic therapy makes sense. I like tucatinib because it does penetrate the blood-brain barrier, but I would still be tempted and would probably talk to my radiation oncology and neurosurgery colleagues. We’d probably end up doing both the SRS and tucatinib-based therapy.
Adam M. Brufsky, MD, PhD: That’s reasonable. VK, do you have any other comments on this?
VK Gadi, MD, PhD: Yes, I agree. The tolerability of the regimen is good. You might even give this lady an opportunity to fly without SRS and have that in your back pocket. If you’re not seeing control, you can go to SRS at a later time. I don’t think there’s a wrong answer here. You could probably do it both ways.
Adam M. Brufsky, MD, PhD: Neil, do you have something to add?
Neil M. Iyengar, MD: No. She fits perfectly into the HER2CLIMB population, so I agree with everything that has been said because there is demonstrated activity of the tucatinib-based regimen in terms of CNS [central nervous system] response. Coupling that with SRS is reasonable. This is the patient we were talking about earlier with whom we would discuss foregoing local therapy to the brain. That’s a reasonable discussion here. It’s a tricky poll question because my kneejerk response would be to put her on a clinical trial. We should all be trying to prioritize clinical trials, but in the absence of that clinical availability, tucatinib plus or minus radiation is a reasonable option.
Adam M. Brufsky, MD, PhD: There’s a clinical trial that’s great; it’s not scientifically spectacular, but clinically, it’s fabulous. I believe it’s called DESTINY. In fact, I put a patient on it today with trastuzumab deruxtecan and tucatinib together. That’s a great trial that’s going to accrue quickly. If we could put as many people as we can on that, we can answer the clinical question quickly. I would agree.
I have 1 last question before we go on to the last 25 minutes and the last segment. What do you tell people about [adverse] effects? Are you seeing a lot of [adverse] effects with tucatinib? Do you have to dose reduce it at all when you give it? These are questions people who haven’t had a lot of experience with it usually ask. I’ll start with Neil. Do you see a lot of diarrhea? Do you have to dose reduce with tucatinib?
Neil M. Iyengar, MD: In my experience, this regimen is quite tolerable. We all, as oncologists, have unfortunately become very comfortable with managing diarrhea, along with oncology nursing and so forth. What I have found with the tucatinib-based regimen is that with the initiation of antidiarrheal agents, the diarrhea usually resolves or improves pretty quickly. People have to know about it and be prepared to deal with it immediately. It does come on early, usually within the first cycle.
The other consideration to keep in mind with tucatinib is that many of the [adverse] effects are likely related to capecitabine. We’re all very comfortable with managing capecitabine-related toxicity and dose modifying capecitabine as needed. We see in the HER2CLIMB data that patients in the tucatinib arm stayed on study longer and were therefore exposed to capecitabine for longer than those in the placebo arm. I think a lot of the toxicities are familiar ones that are related to capecitabine and are quite manageable.
Adam M. Brufsky, MD, PhD: Great. VK and Sara, do you have any other comments about this toxicity? Do you see any toxicity at all with this, more than you’d expect?
Sara A. Hurvitz, MD, FACP: It’s well tolerated. About 13% had grade 3/4 diarrhea. Before getting on this call, I had to dose reduce a patient on this therapy. It’s hard to tell. On the clinical trial we enrolled patients, and I had a patient on who had severe colitis, hospitalization, etc, and I was sure she was getting tucatinib. When she was unblinded after the data came out, it turned out that she wasn’t on tucatinib. She was on placebo. I completely agree that these are [adverse] effects we’re used to with capecitabine. There’s not a whole lot of difference. Tucatinib is pretty well tolerated.
VK Gadi, MD, PhD: I agree. I think the capecitabine is the real culprit. The people on the trial were actually on it for so much longer that the toxicities from capecitabine emerged ongoing on the study. That has been my experience. Something important we don’t yet have is the PRO [patient-reported outcomes] data from these studies. A lot of my colleagues, especially those in communities where patients come in from a long way away, know that this is a tremendous pill burden with this regimen. Sometimes a parenteral regimen that you’re giving every 3 weeks is better for patients. I’m curious to see what those data look like when they come out. From our perspective as physicians, this is a slam dunk and it’s easy to give, but that’s not always the perspective that matters.
Adam M. Brufsky, MD, PhD: I agree.
Sara A. Hurvitz, MD, FACP: Yes, I think the quality of life PRO data were presented at the San Antonio [Breast Cancer Symposium]. I’m trying to pull it up. I don’t have it right at my fingertips, but my recollection was that it looked fairly good, that the quality of life was maintained.
Adam M. Brufsky, MD, PhD: Right, but they’re not going to tell you that they’re struggling to take all those pills. It’s a lot.
Sara A. Hurvitz, MD, FACP: That’s true.
Adam M. Brufsky, MD, PhD: It’s about 9 pills a day, which is a lot.
Neil M. Iyengar, MD: The quality of life data are always interesting because the end point of choice is time to deterioration and whether we are avoiding that. I think that’s a fairly low bar.
Adam M. Brufsky, MD, PhD: Exactly. Women are going to do anything they can.
Transcript edited for clarity.
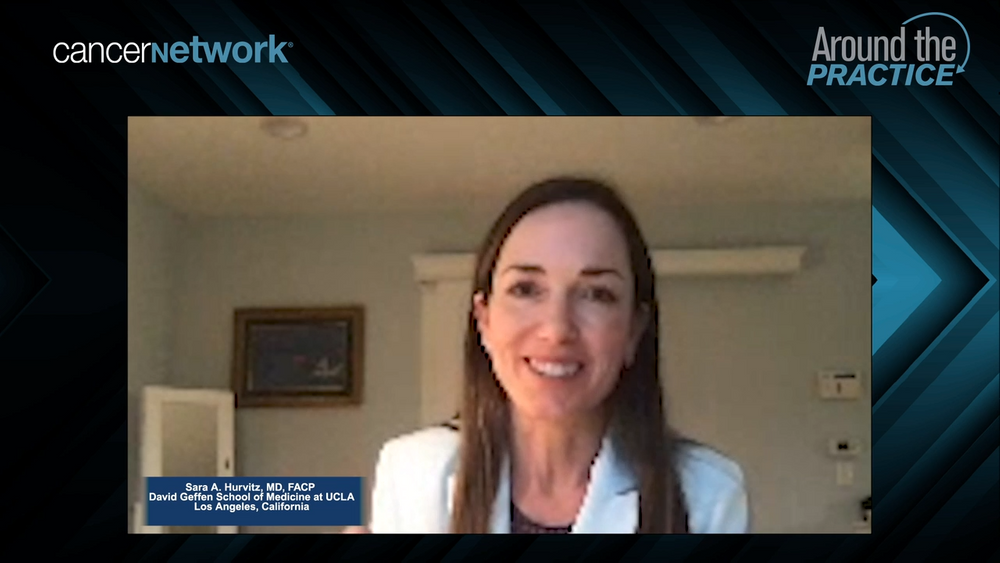
Together we are beating cancer
About cancer
Cancer types
- Breast cancer
- Bowel cancer
- Lung cancer
- Prostate cancer
Cancers in general
- Clinical trials
Causes of cancer
Coping with cancer
- Managing symptoms and side effects
- Mental health and cancer
- Money and travel
- Death and dying
- Cancer Chat forum
Health Professionals
- Cancer Statistics
- Cancer Screening
- Learning and Support
- NICE suspected cancer referral guidelines
Get involved
- Make a donation
By cancer type
- Leave a legacy gift
- Donate in Memory
Find an event
- Race for Life
- Charity runs
- Charity walks
- Search events
- Relay For Life
- Volunteer in our shops
- Help at an event
- Help us raise money
- Campaign for us
Do your own fundraising
- Fundraising ideas
- Get a fundraising pack
- Return fundraising money
- Fundraise by cancer type
- Set up a Cancer Research UK Giving Page
- Find a shop or superstore
- Become a partner
- Cancer Research UK for Children & Young People
- Our We Are campaign
Our research
- Brain tumours
- Skin cancer
- All cancer types
By cancer topic
- New treatments
- Cancer biology
- Cancer drugs
- All cancer subjects
- All locations
By Researcher
- Professor Duncan Baird
- Professor Fran Balkwill
- Professor Andrew Biankin
- See all researchers
- Our achievements timeline
- Our research strategy
- Involving animals in research
Funding for researchers
Research opportunities
- For discovery researchers
- For clinical researchers
- For population researchers
- In drug discovery & development
- In early detection & diagnosis
- For students & postdocs
Our funding schemes
- Career Development Fellowship
- Discovery Programme Awards
- Clinical Trial Award
- Biology to Prevention Award
- View all schemes and deadlines
Applying for funding
- Start your application online
- How to make a successful application
- Funding committees
- Successful applicant case studies
How we deliver research
- Our research infrastructure
- Events and conferences
- Our research partnerships
- Facts & figures about our funding
- Develop your research career
- Recently funded awards
- Manage your research grant
- Notify us of new publications
Find a shop
- Volunteer in a shop
- Donate goods to a shop
- Our superstores
Shop online
- Wedding favours
- Cancer Care
- Flower Shop
Our eBay store
- Shoes and boots
- Bags and purses
- We beat cancer
- We fundraise
- We develop policy
- Our global role
Our organisation
- Our strategy
- Our Trustees
- CEO and Executive Board
- How we spend your money
- Early careers
Cancer news
- Cancer News
- For Researchers
- For Supporters
- Press office
- Publications
- Update your contact preferences
ABOUT CANCER
GET INVOLVED
NEWS & RESOURCES
FUNDING & RESEARCH
You are here

Patient data use case studies
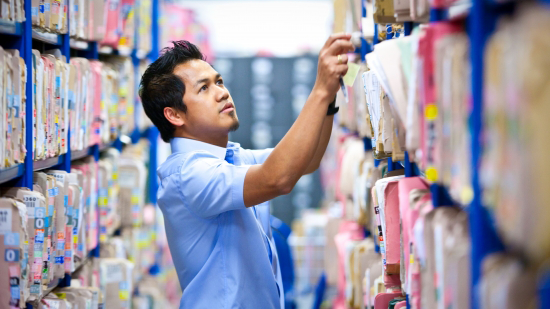
Questions about these case studies or how patient data is handled?
Contact the team
During our Review of Informed Choice for Cancer Registration, patients clearly told us they would like to know what their data are being used for. We agreed that as a charity, we would highlight where we are using patient-data for research and analysis. Relevant examples of work by the Cancer Intelligence team at Cancer Research UK will be available here, and we will add to this as we embark on new projects.
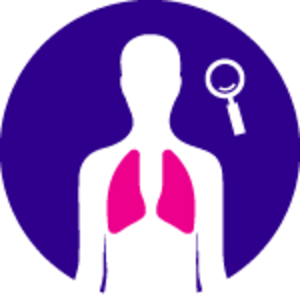
Lung cancer diagnostic pathways
Identifying common lung cancer diagnostic pathways using linked datasets.
Small proportions of patients in 2013-2015 meet the timings of the optimal pathway. Diagnostic pathway length varies by source of imaging request.
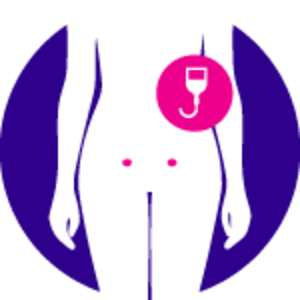
Variation in ovarian cancer treatment rates
Investigating variation in treatment for ovarian cancer patients.
We expect to establish if there is variation between Cancer Alliances/Trusts, identify the odds ratios/ regression co-efficients for predictor variables, and if it's the affect of healthcare system level factors.
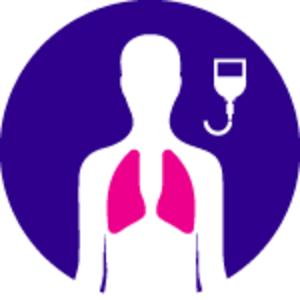
Variation in lung cancer treatment rates
Investigating variation in treatment for non-small cell lung cancer patients.
We expect to establish if there is significant variation between Cancer Alliances/Trusts (geography still to TBC) and identify the odds ratios/regression co-effecients associated with each predictor variable.
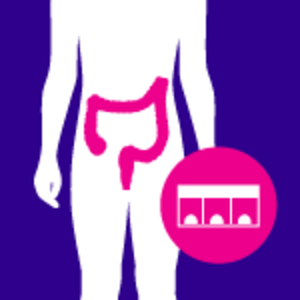
Bowel cancer screening campaign evaluation
Evaluating the bowel cancer screening regional pilot campaign in the North West of England.
Analysis of interim results following the campaign are promising. We are currently working to finalise the analysis and results will follow in due course.
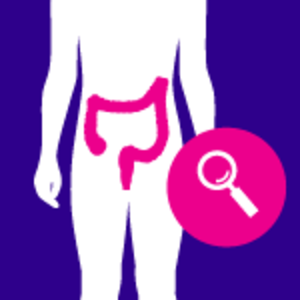
Colorectal cancer diagnostic pathways
Coming soon.
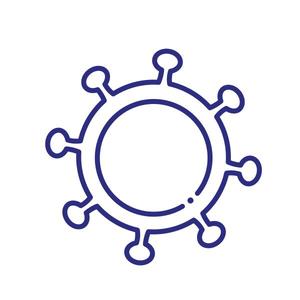
The impact of COVID-19 on radiotherapy in the UK
The aims of COVID RT are to understand why changes in radiotherapy treatment schedules were implemented during the pandemic and to then explore the impact of these changes on patient outcomes and the UK radiotherapy services. This project aims purely to understand the changes in patient's radiotherapy treatment from COVID-19.
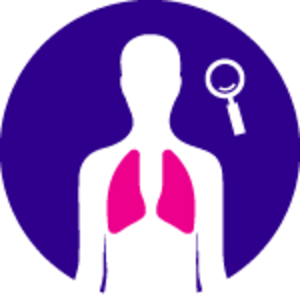
Patient data – a vital tool that will help beat cancer
Cancer is the most complex health challenge that we face. There are 200 different types of cancer but even that is an oversimplification.
At a genetic level each individual cancer is as unique as the person with the disease. Despite this complexity our research has helped double cancer survival over the last 40 years. This extraordinary progress has been powered by relentlessly increasing our understanding of cancer’s intricacies.
But big questions about cancer remain, and our scientists are seeking the answers. Patient data has the potential to reveal some of these. Here we meet some outstanding scientists who are using patient data to grow our understanding even further, work that could underpin tomorrow’s cures.
Case study 1 – Prof. Crispin Miller
Professor_crispin_miller.jpg.
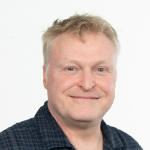
At the heart of the CRUK Scotland Institute is a team of brilliant scientists, led by Professor Crispin Miller, who specialise in using super computers to understand how cancer behaves.
This diverse and talented team - which includes mathematicians, physicists, biologists and software engineers - are using a range of techniques to dig deep into the huge amounts of data we have on the genetics and anatomy of tumours.
“Biology is exciting” says Crispin. “The questions of modern cancer research, like how the DNA in one cell can define an entire living person – to me that’s as exciting as understanding the first few microseconds of the Big Bang.”
Crispin’s goal is to identify patterns in a tumour’s DNA or its structure that might help us better understand why and how cancers develop. And ultimately this could inform how best to treat them.
But spotting those crucial patterns is difficult because tumours are so diverse. And so Crispin’s team turn to supercomputers. These powerful tools can sift through vast amounts of information to find patterns that would be impossible for a human to spot. Then the scientists can do what they do best – work out how the patterns are driving cancer progression or making a tumour more or less sensitive to an anticancer drug.
Professor Miller explains: “Data gives us insight into things you can’t see down a microscope. It has the potential to start developing truly targeted therapies. To find the right therapy for the right person at the right time that is the goal.”
His team are using a data approach on many different cancer-related questions. In one project, patients have kindly allowed their doctors to share anonymised scan images of tumours and the tumour genetic data. Crispin’s team are seeing whether they can build a sophisticated AI approach that has the power to use this data to predict how tumours are going to respond to treatment. This could help doctors pick the best treatment for each person.
What nearly all Professor Miller’s work has in common is that it is only possible due to the data that people with cancer have shared.
“Data is central to the research we are doing. The more tumours we study, the more we understand how they differ from each other and how we can use this information to improve outcomes for people with cancer. I am incredibly grateful to the patients who donate their data and tissue, I couldn’t do my work without it.”
Case study 2 – Dr Irene Lobon
“Data is everything” – how patient data is helping us understand how cancer spreads and resists treatment
For Dr Irene Lobon, everything is data and data is everything. From calculating nutrients in new recipes to studying tumour samples, analysing data is central to her life and work. While in her spare time Dr Lobon’s analytical skills help her to optimise her favourite recipes, in the laboratory, these skills could lead to lifesaving discoveries.
Dr Lobon is a biomedical researcher in the Cancer Dynamics Laboratory, led by Professor Samra Turajlic at The Francis Crick Institute. She works on a multidisciplinary team featuring scientists and clinicians, all working together to better understand cancer.
The team are studying advanced, spreading melanoma, called metastatic melanoma. This is a type of skin cancer that is particularly hard to treat and often becomes resistant to drugs. Professor Samra’s group want to know how melanoma changes over time, what happens inside the tumour as it spreads and what features lead to drug resistance. To answer these questions, the team relies on patient data. Specifically, samples from patients who have passed away from cancer.
To access these samples, the team makes use of the PEACE (Posthumous Evaluation of Advanced Cancer Environment) study, a huge collaborative project, set up to help researchers collect samples from patients who have died from cancer. In this study, researchers take samples from participants during their treatment journey and after they have died. This huge assembly of data has allowed clinicians and scientists to study cancer in a way that was not possible before.
Professor Turajlic’s team employed sophisticated genetic sequencing techniques to study nearly 600 separate samples from 14 different patients with metastatic melanoma from the PEACE study. “What we’ve observed is that there are many variables that contribute tiny bits to the cancer progression” said Dr Lobon.
Uncovering these small contributions allowed the team to piece together a complex timeline of how the cancer develops. “It’s like having a fossil. We can study cancers at different points in time.”
The team shared the results of this extensive study with the scientific community earlier this year. The incredible information uncovered has helped scientists to understand the complex ways that melanomas use to evade drugs. The researchers hope that it could also lead to the development of completely new drugs that could provide hope for those diagnosed with metastatic melanoma.
While Professor Samra’s team are using the PEACE study to study metastatic melanoma, there are many other scientists using the valuable data collected to investigate other cancer types. There are researchers investigating lung cancer, renal cancer and many more. What they have in common, is their reliance on patient data.
Dr Lobon emphasised that her work would be impossible without the generous people who agree to donate their samples after they pass. “It’s extremely important. There is no other way we could get such specific information without these samples.”
“Data is everything.”
Case study 3 – Prof Rebecca Fitzgerald
Professor_rebecca_fitzgerald.jpg.
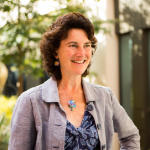
Oesophageal cancer is one of the biggest challenges in cancer research today. The underlying biology of the disease is poorly understood and survival remains very low, with only 1 in 10 people surviving their disease for 10 years or more. It’s a big challenge – and one that researchers and clinicians can’t tackle alone.
For over 10 years, Professor Rebecca Fitzgerald at the University of Cambridge has been spearheading a huge project that brings together scientists, doctors and nurses from across the UK with the goal of better understanding oesophageal cancer.
Known in the research community as OCCAMS (Oesophageal Cancer Clinical and Molecular Stratification), this impressive programme of work has seen researchers collecting tumour and blood samples from people with oesophageal cancer and decoding the cancer’s genetic sequence so that they have a complete map of the cancer’s DNA.
These samples provide a treasure trove of information, allowing scientists to see how changes in DNA sequence affects progression of oesophageal cancer. And every person’s data provides a new piece of the puzzle.
“This really is a team effort,” says Rebecca. “By sharing information, we can see the patterns and the trends and that’s what allows the breakthroughs to happen. Right now we can’t predict how well someone will do just by how they look when we first see them in the clinic. Some people do well, and sadly some people do badly, and we need to know why.”
And there are a lot of questions that need answering. How do genetic changes in the cancer change the way it develops over time? Are there specific risk factors that could predict if oesophageal cancer is going to recur? Why do some people respond well to treatment and some people don’t? All these questions rely on matching up clinical data (data collected by doctors about how each person’s cancer progresses) with laboratory data (data that comes from samples that scientists take away to analyse in the lab).
“And we need a lot of data,” explains Rebecca. “Oesophageal cancer is really complicated. We can’t find the needle in the haystack by just using data from a few people. The current problem my team is working on – working out whether all oesophageal cancers start from the precursor condition Barrett’s oesophagus – is using data from 4,000 patients. And that is why we are so grateful to all the patients who allow us to do this work.”
In the end, this work is all driven by the desire to make the situation better for people with oesophageal cancer. Already the team have been able to describe for the first time some of the DNA mutations that they think cause cancer, and there are likely to be many more findings to come.
“We have seen some improvements over the 20 years I have been working on this,” reflects Rebecca, “but we’ve got a long way to go. Solving this problem is what gets me out of bed in the morning. And I want to get to the end of my career and see that it is really different. I can’t do what I do without patient data – and I am not done yet.”
Case study 4 – Dr Rajesh Jena
Personalising radiotherapy – how patient data is helping reduce the side effects of treatment
Radiotherapy is the gold standard of treatment for many types of cancers. In fact, more than 130,000 patients benefit from radiotherapy every year in the UK. Today, most people receive image-guided radiotherapy – that is, using imaging such as x-rays and MRIs to target the beam of radiation to the tumour site, to make it as accurate as possible.
“Many people think about imaging at the point of diagnosis to find a tumour, or to follow up on treatment to see if its working,” says Dr Rajesh Jena, a clinician scientist based in Cambridge who is finding ways to improve radiotherapy for people with tumours of the brain and spine. “But imaging can be so much more: it can be used to personalise and even predict response to treatment.”
With radiotherapy there can be side effects because the beam of radiation can also affect healthy tissue surrounding the tumour. Combining imaging while delivering radiotherapy can help minimise these side effects, and getting better at combining these approaches is where patient data can be so important.
By studying images taken during radiotherapy, researchers can determine which healthy tissues were affected by the radiation, and then use that information to help predict how a new patient might respond to treatment, potentially altering the treatment plan to make it as side-effect free as possible.
“The wealth of information an image holds comes with an incredible potential,” says Dr Jena. Such potential to inform patient care led Dr Jena and his team to develop the first continuously learning artificial intelligence (AI) medical device in his hospital. Using patient data to train the AI, they were able to develop a tool that speeds up analysis of images. The technology helps doctors by cutting the amount of time spent ‘sketching’ around healthy organs, as they create radiotherapy plans, a painstaking yet vital step in radiotherapy treatment to ensure healthy tissue is protected.
And this data offers value far beyond direct medical treatment. “It’s not only doctors that need access to imaging data like this,” Dr Jena adds. “Patients are so generous allowing us to use this data, so we try to maximise its value by working with mathematicians, physicists, biologists and many other experts.” Bringing together different specialisms like this gives the scientists the best chance of understanding a disease as complex as cancer.
“And we also look back at historical patient images – this is important to provide context, improve our understanding and build up a valuable database of information.”
Thanks to patient data, Dr Jena and his team have already been able to create a technology that frees up valuable time and ultimately enable patients to receive treatment sooner. Over the next year, Dr Jena and his team are working on improving this technology by providing the AI with even more examples of patient images to learn from, allowing it to become better than ever at identifying healthy tissue.
“We’re so grateful to have access to patient imaging data because without it we wouldn’t be able to develop technologies like this, which can make a real difference to people with cancer. We’re very careful with how we use patient data, we take care to ensure that all data is anonymised and is treated with respect.”
Case studies in detail
The earlier diagnosis of lung cancer will save lives but also puts additional pressure on diagnostic services. It is therefore important that lung cancer pathways are organised to be as effective and efficient as possible and to ensure patients are given their diagnosis as soon as possible.
We hoped to improve understanding of pre-diagnostic events, intervals and patterns for lung cancer patients on a national scale, and to benchmark to the timings in the newly adopted National Optimal Lung Cancer Pathway (NOLCP).
The data used included: lung cancer registrations (2013-2015) from National Cancer Registration Analysis Service (NCRAS), diagnostic imaging data (DID) and cancer waiting times (CWT) data from NHS England; and involved data from over 100,000 patients.
Following data linkage, time intervals between events were calculated, different scenarios of events were investigated and timings of events were compared with those from the NOLCP.
Many different diagnostic scenarios exist, from simple to complex, which varies by CCG. Time intervals from imaging to diagnosis differed by source of image referral, with those ordered by GP direct access imaging having longer pathways. Benchmarking to the NOLCP timings showed small proportions (less than 6%) of patients meeting timings, this also varied widely by CCG.
Partners: ACE Programme (funded by CRUK, Macmillan, NHS England), CRUK-NHS England Partnership, NCRAS
See also: ACE Programme website , More in-depth findings
Survival for ovarian cancer in the UK is lower than other comparable high-income countries and there is substantial regional variation within the UK. Access to and/or quality of treatment may be contributing factors.
This analysis will establish a detailed picture of ovarian cancer treatments and outcomes and is intended to provide initial insights into a planned national ovarian cancer audit benchmarking pilot, with the ultimate aim to act as a catalyst to reduce variation and drive improvements to clinical practice.
Data used will be: patients diagnosed with ovarian cancer (1st July 2014 to 31st March 2015) who were eligible for chemotherapy; information about their tumour, chemotherapy treatment received, and demographic information.
The linked data will be used to compare treatment access rates between Cancer Alliances and/ or Trust of Multi-Disciplinary Team/diagnosis, to determine the extent to which this can be explained by regional differences in patient demographics, such as age and socioeconomic status, and we will investigate the influence of healthcare system levels factors on access to treatment, such as provider and type, and consultant volume and specialisation.
Findings are due Winter 2018, but we expect to find how much geographical variation in access to treatment for ovarian cancer there is in England, and to what extent is this affected by patient demographics and healthcare system level factors.
Partners: NHS England
The purpose of this service evaluation is to establish; factors that affect access to lung cancer treatments for non-small cell lung cancer (NSCLC) patients, whether there is variation between Cancer Alliances/ hospital trusts in access to a range of treatment pathways, and the impact of patient, tumour and provider characteristics on access to treatments.
Data used will be: patients diagnosed with lung cancer (1st April 2014 to 31st March 2015) who were eligible for chemotherapy; information about their tumour, chemotherapy treatment received, and demographic information.
Understanding variation in accessing treatments will inform policy makers and commissioners regarding where efforts should be focused, to ensure equitable access to effective treatments for NSCLC, and improve patient outcomes.
The linked data will be used to calculate access rates for different treatments, and then statistical tests will measure the overall geographic variation and significance. Finally, logistic regression will identify which factors are predictive of whether people receive various treatments (e.g. age, deprivation, ethnicity).
Findings are due early 2019: we expect to establish the presence of variation at a geographic level, and the reaso
In England, less than 60% of eligible bowel cancer screening participants take part in the programme. Cancer Research UK, in partnership with Public Health England, carried out a regional Be Clear on Cancer pilot campaign in the North West of England to encourage participation.
The campaign ran in 33 CCGs and consisted of advertising (including TV) and direct mail. Advertising ran alone for 6 weeks and was later combined for 6 weeks with direct mail in 22 CCGs to first-timers and previous non-responders. The remaining 11 CCGs continued to receive advertising only. The campaign was aimed at people aged 55-74 in the lower socioeconomic groups (C2DE) and skewed towards men; this was based on pilots previously run by Cancer Research UK.
We evaluated whether the campaign advertising, or advertising paired with sending a CRUK-endorsement letter with the kit increased uptake. Differences have also been compared across different socioeconomic groups.
The data we used to do this were extracted from the national bowel cancer screening database. We used age, location, date of test kit received, and date kit sent back. For the direct mail element of the campaign, we obtained a set of data for bowel screening test kit recipients who received a CRUK-endorsement letter, and a separate set of data for bowel screening test kit recipients who did not. We did not receive any names, addresses, or NHS numbers, so no individuals could be identified.
We split participants into 3 groups for the evaluation:
- First timers – people who are invited for bowel screening for the first time
- Previously screened – those who were invited for screening previously and returned completed kits
- Previous non-responders – those who were invited previously but didn’t return completed kits
Results are promising. They showed an increase in uptake of the screening test kit during the live campaign for all groups, as well as a smaller sustained increase in the 3 months following the campaign.
There are upcoming changes to the bowel cancer screening programme which may impact on screening uptake. We recommend waiting for an appropriate time, once changes have been implemented, to consider another campaign.
See also: Definitions of socioeconomic groups , Previous pilots .
COVID RT – assessing the impact of COVID-19 on radiotherapy in the UK
The COVID-19 pandemic has had a significant impact on cancer patients and cancer services across the UK. During the peak of the pandemic, radiotherapy services across the UK continued to treat cancer patients in often challenging circumstances and implemented significant changes to standard practice to minimise the risks to patients of contracting COVID-19 and focus radiotherapy resources where they were most needed. The scale of these changes in radiotherapy practice, the clinical decision-making underpinning them and their impact on cancer patient outcomes is unknown. This study will give a national picture of the decision making by patients and clinical staff and the impact on patient's treatment. It also provides knowledge of how RT was used as a bridge to surgery when surgical services weren't viable due to the pandemic and therefore what are the further requirements for cohorts of patients.
Data have been collected by radiotherapy centres across England specifically for this study as part of patient's routine care. These data were submitted to NHS England (previously Public Health England) under regulation 2 of the Health Service Regulations 2002. NHS England (previously Public Health England) collected and stored these data. A pseudonymised extract of these data was created and transferred to our Cancer Research UK Secure Data Environment (SDE).
These data are being analysed within the CRUK SDE, by named analytical staff at Cancer Research UK and University of Leeds, alongside similar data for other UK nations. Summary results will be calculated and used as the first research project to understand the affect Covid had on radiotherapy treatment. These data will not be linked to other datasets.
Join us on X (previously known as Twitter)
Information on the patient-level data we hold
- Skip to main content
- Keyboard shortcuts for audio player

- Your Health
- Treatments & Tests
- Health Inc.
- Public Health
After 40 years of smoking, she survived lung cancer thanks to new treatments

Yuki Noguchi

Denise Lee on her last day of chemo. In addition to chemo and surgery, she was treated with immunotherapy. She's currently in remission. Denise Lee hide caption
Denise Lee on her last day of chemo. In addition to chemo and surgery, she was treated with immunotherapy. She's currently in remission.
Denise Lee grew up in Detroit in the mid-1970s and went to an all-girls Catholic high school. She smoked her first cigarette at age 14 at school, where cigarettes were a popular way of trying to lose weight.
Instead, her nicotine addiction lasted four decades until she quit in her mid-50s.
"At some point it got up as high as 2.5 packs a day," Lee, 62, recalls.
Yet she didn't think about lung cancer risk — until she saw a billboard urging former smokers to get screened. Lee, a retired lawyer living in Fremont, Calif., used to drive past it on her way to work.
"The thing that caught my attention was the fact that it was an African American female on the front," she recalls.

Shots - Health News
The american cancer society says more people should get screened for lung cancer.
She eventually got the low-dose CT scan recommended for current and former smokers. When doctors found an early, but dangerous, tumor, Lee cried and panicked. Her mother had cared for her father, who'd died of prostate cancer. "My biggest concern was telling my mom," she says.
But that was six years ago, and Lee is cancer free today. Surgery removed the 2-inch tumor in her lung, then new treatments also boosted her immune system, fighting off any recurrence.
Lung cancer remains the most lethal form of the disease, killing about 135,000 Americans a year – more than breast, prostate and colon cancer combined – which is why many people still think of a diagnosis as synonymous with a death sentence. But with new treatments and technology, the survival rates from lung cancer are dramatically improving, allowing some patients with relatively late-stage cancers to live for years longer.
"If you're gonna have lung cancer, now is a good time," Lee says of the advances that saved her.

Denise Lee has been cancer-free for six years. She says she's grateful she got screened and caught her lung cancer early enough that treatment has been effective. Denise Lee hide caption
Denise Lee has been cancer-free for six years. She says she's grateful she got screened and caught her lung cancer early enough that treatment has been effective.
The key breakthrough, says Robert Winn, a lung cancer specialist at Virginia Commonwealth University, is the ability to better pinpoint the mutations of a patient's particular form of cancer. In the past, treatments were blunt tools that caused lots of collateral damage to healthy parts of the body while treating cancer.
"We've gone from that to molecular characterization of your lung cancer, and it has been a game changer," Winn says. "This is where science and innovation has an impact."
One of those game-changing treatments is called targeted therapy . Scientists identify genetic biomarkers in the mutated cancer cells to target and then deliver drugs that attack those targets, shrinking tumors.

CRISPR gene-editing may boost cancer immunotherapy, new study finds
Another is immunotherapy, usually taken as a pill, which stimulates the body's own defense system to identify foreign cells, then uses the immune system's own power to fight the cancer as if it were a virus.
As scientists identify new cancer genes, they're creating an ever-broader array of these drugs.
Combined, these treatments have helped increase national survival rates by 22% in the past five years – a rapid improvement over a relatively short time, despite the fact that screening rates are very slow to increase. Winn says as these treatments get cheaper and readily available, the benefits are even reaching rural and Black populations with historic challenges accessing health care.
The most remarkable thing about the drugs is their ability to, in some cases, reverse late-stage cancers. Chi-Fu Jeffrey Yang, a thoracic surgeon at Massachusetts General Hospital and faculty at Harvard Medical School, recalls seeing scans where large dark shadows of tumor would disappear: "It was remarkable to see the lung cancer completely melting away."
To Yang, such progress feels personal. He lost his beloved grandfather to the disease when Yang was in college. If he were diagnosed today, he might still be alive.
"Helping to take care of him was a big reason why I wanted to be a doctor," Yang says.
But the work of combating lung cancer is far from over; further progress in lung cancer survival hinges largely on getting more people screened.
Low-dose CT scans are recommended annually for those over 50 who smoked the equivalent of a pack a day for 20 years. But nationally, only 4.5% of those eligible get those scans , compared to rates of more than 75% for mammograms.
Andrea McKee, a radiation oncologist and spokesperson for the American Lung Association, says part of the problem is that lung cancer is associated with the stigma of smoking. Patients often blame themselves for the disease, saying: "'I know I did this to myself. And so I don't I don't think I deserve to get screened.'"
McKee says that's a challenge unique to lung cancer. "And it just boggles my mind when I hear that, because, of course, nobody deserves to die of lung cancer."
Denise Lee acknowledges that fear. "I was afraid of what they would find," she admits. But she urges friends and family to get yearly scans, anyway.
"I'm just so grateful that my diagnosis was early because then I had options," she says. "I could have surgery, I could have chemotherapy, I could be a part of a clinical trial."
And all of that saved her life.
- lung cancer screening
- immunotherapy
- lung cancer
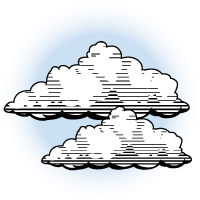
New study offers hope for a rare and devastating eye cancer
After more than a decade studying a rare eye cancer that produces some of the hardest-to-fight tumors, researchers from University of Pittsburgh Medical Center have found a treatment that works on some patients and, more importantly, a tool that can predict when it is likely to succeed.
The work, published in Nature Communications, is being validated in a clinical trial involving at least 30 patients. It could pave the way for similar methods designed to overcome one of the enduring frustrations of cancer care.
Because tumors differ, not only between patients but even inside the same patient, a treatment that works on one mass may fail on another, even when both are of the same cancer type.
The researchers in Pittsburgh tackled this problem in uveal melanoma, an eye cancer that afflicts only 5 people in a million, but that half the time spreads to other parts of the body, often the liver. The median survival once uveal melanoma has spread has been less than seven months, according to a 2018 study in the journal JAMA Ophthalmology.
“We chose this because it was one of the only cancers that 10 years ago when we started, there was nothing approved for it,” said Udai Kammula, who led the study and directs the Solid Tumor Cell Therapy Program at UPMC Hillman Cancer Center in Pittsburgh.
Scientists had long speculated that the reason uveal melanoma is so tough to fight is that something helps the tumor keep out T cells, a key part of the body’s immune system that develops in bone marrow. However, previous studies by Kammula and his colleagues showed that uveal melanoma tumors actually have T cells inside, and they are turned on.
The problem? The cells lie dormant instead of multiplying and reaching numbers large enough to overwhelm the tumor.
The culprit appears to reside somewhere inside the tumor’s ecosystem of cells, molecules and blood vessels, known formally as the tumor’s “microenvironment.” Kammula compares this ecosystem to the infrastructure that supports a city. Something in that infrastructure helps protect uveal melanoma tumors by preventing the critical T cells from multiplying.
“Ultimately, if we’re going to get rid of cancer, we have to get rid of this infrastructure,” Kammula said.
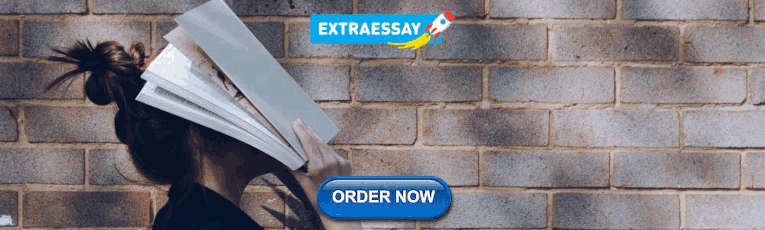
A tool for predicting success
He and his colleagues have had some success using a treatment known as adoptive cell therapy, which was developed in the 1980s by Steven Rosenberg at the National Institutes of Health.
The treatment involves removing the T cells from the tumor, where they have been unable to proliferate. Scientists then take those T cells and grow them outside the body in a lab dish. They treat patients with chemotherapy to kill off the last of their old immune systems. Finally, they reinfuse the lab-grown T cells into the patient’s blood stream and the cells, now in much greater numbers, go on to attack the tumor.
In this treatment, the T cells are often referred to as tumor-infiltrating leukocytes, or TILs.
Kammula said his team has found that tumors shrink partially or completely in about 35% of patients who receive the treatment. But they wanted to know why it doesn’t work in the majority of cases, and whether there might be some way to predict beforehand when it will succeed.
To find out, the researchers analyzed samples from 100 different uveal melanoma tumors that had spread to different parts of the body in 84 patients, seeking to examine all of the tumors’ genetic material.
“We basically put the tumor biopsy in a blender that had the stroma (supportive tissue), the blood vessels, the immune cells, the tumor cells. It had everything,” Kammula said, explaining that they then analyzed all of the tumor’s genetic material.
They found 2,394 genes that could have helped make the tumor susceptible to treatment, some of them genes that experts would regard as “the usual suspects” and others that were unexpected. Using this long list of genes, the scientists searched for characteristics that they shared.
The genes were predominantly involved in helping the body defend itself against viruses, bacteria and other foreign invaders by removing the invaders and helping tissue heal. Kammula and the study’s lead author, Shravan Leonard-Murali, a postdoctoral fellow in the lab, used the different activity levels of these genes to develop a clinical tool.
The tool, known as a biomarker, assigns a score to a uveal melanoma tumor based on the likelihood that it will respond well to the treatment – removing T cells, growing them outside the body, then reinfusing them.
So far, Kammula said, the biomarker has been “extremely good,” in predicting when the treatment will be effective, though he added, “these findings will need confirmation in the current ongoing clinical trial.”
“I thought it was somewhat of a tour de force, honestly,” said Eric Tran, an associate member of the Earle A. Chiles Research Institute, a division of Providence Cancer Institute in Portland, Ore. Tran did not participate in the study.
He said that while it will be important to validate these results, “I was certainly encouraged by their studies. And from my perspective, I wonder if that sort of strategy can be deployed in other cancers.”
Ryan J. Sullivan, an oncologist at Massachusetts General Hospital and associate professor at Harvard Medical School who was not involved in the study, called the team’s work “timely” and said “it is even more significant that they appear to have a [tool] that appears to predict which patients will benefit.”
The team at UPMC is already investigating possible wider application of the treatment and the biomarker in a second clinical trial that involves a dozen different cancers.
Returning to the ranch: Roy’s stroke survival story
Thanks to a persistent wife, a fast-acting care team, and a serendipitous day off, Roy Hallmark has returned to life on his rural Priest Lake ranch after suffering a stroke.
- Open access
- Published: 26 April 2024
From algorithms to action: improving patient care requires causality
- Wouter A. C. van Amsterdam 1 , 2 ,
- Pim A. de Jong 2 ,
- Joost J. C. Verhoeff 3 ,
- Tim Leiner 2 , 4 &
- Rajesh Ranganath 5
BMC Medical Informatics and Decision Making volume 24 , Article number: 111 ( 2024 ) Cite this article
Metrics details
In cancer research there is much interest in building and validating outcome prediction models to support treatment decisions. However, because most outcome prediction models are developed and validated without regard to the causal aspects of treatment decision making, many published outcome prediction models may cause harm when used for decision making, despite being found accurate in validation studies. Guidelines on prediction model validation and the checklist for risk model endorsement by the American Joint Committee on Cancer do not protect against prediction models that are accurate during development and validation but harmful when used for decision making. We explain why this is the case and how to build and validate models that are useful for decision making.
Peer Review reports
Introduction
Treatment decisions in cancer care are guided by treatment effect estimates from randomized controlled trials (RCTs). RCTs estimate the average effect of one treatment versus another in a certain population. However, treatments may not be equally effective for every patient in a population. Knowing the effectiveness of treatments tailored to specific patient and tumor characteristics would enable individualized treatment decisions. Getting tailored treatment effects by averaging outcomes in different patient subgroups in RCTs requires an infeasible number of patients to have sufficient statistical power in all relevant subgroups for all possible treatments. Instead, we must rely on statistical modeling, potentially using observational data from non-randomized studies to further the individualization of treatment decisions.
The American Joint Committee on Cancer (AJCC) recommends that researchers develop outcome prediction models in an effort to individualize treatment decisions [ 1 , 2 ]. Outcome prediction models, sometimes called risk models or prognosis models, use patient and tumor characteristics to predict a patient outcome such as cancer recurrence or overall survival. The assumption is that the predictions are useful for treatment decisions using rules such as “prescribe chemotherapy only if the outcome prediction model predicts the patient has a high risk of recurrence”. Many outcome prediction models are published every year. Recognizing the importance of reliable predictions, the AJCC published a checklist for outcome prediction models to ensure dependable prediction accuracy in the patient population for which the outcome prediction model was designed [ 1 ]. However, accurate outcome predictions do not imply that these predictions yield good treatment decisions. In this comment, we show that outcome prediction models rely on a fixed treatment policy which implies that outcome prediction models that were found to accurately predict outcomes in validation studies can still lead to patient harm when used to inform treatment decisions. We then give guidance on how to evaluate whether a model has value for decision-making and how to develop models that are useful for individualized treatment decisions.
Accurate predictions have unknown value for decision-making
Individualizing treatment decisions means changing the treatment policy . For example, if for a specific cancer type and stage the current treatment policy is to give the same treatment to all patients, then individualizing treatment decisions means recommending treatments tailored to a patient’s characteristics. The value of an outcome prediction model is not in how well it predicts under a certain historic treatment policy, but rather what is the effect of deploying this model on treatment decisions and patient outcomes?
Consider an outcome prediction model that uses pre-treatment tumor characteristics to predict an outcome but ignores whatever treatment the patients may have had, i.e. treatment-naive models (e.g. [ 3 , 4 , 5 ]). Interestingly, the decision to ignore treatments in the outcome prediction model is in line with the AJCC checklist for outcome prediction models (item 12 [ 1 ]). However, these outcome prediction models can cause more harm than good when used to support treatment decisions, even when they are accurate under the historic treatment policy. Consider for example an outcome prediction model that predicts overall survival for stage IV lung cancer patients based on the pre-treatment growth-rate of the tumor. An accurate model would predict shorter survival for patients with faster growing tumors. Applying this outcome prediction model, a clinician could decide to refrain from palliative radiotherapy in patients with faster growing tumors under the assumption that their life expectancy is too short to benefit from radiotherapy. This decision based on the outcome prediction model would be unjustified and harmful, as faster growing tumors are more susceptible to radiotherapy [ 6 ]. See Fig. 1 for an illustration of introducing an outcome prediction model for treatment decisions.
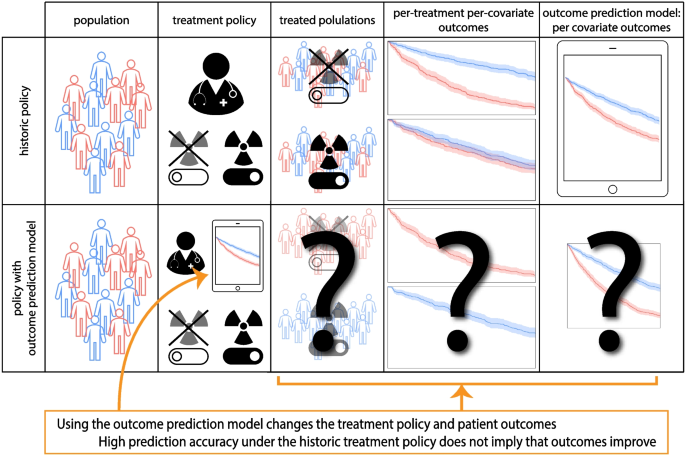
Illustration of the use of outcome prediction models that ignore treatment allocations in the historical data (i.e. are treatment naive ) for treatment decision making. These models change the treatment decisions and thus patient outcomes but whether this change improves patient outcomes is not determined by the prediction accuracy of the outcome prediction model
Prospective validation does not test value for decision-making
The gold standard for evaluating the accuracy of an outcome prediction model is prospective validation [ 1 , 7 ]. In a prospective validation, patient characteristics and outcomes are recorded for a new patient cohort according to a predefined protocol. Comparing the outcome prediction model’s predictions with the observed outcomes provides an estimate of how accurate the outcome prediction model is outside the cohort in which the model was developed. The outcome prediction model from the lung cancer example above, if well-estimated, would be found accurate in a prospective validation that uses the historic treatment policy because the outcome prediction model was developed under the same historic policy. It would then fulfill all the AJCC checklist items but still lead to patient harm when used for treatment decisions because the differential effect of radiotherapy depending on tumor growth-rate is not accounted for in the outcome prediction model.
As an additional validation step, one may conduct a prospective validation study where the outcome prediction model is used for treatment decisions in new patients, thus changing the treatment policy. If such a validation were carried out for the lung cancer survival outcome prediction model, the patients with fast-growing tumors would be given radiotherapy less often due to the predictions of the outcome prediction model, leading to even worse survival for these patients than before introduction of the outcome prediction model. Introducing the outcome prediction model for decision making caused harm because under the new policy treatments are withheld from those who would have benefited most (the patients with fast-growing tumors). However, in this validation study with model deployment the prediction model is still accurate as the model already predicted that patients with fast-growing tumors have a poor prognosis.
Models should improve decisions
The crux of the issue with outcome prediction models is that they answer the question “What is the chance of the outcome given these patient and tumor characteristics, with the assumption that we will keep making the same treatment decisions as we always did ?”. Similar issues exist with other kinds of outcome prediction models which make predictions using the historical treatments but without regards to the policy for how those treatments were assigned (i.e. post-decision models such as [ 8 , 9 , 10 , 11 , 12 ]). Post-decision outcome prediction models are also in line with the AJCC checklist (item 12 [ 1 ]). To improve treatment decisions however, we need models with a foreseeable positive effect on outcomes when used in decision-making.
Outcome prediction models assume treatment decisions follow the historical policy and thereby cannot inform us on the effect of a new policy derived from the outcome prediction model. This reliance on the historical treatment policy leads to a fundamental gap between a prediction model’s accuracy and its value for treatment decision-making in clinical practice (Fig. 2 ). Bridging the gap from prediction accuracy to value for decision making is only possible with causality . Evaluating the effect of a prediction model-based treatment policy on patient outcomes requires a causal study design or causal assumptions.
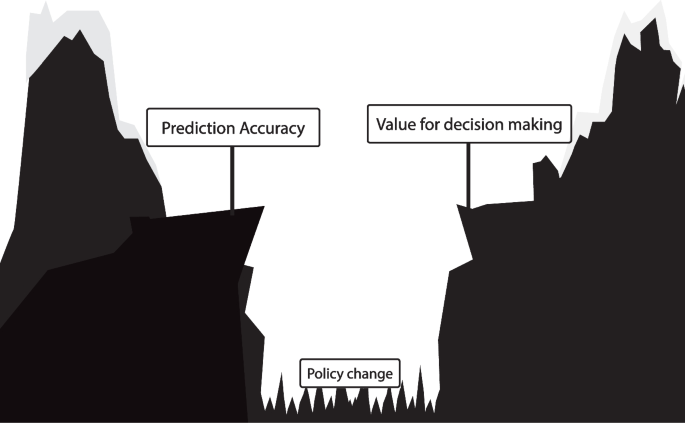
Illustration of the difference between outcome prediction model accuracy and its value for treatment decision making. Validation of an outcome prediction model following the AJCC checklist leads to a reliable estimate of the outcome prediction model’s accuracy if the treatment policy does not change. However, because the outcome prediction model relies on a fixed historic treatment policy, prediction accuracy does not imply value for decision making, as visualized with the gap. This gap can only be bridged with causality
How to validate models used for treatment decisions?
The ultimate test of the effect of introducing a new treatment policy for example based on an outcome prediction model is a cluster randomized controlled trial [ 7 , 13 ]. In a cluster RCT with outcome prediction models, some groups of clinicians are randomly selected to get access to the model while others are not. This allows for the estimation of the effect of introducing the model on treatment decisions and patient outcomes. For example, the cluster RCT could demonstrate that using the model leads to fewer treatment side effects and better overall survival. However, in the context of shared decision-making, patients may weigh the value of overall survival versus treatment discomfort differently [ 14 ]. These individual preferences need to be taken into account in the cluster RCT when calculating the value of introducing a model for decision-making.
As an alternative to cluster RCTs, the expected outcomes under a treatment policy (e.g. based on a prediction model) can be evaluated in data from a standard RCT. This can be done by calculating the average outcome in the subgroup of patients for whom the randomized treatment assignment was concordant with the policy [ 15 ]. Multiple policies can be compared this way, for example comparing a policy based on a new prediction model with current clinical practice. The policy with the best outcomes is preferable. However, such an analysis does not take into account that in practice the compliance with the new treatment policy might not be perfect. Notably, the validation steps recommended in the AJCC checklist [ 1 ] provide no information on what the effect is of deploying an outcome prediction model on treatment decisions and patient outcomes.
Building models to individualize treatment decisions
Cluster RCTs are costly and time consuming. With tools from causal inference we can improve the chance of success of models for decision making. One way to construct a good individualized treatment policy is with models that predict the outcome under hypothetical interventions, where the intervention is the decision to give a certain treatment. The optimal treatment policy selects the treatment that leads to the most beneficial expected outcome.
Estimating such models for prediction under intervention requires unconfoundedness, which holds when there are no unknown variables that influence both the treatment assignment and the outcome (i.e. confounders). RCTs are ideal for this as unconfoundedness holds by design because the treatment assignment is random. However, individual RCTs are generally too small to include many important patient and tumor characteristics in the modeling. Observational data from regular clinical practice on the other hand are often more readily available. If all variables that influence the treatment policy are available in a particular dataset, meaning that unconfoundedness holds, there are many approaches to prediction under intervention. These include ‘conventional’ statistical approaches such as regression, or machine learning approaches, for example using neural networks [ 16 ].
To express available background knowledge and judge whether unconfoundedness holds in observational data, researchers can use directed acyclic graphs (DAGs) [ 17 ]. DAGs depict variables (such as treatment, outcome and confounders) and causal dependencies between the variables as arrows that point from a cause variable to an effect variable, see Fig. 3 for an example. Using tools from causal inference , the DAG determines whether a prediction under intervention model can be estimated and if so what confounders need to be accounted for [ 17 ].
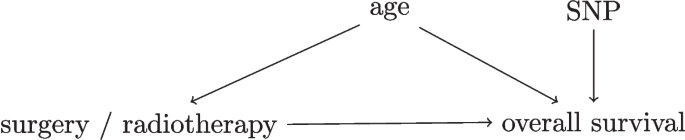
Simplified Directed Acyclic Graph for the decision between surgery and radiotherapy for overall survival in lung cancer patients. As an example, consider a hypothetical study in early-stage lung cancer where researchers investigate whether the relative effectiveness of surgery versus radiotherapy for overall survival depends on a certain single-nucleotide polymorphism (SNP). The SNP assay was performed for the study only so this information did not affect the treatment decision. A DAG with four variables for this study is presented in this Figure. In this DAG, the variables age and SNP both have arrows to overall survival, but only age influences the treatment decision as older patients are less likely to get surgery. This DAG indicates that unconfoundedness holds when age is conditioned on in the analysis, as age is the only confounder between the treatment and the outcome [ 17 ]
In some cases not all confounders are available. In this setting with unobserved confounding standard methods based on confounder adjustment cannot be used, but sometimes prediction under intervention models may be estimated using specialized methods. Two examples are methods based on proxy-variables of unmeasured confounders [ 18 , 19 ] and instrumental variable methods [ 20 ] and their machine learning variants [ 21 , 22 ]. These methods rely on assumptions that may not hold perfectly in reality, so figuratively speaking they might reduce the gap between model accuracy and treatment policy value, but not close the gap entirely. DAGs encode assumptions about the data which may not hold perfectly in practice. The effects of potential violations of these assumptions may be estimated using sensitivity analyses [ 23 ].
A special case for prediction under intervention is the untreated risk, which is the hypothetical outcome under no treatment (or some baseline treatment) and would be observed in the control group of an RCT. For instance, when deciding to give adjuvant therapy after breast cancer surgery, the untreated risk of recurrence is the risk of recurrence when no adjuvant therapy would be given [ 24 ]. Knowing the untreated risk is valuable when considering giving no further treatment, and as a baseline to compare other potential treatments against. Although estimating the untreated risk requires unconfoundedness, in some cases it may be estimated quite accurately even from confounded data using specialized methods [ 25 ].
Because RCTs randomly assign patients to interventions, models for prediction under intervention can be validated in RCTs with standard prediction validation approaches [ 7 ]. For shared decision-making, prediction under intervention of different treatment options allows the patient to make their own judgment on how to weigh e.g. expected overall survival with expected treatment discomfort. Whereas individual RCTs randomize a patient to a certain treatment, cluster RCTs randomize clinicians’ access to a model for decision support. Thereby individual treatment decisions may still be confounded in cluster RCTs meaning that cluster RCTs cannot validate predictions from prediction under intervention -models directly. Both policy evaluation with cluster RCTs and prediction-under-intervention validation in standard RCTs are also possible in observational data but require unconfoundedness and thereby sensitivity analyses for potentially omitted confounders [ 23 , 26 ].
In line with American Joint Committee on Cancer recommendations [ 1 , 2 ] many researchers develop outcome prediction models to individualize treatment decisions. The AJCC checklist provides important guidelines for outcome prediction model development and validation, such as clearly defining the patient population, predictor variables and prediction time-point, in addition to validation in external datasets. These items improve the dependability of outcome prediction models for predicting outcomes in the intended patient population if there are no changes in the treatment policy [ 1 ]. However, not changing the treatment policy directly contradicts the intended purpose of these models. Outcome prediction models that satisfy all the criteria in the checklist still have unknown clinical utility because high prediction accuracy in prospective validation studies does not imply value for treatment decision-making in clinical practice [ 27 ]. Because the gap between outcome prediction model accuracy and value for decision-making is due to causal issues, it is not resolved by larger datasets, more flexible prediction algorithms (e.g. machine learning) or even by prospective validation with model deployment. In contrast, we explained how models for prediction under intervention are useful for decision-making and how to validate any model used in decision-making.
The gap between outcome prediction model accuracy and value for decision-making is due to causal issues, but it is different from the standard “correlation does not imply causation”. In the standard “correlation is not causation” setting, all variables (treatment, outcome, patient/tumor characteristics) are already present in the historical data, whereas in this case, the output of the outcome prediction model cannot be a cause of the outcome. This is because the outcome prediction model is not a variable in historical data, but a shift in policy that changes the distribution of the treatment.
It was noted before that cluster RCTs are the ultimate test for the impact of a new prediction model on clinical practice due to issues related to compliance with treatment recommendations [ 13 ]. We show that because of the gap between prediction accuracy and value for treatment decision-making, many accurate outcome prediction models will fail to demonstrate value in cluster RCTs. Also, cluster RCTs measure the effect of a new policy on average outcomes but do not directly measure whether a model accurately predicts the outcome under intervening to give a certain treatment, for this individually randomized data are most valuable. For shared decision-making accurate predictions-under-intervention may be most important. Two patients with the same predicted outcomes may make a different treatment decision because each patient has their own values and preferences. In a cluster RCT, these individual values need to be accounted for when evaluating a treatment policy, for example by eliciting the values and incorporating them in the analysis when weighing for example overall survival and treatment discomfort.
Previous work underlined the value of prediction-under-intervention models (sometimes referred to as counterfactual prediction ) for supporting treatment decisions [ 28 , 29 ]. Our comment highlights the potential harm of current common practice where outcome prediction models are deployed for decision making based on prediction accuracy alone, further emphasizing the relevance of prediction-under-intervention. In addition, we note how models may be validated for decision support for example with cluster RCTs.
Building models for prediction under intervention is harder than developing outcome prediction models due to the extra requirement of unconfoundedness, which involves formalizing assumptions about confounders for example with DAGs, gathering data on all confounders, often more complex statistical estimation, and sensitivity analyses. When the cost to do a cluster RCT is low, it may suffice to build outcome prediction models in line with the AJCC checklist and test them in cluster RCTs before model deployment. As illustrated in Fig. 4 , when cluster RCTs are costly, impractical or unethical, models that predict under interventions are preferable as they have foreseeable effects when used for treatment decision-making.
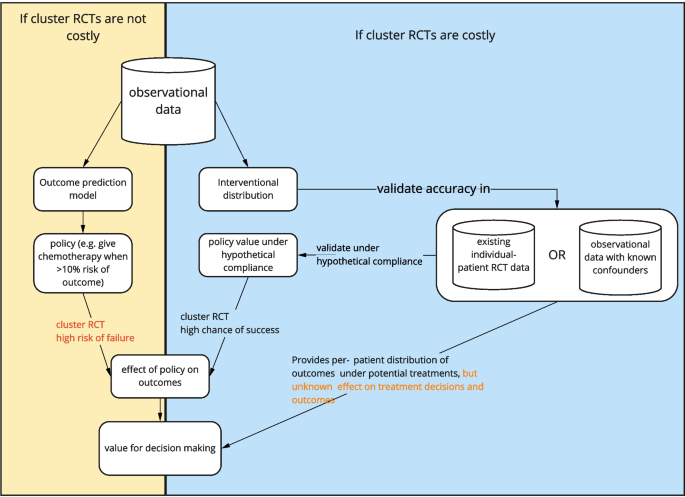
Flowchart of what to do depending on the costliness of cluster randomized controlled trials. Costliness of cluster RCTs should be taken broadly, including time, money and ethical considerations
There is a classical distinction between treatment effect estimation and prediction that amounts to “treatment effect estimation is causal (and thus requires RCTs)” but “prediction is not causal”. When it comes to individualizing treatment decisions with prediction models, this distinction is unhelpful and confusing as the goal is to predict what would happen under different interventions. Selecting the best treatment for a patient is a causal question and requires causal answers.
Availability of data and materials
Not applicable.
Kattan MW, Hess KR, Amin MB, Lu Y, Moons KGM, Gershenwald JE, et al. American Joint Committee on Cancer acceptance criteria for inclusion of risk models for individualized prognosis in the practice of precision medicine. CA Cancer J Clin. 2016;66(5):370–4. https://doi.org/10.3322/caac.21339 .
Article PubMed PubMed Central Google Scholar
Amin MB, Greene FL, Edge SB, Compton CC, Gershenwald JE, Brookland RK, et al. The Eighth Edition AJCC Cancer Staging Manual: Continuing to build a bridge from a population-based to a more “personalized” approach to cancer staging: The Eighth Edition AJCC Cancer Staging Manual. CA Cancer J Clin. 2017;67(2):93–99. https://doi.org/10.3322/caac.21388 .
Salazar R, Roepman P, Capella G, Moreno V, Simon I, Dreezen C, et al. Gene Expression Signature to Improve Prognosis Prediction of Stage II and III Colorectal Cancer. J Clin Oncol. 2011;29(1):17–24. https://doi.org/10.1200/JCO.2010.30.1077 .
Article PubMed Google Scholar
Merli F, Luminari S, Tucci A, Arcari A, Rigacci L, Hawkes E, et al. Simplified Geriatric Assessment in Older Patients With Diffuse Large B-Cell Lymphoma: The Prospective Elderly Project of the Fondazione Italiana Linfomi. J Clin Oncol Off J Am Soc Clin Oncol. 2021;39(11):1214–22. https://doi.org/10.1200/JCO.20.02465 .
Article CAS Google Scholar
Courtiol P, Maussion C, Moarii M, Pronier E, Pilcer S, Sefta M, et al. Deep learning-based classification of mesothelioma improves prediction of patient outcome. Nat Med. 2019;25(10):1519–25. https://doi.org/10.1038/s41591-019-0583-3 .
Article CAS PubMed Google Scholar
Breur K. Growth rate and radiosensitivity of human tumours—II: Radiosensitivity of human tumours. Eur J Cancer (1965). 1966;2(2):173–188. https://doi.org/10.1016/0014-2964(66)90009-0 .
Moons KGM, Altman DG, Reitsma JB, Ioannidis JPA, Macaskill P, Steyerberg EW, et al. Transparent Reporting of a multivariable prediction model for Individual Prognosis Or Diagnosis (TRIPOD): Explanation and Elaboration. Ann Intern Med. 2015;162(1):W1. https://doi.org/10.7326/M14-0698 .
Ryu JS, Ryu HJ, Lee SN, Memon A, Lee SK, Nam HS, et al. Prognostic impact of minimal pleural effusion in non-small-cell lung cancer. J Clin Oncol Off J Am Soc Clin Oncol. 2014;32(9):960–7. https://doi.org/10.1200/JCO.2013.50.5453 .
Article Google Scholar
Fried DV, Mawlawi O, Zhang L, Fave X, Zhou S, Ibbott G, et al. Stage III Non-Small Cell Lung Cancer: Prognostic Value of FDG PET Quantitative Imaging Features Combined with Clinical Prognostic Factors. Radiology. 2016;278(1):214–22. https://doi.org/10.1148/radiol.2015142920 .
Hippisley-Cox J, Coupland C. Development and validation of risk prediction equations to estimate survival in patients with colorectal cancer: cohort study. BMJ (Clin Res Ed). 2017;357:j2497. https://doi.org/10.1136/bmj.j2497 .
Liu R, Rizzo S, Waliany S, Garmhausen MR, Pal N, Huang Z, et al. Systematic pan-cancer analysis of mutation-treatment interactions using large real-world clinicogenomics data. Nat Med. 2022. https://doi.org/10.1038/s41591-022-01873-5 .
Pires da Silva I, Ahmed T, McQuade JL, Nebhan CA, Park JJ, Versluis JM, et al. Clinical Models to Define Response and Survival With Anti-PD-1 Antibodies Alone or Combined With Ipilimumab in Metastatic Melanoma. J Clin Oncol Off J Am Soc Clin Oncol. 2022;40(10):1068–1080. https://doi.org/10.1200/JCO.21.01701 .
Moons KGM, Altman DG, Vergouwe Y, Royston P. Prognosis and prognostic research: application and impact of prognostic models in clinical practice. BMJ. 2009;338:b606. https://doi.org/10.1136/bmj.b606 .
Barry MJ, Edgman-Levitan S. Shared Decision Making – The Pinnacle of Patient-Centered Care. N Engl J Med. 2012;366(9):780–1. https://doi.org/10.1056/NEJMp1109283 .
Karmali KN, Lloyd-Jones DM, van der Leeuw J, Goff Jr DC, Yusuf S, Zanchetti A, et al. Blood pressure-lowering treatment strategies based on cardiovascular risk versus blood pressure: A meta-analysis of individual participant data. PLoS Med. 2018;15(3):e1002538. https://doi.org/10.1371/journal.pmed.1002538 .
Shalit U, Johansson FD, Sontag D. Estimating individual treatment effect: generalization bounds and algorithms. 2017. arXiv:1606.03976 .
Pearl J. Causality. Cambridge University Press; 2009.
Miao W, Geng Z, Tchetgen Tchetgen EJ. Identifying causal effects with proxy variables of an unmeasured confounder. Biometrika. 2018;105(4):987–93. https://doi.org/10.1093/biomet/asy038 .
van Amsterdam WAC, Verhoeff JJC, Harlianto NI, Bartholomeus GA, Puli AM, de Jong PA, et al. Individual treatment effect estimation in the presence of unobserved confounding using proxies: a cohort study in stage III non-small cell lung cancer. Sci Rep. 2022;12(1):5848. https://doi.org/10.1038/s41598-022-09775-9 .
Article CAS PubMed PubMed Central Google Scholar
Wald A. The Fitting of Straight Lines if Both Variables are Subject to Error. Ann Math Stat. 1940;11(3):284–300. https://doi.org/10.1214/aoms/1177731868 .
Hartford J, Lewis G, Leyton-Brown K, Taddy M. Deep IV: a flexible approach for counterfactual prediction. In: International Conference on Machine Learning. PMLR; 2017. p. 1414–1423.
Puli A, Ranganath R. General Control Functions for Causal Effect Estimation from IVs. Adv Neural Inf Process Syst. 2020;33:8440–51.
PubMed PubMed Central Google Scholar
Greenland S. Basic methods for sensitivity analysis of biases. Int J Epidemiol. 1996;25(6):1107–16. https://doi.org/10.1093/ije/25.6.1107-a .
Candido dos Reis FJ, Wishart GC, Dicks EM, Greenberg D, Rashbass J, Schmidt MK, et al. An updated PREDICT breast cancer prognostication and treatment benefit prediction model with independent validation. Breast Cancer Res. 2017;19(1):58. https://doi.org/10.1186/s13058-017-0852-3 .
Van Amsterdam WAC, Ranganath R. Conditional average treatment effect estimation with marginally constrained models. J Causal Infer. 2023;11(1):20220027. https://doi.org/10.1515/jci-2022-0027 .
Keogh RH, van Geloven N. Prediction under interventions: evaluation of counterfactual performance using longitudinal observational data. 2024. https://doi.org/10.48550/arXiv.2304.10005 .
van Amsterdam WAC, van Geloven N, Krijthe JH, Ranganath R, Ciná G. When accurate prediction models yield harmful self-fulfilling prophecies. 2024. https://doi.org/10.48550/arXiv.2312.01210 .
Prosperi M, Guo Y, Sperrin M, Koopman JS, Min JS, He X, et al. Causal inference and counterfactual prediction in machine learning for actionable healthcare. Nat Mach Intel. 2020;2(7):369–75. https://doi.org/10.1038/s42256-020-0197-y .
van Geloven N, Swanson SA, Ramspek CL, Luijken K, van Diepen M, Morris TP, et al. Prediction meets causal inference: the role of treatment in clinical prediction models. Eur J Epidemiol. 2020;35(7):619–30. https://doi.org/10.1007/s10654-020-00636-1 .
Download references
Acknowledgements
Drs. Lidia Barberio, Director of “Longkanker Nederland” (the Dutch patient association for lung cancer) provided feedback on this comment. Her input broadened the scope of this work making it more relevant for patients. Specifically, we added more emphasis on the importance of including the values of the patient in treatment decision-making.
There was no specific funding for this comment.
Author information
Authors and affiliations.
Julius Center for Health Sciences and Primary Care, University Medical Center Utrecht, Utrecht, the Netherlands
Wouter A. C. van Amsterdam
Department of Radiology, University Medical Center Utrecht, Utrecht, the Netherlands
Wouter A. C. van Amsterdam, Pim A. de Jong & Tim Leiner
Department of Radiotherapy, University Medical Center Utrecht, Utrecht, the Netherlands
Joost J. C. Verhoeff
Mayo Clinic, Rochester, MN, USA
Courant Institute of Mathematical Sciences, Department of Computer Science, New York University, New York City, NY, USA
Rajesh Ranganath
You can also search for this author in PubMed Google Scholar
Contributions
Conceptualization: WA, PJ, JV, TL, RR. Writing of draft manuscript: WA and RR. Editing and revision: all authors.
Corresponding author
Correspondence to Wouter A. C. van Amsterdam .
Ethics declarations
Ethics approval and consent to participate, consent for publication.
All authors consent with publication.
Competing interests
The authors declare no competing interests.
Additional information
Publisher's note.
Springer Nature remains neutral with regard to jurisdictional claims in published maps and institutional affiliations.
Rights and permissions
Open Access This article is licensed under a Creative Commons Attribution 4.0 International License, which permits use, sharing, adaptation, distribution and reproduction in any medium or format, as long as you give appropriate credit to the original author(s) and the source, provide a link to the Creative Commons licence, and indicate if changes were made. The images or other third party material in this article are included in the article's Creative Commons licence, unless indicated otherwise in a credit line to the material. If material is not included in the article's Creative Commons licence and your intended use is not permitted by statutory regulation or exceeds the permitted use, you will need to obtain permission directly from the copyright holder. To view a copy of this licence, visit http://creativecommons.org/licenses/by/4.0/ . The Creative Commons Public Domain Dedication waiver ( http://creativecommons.org/publicdomain/zero/1.0/ ) applies to the data made available in this article, unless otherwise stated in a credit line to the data.
Reprints and permissions
About this article
Cite this article.
van Amsterdam, W.A.C., de Jong, P.A., Verhoeff, J.J.C. et al. From algorithms to action: improving patient care requires causality. BMC Med Inform Decis Mak 24 , 111 (2024). https://doi.org/10.1186/s12911-024-02513-3
Download citation
Received : 31 January 2023
Accepted : 15 April 2024
Published : 26 April 2024
DOI : https://doi.org/10.1186/s12911-024-02513-3
Share this article
Anyone you share the following link with will be able to read this content:
Sorry, a shareable link is not currently available for this article.
Provided by the Springer Nature SharedIt content-sharing initiative
- Causal inference
- Tailored treatment decision making
- Prediction research
- Prognosis research
BMC Medical Informatics and Decision Making
ISSN: 1472-6947
- General enquiries: [email protected]

An official website of the United States government
The .gov means it’s official. Federal government websites often end in .gov or .mil. Before sharing sensitive information, make sure you’re on a federal government site.
The site is secure. The https:// ensures that you are connecting to the official website and that any information you provide is encrypted and transmitted securely.
- Publications
- Account settings
Preview improvements coming to the PMC website in October 2024. Learn More or Try it out now .
- Advanced Search
- Journal List
- Front Oncol
- PMC10987809
Assessing of case–cohort design: a case study for breast cancer patients in Xinjiang, China
Mengjuan wu.
1 Country College of Public Health, Xinjiang Medical University, Urumqi, China
Chunjie Gao
2 Department of Medical Record Management, The Affiliated Cancer Hospital of Xinjiang Medical University, Urumqi Xinjiang, China
3 Department of Medical Engineering and Technology, Xinjiang Medical University, Urumqi Xinjiang, China
4 Xinjiang Cancer Center/ Key Laboratory of Oncology of Xinjiang Uyghur Autonomous Region, Urumqi, Xinjiang, China
5 Department of Breast and Thyroid Surgery, The Affiliated Cancer Hospital of Xinjiang Medical University, Urumqi, Xinjiang, China
Shuheng Bai, The First Affiliated Hospital of Xi’an Jiaotong University, China
Associated Data
The data that support the findings of this study are available from the Affiliated Cancer Hospital of Xinjiang Medical University but restrictions apply to the availability of these data, which were used under license for the current study, and so are not publicly available. Requests to access the datasets should be directed to TiZ, [email protected].
To assess the effectiveness and clinical value of case–cohort design and determine prognostic factors of breast cancer patients in Xinjiang on the basis of case–cohort design.
The survival data with different sample characteristics were simulated by using Cox proportional risk models. To evaluate the effectiveness for the case–cohort, entire cohort, and simple random sampling design by comparing the mean, coefficient of variation, etc., of covariate parameters. Furthermore, the prognostic factors of breast cancer patients in Xinjiang were determined based on case–cohort sampling designs. The models were comprehensively evaluated by likelihood ratio test, the area under the receiver operating characteristic curve (AUC), and Akaike Information Criterion (AIC).
In a simulations study, the case–cohort design shows better stability and improves the estimation efficiency when the censored rate is high. In the breast cancer data, molecular subtypes, T-stage, N-stage, M-stage, types of surgery, and postoperative chemotherapy were identified as the prognostic factors of patients in Xinjiang. These models based on the different sampling designs both passed the likelihood ratio test ( p <0.05). Moreover, the model constructed under the case–cohort design had better fitting effect (AIC=3,999.96) and better discrimination (AUC=0.807).
Simulations study confirmed the effectiveness of case–cohort design and further determined the prognostic factors of breast cancer patients in Xinjiang based on this design, which presented the practicality of case–cohort design in actual data.
1. Introduction
Breast cancer with a high mortality rate is one of the most widespread malignant tumors, which seriously threatens women’s health and safety. Global Cancer Statistics 2020 pointed out that there were 2.27 million new cases of breast cancer worldwide, and approximately one in eight patients died of breast cancer in 2020 ( 1 ). Since the twenty-first century, the morbidity and mortality of female breast cancer in China have been continuously increasing ( 2 ), which would cause tremendous burden of breast cancer. Furthermore, breast cancer is highly heterogeneous, with the variety in molecular subtype, clinical stage, and other pathological features ( 3 ). The differences in tumor cell growth rate, invasion ability, and potential metastasis are strongly correlated with patients’ survival prognosis ( 4 ). Survival analysis is widely applied to investigate the relationship among survival time, survival state, and important influencing factors of breast cancer patients. For instance, Ma et al. ( 5 ) studied the serum lipid changes in breast cancer patients during neoadjuvant chemotherapy and the impact of dyslipidemia on their prognosis. Zhou et al. ( 6 ) identified the potential prognostic factors of patients with triple-negative breast cancer and built the corresponding prediction model.
In China, there are endemical variety in the morbidity and mortality of breast cancer ( 7 ). Relevant studies ( 8 – 10 ) showed that the current situation of breast cancer in Xinjiang is different from that in other regions, with such features as lower incidence rate, luminal breast cancer appearing more frequently, and women aged 45–55 having a higher risk of developing this disease. At present, there have been many studies evaluating the prognostic risk factors of patients with breast cancer in Xinjiang ( 11 – 14 ); for instance, Shan et al. ( 11 ) investigated the clinicopathological features and prognostic characteristics of patients with triple-negative breast cancer in Xinjiang, based on clinical information for 319 patients. Fu et al. ( 13 ) focused on the difference in survival and prognosis of breast cancer patients with different molecular subtypes in Xinjiang. Cao et al. ( 14 ) evaluated the association of hypoxia-inducible factor-1α and survivin with breast cancer prognosis in breast cancer patients. However, the sample size of some studies was relatively small ( 11 , 12 ), and those studies were mainly focus on exploring the impact of molecular subtypes or gene expression on the prognosis of breast cancer patients ( 11 , 13 , 14 ). On the other hand, it is necessary to follow up a large number of research subjects over the long-term in survival analysis, which may inevitably cause certain omissions in the process of data collection. Realistically, the breast cancer patients followed up by the hospitals or cancer centers are equivalent to random sample from the overall population. Therefore, it could not totally represent the basic characteristics of the overall population to a certain extent. In particular, a previous study showed that the mortality rate of breast cancer in Xinjiang Cancer Registration Area was only approximately 8.72% ( 15 ). When the incidence of interested event in the follow-up subjects is lower, directly using the data of random samples would cause the insufficient power of statistical analysis ( 16 ). To decrease the sampling error produced by simple random sampling, Prentice ( 17 ) proposed the case–cohort design in 1986. On the basis of simple random sampling, the case–cohort design analyzes those patients who have experienced outcome events in the full cohort, which is suitable for these studies with lower incidence of disease outcomes or higher costs of covariate collections ( 18 – 20 ), and compared with the simple random sampling, the case–cohort design may decrease the sample error ( 21 , 22 ). Yu et al. ( 18 ) separately investigated the relationship between demographic characteristics, tumor histology, and time of onset and recurrence of nephroblastoma patients, under a case–cohort design. Cai et al. ( 19 ) employed a case–cohort design to identify the influencing factors of fungal infection in patients with hematopoietic cell transplant. Particularly, the case–cohort design is widely used to analyze the factors influencing morbidity or mortality of breast cancer ( 20 – 22 ). For example, based on the case–cohort design, Yang et al. ( 20 ) used additive risk model to explore the major prognostic factors of patients with breast cancer. The case–cohort design was employed to evaluate the prospective associations between perfluoroalkyl substances and breast cancer risk in ( 21 ). Yao et al. ( 22 ) used case–cohort design to investigate the association of serum biomarker of vitamin D status, 25-hydroxyvitamin D values with breast cancer recurrence, and survival prognosis. It was indicated that the results based on the case–cohort design with fewer samples were similar to those based on the full cohort. The case–cohort design could be not only suitable for large cohort studies with low incidence but also availably reduce the cost and improve the efficiency. Furthermore, there may be a lack of repeatability in the analysis of actual clinical data; thus, using a case–cohort design could partly decrease the bias generated by random sampling. Therefore, it is significant to further determine the prognostic factors of breast cancer patients in Xinjiang by using a case–cohort design, which could contribute to explore patients’ clinical treatments and improve their survival probability.
Inspired by the aforementioned discussion, in this paper, we first explored the effectiveness of the case–cohort sampling design by using simulated data. To do this, we employed the Cox proportional hazards model to fit the parameters of covariates in these models under full cohort, case–cohort with different sampling proportions, and simple random sampling designs, respectively, and then, we compared these estimated values of parameters for those models (such as the mean, standard deviation, coefficient of variation, and bias). Second, due to the fact that the mortality for the Xinjiang breast cancer patients was relatively lower, we further discussed the applicability of the case–cohort design in identifying the prognostic factors of breast cancer patients in Xinjiang, by comparing the comprehensive performance of these models established under the case–cohort and full cohort sampling designs. These results could offer scientific basis for evaluating the prognosis of breast cancer patients in Xinjiang.
2.1. Case–cohort design
In the case–cohort design, the random subcohort (denoted as S ) was selected by simple random sampling from the full cohort. We denoted S i and δ i as indicator variables, respectively, whether the i th patient was included in the random subcohort and whether the i th patient experienced outcome events. That is, if the i th patient was included in the random subcohort, then S i = 1 , and if the i th patient experienced the outcome event (i.e., case), then δ i = 1 . The case–cohort samples included the random subcohort and all cases outside the random subcohort ( 20 ) (see Figure 1 ). Denote C C i as an indicator variable, the explicit expression is as follows,

Schematic diagram of the case–cohort design.
In this paper, it is assumed that there are N independent individuals in total. For the survival data with censored, the Cox proportional hazards model is used for analysis. Let X be the covariable for the i th individual and β be the partial regression coefficient, then the basic form of the Cox proportional hazards model is,
where h 0 ( t ) denotes the baseline risk function. Since the case–cohort design is a biased sampling, the cases and non-cases in the case–cohort design are equally weighted. The pseudolikelihood is used to infer the partial regression coefficient β , then an estimator for β may be obtained by maximizing the pseudolikelihood function
where T i represents the observed true event time for i th patient, the risk set at time t denoted by ℛ ( t ) = { j : T j ≥ t , j ∈ D ( t ) ∪ S } , and D ( t ) is the collection of cases at time t . Then, the maximum pseudolikelihood estimator for β be solved as
2.2. Simulation study
Let T i * and C i be the time that the interested event occurs or fails and the time that the i th patient was followed up or censored ( i = 1 , … , N ), respectively. If T i * ≤ C i , then the i th patient experienced the outcome event before the end of the observation period. Otherwise, if T i * > C i , then the i th patient is censored. Thus, the observed true event time is defined as T i = min ( T i * , C i ) . Whether or not each patient experienced the outcome event is given by the right censored indicative variable δ i = I ( T i * ≤ C i ) , where I ( · ) is an indicator function.
The time that the interested event occurs or fails for an individual is usually described by using exponential, Weibull, lognormal, and Gamma distributions, etc. The censored time usually follows uniform, exponential distribution and so on ( 23 ). In this paper, the survival data were simulated based on the total number of the full cohort sample N = 5,000 , T i * ~ Weibull ( α , λ ) , and C i ~ uniform ( 0 , θ ) , where the scale parameter λ = 4 , the shape parameter α = 2 , and θ denotes as censored rate. Given that this paper mainly focuses on categorical variables, Bernoulli distributions with different probabilities of occurrence were chosen to fit covariates during the simulation process. Therefore, assuming that there are three covariates for each individual, namely, X 1 , X 2 , and X 3 , generated from Bernoulli distributions with success rates of 0.1, 0.5, and 0.9, respectively, i.e., X 1 ~ B ( 0.1 ) , X 2 ~ B ( 0.5 ) , and X 3 ~ B ( 0.9 ) ( Table 1 ). Then, the Cox proportional hazards model is considered as follows:
Table 1
Different sample characteristics of simulated data.
θ denotes as censored rate. X 1 , X 2 , and X 3 indicate covariates. β denotes the corresponding estimated parameters.
The simulated data with six different sample characteristics was simulated based on different censored rates and regression coefficients ( Table 1 ).
In the following, we compared the parameter estimations of these sampling designs:
FC: parameter estimations based on full cohort ( β ^ FC ).
CCI: parameter estimations based on a case–cohort design with one-third proportion sample ( β ^ CCI ).
CCII: parameter estimations based on a case–cohort design with one-sixth proportion sample ( β ^ CCII ).
RS: parameter estimations based on random subcohort with one-third proportion sample ( β ^ RS ).
The simulated data were sampled 1,000 times for parameter estimations. The mean, standard error of the mean (SE.mean), standard deviation (SD), coefficient of variation (CV), range, and bias of these parameters were compared to assess the performance of different sampling designs.
2.3. Analysis of breast cancer data
The breast cancer patients collected in this paper was sourced from the Affiliated Cancer Hospital of Xinjiang Medical University. Based on full cohort and case–cohort sampling designs, the survival data of these patients were analyzed to identify the independent prognostic factors of breast cancer patients in Xinjiang, by using Kaplan–Meier analysis, Cox proportional hazards model, and stepwise regression. Meanwhile, the parameter estimations of those models were compared to evaluate the comprehensive performance of these models based on case–cohort and full cohort sampling designs and then assess the effectiveness and clinical value of the case–cohort design.
Potential influencing factors such as survival status (life or death), survival time, basic demographic, and clinicopathological of patients were gathered. The patients’ histological grades of tumors are divided into low, medium, and high. According to immunohistochemical technique, there are luminal A, luminal B, HER2 overexpression, and triple-negative breast cancer. The TNM staging system is divided into T stage (primary tumor), N stage (regional lymph nodes), and M stage (distant organ metastases). T stage was divided into T1 (tumor size, ≤2 cm), T2 (tumor size, 2–5 cm), T3 (tumor size, >5 cm), and T4 (tumors of any size with direct extension to the chest wall and/or to the skin, that is ulceration or skin nodules, macroscopic nodules); N stage included N0 (no regional lymph node metastases), N1 (micrometastases, or metastases in one to three axillary lymph nodes), N2 (metastases in four to nine axillary lymph nodes), and N3 (metastases in 10 or more axillary lymph nodes); and M stage was split into M0 (no clinical or radiographic evidence of distant metastases) and M1 (distant metastases) ( 24 ). The types of surgery that patients underwent included no surgery, radical surgery, and breast-conserving surgery. In addition, the age [classified into three categories: younger group (≤45 years), middle-aged group (46–69 years), and the elderly group (≥70 years)] and postoperative chemotherapy of patient were also included.
The inclusion criteria for patients were 1) the age of patient was above 18, 2) tumor of primary site was only identified as breast cancer, and 3) the information of clinicopathological and follow-up were complete. Patients were excluded if 1) medical documents were unsigned, such as informed consent and patient instructions, at the time of admission, and 2) the information about the molecular subtypes, clinical stage, types of surgery, etc., were partial. A total of 8,226 breast cancer patients were followed up in this paper, and the end of the follow-up period was 31 December 2021. Among them, 7,948 patients were effectively followed up, with a follow-up rate of 96.62%. According to the inclusion and exclusion criteria, a total of 3,641 patients were ultimately included, of which 326 patients died (i.e., the censored rate more than 90%).
In this paper, all statistical analysis and visualization were conducted using R 4.1.3 software. A p <0.05 based on a two-tailed test was considered statistically significant.
2.4. Model evaluation
2.4.1. likelihood ratio test.
The likelihood ratio test was used to evaluate Cox regression models in general and reflect the fitting effect of the models ( 25 ), based on the following formula,
where χ v 2 ~ χ 2 ( v ) , − 2 L o g L i represents the log-likelihood function value of a regression model with i parameters. The smaller the value of χ v 2 , the better the fitting effect of the model.
2.4.2. Akaike Information Criterion
Akaike Information Criterion (AIC) ( 26 ) is applicable to select the most effective model from various models and evaluate the validity of the modeling results. The general form of this is as follows
where L and K is the maximum likelihood function and the number of independent parameters, respectively. The smaller the AIC value is, which indicates a minimum discrepancy between the probability and the true distribution, the better the model is.
2.4.3. Discrimination
The accuracy of the model predictions is evaluated on the basis of the discrimination. A model showed good discrimination if this model can distinguish whether the patient has reached the endpoint. The area under the receiver operating characteristic (ROC) curve (AUC), which has a value of 0.5–1.0 and the discrimination is better with the higher value of AUC, was used to assess the discrimination of models ( 27 ).
3.1. Results of simulation
In the simulation data of six different sample characteristics, the parameters β 1 , β 2 , and β 3 of these models constructed in FC, RS, CCI, and CCII sampling designs were estimated, where full cohort N = N FC = 5,000 and subcohort N RS = 1,666 (see Table 2 , Supplementary Tables S1 , S2 , respectively).
Table 2
The simulation results of β 1 under different censored rate and sampling design.
θ denotes as censored rate. β 1 , β 2 , and β 3 indicate the estimated parameters.
SE, mean standard error of the mean; SD, standard deviation; CV, coefficient of variation; FC, full cohort; RS, random subcohort; CCI, case–cohort design with one-third proportion sample; CCII, case–cohort design with one-sixth proportion sample.
The estimated results of β 1 , β 2 , and β 3 showed that its mean values were relatively close under different parameter settings of four sampling designs. Its SE.mean, SD, CV, range, and bias were small, which demonstrated that Cox proportional hazards model presents the better ability in the analysis of the simulated data. Moreover, the findings showed that the fitting results of parameters in the RS and CCI sampling designs approached to the same with a large bias in the results of CCII sampling design when θ = 50 % . For instance, in the scenario of θ = 50 % and β 1 = β 2 = β 3 = -1.5 ( Supplementary Table S2 ), the bias value of β 3 in CCII is approximately 0.02, and its SD, CV, and range are also larger than those of other sampling designs.
On the other hand, it was found that when the censored rate increases, the efficiency of simple random sampling design decreases, the range, SD, and CV of parameter estimations under this sampling design become larger, and then the possibility of outlier is increased. In actual application, there may be a large bias in the results of simple random sampling design without repeated sampling. For instance, under the RS sampling design, when θ = 90 % , β 1 = β 2 = β 3 = -1.5 ( Figure 2C ) or 1.5 ( Figure 2D ), respectively, there are many outliers with the ranges of approximately 18 in the fitted values of β 3 and β 1 , which greatly exceeds the ranges of the estimated values under other sampling designs.

Fitting values of β 1 , β 2 , and β 3 under different sampling designs ( θ = 90%). The yellow dashed line represents the initial value of the regression coefficients. (A–C) The fitting values of β 1 , β 2 , and β 3 when the initial regression coefficients are 1.5; (D–F) the fitting values of β 1 , β 2 , and β 3 when the initial regression coefficients are −1.5. FC, full cohort; RS, random subcohort; CCI, case–cohort design with one-third proportion sample; CCII, case–cohort design with one-sixth proportion sample.
Moreover, when the censored rate is high (i.e., θ = 80 % or θ = 90 % ), CCI and CCII sampling designs have good stability, with smaller dispersion degree and variation index of the parameters, especially CCI. CCI sampling design improves the estimation efficiency because only partial samples (approximately 40%) of the full cohort samples were used by this sampling design to reach the fitting result of FC sampling design, as shown in Table 2 , Figure 2 , and Supplementary Figures S1 , S2 . Therefore, when the sample censored rate was 90%, the sample error of the case–cohort design is smaller than that of simple random sampling.
3.2. Results of breast cancer data
In this paper, there were 3,641 breast cancer patients in Xinjiang with a censored rate of more than 90% as full cohort samples, of which only 326 patients experienced the outcome event (i.e., death). Hence, based on the results of the simulation in Section 3.1 , the case–cohort design with a one-third sample proportion was selected to analyze these data. First, one-third of the patients were randomly selected as a random subcohort (1,214 patients) combining with all cases outside the subcohort, and then, a case–cohort sample with 1,418 patients was formed. The basic information about clinicopathological characteristics of patients is shown in Table 3 . Furthermore, Kaplan–Meier analysis was performed to analyze the clinical data of patients based on the full cohort and case–cohort sampling designs, as shown in Figures 3 and 4 , respectively. Then, the statistically significant factors ( p < 0.05) in Kaplan–Meier analysis and factors with clinical practice value were added to the Cox regression model, and the significant prognostic factors were selected by bidirectional stepwise regression.
Table 3
Basic information about clinicopathological characteristics of breast cancer patients in Xinjiang.
x ¯ ± s d denotes mean ± standard deviation.

Results of Kaplan–Meier analysis for the clinical data of breast cancer patients based on the full cohort sampling designs. (A) Age; (B) histological grade; (C) molecular subtyping; (D) T stage; (E) N stage; (F) M stage; (G) types of surgery; and (H) postoperative chemotherapy.

Results of Kaplan–Meier analysis for the clinical data of breast cancer patients based on the case–cohort sampling designs. (A) Age; (B) histological grade; (C) molecular subtyping; (D) T stage; (E) N stage; (F) M stage; (G) types of surgery; and (H) postoperative chemotherapy.
The fitting results of Cox regression model showed that the parameter estimations under the two sampling designs were very close (see Figure 5 ). It was finally determined that molecular subtypes, T stage, N stage, and M stage were the risk factors for prognosis of Xinjiang breast cancer patients ( p <0.05 and HR>1). In detail, patients with clinicopathological features of triple-negative breast cancer, T3, N3, and M1 substages had the highest risk of death. Simultaneously, types of surgery and postoperative chemotherapy were protective factors for independent prognosis ( p <0.05 and HR<1). Patients who underwent breast-conserving surgery, radical surgery, and postoperative chemotherapy had a lower risk of death than others who did not have surgery. Thus, a model that can effectively predict prognosis of patients has been established as follows:

Multivariate Cox regression models of breast cancer patients in Xinjiang with full cohort and case–cohort sampling designs. The red lines and squares reflect the HR and 95%CrI for risk factors, while green reflects the HR and 95%CrI for protective factors. HR, hazard ratio; CrI, credibility interval.
Finally, the performances of these models established on the basis of the case–cohort (CCI) and full cohort (FC) sampling designs were comprehensively evaluated, as shown in Table 4 . Both Cox proportional hazards models established under the two sampling designs passed the likelihood ratio test ( p <0.05), where χ v CCI 2 = 490.05 < χ v FC 2 = 518.80 . In addition, the AIC value (3,999.96) obtained by the CCI was also smaller compared with the FC, which indicated that the fitting effect of the case–cohort sampling design was better.
Table 4
Evaluation indexes of Cox regression models under different sampling designs.
AIC, Akaike Information Criterion; AUC, the area under the receiver operating characteristic curve. CrI, credible interval.
Moreover, ROC curves of Cox regression models under FC and CCI sampling designs were separately drawn to compare the discrimination of these models ( Figure 6 ). It was shown that both AUC values were >0.8, and they were very close, which confirmed a good discrimination for the prognostic model constructed in this paper and also further verified that the case–cohort sampling design reached a better fitting effect only using approximately 38.9% of the full cohort samples.

ROC curves of Cox regression models under different sampling designs. (A) Full cohort design; (B) case–cohort design. AUC, the area under the receiver operating characteristic curve.
4. Discussion
Case–cohort design is suitable for cancer research with large cohort and low incidence, which could improve efficiency and reduce the cost of collecting redundant non-case data ( 18 ). One of the highlights of this paper is that the effectiveness of the case–cohort design was verified based on the Cox proportional risk model, and the different censored rates (50%, 80%, and 90%) and different sampling ratios (1/3, 1/6) were conducted in the simulation study. By simulating the survival data with different sample characteristics, this study estimated the coefficients of Cox regression models in FC, CCI, CCII, and RS sampling designs to assess the performance of the models and sampling designs, respectively. Our findings showed that the case–cohort design could improve the estimation efficiency, especially the higher censored rate. Since the morbidity of breast cancer has been an increasing tendency year by year in Xinjiang ( 10 ), and the mortality for the followed up Xinjiang breast cancer patients was relatively lower, using the case–cohort design could reduce the bias caused by random sampling, more effectively identify prognostic factors, and further promote the improvement of clinical prognostic methods. Therefore, based on the case–cohort design, this study analyzed the actual clinical data of breast cancer patients in Xinjiang to identify independent prognostic factors (molecular subtypes, T stage, N stage, M stage, types of surgery, and postoperative chemotherapy). Another innovation of this paper is that the performance of the model established under the full cohort and case–cohort in the actual data were comprehensively evaluated in breast cancer patients in Xinjiang by likelihood ratio test, AIC criterion, and discrimination. This further confirmed that the prognosis model constructed under the case–cohort sampling design had better fitting effect than that based on the full cohort sampling design, and the case–cohort sampling design showed certain applicability in the actual data.
The results of simulations in this paper displayed that the estimated mean values of regression coefficients were close to the given initial values in the survival data with different scenarios, indicating that Cox proportional hazards model could achieve the better fitting effect. In addition, when the censored rate was lower, the fitting results of the regression coefficients under the RS and CCI sampling designs were nearly the same, while there was a lager bias of the parameter estimations under CCII sampling designs. It demonstrated that not only the suitable sampling designs should be selected but also the sampling proportion should not be too small in the analysis; otherwise, it would also reduce the statistical efficiency. On the other hand, when the censored rate gradually increased, the parameter estimations under the single simple random sampling design would be more likely to generate outliers, which could result in the gradual decrease in efficiency under this sampling design. However, in actual applications, it is often difficult to conduct multiply repeated sampling, which may lead to a significant deviation in the obtained results. Meanwhile, our findings revealed that when the censored rate was higher, the CCI and CCII sampling designs had superior stability (i.e., there are fewer outliers and smaller deviations), especially CCI sampling design. Both estimated mean values under CCI and CCII sampling designs had smaller dispersion degree and variation index, and the CCI design results that only used 38.9% samples of the full cohort samples were close to FC design results. Moreover, using different types of covariates may have a certain influence on the simulation results, but this impact is relatively small, as demonstrated in the paper by Yang et al. ( 20 ), where there were slight differences between simulation results of the normal and uniform distribution. To sum up, the simulation results of this paper confirmed that the case–cohort design is a cost-effective sampling design compared with simple random sampling design, which could improve the efficiency of estimation. In particular, the case–cohort design was more effective and stable when the interested events had a relatively lower incidence, which was consistent with the results in these references ( 18 , 20 , 28 ).
In this paper, the breast cancer patients followed up were registered in the Affiliated Cancer Hospital of Xinjiang Medical University, which could be regarded as a random sample from the overall population, with more than 90% censored rate. Thus, a one-third proportion of case–cohort sampling design was used to analyze these data, and the same Cox regression model was also simultaneously implemented in the full cohort sampling design to compare the difference between the two designs’ results. The results showed that the prognosis of patients with triple-negative breast cancer was the worst, which may be the cause of the tumor cells of those patients being more aggressive and more prone to recurrence and metastasis ( 29 ). Luminal breast cancer patients had better prognosis and higher survival rate than other non-luminal patients. Moreover, T, N, and M stages were independent risk prognostic factors of breast cancer patients. Patients with advanced T stage had larger tumors, more tumor cells, and the longer time for the tumor formation, so these patients would be more likely to develop into distant metastasis breast cancer ones. The later stage of N stage indicated greater probability, more numbers of lymph node metastases, and higher risk of death, which are typical clinical features of breast cancer progression ( 30 ). Because distant metastasis of breast cancer (i.e., M stage) means that the tumors of breast cancer could spread to the lung, liver, brain, and other parts of the body, the occurrence of distant metastasis (i.e., advanced breast cancer) would result in more difficult clinical treatment ( 31 ). Therefore, regular breast self-examination and clinical screening for women were recommended to achieve the purpose of early detection, early diagnosis, and early treatment, and then reduce the mortality and improve the prognosis of breast cancer patients. At the same time, it was also shown that the breast-conserving surgery [HR=0.30, 95%CrI: (0.17, 0.55)], radical surgery [HR=0.52, 95%CrI: (0.35, 0.76)], and postoperative chemotherapy [HR=0.43, 95%CrI: (0.30, 0.61)] were protective factors for breast cancer patients in Xinjiang. These surgeries could effectively reduce the size of the tumor, reduce the number of tumors, and control the spread of the disease, thereby greatly improving the survival probability for breast cancer patients. Initially, the radical surgery, as a common treatment, occupied a very important position. But now, breast-conserving surgery is more widely used to treat patients with early disease progression, with the characteristics of shorter operation time and lower incidence of postoperative complications ( 32 ). Standard postoperative adjuvant chemotherapy for patients could prevent the recurrence and control the metastasis of cancer to a certain extent, and it could reduce the pain, improve the quality of life, and then extend their life cycle for some patients with advanced stage ( 33 ). Finally, the likelihood ratio test, ROC curve, and AIC criteria were used to compare the superiority of model prediction in the full cohort and the case–cohort sampling designs. The comparison findings showed that both models under FC and CCI sampling designs passed the likelihood ratio test ( p <0.05), and the model constructed under the CCI design had better fitting effect (AIC=3,999.96) and better discrimination [AUC=0.807, 95%CrI: (0.780, 0.835)], which demonstrated that the case–cohort design was suitable to analyze the prognosis of breast cancer patients in Xinjiang.
There are some limitations in this study. On the one hand, we only employed Cox proportional hazards model with Prentice’s weight method to investigate the effectivity and stability of the case–cohort design. However, different weighted estimation methods (such as Barlow and Self-Prentice method) or different statistical models (such as additive risk model) could also be applied to make statistical inference to be more accurate and effective under the case–cohort design when the weights of case–cohort samples are not mutually independent or the actual data do not follow the proportional hazards assumption. On the other hand, only the clinical data of breast cancer patients in Xinjiang were analyzed in this paper, but the applicability of the case–cohort design in the other regions or other cancers deserves to be further explored. Last but not least, the main purpose of our paper is to explore the factors affecting the prognosis of breast cancer patients in Xinjiang, based on the case–cohort design and Cox proportional risk model. Hence, we focused on the influence degree of different factors on the occurrence time of the event. It was needed to consider the impact of covariates on survival time and the chronological order of events; therefore, we only reported HR values in the outcome in this paper. In our future work, we will consider different methods such as logistic regression or propensity score to calculate different statistical indicators (such as OR and RR values) ( 34 – 36 ), in order to find the best reporting indicator for actual data with different sample characteristics.
5. Conclusion
In summary, this study demonstrated the effectivity and stability of the case–cohort design through simulating data and confirmed that this design could maintain a better estimation efficiency in cancers with high censored rate. Furthermore, independent prognostic factors of breast cancer patients in Xinjiang were determined under the case–cohort design, and the practical fitting effect and useful application of the case–cohort design were demonstrated by comparing with the results based on full cohort design.
Data availability statement
Ethics statement.
The studies involving human participants were reviewed and approved by Medical Ethics Committee of the Affiliated Cancer Hospital of Xinjiang Medical University (approval number: K-2023001). The participants provided their written informed consent to participate in this study.
Author contributions
MW: Conceptualization, Software, Writing – original draft, Writing – review & editing. GS: Data curation, Funding acquisition, Writing – review & editing. TaZ: Software, Writing – original draft. CG: Methodology, Writing – original draft. TiZ: Data curation, Resources, Writing – review & editing. QZ: Data curation, Resources, Writing – review & editing. LW: Conceptualization, Methodology, Writing – original draft, Writing – review & editing.
Funding Statement
The author(s) declare financial support was received for the research, authorship, and/or publication of this article. This work was supported by the National Natural Science Foundation of China (Grant Nos. 12061079 and 82060520), the Project of Top-notch Talents of Technological Youth of Xinjiang (Grant No. 2022TSYCCX0108), the Natural Science Foundation of Xinjiang (Grant No. 2022D01C287), and the Tianshan Cedar Talent Training Project of Science and Technology Department of Xinjiang Uygur Autonomous Region (Grant No. 2020XS14).
Conflict of interest
The authors declare that the research was conducted in the absence of any commercial or financial relationships that could be construed as a potential conflict of interest.
Publisher’s note
All claims expressed in this article are solely those of the authors and do not necessarily represent those of their affiliated organizations, or those of the publisher, the editors and the reviewers. Any product that may be evaluated in this article, or claim that may be made by its manufacturer, is not guaranteed or endorsed by the publisher.
Supplementary material
The Supplementary Material for this article can be found online at: https://www.frontiersin.org/articles/10.3389/fonc.2024.1306255/full#supplementary-material
ORIGINAL RESEARCH article
Overweight as a biomarker for concomitant thyroid cancer in patients with graves’ disease.
- Department of Surgery, College of Medicine, The Catholic University of Korea, Seoul, Republic of Korea
The incidence of concomitant thyroid cancer in Graves’ disease varies and Graves’ disease can make the diagnosis and management of thyroid nodules more challenging. Since the majority of Graves’ disease patients primarily received non-surgical treatment, identifying biomarkers for concomitant thyroid cancer in patients with Graves’ disease may facilitate planning the surgery. The aim of this study is to identify the biomarkers for concurrent thyroid cancer in Graves’ disease patients and evaluate the impact of being overweight on cancer risk. This retrospective cohort study analyzed 122 patients with Graves’ disease who underwent thyroid surgery at Seoul St. Mary’s Hospital (Seoul, Korea) from May 2010 to December 2022. Body mass index (BMI), preoperative thyroid function test, and thyroid stimulating hormone receptor antibody (TR-Ab) were measured. Overweight was defined as a BMI of 25 kg/m² or higher according to the World Health Organization (WHO). Most patients (88.5%) underwent total or near-total thyroidectomy. Multivariate analysis revealed that patients who were overweight had a higher risk of malignancy (Odds ratios, 3.108; 95% confidence intervals, 1.196–8.831; p = 0.021). Lower gland weight and lower preoperative TR-Ab were also biomarkers for malignancy in Graves’ disease. Overweight patients with Graves’ disease had a higher risk of thyroid cancer than non-overweight patients. A comprehensive assessment of overweight patients with Graves’ disease is imperative for identifying concomitant thyroid cancer.
1 Introduction
Graves’ disease (GD) is an autoimmune disease that causes hyperthyroidism by stimulating the thyroid gland to produce excessive thyroid hormone due to the presence of thyroid stimulating hormone receptor antibody (TR-Ab) ( 1 – 4 ). Surgical intervention is required for the management of GD in cases of failed medical therapy, severe or rapidly progressing disease with compressive symptoms, concomitant thyroid cancer, worsening Graves’ ophthalmopathy, or based on patient’s preference ( 1 , 5 – 7 ).
The reported incidence of concomitant thyroid cancer in patients with GD varies, ranging from 1% to 22%, and some studies reported that the incidence of thyroid cancer is higher in patients with GD than the incidence in the general population ( 8 – 11 ). Although the relationship between GD and thyroid cancer is unclear, GD can make the diagnosis and management of thyroid nodules more challenging ( 12 – 16 ). In patients with GD and concomitant thyroid cancer, most surgeries are planned after nodules are diagnosed by ultrasound or fine-needle aspiration biopsy (FNAB). However, thyroid cancer is occasionally identified incidentally in the pathologic examination after surgery ( 17 – 19 ). These cases are indications that surgery was necessary, and cancer could have been missed if surgery had not been performed for other reasons. Therefore, identifying biomarkers for concomitant thyroid cancer in patients with GD may facilitate planning the surgery and more thorough screening, even if a nodule is not discovered before surgery.
Previous studies have identified risk factors for concomitant thyroid cancer in patients with GD, including TR-Ab, preoperative nodules, previous external radiation, and younger age ( 13 , 20 – 24 ). Regardless of the existence of GD, morbid obesity affects the incidence and aggressiveness of thyroid cancer in euthyroid patients ( 25 – 29 ). However, few studies have investigated the relationship between thyroid cancer in patients with GD and obesity. In a study of 216 GD patients, those with thyroid cancer had significantly higher body mass index (BMI) compared to those without thyroid cancer ( 30 ). Since weight loss is common in patients with GD ( 31 ), investigations into the relationship between being overweight or obese and GD are needed. The aim of this study was to identify biomarkers for concurrent thyroid cancer in patients with GD and identify the effects of being overweight on cancer risk.
2 Materials and methods
2.1 patients.
We retrospectively reviewed the medical charts and pathology reports of 132 patients with GD who underwent thyroid surgery from May 2010 to December 2022 at Seoul St. Mary’s Hospital (Seoul, Korea). Five patients with newly diagnosed GD after lobectomy, one patient with distant metastasis of thyroid cancer at initial diagnosis, one patient who underwent the initial operation at a different hospital, two patients with insufficient data, and one patient who was lost to follow-up were excluded from the study. Thus, 122 patients were included in the analysis ( Figure 1 ). The mean follow-up duration was 52.8 ± 39.6 months (range, 4.8–144.0 months).
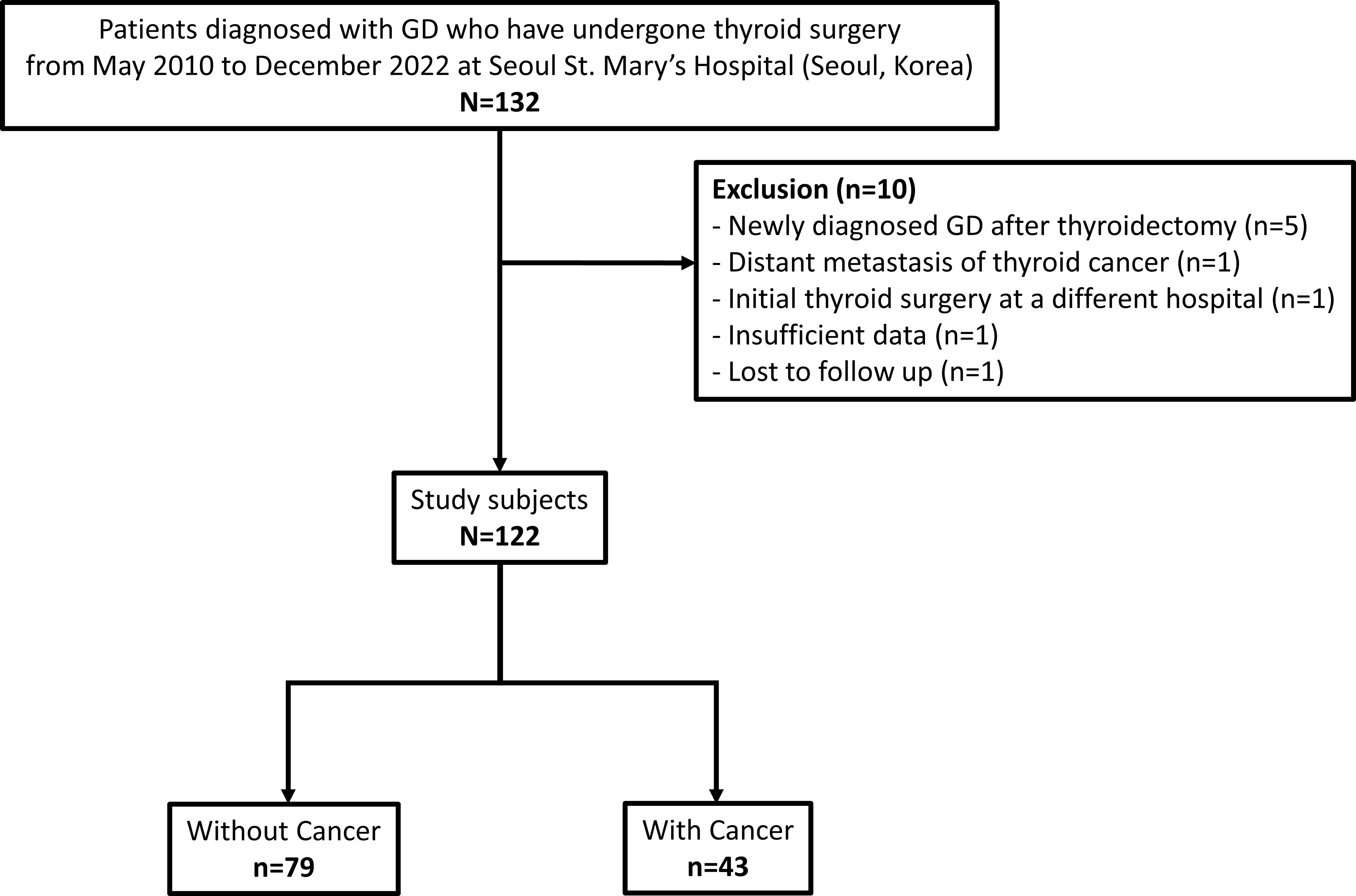
Figure 1 Participant flow diagram of patient selection. GD, Graves’ disease.
Overweight was defined as a BMI of 25 kg/m² or higher according to the World Health Organization (WHO) and the International Association for the Study of Obesity (IASO) ( 32 ). WHO and IASO define obesity as a BMI of 30 or above ( 33 , 34 ). However, only 7 (5.7%) patients were obese in the present study, according to these criteria (BMI ≥ 30 kg/m²). Moreover, Asian countries have lower cut-off values due to a higher prevalence of obesity-related diseases at lower BMI levels ( 35 ). As this study included Korean individuals, the patients were divided by a BMI of 25, which is the standard for overweight defined by WHO and for obesity in Asia ( 36 ).
2.2 Preoperative management and follow-up assessment
Height and weight were assessed in all patients the day prior to surgery to mitigate potential measurement and temporal biases. BMI was calculated by dividing the weight in kilograms by the square of their height in meters (kg/m2). The duration of GD was defined as the number of years between the date of initial diagnosis and the date of surgery. Disease status was assessed using the serum thyroid function test (TFT), including thyroid stimulating hormone (TSH), triiodothyronine (T3), free thyroxine (T4), and TR-Ab levels before surgery, either as outpatients or after hospital admission. Pathology reports were used to review the final results after surgery.
Patients with GD received treatment based on the 2016 American Thyroid Association (ATA) guidelines for hyperthyroidism ( 1 ). Patients with concomitant thyroid cancer were managed according to the 2015 ATA management guidelines for differentiated thyroid cancer ( 37 ). After the thyroidectomy, all patients discontinued antithyroid drugs and started taking L-T4 at a daily dosage suitable for their body weight (1.6 μg/kg). Patients with concomitant thyroid cancer were closely monitored every 3–6 months during the first year and then annually thereafter. Thyroid ultrasonography was conducted annually for patients with cancer.
2.3 Primary endpoint
The primary endpoint was the rate of overweight in GD patients with and without concomitant thyroid cancer.
2.4 Statistical analysis
Continuous variables were reported as means with standard deviations, while categorical variables were presented as numbers with percentages. Continuous variables were compared with Student’s t-tests and Mann-Whitney test, and categorical characteristics were compared using Pearson’s chi-square tests or Fisher’s exact tests. Univariate Cox regression analyses were conducted to determine the biomarkers for postoperative hypoparathyroidism and malignancy in patients with GD. Statistically significant variables were included in the multivariate Cox proportional hazard model. Odds ratios (ORs) with 95% confidence intervals (CIs) were calculated. Statistical significance was defined as p-values < 0.05. The Statistical Package for the Social Sciences (version 24.0; IBM Corp., Armonk, NY, USA) was used for all statistical analyses.
3.1 Baseline clinicopathological characteristics of the study population
Table 1 presents the clinicopathological characteristics of the 122 patients in the study. The average age was 45.7 years (range, 15–77), and the average BMI was 23.4 kg/m2 (range, 17.2–37.0). 35 patients (28.7%) were classified as overweight. The mean disease duration was 5.9 years, and the mean gland weight was 105.6 grams (range, 7.6–471.4). Most patients (110 patients, 90.2%) underwent total or near-total thyroidectomy; 11 (9.0%) patients underwent lobectomy, and one patient (0.8%) underwent total thyroidectomy with modified radical neck dissection (mRND). The 11 patients who underwent lobectomy exhibited proper regulation of thyroid function prior to surgery, and preoperative diagnosis confirmed the existence of unifocal cancer or follicular neoplasm with a size smaller than 2cm (range, 0.3-1.8). The pathology was benign in 79 (64.8%) patients, while 43 (35.2%) patients exhibited malignant pathology. The preoperative TFT showed a mean TSH level of 1.7 ± 7.8 mIU/L (range, 0.0–77.9), a mean T3 level of 1.7 ± 0.7 ng/mL (range, 0.5–5.1), a mean free T4 level of 1.4 ± 0.7 ng/dL (range, 0.3–4.1), and a mean TR-Ab level of 26.4 ± 35.7 IU/L (range, 0.3–292.8). Forty-four patients (36.1%) underwent surgery due to refractory disease or medication complications, 31 (25.4%) patients underwent surgery due to huge goiters with compressive symptoms, 10 patients (8.2%) underwent surgery due to ophthalmopathies, and 37 (30.3%) patients underwent surgery due to cancer or follicular neoplasm diagnoses before surgery. Postoperative complications were described in Supplementary Table 1 . Unilateral vocal cord palsy (VCP) occurred in 3 (2.5%) patients, and no bilateral VCP occurred. Hypoparathyroidism was transient in 48 (39.3%) patients and permanent in 3 (2.5%) patients. No cases of hematoma or thyroid storm occurred.
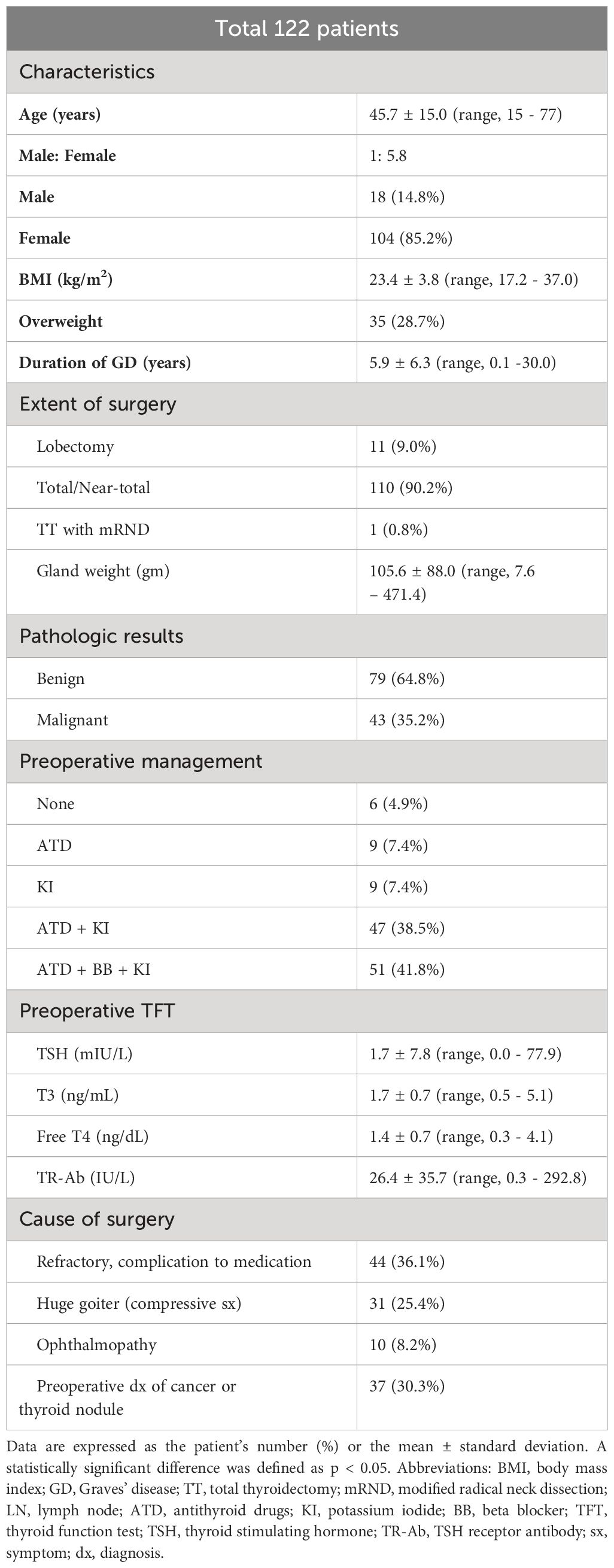
Table 1 Baseline clinicopathological characteristics of the study population.
3.2 Clinicopathological characteristics of thyroid cancer in patients with Graves’ disease
Table 2 shows the clinicopathological characteristics of the 43 patients diagnosed with thyroid cancer. 42 (97.7%) patients were diagnosed with PTC, while 1 (2.3%) patient had minimally invasive Hürthle cell carcinoma. Thirty-four (79.1%) patients were preoperatively diagnosed with papillary thyroid cancer (PTC) or Hürthle cell neoplasm, while cancers were discovered incidentally in 9 (20.9%) patients. Ten (23.3%) patients underwent lobectomy, 32 (74.4%) patients underwent total or near-total thyroidectomy, and one (2.3%) patient underwent total thyroidectomy with mRND. The most prevalent subtype of PTC was the classic type, accounting for 81.0% of PTC cases. Follicular, tall cell, and oncocytic variants comprised 7.1%, 4.8%, and 7.1% of PTC cases, respectively. The average tumor size was 0.9 cm (range, 0.1–3.4 cm). Multifocalities were observed in 19 (44.2%) patients and bilaterality was observed in 11 (25.6%) patients. Lymphatic invasion, vascular invasion, and perineural invasion were observed in 12 (27.9%), 1 (2.3%), and 2 (4.7%) patients, respectively.
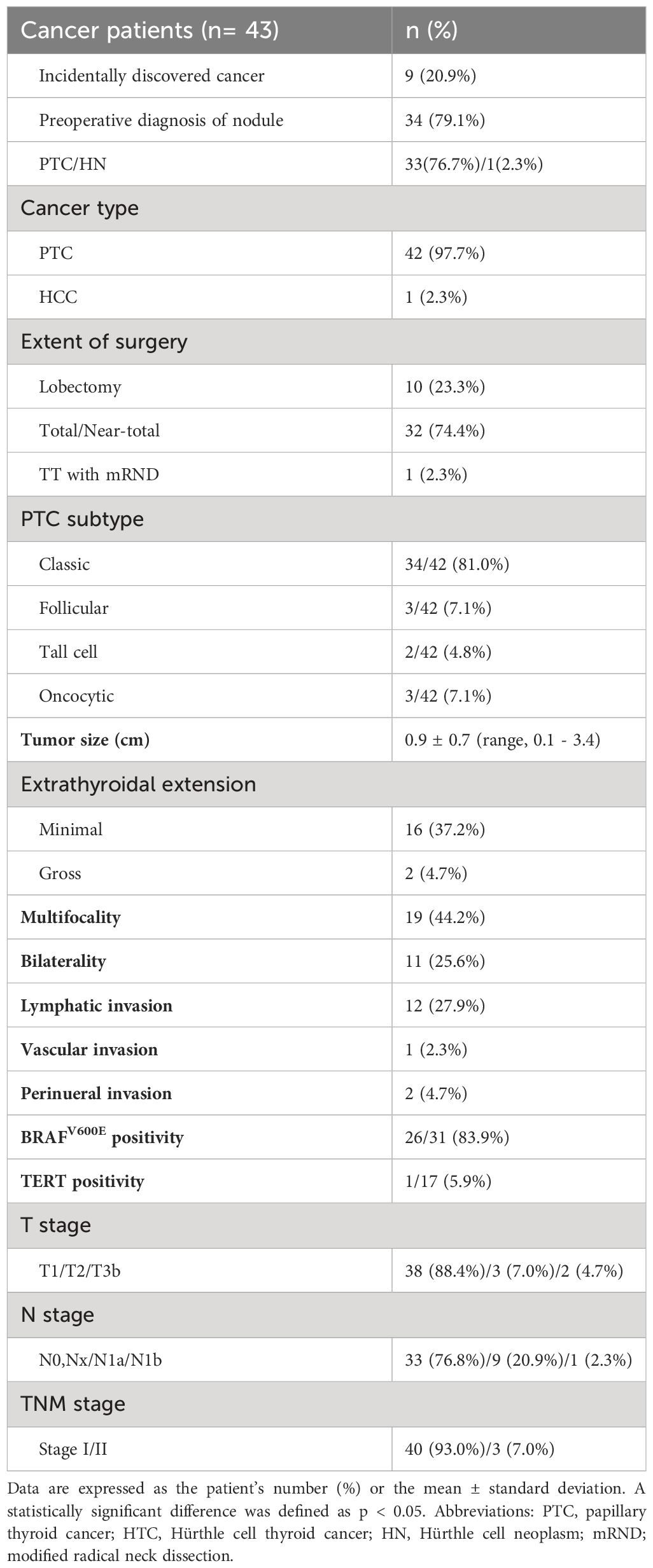
Table 2 Clinicopathological characteristics of thyroid cancer in Graves’ disease.
As shown in Table 3 , the 34 patients who were preoperatively diagnosed with cancers were compared with the 9 patients with incidentally discovered cancers after surgery. No differences in BMI were detected between the two groups (23.3 ± 3.7 vs. 24.4 ± 4.1; p = 0.450). Gland weight was significantly lighter in patients with preoperatively diagnosed cancers compared with gland weights in the incidentally discovered group (35.3 ± 40.1 vs. 119.2 ± 62.9; p < 0.001). TR-Ab levels were significantly lower in the preoperatively diagnosed group compared with the levels in the incidentally discovered group (5.5 ± 5.3 vs. 31.4 ± 28.9; p = 0.005). Tumor size was significantly larger in the preoperatively diagnosed group compared with the size in the incidentally discovered group (1.0 ± 0.7 vs. 0.4 ± 0.2, p = 0.001). The causes of surgery were also significantly different between the two groups ( p < 0.001). In the incidentally discovered cancer group, 66.7% of the patients underwent surgery due to refractory disease or medication complications, 22.2% due to large goiters, and 11.1% due to nodules detected on preoperative ultrasound. In contrast, all surgeries were performed due to the preoperative detection of cancer in the group with preoperative diagnosis.
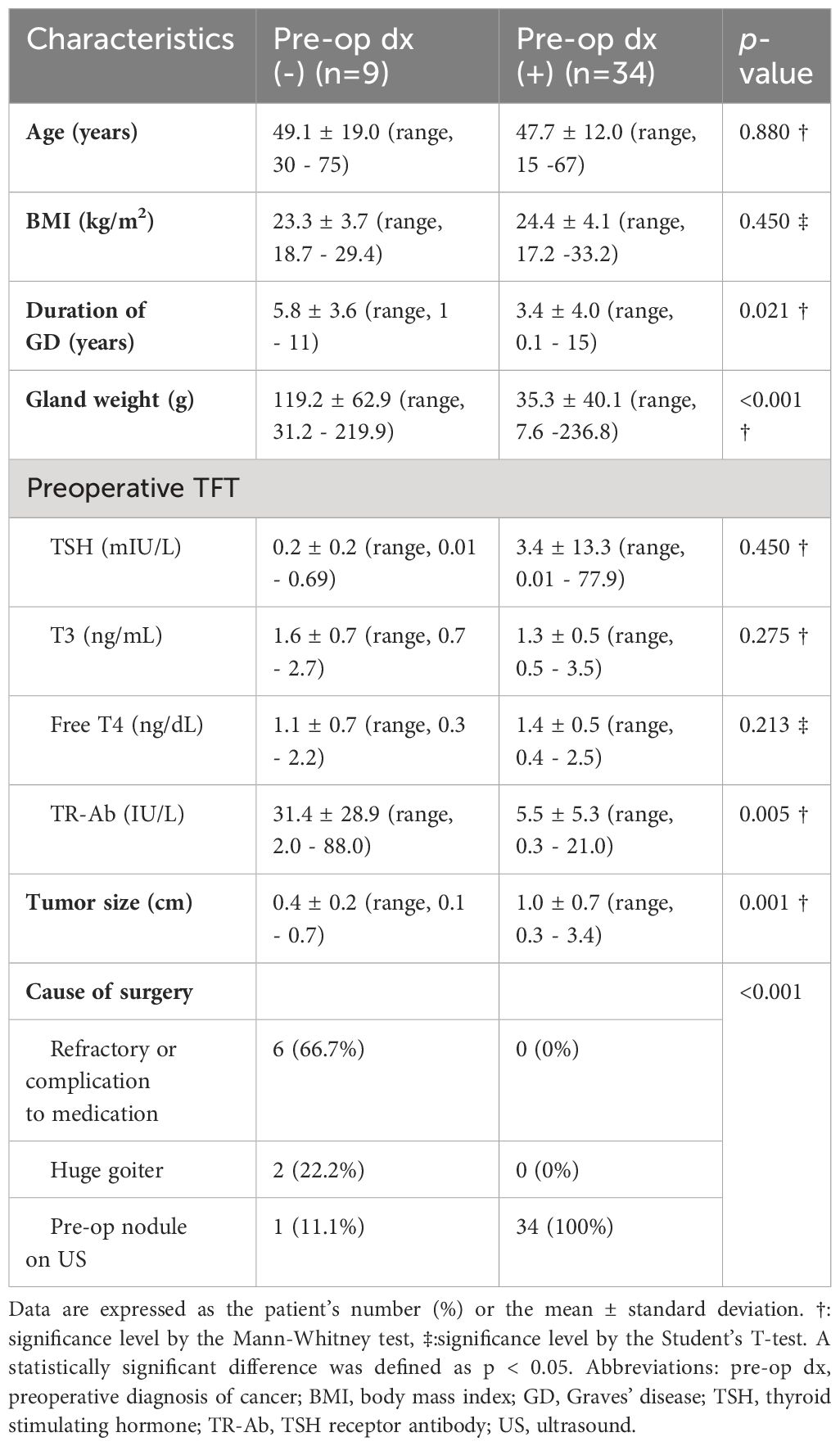
Table 3 Comparison of thyroid cancers in Graves’ disease with or without preoperative pathologic diagnosis.
3.3 Comparison of Graves’ disease subgroups with or without thyroid cancer
Patients with GD with or without thyroid cancer were compared, as shown in Table 4 . Patients with GD and thyroid cancer were significantly more overweight (BMI ≥ 25 kg/m2) than patients with GD without thyroid cancer (44.2% vs. 20.3%; p = 0.005). The duration of GD was longer in patients without cancer than the duration in patients with cancer (6.9 ± 7.1 vs. 3.9 ± 4.0 years; p = 0.003). Gland weights were significantly heavier in patients without cancer compared with patients with cancer (134.7 ± 88.9 vs. 52.9 ± 56.6 g; p < 0.001). Preoperative TR-Ab was significantly higher in patients without cancer compared with TR-Ab levels in patients with cancer (34.9 ± 40.1 vs. 10.9 ± 17.2 IU/L; p < 0.001).
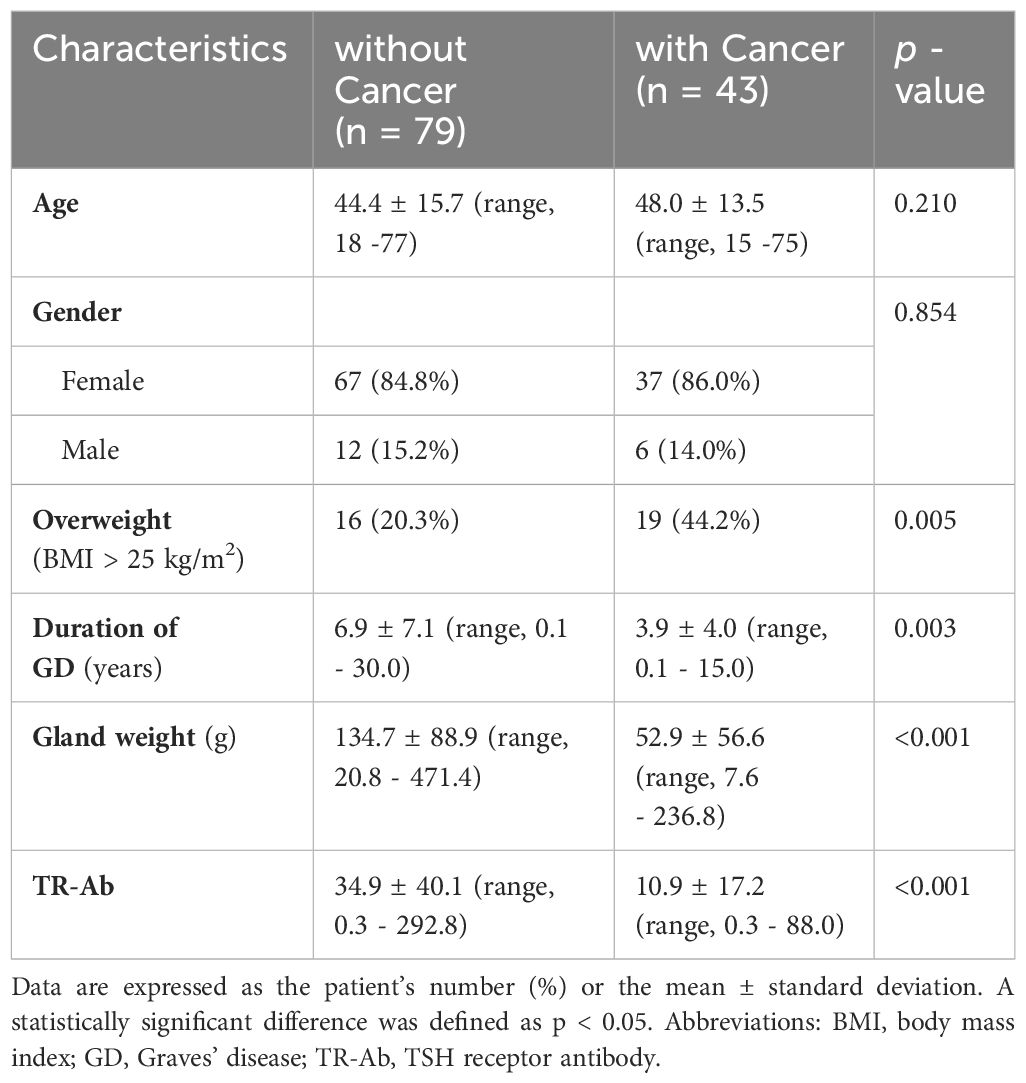
Table 4 Comparison between sub-groups of Graves’ disease with or without thyroid cancer.
3.4 Univariate and multivariate analyses of biomarkers for malignancy in patients with Graves’ disease
Univariate analysis revealed that being overweight, the duration of GD, gland weight, and preoperative TR-Ab were significant biomarkers for malignancy in patients with GD ( Table 5 ). In the multivariate analysis, being overweight, lighter gland weight, and lower postoperative TR-Ab levels were confirmed as biomarkers for malignancy. Being overweight emerged as the most significant biomarker for malignancy (OR, 3.108; 95% CI, 1.196–8.831; p = 0.021).
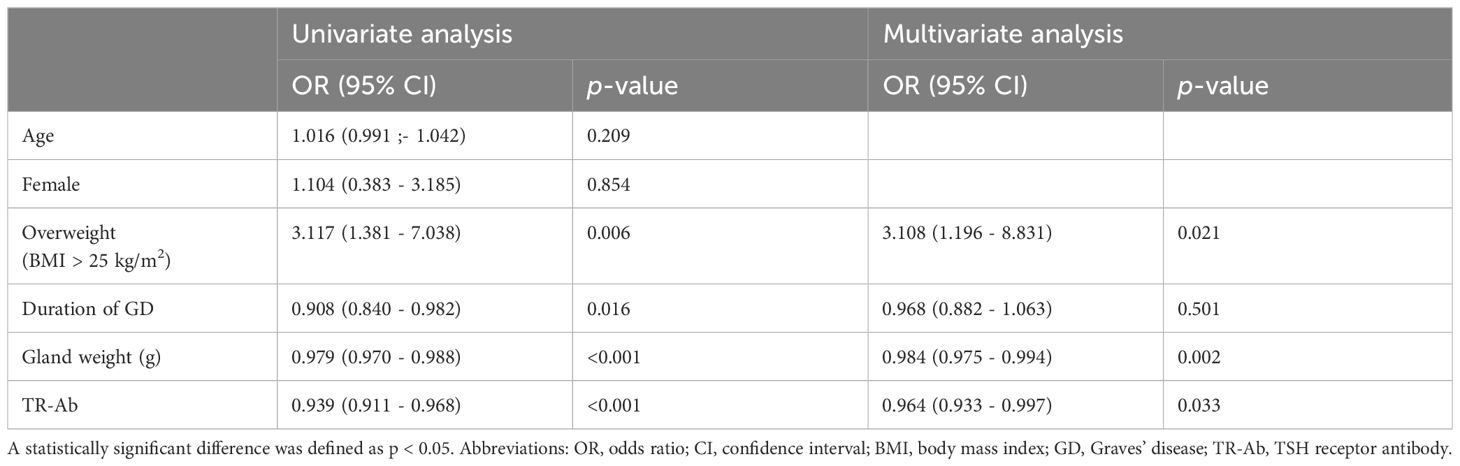
Table 5 Univariate and multivariate analyses of biomarkers for malignancy in patients with Graves’ disease.
4 Discussion
The present study aimed to investigate the biomarkers for concomitant thyroid cancer in patients with GD and identify the effects of being overweight on cancer risk. Patients with GD and concomitant thyroid cancer were more likely to be overweight compared to patients with GD without cancer. In addition, overweight patients had a significantly increased risk of developing thyroid cancer compared to non-overweight patients.
In GD, TR-Ab stimulates the TSH receptor, leading to increased production and release of thyroid hormones. Excessive thyroid hormone affects entire body tissues, including thermogenesis and metabolic rate. GD symptoms vary by hyperthyroidism severity and duration ( 1 , 2 , 31 ).
The reported incidence of concomitant thyroid cancer in GD ranges from 1% to 22% ( 8 – 11 , 38 , 39 ). Since this study included GD patients who meet the surgical indications, the cohort demonstrated a higher prevalence of thyroid cancer compared to the general GD population. The frequency of cancer in patients with GD is consistent with the frequency in the general population. All types of thyroid cancer can occur in GD patients; PTC is the most common cancer followed by FTC ( 8 , 40 ). While surgery is not the primary treatment for GD, surgical intervention may be performed in cases that meet specific surgical indications ( 1 , 2 ). According to the 2016 ATA guidelines for hyperthyroidism, near-total or total thyroidectomy is recommended for surgical intervention of GD ( 1 ). However, 11 patients underwent lobectomy in our study; these patients maintained a euthyroid state with preoperatively detected nodules, and the decision to perform lobectomy was made based on the individual preferences of the patients and the multidisciplinary medical team. GD did not recur in any of the 11 patients who underwent lobectomies.
Numerous studies have demonstrated that thyroid cancer is more aggressive in obese and overweight patients, irrespective of the coexistence of GD ( 26 – 28 , 41 , 42 ). In a case-control study, Marcello et al. showed that being overweight (BMI ≥ 25 kg/m2) is associated with an increased risk of thyroid cancer (OR, 3.787; 95% CI, 1.110–6.814, p < 0.001) ( 27 ). GD is a hypermetabolic disease, which usually causes weight loss, and obesity is not common in patients with GD ( 31 ). Weight gain is a useful indicator for evaluating initial treatment success for hyperthyroidism, but weight loss should be considered differently in obese patients. Hoogwerf et al. reported that despite greater weight loss at the time of the initial diagnosis of GD, obese patients were still morbidly obese and had higher thyroid function values compared to non-obese patients ( 43 ). The diagnosis of hyperthyroidism may be delayed in these patients as weight loss is often perceived as a positive outcome. The results of our study agree with earlier studies and are supported by an OR of 3.108, which is similar to the OR of 3.787 reported in the Marcello study ( 27 ).
The mean tumor size in this study was 0.9 cm, which was similar to previous studies concerning thyroid cancer in patients with GD. In a study by Hales et al., the average size of thyroid cancer in patients with GD was 0.91 cm, which was significantly smaller than the average size in the euthyroid group (0.91 vs. 2.33 cm) ( 44 ). However, previous studies demonstrated a more aggressive thyroid cancer phenotype in patients with GD ( 9 , 45 ). In addition, Marongju et al. revealed a higher degree of aggressiveness in some patients with microcarcinoma and GD compared to controls, even when tumor characteristics were favorable, which conflicts with other studies ( 45 ). The presence of both thyroid cancer and GD is a surgical indication, regardless of the size of the cancer. Thus, microcarcinoma in GD should not be overlooked.
Lower preoperative TR-Ab were biomarkers for malignancy in patients with GD in this study. TR-Ab, which promotes hyperthyroidism by inducing the production and release of thyroid hormones, is a diagnostic biomarker for GD ( 13 , 20 ). Several studies have explored the link between TR-Ab and concurrent thyroid cancer in patients with GD and showed that TR-Ab can potentially trigger thyroid cancer by continuously stimulating thyroid cells ( 20 , 46 ). However, other studies did not detect an association between TR-Ab and concomitant thyroid cancer in patients with GD, which is consistent with our findings ( 16 , 40 , 47 ). Yano et al. demonstrated that elevated TR-Ab was significantly associated with smaller tumor size in patients with GD and had no significant impact on multifocality or lymph node metastasis ( 40 ). Similarly, Kim et al. concluded that the behavior of thyroid cancer is not affected by TR-Ab ( 16 ). We attributed these results to the fact that patients with GD and cancer may undergo surgery due to the detection of nodules that were relatively well-controlled with medication for a long time. On the other hand, in the GD without cancer group, surgery is often performed due to uncontrolled hyperthyroidism despite medication, and TR-Ab levels may be higher. Future research should investigate the association between TR-Ab levels and thyroid cancer risk in larger studies to clarify the contradictory findings in previous studies.
The lighter gland weight was a biomarker for the concomitant thyroid cancer; however, measuring the gland weight before surgery is not feasible in clinical practice. Nonetheless, ultrasound can estimate thyroid volume preoperatively using the ellipsoidal formula: Volume = (π/6) × Length × Width × Depth. The overall thyroid volume can be derived by adding together the volume calculations for both lobes ( 48 ). Future studies will focus on applying this method clinically and investigating the link between preoperative thyroid dimensions and the prevalence of concomitant thyroid cancer.
This study’s strengths include long follow-up duration with more than 100 patients, providing robust results. Additionally, the study included various demographic and clinical factors, providing a comprehensive evaluation of thyroid cancer biomarkers in patients with GD. Of note, this study focused on the effect of being overweight in patients with GD, rather than the general population. However, the relationship between GD, thyroid cancer, and overweight is complex and may involve a variety of factors, including genetics, hormonal imbalances, and lifestyle factors.
This study has several limitations. First, its retrospective design and relatively small sample size may have introduced selection and information bias. Second, the study was conducted in the Korean population, limiting generalizability to other populations. Lastly, BRAF and TERT assessments were conducted in a limited cohort, insufficient to represent the entire study population, and there is a paucity of data on the molecular characteristics and genetic information for thyroid cancer. Further research should investigate the effects of being overweight on thyroid cancer risk in a diverse population of patients with GD to determine whether the results are generalizable. In addition, more investigations into the long-term postoperative outcomes of patients with GD with and without concomitant thyroid cancer may provide a more comprehensive evaluation of surgical outcomes.
5 Conclusions
Overweight individuals with GD have a higher risk of developing concomitant thyroid cancer. This highlights the importance of thorough screening and comprehensive evaluations specifically tailored to overweight GD patients to detect and prevent thyroid cancer. Further research is needed to elucidate the underlying mechanisms and the effects of being overweight on thyroid cancer risk in GD patients in the general population.
Data availability statement
The raw data supporting the conclusions of this article will be made available by the authors, without undue reservation.
Ethics statement
The studies involving humans were approved by Institutional Review Board of Seoul St. Mary’s Hospital, The Catholic University of Korea (IRB No: KC23RISI0054 and date of approval: 2023.04.21). The studies were conducted in accordance with the local legislation and institutional requirements. The ethics committee/institutional review board waived the requirement of written informed consent for participation from the participants or the participants’ legal guardians/next of kin because due to the retrospective nature of this study.
Author contributions
JP: Writing – review & editing, Writing – original draft, Visualization, Validation, Investigation, Formal analysis, Data curation, Conceptualization. SA: Writing – review & editing, Software, Data curation. JB: Writing – review & editing, Supervision, Software, Resources, Methodology. JK: Writing – review & editing, Supervision, Resources, Methodology. KK: Writing – review & editing, Writing – original draft, Visualization, Validation, Software, Resources, Project administration, Methodology, Investigation, Formal analysis, Data curation, Conceptualization.
The author(s) declare that no financial support was received for the research, authorship, and/or publication of this article.
Conflict of interest
The authors declare that the research was conducted in the absence of any commercial or financial relationships that could be construed as a potential conflict of interest.
Publisher’s note
All claims expressed in this article are solely those of the authors and do not necessarily represent those of their affiliated organizations, or those of the publisher, the editors and the reviewers. Any product that may be evaluated in this article, or claim that may be made by its manufacturer, is not guaranteed or endorsed by the publisher.
Supplementary material
The Supplementary Material for this article can be found online at: https://www.frontiersin.org/articles/10.3389/fendo.2024.1382124/full#supplementary-material
1. RossDouglas S, BurchHenry B, CooperDavid S, Carol G, Luiza M, RivkeesScott A, et al. 2016 American Thyroid Association guidelines for diagnosis and management of hyperthyroidism and other causes of thyrotoxicosis. Thyroid . (2016). doi: 10.1089/thy.2016.0229
CrossRef Full Text | Google Scholar
2. Smith TJ, Hegedüs L. Graves’ disease. New Engl J Med . (2016) 375:1552–65. doi: 10.1056/NEJMra1510030
PubMed Abstract | CrossRef Full Text | Google Scholar
3. Pearce EN, Farwell AP, Braverman LE. Thyroiditis. New Engl J Med . (2003) 348:2646–55. doi: 10.1056/NEJMra021194
4. Davies TF, Andersen S, Latif R, Nagayama Y, Barbesino G, Brito M, et al. Graves’ disease. Nat Rev Dis primers . (2020) 6:52. doi: 10.1038/s41572-020-0184-y
5. Bahn RS. Graves' ophthalmopathy. New Engl J Med . (2010) 362:726–38. doi: 10.1056/NEJMra0905750
6. Burch HB, Cooper DS. Management of Graves disease: a review. Jama . (2015) 314:2544–54. doi: 10.1001/jama.2015.16535
7. Ginsberg J. Diagnosis and management of Graves' disease. Cmaj . (2003) 168:575–85.
PubMed Abstract | Google Scholar
8. Wahl RA, Goretzki P, Meybier H, Nitschke J, Linder M, Röher H-D. Coexistence of hyperthyroidism and thyroid cancer. World J Surgery . (1982) 6:385–9. doi: 10.1007/BF01657662
9. Belfiore A, Garofalo MR, Giuffrida D, Runello F, Filetti S, Fiumara A, et al. Increased aggressiveness of thyroid cancer in patients with Graves' disease. J Clin Endocrinol Metab . (1990) 70:830–5. doi: 10.1210/jcem-70-4-830
10. Kraimps J, Bouin-Pineau M, Mathonnet M, De Calan L, Ronceray J, Visset J, et al. Multicentre study of thyroid nodules in patients with Graves' disease. J Br Surgery . (2000) 87:1111–3. doi: 10.1046/j.1365-2168.2000.01504.x
11. Pacini F, Elisei R, Di Coscio G, Anelli S, Macchia E, Concetti R, et al. Thyroid carcinoma in thyrotoxic patients treated by surgery. J endocrinological Invest . (1988) 11:107–12. doi: 10.1007/BF03350115
12. Durante C, Grani G, Lamartina L, Filetti S, Mandel SJ, Cooper DS. The diagnosis and management of thyroid nodules: a review. Jama . (2018) 319:914–24. doi: 10.1001/jama.2018.0898
13. Belfiore A, Russo D, Vigneri R, Filetti S. Graves' disease, thyroid nodules and thyroid cancer. Clin endocrinology . (2001) 55:711–8. doi: 10.1046/j.1365-2265.2001.01415.x
14. Arslan H, Unal O, Algün E, Harman M, Sakarya ME. Power Doppler sonography in the diagnosis of Graves’ disease. Eur J Ultrasound . (2000) 11:117–22. doi: 10.1016/S0929-8266(99)00079-8
15. Vitti P, Rago T, Mazzeo S, Brogioni S, Lampis M, De Liperi A, et al. Thyroid blood flow evaluation by color-flow Doppler sonography distinguishes Graves’ disease from Hashimoto’s thyroiditis. J endocrinological Invest . (1995) 18:857–61. doi: 10.1007/BF03349833
16. Kim WB, Han SM, Kim TY, Nam-Goong IS, Gong G, Lee HK, et al. Ultrasonographic screening for detection of thyroid cancer in patients with Graves’ disease. Clin endocrinology . (2004) 60:719–25. doi: 10.1111/j.1365-2265.2004.02043.x
17. Phitayakorn R, McHenry CR. Incidental thyroid carcinoma in patients with Graves’ disease. Am J surgery . (2008) 195:292–7. doi: 10.1016/j.amjsurg.2007.12.006
18. Dănilă R, Karakas E, Osei-Agyemang T, Hassan I. Outcome of incidental thyroid carcinoma in patients undergoing surgery for Graves' disease. Rev Medico-chirurgicala Societatii Medici si Naturalisti din Iasi . (2008) 112:115–8.
Google Scholar
19. Jia Q, Li X, Liu Y, Li L, Kwong JS, Ren K, et al. Incidental thyroid carcinoma in surgery-treated hyperthyroid patients with Graves’ disease: a systematic review and meta-analysis of cohort studies. Cancer Manage Res . (2018) 10:1201–7. doi: 10.2147/CMAR
20. Filetti S, Belfiore A, Amir SM, Daniels GH, Ippolito O, Vigneri R, et al. The role of thyroid-stimulating antibodies of Graves' disease in differentiated thyroid cancer. New Engl J Med . (1988) 318:753–9. doi: 10.1056/NEJM198803243181206
21. Potter E, Horn R, Scheumann G, Dralle H, Costagliola S, Ludgate M, et al. Western blot analysis of thyrotropin receptor expression in human thyroid tumors and correlation with TSH binding. Biochem Biophys Res Commun . (1994) 205:361–7. doi: 10.1006/bbrc.1994.2673
22. Papanastasiou A, Sapalidis K, Goulis DG, Michalopoulos N, Mareti E, Mantalovas S, et al. Thyroid nodules as a risk factor for thyroid cancer in patients with Graves’ disease: A systematic review and meta-analysis of observational studies in surgically treated patients. Clin Endocrinology . (2019) 91:571–7. doi: 10.1111/cen.14069
23. Behar R, Arganini M, Wu T-C, McCormick M, Straus F 2nd, DeGroot L, et al. Graves' disease and thyroid cancer. Surgery . (1986) 100:1121–7.
24. Ren M, Wu MC, Shang CZ, Wang XY, Zhang JL, Cheng H, et al. Predictive factors of thyroid cancer in patients with Graves’ disease. World J surgery . (2014) 38:80–7. doi: 10.1007/s00268-013-2287-z
25. Franchini F, Palatucci G, Colao A, Ungaro P, Macchia PE, Nettore IC. Obesity and thyroid cancer risk: an update. Int J Environ Res Public Health . (2022) 19:1116. doi: 10.3390/ijerph19031116
26. Kaliszewski K, Diakowska D, Rzeszutko M, Rudnicki J. Obesity and overweight are associated with minimal extrathyroidal extension, multifocality and bilaterality of papillary thyroid cancer. J Clin Med . (2021) 10:970. doi: 10.3390/jcm10050970
27. Marcello MA, Sampaio AC, Geloneze B, Vasques ACJ, Assumpção LVM, Ward LS. Obesity and excess protein and carbohydrate consumption are risk factors for thyroid cancer. Nutr cancer . (2012) 64:1190–5. doi: 10.1080/01635581.2012.721154
28. Matrone A, Ferrari F, Santini F, Elisei R. Obesity as a risk factor for thyroid cancer. Curr Opin Endocrinology Diabetes Obes . (2020) 27:358–63. doi: 10.1097/MED.0000000000000556
29. Xu L, Port M, Landi S, Gemignani F, Cipollini M, Elisei R, et al. Obesity and the risk of papillary thyroid cancer: a pooled analysis of three case–control studies. Thyroid . (2014) 24:966–74. doi: 10.1089/thy.2013.0566
30. Sun H, Tong H, Shen X, Gao H, Kuang J, Chen X, et al. Outcomes of surgical treatment for graves’ Disease: A single-center experience of 216 cases. J Clin Med . (2023) 12:1308. doi: 10.3390/jcm12041308
31. Brent GA. Graves' disease. New Engl J Med . (2008) 358:2594–605. doi: 10.1056/NEJMcp0801880
32. James PT. Obesity: the worldwide epidemic. Clinics Dermatol . (2004) 22:276–80. doi: 10.1016/j.clindermatol.2004.01.010
33. Organization WH. Follow-up to the political declaration of the high-level meeting of the general assembly on the prevention and control of non-communicable diseases. Sixty-sixth World Health Assembly Agenda item . (2013) 13:43–4.
34. Deitel M. Overweight and obesity worldwide now estimated to involve 1.7 billion people. Obes surgery . (2003) 13:329–30. doi: 10.1381/096089203765887598
35. Consultation WE. Appropriate body-mass index for Asian populations and its implications for policy and intervention strategies. Lancet Lond Engl . (2004) 363:157–63. doi: 10.1016/S0140-6736(03)15268-3
36. Fan J-G, Kim S-U, Wong VW-S. New trends on obesity and NAFLD in Asia. J hepatology . (2017) 67:862–73. doi: 10.1016/j.jhep.2017.06.003
37. Haugen BR, Alexander EK, Bible KC, Doherty GM, Mandel SJ, Nikiforov YE, et al. 2015 American Thyroid Association management guidelines for adult patients with thyroid nodules and differentiated thyroid cancer: the American Thyroid Association guidelines task force on thyroid nodules and differentiated thyroid cancer. Thyroid . (2016) 26:1–133. doi: 10.1089/thy.2015.0020
38. Erbil Y, Barbaros U, Özbey N, Kapran Y, Tükenmez M, Bozbora A, et al. Graves' disease, with and without nodules, and the risk of thyroid carcinoma. J Laryngology Otology . (2008) 122:291–5. doi: 10.1017/S0022215107000448
39. Cantalamessa L, Baldini M, Orsatti A, Meroni L, Amodei V, Castagnone D. Thyroid nodules in Graves disease and the risk of thyroid carcinoma. Arch Internal Med . (1999) 159:1705–8. doi: 10.1001/archinte.159.15.1705
40. Yano Y, Shibuya H, Kitagawa W, Nagahama M, Sugino K, Ito K, et al. Recent outcome of Graves’ disease patients with papillary thyroid cancer. Eur J endocrinology . (2007) 157:325–9. doi: 10.1530/EJE-07-0136
41. Pappa T, Alevizaki M. Obesity and thyroid cancer: a clinical update. Thyroid . (2014) 24:190–9. doi: 10.1089/thy.2013.0232
42. Kitahara CM, Platz EA, Freeman LEB, Hsing AW, Linet MS, Park Y, et al. Obesity and thyroid cancer risk among US men and women: a pooled analysis of five prospective studies. Cancer epidemiology Biomarkers Prev . (2011) 20:464–72. doi: 10.1158/1055-9965.EPI-10-1220
43. Hoogwerf BJ, Nuttall FQ. Long-term weight regulation in treated hyperthyroid and hypothyroid subjects. Am J Med . (1984) 76:963–70. doi: 10.1016/0002-9343(84)90842-8
44. Hales I, McElduff A, Crummer P, Clifton-Bligh P, Delbridge L, Hoschl R, et al. Does Graves' disease or thyrotoxicosis affect the prognosis of thyroid cancer. J Clin Endocrinol Metab . (1992) 75:886–9. doi: 10.1210/jcem.75.3.1517381
45. Marongiu A, Nuvoli S, De Vito A, Rondini M, Spanu A, Madeddu G. A comparative follow-up study of patients with papillary thyroid carcinoma associated or not with graves’ Disease. Diagnostics . (2022) 12:2801. doi: 10.3390/diagnostics12112801
46. Katz S, Garcia A, Niepomniszcze H. Development of Graves' disease nine years after total thyroidectomy due to follicular carcinoma of the thyroid. Thyroid . (1997) 7:909–11. doi: 10.1089/thy.1997.7.909
47. Tanaka K, Inoue H, Miki H, Masuda E, Kitaichi M, Komaki K, et al. Relationship between prognostic score and thyrotropin receptor (TSH-R) in papillary thyroid carcinoma: immunohistochemical detection of TSH-R. Br J cancer . (1997) 76:594–9. doi: 10.1038/bjc.1997.431
48. Viduetsky A, Herrejon CL. Sonographic evaluation of thyroid size: a review of important measurement parameters. J Diagn Med Sonography . (2019) 35:206–10. doi: 10.1177/8756479318824290
Keywords: Graves’ disease, thyroid cancer, overweight, thyroid stimulating hormone receptor antibodies, BMI - body mass index
Citation: Park J, An S, Bae JS, Kim JS and Kim K (2024) Overweight as a biomarker for concomitant thyroid cancer in patients with Graves’ disease. Front. Endocrinol. 15:1382124. doi: 10.3389/fendo.2024.1382124
Received: 05 February 2024; Accepted: 03 April 2024; Published: 22 April 2024.
Reviewed by:
Copyright © 2024 Park, An, Bae, Kim and Kim. This is an open-access article distributed under the terms of the Creative Commons Attribution License (CC BY) . The use, distribution or reproduction in other forums is permitted, provided the original author(s) and the copyright owner(s) are credited and that the original publication in this journal is cited, in accordance with accepted academic practice. No use, distribution or reproduction is permitted which does not comply with these terms.
*Correspondence: Kwangsoon Kim, [email protected]
Disclaimer: All claims expressed in this article are solely those of the authors and do not necessarily represent those of their affiliated organizations, or those of the publisher, the editors and the reviewers. Any product that may be evaluated in this article or claim that may be made by its manufacturer is not guaranteed or endorsed by the publisher.
Knowledge, Attitude and Practice Related to Chemotherapy Among Cancer Patients
Affiliation.
- 1 Akhtar Saeed Medical and Dental College, Lahore, Pakistan.
- PMID: 38616652
- PMCID: PMC11017809
- DOI: 10.1177/00469580241246460
Cancer is a leading cause of death, with a rapidly increasing global burden. Chemotherapy is the most effective cancer treatment, and with its benefits, there exist potential problems. The present study assesses cancer patients' knowledge, attitude, and practice toward chemotherapy use. A descriptive cross-sectional study was conducted in the oncology wards of various tertiary care hospitals and cancer care centers in Lahore, Pakistan. Patients were included in the study based on convenient sampling. A structured questionnaire with 25 (close-ended) questions and a demographic profile was used to collect data. Descriptive statistics was used to analyze frequencies and percentages. Independent sample t -test and ANOVA were used to calculate the mean and standard deviation. Most patients were females (54%) and married (52.6%), with an unemployment rate of 39%. Patients with higher education depicted significantly higher scores in knowledge (9.61 ± 2.65), attitude (19.37 ± 2.70), and practice (3.89 ± 1.03) domains. Surprisingly, throughout the whole KAP domain, the patient's attitude (18.42 ± 3.31) toward chemotherapy use, showed higher values, as compared to their knowledge (7.78 ± 3.26) and practice (3.66 ± 1.08) scores. The majority of the study participants had a positive attitude toward chemotherapy use, with limited knowledge, and practice.
Keywords: attitude; cancer; chemotherapy; knowledge; practice.
- Cross-Sectional Studies
- Health Knowledge, Attitudes, Practice*
- Neoplasms* / drug therapy
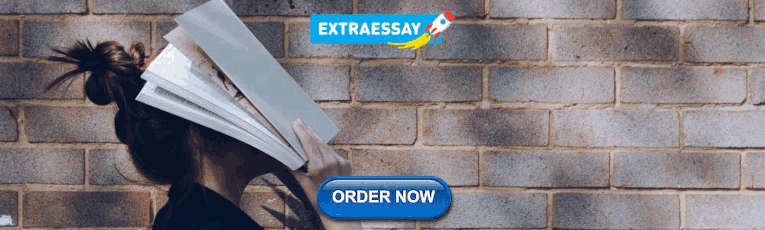
COMMENTS
In one study, 69.8% of the patients had a partial or complete response after receiving an ... Mehta V, Goel S, Kabarriti R, et al. Case fatality rate of cancer patients with COVID-19 in a New York ...
Cancer is a risk factor for venous thromboembolism, and this patient presents with chest pain, dyspnea, and unexplained tachycardia. In the context of cancer, an elevated d -dimer level can ...
Presentation of Case. Dr. Christopher T. Chen: A 34-year-old woman was evaluated in the oncology clinic of this hospital for the management of relapsed, metastatic intrahepatic cholangiocarcinoma ...
Methods. A case study approach was used because it allowed us to examine the complexity of cancer management from the perspective of one person's case as interpreted by multiple people, retaining its holistic and meaningful characteristics while being studied 17 answering how and why questions. 18 Interviews from four participants presented multiple perspectives of the same interested topic ...
This patient has a stomach cancer that appears to be locoregionally advanced on imaging studies. The first decision regarding management is whether to resect it immediately or to administer preoperative chemotherapy, with or without preoperative radiation therapy. Treatment of Locoregionally Advanced Gastric Cancer
Breast cancer is the most common cancer and the one that associates the highest mortality rates among Spanish women, with 32,953 new cases estimated to be diagnosed in Spain in 2020 [ 1 ]. Thanks to early diagnosis and therapeutic advances, survival has increased in recent years [ 2 ]. The 5-year survival rate is currently around 85% [ 3, 4 ].
example, in a series of 8422 patients enrolled on International Breast Cancer Study Group trials between 1978 and 1999, the rate of node-negativity for medial compared to lateral/central tumors was 44 versus 33 percent, respectively. The most likely explanation for this difference is preferential drainage of some medial tumors to the IM nodes.
Introduction. Cervical cancer is one of the major causes of cancer-related death in women worldwide [].It is the fourth most prevalent cause of malignancy in women after breast, colorectal, and lung cancer [].]. The incidence of cervical cancer and mortality rate has declined by 70% in the United States since the 1950s because of age-appropriate screening [].
Patient factors and cancer characteristics are key to deciding between focal therapies and whole gland treatment. October 30, 2023 / Cancer / Surgical Oncology. ... A Case Study. Two-day procedure prevents neurologic compromise for octogenarian. March 10, 2022 / Cancer / Surgical Oncology.
s to identify and synthesize the evidence of randomized controlled trial studies to prove that case management could be one way to address the quality of life of cancer patients. Methods: We performed a literature search in 4 electronic bibliographic databases and snowball searches were performed to ensure a complete collection. Two review authors independently extracted and analyzed data. A ...
Case study. Patient T was a 48-year-old male who presented on 9 February 2021 with a non-benign lesion in his throat and progressive shortness of breath. He had a two-month history of loss of weight (approximately 20 kg), dysphagia (grade III-IV) with poor oral intake during this period, coughing and voice changes.
According to another retrospective study, 20 of 137 patients with EGFR-mutant lung adenocarcinoma survived for ≥5 years, and exon 19 deletion, absence of extrathoracic metastases, absence of brain metastasis, and current non-smoking status were reportedly good prognostic factors. Our case corroborated the good prognostic factors reported in ...
Case management (CM) is widely utilized to improve health outcomes of cancer patients, enhance their experience of health care, and reduce the cost of care. While numbers of systematic reviews are available on the effectiveness of CM for cancer patients, they often arrive at discordant conclusions that may confuse or mislead the future case management development for cancer patients and ...
Drew Bouton, from Olympia, Wash., is one of those patients. Drew lives with metastatic prostate cancer and receives treatment at the Fred Hutchinson Cancer Center in Seattle, Wash. Diagnosed at the age of 45, he was reluctantly told by his doctor that though survival is a bell curve, he likely had just two years to live. That was 22 years ago.
Lessons for the future. "This case is unusual, not only because of the multimodal approach but also the age of the patient," explains Dr. Kamath. "It underscores the unfortunate rise of young-onset colorectal cancer. Thirty years ago, the median age at onset of colorectal cancer was 72 years. It has fallen to 66 years, and we are seeing ...
And this person certainly did. This patient had 2 years from completion of her platinum-based chemotherapy before she had evidence of recurrence. These bigger platinum-free intervals are also associated with better likelihood to respond to a platinum rechallenge. Case Overview: A 57-Year-Old Woman With Ovarian Cancer. Initial Presentation
During a Case-Based Roundtable® event, Madappa Kundranda, MD, PhD, discussed recent retrospective studies that compared outcomes between the available treatment options in patients with relapsed/refractory advanced colorectal cancer in the first article of a 2-part series.
Case 2: FLAURA Study for EGFR+ Metastatic NSCLC. EP: 11 ... It's my pleasure to walk us through the first case, which is small cell lung cancer. This is a case with a 72-year-old woman who presents with shortness of breath, a productive cough, chest pain, some fatigue, anorexia, a recent 18-pound weight loss, and a history of hypertension ...
Dr. Mathew S. Lopes: A 65-year-old woman was transferred to this hospital because of chest pain. Six months before the current presentation, the patient presented to a hospital affiliated with ...
Patient Case Presentation. Patient Mrs. B.C. is a 56 year old female who is presenting to her WHNP for her annual exam. She had to cancel her appointment two months ago and didn't reschedule until now. Her last pap smear and mammogram were normal. Today, while performing her breast exam, her nurse practitioner notices dimpling in the left ...
Application error: a client-side exception has occurred (see the browser console for more information). Home to the journal Oncology, Cancer Network provides research and opinion on the screening, early detection, diagnosis, treatment, and prevention of cancers.
Case study 4 - Dr Rajesh Jena. Personalising radiotherapy - how patient data is helping reduce the side effects of treatment. Radiotherapy is the gold standard of treatment for many types of cancers. In fact, more than 130,000 patients benefit from radiotherapy every year in the UK.
Data suggest there were 19.96 million new cancer cases and 9.74 million deaths from cancer in 2022. Researchers are predicting that global cancer cases will continue to rise, exceeding 35 million ...
The key breakthrough, says Robert Winn, a lung cancer specialist at Virginia Commonwealth University, is the ability to better pinpoint the mutations of a patient's particular form of cancer.
5 Methods Used By the Ambulatory Safety Nets to Ensure Success . CRICO and members of the Harvard medical community have developed and deployed a collaborative Ambulatory Safety Net (ASN) to identify patients who previously fell through the cracks related to follow-up cancer screening.
News; Health; New study offers hope for a rare and devastating eye cancer Thu., April 25, 2024 Udai Kammula of University of Pittsburgh Medical Center stands with a patient who was treated with ...
In cancer research there is much interest in building and validating outcome prediction models to support treatment decisions. However, because most outcome prediction models are developed and validated without regard to the causal aspects of treatment decision making, many published outcome prediction models may cause harm when used for decision making, despite being found accurate in ...
At present, there have been many studies evaluating the prognostic risk factors of patients with breast cancer in Xinjiang (11-14); for instance, Shan et al. investigated the clinicopathological features and prognostic characteristics of patients with triple-negative breast cancer in Xinjiang, based on clinical information for 319 patients.
The mean tumor size in this study was 0.9 cm, which was similar to previous studies concerning thyroid cancer in patients with GD. In a study by Hales et al., the average size of thyroid cancer in patients with GD was 0.91 cm, which was significantly smaller than the average size in the euthyroid group (0.91 vs. 2.33 cm) .
Cancer is a leading cause of death, with a rapidly increasing global burden. Chemotherapy is the most effective cancer treatment, and with its benefits, there exist potential problems. The present study assesses cancer patients' knowledge, attitude, and practice toward chemotherapy use. A descriptiv …