Cite this chapter
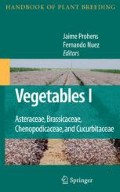
- Beiquan Mou 4
Part of the book series: Handbook of Plant Breeding ((HBPB,volume 1))
5167 Accesses
38 Citations
1 Altmetric
Lettuce ( Lactuca sativa L.) is a major fresh vegetable and its leaves are commonly found in salad mixtures and sandwiches. In some eastern countries like China and Egypt, stems instead of leaves of lettuce are consumed, either cooked, raw, pickled, dried, or as a sauce. Some less common uses for lettuce include a cigarette without nicotine made from lettuce leaves, edible oil extracted from seeds of a primitive lettuce, and a sedative made of dried latex contained in stems and other tissues. Lactucarium, the dried latex produced from a wild lettuce relative, Lactuca virosa L., is used to make a sleep-inducing medicine (Ryder, 1986).
This is a preview of subscription content, log in via an institution to check access.
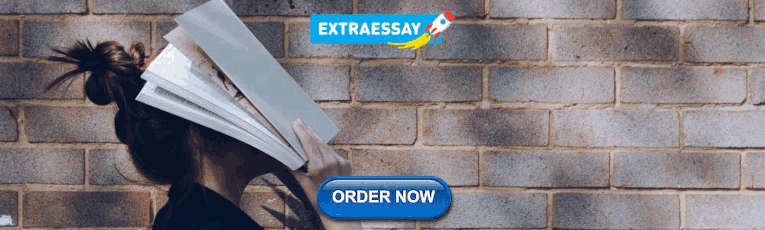
Access this chapter
- Available as PDF
- Read on any device
- Instant download
- Own it forever
- Compact, lightweight edition
- Dispatched in 3 to 5 business days
- Free shipping worldwide - see info
- Durable hardcover edition
Tax calculation will be finalised at checkout
Purchases are for personal use only
Institutional subscriptions
Unable to display preview. Download preview PDF.
Author information
Authors and affiliations.
United States Department of Agriculture, Agricultural Research Service, 1636 E.Alisal St., Salinas, 93905, CA, USA
Beiquan Mou
You can also search for this author in PubMed Google Scholar
Editor information
Editors and affiliations.
COMAV-UPV, Universidad Politécnica de Valencia, 14 Camino de Vera, Valencia, 46022, Spain
Jaime Prohens & Fernando Nuez &
Rights and permissions
Reprints and permissions
Copyright information
© 2008 Springer Science+Business Media, LLC
About this chapter
Mou, B. (2008). Lettuce. In: Prohens, J., Nuez, F. (eds) Vegetables I. Handbook of Plant Breeding, vol 1. Springer, New York, NY. https://doi.org/10.1007/978-0-387-30443-4_3
Download citation
DOI : https://doi.org/10.1007/978-0-387-30443-4_3
Publisher Name : Springer, New York, NY
Print ISBN : 978-0-387-72291-7
Online ISBN : 978-0-387-30443-4
eBook Packages : Biomedical and Life Sciences Biomedical and Life Sciences (R0)
Share this chapter
Anyone you share the following link with will be able to read this content:
Sorry, a shareable link is not currently available for this article.
Provided by the Springer Nature SharedIt content-sharing initiative
- Publish with us
Policies and ethics
- Find a journal
- Track your research
Thank you for visiting nature.com. You are using a browser version with limited support for CSS. To obtain the best experience, we recommend you use a more up to date browser (or turn off compatibility mode in Internet Explorer). In the meantime, to ensure continued support, we are displaying the site without styles and JavaScript.
- View all journals
- My Account Login
- Explore content
- About the journal
- Publish with us
- Open access
- Published: 01 August 2020
Growth monitoring of greenhouse lettuce based on a convolutional neural network
- Lingxian Zhang 1 , 2 ,
- Zanyu Xu 1 ,
- Juncheng Ma 3 ,
- Yingyi Chen 1 &
- Zetian Fu 1
Horticulture Research volume 7 , Article number: 124 ( 2020 ) Cite this article
10k Accesses
44 Citations
Metrics details
- Developmental biology
- Plant breeding
Growth-related traits, such as aboveground biomass and leaf area, are critical indicators to characterize the growth of greenhouse lettuce. Currently, nondestructive methods for estimating growth-related traits are subject to limitations in that the methods are susceptible to noise and heavily rely on manually designed features. In this study, a method for monitoring the growth of greenhouse lettuce was proposed by using digital images and a convolutional neural network (CNN). Taking lettuce images as the input, a CNN model was trained to learn the relationship between images and the corresponding growth-related traits, i.e., leaf fresh weight (LFW), leaf dry weight (LDW), and leaf area (LA). To compare the results of the CNN model, widely adopted methods were also used. The results showed that the values estimated by CNN had good agreement with the actual measurements, with R 2 values of 0.8938, 0.8910, and 0.9156 and normalized root mean square error (NRMSE) values of 26.00, 22.07, and 19.94%, outperforming the compared methods for all three growth-related traits. The obtained results showed that the CNN demonstrated superior estimation performance for the flat-type cultivars of Flandria and Tiberius compared with the curled-type cultivar of Locarno. Generalization tests were conducted by using images of Tiberius from another growing season. The results showed that the CNN was still capable of achieving accurate estimation of the growth-related traits, with R 2 values of 0.9277, 0.9126, and 0.9251 and NRMSE values of 22.96, 37.29, and 27.60%. The results indicated that a CNN with digital images is a robust tool for the monitoring of the growth of greenhouse lettuce.
Similar content being viewed by others
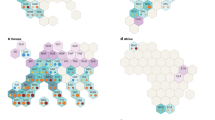
Climate change impacts and adaptations of wine production
Cornelis van Leeuwen, Giovanni Sgubin, … Gregory A. Gambetta
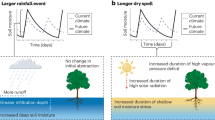
Plant responses to changing rainfall frequency and intensity
Andrew F. Feldman, Xue Feng, … Benjamin Poulter
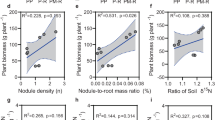
Legume rhizodeposition promotes nitrogen fixation by soil microbiota under crop diversification
Mengjie Qiao, Ruibo Sun, … Yan Chen
Introduction
Growth monitoring is essential for optimizing management and maximizing the production of greenhouse lettuce. Leaf fresh weight (LFW), leaf dry weight (LDW), and leaf area (LA) are critical indicators for characterizing growth 1 , 2 . Monitoring the growth of greenhouse lettuce by accurately obtaining growth-related traits (LFW, LDW, and LA) is of great practical significance for improving the yield and quality of lettuce 3 . The traditional methods for measuring growth-related traits, which are relatively straightforward, can achieve relatively accurate results 4 . However, the methods require destructive sampling, thus making it time-consuming and laborious 5 , 6 , 7 .
In recent years, nondestructive monitoring approaches have become a hot research topic. With the development of computer vision technology, image-based approaches have been widely applied to the nondestructive monitoring of crop growth 6 , 8 , 9 , 10 . Specifically, the image-based approaches extract low-level features from digital images and establish the relationship between the low-level features and manually measured growth-related traits, such as LA, LFW, and LDW. Based on this relationship, the image-derived features can estimate the growth-related traits, thus achieving nondestructive growth monitoring. For example, Chen et al. 6 proposed method for the estimation of barley biomass. The authors extracted structure properties, color-related features, near-infrared (NIR) signals, and fluorescence-based features from images. Based on the above features, they built multiple models, i.e., support vector regression (SVR), random forest (RF), multivariate linear regression (MLR), and multivariate adaptive regression splines, to estimate barley biomass. The results showed that the RF model was able to accurately estimate the biomass of barley and better quantify the relationship between image-based features and barley biomass than the other methods. Tackenberg et al. 11 proposed a method for estimating the growth-related traits of grass based on digital image analysis. Image features, such as the projected area (PA) and proportion of greenish pixels, were extracted, which were then fitted to the actual measured values of the aboveground fresh biomass, oven-dried biomass, and dry matter content by linear regression (LR). The results showed that all the determined coefficients of the constructed models were higher than 0.85, indicating that these features exhibited good linear relationship with growth-related traits. Casadesús and Villegas 5 used color-based image features to estimate the leaf area index (LAI), green area index (GAI), and crop dry weight biomass (CDW) of two genotypes of barley. The image features included the H component of the HSI color space, the a* component of the CIEL*a*b* color space, and the U components of the CIELUV color space. In addition, the green fraction and greener fraction were also extracted. The features were linearly fitted to the measured values of LAI, GAI, and CDW at different growth stages. The results showed that the image features based on color had strong correlations with growth-related traits. Fan et al. 12 developed a simple visible and NIR (near-infrared) camera system to capture time-series images of Italian ryegrass. Based on the digital number values of the R, G, and NIR channels of the raw images, MLR models for LAI estimation were built. The results showed that the image features derived from segmented images yielded better accuracy than those from non-segmented images, with an R 2 value of 0.79 for LAI estimation. Liu and Pattey 13 extracted the vertical gap fraction from digital images captured from nadir to estimate the LAI of corn, soybean, and wheat. Prior to the extraction of the canopy vertical gap fraction, the authors adopted the histogram-based threshold method to segment the green vegetative pixels. The results showed that the LAI estimated by the digital images before canopy closure was correlated with the field measurements. Sakamoto et al. 14 used vegetation indices derived from digital images, i.e., the visible atmospherically resistant index (VARI) and excess green (ExG), to estimate the biophysical characteristics of maize during the daytime. The results showed that the VARI could accurately estimate the green LAI, and the ExG was able to accurately estimate the total LAI.
Although computer vision-based methods for estimating growth-related traits have achieved promising results, they are subject to two issues. First, the methods are susceptible to noise. Since the images are captured under field conditions, noise caused by uneven illumination and cluttered backgrounds is inevitable, which will affect image segmentation and feature extraction, thus potentially reducing the accuracy 15 . Second, the methods greatly rely on manually designed image features, which have large computational complexity. Moreover, the generalization ability of the extracted low-level image features is poor 16 , 17 . Therefore, a more feasible and robust approach should be explored.
Convolutional neural networks (CNNs), which is a state-of-the-art deep learning approach, can directly take images as input to automatically learn complex feature representations 18 , 19 . With a sufficient amount of data, CNNs can achieve better precision than conventional methods 20 , 21 . Therefore, CNNs have been used in a wide range of agricultural applications, such as weed and crop recognition 19 , 22 , 23 , plant disease diagnosis 24 , 25 , 26 , 27 , 28 , and plant organ detection and counting 21 , 29 . However, despite its extensive use in classification tasks, CNNs have rarely been applied to regression applications, and there are few reports on how CNNs have been used for the estimation of growth-related traits of greenhouse lettuce. Inspired by Ma et al. 18 , who accurately estimated the aboveground biomass of winter wheat at early growth stages by using a deep CNN, which is a CNN with a deep network structure, this study intended to adopt a CNN to construct an estimation model for growth monitoring of greenhouse lettuce based on digital images and to compare the results with conventional methods that have been widely adopted to estimate growth-related traits.
The objective of this study is to achieve accurate estimations of growth-related traits for greenhouse lettuce. A CNN is used to model the relationship between an RGB image of greenhouse lettuce and the corresponding growth-related traits (LFW, LDW, and LA). By following the proposed framework, including lettuce image preprocessing, image augmentation, and CNN construction, this study will investigate the potential of using CNNs with digital images to estimate the growth-related traits of greenhouse lettuce throughout the entire growing season, thus exploring a feasible and robust approach for growth monitoring.
Material and methods
Greenhouse lettuce image collection and preprocessing.
The experiment was conducted at the experimental greenhouse of the Institute of Environment and Sustainable Development in Agriculture, Chinese Academy of Agricultural Sciences, Beijing, China (N39°57′, E116°19′). Three cultivars of greenhouse lettuce, i.e., Flandria, Tiberius, and Locarno, were grown under controlled climate conditions with 29/24 °C day/night temperatures and an average relative humidity of 58%. During the experiment, natural light was used for illumination, and a nutrient solution was circulated twice a day. The experiment was performed from April 22, 2019, to June 1, 2019. Six shelves were adopted in the experiment. Each shelf had a size of 3.48 × 0.6 m, and each lettuce cultivar occupied two shelves.
The number of plants for each lettuce cultivar was 96, which were sequentially labeled. Image collection was performed using a low-cost Kinect 2.0 depth sensor 30 . During the image collection, the sensor was mounted on a tripod at a distance of 78 cm to the ground and was oriented vertically downwards over the lettuce canopy to capture digital images and depth images. The original pixel resolutions of the digital images and depth images were 1920 × 1080 and 512 × 424, respectively. The digital images were stored in JPG format, while the depth images were stored in PNG format. The image collection was performed seven times 1 week after transplanting between 9:00 a.m. and 12:00 a.m. Finally, two image datasets were constructed, i.e., a digital image dataset containing 286 digital images and a depth image dataset containing 286 depth images. The number of digital images for Flandria, Tiberius, and Locarno was 96, 94 (two plants did not survive), and 96, respectively, and the number of depth images for the three cultivars was the same.
Since the original digital images of greenhouse lettuce contained an excess of background pixels, this study manually cropped images to eliminate the extra background pixels, after which images were uniformly adjusted to 900 × 900 pixel resolution. Figure 1 shows examples of the cropped digital images for the three cultivars. Prior to the construction of the CNN model, the original digital image dataset was divided into two datasets in a ratio of 8:2, i.e., a training dataset and a test dataset. The two datasets both covered all three cultivars and sampling intervals. The number of images for the training dataset was 229, where 20% of the images were randomly selected for the validation dataset. The test dataset contained 57 digital images. To enhance data diversity and prevent overfitting, a data augmentation method was used to enlarge the training dataset (Fig. 2 ). The augmentations were as follows: first, the images were rotated by 90°, 180°, and 270°, and then flipped horizontally and vertically. To adapt the CNN model to the changing illumination of the greenhouse, the images in the training dataset were converted to the HSV color space, and the brightness of the images was adjusted by changing the V channel 31 . The brightness of the images was adjusted to 0.8, 0.9, 1.1, and 1.2 times that of the original images to simulate the change in daylight. In total, the training dataset was enlarged by 26 times, resulting in 5954 digital images.
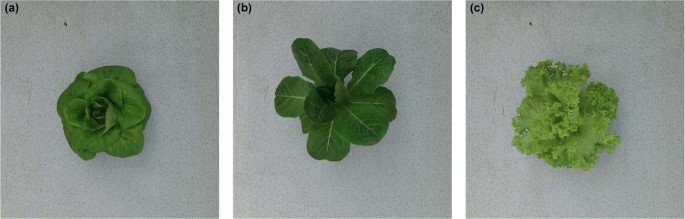
a , b , and c shows the cultivar of Flandria, Tiberius, and Locarno
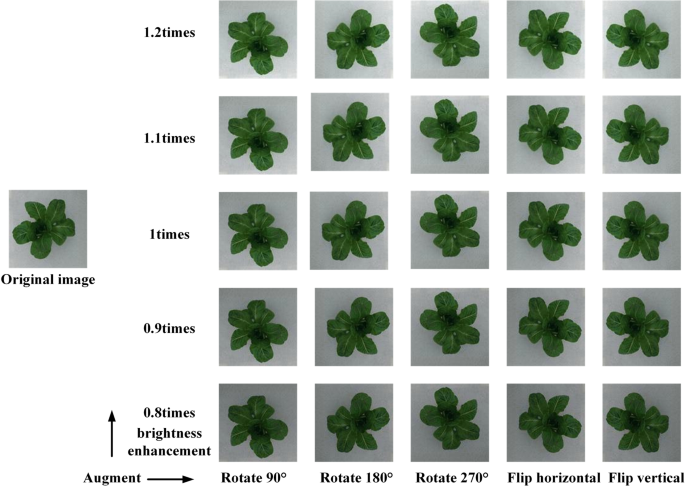
Image augmentation scheme
Measurement of greenhouse lettuce growth-related traits
Field measurements of LFW, LDW, and LA were performed simultaneously with image collection. These measurements were conducted at an interval of seven days, specifically on April 29, May 6, May 13, May 20, May 27, May 31, and June 1 of 2019. For the first six measurements, ten plants of greenhouse lettuce were randomly sampled each time for each cultivar. The measurements were obtained using a destructive sampling method. The sample was placed on a balance with a precision of 0.01 g after root removal, and the LFW was measured. The LA of the corresponding sample was obtained by a LA meter (LI-3100 AREA METER; LI-COR Inc. Lincoln, Nebraska, USA). Given the relatively large leaves of lettuce during the late growing season, the sample was sealed in an envelope and oven-dried at 80 °C for 72 h, after which the sample was weighed to obtain the LDW. For the last measurement, all the remaining lettuce plants were harvested, and the measurements were obtained by using the same method.
Construction of the CNN
The architecture of the CNN model is shown in Fig. 3 . The CNN model consisted of five convolutional layers, four pooling layers, and one fully connected layer. The input to the CNN model was digital images of greenhouse lettuce with a size of 128 × 128 × 3 (width × height (H) × channel). The convolutional layers adopted kernels with a size of 5 × 5 to extract features. The number of kernels in the five convolutional layers were 32, 64, 128, 216, and 512. To keep the size of the feature maps as an integer, zero-padding was employed in the second and third convolutional layers. The kernels in the pooling layers had a size of 2 × 2 and a stride of 2, which was able to reduce the size of feature maps by a factor of two. The average pooling function was adopted in the pooling layers instead of the max pooling function. The number of hidden neurons in the fully connected layer was three, corresponding to the three outputs of the model, i.e., the LFW, LDW, and LA. Therefore, the CNN model could estimate the three growth-related traits simultaneously. Dropout was used, and the rate was 0.5. In this study, the CNN model used stochastic gradient descent to optimize the network weights. The initial learning rate of the model was set to 0.001 and dropped every 20 epochs by a drop factor of 0.1. The mini-batch size was set to 128, and the maximum number of epochs for training was set to 300.
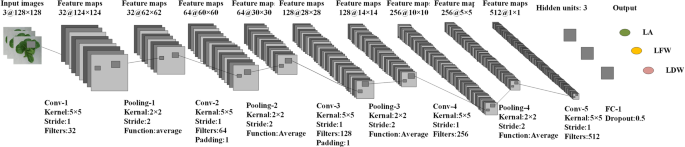
Architecture of the CNN model
Performance evaluation
To evaluate the performance of the CNN model, tests were performed with the widely adopted estimation methods. Two shallow machine learning classifiers, i.e., SVR 32 , 33 and RF 34 , were adopted to estimate the growth-related traits of greenhouse lettuce since these two methods have been reported to achieve good performance in crop growth monitoring. According to “Greenhouse lettuce image collection and preprocessing,” there was a large number of background pixels in the captured images of greenhouse lettuce. Therefore, it was necessary to conduct image segmentation to extract the lettuce pixels, thus ensuring that the extracted features in the following step were presenting the lettuce plants. For the digital images of the greenhouse lettuce, since the color contrast between the lettuce plant and the background was very obvious, image segmentation was achieved by using the adaptive threshold method for the color information. Some segmentation results are shown in Fig. 4 .
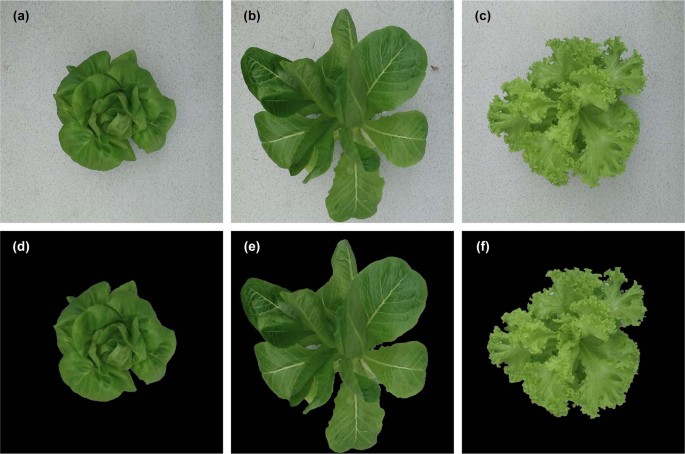
a – c shows the original images of greenhouse lettuce, and d – f shows the corresponding segmentation results
To build the shallow machine learning classifiers, feature extraction was performed on the segmented images of greenhouse lettuce. According to the characteristics of the three cultivars of greenhouse lettuce, low-level image features, including color, texture, and shape features, were extracted 35 . The color features included the average and standard deviation of 15 color components of five color spaces (RGB, HSV, CIEL*a*b, YCbCr, and HSI) 36 . Based on the color components, the gray level co-occurrence matrix 37 was combined to extract the texture features. The texture features included the contrast, correlation, energy, and homogeneity of the 15 color components. The shape features of the greenhouse lettuce that were extracted were area and perimeter in this study. The area was the area enclosed by the outline, and the perimeter was the total length of the blade outline. After extracting the image features, the Pearson coefficient was used to perform correlation analysis between the extracted features and the actual values of the LFW, LDW, and LA of greenhouse lettuce. The features with relatively high correlation values were used to build the shallow machine learning classifiers.
In addition to the above image features, structural features derived from the depth images, including H, PA, and digital volume (V), were also used to estimate the growth-related traits of the greenhouse lettuce 8 , 38 , 39 , 40 . Three LR models using H, PA, and V as the predictor variables (LR-H, LR-PA, and LR-V) were also used for comparison. Similar to the processing of digital images, image segmentation was also conducted on the depth images, which was achieved by the entropy rate superpixel segmentation method 41 . The lettuce plant could be extracted using the Euclidean distance to find the superpixel that was closest to the center of the image (Fig. 5 ). Once the lettuce plant was obtained, the structural features could be calculated (Fig. 6 ). Since the pixel value of the depth image was the actual distance from the sensor to the object, it reflected the depth information. Therefore, PA could be obtained by counting the number of pixels in the lettuce plant area. H could be obtained by averaging the H of the pixels in the lettuce plant area, which was obtained by using the H of the sensor minus the pixel values in the lettuce plant area. V could be obtained by multiplying PA by H.
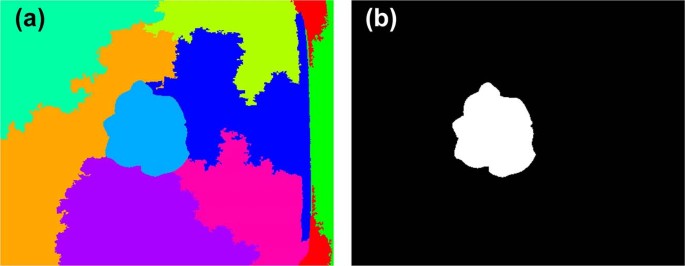
a shows the randomly colored superpixels, and b show the segmented lettuce plant
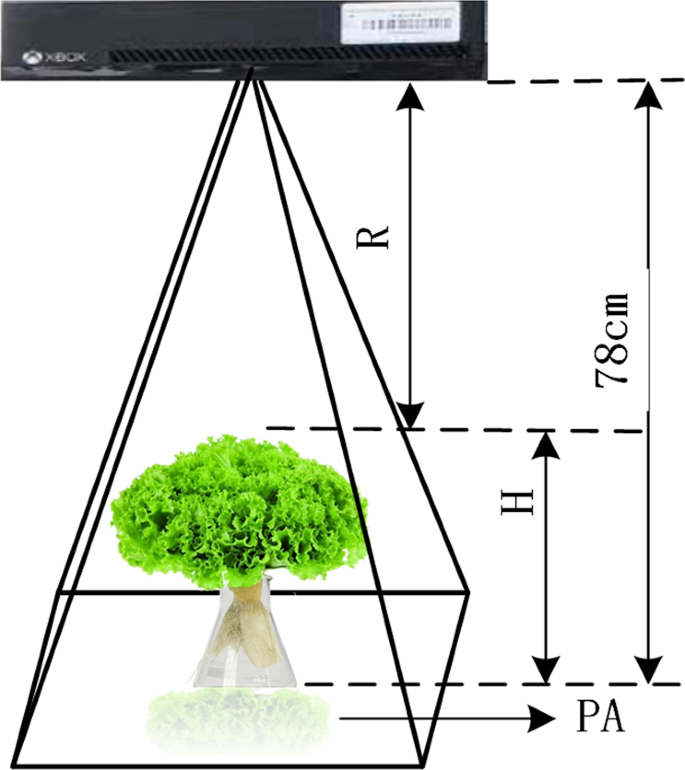
The calculations for PA and H
In this study, the coefficient of determination ( R 2 ) and the normalized root mean square error (NRMSE) were used as the criteria for evaluating the performances of all the estimation models.
In this study, the construction of the estimation models and image preprocessing were implemented using MATLAB 2018b (MathWorks Inc., USA). The software environment was Windows 10 Professional Edition, the hardware environment was an Intel i7 processor, CPU 3.20 GHz, with 8 GB memory, and the GPU was NVIDIA GeForce GTX1060.
Estimation results of the CNN model
When the training process finished, the test dataset was used to test the performance of the CNN model. The performance of the CNN model evaluated over the test dataset is shown in Fig. 7 . The results showed strong correlations between the actual measurements of the growth-related traits and those estimated by the CNN model. It can also be seen that the CNN model demonstrated the best performance on the estimation of LA, achieving the highest R 2 and the lowest NRMSE ( R 2 = 0.9156, NRMSE = 19.94%). The performance of the CNN model for LFW and LDW was similar, with R 2 values of 0.8983 and 0.8910, respectively, and NRMSE values of 26.00% and 22.07%, respectively. For the lettuce cultivars (Fig. 8 and Table 1 ), the CNN model showed different performances. Generally, the CNN model was better at estimating the growth-related traits of Flandria and Tiberius than Locarno, which might be due to the differences in the leaf shape of the lettuce. Flandria and Tiberius have flat-leaf types with relatively stretched leaves, while Locarno is a curled-leaf type with uneven curling leaves. Therefore, the CNN model was able to obtain more comprehensive information when extracting the features of Flandria and Tiberius. However, the leaves of Locarno were more curled, resulting in the learned features not being comprehensive enough to account for the covering and hiding of leaf sections, which affected the estimation accuracy. Therefore, the CNN model achieved the highest prediction accuracy for Flandria and Tiberius.
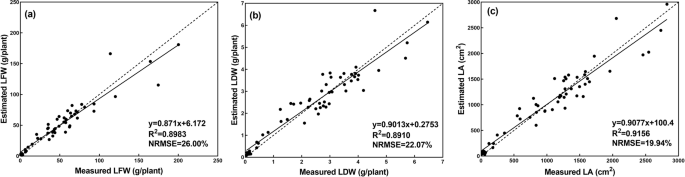
a , b , and c shows the estimation results of LFW, LDW, and LA, dashed line indicates the 1:1 line
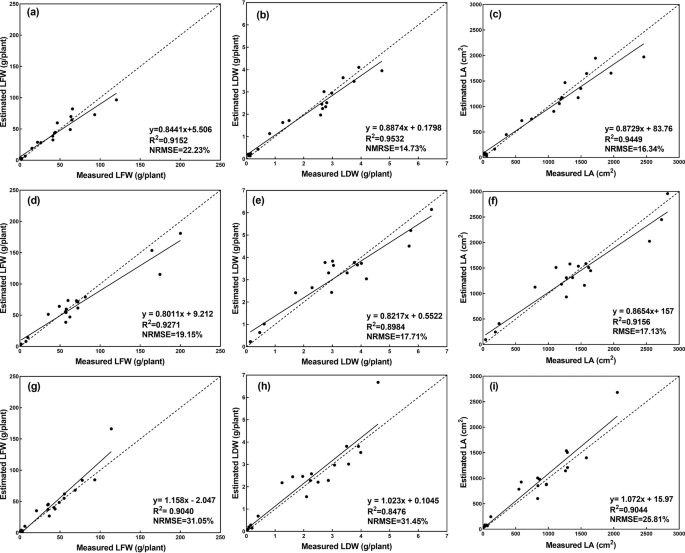
a – c shows the estimation results of LFW, LDW, and LA for Flandria, d – f shows the estimation results of LFW, LDW, and LA for Tiberius, g – i shows the estimation results of LFW, LDW, and LA for Locarno, the dashed line indicates the 1:1 line
Comparison of the results with the conventional estimation methods
Prior to the construction of shallow machine learning classifiers, correlation analysis was performed between pairs of parameters that included the low-level image features and the three growth-related traits. The features that were highly correlated to the actual values of the growth-related traits were used to build the classifiers. The selected features for building the classifiers to estimate LFW, LDW, and LA are shown in Tables 2 – 4 , respectively.
Based on the selected features, SVR and RF models were constructed. The estimation results by the two classifiers are shown in Fig. 9 . For the growth-related trait of LFW, SVR demonstrated better performance than RF, while for the growth-related traits of LDW and LA, RF achieved superior results to SVR. Compared with the performance of the CNN model (Table 5 ), although the R 2 value of RF for estimating LDW was very close, its NRMSE value was approximately 3.5% higher. Considering the R 2 and NRMSE comprehensively, the CNN model indicated better performance on estimating LDW than RF. According to Table 5 , it can be concluded that the CNN models outperformed the two classifiers in estimating all three growth-related traits with higher R 2 values and lower NRMSE values. A possible explanation for the results might be that the construction of SVR and RF was based on the low-level features of the digital images, which could be extracted based on the image segmentation of lettuce plants. This method may be unreliable due to an uneven external illumination and other factors, potentially resulting in a low accuracy image segmentation, which decreased the accuracy of the feature extraction 15 . Furthermore, the low-level image features were artificially designed, indicating that the generalization ability of SVR and RF models was poor 18 , 28 . Therefore, the estimation accuracy for the growth-related traits of the greenhouse lettuce was worse than that of the CNN model.
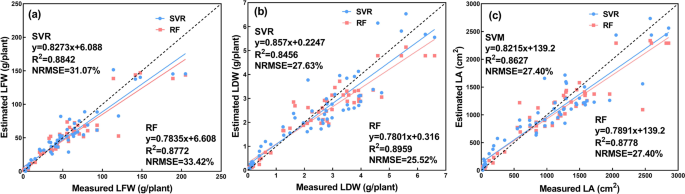
It can be seen from Fig. 10 that the LR-V and LR-PA models demonstrated better estimation performance than the LR-H model. The research objects of this paper were vegetables. From the perspective of horticulture research, the H of lettuce was an essential trait for growth monitoring. However, it is not fair to say, the higher, the better. In the case of nutrient deficiencies, excessive growth can also occur 42 . Therefore, the estimated biomass (LFW and LDW) and LA from the H of greenhouse lettuce can be inaccurate. In addition, existing research has shown that V and PA have relatively strong correlations with the growth-related traits of crops 43 , 44 . Therefore, the results that the LR models that used V and PA as predictor variables exhibited better estimation accuracy than those using H as the predictor variable were within expectation. Compared with the CNN model (Table 5 ), the three LR models (LR-V, LR-PA, and LR-H) based on structural features had low R 2 values and high NRMSE values. In addition, the two shallow machine learning classifiers outperformed the LR models (Table 5 ). This result might be explained by the fact that the growth of lettuce was not only related to geometric features but also related to the color and texture features of lettuce. The structural features derived from depth images were geometric, thus containing no color or texture information of the greenhouse lettuce. Therefore, the LR models based on structural features derived from depth images yielded the worst prediction accuracy.
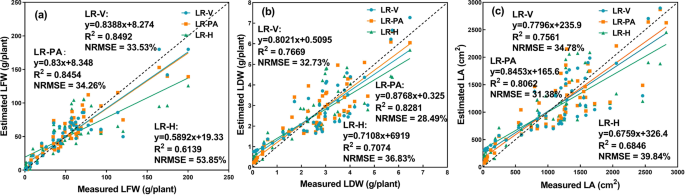
a LFW, b LDW, c LA, dashed line indicates the 1:1 line
Generalization test results
The evaluation of the performance of the proposed estimation method using images from different growing seasons would strengthen the belief in its validity and generalization 45 . Therefore, we performed a generalization test by directly applying the pretrained CNN model to images of Tiberius planted in another growing season (Season 2). We adopted the same experimental design as in “Greenhouse lettuce image collection and preprocessing,” resulting in a dataset containing 200 images and corresponding growth-related traits covering the entire growing season of the greenhouse lettuce.
The estimation results are shown in Fig. 11 . Regression analysis suggested that the values of the three growth-related traits estimated from the images in Season 2 agreed well with the corresponding values derived from field measurements. For the three growth-related traits of LFW, LDW, and LA, the CNN model had R 2 values equal to 0.9277, 0.9126, and 0.9251, respectively, and NRMSE values equal to 22.96%, 37.92%, and 27.60%, respectively. The results revealed that the proposed estimation method had a strong generalization ability. On the other hand, the temperature and humidity in the greenhouse changed with the seasons, which would cause the growth of lettuce to change. Promisingly, the estimation results of the CNN model were still accurate, demonstrating that the proposed estimation method achieved excellent robustness and made a reliable tool for monitoring the growth of greenhouse lettuce.
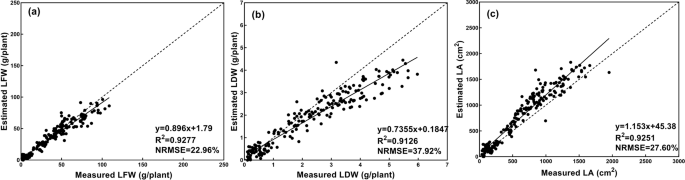
a , b , and c shows the estimation results of LFW, LDW, and LA, dashed line indicates the 1:1
The close and accurate monitoring of crop growth is critical for the optimized management of crop production 46 . Direct measurement of growth-related traits is destructive and inefficient. Nondestructive monitoring has emerged and become a hot topic of current research. Computer vision technology has been widely used in nondestructive monitoring, providing great convenience to growth-related trait acquisition by using conventional methods, i.e., SVR, RF, and LR. However, these methods are limited in practical applications. Therefore, with the rapid development of deep learning, CNN has become preferred by researchers for its advantages, such as no need to manually extract features. In this study, we demonstrated that CNN can serve as a convenient and accurate tool to obtain growth-related traits for greenhouse lettuce. In comparison with the conventional methods, the proposed CNN model showed superior estimation performances in estimating the three growth-related traits for multiple cultivars of greenhouse lettuce, as shown in Table 5 . Specifically, the estimated results of the CNN model on all three growth-related traits had R 2 values above 0.89 and NRMSE values below 27%. The results demonstrated the advantages of the CNN model in that it was able to automatically learn complex feature representations from digital images, which can be translated to a strong generalization ability 47 . The obtained results agreed with previous studies by Ma et al. 18 and Grinblat et al. 23 .
Limitations and future work
Although the proposed method has been shown to be accurate and efficient, there are still limitations that we need to take into account. One limitation is that images were acquired from only the top view, indicating that the error may increase if there are too many overlaps between the leaves. Another limitation is the fixed H during image collection. If the H changes, the estimated results may be biased.
Future studies will continue to collect more images to enlarge our dataset, such as images of other lettuce cultivars. To improve the efficiency of the method, we will explore the growth-related traits of multiple lettuce plants in a single image. In addition, the factors that may influence the performance of the CNN model, such as stress and H for image collection, will also be explored.
Prospective
Growth monitoring can indicate the status of greenhouse lettuce, which is critical for intelligent field management to control the greenhouse environment and establish nutrition strategies. The proposed estimation method allowed us to estimate LFW, LDW, and LA for multiple cultivars of greenhouse lettuce by using digital images, which are low cost and easy to use. The method has great potential for being used in the field when combined with mobile devices or when integrated into other automatic platforms since its input images can be captured by low-cost digital cameras.
Conclusions
In this study, a method for the estimation of growth-related traits of multiple cultivars of greenhouse lettuce was proposed by using digital images and CNN, which could provide support for growth monitoring. The estimated growth-related traits had good agreement with the actual measurements, with R 2 values of ~0.9 and NRMSE values of ~20%. Furthermore, the performance of the proposed method was superior to that of the conventional methods that are widely adopted to estimate growth-related traits. The obtained results showed that the proposed method in this study achieved better estimation performance for Flandria and Tiberius cultivars than Locarno. After another batch of images were acquired of the Tiberius cultivar that was planted in Season 2 for verification, the results reinforced that the proposed estimation method had a strong generalization ability, as well as robust estimation performance despite the seasonal factors. It can be concluded that the proposed method is a reliable tool for estimating the growth-related traits of greenhouse lettuce and has excellent potential in the application of growth monitoring. Furthermore, the accurate monitoring of growth-related traits can provide support for scientific management decision-making.
Data availability
The authors declare that all data supporting the findings of this study are available within the paper and its supplementary information files.
Code availability
Computer program codes and image data used in this study can be accessed through https://figshare.com/s/4e27e3ba666d32daf5c5 .
Teobaldelli, M. et al. Developing an accurate and fast non-destructive single leaf area model for loquat (Eriobotrya japonica Lindl) cultivars. Plants 8 , 1–12 (2019).
Google Scholar
Lati, R. N., Filin, S. & Eizenberg, H. Estimation of plants’ growth parameters via image-based reconstruction of their three-dimensional shape. Agron. J. 105 , 191–198 (2013).
Bauer, A. et al. Combining computer vision and deep learning to enable ultra-scale aerial phenotyping and precision agriculture: a case study of lettuce production. Hortic. Res. 6 , 1–12 (2019).
Levy, P. E. & Jarvis, P. G. Direct and indirect measurements of LAI in millet and fallow vegetation in HAPEX-Sahel. Agric. Meteorol. 97 , 199–212 (1999).
Casadesús, J. & Villegas, D. Conventional digital cameras as a tool for assessing leaf area index and biomass for cereal breeding. Plant Biol. 56 , 7–14 (2014).
Chen, D. et al. Predicting plant biomass accumulation from image-derived parameters. Gigascience 7 , 5–27 (2018).
Zhang, L., Verma, B., Stockwell, D. & Chowdhury, S. Density weighted connectivity of grass pixels in image frames for biomass estimation. Expert Syst. Appl. 101 , 213–227 (2018).
Hu, Y., Wang, L., Xiang, L., Wu, Q. & Jiang, H. Automatic non-destructive growth measurement of leafy vegetables based on kinect. Sensors 18 , 1–23 (2018).
Krishna, G., Sahoo, R. N., Singh, P. & Bajpai, V. Comparison of various modelling approaches for water deficit stress monitoring in rice crop through hyperspectral remote sensing. Agric Water Manag. 213 , 231–244 (2019).
Yu, J., Li, C. & Paterson, A. H. High-throughput phenotyping of cotton plant height using depth images under field conditions. Comput. Electron. Agric 130 , 57–68 (2016).
Tackenberg, O. A new method for non-destructive measurement of biomass, growth rates, vertical biomass distribution and dry matter content based on digital image analysis. Ann. Bot. 99 , 777–783 (2007).
PubMed PubMed Central Google Scholar
Fan, X. et al. A simple visible and near-infrared (V-NIR) camera system for monitoring the leaf area index and growth stage of Italian ryegrass. Comput. Electron. Agric. 144 , 314–323 (2018).
Liu, J. & Pattey, E. Retrieval of leaf area index from top-of-canopy digital photography over agricultural crops. Agric. Meteorol. 150 , 1485–1490 (2010).
Sakamoto, T. et al. Application of day and night digital photographs for estimating maize biophysical characteristics. Precis. Agric. 13 , 285–301 (2012).
Ma, J. et al. A segmentation method for greenhouse vegetable foliar disease spots images using color information and region growing. Comput. Electron. Agric. 142 , 110–117 (2017).
Wan, J., Wang, D., Hoi, S.C.H. & Wu, P. Deep learning for content-based image retrieval: a comprehensive study. In Proc. 22nd ACM International Conference on Multimedia , 157–166 (Istanbul, Turkey, 2014).
Pound, M. P. et al. Deep machine learning provides state-of-the-art performance in image-based plant phenotyping. Gigascience 6 , 1–10 (2017).
Ma, J. et al. Estimating above ground biomass of winter wheat at early growth stages using digital images and deep convolutional neural network. Eur. J. Agron. 103 , 117–129 (2019).
Ferreira, S., Freitas, D. M., Gonçalves, G., Pistori, H. & Theophilo, M. Weed detection in soybean crops using ConvNets. Comput. Electron. Agric 143 , 314–324 (2017).
Ghosal, S. et al. An explainable deep machine vision framework for plant stress phenotyping. Proc. Natl Acad. Sci. 115 , 4613–4618 (2018).
CAS PubMed Google Scholar
Uzal, L. C. et al. Seed-per-pod estimation for plant breeding using deep learning. Comput. Electron. Agric. 150 , 196–204 (2018).
Dyrmann, M., Karstoft, H. & Midtiby, H. S. Plant species classification using deep convolutional neural network. Biosyst. Eng. 151 , 72–80 (2016).
Grinblat, G. L., Uzal, L. C., Larese, M. G. & Granitto, P. M. Deep learning for plant identification using vein morphological patterns. Comput. Electron. Agric. 127 , 418–424 (2016).
Nachtigall, L. G., Araujo, R. M. & Nachtigall, G. R. Classification of apple tree disorders using convolutional neural networks. In Proc. 2016 IEEE 28th International Conference on Tools with Artificial Intelligence (San Jose, California, 2016).
Mohanty, S. P., Hughes, D. P. & Salathé, M. Using deep learning for image-based plant disease detection. Front Plant Sci. 7 , 1–10 (2016).
Ferentinos, K. P. Deep learning models for plant disease detection and diagnosis. Comput. Electron. Agric. 145 , 311–318 (2018).
Ramcharan, A. et al. Deep learning for image-based cassava disease detection. Front Plant Sci. 8 , 1–7 (2017).
Ma, J. et al. A recognition method for cucumber diseases using leaf symptom images based on deep convolutional neural network. Comput. Electron. Agric. 154 , 18–24 (2018).
Ubbens, J., Cieslak, M., Prusinkiewicz, P. & Stavness, I. The use of plant models in deep learning: an application to leaf counting in rosette plants. Plant Methods 14 , 1–10 (2018).
Zhang, Z. Microsoft kinect sensor and its effect. IEEE Multimed. 19 , 4–10 (2012).
Xiong, X. et al. Panicle - SEG: a robust image segmentation method for rice panicles in the field based on deep learning and superpixel optimization. Plant Methods 13 , 1–15 (2017).
Vapnik, V. N. An overview of statistical learning theory. IEEE Trans. Neural Netw. 10 , 988–999 (1999).
Smits, G. & Jordaan, E. M. Improved SVM regression using mixtures of kernels. In Proc. 2002 International Joint Conference on Neural Networks , 2785–2790 (Honolulu, Hawaii, 2002).
Breiman, L. Random forests. Mach. Learn. 45 , 5–32 (2001).
Lin, C. H., Chen, R. T. & Chan, Y. K. A smart content-based image retrieval system based on color and texture feature. Image Vis. Comput. 27 , 658–665 (2009).
Guo, W., Rage, U. K. & Ninomiya, S. Illumination invariant segmentation of vegetation for time series wheat images based on decision tree model. Comput. Electron. Agric 96 , 58–66 (2013).
Donis-González, I. R., Guyer, D. E. & Pease, A. Postharvest noninvasive classification of tough-fibrous asparagus using computed tomography images. Postharvest Biol. Technol. 121 , 27–35 (2016).
Xiong, X. et al. A high‑throughput stereo‑imaging system for quantifying rape leaf traits during the seedling stage. Plant Methods 13 , 1–17 (2017).
Hämmerle, M. & Höfle, B. Direct derivation of maize plant and crop height from low-cost time-of-flight camera measurements. Plant Methods 12 , 1–13 (2016).
Andújar, D., Ribeiro, A., Fernández-quintanilla, C. & Dorado, J. Using depth cameras to extract structural parameters to assess the growth state and yield of cauliflower crops. Comput. Electron. Agric 122 , 67–73 (2016).
Liu, M., Tuzel, O., Ramalingam, S. & Chellappa, R. Entropy rate superpixel segmentation. In Proc. 2011 IEEE Conference on Computer Vision and Pattern Recognition, 2097–2104 (Providence, Rhode Island, 2011).
Yang, S. et al. Method for measurement of vegetable seedlings height based on RGB-D camera. Trans. Chin. Soc. Agric. Machinery. 50 , 128–135 (2019).
Chen, D. et al. Dissecting the phenotypic components of crop plant growthand drought responses based on high-throughput image analysis w open. Plant Cell Online 26 , 4636–4655 (2014).
CAS Google Scholar
Golzarian, M. R., Frick, R. A., Rajendran, K., Berger, B. & Lun, D. S. Accurate inference of shoot biomass from high-throughput images of cereal plants. Plant Methods 7 , 1–11 (2011).
Mortensen, A. K., Bender, A., Whelan, B. & Barbour, M. M. Segmentation of lettuce in coloured 3D point clouds for fresh weight estimation. Comput. Electron. Agric. 154 , 373–381 (2018).
Tudela, J. A., Hernández, N., Pérez-Vicente, A. & Gil, M. I. Postharvest biology and technology growing season climates affect quality of fresh-cut lettuce. Postharvest Biol. Technol. 123 , 60–68 (2017).
Lecun, Y., Bengio, Y. & Hinton, G. Deep learning. Nature 521 , 436–444 (2015).
Download references
Acknowledgements
This study is supported by the Beijing Leafy Vegetables Innovation Team of Modern Agro-industry Technology Research System (BAIC07-2020) and the National Key Research and Development Project of Shandong (2017CXGC0201).
Author information
Authors and affiliations.
China Agricultural University, Beijing, 100083, China
Lingxian Zhang, Zanyu Xu, Yingyi Chen & Zetian Fu
Key Laboratory of Agricultural Informationization Standardization, Ministry of Agriculture and Rural Affairs, Beijing, China
Lingxian Zhang
Institute of Environment and Sustainable Development in Agriculture, Chinese Academy of Agricultural Sciences, Beijing, 100081, China
Dan Xu & Juncheng Ma
You can also search for this author in PubMed Google Scholar
Contributions
L.Z., Z.X., and J.M. wrote the manuscript. Z.X. and D.X. designed and performed the field experiments. J.M., Z.X., and Z.F. designed and implemented the image processing algorithm and the deep learning models. J.M. and Z.X. performed the data analysis. J.M., D.X., L.Z., and Y.C. revised the manuscript. All authors read and approved the final manuscript.
Corresponding authors
Correspondence to Lingxian Zhang or Juncheng Ma .
Ethics declarations
Conflict of interest.
The authors declare that they have no conflict of interest.
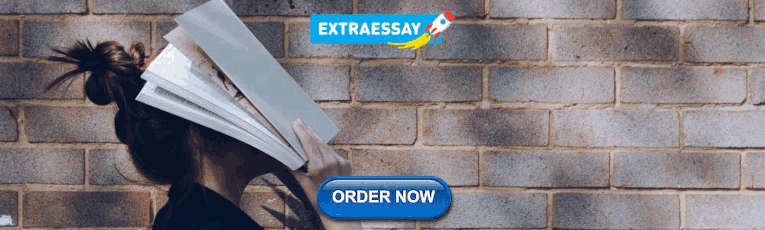
Rights and permissions
Open Access This article is licensed under a Creative Commons Attribution 4.0 International License, which permits use, sharing, adaptation, distribution and reproduction in any medium or format, as long as you give appropriate credit to the original author(s) and the source, provide a link to the Creative Commons license, and indicate if changes were made. The images or other third party material in this article are included in the article’s Creative Commons license, unless indicated otherwise in a credit line to the material. If material is not included in the article’s Creative Commons license and your intended use is not permitted by statutory regulation or exceeds the permitted use, you will need to obtain permission directly from the copyright holder. To view a copy of this license, visit http://creativecommons.org/licenses/by/4.0/ .
Reprints and permissions
About this article
Cite this article.
Zhang, L., Xu, Z., Xu, D. et al. Growth monitoring of greenhouse lettuce based on a convolutional neural network. Hortic Res 7 , 124 (2020). https://doi.org/10.1038/s41438-020-00345-6
Download citation
Received : 29 January 2020
Revised : 20 April 2020
Accepted : 17 May 2020
Published : 01 August 2020
DOI : https://doi.org/10.1038/s41438-020-00345-6
Share this article
Anyone you share the following link with will be able to read this content:
Sorry, a shareable link is not currently available for this article.
Provided by the Springer Nature SharedIt content-sharing initiative
This article is cited by
A hyperspectral deep learning attention model for predicting lettuce chlorophyll content.
- Xiangfeng Tan
- Dedong Kong
Plant Methods (2024)
Quantitative phenotyping and evaluation for lettuce leaves of multiple semantic components
- Chunjiang Zhao
Plant Methods (2022)
Quick links
- Explore articles by subject
- Guide to authors
- Editorial policies


An official website of the United States government
The .gov means it’s official. Federal government websites often end in .gov or .mil. Before sharing sensitive information, make sure you’re on a federal government site.
The site is secure. The https:// ensures that you are connecting to the official website and that any information you provide is encrypted and transmitted securely.
- Publications
- Account settings
Preview improvements coming to the PMC website in October 2024. Learn More or Try it out now .
- Advanced Search
- Journal List
- Front Plant Sci
Morphology, Photosynthetic Traits, and Nutritional Quality of Lettuce Plants as Affected by Green Light Substituting Proportion of Blue and Red Light
1 Institute of Environment and Sustainable Development in Agriculture, Chinese Academy of Agricultural Sciences, Beijing, China
2 Key Laboratory of Energy Conservation and Waste Management of Agricultural Structures, Ministry of Agriculture, Beijing, China
Yu-xin Tong
Jun-ling lu, yang-mei li, rui-feng cheng, associated data.
The original contributions presented in the study are included in the article/ Supplementary Material , further inquiries can be directed to the corresponding author/s.
Green light, as part of the photosynthetically active radiation, has been proven to have high photosynthetic efficiency once absorbed by plant leaves and can regulate plant physiological activities. However, few studies have investigated the appropriate and efficient way of using the green light for plant production. Thus, the objective of this study was to investigate a moderate amount of green light, partially replacing red and blue light, for plant growth and development. In this experiment, four treatments were set up by adjusting the relative amount of green light as 0 (RB), 30 (G30), 60 (G60), and 90 (G90) μmol m −2 s −1 , respectively, with a total photosynthetic photon flux density of 200 μmol m −2 s −1 and a fixed red-to-blue ratio of 4:1. Lettuce ( Lactuca sativa cv. ‘Tiberius’) plant growth and morphology, stomatal characteristics, light absorptance and transmittance, photosynthetic characteristics, and nutritional quality were investigated. The results showed that: (1) shoot dry weight increased by 16.3 and 24.5% and leaf area increased by 11.9 and 16.2% under G30 and G60, respectively, compared with those under RB. Plant stem length increased linearly with increasing green-to-blue light ratio; (2) light transmittance of lettuce leaf under treatments employing green light was higher than that under RB, especially in the green region; (3) stomatal density increased, whereas stomatal aperture area decreased with the increase in the relative amount of green light; and (4) carbohydrate accumulation increased under G60 and G90. Soluble sugar contents under G60 and G90 increased by 39.4 and 19.4%, respectively. Nitrate contents under G30, G60, and G90 decreased by 26.2, 40.3, and 43.4%, respectively. The above results indicated that 15–30% green light replacing red and blue light effectively increased the yield and nutritional quality of lettuce plants.
Introduction
Plants perceive not only light intensity and photoperiod but also light quality, including monochromatic and polychromatic light, as ambient growth environment signals that induce a large number of physiological responses (Kami et al., 2010 ). Different light qualities have distinctly different biological effects on plants (Li et al., 2020 ). There is a misconception that green light is less useful for plant photosynthesis, probably because the light absorption of photosynthetic pigments is relatively low within the green region compared with that within the red and blue regions, especially in the plant canopy (McCree, 1972 ; Smith et al., 2017 ). This is also the reason that red and blue light, rather than other lights, are widely used in recently developed plant factories with artificial lighting for plant production (Wang et al., 2016 ). Moreover, the photoreceptors of red and far-red, blue, and UV-B have been identified as phytochromes, cryptochromes and phototropins, and UV Resistance Locus 8 (Bantis et al., 2018 ), respectively, but no specific green light photoreceptor was detected in previous studies. Even so, the vital role of green light affecting plant physiological activities was gradually proved in previous studies (Johkan et al., 2012 ; Wang and Folta, 2013 ; Materová et al., 2017 ).
A recently published review suggested that green light distributed energy among plant leaves and canopies (Smith et al., 2017 ) because green light was capable of reaching deeper and drives CO 2 fixation under plant canopy, whereas most of the red and blue light is generally absorbed by the upper part of plant leaves (Sun et al., 1998 ; Schenkels et al., 2020 ). In addition, a green light could easily induce a shade avoidance syndrome in plants because, in natural conditions, green light transmitted through the plant canopy, causing a sharp decline in blue-to-green (B/G) ratio from the top to the bottom layers of plants (Zadoks et al., 1974 ). Similar to the shading response caused by far-red light, plant stem elongation and leaf area expansion also occurred under green light (Sellaro et al., 2010 ; Zhang et al., 2011 ). Besides, green light shifted cryptochromes from semi-reduced active state caused by blue light to the fully reduced and inactive state. Thus, green light could inactivate blue-mediated responses (Bouly et al., 2007 ; Liu et al., 2010 ; Sellaro et al., 2010 ).
Recent studies suggested that green light should not be ignored in plant growth and development. Kaiser et al. ( 2019 ) reported that, by replacing red and blue with green light, the fresh and dry weights of tomato increased linearly with an increase in the percentage of green light. Schenkels et al. ( 2020 ) have shown that additional green light significantly increased the total fresh and dry weights of plant seedlings, whereas when replacing red and blue with green light, no significant increase was found in dry weight. The above inconsistent results were mainly caused by the different proportions of green light in the light source. According to McCree ( 1972 ), once absorbed by plant leaves, green light showed a higher relative quantum efficiency than blue light. Moreover, the shade avoidance syndrome induced by green light was capable of increasing the stem length and leaf area of plants (Johkan et al., 2012 ). These changes in plant morphology effectively enhance the photons captured by plant leaves, improving the photosynthetic efficiency (Park and Runkle, 2017 ). Thus, investigating a moderate proportion of green light can optimize the photosynthesis of plant leaves to maximize the yield in plant production.
Therefore, this study aimed to investigate the optimal proportion of green light for lettuce growth and development by adjusting the relative amount of green light. Combined red and blue light were used as the fundamental light. Lettuce ( Lactuca sativa cv. ‘Tiberius’) was used in this experiment because it is one of the most popular horticulture vegetables produced in plant factories with artificial lighting. Lettuce morphology, biomass, stomatal characteristics, light absorptance and transmittance, photosynthetic traits, and nutritional quality were evaluated.
Materials and Methods
Plant material and growth condition.
Lettuce ( Lactuca sativa cv. ‘Tiberius’) seeds were sown in sponge blocks filled in plastic trays. Seedlings were developed under fluorescent lamps (TL-D56W, Osram, Munich, Germany) with a light intensity of 150 μmol m −2 s −1 and a photoperiod of 16 h day −1 . Upon emerging three true leaves (15 days after sowing), seedlings were transplanted in a plant factory with artificial lighting (PFAL), in which the day/night temperature was 24/20°C, relative humidity was 60–70%, and the concentration of CO 2 (supplied by a CO 2 gas cylinder) was 1,000 μmol mol −1 . Plants were cultivated in a customized circulation system of nutrient solution with a planting density of 32 plants m −2 . The light-emitting diode (LED) panels (Datang New Energy Technology Co., Ltd., Shenzhen, China) were equipped on cultivation shelves. The distance between the LED panels and the culture bed was 0.3 m. An opaque white plastic reflective film was placed around the LED panels to ensure uniform radiation on the surface of the culture beds and to prevent light pollution from the adjacent treatments. Modified Yamasaki nutrient solution (EC = 1.2 dS m −1 , pH = 5.8) was applied for the plant growth. The circulation system was automatically operated for 1 h day −1 .
Experimental Setup
The photosynthetic photon flux density (PPFD) and red (600–690 nm, peak at 660 nm) to blue (410–490 nm, peak at 450 nm) (R/B) ratio of all treatments were maintained at 200 μmol m −2 s −1 and 4:1, respectively. The relative amount of green light (490–590 nm, peak at 525 nm) of each treatment was adjusted to 0 (RB), 30 (G30), 60 (G60), and 90 (G90) μmol m −2 s −1 ( Table 1 ). The light spectrum of each treatment was presented in Figure 1 . Plants were harvested on day 20 after transplanting. This experiment was repeated three times.
Different light intensity (μmol m −2 s −1 ) of red, blue, and green light of four treatments.

The spectral distribution of different treatments (A) and photograph of lettuce grown under treatments (B) .
Determinations of Plant Growth and Morphology
For the growth and morphology analysis, destructive measurements were taken on five plants of each treatment on day 20 after transplanting. Lettuce samples were separated into shoot and root using sharp scalpels and forceps. The stem length was the average value of stem lengths in fully expanded leaves of each plant. The leaf area was measured by an area meter (LI-3100C, LI-COR Biosciences, Lincoln, NE, USA). The fresh and dry weights of shoot and roots were measured by an electronic balance (Si-234; Denver Instrument, Bohemia, NY, USA). Whole-plant net assimilation was calculated by dividing shoot dry weight by total leaf area for each plant.
Measurements of Light Absorptance and Light Transmittance of Leaves
Light reflectance (Rf) and light transmittance (Tr) were measured on the fully expanded second leaves with a spectroradiometer (Ocean Optics USB2000+, Dunedin, FL, USA) in combination with two integrating spheres (FOIS-1, ISP-REF, Ocean Optics Inc., Dunedin, FL, USA). Light absorptance (Ab) was calculated as: Ab = 1 – (Rf + Tr). The diagram of the measuring apparatus is showed in Supplementary Figure 1 .
Observation of Stomata
Leaf samples were collected from the fully expanded second young leaves of five plants in the same region of each treatment. Leaf stomatal characteristics were measured using the method of Wang et al. ( 2016 ). The stomata of plants are regarded as rhombus. Stomatal aperture area was calculated by long axis length of aperture (Al) and short axis length of aperture (As). Stomatal aperture area = Al × As/2.
Determinations of Photosynthetic Pigments and Photosynthetic Characteristics
From the top to the bottom, the leaves of 2, 4, 6, 8, 10, 12, 14, 16, 18, and 20 from four plants in each treatment were collected at the same point with 1 cm 2 by using a punch. Subsequently, 96% ethanol was used as a solvent for these samples to measure chlorophyll a (Chl a), chlorophyll b (Chl b), and carotenoid content. The absorbance was measured at 665, 649, and 470 nm by using a spectrophotometer (UV-1800, Shimadzu Corp., Kyoto, Japan). Concentrations of chlorophyll and carotenoid were determined using the equations reported by Lichtenthaler and Wellburn ( 1983 ). All of the data measured were used for the normal distribution analysis shown in Figure 7 .

Box-plot and normal distribution of concentrations of total chlorophyll (A) , carotenoid (B) , and Chl a/Chl b (C) of different layers of lettuce under different proportions of green light.
Net photosynthetic rate (P n ), gas exchange (g s ), and intercellular CO 2 concentration (Ci) of lettuce fully expanded second leaves were measured using a portable photosynthetic instrument (LI-6400XT, LI-COR Biosciences, Lincoln, NE, USA) with a transparent leaf chamber (6400-08, LI-COR Biosciences, Lincoln, NE, USA) under the actual light condition of plant growth. The environmental conditions of the leaf chamber were maintained at 24°C, 1,000 μmol m −2 s −1 CO 2 level, and 60–70% relative humidity (Li et al., 2020 ). The order of measurements was arranged randomly for each repetition, and each treatment was repeated three times. P n and g s of lettuce leaves measured in LED leaf chamber were represented by P n−T and g s−T , respectively.
P n and g s to light intensity response curves were taken on the fully expanded second leaves by using the same portable photosynthetic instrument (LI-6400XT, LI-COR Biosciences, Lincoln, NE, USA) with a red–blue LED leaf chamber (6400-02B, LI-COR Biosciences, Lincoln, NE, USA) installed. The leaf chamber temperature, the concentration of CO 2 , relative humidity, airflow rate, and leaf-to-air vapor pressure difference (VPD leaf−air ) in the leaf chamber were 24°C, 1,000 μmol mol −1 , 60–70%, 500 μmol s −1 , and 1.0 ± 0.1 kPa, respectively. P n and g s under different light intensities were measured subsequently. The starting light intensity was 200 μmol m −2 s −1 , followed by 100, 0, 200, 400, 600, 800, 1,000, and 1,200 μmol m −2 s −1 . Light intensity decreasing from the actual growth level to dark and then gradually increasing to high light intensities was needed when measuring light intensity-photosynthesis response curves of plant leaves (Wang et al., 2016 ). The light source was red and blue light with an R/B ratio of 4:1. P n and g s were recorded when P n reached a steady state at each light intensity. Measurement order was arranged randomly for every repetition, and each treatment was repeated three times. P n and g s of lettuce leaves measured in LED leaf chamber were represented by P n−L and g s−L , respectively.
Determinations of Sucrose, Starch, and Nutritional Quality
Lettuce leaves were collected in sample bottles, quick-frozen by using liquid nitrogen, and stored in an ultralow temperature freezer before the end of the dark period. Five plants of each treatment were taken at the end of the light period on day 20 after transplanting. The samples were used to measure sucrose, starch, and nutritional quality contents, including soluble sugar, nitrate, and crude fiber. Sucrose content was determined using the method described by Fils-Lycaon et al. ( 2011 ) and measured at 480 nm. Starch content was measured and calculated according to Clegg ( 1956 ). Soluble sugar content was determined using the method described by Fairbairn ( 1953 ). Nitrate content was determined by the method described by Cataldo et al. ( 1975 ). The ELISA performed by using the double-antibody one-step sandwich method was used to determine the crude fiber content of lettuce leaves. The absorbance was measured at 450 nm wavelength with a spectrophotometer (Shimadzu UV-1800, Shimadzu Corp., Kyoto, Japan).
Statistical Analysis
This experiment was designed as a single factor experiment. A one-way ANOVA was performed for each treatment. When the ANOVA result was significant, Duncan's multiple range test at p < 0.05 was used for mean separation. The linear relationships between plant stem length and G/B ratio were determined by GraphPad Prism 8 (GraphPad Software Inc., San Diego, CA, USA), using simple linear regression. The fitting light intensity response curves of P n and g s with non-linear regression were performed by the GraphPad Prism 8 (GraphPad Software Inc., San Diego, CA, USA), using [Agonist] vs. response–variable slope (four parameters) equation. The data of chlorophyll concentration in different layers of lettuce plants were performed by a box-plot and drawn with the Origin 2020 software (OriginLab Inc., Northampton, MA, USA). The desperation of data is proportional to the height of the box. The lines and dots in the box represent the median and the average values, respectively. The highest and the lowest point of the vertical line represent the maximum and minimum values. All statistical analyses were performed by the IBM SPSS Statistics 26 program (SPSS Inc., Chicago, IL, USA). This experiment was repeated three times.
Growth and Morphology
As shown in Figure 2 , plant fresh weight, leaf area, and shoot dry weight were 12–25% greater under G30 and G60 than those under RB or G90. The increased leaf area accounted for most of the increased plant mass so that the trend of whole-plant net assimilation was nearly constant with the leaf area among four treatments. The stem length of lettuce increased linearly with increasing G/B ratio ( Figure 3 ).

Plant fresh weight (A) , leaf area (B) , shoot dry weight (C) , and whole-plant net assimilation (D) of lettuce plants as affected by the different proportions of green light. Different letters in each column indicate significant differences at p < 0.05, according to the least significant difference (LSD) test.

Stem length of lettuce leaves as affected by green-to-blue (G/B) ratio. Associated correlation of coefficients ( R 2 ) and regression equations presented here indicate significant differences at p < 0.05.
Light Absorptance and Light Transmittance of Lettuce Leaves
As shown in Figure 4 , the lowest light absorptance and highest light transmittance of lettuce leaves were observed in the green range within the visible spectrum of 400–700 nm. Meanwhile, the light transmittance was higher under treatments employing green light than that under RB, whereas the light absorptance was, on the contrary, especially within the green region. The minimal absorptance and maximal transmittance found at the same wavelength point (555 nm) were 80% under G30 and 8% under G60, respectively.

Light absorptance (A) and light transmittance (B) as affected by the different proportions of green light.
Stomatal Characteristics
As shown in Table 2 , the stomatal development was significantly affected by the relative amount of green light. Stomatal density increased by 47, 38, and 71% under G30, G60, and G90, respectively, compared with that under RB, whereas epidermal cell density only increased under G90. With the increase in green proportion from 15 to 45%, the long axis length and short axis length of aperture decreased by 7–25% and 14–51%, respectively. The reduction of long and short axis length of aperture caused a linear decrease in aperture area from 45.1 to 16.5 μm 2 with an increase in the relative amount of green light ( Figure 5 ).
Effects of green light on stomatal characteristics of lettuce.
The data are means ± SDs (n = 5). Different lowercase letters in the same line show significant difference at p < 0.05, according to the least significant difference (LSD) test .

Photos of stomatal characteristics under the different proportions of green light.
Leaf Photosynthetic Pigments
The concentrations of total chlorophyll and carotenoids, and Chl a/Chl b in different leaf layers of lettuce were different. As shown in Figure 6 , these three pigment parameters increased dramatically from the 2nd to the 4th leaf. Meanwhile, the total chlorophyll and carotenoids under G60 and G90 reached their peak at the 4th leaf, whereas they decreased dramatically at the 6th leaf. Then, the curves of the three pigment parameters were relatively flat under G30, G60, and G90 from the 6th to the 14th leaf, and gradually dropped from the 14th to the 20th leaf. A significantly decrease under RB was observed on the three pigment parameters from the 12th to the 20th leaf and finally reached the lowest level among all treatments.

The concentrations of total chlorophyll (A) , carotenoids (B) , and of leaves and Chl a/Chl b (C) of different layers of lettuce plants as affected by the different proportions of green light.
As shown in Figure 7 , the data of the three pigment parameters of lettuce leaves in different layers under G60 are the most compact in all treatments, whether from the difference between the maximum and minimum values or the height of the box. Employing green light, the difference between maximum and minimum values was decreased significantly, though the highest values of the three pigment parameters were observed under RB. These results suggested that green light reduced the gap of the concentrations of pigments between upper and lower leaves of plants, especially when green light accounts for 30% (G60) in the light source.
Leaf Photosynthetic Traits
P n−T under G30 decreased by 6% compared with that under RB, whereas no significant difference in P n−T was found among RB, G60, and G90. g s−T and Ci under treatments employing green light decreased significantly compared with those under RB ( Figure 8 ).

P n−T (A) , g s−T (B) , and Ci (C) under the actual light condition of lettuce leaves as affected by different proportions of green light. Different letters in each column indicate significant differences at p < 0.05, according to the least significant difference (LSD) test.
As shown in Figure 9 , P n−L and g s−L increased with the increasing light intensity. Among treatments employing green light, g s−L increased with an increase in the relative amount of green light. Under G30, g s−L was lower than that under RB, especially when light intensity was lower than 1,000 μmol m −2 s −1 , whereas g s−L under G60 and G90 was higher than that under RB. There was no obvious difference in P n−L among all treatments under the same light intensity ( Figure 9 ).

P n−L (A) and g s−L (B) to light intensity response curves as affected by the different proportions of green light.
Sucrose, Starch, and Nutritional Quality
As shown in Table 3 , an increase in the carbohydrate accumulation of lettuce leaves was found under treatments employing green light compared with that under RB. Sucrose and starch contents increased by 60 and 37% under G60 and by 35 and 40% under G90, respectively, compared with those under RB. In terms of nutritional quality, soluble sugar contents under G60 and G90 increased by 39 and 19%, respectively, compared with that under RB. Nitrate contents of lettuce leaves significantly decreased under treatments employing green light. Nitrate contents under G30, G60, and G90 decreased by 26, 40, and 43%, respectively, compared with that under RB. No significant differences in crude fiber contents were found among all treatments.
The accumulation of carbohydrate and nutritional quality as affected by different relative amount of green light.
Morphology of Lettuce Plants as Affected by Employing Green Light
Light quality, as energy and signal sources, significantly affects plant photomorphogenesis (Bantis et al., 2018 ). Similar to red/far-red (R/FR) ratio, the G/B ratio also could act as a shade signal within a plant canopy and could provide information about the degree of shading, triggering physiological and morphological changes of plants to best intercept available energy (Sellaro et al., 2010 ; Smith et al., 2017 ). In this experiment, lettuce stem length increased with increasing G/B ratio. This result was consistent with the finding that the hypocotyl length of Arabidopsis seedlings increased linearly with increasing G/B ratios within the range of 0.5–1.0 while cultivated in a natural environment (Sellaro et al., 2010 ). The above phenomenon can be explained by the shade avoidance syndrome induced by the green light. As shown in Figure 4 , the light transmittance in the green region was higher than that in the blue region so that an increase in G/B ratio was induced under the lettuce canopy, providing a shade signal for plants. The shade signal can be detected by relevant non-photosynthetic photoreceptors, and it can then induce the shade avoidance syndrome, such as stem elongation and leaf expansion, to intercept more available light energy (Wang and Folta, 2013 ). Kang et al. ( 2016 ) also found that green light increased the leaf length in lettuce plants. Moreover, leaf expansion was also found under G30 and G60 in this study. This was consistent with the finding that lettuce leaf area increased dramatically when green light replaced red and blue light (Kim et al., 2004 ). This kind of morphological changes caused by a high G/B ratio may be attributed to cryptochromes or an unknown role of phytochromes, or perhaps a novel light photoreceptor (Sellaro et al., 2010 ; Zhang et al., 2011 ). However, the leaf area under G90 showed no significant difference with RB. This may be caused by the low yield photon flux density (YPFD) in G90 (Johkan et al., 2012 ).
Gas Exchange of Lettuce Leaves as Affected by Employing Green Light
Stomatal conductance was mainly affected by stomatal density and the degree of stomatal opening (Fanourakis et al., 2015 ). Previous studies have shown that stomatal density in newly developed leaves of C3 plants is independent of their adjacent irradiance (Lake et al., 2001 ; Miyazawa et al., 2006 ). On the contrary, exposure of mature leaves to low light conditions triggered long-distance signaling that controls the stomatal development and caused a reduction in stomatal density in young leaves (Ehonen et al., 2020 ). This phenomenon is called systemic signaling (Lake et al., 2001 ). In this experiment, stomatal density increased with an increase in the relative amount of green light. This should be attributed to green light showing a higher light transmittance to plant canopy in comparison with red and blue light. Meanwhile, the local light intensity in mature leaves of relative lower layers increased with the increasing relative amount of green light. The above result was consistent with the finding that stomatal density increased significantly when blue LEDs were replaced with green LEDs in green leaf lettuce (Son and Oh, 2015 ).
Green light can reverse the stomatal openings stimulated by blue light (Frechilla et al., 2000 ), because the fully oxidized state of the flavin chromophore of cryptochrome in darkness is shifted to a semi-reduced form by blue light, and this semi-reduced form is converted to a reduced form particularly by absorbing green light (Liu et al., 2010 ). This was the reason why stomatal aperture decreased linearly with increasing G/B ratio in this study. This was also the main reason that caused lower g s−T under treatments employing green light than that under RB. However, the stomatal opening could be restored if the green light was followed or replaced by a second blue light in a response analogous to the R/FR reversibility of phytochrome responses (Frechilla et al., 2000 ; Taiz and Zeiger, 2015 ). In this research, the stomatal density of lettuce leaves caused by systemic signaling could not change immediately with the change of light quality; thus, under the same red and blue light condition, g s−L increased with an increase in the relative amount of green light. The above phenomenon gave an efficient way to regulate stomatal behaviors. In future experiments, green light can be considered to increase the stomatal density at the early growth stage of plant leaves, whereas blue light can be considered to increase the stomatal opening at the yield-forming stage of plants so that, especially in plant factories, plant yield can be increased efficiently. Previous studies also demonstrated that stomatal response to the light environment could provide potential targets for increasing photosynthesis, water use efficiency, and overall plant yield (Matthews et al., 2020 ).
Green Light Affected Pigmentation, Light Energy Distribution, and Photosynthetic Characteristics in Lettuce
Most of the previous studies mainly focused on the pigment contents of individual leaves rather than the whole plant. In this study, pigment concentrations of the lettuce leaves in different layers were measured to investigate the effect of green light on pigment concentrations of leaves within the canopy. The light absorptance of red and blue light was higher, but the light transmittance was lower in the upper leaves of lettuce. Thus, the relatively scattered pigment concentrations and Chl a/Chl b were found under RB. Compact distribution of pigment concentrations and Chl a/Chl b under G60 was mainly caused by the maximal light transmittance found under G60, which helped the pigmentation of lower layer leaves of lettuce plants in this study. This was beneficial for whole-plant photosynthesis. In comparison with G60, the lower pigment level of bottom leaves under G30 may be due to the relative lower green light intensity, limiting the irradiance to transmitting through the canopy, whereas under G90, they may be related to a stronger shade condition caused by a higher G/B ratio (Smith and Whitelam, 1997 ). These results suggested that a moderate amount of green light can promote the pigmentation of underlying leaves in the lower layer canopy.
Green light was capable of contributing light energy to photosynthesis both at the leaf level and the canopy level of plants (Smith et al., 2017 ). Up to 80% of green light was detected to pass through chloroplast, when 15–25% of green light was considered to transmit through the canopy of plants, efficiently promoting green light absorbed by chloroplasts either in the deeper mesophyll of a single leaf or lower leaves within the canopy, especially in plants with a folded structure, such as lettuce (Terashima et al., 2009 ). Relative lower light absorptance and higher light transmittance in the green region were also found in this experiment, in comparison with red and blue light. Moreover, higher light transmittance was observed in lettuce leaves under treatments employing green light compared with that under RB, especially in green regions. This result suggested that green light promoted more light energy to transmit through leaves. However, no significant increase in the light transmittance was found by using green light in Ocimum basilicum L. (Schenkels et al., 2020 ). This inconsistent phenomenon may be because the green light accounted for only 12% of the light source in this previous study.
Green light can be transmitted into deeper mesophyll of leaves and promote photosynthesis. In addition, once absorbed by chloroplasts, green light could drive photosynthesis with the close quantum efficiency of red and blue light (McCree, 1972 ; Smith et al., 2017 ). Thus, though the YPFD, which has been used to quantify the net radiation driving photosynthesis, of G60 and G90 was lower than that of RB, no significant differences of P n−T among RB, G60, and G90 were found in this research. However, P n−T under G30 was found to be lower than that under RB. This may be because the YPFD of G30 was lower than that of RB, and the relatively lower amount of green light was not enough to promote photosynthesis in the deep layer of leaves. The relatively lower light absorption found under G30 was also the cause of the lowest P n−T found under G30 in this study. When transferred to a similar red–blue light source, lettuce leaves of four treatments showed no differences on P n−L , though g s−L differed. This was mainly because g s was no longer the limiting factor for plant photosynthesis under high CO 2 levels.
Moderate Amount of Green Light Increased the Biomass in Lettuce
Plant dry mass accumulation was mainly determined by the photosynthetic efficiency of plant leaves and total leaf area of plants. The photosynthetic efficiency was primarily affected by the net photosynthetic rate of leaves. However, the net photosynthetic rate (P n ) under the growth environment did not support the growth results in this study. This phenomenon was also found in previous studies (Kim et al., 2004 ; Son and Oh, 2015 ). The possible explanation for this result was that the P n measured on a single leaf cannot represent the P n of the whole plant (Yorio et al., 2001 ; Li et al., 2020 ). Thus, whole-plant net assimilation was introduced in this study because whole-plant net assimilation has been used to evaluate the photosynthesis of the whole plant (Park and Runkle, 2018 ). In this study, the whole-plant net assimilation under G30 and G60 showed a nearly similar trend to the dry mass. This result may indicate that G30 and G60 had the ability to improve the whole-plant photosynthesis of lettuce plants. Moreover, the total leaf area of plants affects the light energy received by the plants, because the incident radiation interception increases with increasing the total leaf area (Bugbee, 2016 ; Snowden et al., 2016 ). The promotive effects of G30 (15% green light) and G60 (30% green light) on dry matter accumulated by lettuce leaves could be mainly attributed to the increase in leaf area caused by the shade avoidance syndrome, as described in this study. Kim et al. ( 2004 ) also found that fresh and dry weights of the lettuce shoot increased significantly when 24% of red and blue light was replaced with green light.
Moderate Amount of Green Light Increased the Concentration of Carbohydrate and Nutritional Quality in Lettuce
Carbohydrate is the final product of CO 2 absorption in the progress of plant photosynthesis (Taiz and Zeiger, 2015 ). In this experiment, the concentration of carbohydrates under the treatments employing green light was at a higher level than that of P n−T in the difference of statistical analysis. This result implicated replacing red and blue light with 15–45% of green light since the ability of plants to accumulate carbohydrates was stronger under green light than under red and blue light.
In general, higher soluble sugar concentration results in a desirable taste of the plant (Lin et al., 2013 ). In this study, a relatively higher soluble sugar concentration was found under G60. Chen et al. ( 2016 ) also found that supplementing green light to white LEDs increased the soluble sugar content and decreased the nitrate content in green oak leaf lettuce. However, vegetables with high nitrate concentrations are harmful to human health. Especially in China, the allowable highest nitrate concentration of leaf vegetables is 3.0 mg g −1 . In this study, the nitrate concentration of lettuce leaves could be significantly decreased by employing green light. Li et al. ( 2020 ) also found that partially replacing red and blue light with green light decreased the nitrate content in lettuce leaves. Bian et al. ( 2018 ) agreed with the above result that green light could decrease the nitrate contents in lettuce leaves by regulating the expression of some specific genes (e.g., NR and NiR ). Crude fiber, as an important component of a plant cell wall, provided the crisp taste of and nutrients in lettuce for human beings (Taiz and Zeiger, 2015 ). However, no significant influence of green light on lettuce crude fiber was found in this study. Results presented in this study suggested that a moderate amount of green light could enhance the nutritional quality of lettuce plants.
The optimal proportion of green light, partially replacing red and blue light, for promoting plant growth and development was investigated in this study. Our results showed that plant growth and morphology, light absorptance and transmittance, pigment concentration, stomatal characteristics, photosynthetic characteristics, carbohydrates, and nutritional quality were affected by different proportions of green light. The best green proportion was 30% in G60 in this study because G60 not only increased the leaf expansion and lettuce yield but also improved the accumulation of carbohydrate and nutritional quality. In addition, 15% green light in G30 and 45% green light in G90 also showed some advantages in lettuce growth. G30 increased the leaf expansion and yield and decreased the nitrate content of lettuce plants. G90 increased the accumulation of carbohydrates and decreased the nitrate content in lettuce plants, but no significant influence of G90 on lettuce yield was observed. Thus, from the perspective of economic benefits, the proportion of green light substituting red and blue light should be controlled between 15 and 30% in the production of lettuce, according to this experiment. The more accurate proportion still needs to be explored deeply in future studies.
Data Availability Statement
Author contributions.
LL carried out the measurements, data analysis, and drafted the manuscript. J-lL, Y-mL, and XL participated in part of measurements. Y-xT and R-fC made substantial guide about the experiment design and manuscript revision. All authors contributed to the article and approved the submitted version.
Conflict of Interest
The authors declare that the research was conducted in the absence of any commercial or financial relationships that could be construed as a potential conflict of interest.
Funding. This work was financially supported by the National Key Research and Development Program, Ministry of Science and Technology of China (No. 2020YFE0203600) and the Science and Technology Partnership Program, Ministry of Science and Technology of China (No. KY201702008).
Supplementary Material
The Supplementary Material for this article can be found online at: https://www.frontiersin.org/articles/10.3389/fpls.2021.627311/full#supplementary-material
Supplementary Figure 1
The diagram of the measuring apparatus.
- Bantis F., Smirnakou S., Ouzounis T., Koukounaras A., Ntagkas N., Radaglou K. (2018). Current status and recent achievements in the field of horticulture with the use of light-emitting diodes (LEDs) . Sci. Hortic. 235 , 437–451. 10.1016/j.scienta.2018.02.058 [ CrossRef ] [ Google Scholar ]
- Bian Z., Cheng R., Wang Y., Yang Q., Lu C. (2018). Effects of green light on nitrate reduction and edible quality of hydroponically grown lettuce ( Lactuca sativa L.) under short-term continuous light from red and blue light-emitting diodes . Environ. Expt. Bot. 153 , 63–71. 10.1016/j.envexpbot.2018.05.010 [ CrossRef ] [ Google Scholar ]
- Bouly J. P., Schleicher E., Dionisio-Sese M., Vandenbussche F., Straeten D. V. D., Bakrim N., et al.. (2007). Cryptochrome blue light photoreceptors are activated through interconversion of flavin redox states . J. Biol. Chem. 282 , 9383–9391. 10.1074/jbc.M609842200 [ PubMed ] [ CrossRef ] [ Google Scholar ]
- Bugbee B. (2016). Toward an optimal spectral quality for plant growth and development: the importance of radiation capture . Acta Hortic. 1134 , 1–12. 10.17660/ActaHortic.2016.1134.1 [ CrossRef ] [ Google Scholar ]
- Cataldo D. A., Maroon M., Schrader L. E., Youngs V. L. (1975). Rapid colorimetric determination of nitrate in plant tissue by nitration of salicylic acid 1 . Commun. Soil Sci Plant Anal. 6 , 71–80. 10.1080/00103627509366547 [ CrossRef ] [ Google Scholar ]
- Chen X., Xue X., Guo W., Wang L., Qiao X. (2016). Growth and nutritional properties of lettuce affected by mixed irradiation of white and supplemental light provided by light-emitting diode . Sci. Hort. 200 , 111–118. 10.1016/j.scienta.2016.01.007 [ CrossRef ] [ Google Scholar ]
- Clegg K. M. (1956). The application of the anthrone reagent to the estimation of starch in cereals . J. Sci. Food Agric. 7 , 40–44. 10.1002/jsfa.2740070108 [ CrossRef ] [ Google Scholar ]
- Ehonen S., Hölttä T., Kangasjärvi J. (2020). Systemic signaling in the regulation of stomatal conductance . Plant Physiol. 182 , 1829–1832. 10.1104/pp.19.01543 [ PMC free article ] [ PubMed ] [ CrossRef ] [ Google Scholar ]
- Fairbairn N. J. (1953). A modified anthrone reagent . Chem. Ind. 4 :86. [ Google Scholar ]
- Fanourakis D., Giday H., Milla R., Pieruschka R., Kjaer K. H., Vasilevski A., et al.. (2015). Pore size regulates operating stomatal conductance, while stomatal densities drive the partitioning of conductance between leaf sides . Ann. Bot. 115 , 555–565. 10.1093/aob/mcu247 [ PMC free article ] [ PubMed ] [ CrossRef ] [ Google Scholar ]
- Fils-Lycaon B., Julianus P., Chillet M., Galas C., Hubert O., Rinaldo D., et al.. (2011). Acid invertase as a serious candidate to control the balance source versus (glucose+fructose) of banana fruit during ripening . Sci. Hortic. 129 , 197–206. 10.1016/j.scienta.2011.03.029 [ CrossRef ] [ Google Scholar ]
- Frechilla S., Talbott L. D., Bogomolni R. A., Zeiger E. (2000). Reversal of blue light-stimulated stomatal opening by green light . Plant Cell Physiol. 41 , 171–176. 10.1093/pcp/41.2.171 [ PubMed ] [ CrossRef ] [ Google Scholar ]
- Johkan M., Shoji K., Goto F., Hahida S., Yoshihara T. (2012). Effect of green light wavelength and intensity on photomorphogenesis and photosynthesis in Lactuca sativa . Environ. Exp. Bot. 75 , 128–133. 10.1016/j.envexpbot.2011.08.010 [ CrossRef ] [ Google Scholar ]
- Kaiser E., Weerherm K., Schipper R., Dieleman J. A. (2019). Partial replacement of red and blue by green light increases biomass and yield in tomato . Sci. Hortic. 249 , 271–279. 10.1016/j.scienta.2019.02.005 [ CrossRef ] [ Google Scholar ]
- Kami C., Lorrain S., Hornitschek P., Fankhauser C. (2010). Light-regulated plant growth and development . Curr. Top. Dev. Biol. 91 , 29–66. 10.1016/S0070-2153(10)91002-8 [ PubMed ] [ CrossRef ] [ Google Scholar ]
- Kang W. H., Park J. S., Park K. S., Son J. E. (2016). Leaf photosynthetic rate, growth, and morphology of lettuce under different fractions of red, blue, and green light from light-emitting diodes (LEDs) . Hortic. Environ. Biotechnol. 57 , 573–579. 10.1007/s13580-016-0093-x [ CrossRef ] [ Google Scholar ]
- Kim H. H., Goins G. D., Wheeler R. M., Sager J. C. (2004). Green-light supplementation for enhanced lettuce growth under red- and blue-light-emitting diodes . Hort. Sci. 39 , 1617–1622. 10.21273/HORTSCI.39.7.1617 [ PubMed ] [ CrossRef ] [ Google Scholar ]
- Lake J. A., Quick W. P., Beerling D. J., Woodward F. I. (2001). Plant development: Signals from mature to new leaves . Nature 411 :154. 10.1038/35075660 [ PubMed ] [ CrossRef ] [ Google Scholar ]
- Li L., Tong Y., Lu J., Li Y., Yang Q. (2020). Lettuce growth, nutritional quality, and energy use efficiency as affected by red-blue light combined with different monochromatic wavelengths . Hort. Sci. 55 , 613–620. 10.21273/HORTSCI14671-19 [ CrossRef ] [ Google Scholar ]
- Lichtenthaler H. K., Wellburn A. R. (1983). Determination of total carotenoids and chlorophylls a and b of leaf extracts in different solvents . Biochem. Soc. Trans. 603 , 591–592. 10.1042/bst0110591 [ CrossRef ] [ Google Scholar ]
- Lin K., Huang M., Huang W., Hsu M., Yang Z., Yang C. (2013). The effects of red, blue, and white light-emitting diodes on the growth, development, and edible quality of hydroponically grown lettuce ( Lactuca sativa, L . var. capitata). Sci. Hortic. 150 , 86–91. 10.1016/j.scienta.2012.10.002 [ CrossRef ] [ Google Scholar ]
- Liu B., Liu H., Zhong D., Lin C. (2010). Searching for a photocycle of the cryptochrome photoreceptors . Curr. Opin. Plant Biol. 13 , 578–586. 10.1016/j.pbi.2010.09.005 [ PMC free article ] [ PubMed ] [ CrossRef ] [ Google Scholar ]
- Materová Z., Sobotka R., Zdvihalová B., Oravec M., Nezval J., Karlicky V., et al.. (2017). Monochromatic green light induces an aberrant accumulation of geranylgeranyled chlorophylls in plants . Plant Physiol. Biochem. 116 , 48–56. 10.1016/j.plaphy.2017.05.002 [ PubMed ] [ CrossRef ] [ Google Scholar ]
- Matthews J. S. A., Vialet-Chabrand S., Lawson T. (2020). Role of blue and red light in stomatal dynamic behavior . J. Exp. Bot. 71 , 2253–2269. 10.1093/jxb/erz563 [ PMC free article ] [ PubMed ] [ CrossRef ] [ Google Scholar ]
- McCree K. J. (1972). The action spectrum, absorptance and quantum yield of photosynthesis in crop plants . J. Agric. Meteorol. 9 , 191–216. 10.1016/0002-1571(71)90022-7 [ CrossRef ] [ Google Scholar ]
- Miyazawa S., Livingston N. J., Turpin D. H. (2006). Stomatal development in new leaves is related to the stomatal conductance of mature leaves in poplar ( Populus trichocarpa × P . deltoides) . J. Exp. Bot. 57 , 373–380. 10.1093/jxb/eri278 [ PubMed ] [ CrossRef ] [ Google Scholar ]
- Park Y., Runkle E. S. (2017). Far-red radiation promotes growth of seedlings by increasing leaf expansion and whole-plant net assimilation . Environ. Exp. Bot. 136 , 41–49. 10.1016/j.envexpbot.2016.12.013 [ CrossRef ] [ Google Scholar ]
- Park Y., Runkle E. S. (2018). Far-red radiation and photosynthetic photon flux density independently regulate seedling growth but interactively regulate flowering . Environ. Exp. Bot. 155 , 206–216. 10.1016/j.envexpbot.2018.06.033 [ CrossRef ] [ Google Scholar ]
- Sager J. C., Smith W. O., Edwards J. L., Cyr K. L. (1988). Photosynthetic efficiency and phytochrome photoequilibria determination using spectral data . T ASABE . 31 , 1882–1889. 10.13031/2013.30952 [ CrossRef ] [ Google Scholar ]
- Schenkels L., Saeys W., Lauwers A., Proft M. P. D. (2020). Green light induces shade avoidance to alter plant morphology and increases biomass production in Ocimum basilicum, L . Sci. Hortic. 261 :109002. 10.1016/j.scienta.2019.109002 [ CrossRef ] [ Google Scholar ]
- Sellaro R., Creyp M., Trupkin S. A., Karayekov E., Buchovsky A. S., Rossi C., et al.. (2010). Cryptochrome as a sensor of the blue/green ratio of natural radiation in arabidopsis 1[C] [W] [OA] . Plant Physiol. 154 , 401–409. 10.1104/pp.110.160820 [ PMC free article ] [ PubMed ] [ CrossRef ] [ Google Scholar ]
- Smith H., Whitelam G. C. (1997). The shade avoidance syndrome: multiple responses mediated by multiple phytochromes . Plant Cell Environ. 20 , 840–844. 10.1046/j.1365-3040.1997.d01-104.x [ CrossRef ] [ Google Scholar ]
- Smith H. L., McAusland L., Murchie E. H. (2017). Don't ignore the green light: Exploring diverse roles in plant processes . J. Exp. Bot. 68 , 2099–2110. 10.1093/jxb/erx098 [ PubMed ] [ CrossRef ] [ Google Scholar ]
- Snowden M. C., Cope K. R., Bugbee B. (2016). Sensitivity of seven diverse species to blue and green light: interactions with photon flux . PLoS ONE 11 :e0163121. 10.1371/journal.pone.0163121 [ PMC free article ] [ PubMed ] [ CrossRef ] [ Google Scholar ]
- Son K., Oh M. M. (2015). Growth, photosynthetic and antioxidant parameters of two lettuce cultivars as affected by red, green, and blue light-emitting diodes . Hortic. Environ. Biotechnol. 56 , 639–653. 10.1007/s13580-015-1064-3 [ CrossRef ] [ Google Scholar ]
- Sun J., Nishio J. N., Vogelmann T. C. (1998). Green light drives CO 2 fixation deep within leaves . Plant Cell Physiol. 39 , 1020–1026. 10.1093/oxfordjournals.pcp.a029298 [ CrossRef ] [ Google Scholar ]
- Taiz L., Zeiger E. (2015). Plant Physiology, 5th Edn . Sunderland, MA: Sinauer Assoc Inc. [ Google Scholar ]
- Terashima I., Fujita T., Inoue T., Chow W. S., Oguchi R. (2009). Green light drives leaf photosynthesis more efficiently than red light in strong white light: revisiting the enigmatic question of why leaves are green . Plant Cell Physiol. 50 , 684–697. 10.1093/pcp/pcp034 [ PubMed ] [ CrossRef ] [ Google Scholar ]
- Wang J., Lu W., Tong Y., Yang Q. (2016). Leaf morphology, photosynthetic performance, chlorophyll fluorescence, stomatal development of lettuce ( Lactuca sativa L.) exposed to different ratios of red light to blue light . Front. Plant Sci. 7 :250. 10.3389/fpls.2016.00250 [ PMC free article ] [ PubMed ] [ CrossRef ] [ Google Scholar ]
- Wang Y., Folta K. M. (2013). Contributions of green light to plant growth and development . Am. J. Bot. 100 , 70–78. 10.3732/ajb.1200354 [ PubMed ] [ CrossRef ] [ Google Scholar ]
- Yorio N. C., Goin G. D., Kagie H. R., Wheeler R. M., Sager J. C. (2001). Improving spinach, radish, and lettuce growth under red light-emitting diodes (LEDs) with blue light supplementation . Hort. Sci. 36 , 380–383. 10.21273/HORTSCI.36.2.380 [ PubMed ] [ CrossRef ] [ Google Scholar ]
- Zadoks J. C., Chang T. T., Konzak C. F. (1974). A decimal code for the growth stages of cereals . Weed Res. 14 , 415–421. 10.1111/j.1365-3180.1974.tb01084.x [ CrossRef ] [ Google Scholar ]
- Zhang T., Maruhnich S. A., Folta K. M. (2011). Green light induces shade avoidance synmptoms 1[C] [W] [OA] . Plant Physiol. 157 , 1528–1536. 10.1104/pp.111.180661 [ PMC free article ] [ PubMed ] [ CrossRef ] [ Google Scholar ]
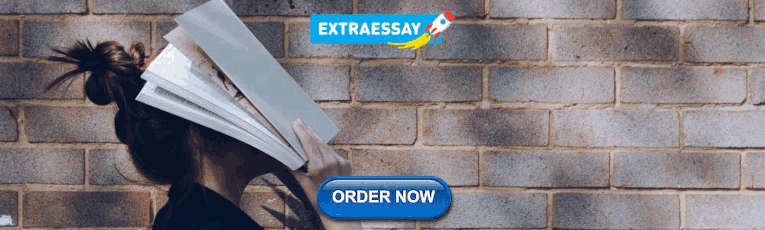
IMAGES
VIDEO
COMMENTS
PDF | On Jun 1, 2017, M. R. Hasan and others published Growth and Yield of Lettuce (Lactuca Sativa L.) Influenced As Nitrogen Fertilizer and Plant Spacing | Find, read and cite all the research ...
Keywords: organic nutrient; hydroponic; production; lettuce; antioxidants; phenols 1. Introduction Lettuce (Lactuca sativa L.) is a member of the Asteraceae family and is widely recognized as one of the most important leafy vegetable crops in terms of crop value [1]. It is a delicious
2. LITERATURE REVIEW Lettuce is able to grown on different types of soil, from light sand to heavy clay soils. A loam-to-clay loam textured soil is considered as the best soil for lettuce production. Good drainage should be ensured when growing lettuce. Preferably the soil pH should be between 5.5 and 7 for optimum growth.
The results found in the literature were related to the effect of the combined RBW (red (R), blue (B), and white (W)) LEDs on some sensory attributes of Boston lettuce [12]. Few studies relating to light requirements in an indoor plant production system have concerned head-forming as romaine lettuce Lactuca sativa var. longifolia [7,17-20].
Lettuce (Lactuca sativa L.) is a major fresh vegetable and its leaves are commonly found in salad mixtures and sandwiches. In some eastern countries like China and Egypt, stems instead of leaves of lettuce are consumed, either cooked, raw, pickled, dried, or as a sauce. Some less common uses for lettuce include a cigarette without
Lettuce. D.A.C. PINK, EAVAN M. KEANE, in Genetic Improvement of Vegetable Crops, 1993 Publisher Summary. Lettuce, Lactuca sativa L., is a cool-season, leafy vegetable that belongs to the Cicoreae tribe of the family Compositae.Lettuce is grown on all continents, but the greatest consumers and producers are the USA (91 000 hectares, of which 60 000 ha are in California) and Europe (total EEC ...
Consuming lettuce is beneficial for human health—related to the bioactive compounds in vegetables . Some epidemiological studies have reported that vegetable consumption is associated with a lower risk of chronic diseases, such as cancer and cardiovascular disease [7,8,9,10,11]. Lettuce is an important natural source of phytochemicals.
Growth-related traits, such as aboveground biomass and leaf area, are critical indicators to characterize the growth of greenhouse lettuce. Currently, nondestructive methods for estimating growth ...
the growth of lettuce and resulted in differences in plant height, number of leaves, stem diameter, leaf area and fresh weight for each lettuce plant. Treatment t6 (continuous nutrition / Non Stop) was known to be the optimum treatment which produced lettuce with a fresh weight of 207.5 g. Keywords: aeroponics; time difference, nutrition ...
Plant Material and Growth Condition. Lettuce (Lactuca sativa cv. 'Tiberius') seeds were sown in sponge blocks filled in plastic trays.Seedlings were developed under fluorescent lamps (TL-D56W, Osram, Munich, Germany) with a light intensity of 150 μmol m −2 s −1 and a photoperiod of 16 h day −1.Upon emerging three true leaves (15 days after sowing), seedlings were transplanted in a ...
about by CNS (inorganic) for almost all parameters, except; for root length and volume of lettuce. The yield achieved by 300 and 600 grams of GC is twice higher than that of CNS. Keywords - Guano char, Lettuce, Residual Effect, Pot experiment INTRODUCTION Lettuce is commonly known as lechugas which is native to Southern Europe and Western Asia.
Lettuce is popular leafy vegetable for its delicate, crisp texture and slightly bitter tang. Lettuce (Lactuca sativa var. Lores, variety Longifolia, Longifolia Group) is often associated with health benefits due to the presence of antioxidant components (María et al.,2013).The nutritive value of lettuce very high but rests largely upon a good ...
Introduction and Literature Review . Hydroponics refers to the technique of growing plants without soil and instead placing roots in a nutrient solution (Jones, 2005; Resh, 2004). It is commonplace to include soilless ... lettuce at 2009 prices is between $158-$198 million per year. Current retail market prices range from $1.50 - $2.00 (Ralf ...
AbstrAct: Lettuce (Lactuca sativa) is the most important crop in the group of leafy vegetables. It is characterized by considerable morphological and genetic variation. The crop comprises seven main groups of cultivars (including oilseed lettuce) difering phenotypically; they are usually described as morphotypes.
NASS 2016). There are five common lettuce types including Summer Crisp, Butterhead, Cos or Romaine, Leaf, and Crisphead (Ryder, 1999). Field production of lettuce is restricted to a short window of time during the late fall to early spring in the southern U. S. because lettuce is susceptible to a number
Lettuce cultivars performance is affected by genotype and environmental conditions. The ability to select the right cultivar contribute highly to realization of increase growth and yield. There is paucity of literature information on the lettuce cultivars that is suitable for increase growth in Cross River, Southern Nigeria.