Have a language expert improve your writing
Run a free plagiarism check in 10 minutes, generate accurate citations for free.
- Knowledge Base
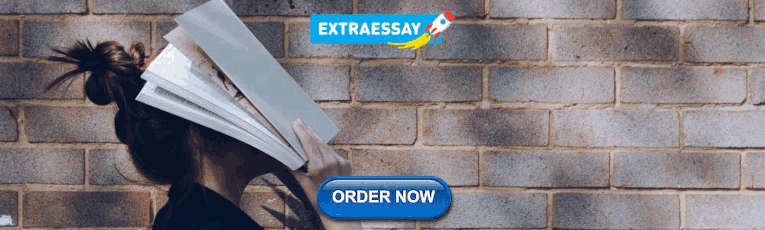
Hypothesis Testing | A Step-by-Step Guide with Easy Examples
Published on November 8, 2019 by Rebecca Bevans . Revised on June 22, 2023.
Hypothesis testing is a formal procedure for investigating our ideas about the world using statistics . It is most often used by scientists to test specific predictions, called hypotheses, that arise from theories.
There are 5 main steps in hypothesis testing:
- State your research hypothesis as a null hypothesis and alternate hypothesis (H o ) and (H a or H 1 ).
- Collect data in a way designed to test the hypothesis.
- Perform an appropriate statistical test .
- Decide whether to reject or fail to reject your null hypothesis.
- Present the findings in your results and discussion section.
Though the specific details might vary, the procedure you will use when testing a hypothesis will always follow some version of these steps.
Table of contents
Step 1: state your null and alternate hypothesis, step 2: collect data, step 3: perform a statistical test, step 4: decide whether to reject or fail to reject your null hypothesis, step 5: present your findings, other interesting articles, frequently asked questions about hypothesis testing.
After developing your initial research hypothesis (the prediction that you want to investigate), it is important to restate it as a null (H o ) and alternate (H a ) hypothesis so that you can test it mathematically.
The alternate hypothesis is usually your initial hypothesis that predicts a relationship between variables. The null hypothesis is a prediction of no relationship between the variables you are interested in.
- H 0 : Men are, on average, not taller than women. H a : Men are, on average, taller than women.
Here's why students love Scribbr's proofreading services
Discover proofreading & editing
For a statistical test to be valid , it is important to perform sampling and collect data in a way that is designed to test your hypothesis. If your data are not representative, then you cannot make statistical inferences about the population you are interested in.
There are a variety of statistical tests available, but they are all based on the comparison of within-group variance (how spread out the data is within a category) versus between-group variance (how different the categories are from one another).
If the between-group variance is large enough that there is little or no overlap between groups, then your statistical test will reflect that by showing a low p -value . This means it is unlikely that the differences between these groups came about by chance.
Alternatively, if there is high within-group variance and low between-group variance, then your statistical test will reflect that with a high p -value. This means it is likely that any difference you measure between groups is due to chance.
Your choice of statistical test will be based on the type of variables and the level of measurement of your collected data .
- an estimate of the difference in average height between the two groups.
- a p -value showing how likely you are to see this difference if the null hypothesis of no difference is true.
Based on the outcome of your statistical test, you will have to decide whether to reject or fail to reject your null hypothesis.
In most cases you will use the p -value generated by your statistical test to guide your decision. And in most cases, your predetermined level of significance for rejecting the null hypothesis will be 0.05 – that is, when there is a less than 5% chance that you would see these results if the null hypothesis were true.
In some cases, researchers choose a more conservative level of significance, such as 0.01 (1%). This minimizes the risk of incorrectly rejecting the null hypothesis ( Type I error ).
The results of hypothesis testing will be presented in the results and discussion sections of your research paper , dissertation or thesis .
In the results section you should give a brief summary of the data and a summary of the results of your statistical test (for example, the estimated difference between group means and associated p -value). In the discussion , you can discuss whether your initial hypothesis was supported by your results or not.
In the formal language of hypothesis testing, we talk about rejecting or failing to reject the null hypothesis. You will probably be asked to do this in your statistics assignments.
However, when presenting research results in academic papers we rarely talk this way. Instead, we go back to our alternate hypothesis (in this case, the hypothesis that men are on average taller than women) and state whether the result of our test did or did not support the alternate hypothesis.
If your null hypothesis was rejected, this result is interpreted as “supported the alternate hypothesis.”
These are superficial differences; you can see that they mean the same thing.
You might notice that we don’t say that we reject or fail to reject the alternate hypothesis . This is because hypothesis testing is not designed to prove or disprove anything. It is only designed to test whether a pattern we measure could have arisen spuriously, or by chance.
If we reject the null hypothesis based on our research (i.e., we find that it is unlikely that the pattern arose by chance), then we can say our test lends support to our hypothesis . But if the pattern does not pass our decision rule, meaning that it could have arisen by chance, then we say the test is inconsistent with our hypothesis .
If you want to know more about statistics , methodology , or research bias , make sure to check out some of our other articles with explanations and examples.
- Normal distribution
- Descriptive statistics
- Measures of central tendency
- Correlation coefficient
Methodology
- Cluster sampling
- Stratified sampling
- Types of interviews
- Cohort study
- Thematic analysis
Research bias
- Implicit bias
- Cognitive bias
- Survivorship bias
- Availability heuristic
- Nonresponse bias
- Regression to the mean
Hypothesis testing is a formal procedure for investigating our ideas about the world using statistics. It is used by scientists to test specific predictions, called hypotheses , by calculating how likely it is that a pattern or relationship between variables could have arisen by chance.
A hypothesis states your predictions about what your research will find. It is a tentative answer to your research question that has not yet been tested. For some research projects, you might have to write several hypotheses that address different aspects of your research question.
A hypothesis is not just a guess — it should be based on existing theories and knowledge. It also has to be testable, which means you can support or refute it through scientific research methods (such as experiments, observations and statistical analysis of data).
Null and alternative hypotheses are used in statistical hypothesis testing . The null hypothesis of a test always predicts no effect or no relationship between variables, while the alternative hypothesis states your research prediction of an effect or relationship.
Cite this Scribbr article
If you want to cite this source, you can copy and paste the citation or click the “Cite this Scribbr article” button to automatically add the citation to our free Citation Generator.
Bevans, R. (2023, June 22). Hypothesis Testing | A Step-by-Step Guide with Easy Examples. Scribbr. Retrieved March 25, 2024, from https://www.scribbr.com/statistics/hypothesis-testing/
Is this article helpful?
Rebecca Bevans
Other students also liked, choosing the right statistical test | types & examples, understanding p values | definition and examples, what is your plagiarism score.
- International
- Schools directory
- Resources Jobs Schools directory News Search
Hypothesis Testing
Subject: Mathematics
Age range: 16+
Resource type: Other

Last updated
29 March 2018
- Share through email
- Share through twitter
- Share through linkedin
- Share through facebook
- Share through pinterest
Creative Commons "Sharealike"
Your rating is required to reflect your happiness.
It's good to leave some feedback.
Something went wrong, please try again later.
Paris_the_Penguin
Excellent resource - really clearly written. Thank you!
Empty reply does not make any sense for the end user
Excellent and well written PPT to introduce the Hypothesis testing. Thank you ever so much for sharing
Detailed notes. Thank you
Excellent set of resources and really easy to use. Many thanks!
Report this resource to let us know if it violates our terms and conditions. Our customer service team will review your report and will be in touch.
Not quite what you were looking for? Search by keyword to find the right resource:
You are using an outdated browser. Please upgrade your browser to improve your experience.
You may love

Hypothesis Testing PowerPoint Template
We will customize this slide for you to fit your exact needs
- Hypothesis-Testing-PowerPoint-Template - 4x3 – $4.99
- Hypothesis-Testing-PowerPoint-Template - 16x9 – $4.99
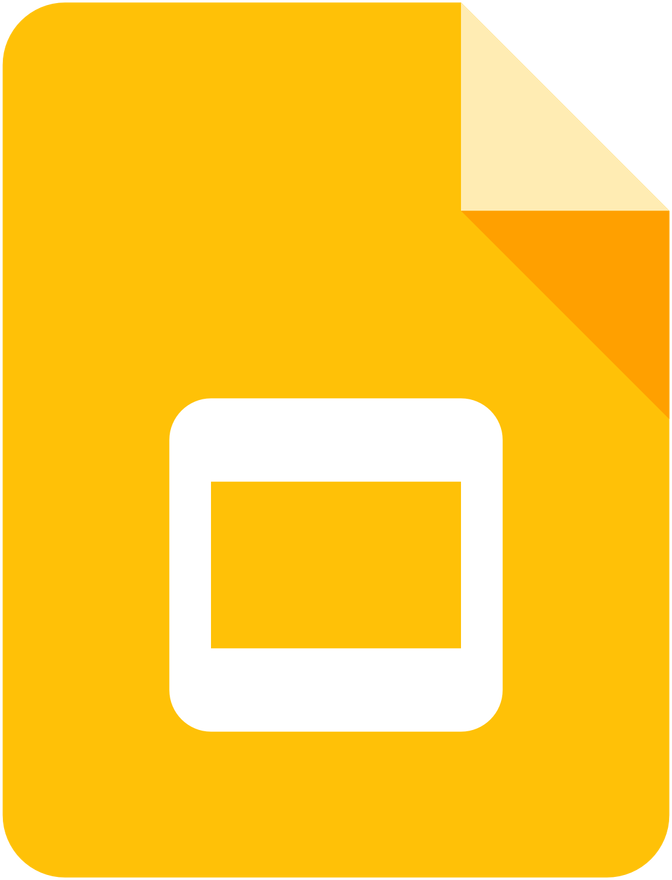
Login to use this feature
Add-to-favs lets you build a list for inspiration and future use.
Log in now to start adding your favs.
If you don't have one. A free account also gives you access to our free templates library
You May Also Like
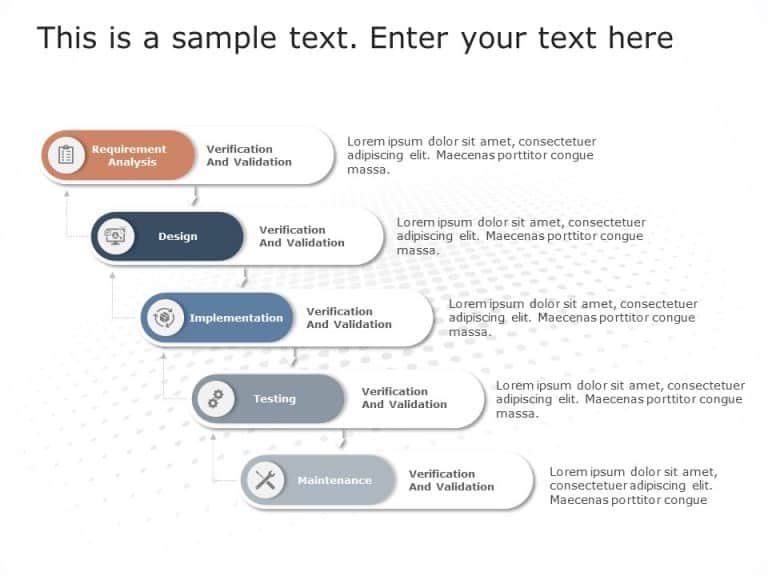
Software Testing Waterfall Model PowerPoint Template
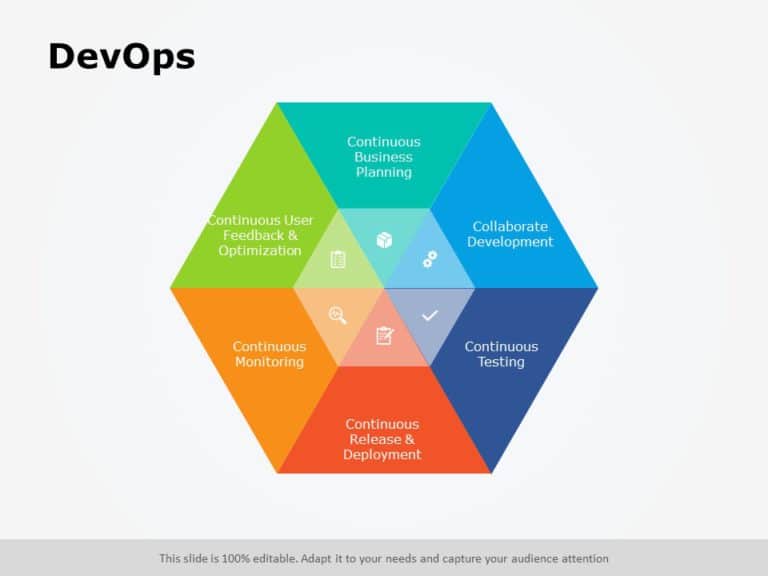
DevOps Cycle PowerPoint Template
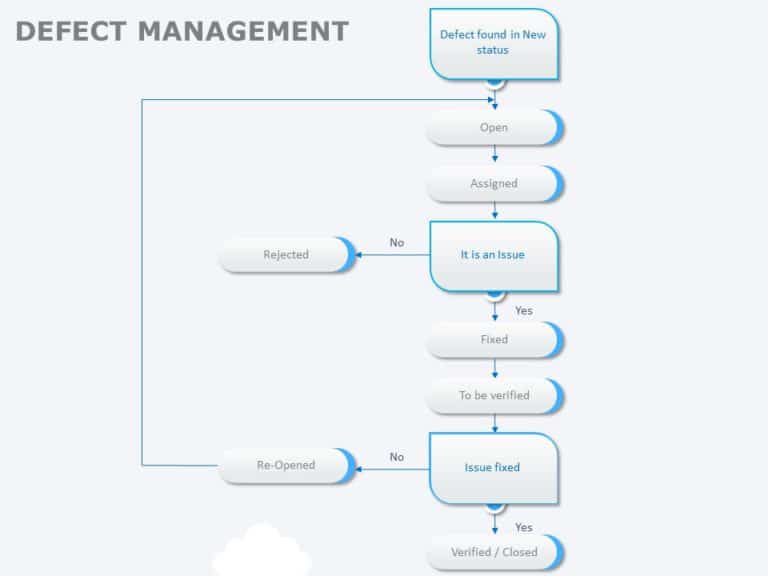
Bug Defect Management PowerPoint Template
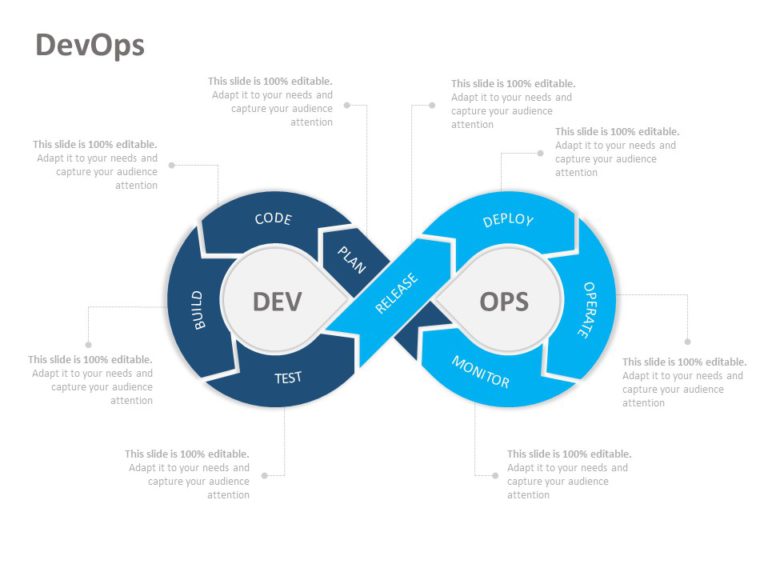
Devops 01 PowerPoint Template
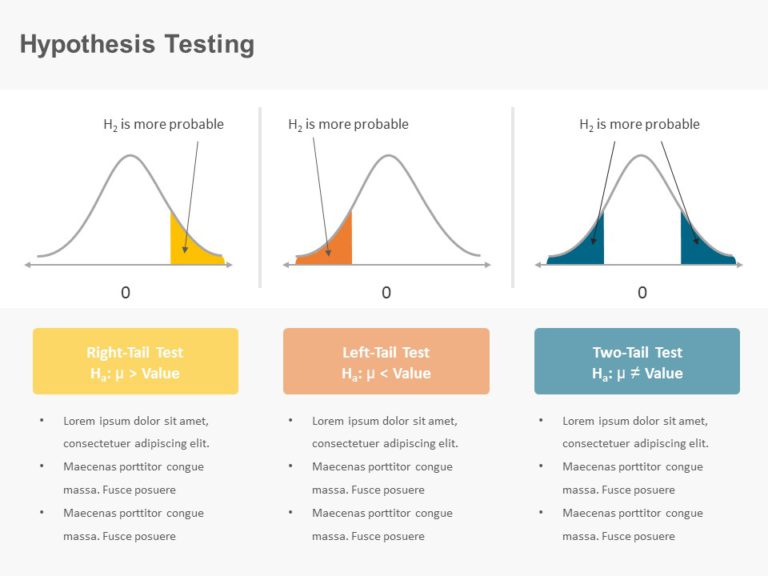
Hypothesis Generation PowerPoint Template
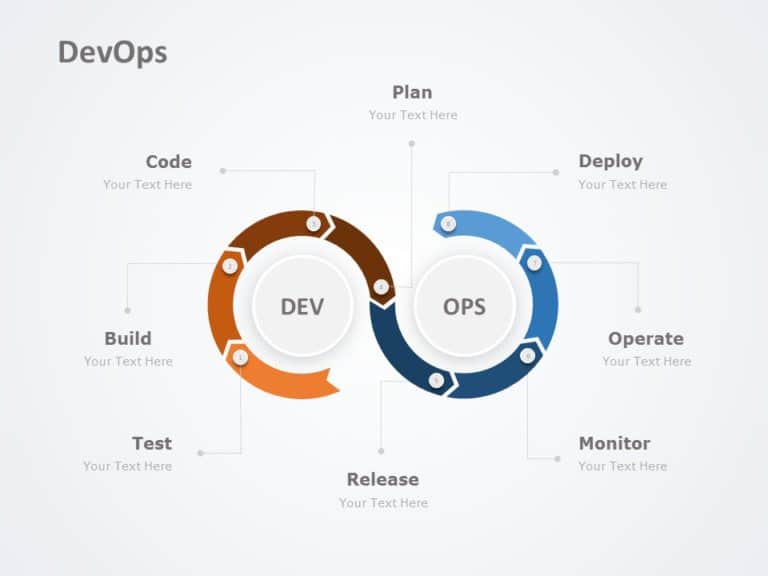
Devops 08 PowerPoint Template
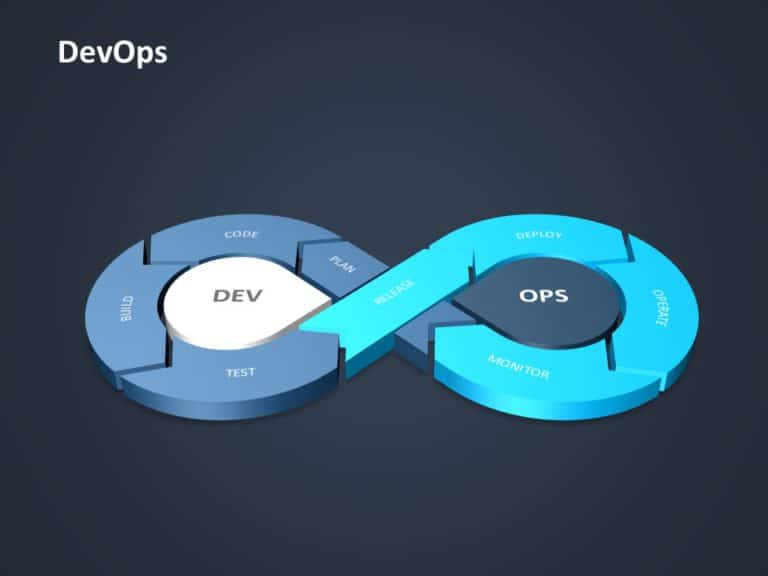
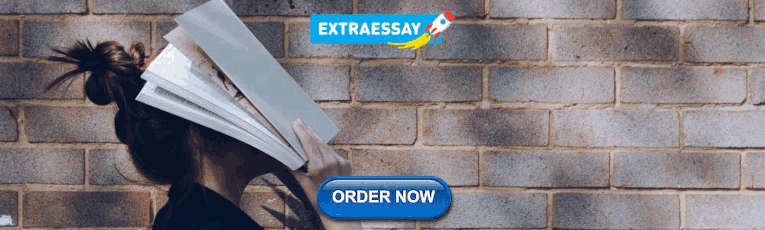
Devops 07 PowerPoint Template
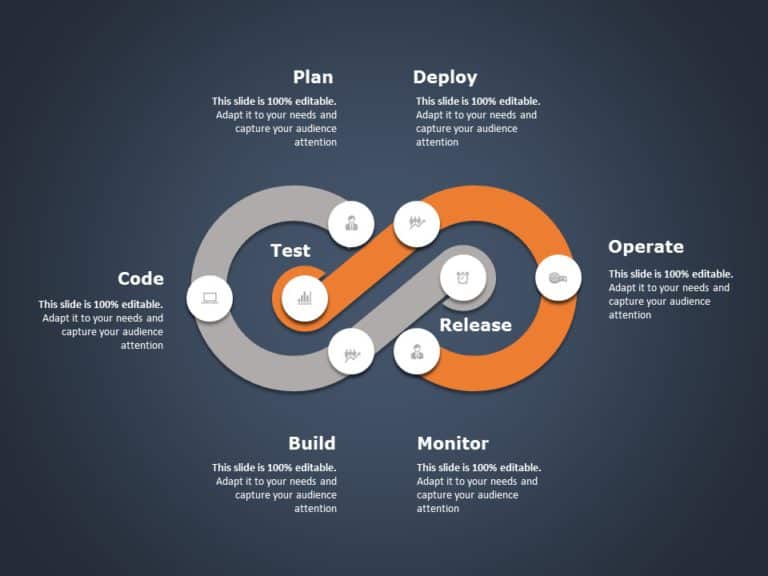
Devops 09 PowerPoint Template
Recommended for you.
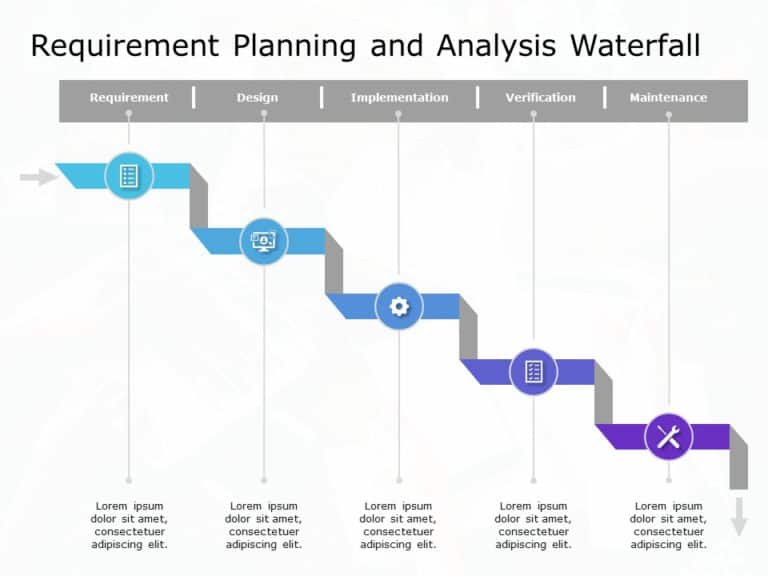
Waterfall Model PowerPoint Template
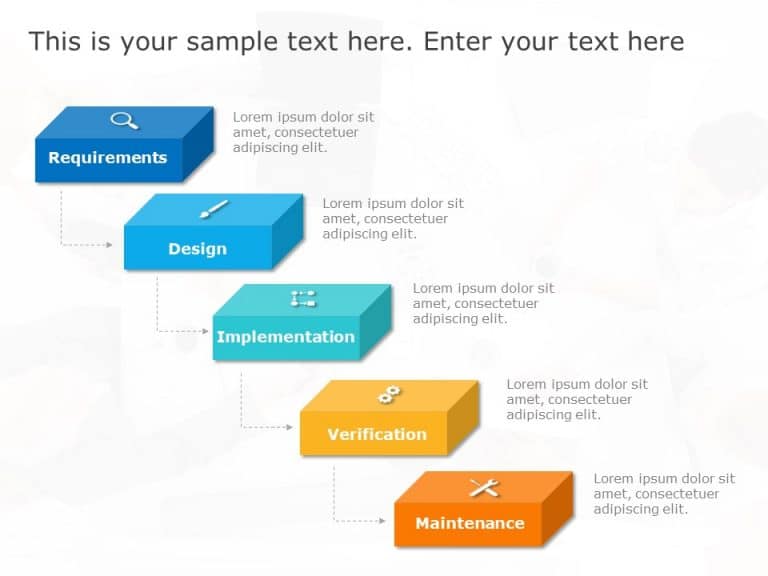
Devops 02 PowerPoint Template
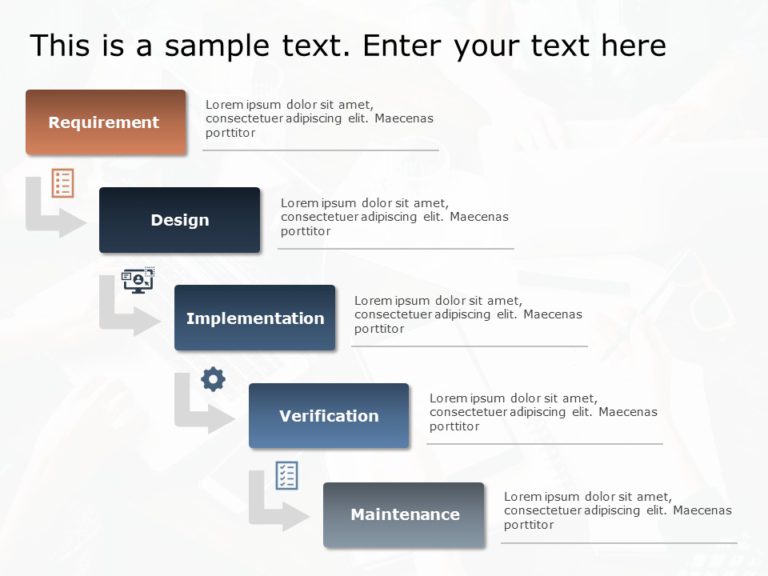
Waterfall Development PowerPoint Template
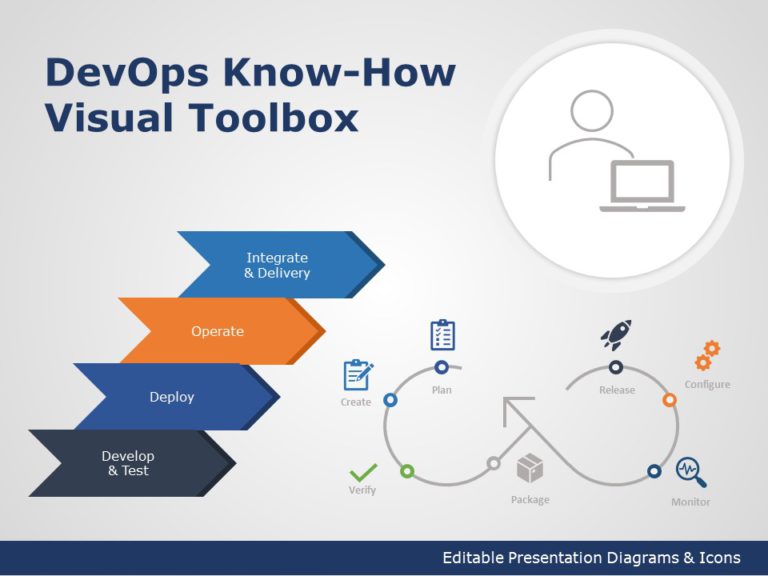
Devops 03 PowerPoint Template
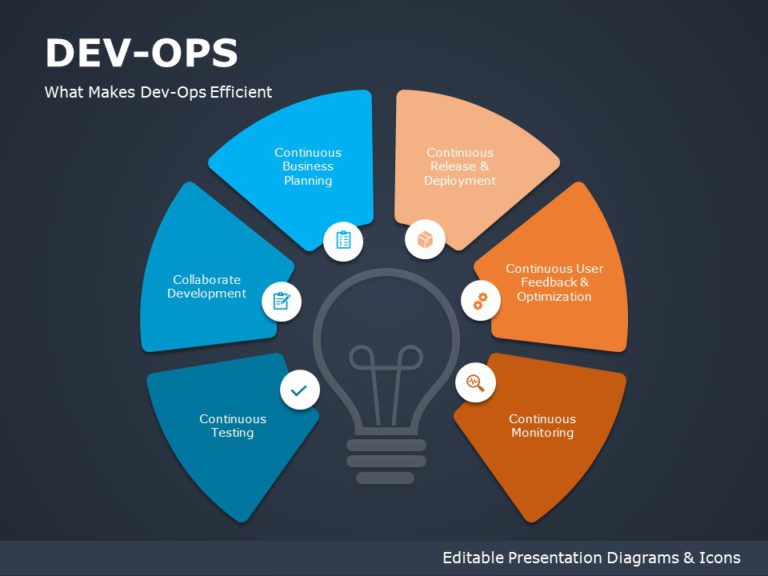
Devops 11 PowerPoint Template
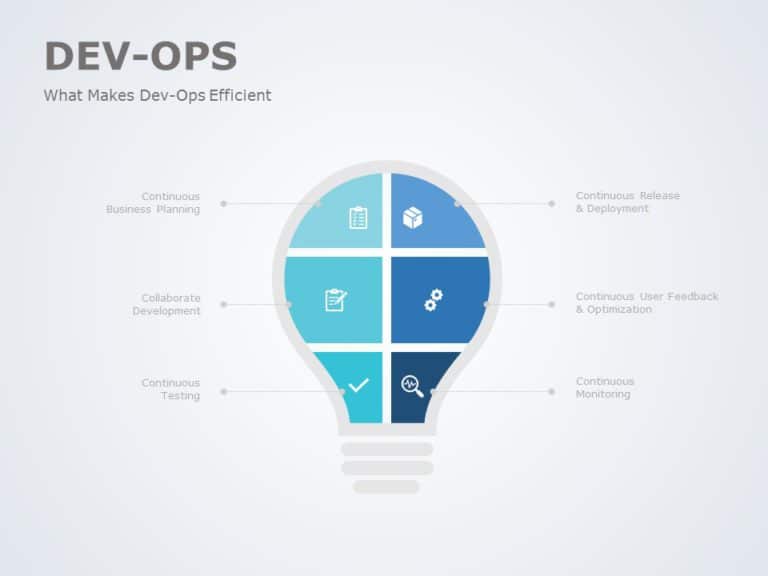
Devops 04 PowerPoint Template
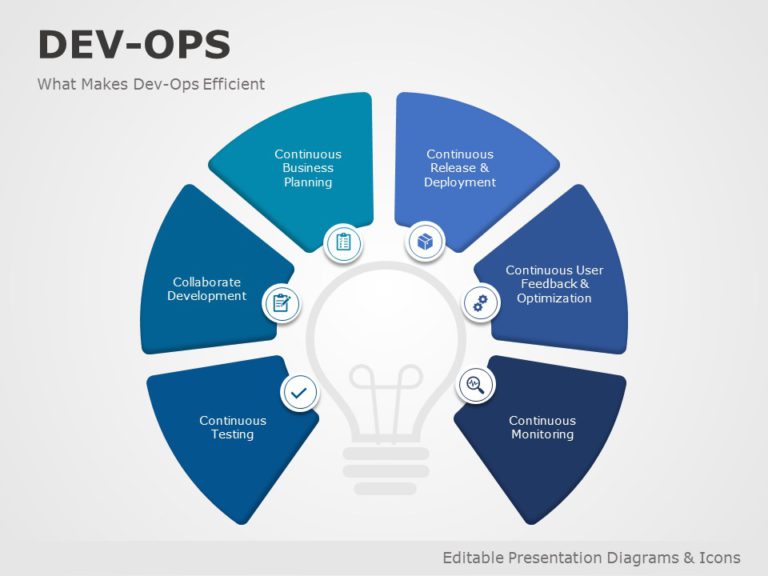
Devops 10 PowerPoint Template
Hypothesis testing presentation template.
Use this Hypothesis Testing PowerPoint template to create visually appealing presentations in any professional setting. Its minimalistic design and ready-to-use features enhance your presentation slides ten folds.
The Hypothesis Testing PPT template is professionally designed with the principles of vision sciences to capture your audience’s attention. Convey your message clearly with our unique set of editable infographics, icons, images, fonts, and presentation backgrounds. Download now and stand out in your next presentation with Hypothesis Testing PowerPoint and Google Slides template.
Ask us to modify or edit any specific element of the Hypothesis Testing template as per your need with our custom slides services. Lets collaborate to blend your ideas with our Hypothesis Testing template and get the final product delivered within 24 hours.
We can also help you and your team create full-fledged presentations from scratch with our presentation services . Explore now!
Features of this PowerPoint Template And Google Slides Theme:
- 100% editable with easy-to-use features.
- Contains 4:3 and 16:9 aspect ratio suitable for all types of screens.
- Includes icons, images, graphics, and infographics to capture audience’s attention.
- Compatible with both Google Slides and Microsoft PowerPoint.
Forgot Password?
Join the SlideUpLift Discount Club- A Lifetime Value
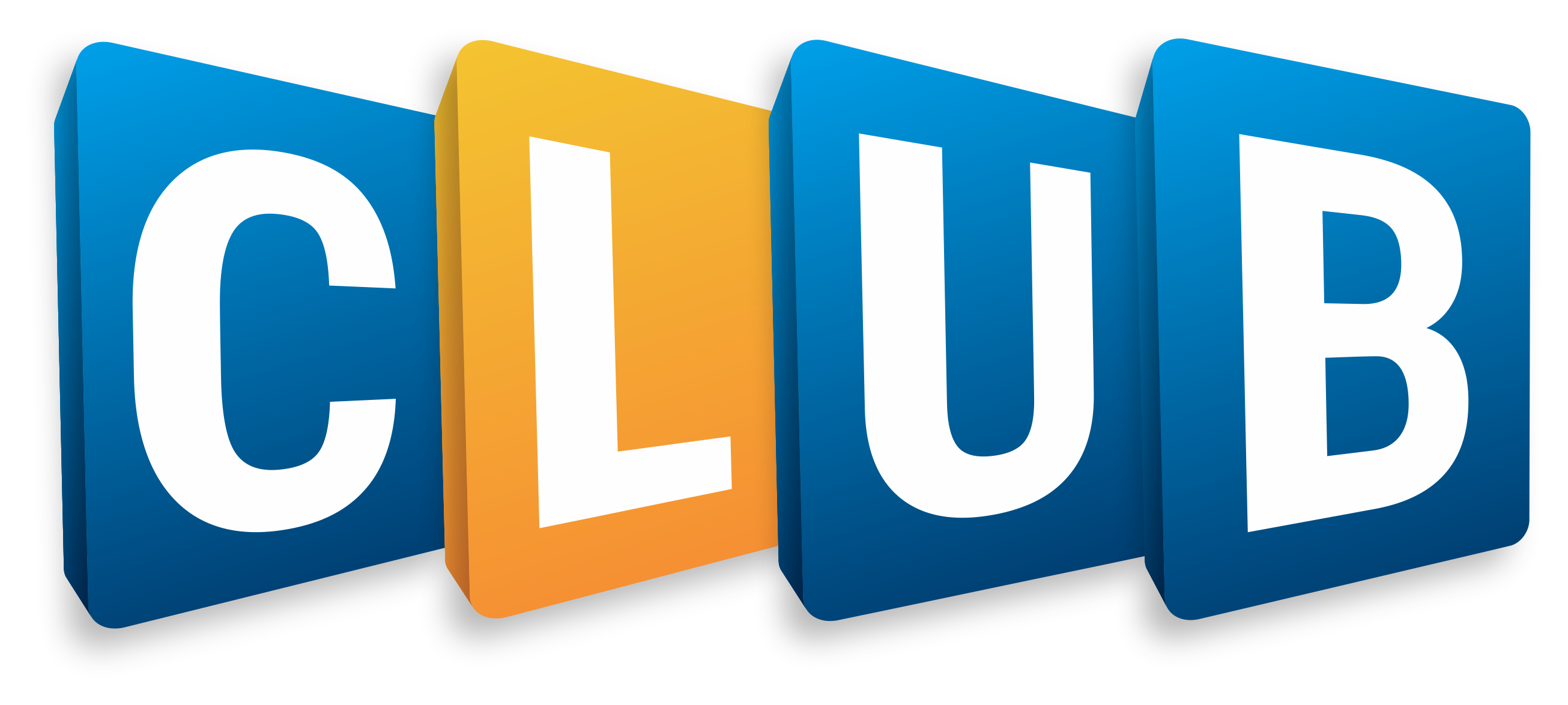
Benefits never expire and apply to the whole SlideUplift library including future additions.
Upon paying a one time fee, you will remain a Discount Clubber for a lifetime and enjoy 20% discounts on all products that you purchase à la carte from SlideUpLift.com
Privacy Overview
Necessary cookies are absolutely essential for the website to function properly. This category only includes cookies that ensures basic functionalities and security features of the website. These cookies do not store any personal information
Any cookies that may not be particularly necessary for the website to function and is used specifically to collect user personal data via ads, other embedded contents are termed as non-necessary cookies. It is mandatory to procure user consent prior to running these cookies on your website.
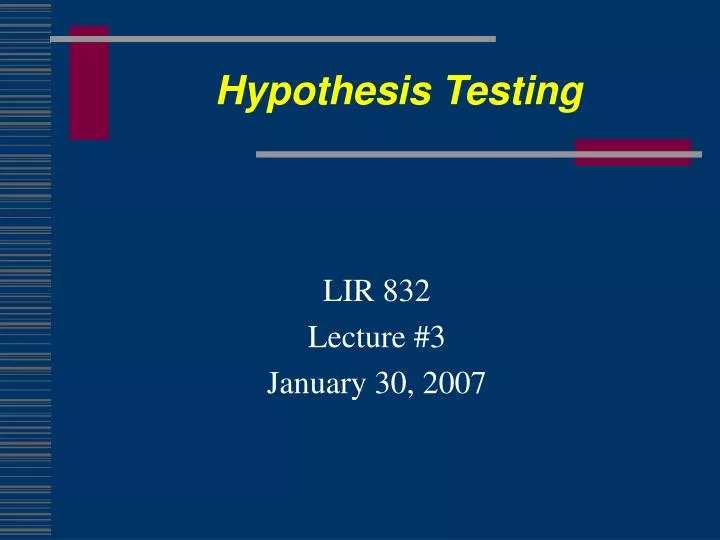
Hypothesis Testing
Apr 07, 2019
1.25k likes | 2.51k Views
Hypothesis Testing. LIR 832 Lecture #3 January 30, 2007. Topics of the Day. A. Our Fundamental Problem Again: Learning About Populations from Samples B. Basic Hypothesis Testing: One Tailed Tests Using a Z Statistic C. Probability and Critical Cutoff Approaches: Really the Same Thing
Share Presentation
- low cut point
- state beliefs
- a graphical approach
- systematic difference
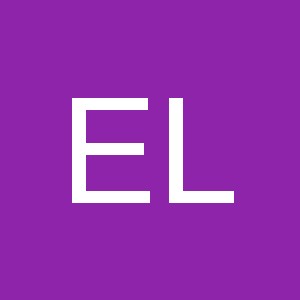
Presentation Transcript
Hypothesis Testing LIR 832 Lecture #3 January 30, 2007
Topics of the Day • A. Our Fundamental Problem Again: Learning About Populations from Samples • B. Basic Hypothesis Testing: One Tailed Tests Using a Z Statistic • C. Probability and Critical Cutoff Approaches: Really the Same Thing • D. How do we do hypothesis tests on small samples (n = 30 or less). • E. How do we do hypothesis testing when we have information on population standard deviation? On sample standard deviations? • F. How do we test a statement such as (two tailed test)? • G. How do we test for differences in means of two populations?
Hypothesis Testing • Fundamental Problem: We want to know about a population which is not observed and want to use a sample to learn about the population. Our problem is that sampling variability makes sample an inexact estimator of the population. We need to come up with a method to learn about the population from samples which allows for sampling variability.
Hypothesis Testing: Example • We are considering implementing a training program which purports to improve quality and reduce the number of defects. Currently, 10 out of every 1000 parts produced are not within spec. The standard deviation of defects is 8 parts (variance is 64). The typical employee produces 1,000 parts per day • The program costs $1,000 per employee and we have 10,000 production employees. We are unwilling to spend $10,000,000 for a pig in the poke. • Instead we decide to run a pilot on 100 employees to determine whether the program is effective for our employees. The firm that does the training will do the program for free, so our only cost is lost production for the time during which employees are trained. Note that the 100 employees are a pilot or, in our terminology, a sample.
Hypothesis Testing: Example • Employees are sent to the program and then given several days under instruction to apply what they have learned to their work. We run a one day test on the employees and find that they average 8 parts per thousand. • Defects are down, but is this really an improvement or is it simply the result of sampling variation? Could we be reasonably certain if we trained a second group of employees, or ran this same test next week, that defects would also be down?
Hypothesis Testing: Example • Abstractly, we are faced with the problem of distinguishing whether the program is effective or whether the improvement is reasonably explained by sampling variation (aka luck).
Hypothesis Testing: Example • Let’s approach this as a statistical problem. We know that historically there have been 10 defects per 1000 with standard deviation of 8. So our question is, “How likely is it that we have pulled a sample of 100 employees with a mean defect rate of 8 if, in fact, the training program did not work (in other words, that the population rate of defects remains 10 per 1000)?
Hypothesis Testing: Example • You can set this problem up as you have been already: • As the sample is larger than 30, we can use our z-table. The P(z<2.5) = 0.62%, very small. There is so small a likelihood that the sample we have observed was drawn from a population with a mean of 10 that we reject the possibility that the training program was ineffective.
Hypothesis Testing: Example • What do I mean when I say that the probability is 0.62%? Two possible interpretations: • 1. Suppose we set up a population with a mean of 10 and a standard deviation of 8 and draw samples of 100 from that population. Now imagine repeating this experiment 1000 times. We would expect that slightly over 6 of those samples would have a value of 8 or less. • 2. Alternatively, if the population had a mean of 10, standard deviation of 8, we would expect that 0.62% of the time we would draw a sample values 8 or less by chance. • I find the first approach makes it easier to understand what I mean when I say there is a 0.62% probability that an event occurred by chance, but you may prefer the second.
Hypothesis Testing: Example • Thinking about this graphically: • Two extreme possibilities: • 1. Training did little or nothing • 2. The training was highly effective (really useful in Thomas the Tank Engine terminology)
Hypothesis Testing: Example • If the training did nothing, we would expect the distribution of defects post training to look a lot like the distribution of defects prior to training.
Hypothesis Testing: Example So we can use the old distribution as a standard against which to judge our sample results. If the sample looks a lot like the old distribution it would be reasonable to believe that the training did not work. 1. For convenience, we add cut points at ± 2 standard deviations (1.6 = 2* .8)
Hypothesis Testing: Example • When we pull our sample, we calculate a mean and compare it to the old distribution. So, for example, our sample returns a value of 8. This lies to the left of the low cut point and we conclude it is very unlikely that the training did nothing.
Hypothesis Testing: Example • Let’s go behind the graphs to the underlying, if unobserved population.
Hypothesis Testing: Example
Hypothesis Testing: Formalizing the Steps • Step 1: State our beliefs about the world clearly (hypotheses). • Example: The consulting firms contention is that their training program reduces defect rates. Another possibility is that the training program is ineffective and that any changes in defect rates are simply the result of sampling variability (randomness)
Hypothesis Testing: Formalizing the Steps • Step 2: Formalize this into the alternative and null hypothesis: • Alternative: HA μpost training < 10: the the training program is effective • Null: HO μpost training ≥10: the training program has no effect on defect rates.
Hypothesis Testing: Formalizing the Steps • More about Step 2: • The two hypotheses are about the unobserved population. We will use samples to test these two hypotheses • Together, the null and alternative cover all possible outcomes. • The null is about change being the result of sampling variability, not the result of systematic difference. So our null for the training program is that it is not effective. Our alternative is that the program has a positive effect. • We always test to determine whether the null is reasonable (is sufficiently likely that we are unwilling to conclude it is false).
Hypothesis Testing: Formalizing the Steps • Step 3: Set up the probability problem: • The sign of the test is taken from the alternative hypothesis. We ask, how likely are we to observe this extreme an outcome if, indeed, the null is true.
Hypothesis Testing: Formalizing the Steps • Step 4: Solve the Probability Problem: • Note that our standard deviation is the root of the population variance divided by sample size. This is the second part of the Central Limit Theorem and provides the predictive power to use of sample means in hypothesis testing. • The result of doing the test is a probability of the null being true given the sample outcome. If that probability is sufficiently small, we conclude the null is not reasonable.
Hypothesis Testing: Formalizing the Steps • Step 5: Decide whether the probability of the null being true, given the sample outcome, is sufficiently low to allow you to reject (not believe) the null. • The 0.62% likelihood is the probability that a population with a mean of 10 and a standard deviation of 8 would produce a sample of 100 observations with a mean of 8. It is very unlikely and we may well decide the null isn’t realistic.
A Graphical Approach
Hypothesis Testing: Example • A second example: We are considering the absence behavior of a new group of workers and wish to determine if it is different from the behavior of our typical company employee. • The company has hired a large group of human resource managers over the last three years. They are well versed in corporate policy and, as they do many of the unpleasant tasks in the firm, tend to have high stress levels. The Vice President of Human Resources has remarked that she is concerned about their stress levels and believes that our new HR managers they are taking more days off than most employees to compensation for their problems. • We collect data on the 225 HR managers in the firm. We find they average 24 days of sick leave annually. The average managers in the firm has taken 22 days of sick leave annually over the last ten years. The standard deviation for the pool of typical managers is 30 days. Is our VP correct that our HR managers take more sick leave than other managers?
Hypothesis Testing: Example • Step 1: State our views of the world. • One view is that HR managers are taking more days of sick leave than has been typical of other managers. • Another is that they are, as a group, no different than other managers.
Hypothesis Testing: Example • Step 2: Formalize the hypotheses we are testing. • Let μd be the population mean days off for the population of HR managers: • H0: Null: HR managers are not different: • (NOTE: the null is about the population mean, not the sample) • μ ≤22 • HA: Alternative: HR managers take more sick time than other managers • (Similarly, the alternative is about the population) • μ > 22
Hypothesis Testing: Example • Step 3: Set up the probability problem. • Note that our sample is 225, so we have central limit theorem results and can use a z distribution.
Hypothesis Testing: Example • Step 4: Solve the probability problem.
Hypothesis Testing: Example • Step 5: Determine whether the likelihood is sufficiently small to reject the null. • Our calculations indicate that there is a 15.87 percent likelihood that a population with a mean of 22 would produce a sample of 225 individuals with a mean of 24. So, we would expect a sample of the type we got in about 1 in 6 experiments when HR managers were no different than other managers. That type of outcome just isn’t too unlikely.
Hypothesis Testing: Example • There are, again, two ways of interpreting this result: • 1. (Repeat experiment approach) If we ran 100 experiments in which we pulled random samples of 225 from a population in which the mean of 22 and a standard deviation of 30, between 15 and 16 of those samples would have a mean of 24 or greater. • 2. (Probability approach) Alternatively, 15.87% of the time when we pull a sample of 225 from a population with a mean of 22 and standard deviation of 30, the mean will be 24 or greater.
Hypothesis Testing • Now consider what we are doing abstractly… • A little background. Employers are often very interested in employees views of their work and firm. There are many ways of measuring employee attitudes, a common method used in survey research are Likert scaled questions. For example, we might ask a question such as: • How would you rate this job? Would you rate it as • 1 - very bad 2 - bad 3 - good 4 - very good
Hypothesis Testing • A Somewhat Cranky Aside: • The response to this question is numeric, we get a number between 1 and 4, but it is ordinal data. We know the ordering of the response, a response of very good is stronger than a response of good; similarly a response of bad is weaker than a response of good.; but there is no arithmetic relationship between the responses. Two responses of “very bad” are not equal to one response of “bad”. Indeed, we cannot even be sure that the responses are equally spaced. The person who responds “bad” may be mildly unhappy relative to someone who responds good; but the person who responds “very bad” could be somewhat more unhappy than the “bad” or they could just about ready to “go postal”. Still the data is ordered. • Despite this limitation, it is common practice in behavior sciences to add the responses to form a mean response.
Hypothesis Testing • Back to our problem: • 1. We begin with a “theory” or observation about the population. These are typically a general statements such as: • a. Employees on evening or night shifts are less satisfied with their work than other workers. • 2. Implicit in this statement is its opposite, the null hypothesis. This is what we are going to test • b. Null: Employees on evening or night shifts are no less satisfied with their work than employees on the day shift.
Hypothesis Testing • 3. We turn these statements into operational hypotheses, statements which can be tested statistically. • c. We have been surveying employees for many years using Likert scaled surveys. We know from these surveys that day shift employees rate the firm as good (3) on a four point Likert scale(very bad, bad, good, very good). Standard deviation is also 3. Our null and alternative would be: • H0: Null: μevening or night ≥3 • HA: Alternative: μevening or night <3 • Note: The null and alternative exhaust all possibilities, no other outcome is possible.
Hypothesis Testing • 4. We pull a sample and test to determine the probability that the sample came from the population with the characteristics of the null. • Suppose we pull a sample of 36 night employees with a sample mean of 2.3 • We ask: • What is the probability of pulling this particular sample if the mean response of our night shift employees really is 3.0 or greater? • P(x-bar < 2.3 if μnight really ≥3) or P(x-bar < 2.3 | μnight ≥3)? • Note: the sign in the statement is taken from the alternative hypothesis.
Hypothesis Testing • 5. We then calculate that probability:
Hypothesis Testing • 6. What does this 8.08% mean: • 1. Suppose we had a population with a mean value of 3 and standard deviation of 3 (this is exactly the population we build our null hypotheses around). Then suppose we ran an experiment in which we drew 36 individuals at random from that population and repeated the experiment 100 times. We would expect that 8.0 of those samples would have a mean of 2.3 or less! • 2. If our population has a mean and standard deviation of 3.0, we would expect that 8.08% of the time we would draw a sample of 36 individuals with a mean of 2.3 or less.
Hypothesis Testing • Criteria for rejecting or not rejecting the null: • We reject the null if it is sufficiently unlikely that the sample would be produced by the “null” population by chance. • We do not reject the null if the probability that the observed sample was pulled from the null population is reasonably large. • So our logic is that we only believe our alternative if the probability of the reverse (the null) is very low. • We have not established how low one has to go (how low the probability has to be) before one rejects the null. We are getting to this.
Hypothesis Testing: Example • We are told by a consultant that their program will, by making workers more aware of the effects of their absence, reduce the use of sick leave and personal leave. We have no reason to disbelieve her, but given the price of the program $2,500 per employee, we want to run a pilot on 50 employees before taking it to the entire population of employees. Reviewing our records, we find that, on average, employees take 10 days of sick leave and personal leave a year. The standard deviation in leave time is 8.5 days per year.
Hypothesis Testing: Example • Set up the null and alternative and test this problem. • 1. Let’s define μ as the number of absences due to sick leave or personal days. • 2. What is the null and alternative? • 3. You run the pilot and find that the mean number of sick and personal days taken was 8.7. Set up the hypothesis test. • 4. What is the z score from this test? What is the probability? • 5. Do you reject the null? Explain.
Graphical Example • We use an examination for testing new employees. We need to calibrate our criteria for passing this test to the performance of our current employees with whom we are very satisfied). • The typical standard for hiring a person who has taken this test is a score of person hired after taking this test scores 500. We believe that our employees are superior and that, if we gave this test to our current employees, the mean of the test would be more than 500. The company which designs the test cannot help us directly as, although they know the typical performance of a test taker, they do not know the typical performance of our employees. They however, agree to administer the test to a sample of 24 of our employees. We are interested in using this sample to test the hypothesis that the mean score for our employees would be more than 500. The standard deviation of the sample, after allowing for sample size, is 4. Once we know this result, we can decide whether we can default to the typical performance standard or need to more closely determine our employees performance.
Graphical Example • Suppose we want to test our belief about the population and are willing to be wrong by chance about 5% of the time. We know that 5% of the area of a normal is 1.645 standard deviations above the means, so we set up an upper cut point at +1.645 standard deviations (which is 506.58 = 1.645*4+ 500). What does this look like graphically? • What does this test look like?
Graphical Example • Note that the limits for accepting the null are set around the hypothesized population
Graphical Example
Establishing Cut Points • Establishing the Appropriate Cut-offs for rejecting the null hypothesis. • To this point, we have produced p-values from our hypothesis tests and then tried to judge whether a null was sufficiently unlikely that we were willing to reject it. • A more conventional approach is it use 10%, 5% and 1% as the standards
- More by User
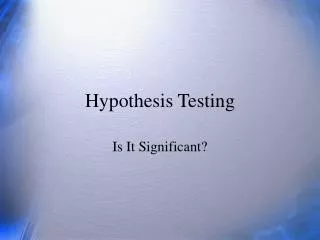
Hypothesis Testing. Is It Significant?. Questions (1). What is a statistical hypothesis? Why is the null hypothesis so important? What is a rejection region? What does it mean to say that a finding is statistically significant ?
1.29k views • 23 slides
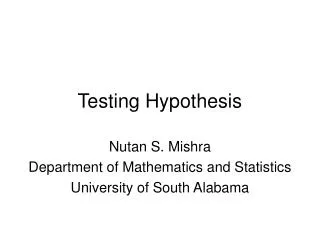
Testing Hypothesis
Testing Hypothesis. Nutan S. Mishra Department of Mathematics and Statistics University of South Alabama. Description of the problem. The population parameter(s) is unknown. Some one (say person A) has some claim about the value of this unknown parameter.
501 views • 24 slides
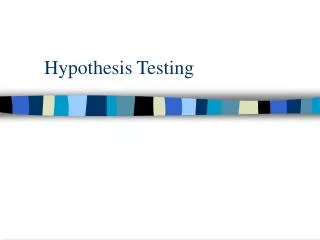
Hypothesis Testing. Another inference method. We’ve used confidence intervals to give an estimate (with a margin of error) of m . We change the question we’re asking… from, “What’s an interval that likely encloses the parameter?”
675 views • 12 slides
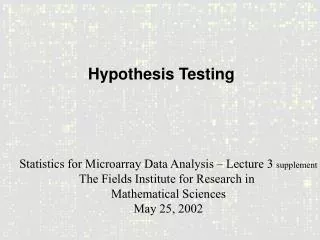
Hypothesis Testing. Statistics for Microarray Data Analysis – Lecture 3 supplement The Fields Institute for Research in Mathematical Sciences May 25, 2002. p -values.
526 views • 28 slides
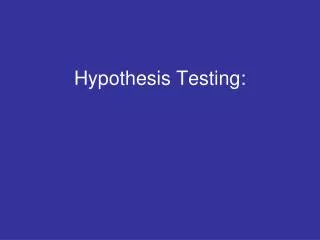
Hypothesis Testing:
Hypothesis Testing:. Inferential statistics. These will help us to decide if we should:. 1) believe that the relationship we found in our sample data is the same as the relationship we would find if we tested the entire population. OR.
793 views • 39 slides
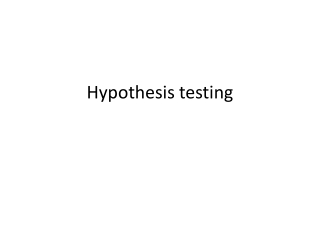
Hypothesis testing
Hypothesis testing. Null hypothesis Ho - this hypothesis holds that if the data deviate from the norm in any way, that deviation is due strictly to chance. Alternative hypothesis Ha - the data show something important.
668 views • 47 slides
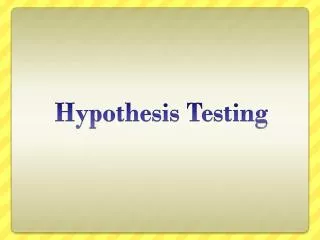
Hypothesis Testing. Overview. This is the other part of inferential statistics, hypothesis testing Hypothesis testing and estimation are two different approaches to two similar problems Estimation is the process of using sample data to estimate the value of a population parameter
1.72k views • 126 slides
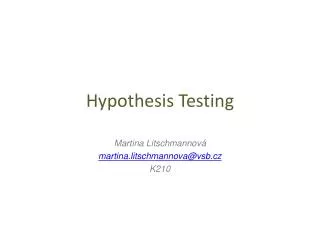
Hypothesis Testing. Martina Litschmannová m artina.litschmannova @vsb.cz K210. Terms Introduce in Prior Chapter. Population … all possible values Sample … a portion of the population Statistical inference … generalizing from a sample to a population with calculated degree of certainty
1.01k views • 37 slides
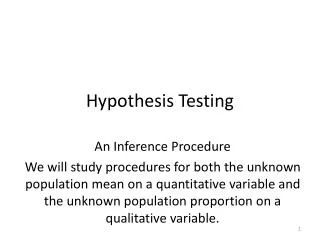
Hypothesis Testing. An Inference Procedure We will study procedures for both the unknown population mean on a quantitative variable and the unknown population proportion on a qualitative variable. Background .
323 views • 18 slides
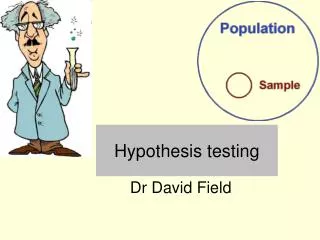
Hypothesis testing. Dr David Field. Summary. Null hypothesis and alternative hypothesis Statistical significance (p-value, alpha level) One tailed and two tailed predictions What is a true experiment? random allocation to conditions Outcomes of experiments Type I and Type II error
817 views • 39 slides
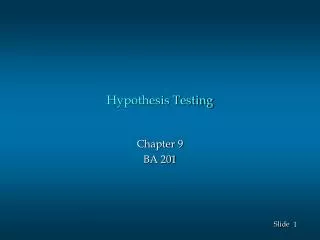
Hypothesis Testing. Chapter 9 BA 201. Hypothesis Testing. The null hypothesis , denoted by H 0 , is a tentative assumption about a population parameter. The alternative hypothesis , denoted by H a , is the opposite of what is stated in the null hypothesis.
693 views • 41 slides
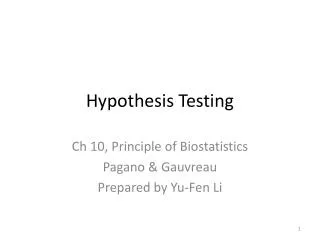
Hypothesis Testing. Ch 10, Principle of Biostatistics Pagano & Gauvreau Prepared by Yu-Fen Li. Statistical Inference. Estimation of parameters point estimation interval estimation Tests of statistical hypotheses construct a confidence interval for the parameter
478 views • 25 slides
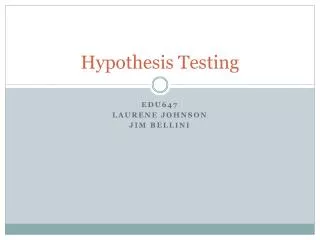
Hypothesis Testing. EDU647 Laurene Johnson Jim bellini. Null Hypothesis. Evaluated using inferential statistics No differences between groups –or– no linear relationship between variables We’ll look at the equations for these later. In terms of Sneetches. Null hypothesis.
559 views • 36 slides
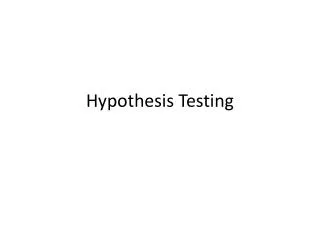
Hypothesis Testing. Coke vs. Pepsi. Hypothesis: tweets reflect market share (people tweet as much as they drink) Market share: 67% vs. 33% From tweets: 71% vs. 29% Happened by chance? Or people tend to talk more about Coke than they drink it?. A simpler hypothesis testing.
218 views • 12 slides
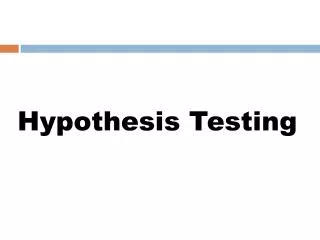
Hypothesis Testing. Hypothesis Testing. Hypothesis is a claim or statement about a property of a population. Hypothesis Testing is to test the claim or statement Example : A conjecture is made that “the average starting salary for computer science gradate is Rs 45,000 per month”.
1k views • 40 slides
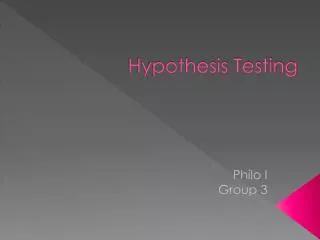
Hypothesis Testing. Philo I Group 3. What is a Hypothesis?. a tentative assumption made in order to draw out and test its logical/analytic or empirical consequences. Problems. Roots of Hypotheses Typical setting for hypothesis formation Can be anything.
360 views • 13 slides
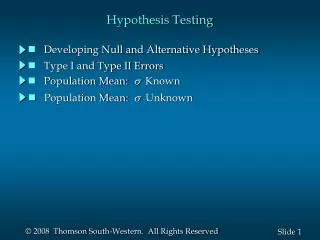
Hypothesis Testing. Developing Null and Alternative Hypotheses. Type I and Type II Errors. Population Mean: s Known. Population Mean: s Unknown. Developing Null and Alternative Hypotheses. Hypothesis testing can be used to determine whether
877 views • 69 slides
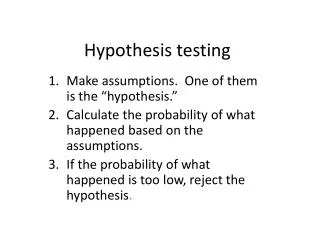
Hypothesis testing. Make assumptions. One of them is the “hypothesis.” Calculate the probability of what happened based on the assumptions. If the probability of what happened is too low, reject the hypothesis. Coin. Assumption: The probability of heads is ½. One toss possibilities:
266 views • 12 slides
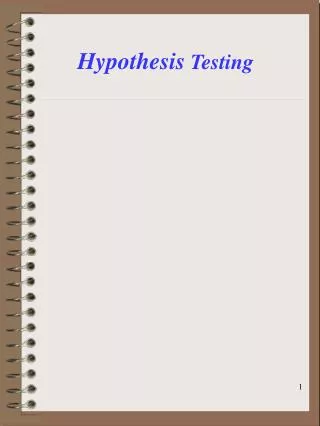
Hypothesis Testing. Hypothesis Testing. Greene: App. C:892-897 Statistical Test: Divide parameter space ( Ω ) into two disjoint sets: Ω 0 , Ω 1 Ω 0 ∩ Ω 1 = and Ω 0 Ω 1 = Ω
754 views • 38 slides
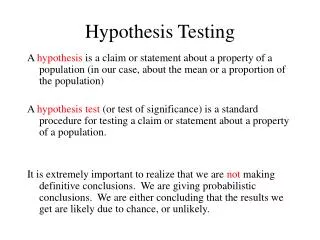
Hypothesis Testing. A hypothesis is a claim or statement about a property of a population (in our case, about the mean or a proportion of the population) A hypothesis test (or test of significance) is a standard procedure for testing a claim or statement about a property of a population.
2.63k views • 17 slides
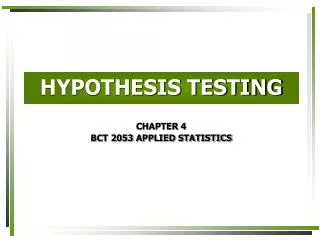
HYPOTHESIS TESTING
HYPOTHESIS TESTING. CHAPTER 4 BCT 2053 APPLIED STATISTICS. CONTENT. 4.1 Introduction to Hypothesis Testing 4.2 Hypothesis Testing for Mean with known and unknown Variance 4.3 Hypothesis Testing for Difference Means with known and unknown Population Variance
1.08k views • 42 slides
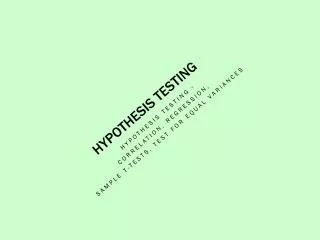
Hypothesis testing. HYPOTHESIS TESTING - CORRELATION, REGRESSION, SAMPLE T-TESTS, TEST FOR EQUAL VARIANCES. What is Hypothesis Testing?.
721 views • 44 slides
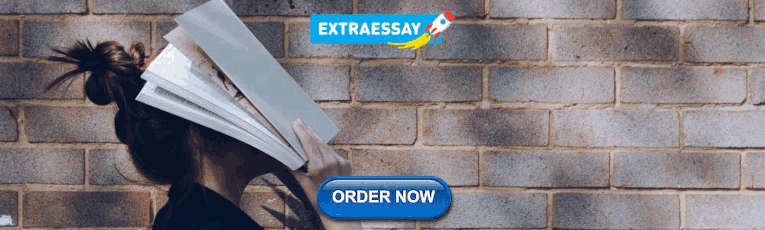
IMAGES
COMMENTS
The intent of hypothesis testing is formally examine two opposing conjectures (hypotheses), H0 and HA. These two hypotheses are mutually exclusive and exhaustive so that one is true to the exclusion of the other. We accumulate evidence - collect and analyze sample information - for the purpose of determining which of the two hypotheses is true ...
Effect size. Significance tests inform us about the likelihood of a meaningful difference between groups, but they don't always tell us the magnitude of that difference. Because any difference will become "significant" with an arbitrarily large sample, it's important to quantify the effect size that you observe.
23.1 How Hypothesis Tests Are Reported in the News 1. Determine the null hypothesis and the alternative hypothesis. 2. Collect and summarize the data into a test statistic. 3. Use the test statistic to determine the p-value. 4. The result is statistically significant if the p-value is less than or equal to the level of significance.
Specify the desired level of significance and the sample size Suppose that = 0.05 and n = 100 are chosen for this test DCOVA Hypothesis Testing Example 3. Determine the appropriate technique σ is assumed known so this is a Z test 4. Determine the critical values For = 0.05 the critical Z values are ±1.96 5.
Review: steps in hypothesis testing about the mean 1.Hypothesis a value ( 0) and set up H 0 and H 1 2.Take a random sample of size n and calculate summary statistics (e.g., sample mean and sample variance) 3.Determine whether it is likely or unlikely that the sample, or one even more extreme, came from a population with mean
Step 1: State your null and alternate hypothesis. Step 2: Collect data. Step 3: Perform a statistical test. Step 4: Decide whether to reject or fail to reject your null hypothesis. Step 5: Present your findings. Other interesting articles. Frequently asked questions about hypothesis testing.
7-1 Basics of Hypothesis Testing 7-2 Testing a Claim about a Mean: Large Samples 7-3 Testing a Claim about a Mean: Small Samples 7-4 Testing a Claim about a Proportion 7- 5 Testing a Claim about a Standard Deviation (will cover with chap 8) Chapter 7Hypothesis Testing. 7-1 Basics of Hypothesis Testing. Hypothesis in statistics, is a statement regarding a characteristic of one or more ...
Hypothesis Testing Allows us to use sample data to test a claim about a population, such as testing whether a population proportion or population mean equals some number. Example: Is the true average amount that students spent weekly on alcohol $20? General Idea of Hypothesis Testing Make an initial assumption. Collect evidence (data).
Hypothesis+Testing+Complete+Slides - Free download as Powerpoint Presentation (.ppt), PDF File (.pdf), Text File (.txt) or view presentation slides online. This document provides an overview of statistical inference and hypothesis testing. It discusses key concepts like point estimation, confidence intervals, sample means, and hypothesis testing errors.
Hypothesis Test for the Difference of Two Population Proportions • Step 1. Set up hypotheses Ho: p1 = p2 and three possible Ha's: Ha: p1 = p2 (two-tailed) or Ha: p1 < p2 (lower-tailed) or Ha: p1 > p2 (upper-tailed) Hypothesis Test for the Difference between Two Population Proportions • Step 2. calculate test statistic where.
Chapter 8 Introduction to Hypothesis Testing. PowerPoint Lecture Slides Essentials of Statistics for the Behavioral Sciences Seventh Edition by Frederick J. Gravetter and Larry B. Wallnau. Chapter 8 Learning Outcomes. Concepts to review. z- Scores (Chapter 5)
Hypothesis Testing. Chapter 6. Steps for Conducting Scientific Research Study Design This study will examine the effects of temperature on irritability. Subjects will be randomly assigned to one of three temperature conditions (low [70o-72o], moderate [80o-82o], or a high [90o-92o] temperature room). While in the room an assistant will irritate ...
File previews. pptx, 616.63 KB. A powerpoint giving an introduction to hypothesis testing using the normal distribution. It also covers type I and type II errors, and has some examples of hypothesis testing using the Poisson and Binomial distributions, plus various exam questions at the end.
The alternative hypothesis (denoted H1 or Ha) is a statement that the value of a population parameter somehow differs from the null hypothesis. The symbolic form must be a >, < or ≠ statement. We will be testing the null hypothesis directly (by assuming it's true) to reach a conclusion to either reject H0 or fail to reject H0.
Presenting this set of slides with name statistical hypothesis testing ppt powerpoint presentation slides aids cpb. This is an editable Powerpoint one stages graphic that deals with topics like statistical hypothesis testing to help convey your message better graphically. This product is a premium product available for immediate download and is ...
Presentation Transcript. Hypothesis Testing: Inferential statistics These will help us to decide if we should: 1) believe that the relationship we found in our sample data is the same as the relationship we would find if we tested the entire population OR 2) believe that the relationship we found in our sample data is a coincidence produced by ...
Hypothesis-Testing-PowerPoint-Template - 16x9. - $4.99. Add to Cart Buy Membership. Also available in Google Slides. Add to Favorite. Rating: ★ ★ ★ ★ ★. Download 100% editable Hypothesis Testing PowerPoint Template to visualize your presentation content effectively.
Hypothesis Testing - Two Samples. Chapters 12 & 13. Chapter Topics. Comparing Two Independent Samples Independent samples Z test for the difference in two means Pooled-variance t test for the difference in two means F Test for the Difference in Two Variances. Download Presentation.
Hypothesis Testing. LIR 832 Lecture #3 January 30, 2007. Topics of the Day. A. Our Fundamental Problem Again: Learning About Populations from Samples B. Basic Hypothesis Testing: One Tailed Tests Using a Z Statistic C. Probability and Critical Cutoff Approaches: Really the Same Thing