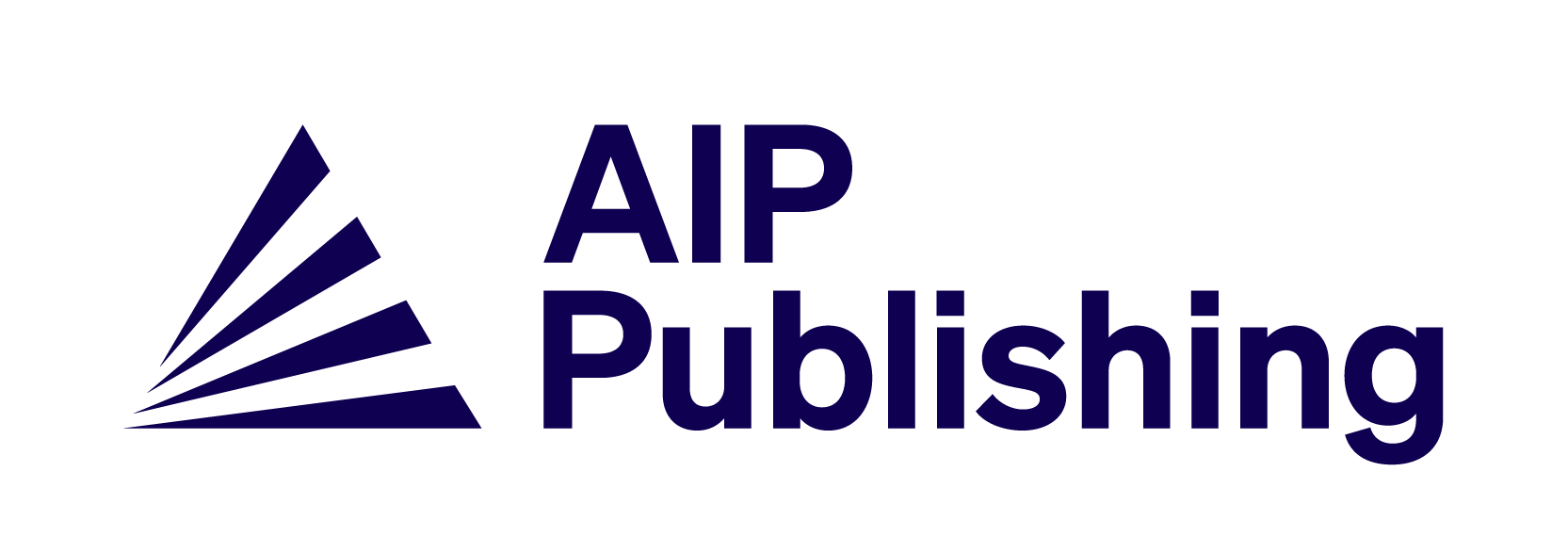
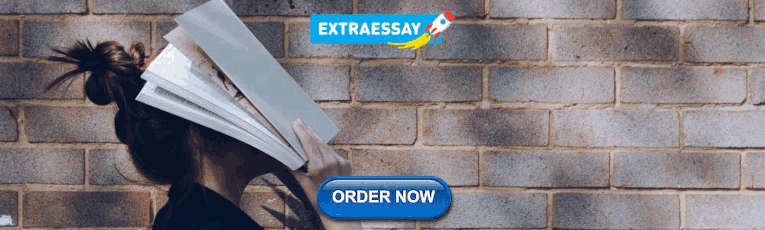
Using Conceptual Scaffolding to Foster Effective Problem Solving
- Article contents
- Figures & tables
- Supplementary Data
- Peer Review
- Reprints and Permissions
- Cite Icon Cite
- Search Site
Lin Ding , Neville Reay , Albert Lee , Lei Bao; Using Conceptual Scaffolding to Foster Effective Problem Solving. AIP Conf. Proc. 5 November 2009; 1179 (1): 129–132. https://doi.org/10.1063/1.3266695
Download citation file:
- Ris (Zotero)
- Reference Manager
Traditional end‐of‐chapter problems often are localized, requiring formulas only within a single chapter. Students frequently can solve these problems by performing “plug‐and‐chug” without recognizing underlying concepts. We designed open‐ended problems that require a synthesis of concepts that are broadly separated in the teaching time line, militating against students’ blindly invoking locally introduced formulas. Each problem was encapsulated into a sequence with two preceding conceptually‐based multiple‐choice questions. These conceptual questions address the same underlying concepts as the subsequent problem, providing students with guided conceptual scaffolding. When solving the problem, students were explicitly advised to search for underlying connections based on the conceptual questions. Both small‐scale interviews and a large‐scale written test were conducted to investigate the effects of guided conceptual scaffolding on student problem solving. Specifically, student performance on the open‐ended problems was compared between those who received scaffolding and those who did not. A further analysis of whether the conceptual scaffolding was equivalent to mere cueing also was conducted.
Sign in via your Institution
Citing articles via, publish with us - request a quote.
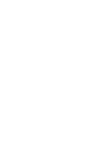
Sign up for alerts
- Online ISSN 1551-7616
- Print ISSN 0094-243X
- For Researchers
- For Librarians
- For Advertisers
- Our Publishing Partners
- Physics Today
- Conference Proceedings
- Special Topics
pubs.aip.org
- Privacy Policy
- Terms of Use
Connect with AIP Publishing
This feature is available to subscribers only.
Sign In or Create an Account

- Distance Learning
- Print Resource Orders
Subscribe to our Creative Problem Solving Online Learning Course today!
About problem solving styles.

Knowledge of style is important in education in a number of ways. It contributes to adults’ ability to work together effectively in teams and in large groups. It provides information that helps educators understand their own personal strengths and how to put them to work as effectively as possible across many tasks and challenges. It helps educators communicate more effectively with each other, but also with parents, community members, and, of course, with students. In addition to its importance for adults, style can also be important in designing and differentiating instruction.
The VIEW Model
Our approach to problem solving style (the VIEW model) represents and assesses three dimensions and six specific styles that are unique and important in understanding and guiding the efforts of individuals and groups to manage their creative problem solving and change management as effectively as possible.
Orientation to Change
The first VIEW dimension involves your preferences in two general styles for managing change and solving problems creatively. We identify this as “Orientation to Change;” its two contrasting styles are the “Explorer” and the “Developer.” Explorers thrive on and seek out novelty and original ideas (“thinking out of the box”), and they may find externally imposed procedures and structures confining and limiting to their imagination and energy. Developers are concerned with practical applications and the reality of the task, and they use their creative and critical thinking in ways that are clearly recognized by others as being helpful and valuable. They’re good at finding workable possibilities and guiding them to successful implementation. They are creative in “thinking better inside the box.”
Manner of Processing
The second dimension of VIEW, Manner of Processing describes the person’s preference for working externally (i.e., with other people throughout the process) or internally (i.e., thinking and working alone before sharing ideas with others) when managing change and solving problems.
Ways of Deciding
The third dimension of VIEW describes the major emphasis the person gives to people (i.e., maintaining harmony and interpersonal relationships) or to tasks (i.e., emphasizing logical, rational, and appropriate decisions) when making decisions during problem solving or when managing change.
Through our research and development efforts, our instrument, VIEW: An Assessment of Problem Solving Style, translates the VIEW model of style into measurable dimensions. The VIEW assessment is a practical and useful tool for anyone who wishes to understand his or her own approach to change or problem solving. Contact us for more information about the VIEW instrument.
Practical Applications of VIEW
Understanding problem solving styles can be helpful in many ways to individuals, teams, small groups, and organizations. VIEW: An Assessment of Problem Solving Style is a carefully researched, but simple and easy-to-use tool that can enable people to understand their style preferences and to use that knowledge in many powerful ways. These pages illustrate briefly a variety of practical applications of the VIEW assessment that cut across many settings or contexts (including, for example: large, global organizations; smaller business and professional settings; educational institutions, hospitals, religious organizations, arts organizations, or other non-profits). VIEW can be a valuable tool for individuals who are concerned with understanding their personal style preferences and improving their problem solving effectiveness, for teams or groups who need to work together successfully, and to organizations in their efforts to build a constructive work climate, to recognize and value diversity, and to manage change for long-term success. Click on any of the following ten applications of VIEW to see examples for that area. (You may also click here to Download a PDF file with all the applications examples in one file.)
- Improving Problem Solving
- Communicating Effectively
- Enhancing Personal Productivity
- Providing and Receiving Feedback
- Facilitating Groups
- Managing Change
- Developing Leadership
- Designing Instruction
- Building Teams
- Coaching and Mentoring
Free Resources
Click here to find a number of free resources that will explain CPS, to obtain articles that deal with both research and practice, and to obtain an extensive bibliography to give you direction for future reading and study.
Online Resources:
Click here for advanced online resources in PDF format that deal with applications of the VIEW Problem Solving Style model. These resources are available at a reasonable cost for immediate download. The cost of each one includes permission to duplicate the file for up to three other individuals at no additional charge.
Distance Learning Resources:
Click here for information about our extensive (newly revised and updated) distance learning modules on CPS.
Print Resources:
We also have print publications about problem-solving style that you can purchase. Click here to view those publications.
Workshops, Training, Consulting Services:
Our CPS programs and services are custom-tailored to meet your needs and interests. We will confer with you, create a complete proposal to meet your unique needs, and work closely with you to carry out our collaborative plan. Click here for more.
We believe that all people have strengths and talents that are important to recognize, develop, and use throughout life. Read more.
- https://sodo-group.com
- s999 casino
- sodo casino
- link sodo66
- trang chu sodo66
- 79sodo đăng nhập
- nhà cái s666
- BONG DA LUUU
- CV88 CASINO
- ZM88 TẶNG 100K
- VZ99 TẶNG 100K
- QH88 TẶNG 100K
- xổ số miền bắc 500 ngày
- nhà cái J88
- nhà cái SV88
- BAYVIP club
- KEN88 game bài
- nhà cái 6BET
- nhà cái 8DAY
- lô khung 1 ngày
- dàn de 16 số khung 5 ngày
- bạch thủ kép
- kèo nhà cái hôm nay
- nhà cái 8kbet
- lk88 casino
- BETLV casino
- trực tiếp 3s
- soi kèo ngoại hạng anh
- https://vj9999.site
- https://uw88.online
- https://vwinbets.com
- xổ số đại phát
- xổ số miền bắc đại phát
- xổ số miền nam đại phát
- kqxs đại phát
- xổ số thiên phú
- Xổ số phương trang
- linkviva88.net
- đăng ký w88
- casino trực tuyến uy tín
- tải game tài xỉu online
- xoc dia online
- top nhà cái uy tín
- nhà cái uy tín
- sp666 casino
- nhà cái bwing
- nha cai tk88
- https://tk886.com
- trang chu sodo
- nhà cái mb88
- app baby live
- app tiktok live
- https://one88vn.net/
- https://vb68.live/
- https://nhacaivb68.org/
- https://vb68.info/
- https://vb68.one/
- https://mu88gamebai.com/
- https://dulieunhacai.com/
- https://vn89nhacai.com/
- https://tk88vnd.com/
- https://tk88a.org/
- https://tk88pro.net/
- https://tk88a.net/
- https://tk88viet.com/
- https://tk88viet.org/
- https://tk88vietnam.com/
- https://tk88vietnam.org/
- https://kimsa88no1.com/
- https://sm66link.com/
- https://bet168no1.com/
- https://tk860.com/
- https://richditch.com/
- https://zb-bet.com/
- https://vivanau.net/
- https://fiyrra.com/
- https://tf88vn.fun/
- https://365rrd.com/
- https://78wed.com/
- https://ee8881.com/
- https://tk66live.live/
- https://qh888.net/
- https://funews.net/
- https://rodball.com/
- https://shbenqi.com/
- https://888wpc.com/
- https://3344ys.com/
- https://m88q.com/
- https://w88ap.net/
- https://jun-room.com/
- https://me8e.com/
- https://xsvwines.com/
- https://fbk8.com/
- https://jbohm.com/
- https://scotlandroyalty.org/
- https://newsbd7.com/
- skylush.net
- https://123bno1.com/
- https://188betno1.com/
- https://789betno1.com/
- https://78winno1.com/
- https://79sodono1.com/
- https://bet66no1.com/
- https://ttk86.com/
- https://hr99link.com/
- https://lixi88no1.com/
- https://mu88link.com/
- https://mu88no1.com/
- https://qh88link.com/
- https://qh88no1.com/
- https://s666no1.com/
- https://sm66no1.com/
- https://sodo66no1.com/
- https://vb138betno1.com/
- https://vx88no1.com/
- link sodo casino
- nhà cái jbo
- link ole777
- đăng ký vz99
- xổ số miền bắc minh chính
- sm66 nha cai
- soi cầu xổ số miền bắc
- trực tiếp kết quả xổ số
- lô đề online
- d9bet casino
- nhà cái pog79
- tỷ số trực tuyến 7m
- link vao fb88
- nha cai 188bet
- nha cai vn88
- nha cai m88
- trang chủ 789bet
- bet88vn.net
- soi kèo world cup 2022
- nhà cái bet88
- https://xocdia.biz/
- soi cầu xổ số
- fb88next.com
- fb88thai.com
Leadership Team
Our work builds on more than five decades of research, development, and practical experience in organizations. Learn more about our team .
Contact Information
Center for Creative Learning, LLC 2015 Grant Place Melbourne, Florida, 32901 USA Email: [email protected]
- deneme bonusu veren siteler
- geres-cambodia.org
- capitalhillrealty.net
- bahis siteleri
- johnacarroll.com ile En güvenilir deneme bonusu veren bahis siteleri listesine erişin.
- beautyskinlaserga.com ile 2024 yeni deneme bonusu veren siteler listesine erişin.
How to master the seven-step problem-solving process
In this episode of the McKinsey Podcast , Simon London speaks with Charles Conn, CEO of venture-capital firm Oxford Sciences Innovation, and McKinsey senior partner Hugo Sarrazin about the complexities of different problem-solving strategies.
Podcast transcript
Simon London: Hello, and welcome to this episode of the McKinsey Podcast , with me, Simon London. What’s the number-one skill you need to succeed professionally? Salesmanship, perhaps? Or a facility with statistics? Or maybe the ability to communicate crisply and clearly? Many would argue that at the very top of the list comes problem solving: that is, the ability to think through and come up with an optimal course of action to address any complex challenge—in business, in public policy, or indeed in life.
Looked at this way, it’s no surprise that McKinsey takes problem solving very seriously, testing for it during the recruiting process and then honing it, in McKinsey consultants, through immersion in a structured seven-step method. To discuss the art of problem solving, I sat down in California with McKinsey senior partner Hugo Sarrazin and also with Charles Conn. Charles is a former McKinsey partner, entrepreneur, executive, and coauthor of the book Bulletproof Problem Solving: The One Skill That Changes Everything [John Wiley & Sons, 2018].
Charles and Hugo, welcome to the podcast. Thank you for being here.
Hugo Sarrazin: Our pleasure.
Charles Conn: It’s terrific to be here.
Simon London: Problem solving is a really interesting piece of terminology. It could mean so many different things. I have a son who’s a teenage climber. They talk about solving problems. Climbing is problem solving. Charles, when you talk about problem solving, what are you talking about?
Charles Conn: For me, problem solving is the answer to the question “What should I do?” It’s interesting when there’s uncertainty and complexity, and when it’s meaningful because there are consequences. Your son’s climbing is a perfect example. There are consequences, and it’s complicated, and there’s uncertainty—can he make that grab? I think we can apply that same frame almost at any level. You can think about questions like “What town would I like to live in?” or “Should I put solar panels on my roof?”
You might think that’s a funny thing to apply problem solving to, but in my mind it’s not fundamentally different from business problem solving, which answers the question “What should my strategy be?” Or problem solving at the policy level: “How do we combat climate change?” “Should I support the local school bond?” I think these are all part and parcel of the same type of question, “What should I do?”
I’m a big fan of structured problem solving. By following steps, we can more clearly understand what problem it is we’re solving, what are the components of the problem that we’re solving, which components are the most important ones for us to pay attention to, which analytic techniques we should apply to those, and how we can synthesize what we’ve learned back into a compelling story. That’s all it is, at its heart.
I think sometimes when people think about seven steps, they assume that there’s a rigidity to this. That’s not it at all. It’s actually to give you the scope for creativity, which often doesn’t exist when your problem solving is muddled.
Simon London: You were just talking about the seven-step process. That’s what’s written down in the book, but it’s a very McKinsey process as well. Without getting too deep into the weeds, let’s go through the steps, one by one. You were just talking about problem definition as being a particularly important thing to get right first. That’s the first step. Hugo, tell us about that.
Hugo Sarrazin: It is surprising how often people jump past this step and make a bunch of assumptions. The most powerful thing is to step back and ask the basic questions—“What are we trying to solve? What are the constraints that exist? What are the dependencies?” Let’s make those explicit and really push the thinking and defining. At McKinsey, we spend an enormous amount of time in writing that little statement, and the statement, if you’re a logic purist, is great. You debate. “Is it an ‘or’? Is it an ‘and’? What’s the action verb?” Because all these specific words help you get to the heart of what matters.
Want to subscribe to The McKinsey Podcast ?
Simon London: So this is a concise problem statement.
Hugo Sarrazin: Yeah. It’s not like “Can we grow in Japan?” That’s interesting, but it is “What, specifically, are we trying to uncover in the growth of a product in Japan? Or a segment in Japan? Or a channel in Japan?” When you spend an enormous amount of time, in the first meeting of the different stakeholders, debating this and having different people put forward what they think the problem definition is, you realize that people have completely different views of why they’re here. That, to me, is the most important step.
Charles Conn: I would agree with that. For me, the problem context is critical. When we understand “What are the forces acting upon your decision maker? How quickly is the answer needed? With what precision is the answer needed? Are there areas that are off limits or areas where we would particularly like to find our solution? Is the decision maker open to exploring other areas?” then you not only become more efficient, and move toward what we call the critical path in problem solving, but you also make it so much more likely that you’re not going to waste your time or your decision maker’s time.
How often do especially bright young people run off with half of the idea about what the problem is and start collecting data and start building models—only to discover that they’ve really gone off half-cocked.
Hugo Sarrazin: Yeah.
Charles Conn: And in the wrong direction.
Simon London: OK. So step one—and there is a real art and a structure to it—is define the problem. Step two, Charles?
Charles Conn: My favorite step is step two, which is to use logic trees to disaggregate the problem. Every problem we’re solving has some complexity and some uncertainty in it. The only way that we can really get our team working on the problem is to take the problem apart into logical pieces.
What we find, of course, is that the way to disaggregate the problem often gives you an insight into the answer to the problem quite quickly. I love to do two or three different cuts at it, each one giving a bit of a different insight into what might be going wrong. By doing sensible disaggregations, using logic trees, we can figure out which parts of the problem we should be looking at, and we can assign those different parts to team members.
Simon London: What’s a good example of a logic tree on a sort of ratable problem?
Charles Conn: Maybe the easiest one is the classic profit tree. Almost in every business that I would take a look at, I would start with a profit or return-on-assets tree. In its simplest form, you have the components of revenue, which are price and quantity, and the components of cost, which are cost and quantity. Each of those can be broken out. Cost can be broken into variable cost and fixed cost. The components of price can be broken into what your pricing scheme is. That simple tree often provides insight into what’s going on in a business or what the difference is between that business and the competitors.
If we add the leg, which is “What’s the asset base or investment element?”—so profit divided by assets—then we can ask the question “Is the business using its investments sensibly?” whether that’s in stores or in manufacturing or in transportation assets. I hope we can see just how simple this is, even though we’re describing it in words.
When I went to work with Gordon Moore at the Moore Foundation, the problem that he asked us to look at was “How can we save Pacific salmon?” Now, that sounds like an impossible question, but it was amenable to precisely the same type of disaggregation and allowed us to organize what became a 15-year effort to improve the likelihood of good outcomes for Pacific salmon.
Simon London: Now, is there a danger that your logic tree can be impossibly large? This, I think, brings us onto the third step in the process, which is that you have to prioritize.
Charles Conn: Absolutely. The third step, which we also emphasize, along with good problem definition, is rigorous prioritization—we ask the questions “How important is this lever or this branch of the tree in the overall outcome that we seek to achieve? How much can I move that lever?” Obviously, we try and focus our efforts on ones that have a big impact on the problem and the ones that we have the ability to change. With salmon, ocean conditions turned out to be a big lever, but not one that we could adjust. We focused our attention on fish habitats and fish-harvesting practices, which were big levers that we could affect.
People spend a lot of time arguing about branches that are either not important or that none of us can change. We see it in the public square. When we deal with questions at the policy level—“Should you support the death penalty?” “How do we affect climate change?” “How can we uncover the causes and address homelessness?”—it’s even more important that we’re focusing on levers that are big and movable.
Would you like to learn more about our Strategy & Corporate Finance Practice ?
Simon London: Let’s move swiftly on to step four. You’ve defined your problem, you disaggregate it, you prioritize where you want to analyze—what you want to really look at hard. Then you got to the work plan. Now, what does that mean in practice?
Hugo Sarrazin: Depending on what you’ve prioritized, there are many things you could do. It could be breaking the work among the team members so that people have a clear piece of the work to do. It could be defining the specific analyses that need to get done and executed, and being clear on time lines. There’s always a level-one answer, there’s a level-two answer, there’s a level-three answer. Without being too flippant, I can solve any problem during a good dinner with wine. It won’t have a whole lot of backing.
Simon London: Not going to have a lot of depth to it.
Hugo Sarrazin: No, but it may be useful as a starting point. If the stakes are not that high, that could be OK. If it’s really high stakes, you may need level three and have the whole model validated in three different ways. You need to find a work plan that reflects the level of precision, the time frame you have, and the stakeholders you need to bring along in the exercise.
Charles Conn: I love the way you’ve described that, because, again, some people think of problem solving as a linear thing, but of course what’s critical is that it’s iterative. As you say, you can solve the problem in one day or even one hour.
Charles Conn: We encourage our teams everywhere to do that. We call it the one-day answer or the one-hour answer. In work planning, we’re always iterating. Every time you see a 50-page work plan that stretches out to three months, you know it’s wrong. It will be outmoded very quickly by that learning process that you described. Iterative problem solving is a critical part of this. Sometimes, people think work planning sounds dull, but it isn’t. It’s how we know what’s expected of us and when we need to deliver it and how we’re progressing toward the answer. It’s also the place where we can deal with biases. Bias is a feature of every human decision-making process. If we design our team interactions intelligently, we can avoid the worst sort of biases.
Simon London: Here we’re talking about cognitive biases primarily, right? It’s not that I’m biased against you because of your accent or something. These are the cognitive biases that behavioral sciences have shown we all carry around, things like anchoring, overoptimism—these kinds of things.
Both: Yeah.
Charles Conn: Availability bias is the one that I’m always alert to. You think you’ve seen the problem before, and therefore what’s available is your previous conception of it—and we have to be most careful about that. In any human setting, we also have to be careful about biases that are based on hierarchies, sometimes called sunflower bias. I’m sure, Hugo, with your teams, you make sure that the youngest team members speak first. Not the oldest team members, because it’s easy for people to look at who’s senior and alter their own creative approaches.
Hugo Sarrazin: It’s helpful, at that moment—if someone is asserting a point of view—to ask the question “This was true in what context?” You’re trying to apply something that worked in one context to a different one. That can be deadly if the context has changed, and that’s why organizations struggle to change. You promote all these people because they did something that worked well in the past, and then there’s a disruption in the industry, and they keep doing what got them promoted even though the context has changed.
Simon London: Right. Right.
Hugo Sarrazin: So it’s the same thing in problem solving.
Charles Conn: And it’s why diversity in our teams is so important. It’s one of the best things about the world that we’re in now. We’re likely to have people from different socioeconomic, ethnic, and national backgrounds, each of whom sees problems from a slightly different perspective. It is therefore much more likely that the team will uncover a truly creative and clever approach to problem solving.
Simon London: Let’s move on to step five. You’ve done your work plan. Now you’ve actually got to do the analysis. The thing that strikes me here is that the range of tools that we have at our disposal now, of course, is just huge, particularly with advances in computation, advanced analytics. There’s so many things that you can apply here. Just talk about the analysis stage. How do you pick the right tools?
Charles Conn: For me, the most important thing is that we start with simple heuristics and explanatory statistics before we go off and use the big-gun tools. We need to understand the shape and scope of our problem before we start applying these massive and complex analytical approaches.
Simon London: Would you agree with that?
Hugo Sarrazin: I agree. I think there are so many wonderful heuristics. You need to start there before you go deep into the modeling exercise. There’s an interesting dynamic that’s happening, though. In some cases, for some types of problems, it is even better to set yourself up to maximize your learning. Your problem-solving methodology is test and learn, test and learn, test and learn, and iterate. That is a heuristic in itself, the A/B testing that is used in many parts of the world. So that’s a problem-solving methodology. It’s nothing different. It just uses technology and feedback loops in a fast way. The other one is exploratory data analysis. When you’re dealing with a large-scale problem, and there’s so much data, I can get to the heuristics that Charles was talking about through very clever visualization of data.
You test with your data. You need to set up an environment to do so, but don’t get caught up in neural-network modeling immediately. You’re testing, you’re checking—“Is the data right? Is it sound? Does it make sense?”—before you launch too far.
Simon London: You do hear these ideas—that if you have a big enough data set and enough algorithms, they’re going to find things that you just wouldn’t have spotted, find solutions that maybe you wouldn’t have thought of. Does machine learning sort of revolutionize the problem-solving process? Or are these actually just other tools in the toolbox for structured problem solving?
Charles Conn: It can be revolutionary. There are some areas in which the pattern recognition of large data sets and good algorithms can help us see things that we otherwise couldn’t see. But I do think it’s terribly important we don’t think that this particular technique is a substitute for superb problem solving, starting with good problem definition. Many people use machine learning without understanding algorithms that themselves can have biases built into them. Just as 20 years ago, when we were doing statistical analysis, we knew that we needed good model definition, we still need a good understanding of our algorithms and really good problem definition before we launch off into big data sets and unknown algorithms.
Simon London: Step six. You’ve done your analysis.
Charles Conn: I take six and seven together, and this is the place where young problem solvers often make a mistake. They’ve got their analysis, and they assume that’s the answer, and of course it isn’t the answer. The ability to synthesize the pieces that came out of the analysis and begin to weave those into a story that helps people answer the question “What should I do?” This is back to where we started. If we can’t synthesize, and we can’t tell a story, then our decision maker can’t find the answer to “What should I do?”
Simon London: But, again, these final steps are about motivating people to action, right?
Charles Conn: Yeah.
Simon London: I am slightly torn about the nomenclature of problem solving because it’s on paper, right? Until you motivate people to action, you actually haven’t solved anything.
Charles Conn: I love this question because I think decision-making theory, without a bias to action, is a waste of time. Everything in how I approach this is to help people take action that makes the world better.
Simon London: Hence, these are absolutely critical steps. If you don’t do this well, you’ve just got a bunch of analysis.
Charles Conn: We end up in exactly the same place where we started, which is people speaking across each other, past each other in the public square, rather than actually working together, shoulder to shoulder, to crack these important problems.
Simon London: In the real world, we have a lot of uncertainty—arguably, increasing uncertainty. How do good problem solvers deal with that?
Hugo Sarrazin: At every step of the process. In the problem definition, when you’re defining the context, you need to understand those sources of uncertainty and whether they’re important or not important. It becomes important in the definition of the tree.
You need to think carefully about the branches of the tree that are more certain and less certain as you define them. They don’t have equal weight just because they’ve got equal space on the page. Then, when you’re prioritizing, your prioritization approach may put more emphasis on things that have low probability but huge impact—or, vice versa, may put a lot of priority on things that are very likely and, hopefully, have a reasonable impact. You can introduce that along the way. When you come back to the synthesis, you just need to be nuanced about what you’re understanding, the likelihood.
Often, people lack humility in the way they make their recommendations: “This is the answer.” They’re very precise, and I think we would all be well-served to say, “This is a likely answer under the following sets of conditions” and then make the level of uncertainty clearer, if that is appropriate. It doesn’t mean you’re always in the gray zone; it doesn’t mean you don’t have a point of view. It just means that you can be explicit about the certainty of your answer when you make that recommendation.
Simon London: So it sounds like there is an underlying principle: “Acknowledge and embrace the uncertainty. Don’t pretend that it isn’t there. Be very clear about what the uncertainties are up front, and then build that into every step of the process.”
Hugo Sarrazin: Every step of the process.
Simon London: Yeah. We have just walked through a particular structured methodology for problem solving. But, of course, this is not the only structured methodology for problem solving. One that is also very well-known is design thinking, which comes at things very differently. So, Hugo, I know you have worked with a lot of designers. Just give us a very quick summary. Design thinking—what is it, and how does it relate?
Hugo Sarrazin: It starts with an incredible amount of empathy for the user and uses that to define the problem. It does pause and go out in the wild and spend an enormous amount of time seeing how people interact with objects, seeing the experience they’re getting, seeing the pain points or joy—and uses that to infer and define the problem.
Simon London: Problem definition, but out in the world.
Hugo Sarrazin: With an enormous amount of empathy. There’s a huge emphasis on empathy. Traditional, more classic problem solving is you define the problem based on an understanding of the situation. This one almost presupposes that we don’t know the problem until we go see it. The second thing is you need to come up with multiple scenarios or answers or ideas or concepts, and there’s a lot of divergent thinking initially. That’s slightly different, versus the prioritization, but not for long. Eventually, you need to kind of say, “OK, I’m going to converge again.” Then you go and you bring things back to the customer and get feedback and iterate. Then you rinse and repeat, rinse and repeat. There’s a lot of tactile building, along the way, of prototypes and things like that. It’s very iterative.
Simon London: So, Charles, are these complements or are these alternatives?
Charles Conn: I think they’re entirely complementary, and I think Hugo’s description is perfect. When we do problem definition well in classic problem solving, we are demonstrating the kind of empathy, at the very beginning of our problem, that design thinking asks us to approach. When we ideate—and that’s very similar to the disaggregation, prioritization, and work-planning steps—we do precisely the same thing, and often we use contrasting teams, so that we do have divergent thinking. The best teams allow divergent thinking to bump them off whatever their initial biases in problem solving are. For me, design thinking gives us a constant reminder of creativity, empathy, and the tactile nature of problem solving, but it’s absolutely complementary, not alternative.
Simon London: I think, in a world of cross-functional teams, an interesting question is do people with design-thinking backgrounds really work well together with classical problem solvers? How do you make that chemistry happen?
Hugo Sarrazin: Yeah, it is not easy when people have spent an enormous amount of time seeped in design thinking or user-centric design, whichever word you want to use. If the person who’s applying classic problem-solving methodology is very rigid and mechanical in the way they’re doing it, there could be an enormous amount of tension. If there’s not clarity in the role and not clarity in the process, I think having the two together can be, sometimes, problematic.
The second thing that happens often is that the artifacts the two methodologies try to gravitate toward can be different. Classic problem solving often gravitates toward a model; design thinking migrates toward a prototype. Rather than writing a big deck with all my supporting evidence, they’ll bring an example, a thing, and that feels different. Then you spend your time differently to achieve those two end products, so that’s another source of friction.
Now, I still think it can be an incredibly powerful thing to have the two—if there are the right people with the right mind-set, if there is a team that is explicit about the roles, if we’re clear about the kind of outcomes we are attempting to bring forward. There’s an enormous amount of collaborativeness and respect.
Simon London: But they have to respect each other’s methodology and be prepared to flex, maybe, a little bit, in how this process is going to work.
Hugo Sarrazin: Absolutely.
Simon London: The other area where, it strikes me, there could be a little bit of a different sort of friction is this whole concept of the day-one answer, which is what we were just talking about in classical problem solving. Now, you know that this is probably not going to be your final answer, but that’s how you begin to structure the problem. Whereas I would imagine your design thinkers—no, they’re going off to do their ethnographic research and get out into the field, potentially for a long time, before they come back with at least an initial hypothesis.

Want better strategies? Become a bulletproof problem solver
Hugo Sarrazin: That is a great callout, and that’s another difference. Designers typically will like to soak into the situation and avoid converging too quickly. There’s optionality and exploring different options. There’s a strong belief that keeps the solution space wide enough that you can come up with more radical ideas. If there’s a large design team or many designers on the team, and you come on Friday and say, “What’s our week-one answer?” they’re going to struggle. They’re not going to be comfortable, naturally, to give that answer. It doesn’t mean they don’t have an answer; it’s just not where they are in their thinking process.
Simon London: I think we are, sadly, out of time for today. But Charles and Hugo, thank you so much.
Charles Conn: It was a pleasure to be here, Simon.
Hugo Sarrazin: It was a pleasure. Thank you.
Simon London: And thanks, as always, to you, our listeners, for tuning into this episode of the McKinsey Podcast . If you want to learn more about problem solving, you can find the book, Bulletproof Problem Solving: The One Skill That Changes Everything , online or order it through your local bookstore. To learn more about McKinsey, you can of course find us at McKinsey.com.
Charles Conn is CEO of Oxford Sciences Innovation and an alumnus of McKinsey’s Sydney office. Hugo Sarrazin is a senior partner in the Silicon Valley office, where Simon London, a member of McKinsey Publishing, is also based.
Explore a career with us
Related articles.
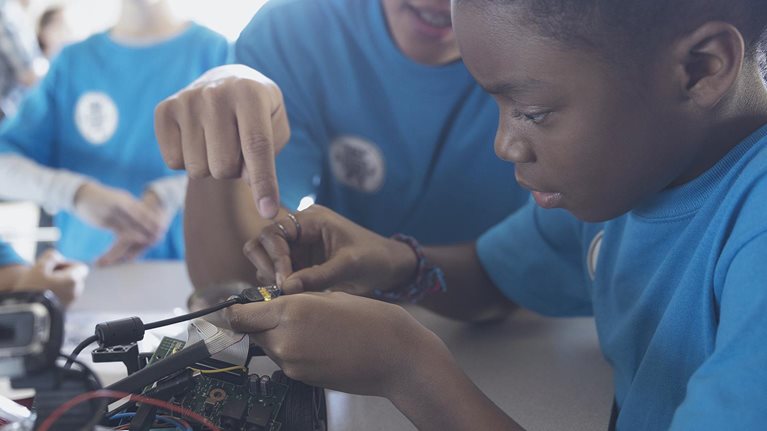
Strategy to beat the odds
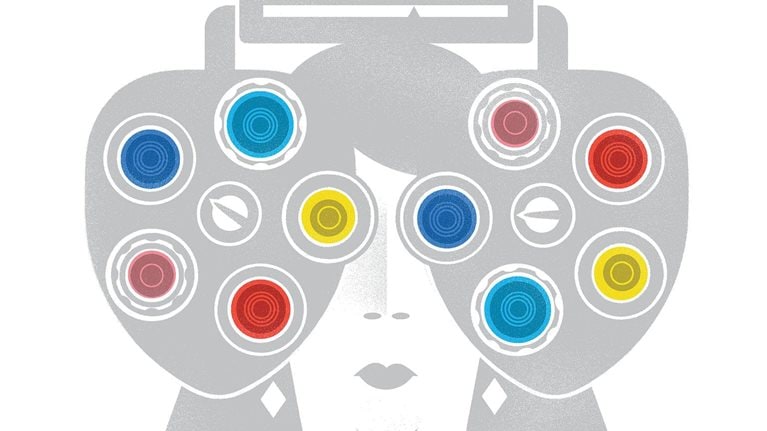
Five routes to more innovative problem solving
Thank you for visiting nature.com. You are using a browser version with limited support for CSS. To obtain the best experience, we recommend you use a more up to date browser (or turn off compatibility mode in Internet Explorer). In the meantime, to ensure continued support, we are displaying the site without styles and JavaScript.
- View all journals
- My Account Login
- Explore content
- About the journal
- Publish with us
- Sign up for alerts
- Review Article
- Open access
- Published: 10 August 2016
Learning strategies: a synthesis and conceptual model
- John A C Hattie 1 &
- Gregory M Donoghue 1
npj Science of Learning volume 1 , Article number: 16013 ( 2016 ) Cite this article
169k Accesses
178 Citations
681 Altmetric
Metrics details
- Social sciences
The purpose of this article is to explore a model of learning that proposes that various learning strategies are powerful at certain stages in the learning cycle. The model describes three inputs and outcomes (skill, will and thrill), success criteria, three phases of learning (surface, deep and transfer) and an acquiring and consolidation phase within each of the surface and deep phases. A synthesis of 228 meta-analyses led to the identification of the most effective strategies. The results indicate that there is a subset of strategies that are effective, but this effectiveness depends on the phase of the model in which they are implemented. Further, it is best not to run separate sessions on learning strategies but to embed the various strategies within the content of the subject, to be clearer about developing both surface and deep learning, and promoting their associated optimal strategies and to teach the skills of transfer of learning. The article concludes with a discussion of questions raised by the model that need further research.
Similar content being viewed by others
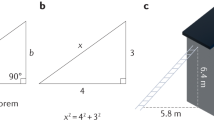
The science of effective learning with spacing and retrieval practice
Shana K. Carpenter, Steven C. Pan & Andrew C. Butler
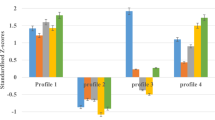
Profiles of learners based on their cognitive and metacognitive learning strategy use: occurrence and relations with gender, intrinsic motivation, and perceived autonomy support
Diana Kwarikunda, Ulrich Schiefele, … Joseph Ssenyonga
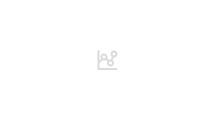
What makes the difference? An empirical comparison of critical aspects identified in phenomenographic and variation theory analyses
Mona Holmqvist & Per Selin
There has been a long debate about the purpose of schooling. These debates include claims that schooling is about passing on core notions of humanity and civilisation (or at least one’s own society’s view of these matters). They include claims that schooling should prepare students to live pragmatically and immediately in their current environment, should prepare students for the work force, should equip students to live independently, to participate in the life of their community, to learn to ‘give back’, to develop personal growth. 1
In the past 30 years, however, the emphasis in many western systems of education has been more on enhancing academic achievement—in domains such as reading, mathematics, and science—as the primary purpose of schooling. 2 Such an emphasis has led to curricula being increasingly based on achievement in a few privileged domains, and ‘great’ students are deemed those who attain high levels of proficiency in these narrow domains.
This has led to many countries aiming to be in the top echelon of worldwide achievement measures in a narrow range of subjects; for example, achievement measures such as PISA (tests of 15-year olds in mathematics, reading and science, across 65 countries in 2012) or PIRLS (Year-5 tests of mathematics, reading and science, across 57 countries in 2011). Indeed, within most school systems there is a plethora of achievement tests; many countries have introduced accountability pressures based on high levels of testing of achievement; and communities typically value high achievement or levels of knowledge. 3 The mantra underpinning these claims has been cast in terms of what students know and are able to do; the curriculum is compartmentalised into various disciplines of achievement; and students, teachers, parents and policy makers talk in terms of success in these achievement domains.
Despite the recent emphasis on achievement, the day-to-day focus of schools has always been on learning—how to know, how to know more efficiently and how to know more effectively. The underlying philosophy is more about what students are now ready to learn, how their learning can be enabled, and increasing the ‘how to learn’ proficiencies of students. In this scenario, the purpose of schooling is to equip students with learning strategies, or the skills of learning how to learn. Of course, learning and achievement are not dichotomous; they are related. 4 Through growth in learning in specific domains comes achievement and from achievement there can be much learning. The question in this article relates to identifying the most effective strategies for learning.
In our search, we identified >400 learning strategies: that is, those processes which learners use to enhance their own learning. Many were relabelled versions of others, some were minor modifications of others, but there remained many contenders purported to be powerful learning strategies. Such strategies help the learner structure his or her thinking so as to plan, set goals and monitor progress, make adjustments, and evaluate the process of learning and the outcomes. These strategies can be categorised in many ways according to various taxonomies and classifications (e.g., references 5 , 6 , 7 ). Boekaerts, 8 for example, argued for three types of learning strategies: (1) cognitive strategies such as elaboration, to deepen the understanding of the domain studied; (2) metacognitive strategies such as planning, to regulate the learning process; and (3) motivational strategies such as self-efficacy, to motivate oneself to engage in learning. Given the advent of newer ways to access information (e.g., the internet) and the mountain of information now at students’ fingertips, it is appropriate that Dignath, Buettner and Langfeldt 9 added a fourth category—management strategies such as finding, navigating, and evaluating resources.
But merely investigating these 400-plus strategies as if they were independent is not defensible. Thus, we begin with the development of a model of learning to provide a basis for interpreting the evidence from our meta-synthesis. The argument is that learning strategies can most effectively enhance performance when they are matched to the requirements of tasks (cf. 10 ).
A model of learning
The model comprises the following components: three inputs and three outcomes; student knowledge of the success criteria for the task; three phases of the learning process (surface, deep and transfer), with surface and deep learning each comprising an acquisition phase and a consolidation phase; and an environment for the learning ( Figure 1 ). We are proposing that various learning strategies are differentially effective depending on the degree to which the students are aware of the criteria of success, on the phases of learning process in which the strategies are used, and on whether the student is acquiring or consolidating their understanding. The following provides an overview of the components of the model (see reference 11 for a more detailed explanation of the model).
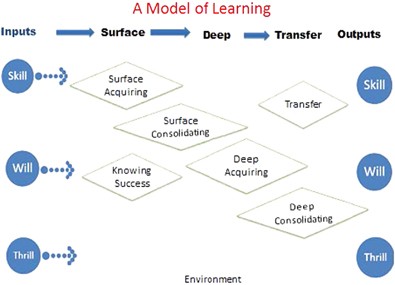
A model of learning.
Input and outcomes
The model starts with three major sources of inputs: the skill, the will and the thrill. The ‘skill’ is the student’s prior or subsequent achievement, the ‘will’ relates to the student’s various dispositions towards learning, and the ‘thrill’ refers to the motivations held by the student. In our model, these inputs are also the major outcomes of learning. That is, developing outcomes in achievement (skill) is as valuable as enhancing the dispositions towards learning (will) and as valuable as inviting students to reinvest more into their mastery of learning (thrill or motivations).
The first component describes the prior achievement the student brings to the task. As Ausubel 12 claimed ‘if I had to reduce all of educational psychology to just one principle, I would say this ‘The most important single factor influencing learning is what the leaner already knows. Ascertain this and teach him accordingly. Other influences related to the skills students bring to learning include their working memory, beliefs, encouragement and expectations from the student’s cultural background and home.
Dispositions are more habits of mind or tendencies to respond to situations in certain ways. Claxton 13 claimed that the mind frame of a ‘powerful learner’ is based on the four major dispositions: resilience or emotional strength, resourcefulness or cognitive capabilities, reflection or strategic awareness, and relating or social sophistication. These dispositions involve the proficiency to edit, select, adapt and respond to the environment in a recurrent, characteristic manner. 14 But dispositions alone are not enough. Perkins et al. 15 outlined a model with three psychological components which must be present in order to spark dispositional behaviour: sensitivity—the perception of the appropriateness of a particular behaviour; inclination—the felt impetus toward a behaviour; and ability—the basic capacity and confidence to follow through with the behaviour.
There can be a thrill in learning but for many students, learning in some domains can be dull, uninviting and boring. There is a huge literature on various motivational aspects of learning, and a smaller literature on how the more effective motivational aspects can be taught. A typical demarcation is between mastery and performance orientations. Mastery goals are seen as being associated with intellectual development, the acquisition of knowledge and new skills, investment of greater effort, and higher-order cognitive strategies and learning outcomes. 16 Performance goals, on the other hand, have a focus on outperforming others or completing tasks to please others. A further distinction has been made between approach and avoidance performance goals. 17 – 19 The correlations of mastery and performance goals with achievement, however, are not as high as many have claimed. A recent meta-analysis found 48 studies relating goals to achievement (based on 12,466 students), and the overall correlation was 0.12 for mastery and 0.05 for performance goals on outcomes. 20 Similarly, Hulleman et al. 21 reviewed 249 studies ( N =91,087) and found an overall correlation between mastery goal and outcomes of 0.05 and performance goals and outcomes of 0.14. These are small effects and show the relatively low importance of these motivational attributes in relation to academic achievement.
An alternative model of motivation is based on Biggs 22 learning processes model, which combines motivation (why the student wants to study the task) and their related strategies (how the student approaches the task). He outlined three common approaches to learning: deep, surface and achieving. When students are taking a deep strategy, they aim to develop understanding and make sense of what they are learning, and create meaning and make ideas their own. This means they focus on the meaning of what they are learning, aim to develop their own understanding, relate ideas together and make connections with previous experiences, ask themselves questions about what they are learning, discuss their ideas with others and compare different perspectives. When students are taking a surface strategy, they aim to reproduce information and learn the facts and ideas—with little recourse to seeing relations or connections between ideas. When students are using an achieving strategy, they use a ‘minimax’ notion—minimum amount of effort for maximum return in terms of passing tests, complying with instructions, and operating strategically to meet a desired grade. It is the achieving strategy that seems most related to school outcomes.
Success criteria
The model includes a prelearning phase relating to whether the students are aware of the criteria of success in the learning task. This phase is less about whether the student desires to attain the target of the learning (which is more about motivation), but whether he or she understands what it means to be successful at the task at hand. When a student is aware of what it means to be successful before undertaking the task, this awareness leads to more goal-directed behaviours. Students who can articulate or are taught these success criteria are more likely to be strategic in their choice of learning strategies, more likely to enjoy the thrill of success in learning, and more likely to reinvest in attaining even more success criteria.
Success criteria can be taught. 23 , 24 Teachers can help students understand the criteria used for judging the students’ work, and thus teachers need to be clear about the criteria used to determine whether the learning intentions have been successfully achieved. Too often students may know the learning intention, but do not how the teacher is going to judge their performance, or how the teacher knows when or whether students have been successful. 25 The success criteria need to be as clear and specific as possible (at surface, deep, or transfer level) as this enables the teacher (and learner) to monitor progress throughout the lesson to make sure students understand and, as far as possible, attain the intended notions of success. Learning strategies that help students get an overview of what success looks like include planning and prediction, having intentions to implement goals, setting standards for judgement success, advance organisers, high levels of commitment to achieve success, and knowing about worked examples of what success looks like. 23
Environment
Underlying all components in the model is the environment in which the student is studying. Many books and internet sites on study skills claim that it is important to attend to various features of the environment such as a quiet room, no music or television, high levels of social support, giving students control over their learning, allowing students to study at preferred times of the day and ensuring sufficient sleep and exercise.
The three phases of learning: surface, deep and transfer
The model highlights the importance of both surface and deep learning and does not privilege one over the other, but rather insists that both are critical. Although the model does seem to imply an order, it must be noted that these are fuzzy distinctions (surface and deep learning can be accomplished simultaneously), but it is useful to separate them to identify the most effective learning strategies. More often than not, a student must have sufficient surface knowledge before moving to deep learning and then to the transfer of these understandings. As Entwistle 26 noted, ‘The verb ‘to learn’ takes the accusative’; that is, it only makes sense to analyse learning in relation to the subject or content area and the particular piece of work towards which the learning is directed, and also the context within which the learning takes place. The key debate, therefore, is whether the learning is directed content that is meaningful to the student, as this will directly affect student dispositions, in particular a student’s motivation to learn and willingness to reinvest in their learning.
A most powerful model to illustrate this distinction between surface and deep is the structure of observed learning outcomes, or SOLO, 27 , 28 as discussed above. The model has four levels: unistructural, multistructural, relational and extended abstract. A unistructural intervention is based on teaching or learning one idea, such as coaching one algorithm, training in underlining, using a mnemonic or anxiety reduction. The essential feature is that this idea alone is the focus, independent of the context or its adaption to or modification by content. A multistructural intervention involves a range of independent strategies or procedures, but without integrating or orchestration as to the individual differences or demands of content or context (such as teaching time management, note taking and setting goals with no attention to any strategic or higher-order understandings of these many techniques). Relational interventions involve bringing together these various multistructural ideas, and seeing patterns; it can involve the strategies of self-monitoring and self-regulation. Extended abstract interventions aim at far transfer (transfer between contexts that, initally, appear remote to one another) such that they produce structural changes in an individual’s cognitive functioning to the point where autonomous or independent learning can occur. The first two levels (one then many ideas) refer to developing surface knowing and the latter two levels (relate and extend) refer to developing deeper knowing. The parallel in learning strategies is that surface learning refers to studying without much reflecting on either purpose or strategy, learning many ideas without necessarily relating them and memorising facts and procedures routinely. Deep learning refers to seeking meaning, relating and extending ideas, looking for patterns and underlying principles, checking evidence and relating it to conclusions, examining arguments cautiously and critically, and becoming actively interested in course content (see reference 29 ).
Our model also makes a distinction between first acquiring knowledge and then consolidating it. During the acquisition phase, information from a teacher or instructional materials is attended to by the student and this is taken into short-term memory. During the consolidation phase, a learner then needs to actively process and rehearse the material as this increases the likelihood of moving that knowledge to longer-term memory. At both phases there can be a retrieval process, which involves transferring the knowing and understanding from long-term memory back into short-term working memory. 30 , 31
Acquiring surface learning
In their meta-analysis of various interventions, Hattie et al. 32 found that many learning strategies were highly effective in enhancing reproductive performances (surface learning) for virtually all students. Surface learning includes subject matter vocabulary, the content of the lesson and knowing much more. Strategies include record keeping, summarisation, underlining and highlighting, note taking, mnemonics, outlining and transforming, organising notes, training working memory, and imagery.
Consolidating surface learning
Once a student has begun to develop surface knowing it is then important to encode it in a manner such that it can retrieved at later appropriate moments. This encoding involves two groups of learning strategies: the first develops storage strength (the degree to which a memory is durably established or ‘well learned’) and the second develops strategies that develop retrieval strength (the degree to which a memory is accessible at a given point in time). 33 ‘Encoding’ strategies are aimed to develop both, but with a particular emphasis on developing retrieval strength. 34 Both groups of strategies invoke an investment in learning, and this involves ‘the tendency to seek out, engage in, enjoy and continuously pursue opportunities for effortful cognitive activity. 35 Although some may not ‘enjoy’ this phase, it does involve a willingness to practice, to be curious and to explore again, and a willingness to tolerate ambiguity and uncertainty during this investment phase. In turn, this requires sufficient metacognition and a calibrated sense of progress towards the desired learning outcomes. Strategies include practice testing, spaced versus mass practice, teaching test taking, interleaved practice, rehearsal, maximising effort, help seeking, time on task, reviewing records, learning how to receive feedback and deliberate practice (i.e., practice with help of an expert, or receiving feedback during practice).
Acquiring deep learning
Students who have high levels of awareness, control or strategic choice of multiple strategies are often referred to as ‘self-regulated’ or having high levels of metacognition. In Visible Learning , Hattie 36 described these self-regulated students as ‘becoming like teachers’, as they had a repertoire of strategies to apply when their current strategy was not working, and they had clear conceptions of what success on the task looked like. 37 More technically, Pintrich et al. 38 described self-regulation as ‘an active, constructive process whereby learners set goals for their learning and then attempt to monitor, regulate and control their cognition, motivation and behaviour, guided and constrained by their goals and the contextual features in the environment’. These students know the what, where, who, when and why of learning, and the how, when and why to use which learning strategies. 39 They know what to do when they do not know what to do. Self-regulation strategies include elaboration and organisation, strategy monitoring, concept mapping, metacognitive strategies, self-regulation and elaborative interrogation.
Consolidating deep learning
Once a student has acquired surface and deep learning to the extent that it becomes part of their repertoire of skills and strategies, we may claim that they have ‘automatised’ such learning—and in many senses this automatisation becomes an ‘idea’, and so the cycle continues from surface idea to deeper knowing that then becomes a surface idea, and so on. 40 There is a series of learning strategies that develop the learner’s proficiency to consolidate deeper thinking and to be more strategic about learning. These include self-verbalisation, self-questioning, self-monitoring, self-explanation, self-verbalising the steps in a problem, seeking help from peers and peer tutoring, collaborative learning, evaluation and reflection, problem solving and critical thinking techniques.
There are skills involved in transferring knowledge and understanding from one situation to a new situation. Indeed, some have considered that successful transfer could be thought as synonymous with learning. 41 , 42 There are many distinctions relating to transfer: near and far transfer, 43 low and high transfer, 44 transfer to new situations and problem solving transfer, 5 and positive and negative transfer. 45 Transfer is a dynamic, not static, process that requires learners to actively choose and evaluate strategies, consider resources and surface information, and, when available, to receive or seek feedback to enhance these adaptive skills. Reciprocal teaching is one program specifically aiming to teach these skills; for example, Bereiter and Scardamalia 46 have developed programs in the teaching of transfer in writing, where students are taught to identify goals, improve and elaborate existing ideas, strive for idea cohesion, present their ideas to groups and think aloud about how they might proceed. Similarly, Schoenfeld 47 outlined a problem-solving approach to mathematics that involves the transfer of skills and knowledge from one situation to another. Marton 48 argued that transfer occurs when the learner learns strategies that apply in a certain situation such that they are enabled to do the same thing in another situation when they realise that the second situation resembles (or is perceived to resemble) the first situation. He claimed that not only sameness, similarity, or identity might connect situations to each other, but also small differences might connect them as well. Learning how to detect such differences is critical for the transfer of learning. As Heraclitus claimed, no two experiences are identical; you do not step into the same river twice.
Overall messages from the model
There are four main messages to be taken from the model. First, if the success criteria is the retention of accurate detail (surface learning) then lower-level learning strategies will be more effective than higher-level strategies. However, if the intention is to help students understand context (deeper learning) with a view to applying it in a new context (transfer), then higher level strategies are also needed. An explicit assumption is that higher level thinking requires a sufficient corpus of lower level surface knowledge to be effective—one cannot move straight to higher level thinking (e.g., problem solving and creative thought) without sufficient level of content knowledge. Second, the model proposes that when students are made aware of the nature of success for the task, they are more likely to be more involved in investing in the strategies to attain this target. Third, transfer is a major outcome of learning and is more likely to occur if students are taught how to detect similarities and differences between one situation and a new situation before they try to transfer their learning to the new situation. Hence, not one strategy may necessarily be best for all purposes. Fourth, the model also suggests that students can be advantaged when strategy training is taught with an understanding of the conditions under which the strategy best works—when and under what circumstance it is most appropriate.
The current study
The current study synthesises the many studies that have related various learning strategies to outcomes. This study only pertains to achievement outcomes (skill, on the model of learning); further work is needed to identify the strategies that optimise the dispositions (will) and the motivation (thrill) outcomes. The studies synthesised here are from four sources. First, there are the meta-analyses among the 1,200 meta-analyses in Visible Learning that relate to strategies for learning. 36 , 49 , 50 Second, there is the meta-analysis conducted by Lavery 51 on 223 effect-sizes derived from 31 studies relating to self-regulated learning interventions. The third source is two major meta-analyses by a Dutch team of various learning strategies, especially self-regulation. And the fourth is a meta-analysis conducted by Donoghue et al. 52 based on a previous analysis by Dunlosky et al. 53
The data in Visible Learning is based on 800 meta-analyses relating influences from the home, school, teacher, curriculum and teaching methods to academic achievement. Since its publication in 2009, the number of meta-analyses now exceeds 1,200, and those influences specific to learning strategies are retained in the present study. Lavery 51 identified 14 different learning strategies and the overall effect was 0.46—with greater effects for organising and transforming (i.e., deliberate rearrangement of instructional materials to improve learning, d =0.85) and self-consequences (i.e., student expectation of rewards or punishment for success or failure, d =0.70). The lowest effects were for imagery (i.e., creating or recalling vivid mental images to assist learning, d =0.44) and environmental restructuring (i.e., efforts to select or arrange the physical setting to make learning easier, d =0.22). She concluded that the higher effects involved ‘teaching techniques’ and related to more ‘deep learning strategies’, such as organising and transforming, self-consequences, self-instruction, self-evaluation, help-seeking, keeping records, rehearsing/memorising, reviewing and goal-setting. The lower ranked strategies were more ‘surface learning strategies’, such as time management and environmental restructuring.
Of the two meta-analyses conducted by the Dutch team, the first study, by Dignath et al. 9 analysed 357 effects from 74 studies ( N =8,619). They found an overall effect of 0.73 from teaching methods of self-regulation. The effects were large for achievement (elementary school, 0.68; high school, 0.71), mathematics (0.96, 1.21), reading and writing (0.44, 0.55), strategy use (0.72, 0.79) and motivation (0.75, 0.92). In the second study, Donker et al. 54 reviewed 180 effects from 58 studies relating to self-regulation training, reporting an overall effect of 0.73 in science, 0.66 in mathematics and 0.36 in reading comprehension. The most effective strategies were cognitive strategies (rehearsal 1.39, organisation 0.81 and elaboration 0.75), metacognitive strategies (planning 0.80, monitoring 0.71 and evaluation 0.75) and management strategies (effort 0.77, peer tutoring 0.83, environment 0.59 and metacognitive knowledge 0.97). Performance was almost always improved by a combination of strategies, as was metacognitive knowledge. This led to their conclusion that students should not only be taught which strategies to use and how to apply them (declarative knowledge or factual knowledge) but also when (procedural or how to use the strategies) and why to use them (conditional knowledge or knowing when to use a strategy).
Donoghue et al. 52 conducted a meta-analysis based on the articles referenced in Dunlosky et al. 53 They reviewed 10 learning strategies and a feature of their review is a careful analysis of possible moderators to the conclusions about the effectiveness of these learning strategies, such as learning conditions (e.g., study alone or in groups), student characteristics (e.g., age, ability), materials (e.g., simple concepts to problem-based analyses) and criterion tasks (different outcome measures).
In the current study, we independently assigned all strategies to the various parts of the model—this was a straightforward process, and the few minor disagreements were resolved by mutual agreement. All results are presented in Appendix 1.
Results: the meta-synthesis of learning strategies
There are 302 effects derived from the 228 meta-analyses from the above four sources that have related some form of learning strategy to an achievement outcome. Most are experimental–control studies or pre–post studies, whereas some are correlations ( N =37). There are 18,956 studies (although some may overlap across meta-analyses). Only 125 meta-analyses reported the sample size ( N =11,006,839), but if the average (excluding the outlier 7 million from one meta-analysis) is used for the missing sample sizes, the best estimate of sample size is between 13 and 20 million students.
The average effect is 0.53 but there is considerable variance ( Figure 2 ), and the overall number of meta-analyses, studies, number of people (where provided), effects and average effect-sizes for the various phases of the model are provided in Table 1 . The effects are lowest for management of the environment and ‘thrill’ (motivation), and highest for developing success criteria across the learning phases. The variance is sufficiently large, however, that it is important to look at specific strategies within each phase of the model.
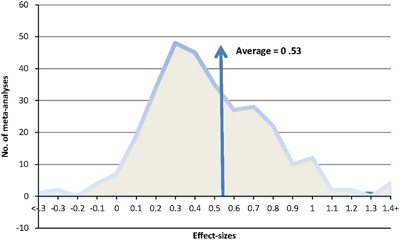
The average and the distribution of all effect sizes.
Synthesis of the input phases of the model
The inputs: skills.
There are nine meta-analyses that have investigated the relation between prior achievement and subsequent achievement, and not surprisingly these relations are high ( Table 2 ). The average effect-size is 0.77 (s.e.=0.10), which translates to a correlation of 0.36—substantial for any single variable. The effects of prior achievement are lowest in the early years, and highest from high school to university. One of the purposes of school, however, is to identify those students who are underperforming relative to their abilities and thus to not merely accept prior achievement as destiny. The other important skill is working memory—which relates to the amount of information that can be retained in short-term working memory when engaged in processing, learning, comprehension, problem solving or goal-directed thinking. 55 Working memory is strongly related to a person’s ability to reason with novel information (i.e., general fluid intelligence. 56
The inputs: will
There are 28 meta-analyses related to the dispositions of learning from 1,304 studies and the average effect-size is 0.48 (s.e.=0.09; Table 3 ). The effect of self-efficacy is highest ( d =0.90), followed by increasing the perceived value of the task ( d = 0.46), reducing anxiety ( d =0.45) and enhancing the attitude to the content ( d =0.35). Teachers could profitably increase students’ levels of confidence and efficacy to tackle difficult problems; not only does this increase the probability of subsequent learning but it can also help reduce students’ levels of anxiety. It is worth noting the major movement in the anxiety and stress literature in the 1980s moved from a preoccupation on understanding levels of stress to providing coping strategies—and these strategies were powerful mediators in whether people coped or not. 57 Similarly in learning, it is less the levels of anxiety and stress but the development of coping strategies to deal with anxiety and stress. These strategies include being taught to effectively regulate negative emotions; 58 increasing self-efficacy, which relates to developing the students conviction in their own competence to attain desired outcomes; 59 focusing on the positive skills already developed; increasing social support and help seeking; reducing self-blame; and learning to cope with error and making mistakes. 60 Increasing coping strategies to deal with anxiety and promoting confidence to tackle difficult and challenging learning tasks frees up essential cognitive resources required for the academic work.
There has been much discussion about students having growth—or incremental—mindsets (human attributes are malleable not fixed) rather than fixed mindsets (attributes are fixed and invariant). 61 However, the evidence in Table 3 ( d =0.19) shows how difficult it is to change to growth mindsets, which should not be surprising as many students work in a world of schools dominated by fixed notions—high achievement, ability groups, and peer comparison.
The inputs: thrill
The thrill relates to the motivation for learning: what is the purpose or approach to learning that the student adopts? Having a surface or performance approach motivation (learning to merely pass tests or for short-term gains) or mastery goals is not conducive to maximising learning, whereas having a deep or achieving approach or motivation is helpful ( Table 4 ). A possible reason why mastery goals are not successful is that too often the outcomes of tasks and assessments are at the surface level and having mastery goals with no strategic sense of when to maximise them can be counter-productive. 62 Having goals, per se , is worthwhile—and this relates back to the general principle of having notions of what success looks like before investing in the learning. The first step is to teach students to have goals relating to their upcoming work, preferably the appropriate mix of achieving and deep goals, ensure the goals are appropriately challenging and then encourage students to have specific intentions to achieve these goals. Teaching students that success can then be attributed to their effort and investment can help cement this power of goal setting, alongside deliberate teaching.
The environment
Despite the inordinate attention, particularly by parents, on structuring the environment as a precondition for effective study, such effects are generally relatively small ( Table 5 ). It seems to make no differences if there is background music, a sense of control over learning, the time of day to study, the degree of social support or the use of exercise. Given that most students receive sufficient sleep and exercise, it is perhaps not surprising that these are low effects; of course, extreme sleep or food deprivation may have marked effects.
Knowing the success criteria
A prediction from the model of learning is that when students learn how to gain an overall picture of what is to be learnt, have an understanding of the success criteria for the lessons to come and are somewhat clear at the outset about what it means to master the lessons, then their subsequent learning is maximised. The overall effect across the 31 meta-analyses is 0.54, with the greatest effects relating to providing students with success criteria, planning and prediction, having intentions to implement goals, setting standards for self-judgements and the difficulty of goals ( Table 6 ). All these learning strategies allow students to see the ‘whole’ or the gestalt of what is targeted to learn before starting the series of lessons. It thus provides a ‘coat hanger’ on which surface-level knowledge can be organised. When a teacher provides students with a concept map, for example, the effect on student learning is very low; but in contrast, when teachers work together with students to develop a concept map, the effect is much higher. It is the working with students to develop the main ideas, and to show the relations between these ideas to allow students to see higher-order notions, that influences learning. Thus, when students begin learning of the ideas, they can begin to know how these ideas relate to each other, how the ideas are meant to form higher order notions, and how they can begin to have some control or self-regulation on the relation between the ideas.
Synthesis of the learning phases of the model
There are many strategies, such as organising, summarising, underlining, note taking and mnemonics that can help students master the surface knowledge ( Table 7 ). These strategies can be deliberately taught, and indeed may be the only set of strategies that can be taught irrespective of the content. However, it may be that for some of these strategies, the impact is likely to be higher if they are taught within each content domain, as some of the skills (such as highlighting, note taking and summarising) may require specific ideas germane to the content being studied.
While it appears that training working memory can have reasonable effects ( d =0.53) there is less evidence that training working memory transfers into substantial gains in academic attainment. 63 There are many emerging and popular computer games that aim to increase working memory. For example, CogMed is a computer set of adaptive routines that is intended to be used 30–40 min a day for 25 days. A recent meta-analysis (by the commercial owners 64 ) found average effect-sizes (across 43 studies) exceed 0.70, but in a separate meta-analysis of 21 studies on the longer term effects of CogMed, there was zero evidence of transfer to subjects such as mathematics or reading 65 . Although there were large effects in the short term, they found that these gains were not maintained at follow up (about 9 months later) and no evidence to support the claim that working memory training produces generalised gains to the other skills that have been investigated (verbal ability, word decoding or arithmetic) even when assessment takes place immediately after training. For the most robust studies, the effect of transfer is zero. It may be better to reduce working memory demands in the classroom. 66
The investment of effort and deliberate practice is critical at this consolidation phase, as are the abilities to listen, seek and interpret the feedback that is provided ( Table 8 ). At this consolidation phase, the task is to review and practice (or overlearn) the material. Such investment is more valuable if it is spaced over time rather than massed. Rehearsal and memorisation is valuable—but note that memorisation is not so worthwhile at the acquisition phase. The difficult task is to make this investment in learning worthwhile, to make adjustments to the rehearsal as it progresses in light of high levels of feedback, and not engage in drill and practice. These strategies relating to consolidating learning are heavily dependent on the student’s proficiency to invest time on task wisely, 67 to practice and learn from this practice and to overlearn such that the learning is more readily available in working memory for the deeper understanding.
Acquiring deeper learning
Nearly all the strategies at this phase are powerful in enhancing learning ( Table 9 ). The ability to elaborate and organise, monitor the uses of the learning strategies, and have a variety of metacognitive strategies are the critical determinants of success at this phase of learning. A major purpose is for the student to deliberately activate prior knowledge and then make relations and extensions beyond what they have learned at the surface phase.
At this phase, the power of working with others is most apparent ( Table 10 ). This involves skills in seeking help from others, listening to others in discussion and developing strategies to ‘speak’ the language of learning. It is through such listening and speaking about their learning that students and teachers realise what they do deeply know, what they do not know and where they are struggling to find relations and extensions. An important strategy is when students become teachers of others and learn from peers, as this involves high levels of regulation, monitoring, anticipation and listening to their impact on the learner.
There has been much research confirming that teaching help-seeking strategies is successful, but how this strategy then works in classrooms is more complex. Teachers have to welcome students seeking help, and there needs to be knowledgeable others (e.g., peers) from whom to seek the help—too often students left in unsupported environments can seek and gain incorrect help and not know the help is incorrect. 68 Ryan and Shin 69 also distinguished between adaptive help seeking (seeking help from others, such as an explanation, a hint, or an example, that would further learning and promote independent problem solving in the future) and expedient help seeking (seeking help that expedites task completion, such as help that provides the answer and is not focused on learning). They showed that adaptive help seeking from peers declines and expedient help seeking increases during early adolescence. Further, increases in expedient help seeking were associated with declines in achievement but changes in adaptive help seeking were unrelated to achievement. The key is for teachers to teach adaptive help seeking, to ensure the help is dependable and correct and to see this more of a student than a teacher skill. Help seeking needs to be welcomed before it can have an effect.
The transfer model promoted by Marton 48 seems to be supported in that a key in teaching for transfer involves understanding the patterns, similarities and differences in the transfer before applying the strategies to new task ( Table 11 ). Marton argued that transfer occurs when students learn strategies that apply in a certain situation such that they are enabled to do the same thing in another situation to the degree that they realise how the second situation does (or does not) resemble the first situation. It is learning to detect differences and similarities that is the key that leads to transfer of learning.
Discussion and Conclusions
There is much debate about the optimal strategies of learning, and indeed we identified >400 terms used to describe these strategies. Our initial aim was to rank the various strategies in terms of their effectiveness but this soon was abandoned. There was too much variability in the effectiveness of most strategies depending on when they were used during the learning process, and thus we developed the model of learning presented in this article. Like all models, it is a conjecture, it aims to say much and it is falsifiable. The efficacy of any model can be seen as an expression of its capacity to generate a scalable solution to a problem or need in ways that resolve more issues than prevailing theories or approaches. 70 The model posits that learning must be embedded in some content (something worth knowing) and thus the current claims about developing 21st century skills sui generis are most misleading. These skills often are promoted as content free and are able to be developed in separate courses (e.g., critical thinking, resilience). Our model, however, suggests that such skills are likely to be best developed relative to some content. There is no need to develop learning strategy courses, or teach the various strategies outside the context of the content. Instead, the strategies should be an integral part of the teaching and learning process, and can be taught within this process.
The model includes three major inputs and outcomes. These relate to what the students bring to the learning encounter (skill), their dispositions about learning (will) and their motivations towards the task (thrill). The first set of strategies relate to teaching students the standards for what is to be learned (the success criteria). We propose that effective learning strategies will be different depending on the phase of the learning—the strategies will be different when a student is first acquiring the matters to be learnt compared with when the student is embedding or consolidating this learning. That is, the strategies are differentially effective depending on whether the learning intention is surface learning (the content), deep learning (the relations between content) or the transfer of the skills to new situations or tasks. In many ways this demarcation is arbitrary (but not capricious) and more experimental research is needed to explore these conjectures. Further, the model is presented as linear whereas there is often much overlap in the various phases. For example, to learn subject matter (surface) deeply (i.e., to encode in memory) is helped by exploring and understanding its meaning; success criteria can have a mix of surface and deep and even demonstrate the transfer to other (real world) situations; and often deep learning necessitates returning to acquire specific surface level vocabulary and understanding. In some cases, there can be multiple overlapping processes. A reviewer provided a clear example: in learning that the internal angles of a quadrilateral add up to 360°, this might involve surface learning, which then requires rehearsal to consolidate, some self-questioning to apply, some detection of similarities to then work out what the internal angles of a hexagon might be, and spotting similarities to the triangle rule. There may be no easy way to know the right moment, or no easy demarcation of the various phases. The proposal in this paper is but a ‘model’ to help clarify the various phases of learning, and in many real world situations there can be considerable overlap.
We have derived six sets of propositions from our conceptual model of learning and the results of our meta-synthesis of research on learning strategies. The first set relates to the differential role played by what students bring to and take from the learning encounter—the inputs and outcomes. Second, there are some strategies that are more effective than others—but their relative effectiveness depends on the phase in the model of learning in which they take place. Third is the distinction between surface learning, deep learning and the transfer of learning. The fourth set relates to the skills of transfer, the fifth to how the model of learning can be used to resolve some unexpected findings about the effectiveness of some strategies, and the sixth set discusses the question ‘what is learning?’.
The intertwining role of skill, will, and thrill
Our first set of claims relates to the differential role of what students bring to and take from the learning encounter. Rather than arguing that many factors contribute to achievement (an important but sometimes the only privileged outcome of learning), we are promoting the notion that the skill, will and thrill can intertwine during learning and that these three inputs are also important outcomes of learning—the aim is to enhance the will (e.g., the willingness to reinvest in more and deeper learning), the thrill (e.g., the emotions associated with successful learning, the curiosity and the willingness to explore what one does not know) and the skills (e.g., the content and the deeper understanding). The relation between the thrill, will and skill can vary depending on the student and the requirements of the task. Certainly, negative emotions, such as those induced by fear, anxiety, and stress can directly and negatively affect learning and memory. Such negative emotions block learning: ‘If the student is faced with sources of stress in an educational context which go beyond the positive challenge threshold—for instance, aggressive teachers, bullying students or incomprehensible learning materials whether books or computers—it triggers fear and cognitive function is negatively affected. 71 Our argument is that learning can lead to enhanced skills, dispositions, motivations and excitements that can be reinvested in learning, and can lead to students setting higher standards for their success criteria. When skill, will, and thrill overlap, this should be considered a bonus; developing each is a worthwhile outcome of schooling in its own right.
It is all in the timing
Our second set of claims is that while it is possible to nominate the top 10 learning strategies the more critical conclusion is that the optimal strategies depend on where in the learning cycle the student is located. This strategic skill in using the strategies at the right moment is akin to the message in the Kenny Rogers song—you need to ‘know when to hold ‘em, know when to fold ‘em’. For example, when starting a teaching sequence, it is most important to be concerned that students have confidence they can understand the lessons, see value in the lessons and are not overly anxious about their skills to be mastered. Providing them early on with an overview of what successful learning in the lessons will look like (knowing the success criteria) will help them reduce their anxiety, increase their motivation, and build both surface and deeper understandings.
To acquire surface learning, it is worthwhile knowing how to summarise, outline and relate the learning to prior achievement; and then to consolidate this learning by engaging in deliberate practice, rehearsing over time and learning how to seek and receive feedback to modify this effort. To acquire deep understanding requires the strategies of planning and evaluation and learning to monitor the use of one’s learning strategies; and then to consolidate deep understanding calls on the strategy of self-talk, self-evaluation and self-questioning and seeking help from peers. Such consolidation requires the learner to think aloud, learn the ‘language of thinking’, 72 know how to seek help, self-question and work through the consequences of the next steps in learning. To transfer learning to new situations involves knowing how to detect similarities and differences between the old and the new problem or situations.
We recommend that these strategies are developed by embedding them into the cycle of teaching rather than by running separate sessions, such as ‘how to learn’ or study skills courses. There is a disappointing history of educational programs aimed at teaching students how to learn. 30 , 73 , 74 Wiliam 75 made this case for why teaching these learning strategies (e.g., critical thinking) out of context is unlikely to develop a generic skill applicable to many subjects. He noted that in a ‘mathematics proof, critical thinking might involve ensuring that each step follows from the previous one (e.g., by checking that there has not been a division by zero). In reading a historical account, critical thinking might involve considering the author of the account, the potential biases and limitations that the author may be bringing to the account, and what other knowledge the reader has about the events being described. The important point here is that although there is some commonality between the processes in mathematics and history, they are not the same. Developing a capacity for critical thinking in history does not make one better at critical thinking in mathematics. For all of the apparent similarities, critical thinking in history and critical thinking in mathematics are different, and they are developed in different ways’. Many others have noted that metacognition is not knowledge-free but needs to be taught in the context of the individual subject areas. 76 , 77 Perkins 78 also noted that there is a certain art to infusing the teaching of thinking into content learning. Sometimes, ‘teachers think it is enough simply to establish a generally thoughtful atmosphere in a classroom, with regular expectations for thinking critically and creatively...teaching for know-how about learning to learn is a much more time-consuming enterprise than teaching for just learning the ideas... Building active know-how requires much more attention’.
Another aspect to consider is the difference, identified in the model, between being first exposed to learning and the consolidation of this learning. This distinction is far from novel. Shuell, 79 for example, distinguished between initial, intermediate, and final phases of learning. In the initial phase, the students can encounter a ‘large array of facts and pieces of information that are more-or-less isolated conceptually... there appears to be little more than a wasteland with few landmarks to guide the traveller on his or her journey towards understanding and mastery’. Students can use existing schema to make sense of this new information, or can be guided to have more appropriate schema (and thus experience early stages of concept learning and relation between ideas) otherwise the information may remain as isolated facts, or be linked erroneously to previous understandings. At the intermediate phase, the learner begins to see similarities and relationships among these seemingly conceptually isolated pieces of information. ‘The fog continues to lift but still has not burnt off completely’. During the final phase, the knowledge structure becomes well integrated and functions more autonomously, and the emphasis is more on performance or exhibiting the outcome of learning.
Horses for courses: matching strategies with phases
The third set of claims relates to the distinction between surface, deep, and transfer of learning. Although not a hard and fast set of demarcations, surface learning refers more to the content and underlying skills; deep learning to the relationships between, and extensions of, ideas; and transfer to the proficiency to apply learning to new problems and situations. During the surface learning phase, an aim is to assist students to overlearn certain ideas and thus reduce the needs of their working memory to work with these new facts when moving into the deeper understanding phase. Note, for example, that Marton et al. 80 made an important distinction between memorising without understanding first and called this rote memorisation (which has long term effect), and memorisation when you have understood and called this meaningful memorisation (which can be powerful). The evidence in the current study supports this distinction.
It is when students have much information, or many seemingly unrelated ideas, that the learning strategies for the deep phase are optimally invoked. This is when they should be asked to integrate ideas with previous schema or modify their previous schema to integrate new ideas and ways of thinking. The key to this process is first gaining ideas—a fact often missed by those advocating deeper thinking strategies when they try to teach these skills prior to developing sufficient knowledge within the content domain. The students need to first have ideas before they can relate them. The model does not propose discarding the teaching or learning skills that have been developed to learn surface knowing, but advocates the benefits of a more appropriate balance of surface and deeper strategies and skills that then lead to transfer. The correct balance of surface to deep learning depends on the demands of the task. It is likely that more emphasis on surface strategies is probably needed as students learn new ideas, moving to an emphasis on deeper strategies as they become more proficient.
Pause and reflect: detecting similarities and differences
The fourth set of claims relate to the skills of transfer, and how important it is to teach students to pause and detect the similarities and differences between previous tasks and the new one, before attempting to answer a new problem. Such transfer can be positive, such as when a learner accurately remembers a learning outcome reached in a certain situation and appropriately applies it in a new and similar situation, or negative, such as when a learner applies a strategy used successfully in one situation in a new situation where this strategy is not appropriate. Too many (particularly struggling) students over-rehearse a few learning strategies (e.g., copying and highlighting) and apply them in situations regardless of the demands of new tasks. Certainly, the fundamental skill for positive transfer is stopping before addressing the problem and asking about the differences and similarities of the new to any older task situation. This skill can be taught.
This ability to notice similarities and differences over content is quite different for novices and experts 81 , 82 and we do not simply learn from experience but we also learn to experience. 83 Preparation for future learning involves opportunities to try our hunches in different contexts, receive feedback, engage in productive failure and learn to revise our knowing based on feedback. The aim is to solve problems more efficiently, and also to ‘let go’ of previously acquired knowledge in light of more sophisticated understandings—and this can have emotional consequences: ‘Failure to change strategies in new situations has been described as the tyranny of success’. 84 It is not always productive for students to try the same thing that worked last time. Hence there may need to be an emphasis on knowledge-building rather than knowledge-telling, 85 and systematic inquiry based on theory-building and disconfirmation rather than simply following processes for how to find some result.
Why some strategies do not work
The fifth set of claims relate to how the model can be used to resolve some of the unexpected findings about the impact of various teaching methods. In Visible Learning , 36 it was noted that many programs that seem to lead to developing deeper processing have very low effect sizes (e.g., inquiry based methods, d =0.31; problem-based learning, d =0.15). For example, there have been 11 meta-analyses relating to problem-based learning based on 509 studies, leading to an average small effect ( d =0.15). It hardly seems necessary to run another problem-based program (particularly in first-year medicine, where four of the meta-analyses were completed) to know that the effects of problem-based learning on outcomes are small. The reason for this low effect seems to be related to using problem-based methods before attaining sufficient surface knowledge. When problem-based learning is used in later medical years, the effects seem to increase. Albanese and Mitchell 86 claimed that increased years of exposure to medical education increases the effect of problem-based learning. They argued that lack of experience (and lack of essential surface knowledge) leads the student to make more errors in their knowledge base, add irrelevant material to their explanations and engage in backward reasoning (from the unknown to the givens), whereas experts engaged in forward reasoning (also see references 87 , 88 ). Walker et al. 89 also noted that novice problem-based learning students tended to engage in far more backward-driven reasoning, which results in more errors during problem solving and may persist even after the educational intervention is complete. It is likely that problem-based learning works more successfully when students engage in forward reasoning and this depends on having sufficient content knowledge to make connections.
Deep understanding in problem-based learning requires a differentiated knowledge structure, 90 and this may need to be explicitly taught—as there is no assumption that students will see similarities and differences in contexts by themselves. There is a limit to what we can reasonably expect students to discover, and it may require teaching students to make predictions based on features that were told to them and that they may not notice on their own. Deliberate teaching of these surface features can offer a higher level of explanation that would be difficult or time consuming to discover. A higher level explanation is important because it provides a generative framework that can extend one understanding beyond the specific cases that have been analysed and experienced. On the other hand, the problems need not be too overly structured, as then students do not gain experience of searching out conceptual tools or homing in on particular cases of application. 78
Another example of the different requirements of surface and deep learning is the effect of asking students to explore errors and misconceptions during their learning. Using meta-analysis, Keith and Frese 91 found that the average effect of using these strategies when the outcome was surface learning was −0.15 and when the outcome was deep learning and far transfer to new problems, it was 0.80.
So: what is learning?
The sixth set of claims relate to the notion of ‘what is learning?’. The argument in this article is that learning is the outcome of the processes of moving from surface to deep to transfer. Only then will students be able to go beyond the information given to ‘figure things out’, which is one of the few untarnishable joys of life. 92 One of the greatest triumphs of learning is what Perkins 78 calls ‘knowing one’s way around’ a particular topic or ‘playing the whole game’ of history, mathematics, science or whatever. This is a function of knowing much and then using this knowledge in the exploration of relations and to make extensions to other ideas, and being able to know what to do when one does not know what to do (the act of transfer).
Concluding comments
Like all models, the one proposed in this article invites as many conjectures and directions for further research as it provide a basis for interpreting the evidence from the meta-synthesis. It helps make sense of much of the current literature but it is speculative in that it also makes some untested predictions. There is much solace in Popper's 93 claim that ‘Bold ideas, unjustified anticipations, and speculative thought, are our only means for interpreting nature: our only organon, our only instrument, for grasping her. And we must hazard them to win our prize. Those among us who are unwilling to expose their ideas to the hazard of refutation do not take part in the scientific game.’ Further research is needed, for example, to better understand the optimal order through the various phases; there may be circumstances where it may be beneficial to learn the deeper notions before developing the surface knowledge. It is highly likely that as one develops many ideas and even relates and extends them, these become ‘ideas’ and the cycle continues. 94 We know much, but we need to know much more, and in particular we need to know how these many learning strategies might be better presented in another competing model. Such testing of a bold model and making predictions from models is, according to Popper, how science progresses.
Further research is needed that asks whether the distinction between the acquisition and the consolidation of learning is a distinctive difference, a melding from one to the other or whether both can occur simultaneously. If there is a difference, then more research on ascertaining the best time to move from acquisition to consolidation would be informative. Similarly, there is no hard rule in the model of a sequence from surface to deep to transfer. In some ways, teaching the strategies of knowing what success looks like upfront implies an exposure to both surface and deep learning. Also, the many arguments (but surprisingly there is a lack of evidence) for the popular notions of flipped classrooms could be supported with more evidence of introducing the success criteria upfront to students. A typical flipped lesson starts with students accessing online video lectures or resources prior to in-class sessions so that students are prepared to participate in more interactive and higher-order activities such as problem solving, discussions and debates. 95 The most needed research concerns transfer—the variation theory of Marton, 48 the claims by Perkins 78 and others need more focused attention and the usual (and often unsubstantiated) claims that doing x will assist learning y should come back as a focus of learning sciences.
We are proposing that it is worthwhile to develop the skill, will and thrill of learning, and that there are many powerful strategies for learning. Students can be taught these strategies (declarative knowledge), how to use them (procedural knowledge), under what conditions it may be more or less useful to apply them (conditional knowledge) and how to evaluate them. It may be necessary to teach when best to use these strategies according the nature of the outcomes (surface and deep), according to the timing of learning (first acquiring and then consolidating learning) and to teach the skill of transferring learning to new situations. We need to think in terms of ‘surface to deep’ and not one alone; we need to think in terms of developing dispositions, motivations and achievement, and not one alone. This invites considering multiple outcomes from our schools. Singapore, 96 for example, is now committed to developing an educational system which will produce young people who have the moral courage to stand up for what is right; pursue a healthy lifestyle and have an appreciation of aesthetics; are proud to be Singaporeans; are resilient in the face of difficulty, innovative and enterprising; are purposeful in the pursuit of excellence; are able to collaborate across cultures; and can think critically and communicate persuasively. Academic achievement is but one desirable learning outcomes of many.
Another important message is that developing a few learning strategies may not be optimal. The failure to change strategies in new situations has been described as the tyranny of success; 84 and the current meta-synthesis suggests that choosing different strategies as one progresses through the learning cycle (from first exposure to embedding, from surface to deep to transfer) demands cognitive flexibility. It may not be the best option for students to use the same strategies that worked last time, as when the context is changed the old strategies may no longer work.
Widdowson, D. A., Dixon, R. S., Peterson, E. R., Rubie-Davies, C. M. & Irving, S. E. Why go to school? Student, parent and teacher beliefs about the purposes of schooling. Asia Pac. J. Educ. 35 , 1–14 (2014).
Google Scholar
Biesta, G. J. Good Education in an Age of Measurement: Ethics, Politics, democracy , (Routledge, 2015).
Tröhler, D., Meyer, H. D., Labaree, D. F. & Hutt, E. L. Accountability: antecedents, power, and processes. Teach. Coll. Rec. 116 , 1–12 (2014).
Soderstrom, N. C. & Bjork, R. A. Learning versus performance: an integrative review. Perspect. Psychol. Sci. 10 , 176–199 (2015).
Article Google Scholar
Mayer, R. E. Applying the science of learning: Evidence-based principles for the design of multimedia instruction. Am. Psychol. 63 , 760–769 (2008).
Pressley, M. Comprehension Instruction: Research-Based Best Practices 11–27 (Guilford Press, 2002).
Weinstein, C. E. & Mayer, R. E. in Handbook of Research on Teaching: a Project of the American Educational Research Association (ed. Wittrock, M. C.) 3rd edn., 315–327 (Macmillan, 1986).
Boekaerts, M. Self-regulated learning: a new concept embraced by researchers, policy makers, educators, teachers, and students. Learn. Instruct. 7 , 161–186 (1997).
Dignath, C., Buettner, G. & Langfeldt, H. P. How can primary school students learn self-regulated learning strategies most effectively? A meta-analysis on self-regulation training programmes. Educ. Res. Rev. 3 , 101–129 (2008).
Pressley, M., Goodchild, F., Fleet, J., Zajchowski, R. & Evans, E. D. The challenges of classroom strategy instruction. The Elementary School Journal 89 , 301–342 (1989).
Hattie, J. A. C. The 34th Vernon-Wall Lecture (British Psychological Society, 2014).
Ausubel, D. P. Educational Psychology: a Cognitive View (Holt, Rinehart, & Winston, 1968).
Claxton, G. What's the Point of School? Rediscovering the Heart of Education (Oneworld Publications, 2013).
Carr, M. & Claxton, G. Tracking the development of learning dispositions. Assessment in Education: Principles, Policy & Practice 9 , 9–37 (2002).
Perkins, D. N., Jay, E. & Tishman, S. Beyond abilities: A dispositional theory of thinking. Merrill-Palmer Quarterly 39 , 1–21 (1993).
Anderman, E. M. & Patrick, H. in Handbook of Research on Student Engagement , 173–191 (Springer, USA, 2012).
Elliot, A. J. & Harackiewicz, J. M. Approach and avoidance achievement goals and intrinsic motivation: A mediational analysis. J. Personal. Soc. Psychol. 70 , 461–475 (1996).
Middleton, M. J. & Midgley, C. Avoiding the demonstration of lack of ability: An underexplored aspect of goal theory. J. Educ. Psychol. 89 , 710 (1997).
Skaalvik, E. M. in Advances in Motivation and Achievement (eds Maehr, M. L. & Pintrich, P. R.) 51–97 (JAI Press, 1997).
Carpenter, S. L. A Comparison of the Relationships of Students' Self-Efficacy, Goal Orientation, and Achievement Across Grade Levels: a Meta-Analysis (Unpublished doctoral dissertation Faculty of Education, Simon Fraser Univ., 2007).
Hulleman, C. S., Schrager, S. M., Bodmann, S. M. & Harackiewicz, J. M. A meta-analytic review of achievement goal measures: Different labels for the same constructs or different constructs with similar labels? Psychol. Bull. 136 , 422 (2010).
Biggs, J. B. What do inventories of students’ learning processes really measure? A theoretical review and clarification. British J. Educ. Psychol. 63 , 3–19 (1993).
Clarke, S. Formative assessment in the secondary classroom (Hodder Murray, 2005).
Heritage, M. Formative assessment: What do teachers need to know and do? Phi Delta Kappan 89 , 140–145 (2007).
Boekaerts, M. Self-regulated learning: Where we are today. Int. J. Educ. Res. 31 , 445–457 (1999).
Entwistle, N. J. The verb ‘to learn’takes the accusative. Br. J. Educ. Psychol. 46 , 1–3 (1976).
Biggs, J. B. & Collis, K. F. Evaluating the Quality of Learning: the SOLO Taxonomy (Structure of the Observed Learning Outcome) (Academic Press, 1982).
Hattie, J. A. C. & Brown, G. T. L. Cognitive processes in asTTle: the SOLO taxonomy. asTTle technical report (No. 43) (University of Auckland & Ministry of Education, 2004).
Enwistle, N. J. Approaches to studying and levels of understanding: the influences of teaching and assessment . High. Educ. 15 , 156–218. (2000).
Mayer, R. E. The Cambridge Handbook of Multimedia Learning , 2nd edn, 43–71 (Cambridge Univ. Press, 2014).
Robertson, E. M., Pascual-Leone, A. & Miall, R. C. Current concepts in procedural consolidation. Nat. Rev. Neurosci. 5 , 576–582 (2004).
Article CAS Google Scholar
Hattie, J. A. C., Biggs, J. & Purdie, N. Effects of learning skills interventions on student learning: A meta-analysis. Rev. Educ. Res. 66 , 99–136 (1996).
Bjork, R. A. & Bjork, E. L. in Learning Processes to Cognitive Processes: essays in Honor of William K. Estes (eds Healy, A. et al. ) Vol. 2, 35–67 (Erlbaum, 1992).
Bjork, E. L., de Winstanley, P. A. & Storm, B. C. Learning how to learn: can experiencing the outcome of different encoding strategies enhance subsequent encoding? Psychon. Bull. Rev. 14 , 207–211 (2007).
von Stumm, S., Chamorro-Premuzic, T. & Ackerman, P. L. in Wiley-Blackwell Handbook of Individual Differences (eds Chamorr-Premuzic, T. et al. ) 217–241 (Wiley-Blackwell, 2011).
Hattie, J. A. C. Visible Learning: a Synthesis of Over 800 Meta-Analyses Relating to Achievement (Routledge, 2009).
Purdie, N. & Hattie, J. A. C. Assessing students’ conceptions of learning. Austr. J. Dev. Educ. Psychol. 2 , 17–32 (2002).
Pintrich, P. R. Multiple goals, multiple pathways: the role of goal orientation in learning and achievement. J. Educ. Psychol. 92 , 544–55 (2000).
Schraw, G. & Dennison, R. S. Assessing metacognitive awareness. Contemp. Educ. Psychol. 19 , 460–475 (1994).
Pegg, J. & Tall, D. in Theories of Mathematics Education 173–192 (Springer, 2010).
Perkins, D. N. & Salomon, G. Knowledge to go: a motivational and dispositional view of transfer. Educ. Psychol. 47 , 248–258 (2012).
Smedslund, J. The problem of “what is learned?”. Psychol. Rev. 60 , 157–158 (1953).
Barnett, S. M. & Ceci, S. J. When and where do we apply what we learn? A taxonomy for far transfer. Psychol. Bull. 128 , 612–637 (2002).
Salomon, G. & Perkins, D. N. Rocky roads to transfer: rethinking mechanism of a neglected phenomenon. Educ. Psychol. 24 , 113–142 (1989).
Osgood, C. E. The similarity paradox in Hum. Learn.: A resolution. Psychol. Rev. 56 , 132–143 (1949).
Bereiter, C. & Scardamalia, M. in Knowledge Creation in Education (eds Tan, S. C. et al. ) 35–52 (Springer, 2014).
Schoenfeld, A. H. in Handbook of Research on Mathematics Learning and Teaching (ed. Grouws, D. A.) 334–370 (Macmillan, 1992).
Marton, F. Sameness and difference in transfer. J. Learn. Sci. 15 , 499–535 (2006).
Hattie, J. A. C. Visible Learning for Teachers (Routledge, 2012).
Book Google Scholar
Hattie, J. A. C. The applicability of Visible Learning to higher education. Scholarship Teach. Learn. Psychol. 1 , 79–91 (2015).
Lavery, L. Self-regulated Learning For Academic Success: an Evaluation Of Instructional Techniques (Unpublished doctoral thesis Univ. Auckland, 2008).
Donoghue, G. & Hattie, J. A. C. A Meta-Analysis of Learning Strategies based on Dunlosky et al. (Unpublished paper Science of Learning Research Centre, 2015).
Dunlosky, J., Rawson, K. A., Marsh, E. J., Nathan, M. J. & Willingham, D. T. Improving students’ learning with effective learning techniques promising directions from cognitive and educational psychology. Psychol. Sci. Public Interest 14 , 4–58 (2013).
Donker, A. S., de Boer, H., Kostons, D., van Ewijk, C. D. & van der Werf, M. P. C. Effectiveness of learning strategy instruction on academic performance: A meta-analysis. Educ. Res. Rev. 11 , 1–26 (2013).
Cowan, N. in Neuroscience in Education: the Good, the Bad and the Ugly (eds Della Sala, S. & Anderson, M.) 111–127 (Oxford Univ. Press, 2012).
Ackerman, P. L., Beier, M. E. & Boyle, M. O. Working memory and intelligence: The same or different constructs? Psychol. Bull. 131 , 30–60 (2005).
Frydenberg, E. & Lewis, R. Coping Scale for Adults (ACER, 2015).
Galla, B. M. & Wood, J. J. Emotional self-efficacy moderates anxiety-related impairments in math performance in elementary school-age youth. Pers. Indiv. Differ. 52 , 118–122 (2012).
Bandura, A. Self-efficacy: the Exercise of Control (Macmillan, 1997).
Frydenberg, E. Think positively!: a Course For Developing Coping Skills In Adolescents (A&C Black, 2010).
Dweck, C. Mindset: How You Can Fulfil Your Potential (Hachette, 2012).
Watkins, D. & Hattie, J. A. A longitudinal study of the approaches to learning of Australian Tertiary students. Hum. Learn. 4 , 127–141 (1985).
Turley-Ames, K. J. & Whitfield, M. M. Strategy training and working memory task performance. J. Mem. Lang. 49 , 446–468 (2003).
Owen, A. M. et al. Putting brain training to the test. Nature 465 , 775–778 (2010).
Melby-Lervåg, M. & Hulme, C. Is working memory training effective? A meta-analytic review. Dev. Psychol. 49 , 270–291 (2013).
Alloway, T. P. How does working memory work in the classroom? Educ. Res. Rev. 1 , 134–139 (2006).
Claessens, B. J., Van Eerde, W., Rutte, C. G. & Roe, R. A. Things to do today: a daily diary study on task completion at work. Appl. Psychol. 59 , 273–295 (2010).
Nuthall, G. A. The Hidden Lives of Learners (New Zealand Council for Educational Research, 2007).
Ryan, A. M. & Shin, H. Help-seeking tendencies during early adolescence: an examination of motivational correlates and consequences for achievement. Learn. Instruct. 21 , 247–256 (2011).
Kuhn, T. S. The structure of scientific revolutions (The University of Chicago Press, 1962).
CERI. 40th Anniversary International Conference on Learning in the 21st century , May 2008. (2008).
Zohar, A. in Metacognition in Science Education 197–223 (Springer, 2012).
Lockhead J. & Clement J. (eds) Research on Teaching Thinking Skills (Franklin Institute Press, 1979).
Mayer, R. E. Multimedia learning: Are we asking the right questions? Educ. Psychol. 32 , 1–19 (1997).
Wiliam, D. Presentation to the Salzburg Global Seminar. Available at www.dylanwiliam.org/Dylan_Wiliams.../Salzburg%20Seminar%20talk (2014).
Donovan M. S. & Bransford J. D. (eds). How Students Learn: History in the Classroom (National Academies Press, 2005).
Vye, N., Schwartz, D. L., Bransford, J. D., Barron, B. J. & Zech, L. in Metacognition in Educational Theory And Practice (eds Dunlosky, H. & Graesser, A.) 305–347 (Lawrence Erlbaum, 1998).
Perkins, D. Future Wise: Educating our Children for a Changing World (John Wiley & Sons, 2014).
Shuell, T. J. Teaching and learning as problem solving. Theor. Pract. 29 , 102–108 (1990).
Marton, F., Wen, Q. & Wong, K. C. ‘Read a hundred times and the meaning will appear’. Changes in Chinese university students’ views of the temporal structure of learning. High. Educ. 49 , 291–318 (2005).
Chase, W. G. & Simon, H. A. Perception in chess. Cogn. Psychol. 4 , 55–81 (1973).
Berliner, D. C. Learning about and learning from expert teachers. Int. J. Educ. Res. 35 , 463–482 (2001).
Bransford, J. D., Brown, A. L. & Cocking, R. R. How People Learn: Brain, Mind, Experience, and School (National Academy Press, 1999).
Robinson, A. G., Stern, S. & Stern, S. Corporate creativity: How Innovation and Improvement Actually Happen (Berrett-Koehler Publishers, 1997).
Bereiter, C. & Scardamalia, M. An Inquiry Into the Nature and Implications of Expertise , (Open Court, 1993).
Albanese, M. A. & Mitchell, S. Problem-based learning: A review of literature on its outcomes and implementation issues. Acad. Med. 68 , 52–81 (1993).
Gijbels, D., Dochy, F., Van den Bossche, P. & Segers, M. Effects of problem-based learning: A meta-analysis from the angle of assessment. Rev. Educ. Res. 75 , 27–61 (2005).
Gilhooly, K. J. Cognitive psychology and medical diagnosis. Appl. Cogn. Psychol. 4 , 261–272 (1990).
Walker, A. & Leary, H. M. A problem based learning meta-analysis: differences across problem types, implementation types, disciplines, and assessment levels. Interdiscipl. J. Probl. Based Learn. 3 , 12–43 (2009).
Schwartz, D. L. & Bransford, J. D. A time for telling. Cogn. Instruct. 16 , 475–522 (1998).
Keith, N. & Frese, M. Effectiveness of error management training: A meta-analysis. J. Appl. Psychol. 93 , 59 (2008).
Bruner, J. S. The Culture of Education (Harvard Univ. Press, 1996).
Popper, K. R. The Logic of Scientific Discovery , 3rd edn (Hutchinson, 1968).
Pegg, J. in Encyclopedia of Mathematics Education , 570–572 (Springer, 2014).
DeWitt, P. M. Flipping Leadership Doesn’t Mean Reinventing the Wheel (Corwin Press, 2014).
Tan, C. Y. & Dimmock, C. How a ‘top performing’ Asian school system formulates and implements policy: The case of Singapore. Educ. Manage. Admin. Leadership 42 , 743–763 (2014).
Download references
Acknowledgements
The Science of Learning Research Centre is a Special Research Initiative of the Australian Research Council. Project Number SR120300015. We thank the following for critiquing earlier drafts of this article: Dan Willingham, Jason Lodge, Debra Masters, Rob Hester, Jared Horvath and Luke Rowe.
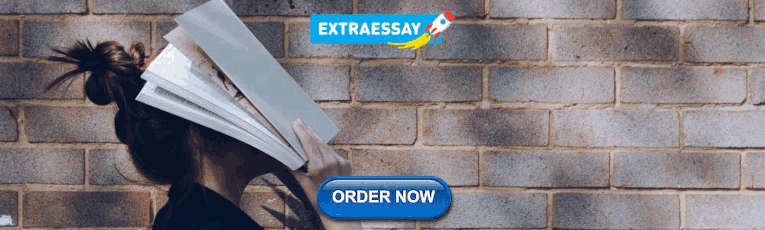
Author information
Authors and affiliations.
Science of Learning Research Centre, Graduate School of Education, University of Melbourne, Carlton, VIC, Australia
John A C Hattie & Gregory M Donoghue
You can also search for this author in PubMed Google Scholar
Contributions
The authors contributed equally to the project and writing of this paper.
Corresponding author
Correspondence to John A C Hattie .
Ethics declarations
Competing interests.
The authors declare no conflict of interest.
Supplementary information
Supplementary appendix (doc 899 kb), rights and permissions.
This work is licensed under a Creative Commons Attribution 4.0 International License. The images or other third party material in this article are included in the article’s Creative Commons license, unless indicated otherwise in the credit line; if the material is not included under the Creative Commons license, users will need to obtain permission from the license holder to reproduce the material. To view a copy of this license, visit http://creativecommons.org/licenses/by/4.0/
Reprints and permissions
About this article
Cite this article.
Hattie, J., Donoghue, G. Learning strategies: a synthesis and conceptual model. npj Science Learn 1 , 16013 (2016). https://doi.org/10.1038/npjscilearn.2016.13
Download citation
Received : 30 December 2015
Revised : 12 April 2016
Accepted : 23 May 2016
Published : 10 August 2016
DOI : https://doi.org/10.1038/npjscilearn.2016.13
Share this article
Anyone you share the following link with will be able to read this content:
Sorry, a shareable link is not currently available for this article.
Provided by the Springer Nature SharedIt content-sharing initiative
This article is cited by
Effect of instruction and experience on students’ learning strategies.
- Ezgi Melisa Yüksel
- C. Shawn Green
- Haley A. Vlach
Metacognition and Learning (2024)
Where is Ethics in the Evolution of AIED’s Future?
- Arthur C. Graesser
- Colin M. Carmon
International Journal of Artificial Intelligence in Education (2024)
Analyzing the associations between motivation and academic performance via the mediator variables of specific mathematic cognitive learning strategies in different subject domains of higher education
- Steffen Wild
- Christoph Neef
International Journal of STEM Education (2023)
Teachers’ assessment of self-regulated learning: Linking professional competences, assessment practices, and judgment accuracy
- Yves Karlen
- Kerstin Bäuerlein
- Sabrina Brunner
Social Psychology of Education (2023)
Quick links
- Explore articles by subject
- Guide to authors
- Editorial policies
Sign up for the Nature Briefing newsletter — what matters in science, free to your inbox daily.

Physical Review Physics Education Research
- Collections
- Editorial Team
- Open Access
Conceptual problem solving in high school physics
Jennifer l. docktor, natalie e. strand, josé p. mestre, and brian h. ross, phys. rev. st phys. educ. res. 11 , 020106 – published 1 september 2015.
- Citing Articles (51)
Supplemental Material
- INTRODUCTION
- RESULTS AND DISCUSSION
- GENERAL DISCUSSION
- ACKNOWLEDGMENTS
Problem solving is a critical element of learning physics. However, traditional instruction often emphasizes the quantitative aspects of problem solving such as equations and mathematical procedures rather than qualitative analysis for selecting appropriate concepts and principles. This study describes the development and evaluation of an instructional approach called Conceptual Problem Solving (CPS) which guides students to identify principles, justify their use, and plan their solution in writing before solving a problem. The CPS approach was implemented by high school physics teachers at three schools for major theorems and conservation laws in mechanics and CPS-taught classes were compared to control classes taught using traditional problem solving methods. Information about the teachers’ implementation of the approach was gathered from classroom observations and interviews, and the effectiveness of the approach was evaluated from a series of written assessments. Results indicated that teachers found CPS easy to integrate into their curricula, students engaged in classroom discussions and produced problem solutions of a higher quality than before, and students scored higher on conceptual and problem solving measures.
- Received 30 April 2015
DOI: https://doi.org/10.1103/PhysRevSTPER.11.020106

This article is available under the terms of the Creative Commons Attribution 3.0 License . Further distribution of this work must maintain attribution to the author(s) and the published article’s title, journal citation, and DOI.
Published by the American Physical Society
Authors & Affiliations
- 1 Department of Physics, University of Wisconsin–La Crosse, La Crosse, Wisconsin 54601, USA
- 2 Department of Physics, University of Illinois, Urbana, Illinois 61801, USA
- 3 Beckman Institute for Advanced Science and Technology, University of Illinois, Urbana, Illinois 61801, USA
- 4 Department of Educational Psychology, University of Illinois, Champaign, Illinois 61820, USA
- 5 Department of Psychology, University of Illinois, Champaign, Illinois 61820, USA
- * [email protected]
Article Text
Vol. 11, Iss. 2 — July - December 2015
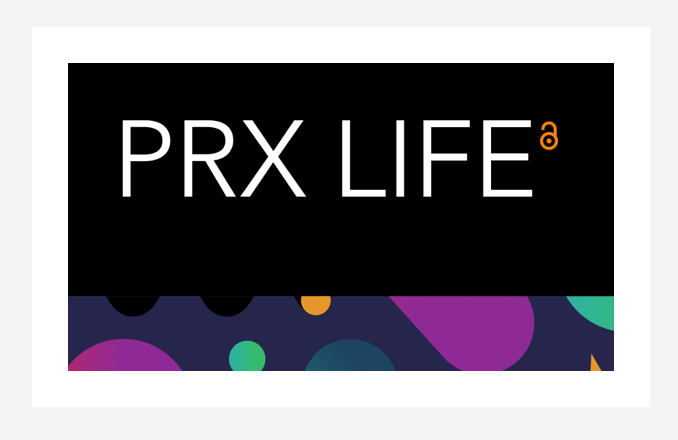
Authorization Required
Other options.
- Buy Article »
- Find an Institution with the Article »
Download & Share
Sample problem and strategy (principle-justification-plan).
Sample two-column solution.
Sign up to receive regular email alerts from Physical Review Physics Education Research
Reuse & Permissions
It is not necessary to obtain permission to reuse this article or its components as it is available under the terms of the Creative Commons Attribution 3.0 License . This license permits unrestricted use, distribution, and reproduction in any medium, provided attribution to the author(s) and the published article's title, journal citation, and DOI are maintained. Please note that some figures may have been included with permission from other third parties. It is your responsibility to obtain the proper permission from the rights holder directly for these figures.
- Forgot your username/password?
- Create an account
Article Lookup
Paste a citation or doi, enter a citation.
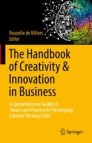
The Handbook of Creativity & Innovation in Business pp 197–221 Cite as
Creative Thinking, Problem Solving and Ideation Tools
- Rouxelle de Villiers 2
- First Online: 05 August 2022
2727 Accesses
It is a myth that creative genii sit quietly hoping for inspiration and insight come to them in a flash of illumination. Some discoveries are either by accident or the result of some form of creative “spark of genius”, but for most novel, valuable business solutions, problem-solving often starts with a design challenge/problem or opportunity. Thanks to decades of research and tried-and-tested tools applied in a range of domains and business disciplines, a treasure trove of creative problem-solving tools and techniques is available to creatives and innovators. These tools turn problems into possibilities, and provide opportunities to develop ideas, processes, products, or procedures that are new to that job, team or organization. Business Schools and industries have no shortage of models, frameworks and tools to improve business effectiveness or generate new and interesting problem solutions for clients. Thinking tools have merit and include divergent or convergent (or both) thinking techniques. We cover thirteen of the most well-known and useful tools and techniques in this chapter. There are many more, but the scope of this book limits what can be covered here.
- Analogical thinking
- Brainstorming
- Convergent thinking
- Divergent thinking
- Facilitation
- Lateral thinking
- Mind-mapping
- Problem-solving tools
- Six thinking hats
This is a preview of subscription content, log in via an institution .
Buying options
- Available as PDF
- Read on any device
- Instant download
- Own it forever
- Available as EPUB and PDF
- Compact, lightweight edition
- Dispatched in 3 to 5 business days
- Free shipping worldwide - see info
- Durable hardcover edition
Tax calculation will be finalised at checkout
Purchases are for personal use only
Read the book by Stokes, P.D. (2006). Creativity from Constraints about the various disciplines, from literature to fashion, advertising and architecture, to music. ISBN 0–8261–7845-6
Osborn AF. Applied imagination. New York: Charles Schribner’s Sons; 1953.
Google Scholar
Janis IL. Groupthink. IEEE Eng Manag Rev. 2008;36(1):36.
Article Google Scholar
Paulus PG, Dzindolet MT, Poletes G, Camacho LM. The illusion of group productivity. Personal Soc Psychol Bull. 1993;19:78–89.
Paulus PG, Dzindolet MT. Social influence processes in group brainstorming. J Pers Soc Psychol. 1993;64:575–86.
McLeod S. Solomon Asch – conformity experiment. SimplyPsychology.org : simply psychology; 2018.
Greenfield R. Brainstorming doesn’t work; try this technique instead. FastCompnay; 2014. Available from: https://www.fastcompany.com/3033567/brainstorming-doesnt-work-try-this-technique-instead
Rietzschel EF, Nijstad BA, Stroebe W. Productivity is not enough: a comparison of interactive and nominal brainstorming groups on idea generation and selection. J Exp Soc Psychol. 2006;42(2):244–51.
Thompson L. Creative conspiracy: the new rules of breakthrough collaboration. Harvard Business Review Press; 2013.
Right Source. 2017. Available from: https://www.rightsourcemarketing.com/content-creation/everything-know-brainstorming-wrong/
Griffiths C, Costi M. The creative thinking handbook: your step-by-step guide to problem solving in business. New York: Kogan Page Ltd; 2019.
Ayshford E. Why your next brainstorm should begin with an embarrassing story. Kellogg School of Management at Northwestern University; 2019. Available from: https://insight.kellogg.northwestern.edu/article/boost-creativity-brainstorm-embarrassment?utm_source=piano&utm_medium=onsite&utm_campaign=364L
Buzan T, Griffiths C. Mind maps for business: revolutionize your business thinking and practice. Harlow: BBC Active; 2010.
Eberle B. Scamper on: Games for imagination development. New York: D.O.K Publisher Inc; 1971.
De Bono E. Six thinking hats. London: Penguin; 2017.
De Bono E. Six thinking hats. New York: Little, Brown & Company; 1985.
Ohno T. Ask ‘why’ five times about every matter. 2006. Available from: https://www.toyota-myanmar.com/about-toyota/toyota-traditions/quality/ask-why-five-times-about-every-matter
Duncan K. The ideas book: 50 ways to general ideas visually. London: LID; 2014.
Gentner D, Bowdle B, Wolff P, Boronat C. Metaphor is like analogy. The analogical mind: perspectives from cognitive science. 2001. p. 99–253.
Gentner D. The mechanisms of analogical learning. In: Vosniadou S, Ortony A, editors. Similarity and analogical reasoning. Cambridge: Cambridge University Press; 1989. p. 199–241.
Chapter Google Scholar
Gordon WJJ. Synetics: the development of creative capacity. New York: Harper & Row; 1961.
Det N. Synectics classroom demo by Dr. Chitra Sohani YouTube; 2019. https://www.youtube.com/watch?v=YwVelaJQCcs
Clapham MM. The development of innovative ideas through creativity training. In: Shavinina LV, editor. The international handbook on innovation. Oxford: Pergamon; 2003. p. 366–76.
Kostoff RN. Stimulating innovation. The international handbook on innovation. 2003. p. 388–400.
Dhanoa HK, Nanda S. Effect of synectics model on language creativity: a review. Int J Multidiscip Educ Res. 2019;8(1).
Prince G. The operational mechanism of synectics. J Creat Behav. 1968;2:1–13.
Nolan V. Whatever happened to Synectics? Creat Innov Manag. 2003;12(1):25.
Starko AJ. Creativity in the classroom: schools of curious delight. London: Routledge; 2010.
Sawyer RK. The science of human innovation: Explaining creativity. New York: Oxford University Press; 2012.
Download references
Author information
Authors and affiliations.
Auckland University of Technology, Auckland, New Zealand
Rouxelle de Villiers
You can also search for this author in PubMed Google Scholar
Corresponding author
Correspondence to Rouxelle de Villiers .
Editor information
Editors and affiliations, creativity laboratory, 1.1 activity i: self-directed brainwriting.
Write down a problem (or design challenge) as concisely and as accurate as possible in the first one of the framed boxes in Fig. 10.2 . ( If you cannot think of one, here is one for you to mentally chew on: Too few parents volunteer for parent-teacher events to help teachers raise funds for additional school resources .)
At the top of each Post-it note, next to the pin, write down a category or discipline you think can think of that may have something/nothing to do with the problem. Suggested: Furniture, Toys, Utensils, Tools, Scientific Instruments, Transportation, Appliances, Weapons, Health/Medicine, Fashion & Personal Accessories). (2) Brainstorm ideas – in your own mind! Do not stop until you have 5 or 6 ideas under each Post-It topic. (Try to list about 20 to 30 under each topic.) Challenge yourself even further: do not leave until you have 50 ideas or have spent 10 min by yourself contemplating the issue and generating ideas.
Set this list aside for at least an hour while you do other things (anything but actively thinking about this matter.)
Return to the Post-it notes and colour the stars next to the best ideas (you determine the criteria to be considered ‘best’.)
Write the starred idea in the next one of the framed boxes and repeat the process. Do this at least three times. Any luck?
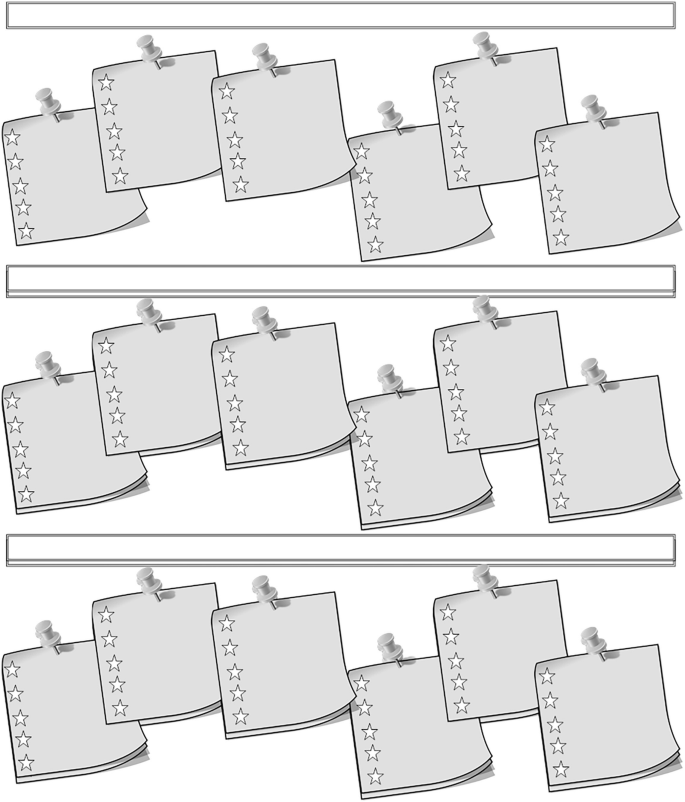
Self-directed Brainwriting here
1.2 Activity II: The Problem with Private Property in Public Transport
Passengers keep leaving their umbrellas on public buses, causing huge problems for the bus company in storing and returning lost property. How can the transport services deal with this costly problem without simply shrugging it off as ‘not our problem’ and creating customer dissatisfaction? Use the three tools (SCAMPER, WWJD and Random Picture Technique) to ideate. Next, apply the 5WHYs framework to consider a range of causes. Then, use those causes to consider alternative solutions the bus company might use to either prevent or overcome this costly customer service. (If you are initially unsure how the job functions listed in the figure relate to the problem, try to make remote associations between the problem and the issues that job/role might deal with. How would someone in that role, solve the lost umbrella problem?) (Figs. 10.3 and 10.4 ).
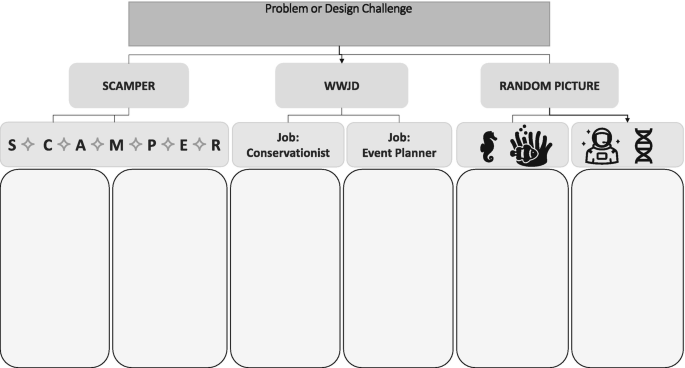
Practice with three thinking tools
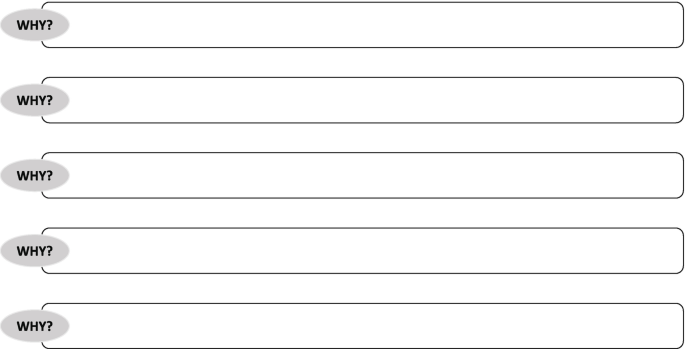
Five Wise Genii
Rights and permissions
Reprints and permissions
Copyright information
© 2022 The Author(s), under exclusive license to Springer Nature Singapore Pte Ltd.
About this chapter
Cite this chapter.
de Villiers, R. (2022). Creative Thinking, Problem Solving and Ideation Tools. In: de Villiers, R. (eds) The Handbook of Creativity & Innovation in Business. Springer, Singapore. https://doi.org/10.1007/978-981-19-2180-3_10
Download citation
DOI : https://doi.org/10.1007/978-981-19-2180-3_10
Published : 05 August 2022
Publisher Name : Springer, Singapore
Print ISBN : 978-981-19-2179-7
Online ISBN : 978-981-19-2180-3
eBook Packages : Business and Management Business and Management (R0)
Share this chapter
Anyone you share the following link with will be able to read this content:
Sorry, a shareable link is not currently available for this article.
Provided by the Springer Nature SharedIt content-sharing initiative
- Publish with us
Policies and ethics
- Find a journal
- Track your research
Filter by Keywords
People Management
4 types of decision-making styles and how to utilize them.
Senior Content Marketing Manager
December 18, 2023
Three billion decisions a year: That’s how many decisions managers and executives make as part of their day job.
Decision-making is a critical part of every manager’s job, yet most don’t do a great job of it. They rely on instinct and intuition to make decisions, with 98% of them not leveraging best practices.
Bad decisions can have grave consequences for the organization, such as bad financial performance (costing 3% of yearly profits !), loss of reputation, employee turnover, compliance issues, etc. Sound decisions can prevent them.
In this blog post, let’s see how you can use the four commonly used decision-making styles to make better business decisions.
What are Decision-Making Styles?
1. understand your team, 2. observe your team, 3. assess your team , 1. directive, 2. analytical, 3. conceptual, 4. behavioral, how to manage your team’s decision making styles.

Decision-making styles are approaches you, as a manager, take to solve a problem, improve a process, or resolve a dispute. Your approach depends on the issue at hand, time constraints, resource constraints, and many other variables.
While we speak of decisions, we don’t just mean the big ones like the marketing strategy for the year or the firing a non-performer. It could also be seemingly insignificant things like the subject line of an email or the right time for a 1-on-1 meeting.
Building thoughtful and repeatable decision-making styles can be a game-changer for someone making thousands of decisions daily. However, before you build your decision-making process , it’s essential to consider your team’s approaches, preferences, personalities, and reactions.
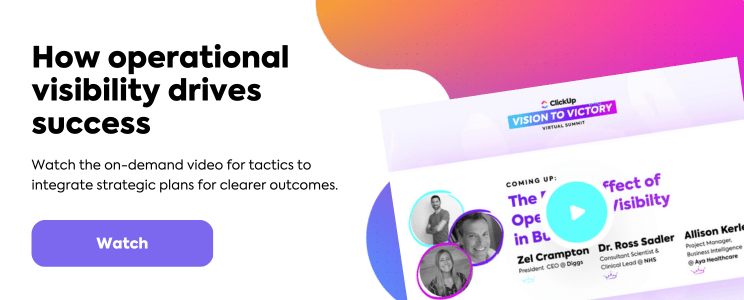
Understanding Your Team’s Decision-Making Styles
People don’t leave organizations. They leave managers.
Every decision you make has an impact on your teams and colleagues. So, before you develop your style, it’s essential to understand theirs.
Talk to your team members to understand them as individuals. Encourage them to speak about their priorities and preferences. For instance, if you’re making decisions about your hybrid work strategy, understand
- What time zones they’re living in
- What time they like working
- When they can take calls
- Do they have children who they drop/pickup from school.
No piece of information is too trivial. Understanding each individual’s motivations, passions, and overall personality helps managers know how to manage them.
Not everyone articulates the same way. Some team members may not speak up. So, observe their decision-making styles over a period of time. When you know how they make decisions, you can mimic their styles to influence them.
- Do they present concrete data at monthly reviews? This shows that they focus on objective data
- Do they come to you regularly for suggestions? They seek consensus and validation
- Do they change their personal processes every other month? They might be hasty or action-oriented, or experimental by nature
There are dozens of proven ways to examine and understand your team’s decision-making styles. The Myers-Briggs Type Indicator (MBTI) helps identify one’s natural preferences in four aspects of personality.
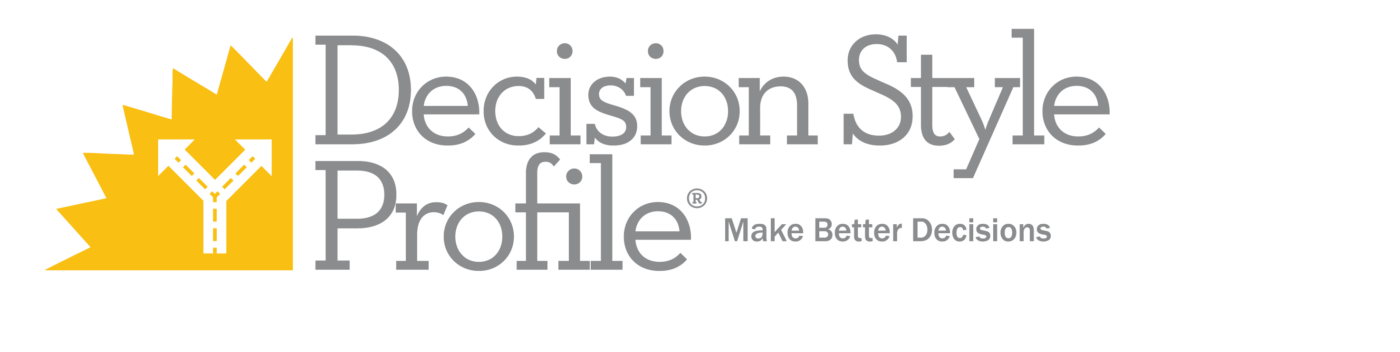
The Impact Associates Decision Style Profile evaluates people in five key factors: clarity, information, commitment, alignment, and time. The Truity Decision Style Test explores how you prioritize differing goals and how they influence your decisions.
Using any of these tools can give you a clearer idea of what moves your teams. However, it is important to note that there are some legal concerns around using personality tests in the workplace. Consider federal, state, and local laws before picking one.
Now that you’ve your groundwork sorted, let’s explore the four decision-making styles and how you can leverage them at work.
4 Different Decision-Making Styles
The directive decision-making style favors using already-known information and past trends to choose future paths. It is rational, data-driven, and logical. Directive decision makers choose to:
- Make decisions themselves
- Use past data and procedures instead of trying new ways
- Prioritize speed of action
- Be straight-forward in their thought process
- Avoid ambiguity
Directive decision-making is perfect for quick, recurring, or short-term decisions. It is also perfect for situations where the goal is clear. Before you make decisions, try this goal setting template to begin right.
Once you’ve set the goal, collecting and analyzing data will become easier. For example, if you’re a project manager estimating time for each task, directive decision-making saves a lot of time while being nearly accurate.
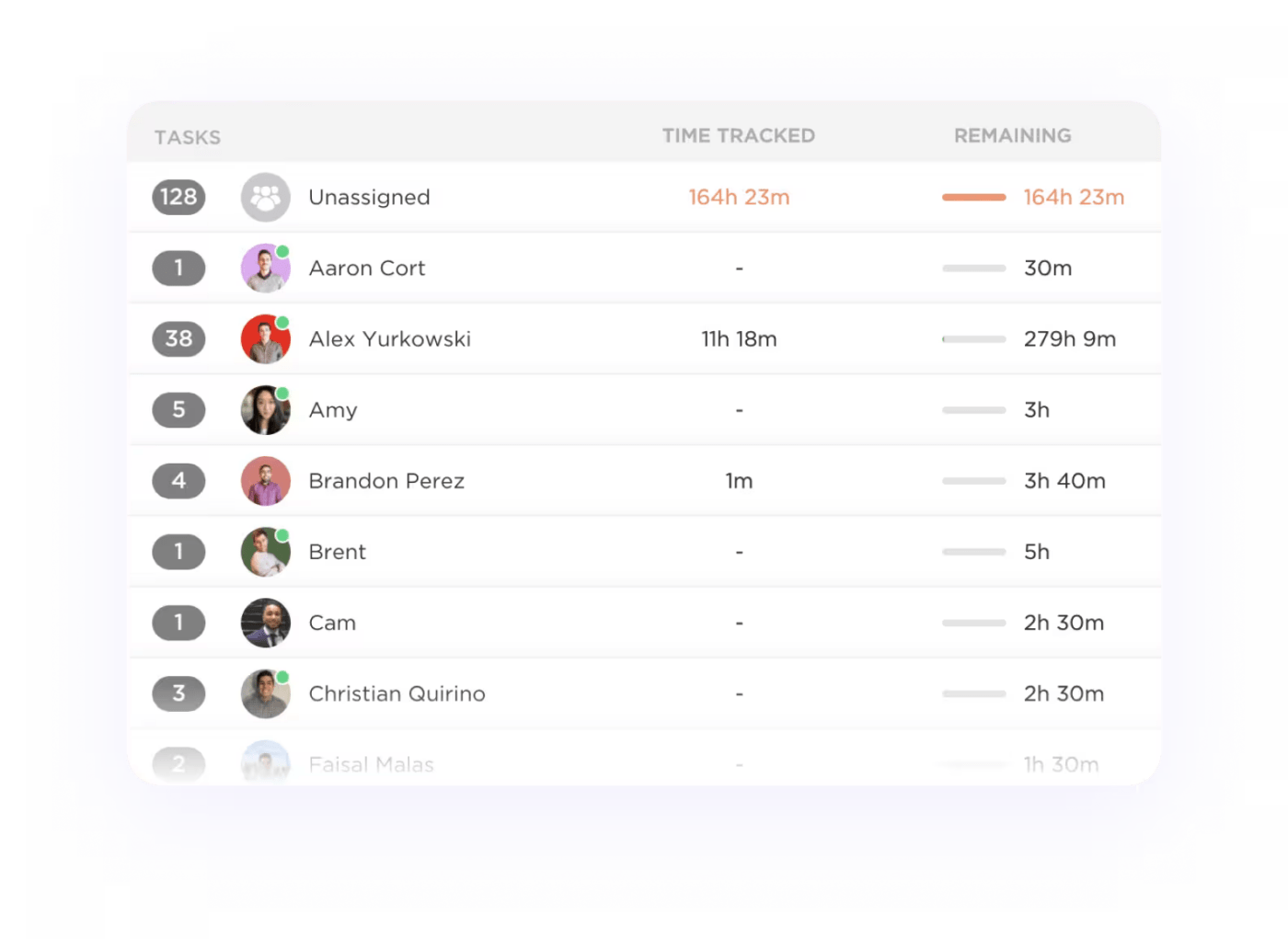
This can sometimes be seen as uncollaborative or authoritative. Therefore, it’s unsuitable for big decisions or changes that impact people’s lives.
Say you’re an HR manager responsible for the remote work strategy. If you make a decision that people need to come to the office from Monday till Friday from 9-6, it will be seen as inflexible and unwelcome, even if you base it on rational thought and data.
This type of decision-making is also unsuitable if you’re managing highly skilled and independent workers who want creative freedom. They wouldn’t appreciate being told what to do.
Analytic decision style is exploratory. Here, you consider all facts, opinions, possible scenarios, risks, costs, and consequences before making a decision. You also take as much time as you need.
Analytic decision making style is employed when there are multiple options, and there is no such thing as ‘one right answer.’
For example, if you are the procurement manager tasked with buying a project management tool for the engineering team, you will take an analytical decision-making approach. Analytic decision makers will:
- Explore all tools available
- Shortlist potential tools based on features and benefits
- Compare price and usability
- Study terms, conditions, and renewal policies of the contract
- Negotiate discounts and deals with vendors
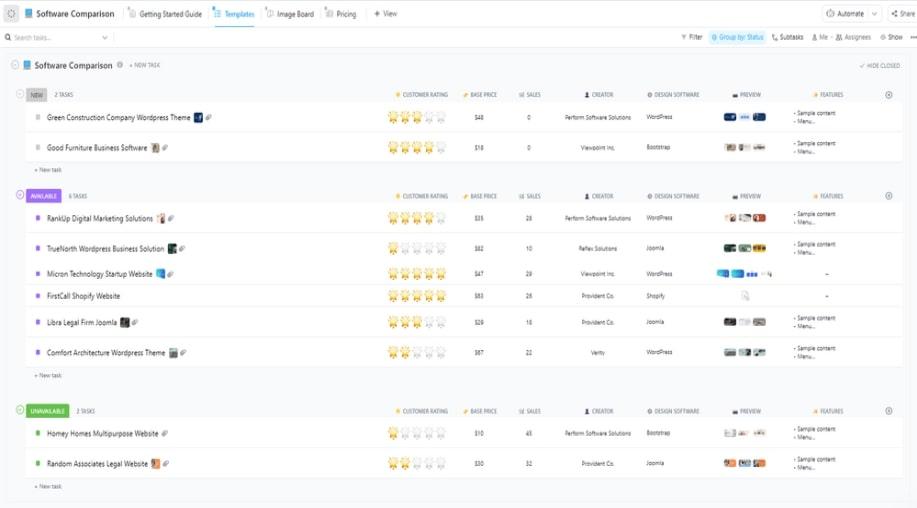
An analytical decision-making approach helps you weigh your options and choose the one most suitable for you, based on available information.
Analytical decision-making style also doesn’t allow much space for ambiguity. To be analytical, it is essential to have all the information clearly in hand. Sometime,s it might be your own knowledge. Therefore, it’s applicable for complex decisions, only if the options/possibilities are clearly defined.
It is not the right approach if the decision involves too many variables or unpredictability, like the feelings of team members. It is certainly not right for issues that need to be solved immediately.
Conceptual style decision making involves a creative approach to problem-solving. When there is a complex problem that involves imagining various potential scenarios and coming up with innovative solutions, this is the best style.
Conceptual decision-makers:
- Love taking risks
- Value creativity over pre-determined processes
- Ask open-ended questions and explore possibilities
- Think holistically, including tangential consequences
- Are flexible when faced with new possibilities
Let’s take an example of a product manager designing the roadmap of their software. This decision-making process is perfect. They will start with documenting their needs, then collaborate on a whiteboard, run polls for feedback, and run scenario planning, before making a decision.
A conceptual decision-maker is collaborative and will bring the whole team together to innovate the decision process. It motivates the team to be creative and consider long-term effects.
Conceptual decision style is great for complex, ambiguous situations; it is unsuitable for small or simple decisions. If you start pulling up a whiteboard and involve group decision making for ‘where to go for team lunch?’ you will waste a lot of time and energy!
If you take a people-centric approach to every decision, you have a behavioral decision-making style. In this style, you will:
- Take into account your team’s feelings and emotions
- Invite active participation from each team member
- Influence people’s decisions slowly and deliberately
- Prioritize the benefit of many over few
This is most commonly followed in human resources or people management teams. Project managers also use this style while making a final decision that can be disruptive or unpleasant.
When terminating a vendor’s contract, firing an employee, changing team structures, etc., this style works best.
However, managers with a conceptual decision-making style run the risk of caring too much for people’s feelings/reactions, which affects their decision itself. The extreme focus on consensus-building might also lead to inordinate delays and disgruntled team members. Having the right communication goals is also important, while taking this approach.
As you see, there is no right or wrong decision-making style. Depending on the situation and consequences, one style might work better than the others. A good manager will learn and apply all styles as appropriate throughout their career.
Whatever style you choose to follow, you can leverage various tools and techniques to make the right decisions. Let’s see how.
A good project management tool will give you everything you need to make the right decisions.
If the situation demands a directive decision-making style, you need data. You can use ClickUp’s Dashboard to create custom reports for any information you need.
You can create custom reports for resource utilization, sprint progress, time estimates, sales trends, goals , and more. You can also convert your dashboard into your calculator to make projections.
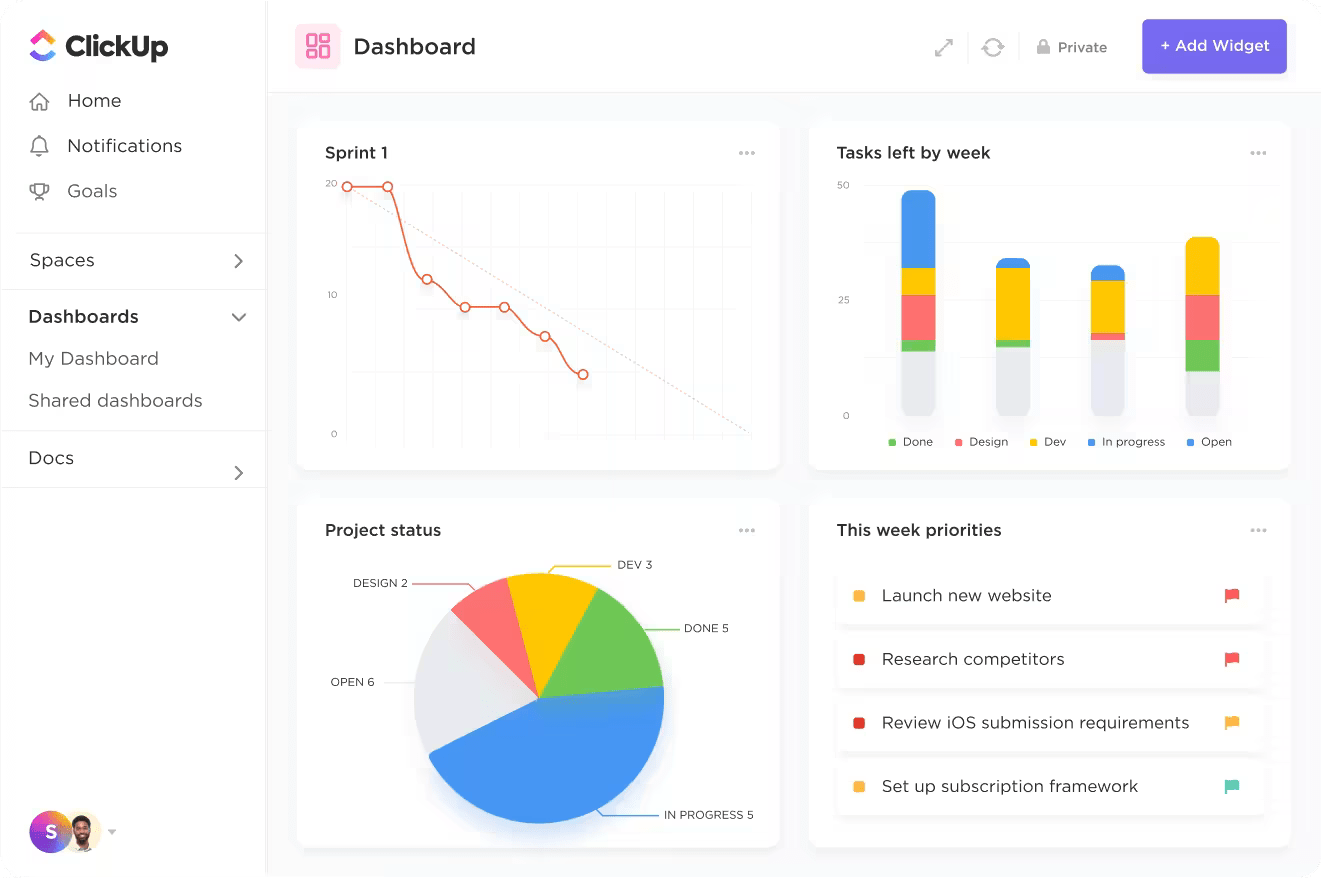
If you’re going to use an analytical style of decision-making, you need a way to document and evaluate multiple options. The simplest form of this is comparing the best and worst features — here are several pros and cons templates to make this process easy for you.
For more complex decisions, ClickUp’s Views are designed to evaluate options in whichever format suits you.
The list or table view gives shows you all the options one below another. You can also compare statuses, price, etc. with this view.
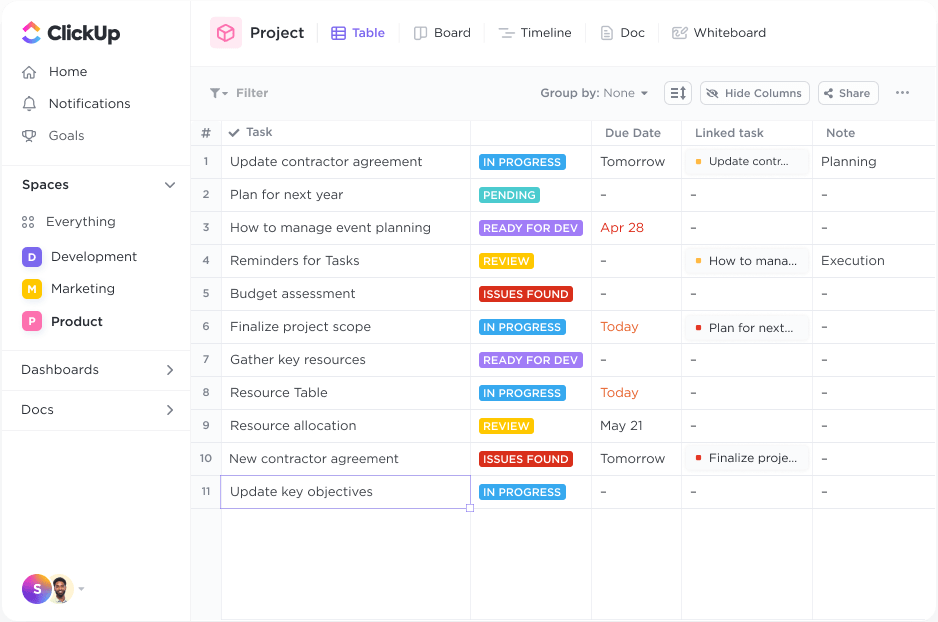
The board view can be used to track options by status or any custom field. For instance, if you compare project management tools, you can set a custom field for app availability such as Android, iOS, Web, etc.
If the Web is an important platform for you, you can sort accordingly and eliminate those unavailable. The calendar view is perfect for making decisions about time. The Gantt chart can be used for scheduling. The Workload and Box views are most useful in resource allocation decisions, especially while managing multiple projects .
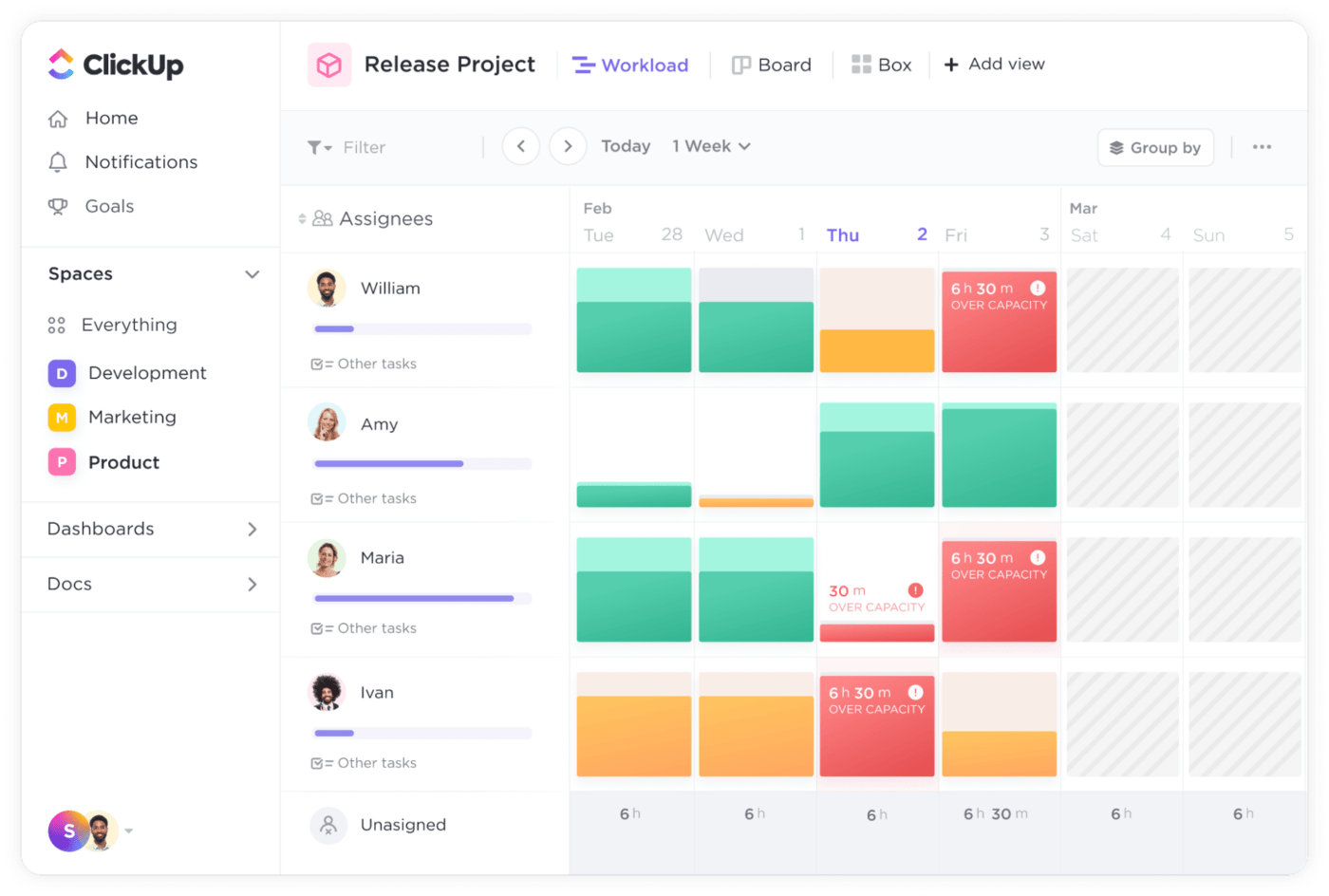
If the problem you’re solving demands a conceptual style, you need creative tools. The ClickUp Whiteboard is designed for precisely this purpose.
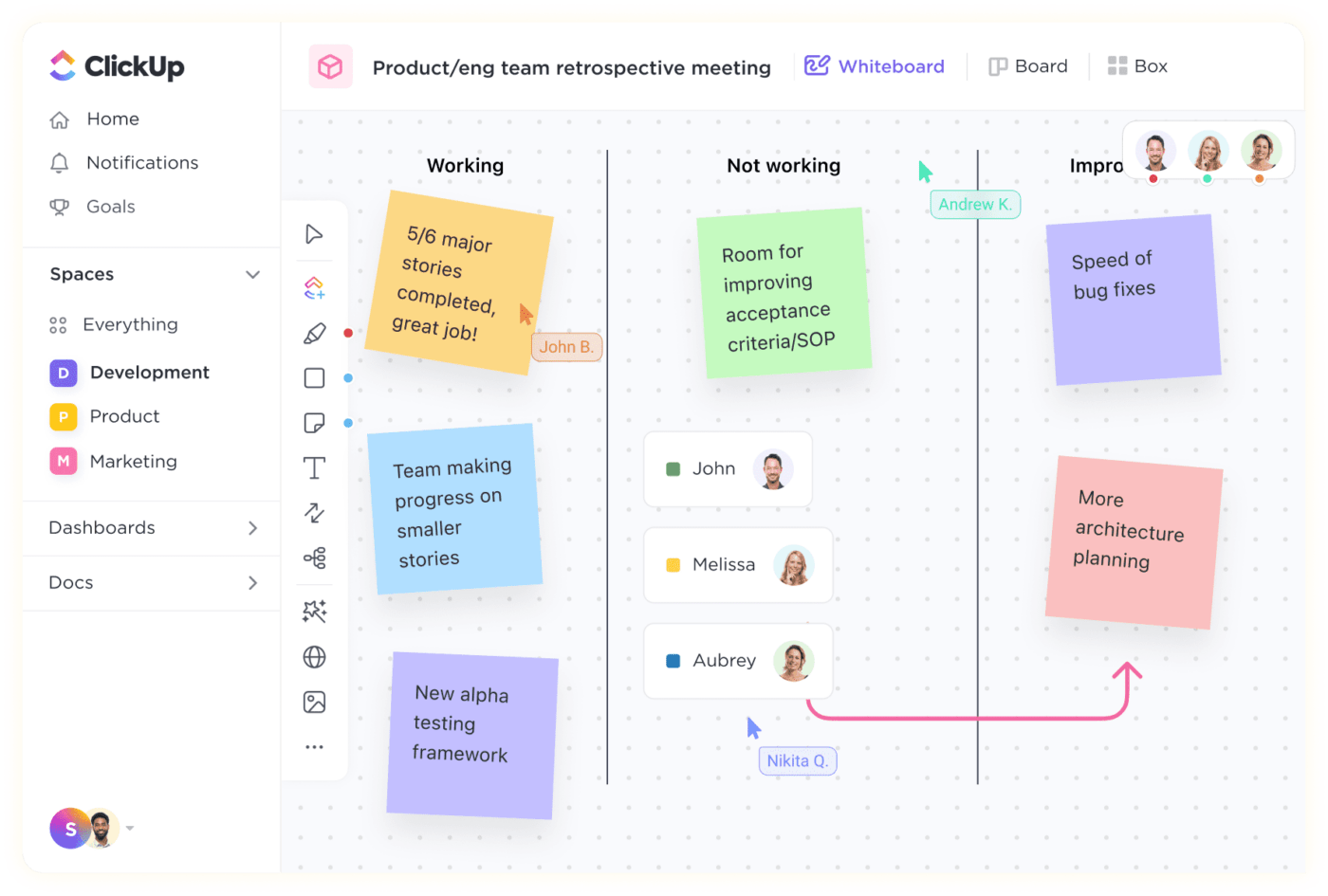
You can brainstorm on a blank slate or use one of ClickUp’s numerous decision-making templates . Track everyone’s activities, add notes, and collaborate in real-time to bring the team together in one place.
We recommend ClickUp’s decision-making framework template to get started.
Behavioral decision-making can be complex. But ClickUp can help there too. When you’re getting acquainted with your team, use forms on ClickUp to collect information and preferences. If you have to manage client expectations, these needs assessment templates will help you map their priorities.
Make Better Decisions Everytime with ClickUp
Whether you make three billion decisions or not, decision-making is the fundamental responsibility of every manager. Your ability to make better decisions will set you up as a reliable and effective manager.
Directive decision makers need data. As an analytical one, you need visibility. As a conceptual one, you foster creativity. And as a behavioral decision-maker, you need insights into your team. ClickUp is your singular place to get all this and more.
ClickUp helps you capture, organize and analyze data in the way that works for you. Track with Kanban boards, compare with table views, measure with reports/dashboard, collaborate with whiteboards, take action with tasks, and so much more! Try ClickUp for free now !
Questions? Comments? Visit our Help Center for support.
Receive the latest WriteClick Newsletter updates.
Thanks for subscribing to our blog!
Please enter a valid email
- Free training & 24-hour support
- Serious about security & privacy
- 99.99% uptime the last 12 months
.css-s5s6ko{margin-right:42px;color:#F5F4F3;}@media (max-width: 1120px){.css-s5s6ko{margin-right:12px;}} Join us: Learn how to build a trusted AI strategy to support your company's intelligent transformation, featuring Forrester .css-1ixh9fn{display:inline-block;}@media (max-width: 480px){.css-1ixh9fn{display:block;margin-top:12px;}} .css-1uaoevr-heading-6{font-size:14px;line-height:24px;font-weight:500;-webkit-text-decoration:underline;text-decoration:underline;color:#F5F4F3;}.css-1uaoevr-heading-6:hover{color:#F5F4F3;} .css-ora5nu-heading-6{display:-webkit-box;display:-webkit-flex;display:-ms-flexbox;display:flex;-webkit-align-items:center;-webkit-box-align:center;-ms-flex-align:center;align-items:center;-webkit-box-pack:start;-ms-flex-pack:start;-webkit-justify-content:flex-start;justify-content:flex-start;color:#0D0E10;-webkit-transition:all 0.3s;transition:all 0.3s;position:relative;font-size:16px;line-height:28px;padding:0;font-size:14px;line-height:24px;font-weight:500;-webkit-text-decoration:underline;text-decoration:underline;color:#F5F4F3;}.css-ora5nu-heading-6:hover{border-bottom:0;color:#CD4848;}.css-ora5nu-heading-6:hover path{fill:#CD4848;}.css-ora5nu-heading-6:hover div{border-color:#CD4848;}.css-ora5nu-heading-6:hover div:before{border-left-color:#CD4848;}.css-ora5nu-heading-6:active{border-bottom:0;background-color:#EBE8E8;color:#0D0E10;}.css-ora5nu-heading-6:active path{fill:#0D0E10;}.css-ora5nu-heading-6:active div{border-color:#0D0E10;}.css-ora5nu-heading-6:active div:before{border-left-color:#0D0E10;}.css-ora5nu-heading-6:hover{color:#F5F4F3;} Register now .css-1k6cidy{width:11px;height:11px;margin-left:8px;}.css-1k6cidy path{fill:currentColor;}
- Project planning |
- How to solve problems using the design ...
How to solve problems using the design thinking process
The design thinking process is a problem-solving design methodology that helps you develop solutions in a human-focused way. Initially designed at Stanford’s d.school, the five stage design thinking method can help solve ambiguous questions, or more open-ended problems. Learn how these five steps can help your team create innovative solutions to complex problems.
As humans, we’re approached with problems every single day. But how often do we come up with solutions to everyday problems that put the needs of individual humans first?
This is how the design thinking process started.
What is the design thinking process?
The design thinking process is a problem-solving design methodology that helps you tackle complex problems by framing the issue in a human-centric way. The design thinking process works especially well for problems that are not clearly defined or have a more ambiguous goal.
One of the first individuals to write about design thinking was John E. Arnold, a mechanical engineering professor at Stanford. Arnold wrote about four major areas of design thinking in his book, “Creative Engineering” in 1959. His work was later taught at Stanford’s Hasso-Plattner Institute of Design (also known as d.school), a design institute that pioneered the design thinking process.
This eventually led Nobel Prize laureate Herbert Simon to outline one of the first iterations of the design thinking process in his 1969 book, “The Sciences of the Artificial.” While there are many different variations of design thinking, “The Sciences of the Artificial” is often credited as the basis.
Anatomy of Work Special Report: How to spot—and overcome—the most crucial enterprise challenges
Learn how enterprises can improve processes and productivity, no matter how complex your organization is. With fewer redundancies, leaders and their teams can hit goals faster.
A non-linear design thinking approach
Design thinking is not a linear process. It’s important to understand that each stage of the process can (and should) inform the other steps. For example, when you’re going through user testing, you may learn about a new problem that didn’t come up during any of the previous stages. You may learn more about your target personas during the final testing phase, or discover that your initial problem statement can actually help solve even more problems, so you need to redefine the statement to include those as well.
Why use the design thinking process
The design thinking process is not the most intuitive way to solve a problem, but the results that come from it are worth the effort. Here are a few other reasons why implementing the design thinking process for your team is worth it.
Focus on problem solving
As human beings, we often don’t go out of our way to find problems. Since there’s always an abundance of problems to solve, we’re used to solving problems as they occur. The design thinking process forces you to look at problems from many different points of view.
The design thinking process requires focusing on human needs and behaviors, and how to create a solution to match those needs. This focus on problem solving can help your design team come up with creative solutions for complex problems.
Encourages collaboration and teamwork
The design thinking process cannot happen in a silo. It requires many different viewpoints from designers, future customers, and other stakeholders . Brainstorming sessions and collaboration are the backbone of the design thinking process.
Foster innovation
The design thinking process focuses on finding creative solutions that cater to human needs. This means your team is looking to find creative solutions for hyper specific and complex problems. If they’re solving unique problems, then the solutions they’re creating must be equally unique.
The iterative process of the design thinking process means that the innovation doesn’t have to end—your team can continue to update the usability of your product to ensure that your target audience’s problems are effectively solved.
The 5 stages of design thinking
Currently, one of the more popular models of design thinking is the model proposed by the Hasso-Plattner Institute of Design (or d.school) at Stanford. The main reason for its popularity is because of the success this process had in successful companies like Google, Apple, Toyota, and Nike. Here are the five steps designated by the d.school model that have helped many companies succeed.
1. Empathize stage
The first stage of the design thinking process is to look at the problem you’re trying to solve in an empathetic manner. To get an accurate representation of how the problem affects people, actively look for people who encountered this problem previously. Asking them how they would have liked to have the issue resolved is a good place to start, especially because of the human-centric nature of the design thinking process.
Empathy is an incredibly important aspect of the design thinking process. The design thinking process requires the designers to put aside any assumptions and unconscious biases they may have about the situation and put themselves in someone else’s shoes.
For example, if your team is looking to fix the employee onboarding process at your company, you may interview recent new hires to see how their onboarding experience went. Another option is to have a more tenured team member go through the onboarding process so they can experience exactly what a new hire experiences.
2. Define stage
Sometimes a designer will encounter a situation when there’s a general issue, but not a specific problem that needs to be solved. One way to help designers clearly define and outline a problem is to create human-centric problem statements.
A problem statement helps frame a problem in a way that provides relevant context in an easy to comprehend way. The main goal of a problem statement is to guide designers working on possible solutions for this problem. A problem statement frames the problem in a way that easily highlights the gap between the current state of things and the end goal.
Tip: Problem statements are best framed as a need for a specific individual. The more specific you are with your problem statement, the better designers can create a human-centric solution to the problem.
Examples of good problem statements:
We need to decrease the number of clicks a potential customer takes to go through the sign-up process.
We need to decrease the new subscriber unsubscribe rate by 10%.
We need to increase the Android app adoption rate by 20%.
3. Ideate stage
This is the stage where designers create potential solutions to solve the problem outlined in the problem statement. Use brainstorming techniques with your team to identify the human-centric solution to the problem defined in step two.
Here are a few brainstorming strategies you can use with your team to come up with a solution:
Standard brainstorm session: Your team gathers together and verbally discusses different ideas out loud.
Brainwrite: Everyone writes their ideas down on a piece of paper or a sticky note and each team member puts their ideas up on the whiteboard.
Worst possible idea: The inverse of your end goal. Your team produces the most goofy idea so nobody will look silly. This takes out the rigidity of other brainstorming techniques. This technique also helps you identify areas that you can improve upon in your actual solution by looking at the worst parts of an absurd solution.
It’s important that you don’t discount any ideas during the ideation phase of brainstorming. You want to have as many potential solutions as possible, as new ideas can help trigger even better ideas. Sometimes the most creative solution to a problem is the combination of many different ideas put together.
4. Prototype stage
During the prototype phase, you and your team design a few different variations of inexpensive or scaled down versions of the potential solution to the problem. Having different versions of the prototype gives your team opportunities to test out the solution and make any refinements.
Prototypes are often tested by other designers, team members outside of the initial design department, and trusted customers or members of the target audience. Having multiple versions of the product gives your team the opportunity to tweak and refine the design before testing with real users. During this process, it’s important to document the testers using the end product. This will give you valuable information as to what parts of the solution are good, and which require more changes.
After testing different prototypes out with teasers, your team should have different solutions for how your product can be improved. The testing and prototyping phase is an iterative process—so much so that it’s possible that some design projects never end.
After designers take the time to test, reiterate, and redesign new products, they may find new problems, different solutions, and gain an overall better understanding of the end-user. The design thinking framework is flexible and non-linear, so it’s totally normal for the process itself to influence the end design.
Tips for incorporating the design thinking process into your team
If you want your team to start using the design thinking process, but you’re unsure of how to start, here are a few tips to help you out.
Start small: Similar to how you would test a prototype on a small group of people, you want to test out the design thinking process with a smaller team to see how your team functions. Give this test team some small projects to work on so you can see how this team reacts. If it works out, you can slowly start rolling this process out to other teams.
Incorporate cross-functional team members : The design thinking process works best when your team members collaborate and brainstorm together. Identify who your designer’s key stakeholders are and ensure they’re included in the small test team.
Organize work in a collaborative project management software : Keep important design project documents such as user research, wireframes, and brainstorms in a collaborative tool like Asana . This way, team members will have one central source of truth for anything relating to the project they’re working on.
Foster collaborative design thinking with Asana
The design thinking process works best when your team works collaboratively. You don’t want something as simple as miscommunication to hinder your projects. Instead, compile all of the information your team needs about a design project in one place with Asana.
Related resources
Unmanaged business goals don’t work. Here’s what does.
How Asana uses work management to drive product development
How Asana uses work management to streamline project intake processes
How Asana uses work management for smoother creative production
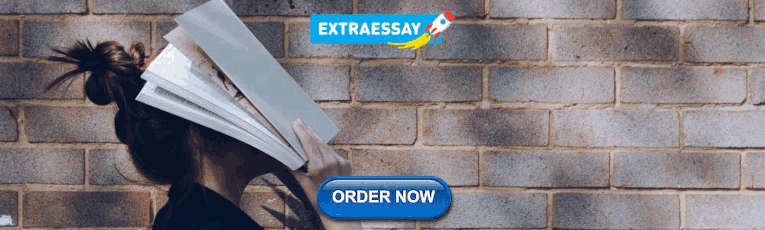
IMAGES
VIDEO
COMMENTS
The links between the mathematical and cognitive models that interact during problem solving are explored with the purpose of developing a reference framework for designing problem-posing tasks. When the process of solving is a successful one, a solver successively changes his/her cognitive stances related to the problem via transformations that allow different levels of description of the ...
When solving the problem, students were explicitly advised to search for underlying connections based on the conceptual questions. Both small‐scale interviews and a large‐scale written test were conducted to investigate the effects of guided conceptual scaffolding on student problem solving.
That evolution also involves an emerging understanding of the construct of problem-solving style and the influence that construct holds on creative productivity. VIEW: An assessment of problem solving style is a new instrument for assessing problem-solving style. It is the result of more than five years of research and development.
Humans are innate creative problem-solvers. Since early humans developed the first stone tools to crack open fruit and nuts more than 2 million years ago, the application of creative thinking to solve problems has been a distinct competitive advantage for our species (Puccio 2017).Originally used to solve problems related to survival, the tendency toward the use of creative problem-solving to ...
Advances in our understanding of the Creative Problem Solving framework, problem-solving style, and their interactions hold great promise for future practice and research, and especially for those concerned with understanding and enhancing creative human behavior and organizational innovation (e.g., Isaksen, 2004, Isaksen and Dorval, 1993 ...
six problem-solving styles aligned over three dimensions have been identified that describe a person's ... psychology of style theory hypothesizes that "matches" of styles and situations will be most effective (Zhang, 2006a, 2006b, 2008). The purpose of the present study was to test the relationship of style to achievement. First, if the
Problem-solving styles are consistent individual differences in the ways people prefer to deal with new ideas, manage change, and respond effectively to complex, open-ended opportunities and challenges. Knowledge of style is important in education in a number of ways. It contributes to adults' ability to work together effectively in teams and ...
When, how, and why students use conceptual knowledge during math problem solving is not well understood. We propose that when solving routine problems, students are more likely to recruit conceptual knowledge if their procedural knowledge is weak than if it is strong, and that in this context, metacognitive processes, specifically feelings of doubt, mediate interactions between procedural and ...
When we do problem definition well in classic problem solving, we are demonstrating the kind of empathy, at the very beginning of our problem, that design thinking asks us to approach. When we ideate—and that's very similar to the disaggregation, prioritization, and work-planning steps—we do precisely the same thing, and often we use ...
Walker et al. 89 also noted that novice problem-based learning students tended to engage in far more backward-driven reasoning, which results in more errors during problem solving and may persist ...
Susie Steel prefers to use the conceptual style of decision-making in her job. She is more concerned with long-term results, brainstorming of alternatives, creative approaches to problem-solving ...
This study describes the development and evaluation of an instructional approach called Conceptual Problem Solving (CPS) which guides students to identify principles, justify their use, and plan their solution in writing before solving a problem. The CPS approach was implemented by high school physics teachers at three schools for major ...
On completion of this chapter, the readers will be able to: Identify the appropriate problem-solving tool to use for a specific situation or to match his or her preferred thinking style. Apply a range of thinking tools to assist in the ideation and idea refinement stages of creative thinking tasks. Deliver creative ideas for further development ...
The relationships between cognitive style, conceptual tempo and problem solving strategies of 81 fifth-grade students were examined. While cognitive style was not found to be related to strategies employed during problem solving, conceptual tempo influenced both time spent and quantity and quality of questions asked. Effectiveness of programmed
to problem solving and posing. Second, we observed habits and attitudes of the teachers involved in our study (the first sample) when they designed problem-solving tasks for students. At the beginning of a four. day training program, teachers received a 2-h instruction in PP based on a variety of.
3. Conceptual. Conceptual style decision making involves a creative approach to problem-solving. When there is a complex problem that involves imagining various potential scenarios and coming up with innovative solutions, this is the best style. Conceptual decision-makers: Love taking risks; Value creativity over pre-determined processes
Skills in this area include restructuring skills, abstractive thinking, innovation, open-mindedness, and strategic planning. Problem-solving skills such as decision-making, troubleshooting, solution execution, and logical thinking are essential for professionals employing their conceptual skills. These skills take the idea through to execution.
Abstract and Figures. The links between the mathematical and cognitive models that interact during problem solving are explored with the purpose of developing a reference framework for designing ...
The design thinking process is a problem-solving design methodology that helps you develop solutions in a human-focused way. Initially designed at Stanford's d.school, the five stage design thinking method can help solve ambiguous questions, or more open-ended problems. Learn how these five steps can help your team create innovative solutions ...
This eventually leads to many misconceptions and errors in problem-solving. This is also aligned with the results found in Jazuli et al. (2017) where the authors concluded that most students find ...
Best Teaching Practices Conceptual Understanding of Problem Solving. Research Findings. Research at the secondary and even post-secondary level on understanding of basic concepts that are involved in solving biology, chemistry, and physics problems (many of which require the application of algebraic or other mathematical concepts) indicates that students do not understand the concepts.
there are emotionally charged problems. you know there is just one correct answer. 5.The Conceptual problem-solving style is most effective when... select best answer? a more social approach is needed. you are in a hurry and need to move quickly through the problem-solving and decision-making processes. there is no clarity about the possible ...
A) The problem factual information should be differentiated from opinion or speculation. B) The problem should be stated explicitly. C) The problem definition should be different than a disguised solution. D) The problem definition should be written down. B.