Carsharing: a systematic literature review and research agenda
Journal of Service Management
ISSN : 1757-5818
Article publication date: 23 July 2021
Issue publication date: 17 December 2021
Following the recent surge in research on carsharing, the paper synthesizes this growing literature to provide a comprehensive understanding of the current state of research and to identify directions for future work. Specifically, this study details implications for service theory and practice.
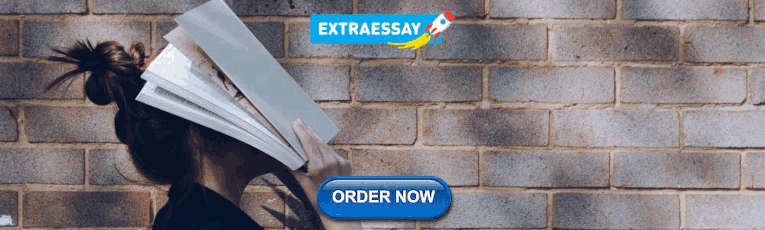
Design/methodology/approach
Systematic selection and analysis of 279 papers from the existing literature, published between 1996 and 2020.
The literature review identified four key themes: business models, drivers and barriers, customer behavior, and vehicle balancing.
Practical implications
For managers, the study illuminates the importance of collaboration among stakeholders within the automotive sector for purposes of widening their customer base and maximizing utilization and profits. For policy makers, their important role in supporting carsharing take-off is highlighted with emphasis on balancing support rendered to different mobility services to promote mutual success.
Originality/value
This is the first systematic multi-disciplinary literature review of carsharing. It integrates insights from transportation, environmental, and business studies, identifying gaps in the existing research and specifically suggesting implications for service research.
- Nonownership
- Vehicle balancing
- Business model
- Access-based consumption
Nansubuga, B. and Kowalkowski, C. (2021), "Carsharing: a systematic literature review and research agenda", Journal of Service Management , Vol. 32 No. 6, pp. 55-91. https://doi.org/10.1108/JOSM-10-2020-0344
Emerald Publishing Limited
Copyright © 2021, Brenda Nansubuga and Christian Kowalkowski
Published by Emerald Publishing Limited. This article is published under the Creative Commons Attribution (CC BY 4.0) licence. Anyone may reproduce, distribute, translate and create derivative works of this article (for both commercial and non-commercial purposes), subject to full attribution to the original publication and authors. The full terms of this licence may be seen at http://creativecommons.org/licences/by/4.0/legalcode
1. Introduction
Environmental concerns and the specter of “peak car” – the idea that per capita distance traveled by an automobile will now fall, threatening the traditional mass-market car business – have prompted automotive manufacturers, service providers, NGOs, and policy makers worldwide to devise and offer a wide range of carsharing services. Carsharing refers to the temporary right to exclusive use of a car without the responsibilities of ownership, with payments linked to usage and/or subscription fees. Unlike traditional car rental and leasing, carsharing relies on platform mediation to identify appropriate matches between provider resources and users and to facilitate exchange ( Eckhardt et al. , 2019 ). While car rentals require a contractual agreement each time one rents a car, carsharing typically requires a membership and subscription. Lovelock and Gummesson (2004) suggested that such nonownership services – that is, marketing exchanges, which do not involve a transfer of ownership – differ distinctly from those that do, conveying benefits through temporary access rather than ownership. By securing the temporary right to use a good, customers avoid “the burdens of ownership” ( Moeller and Wittkowski, 2010 ), such as responsibility for maintenance and repair. Although accumulated service fees over time may be higher than the purchase price, individual payments are lower, making nonownership more affordable by comparison ( Schaefers et al. , 2018 ). Examples of carsharing include the pioneering Zipcar, which commenced operations in Massachusetts in 2000, and ShareNow, a collaboration between German automotive manufacturers BMW and Daimler. Achieving economic viability has proved challenging, and many projects are in retreat, for example, most of ShareNow's international operations have been closed down since 2019 ( Miller, 2019 ). Nevertheless, industry and public research centers continue to invest heavily in new initiatives ( Bocken et al. , 2020 ), and many carsharing service providers see long-term market potential. In 2019, the global carsharing market exceeded US$2.5 billion, with predicted growth of over 24% by 2026 ( Global Growth Trends, 2021 ).
Mirroring these developments, research interest in carsharing has increased across diverse fields that include transportation, environmental and energy studies, and business and management. This research activity has yielded a wide array of analyses and is also an indicator that carsharing is seen to have wide-ranging implications for society. Research on carsharing also reflects the growing interest in various nonownership services ( Schaefers et al. , 2016a ; Fritze et al. , 2020 ). However, despite extensive attention from transportation and environmental researchers – as well as increasing popular interest and media coverage – few of these studies are grounded in service management research.
Against this backdrop, we conducted a systematic review to synthesize the growing literature on carsharing. The dramatic increase in publications in recent years highlights the need for such a review, with more than 60 scientific journal articles published in 2020 alone. The review had three aims: (1) to consolidate existing knowledge and to extract key insights across disciplines; (2) to identify implications for managers and policy makers; and (3) to outline an agenda for service research. The study addresses two main gaps in existing literature reviews. First, as most of these reviews focus on specific issues and fail to acknowledge the full array of relevant research themes, they are not directly comparable. Among these, Jorge and Correia's (2013) review focused specifically on vehicle relocation. Although Ferrero et al. 's (2018) review was (we believe) the first attempt to comprehend the existing carsharing literature, it was not systematic, and their search covered only papers on access-based carsharing published during the period 2001–2016. As more than 180 papers on carsharing have since been published, an updated review is needed to capture newer and more relevant insights on subjects such as peer-to-peer (P2P) carsharing. Furthermore, Ferrero et al. 's study focuses on transportation-related outlets and issues, so neglecting important service management and marketing-related issues. On that basis, the present study addresses the need for an exhaustive thematic review.
The rest of the paper is structured as follows: Section 2 describes the methodology for the systematic selection and analysis of relevant publications. Section 3 presents the study findings, which are grouped under four themes: business models, drivers and barriers, customer behavior, and vehicle balancing. Section 4 outlines a carsharing research agenda that addresses key questions and challenges for service providers and customers. In conclusion, Section 5 identifies some key implications for managers and policy makers.
2. Methodology
The growing volume of fragmented research across disciplines makes it challenging to keep up with existing knowledge of carsharing, and a systematic literature review is needed to integrate findings and perspectives while minimizing bias ( Snyder, 2019 ). A systematic review is an effective means of critically evaluating a body of literature in an objective, transparent, unbiased, and rigorous way ( Boell and Cecez-Kecmanovic, 2015 ; Lyngdoh et al. , 2021 ) and increases the validity of the review process ( Palmatier et al. , 2018 ). Following Tranfield et al. (2003) , our approach involved three stages: planning, conducting and reporting the review.
2.1 Planning and conducting the review
In the first stage ( planning ), we assessed the need for a systematic review of the carsharing literature. In the second stage ( conducting the review), we identified and selected relevant publications, using the specified keywords (“carsharing,” “shared mobility,” “mobility services” and “mobility-as-a-service”) to search the Scopus database. As a leading database, Scopus is widely used for systematic reviews (e.g. Raddats et al. , 2019 ; Witell et al. , 2016 ). To ensure that the search was comprehensive, we used the Boolean operators “AND” and “OR” ( Cronin et al. , 2008 ). As keywords are the cornerstone of a systematic literature review ( Timmins and McCabe, 2005 ), we selected terms that were contextually relevant and reflected the scope of the carsharing literature, excluding related but distinct terms like “ride sharing” or “ride hailing.” Although terms like “shared mobility” and “mobility-as-a-service” tend to be broader in scope than carsharing, some such studies nevertheless relate to carsharing and are likely to provide relevant insights (e.g. Cohen and Kietzmann, 2014 ). More generic terms like “sharing economy” or “access-based services” were not included because a majority of these studies only refer to carsharing as an example among many other services rather than as the focal concern (e.g. Eckhardt et al. , 2019 ; Fritze et al. , 2020 ). Studies that discuss carsharing in detail, like Bardhi and Eckhardt (2012) , appear in searches using the term “carsharing,” but we deliberately excluded studies dealing with other nonownership services that do not involve shared car use, such as car leasing (e.g. Wittkowski et al. , 2013 ).
The search covered peer-reviewed journal papers published in English up to the end of 2020. To ensure a comprehensive overview of the field, the search was not confined to particular subjects or journals ( Snyder et al. , 2016 ), yielding an initial total of 1,417 papers. To begin the exclusion process, we read the abstracts and eliminated 1,051 papers that we deemed to be beyond the scope of our review. Some of the excluded papers related to topics like carbon emissions, autonomous vehicles, electric vehicle ownership, ride hailing, and ride sharing. Some addressed topics like mobile networks or mobility aids for the visually impaired but did not focus on automotive mobility, and these were also eliminated at this stage, leaving 366 papers in total. In the second round of the review, we read the remaining papers in full to assess their quality and to extract relevant information. We excluded any publications whose content failed to meet the specified criteria, for example, shared mobility studies with a very limited discussion of carsharing, or papers that focused on other vehicle sharing systems like bike sharing. As a result, a further 87 papers were excluded, leaving a final sample of 279 papers (see Appendix for subject areas, journals by subject, and number of papers published in each journal). Figure 1 summarizes the search and selection process.
To begin the review stage, the lead author read and categorized each paper in relation to the research topic. The research team then discussed the results, paying particular attention to papers in which the dominant theme was less easily discerned. For example, although electric vehicle carsharing was originally identified as a distinct theme, it was subsequently assimilated to other themes because it was the focus of interest in only a limited number of papers. This iterative analysis yielded four major themes: (1) the wide variety of carsharing business models ( n = 62); (2) customer and service provider drivers and barriers ( n = 56); (3) usage characteristics of carsharing customers, including behaviors and motives ( n = 82); and (4) vehicle balancing, encompassing issues related to station location and vehicle relocation ( n = 79). To assess the reliability of this categorization, another researcher independently reviewed the final sample of 279 papers. We assessed inter-judge reliability in terms of the proportional reduction in loss, which at 0.96 was well above the recommended 0.90 threshold for advanced practice ( Rust and Cooil, 1994 ). In cases where the two researchers differed – usually where a paper covered more than one theme – the research team jointly reviewed and discussed the study before agreeing a mutually acceptable categorization. Any additional relevant insights beyond a study's core theme are noted in the results.
2.2 Descriptive analysis of the field
Research on carsharing has grown rapidly, and the number of papers published in the last four years was more than double the total for all previous years combined. On average, less than two papers were published annually between 1996 (when the first paper appeared) and 2011. The topic began to attract greater research interest in 2012, when 15 papers were published, almost equaling the number of papers published in all previous years combined. Since then, the topic has attracted increasing research attention, and more than 60 papers were published in 2020 alone. Research initially focused on the nature of existing and potential business models for carsharing, customer reasons for engagement and customer behavior; over the years, interest has extended to key challenges like vehicle balancing. Table 1 shows the evolution of coverage of the four themes.
Overall, research on carsharing has most often appeared in transportation journals (125/279), with fewer (43/279) in business and management publications (including service and marketing) or environmental and energy journals (58/279) (see Appendix ). However, the three most cited papers for each theme appear in business and management journals (four papers), transportation journals (six papers), and environmental and energy journals (two papers) (see Table 2 ). While citation analysis is biased toward older publications, it is a useful way of identifying the main work in a given field ( Raddats et al. , 2019 ; Zupic and Čater, 2015 ). It is interesting that while the majority of papers were published in transportation journals, the two most cited papers were consumer studies that appeared in business and management publications. It is also notable that despite the growing importance for service and marketing researchers of concepts like the sharing economy and collaborative consumption (e.g. Eckhardt et al. , 2019 ; Guyader, 2018 ), few such studies have specifically investigated carsharing. As discussed below, it therefore seems useful to develop a fuller account of user needs and the challenges faced by carsharing service providers from the perspective of service management.
This section discusses the four themes elaborated in the third review stage (reporting and dissemination) ( Tranfield et al. , 2003 ). To begin, the evolution of carsharing is briefly summarized.
3.1 Evolution of carsharing
The concept of carsharing can be traced back to 1948 in Zurich, Switzerland, when the earliest known carsharing cooperative was formed by a group of private individuals. This initiative was followed soon after by similar ventures in other European countries, including France and the Netherlands ( Shaheen et al. , 1998 ). Early carsharing schemes operated on a round-trip basis, in which the customer returned the car to its original pick-up location at the end of each rent period. The earliest known one-way carsharing scheme (known as Procotip) was established in France in 1971, but it failed as a result of technological issues and a lack of appropriate control systems ( Shaheen et al. , 2015 ). Despite these early efforts, successful cases of carsharing were not identified until the late 1980 and 1990s, when various carsharing cooperatives in Switzerland (e.g. Mobility) were established. In 2008, Daimler formed the first free-floating carsharing scheme (then called Car2go and now known as ShareNow) in Ulm, Germany ( Firnkorn and Müller, 2012 ).
As concern grew about traffic congestion caused by increased car ownership ( Steininger et al. , 1996 ), carsharing was seen to offer multiple benefits to users, governments and service providers ( Tuan Seik, 2000 ). Beyond its initial appeal to environmentalists and community activists, today's users are motivated by factors like personal convenience and cost savings ( Orski, 2001 ). Other reported reasons for adopting carsharing include time savings, traffic mitigation, and individual attitudes ( Fellows and Pitfield, 2000 ). Shaheen et al. (1999) predicted that carsharing providers might combine their operational expertise with other advanced technology suppliers to offer mobility services with social, economic and environmental benefits. In many respects, this is true of carsharing today, which has been transformed by advances such as P2P services and technologies, growing stakeholder cooperation and integration, new automotive ventures, and a renewed emphasis on electric vehicles (EVs).
3.2 Carsharing business models
A business model describes how a business creates and captures value, articulating the value proposition, resources, and associated costs and revenue mechanisms ( Teece, 2010 ). Among carsharing services providers, business models vary widely from access-based services such as business-to-consumer (B2C) and business-to-business (B2B) models and cooperatives to platform-based peer-to-peer (P2P) models.
Most carsharing business models fall into the category of access-based services ( Bardhi and Eckhardt, 2012 ), which allow customers to access a product for a specified period in return for an access payment while the service provider retains legal ownership ( Schaefers et al. , 2016b ). Access-based services are considered suitable for convenience-seeking customers who do not care about the value of ownership and favor monetary savings ( Hazée et al. , 2017 ). According to Hazée et al. (2017) , such services entail a high level of customer involvement, minimal supervision by the service provider and high levels of interpersonal anonymity, as customers have minimal contact with other customers or employees when accessing the products.
3.2.1 Business-to-consumer carsharing
As the most common type of carsharing, B2C models have so far received the most research attention. This form of carsharing entails ownership of a fleet of cars by a firm, which rents them on demand to private individuals for short periods of time ( Münzel et al. , 2018 ). In addition to these independent service providers, car manufacturers also engage in B2C carsharing in their search for new markets ( Bellos et al. , 2017 ; Perboli et al. , 2018 ). As the owner (or lessee), the service provider assumes responsibility for car maintenance and marketing transactions ( Wilhelms et al. , 2017a ). B2C carsharing is generally usage-based, that is, members pay a service provider for access to cars at a given rate based on minutes or hours, or at a daily rate for distance traveled ( Schmöller et al. , 2015 ). Different membership types are often available – for example, pay-per-use or monthly subscription – according to the customer's expected level of usage ( Bocken et al. , 2020 ). B2C carsharing may be one-way or round-trip (in which the car is returned to the pick-up location after use) ( Cohen and Kietzmann, 2014 ; Le Vine et al. , 2014 ). Additionally, membership may be station-based or free-floating; in the former case, the customer must leave the car at a designated station within a given area, while a free-floating arrangement means they can leave the car at any location within the designated area ( Shaheen et al. , 2015 ). Users of free-floating and station-based services typically use the cars for short trips while round-trip customers mostly take longer trips ( Alencar et al. , 2019 ). Use of free-floating or station-based carsharing also varies according to trip length and purpose ( Heilig et al. , 2018 ); while station-based carsharing is favored mainly when a car is the most effective solution, the free-floating option is often preferred for nonregular trips ( Becker et al. , 2018 ) and saves time as compared to alternatives like public transport ( Becker et al. , 2017a ). Examples of B2C carsharing include Zipcar (founded in 2000 and acquired by Avis Budget Group in 2013) and ShareNow, a joint venture involving the German manufacturers BMW and Daimler.
3.2.2 Business-to-business carsharing
B2B carsharing – also referred to as corporate carsharing or employer-based carsharing ( Clark et al. , 2015 ) – is an option for organizations that do not wish to own or lease a fleet. Using this form of carsharing, employers provide access through a service provider to shared cars for employees' work-related trips ( Fleury et al. , 2017 ). B2B carsharing customers may be private or public sector organizations ( Clark et al. , 2015 ). B2B carsharing is usually offered by B2C service providers (e.g. ShareNow and Volvo Car's M), and the two models are broadly similar, aside from the fact that the B2B customers are organizations, and subscriptions or payments are made by the organization rather than the individual user. Typically, an employer signs a contract with the provider that specifies fees and terms of payment for the service. Potential customer benefits include lower travel costs as compared to car rental or taxis, as well as flexible fleet size, customized services and price models, and consolidated invoicing. For larger customers especially, service providers must have the necessary resources to provide personal support (e.g. through key account managers) and to ensure that the value potential is realized. Although one study of carsharing behavior in Toronto reported that B2B customers use carsharing more frequently than B2C customers ( Costain et al. , 2012 ), B2B carsharing has so far received much less research attention.
3.2.3 Cooperative carsharing
A third type of access-based carsharing business model is also known as the nonprofit car club ( Bonsall et al. , 2002 ) or self-regulating community ( Hofmann et al. , 2017 ), based on collective car ownership and usage within a defined or institutionalized local group such as friends, neighbors or a nonprofit organization ( Nitschke, 2020 ). This model is typically characterized by a communal interest in sharing cars rather than any profit motive ( Cohen and Kietzmann, 2014 ; Münzel et al. , 2018 ). Members of cooperative carsharing schemes usually pay an annual membership deposit or monthly mileage fees, with contractual obligations governing car maintenance and other administrative responsibilities ( Bocken et al. , 2020 ). As nonprofit organizations, cooperative carsharing schemes can often secure funding from government agencies and foundations. As they are usually community-based, these schemes tend to be small in size, favor a round-trip approach and offer only limited choices of car. One example of cooperative carsharing is the member-owned Modo, which was founded in 1997 in Vancouver, Canada.
3.2.4 Peer-to-peer carsharing
P2P carsharing business models can be classified under the broader term collaborative consumption , which is defined as the use of online marketplaces and social networking technologies to facilitate peer-to-peer resource sharing ( Barnes and Mattsson, 2016 ; Hamari et al. , 2016 ). P2P carsharing involves a triadic relationship between a car owner, a platform provider and a car renter (the customer) ( Wilhelms et al. , 2017a ). For the car owner, this involves renting out one's personal car and collecting the agreed monetary compensation ( Barbour et al. , 2020 ); for the customer, it entails renting a car from a private individual through an online platform ( Meelen et al. , 2019 ). As the platform provider does not own any cars, its role in P2P carsharing is to act as an intermediary between the car owner and customer ( Meelen et al. , 2019 ; Münzel et al. , 2018 ). P2P carsharing customers often pay a fee per use, and the platform provider takes a percentage of the rental fee paid by the customer. Under the P2P arrangement, the car owner assumes responsibility for maintaining and cleaning the car ( Hartl et al. , 2018 ) and determines the rental duration ( Bocken et al. , 2020 ). As P2P platform providers do not incur fleet investment costs, this form of carsharing has higher scalability potential than the access-based model ( Hampshire and Gaites, 2011 ; Meelen et al. , 2019 ).
While sharing of goods between individuals has always existed, the idea of P2P carsharing as a business is quite a recent development. Despite its growing popularity, this model has not received much research attention to date, and most studies focus on customer motivations (e.g. Barbour et al. , 2020 ; Dill et al. , 2019 ; Wilhelms et al. , 2017b ). Examples of P2P carsharing platforms include Getaround, which operates in the US and in some European markets (including France and the UK) and Turo, which operates in the US, Canada and the UK.
3.2.5 Business model typology
Based on the work of Hahn et al. (2020) and Wilhelms et al. (2017b) , Table 3 outlines the main characteristics of each business model. There are several differences between carsharing business models beyond value proposition, resources and revenue mechanism. Pricing is one of the most important factors, as it plays a role in determining the customer type and level of usage ( Balac et al. , 2017 ). Various strategies have been devised to make carsharing profitable, including dynamic pricing schemes based on availability and time ( Giorgione et al. , 2020 ); for a company targeting a younger clientele, for example, the ideal pricing model combines usage-based and monthly fees ( Hahn et al. , 2020 ). In the early phase of P2P carsharing, there were several experiments in the absence of an established pricing model; for instance, some operators allowed car owners to specify their own rental prices while others allowed the platform provider to set the price ( Barbour et al. , 2020 ).
Both P2P and cooperative carsharing have a cost advantage over B2C and B2B. As cooperative schemes are not profit-driven, and P2P car owners usually only want to earn some additional income from their cars, rental prices are usually lower than those of B2C and B2B providers, who need to make a profit on their investment ( Hofmann et al. , 2017 ; Münzel et al. , 2018 ). A second difference relates to fleet size; while cooperative schemes often have only a few cars, B2C and B2B providers range from hundreds to more than a thousand cars (depending on the city size), or indeed several thousand in the case of P2P ( Münzel et al. , 2018 ). In terms of required investment, this means that both B2B and B2C carsharing providers need significantly more capital than cooperative or P2P schemes. Additionally, as P2P cars are owned by individuals, the level of service provided may vary from one individual car owner to another.
3.2.6 Digital technologies
Digital technologies have had a major impact on the development of carsharing business models, especially P2P. Lagadic et al. (2019) observed that while carsharing is not a new service type, it has been completely transformed by advances in digital technologies. Although access-based services derive from traditional marketplace rental models, they have evolved through digital technology into a more collaborative and self-service approach ( Bardhi and Eckhardt, 2012 ). Similarly, the growth of carsharing has been aided by advances such as mobile apps, global positioning system (GPS), software that monitors fuel and battery usage, open-ended booking and smart locks that allow instant access ( Alfian et al. , 2015 ; Münzel et al. , 2018 ). In B2B carsharing setups, online dashboards can offer customers a consolidated overview of all user activities.
While technology plays an integral role in all types of carsharing, P2P carsharing relies more than other business models on technological innovations ( Julsrud and Farstad, 2020 ). In particular, P2P carsharing must make booking and key exchange as convenient as possible ( Sprei and Ginnebaugh, 2018 ), and this has become easier with smart locks that allow renters to access a car without having to meet the owner ( Münzel et al. , 2018 ). The increased incorporation of technology into carsharing services enhances the value proposition for the customer by making the service more flexible and convenient ( Lagadic et al. , 2019 ). As noted above, from a service research perspective, P2P carsharing differs from other carsharing models by virtue of its triadic structure. While many of the factors discussed here are relevant to all business models, most studies of carsharing explicitly or implicitly refer to B2C models or to access-based models in general. For that reason, it is important to highlight the differences between B2C and P2P models, especially with regard to customer behavior drivers and barriers.
3.3 Drivers and barriers
Individuals and business customers increasingly choose to rent objects for specific periods of time as needed rather than owning them; Lovelock and Gummesson (2004) refer to this as the rental/access paradigm. This is explained by Moeller and Wittkowski (2010) in terms of what they refer to as the burdens of ownership ; that is, product ownership entails certain burdens that users may prefer to avoid, such as risks related to product updates and obsolescence, responsibility for product maintenance and repair, and incurring the full cost of the product. In the same way, carsharing customers are driven by a desire to avoid the burdens of car ownership. Carsharing has multiple benefits as an affordable mobility alternative for lower-income groups like students and seniors, and as a substitute for transport alternatives such as walking and biking ( Cohen et al. , 2008 ). Most studies of the decision to use carsharing relate to B2C business models, and some recent studies have specifically discussed the issue in the context of B2B or communal carsharing, but few studies to date have dealt specifically with drivers and barriers in relation to P2P carsharing.
3.3.1 Key factors driving the demand and use of carsharing
Multiple studies have found that propensity to adopt carsharing in general is commonly influenced by sociodemographic, geographic and socioeconomic factors (e.g. Cartenì et al. , 2016 ; Coll et al. , 2014 ; Kang et al. , 2016 ). Other factors include convenience ( Orski, 2001 ; Peterson and Simkins, 2019 ), mobility patterns, family decisions, cost, and quality of alternative transport modes, lifestyle, and customer segment ( Chun et al. , 2019 ; Hahn et al. , 2020 ).
Sociodemographic factors like age and gender are often mentioned as influencing factors for adoption of carsharing (e.g. Carteni et al. , 2016 ; Juschten et al. , 2019 ; Shaheen and Martin, 2010 ). Some studies report that the average carsharing customer is young, male, middle class, more highly educated and from a household of below-average size (e.g. Becker et al. , 2017a ; Clewlow, 2016 ; De Luca and Di Pace, 2015 ; Hjorteset and Böcker, 2020 ; Tyndall, 2017 ). In a web-based study of vehicle availability in ten different European cities, Boldrini et al. (2019) found a positive correlation between carsharing demand and sociodemographic and urban indicators such as high educational attainment. In a survey of women's carsharing usage, del Mar Alonso-Almeida (2019) attributed the predominance of male carsharing customers to the fact that the mobile technology platforms on which carsharing services are based are less appealing to women than to men. Similarly, the predominance of younger carsharing customers can be attributed to their greater familiarity with technological devices like smartphones ( Burlando et al. , 2019 ; Guglielmetti Mugion et al. , 2019 ).
Geographically, carsharing demand is higher in urban centers than in rural areas ( Prieto et al. , 2017 ); this can be attributed to factors that make car ownership difficult and more expensive in urban centers, such as limited parking space. Carsharing adoption is positively affected by availability of other transport modes and scarcity of parking space ( Csonka and Csiszar, 2016 : Juschten et al. , 2019 ; Münzel et al. , 2020 ). In addition, close proximity to a carsharing station or to available cars increases the probability that individuals will use carsharing ( Diana and Ceccato, 2019 ; Jian et al. , 2016 ; Juschten et al. , 2019 ; Kent et al. , 2017 ; Zhou and Kockelman, 2011 ). A survey in Beijing by Shaheen and Martin (2010) established that one kilometer is the maximum distance that users are willing to walk to a carsharing station.
Regarding socioeconomic factors, the claim that carsharing is valued for its ability to provide cost savings for customers ( Duncan, 2011 ; Orski, 2001 ) is contradicted by Hjorteset and Böcker (2020) . In their investigation of propensity to adopt carsharing in Norway, they showed that carsharing appeals mainly to individuals who are unconcerned about reducing their expenditure, indicating that the choice is not motivated by cost reduction. Similarly, in a study of carsharing in Switzerland, Juschten et al. (2019) reported that high income increases the probability of adopting carsharing. However, Hjorteset and Böcker (2020) did not distinguish between P2P and B2C customers, and results may vary by carsharing type. While carsharing in the developed economies of Europe and North America is used predominantly by highly educated individuals ( Boldrini et al. , 2019 ; Clewlow, 2016 ; Coll et al. , 2014 ; Hjorteset and Böcker, 2020 ; Münzel et al. , 2020 ), Chun et al. (2019) reported that the opposite is true for developing economies, where highly educated individuals are more likely to favor car ownership.
Studies of the drivers of carsharing indicate a clear relationship between carsharing and urban population density (e.g. de Lorimier and El-Geneidy, 2013 ; Cohen et al. , 2008 ; Coll et al. , 2014 ; Csonka and Csiszar, 2016 ). For example, Becker et al. (2017b) found that free-floating carsharing in Switzerland scales with population density and social activity in a given area. As it is also more prevalent in areas with low car ownership and public transport, they concluded that carsharing serves as an alternative to these transport modes. Similarly, in their analysis of factors that favor carsharing, Coll et al. (2014) identified urban sprawl and a fairly good public transport system as drivers of carsharing.
Carsharing is often linked to general environmental benefits such as reduction of greenhouse gas emissions ( Cohen et al. , 2008 ; Fleury et al. , 2017 ). According to a study investigating choice of transport mode under uncertainty ( Kim et al. , 2017a ), improving the symbolic value of carsharing as an environmentally friendly solution by use of EVs makes it a more attractive option and increases market share. Carsharing is perceived as more environmentally friendly than private car ownership ( Münzel et al. , 2020 ) but less environmentally friendly than public transport ( Kim et al. , 2017a ). However, in a study of EV carsharing in Germany, Schwabe (2020) found that customer adoption of carsharing depends on more than perceived environmental friendliness. A study based on in-depth interviews with carsharing users in Norway ( Julsrud and Farstad, 2020 ) reported similar findings in relation to cooperative carsharing, revealing that in addition to environmental benefits, customers chose cooperative carsharing for its practical benefits, such as the convenience of access to a car. In short, as with carsharing in general, environmental consciousness may be one key driver but is insufficient alone.
Factors affecting demand may also depend on the type of carsharing. For example, if B2B corporate customers are to adopt carsharing, providers must meet high expectations in terms of flexibility and service quality ( Loose et al. , 2006 ). Fleury et al. 's (2017) survey of intention to use B2B carsharing in France indicates that motivating factors – especially for first time users – include tutorial videos, training and ease of access to the service provider. Probability of adoption is further increased if the service provided is perceived as environmentally friendly, which resonates with the growing emphasis on embedding sustainability goals in corporate strategies.
While most existing studies focus on access-based services (especially B2C), the factors that affect demand for P2P carsharing may differ for access-based carsharing models. In a study of five European cities, Münzel et al. (2020) reported that, in contrast to B2C, demand for P2P carsharing was not affected by population density. For car owners and service providers, the greater scalability of P2P overcomes the geographic limitations of B2C carsharing as it is not dependent on population density ( Dill et al. , 2019 ).
3.3.2 Key barriers to carsharing demand and use
The success of carsharing depends on making it more attractive to potential customers by overcoming perceived barriers ( Kim et al. , 2017b ). While previous research focused on the burden of ownership (e.g. Moeller and Wittkowski, 2010 ), Hazée et al. (2017) and Valor (2020) discuss the burdens of access and sharing, respectively. Hazée et al. (2017) classified the burdens of access in terms of functional and psychological barriers. Functional barriers included service complexity, which is associated with perceived access, usage, and understanding, and reliability, which refers to uncertainty about product performance and related technology. Psychological barriers included perceived contamination, referring to the fact that the product is also accessed and used by other (unknown) individuals, and responsibility, referring to customer concerns about being held accountable for their own and others' usage of available services.
These barriers also affect carsharing. In an early study, Shaheen et al. (1998) identified the limited availability of cars as one factor that limited the use of carsharing in residential areas. This finding relates to the issues of complexity and reliability identified by Hazée et al. (2017) as difficulty in accessing carsharing services when required undermines reliability and so acts as a barrier to adoption and use. Using a two-phase online survey, Kim et al. (2017a) confirmed that likelihood of carsharing use diminishes as waiting time increases. In the case of access-based services, cars must therefore be strategically located to service a wider pool of customers ( Kim et al. , 2019 ). In their study of Communauto in Montreal, Canada, de Lorimier and El-Geneidy's (2013) multilevel regression analysis showed that an efficient carsharing system must constantly attract new customers to compensate for lower use among seasoned members. To ensure customer satisfaction, the ideal situation combines high car availability and high car usage.
Low public awareness was identified by Zhou et al. (2017) as another significant obstacle to carsharing adoption in their survey of projected carsharing in Australia, Indonesia, Malaysia and Thailand. This problem relates to the compatibility barrier, where unfamiliarity can be a limiting factor. For example, lack of prior experience of similar services (such as car rental) is likely to deter certain potential customer groups from using carsharing. Both Duan et al. (2020) , who used a stated preference survey to quantify the impacts of potential carsharing demands in Shanghai, and Zhang and Li (2020) , who tested a model based on the theory of planned behavior in a study involving university students in Qingdao, China, reported that individuals who are familiar with car rental programs are more inclined to use carsharing.
In addition, nonmonetary costs (such as the inconvenience of having to reserve a car each time it is needed) may act as a barrier to carsharing ( Duncan, 2011 ). In a survey to understand the factors that influence carsharing adoption, Burlando et al. (2019) found that issues like comfort and independence, which are often associated with private car ownership, may discourage carsharing adoption. Table 4 summarizes the main customer drivers and barriers identified in the literature.
3.3.3 Drivers and barriers for service providers and car owners
While much of the research on carsharing drivers and barriers has focused on the customer (especially in relation to B2C carsharing), only a handful of studies have discussed the service provider perspective. Service providers offer carsharing services for different reasons. Among profit-driven ventures, pure B2C and B2B service providers are motivated mainly by the desire to make a profit on their services, while car manufacturers offer carsharing services mainly to expand their market and to explore untapped niches ( Bellos et al. , 2017 ; Perboli et al. , 2018 ). On the other hand, in a study of 235 car owners in Portland, Oregon, who rented out their cars for P2P carsharing, Dill et al. (2019) found that they were motivated by the prospect of generating extra income, low levels of car use and willingness to help others. Even if not in financial need, car owners in P2P carsharing schemes may be motivated by a desire to maximize car utilization or to make use of an otherwise underutilized asset ( Ballús-Armet et al. , 2014 ; Barbour et al. , 2020 ; Dill et al. , 2019 ). The claim that public awareness drives engagement in carsharing is further supported by the evidence that car owners who are familiar with the concept are more likely to offer their cars for P2P carsharing than those who are not ( Münzel et al. , 2019 ).
In addition to the high levels of investment required to engage in B2C and B2B carsharing, operational hurdles such as vehicle balancing (especially in one-way schemes, as discussed in detail in section 3.5 ) present serious challenges for service providers. While many of the barriers that affect platform-based services have also been identified in studies of access-based services, P2P carsharing must overcome some specific additional hurdles. P2P platform providers often face the double challenge of attracting both car owners and customers ( Wilhelms et al. , 2017b ). Based on 20 in-depth interviews with early adopters in Spain, Valor (2020) identified burdens for car owners that included emotional costs associated with the difficulties of sharing, the possibility of damage and perceived physical insecurity when driving the returned car. For customers, burdens included anticipated friction with owners, uncertainty about contractual conditions, limited reliability and availability, and perceived insecurity when driving a P2P-rented car.
Access-related barriers for P2P car owners include the perceived risk of limited access to their car ( Hazée et al. , 2020 ) and possible damage to the car while rented out. In a study of P2P carsharing in California, Ballús et al. (2014) noted that where the platform provider's package did not include insurance, fewer owners were willing to offer their car, as they would incur higher costs in the event of it being damaged. Hazée et al. (2020) discuss the image barrier as a common feature of collaborative consumption. As users are required to evaluate each other, car owners who worry that P2P carsharing may affect their standing in subsequent service requests may decide to withdraw.
3.4 Customer behavior
This theme relates to the travel behavior of carsharing customers, including specific customer traits and how they use carsharing services.
3.4.1 Usage characteristics of carsharing customers
Depending on the type of customer, carsharing services are used in different ways. In the case of B2C services, private individuals typically use carsharing for leisure travel and shopping ( Sioui et al. , 2012 ), while organizations use B2B carsharing for employees' work-related trips ( Clark et al. , 2015 ). In terms of demand, it may prove worthwhile to attract both private individuals and organizations as two customer groups that complement each other because they use cars at different times. In other words, private individuals might use the service for evening and weekend errands and leisure activities ( Costain et al. , 2012 ; de Lorimier and El-Geneidy, 2013 ), while organizations are likely to use the service during weekday working hours, so maximizing car utilization ( Loose et al. , 2006 ).
Charoniti (2020) investigated stated preferences for carsharing under uncertain travel times and noted that use of carsharing is strongly dependent on activity type and context. For example, Wu et al. 's (2020a) analysis of carsharing in London indicates that infrequent customers often use carsharing to transport bulky luggage while frequent customers are typically commuters. According to Jian et al. (2017) , while high-income customers who choose more luxurious brands use carsharing for leisure and business purposes, lower-income customers often use carsharing for special purposes like vans for moving or traveling with large groups of people.
Many individuals who register for carsharing schemes are not necessarily active customers and use the service only occasionally. In the case of Autolib, an early French EV carsharing initiative, frequency of use per customer continued to decline despite increasing membership; as many registered subscribers used the service infrequently, growth did not translate into increased revenues for the provider ( Lagadic et al. , 2019 ). This issue is less significant for P2P car owners, who incur very low marginal costs in comparison to B2C providers, who own or lease large fleets ( Meelen et al. , 2019 ).
For frequent users, carsharing is not their main mode of transport but is used in combination with other modes ( Ruhrort et al. , 2014 ). Carsharing customers are more likely than car owners to use other complementary mobility services such as bike sharing, walking and public transport ( Clewlow, 2016 : Mishra et al. , 2015 ; Münzel et al. , 2019 ). For example, in comparisons of free-floating carsharing and public transport in Spain and Denmark, respectively, Ampudia-Renuncio et al. (2020) and Carrone et al. (2020) reported that free-floating carsharing is often used as a substitute for public transport. Becker et al. 's (2017a) comparative study of station-based and free-floating carsharing schemes in Switzerland reports similar findings.
Multiple studies have discussed the impact of carsharing on car ownership, and whether the need for a personal car is reduced by replacing it with a shared one (e.g. Firnkorn and Müller, 2015 ; Jochem et al. , 2020 ; Le Vine and Polak, 2019 ). While findings vary, some studies report evidence of a reduction in car ownership as a result of carsharing. For example, in a survey of B2C carsharing members in Seoul, South Korea, Ko et al. (2019) estimated that each shared car replaced 3.3 private cars. Similarly, a study of B2C carsharing providers in Germany found that car owners who adopted carsharing tended to use their own car less frequently ( Loose et al. , 2006 ).
3.4.2 Trust
While trust is an important issue for all business models, its significance differs for B2C and P2P models. Several studies note the particular importance of trust for P2P carsharing; for example, in a study of German P2P providers, Wilhelms et al. (2017a) found that trust between car owners and customers was important, especially in relation to car usage behavior and maintenance. In their comparative study of B2C and P2P carsharing, Hartl et al. (2018) also found that because P2P carsharing involves transactions between strangers, the potentially higher economic risks and lack of regulation make trust more important. When offered a choice between B2C and P2P carsharing, customers tended to choose B2C because interacting with a company was perceived as less risky than with an individual. Similarly, Julsrud and Farstad (2020) reported that customers regarded B2C carsharing as (more) trustworthy and professional. According to Ma et al. (2020) , trust can affect customer loyalty both directly and indirectly, prompting service providers to address this challenge by eliminating factors that might lead to loss of customer trust, such as high transaction costs. Table 5 summarizes the main reported usage characteristics of carsharing customers.
3.4.3 Customer misbehavior
As a form of service cocreation, the carsharing system involves customer interdependency in ensuring that the car is in good condition for the next customer ( Bardhi and Eckhardt, 2012 ). Schaefers et al. (2016b) investigated misbehavior among users of sharing services such as carsharing and identified a parallel with broken windows theory, in that one customer's misbehavior affects the next customer's behavior – in other words, misbehavior can be contagious. However, as the opposite is true in groups exhibiting high communal identification, it was considered more useful for service providers to strengthen their brand identity rather than investing in surveillance. Bardhi and Eckhardt (2012) conducted 40 interviews with Zipcar customers and found no sense of perceived ownership when using a car; in some cases, users preferred not to be associated with carsharing services. According to Schaefers et al. (2016b) , the contagious effects of customer misbehavior suggest that deanonymizing service providers and customers may help to reduce misbehavior. In an experiment on motivating car inspection, Namazu et al. (2018) found that in the absence of a reminder, most customers did not inspect the car before use. Other undesirable behaviors such as late car return are more likely among P2P users than in B2C carsharing because customers may believe it is easier to discuss misbehavior with an individual than with a company ( Namazu et al. , 2018 ).
3.5 Vehicle balancing
This aspect of one-way carsharing remains challenging for many providers of access-based services. In one-way carsharing, a short-term imbalance of cars often occurs at certain locations because of the uneven distribution of cars, that is, cars may be unavailable where they are needed while oversupplied at redundant locations. This is a likely consequence of flexible return times and locations, and the uneven flow of cars between stations means there may be too few cars at popular pick-up points ( Nair and Miller-Hooks, 2011 ). The issue of vehicle imbalance is especially challenging for B2C providers as this business model most commonly offers one-way carsharing. The lack of adequate data makes it more difficult to resolve this problem ( Ren et al. , 2020 ), and some studies have developed demand forecasting models to predict carsharing demand in pursuit of optimal business performance and customer satisfaction (e.g. Moein and Awasthi, 2020 ; Müller et al. , 2017 ). The next section discusses the challenge of selecting appropriate locations for carsharing stations and refers to studies that address the issue of vehicle relocation.
3.5.1 Station location
Appropriate station selection includes identification of lucrative locations ( Cheng et al. , 2019 ) and ease of relocating cars between stations to meet demand ( Boyaci et al. , 2015 ). In many cases, limited access means that some potential customers may be excluded, while those who can access the cars may not use them. Clark and Curl (2016) , who studied UK bicycle and carsharing schemes, noted the importance of the station location in efforts to target potential and willing customers.
Hua et al. (2019) proposed an innovative framework for the deployment of one-way EV carsharing. They emphasized that identification of suitable stations is even more important for EV carsharing fleets because of issues such as battery charging and fluctuating travel time and demand. Seeking to identify optimal charging locations for one-way EV carsharing, Deza et al. (2020) showed how this can maximize balanced flow in carsharing networks. Given the uncertainty of travel demand, simulation models have been used to aid identification of optimal charging station locations for EV fleets (e.g. Brandstätter et al. , 2020 ; Kuwahara et al. , 2020 ; Lu et al. , 2020 ) and to assist fleet allocation (e.g. Deveci et al. , 2018 ). In their investigation of the factors that affect turnover at carsharing stations, Hu et al. (2018) found that in addition to spatial and temporal elements, station-level turnover and the relationship between transit and carsharing affect the use of shared cars. In identifying appropriate locations for carsharing stations, carsharing providers are encouraged to optimize efficiency, especially in densely populated areas, where cars occupy a significant amount of expensive parking space ( Hu et al. , 2018 ). Sai et al. (2020) designed a model for EV carsharing and concluded that city population, proportion of available travel modes, station construction costs and budget should be considered when selecting station locations.
3.5.2 Vehicle relocation
Spatio-temporal imbalances in demand for one-way carsharing mean that cars must be constantly redistributed ( Balac et al. , 2019 ), relocating them periodically between stations to ensure availability where they are most needed ( Kim and Lee, 2017 ). Any imbalance in distribution makes carsharing less reliable ( Balac et al. , 2019 ) and creates complexity barriers (cf. Hazeé et al. , 2017 ) for service providers, who must relocate cars to wherever they are required. Vehicle balancing efforts lead to various trade-offs; for example, Nourinejad and Roorda (2014) evaluated one-way carsharing performance by measuring effectiveness and proposed an optimization model for operational problems involving vehicle relocation. In the proposed model, increased fleet size means reduced time for relocation but also leads to increased costs. As another alternative, the same study proposed that fleet size could be reduced by extending reservation time to about 30 min. However, this might also result in reduced demand, as user privacy could be compromised in some instances by having to specify a return time window to facilitate vehicle relocation forecasting ( Repoux et al. , 2019 ).
Over time, vehicle relocation models have been developed for operator-based, user-based and combined vehicle relocation. While operator-based models require staff members to move cars, user-based models rely on customers to do so ( Brendel et al. , 2018 ). Testing the impact of vehicle relocation on competition among carsharing operators in Switzerland, Balac et al. (2019) found that to substantially affect demand, more employees are needed to perform the required relocations. Additionally, as cars are unavailable to customers while being relocated, no revenue is earned, making relocation less profitable ( Balac et al. , 2019 ). These issues were further highlighted by Kypriadis et al. (2020) , who noted that operator-based relocation requires multiple employees to ensure timely relocation. To minimize redundancy, it is also important to coordinate user-based and operator-based relocation ( Brendel et al. , 2020a ). This view is echoed by Huang et al. (2020) , who note that user-based and operator-based relocation can be combined on the basis of pricing and the number of shifts, respectively, to correct any vehicle imbalance in one-way carsharing systems. In creating a decision support system for vehicle relocation, Kek et al. (2009) found that customers could be offered incentives in the form of reduced travel costs to encourage compliance with user-based relocation. Alternatively, this can be achieved by allowing customers to choose their own incentive, such as vehicle delivery, alternate pick-up location, alternate drop-off location or paid user relocation ( Wu et al. , 2020b ). Table 6 summarizes key findings related to vehicle relocation models.
Many existing vehicle balancing models are incremental improvements of earlier models. For example, Correia et al. (2014) discussed further options for customer pick-up and car drop-off, and Jorge et al. (2014) combined a mathematical model and a simulation model for optimal vehicle relocation and real-time relocation policy. Unlike simulation models that focus on one-way carsharing, Nourinejad and Roorda (2015) described a bi-modal scheme combining round-trip and one-way modes as one solution to the vehicle relocation problem in one-way carsharing services. Although convenient for customers, one-way services are costly for service providers because they require constant vehicle relocation. In contrast, round-trip systems are convenient for service providers because there is no vehicle relocation requirement, but this is less convenient for customers, who must return the car to its original pick-up point at the end of each trip ( Jorge et al. , 2015 ). With the increasing popularity of EV carsharing fleets, more recent models take account of new requirements such as unplugging and recharging (e.g. Boyaci et al. , 2015 ; Weikl and Bogenberger, 2015 ). For example, based on two optimization models, Caggiani et al. (2020) proposed a shared (vehicle-to-grid) charging system that can help to minimize losses during charging times, so maximizing profits.
4. Discussion and research agenda
Despite the growing number of studies on carsharing, service scholars have shown surprisingly little interest to date, and there are ample opportunities for further research. As our review indicates, transport and engineering studies predominate, advancing solutions to challenges like vehicle balancing. Based on our systematic review of the literature, we identified four themes as the basis for a service research agenda encompassing theory development and managerial practice. The proposed agenda addresses critical factors for the further growth of carsharing, including customer requirements, balancing supply and demand, and the effects of carsharing on car shedding. While ongoing experimentation continues to explore why certain provisions work better in some locations and markets than in others, a wide range of tools and methods is already available to address pressing issues such as the societal relevance of carsharing ventures and how best to ensure their success.
The research agenda addresses four key areas and associated questions, including key issues for managers and policymakers (see Table 7 ). While several of these topics are specific to carsharing services, we believe they also have broader ramifications for other mobility service issues, including multi-modal mobility and the rental/access paradigm in general ( Lovelock and Gummesson, 2004 ). As our review illustrates, there is considerable heterogeneity in terms of business models and service systems across the various forms of mobility service. Even in the case of similar value propositions such as B2C and P2P carsharing, there are fundamental differences between their respective access-based and platform-based models and systems. Our findings also provide relevant insights and research directions for other forms of nonownership, shared use of other goods, or other types of short-term access to goods (e.g. bike sharing).
Carsharing is part of the wider shift from products to services commonly referred to as servitization . Although servitization research is among the most active domains in the field of service research and attracts interest from multiple disciplines, it has focused almost exclusively on B2B markets ( Raddats et al. , 2019 ). In light of the rapid growth of servitization initiatives in consumer markets (e.g. subscription-as-a-service, sharing economy offerings), there is a need for a systematic overview and a roadmap for future research directions. In this context, it also seems timely to look at carsharing from a wider service management perspective as a market driven by technological and digital transformation.
4.1 Critical factors for the growth of carsharing
Despite the evidence that some carsharing service providers are struggling, as seen in the closures across different markets, little of the existing research has sought to develop a better understanding of the key challenges. The present study identifies four main directions for future research. First, it will be important to identify the conditions necessary for the growth of carsharing. Carsharing is known to scale with population density and social activity – for example, in metropolitan cities ( Clewlow, 2016 ) – and is also more prevalent in areas with lower car ownership and efficient public transport ( Becker et al. , 2017b ). However, a comparison of carsharing services and levels of substitution for other transport modes shows that carsharing usage levels remain low ( Rotaris et al. , 2019 ). To address this issue, researchers should explore the factors impeding the progress of carsharing and how these can be managed.
Second, while studies point to high population density as a requirement for successful operation of carsharing, the required critical mass of users remains an unresearched issue. Studies of access-based carsharing have shown that most businesses need to achieve a critical mass to break even ( Acheampong and Siiba, 2020 ; Lagadic et al. , 2019 ; Terama et al. , 2018 ). However, this is effectively a chicken-and-egg problem; while a critical mass of service users is required for scalability, users must be able to see scalability before being convinced to abandon their private cars in favor of carsharing. Furthermore, many members of carsharing schemes are not necessarily active customers, as many of those who register for these services use them only occasionally. As mentioned earlier, cost issues make this a more significant problem for B2C providers than for P2P owners and platform providers. Further research on the critical mass of users needed for different business models may enable service providers to solve the problem of maximum vehicle utilization.
Third, carsharing research should explore the different business models in greater depth to understand how they can be harnessed to promote carsharing, either as standalone models or in combination. As most research to date has focused on B2C carsharing, further research should place greater emphasis on P2P and B2B models. For example, as trust is an especially important issue for P2P carsharing, it would be useful to analyze how peer service providers can enhance profits by gaining their customers' trust ( Benoit et al. , 2017 ). This in turn should contribute to the identification of underlying success factors that can be generalized to various forms of access-based carsharing. In practice, many commercial providers offer parallel B2C and B2B services and may have to do so to achieve the requisite utilization rate. Researchers should seek to determine how synergies between business models can best be managed and developed.
Finally, as technology plays an important role in the growth of carsharing, future research should explore how recent and imminent technological advances in the automotive sector can be harnessed to drive innovation and enhance the customer experience of carsharing. For example, some providers have incorporated EVs into carsharing fleets as a means of promoting sustainability and optimizing operational costs ( Jacquillat and Zoepf, 2018 ). However, the challenges associated with EV fleets, including customers' inexperience and the need for a distinct service system, remain to be addressed. Advances in driving automation technology also afford opportunities to utilize customer data to provide better services for customers. Further research on these issues can help carsharing service providers to develop the competences they need to manage the industry's digital transformation.
4.2 Key customer requirements
Carsharing adoption has been attributed to the potential for exploiting the benefits of car ownership without bearing the costs ( Shaheen et al. , 1998 ). At present, the known prerequisites include collaboration and transparency between stakeholders, demand synchronization, solutions for a diverse group of customers that are accessible and easy to use and adopt, the potential to combine mobility of people and goods, and public information about the availability of mobility services ( Eckhardt et al. , 2019 ). However, service researchers have made only limited efforts to identify the key customer requirements for an optimal carsharing experience. On that basis, we can identify three key areas for future research.
First, while extensive research has focused on the customer, including customer behavior, drivers and barriers (especially in relation to access-based business models), few studies have addressed the motives of carsharing service providers. Different types of providers (e.g. pure service firms or automotive manufacturers) may have different reasons for venturing into carsharing, reflecting two broad and fundamentally distinct business models: car sales and leasing (which is managed primarily through third-party dealers) and carsharing. Future studies on service providers can also contribute to the development of balanced solutions based on better alignment of the service provider and customer requirements. Additionally, the perspective of car owners who rent their cars for P2P carsharing has been neglected by existing research ( Hazée et al. , 2020 ).
A second direction for further research relates to payment schemes. To promote maximum car utilization and customer satisfaction, some studies (e.g. Le Vine et al. , 2014 ; Molnar and de Almeida Correia, 2019 ) have proposed the introduction of reservation systems to eliminate the current first-come-first-served approach. However, while such systems might seem to address the challenge of maximum utilization, they may have an opposite effect on long-term customer satisfaction, as customers are more willing to pay for a guaranteed reservation than for virtual queuing ( Wu et al. , 2020a ). The different types of carsharing providers have experimented with various pricing and payment schemes, such as pay-per-use or P2P's dynamic pricing based on time and usage. Lovelock and Gummesson (2004) proposed that pricing access-based services should relate to units of time but also noted that pricing schemes may need to be modified according to circumstance. Dowling et al. 's (2020) analysis of how users choose between different payment plans showed that carsharing customers typically opt for pay-per-use favor flexibility but tend to underestimate their usage. In general, further research is warranted on perceived utility and willingness to pay, as this may yield solutions to issues of scalability and stakeholder goal alignment.
A final area of concern is that much of the existing research has focused on developed countries in Europe, North America and Asia (including China) while neglecting developing economies with lower purchasing power parity. These less developed countries (in Africa, Asia and Latin America) account for the greater part of the world's population and stand for essentially all world population growth. Many of these markets are undergoing rapid urbanization but often lack efficient public transport and suffer from traffic congestion and poor air quality. As Lovelock and Gummesson (2004) noted, people in developing countries often find ways of improving their prospects through innovative sharing of goods and services. While such practices may not be directly replicable, mobility solutions in these markets may provide valuable insights for improving overall quality of life. Future research should seek to identify the key requirements for progressing carsharing in different customer groups and how these can be implemented in markets with service systems or institutional arrangements that differ fundamentally from high-income economies.
4.3 Addressing the balance between supply and demand
This problem occurs mainly in schemes that operate one-way carsharing although it is also relevant to the challenges of vehicle balancing management in general. To date, research in this area has been largely confined to the fields of computer science, engineering and mathematics, focusing mainly on simulation models to assist vehicle balancing. We contend that service researchers can address the imbalance between vehicle supply and demand in three areas.
First, it is clear from the various trade-offs associated with different approaches to vehicle balancing that there is no quick fix for this problem in the context of one-way carsharing. Specifically, service providers must choose between increased costs, reducing the time spent relocating vehicles and reduced customer demand. Service researchers should examine ways of striking a better balance between fleet size/mix and fixed and variable costs by incorporating factors such as customer preferences and behavior.
Second, while a number of studies have advanced user-based vehicle relocation models or a combination of user-based and operator-based models as cost-saving alternatives for vehicle balancing, there is some skepticism about customers' willingness to participate in vehicle relocation, regardless of incentives offered ( Brendel et al. , 2020b ). The question of whether user-based relocation models offer a viable solution remains unanswered in the absence of empirical studies to test these claims. Further research should therefore include empirical studies of user-based vehicle relocation as an alternative or a complement to operator-based relocation, as well as the range of customer motivations and their effects on engagement, usage and loyalty.
Finally, despite growing research interest and ongoing development of new relocation models, some issues remain unresolved. For example, as some relocation models are context-specific and refer to parameters for the city in question, generalizability is limited (e.g. Boyaci et al. , 2015 ; Di Febbraro et al. , 2018 ). Nevertheless, any lessons learned from these context-specific models should be disseminated to enhance general understanding and to inform further research on vehicle balancing strategies. Additionally, the interdependence of supply and demand is a crucial issue for the design of carsharing schemes but is often neglected in vehicle relocation models ( Jian et al. , 2019 ). Finally, questions remain to be answered regarding the optimal inventory level at each carsharing station ( Laporte et al. , 2018 ).
4.4 The effect of carsharing on car shedding
One matter of longstanding debate is whether carsharing might reduce traffic congestion by encouraging car shedding. Dowling and Simpson (2013) predicted that people's relationship with their cars would change, and that young people in particular would increasingly favor carsharing. This resonates with industry forecasts of a cultural shift in which the car ceases to be an object of ownership and instead becomes part of a service or a network of vehicles for collective use. However, in a study examining the effects of free-floating carsharing on the purchase of new vehicles in cities, Schmidt (2020) found that the claim that one shared car eliminates roughly three car purchases annually does not apply to high status cars such as SUVs or larger vehicles like vans. Similarly, Li and Kamargianni's (2020) research on how carsharing affects private car ownership and public transport demand established that people who take long trips are less likely to give up their private car in favor of carsharing. As one may reasonably assume that P2P sharing is also a less attractive option in such cases, the debate around carsharing as a means of reducing car traffic remains unresolved.
Further research on this topic should address three issues. First, the conditions under which carsharing might encourage car shedding remain unclear, and further research is needed. Ikezoe et al. 's (2020) survey of car owners in Japan found that car owners tend to have an emotional attachment to their cars and would only abandon them if carsharing tapped into factors beyond economic rationality (such as convenience). To position access-based and platform-based carsharing services as an attractive alternative to car ownership, researchers should explore the nature and impact of these underlying factors.
A second research direction relates to the fact that customer willingness to forego car purchases after joining a carsharing scheme may be contingent on carsharing type ( Mishra et al. , 2015 ). For example, round-trip customers are more likely to abandon private ownership if they reduce that dependency by adopting other modes of mobility such as walking or biking. In contrast, one-way customers employ carsharing to replace modes such as taxi and ride hailing ( Lempert et al. , 2019 ). Further research should therefore strive for a deeper understanding of how the various carsharing models impact car shedding. Such insights would help to develop an understanding of service outcomes that can guide resource allocation in carsharing schemes.
Finally, more research is needed to specify the ideal conditions for the co-existence of carsharing and other transport modes such as public transport and biking, both as separate services and for multimodal mobility. Contrary to popular belief, carsharing alone may not suffice to encourage car shedding but is more likely to succeed if combined with other transport modes. In fact, access-based carsharing is often most successful when combined with other complementary transport options ( Csonka and Csiszár, 2016 ; Shaheen et al. , 1998 ). Future research should also explore how digital technology can be used to facilitate seamless multi-modal mobility services that align customer preferences and policy goals.
5. Conclusions and implications for practice
As evidenced by a growing number of publications over the last five years, carsharing has attracted significant attention from scholars, industry stakeholders and government organizations, reflecting ongoing challenges and the need for innovative mobility solutions. The aims of this literature review were to synthesize the extensive existing literature and to identify key areas for future research. Using a systematic approach, the review analyzed 279 studies published between 1996 and 2020, addressing issues that include existing business models, customer behavior, and user drivers and barriers, as well as the ongoing challenge of vehicle balancing. On that basis, we advanced a research agenda to address critical issues and to propose how existing challenges and opportunities can best be managed.
5.1 Managerial implications
The review identifies three main implications for carsharing service providers. First, managers need to understand the importance of building relationships with a wider range of stakeholders, especially when dealing with EV fleets. They must also understand the importance of customer and market factors; for example, while providers may be involved in the design of greenfield service systems from an early stage, initiatives in densely populated areas are different because parking space and charging infrastructure are scarce resources. Similarly, the requirements for P2P carsharing may differ significantly from B2C and B2B contexts. In every case, managers must understand customer needs, shape expectations and articulate potential benefits in terms of the appropriate service business model and ecosystem.
Second, managers must pursue innovations that will help to make carsharing attractive to the wider population; for example, Jian et al. (2020) suggested providing shared parking spaces for carsharing customers rather than simply providing carsharing services as is the current norm. This would enable carsharing service providers to make a profit by renting parking spaces from parking suppliers and then renting the same spaces to carsharing customers at a higher price. This would in turn deliver a higher utilization rate and a further increase in profits. Managers should also look to exploit ongoing technological advances to achieve synergies between business models, for instance, by leveraging customer data to reveal user patterns and so facilitate maximum utilization.
Finally, by targeting a broad base of customers through collaboration with other mobility providers (such as public transport), managers can identify key competences that can be utilized or synchronized to improve services. To that end, it will also be important to assess the potential market size in order to anticipate the impact of other mobility modes and to identify usage patterns that will optimize utilization rates and customer engagement.
5.2 Policy implications
For those making and implementing public policy, the present review has two main implications. First, given the potential of carsharing to resolve many pressing societal and environmental challenges such as traffic congestion and air pollution, such initiatives warrant the support of public and private stakeholders alike. However, policy interventions cannot simply support carsharing at the expense of public transport, nor can they enforce policies that simply make carsharing attractive to non-car owners; instead any such intervention must balance and direct provisions to maximize societal benefit. While service providers are often motivated by profit, regulatory bodies are driven by their duty to protect the interests of both users and providers ( Lindloff et al. , 2014 ) and must therefore base supports on assessments of local need and the common good. Rather than undermining support for public transport and funding, policy can make carsharing a more attractive alternative to car ownership through a combination of rewards and penalties, for example, lower taxes on revenues from P2P carsharing in combination with more restricted or more expensive public parking. Policies should not simply aim to make carsharing more attractive, as this may prove counterproductive by attracting non-car owners who would otherwise use public transport.
Secondly, to improve traffic conditions and pollution, more support should be provided for EV carsharing fleets, engaging actively with challenges such as the provision of optimized charging stations. In their study of the optimal use of EVs in carsharing, Abouee‐Mehrizi et al. (2021) identified three key factors: charging speed, charging station availability and battery range (in that order). Even if most carsharing users are not driving long distances, providers need to ensure that their fleets are charged – ideally, where they are parked. Automotive manufacturer Tesla owns and operates the largest global-charging network in the world (25,000+ Superchargers as of June 2021), but too much reliance on commercial actors may create brand-specific lock-in effects that inhibit growth and provision of the necessary infrastructure for local ventures. In short, public decision makers can play a more active role in facilitating the shift to electrification and shared mobility.
Literature search and selection process
Publications by topic and year
Most cited papers by theme
Main usage characteristics of carsharing customers
User-based and operator-based relocation models
Research agenda and managerial implications
Reviewed journals and research streams
Abouee-Mehrizi , H. , Baron , O. , Berman , O. and Chen , D. ( 2021 ), “ Adoption of electric vehicles in car sharing market ”, Production and Operations Management , Vol. 30 No. 1 , pp. 190 - 209 .
Acheampong , R.A. and Siiba , A. ( 2020 ), “ Modelling the determinants of car-sharing adoption intentions among young adults: the role of attitude, perceived benefits, travel expectations and socio-demographic factors ”, Transportation , Vol. 47 No. 5 , pp. 2557 - 2580 .
Alencar , V.A. , Rooke , F. , Cocca , M. , Vassio , L. , Almeida , J. and Vieira , A.B. ( 2019 ), “ Characterizing client usage patterns and service demand for car-sharing systems ”, Information Systems , Article in press , 101448 .
Alfian , G. , Rhee , J. , Kang , Y.S. and Yoon , B. ( 2015 ), “ Performance comparison of reservation based and instant access one-way car sharing service through discrete event simulation ”, Sustainability , Vol. 7 No. 9 , pp. 12465 - 12489 .
Ampudia-Renuncio , M. , Guirao , B. , Molina-Sánchez , R. and de Alvarez , C.E. ( 2020 ), “ Understanding the spatial distribution of free-floating carsharing in cities: analysis of the new Madrid experience through a web-based platform ”, Cities , Vol. 98 , p. 102593 .
Balac , M. , Ciari , F. and Axhausen , K.W. ( 2017 ), “ Modeling the impact of parking price policy on free-floating carsharing: case study for Zurich, Switzerland ”, Transportation Research Part C: Emerging Technologies , Vol. 77 , pp. 207 - 225 .
Balac , M. , Becker , H. , Ciari , F. and Axhausen , K.W. ( 2019 ), “ Modeling competing free-floating carsharing operators–A case study for Zurich, Switzerland ”, Transportation Research Part C: Emerging Technologies , Vol. 98 , pp. 101 - 117 .
Ballús-Armet , I. , Shaheen , S.A. , Clonts , K. and Weinzimmer , D. ( 2014 ), “ Peer-to-peer carsharing: exploring public perception and market characteristics in the San Francisco Bay area, California ”, Transportation Research Record , Vol. 2416 No. 1 , pp. 27 - 36 .
Barbour , N. , Zhang , Y. and Mannering , F. ( 2020 ), “ Individuals' willingness to rent their personal vehicle to others: an exploratory assessment of peer-to-peer carsharing ”, Transportation Research Interdisciplinary Perspectives , Vol. 5 , p. 100138 .
Bardhi , F. and Eckhardt , G.M. ( 2012 ), “ Access-based consumption: the case of car sharing ”, Journal of Consumer Research , Vol. 39 No. 4 , pp. 881 - 898 .
Barnes , S.J. and Mattsson , J. ( 2016 ), “ Understanding current and future issues in collaborative consumption: a four-stage Delphi study ”, Technological Forecasting and Social Change , Vol. 104 , pp. 200 - 211 .
Becker , H. , Ciari , F. and Axhausen , K.W. ( 2017a ), “ Comparing car-sharing schemes in Switzerland: user groups and usage patterns ”, Transportation Research Part A: Policy and Practice , Vol. 97 , pp. 17 - 29 .
Becker , H. , Ciari , F. and Axhausen , K.W. ( 2017b ), “ Modeling free-floating car-sharing use in Switzerland: a spatial regression and conditional logit approach ”, Transportation Research Part C: Emerging Technologies , Vol. 81 , pp. 286 - 299 .
Becker , H. , Ciari , F. and Axhausen , K.W. ( 2018 ), “ Measuring the car ownership impact of free-floating car-sharing–A case study in Basel, Switzerland ”, Transportation Research Part D: Transport and Environment , Vol. 65 , pp. 51 - 62 .
Bellos , I. , Ferguson , M. and Toktay , L.B. ( 2017 ), “ The car sharing economy: interaction of business model choice and product line design ”, Manufacturing and Service Operations Management , Vol. 19 No. 2 , pp. 185 - 201 .
Benoit , S. , Baker , T.L. , Bolton , R.N. , Gruber , T. and Kandampully , J. ( 2017 ), “ A triadic framework for collaborative consumption (CC): motives, activities and resources and capabilities of actors ”, Journal of Business Research , Vol. 79 , pp. 219 - 227 .
Bocken , N. , Jonca , A. , Södergren , K. and Palm , J. ( 2020 ), “ Emergence of carsharing business models and sustainability impacts in Swedish cities ”, Sustainability , Vol. 12 No. 4 , p. 1594 .
Boell , S.K. and Cecez-Kecmanovic , D. ( 2015 ), “ On being ‘systematic’ in literature reviews ”, Journal of Information Technology , Vol. 30 , pp. 161 - 173 .
Boldrini , C. , Bruno , R. and Laarabi , M.H. ( 2019 ), “ Weak signals in the mobility landscape: car sharing in ten European cities ”, EPJ Data Science , Vol. 8 No. 1 , p. 7 .
Bonsall , P. , Jopson , A. , Pridmore , A. , Ryan , A. and Firmin , P. ( 2002 ), “ Car share and car clubs: potential impacts ”, Department of Transport (Great Britain), Local Government and the Regions and the Motorists Forum , London .
Boyaci , B. , Zografos , K.G. and Geroliminis , N. ( 2015 ), “ An optimization framework for the development of efficient one-way car-sharing systems ”, European Journal of Operational Research , Vol. 240 No. 3 , pp. 718 - 733 .
Brandstätter , G. , Leitner , M. and Ljubić , I. ( 2020 ), “ Location of charging stations in electric car sharing systems ”, Transportation Science , Vol. 54 No. 5 , pp. 1408 - 1438 .
Brendel , A.B. , Lichtenberg , S. , Brauer , B. , Nastjuk , I. and Kolbe , L.M. ( 2018 ), “ Improving electric vehicle utilization in carsharing: a framework and simulation of an e-carsharing vehicle utilization management system ”, Transportation Research Part D: Transport and Environment , Vol. 64 , pp. 230 - 245 .
Brendel , A.B. , Brennecke , J.T. , Hillmann , B.M. and Kolbe , L.M. ( 2020a ), “ The design of a decision support system for computation of carsharing pricing areas and its influence on vehicle distribution ”, IEEE Transactions on Engineering Management , Article in press , pp. 1 - 15 .
Brendel , A.B. , Lichtenberg , S. , Prinz , C. and Herrenkind , B. ( 2020b ), “ Increasing the value of shared vehicles: insights from an implementation of user-based relocation in station-based one-way carsharing ”, Sustainability , Vol. 12 No. 21 , p. 8800 .
Bruglieri , M. , Colorni , A. and Luè , A. ( 2014 ), “ The relocation problem for the one‐way electric vehicle sharing ”, Networks , Vol. 64 No. 4 , pp. 292 - 305 .
Burlando , C. , Ivaldi , E. , Saiani , P.P. and Penco , L. ( 2019 ), “ To own or not to own? Car ownership and consumer awareness: evidence from an Italian survey ”, Research in Transportation Business and Management , Vol. 33 , p. 100435 .
Caggiani , L. , Prencipe , L.P. and Ottomanelli , M. ( 2020 ), “ A static relocation strategy for electric car-sharing systems in a vehicle-to-grid framework ”, Transportation Letters , Article in press , pp. 1 - 10 .
Carrone , A.P. , Hoening , V.M. , Jensen , A.F. , Mabit , S.E. and Rich , J. ( 2020 ), “ Understanding car sharing preferences and mode substitution patterns: a stated preference experiment ”, Transport Policy , Vol. 98 , pp. 139 - 147 .
Cartenì , A. , Cascetta , E. and de Luca , S. ( 2016 ), “ A random utility model for park and carsharing services and the pure preference for electric vehicles ”, Transport Policy , Vol. 48 , pp. 49 - 59 .
Charoniti , E. , Kim , J. , Rasouli , S. and Timmermans , H.J. ( 2020 ), “ Intrapersonal heterogeneity in car-sharing decision-making processes by activity-travel contexts: a context-dependent latent class random utility–random regret model ”, International Journal of Sustainable Transportation , Article in press , pp. 1 - 11 .
Cheng , Y. , Chen , X. , Ding , X. and Zeng , L. ( 2019 ), “ Optimizing location of car-sharing stations based on potential travel demand and present operation characteristics: the case of chengdu ”, Journal of Advanced Transportation , Vol. 2019 , pp. 1 - 13 .
Chun , Y.-Y. , Matsumoto , M. , Tahara , K. , Chinen , K. and Endo , H. ( 2019 ), “ Exploring factors affecting car sharing use intention in the Southeast-Asia region: a case study in Java, Indonesia ”, Sustainability , Vol. 11 No. 18 , p. 5103 .
Clark , J. and Curl , A. ( 2016 ), “ Bicycle and car share schemes as inclusive modes of travel? A socio-spatial Analysis in Glasgow, UK ”, Social Inclusion , Vol. 4 No. 3 , pp. 83 - 99 .
Clark , M. , Gifford , K. , Anable , J. and Le Vine , S. ( 2015 ), “ Business-to-business carsharing: evidence from Britain of factors associated with employer-based carsharing membership and its impacts ”, Transportation , Vol. 42 No. 3 , pp. 471 - 495 .
Clewlow , R.R. ( 2016 ), “ Carsharing and sustainable travel behavior: results from the san Francisco Bay area ”, Transport Policy , Vol. 51 , pp. 158 - 164 .
Cohen , B. and Kietzmann , J. ( 2014 ), “ Ride on! Mobility business models for the sharing economy ”, Organization and Environment , Vol. 27 No. 3 , pp. 279 - 296 .
Cohen , A.P. , Shaheen , S. and McKenzie , R. ( 2008 ), Carsharing: A Guide for Local Planners , American Planning Association , California .
Coll , M.H. , Vandersmissen , M.H. and Thériault , M. ( 2014 ), “ Modeling spatio-temporal diffusion of carsharing membership in Québec City ”, Journal of Transport Geography , Vol. 38 , pp. 22 - 37 .
Correia , G.H.D.A. and Antunes , A.P. ( 2012 ), “ Optimization approach to depot location and trip selection in one-way carsharing systems ”, Transportation Research Part E: Logistics and Transportation Review , Vol. 48 No. 1 , pp. 233 - 247 .
Correia , G.H.D.A. , Jorge , D.R. and Antunes , D.M. ( 2014 ), “ The added value of accounting for users' flexibility and information on the potential of a station-based one-way car-sharing system: an application in Lisbon, Portugal ”, Journal of Intelligent Transportation Systems , Vol. 18 No. 3 , pp. 299 - 308 .
Costain , C. , Ardron , C. and Habib , K.N. ( 2012 ), “ Synopsis of users' behavior of a carsharing program: a case study in Toronto ”, Transportation Research Part A: Policy and Practice , Vol. 46 No. 3 , pp. 421 - 434 .
Cronin , P. , Ryan , F. and Coughlan , M. ( 2008 ), “ Undertaking a literature review: a step-by-step approach ”, British Journal of Nursing , Vol. 17 No. 1 , pp. 38 - 43 .
Csonka , B. and Csiszár , C. ( 2016 ), “ Service quality analysis and assessment method for European carsharing systems ”, Periodica Polytechnica Transportation Engineering , Vol. 44 No. 2 , pp. 80 - 88 .
de Lorimier , A. and El-Geneidy , A.M. ( 2013 ), “ Understanding the factors affecting vehicle usage and availability in carsharing networks: a case study of Communauto carsharing system from Montréal, Canada ”, International Journal of Sustainable Transportation , Vol. 7 No. 1 , pp. 35 - 51 .
De Luca , S. and Di Pace , R. ( 2015 ), “ Modelling users' behavior in inter-urban carsharing program: a stated preference approach ”, Transportation Research Part A: Policy and Practice , Vol. 71 , pp. 59 - 76 .
del Mar Alonso-Almeida , M. ( 2019 ), “ Carsharing: another gender issue? Drivers of carsharing usage among women and relationship to perceived value ”, Travel Behaviour and Society , Vol. 17 , pp. 36 - 45 .
Deveci , M. , Canıtez , F. and Gökaşar , I. ( 2018 ), “ WASPAS and TOPSIS based interval type-2 fuzzy MCDM method for a selection of a car sharing station ”, Sustainable Cities and Society , Vol. 41 , pp. 777 - 791 .
Deza , A. , Huang , K. and Metel , M.R. ( 2020 ), “ Charging station optimization for balanced electric car sharing ”, Discrete Applied Mathematics , Article in press .
Diana , M. and Ceccato , R. ( 2019 ), “ A multimodal perspective in the study of car sharing switching intentions ”, Transportation Letters , Article in press , pp. 1 - 7 .
Di Febbraro , A. , Sacco , N. and Saeednia , M. ( 2018 ), “ One-way car-sharing profit maximization by means of user-based vehicle relocation ”, IEEE Transactions on Intelligent Transportation Systems , Vol. 20 No. 2 , pp. 628 - 641 .
Dijk , M. , Orsato , R.J. and Kemp , R. ( 2013 ), “ The emergence of an electric mobility trajectory ”, Energy Policy , Vol. 52 , pp. 135 - 145 .
Dill , J. , McNeil , N. and Howland , S. ( 2019 ), “ Effects of peer-to-peer carsharing on vehicle owners' travel behavior ”, Transportation Research Part C: Emerging Technologies , Vol. 101 , pp. 70 - 78 .
Dowling , R. and Simpson , C. ( 2013 ), “ Shift-the way you move: reconstituting automobility ”, Continuum , Vol. 27 No. 3 , pp. 421 - 433 .
Dowling , K. , Manchanda , P. and Spann , M. ( 2020 ), “ The existence and persistence of the pay-per-use bias in car sharing services ”, International Journal of Research in Marketing , Article in press .
Duan , Q. , Ye , X. , Li , J. and Wang , K. ( 2020 ), “ Empirical modeling analysis of potential commute demand for carsharing in shanghai, China ”, Sustainability , Vol. 12 No. 2 , p. 620 .
Duncan , M. ( 2011 ), “ The cost saving potential of carsharing in a US context ”, Transportation , Vol. 38 No. 2 , pp. 363 - 382 .
Eckhardt , G.M. , Houston , M.B. , Jiang , B. , Lamberton , C. , Rindfleisch , A. and Zervas , G. ( 2019 ), “ Marketing in the sharing economy ”, Journal of Marketing , Vol. 83 No. 5 , pp. 5 - 27 .
Efthymiou , D. , Antoniou , C. and Waddell , P. ( 2013 ), “ Factors affecting the adoption of vehicle sharing systems by young drivers ”, Transport Policy , Vol. 29 , pp. 64 - 73 .
Fellows , N.T. and Pitfield , D.E. ( 2000 ), “ An economic and operational evaluation of urban car-sharing ”, Transportation Research Part D: Transport and Environment , Vol. 5 No. 1 , pp. 1 - 10 .
Ferrero , F. , Perboli , G. , Rosano , M. and Vesco , A. ( 2018 ), “ Car-sharing services: an annotated review ”, Sustainable Cities and Society , Vol. 37 , pp. 501 - 518 .
Firnkorn , J. and Müller , M. ( 2012 ), “ Selling mobility instead of cars: new business strategies of automakers and the impact on private vehicle holding ”, Business Strategy and the Environment , Vol. 21 No. 4 , pp. 264 - 280 .
Firnkorn , J. and Müller , M. ( 2015 ), “ Free-floating electric carsharing-fleets in smart cities: the dawning of a post-private car era in urban environments? ”, Environmental Science and Policy , Vol. 45 , pp. 30 - 40 .
Fleury , S. , Tom , A. , Jamet , E. and Colas-Maheux , E. ( 2017 ), “ What drives corporate carsharing acceptance? A French case study ”, Transportation Research Part F: Traffic Psychology and Behaviour , Vol. 45 , pp. 218 - 227 .
Fritze , M.P. , Marchand , A. , Eisingerich , A.B. and Benkenstein , M. ( 2020 ), “ Access-based services as substitutes for material possessions: the role of psychological ownership ”, Journal of Service Research , Vol. 23 No. 3 , pp. 368 - 385 .
Giorgione , G. , Ciari , F. and Viti , F. ( 2020 ), “ Dynamic pricing on round-trip carsharing services: travel behavior and equity impact analysis through an agent-based simulation ”, Sustainability , Vol. 12 No. 17 , p. 6727 .
Global Market Insights, Inc. ( 2021 ), “ Car sharing market statistics - global growth trends 2026 ”, available at: https://www.gminsights.com/industry-analysis/carsharing-market ( accessed 13 June 2021 ).
Guglielmetti Mugion , R. , Toni , M. , Di Pietro , L. , Pasca , M. and Renzi , M. ( 2019 ), “ Understanding the antecedents of car sharing usage: an empirical study in Italy ”, International Journal of Quality and Service Sciences , Vol. 11 No. 4 , pp. 523 - 541 .
Guyader , H. ( 2018 ), “ No one rides for free! Three styles of collaborative consumption ”, Journal of Services Marketing , Vol. 32 No. 6 , pp. 692 - 714 .
Hahn , R. , Ostertag , F. , Lehr , A. , Büttgen , M. and Benoit , S. ( 2020 ), “ ‘I like it, but I don't use it’: impact of carsharing business models on usage intentions in the sharing economy ”, Business Strategy and the Environment , Vol. 29 No. 3 , pp. 1404 - 1418 .
Hamari , J. , Sjöklint , M. and Ukkonen , A. ( 2016 ), “ The sharing economy: why people participate in collaborative consumption ”, Journal of the Association for Information Science and Technology , Vol. 67 No. 9 , pp. 2047 - 2059 .
Hampshire , R.C. and Gaites , C. ( 2011 ), “ Peer-to-peer carsharing: market analysis and potential growth ”, Transportation Research Record , Vol. 2217 No. 1 , pp. 119 - 126 .
Hartl , B. , Sabitzer , T. , Hofmann , E. and Penz , E. ( 2018 ), “ ‘Sustainability is a nice bonus’ the role of sustainability in carsharing from a consumer perspective ”, Journal of Cleaner Production , Vol. 202 , pp. 88 - 100 .
Hazée , S. , Delcourt , C. and Van Vaerenbergh , Y. ( 2017 ), “ Burdens of access: understanding customer barriers and barrier-attenuating practices in access-based services ”, Journal of Service Research , Vol. 20 No. 4 , pp. 441 - 456 .
Hazée , S. , Zwienenberg , T.J. , Van Vaerenbergh , Y. , Faseur , T. , Vandenberghe , A. and Keutgens , O. ( 2020 ), “ Why customers and peer service providers do not participate in collaborative consumption ”, Journal of Service Management , Vol. 31 , pp. 397 - 419 .
Heilig , M. , Mallig , N. , Schröder , O. , Kagerbauer , M. and Vortisch , P. ( 2018 ), “ Implementation of free-floating and station-based carsharing in an agent-based travel demand model ”, Travel Behaviour and Society , Hong Kong Society for Transportation Studies , Vol. 12 , pp. 151 - 158 .
Hjorteset , M.A. and Böcker , L. ( 2020 ), “ Car sharing in Norwegian urban areas: examining interest, intention and the decision to enrol ”, Transportation Research Part D: Transport and Environment , Vol. 84 , p. 102322 .
Hofmann , E. , Hartl , B. and Penz , E. ( 2017 ), “ Power versus trust – what matters more in collaborative consumption? ”, Journal of Services Marketing , Vol. 31 No. 6 , pp. 589 - 603 .
Hu , S. , Chen , P. , Lin , H. , Xie , C. and Chen , X. ( 2018 ), “ Promoting carsharing attractiveness and efficiency: an exploratory analysis ”, Transportation Research Part D: Transport and Environment , Vol. 65 , pp. 229 - 243 .
Hua , Y. , Zhao , D. , Wang , X. and Li , X. ( 2019 ), “ Joint infrastructure planning and fleet management for one-way electric car sharing under time-varying uncertain demand ”, Transportation Research Part B: Methodological , Vol. 128 , pp. 185 - 206 .
Huang , K. , An , K. and de Almeida Correia , G.H. ( 2020 ), “ Planning station capacity and fleet size of one-way electric carsharing systems with continuous state of charge functions ”, European Journal of Operational Research , Vol. 287 No. 3 , pp. 1075 - 1091 .
Ikezoe , K. , Kiriyama , E. and Fujimura , S. ( 2020 ), “ Car-sharing intention analysis in Japan by comparing the utility of car ownership for car-owners and non-car owners ”, Transport Policy , Vol. 96 , pp. 1 - 14 .
Jacquillat , A. and Zoepf , S. ( 2018 ), “ Deployment and utilization of plug-in electric vehicles in round-trip carsharing systems ”, International Journal of Sustainable Transportation , Vol. 12 No. 2 , pp. 75 - 91 .
Jian , S. , Hossein Rashidi , T. , Wijayaratna , K.P. and Dixit , V.V. ( 2016 ), “ A Spatial Hazard-Based analysis for modelling vehicle selection in station-based carsharing systems ”, Transportation Research Part C: Emerging Technologies , Vol. 72 , pp. 130 - 142 .
Jian , S. , Rashidi , T.H. and Dixit , V. ( 2017 ), “ An analysis of carsharing vehicle choice and utilization patterns using multiple discrete-continuous extreme value (MDCEV) models ”, Transportation Research Part A: Policy and Practice , Vol. 103 , pp. 362 - 376 .
Jian , S. , Rey , D. and Dixit , V. ( 2019 ), “ An integrated supply-demand approach to solving optimal relocations in station-based carsharing systems ”, Networks and Spatial Economics , Networks and Spatial Economics , Vol. 19 No. 2 , pp. 611 - 632 .
Jian , S. , Liu , W. , Wang , X. , Yang , H. and Waller , S.T. ( 2020 ), “ On integrating carsharing and parking sharing services ”, Transportation Research Part B: Methodological , Vol. 142 , pp. 19 - 44 .
Jochem , P. , Frankenhauser , D. , Ewald , L. , Ensslen , A. and Fromm , H. ( 2020 ), “ Does free-floating carsharing reduce private vehicle ownership? The case of SHARE NOW in European cities ”, Transportation Research Part A: Policy and Practice , Vol. 141 , pp. 373 - 395 .
Jorge , D. and Correia , G. ( 2013 ), “ Carsharing systems demand estimation and defined operations: a literature review ”, European Journal of Transport and Infrastructure Research , Vol. 13 No. 3 , pp. 201 - 220 .
Jorge , D. , Correia , G.H.A. and Barnhart , C. ( 2014 ), “ Comparing optimal relocation operations with simulated relocation policies in one-way carsharing systems ”, IEEE Transactions on Intelligent Transportation Systems , Vol. 15 No. 4 , pp. 1667 - 1675 .
Jorge , D. , Barnhart , C. and de Almeida Correia , G.H. ( 2015 ), “ Assessing the viability of enabling a round-trip carsharing system to accept one-way trips: application to Logan Airport in Boston ”, Transportation Research Part C: Emerging Technologies , Vol. 56 , pp. 359 - 372 .
Julsrud , T.E. and Farstad , E. ( 2020 ), “ Car sharing and transformations in households travel patterns: insights from emerging proto-practices in Norway ”, Energy Research and Social Science , Vol. 66 , p. 101497 .
Juschten , M. , Ohnmacht , T. , Thao , V.T. , Gerike , R. and Hössinger , R. ( 2019 ), “ Carsharing in Switzerland: identifying new markets by predicting membership based on data on supply and demand ”, Transportation , Vol. 46 No. 4 , pp. 1171 - 1194 .
Kang , J. , Hwang , K. and Park , S. ( 2016 ), “ Finding factors that influence carsharing usage: case study in seoul ”, Sustainability , Vol. 8 No. 8 , p. 709 .
Kek , A.G.H. , Cheu , R.L. , Meng , Q. and Fung , C.H. ( 2009 ), “ A decision support system for vehicle relocation operations in carsharing systems ”, Transportation Research Part E: Logistics and Transportation Review , Vol. 45 No. 1 , pp. 149 - 158 .
Kent , J. , Dowling , R. and Maalsen , S. ( 2017 ), “ Catalysts for transport transitions: bridging the gap between disruptions and change ”, Journal of Transport Geography , Vol. 60 , pp. 200 - 207 .
Kim , K.H. and Lee , Y.H. ( 2017 ), “ Vehicle-relocation optimization for one-way carsharing ”, International Journal of Industrial Engineering , Vol. 24 No. 5 , pp. 468 - 482 .
Kim , J. , Rasouli , S. and Timmermans , H.J.P. ( 2017a ), “ The effects of activity-travel context and individual attitudes on car-sharing decisions under travel time uncertainty: a hybrid choice modeling approach ”, Transportation Research Part D: Transport and Environment , Vol. 56 , pp. 189 - 202 .
Kim , J. , Rasouli , S. and Timmermans , H.J.P. ( 2017b ), “ Satisfaction and uncertainty in car-sharing decisions: an integration of hybrid choice and random regret-based models ”, Transportation Research Part A: Policy and Practice , Vol. 95 , pp. 13 - 33 .
Kim , D. , Park , Y. and Ko , J. ( 2019 ), “ Factors underlying vehicle ownership reduction among carsharing users: a repeated cross-sectional analysis ”, Transportation Research Part D: Transport and Environment , Vol. 76 , pp. 123 - 137 .
Ko , J. , Ki , H. and Lee , S. ( 2019 ), “ Factors affecting carsharing program participants' car ownership changes ”, Transportation Letters , Vol. 11 No. 4 , pp. 208 - 218 .
Kuwahara , M. , Yoshioka , A. and Uno , N. ( 2020 ), “ Practical searching optimal one-way carsharing stations to Be equipped with additional chargers for preventing opportunity loss caused by low SoC ”, International Journal of Intelligent Transportation Systems Research , pp. 1 - 10 .
Kypriadis , D. , Pantziou , G. , Konstantopoulos , C. and Gavalas , D. ( 2020 ), “ Optimizing relocation cost in free-floating car-sharing systems ”, IEEE Transactions on Intelligent Transportation Systems , Vol. 21 No. 9 , pp. 4017 - 4030 .
Lagadic , M. , Verloes , A. and Louvet , N. ( 2019 ), “ Can carsharing services be profitable? A critical review of established and developing business models ”, Transport Policy , Vol. 77 , pp. 68 - 78 .
Laporte , G. , Meunier , F. and Wolfler Calvo , R. ( 2018 ), “ Shared mobility systems: an updated survey ”, Annals of Operations Research , Vol. 271 No. 1 , pp. 105 - 126 .
Le Vine , S. and Polak , J. ( 2019 ), “ The impact of free-floating carsharing on car ownership: early-stage findings from London ”, Transport Policy , Vol. 75 , pp. 119 - 127 .
Le Vine , S. , Lee-Gosselin , M. , Sivakumar , A. and Polak , J. ( 2014 ), “ A new approach to predict the market and impacts of round-trip and point-to-point carsharing systems: case study of London ”, Transportation Research Part D: Transport and Environment , Vol. 32 , pp. 218 - 229 .
Lempert , R. , Zhao , J. and Dowlatabadi , H. ( 2019 ), “ Convenience, savings, or lifestyle? Distinct motivations and travel patterns of one-way and two-way carsharing members in Vancouver, Canada ”, Transportation Research Part D: Transport and Environment , Vol. 71 , pp. 141 - 152 .
Li , W. and Kamargianni , M. ( 2020 ), “ Steering short-term demand for car-sharing: a mode choice and policy impact analysis by trip distance ”, Transportation , Vol. 47 No. 5 , pp. 2233 - 2265 .
Lindloff , K. , Pieper , N. , Bandelow , N.C. and Woisetschläger , D.M. ( 2014 ), “ Drivers of carsharing diffusion in Germany: an actor-centred approach ”, International Journal of Automotive Technology and Management , Vol. 14 Nos 3-4 , pp. 217 - 245 .
Loose , W. , Mohr , M. and Nobis , C. ( 2006 ), “ Assessment of the future development of car sharing in Germany and related opportunities ”, Transport Reviews , Vol. 26 No. 3 , pp. 365 - 382 .
Lovelock , C. and Gummesson , E. ( 2004 ), “ Whither services marketing? In search of a new paradigm and fresh perspectives ”, Journal of Service Research , Vol. 7 No. 1 , pp. 20 - 41 .
Lu , X. , Zhang , Q. , Peng , Z. , Shao , Z. , Song , H. and Wang , W. ( 2020 ), “ Charging and relocating optimization for electric vehicle car-sharing: an event-based strategy improvement approach ”, Energy , Vol. 207 , p. 118285 .
Lyngdoh , T. , Chefor , E. , Hochstein , B. , Britton , B.P. and Amyx , D. ( 2021 ), “ A systematic literature review of negative psychological states and behaviors in sales ”, Journal of Business Research , Vol. 122 , pp. 518 - 533 .
Ma , F. , Guo , D. , Yuen , K.F. , Sun , Q. , Ren , F. , Xu , X. and Zhao , C. ( 2020 ), “ The influence of continuous improvement of public car-sharing platforms on passenger loyalty: a mediation and moderation analysis ”, International Journal of Environmental Research and Public Health , Vol. 17 No. 8 , p. 2756 .
Meelen , T. , Frenken , K. and Hobrink , S. ( 2019 ), “ Weak spots for car-sharing in The Netherlands? The geography of socio-technical regimes and the adoption of niche innovations ”, Energy Research and Social Science , Vol. 52 , pp. 132 - 143 .
Miller , J. ( 2019 ), “ German automakers do U-turn on car-sharing push ”, Financial Times .
Mishra , G.S. , Clewlow , R.R. , Mokhtarian , P.L. and Widaman , K.F. ( 2015 ), “ The effect of carsharing on vehicle holdings and travel behavior: a propensity score and causal mediation analysis of the San Francisco Bay Area ”, Research in Transportation Economics , Vol. 52 , pp. 46 - 55 .
Möhlmann , M. ( 2015 ), “ Collaborative consumption: determinants of satisfaction and the likelihood of using a sharing economy option again ”, Journal of Consumer Behaviour , Vol. 14 No. 3 , pp. 193 - 207 .
Moein , E. and Awasthi , A. ( 2020 ), “ Carsharing customer demand forecasting using causal, time series and neural network methods: a case study ”, International Journal of Services and Operations Management , Vol. 35 No. 1 , pp. 36 - 57 .
Moeller , S. and Wittkowski , K. ( 2010 ), “ The burdens of ownership: reasons for preferring renting ”, Managing Service Quality: An International Journal , Vol. 20 No. 2 , pp. 176 - 191 .
Molnar , G. and de Almeida Correia , G.H. ( 2019 ), “ Long-term vehicle reservations in one-way free-floating carsharing systems: a variable quality of service model ”, Transportation Research Part C: Emerging Technologies , Vol. 98 , pp. 298 - 322 .
Müller , J. , Correia , G.H.D.A. and Bogenberger , K. ( 2017 ), “ An explanatory model approach for the spatial distribution of free-floating carsharing bookings: a case-study of German cities ”, Sustainability , Vol. 9 No. 7 , p. 1290 .
Münzel , K. , Boon , W. , Frenken , K. and Vaskelainen , T. ( 2018 ), “ Carsharing business models in Germany: characteristics, success and future prospects ”, Information Systems and E-Business Management , Vol. 16 No. 2 , pp. 271 - 291 .
Münzel , K. , Piscicelli , L. , Boon , W. and Frenken , K. ( 2019 ), “ Different business models–different users? Uncovering the motives and characteristics of business-to-consumer and peer-to-peer carsharing adopters in The Netherlands ”, Transportation Research Part D: Transport and Environment , Vol. 73 , pp. 276 - 306 .
Münzel , K. , Boon , W. , Frenken , K. , Blomme , J. and van der Linden , D. ( 2020 ), “ Explaining carsharing supply across Western European cities ”, International Journal of Sustainable Transportation , Vol. 14 No. 4 , pp. 243 - 254 .
Nair , R. and Miller-Hooks , E. ( 2011 ), “ Fleet management for vehicle sharing operations ”, Transportation Science , Vol. 45 No. 4 , pp. 524 - 540 .
Namazu , M. , Zhao , J. and Dowlatabadi , H. ( 2018 ), “ Nudging for responsible carsharing: using behavioral economics to change transportation behavior ”, Transportation , Vol. 45 No. 1 , pp. 105 - 119 .
Nitschke , L. ( 2020 ), “ Reconstituting automobility: the influence of non-commercial carsharing on the meanings of automobility and the car ”, Sustainability , Vol. 12 No. 17 , p. 7062 .
Nourinejad , M. and Roorda , M.J. ( 2014 ), “ A dynamic carsharing decision support system ”, Transportation Research Part E: Logistics and Transportation Review , Vol. 66 , pp. 36 - 50 .
Nourinejad , M. and Roorda , M.J. ( 2015 ), “ Carsharing operations policies: a comparison between one-way and two-way systems ”, Transportation , Vol. 42 No. 3 , pp. 497 - 518 .
Nourinejad , M. , Zhu , S. , Bahrami , S. and Roorda , M.J. ( 2015 ), “ Vehicle relocation and staff rebalancing in one-way carsharing systems ”, Transportation Research Part E: Logistics and Transportation Review , Vol. 81 , pp. 98 - 113 .
Orski , K. ( 2001 ), “ Car sharing ”, Transport Quaterly , Vol. 55 No. 4 , pp. 13 - 15 .
Palmatier , R.W. , Houston , M.B. and Hulland , J. ( 2018 ), “ Review articles: purpose, process, and structure ”, Journal of the Academy of Marketing Science , Vol. 46 No. 1 , pp. 1 - 5 .
Paundra , J. , Rook , L. , van Dalen , J. and Ketter , W. ( 2017 ), “ Preferences for car sharing services: effects of instrumental attributes and psychological ownership ”, Journal of Environmental Psychology , Vol. 53 , pp. 121 - 130 .
Perboli , G. , Ferrero , F. , Musso , S. and Vesco , A. ( 2018 ), “ Business models and tariff simulation in car-sharing services ”, Transportation Research Part A: Policy and Practice , Vol. 115 , pp. 32 - 48 .
Peterson , M. and Simkins , T. ( 2019 ), “ Consumers' processing of mindful commercial car sharing ”, Business Strategy and the Environment , Vol. 28 No. 3 , pp. 457 - 465 .
Prieto , M. , Baltas , G. and Stan , V. ( 2017 ), “ Car sharing adoption intention in urban areas: what are the key sociodemographic drivers? ”, Transportation Research Part A: Policy and Practice , Vol. 101 , pp. 218 - 227 .
Raddats , C. , Kowalkowski , C. , Benedettini , O. , Burton , J. and Gebauer , H. ( 2019 ), “ Servitization: a contemporary thematic review of four major research streams ”, Industrial Marketing Management , Vol. 83 , pp. 207 - 223 .
Ren , C. , An , L. , Gu , Z. , Wang , Y. and Gao , Y. ( 2020 ), “ Rebalancing the car-sharing system with reinforcement learning ”, World Wide Web , Vol. 23 No. 4 , pp. 2491 - 2511 .
Repoux , M. , Kaspi , M. , Boyacı , B. and Geroliminis , N. ( 2019 ), “ Dynamic prediction-based relocation policies in one-way station-based carsharing systems with complete journey reservations ”, Transportation Research Part B: Methodological , Vol. 130 , pp. 82 - 104 .
Rotaris , L. , Danielis , R. and Maltese , I. ( 2019 ), “ Carsharing use by college students: the case of Milan and Rome ”, Transportation Research Part A: Policy and Practice , Vol. 120 , pp. 239 - 251 .
Ruhrort , L. , Steiner , J. , Graff , A. , Hinkeldein , D. and Hoffmann , C. ( 2014 ), “ Carsharing with electric vehicles in the context of users' mobility needs - results from user-centred research from the BeMobility field trial (Berlin) ”, International Journal of Automotive Technology and Management , Vol. 14 Nos 3-4 , pp. 286 - 305 .
Rust , R.T. and Cooil , B. ( 1994 ), “ Reliability measures for qualitative data: theory and implications ”, Journal of Marketing Research , Vol. 31 No. 1 , pp. 1 - 14 .
Sai , Q. , Bi , J. and Chai , J. ( 2020 ), “ Optimal model for carsharing station location based on multi-factor constraints ”, Algorithms , Vol. 13 No. 2 , p. 43 .
Santos , G.G.D. and de Almeida Correia , G.H. ( 2019 ), “ Finding the relevance of staff-based vehicle relocations in one-way carsharing systems through the use of a simulation-based optimization tool ”, Journal of Intelligent Transportation Systems: Technology, Planning, and Operations , Vol. 23 No. 6 , pp. 583 - 604 .
Schaefers , T. ( 2013 ), “ Exploring carsharing usage motives: a hierarchical means-end chain analysis ”, Transportation Research Part A: Policy and Practice , Vol. 47 , pp. 69 - 77 .
Schaefers , T. , Lawson , S.J. and Kukar-Kinney , M. ( 2016a ), “ How the burdens of ownership promote consumer usage of access-based services ”, Marketing Letters , Vol. 27 No. 3 , pp. 569 - 577 .
Schaefers , T. , Wittkowski , K. , Benoit (née Moeller) , S. and Ferraro , R. ( 2016b ), “ Contagious effects of customer misbehavior in access-based services ”, Journal of Service Research , Vol. 19 No. 1 , pp. 3 - 21 .
Schaefers , T. , Moser , R. and Narayanamurthy , G. ( 2018 ), “ Access-based services for the base of the pyramid ”, Journal of Service Research , Vol. 21 No. 4 , pp. 421 - 437 .
Schmidt , P. ( 2020 ), “ The effect of car sharing on car sales ”, International Journal of Industrial Organization , Vol. 71 , p. 102622 .
Schmöller , S. , Weikl , S. , Müller , J. and Bogenberger , K. ( 2015 ), “ Empirical analysis of free-floating carsharing usage: the munich and berlin case ”, Transportation Research Part C: Emerging Technologies , Vol. 56 , pp. 34 - 51 .
Schwabe , J. ( 2020 ), “ The evolution of cooperative electric carsharing in Germany and the role of intermediaries ”, Environmental Innovation and Societal Transitions , Vol. 37 , pp. 108 - 119 .
Shaheen , S.A. and Cohen , A.P. ( 2012 ), “ Carsharing and personal vehicle services: worldwide market developments and emerging Trends ”, International Journal of Sustainable Transportation , Vol. 7 No. 1 , pp. 5 - 34 .
Shaheen , S.A. and Martin , E. ( 2010 ), “ Demand for carsharing systems in Beijing, China: an exploratory study ”, International Journal of Sustainable Transportation , Vol. 4 No. 1 , pp. 41 - 55 .
Shaheen , S. , Sperling , D. and Wagner , C. ( 1998 ), “ Carsharing in Europe and North America: past, present and future ”, Transport Quaterly , Vol. 52 No. 3 , pp. 35 - 52 .
Shaheen , S. , Sperling , D. and Wagner , C. ( 1999 ), “ Carsharing and partnership management: an international perspective ”, Transportation Research Record , Vol. 1666 No. 1 , pp. 118 - 124 .
Shaheen , S.A. , Chan , N.D. and Micheaux , H. ( 2015 ), “ One-way carsharing's evolution and operator perspectives from the Americas ”, Transportation , Vol. 42 No. 3 , pp. 519 - 536 .
Sioui , L. , Morency , C. and Trépanier , M. ( 2012 ), “ How carsharing affects the travel behavior of households: a case study of Montréal, Canada ”, International Journal of Sustainable Transportation , Vol. 7 No. 1 , pp. 52 - 69 .
Snyder , H. ( 2019 ), “ Literature review as a research methodology: an overview and guidelines ”, Journal of Business Research , Vol. 104 , pp. 333 - 339 .
Snyder , H. , Witell , L. , Gustafsson , A. , Fombelle , P. and Kristensson , P. ( 2016 ), “ Identifying categories of service innovation: a review and synthesis of the literature ”, Journal of Business Research , Vol. 69 No. 7 , pp. 2401 - 2408 .
Sprei , F. and Ginnebaugh , D. ( 2018 ), “ Unbundling cars to daily use and infrequent use vehicles—the potential role of car sharing ”, Energy Efficiency , Vol. 11 No. 6 , pp. 1433 - 1447 .
Steininger , K. , Vogl , C. and Zettl , R. ( 1996 ), “ Car-sharing organizations in mobility behavior ”, Transport Policy , Vol. 3 No. 4 , pp. 177 - 185 .
Teece , D.J. ( 2010 ), “ Business models, business strategy and innovation ”, Long Range Planning , Vol. 43 Nos 2-3 , pp. 172 - 194 .
Terama , E. , Peltomaa , J. , Rolim , C. and Baptista , P. ( 2018 ), “ The contribution of car sharing to the sustainable mobility transition ”, Transfers , Vol. 8 No. 2 , pp. 113 - 121 .
Timmins , F. and McCabe , C. ( 2005 ), “ How to conduct an effective literature search ”, Nursing Standard , Vol. 20 No. 11 , pp. 41 - 47 .
Tran , V. , Zhao , S. , Diop , E.B. and Song , W. ( 2019 ), “ Travelers' acceptance of electric carsharing systems in developing countries: the case of China ”, Sustainability , Vol. 11 No. 19 , p. 5348 .
Tranfield , D. , Denyer , D. and Smart , P. ( 2003 ), “ Towards a methodology for developing evidence-informed management knowledge by means of systematic review ”, British Journal of Management , Vol. 14 No. 3 , pp. 207 - 222 .
Tuan Seik , F. ( 2000 ), “ Vehicle ownership restraints and car sharing in Singapore ”, Habitat International , Vol. 24 No. 1 , pp. 75 - 90 .
Tyndall , J. ( 2017 ), “ Where no cars go: free-floating carshare and inequality of access ”, International Journal of Sustainable Transportation , Vol. 11 No. 6 , pp. 433 - 442 .
Valor , C. ( 2020 ), “ Anticipated emotions and resistance to innovations: the case of p2p car sharing ”, Environmental Innovation and Societal Transitions , Vol. 37 , pp. 50 - 65 .
Wang , N. , Guo , J. , Liu , X. and Liang , Y. ( 2020 ), “ Electric vehicle car-sharing optimization relocation model combining user relocation and staff relocation ”, Transportation Letters , Article in press , pp. 1 - 12 .
Weikl , S. and Bogenberger , K. ( 2013 ), “ Relocation strategies and algorithms for free-floating car sharing systems ”, IEEE Intelligent Transportation Systems Magazine , Vol. 5 No. 4 , pp. 100 - 111 .
Weikl , S. and Bogenberger , K. ( 2015 ), “ A practice-ready relocation model for free-floating carsharing systems with electric vehicles - mesoscopic approach and field trial results ”, Transportation Research Part C: Emerging Technologies , Vol. 57 , pp. 206 - 223 .
Wilhelms , M.P. , Henkel , S. and Falk , T. ( 2017a ), “ To earn is not enough: a means-end analysis to uncover peer-providers' participation motives in peer-to-peer carsharing ”, Technological Forecasting and Social Change , Vol. 125 , pp. 38 - 47 .
Wilhelms , M.P. , Merfeld , K. and Henkel , S. ( 2017b ), “ Yours, mine, and ours: a user-centric analysis of opportunities and challenges in peer-to-peer asset sharing ”, Business Horizons , Vol. 60 No. 6 , pp. 771 - 781 .
Witell , L. , Snyder , H. , Gustafsson , A. , Fombelle , P. and Kristensson , P. ( 2016 ), “ Defining service innovation: a review and synthesis ”, Journal of Business Research , Vol. 69 No. 8 , pp. 2863 - 2872 .
Wittkowski , K. , Moeller , S. and Wirtz , J. ( 2013 ), “ Firm's intentions to use nonownership services ”, Journal of Service Research , Vol. 16 No. 2 , pp. 171 - 185 .
Wu , C. , Le Vine , S. , Clark , M. , Gifford , K. and Polak , J. ( 2020a ), “ Factors associated with round-trip carsharing frequency and driving-mileage impacts in London ”, International Journal of Sustainable Transportation , Vol. 14 No. 3 , pp. 177 - 186 .
Wu , C. , Le Vine , S. , Philips , S. , Tang , W. and Polak , J. ( 2020b ), “ Free-floating carsharing users' willingness-to-pay/accept for logistics management mechanisms ”, Travel Behavior and Society , Vol. 21 , pp. 154 - 166 .
Zhang , Y. and Li , L. ( 2020 ), “ Intention of Chinese college students to use carsharing: an application of the theory of planned behavior ”, Transportation Research Part F: Traffic Psychology and Behavior , Vol. 75 , pp. 106 - 119 .
Zhou , B. and Kockelman , K.M. ( 2011 ), “ Opportunities for and impacts of carsharing: a survey of the Austin, Texas market ”, International Journal of Sustainable Transportation , Vol. 5 No. 3 , pp. 135 - 152 .
Zhou , F. , Zheng , Z. , Whitehead , J. , Perrons , R. , Page , L. and Washington , S. ( 2017 ), “ Projected prevalence of car-sharing in four Asian-Pacific countries in 2030: what the experts think ”, Transportation Research Part C: Emerging Technologies , Vol. 84 , pp. 158 - 177 .
Zupic , I. and Čater , T. ( 2015 ), “ Bibliometric methods in management and organization ”, Organizational Research Methods , Vol. 18 No. 3 , pp. 429 - 472 .
Acknowledgements
This research received financial support from the CarE-Service project (which received funding from the European Union's Horizon 2020 research and innovation programme under grant agreement No 776851), and the SE:Kond 2 LIFE project (2019-04463), which was funded by Sweden's innovation agency Vinnova.
Corresponding author
About the authors.
Brenda Nansubuga is a Ph.D. student in industrial marketing at Linköping University, Sweden, and her research interests include service-based business model innovation, with focus on the automotive sector.
Christian Kowalkowski is a Professor of Industrial Marketing at Linköping University and is affiliated with the Centre for Relationship Marketing and Service Management at Hanken School of Economics in Helsinki. Dr. Kowalkowski's research interests include service growth strategies, solutions marketing, service innovation and subscription business models. His work has been published in journals such as Journal of Service Research, Journal of Service Management, Journal of Service Theory and Practice, Service Industries Journal, Industrial Marketing Management and Journal of Business Research . He is the servitization editor for the Journal of Service Management , associate editor of the Journal of Services Marketing and advisory board member of Industrial Marketing Management .
Related articles
We’re listening — tell us what you think, something didn’t work….
Report bugs here
All feedback is valuable
Please share your general feedback
Join us on our journey
Platform update page.
Visit emeraldpublishing.com/platformupdate to discover the latest news and updates
Questions & More Information
Answers to the most commonly asked questions here
The Economics of Multi-Hop Ride Sharing
Creating New Mobility Networks Through IS
- Research Paper
- Published: 06 August 2015
- Volume 57 , pages 311–324, ( 2015 )
Cite this article
- Timm Teubner 1 &
- Christoph M. Flath 2
4565 Accesses
76 Citations
1 Altmetric
Explore all metrics
Ride sharing allows to share costs of traveling by car, e.g., for fuel or highway tolls. Furthermore, it reduces congestion and emissions by making better use of vehicle capacities. Ride sharing is hence beneficial for drivers, riders, as well as society. While the concept has existed for decades, ubiquity of digital and mobile technology and user habituation to peer-to-peer services and electronic markets have resulted in particular growth in recent years. This paper explores the novel idea of multi-hop ride sharing and illustrates how information systems can leverage its potential. Based on empirical ride sharing data, we provide a quantitative analysis of the structure and the economics of electronic ride sharing markets. We explore the potential and competitiveness of multi-hop ride sharing and analyze its implications for platform operators. We find that multi-hop ride sharing proves competitive against other modes of transportation and has the potential to greatly increase ride availability and city connectedness, especially under high reliability requirements. To fully realize this potential, platform operators should implement multi-hop search, assume active control of pricing and booking processes, improve coordination of transfers, enhance data services, and try to expand their market share.
This is a preview of subscription content, log in via an institution to check access.
Access this article
Price includes VAT (Russian Federation)
Instant access to the full article PDF.
Rent this article via DeepDyve
Institutional subscriptions
Similar content being viewed by others
Ride Sharing: A Network Perspective
A Study of Ride Sharing Opportunities in the City of Santiago de Chile
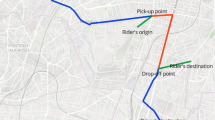
Ride-sharing with inflexible drivers in the Paris metropolitan area
André de Palma, Lucas Javaudin, … Léandre Tarpin-Pitre
While writing this paper, Carpooling.com has been taken over by its France-based competitor BlaBlaCar which, however, does not reduce the validity of our data, analyses, or conclusions as the market models of both firms are virtually identical.
These are (in descending order): Berlin (B), Hamburg (HH), Munich (M), Cologne (K), Frankfurt/Main (F), Stuttgart (S), Düsseldorf (D), Dortmund (DO), Essen (E), Bremen (HB), Leipzig (L), Dresden (DD), Hanover (H), Nuremberg (N), Duisburg (DU), Bochum (BO), Wuppertal (W), Bonn (BN), Bielefeld (BI), Mannheim (MA), and Karlsruhe (KA).
In this study, we limit the analysis to rides with one transfer (i.e., two hops). Drews and Luxen ( 2013 ), found negligible improvements when allowing for more than two hops.
This observation can be explained through the temporal trip distribution as shown in Fig. 2 : the morning and evening ride supply peaks are fairly compact. Hence, given a short first-hop ride, waiting times above 80 minutes fail to tap into much extra supply, as the peak has flattened out by then.
Based on the edges served by both direct and MHRS rides, MHRS trips are on average 10 % longer in distance, 17 % more expensive, and take 40 % more time than their direct counterparts.
Anecdotal evidence confirms this notion, as Carpooling.com also retains fully booked rides in its search results, indicating that demand may surpass supply.
The results do not change qualitatively for more complex, e.g., non-linear, relationships.
This analysis is based on train prices discounted by 50 % to reflect the possibility of obtaining low-cost tickets or other discount programs (“BahnCard50”). The analysis favors MHRS even more when assuming regular train fares.
This difference is significant for any conventional threshold. Using Fisher’s Exact Test with groups “direct only” vs. “direct + MHRS” and outcomes “link exists (#rides \(\ge \tau \) ) and “no link (#rides \(<\tau \) ),” and for all \(\tau \in \{1,2,\ldots ,20\},\) yields p -values \(<.0001\) .
Formally, this is given by \(\sqrt{p_1 p_2}\) where \(p_1\) and \(p_2\) denote the population figures (in millions) of city 1 and 2, respectively.
Note that 90 % of all relations fall within a range from 80 to 600 km.
The price decrease is 38.5 cents per 100 km for a 1 million increase in the geometric population mean. For the two largest cities this would suggest a reduction of 95 cents per 100 km compared with a reduction of 36.5 cents between the two smallest cities.
These findings are in line with standard gravity models as used in transportation analysis (Erlander and Stewart 1990 ).
http://techcrunch.com/2015/04/15/blablacar-acquires-its-biggest-competitor-carpooling-com-to-dominate-european-market/ .
Making it from one meeting point to another introduces hassle. Everyone who ever changed trains from Gare de L’Est to Gare de Lyon in Paris will certainly agree.
These are conveniently located and should be interested in ride sharing activities for at least two reasons: i) Drivers are likely to fuel their cars and purchase other products and ii), petrol companies may improve their image by actively supporting ride sharing – a fuel-efficient and thus sustainable activity beyond all doubt.
Such optimization could leverage the rich body of research on optimal transit design and train scheduling. See Cordeau et al. ( 1998 ) and Guihaire and Hao ( 2008 ) for comprehensive reviews.
Amey A, Attanucci J, Mishalani R (2011) Real-time ridesharing. Transp Res Rec: J Transp Res Board 2217(1):103–110
Article Google Scholar
Bardhi F, Eckhardt GM (2012) Access-based consumption: the case of car sharing. J Consum Res 39(4):881–898
Basole RC, Rouse WB (2008) Complexity of service value networks: conceptualization and empirical investigation. IBM Syst J 47(1):53–70
Beul-Leusmann S, Samsel C, Wiederhold M, Krempels K-H, Jakobs E-M, Ziefle M (2014) Usability evaluation of mobile passenger information systems. In: Design, user experience, and usability. Theories, methods, and tools for designing the user experience. Springer, pp 217–228
Bicocchi N, Mamei M (2014) Investigating ride sharing opportunities through mobility data analysis. Pervasive Mob Comput 14(2014):83–94
Blau B, Conte T, van Dinther C (2010) A multidimensional procurement auction for trading composite services. Electron Commer Res Appl 9(5):460–472
Blau B, van Dinther C, Conte T, Xu Y, Weinhardt C (2009) How to coordinate value generation in service networks. Bus Inf Syst Eng 1(5):343–356
Bohmann T, Leimeister JM, Möslein K (2014) Service systems engineering – a field for future information systems research. Bus Inf Syst Eng 6(2):73–79
Botsman R, Rogers R (2010) What’s mine is yours. HarperBusiness, New York
Google Scholar
Brereton M, Roe P, Foth M, Bunker JM, Buys L (2009) Designing participation in agile ridesharing with mobile social software. In: Proc 21st Annu Conf Australian Comput-Human Interact Special Interest Group. ACM, New York, pp 257–260
Chan ND, Shaheen SA (2012) Ridesharing in North America: past, present, and future. Transp Rev 32(1):93–112
Cohen B, Kietzmann J (2014) Ride On! Mobility business models for the sharing economy. Org Environ 27(3):279–296
Coltin BJ, Veloso M (2013) Towards ridesharing with passenger transfers. In: Proceedings of the 2013 international conference on Autonomous agents and multi-agent systems, pp 1299–1300
Cordeau J-F, Toth P, Vigo D (1998) A survey of optimization models for train routing and scheduling. Transp Sci 32(4):380–404
Cusumano MA (2014) How traditional firms must compete in the sharing economy. Commun ACM 58(1):32–34
Deakin E, Frick KT, Shively KM (2010) Markets for dynamic ridesharing? Transp Res Rec: J Transp Res Board 2187(1):131–137
Dedrick J (2010) Green IS: concepts and issues for information systems research. Commun Assoc Inf Syst 27(1):173–184
Drews F, Luxen D (2013) Multi-hop ride sharing. In: Proceedings of the Sixth International Symposium on Combinatorial Search, pp 71–79
Eisenmann T, Parker G, Van Alstyne M (2011a) Platform envelopment. Strat Manag J 32(12):1270–1285
Eisenmann TR, Parker G, Van Alstyne M (2011b) Opening platforms: how, when and why? In: Gawer A (ed) Platforms, markets and innovation. Edward Elgar, pp 131–162
Eisenmann T, Parker G, Van Alstyne MW (2006) Strategies for two-sided markets. Harv Bus Rev 84(10):92–101
Erlander S, Stewart NF (1990) The gravity model in transportation analysis: theory and extensions, vol 3. Brill Academic, Utrecht
Evans DS (2003) The antitrust economics of multi-sided platform markets. Yale J Regul 20(2):325–382
Furuhata M, Dessouky M, Ordóñez F, Brunet M-E, Wang X, Koenig S (2013) Ridesharing: the state-of-the-art and future directions. Transp Res Part B: Methodol 57(2013):28–46
Gefen D, Detmar WS (2004) Consumer trust in B2C e-commerce and the importance of social presence: experiments in e-products and e-services. Omega 32(6):407–424
Goebel C, Jacobsen H-A, del Razo V, Doblander C, Rivera J, Ilg J, Flath C, Schmeck H, Weinhardt C, Pathmaperuma D, Appelrath H-J, Sonnenschein M, Lehnhoff S, Kramer O, Staake T, Fleisch E, Neumann D, Strüker J, Erek K, Zarnekow R, Ziekow H, Lässig J (2014) Energy informatics. Bus Inf Syst Eng 6(1):25–31
Guihaire V, Hao J-K (2008) Transit network design and scheduling: a global review. Transp Res Part A: Policy Pract 42(10):1251–1273
Gurvich I, Lariviere M, Moreno A (2014) Staffing service systems when capacity has a mind of its own. Working paper. Available at SSRN 2336514
Herbawi WM, Weber M (2012) A genetic and insertion heuristic algorithm for solving the dynamic ridematching problem with time windows. In: Proceedings of the fourteenth international conference on Genetic and evolutionary computation conference. ACM, pp 385–392
Hou Y, Li X, Qiao C (2012) TicTac: from transfer-incapable carpooling to transfer-allowed carpooling. In: Global Communications Conference (GLOBECOM). IEEE, pp 268–273
Jones K, Leonard LNK (2008) Trust in consumer-to-consumer electronic commerce. Inf Manag 45(2):88–95
Kim C, Mirusmonov M, Lee I (2010) An empirical examination of factors influencing the intention to use mobile payment. Comput Hum Behav 26(3):310–322
Kleiner A, Nebel B, Ziparo VA (2011) A mechanism for dynamic ride sharing based on parallel auctions. IJCAI 11:266–272
Lequerica I, Longaron MG, Ruiz PM (2010) Drive and share: efficient provisioning of social networks in vehicular scenarios. IEEE Commun Mag 48(11):90–97
Leukel J, Kirn S, Schlegel T (2011) Supply chain as a service: a cloud perspective on supply chain systems. IEEE Syst J 5(1):16–27
Malhotra A, Van Alstyne M (2014) The dark side of the sharing economy... and how to lighten it. Commun ACM 57(11):24–27
Rayle L, Shaheen S, Chan N, Dai D, Cervero R (2014) App-based, on-demand ride services: comparing taxi and ridesourcing trips and user characteristics in San Francisco. Working Paper
Rochet J-C, Tirole J (2003) Platform competition in two-sided markets. J Eur Econ Assoc 1(4):990–1029
Rochet J-C, Tirole J (2006) Two-sided markets: a progress report. RAND J Econ 37(3):645–667
Shaheen S, Sperling D, Wagner C (1998) Carsharing in Europe and North American: past, present, and future. Transp Q 52(3):35–52
Shaheen SA, Mallery MA, Kingsley KJ (2012) Personal vehicle sharing services in North America. Res Transp Bus Manag 3(2012):71–81
Slee T (2013) Some obvious things about internet reputation systems. Working Paper
Sundararajan A (2013) From zipcar to the sharing economy. Harv Bus Rev
Teal RF (1987) Carpooling: who, how and why. Transp Res Part A: Gen 21(3):203–214
Teubner T (2014) Thoughts on the sharing economy. In: Proceedings of the International Conference on e-Commerce, pp 322–326
Teubner T, Adam MTP, Camacho S, Hassanein K (2014) Understanding resource sharing in C2C platforms: the role of picture humanization. In: Proc 25th Australasian Conf Inf Syst
van Heck E, Vervest P (2007) Smart business networks: how the network wins. Commun ACM 50(6):28–37
vom Brocke J, Jan, Watson RT, Dwyer C, Elliot S, Melville N (2013) Green information systems: directives for the IS Discipline. Commun Assoc Inf Syst 33(30):509–520
Watson RT, Boudreau M-C, Chen AJ (2010) Information systems and environmentally sustainable development: energy informatics and new directions for the IS community. Manag Inf Syst Q 34(1):23–38
Zhao D, Zhang D, Enrico HG, Yuko S, Makoto Y (2014) Incentives in ridesharing with deficit control. In: Proceedings of the 2014 International Conference on Autonomous Agents and Multi-agent Systems (AAMAS), pp 1021–1028
Download references
Acknowledgements
The authors would like to thank Matthias Hauser as well as seminar participants at the Karlsruhe Institute of Technology and the University of Würzburg.
Author information
Authors and affiliations.
Institute of Information Systems and Marketing (IISM), Karlsruhe Institute of Technology (KIT), Englerstraße 14, 76131, Karlsruhe, Germany
Timm Teubner
Department of Business Management, Universität Würzburg, 97070, Würzburg, Germany
Christoph M. Flath
You can also search for this author in PubMed Google Scholar
Corresponding author
Correspondence to Timm Teubner .
Additional information
Accepted after three revisions by the editors of the special issue.
Rights and permissions
Reprints and permissions
About this article
Teubner, T., Flath, C.M. The Economics of Multi-Hop Ride Sharing. Bus Inf Syst Eng 57 , 311–324 (2015). https://doi.org/10.1007/s12599-015-0396-y
Download citation
Received : 29 October 2014
Accepted : 08 July 2015
Published : 06 August 2015
Issue Date : October 2015
DOI : https://doi.org/10.1007/s12599-015-0396-y
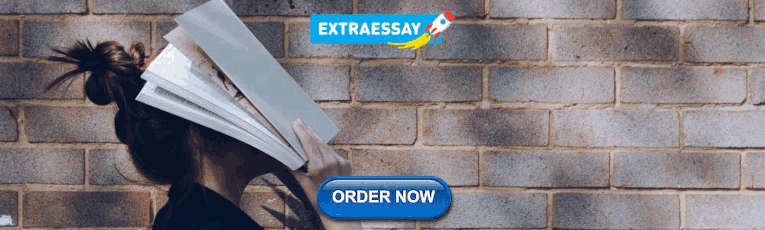
Share this article
Anyone you share the following link with will be able to read this content:
Sorry, a shareable link is not currently available for this article.
Provided by the Springer Nature SharedIt content-sharing initiative
- Multi-hop ride sharing
- Sharing economy
- Mobility networks
- Platform economics
- Find a journal
- Publish with us
- Track your research
Help | Advanced Search
Computer Science > Data Structures and Algorithms
Title: a dynamic tree algorithm for peer-to-peer ride-sharing matching.
Abstract: On-demand peer-to-peer ride-sharing services provide flexible mobility options, and are expected to alleviate congestion by sharing empty car seats. An efficient matching algorithm is essential to the success of a ride-sharing system. The matching problem is related to the well-known dial-a-ride problem, which also tries to find the optimal pickup and delivery sequence for a given set of passengers. In this paper, we propose an efficient dynamic tree algorithm to solve the on-demand peer-to-peer ride-sharing matching problem. The dynamic tree algorithm benefits from given ride-sharing driver schedules, and provides satisfactory runtime performances. In addition, an efficient pre-processing procedure to select candidate passenger requests is proposed, which further improves the algorithm performance. Numerical experiments conducted in a small network show that the dynamic tree algorithm reaches the same objective function values of the exact algorithm, but with shorter runtimes. Furthermore, the proposed method is applied to a larger size problem. Results show that the spatial distribution of ride-sharing participants influences the algorithm performance. Sensitivity analysis confirms that the most critical ride-sharing matching constraints are the excess travel times. The network analysis suggests that small vehicle capacities do not guarantee overall vehicle-kilometer travel savings.
Submission history
Access paper:.
- Download PDF
- Other Formats
References & Citations
- Google Scholar
- Semantic Scholar
DBLP - CS Bibliography
Bibtex formatted citation.

Bibliographic and Citation Tools
Code, data and media associated with this article, recommenders and search tools.
- Institution
arXivLabs: experimental projects with community collaborators
arXivLabs is a framework that allows collaborators to develop and share new arXiv features directly on our website.
Both individuals and organizations that work with arXivLabs have embraced and accepted our values of openness, community, excellence, and user data privacy. arXiv is committed to these values and only works with partners that adhere to them.
Have an idea for a project that will add value for arXiv's community? Learn more about arXivLabs .
Suggestions or feedback?
MIT News | Massachusetts Institute of Technology
- Machine learning
- Social justice
- Black holes
- Classes and programs
Departments
- Aeronautics and Astronautics
- Brain and Cognitive Sciences
- Architecture
- Political Science
- Mechanical Engineering
Centers, Labs, & Programs
- Abdul Latif Jameel Poverty Action Lab (J-PAL)
- Picower Institute for Learning and Memory
- Lincoln Laboratory
- School of Architecture + Planning
- School of Engineering
- School of Humanities, Arts, and Social Sciences
- Sloan School of Management
- School of Science
- MIT Schwarzman College of Computing
Study finds ride-sharing intensifies urban road congestion
Press contact :.
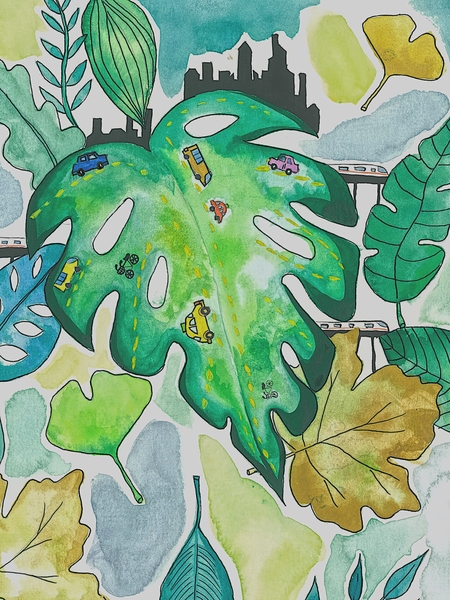
Previous image Next image
Transport network companies (TNCs), or ride-sharing companies, have gained widespread popularity across much of the world, with more and more cities adopting the phenomenon. While ride-sharing has been credited with being more environmentally friendly than taxis and private vehicles, is that really the case today, or do they rather contribute to urban congestion?
Researchers at the Future Urban Mobility (FM) Interdisciplinary Research Group (IRG) at Singapore-MIT Alliance for Research and Technology (SMART), MIT, and Tongji University conducted a study to find out.
In a paper titled “ Impacts of transportation network companies on urban mobility ” recently published in Nature Sustainability , the first-of-its-kind study assessed three aspects of how ride-sharing (more accurately called ride-hailing) impacts urban mobility in the United States — road congestion, public transport ridership, and private vehicle ownership — and how they have evolved over time.
“While public transportation provides high-efficiency shared services, it can only accommodate a small portion of commuters, as their coverage is limited in most places,” says Jinhua Zhao, SMART FM principal investigator and associate professor at MIT Department of Urban Studies and Planning. “While mathematical models in prior studies showed that the potential benefit of on-demand shared mobility could be tremendous, our study suggests that translating this potential into actual gains is much more complicated in the real world.”
Using a panel dataset covering mobility trends, socio-demographic changes, and TNC entry at the metropolitan statistical areas level to construct a set of fixed-effect panel models, the researchers found the entrance of TNCs led to increased road congestion in terms of both intensity and duration. Specifically, they noted that congestion increased by almost 1 percent while the duration of congestion rose by 4.5 percent. They also found a 8.9 percent drop in public transport ridership and an insignificant decrease of only 1 percent in private vehicle ownership.
While many previous studies have focused on Uber alone, this study takes into account both Uber and Lyft — the two most popular ride-sharing companies in the United States. While Uber accounts for 69 percent of the market, Lyft accounts for a significant 29 percent, and its inclusion into the dataset would give a more holistic and unbiased estimate of the TNC effect.
The study also finds easy access to ride-sharing discourages commuters from taking greener alternatives, such as walking or public transportation. Survey data from various U.S. cities also showed that approximately half of TNC trips would otherwise have been made by walking, cycling, public transport, or would not have been made at all.
“We are still in the early stages of TNCs and we are likely to see many changes in how these ride-sharing businesses operate,” says Hui Kong, SMART-FM alumna and postdoc at the MIT Urban Mobility Lab, and an author of the paper. “Our research shows that over time TNCs have intensified urban transport challenges and road congestion in the United States, mainly through the extended duration and slightly through the increased intensity. With this information, policies can then be introduced that could lead to positive changes.”
The researchers think that the substantial deadheading miles (miles traveled without a passenger) by TNCs could contribute to the TNC’s negative impact on road congestion. According to some other studies, approximately 40.8 percent of TNC miles are deadheading miles.
“Our findings can provide useful insights into the role that TNCs have played in urban transport systems,” says Professor Mi Diao of Tongji University and SMART-FM alumnus, who is the lead author of the paper. “It can be very useful in supporting transportation planners and policymakers in their decisions and regulations with regard to TNCs.”
The research is carried out by SMART and supported by the National Research Foundation (NRF) Singapore under its Campus for Research Excellence And Technological Enterprise (CREATE) program.
FM is one of five IRGs in SMART. FM harnesses new technological and institutional innovations to create the next generation of urban mobility systems to increase accessibility, equity, safety, and environmental performance for the citizens and businesses of Singapore and other metropolitan areas, worldwide.
SMART is MIT’s research enterprise in Singapore, established in partnership with the NRF in 2007. SMART is the first entity in the CREATE. SMART serves as an intellectual and innovation hub for research interactions between MIT and Singapore, undertaking cutting-edge research projects in areas of interest to both Singapore and MIT. SMART currently comprises an Innovation Center and five IRGs: Antimicrobial Resistance, Critical Analytics for Manufacturing Personalized-Medicine, Disruptive and Sustainable Technologies for Agricultural Precision, FM, and Low Energy Electronic Systems.
Share this news article on:
Press mentions.
Gizmodo reporter Victoria Song writes that a new study by researchers from the Singapore-MIT Alliance for Research and Technology (SMART) finds that “not only do rideshares increase congestion, but they also made traffic jams longer , led to a significant decline in people taking public transit, and haven’t really impacted car ownership.”
Previous item Next item
Related Links
- Singapore-MIT Alliance for Research and Technology (SMART)
- Urban Mobility Lab at MIT
- Department of Urban Studies and Planning
- School of Architecture and Planning
Related Topics
- Transportation
- Urban studies and planning
- Automobiles
- Collaboration
- Public service
- Sustainability
- Technology and society
Related Articles
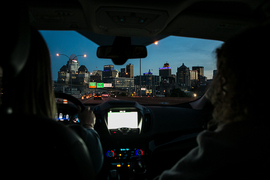
3 Questions: The price of privacy in ride-sharing app performance
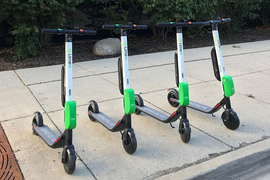
Comparing the benefits of scooter-sharing vs. bike-sharing
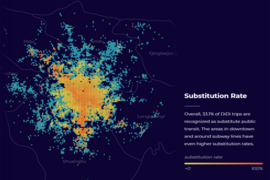
Does ride-sharing substitute for or complement public transit?
More mit news.
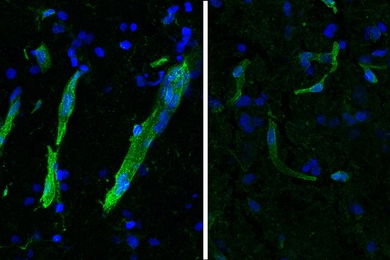
Study: Movement disorder ALS and cognitive disorder FTLD show strong molecular overlaps
Read full story →
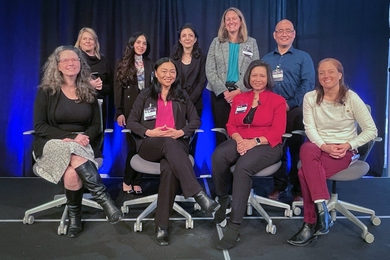
Students explore career opportunities in semiconductors
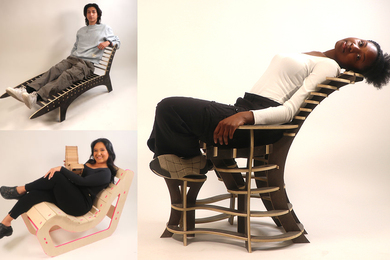
For MIT students, there is much to learn from crafting a chair
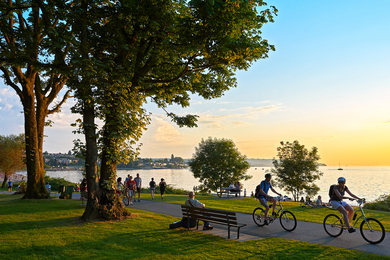
A new way to quantify climate change impacts: “Outdoor days”
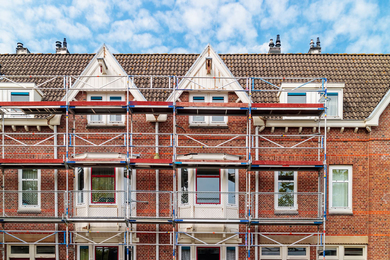
Think globally, rebuild locally
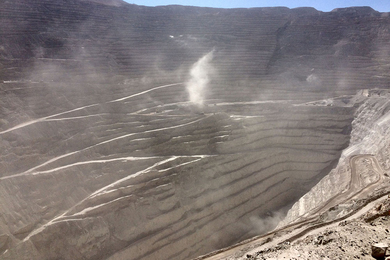
Understanding the impacts of mining on local environments and communities
- More news on MIT News homepage →
Massachusetts Institute of Technology 77 Massachusetts Avenue, Cambridge, MA, USA
- Map (opens in new window)
- Events (opens in new window)
- People (opens in new window)
- Careers (opens in new window)
- Accessibility
- Social Media Hub
- MIT on Facebook
- MIT on YouTube
- MIT on Instagram

An official website of the United States government
The .gov means it’s official. Federal government websites often end in .gov or .mil. Before sharing sensitive information, make sure you’re on a federal government site.
The site is secure. The https:// ensures that you are connecting to the official website and that any information you provide is encrypted and transmitted securely.
- Publications
- Account settings
Preview improvements coming to the PMC website in October 2024. Learn More or Try it out now .
- Advanced Search
- Journal List
- Springer Nature - PMC COVID-19 Collection

A systematic literature review of ride-sharing platforms, user factors and barriers
Lambros mitropoulos.
Centre for Research and Technology Hellas, Hellenic Institute of Transport, 52 Egialias Street, 15125 Marousi, Greece
Annie Kortsari
Georgia ayfantopoulou, associated data.
The datasets generated and/or analyzed during the current study are partly publicly available due to contractual restrictions. These can be found in Deliverable 2.2. State-of-the-art of ride-sharing in target EU countries, Horizon EU funded project Ride2Rail.
Ride-sharing is an innovative on-demand transport service that aims to promote sustainable transport, reduce car utilization, increase vehicle occupancy and public transport ridership. By reviewing ride-sharing studies around the world, this paper aims to map major aspects of ride-sharing, including online platforms, user factors and barriers that affect ride-sharing services, and extract useful insights regarding their successful implementation.
A systematic literature review is conducted on scientific publications in English language. Articles are eligible if they report a study on user factors affecting ride-sharing use and/or barriers preventing ride-sharing implementation; ride-sharing online platforms in these articles are also recorded and are further explored through their official websites. A database is built that organizes articles per author, year and location, summarizes online platform attributes, and groups user factors associated with the likelihood to ride-share.
The review shows that the term “ride-sharing” is used in the literature for both profit and non-profit ride-sharing services. In total, twenty-nine ride-sharing online platforms are recorded and analyzed according to specific characteristics. Sixteen user factors related to the likelihood to ride-share are recorded and grouped into sociodemographic, location and system factors. While location and system factors are found to follow a pattern among studies, mixed findings are recorded on the relationship between sociodemographic factors and ride-sharing. Factors that may hinder the development of ride-sharing systems are grouped into economic, technological, business, behavioral and regulatory barriers.
Opportunities exist to improve the quality of existing ride-sharing services and plan successful new ones. Future research efforts should focus towards studying ride-sharing users' trip purpose (i.e., work, university, shopping, etc.), investigating factors associated to ride-sharing before and after implementation of the service, and perform cross-case studies between cities and countries of the same continent to compare findings.
Introduction
Ride-sharing aims to minimize negative impacts related to emissions, reduce travelling costs and congestion [ 20 , 40 ], and increase passenger vehicle occupancy and public transit ridership. During the last decade, innovative mobility solutions were introduced, including on-demand mobility services and Mobility as a Service (MaaS), that focused on daily travel needs to promote sustainable transport [ 20 ].
The literature uses the term “ride-sharing” to describe various mobility sharing concepts. Ride-sharing refers to the common use of a motor vehicle by a driver and one or several passengers, in order to share the costs (non-profit) or to compensate the driver (i.e., paid service) using billing information provided by the participants (for profit). In this study the term is used to describe the common use of a motor vehicle for cost compensation, in the context of a ride, that the driver performs for its own account (referred also as Carpooling); thus, it is not intended to result in any financial gain [ 20 ].
Practical experience shows that ride-sharing trips are usually pre-arranged through matching applications, that allow drivers and passengers to find potential rides. They often include community-based trust mechanisms, such as user-ratings and provide links to social networks to allow prospective sharers to check each other. Ride-sharing has demonstrated limited uptake so far, due to business, economic and technological barriers [ 37 , 38 , 48 , 50 ]. Past ride-sharing studies focused mainly on ride-matching algorithms for ride-sharing optimization [ 2 , 47 , 63 ], dynamic ride-sharing pricing [ 2 , 3 ], and the economic, social, transport, and environmental benefits of ride-sharing [ 19 , 20 , 83 , 95 , 111 ]. Studies on factors affecting ride-sharing use have been increased within the last decade (e.g., [ 11 , 13 , 14 , 23 ]) showing the challenges and diversity of results per case study. A synthesis of information about factors that affect ride-sharing use and implementation barriers, is required to inform interested stakeholders and planners. To the best of our knowledge, there are no previous studies that review the user factors and barriers when implementing a ride-sharing service.
The aim of this systematic review is to understand, how successful ride-sharing services could be implemented and operated. This is achieved by recording and synthesizing data for online ride-sharing platforms, factors affecting users to ride-share (i.e., increase and decrease the likelihood to ride-share), and potential implementation barriers. The remainder of this paper is organized as follows: Sect. 2 outlines the methodological steps of this research and provides details for the publications that were collected and analyzed. Section 3 summarizes literature findings and results. More specifically, authors first review ride-sharing definitions and identify how the term is used in literature. Next, online ride-sharing platforms that were identified in literature are further explored in terms of operation status, starting year, location, and distance of service. User factors that are associated with the likelihood to ride-share are also recorded and presented. The third section synthesizes data from previous sections to discuss implementation barriers for ride-sharing services and make recommendations.
To provide a detailed understanding of ride-sharing it should be noted that users in this study are divided into drivers and passengers. Ride-sharing platforms refer to official providers or companies of ride-sharing services. Other topics, such as ride-sharing financial, economic or business models are not covered herein. Venues for further research are highlighted through the article.
Methodology
This research focuses on a state-of-the-art analysis of ride-sharing that constitutes the basis for understanding different aspects, including online platforms and user factors and discusses potential barriers that prevent the successful implementation of ride-sharing systems. To achieve its purpose, the methodological approach builds on the principles of systematic literature review. A systematic review method helps researchers to develop a high-level overview of knowledge on a particular research area [ 22 , 27 , 56 ]. A systematic review means adopting a replicable, scientific and transparent process, in other words a detailed process that minimizes bias, through exhaustive literature searches of published and unpublished studies and by providing an audit trail of the reviewers’ decisions, procedures and conclusions [ 27 ].
The methodology focuses on the content of the publications, the research per se, rather than on their metrics. Although, more information regarding local ride-sharing systems may exist in different languages, we have limited the scope of this study to English-speaking publications, and we focus only on papers published in academic journals and conference proceedings, excluding books, chapters of books, thesis and dissertations. Following Moustaghfir [ 69 ], the methodological approach adopted, comprises of six parts (Fig. 1 ), as follows:

Methodological structure
Identification of objectives
Adapting the paper’s goal and the steps for performing a systematic literature review, the research questions (RQ) are shaped before starting to perform the review [ 27 ]. These are:
- RQ1: Does a universal definition for “ride-sharing” exist in literature, and how is ride-sharing defined?
- RQ2: Do ride-sharing online platforms (i.e., in operation and inactive) share common attributes?
- RQ3: What factors affect passenger and drivers to use ride-sharing?
- RQ4: What prevents ride-sharing systems from being successful?
Based on these four questions—four main objectives were identified as of high relevance to the understanding of ride-sharing services:
- Definition of a ride-sharing;
- State-of-the-art analysis of ride-sharing online platforms;
- Identification of factors affecting current and potential ride-sharing passenger and drivers.
- Synthesis and discussion of barriers for implementing a successful ride-sharing system.
Identification of data sources and databases
The purpose of data collection is to collect the most representing research material and use the most recent information available. This step is composed of three sub-steps: Primary studies, search keywords, search database. Primary studies refer to the identification of relevant studies, to ensure first that the set research questions-objectives are valid, avoid duplication of previous work, and ensure that enough material is available to conduct the analysis. An initial search in “Google Scholars” and “science direct” by using the term “ridesharing” AND “review” resulted to three relevant studies, that review dynamic ride-sharing concept [ 2 ], ridesharing and matching criteria [ 38 ], and a meta-analysis exploring the factors that affect ride-sharing, which included 19 papers in the analysis [ 73 ]; however, none of them includes a review on ride-sharing platforms, user factors and barriers.
As a first step the keywords were identified to enable the conceptualization of the research and helped to target relevant articles. Prior selecting keywords, a shortlist of sharing mobility services was made. The keywords were defined by the authors based on their professional experience. Keywords related to shared mobility definition included: ride-sharing, carpooling, mobility as a service, MaaS, innovative mobility. Car-sharing publications, which refer to short-term auto use [ 20 ], were excluded from this research to focus exclusively on on-demand transport for passengers.
The terms “Ride-hailing” and “on-demand ride” were also excluded, as these two terms returned publications relevant to ride-sharing services that aim to financial gain (e.g., Uber, Lyft, etc.).
In literature, carpooling is a synonym for ride-sharing for non-profit reasons. The keywords ride-sharing and carpooling were constructed into search strings by using other keywords relative to the objectives, such as factors, users, passengers, barriers, constraints, legal-framework, drivers; resulting to strings: ride-sharing factors, ride-sharing users, etc. These search strings were used to conduct searches for all geographical areas. Factors that decrease the likelihood to ride-share and thus prevent ride-sharing implementation may be considered as barriers or constraints. Thus, authors included both terms as separate search terms for performing a complete review and synthesizing results. It should be noted that keywords ride-sharing and carpooling were typed in all possible formats, as these were found in literature: with a dash (–), with a space and as single words. We limited our research to articles published in English language within the last 30 years, from 1990 to 2020. Concurrently, authors and year of publication were also identified to perform a second search based on their names.
The data sources that were used to collect the necessary information and data include published journal and conference papers (Science Direct, Web of Science, Google Scholar, Wiley Online Library and Springer). Online platforms that were identified in these data sources, were further explored. The status and attributes of identified ride-sharing online platforms were not disclosed in the scientific manuscripts; therefore, a follow-up desk review conducted by focusing on online official websites and social-media of each provider.
Selection of publications
The first task was to merge publications and exclude potential duplicates, thesis or dissertations, and publications that were not related to ride-sharing, such as publications focusing on taxi ride-sharing services. All duplicate publications were deleted; the remaining ones were exported to an excel file for screening. Definitions for different and partially overlapping concepts have emerged in publications’ titles, including ride-hailing (commercial, organized by companies), ride-sourcing and ride-pooling (commercial, organized by public institutions) [ 29 , 35 ]. Publications not referring to ride-sharing or carpooling were eliminated by title screening. The second task was to identify if these publications refer to ride-sharing, carpooling or ride-hailing. This was achieved by reviewing each publication’s abstract. Abstract reviewing was performed by authors who are transportation experts. In some cases, the ride-sharing definition that was used in the study was not clear and authors had to review the introduction or/and the methodology of each publication (i.e., text review).
Each publication was recorded according to title, authors, year of publication and location of the study, and then it was reviewed to record specific features (when available) and build the database. These features refered to: (a) Ride-sharing definition, (b) Ride-sharing platforms (i.e., specific ride-sharing online platforms by name), (c) User factors—referring to factors affecting users (i.e., passengers and drivers) to use ride-sharing services, and (d) Barriers—referring to potential barriers and constraints that are faced in the implementation of ride-sharing services.
Development of tools for data collection
For facilitating the data collection process, a template was developed. The developed template aimed to collect and organize information relative to ride-sharing online platforms, which is provided on the websites and social media of ride-sharing companies or related services, according to the following characteristics:
- Name of company/ride-sharing platform
- Potential barriers and provided incentives
- Country of operation
- Company/provider website
- Current status of ride-sharing platform (in/not in operation)
- Period of operation of the ride-sharing platform
- Provision of urban/interurban transport services (i.e., urban trips here are considered within the same city; interurban include all other trip types).
Collected information is analyzed and used as input to support each of the four objectives. Data are tabulated when possible, to support the objectives and are presented in the following sections.
Figure 2 provides the flow diagram of publications included in the review [ 67 ]. The initial combined total number of publications was 363 articles. Following the first screening, 113 publications remained. The second screening identified if these publications refer to ride-sharing, carpooling or ride-hailing by reviewing their abstracts. Three articles that fulfilled the criteria, were not available in a database and thus were eliminated. Following the second screening, 84 publications remained. Following the text review, twenty-eight publications were found to use the term ride-sharing while referring to for-profit ride-sharing services such as Uber and Lyft (i.e., ride-hailing). Finally, 56 articles met the inclusion criteria for our review.

Number of publications in the review process
The majority of them use the term ride-sharing (n = 32) and carpooling (n = 23). It should be noted that one publication uses both the term ride-sharing and ride-hailing. Almost half of the studies were conducted in the US (n = 25) and one-quarter in EU and the UK (n = 19), with the rest being global (n = 2), in China (n = 4), in Canada (n = 3), in Australia, in New Zealand and in Asia (all n = 1). The majority of the studies focus on user factors (n = 32), while 15 of them discuss barriers related to planning and implementation of ride-sharing, and 18 mention at least one ride-sharing online platform.
Exploration and synthesis
For each of the four objectives a discussion and synthesis of information is provided in respective sections, as outlined in the introduction.
The results of the literature review are summarized in Table Table1 1 .
Summary of ride-sharing publications
Ride-sharing definition
Table Table2 2 presents a sample of recent publications and ride-sharing definitions. A universally accepted definition for “ride-sharing” does not exist and the term “ride-sharing” is defined based on the context of each study.
Ride-sharing definitions within literature
Ride-sharing typically includes carpooling and vanpooling [ 20 ], while the term does not necessarily refer to consistent participation in the same ride-share service every day [ 20 ] neither to daily use of the service. Ride-sharing may be used by its passengers as a mode to complete their whole trip (i.e., origin to destination) or to complement public transport, with the focus of further incorporating public transport in the multimodal transport chain. In the latter context, ride-sharing aims to facilitate access for the first/last mile to public transport services, to optimize multimodality and on-demand mobility, thus reducing single-occupant trips, and finally to develop smart urban/rural transport areas. A ride-sharing definition that may be used for non-profit ride-sharing services is proposed according to Code of Virginia US [ 26 ] that defines “Ride-sharing” as the transport of persons in a motor vehicle when such transportation is incidental to the principal purpose of the driver, which is to reach a destination and not to transport persons for profit.
Ride-sharing platforms
In total 29 ride-sharing online platforms have been identified in the reviewed literature (Table (Table3). 3 ). The platform recommends a ride fee and passengers decide to accept it or not; from the total fee the provider retain a fixed amount to cover the transaction cost. Although this is the most common practice, in very few occasions (only 2% of the cases), drivers may decide what to charge passengers after reviewing the platform’s recommendation and this occurs for interurban ride-sharing services.
Summary of ride-sharing platforms
In terms of geographical coverage, ride-sharing platforms operate in US, EU, Asia, and Latin America. Ride-sharing platforms that provide services to more than one of these geographic areas are classified as global. The majority of the ride-sharing platforms were found to operate in EU (48%) with 27% of them being in Italy; a high share compared to the rest of the EU countries, showing the attempts to promote ride-sharing in Italy. US- and Asia-based platforms accounted for 20% and 10% of all platforms, respectively, while 20% operate globally. Although, this geographic classification refers to countries or continents, rarely one service covers the totality of a country as in most cases, services operate in a specific city or several close-by cities.
Urban and interurban platforms cover roughly 42% and 20% of all platforms, respectively, while ride-sharing platforms that cover both urban and interurban trips account for 38% of all. Urban trips here are considered within the same city; interurban include all other trip types. Often, ride-sharing platforms that provide only interurban services provide booking access through a website platform, whereas access through a mobile application is not available. To our understanding this occurs because interurban ride-sharing platforms require low maintenance in terms of administration and matching algorithms. In these cases, drivers publish their trip in advance and passengers review trip details (i.e., trip cost, destination, time of departure, driver profile) and decide to join or not. Therefore, to avoid extra maintenance costs for the service, a mobile application is not available. Several ride-sharing platforms have ceased operations due to low demand; some of them have re-started operation under a different name or/and follow a different business model. Approximately, 62% of the surveyed ride-sharing platforms are currently in operation, whereas 38% have ceased their operation. The vast majority of ride-sharing platforms (93%) have started their operation in 2005 or after, while 62% were found to start operations in or after 2010, which might be explained by the rapid development of mobile applications and spread of smartphones. Smartphone annual sales doubled between 2007 and 2010 (i.e., 122.32 vs. 296.65 million units), and increased by a factor of 4.2 between 2010 and 2014 (i.e., 296.65 vs. 969.72 million units), to reach 1540.66 million sold units in 2019 [ 89 ].
An important aspect, to address safety and security concerns and improve the overall level of services, is users’ feedback, as all of the ride-sharing platforms allow users to provide “feedback” either through the provided platform, through the application, or both. The feedback platform allows users to comment and evaluate the seriousness and reliability of drivers and vice versa. To further increased sense of safety, some platforms provide the option to women to travel only with other women as co-passengers or even drivers (i.e., Avacar).
The procedure to access ride-sharing is the same in all cases: users enter the platform, register and then search for offered trips. Trips can be organized last-minute, however, some platforms (18%) offer the opportunity to pre-plan trips one to two days in advance (e.g., for interurban trips).
The matching mechanisms for 90% of the platforms are destination-based. Drivers, who offer a ride, insert the departure and arrival locations and wait for those looking for the ride to that destination or a location along the way. The passenger consults a list of available to find the one that best meets their needs (i.e., departure, arrival, time, crew members, etc.). Once the passenger selects the path of their interest, they may undertake the necessary agreements (e.g., meeting point, how to recognize themself, etc.). Ride-sharing platforms do not use a sophisticated algorithm with multiple criteria to find the perfect ride-match, opposed to ride-hailing platforms that incorporate more travel and user criteria [ 64 ]. Only one platform (i.e., TwoGo) was found to use an intelligent technology to analyze rides from all users to find the best fit for each user, and factor in real-time traffic data to calculate precise routes and arrival times.
Several incentives are used to promote ride-sharing, such as toll cost reduction [ 6 ], High Occupancy Vehicle (HOV) lanes in US [ 18 , 43 ], free or discounted parking access in public or private areas [ 51 , 88 ], public transport ticket discounts and collection of points that may be redeemed in companies that collaborate with ride-sharing services [ 8 , 51 ]. For example, Autostrade [ 6 ] carpooling with at least 4 passengers pays 0.50 euros toll, instead of 1.70 euros, from Monday to Friday; or GoCarma [ 43 ] that uses Bluetooth to automatically detect if there are at least 2 people in the car so as to qualify for an HOV toll discount.
User factors
Several studies in the literature focused on the exploration of users’ factors when using ride-sharing services (Table (Table1). 1 ). User factors may be associated in a positive or negative way with ride-sharing. In the latter case they may also be considered as barriers to ride-sharing implementation. The literature shows that the strongest identified barriers for ride-sharing users are mainly psychological [ 1 , 52 , 91 ] with the most common ones being personal security, comfort and privacy [ 1 , 52 , 91 ]. This section summarizes these findings and identifies the factors that are associated with the likelihood of ride-sharing for passengers and drivers. The following subsections summarize factors and results for ride-sharing passengers and drivers, and Table Table4 4 summarizes the studies and factors that are associated with the likelihood of ride-sharing.
Summary of user factors associated with the likelihood to ride-share
* Incentives: Free parking, use of HOV lanes, ride-sharing services available in a company or University
Ride-sharing passengers
Ride-sharing research on passengers’ behavior tend to refer to identical factors, which can be grouped in various ways; for example, Buliung et al. [ 13 ] classified ride-sharing factors as socio-demographic, spatial, temporal, automobile availability, and attitudinal, whereas Neoh et al. [ 73 ] grouped them into internal (i.e., individual characteristics and reasons to ride-share) and external (i.e., policy measures to facilitate ride-sharing, location-based factors). Our study adapts Neoh et al. [ 73 ] approach with some minor adjustments, and groups factors into sociodemographic, location and system factors. Sociodemographic factors are factors associated with the passenger’s demographic and socioeconomic status, and beliefs such as environmental concerns; location factors refer to spatial characteristics of travelling, such as trip distance and time, and area density. System factors refer to the ride-sharing service environment, such as policies and incentives; system factors may be adjusted by the ride-sharing service provider. The factors per study that are reported in Table Table4 4 were found to be statistically significant.
Several studies (e.g., [ 13 , 14 ]) concluded that socio-demographic characteristics, such as marital status, gender, age and educational level are not significant; whereas behavioral factors are. Other studies, however, concluded that some socio-demographic characteristics, such as age, income and age, are associated to ride-sharing [ 28 ]. Females, younger workers, and those who live with others were found to be more likely to ride-share [ 58 , 73 ]. Delhomme and Gheorghiu [ 31 ] found that women are almost three times more likely to use ride-sharing compared to men, while Lee [ 58 ] concluded that females who are younger than 55 years old are more likely to ride-share than older males. However, Ciari and Axhausen [ 25 ] concluded that female individuals in Switzerland are less attracted to ride-sharing, maybe for security concerns.
Education level was not a significant factor in the majority of the studies, while just a few found that education is related to ride-sharing, and more specifically, users that do not hold a degree are more likely to ride-share [ 58 ]. In terms of marital status, passengers between the ages of 25 and 34 were more likely to make commute trips (96%) versus non-commute trips (80%) by using ride-sharing services, and they were more likely to be single or married without children [ 92 ]. Specifically, a propensity towards ride-sharing is demonstrated among unmarried and divorced commuters.
The user or household income was not associated with increased likelihood to ride-share for the majority of the studies. Monchambert [ 65 ] used discrete mixed logit models to estimate the probability of mode choice and found that the ride-share value of travel time correlates with socio-economic variables. In other words, wealthier individuals seem to be willing to pay more to save travel time. Also, Ciari and Axhausen [ 25 ] concluded that persons with higher income and shorter trips tend to have a higher value of travel time savings, and thus, prefer ride-sharing compared to car, suggesting that it is also preferred to the other available modes.
Recent data, however, from the National Household Travel Survey in the US [ 72 ] indicated that ride-sharing passengers that have generally lower incomes, and minorities (typically Hispanics and African Americans) tend to ride-share more than other racial and ethnic groups [ 83 ]. Similarly, other studies concluded that lower income passengers are more likely to ride-share [ 14 ] or that ride-sharing maintains mobility for low-income passengers [ 4 ]. Ferguson [ 37 ] found that income has only an indirect impact on the choice to ride-share in lower income households, as income influences auto ownership and use. Higher vehicle ownership does not favor the utilization of ride-sharing services [ 37 ]; though, a study in China showed that the ride-sharing adoption rate was similar between households with cars and those without [ 100 ].
A strong relation was found between having ride-sharers among family/friends and colleagues, and engaging in ride-sharing [ 14 , 33 ]. The tendency to adopt ride-sharing services is higher for multi-person households and households having more licensed drivers than vehicles [ 58 ]. The presence of children, elderly persons, or both, in the household is likely to have a negative effect on the adoption and frequency of use.
Findings on sociodemographic factors show that while these may be limited in their effect, when combined with system factors they may reveal a more stable status. As Olsson et al. [ 75 ] stated, other factors become more important for mode choice and are the focus of transport research.
In terms of trip characteristics, commuters who travel longer distances were found to be more willing to use ride-sharing services [ 58 ]. However, the in-vehicle time for public transport services was found to have a marginal impact on passengers’ propensity toward ride-sharing [ 64 ]. Based on transport mode shares for US, Australia, UK and Canada, there is some evidence that in the absence of adequate public transport services, commuters opt for ride-sharing [ 11 , 33 , 42 , 58 , 61 , 104 ]. The purpose of the trip also plays a role, as ride-sharing is more likely to be used for work trips [ 24 , 61 ] and for persons that have a full working or studying day. People who work full time and with flexible schedules are more likely than other workers and non-workers to adopt and frequently use ride-sharing.
Travel cost and travel time are associated with ride-sharing and are two of the main reasons for participating in ride-sharing services [ 14 , 20 , 61 , 73 , 105 ]. Commuters who travel short distances of a mile or two are less interested in dynamic ride-sharing than those who travel further because for short distances, the time required to arrange a ride is excessive [ 30 ]. For student passengers the desire to save on gasoline costs, followed by a preference to do other things during travelling, the reduced stress and travel time savings, increase the likelihood to ride-share [ 92 ].
Although, density employment centers in suburban areas were found to benefit public transit and nonmotorized modes more than ride-sharing [ 37 ], building and population density seem to increase the likelihood of ride-sharing [ 31 , 58 , 73 ].
Using microsimulation, Dubernet et al. [ 34 ] found that behavioral factors are the most limiting factor of ride-sharing; behavioral barriers, attitudes and perceptions were found to affect more the decision to use ride-sharing services than socio-demographics [ 97 ]. Research showed that enjoying travel with others, environmental considerations [ 31 , 42 ] and socializing [ 39 ] affect at a significant level the choice to use ride-sharing services [ 61 ]. Other important factors for ride-sharing include security and trust [ 28 , 48 ].
Several incentives have been provided occasionally to ride-sharing passengers, including reward programs that may provide money or gift cards for ride-sharing, access to green zones, (i.e., commuter rewards programmes that may provide money or gift cards for ride-sharing), etc. Such incentives showed that may attract ride-sharing participants from either single occupancy vehicles and/or public transit [ 28 , 75 , 82 ].
Although, the most prevailing results are summarized in this section, the literature review showed that factors affecting travellers to use ride-sharing services in some cases may differ among studies. For example, “income” is associated negatively [ 4 , 13 , 14 , 82 , 91 ] and positively [ 73 , 103 ] with ride-sharing; “education” is associated negatively [ 58 ] and positively [ 73 ]; and “age” is associated negatively [ 58 , 73 ] and positively [ 91 ]. Similarly, the location factor “area density” is associated negatively [ 4 ] and positively [ 31 , 58 , 73 ] with ride-sharing. Readers are strongly recommended to follow-up the study they are interested in, since different methods and statistics may have been used; thus, resulting to different factor results (i.e., not statistically significant) for specific cases.
Ride-sharing drivers
Ride-sharing users can offer a ride as a driver or request transport as a passenger. Drivers provide ride-sharing services and thus they are considered independent private entities. This approach is different from most traditional forms of passenger transport, where an authority or company owns vehicles and/or employs drivers. If the driver and the passenger agree on the proposed arrangement, the driver picks up the passenger at the agreed time and location.
Several surveys have been conducted to study the passenger’s behavior, however, few of these focused on the driver’s behavior. Respondents with a preference for driving only accounted nearly for 50% [ 13 ]. Approximately, 33% of the respondents stated that they would rather not offer a ride in the evening (18:00–24:00), while more than 52% of passengers stated that they would not accept a ride in the evening (18:00–24:00) [ 28 ]. Drivers indicated that departure time flexibility is the primary reason for driving instead of riding, as the highest share of them (74%) agrees that reducing flexibility is among reasons not offering a ride [ 33 ]. It is worth mentioning that other studies concluded that younger and older people tend to be passengers, while middle-aged people tend to be drivers [ 92 ]. Drivers appear to avoid ride-sharing as passengers as they feel anxious and stressed (usually studied as ‘locus of control’) when delegating the driving task to others [ 73 , 97 ].
For drivers, a passenger’s profile is an important factor. Passengers, whose social network profile appears unattractive, incomplete or has low rating, have a lower chance of finding a ride offer [ 92 ]. Therefore, it becomes essential for potential passengers to have a trustworthy profile, including a picture, profile details, and contact information on a social network (e.g., LinkedIn, Facebook or Ride-sharing application). Similarly, the driver’s profile plays the most significant role in one’s decision to accept an offered ride [ 91 ]. This challenge has been largely addressed through the development of increasingly sophisticated ride-matching platforms. Another factor that differs between passengers and drivers is the payment method. Drivers prefer to receive the reimbursement in cash but passengers prefer to pay through a mobile payment platform, revealing drivers’ concerns over the certainty of the reimbursement [ 39 ].
Following the results of ride-sharing definitions, online platforms and user factors, this section synthesizes findings with barriers identified in literature (Table (Table1). 1 ). Factors that prevent the successful implementation of ride-sharing services are grouped into economic, business, technological, behavioral and regulatory, to stimulate a discussion for implementing successful ride-sharing services.
Economic barriers
Cost and convenience are important factors associated with the intention to start ride-sharing [ 1 ]. Time costs include the time that is required to set up an account in the ride-sharing application/website, the time it takes to find and book a ride through the application and the waiting time to join a ride. Booking time be insignificant when interurban rides are arranged but for daily rides this cost may seem significant to potential users [ 1 ]. Booking trips in advance is not convenient and may not suit to users that prefer instant arrangements and flexibility in their schedule [ 48 ]. Similarly, ride-sharing drivers are unwilling to experience more than 5–10 min delay in order to pick-up and drop-off passengers [ 64 ], suggesting time delay is a significant factor for joining a ride-sharing service as a driver. Ride-sharing platforms should try to minimize the time that it takes for different users to register, book and wait for a ride. Different users (e.g., based on trip purpose) show different sensitivity to waiting time, and the time range that each user may accepts should be investigated. The outcome of such research should be incorporated in the matching algorithm of the ride-sharing platform to address the needs for each user group. In this way it will be more likely these users to use more often ride-sharing services.
Also, fuel prices and fuel efficiency improvements for internal combustion engine vehicles seem to affect ride-sharing; in 1990s the decline in oil prices matched the decline in ride-sharing [ 37 ] from 20 to 13% [ 20 ]. Personal travel is less sensitive to gasoline price fluctuations than vehicular travel is, due to the ready availability of empty seats, which means that increased fuel prices will likely reduce vehicles on the roads, but not passenger travel. As fuel prices are not expected to decrease significantly in the short term and vehicle fuel efficiency improves in the meantime, ride-sharing may offer personal travelling until a cheaper alternative fuel replaces internal combustion engine vehicles [ 48 ].
Business barriers
Ride-sharing platforms may integrate different business models to generate revenue. The two most used models are a commission fee based on the overall ride cost or a flat rate fee. The third alternative does not integrate any direct fee, and may rely solely on revenues from advertisements on the platform. In our data, only 7% of the platforms appear to charge a direct fee by either way [ 8 , 91 ]. This implies that 26 platforms are neither set up as enterprises that aim to be economically sustainable in the future, nor they focus on growing their user base, thus they do not currently generate any profit. The level of success of these practices is questionable as several ride-sharing platforms stopped operating as outlined in Sect. 3.2 or they were transformed to ride-hailing services (e.g., Zimride became Lyft).
A solution proposed by Olsson et al. [ 75 ] to integrate ride-sharing platforms into the Mobility as a Service (MaaS) concept, where users shift from privately owned vehicles to monthly subscriptions for mobility services. Another recommendation is to integrate ride-sharing services with public transport in locations, where access to public transport is limited or frequency is low. Research showed that in these locations the likelihood to use ride-sharing services increases [ 64 , 102 ]. In this way ride-sharing services should be partially subsidized to transfer travellers to public transport hubs.
Kelly [ 54 ] proposed to add ride-sharing to the list of modalities (currently public transit or vanpools) that are eligible for tax benefits. In this case the largest source of funds should come from the Regional Transportation Boards and state and federal agencies (in the case of US) that have as their mandate the construction and operation of transport systems.
Business models should focus on the community goals (e.g., reduce single occupancy vehicles, provide last mile rides) and users’ needs for each location. More experimentation is needed for designing and testing different types of incentives for different travel activities (work and non-work) to customize solutions per case [ 64 , 75 ]. Incentives and subsidies should take into consideration the ride-sharing impacts to avoid under-subsidizing public transport modes or modes that generate less emissions (i.e., bike and micromobility). Unwanted barriers to ride-sharing such as taxation and insurance issues should be regulated to provide trust and confidence to its users. Analogously, ride-sharing parking and park and ride facilities should be carefully planned since they may generate additional traffic [ 97 ].
Technological barriers
Ride-sharing platforms are supported by a mobile application or/and website to match potential drivers with passengers. The level of sophistication of the matching algorithm affects the ride-sharing participation either for existing or potential users. Also, even if drivers and passengers can be successfully matched, little is known about each individual participant regarding their driving history, annoying habits to co-passengers while ride-sharing (e.g., eating, smoking), criminal record, etc. [ 1 ]. People are significantly less willing to share a ride with strangers than with direct or indirect friends [ 102 , 103 ]. The majority of the ride-sharing platforms rely on the user’s feedback to provide a secure ride to their participants. Therefore, imprecise or imperfect information to participants may hinder significantly ride-sharing.
A solution to this barrier could be the development of a greater ride-sharing database with collaborating capabilities with other databases, that can aggregate user data to increase the probability of matching up a driver and a passenger. As such, the integration of users’ information with other criminal or identification databases is an important step towards encouraging greater ride-sharing participation. Other social networking platforms like Google and Facebook can be incorporated in the ride-sharing platform to add extra credibility, and enable them as platforms to match ride-share users [ 57 ]. People with active profiles on social networking websites are less affected by trust issues when it comes to sharing a ride with people they have never met [ 39 ].
However, there are several emerging ethical concerns in big data analytics applications in public transport systems and ethical frameworks are required to provide a careful balance of benefits and risks driven by disruptive technologies [ 21 ]. A range of ethical impacts are identified relative to the implementation of data-driven transport systems, that constitute barriers to the development of smart mobility. Including but not limited to: trust, surveillance, privacy (including transparency, consent and control), free will, personal data ownership, data-driven social discrimination and equity [ 59 ]. The massive amount of information collected about people, privacy and security are reported as the main concern [ 77 ]. Concerning transport network companies, such as Uber or Lyft, significant evidence of racial and gender discrimination was documented in various experiments [ 41 ]. Additionally, elderly, people with low education and/or physical or mental problems are facing difficulties adopting emerging technologies, and may be excluded from a data-driven transportation system [ 21 ]. A recent study [ 88 ] noted the importance of social equity in smart cities and the need to address elderly people needs across various dimensions, including transportation.
Additionally, the outdated algorithms that are used in traditional ride-sharing platforms make difficult any last-minute schedule changes that a user would like to make [ 38 ]. One of the main reasons that ride-sharing, has fallen off dramatically over the past decade, at least in the US, is largely due to the inflexible nature of pre-arranged ride-sharing [ 68 ]. The maturing of internet adoption and more sophisticated algorithms allow internet-based ride-sharing platforms to overcome problems with schedule inflexibility [ 73 ]. Correia et al. [ 28 ] proposed that for managing schedule variations, a ride-sharing platform can be set to manage both traditional stable groups and a dynamic ride matching service. Dynamic ride matching services have proved to be very ineffective when applied independently; their success, however, strongly depends on the participants’ willingness to share a ride with a possible stranger [ 28 , 102 ].
Despite multiple algorithmic improvements for ride-sharing, including real-time en-route planning, the mainstream ride-sharing applications are almost all trip-based, with specified fixed origin/destination pairs and thus low flexibility for destination choices. Frequently cited barriers to ride-sharing formation and use include: rigid scheduling and lack of matches between drivers and travellers [ 49 , 66 ]. A gap that can be bridged by advanced software and algorithms, to provide enhanced matching. A new ride-sharing algorithm, called collaborative activity-based ride-sharing to address the barriers of trust and flexibility in ride-sharing was proposed [ 103 ], to increase favorable rides without sacrificing more detour time, which potentially encourages public acceptance of ride-sharing.
Lastly, acknowledgment of users' preferences will help service providers to build customized services to meet their travelling and behavioral needs. For example, older adults may require more space for wheelchairs [ 58 ] or students for special equipment, such as cameras or drawing equipment. Future research should focus on the effectiveness of matching algorithms by integrating more travelling and personal criteria to transform ride-sharing into a safe and entertaining mode.
Other major barriers that can be faced by enhanced mobile applications, include lack of information [ 4 ], belief that “nobody is going my way” [ 92 ], and aversion to handle direct money transactions [ 30 ].
Behavioral barriers
Behavioral barriers have found to affect more the decision to use ride-sharing services than socio-demographics [ 97 ]. Research showed that enjoying travel with others, environmental and social consideration, trust and security affect at a significant level the choice to use ride-sharing services [ 48 , 61 ]. Participation in activities such as reading a book, texting, or surfing the internet on their smartphone during the commute may be another influential factor relating to ride-sharing demand [ 92 ].
Ride-sharing systems that fail to provide the conditions for secure travelling pose barriers to a successful implementation of a ride-sharing system. The feeling of unsecure travelling may grow either by not sharing user profiles, user matching not based on user criteria, or lack of mobile applications that enhance security, for example not sharing your location. Research showed that the more information shared by users (i.e., time and place of the ride and information on interests and preferences), the more likely a matched ride could occur [ 65 ]. Poor flexibility is associate negatively with ride-sharing [ 28 ] and is also the main reason against sharing rides as passenger, with 66% supporting this argument [ 33 ]. Lee [ 58 ] suggests that having work schedule flexibility is associated with those who are more likely to use a non-rideshare mode, and most likely to telecommute, than to rideshare.
Also, ride-sharing services are more likely to be successful when an organization, resembling small communities, such as a company or a university provides these services in its premises [ 92 ]. Commuting with colleagues is probable increasing the levels of security, and provides an opportunity for socializing by sharing common topics of discussion.
Sharing roles, as opposed to drive-only or travel-only, has shown to affect success of ride-sharing, and appears to be the preferred approach by users, as they look to acquire both the economic advantages of driving some of the time, and the perceived psychological/comfort benefit of being a passenger [ 60 ].
As mentioned, and presented, the literature offers mixed findings on the relationship between demographic, behavioral characteristics and ride-sharing. Some relationships might exist between ride-sharing, specific users and their characteristics. However, after a specific user group adopts ride-sharing services, the practice may vary greatly within the user group, hence more complex relationships may ultimately describe the interactions that lead to such decisions [ 13 ]. A further analysis, will be able to explore the user characteristics for specific locations and travel purposes, and reveal clusters of users having similar characteristics, behavior and needs, to customize ride-sharing services, and to target specific users.
Regulatory barriers
The European Union transport policy aims to ensure the movement of people and goods throughout the EU by means of integrated networks using all modes of transport (road, rail, water and air). However, within the existing transport legislation a common directive, among EU countries, for ride-sharing is not shared [ 36 ]. To best understand the ride-sharing, it becomes essential to understand the regulatory environment in which the services operate. The majority of EU-Members do not define or regulate ride-sharing; however, only 5 out of the 28 countries (i.e., France, Germany, the Netherlands, Spain and Sweden) provide a ride-sharing definition for non-commercial reasons (i.e., use of a motor vehicle with a driver and one or more passengers as part of a journey; the driver performs the trip on their own account and no remuneration is involved except the costs for the driver). Similarly, in US and Canada ride-sharing is not regulated as it operates on a non-profit basis. Setting an adequate legislative framework for innovative transport solutions is a prerequisite for their successful integration and implementation in existing transport systems. For example, countries that failed to set such a legislative framework for ride-hailing services (e.g., Uber in Denmark and Bulgaria) or for electric-scooters (e.g., Hive in Greece) were forced to cease the operation of these companies.
Exploring users’ perceptions to develop a ride-sharing system
Limited information exists on the trip purpose of ride-sharing users, compared to the exploration of factors for passengers. Only a few studies in the literature review focused on travelling for work or educational purposes (i.e., travel to campus/university), while leisure/recreation and shopping trips are usually not considered. Similarly, Wilkowska et al. [ 107 ] suggested that little analysis is performed on trip purposes other than work. Teal [ 94 ] identified three types of ride-share users based on how they ride-share: (1) Household (travel only with household members), (2) External (travel with unknown individuals), and (3) Passengers. Gheorghiu and Delhomme [ 42 ] identified ride-sharing trips for work, children (picking up and/or taking other children to school and for children’s leisure activities), leisure, and shopping. The same study concluded that the longest ride-sharing trips were attributed to work purposes, the shortest to shopping, while leisure and children-related trips had approximately the same reported average length. Vanoutrive et al. [ 97 ] investigated the influential factors for pre-organized ride-sharing and found that different travel purposes (e.g., to home versus to workplace) bounded with their corresponding travel directions, yielded different ride-sharing rates. Also, the spatial distribution of travel demands and social networks affected matching rates [ 103 ].
Aforementioned barriers show that an understanding of the users’ behavior has the potential to provide insights and result to customized user recommendations for developing a successful ride-sharing services. A grouping of ride-sharing users is suggested on the basis of trip purpose, based on literature findings as presented above. Four user types are considered to cover the majority of trip activities, thus the majority of users:
- Household work user (Trip to work with at least one person from the same household),
- Solo work user (Trip to work with unrelated individuals),
- University and college user (Trip for educational purposes with or w/o unrelated individuals)
- Entertainment/shopping user (Trip for recreation and entertainment purposes (shopping is included here) with or w/o unrelated individuals).
Work users are divided into household and solo driving as several studies have focused on ride-sharing and commuting to work [ 30 , 42 , 97 ], and recent data suggested that household ride-sharing likely represent the largest share of arrangements [ 66 ]. Solo drivers appear not to be so favorable about using ride-sharing services [ 1 ], thus, the research findings (i.e., increased work-based ride-sharing shares and low penetration upon solo drivers), stress the need to consider and study this user type separately in order to design and form customized initiatives to promote ride-sharing. Ride-sharing should be also considered for recreation/entertainment activities, since some of these activities are fixed in terms of time, day and place (e.g., grocery shopping, training)”. The user types apply to both passengers and drivers, as there is no evidence that role preferences (i.e., passenger or driver) are associated with specific trip purposes.
Finally, further research to accommodate the needs of passengers that may combine ride-sharing with public transport (i.e., bus, rail, metro) is required to explore and determine the factors that affect use of ride-sharing. Apart from factors discussed in earlier sections, other factors may be considered, such as travelling time when using ride-sharing with public transport, and travel preferences (e.g., seat preferences, accessibility needs) when travelling with public transport.
Practical implications
Our review findings are used to summarize and propose practical recommendations to service providers to enhance the popularity of ride-sharing systems; thus, increase ride-sharing demand. Economic factors, including time, appear to affect the willingness of users to use ride-sharing systems. The time to register in a platform and the process to find and book a ride either instantly or in advance, and the economic benefits of using ride-sharing are dominant factors for potential users. Ride-sharing service providers should develop and release an easy-to-use mobile application to support their services, which will be linked to a web-based platform to provide access for all travellers complying with local accessibility regulations; in this way a one-time registration will be required. Pre-booking rides is also perceived inconvenient by some users [ 48 ], which prohibit them from ride-sharing. Real-time ride-sharing [ 2 ] which brings together travellers with similar itineraries and time schedules on short-notice should be considered and adopted. Minimization of drop-off/pick up locations through optimization of meeting points and routes is also proposed to relax time constraints for potential passengers that appear to be sensitive to time delay.
Although, the studied ride-sharing systems do not offer financial benefits for the driver and the passengers, incentives are essential towards attracting more users. The service provider through the application should provide various financial incentives to increase the number of people who are eager to provide ride-sharing services (i.e., drivers); such incentives may include booking of parking spots, parking discounts and/or free passes in parking lots. Additionally, ride-sharing incentive programs for passengers may be developed to integrate cash or/and reward incentives. Direct cash incentives may be offered by companies to their employees in exchange for their parking space at work, while public authorities may also provide short-term cash incentives to new ride-sharing users. Georgia’s Cash for Commuters program offered a $3 USD per day incentive per new user for 90-days to try ride-sharing. It was found that 57% continued to ride-share 18 to 21 months after the initial incentive period [ 86 ]. Awarding points for ride-sharing trips that may redeemed in collaborative green-businesses and public transport schemes will also attract more users and highlight the relationship between ride-sharing and sustainability.
Marketing and promotion of ride-sharing services and their benefits will likely introduce the concept of ride-sharing to new users. The mobile applications and platforms may highlight the benefits to environment when travelling with others, while also disclosing that this mobility solution complies with national regulations related to COVID-19 passenger restrictions. Mobile applications, in the trip booking page, should provide a comparison of carbon dioxide and cost savings between private vehicle and ride-sharing to provide instant comparisons.
Mobility by public transport, railway, airplanes and ferries has been characterized as of high-risk activity that enables COVID-19 transmission, due to limited space that users have to share. As a result, ridership in public transport systems has decreased, while use of private vehicles has increased [ 64 ]. However, the share of travellers before and after the first COVID-19 lockdown period remained approximately constant. Ride-sharing provides a transport alternative that has the potential to provide mobility in a safe and controlled environment, that public transport may not be capable of guarantying. For example, the mobile application may ask users to provide their vaccine certificate in order to use the service.
Enhancing security by using several methods should be a priority for all ride-sharing services, since it affects the willingness of users to ride-share [ 48 , 61 ]. The option to users to share their location in real-time with their contacts or other ride-sharing users should be implemented in the mobile application. A rating system, for both passengers and drivers, should be developed to provide feedback for all ride-sharing users. Such a mechanism will allow users to judge whether to accept or decline the offered ride, based on their perception. In this way, users may feel in control of their ride, and enjoying a sense of security. A list of regulations to ensure a safe and secure ride should be also provided to potential travellers, including abusive language, physical contact, unsafe driving, etc. Finally, an alarm button in the application could be added to notify the service provider in case of emergency by recording and forwarding the location and travellers’ information at the time of the incident.
Limitations and strengths
The present systematic literature review focused on ride-sharing online platforms, factors and barriers, and did not include impacts or ride-matching algorithms. While these aspects are equally significant to the design of a successful ride-sharing service, the present study was conducted by recognizing that: (a) studies in the field of optimization and matching algorithm should be studied separately to focus on programming and technology aspects, and (b) studies on impacts of innovative transport systems, such as for ride-sharing, are challenging since the methods and tools to perform exhaustive life cycle assessments are limited.
We performed an extensive literature review that included 56 publications, while for 32 of them the factors that affect ride-sharing were extracted. Our results may help ride-sharing providers and transport planners to design and implemented successful ride-sharing services. However, the study suffers from certain limitations. The exclusion of grey literature and project reports could have been a limiting factor, in that it is possible that significant new findings might have been overlooked related to ride-sharing services. However, it should be noted that official websites of identified ride-sharing platforms were reviewed to collect specific data per platform. Also, the small number of ride-sharing platforms that was identified might led to not sufficient interpretation of the situation. In this aspect the informal character of ride-sharing should be considered, which leads to platforms that are not recorded or are not possible to target them as they operate in local social media and languages. Similarly, exploring regulatory barriers per country is hindered by language restrictions; likely local governmental documents may contain more information. Aspects of automated vehicles in ride-sharing were not considered either, which is an emerging field of discussion. Whether automated vehicles will be used for ride-sharing, as privately owned cars or in the form of service by ride-hailing services (e.g., Uber or Lyft) remains unknown [ 75 ]. The vague definition of ride-sharing might has also limited our findings. We are aware that there exist other forms of ride-sharing such as vanpooling, hitchhiking or slugging, that have not been considered.
Acknowledging these limitations, we do believe that this review provides important insights about official online platforms, what barriers exist, and who is likely to ride-share. Considering these aspects, transportation planners could be assisted and guided when planning a ride-sharing service, and choose more wisely which parameters should be customized and what users should target for, to implement a successful ride-sharing service.
The systematic literature review of ride-sharing studies allowed us to have a comprehensive overview of academic publications dealing with ride-sharing platforms, user factors and barriers. These publications were selected using keywords that refer to ride-sharing, carpooling, barriers and factors. The systematic and comprehensive approach in this review adds strength to the research of economic, technological, business, behavioral and regulatory barriers on ride-sharing operation and success. Improving ride-sharing online platforms and applications and providing more features to users to customize their ride will likely generate positive impacts for ride-sharing.
Findings from this study provide insights and aspire to provide a comprehensive understanding of barriers and factors in decision-making process about ride-sharing. These findings could have important implications for urban and transport planners and policy makers to implement tailored solutions to users’ needs and socio-demographic characteristics. The results can be used as input to transport planning, policy-making and ride-sharing providers: revealing the potential barriers, enabling user-centered design environment, and providing recommendations for a successful ride-sharing service.
It appears to be a norm for location and system factors that affect users’ willingness to ride-share, however in some cases mixed findings exist between socio-demographic factors and ride-sharing. A limitation in existing research is the time of the study or the absence of studies before and after implementing a ride-sharing service. After a specific user group adopts ride-sharing, the practice may vary greatly within this user group, resulting to more complex relationships [ 14 ]. An ex-post evaluation of new introduced ride-sharing services has the potential to study and capture these relationships.
Additionally, it becomes important to examine the factors related to solo driving in each society for all travel activities and design customized interventions to target the behavior of solo drivers. Initiatives that aim to encourage solo drivers to start ride-sharing, could address some of the perceptions around the comfort and the convenience of driving alone versus ride-sharing. Public transport, walking, and biking are strong alternatives for passengers that avoid travelling alone, reducing the potential market for ride-sharing. For this reason, the estimates of participation rates must be considered case-specific, and decision makers have to consider whether to open and market the service to all or to focus on solo drivers. Continuous collection of user feedback through the ride-sharing platforms, and periodic reports from ride-sharing users is an important aspect in developing and improving ride-sharing programs.
The provision of ride-sharing policy is a rather interesting and complicated task that should take into account local and regional characteristics (i.e., demographics, economy, users, geography, transport). Further research is required to evaluate the relationship that exist between users and ride-sharing for existing (i.e., revealed experience) and potential (i.e., stated preference) users. Future directions will be towards exploring the user factors related to specific user-activities and ride-sharing. Additional system factors (e.g., ride safety, information regarding the vehicle condition, feedback method, etc.) should be explored to assess their impact on using ride-sharing services, while the most significant ones should be further investigated (e.g., to explore ride safety in terms of user identification method, sharing the ride online and payment method, etc.) to provide customized criteria that may be implemented within ride-sharing algorithms to optimize user-matching and experience.
Acknowledgements
The authors would like to thank the anonymous reviewers for their comments and suggestions.
Authors' contributions
LM developed the study methodology, collected the data for ride-sharing systems, and users, analyzed the data and made a major contribution to writing the manuscript. AK collected the data for ride-sharing systems, analyzed the data, and corrected the manuscript. GA analyzed the data for ride-sharing definitions and corrected the manuscript. All authors read and approved the final manuscript.
This research was funded by the Shift2Rail Joint Undertaking under the European Union’s Horizon 2020 research and innovation programme under Grant Agreement No. 881825.
Availability of data and materials
Declarations.
The authors declare that they have no competing interests.
Publisher's Note
Springer Nature remains neutral with regard to jurisdictional claims in published maps and institutional affiliations.
Contributor Information
Lambros Mitropoulos, Email: rg.htrec@timl .
Annie Kortsari, Email: rg.htrec@stroka .
Georgia Ayfantopoulou, Email: rg.htrec@aeg .
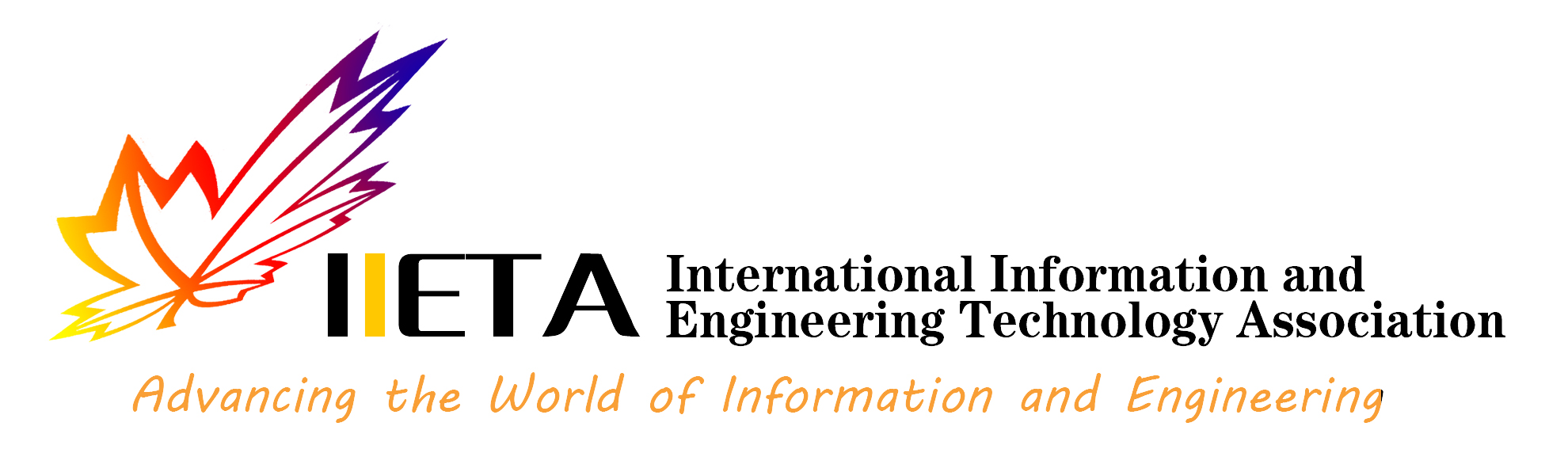
Search form
Implementation of a secure ride-sharing dapp using smart contracts on ethereum blockchain.
Sathya A. Renu * | Barnali Gupta Banik
© 2021 IIETA. This article is published by IIETA and is licensed under the CC BY 4.0 license ( http://creativecommons.org/licenses/by/4.0/ ).
OPEN ACCESS
The idea of sharing economy gives rise to unique ideas and develops innovative businesses. This article aims to relate the smart city concept by introducing the smart transport system and explores the opportunities of adopting blockchain technology in ride-sharing services. Blockchain technology is a distributed, decentralized public ledger that allows peer-to-peer transactions in a secured way without any third party. This paper proposes a blockchain-based framework from the existing centralized framework for a ride-sharing service and implements the same as a decentralized application (DApp) based on smart contracts on Ethereum Blockchain. Using smart contracts facilitate the users with automated transactions, removes the intermediaries, and enables various activities to be carried out safely and securely. Implementation of smart contracts is done using the Solidity programming language. This DApp uses the min matching algorithm to match riders requesting rideshare to save total travel distance. With the overwhelming growth in the usage of cryptocurrencies, smart contracts usage in applications as proposed in this paper can transform the sharing economy.
blockchain, smart city, smart contract, DApp, Ethereum, cryptocurrency, shared economy, greedy algorithm, graph theory
1.1 Background
For the past few decades, Blockchain is revolutionizing every major industry with distinguishing properties and not just with the financial sectors. Researchers are trying to explore the problems where blockchain can fit in and provide exceptional services. Crowdfunding, Insurance, Healthcare, Governance, Energy are few major domains where blockchain is already progressing. For the past few years sharing economy has seen exponential growth [1, 2]. For example, Ola, Uber, Airbnb, Lyft will witness the same [3]. It enabled users to share their assets with others who do not possess them and require them. Sharing of resources will reduce wastes and promotes a lot of people to participate. However, most transactions of sharing economy applications happen on a centralized platform with a third party. The transaction can be an information exchange or payments. Centralized mode of transactions is susceptible to threats like password hacks, misusing of customer data and has increased cost and efforts in maintaining it. One of the best contributors to sharing economy can be shared transport services i.e. taxi rides. Currently, Uber, Ola, and Lyft are leading their way in ride-sharing systems. Almost all ridesharing applications use a centralized approach. But, having a centralized system in ride-sharing services has many downsides like surge fee, convenience fee, GST, payment gateway charges, etc. Thus there’s a need for better approaches. One such solution to overcome the downside of the centralized approach is a decentralized, peer-to-peer approach in the ride-sharing process.
This paper aims to provide a deeper understanding of blockchain technology and give considerations of how to integrate it into their business strategies. The paper humbly claims two-fold contribution:
- A blockchain-based ride-sharing architecture is proposed for safe and secure traveling.
- A prototype of the proposed architecture is developed as a Decentralized Application (DApp) on the Ethereum blockchain.
1.2 Blockchain
Blockchain is a peer-to-peer, distributed ledger technology that progressed from Bitcoin [4], a cryptocurrency developed by Satoshi Nakamoto in 2008. The overwhelming success of Blockchain is due to its distinct properties like distributed, decentralized, immutable, secured, and shared. Every transaction in the blockchain is recorded and anyone in the network can access and verify the transactions. As it follows a peer-to-peer(P2P) model blockchain eliminates the need for intermediaries. Adapting Blockchain in a smart city can lead to various benefits like security, transparency, trust-free, and automation. A smart city aims in improving the standard of living and creating a sustainable environment through Information and Communication Technology (ICT) [5]. The conjunction of Smart city and Blockchain can improve the performance, efficiency, security, and smartness of the environment. The most commonly used distributed ledger technology is Blockchain. It organizes the data into several blocks interconnected with each other. Blockchain systems are of three different types: public, private, and consortium [6]. A public blockchain is permission-less and anyone being in the network can see and make transactions, validate them and manage the shared ledger. Bitcoin and Ethereum are examples of the public blockchain. Whereas private blockchain is a decentralized yet centralized network possessed by a single entity. A consortium blockchain is also a private network that handles cross-organizational transactions.
1.3 Ethereum
Ethereum is a public and permissionless blockchain introduced by Vitalik Buterin. Ethereum is built with Turing complete programming language and overcomes several limitations of Bitcoin’s scripting language difficulty. Transactions on Ethereum are done by Smart Contracts. Smart contracts are nothing but executing some set of predefined rules when certain conditions are met [7]. Transactions in Ethereum are cryptographically signed instructions. The fee for these transactions is paid as Ether. Ether is the built-in currency of Ethereum. Apart from payment Ether is also used as a pricing instrument for running DApps in the system. Ethereum defines cost for every computation in the form of Gas. These instructions are executed by Ethereum Virtual Machine (EVM) running in every node of the network. Some of the potential domains of Ethereum are Insurance, File Storage, Market Predictions, FinTech, etc. However, one of the significant use-cases of Ethereum is Decentralized applications (DApps) which will be discussed in depth in this paper.
1.4 Smart contract
A smart contract based on the Ethereum blockchain is a cryptographic bundle that takes and processes inputs, writes outputs, and stores information. The public can access the output only when some predefined conditions are met [7]. Kevin Delmolino et al., have explained a step-by-step procedure of implementing a smart contract in their Safe Smart Contract paper [8]. The smart contracts generate bytecode at compilation and are stored in blockchain. These byte codes are run by EVM which is running in each node of the network [9]. Solidity is the most common programming language in creating smart contracts. Solidity is derived from C++, JavaScript, and Python. Smart contracts enable the solidity code to run as decentralized applications on a blockchain system. Smart contracts are considered the backbone of decentralized applications [10].
1.5 Decentralized Applications (DApp)
DApp is a decentralized application hosted on a P2P blockchain network. Ethereum hosted DApps on different domains like Insurance, Energy, Finance, Health, and many more. But, many of the DApps are only partially decentralized. As per [11], the properties of DApp are characterized as,
- Open Source: DApp codes are open source and audit from a third party is possible.
- No single-point Failure: Due to its decentralized nature no central point of failure.
- Consensus: To enable transparency consensus among the nodes is inevitable.
- Currency Support: Internal cryptocurrency is the major factor that runs the ecosystem of any decentralized application.
Key differences between centralized web applications and decentralized blockchain applications are shown in Table 1 below.
Table 1. Web App Vs DApp
1.5.1 DApp architecture
DApp is two-tier architecture, one tier being the front-end client-side application and the other being the back-end server-side tier where the smart contract is deployed in the blockchain network. Figure 1. below shows the general architecture of the decentralized application and the interaction between a client and a server-side application. The tools that are used in developing the ridesharing Dapp are discussed in the later sections.
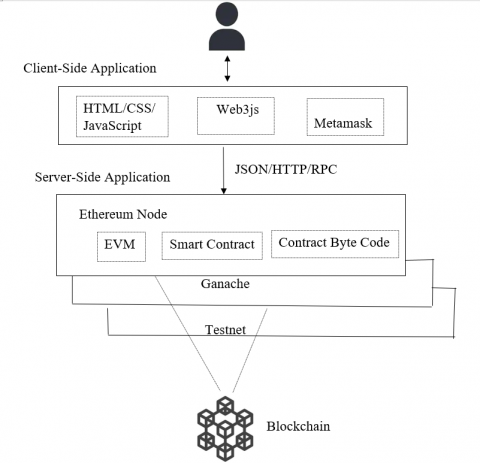
Figure 1. DApp architecture
As mentioned above any DApp has a backend and a front-end application. Unlike the centralized applications where the backend code runs on centralized servers, a DApp’s backend code is distributed through a decentralized peer-to-peer network. The front-end code of a DApp can be written in any language and API calls can be made to the backend.
Ethereum was the first blockchain-based platform to create a Turing complete language for writing smart contracts and a DApp development platform. The standard language for building DApp on the Ethereum platform is solidity. In this project, the Ethereum Truffle suite was used to develop and deploy smart contracts.
Truffle suite The Truffle suite comprises several components that are needed for developing a Dapp. It facilitates to development, compilation, deploy and test the smart contracts. It also allows the creation of front-end user interfaces for Dapps. The three major components of the Truffle suite are 1. Truffle framework, the developmental framework where the smart contracts are created, deployed, and tested. 2. Ganache, the simulator where the Dapp’s Graphical User Interface (GUI) is developed and tested.3. Drizzle: the front end library for Dapp development.
Web3.js The client-side application is just like any other web application built using HTML, CSS, and Javascript. Web3.js is a set of libraries that enables the Dapp browser to interact with the blockchain. It also helps in reading and writing data from smart contracts, transacting ethers from one account to another, and so on. We have used Metamask in our project that includes its web3 provider in the browser. Metamask is a chrome browser extension that allows users to manage their private key and Ethereum accounts in a secured manner. It uses the Ethereum accounts to interact with websites that use web3.js.
Ethereum Virtual Machine (EVM) In an Ethereum network, every Ethereum node runs its EVM and is involved in running the same instructions of smart contracts over the entire Ethereum network.
Let’s take a look at Uber and Ola where they offer transactions between the driver and the user. They help the customers to make a safe payment and flexibility in finding the cabs and other amenities while traveling. This creates a dependency between the customer and the driver and assists the intermediaries to increase their prices. According to [12], these intermediaries charge 10-20 percent higher than the actual charges to the customers.
B. Privacy and Security
As per [4], Uber even uses algorithmic predictions to find how much the customers are willing to pay more than the services they receive which is highly a security breach. Also, having all customer details in centralized servers can invite hackers for numerous attacks.
C. Lack of Transparency
Most of these ridesharing companies adapt surging price concepts where based on the demand they may charge the customers high especially in metro cities. The majority of the customers do not understand how these operations take place. There’s no transparency between the customers and the organizations.
D. Safety standards
Nowadays, the most important issue faced by people who travel through Uber, Ola, or any riding platforms is safety. The safety of both driver and the customers are at stake. Despite spending a huge amount in background verification processes this problem persists. The working process of the centralized ride-sharing model is shown below in Figure 2.
The process model used in the centralized storage model is shown in Figure 2. The steps involved in the process are as follows.
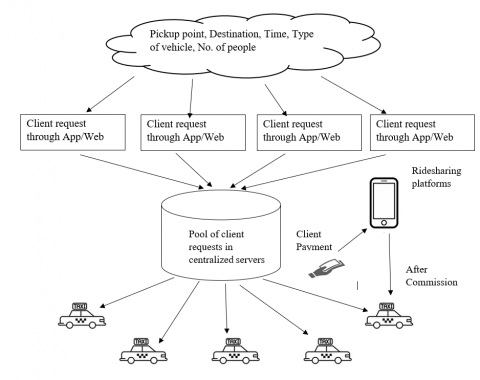
Figure 2. Existing ride-sharing framework
- The user looks for the cab through a web or mobile app of the cab providing platforms.
- The user enters the details like pick-up location, drop point, time, no. of persons, type of car, and mode of payment.
- The request is queued in a transaction pool and is routed to the nearby driver.
- The driver’s and user’s information are exchanged with each other.
- Once the trip is completed, the user will pay through the app and the cab provider receives and will send the driver’s charge after reducing a considerable amount of transaction fee.
- All transactions or information exchange here happens through the service provider who is the central authority and has control over the data.
To eliminate the issues mentioned in section 2, of the current centralized model. A decentralized approach is suggested. Blockchain as we know is a peer-to-peer decentralized, public ledger that allows transactions to happen without any intermediaries. And any transaction on a blockchain network is transparent, immutable, and secured. Therefore, adopting blockchain in ridesharing services removes the middle man and makes the transaction between the drivers and users at a reasonable cost. This attracts more users onboard and benefits them financially. S. E. Chang et al., in his work Application of Blockchain Technology to Smart City Service, mentioned that adopting Blockchain Technology in ridesharing services will make us closer to the concept of Smart City [13] as it embeds the technology within the city and adds different social dimensions for efficient allocation of resources, providing high-value services and improvising the quality of life for the public. Some of the benefits provided by blockchain technology compared to the centralized model are listed below [14].
- Less Cost: Apparently, blockchain removes the intermediated for the information exchange and thereby slashing the costs involved in the transaction to a greater extent. Moreover, it enables the user to trust the data on blockchain than an unknown third-party.
- Transparency: Although Blockchain is anonymous it provides transparency of the transactions at the same time. Therefore, it is always possible to trace back to the information if something goes wrong.
- Safety: Authenticity of data is assured as all the data blockchain are cryptographically protected. It is impossible to have any fraudulent data over the network.
- Transparency: In the blockchain, the data is available over the network and the raiding parties can have a transparent view of each other.
- Safe Payments: Users will be able to make a safe payment directly to the drivers using smart contracts, another unique feature of blockchain.
A decentralized P2P framework using Blockchain is proposed based on an existing framework. A decentralized application (DApp) is developed which will act as a front-end interface and is built on a decentralized Ethereum blockchain at the backend. In this framework, both the user and the driver get registered in the blockchain network with the necessary details. This meta-data information is attached to each of their profile which would be viewed by every node of the network. Three user roles were created as a part of this framework one being the driver, the other the user, and some legal authorities for verification. The working process is as given below [15].
1. The driver creates his profile in the network by submitting his driving license and other necessary documents. MongoDB is used as a storage mechanism and the hashed value is stored in the blockchain.
2. The Legal authorities who are also part of the network will be notified once the driver gives the information. Background verification will be done and the remarks would be added to the driver’s profile. A smart contract is used to generate the review or ratings of the driver based on which the rider can decide to take a ride with the driver.
3. Similarly, users who want to take a ride should also get registered in the network by providing some essential information like name, phone, etc., and background verification will happen similarly. This assures the safety of drivers.
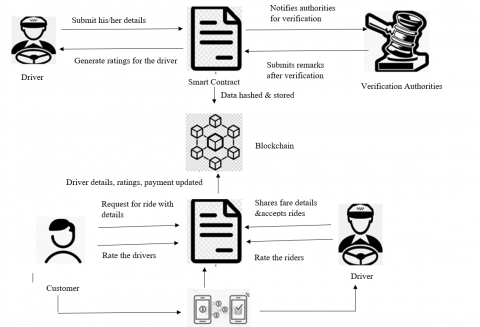
Figure 3. Proposed blockchain-based framework for ridesharing
4. After the verification is done, the user can request cabs by providing his/her location details and other trip details. The driver on such requests calculates the cost of the ride using various parameters like the distance to be traveled, type of car, and a fixed price per kilometer and shares to the users’ request.
5. The rider can now choose the ride as per their comfort.
6. Once the ride is completed payment can be automated from one wallet to the other as crypto payments.
Figure 3 summarizes the decentralized P2P ridesharing model.
5.1 Environment set-up
- Tools and Libraries
Several tools and libraries are available for DApp development. However, the tools and libraries used in developing this ride-sharing DApp are briefly discussed below in Table 2.
Table 2. Tools and libraries used in ridesharing DApp
- Process Flow of Ridesharing DApp
To develop the prototype of the proposed framework we make use of the local Ethereum framework, MetaMask, Web3js, Nodejs, and MongoDB. Two kinds of stakeholders are identified for this DApp i.e. the driver and the rider. Each user has different roles and responsibilities that are offered using different dashboards of the DApp. Driver Dashboard shows, i) The pick-up and Drop details of the user ii) The ride fare and iii) Payment Status whereas, the Rider Dashboard shows, i) Pick up and Drop details and ii) Ride Fare. Whenever a user detail is entered, the details are stored in MongoDB and the metadata of the credential is pushed into the blockchain. The DApp contains a front end which at the back end runs a decentralized platform. The workflow of the DApp is shown in Figure 4.
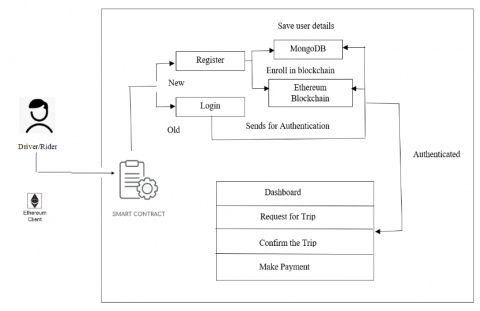
Figure 4. The ridesharing DApp workflow
- Ride Matching Problems
One of the major problems of the P2P ridesharing system is the ride-matching problem. Any ridesharing system's ability to direct drivers to passengers in the most efficient way is a distinguishing attribute. Any flexible ridesharing system must be prepared to provide an optimal solution to ride-matching problems. Some of the algorithms which give an optimal solution to ride-matching are greedy heuristic optimization, meta-heuristic optimization, Exact formulation and heuristic solution, decomposition algorithm, and dynamic programming [16]. The DApp implemented solves the matching problem by using a min matching algorithm to match riders requesting rideshare to save total travel distance.
5.2 Matching algorithm
Matching is a concept in mathematics, graph theory in specific. Matching is a group of edges without any common vertices in an undirected graph. For this ride-sharing problem, an undirected graph is formed with passengers being the nodes and their sharing plan being the edges [17]. With a maximum matching with minimum weight algorithm, it is possible to find the best sharing plan with minimal total distance. The algorithm calculates the distance between every two passengers is defined in different scenarios. The Manhattan distance between two passengers is calculated as,
$(\mathrm{A}(\mathrm{a} 1, \mathrm{a} 2), \mathrm{B}(\mathrm{b} 1, \mathrm{~b} 2), \mathrm{d}(\mathrm{A}, \mathrm{B})=|\mathrm{a} 1-\mathrm{b} 1|+|\mathrm{a} 2-\mathrm{b} 2|)$ (1)
The five different scenarios in which the distance between each two passengers d (A, B) is defined are:
- Pick up A and then pick-up B then drop A and then drop off B
$\mathrm{d}(\mathrm{AB})=\mathrm{d}(1)=\mathrm{d}(\mathrm{A} 1 \mathrm{~B} 1)+\mathrm{d}(\mathrm{B} 1 \mathrm{~A} 2)+\mathrm{d}(\mathrm{A} 2 \mathrm{~B} 2)$ (2)
- Pick up A then Pickup B then drop B and then drop A
$\mathrm{d}(\mathrm{AB})=\mathrm{d}(2)=\mathrm{d}(\mathrm{A} 1 \mathrm{~B} 1)+\mathrm{d}(\mathrm{B} 1 \mathrm{~B} 2)+\mathrm{d}(\mathrm{B} 2 \mathrm{~A} 2)$ (3)
- Pick up B and then pick up A then drop A and then B
$d(\mathrm{AB})=d(3)=d(\mathrm{~B} 1 \mathrm{~A} 1)+d(\mathrm{~A} 1 \mathrm{~A} 2)+d(\mathrm{~A} 2 \mathrm{~B} 2)$ (4)
- Pick up B then pick up A then drop B and then drop off A
$d(\mathrm{AB})=d(4)=d(\mathrm{~B} 1 \mathrm{~A} 1)+d(\mathrm{~A} 1 \mathrm{~B} 2)+d(\mathrm{~B} 2 \mathrm{~A} 2)$ (5)
- A and B travel on their own
$d(\mathrm{AB})=d(5)=d(\mathrm{~A} 1 \mathrm{~A} 2)+d(\mathrm{~B} 1 \mathrm{~B} 2)$ (6)
To assure the quality of sharing vehicles, the algorithm does not allow people to share vehicles if their total distance exceeds 25% more than their travel. Repeat the process below for all the passengers and find the distance.
For passenger(A)
if $d(\mathrm{~A} 1 \mathrm{~B} 1)+d(\mathrm{~B} 1 \mathrm{~A} 2)>1.25 \mathrm{~d}(\mathrm{~B} 1 \mathrm{~A} 1)$ set $d(1)$ to $+\propto$
if $d(\mathrm{~A} 1 \mathrm{~B} 1)+d(\mathrm{~B} 1 \mathrm{~B} 2)+d(\mathrm{~B} 2 \mathrm{~A} 2)>1.25 d(\mathrm{~B} 1 \mathrm{~A} 1)$ set $d(2)$ to $+\propto$
if $d(\mathrm{~A} 1 \mathrm{~B} 2)+d(\mathrm{~B} 2 \mathrm{~A} 2)>1.25 d(\mathrm{~B} 1 \mathrm{~A} 1):$ operatorname{set} ${d}(4)$ to $+\propto$
The weight of any two passengers can be: $d(\mathrm{AB})=\min {{d}(1), d(2), d(3), d(4), d(5)}$
5.3 Minimum matching
A complete graph is formulated to denote passengers and their suitable way of pooling each other once the weights of the edges are defined. The pseudocode of the minimum matching algorithm is given in Table 3. Using one of the scenarios (1) mentioned above, the matching of passengers for ridesharing is shown below. A, B, C, and D are the four passengers and are assigned some weights. The edges are marked with the way of pooling [18]. For all passengers with minimum total weight, the maximum match is pair A with D utilizing scenario 1, and pair B and C using scenario 1. The digramatic representation of rider matching problem based an greedy algorithm is given in Figure 5.
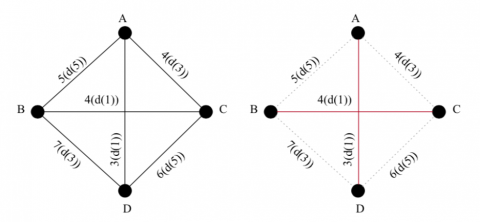
Figure 5. Riders matching problems using Greedy algorithm
Table 3. Minimum Matching Algorithm used to match rides with minimum distance
- Experimental results
The developed decentralized application is tested by running different experiments over a custom network where the Ganache was running. However, it can be tested using Rinkeby (Ethereum’s) test network as well. The cost for creating the contracts and calling the contracts is given in Table 4 below.
Several ridesharing systems are available having their own merits and demerits. A comparison of the existing centralized model with blockchain based model is tabulated in Table 5.
Table 4. Smart contract deployment cost
Table 5. Comparison of blockchain-based ride-sharing and other ride-sharing systems
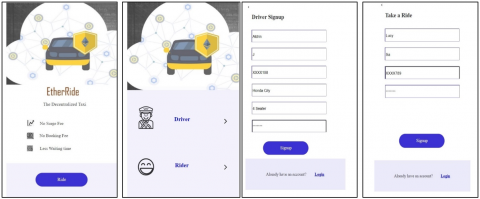
The main goal of this paper is to look at the revolutionary technology Blockchain and its use in the shared economy, which can serve as a framework for the smart city idea. This article presents an existing framework for decentralized, P2P, blockchain-based ridesharing services and proposing a more improved version for the same. Further, to support this ride-sharing framework, a decentralized application (DApp) is developed. It will act as a front-end user interface assisted by blockchain. Ethereum, a permissionless public blockchain is used in this DApp and the transactions and information exchange over the network is automated using smart contracts.
To summarize, blockchain can be used to create a system in which smart contracts incorporated in digital code are maintained in decentralized and transparent databases. The data in these databases are considered to be mutable. Every process and task is expected to have a digital record that will be identified, validated using a digital signature. We can have an ecosystem where no more intermediaries are needed.
Indeed, blockchain leads to a transformation of business models and governance but practically it is still many years away. Blockchain is not a disruptive technology intended to eradicate the traditional business models by providing low-cost solutions. Rather, it can be seen as a foundational technology capable of laying new frameworks for economic and social issues. Blockchain isn't a band-aid solution to a common technological problem. Although it can help with the transition, a clear plan based on proof of concepts for opportunities must be established. However, while blockchain will have a huge effect, it will take decades for it to pervade our socioeconomic infrastructure. As waves of technical and structural change make headway, acceptance will be incremental and steady, rather than abrupt.
Although blockchain could be used on its own, it would be more likely to have a greater impact while integrated with other technologies like the Internet of Things, Artificial Intelligence, and Big data. This could lead to better solutions for location-based automotive services. In the future we intend to extend the work by i) analyze the cost and performance of the application developed. ii) to explore the technology from a data processing perspective as blockchain technology is a trust-free system and enables users to trust data. Although the overall data quality is improved in blockchain technology it is essential to know the data processing abilities while integrating the blockchain with a larger software system. iii) Analyse the data processing workloads on different types of blockchain.
[1] Chang, S.E., Chang, C. (2018). Application of blockchain technology to smart city service: A case of ridesharing. 2018 IEEE International Conference on Internet of Things (iThings) and IEEE Green Computing and Communications (GreenCom) and IEEE Cyber, Physical and Social Computing (CPSCom) and IEEE Smart Data (SmartData), Halifax, NS, Canada, pp. 664-671. https://doi.org/10.1109/Cybermatics_2018.2018.00134 [2] Ramachandran, G.S., Radhakrishnan, R., Krishnamachari, B. (2018). Towards a decentralized data marketplace for smart cities. 2018 IEEE International Smart Cities Conference (ISC2), Kansas City, MO, USA, pp. 1-8. https://doi.org/10.1109/ISC2.2018.8656952 [3] Marchi, A., Parekh, E.J. (2016). How the sharing economy can make its case. McKinsey Quarterly, 1: 112. [4] Nakamoto, S. (2008). Bitcoin: A peer-to-peer electronic cash system. Available: http://www.ildgroup.si/uploads/product/20/bitcoin.pdf. [5] Yin, C., Xiong, Z., Chen, H., Wang, J., Cooper, D., David, B. (2015). A literature survey on smart cities. Science China Information Sciences, 58(10): 1-18. https://doi.org/10.1007/s11432-015-5397-4 [6] Zheng, Z., Xie, S., Dai, H., Chen, X., Wang, H. (2017). An overview of blockchain technology: Architecture, consensus, and future trends. Proc. IEEE BigDataCongress’17, Honolulu, HI, USA, pp. 557-564. https://doi.org/10.1109/BigDataCongress.2017.85 [7] Buterin, V. (2014). A next-generation smart contract and decentralized application platform. Ethereum white paper. [8] Delmolino, K., Arnett, M., Kosba, A., Miller, A., Shi, E. (2015). Step by step towards creating a safe smart contract: Lessons and insights from a cryptocurrency lab. In: Clark J., Meiklejohn S., Ryan P., Wallach D., Brenner M., Rohloff K. (eds) Financial Cryptography and Data Security. FC 2016. Lecture Notes in Computer Science, vol 9604. Springer, Berlin, Heidelberg. https://doi.org/10.1007/978-3-662-53357-4_6 [9] Wood, G. (2018). Ethereum: A secure decentralized generalized transaction ledger, Byzantium version. available at: https://ethereum.github.io/yellowpaper/paper.pdf. [10] Ibba, S., Pinna, A., Seu, M., Pani, F.E. (2017). Citysense: Blockchain-oriented smart cities. In Proceedings of the XP2017 Scientific Workshops, XP ’17, pp. 12:1-12:5. https://doi.org/10.1145/3120459.3120472 [11] Raval, S. (2016). Decentralized applications: Harnessing Bitcoin’s blockchain technology. O’Reilly Media, 1 ed. [12] Huckle, S., Bhattacharya, R., White, M., Beloff, N. (2016). Internet of things, blockchain and shared economy applications. Procedia Computer Science, 89: 461-466. https://doi.org/10.1016/j.procs.2016.09.074 [13] Chang, S.E., Chang, C. (2018). Application of blockchain technology to smart city service: A case of ridesharing. 2018 IEEE International Conference on Internet of Things (iThings) and IEEE Green Computing and Communications (GreenCom) and IEEE Cyber, Physical and Social Computing (CPSCom) and IEEE Smart Data (SmartData), Halifax, NS, Canada, pp. 664-671. https://doi.org/10.1109/Cybermatics_2018.2018.00134 [14] Yuan, Y., Wang, F. (2018). Towards blockchain-based intelligent transportation systems. 2016 IEEE 19th International Conference on Intelligent Transportation Systems (ITSC), Rio de Janeiro, pp. 2663-2668. https://doi.org/10.1109/ITSC.2016.7795984 [15] Victor, F., Zickau, S. (2018). Geofences on the blockchain: Enabling decentralized location-based services. 2018 IEEE International Conference on Data Mining Workshops (ICDMW), Singapore, Singapore, pp. 97-104. https://doi.org/10.1109/ICDMW.2018.00021 [16] Schreieck, M., Safetli, H., Siddiqui, S., Pflügler, C., Wiesche, M., Krcmar, H. (2016). A matching algorithm for dynamic ridesharing. Transportation Research Procedia, 19: 272-285. https://doi.org/10.1016/j.trpro.2016.12.087 [17] Baza, M., Lasla, N., Mahmoud, M., Srivastava, G., Abdallah, M. (2019). B-ride: Ride sharing with privacy-preservation, trust and fair payment atop public blockchain. IEEE Transactions on Network Science and Engineering. https://doi.org/10.1109/TNSE.2019.2959230 [18] Li, M., Zhu, L., Lin, X. (2019). Efficient and privacy-preserving carpooling using blockchain-assisted vehicular fog computing. IEEE Internet of Things Journal, 6(3): 4573-4584. https://doi.org/10.1109/JIOT.2018.2868076 [19] Aïvodji, U.M., Huguenin, K., Huguet, M.J., Killijian, M.O. (2018). Sride: A privacy-preserving ridesharing system. Proc. of the 11th ACM Conference on Security & Privacy in Wireless and Mobile Networks, pp. 40-50. https://doi.org/10.1145/3212480.3212483 [20] Arcade city. [Online]. Available: https://arcade.city/, accessed on 20 July 2020.
Phone: + 1 825 436 9306
Email: [email protected]
Subscription
Language support
Please sign up to receive notifications on new issues and newsletters from IIETA
Select Journal/Journals:
Copyright © 2024 IIETA. All Rights Reserved.
- Share full article
Advertisement
Supported by
In One Key A.I. Metric, China Pulls Ahead of the U.S.: Talent
China has produced a huge number of top A.I. engineers in recent years. New research shows that, by some measures, it has already eclipsed the United States.

By Paul Mozur and Cade Metz
Paul Mozur reported from Taipei, Taiwan, and Cade Metz from San Francisco.
When it comes to the artificial intelligence that powers chatbots like ChatGPT, China lags behind the United States . But when it comes to producing the scientists behind a new generation of humanoid technologies, China is pulling ahead.
New research shows that China has by some metrics eclipsed the United States as the biggest producer of A.I. talent, with the country generating almost half the world’s top A.I. researchers. By contrast, about 18 percent come from U.S. undergraduate institutions, according to the study , from MacroPolo, a think tank run by the Paulson Institute, which promotes constructive ties between the United States and China.
The findings show a jump for China, which produced about one-third of the world’s top talent three years earlier. The United States, by contrast, remained mostly the same. The research is based on the backgrounds of researchers whose papers were published at 2022’s Conference on Neural Information Processing Systems. NeurIPS, as it is known, is focused on advances in neural networks , which have anchored recent developments in generative A.I.
The talent imbalance has been building for the better part of a decade. During much of the 2010s, the United States benefited as large numbers of China’s top minds moved to American universities to complete doctoral degrees. A majority of them stayed in the United States. But the research shows that trend has also begun to turn, with growing numbers of Chinese researchers staying in China.
What happens in the next few years could be critical as China and the United States jockey for primacy in A.I. — a technology that can potentially increase productivity, strengthen industries and drive innovation — turning the researchers into one of the most geopolitically important groups in the world.
Generative A.I. has captured the tech industry in Silicon Valley and in China, causing a frenzy in funding and investment. The boom has been led by U.S. tech giants such as Google and start-ups like OpenAI. That could attract China’s researchers, though rising tensions between Beijing and Washington could also deter some, experts said.
(The New York Times has sued OpenAI and Microsoft for copyright infringement of news content related to A.I. systems.)
China has nurtured so much A.I. talent partly because it invested heavily in A.I. education. Since 2018, the country has added more than 2,000 undergraduate A.I. programs, with more than 300 at its most elite universities, said Damien Ma, the managing director of MacroPolo, though he noted the programs were not heavily focused on the technology that had driven breakthroughs by chatbots like ChatGPT.
“A lot of the programs are about A.I. applications in industry and manufacturing, not so much the generative A.I. stuff that’s come to dominate the American A.I. industry at the moment,” he said.
While the United States has pioneered breakthroughs in A.I., most recently with the uncanny humanlike abilities of chatbots , a significant portion of that work was done by researchers educated in China.
Researchers originally from China now make up 38 percent of the top A.I. researchers working in the United States, with Americans making up 37 percent, according to the research. Three years earlier, those from China made up 27 percent of top talent working in the United States, compared with 31 percent from the United States.
“The data shows just how critical Chinese-born researchers are to the United States for A.I. competitiveness,” said Matt Sheehan, a fellow at the Carnegie Endowment for International Peace who studies Chinese A.I.
He added that the data seemed to show the United States was still attractive. “We’re the world leader in A.I. because we continue to attract and retain talent from all over the world, but especially China,” he said.
Pieter Abbeel, a professor at the University of California, Berkeley, and a founder of Covariant , an A.I. and robotics start-up, said working alongside large numbers of Chinese researchers was taken for granted inside the leading American companies and universities.
“It’s just a natural state of affairs,” he said.
In the past, U.S. defense officials were not too concerned about A.I. talent flows from China, partly because many of the biggest A.I. projects did not deal with classified data and partly because they reasoned that it was better to have the best minds available. That so much of the leading research in A.I. is published openly also held back worries.
Despite bans introduced by the Trump administration that prohibit entry to the United States for students from some military-linked universities in China and a relative slowdown in the flow of Chinese students into the country during Covid, the research showed large numbers of the most promising A.I. minds continued coming to the United States to study.
But this month, a Chinese citizen who was an engineer at Google was charged with trying to transfer A.I. technology — including critical microchip architecture — to a Beijing-based company that paid him in secret , according to a federal indictment.
The substantial numbers of Chinese A.I. researchers working in the United States now present a conundrum for policymakers, who want to counter Chinese espionage while not discouraging the continued flow of top Chinese computer engineers into the United States, according to experts focused on American competitiveness.
“Chinese scholars are almost leading the way in the A.I. field,” said Subbarao Kambhampati, a professor and researcher of A.I. at Arizona State University. If policymakers try to bar Chinese nationals from research in the United States, he said, they are “shooting themselves in the foot.”
The track record of U.S. policymakers is mixed. A policy by the Trump administration aimed at curbing Chinese industrial espionage and intellectual property theft has since been criticized for errantly prosecuting a number of professors. Such programs, Chinese immigrants said, have encouraged some to stay in China.
For now, the research showed, most Chinese who complete doctorates in the United States stay in the country, helping to make it the global center of the A.I. world. Even so, the U.S. lead has begun to slip, to hosting about 42 percent of the world’s top talent, down from about 59 percent three years ago, according to the research.
Paul Mozur is the global technology correspondent for The Times, based in Taipei. Previously he wrote about technology and politics in Asia from Hong Kong, Shanghai and Seoul. More about Paul Mozur
Cade Metz writes about artificial intelligence, driverless cars, robotics, virtual reality and other emerging areas of technology. More about Cade Metz
Intermittent fasting linked to higher risk of cardiovascular death, research suggests
Intermittent fasting, a diet pattern that involves alternating between periods of fasting and eating, can lower blood pressure and help some people lose weight , past research has indicated.
But an analysis presented Monday at the American Heart Association’s scientific sessions in Chicago challenges the notion that intermittent fasting is good for heart health. Instead, researchers from Shanghai Jiao Tong University School of Medicine in China found that people who restricted food consumption to less than eight hours per day had a 91% higher risk of dying from cardiovascular disease over a median period of eight years, relative to people who ate across 12 to 16 hours.
It’s some of the first research investigating the association between time-restricted eating (a type of intermittent fasting) and the risk of death from cardiovascular disease.
The analysis — which has not yet been peer-reviewed or published in an academic journal — is based on data from the Centers for Disease Control and Prevention’s National Health and Nutrition Examination Survey collected between 2003 and 2018. The researchers analyzed responses from around 20,000 adults who recorded what they ate for at least two days, then looked at who had died from cardiovascular disease after a median follow-up period of eight years.
However, Victor Wenze Zhong, a co-author of the analysis, said it’s too early to make specific recommendations about intermittent fasting based on his research alone.
“Practicing intermittent fasting for a short period such as 3 months may likely lead to benefits on reducing weight and improving cardiometabolic health,” Zhong said via email. But he added that people “should be extremely cautious” about intermittent fasting for longer periods of time, such as years.
Intermittent fasting regimens vary widely. A common schedule is to restrict eating to a period of six to eight hours per day, which can lead people to consume fewer calories, though some eat the same amount in a shorter time. Another popular schedule is the "5:2 diet," which involves eating 500 to 600 calories on two nonconsecutive days of the week but eating normally for the other five.
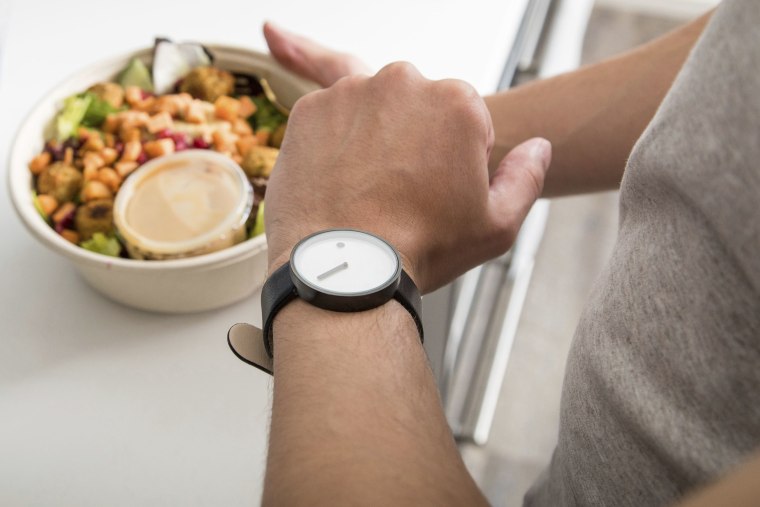
Zhong said it’s not clear why his research found an association between time-restricted eating and a risk of death from cardiovascular disease. He offered an observation, though: People who limited their eating to fewer than eight hours per day had less lean muscle mass than those who ate for 12 to 16 hours. Low lean muscle mass has been linked to a higher risk of cardiovascular death .
Cardiovascular and nutrition experts who were not involved in the analysis offered several theories about what might explain the results.
Dr. Benjamin Horne, a research professor at Intermountain Health in Salt Lake City, said fasting can increase stress hormones such as cortisol and adrenaline, since the body doesn’t know when to expect food next and goes into survival mode. That added stress may raise the short-term risk of heart problems among vulnerable groups, he said, particularly elderly people or those with chronic health conditions.
Horne’s research has shown that fasting twice a week for four weeks, then once a week for 22 weeks may increase a person’s risk of dying after one year but decrease their 10-year risk of chronic disease.
“In the long term, what it does is reduces those risk factors for heart disease and reduces the risk factors for diabetes and so forth — but in the short term, while you’re actually doing it, your body is in a state where it’s at a higher risk of having problems,” he said.
Even so, Horne added, the analysis “doesn’t change my perspective that there are definite benefits from fasting, but it’s a cautionary tale that we need to be aware that there are definite, potentially major, adverse effects.”
Intermittent fasting gained popularity about a decade ago, when the 5:2 diet was touted as a weight loss strategy in the U.K. In the years to follow, several celebrities espoused the benefits of an eight-hour eating window for weight loss, while some Silicon Valley tech workers believed that extreme periods of fasting boosted productivity . Some studies have also suggested that intermittent fasting might help extend people’s lifespans by warding off disease .
However, a lot of early research on intermittent fasting involved animals. In the last seven years or so, various clinical trials have investigated potential benefits for humans, including for heart health.
“The purpose of intermittent fasting is to cut calories, lose weight,” said Penny Kris-Etherton, emeritus professor of nutritional sciences at Penn State University and a member of the American Heart Association nutrition committee. “It’s really how intermittent fasting is implemented that’s going to explain a lot of the benefits or adverse associations.”
Dr. Francisco Lopez-Jimenez, a cardiologist at Mayo Clinic, said the timing of when people eat may influence the effects they see.
“I haven’t met a single person or patient that has been practicing intermittent fasting by skipping dinner,” he said, noting that people more often skip breakfast, a schedule associated with an increased risk of heart disease and death .
The new research comes with limitations: It relies on people’s memories of what they consumed over a 24-hour period and doesn’t consider the nutritional quality of the food they ate or how many calories they consumed during an eating window.
So some experts found the analysis too narrow.
“It’s a retrospective study looking at two days’ worth of data, and drawing some very big conclusions from a very limited snapshot into a person’s lifestyle habits,” said Dr. Pam Taub, a cardiologist at UC San Diego Health.
Taub said her patients have seen “incredible benefits” from fasting regimens.
“I would continue doing it,” she said. “For people that do intermittent fasting, their individual results speak for themselves. Most people that do intermittent fasting, the reason they continue it is they see a decrease in their weight. They see a decrease in blood pressure. They see an improvement in their LDL cholesterol.”
Kris-Etherton, however, urged caution: “Maybe consider a pause in intermittent fasting until we have more information or until the results of the study can be better explained,” she said.

Aria Bendix is the breaking health reporter for NBC News Digital.
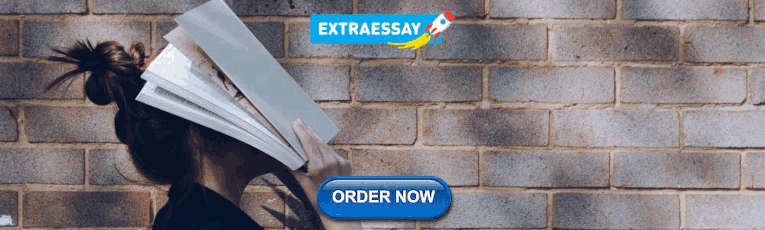
IMAGES
VIDEO
COMMENTS
By reviewing ride-sharing studies around the world, this paper aims to map major aspects of ride-sharing, including online platforms, user factors and barriers that affect ride-sharing services ...
Ride-sharing is an innovative on-demand transport service that aims to promote sustainable transport, reduce car utilization, increase vehicle occupancy and public transport ridership. By reviewing ride-sharing studies around the world, this paper aims to map major aspects of ride-sharing, including online platforms, user factors and barriers that affect ride-sharing services, and extract ...
studies, that review dynamic ride-sharing concept [], 2 ridesharing and matching criteria [38], and a meta-analysis exploring the factors that aect ride-sharing, which included 19 papers in the analysis [73]; however, none of them includes a review on ride-sharing plat-forms, user factors and barriers.
Some of the excluded papers related to topics like carbon emissions, autonomous vehicles, electric vehicle ownership, ride hailing, and ride sharing. Some addressed topics like mobile networks or mobility aids for the visually impaired but did not focus on automotive mobility, and these were also eliminated at this stage, leaving 366 papers in ...
Finally, in Section 6, we summarize our main insights and discuss directions for future research. Throughout the paper we also provide an overview of related problems in passenger and freight transportation optimization. 2. Problem characteristics2.1. Features of dynamic ride-sharing. In this paper, we use the term dynamic ride-sharing to ...
Aim Ride-sharing is an innovative on-demand transport service that aims to promote sustainable transport, reduce car utilization, increase vehicle occupancy and public transport ridership. By reviewing ride-sharing studies around the world, this paper aims to map major aspects of ride-sharing, including online platforms, user factors and barriers that affect ride-sharing services, and extract ...
The contribution of this research study is threefold: (1) First, we propose a novel multi-objective operational model that encompasses both ride-sharing modes, as well as indicators in all three sustainability dimensions. ... In this paper, we study the ride-sharing problem by taking a novel perspective, namely the complete sustainability ...
A research paper was . only take n into consideration if it consisted elements of ridesharing drivers ... Ride-Sharing Services : The Tumultuous Tale of the Rural Urban Divide.
By reviewing ride-sharing studies around the world, this paper aims to map major aspects of ride-sharing, including online platforms, user factors and barriers that affect ride-sharing services, and extract useful insights regarding their successful implementation. Method A systematic literature review is conducted on scientific publications in ...
Paper prepared for Oxford University Press ... we provide an overview of current research on the ... 6 The literature on ride-sharing sometime refers to drivers and "driver-partners". We use ...
Also, ride-sharing allows passengers to build a pro-social or altruistic image, thus increasing their social status and popularity (Ashrafi et al., Citation 2021). However, existing studies have not found social engagement and altruism to be significant influences in explaining ridesharing behaviour (Amirkiaee & Evangelopoulos, Citation 2018 ).
The focus of this paper is commercial ride-sharing (not carsharing or carpooling by neighbours going to the same destination) using hailing technology. ... The authors hope that this paper will contribute to the discussion around ride-sharing research and the various possible future transport solutions. Although the applied method has ...
The ride-sharing system gained popularity because of its efficient transportation. This literature review aims to provide an overview of the existing research and findings related to the ride-sharing system. A. Charnes, W. W. Cooper and R. O. Ferguson were the pioneers of Goal Programming. In 1955, they presented a paper in which they have ...
Multi-hop ride sharing touches upon different research branches. Firstly, shared mobility systems are a central part of the Sharing Economy which has recently seen great attention (Botsman and Rogers 2010; Cusumano 2014; Teubner 2014).Furthermore, ride sharing can be considered a two-sided market as platform operators cater to two inter-dependent customer groups - drivers and riders ...
In this paper, we study the ride-sharing problem where each vehicle is shared by exactly riders for any fixed > 0, and the goal is to minimize the total travel distance. The min-cost ride-sharing problem is intractable even in the case of exactly two riders sharing a vehicle [2], and hence we can only hope for an approximate solution.
We formally define dynamic ride-sharing and outline the optimization challenges that arise when developing technology to support ride-sharing. We hope that this paper will encourage more research by the transportation science and logistics community in this exciting, emerging area of public transportation.
Many types of research have addressed the optimal pricing of ride-sharing platforms. For instance, in the work (Al-Abbasi et al., Citation 2019), the authors propose DeepPool platform, which is a distributed free-model that uses Q-network techniques. Ride-sharing services were improved by incorporating a deep learning model and demand statistics.
In this paper, we propose an efficient dynamic tree algorithm to solve the on-demand peer-to-peer ride-sharing matching problem. The dynamic tree algorithm benefits from given ride-sharing driver schedules, and provides satisfactory runtime performances. In addition, an efficient pre-processing procedure to select candidate passenger requests ...
A study to assess how ride-sharing impacts urban mobility in the United States found ride-sharing increased both intensity and duration of road congestion while there was no significant change in ... and an author of the paper. "Our research shows that over time TNCs have intensified urban transport challenges and road congestion in the ...
Ride-sharing is an innovative on-demand transport service that aims to promote sustainable transport, reduce car utilization, increase vehicle occupancy and public transport ridership. By reviewing ride-sharing studies around the world, this paper aims to map major aspects of ride-sharing, including online platforms, user factors and barriers ...
Feature papers represent the most advanced research with significant potential for high impact in the field. A Feature Paper should be a substantial original Article that involves several techniques or approaches, provides an outlook for future research directions and describes possible research applications. ... The ride sharing problem has ...
5. Implementation. Allows creating DApps on Ethereum network. Provides a suite of tools to write Smart Contracts using Solidity. Provides a framework for testing the contracts. Gives tools to deploy Smart Contracts in the blockchain. Used to develop client-side applications inside truffle.
China has produced a huge number of top A.I. engineers in recent years. New research shows that, by some measures, it has already eclipsed the United States. By Paul Mozur and Cade Metz Paul Mozur ...
Some research papers addressed only the utilization of blockchain in ride-sharing, but others also extending their proposal beyond this utilization to include privacy and confidentiality enhancement. More details and comparisons on almost all blockchain-based ride-sharing works can be found in our work ( Mahmoud et al., 2022 ).
Intermittent fasting regimens vary widely. A common schedule is to restrict eating to a period of six to eight hours per day, which can lead people to consume fewer calories, though some eat the ...