
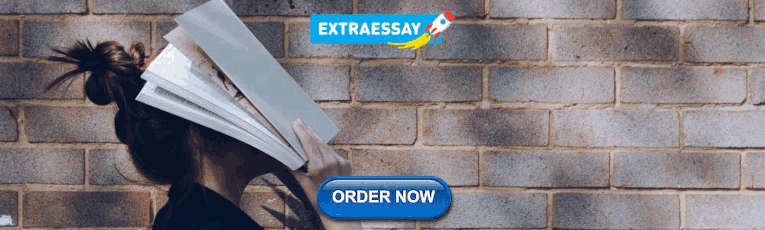
4 Case Studies in Fraud: Social Media and Identity Theft
Does over-sharing leave you open to the risk of identity theft.
Generally speaking, social media is a pretty nifty tool for keeping in touch. Platforms including Facebook, Twitter, Instagram, and LinkedIn offer us a thousand different ways in which we can remain plugged in at all times.
However, our seemingly endless capacity for sharing, swiping, liking, and retweeting has some negative ramifications, not the least of which is that it opens us up as targets for identity theft.
Identity Theft Over the Years
Identity theft isn’t a new criminal activity; in fact, it’s been around for years. What’s new is the method criminals are using to part people from their sensitive information.
Considering how long identity theft has been a consumer threat, it’s unlikely that we’ll be rid of this inconvenience any time soon.
Living Our Lives Online
The police have been using fake social media accounts in order to conduct surveillance and investigations for years. If the police have gotten so good at it, just imagine how skilled the fraudsters must be who rely on stealing social media users’ identities for a living.
People are often surprised at how simple it is for fraudsters to commit identity theft via social media. However, we seem to forget just how much personal information goes onto social media – our names, location, contact info, and personal details – all of this is more than enough for a skilled fraudster to commit identity theft.
In many cases, a fraudster might not even need any personal information at all.
Case Study #1: The Many Sarah Palins
Former Alaska governor Sarah Palin is no stranger to controversy, nor to impostor Twitter accounts. Back in 2011, Palin’s official Twitter account at the time, AKGovSarahPalin (now @SarahPalinUSA ), found itself increasingly lost in a sea of fake accounts.
In one particularly notable incident, a Palin impersonator tweeted out an open invite to Sarah Palin’s family home for a barbecue. As a result, Palin’s security staff had to be dispatched to her Alaska residence to deter would-be partygoers.
This phenomenon is not limited only to Sarah Palin. Many public figures and politicians, particularly controversial ones like the 2016 presidential candidate Donald Trump, have a host of fake accounts assuming their identity.
Case Study #2: Dr. Jubal Yennie
As demonstrated by the above incident, it doesn’t take much information to impersonate someone via social media. In the case of Dr. Jubal Yennie, all it took was a name and a photo.
In 2013, 18-year-old Ira Trey Quesenberry III, a student of the Sullivan County School District in Sullivan County, Tennessee, created a fake Twitter account using the name and likeness of the district superintendent, Dr. Yennie.
After Quesenberry sent out a series of inappropriate tweets using the account, the real Dr. Yennie contacted the police, who arrested the student for identity theft.
Case Study #3: Facebook Security Scam
While the first two examples were intended as (relatively) harmless pranks, this next instance of social media fraud was specifically designed to separate social media users from their money.
In 2012, a scam involving Facebook developed as an attempt to use social media to steal financial information from users.
Hackers hijacked users’ accounts, impersonating Facebook security. These accounts would then send fake messages to other users, warning them that their account was about to be disabled and instructing the users to click on a link to verify their account. The users would be directed to a false Facebook page that asked them to enter their login info, as well as their credit card information to secure their account.
Case Study #4: Desperate Friends and Family
Another scam circulated on Facebook over the last few years bears some resemblance to more classic scams such as the “Nigerian prince” mail scam, but is designed to be more believable and hit much closer to home.
In this case, a fraudster hacked a user’s Facebook profile, then message one of the user’s friends with something along the lines of:
“Help! I’m traveling outside the country right now, but my bag was stolen, along with all my cash, my phone, and my passport. I’m stranded somewhere in South America. Please, please wire me $500 so I can get home!”
Family members, understandably not wanting to leave their loved ones stranded abroad, have obliged, unwittingly wiring the money to a con artist.
Simple phishing software or malware can swipe users’ account information without their having ever known that they were targeted, thus leaving all of the user’s friends and family vulnerable to such attacks.
How to Defend Against Social Media Fraud
For celebrities, politicians, CEOs, and other well-known individuals, it can be much more difficult to defend against social media impersonators, owing simply to the individual’s notoriety. However, for your everyday user, there are steps that we can take to help prevent this form of fraud.
- Make use of any security settings offered by social media platforms. Examples of these include privacy settings, captcha puzzles, and warning pages informing you that you are being redirected offsite.
- Do not share login info, not even with people you trust. Close friends and family might still accidentally make you vulnerable if they are using your account.
- Be wary of what information you share. Keep your personal info under lock and key, and never give out highly sensitive information like your social security number or driver’s license number.
- Do not reuse passwords. Have a unique password for every account you hold.
- Consider changing inessential info. You don’t have to put your real birthday on Facebook.
- Only accept friend requests from people who seem familiar.
Antivirus software, malware blockers, and firewalls can only do so much. In the end, your discretion is your best line of defense against identity fraud.
You might also enjoy a great Motivational Speaker Video for social media safety tips
Jessica Velasco
Bachelor Finale Joey Graziadei gets Engaged In the highly anticipated Bachelor finale, tensions ran high as Joey had to choose who would get the fi…
Mike Tyson and Jake Paul Fight is Streaming on Netflix
Dodgers’ shohei ohtani reveals he is married, subscribe to the skinny.
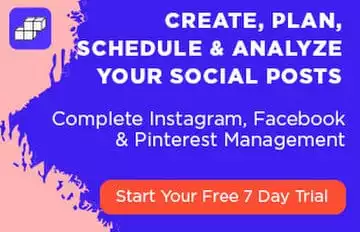
The Rise of Mobile Betting Apps in NBA: Convenience and Accessibility
Strategies for building strong and mutually beneficial vendor relationships, happy april fools day: how to avoid getting tricked online, bachelor finale joey graziadei gets engaged, choosing the right case management software: considerations for law firms, adapting to the business landscape: exploring ai learning, construction, engineering, and home improvements, how and why facial recognition technology is safe, the best sports streaming services in the us.
Exploring the determinants of victimization and fear of online identity theft: an empirical study
- Original Article
- Published: 21 July 2022
- Volume 36 , pages 472–497, ( 2023 )
Cite this article
- Inês Guedes ORCID: orcid.org/0000-0002-4804-9394 1 ,
- Margarida Martins 1 &
- Carla Sofia Cardoso 1
5607 Accesses
8 Citations
1 Altmetric
Explore all metrics
The present study aims at understanding what factors contribute to the explanation of online identity theft (OIT) victimization and fear, using the Routine Activity Theory (RAT). Additionally, it tries to uncover the influence of factors such as sociodemographic variables, offline fear of crime, and computer perception skills. Data for the present study were collected from a self-reported online survey administered to a sample of university students and staff ( N = 832, 66% female). Concerning the OIT victimization, binary logistic regression analysis showed that those who do not used credit card had lower odds of becoming an OIT victim, and those who reported visiting risky contents presented higher odds of becoming an OIT victim. Moreover, males were less likely than females of being an OIT victim. In turn, fear of OIT was explained by socioeconomic status (negatively associated), education (positively associated) and by fear of crime in general (positively associated). In addition, subjects who reported more online interaction with strangers were less fearful, and those reported more avoiding behaviors reported higher levels of fear of OIT. Finally, subjects with higher computer skills are less fearful. These results will be discussed in the line of routine activities approach and implications for online preventive behaviors will be outlined.
Similar content being viewed by others
The Online Behaviour and Victimization Study: The Development of an Experimental Research Instrument for Measuring and Explaining Online Behaviour and Cybercrime Victimization
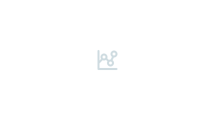
Examining cybercrime victimisation among Turkish women using routine activity theory
Mine Özaşçılar, Can Çalıcı & Zarina Vakhitova
Routine Activities
Avoid common mistakes on your manuscript.
Introduction
The Internet provides unique affordances for criminal activities, especially due to the ease of information dissemination and anonymity of perpetrators. Furthermore, it extends traditional crimes (such as fraud or identity theft) to online offenses or create new types of criminal activity (e.g., malware infection). One of the crimes that can be considered an extension from traditional offenses is the online identity theft (OIT). OIT is considered one of the fastest growing crimes (Holt and Turner 2012 ; Golladay and Holtfreter 2017 ), resulting in relevant financial losses to victims. Knowing what factors might increase the risks of online victimization in general and OIT in particular is crucial. Although there is not one widely accepted definition of identity theft due to its complexity, it can be conceptualized as the “unlawful use of another’s personal identifying information” (Bellah 2001 , p. 222) such as accessing existing credit cards or bank accounts without authorization. Similarly, Koops and Leenes ( 2006 ) define identity theft as the unlawful acquisition and misuse of another individual’s personal information for criminal purposes. Therefore, the information that is obtained by the offender is acquired without the owner’s consent through illegal means. Researchers have been trying to established the factors that affect the likelihood of victimization of OIT. Among the criminological theories that have been used to explain this type of online victimization, Routine Activity Theory (RAT) is one of the most empirically tested (Cohen and Felson 1979 ; Reyns 2013 ; Reyns and Henson 2015 ; Reyns 2015 ; Bossler and Holt 2009 ). Moreover, while the research related to fear of crime in general is vast, the empirical studies aimed at understanding the fear of cybercrime are scarce, especially the fear of OIT. According to the 2019 Special Eurobarometer focusing on the European’s attitudes towards cyber security, although 92% did not report being a victim of identity theft in the last three years, at least two thirds of the sample reported to feel concerned about suffering a victimization of bank card or online banking fraud (67%) and of identity theft (66%). When comparing to the victimization of online crime or even to the fear of general crime, little is known about the online determinants of fear of identity theft.
The present study has two main objectives, namely to analyze the factors that increase (i) the risk of victimization of online identity theft and (ii) the fear and perceived risk of online identity theft. Concretely, the first objective aims at understanding what factors contribute to the explanation of online identity theft victimization , namely the exposure to potential offenders, target suitability and guardianship in the online context. Additionally, it tries to uncover the influence of factors such as sociodemographic variables and computer perception skills. Second, in order to understand what factors are related to fear and perceived risk of online identity theft , the present work will also test the influence of core dimensions of RAT, sociodemographic variables, previous victimization and general fear in those dependent variables.
Concerning the structure through which the present article will be developed, firstly the main crucial concepts and theories (e.g., online identity theft, RAT) will be outlined, followed by the empirical findings on the (i) relationship between RAT, individual variables and victimization of online identity theft and on the (ii) relationship between RAT, individual variables and fear of online identity theft. Next, the methodology of the present study will be described in detail. Finally, the results will be presented and discussed, including the implications of the present study.
- Online identity theft
Identity theft is a term used to classify numerous offenses including fraudulent use of personal information for criminal purposes without individuals’ consent (Reyns 2013 ). Harrell ( 2015 , p. 2) defines identity theft as the “unauthorized use or attempted use of an existing account (such as credit/debit card, savings, telephone, online), the unauthorized use or attempted use of personal information to open a new account or misuse of personal information for a fraudulent purpose such as providing false information to law enforcement”. Accordingly, Copes and Vieraitis ( 2012 ) state that identity theft includes the misuse of another individual’s personal information to commit fraud. Identity theft may or may not be committed through technological and informatic means (Wang et al. 2006 ). Online or cyber identity theft involves the online misappropriation of identity tokens such as email addresses, passwords to access online banking or web-pages (Roberts et al. 2013 ). In the present paper, we define OIT as the illicit and improperly use, via internet, of the personal and financial data which was obtained without prior consent and knowledge by the cyber-criminal. In order to perpetrate this crime, offenders usually employ a mix of Information and Communication Technologies and social engineering, using methods such as hacking, phishing and pharming. Phishing involves attempts to mislead individuals into revealing sensitive information by posing as a legitimate entity such as banks. Hacking is an unauthorized access which may be way of unto itself or for malicious purposes such as spreading malware. Pharming occurs when, through the use of a virus or a similar technique, individual’s browser is hijacked without their knowledge. Therefore, when targets type a legitimate website into the address bar of their browser, the virus will be redirected them to a fake site. In addition to these types of methods, research have been analyzing the relationship between data breaches and identity theft (e.g., Garrison and Ncube 2011 ; Burnes et al. 2020 ). For instance, Tatham ( 2018 ) observed that those who have been impacted by a database breach were 31.7% more likely to experience identity fraud compared to 2.8% of persons not alerted of a data breach. This result can be explained by the fact that the information obtained in a database breach is generally sold online, containing personally identifiable information that can be used to commit identity theft crimes. Therefore, in spite of the relevance of individual online activities, data breaches that target retailers or government entities, for instance, may also increase the risk of online identity theft.
Identity theft is considered one of the most feared, fastest-growing crimes and a public health problem (Burnes et al. 2020 ). In fact, Harrel and Langton ( 2013 ) estimated that the average loss experienced by victims of identity theft was $2183 in 2012. Later, an estimate made by Javelin Strategy and Research showed that the costs of identity theft were near $16 billion (Piquero 2018 ). Besides the financial losses, it has also been observed that, on average, victims spend at least 15–30 h (although it might take several years) solving financial problems related to identity theft. Recently, Golladay and Holtfreter ( 2017 ) analyzed the nonmonetary losses experienced by victims of identity theft through a survey where 3709 individuals reported experiencing some form of identity theft in the past 12 months. The authors found that victims of this crime, as with other forms such as bullying and violence, suffered relevant emotional and physical symptoms. Moreover, that the distress involved in recovering from identity theft could be conceived as a stressor resulting in negative emotions such as depression and anxiety. These results have been consistently reported by other investigations (e.g., Reyns and Randa 2017 ; Li et al. 2019 ; Harrell 2019 ) that found diverse emotional problems such as anxiety, irritation and distress following victimization of (online) identity theft. As we have been seen an increasing in the numbers of victims over the years (Li et al. 2019 ), as well as a set of empirically demonstrated harmful financial and emotional consequences, studying why someone is more prone to be victimized of online identity theft is fundamental. Moreover, it is crucial to understand if individuals fear this type of crime and what are the factors that explain the higher levels of fear of online identity theft. The Routine Activity Theory (or RAT) may help to understand what are the behaviors related to both victimization and fear of OIT.
Routine activity theory
In 1979, Cohen and Felson argued that “structural changes in routine activity patterns can influence crime rates by affecting the convergence in space and time of three minimal elements of direct-contact predatory violations: (1) motivated offenders, (2) suitable targets, and (3) the absence of capable guardians against a violation” (p. 589). Therefore, the RAT was a perspective developed to account for offenses and victimization in the physical world. In the context of cybercrime, authors such as Yar ( 2005 ) and Leukfeldt and Yar ( 2016 ) have been debated the applicability of this framework. In fact, the requirement of offenders and victims converging in time and space for a crime to happen presents a problem when applying the theory to cybercrimes. Gabrowsky ( 2001 ), opposing to ‘transformationists’ like Capeller ( 2001 ) argued that RAT could be applied to crimes in cyberspace since it was just a case of “new wine in old bottles”. In the same direction, Eck and Clark ( 2003 ) suggested that this framework can be useful to explain these types of online crimes since the target and the offender are part of the same geographically dispersed network, and therefore the offender is able to reach the target through network. In accordance, Reyns et al. ( 2011 ) argued that instead of a real-time convergence of victims and offenders within networks, the temporal overlap between them may be lagged for either a short time or a longer period. Therefore, according to RAT, it is expected that online activities—such as banking, shopping, instant messaging or downloading media, might be associated with higher levels of victimization. Moreover, that greater levels of security measures—installing antivirus software, filtering spam email or routinely changing passwords—might decrease opportunities for offenders to access personal information.
Although the RAT has been tested to explain different online forms of victimization such as phishing, hacking, interpersonal violence, malware infection or internet fraud (e.g., Choi 2008 ; Holt and Bossler 2009 ; Marcum et al. 2010 ; Raising et al. 2009 ), few studies have empirically examined OIT victimization from a routine activities’ perspective (e.g., Reyns 2013 ; Reyns and Henson 2015 ; Burnes et al. 2020 ). Furthermore, most studies have not operationalized all of the core dimensions of the theory (i.e. exposure to motivated offenders, target suitability and capable guardianship). Departing from the RAT, and including as well individual variables, we now review the main determinants of victimization and fear of online identity theft.
Determinants of victimization of online crime
Rat core dimensions.
The exposure to risky situations and proximity to offenders (online exposure) is usually conceptualized from the victim’s perspective (Reyns and Henson 2015 ), and have been operationalized through different ways (e.g., ‘the amount of time spent on the internet’ or ‘the amount of time doing specific activities’ Ngo and Paternoster 2011 ; Reyns 2013 ). The results of the studies that explore the relationship between exposure and online victimization are mixed. For instance, Holt and Bossler ( 2013 ) found that internet usage (measured through number of hours per week) did not predict the likelihood of malware infection victimization. Furthermore, in a recent study, Holt et al. ( 2020 ) observed that time spent on specific online activities, such as downloading files and visiting dating websites consistently increased the risk of victimization of malware software infections. This result is consistent with the research employed by Alshalan ( 2006 ) who found that risk exposure (measured through the frequency of using the Internet and duration) was a determinant of victimization of computer virus and cyber-crime. A study by Pratt et al. ( 2010 ) observed that making a purchase online from a website doubled the likelihood of being targeted for online consumer fraud. In turn, Ngo and Paternoster ( 2011 ) found that the number of hours per week that respondents engaged in instant messaging increased the likelihood of experience online harassment by a stranger. However, none of the measures of online routine activities were relevant to phishing victimization. This result was contrary to what was found by Reyns ( 2015 ) who concluded that booking/making reservations, online social networking, online banking and purchasing behaviors were related to phishing. Finally, focusing specifically on OIT, both Reyns ( 2013 ) and Reyns and Henson ( 2015 ) observed that banking and purchasing, as measures of online exposure, positively contributed to OIT victimization. This result was confirmed by a recent study employed by Burnes et al. ( 2020 ). In fact, as the authors concluded, while participating in commercial activities (such as online purchasing) “reflects a major societal innovation and lifestyle shift that has allowed consumers to purchase products conveniently and globally (…) the odds of using an unsecured payment portal or having information exposed in a retail data breach increases” (p. 6).
In the context of cybercrime, target attractiveness is usually operationalized through visiting risky or unprotected websites or divulge personal information online. Even though different studies argue that target suitability is a relevant TAR dimension in explaining online victimization, the results are mixed. For instance, Alshalan ( 2006 ) observed that the more people divulge their credit or debit card number and disseminate their personal information, the more they are at risk of becoming victims of cybercrime. Accordingly, Reyns and Henson ( 2015 ) suggested that having personal information posted online increases victimization of OIT. In 2013, van Wilsem found that spending much time on Internet communication activities increased the risk of victimization including online harassment. Nevertheless, Ngo et al. ( 2020 ) observed that individuals who conduct online banking and plan their travels online had a lower risk of experiencing harassment by a non-stranger. The authors suggest that individuals who conduct these types of activities online were less likely to engage in computer deviance giving the positive relationship between being harassed and deviance online. At the same time, Ngo and Paternoster ( 2011 ) observed that individuals who frequently opened unfamiliar attachments to emails received or frequently opened any file or attachment they received through instant messenger had lower odds of obtaining a computer virus. Lastly, using a sample of 9161 Internet users, Leukfeldt and Yar ( 2016 ) found that only one online activity (targeted browsing, or the search for news or targeted information search) had an effect on identity theft victimization.
Finally, scientific literature has been studying the effects of guardianship on the likelihood of victimization. The online guardianship dimension has been measured through different ways—e.g., shredding personal documents, actively changing account passwords, using antivirus software, deleting emails from unknown senders (Reyns and Henson 2015 ; Ngo and Paternoster 2011 ; Holt and Bossler 2013 ), producing as well mixed results. Holt et al. ( 2020 ) found that having a secured wireless connection decreased the risk of malware victimization. A few years before, explaining the malware victimization, Holt and Bossler ( 2013 ) found that having low-level of computer skills (a measure of lack of guardianship) and using anti-virus software (presence of guardianship) were among the significant predictors of that crime. In turn, Reyns and Henson ( 2015 ) observed that none of the online guardianship measures were relevant to reduce victimization of OIT. One possible explanation is that some victims adopted guardianship routines strategies post to their victimization. Williams ( 2016 ) observed that passive physical guardianship was effective in reducing online identity theft due to the automated form of this type of security when comparing to the others. Moreover, a positive association was found between active personal guardianship and victimization, explained by the post-victimization security reactions. Data collected by Reyns ( 2015 ) suggested a positive relationship between guardianship and victimization for phishing, hacking and malware infection, contrary to what was hypothesized by the author. Accordingly, Williams ( 2016 ) observed that having an anti-virus software was positively related to malware victimization, suggesting that individuals who experienced online victimization were more prone to install this type of software. At the same time, a positive relationship between phishing victimization and deleting emails from unknown senders was observed. Finally, Burnes et al. ( 2020 ) observed that proactive individual behaviors (e.g., shredding personal documents and actively changing account passwords), reduced the likelihood of identity theft.
Individual variables
Numerous research studies have also examined the impact of individual variables on online victimization. Alshalan ( 2006 ) found that gender had an effect on both computer virus victimization and cybercrime victimization, suggesting that males are more victimized than females. Accordingly, Holt and Turner ( 2012 ) and Reyns ( 2013 ) observed that males were at greater risk of identity theft victimization. Conversely, Anderson ( 2006 ) discovered that the estimated risk of experiencing some form of identity theft was 20% more greater for women than for men. Concerning age, while Reyns ( 2013 ) observed that older adults presented higher risk of identity theft victimization, Williams ( 2016 ) and Harrell ( 2015 ) found that younger and middle-aged adults, respectively, reported higher levels of this kind of victimization. Ngo and Paternoster ( 2011 ) observed that each additional year in age decreased the odd of obtaining a computer virus by 2% and of experiencing online defamation by 6%. More recently, Burnes et al. ( 2020 ) observed that individuals between the ages of 39 and 73 were at the highest risk of most types of identity theft, reflecting the socioeconomic capacity and consumption patterns of this generation relative to millennials. Another important result discovered by these authors was that higher educational accomplishment was related with higher risk of existing credit card/bank account identity theft victimization. Accordingly, Reyns ( 2013 ) and Reyns and Henson ( 2015 ) observed that those with higher incomes are most likely to be victimized of OIT. Williams ( 2016 ) showed that social status exhibited a curvilinear association with victimization. Lower and higher status citizens reported highest levels of victimization, while those of average status reported the lowest. Lastly, none of the sociodemographic variables—gender, age, education level, personal or household income and financial assets or possessions explained identity theft victimization in Leukfeldt and Yar’s ( 2005 ) research.
Fear of (online) crime
Fear of crime is considered a serious social problem and has been vastly studied in the last decades (Hale 1996 ). Empirical research has been testing what are the determinants of fearing crime, including individual (e.g., gender, age, social class, education,) and contextual predictors (e.g., incivilities, social cohesion, poor street lighting). Using a wider definition of fear of crime, this construct is conceptualized as a set of three dimensions: the emotional fear of crime, risk perception of victimization and the different behaviors adopted for security reasons (e.g., Gabriel and Greve 2003 ; Liska et al. 1988 ). The emotional fear of crime is a response to crime or symbols associated with it (Ferraro and La Grange 1987 ; Rader et al. 2007 ), different from risk perception which is the likelihood of victimization perceived by an individual (for instance, the likelihood of being a victim of burglary in the next 12 months ). Though they can be related (Mesch 2000 ), the cognitive dimension is distinct from the emotional fear of crime and it refers to an assessment of personal threat or a judgment that individuals make of their risk of victimization. Authors have also been distinguished formless fear from concrete fear. While the first is a generic fear not related to any type of crime, the latter corresponds to specific crimes such as fear of identity theft or fear of robbery (for instance, Keane 1992 ).
Although fear of general crime has been largely studied, limited research has been conducted about fear of online crime and even less on fear of OIT (Henson et al. 2013 ; Virtanen 2017 ; Abdulai 2020 are some exceptions). Hille et al. ( 2015 ) included two main dimensions on the fear of OIT: the fear of financial losses and fear of reputational damage . While the first is the fear of illegal or unethical appropriation and usage of personal and financial data by an unauthorized entity with the goal of getting financial benefits, the second is the fear of misuse of illegally acquired personal data with the objective of impersonation which can cause reputational damage to the victim—for instance, use the victim’s credit card to buy embarrassing products. In the present paper, we follow Hille’s et al. ( 2015 ) definition of fear of OIT.
Exploring fear of OIT is crucial since it is considered one of the most important psychological barriers to consumers (Martin et al. 2017 ), and constitutes a severe threat to the security of online transactions associated with e-commerce services. For instance, Jordan et al. ( 2018 ) explored the impact of fear of identity theft and perceived risk on online purchase intention using a sample of 190 individuals from Slovenia. First, they found a positive correlation between fear of identity theft and perceived risk. Then, they observed that perceived risk decreased the online purchase intention. Now we summarize the main determinants of fear of online crime and the results of the few studies which focused on the explanation of fear of OIT.
Determinants of fear of crime online
Sociodemographic determinants.
Gender is considered the best predictor of fear of crime in general (Hale 1996 ) with women being considered the most fearful group. Under cybercrime research, a set of studies concluded that women are as well the most fearful group, although it depends on concrete crimes. For instance, while Henson et al. ( 2013 ) found higher levels of fear on women of interpersonal violence, Yu ( 2014 ) observed no statistically significant differences between women and men for online identity theft, fraud and virus. Virtanen ( 2017 ) employed an analysis of the fear of eight types of cybercrimes in 28 countries using data from the Special Eurobarometer Survey. The author consistently found that women presented higher levels of fear of cybercrimes comparing to men. Lastly, Roberts et al. ( 2013 ) discovered that gender was not a predictor of fear of OIT which was mainly explained by contextual dimensions and the fear of traditional crime.
Concerning the relationship between age and offline fear of crime, it can be argued that the results are mixed. While some authors found that older people presented higher levels of fear (e.g., Reid and Cornrad 2004 ), others conclude that younger individuals report higher levels of fear when comparing with older adults (e.g., Ziegler and Mitchel 2003 ). In the online context, the relationship between age and fear is also not well established. For instance, Virtanen ( 2017 ) and Henson et al. ( 2013 ) found that younger people fear more online crime comparing to older ones. Roberts et al. ( 2013 ) observed that age (with a positive direction) was the only predictor of fear of OIT but it accounted for less than 1% of the unique variance in fear of OIT and related fraudulent activity. Accordingly, Alshalan ( 2006 ) and Lee et al. ( 2019 ) found that older individuals presented higher levels of fear of online crimes, suggesting that they attribute more value to property and, as a consequence, they fear losing it.
Regarding the impact of socioeconomic status, researchers have been demonstrated that groups with higher disadvantage feel more afraid offline when compared to lesser ones since they present fewer capacity to afford measures to their protection (Hale 1996 ). Accordingly, in the online context, Virtanen ( 2017 ) and Brands and Wilsem ( 2019 ) found that individuals with lower socioeconomic status presented higher levels of fear of online crime, suggesting that less advantage groups might find more difficult to deal with potential costs of an online victimization. In turn, Reisig et al. ( 2009 ) found that those reporting lower levels of socioeconomic status perceived higher levels of risk which, in turn, was associated with online behaviors that reduced the potential likelihood of online theft victimization. Concerning education, typically the literature on traditional fear of crime finds that less educated individuals report higher levels of fear (e.g., Smith and Hill 1991 ). While Alshalan ( 2006 ) and Roberts et al. ( 2013 ) found no direct association between education and general fear of cybercrime, Brands and Wilson ( 2019 ) observed lower levels of fear of online crime in higher educated individuals. On the opposite direction, Akdemir ( 2020 ) found that higher educated individuals reported higher levels of fear of cybercrime comparing to those who were less educated. Accordingly, the author argued that higher educated groups had a higher likelihood of adopting online security measures such as password management (e.g., use of multiple passwords) and elimination of suspect emails.
Previous victimization
The relationship between victimization and fear of traditional crime has been produced mixed results. For instance, Mesch ( 2000 ), Tseloni and Zarafonitou ( 2008 ) and Guedes et al. ( 2018 ) found a positive relationship between perceived risk and victimization. Regarding the relationship between fear of online crime and victimization, the few studies available observed that previous experiences with online crime are important to fear. For instance, Randa ( 2013 ) found that past experiences with cyberbullying increased fear of online crime. Moreover, Alshalan ( 2006 ) also corroborated that previous direct victimizations increased fear of cybercrime, suggesting that the negative consequences of a victimization had a ‘sensitizing effect’ which increased the insecurity on cyberspace. Additionally, Henson et al. ( 2013 ), Virtanen ( 2017 ), Lee et al. ( 2019 ), Brands and Wilsem ( 2019 ) and Abdulai ( 2020 ) suggested that previous online victimization had an impact of fear of online crime. On the contrary, non-significant results were found in Yu’s ( 2014 ) study.
Exposure to online crime and technical skills
The relationship between exposure to online crime and fear has been scarcely investigated. An exception is Roberts and colleagues’ study ( 2013 ) who found that frequency of internet use and use of internet at home where significant predictors of fear of cyber-identity theft and related fraudulent activity. Conversely, Henson et al. ( 2013 ) observed that none of the exposure variables showed statistically significant effects on online fear. Lastly, Virtanen ( 2017 ) showed that frequency of internet use was not associated with fear of cybercrime in models with interactions between gender or social status and victimization. However, the author suggested that the effects of internet use and knowledge of risks were mediated by confidence in one’s abilities. Concerning technical skills, few studies have been investigating if the perception of low technical skills is related with a higher fear of online crime. For instance, Virtanen ( 2017 ) found a negative relationship between these variables, although the author observed that being in the group with the lowest confidence in one’s abilities was not a direct predictor of fear. Finally, Abdulai ( 2020 ) observed that knowledge of cybercrime was not a predictor of fear of becoming a victim of credit/debit card fraud.
Current focus
The present study has the main objective of identifying risk factors both for OIT victimization and fear of OIT (including fear and perceived risk of victimization). Despite some knowledge on the factors associated with several forms of online victimization, there have been relatively few empirical studies on the factors that affect the likelihood of online identity theft. Additionally, existing research rarely addresses all the core dimensions of RAT (online exposure, target suitability and online guardianship). Thus, it is unclear what online activities increase the risk of online identity theft victimization and who are the most targeted individuals in terms of personal characteristics. Furthermore, while the victimization of OIT has been studied in the last years, to what extent and why individuals feel more fearful of OIT has rarely been the focus of scientific research.
Therefore, our investigation is crucial and innovative since: (a) operationalizes all the core concepts included in RAT (online exposure, target suitability and online guardianship) to fully test its prediction of OIT victimization, (b) examines not only the victimization but also the fear and perceived risk of being a victim of OIT; (c) compares the importance of both individual variables and contextual variables both for victimization and fear of this form of victimization.
Data for the current study were collected in 2017 through an online self-report anonymous survey built to explore the variables related to RAT that influenced both the victimization, the perceived risk and the fear of OIT. For that purpose, an email containing the objective of the study and the link of the survey was sent by the University of Porto services to invite students and staff (teaching and non-teaching) to participate in this study. In total, 831 individuals answered the questionnaire.
Dependent variables
In the current study three dependent variables were considered: the OIT victimization, the fear of OIT and the perception of victimization risk concerning the previous referred crime. To measure OIT victimization, respondents were asked: “how many times someone has appropriated, via Internet, personal and financial data without prior consent and knowledge and used them improperly during your lifetime” . Responses varied between 0 to more than 5 times. Lifetime OIT victimization was recoded to a dummy variable (0 = no victimization, 1 = victimization). Fear of OIT was operationalized through an adapted scale of Hille et al. ( 2015 ). This scale conceptualized fear of OIT as having two main dimensions: fear of financial losses and fear of reputational damage. A four-point Likert scale was used to rate the following items varying from 1 (not afraid) to 4 (very afraid): “how fearful do you feel if” … (1) somebody steal your personal and financial data via online? (2) somebody use your personal and financial data via online? (3) somebody damage your reputation based on the illegitimate use of your personal and financial data online?
Concerning the risk perception of victimization related to OIT it was asked the participants to rate in a scale of 1 (not likely) to 5 (very likely) the following items: “ how likely do you think that…” (1) somebody could steal your personal and financial data via online during the next 12 months? (2) somebody could use your personal and financial data via online during the next 12 months? (3) somebody could damage your reputation based on the illegitimate use of your personal and financial data online during the next 12 months? Therefore, perceived risk of OIT was adapted from scale of fear of OIT built by Hille et al. ( 2015 ) and from previous literature that conceptualizes perceived risk as the estimated likelihood of being a victim of crime in the future (e.g., Guedes et al. 2018 ).
Individual characteristics
In order to study how sociodemographic dimensions influenced the dependent variables, insofar as these may be related to patterns of Internet usage and victimization experiences, four individual characteristics of respondents were included: (a) gender (0 = male, 1 = female), (b) age (in years), (c) perceived socioeconomic status (1 = low, 2 = average, 3 = high) and (d) levels of education (1 = up to 4th years of education to 5 = postgraduate studies). Furthermore, a measure of fear of crime offline was included to understand if fear of identity theft online (concrete fear) was influenced by a more general fear of being victimized. The operationalization was based on previous studies and a two item’s measure was undertaken: (a) “How safe do you feel when walking alone in your residential area after dark?” , ( b) How safe do you feel being alone in your home after dark?”.
Three theoretical dimensions from RAT were measured: online exposure to motivated offenders, target suitability, and capable guardianship.
Online exposure
Departing from one of the aims of the present study, analyzing the relationship between exposure and victimization, fear and perceived risk of OIT, respondents were asked a set of questions to measure their online routines. Thus, to examine Internet routine activities, two different measures were used. The first was assessed by the single item “how much time, per day, in average, do you spend online?”, measured in number of hours. Additionally, in an effort to examine specific online routine activities, the frequency of specific online routines activities was rated by participants in a scale of 1 (never) to 5 (always). Following Reyns ( 2013 ), participants were asked “how often do you use the Internet for the ensuing purposes” : (1) online banking or managing finances, (2) e-mail or instant messaging, (3) watching television or listening to the radio, (4) reading online newspapers or news websites, (5) participating in chat rooms or other forums, (6) reading or writing blogs, (7) downloading music, films, or podcasts, (8) social networking (e.g., Facebook, Myspace), (9) for work or study, or (10) buying goods or services (shopping). After performing a factor analysis, the items were aggregated in three main routine activities: (1) financial routines (items 1 and 10), (2) work routines (items 2, and 9), and (3) leisure routines (items 3, 5, 6 and 7). Finally, to understand the types of payments used by respondents, we asked if they used the following forms: home banking, PayPal, credit card, pay safe card, MB Net. Respondents had two response options: ‘yes’ (coded as 1) and ‘no’ (coded as 0).
Target suitability
One of the factors that can increase individual’s likelihood of being victimized (both online and offline) is his or her attractiveness as a potential target (Reyns and Henson 2015 ). In the present study, based on previous works such as those of Paternoster (2011) and Reyns ( 2015 ), online target suitability was measured through the following question: “In the past 12 months have you” : (1) communicated with strangers online, (2) provided personal information to somebody online, (3) opened any unfamiliar attachments to e-mails that they received, (4) clicked on any of the web-links in the emails that they received, (5) opened any file or attachment they received through their instant messengers, (6) clicked on a pop-up message, or (7) visited risky websites . Participants had two response options: ‘yes’ (coded as 1) and ‘no’ (coded as 0). After performing a factor analysis, responses were computed in three indexes: (a) interaction with unknown people (items 1, 2), (b) open dubious links (items 3, 4, 5), and c) visit risky on-line contents (items 6, 7).
Online capable guardianship
Theoretically, higher guardianship might be related to lower levels of victimization in general and victimization of OIT in particular, especially in individuals who protect their personal information through multiple security behaviors. Moreover, it is expected that individuals who fear and perceived more risk of OIT are more prone to adopt these types of security measures. In the present study, to measure the capable guardianship, 13 items based on Williams ( 2016 ) and Ngo and Paternoster’s ( 2011 ) works were used. It was asked participants “For security reasons do you…” (1) avoid online banking, (2) avoid online shopping, (3) use only one computer, (4) use e-mail spam filter, (5) change security settings, (6) use different passwords for different sites, (7) avoid opening emails from people you do not know, (8) visits only trusted websites, (9) has installed and updated antivirus software, (10) installed and upgraded antispyware software, (11) has installed and updated software or hardware firewall, (12) participate in public education workshops on cybercrime, or (13) visits websites aimed at public education on cybercrime. The answers to items were dichotomized (0 = no, 1 = yes) and after a factor analysis they were combined in summated scales corresponding to four types of guardianship: (a) avoiding behaviors (items 1 and 2); (b) Protective software/hardware (items 9, 10, 11); (3) Protective behaviors (items 4, 5, 6); 4) Information (items 12, 13). Additionally, a complementary question to measure the online capable guardianship was included. Therefore, participants had to rate their perception of computer skills ranged between low, medium and high.
Data analysis
Concerning the lifetime OIT victimization, given the dichotomous nature of the variable (0 = no; 1 = yes), binary logistic regression was performed comprising the individual variables but also the variables related to their routine activities.
Moreover, linear regression analysis was executed to analyze the effects of individual and routine activities variables on the dependent variables: fear of crime and risk perception of victimization. In the first model, the individual variables were included to assess their importance in the explanation of each dependent variable (fear and risk). In the second (full) model, contextual variables were added.
A total sample of 831 subjects completed the online survey. Sixty-six percent were females with a mean age of 27 years. The sociodemographic characteristics of the sample and the descriptive results of the studied variables are presented in Table 1 .
OIT victimization
Table 2 presents the results of the logistic regression when lifetime OIT victimization prevalence was regressed on online exposure, target suitability, capable guardianship, and individual variables. Although the model does not reach statistical significance ( p = 0.068) and Nagelkerk R 2 are relatively modest (0.059) four independent variables reached statistical significance. Considering online exposure, the use of credit card form of payment was significantly related to OIT victimization. Specifically, those who do not used these forms of payment had lower odds (34%) of becoming an OIT victim. In the same direction, the use of paysafecard as form of payment was also significantly ( p = 0.05) related to OIT victimization, suggesting that those who do not use this form of on-line payment had lower odds (59%) of becoming victim. None of the other forms of payment and routine activities (financial, work, and leisure) predicted OIT victimization. In what concerns the variables related to target suitability, only one was significantly related to OIT victimization—visit risky contents ( B = 0.283, OR 1.328)—i.e. those who reported to visit risky contents present higher odds (33%) of becoming an OIT victim. None of the studied variables related to capable guardianship were associated with OIT victimization. Considering the individual variables, gender was significantly associated with OIT victimization, with males being less likely (35%) than females of suffering an OIT victimization. Age, perceived socioeconomic status and education were not related to OIT victimization (Table 2 ).
Fear and risk of OIT
To test the correlates of fear of OIT and risk perception of being a victim of OIT, hierarchical regression analysis was performed (Table 3 ). For each dependent variable, two models were tested. The first model includes the individual variables (gender, age, SES, education, OIT victimization and general fear of crime) to control the effects of these variables on the dependent variables. In the second model (full model) the routine activities variables were added (online exposure, target suitability and capable guardianship variables).
In what concerns fear of OIT, the first model explains 10.8% of the variance. Concretely, gender, SES and general fear of crime are significant predictors of fear of OIT. Thus, females ( B = 0.186), and individuals with low SES ( B = − 0.142) present higher levels of fear of OIT. Interestingly, subjects who report more fear of crime in general also reported more fear of OIT ( B = 0.257). The model II explains 16.4% of the variance. Interaction with strangers, avoiding behaviors and computer skills were the variables significantly associated with fear of OIT. Concretely, subjects who reported more interaction with strangers are less fearful ( B = − 0.176), and those who reported to adopt more avoiding behaviors reported higher levels of fear of OIT ( B = 0.145). Finally subjects with higher computer skills are less fearful ( B = − 114). In the full model the effect of the individual variables is not affected substantially with exception of gender which do not reaches statistical significance but maintains the same direction of the association ( B = 0.105, p = 0.086), and the levels of education which reaches significance ( B = 0.084) with fear of OIT.
Regarding perceived risk of OIT victimization, the first model explains 5.3% of the variance. Concretely, gender ( B = 0.107), age ( B = 0.009), and educational levels ( B = 0.009) are positively associated with perceived risk. Moreover, SES is negatively associated with risk perception ( B = − 0.158). Thus, females, older subjects, those with higher levels of education and low SES perceived more risk of being victims of OIT. The full model (model II) explains 8.6% of the variance. It is observed that financial routines ( B = 0.077), open dubious links ( B = 0.091), and avoiding behaviors ( B = 0.080) are positively related with perceived risk. Inversely, computer skills are negatively correlated ( B = − 0.131) with perceived risk. In the full model the effect of the individual variables is not affected substantially with exception of gender which loses the statistical significance.
The current study tested the risk factors or victimization, fear and perceived risk of online identity theft. Concretely, it tried to uncover how RAT and individual variables were important to the explanation of the above referred dependent variables. Using a sample of 831 college students and staff of the University of Porto, the results suggest that RAT can be partially applied, however, other predictors were stronger in explaining these dependent variables.
Concerning the first dependent variable of the present study—victimization—, it was possible to observe a prevalence of 20% of individuals who reported to be a victim of OIT. Taken into consideration that greater exposure to online activities could be associated with higher likelihood of OIT victimization, it could be expected that individuals who spend more time online would be more victimized. In the present study, we found that time spent online does not appear to impact the risk of being victim of OIT. Nevertheless, this result follows prior studies in different cybercrime victimizations (e.g., Ngo and Paternoster 2011 ; Reyns and Henson 2015 ; Holt and Bossler 2013 ; Ngo et al. 2020 ).
Although the model did not reach statistical significance, it was found that two forms of payment (credit card and paysafecard), reflecting online exposure, and one type of suitable target (visit risky contents) were related to victimization of OIT. Furthermore, none of the measures of capable guardianship explained victimization. Concretely, it was observed that those who did not use credit card as a form of payment had lower odds (34%) of becoming an OIT victim. This result shows that not using credit card might be seen as a protective factor and is consistent with the RAT since individuals who pay on-line and use this type of payment need to insert their credit card details. Therefore, they are more exposed to potential offenders who wish to illegally obtain financial information. Besides the implication of the need to use more secure forms of online payments (e.g., PayPal), this result is especially important since the COVID-19 pandemic had necessarily increased internet usage and online purchasing. Consequently, if no security measures are adopted, these shifts in online behaviors might be exploited by fraudsters and increase victimizations levels. Even though the data from this study was collected before the pandemic, multiple reports and studies showed that cybercrime increased during and after COVID-19 pandemic. Therefore, in future studies it would be relevant to understand the development of routine activities adopted by individuals and how these are influencing the levels of cybervictimization.
Contrary to prior studies such as Reyns and Henson ( 2015 ) and Burnes et al. ( 2020 ), banking and purchasing online did not contribute to an increased risk of OIT. When analyzing differences between men and women, a few explanations can be presented to understand this result. Firstly, men reported higher mean levels of financial routines ( X = 2.61) than women ( X = 2.32, p < 0.001). Moreover, men search for more information to protect themselves online and present higher perception of technical skills ( X = 2.21) than females ( X = 1.77, p < 0.001). Therefore, the fact they have more confident in their online skills might influence the relationship between financial activities and OIT victimization. Further research needs better address the relationship between this type of exposure, gender and increased likelihood of victimization of OIT.
Next, one dimension of target suitability in this study, namely visiting risky contents, had an impact in the increased likelihood of victimization. This outcome was expressive since the composite measure including ‘clicked on a pop-up message’ and ‘visited risky websites’ amplified the likelihood of becoming a victim of OIT in 34%. Therefore, risky behaviors such as visiting not very well-known websites might increase the likelihood of OIT victimization. Results concerning the impact of target suitability have been produced mixed-results. For instance, while Leukfeldt and Yar ( 2016 ) found that only one measure of online activity (targeted browsing) had an effect on OIT victimization, Ngo and Paternoster ( 2011 ) observed that click/open links decreased the likelihood of being a victim of computer virus. In Reyns and Henson’s ( 2015 ) study, only posting personal information online was significative in explaining OIT. Nevertheless, our finding shows that increasing technical skills and sensitization on the type of websites visited by individuals might be important to avoid OIT victimization.
Concerning the impact of sociodemographic variables, the only relevant variable was gender. Interestingly, and contrary to what was expecting, males were less likely (36%) than females of being victim of OIT. In fact, this results it at odds with what was found by Holt and Turner ( 2012 ) and Reyns ( 2013 ) which observed higher levels of victimization in males. In turn, Anderson ( 2006 ) observed that females had a 20% higher likelihood of being a victim of OIT and 50% of being a victim of other frauds when comparing to men. One possible explanation for our result is the fact that the higher perception of technical skills reported by men contributed to the decreased possibility of being victimized comparing to women. However, as it was previous mentioned, since the model did not reach statistical significance, it is necessary to analyze the data with precaution. In future studies, it would be important to include other factors that might contribute to the explanation of OIT victimization. For instance, one of the most relevant risk factors that may impact identity theft is the online deviance (Ngo and Paternoster 2011 ; Holt and Turner 2012 ). Previous studies showed that individuals who reported either malicious software infections (Boss and Holt 2009 ) or harassment (Holt and Bossler 2009 ) also reported participation in online deviance. A great deal of research is needed to expand our understanding of RAT, cybercrime and OIT victimization.
We now turn our attention to the results concerning fear and risk of OIT. Although online victimization has been extendedly tested, the same has not been observed in fear and risk perception of OIT. Research has been showing that higher levels of risk perception decrease the likelihood of online purchase intentions (Reisig et al. 2009 ). Moreover, fear of OIT is decreasing consumer trust and confidence in using Internet to conduct business (Roberts et al. 2013 ). Our results attribute partially support to RAT but contribute with novel insights to the understanding of fear and risk perception of OIT.
First, fear was not explained by previous victimization of OIT, confirming the existence of mixed results concerning the relationship between fear and victimization. Instead, and in accordance with Roberts et al. ( 2013 ) the strongest predictor of fear of OIT was general fear of crime. This finding means that individuals who score higher on general fear of crime will present greater fear online. One can argue that dispositional factors such as general fear might be more important than daily internet routines to the understanding of fear of OIT. Studying the relationship between fear of crime, dispositional fear and personality dimensions, Guedes et al. ( 2018 ) found a positive correlation between dispositional fear and fear of crime. This result is in accordance with Gabriel and Greve’s ( 2003 ) theoretical work who argued that fear of concrete situations is related with a more general tendency of experiencing fear. Interestingly, general fear of crime in our sample did not explain risk perception of OIT, suggesting that fear and risk are two different dimensions. Accordingly, while fear of crime is emotional in nature, risk perception is a cognition. In fact, fear of crime is not a perception of the environment, but instead an emotional reaction to the perceived environment (Warr 2000 ), involving a set of different emotions towards the possibility of victimization (Jackson and Gouseti 2012 ). On the other hand, perceived risk is usually conceptualized as a cognitive judgment or an estimate of the risk of victimization (e.g., Ferraro and LaGrange 1987 ). Even though the emotional fear of crime and perceived risk might be highly correlated (e.g., Mesch 2000 ), different predictors explain each type of the dimensions (e.g., Rountree and Land 1996 ; Guedes et al. 2018 ). Therefore, the current results might shed some light to the debate of the multidimensionality of fear of crime.
The second important result is related to the applicability of RAT in the explanation of both fear and risk perception. Concerning fear of OIT, none of the online exposure dimensions of RAT explained fear, as in Henson et al. ( 2013 ). In turn, subjects who reported more interactions with strangers were less fearful. This result can be explained by the fact that interacting with strangers, reflecting the target suitability, might be a routine of individuals who perceive less risk of victimization which, in turn, is positively correlated with fear. Given this intriguing result, more research is needed to understand the impact of target suitability on fear of OIT. Other finding was that those who reported to adopt more avoiding behaviors presented higher levels of fear. Concretely, avoiding online banking and shopping online were predictors of fear of OIT. It is plausible to affirm that these online guardianship dimensions are also proxy measures of the behavioral dimension of fear of crime which, in turn, is generally related either to emotional and cognitive dimensions of the fear of crime in a larger sense. Liska et al. ( 1988 ) observed that fear and constrained social behavior were part of a positive escalating loop, where fear constrained social behavior which, in turn, increased fear. It would be interesting to apply the same model to fear of OIT. Furthermore, as in Virtanen ( 2017 ), we found that subjects with higher computer skills were less fearful and perceived lower risk of victimization. According to Jackson ( 2004 ), worry about crime is related to the seriousness of the consequences of victimization, the likelihood of the event occurring and the ability to control its occurrence. Therefore, one can argue that augmented levels of perception of technical skills might increase the feelings of control over the risk of being victim of OIT. The confidence in individual’s own technical skills was also a predictor of risk perception of OIT, showing the importance of this variable in the present study. Under the applicability of RAT to perceived risk of victimization, it is also important to note that different predictors, comparing to fear, explained risk perception. In fact, one measure of online exposure (financial activities) is positively related to higher levels of perceived risk of victimization. Moreover, open dubious links increase perceived risk of OIT but not the fear of this type of crime. Therefore, these results shed light to the importance of implementing preventive actions and increased knowledge of the risks of online activities since, as it was previously mentioned, higher risk perception of OIT is related to lower levels of online purchase intentions.
Lastly, the present study also intended to analyze the impact of sociodemographic variables on both fear and risk perception of victimization online. Generally, the best predictor of fear of crime offline is gender (Hale 1996 ). However, in the final model of the present study, gender lost its significance in the explanation of fear of OIT. This result is in accordance with others such as Yu ( 2014 ), who observed no statistical significative differences between women and men for OIT, fraud and virus. Moreover, gender was not a predictor of fear of OIT in Roberts et al. ( 2013 ) research. Instead, our findings suggest that education and socioeconomic status are predictors not only of fear but also of risk of OIT. While more educated individuals presented higher levels of fear and risk perception, as in accordance with Akdemir ( 2020 ), lower socioeconomic status predicted both fear and risk of OIT, as in Virtanen ( 2017 ) and Brands and Wilsem ( 2019 ). This result suggests that those individuals are most affected by property-focused victimization and predict more difficulties in dealing with potential costs of that victimization.
Finally, it is important to acknowledge the limitations of the present study. Our data stems from a convenience sample of university population, that includes students and staff of the same educational environment, not allowing generalization to a larger population. While a more diverse and representative population would permit a more diversified experiences and contexts, the positive aspect is that they are a population that uses computer and internet daily. Moreover, previous studies have also utilized samples of college students (e.g., Bossler and Holt 2009 ; Holt and Bossler 2009 ).
Future research can expand to other types of online victimization and increase the list of online activities with the objective of identifying common and specific risk/protective factors associated with cybervictimization. Since in our study individual computer skills were an important predictor of fear and risk of OIT, we suggest a deeper exploration of this variable as a mediator between online exposure and victimization. Finally, qualitative studies on this topic will be very useful to explore the (in)security experiences of the individuals, their online routines, and subjective perception of the risks to which they are exposed and in what activities and contexts. Another important limitation of the present study is the fact that it only relies on individual online activities to understand the victimization of online identity theft. In future studies it would be important to measure if the identity theft victimization occurred due to a data breach which put in risk an individual’s personal/financial information stored by a company or a government. For instance, Burnes et al. ( 2020 ) showed that individuals reporting breached personal information from a company or government were more likely to experience multiple forms of identity theft.
Given the costs associated with cybercrime, and the increasing use and importance of the internet in our daily lives, the identification of risk and protective factors for cybervictimization and fear of cybercrime will be very relevant for prevention strategies.
Anderson, K.B. 2006. Who are the victims of identity theft? The effect of demographics. Journal of Public Policy and Marketing 25 (2): 160–171.
Article Google Scholar
Abdulai, M. 2020. Examining the effect of victimization experience on fear of cybercrime: University student’s experience of credit/debit card fraud. International Journal of Cyber Criminology 14 (1): 157–1754.
Google Scholar
Akdemir, N. 2020. Examining the impact of fear of cybercrime on internet users’ behavioral adaptations, privacy calculus and security intentions. International Journal of Eurasia Social Sciences 11 (40): 606–648.
Alshalan, A. 2006. Cyber-crime fear and victimization: An analysis of a national survey . Mississippi: Mississippi State University.
Bellah, J. 2001. Training: Identity theft. Law and Order 49 (10): 222–226.
Bossler, A.M., and T.J. Holt. 2009. On-line activities, guardianship, and malware infection: An examination of routine activities theory. International Journal of Cyber Criminology 3 (1): 974–2891.
Brands & Wilsem. 2019. Connected and fearful? Exploring fear of online financial crime, Internet behaviour and their relationship. European Journal of Criminology 00: 1–12.
Burnes, D., M. DeLiema, and L. Langton. 2020. Risk and protective factors of identity theft victimization in the United States. Preventive Medicine Reports 17: 1–8.
Capeller, W. 2001. Not such a neat net: Some comments on virtual criminality. Social & Legal Studies 10 (2): 229–242.
Choi, K. 2008. An empirical assessment of an integrated theory of computer crime victimisation. International Journal of Cyber Criminology 2 (1): 308–333.
Cohen, L.E., and M. Felson. 1979. Social change and crime rate trends: A routine activity approach. American Sociological Review 44: 588–608.
Copes, H., and L. Vieraitis, L. 2012. identity theft. In The Oxford handbook of crime and public policy , ed. M. Tonry. https://doi.org/10.1093/oxfordhb/9780199844654.001.0001
Eck, J.E., and R.V. Clarke. 2003. classifying common police problems: A routine activity approach. Crime Prevention Studies 16: 7–39.
Ferraro, K., and R. LaGrange. 1987. The measurement of fear of crime. Sociological Inquiry 57 (1): 70–97.
Gabriel, U., and W. Greve. 2003. The psychology of fear of crime: Conceptual and methodological Perspectives. British Journal of Criminology 43 (1): 600–614.
Garrison, C.P., and M. Ncube. 2011. A longitudinal analysis of data breaches. Information Management & Computer Security 19 (4): 216–230.
Golladay, K., and K. Holtfreter. 2017. The consequences of identity theft victimization: An examination of emotional and physical health outcomes. Victims & Offenders 12 (5): 741–760.
Grabosky, P. 2001. Virtual criminality: Old wine in new bottles? Social & Legal Studies 10 (2): 243–249.
Guedes, I., S. Domingos, and C. Cardoso. 2018. Fear of crime, personality and trait emotions: An empirical study. European Journal of Criminology 15 (6): 658–679.
Hale, C. 1996. Fear of crime: A review of the literature. International Review of Victimology 4: 79–150.
Harrell, E., and L. Langton. 2013. Victims of identity theft, 2012 (NCJ 243779) . Washington: Bureau of Justice Statistics.
Harrell, E. 2015. Victims of identity theft , 2014, Bureau of Justice Statistics, NCJ 248991.
Harrell, E. 2019. Victims of identity theft , 2016: Bulletin.
Henson, B., B.W. Reyns, and B.S. Fisher. 2013. Fear of crime online? Examining the effect of risk, previous victimization, and exposure on fear of online interpersonal victimization. Journal of Contemporary Criminal Justice 29 (4): 475–497.
Hille, P., G. Walsh, and M. Cleveland. 2015. Consumer fear of online identity theft: Scale development and validation. Journal of Interactive Marketing 30: 1–19.
Holt, T.J., and A.M. Bossler. 2009. Examining the applicability of lifestyle-routine activities theory for cybercrime victimization. Deviant Behavior 30 (1): 1–25.
Holt, T.J., and A.M. Bossler. 2013. Examining the relationship between routine activities and malware infection indicators. Journal of Contemporary Criminal Justice 29 (4): 420–436.
Holt, T.J., and M. Turner. 2012. Examining risks and protective factors of on-line identity theft. Deviant Behavior 33 (4): 308–323.
Holt, T.J., J. van Wilsem, S. van de Weijer, and R. Leukfeldt. 2020. Testing an integrated self-control and routine activities framework to examine malware infection victimization. Social Science Computer Review 38 (2): 187–206.
Jackson, J. 2004. Experience and expression: Social and cultural significance in the fear of crime. British Journal of Criminology 44 (6): 946–966.
Jackson, J., and I. Gouseti (eds). 2012. Fear of crime. In The encyclopedia of theoretical criminology . Hoboken, NJ: Wiley-Blackwell.
Jordan, G., R. Leskovar, and Marič M. 2018. Impact of fear of identity theft and perceived risk on online purchase intention. Organizacija 51 (2): 146–155.
Keane, C. 1992. Fear of crime in Canada: An examination of concrete and formeless fear of victimization. Canadian Journal of Criminology 34 (2): 215–224.
Koops, B.J., and R.E. Leenes. 2006. ID theft, ID fraud and/or ID-related crime-definitions matter. Datenschutz Und Datensicherheit 30 (9): 553–556.
Lee, S., K. Choi, S. Choi, and E. Englander. 2019. A test of structural model for fear of crime in social networking sites. International Journal of Cybersecurity Intelligence Cybercrime 2 (2): 5–22.
Leukfeldt, E.R., and M. Yar. 2016. Applying routine activity theory to cybercrime: A theoretical and empirical analysis. Deviant Behavior 37 (3): 263–280.
Li, Y., A. Yazdanmehr, J. Wang, and H.R. Rao. 2019. Responding to identity theft: A victimization perspective. Decision Support Systems 121: 13–24.
Liska, A.E., A. Sanchirico, and M.D. Reed. 1988. Fear of crime and constrained behavior: Specifying and estimating a reciprocal effects model. Social Forces 66 (3): 827–837.
Marcum, C., G. Higgins, and M. Ricketts. 2010. Potential factors of online victimization of youth: An examination of adolescent online behaviors utilizing routine activity theory. Deviant Behavior 31 (5): 381–410.
Martin, K.D., A. Borah, and R.W. Palmatier. 2017. Data privacy: Effects on customer and firm performance. Journal of Marketing 81 (1): 36–58.
Mesch, G.S. 2000. Perceptions of risk, lifestyle activities, and fear of crime. Deviant Behavior 21 (1): 47–62.
McNeeley, S. 2015. Lifestyle-routine activities and crime events. Journal of Contemporary Criminal Justice 31 (1): 30–52.
Ngo, F., and R. Paternoster. 2011. Cybercrime victimization: An examination of individual and situational level factors. International Journal of Cyber Criminology 5 (1): 773–793.
Ngo, F., A. Piquero, J. LaPrade, and B. Duong. 2020. Victimization in cyberspace: Is it how long we spend online, what we do online, or what we post online? Criminal Justice Review 45 (4): 430–451.
Piquero, N.L. 2018. White-collar crime is crime: Victims hurt just the same. Criminology & Pub. Pol’y 17: 595.
Pratt, T.C., K. Holtfreter, and M.D. Reisig. 2010. Routine online activity and Internet fraud targeting: Extending the generality of routine activity theory. Journal of Research in Crime and Delinquency 47: 267–296.
Rader, N., D. May, and S. Goodrum. 2007. An empirical assessment of the ‘threat of victimization’: Considering fear of crime, perceived risk, avoidance, and defensive behaviors. Sociological Spectrum: MId-Shouth Sociological Association 27 (5): 475–505.
Randa, R. 2013. The influence of the cyber-social environment on fear of victimization: Cyber bullying and school. Security Journal 26: 331–348.
Reid, L.W., and M. Konrad. 2004. The gender gap in fear of crime: Assessing the interactive effects of gender and perceived risk on fear of crime. Sociological Spectrum 24 (4): 399–425.
Reisig, M.D., T.C. Pratt, and K. Holtfreter. 2009. Perceived risk of internet theft victimisation: Examining the effects of social vulnerability and financial impulsivity. Criminal Justice and Behavior 36 (4): 369–384.
Reyns, B.W. 2013. Online routines and identity theft victimization: Further expanding routine activity theory beyond direct-contact offenses. Journal of Research in Crime and Delinquency 50 (2): 216–238.
Reyns, B.W. 2015. A routine activity perspective on online victimisation: Results from the Canadian General Social Survey. Journal of Financial Crime 22 (4): 396–411.
Reyns, B.W., and B. Henson. 2015. The thief with a thousand faces and the victim with none: Identifying determinants for online identity theft victimization with routine activity theory. International Journal of Offender Therapy and Comparative Criminology 60 (10): 1119–1139.
Reyns, B.W., B. Henson, and B.S. Fisher. 2011. Being pursued online: Applying cyberlifestyle–routine activities theory to cyberstalking victimization. Criminal Justice and Behavior 38: 1149–1169.
Reyns, B.W., and R. Randa. 2017. Victim reporting behaviors following identity theft victimization: Results from the National Crime Victimization Survey. Crime & Delinquency 63 (7): 814–838.
Roberts, L.D., D. Indermaur, and C. Spiranovic. 2013. Fear of cyber-identity theft and related fraudulent activity. Psychiatry, Psychology, & Law 20: 315–328.
Rountree, W., and K. Land. 1996. Perceived risk versus fear of crime: Empirical evidence of conceptually distinct reactions in survey data. Social Forces 74 (4): 1353–1376.
Skogan, W., and M. Maxfield. 1981. Coping with crime: Individual and neighborhood reactions . Beverly Hills: Sage.
Smith, L.N., and G.D. Hill. 1991. Perceptions of crime seriousness and fear of crime. Sociological Focus 24 (4): 315–327.
Tatham, M. 2018. “Identity Theft Statistics.” Experian. https://www.experian.com/blogs/ask-experian/identity-theft-statistics/ .
Tseloni, A., and C. Zarafonitou. 2008. Fear of crime and victimization: A multivariate analyses of competing measurements. European Journal of Criminology 5 (4): 387–409.
Van Wilsem, J. 2013. Hacking and harassment—Do they have something in common? Comparing risk factors for online victimization. Journal of Contemporary Criminal Justice 29 (4): 437–453.
Virtanen, S. 2017. Fear of cybercrime in Europe: Examining the effects of victimization and vulnerabilities. Psychiatry, Psychology and Law 24 (3): 323–338.
Wang, W., Y. Yuan, and N. Archer. 2006. A Contextual framework for combating identity theft. IEEE Security and Privacy 4 (2): 30–38.
Warr, M. 1984. Fear of victimization: Why are women and the elderly more afraid? Social Science Quarterly 65 (6): 81–702.
Warr, M. 2000. Fear of crime in the United States: Avenues for research and policy. Measurement and Analysis of Crime and Justice 4: 451–489.
Williams, M. 2016. Guardians upon high: An application of routine activities theory to online identity theft in Europe at the country and individual level. British Journal of Criminology 56: 21–48.
Yar, M. 2005. The Novelty of ‘Cybercrime’: An Assessment in Light of Routine Activity Theory. European Journal of Criminology 2 (4): 407–427.
Yu, S. 2014. Fear of cybercrime among college students in the United States: An exploratory study. International Journal of Cyber Criminology 8 (1): 36.
Ziegler, R., and D. Mitchell. 2003. Aging and fear of crime: An experimental approach to an apparent paradox. Experimental Aging Research 29 (2): 173–187.
Download references
Author information
Authors and affiliations.
School of Criminology, Faculty of Law, University of Porto, Porto, Portugal
Inês Guedes, Margarida Martins & Carla Sofia Cardoso
You can also search for this author in PubMed Google Scholar
Corresponding author
Correspondence to Inês Guedes .
Ethics declarations
Conflict of interest.
On behalf of all authors, the corresponding author states that there is no conflict of interest.
Additional information
Publisher's note.
Springer Nature remains neutral with regard to jurisdictional claims in published maps and institutional affiliations.
Rights and permissions
Reprints and permissions
About this article
Guedes, I., Martins, M. & Cardoso, C.S. Exploring the determinants of victimization and fear of online identity theft: an empirical study. Secur J 36 , 472–497 (2023). https://doi.org/10.1057/s41284-022-00350-5
Download citation
Accepted : 01 July 2022
Published : 21 July 2022
Issue Date : September 2023
DOI : https://doi.org/10.1057/s41284-022-00350-5
Share this article
Anyone you share the following link with will be able to read this content:
Sorry, a shareable link is not currently available for this article.
Provided by the Springer Nature SharedIt content-sharing initiative
- Victimization
- Fear of crime
- Routine Activity Theory
- Find a journal
- Publish with us
- Track your research
Ecommerce Fraud Prevention: The 7 Worst Scams and How to Stop Them (10 Prevention Tools)
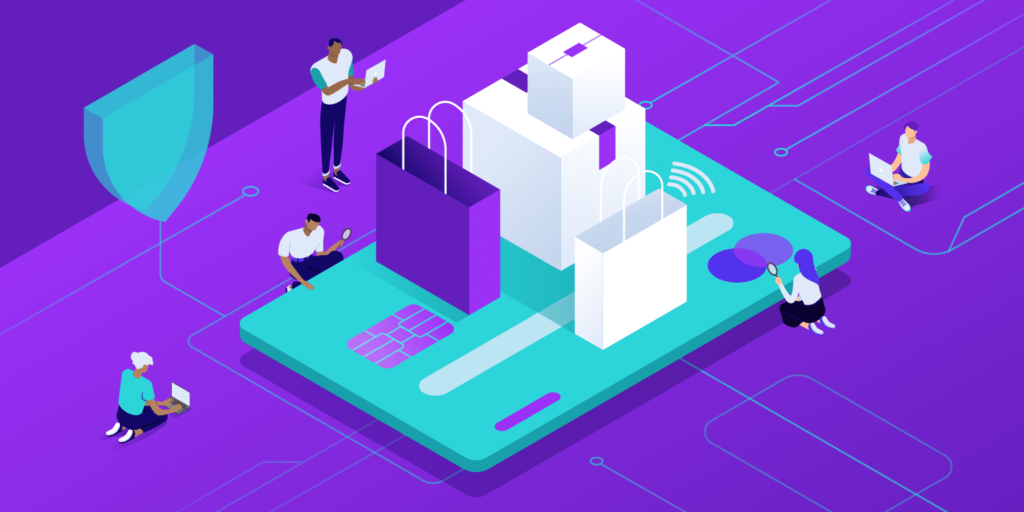
Your suspicions are correct: ecommerce fraud is on the rise. A nearly 30% rise, to put an exact number on it, according to a year-over-year study from LexisNexis Risk Solutions . Even more troubling: that figure is almost twice the growth of ecommerce sales, based on data from Digital Commerce 360 .
To make matters worse, ecommerce merchants have to fight fraud on two different fronts. For one, you have to protect yourselves from schemes targeting merchants specifically, like fraudulent chargebacks or site mimicking (see below).
But on top of that, you also have to protect your customers from scams as well. Harboring scammers on your site is a kiss of death for online stores , even if you’re as much of a victim as your shoppers.
Luckily, ecommerce fraud prevention is also on the rise, with methods just as sophisticated as the scams they thwart. In this article, we outline the 7 worst and most common fraud scams for ecommerce: how they work, what warning signs to watch out for, your best defenses, and 10 ecommerce fraud prevention tools to optimize security.
Let’s get started!
7 Worst Ecommerce Fraud Scams to Watch For
Your first defense against ecommerce fraud is simply knowing what to look out for. Here are the 7 most common scams online stores are vulnerable to.
1. Email Account Phishing
Most people are familiar with email phishing scams , so let’s start there. These are as old as the internet itself, so many of you have likely already opened an email from a stranger asking for sensitive account information.
However, lately we’re seeing an increase in scammers posing not as Nigerian princes, but as ecommerce stores . They send emails disguised as order/delivery confirmations, aimed at either extracting sensitive account data or leading victims to a fraudulent site.
In the best-case scenarios, these lead to an unexpected advertisement page rather than an official store page. Other times, it’s not so harmless; links in phishing emails often go to trap sites with viruses, malware, or other hacking-related misfortunes . That’s why it’s always recommended to hover over suspicious links rather than click them.
2. Identity Theft
If some poor soul loses their account information to a phishing email, what happens next? The scammer takes that info and buys a bunch of expensive gifts for themselves, and guess who pays the bill. Posing as someone else and making purchases with their financial information is known as identity theft.
Strange as it sounds, the retailer is often the one hurt most by identity theft : a credit card company usually initiate chargebacks on behalf of the victim, but with no obligation to return the merchandise. Even if the retailer manages to reclaim products, they’re no longer new. The only way for a retailer to escape identity theft unscathed is to stop it before it starts.
It’s also worth mentioning that online stores also need to watch out for becoming unwitting accomplices in identity theft. If your site is not secure , hackers can steal your customers’ information from right under your nose — as was the million-dollar case in the 2013 Target hacks .
3. Pagejacking
You’re on a site you’ve used hundreds of times before, but this time, on this particular page, something just seems… off. It could be that the site had one of their pages jacked. Pagejacking is when hackers create a fraudulent web page that mimics an existing site.
More advanced cases involve pagejacking a high-ranking site and syphoning off its search engine traffic. Pagejacking is also commonly associated with “mousetrapping,” in which a page prevents users from exiting, for example, by opening a new window every time the user tries to close the browser or flooding their computer with endless pop-ups.
But as far as ecommerce is concerned, pagejacking is another effective technique for phishing, such as mimicking a site’s login page to collect usernames and passwords. The last thing an ecommerce brand wants is their customers second-guessing their legitimacy every time they log in.
4. Chargeback Fraud
Chargeback fraud is painfully simple and woefully common. Basically, the scammer purchases a large ecommerce order and then cancels the payment after it’s shipped. They keep the merchandise when it arrives without paying a cent.
The methods vary, although it can be as easy as the scammer calling the credit card company themselves and saying they had their identity stolen.
Another popular technique is claiming the delivery never arrived so the scammer receives a duplicate order for free. Even if the scam is caught in time, even in the best situations, the merchant still has to investigate false claims.
To make matters worse merchants have to differentiate “friendly fraud” from actual chargeback fraud.
Friendly fraud is when a legitimate customer accidentally causes a chargeback fraud, such as missing a package delivery or entering the wrong payment details. Merchants are stuck in the dark about whether a chargeback had malicious intentions or was just an accident, with fear of offending a well-intentioned customer with accusations of fraud.
Ecommerce brands that operate under a subscription model deal with friendly fraud often, as customers claim they didn’t know the charges were recurring. It’s best for subscription brands to make charges clear and obvious before customers sign up.
5. Triangulation Fraud
Let’s move into more advanced fraud schemes, reserved for more clever and experienced con artists. To explain how triangulation fraud works, let’s break it down into steps.
- The scammer creates a fake listing for a real product with a significant price markup. This isn’t always so fraudulent, either; sites like eBay allow users to post and sell items without verification.
- A customer “buys” the product from the fake listing, giving the scammer all their personal data.
- The scammer takes the customer’s data and buys that same item for them at a different site for less. They have the item shipped to the customer.
- The customer receives the item they bought, without realizing they overpaid. The scammer keeps the markup profit.
One of the most devious parts of this scam is that the victims don’t even necessarily know they’ve been scammed .
Additionally, successful triangulation fraudsters accumulate long lists of account data and credit card numbers. More often than not, they use different credit cards for step 3 to throw off their scent.
That means the victim of triangulation fraud could have their data used again in an unrelated scam months or years later.
6. Affiliate Fraud
Specifically targeting ecommerce merchants with affiliate programs , affiliate fraud refers to scammers manipulating or abusing affiliate links to get a greater payoff. In other words, if an affiliate gets paid for every visitor they send a site, a scammer can make it seem like they sent more visitors than they actually did, earning a bigger paycheck.
Affiliate fraud often involves hacking and automated systems, but in some cases, it can be as simple as using a stable of fake profiles. Scammers usually must have a certain level of computer skills to deftly avoid detection.
7. Supplier Identity Fraud
Last, another merchant-specific fraud scheme: the scammer poses as a manufacturer, wholesale supplier, or other B2B business , promising a service they never intend to deliver. Online stores sign up, hand over some money, but never hear from the supplier again.
These scams draw a lot on other scams like phishing and maybe even pagejacking, with the big difference being they target businesses instead of consumers. It’s one of the reasons we always recommend thoroughly researching who you’re doing business with.
Ecommerce Fraud Warning Signs: Stop Scams Before They Start
An ounce of prevention is worth a pound of cure.
The most effective method of ecommerce fraud prevention is recognizing the warning signs early enough to avoid them. Here are some red flags every online store should keep an eye on:
- Shipping and billing address are different. As if often the case for identity theft and triangulation fraud, the owner of the card is not receiving the goods.
- Multiple orders on the same item. Ecommerce fraudsters tend to target high-ticket items, and when they find one they like, they’ll use it over and over. Often the goods are fenced anyway, so it’s more about the monetary value than the actual product.
- Multiple orders to the same address but different cards. Overusing the same stolen card numbers brings unwanted attention and suspicion, so experienced scammers like to change it up… and it’s easier to use a different credit card number than have goods shipped to a different address (Make sure to check out our guide on how to prevent and reduce credit card fraud by 98% Using Stripe Radar ).
- Suspiciously large orders (especially expedited shipping). As with most crime, scammers want to make sure the rewards are worth the risks. That’s why ecommerce scams frequently involve large orders, in case it’s the fraudster’s last. They also want the exchanges to go through as quickly as possible before their victims catch on, hence expedited shipping.
- Suspicious email addresses or phone numbers. Identity theft is rarely foolproof — there’s usually one or two holes. Keep on the lookout for email addresses that don’t seem to add up (different names, companies posing as individual, etc.) as well as suspicious phone numbers (i.e. different country or area codes than the billing address).
- Repeated declined transactions. Having a transaction declined once or twice happens to everyone, but repeated declined transactions are a red flag. Although sometimes innocent, it can be a sign of someone trying to guess sensitive information they don’t have lawful access to.
Ecommerce Fraud Prevention: Your Ironclad Defense
Now that you know all the misfortunes that can happen to you, let’s move on to the real reason you’re here: making sure it doesn’t! Here’s the best advice on ecommerce fraud prevention and protecting your online store against scams.
PCI Compliance
First, start with the official guidelines for ecommerce fraud prevention: the Payment Card Industry Security Standards Council (PCI SSC). Basically, the biggest credit cards brands around the world got together and outlined a list of best practices for avoiding scams. Think of these as the bare minimum for ecommerce fraud prevention, and a good starting off point.
Luckily, a lot of payment gateways can handle PCI compliance for you, so you can solve the problem straightaway by choosing more secure ones. We explain how to comply to the PCI guidelines here or you can go straight to the source with the PCI SSC blog .
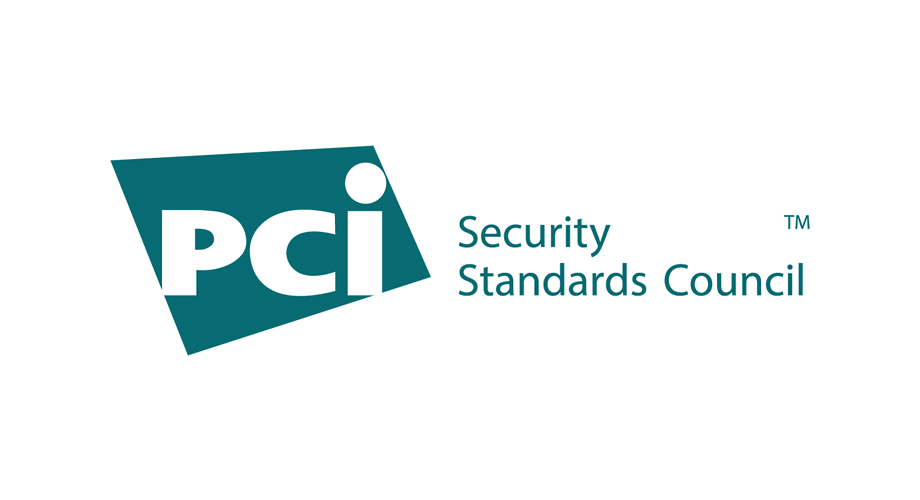
AVS and CVV
More low-hanging fruit for fighting fraud: Address Verification Services (AVS) and Card Verification Value (CVV). These standard security measures are closer to rules than recommendations.
AVS makes sure the billing address entered matches the billing address on file, while CVV requires customers to enter the three-number code on the back of the card (in case the identity thief only stole the card numbers, not the actual card).
Both of these safeguards are typically included in the payment processor, so make sure they’re present before choosing yours.
Required Signature on Delivery
With so many ecommerce scams involving fake identities, a physical signature can carry a lot of weight. Although this option may cost extra depending on your shipping, it’s a great defense against identity theft, fraudulent chargebacks, and triangulation schemes.
If scammers are trying to convince you they’re someone else, or that a delivery never arrived, a required signature corners them in their own trap.
Follow Up Personally
Scammers love lazy victims. They never look twice.
A successful con involves things falling through the cracks, so one of the most effective preventative measures is to follow up on suspicions. If you have the time to spare, a little extra attention and elbow grease can reveal exactly what the scammer doesn’t want you to see. Consider these options:
- Email the customer personally to see if their email address is authentic. Innocent customers won’t mind if you politely explain your suspicions, but scammers won’t know what to do. Pay attention to things like grammar and spelling in their responses to see if English is their second language.
- Verify the person on social media. Search for their name and/or username to see whether or not they’re real, and whether their profiles match their other information.
- Call the customer’s phone. This is the quickest route to verifying someone is who they say.
- Delay the shipment. As we’ve said, scammers want their operations completed as quickly as possible to decrease the chances of getting caught. If you delay a shipment on purpose, and tell them as much, it may scare them off. This is an inconvenience to honest shoppers, so only use it if you have to.
Of course, you don’t have the time to do all this for every order, so a good start is to develop your instincts. Learn to identify suspicious orders early, and if something seems off to you — even just a little — by all means, don’t ignore it.
Always Use HTTPS
What’s the difference between HTTP and HTTPS? In a word, encryption . HTTPS works with another protocol, Secure Sockets Layer (SSL) , to protect data as it “moves” across the internet. HTTP (no S ) does not, so HTTPS is always better for avoiding hackers. Think of it as the S stands for Security.
HTTPS has other benefits too, such as better SEO ranking and more accurate referral data. If you’re using WordPress, read our in-depth guide on how to switch from HTTP to HTTPS . And if you’re managing a WooCommerce store, here’s how to install an SSL certificate .
Safer Passwords
Last but not least, you can share the burden of security with your customers by requiring them to have safer, more elaborate passwords. Sure, no one likes those annoying password requirements, especially if they have accounts on tons of sites… but really, getting victimized by a credit card scam is a lot more inconvenient than remembering a new password.
At the moment, the industry standard is eight characters, one capital letter, and one special character. Another less than this is a risk, and for extra security, you can add more requirements like a number, or even randomly generated passwords.
Top 10 Best Tools for Ecommerce Fraud Prevention
You’re not alone in your fight against fraud, there are tons of allies if you know where to look. Here are the 10 best software for ecommerce fraud prevention.
1. Signifyd
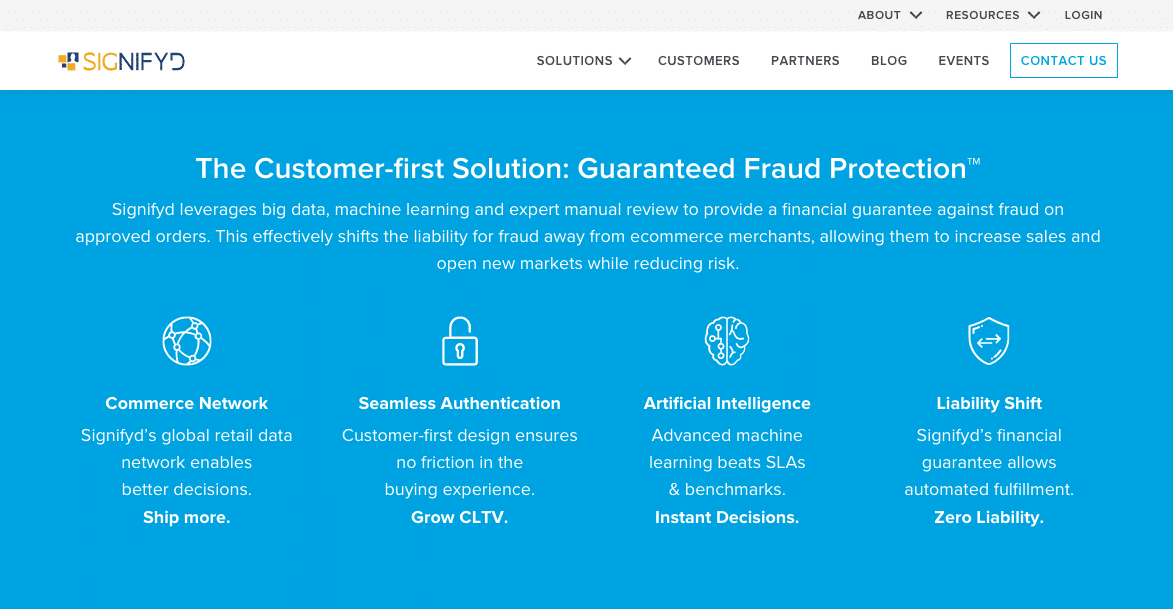
With a sliding scale to accommodate both large and small businesses, Signifyd is one of the first places to look for fraud prevention software. It runs in the backend of your store and assigns every purchase a “score” based on the likelihood that it’s fraud.
Users have the option of handling the case themselves or enlisting help from the Signifyd team. They also offer insurance on select orders, for extra peace of mind in case there’s something suspicious you can’t put your finger on.
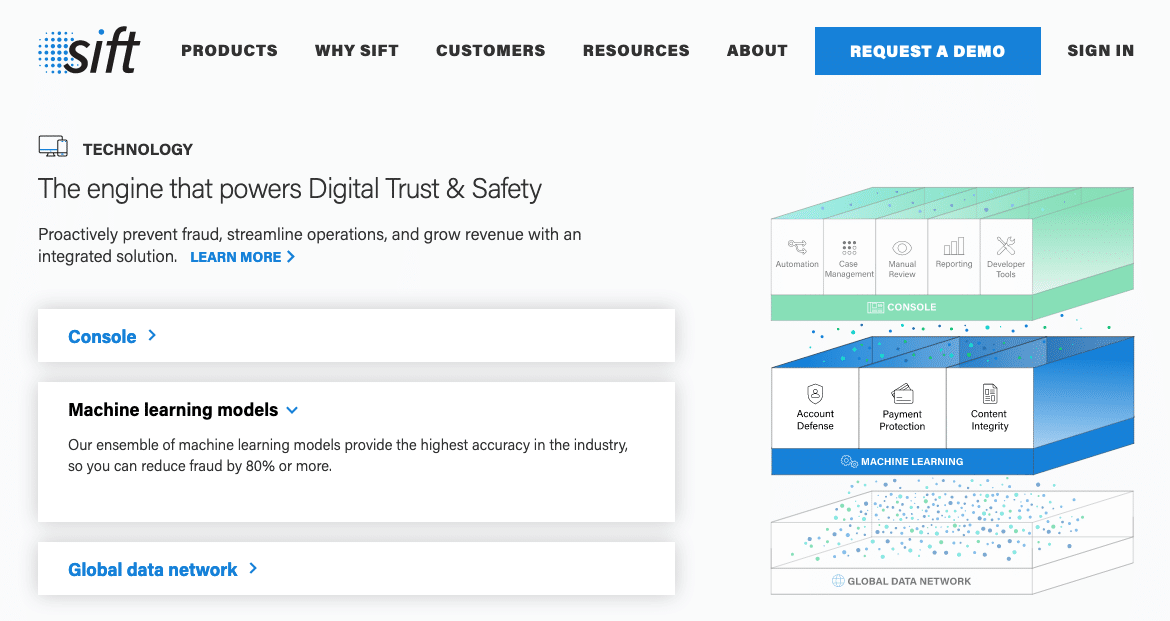
Formerly Sift Science, the fraud prevention tool Sift is aimed at higher-end stores — more features for more money. Although you can buy individual packages, the full suite offers:
- order evaluations
- fake account prevention
- account takeover prevention
- abuse of promotions prevention
- spam prevention for content
- device fingerprinting API
Sift touts its machine learning as one of the best in the industry, so perhaps that, along with the other features, justifies the price tag.
3. Simility
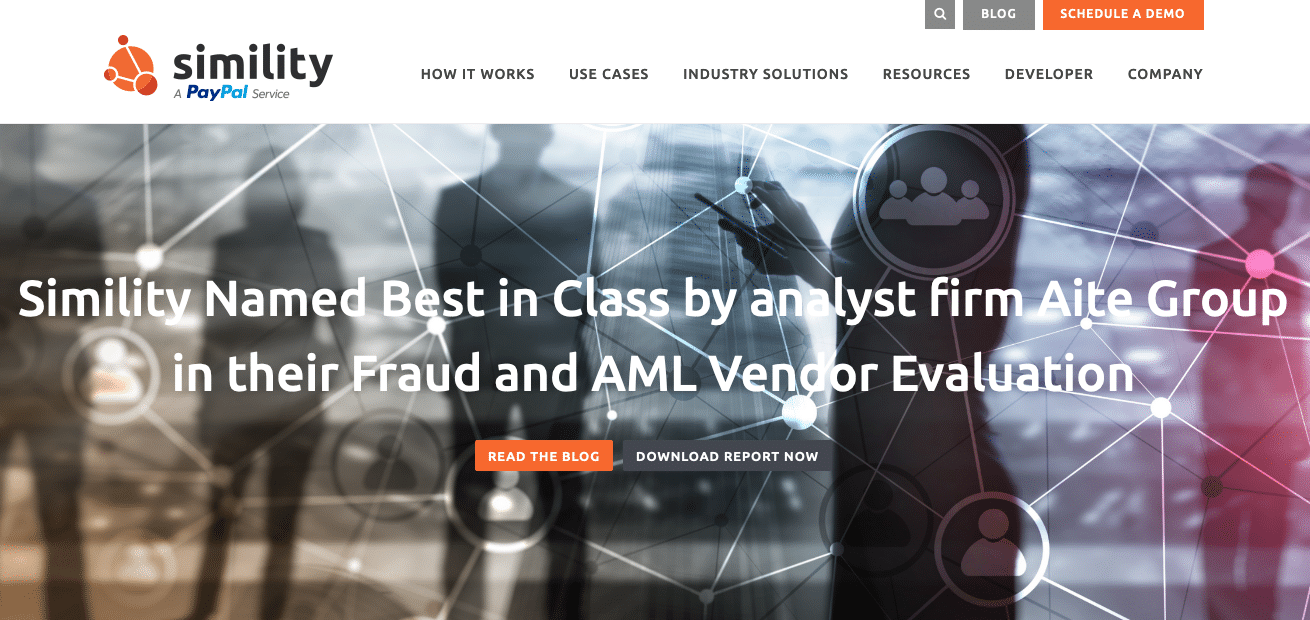
Simility specializes in “device fingerprinting,” identifying a device and evaluating its threat level. By monitoring a device’s data — location, OS, language, web browser, username, even the battery level! — Simility cross-references the device against any blacklists and determines its threat level.
4. DupZapper
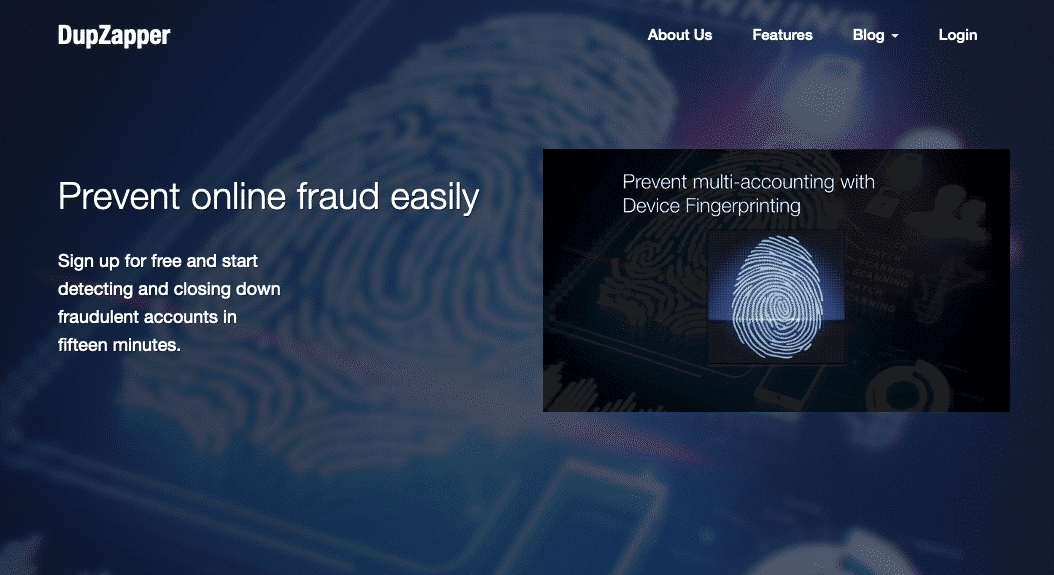
Easy to use, quick to install, and no API integration necessary, DupZapper is a low-maintenance, high-return software. Designed to regulate online gaming, their algorithm detects duplicate accounts, geography consistency, cookie-blocking, and proxy usage, among other things. If you’re looking for painless and effortless fraud prevention tools, this is our recommendation.
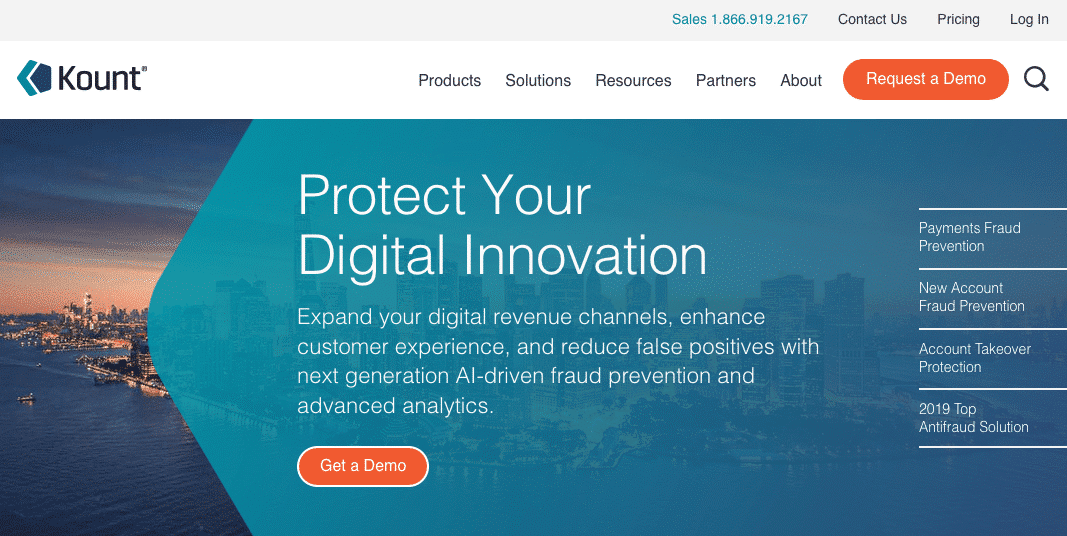
A favorite of global enterprises like Chase Bank and GNC, Kount is another high-cost, high-quality option. If you have the budget for it, Kount enlists some of the most advanced technology to access the risk of a transaction, utilizing over 200 data variables. Their system is also one of the fastest with a response time of less than a second (300 milliseconds, to be precise). For large companies with sizable budgets, that speed can be useful when going through your daily orders.
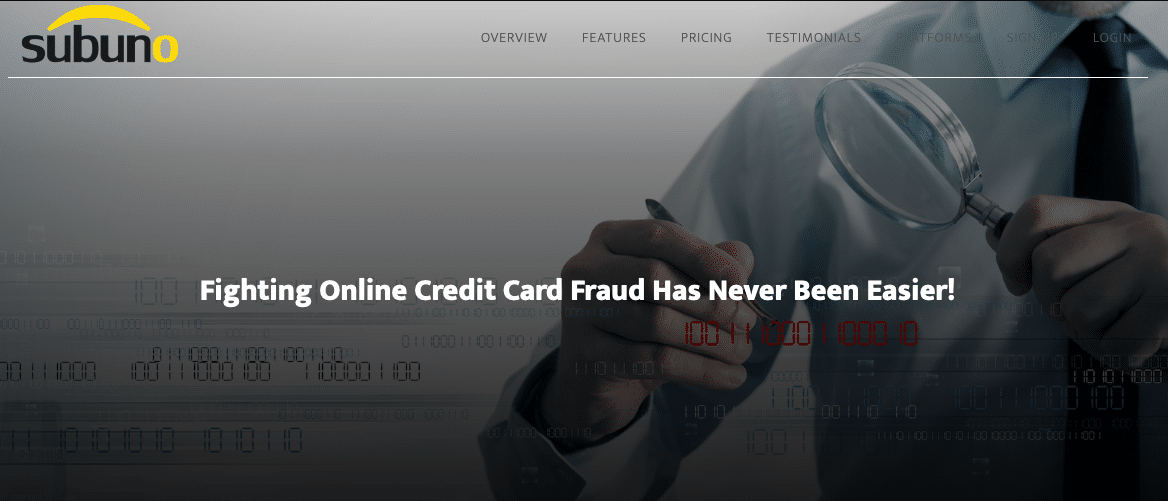
Subuno offers a lot for its low price: over 20 fraud detection tools that analyze over 100 risk factors. It’s a prime choice for those who don’t have a lot in their budget but still prioritize site safety, especially considering it works with ecommerce sites like Shopify and WooCommerce .
7. Riskified
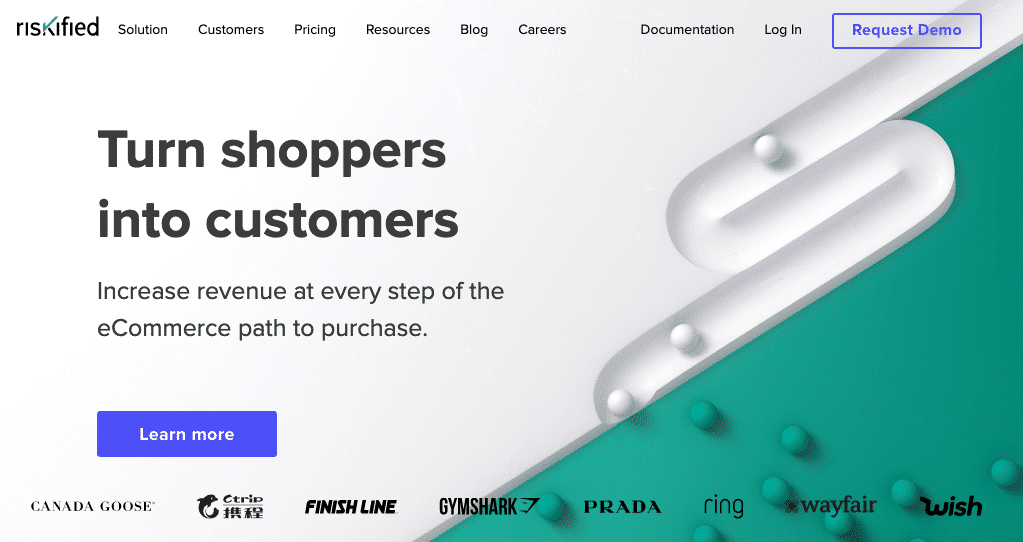
Riskified sets itself apart from other ecommerce fraud prevention software in a few ways. For starters, they use lightning-fast reports in real-time. A good choice if you want the speed of high-end software like Kount, but without the enterprise-level pricing.
Casting aside the “fraud score” model, Riskified presents a clear “approve/decline” analysis for each order. It also works on a sliding scale where you only pay for approved orders that generate sales, making a smart alternative for smaller online stores.
8. FraudLabs Pro
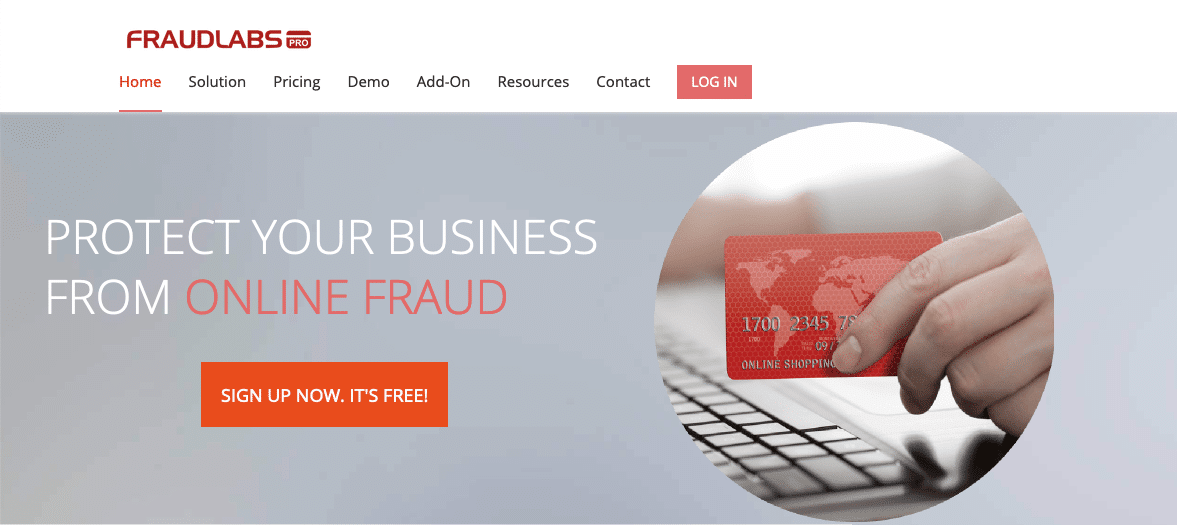
FraudLabs Pro has a couple of strong advantages over the other fraud prevention tools on this list. Namely, it uses unique detection methods, including authenticity checks for email (like email domain age), social media, ISP, and usernames.
But the other advantage is even more appealing: a viable free plan that accepts up to 500 queries per month. For small stores or brands that just launched, this is a lucky find for online security.
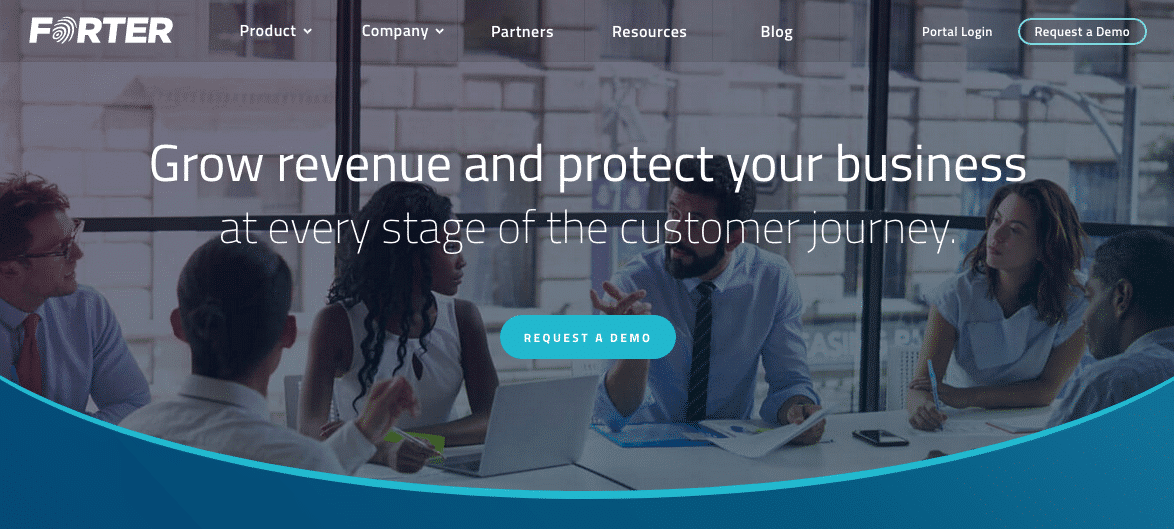
Optimized for mobile transactions, Forter offers broad, blanket coverage on almost nearly any transaction, regardless of geographic location or payment method. One of its favored features is its customization options, allowing users to hone in on specific risk profiles or payment gateways . It also uses quick, real-time reports, with a simple “yes/no” reports rather than a fraud score.
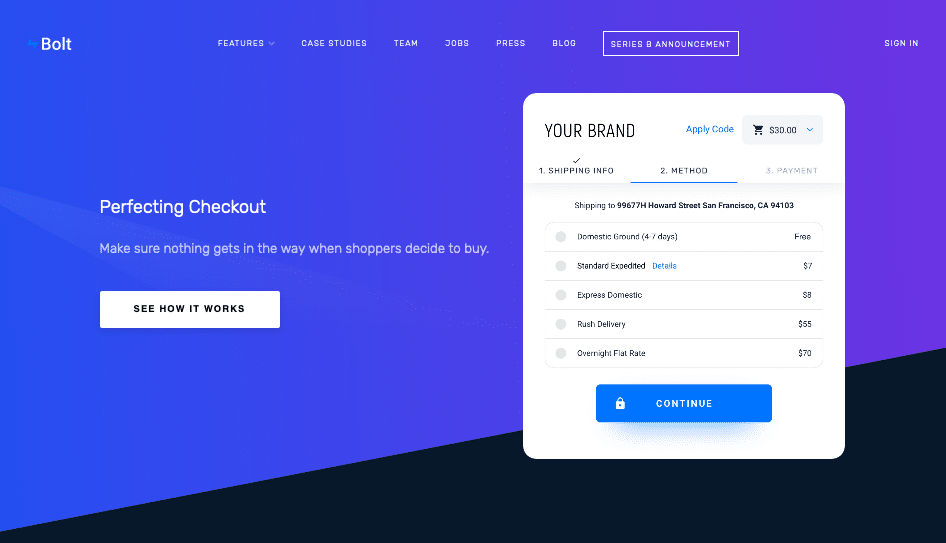
Technically speaking, Bolt is more of a checkout UI solution than a fraud prevention solution… however, because fraud prevention is built-in to its system, it qualifies as both. Bolt is an optimized checkout system for both fraud detection and user experience, aimed at increasing sales and decreasing abandonment through usability.
Bolt scans over 200 behavioral data points during the checkout to assess risks. That, combined with its usability advantages, makes it a great choice for online stores that need help in more areas than just online security.
Your fraud prevention safety measures directly improve your ecommerce brand’s success — or more accurately, your failure to prevent fraud schemes directly impedes your success. And with ecommerce fraud on the rise, security is a higher priority to online stores now than ever before.
Luckily, if you’re vigilant, you can step out of its way. Review the 7 most common scams listed above so you “know your enemy,” so to speak, and prepare yourself for what to expect. Likewise, review the warning signs and red flags we outlined so you can catch fraud attempts while they’re still just “attempts.”
We listed effective, DIY methods of ecommerce fraud prevention — techniques any online store-owner can implement on their own from scratch. But the bigger your ecommerce brand, the more outside help you’ll need. The top 10 fraud prevention tools offer something for everyone, so take a look at our evaluations and find the one that best fits your needs, goals, and limitations.
Matt Ellis is a freelance content writer, specializing in ecommerce and digital marketing. For over a decade, he's been sharing his industry knowledge through ebooks, website copy, and blog articles just like this one. You can learn more about his writing services at www.mattelliscontentwriter.com .
Related Articles and Topics
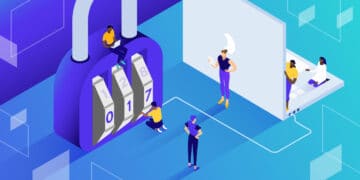
The Best WordPress Security Plugins To Lock Out Malicious Threats
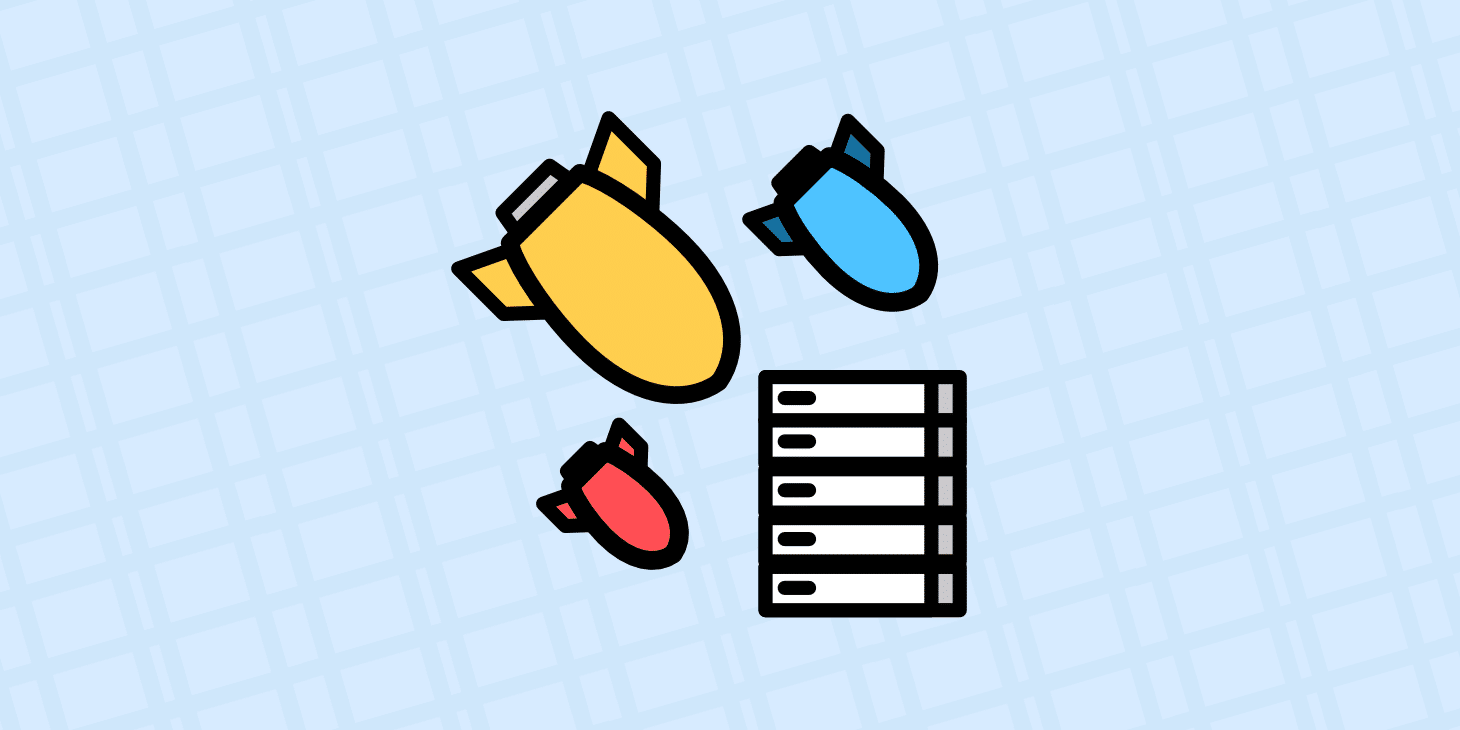
How to Stop a DDoS Attack in Its Tracks (Case Study)
- Local Development
- Web Development
- Website Performance
Online fraud is rampant in ecommerce. Because retailers don’t have a physical store, dissatisfied customers have to deal with a series of barriers for fair treatment.
Here are some common deceptions and practices to be aware of when buying online:
1. Large etailers selling their own brand can “dupe” their online reviews.
2. They can manipulate their return policies (and often do).
3. They often hide their return policies and other policies.
4. Some industries are more dishonest than others. Be wary of health products especially.
*Many reviews on their own websites are fake–written by professional copywriters hired within. Companies can hide their negative reviews on their own website and often do if they have less than 20 reviews on a product.
Leave a Reply Cancel reply
By submitting this form: You agree to the processing of the submitted personal data in accordance with Kinsta's Privacy Policy , including the transfer of data to the United States.
You also agree to receive information from Kinsta related to our services, events, and promotions. You may unsubscribe at any time by following the instructions in the communications received.
HOME SECURITY HEROES
15 Famous Identity Theft Cases That Rocked The Nation
I have had my identity compromised. It sucks. While I shudder to think about what the thief did with the stolen information, it has forced me to develop good online security practices and get the best identity protection services .
Identity theft cases are rising globally, especially in the United States.
The US Federal Trade Commission received nearly 1.4 million identity theft fraud reports in 2021. Apparently, this was fuelled by uncertainty over COVID-19.
But while COVID contributed to this surge, there have been countless identity theft cases long before the pandemic.
In fact, the earliest identity theft case can be traced back to 1548, when an imposter took over Martin Guerre’s identity and lived as him until the real Martin returned.
If you fancy a bit of biblical story, then the first reported identity theft could well be between Jacob and Esau.
But we’re not about to go that far .
Many identity theft cases in recent years are enough to blow you away.
I will try to cover as many cases as possible and the lessons you should learn.
Hopefully, these events will highlight how far a scammer can go to steal your identity. And the terrible consequences that you might suffer – like financial loss, prison, or even death.
✔ Act Now! Protect yourself from identity theft horrors. For a short time, enjoy a discount on Aura Identity Theft Protection!
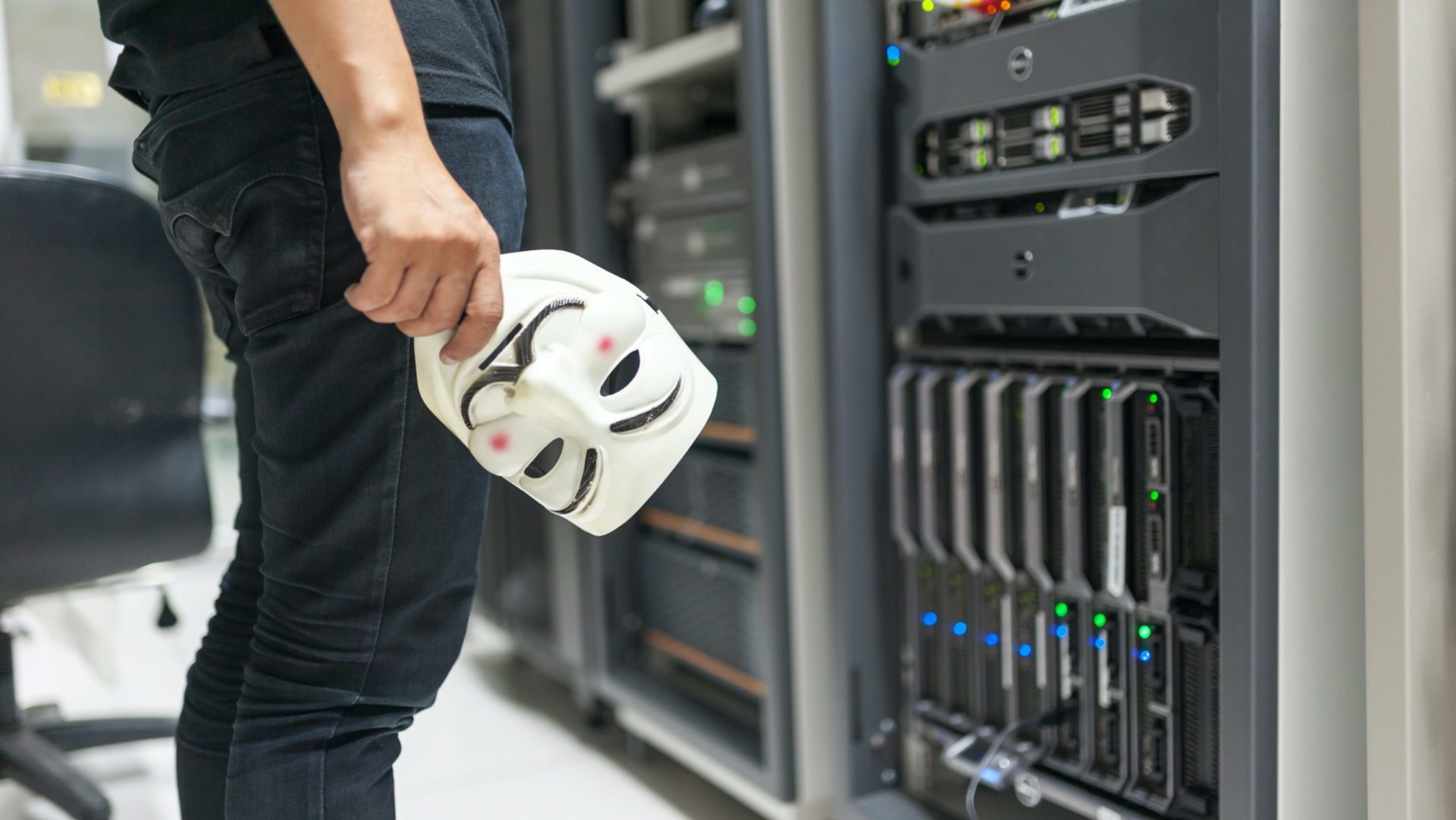
15 Famous Identity Theft Cases
1. the tinder swindler.
There’s a good chance you’ve seen the Netflix documentary, The Tinder Swindler .
If you haven’t, it’s a true crime documentary about an audacious scammer, Shimon Hayut, who posed as a wealthy businessman on Tinder.
Hayut tricked unsuspecting women with his supposedly lavish lifestyle and built fake relationships with them.
After gaining their trust, he would then tell his victims that business rivals were after his life and ask for their credit cards and loans.
Of course, he didn’t pay back. They never do.
As a result, many of Hayut’s victims are now burdened with crippling debt because he maxed out their credit cards.
According to The Times of Israel , he defrauded his victims of $10 million in two years.
Lessons You Should Learn
The Tinder Swindler is a classic example of a romance scam.
It’s a type of scam where scammers use love and romance to gain a victim’s trust.
When you finally find your dream match, they ask for money, gifts, or favors.
Unlike Simon Hayut, not all romance scammers will pose as wealthy people. While you’ll likely find sugar daddies and mommies, the core of this scam is building a romantic relationship with you online.
Anyone can fall victim to a romance scam – whether young or old. And the estimated 73,000 romance scam victims of 2022 alone will tell you that, too.
These Americans lost over $1 billion because they thought they found their knight in shining armor or their queen.
Romance scammers move fast. They’ll often declare their love quickly and make you do the same. Once they build a rapport, they start conjuring up different sob stories – their daughter has cancer, or a loved one needs emergency care.
It could also be the promise of an investment with a guaranteed ROI.
Don’t fall for it.
As a rule of thumb, never give money to someone you have only ever met online.
✎ Related: Signs Someone Is a Military Romance Scammer ⟶
2. Phillip Cummings Stole 33,000 Credit Reports Which Cost Victims Between $50-100 Million
At the time it happened, Philip Cumming was responsible for the largest identity theft case in US history.
Cummings worked as a help desk for a software company in Long Island. After quitting, he took a spreadsheet containing customer login credentials.
Between 2001 and 2002, he sold customers’ credit reports to scammers for around $30 each.
The criminals he sold the sensitive information to netted between $50 to $100 million from roughly 33,000 customers.
Cummings pleaded guilty and was sentenced to 14 years imprisonment in 2005.
Although he claimed he didn’t know the con artists would take that much money, the judge said he caused “almost unimaginable” damage.
Lessons Learned
This scam is an example of a data breach.
A data breach is when criminals assess a company’s database and steal sensitive information.
Since Cummings’ scam, data breaches are now happening at an unprecedented rate – almost every 11 seconds .
To protect yourself, make sure you limit the amount of sensitive information you give companies and services you sign up for.
Protect your banking information, credit file, and social security number (SSN).
You should also review credit card transactions and bank statements regularly. Each credit bureau, Experian, Equifax, and TransUnion, provides a free annual credit report.
Scammers might initiate small transactions to see if you’ll notice. If you or your financial institution don’t flag them, they’ll make more expensive purchases.
✔ Act Now! Don’t let identity theft stories become your reality. Secure a discount on Aura Identity Theft Protection now!
3. Nicole McCabe Was Framed for Assassination
When most people think about the worst that could happen from an identity theft case, it’s usually financial loss.
But not always.
A con artist with your identity can do absolutely anything they want.
It just so happens that most scammers WANT money.
However, what happens when the scammer doesn’t need money?
Nicole McCabe knows a thing or two about this.
In 2010, Australian Nicole McCabe’s identity was allegedly stolen by the Israeli Intelligence Agency, Mossad, and used to assassinate Mahmoud Al-Mabhouh, a Hamas leader, in Dubai.
Imagine being framed for murder. How did it happen?
Her passport had been reportedly compromised.
McCabe was in her car when she heard the assassination news on the radio. And to her surprise, her name was also listed among the suspects.
Apparently, the assassination squad stole her identity along with two other Australians – Adam Korman and Joshua Bruce – to commit the crime. Many other people from the UK, France, Germany, and Ireland had their identities used.
McCabe was lucky because the identity theft was quickly reported, and the issue was resolved.
But not everyone will get off easily.
Her case demonstrates why you must keep sensitive documents, such as bank statements, medical reports, and passports, safe.
If these documents get into the wrong hands, they could be used to perpetuate terrible crimes.
And if you don’t have McCabe’s luck, you’ll find yourself in prison – or worse, on death row.
You can keep confidential materials in a safe or even in the bank.
4. Abraham Abdallah – the Crooked Robinhood
We all know the story of Robinhood. He stole from the rich and gave it to the poor.
Abraham Abdallah targeted the rich, but unlike Robinhood, he didn’t give to the less fortunate.
The con man gained access to the brokerage accounts and credit card numbers for up to six months. He used cell phones, faxes, and delivery services.
With his scheme, Abdallah accessed up to 217 accounts, including those belonging to Warren Buffet, Steven Spielberg, and Oprah Winfrey.
Fortunately, he was caught before all the illegal transactions could be completed – an amount totaling over $22 million.
After admitting to identity theft, wire fraud, and credit card fraud, Abdallah reportedly served 11 years in prison.
Perhaps the biggest lesson with Abdallah’s case is that cyber-criminals can target absolutely anybody. Regardless of social status or wealth, these con artists can and will use your identity to commit crimes if you’re not careful.
Abdallah’s credit card fraud was one of the earliest cases in the digital age.
Since then, scammers have become more creative and now have access to sophisticated technology.
This has made it all the easier to complete scams.
Like in the case of Cummings, it’s important to protect your credit file and other personally identifiable information.
When signing up for an organization that requests your sensitive information, ensure you ask how your documents will be used and who has access to them.
The next case will highlight how cyber-criminals have become highly sophisticated.
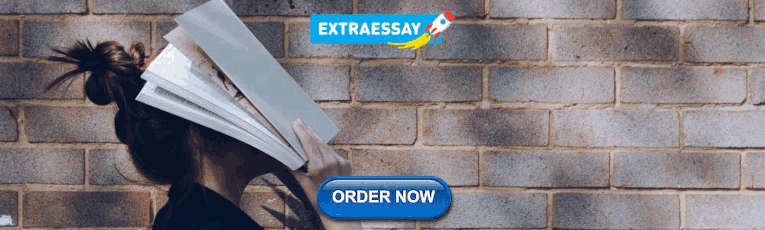
5. Amar Singh and His Wife, Neha Punjani-Singh
Amar Singh and his wife Neha Punjani-Singh entered a guilty plea in a $13 million scam.
They stole credit card numbers from victims and sold the information to buyers of fake cards, who then used it to make purchases worldwide.
The shoppers allegedly rented private jets, high-end cars, and five-star hotels using fake credit cards. When customers swiped their cards at retail or dining establishments, the attackers (Singh) used a skimming device to steal their personal information.
Singh received a sentence of less than 12 years in prison.
According to the FTC, credit card scams top the list of identity theft cases.
If you don’t want to be like Cummings’ or Singh’s thousands of victims, the best thing to do is freeze your credit.
No one can request or see your credit report. So, no one can open an account, apply for a loan, or get a new credit card while it’s frozen.
This service is free, it won’t impact your credit score, and you can temporarily lift the credit freeze whenever you want.
Other safety precautions include requesting annual credit reports, collecting physical mail daily, and reviewing bank statements and credit card transactions.
6. Senita Dill and Ronald Knowles SSN Fraud
Senita Birt Dill and Ronald Jeremy Knowles illegally acquired names, dates of birth, and Social Security numbers from 2009 to 2012.
They submitted over 1,000 false tax returns and received fraudulent tax refunds totaling more than $3.5 million .
Dill and Knowles filed and turned in false federal and state tax returns using tax preparation software.
The information on those filed returns was fake, including the amount of federal tax withheld and the income.
Afterward, they deposit their IRS refund check into a personal account.
The criminals were given prison terms of 324 and 70 months, respectively, and were required to pay the IRS $3,978,211 in restitution.
Dill and Knowles’ scam highlights the importance of protecting your social security number (SSN) .
A hacker with your personal information like name and address can use your SSN, especially the last four digits to steal your money, open accounts in your name, and apply for government benefits.
They might even get tax or healthcare refunds in your name.
Never reveal your SSN in emails, phone calls, or text messages.
A criminal with your SSN can use it to access other personally identifiable information.
It’s also important to educate your children on why they shouldn’t reveal sensitive information to people on social media.
Children and seniors are more prone to identity theft frauds, especially those perpetrated by supposed friends or lovers.
Protect your social number as best as you can.
7. Russian Hackers Send Phishing Email to an American Politician
Some people find out they’ve been a victim of identity theft just right before college.
Others find out during a presidential election.
John Podesta fell for a classic email phishing scam while he was the chairman of Hilary Clinton’s presidential campaign.
Russian hackers posed as Google and emailed Podesta to change his password due to an “unusual activity.”
However, the link redirected to a malicious website where the hackers accessed his email account.
Once they broke in, the hackers released thousands of secret and reputation-damaging emails.
Right in the middle of a presidential election.
Lessons to Learn
Although most people will spot a phishing scam easily, cybercriminals have become increasingly creative.
This type of scam is called spear phishing.
In this kind of cyber attack , the perpetrators gather information about their target and create a scheme they are likelier to fall for.
Spear phishing can also target a group of people, such as seniors aged 60 and above. For example, these people are more likely to have medical issues and be confused about Medicaid and other healthcare programs.
In Podesta’s case, the hackers knew he would be nervous about a potential cyber-attack before the election. So, they preyed on his fears to gain access to his account.
Here’s how to identify and protect yourself from phishing scams:
Ensure you, your kids, and older adults understand how frequent phishing emails are and protect yourselves against these attacks.
8. The EminiFX Cryptocurrency Ponzi Scheme
They say cryptocurrency is the new gold.
But the victims of EminiFX will wish they didn’t believe the company’s CEO, Eddy Alexandre .
According to the FBI, Alexandre used his crypto ponzi scheme to dupe unsuspecting victims up to $59 million.
EminiFX promised investors a “guaranteed” 5% to 9.99% weekly return.
They jumped on it. And Alexandre eventually received almost sixty million dollars.
In reality, most of their money was never invested. Instead, Alexandre took at least $14.7 million into his personal account and bought a BMW with another $168,000.
He pleaded guilty in February 2023, agreeing to forfeit over $248 million. He currently faces up to 10 years in prison.
Cryptocurrencies lack regulation, which explains why scammers like using them.
They will likely create FOMO (fear of missing out) by promising enormous returns. Then, they steal the money that people “invest” or pay out earlier investors, which keeps the scam going.
But only for some time.
The more they pay earlier investors, the more people believe it could be real and keep reinvesting. Once they’ve got enough, they’ll run.
Investment schemes often come with an unbelievable guaranteed return – which is why you shouldn’t believe it.
If it is too good to be true, it probably is.
9. Kenneth Gibson – the Man Who Stole 8,000 Identities
Kenneth Gibson was an IT professional for a software bigwig from 2012 to 2017.
And like Cummings, he had access to the private information of thousands of people, including clients and fellow employees.
Unfortunately, he took advantage of this privilege.
Gibson built a computer software that would automatically create fake PayPal accounts in people’s names using information from the company’s database.
Then, he would use the stolen identities to apply for new credit accounts linked to the fake accounts he created.
Gibson eventually succeeded in creating over 8,000 accounts .
He would only transfer small amounts of money, take cash advances from the credit line, and use his debit card to access the cash.
This helped him continue the scam until he netted around $3.5 million from his victims.
Kenneth Gibson’s scam demonstrates why you must monitor your credit card transactions.
As I mentioned earlier, many scammers will test the waters with a small transaction before making an expensive one.
In Gibson’s case, he didn’t need to make a bigger purchase because he had thousands of accounts to steal from.
His scheme might have still been running till today had he not become careless.
Gibson would usually get cash from an ATM. However, he requested a check from PayPal and one occasion.
The name on one of the checks matched a victim’s name, which gave him away.
He was given a 4-year prison sentence in 2018, had to pay $1 million as compensation, and sold his assets to repay the amount he stole from his victims.
If you didn’t initiate a transaction, report it immediately, no matter how small.
10. SIM Swapping Scam on Jacy Erin
In Jacy Erin’s case , hackers broke into her mother’s email account, stealing sensitive information. This included her phone number and credit card information.
Then, they contacted her phone number to reroute all incoming calls to Erin and her parents to their phones.
Erin found out early and changed her family’s phone service.
But the deed had been done.
A few days later, Erin’s father learned that the scammers had used his credit card to spend almost $40,000.
Of course, the credit card company suspected foul play due to the odd purchase.
However, due to the earlier phone service rerouting, the calls went directly to the fraudsters.
In a SIM swapping scam, a scammer contacts your mobile phone provider and pretends to be you to get them to activate a new SIM card.
This means the fraudster now has a SIM card with your personal information, enabling them to receive your calls or texts.
Unfortunately, this lets them bypass security fraud alerts and two-factor authentication.
To prevent a SIM swapping scam, call your service provider to lock your phone number or SIM to your account.
You’ll typically need to create a secure PIN which only you know.
This means any scammer who wants to “port” your number to a new phone must enter the PIN you’ve created.
Chances are that the scammers will not know this information.
Although unfortunate, Erin’s case demonstrates how scammers can use one weak link in the family to exploit other family members.
This is why it’s essential to protect your children from identity theft . They are the most vulnerable demographic to ID theft.
Once there is a fraud attempt on any family member, or someone close to you who has been a victim, take urgent measures to secure your account.
11. Nakeisha Hall – the IRS Staff
Many identity theft cases involve the IRS .
For example, a scammer calls pretending to be from the IRS and talks to you about tax refunds, etc.
However, what if the scammer actually works for the IRS?
In 2016, Nakeisha Hall pleaded guilty to using taxpayer information to defraud hundreds of victims of $1 million.
Hall was given a nine-year, two-month sentence and was required to pay the IRS $438,187 in restitution.
Hall worked in several IRS offices from 2000 to 2011. The IRS Taxpayer Advocate Service in Birmingham, Alabama, was one of them.
The department was created to help taxpayers who had become identity theft victims.
Since the ID theft came from IRS staff, this is quite tricky.
However, many victims would have avoided this scam if their credit files had been frozen.
You can freeze your credit when not in use and unfreeze it temporarily whenever you want.
This case also demonstrates why you shouldn’t trust anyone – even IRS staff.
It’s important to do your due diligence and beware of contacts that request your personal information.
12. Turhan Lemont Armstrong and the Real Estate Fraud
Real estate is one of the most profitable places to invest in. That’s why scammers use it to entice unsuspecting victims.
Turhan Lemont Armstrong was at the heart of a long-running credit card, loan, and real estate scheme that saw him net $3.3 million using stolen identities , especially children’s.
Armstrong was charged with 51 counts and found guilty on all.
One peculiar thing about this scam is that it targeted the SSNs of children who had left the country.
That’s because these people were more unlikely to monitor their credit reports.
He used these stolen identities and SSNs to open bank accounts, obtain credit cards, apply for loans, set up shell companies, and buy homes and cars.
Armstrong was sentenced to 21 years in federal prison and ordered to pay $3,305,609 in restitution.
This case demonstrates the importance of protecting your children from identity theft.
Around 1 in 50 children become identity theft victims annually.
Scammers can use your child’s credit to stack up debts. And when it’s time to pay for college, you’ll be stuck with unpaid debts.
Here are some steps to protect your child from identity theft:
13. How David Matthew Read Impersonated Demi Moore and Spent Over $169,000
In 2018, David Matthew Read impersonated Hollywood actress Demi Moore.
The 35-year-old con artist reported that her no-limit American Express card had been stolen.
Read had found Moore’s SSN and other personal details online.
Armed with this sensitive information, he intercepted the new card at a FedEx facility pretending to be Moore’s personal assistant.
A 25-day shopping spree followed. And this saw Read spend over $169,000 in luxury stores.
Read wasn’t going to enjoy the money for long as the FBI identified him and another accomplice from surveillance footage of them using the card to make purchases.
The scammer was sentenced to 70 months for his identity theft scheme, including three years of supervised probation and full restitution.
14. Luis Flores Impersonated Kim Kardashian
It seems there is no end to people stealing celebrities’ identities.
But 19-year-old Luis Flores Jr. took it to the next level when he called American Express and claimed to be Kim Kardashian.
He tried to change her SSN and address to his own so that he could receive new cards.
As expected, the credit card company got suspicious and reported Flores and his mom to the Secret Service.
Apparently, Kim Kardashian was the least of his targets.
Investigators found a flash drive containing the private data of Bill Gates, Michelle Obama, Beyonce, and even Paris Hilton!
The driver had their SSN, personal details, and credit card accounts.
But that’s not all.
Flores was also linked to identity theft cases involving fraud against Stacia Hylton, the US Marshals Service Director at the time, and Robert Mueller, a former FBI director.
You’ve got to have some nerve to steal the identity of an FBI director.
He and his mother ordered replacement cards using the personal information and changed the victims’ addresses and phone numbers on their accounts. Then, they made numerous wireless transfers from the targeted accounts to their own.
Lessons Learned
The internet has made it super easy for people to put out personal information online.
Don’t be that person.
Read found Moore’s personal info online.
It’s important to keep details like your SSN, banking statements, credit card information, passports, birth certificates, etc., from social media.
Flores and his mom also compiled the personal data of multiple people to exploit them.
While their targets were mostly celebrities, scammers can easily defraud a regular person who puts their personal information online.
✎ Related: How To Steal an Identity ➔
15. The Fake Hostage Phone Call
An Indiana woman received a fake hostage call from scammers who said they had her mother and would kill her.
The victim received what appeared to be a call from a scammer telling her they had a gun to her mother’s head and would shoot if she didn’t send $1,500 to their Venmo.
She could hear a woman screaming in the background, so she was unsure.
The victim was terrified and sent the money immediately.
After the call, she phoned her mom immediately.
You guessed right – her mom was perfectly fine.
This type of scam is called a hostage scam.
The scammer typically “spoofs” their phone number, making it look like the call was from the victim’s number.
Fraudsters can gather information from your social media and online footprint to know if you’re a possible target.
Another example of this scam is called the grandparent scam. This is when a fraudster calls a senior and pretends to be their grandchild who needs urgent financial help.
The fraudster may also claim that they’re calling on behalf of the grandchild.
For example, an elderly woman in Ohio was scammed of $20,000 in 2019 when the scammer pretended to be law enforcement.
The fraudster said her grandson was under arrest and needed bond money to be released.
To avoid virtual kidnapping schemes , ensure you:
- Never make your dates, travel plans, or locations public.
- Have a secret code with your loved ones that they can use when they’re really in trouble.
- If you’re in doubt, hang up and call the number back.
- Keep the scammer on the phone while you try texting or calling the person supposedly in trouble.
✎ Related: You Could Be Victimized By Someone Like Anna Sorokin ➔
How to Protect Yourself from Identity Theft Scams?
The identity theft cases above are crazy. They also prove that identity theft can happen to anyone , from children to celebrities.
I know that I can never completely eliminate the risk of id theft, but I do my best to steer clear. My strategy involves two steps:
- Practice good hygiene
- Continually monitor
Here’s my 7-step process for practicing good online hygiene
In addition to this, I also use a good identity theft protection service like Aura, which constantly monitors my credit, and public records as well as the dark web and notifies me immediately of any breaches I have been affected by.
I find Aura’s constant monitoring and theft insurance, together with my online hygiene checklist, help me sleep well at night.
You can read my Aura review here or how it is compared to household name LifeLock .
Get Aura Identity Protection If:
- You want the best identity theft protection service for your family
- You have assets worth protecting
- You like the peace of mind that Aura offers
- Up to $5M theft insurance
- Best in class credit and dark web monitoring
- White-glove threat resolution
- 24/7/365 Expert Customer Service
These identity theft cases show how far scammers are willing to go to steal your identity.
It also demonstrates how creative these fraudsters are getting and the sophisticated tech they use.
The best way to protect yourself and your family from being an identity theft victim is to keep sensitive information private.
Learn to identify phishing and social engineering tactics, and always monitor your credit file, transactions, and banking statements.
If you have kids and older adults, they are the likeliest to be victims. Educate them on the importance of being safe online and help them create secure passwords with 2FA.
Don’t forget to use strong antivirus software such as Norton , McAfee, and Proton.
Life can be fast-paced, so it might sometimes be difficult. That’s why you should consider identity theft protection services.
Some examples are Aura, Identity Guard, IdentityForce, LifeLock, IDShield, ID Watchdog, and IdentityIQ.
haveibeenpwned.com is another useful resource to find out if your sensitive data has been affected by a data breach.
✎ Related: Identity Thief Movie Review – Is It Close to Reality? ➔
- https://www.abc.net.au/news/2010-02-27/no-help-for-australian-caught-up-in-dubai-hit/344994
- https://www.smh.com.au/national/three-australians-shocked-by-id-theft-20100225-p5zj.html
- https://www.nbcnews.com/id/wbna6001526
- https://www.justice.gov/usao-nv/pr/reno-man-sentenced-four-years-prison-creating-over-8000-fraudulent-online-accounts-stolen
- https://www.justice.gov/usao-ndal/pr/irs-employee-sentenced-nine-years-and-two-months-prison-leading-1-million-id-theft-tax
- https://www.washingtonpost.com/news/post-nation/wp/2016/08/11/her-job-was-to-help-victims-of-identity-theft-instead-she-used-them-to-steal-from-the-irs/
- https://www.ice.gov/news/releases/los-angeles-area-man-convicted-scheme-used-stolen-identities-obtain-more-3-million
- https://patch.com/california/los-angeles/id-thief-who-used-demi-moores-amex-card-sent-halfway-house
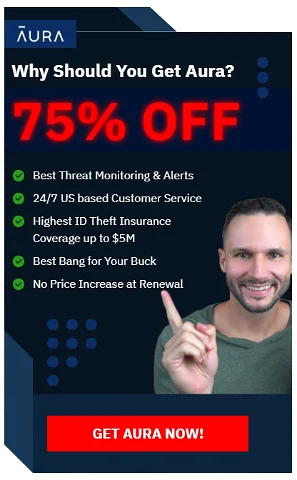
Scam victims tell us their stories
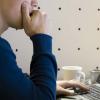
Investment scam: I lost $50,000 in fake online trading
Dating and romance scam: my brother lost $20,000 looking for love
Business email compromise: our business lost $190 000 when our supplier's email was hacked
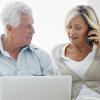
Online shopping scam: we lost $160 on a fake BBQ
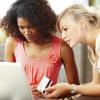
Unusual payment scam: I lost $300 to a Facebook ticket seller
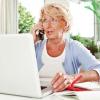
ATO impersonation scam: mother-in-law lost $4000 over a fake tax debt
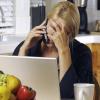
Remote access scam: I lost $520 to fake 'Telstra' employee
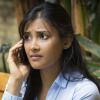
Identity theft: I lost $6028 when scammers stole my identity
Is this page useful.
Thanks for your feedback.
By providing an email address. I agree to the Terms of Use and acknowledge that I have read the Privacy Policy .
Phishing, identity theft top online concerns in PH
MANILA -Phishing and identity theft were the top online fraud concerns in the Philippines last year, which is among the countries where cyberthreats remain pervasive amid the increased digitalization, according to credit agency TransUnion.
In its 2023 State of Omnichannel Fraud Report, the company reported that 8.7 percent of the digital transactions were suspected to be fraudulent last year. This was the third highest among all countries included in the study.
TransUnion, however, said the volume of potential cyberthreats in the country showed a 18-percent decline from the 2019 level.
“[The] Philippines’ digital fraud rate still stands at a much higher level than the global average, leaving no room for complacency,” said Amrita Mitra, chief operating officer at TransUnion Philippines.
“As fraudsters become increasingly sophisticated, businesses must continue to equip themselves with the proper tools to detect fraud at the first warning sign without inhibiting the consumer journey,” the official added.
Phishing and text scams, both at 46 percent, were the most commonly reported fraudulent schemes in the past year.
Phishing is a fraudulent activity where hackers trick users into giving out their personal information, including bank accounts, through suspicious links embedded in emails, social media posts and even QR (quick response) codes. A text scam, meanwhile, is a form of phishing attack launched using mobile messages.
A successful phishing attack can lead to identity theft, which is among Filipinos’ primary concerns as well. Stolen identities could be used for taking over bank accounts.
—Tyrone Jasper C. Piad INQ
Southeast Asia workers vulnerable to phishing
Subscribe to our daily newsletter
Curated business news
Disclaimer: Comments do not represent the views of INQUIRER.net. We reserve the right to exclude comments which are inconsistent with our editorial standards. FULL DISCLAIMER
© copyright 1997-2024 inquirer.net | all rights reserved.
We use cookies to ensure you get the best experience on our website. By continuing, you are agreeing to our use of cookies. To find out more, please click this link.

An official website of the United States government
The .gov means it’s official. Federal government websites often end in .gov or .mil. Before sharing sensitive information, make sure you’re on a federal government site.
The site is secure. The https:// ensures that you are connecting to the official website and that any information you provide is encrypted and transmitted securely.
- Publications
- Account settings
Preview improvements coming to the PMC website in October 2024. Learn More or Try it out now .
- Advanced Search
- Journal List
- Springer Nature - PMC COVID-19 Collection

Exploring the determinants of victimization and fear of online identity theft: an empirical study
Inês guedes.
School of Criminology, Faculty of Law, University of Porto, Porto, Portugal
Margarida Martins
Carla sofia cardoso.
The present study aims at understanding what factors contribute to the explanation of online identity theft (OIT) victimization and fear, using the Routine Activity Theory (RAT). Additionally, it tries to uncover the influence of factors such as sociodemographic variables, offline fear of crime, and computer perception skills. Data for the present study were collected from a self-reported online survey administered to a sample of university students and staff ( N = 832, 66% female). Concerning the OIT victimization, binary logistic regression analysis showed that those who do not used credit card had lower odds of becoming an OIT victim, and those who reported visiting risky contents presented higher odds of becoming an OIT victim. Moreover, males were less likely than females of being an OIT victim. In turn, fear of OIT was explained by socioeconomic status (negatively associated), education (positively associated) and by fear of crime in general (positively associated). In addition, subjects who reported more online interaction with strangers were less fearful, and those reported more avoiding behaviors reported higher levels of fear of OIT. Finally, subjects with higher computer skills are less fearful. These results will be discussed in the line of routine activities approach and implications for online preventive behaviors will be outlined.
Introduction
The Internet provides unique affordances for criminal activities, especially due to the ease of information dissemination and anonymity of perpetrators. Furthermore, it extends traditional crimes (such as fraud or identity theft) to online offenses or create new types of criminal activity (e.g., malware infection). One of the crimes that can be considered an extension from traditional offenses is the online identity theft (OIT). OIT is considered one of the fastest growing crimes (Holt and Turner 2012 ; Golladay and Holtfreter 2017 ), resulting in relevant financial losses to victims. Knowing what factors might increase the risks of online victimization in general and OIT in particular is crucial. Although there is not one widely accepted definition of identity theft due to its complexity, it can be conceptualized as the “unlawful use of another’s personal identifying information” (Bellah 2001 , p. 222) such as accessing existing credit cards or bank accounts without authorization. Similarly, Koops and Leenes ( 2006 ) define identity theft as the unlawful acquisition and misuse of another individual’s personal information for criminal purposes. Therefore, the information that is obtained by the offender is acquired without the owner’s consent through illegal means. Researchers have been trying to established the factors that affect the likelihood of victimization of OIT. Among the criminological theories that have been used to explain this type of online victimization, Routine Activity Theory (RAT) is one of the most empirically tested (Cohen and Felson 1979 ; Reyns 2013 ; Reyns and Henson 2015 ; Reyns 2015 ; Bossler and Holt 2009 ). Moreover, while the research related to fear of crime in general is vast, the empirical studies aimed at understanding the fear of cybercrime are scarce, especially the fear of OIT. According to the 2019 Special Eurobarometer focusing on the European’s attitudes towards cyber security, although 92% did not report being a victim of identity theft in the last three years, at least two thirds of the sample reported to feel concerned about suffering a victimization of bank card or online banking fraud (67%) and of identity theft (66%). When comparing to the victimization of online crime or even to the fear of general crime, little is known about the online determinants of fear of identity theft.
The present study has two main objectives, namely to analyze the factors that increase (i) the risk of victimization of online identity theft and (ii) the fear and perceived risk of online identity theft. Concretely, the first objective aims at understanding what factors contribute to the explanation of online identity theft victimization , namely the exposure to potential offenders, target suitability and guardianship in the online context. Additionally, it tries to uncover the influence of factors such as sociodemographic variables and computer perception skills. Second, in order to understand what factors are related to fear and perceived risk of online identity theft , the present work will also test the influence of core dimensions of RAT, sociodemographic variables, previous victimization and general fear in those dependent variables.
Concerning the structure through which the present article will be developed, firstly the main crucial concepts and theories (e.g., online identity theft, RAT) will be outlined, followed by the empirical findings on the (i) relationship between RAT, individual variables and victimization of online identity theft and on the (ii) relationship between RAT, individual variables and fear of online identity theft. Next, the methodology of the present study will be described in detail. Finally, the results will be presented and discussed, including the implications of the present study.
Online identity theft
Identity theft is a term used to classify numerous offenses including fraudulent use of personal information for criminal purposes without individuals’ consent (Reyns 2013 ). Harrell ( 2015 , p. 2) defines identity theft as the “unauthorized use or attempted use of an existing account (such as credit/debit card, savings, telephone, online), the unauthorized use or attempted use of personal information to open a new account or misuse of personal information for a fraudulent purpose such as providing false information to law enforcement”. Accordingly, Copes and Vieraitis ( 2012 ) state that identity theft includes the misuse of another individual’s personal information to commit fraud. Identity theft may or may not be committed through technological and informatic means (Wang et al. 2006 ). Online or cyber identity theft involves the online misappropriation of identity tokens such as email addresses, passwords to access online banking or web-pages (Roberts et al. 2013 ). In the present paper, we define OIT as the illicit and improperly use, via internet, of the personal and financial data which was obtained without prior consent and knowledge by the cyber-criminal. In order to perpetrate this crime, offenders usually employ a mix of Information and Communication Technologies and social engineering, using methods such as hacking, phishing and pharming. Phishing involves attempts to mislead individuals into revealing sensitive information by posing as a legitimate entity such as banks. Hacking is an unauthorized access which may be way of unto itself or for malicious purposes such as spreading malware. Pharming occurs when, through the use of a virus or a similar technique, individual’s browser is hijacked without their knowledge. Therefore, when targets type a legitimate website into the address bar of their browser, the virus will be redirected them to a fake site. In addition to these types of methods, research have been analyzing the relationship between data breaches and identity theft (e.g., Garrison and Ncube 2011 ; Burnes et al. 2020 ). For instance, Tatham ( 2018 ) observed that those who have been impacted by a database breach were 31.7% more likely to experience identity fraud compared to 2.8% of persons not alerted of a data breach. This result can be explained by the fact that the information obtained in a database breach is generally sold online, containing personally identifiable information that can be used to commit identity theft crimes. Therefore, in spite of the relevance of individual online activities, data breaches that target retailers or government entities, for instance, may also increase the risk of online identity theft.
Identity theft is considered one of the most feared, fastest-growing crimes and a public health problem (Burnes et al. 2020 ). In fact, Harrel and Langton ( 2013 ) estimated that the average loss experienced by victims of identity theft was $2183 in 2012. Later, an estimate made by Javelin Strategy and Research showed that the costs of identity theft were near $16 billion (Piquero 2018 ). Besides the financial losses, it has also been observed that, on average, victims spend at least 15–30 h (although it might take several years) solving financial problems related to identity theft. Recently, Golladay and Holtfreter ( 2017 ) analyzed the nonmonetary losses experienced by victims of identity theft through a survey where 3709 individuals reported experiencing some form of identity theft in the past 12 months. The authors found that victims of this crime, as with other forms such as bullying and violence, suffered relevant emotional and physical symptoms. Moreover, that the distress involved in recovering from identity theft could be conceived as a stressor resulting in negative emotions such as depression and anxiety. These results have been consistently reported by other investigations (e.g., Reyns and Randa 2017 ; Li et al. 2019 ; Harrell 2019 ) that found diverse emotional problems such as anxiety, irritation and distress following victimization of (online) identity theft. As we have been seen an increasing in the numbers of victims over the years (Li et al. 2019 ), as well as a set of empirically demonstrated harmful financial and emotional consequences, studying why someone is more prone to be victimized of online identity theft is fundamental. Moreover, it is crucial to understand if individuals fear this type of crime and what are the factors that explain the higher levels of fear of online identity theft. The Routine Activity Theory (or RAT) may help to understand what are the behaviors related to both victimization and fear of OIT.
Routine activity theory
In 1979, Cohen and Felson argued that “structural changes in routine activity patterns can influence crime rates by affecting the convergence in space and time of three minimal elements of direct-contact predatory violations: (1) motivated offenders, (2) suitable targets, and (3) the absence of capable guardians against a violation” (p. 589). Therefore, the RAT was a perspective developed to account for offenses and victimization in the physical world. In the context of cybercrime, authors such as Yar ( 2005 ) and Leukfeldt and Yar ( 2016 ) have been debated the applicability of this framework. In fact, the requirement of offenders and victims converging in time and space for a crime to happen presents a problem when applying the theory to cybercrimes. Gabrowsky ( 2001 ), opposing to ‘transformationists’ like Capeller ( 2001 ) argued that RAT could be applied to crimes in cyberspace since it was just a case of “new wine in old bottles”. In the same direction, Eck and Clark ( 2003 ) suggested that this framework can be useful to explain these types of online crimes since the target and the offender are part of the same geographically dispersed network, and therefore the offender is able to reach the target through network. In accordance, Reyns et al. ( 2011 ) argued that instead of a real-time convergence of victims and offenders within networks, the temporal overlap between them may be lagged for either a short time or a longer period. Therefore, according to RAT, it is expected that online activities—such as banking, shopping, instant messaging or downloading media, might be associated with higher levels of victimization. Moreover, that greater levels of security measures—installing antivirus software, filtering spam email or routinely changing passwords—might decrease opportunities for offenders to access personal information.
Although the RAT has been tested to explain different online forms of victimization such as phishing, hacking, interpersonal violence, malware infection or internet fraud (e.g., Choi 2008 ; Holt and Bossler 2009 ; Marcum et al. 2010 ; Raising et al. 2009 ), few studies have empirically examined OIT victimization from a routine activities’ perspective (e.g., Reyns 2013 ; Reyns and Henson 2015 ; Burnes et al. 2020 ). Furthermore, most studies have not operationalized all of the core dimensions of the theory (i.e. exposure to motivated offenders, target suitability and capable guardianship). Departing from the RAT, and including as well individual variables, we now review the main determinants of victimization and fear of online identity theft.
Determinants of victimization of online crime
Rat core dimensions.
The exposure to risky situations and proximity to offenders (online exposure) is usually conceptualized from the victim’s perspective (Reyns and Henson 2015 ), and have been operationalized through different ways (e.g., ‘the amount of time spent on the internet’ or ‘the amount of time doing specific activities’ Ngo and Paternoster 2011 ; Reyns 2013 ). The results of the studies that explore the relationship between exposure and online victimization are mixed. For instance, Holt and Bossler ( 2013 ) found that internet usage (measured through number of hours per week) did not predict the likelihood of malware infection victimization. Furthermore, in a recent study, Holt et al. ( 2020 ) observed that time spent on specific online activities, such as downloading files and visiting dating websites consistently increased the risk of victimization of malware software infections. This result is consistent with the research employed by Alshalan ( 2006 ) who found that risk exposure (measured through the frequency of using the Internet and duration) was a determinant of victimization of computer virus and cyber-crime. A study by Pratt et al. ( 2010 ) observed that making a purchase online from a website doubled the likelihood of being targeted for online consumer fraud. In turn, Ngo and Paternoster ( 2011 ) found that the number of hours per week that respondents engaged in instant messaging increased the likelihood of experience online harassment by a stranger. However, none of the measures of online routine activities were relevant to phishing victimization. This result was contrary to what was found by Reyns ( 2015 ) who concluded that booking/making reservations, online social networking, online banking and purchasing behaviors were related to phishing. Finally, focusing specifically on OIT, both Reyns ( 2013 ) and Reyns and Henson ( 2015 ) observed that banking and purchasing, as measures of online exposure, positively contributed to OIT victimization. This result was confirmed by a recent study employed by Burnes et al. ( 2020 ). In fact, as the authors concluded, while participating in commercial activities (such as online purchasing) “reflects a major societal innovation and lifestyle shift that has allowed consumers to purchase products conveniently and globally (…) the odds of using an unsecured payment portal or having information exposed in a retail data breach increases” (p. 6).
In the context of cybercrime, target attractiveness is usually operationalized through visiting risky or unprotected websites or divulge personal information online. Even though different studies argue that target suitability is a relevant TAR dimension in explaining online victimization, the results are mixed. For instance, Alshalan ( 2006 ) observed that the more people divulge their credit or debit card number and disseminate their personal information, the more they are at risk of becoming victims of cybercrime. Accordingly, Reyns and Henson ( 2015 ) suggested that having personal information posted online increases victimization of OIT. In 2013, van Wilsem found that spending much time on Internet communication activities increased the risk of victimization including online harassment. Nevertheless, Ngo et al. ( 2020 ) observed that individuals who conduct online banking and plan their travels online had a lower risk of experiencing harassment by a non-stranger. The authors suggest that individuals who conduct these types of activities online were less likely to engage in computer deviance giving the positive relationship between being harassed and deviance online. At the same time, Ngo and Paternoster ( 2011 ) observed that individuals who frequently opened unfamiliar attachments to emails received or frequently opened any file or attachment they received through instant messenger had lower odds of obtaining a computer virus. Lastly, using a sample of 9161 Internet users, Leukfeldt and Yar ( 2016 ) found that only one online activity (targeted browsing, or the search for news or targeted information search) had an effect on identity theft victimization.
Finally, scientific literature has been studying the effects of guardianship on the likelihood of victimization. The online guardianship dimension has been measured through different ways—e.g., shredding personal documents, actively changing account passwords, using antivirus software, deleting emails from unknown senders (Reyns and Henson 2015 ; Ngo and Paternoster 2011 ; Holt and Bossler 2013 ), producing as well mixed results. Holt et al. ( 2020 ) found that having a secured wireless connection decreased the risk of malware victimization. A few years before, explaining the malware victimization, Holt and Bossler ( 2013 ) found that having low-level of computer skills (a measure of lack of guardianship) and using anti-virus software (presence of guardianship) were among the significant predictors of that crime. In turn, Reyns and Henson ( 2015 ) observed that none of the online guardianship measures were relevant to reduce victimization of OIT. One possible explanation is that some victims adopted guardianship routines strategies post to their victimization. Williams ( 2016 ) observed that passive physical guardianship was effective in reducing online identity theft due to the automated form of this type of security when comparing to the others. Moreover, a positive association was found between active personal guardianship and victimization, explained by the post-victimization security reactions. Data collected by Reyns ( 2015 ) suggested a positive relationship between guardianship and victimization for phishing, hacking and malware infection, contrary to what was hypothesized by the author. Accordingly, Williams ( 2016 ) observed that having an anti-virus software was positively related to malware victimization, suggesting that individuals who experienced online victimization were more prone to install this type of software. At the same time, a positive relationship between phishing victimization and deleting emails from unknown senders was observed. Finally, Burnes et al. ( 2020 ) observed that proactive individual behaviors (e.g., shredding personal documents and actively changing account passwords), reduced the likelihood of identity theft.
Individual variables
Numerous research studies have also examined the impact of individual variables on online victimization. Alshalan ( 2006 ) found that gender had an effect on both computer virus victimization and cybercrime victimization, suggesting that males are more victimized than females. Accordingly, Holt and Turner ( 2012 ) and Reyns ( 2013 ) observed that males were at greater risk of identity theft victimization. Conversely, Anderson ( 2006 ) discovered that the estimated risk of experiencing some form of identity theft was 20% more greater for women than for men. Concerning age, while Reyns ( 2013 ) observed that older adults presented higher risk of identity theft victimization, Williams ( 2016 ) and Harrell ( 2015 ) found that younger and middle-aged adults, respectively, reported higher levels of this kind of victimization. Ngo and Paternoster ( 2011 ) observed that each additional year in age decreased the odd of obtaining a computer virus by 2% and of experiencing online defamation by 6%. More recently, Burnes et al. ( 2020 ) observed that individuals between the ages of 39 and 73 were at the highest risk of most types of identity theft, reflecting the socioeconomic capacity and consumption patterns of this generation relative to millennials. Another important result discovered by these authors was that higher educational accomplishment was related with higher risk of existing credit card/bank account identity theft victimization. Accordingly, Reyns ( 2013 ) and Reyns and Henson ( 2015 ) observed that those with higher incomes are most likely to be victimized of OIT. Williams ( 2016 ) showed that social status exhibited a curvilinear association with victimization. Lower and higher status citizens reported highest levels of victimization, while those of average status reported the lowest. Lastly, none of the sociodemographic variables—gender, age, education level, personal or household income and financial assets or possessions explained identity theft victimization in Leukfeldt and Yar’s ( 2005 ) research.
Fear of (online) crime
Fear of crime is considered a serious social problem and has been vastly studied in the last decades (Hale 1996 ). Empirical research has been testing what are the determinants of fearing crime, including individual (e.g., gender, age, social class, education,) and contextual predictors (e.g., incivilities, social cohesion, poor street lighting). Using a wider definition of fear of crime, this construct is conceptualized as a set of three dimensions: the emotional fear of crime, risk perception of victimization and the different behaviors adopted for security reasons (e.g., Gabriel and Greve 2003 ; Liska et al. 1988 ). The emotional fear of crime is a response to crime or symbols associated with it (Ferraro and La Grange 1987 ; Rader et al. 2007 ), different from risk perception which is the likelihood of victimization perceived by an individual (for instance, the likelihood of being a victim of burglary in the next 12 months ). Though they can be related (Mesch 2000 ), the cognitive dimension is distinct from the emotional fear of crime and it refers to an assessment of personal threat or a judgment that individuals make of their risk of victimization. Authors have also been distinguished formless fear from concrete fear. While the first is a generic fear not related to any type of crime, the latter corresponds to specific crimes such as fear of identity theft or fear of robbery (for instance, Keane 1992 ).
Although fear of general crime has been largely studied, limited research has been conducted about fear of online crime and even less on fear of OIT (Henson et al. 2013 ; Virtanen 2017 ; Abdulai 2020 are some exceptions). Hille et al. ( 2015 ) included two main dimensions on the fear of OIT: the fear of financial losses and fear of reputational damage . While the first is the fear of illegal or unethical appropriation and usage of personal and financial data by an unauthorized entity with the goal of getting financial benefits, the second is the fear of misuse of illegally acquired personal data with the objective of impersonation which can cause reputational damage to the victim—for instance, use the victim’s credit card to buy embarrassing products. In the present paper, we follow Hille’s et al. ( 2015 ) definition of fear of OIT.
Exploring fear of OIT is crucial since it is considered one of the most important psychological barriers to consumers (Martin et al. 2017 ), and constitutes a severe threat to the security of online transactions associated with e-commerce services. For instance, Jordan et al. ( 2018 ) explored the impact of fear of identity theft and perceived risk on online purchase intention using a sample of 190 individuals from Slovenia. First, they found a positive correlation between fear of identity theft and perceived risk. Then, they observed that perceived risk decreased the online purchase intention. Now we summarize the main determinants of fear of online crime and the results of the few studies which focused on the explanation of fear of OIT.
Determinants of fear of crime online
Sociodemographic determinants.
Gender is considered the best predictor of fear of crime in general (Hale 1996 ) with women being considered the most fearful group. Under cybercrime research, a set of studies concluded that women are as well the most fearful group, although it depends on concrete crimes. For instance, while Henson et al. ( 2013 ) found higher levels of fear on women of interpersonal violence, Yu ( 2014 ) observed no statistically significant differences between women and men for online identity theft, fraud and virus. Virtanen ( 2017 ) employed an analysis of the fear of eight types of cybercrimes in 28 countries using data from the Special Eurobarometer Survey. The author consistently found that women presented higher levels of fear of cybercrimes comparing to men. Lastly, Roberts et al. ( 2013 ) discovered that gender was not a predictor of fear of OIT which was mainly explained by contextual dimensions and the fear of traditional crime.
Concerning the relationship between age and offline fear of crime, it can be argued that the results are mixed. While some authors found that older people presented higher levels of fear (e.g., Reid and Cornrad 2004 ), others conclude that younger individuals report higher levels of fear when comparing with older adults (e.g., Ziegler and Mitchel 2003 ). In the online context, the relationship between age and fear is also not well established. For instance, Virtanen ( 2017 ) and Henson et al. ( 2013 ) found that younger people fear more online crime comparing to older ones. Roberts et al. ( 2013 ) observed that age (with a positive direction) was the only predictor of fear of OIT but it accounted for less than 1% of the unique variance in fear of OIT and related fraudulent activity. Accordingly, Alshalan ( 2006 ) and Lee et al. ( 2019 ) found that older individuals presented higher levels of fear of online crimes, suggesting that they attribute more value to property and, as a consequence, they fear losing it.
Regarding the impact of socioeconomic status, researchers have been demonstrated that groups with higher disadvantage feel more afraid offline when compared to lesser ones since they present fewer capacity to afford measures to their protection (Hale 1996 ). Accordingly, in the online context, Virtanen ( 2017 ) and Brands and Wilsem ( 2019 ) found that individuals with lower socioeconomic status presented higher levels of fear of online crime, suggesting that less advantage groups might find more difficult to deal with potential costs of an online victimization. In turn, Reisig et al. ( 2009 ) found that those reporting lower levels of socioeconomic status perceived higher levels of risk which, in turn, was associated with online behaviors that reduced the potential likelihood of online theft victimization. Concerning education, typically the literature on traditional fear of crime finds that less educated individuals report higher levels of fear (e.g., Smith and Hill 1991 ). While Alshalan ( 2006 ) and Roberts et al. ( 2013 ) found no direct association between education and general fear of cybercrime, Brands and Wilson ( 2019 ) observed lower levels of fear of online crime in higher educated individuals. On the opposite direction, Akdemir ( 2020 ) found that higher educated individuals reported higher levels of fear of cybercrime comparing to those who were less educated. Accordingly, the author argued that higher educated groups had a higher likelihood of adopting online security measures such as password management (e.g., use of multiple passwords) and elimination of suspect emails.
Previous victimization
The relationship between victimization and fear of traditional crime has been produced mixed results. For instance, Mesch ( 2000 ), Tseloni and Zarafonitou ( 2008 ) and Guedes et al. ( 2018 ) found a positive relationship between perceived risk and victimization. Regarding the relationship between fear of online crime and victimization, the few studies available observed that previous experiences with online crime are important to fear. For instance, Randa ( 2013 ) found that past experiences with cyberbullying increased fear of online crime. Moreover, Alshalan ( 2006 ) also corroborated that previous direct victimizations increased fear of cybercrime, suggesting that the negative consequences of a victimization had a ‘sensitizing effect’ which increased the insecurity on cyberspace. Additionally, Henson et al. ( 2013 ), Virtanen ( 2017 ), Lee et al. ( 2019 ), Brands and Wilsem ( 2019 ) and Abdulai ( 2020 ) suggested that previous online victimization had an impact of fear of online crime. On the contrary, non-significant results were found in Yu’s ( 2014 ) study.
Exposure to online crime and technical skills
The relationship between exposure to online crime and fear has been scarcely investigated. An exception is Roberts and colleagues’ study ( 2013 ) who found that frequency of internet use and use of internet at home where significant predictors of fear of cyber-identity theft and related fraudulent activity. Conversely, Henson et al. ( 2013 ) observed that none of the exposure variables showed statistically significant effects on online fear. Lastly, Virtanen ( 2017 ) showed that frequency of internet use was not associated with fear of cybercrime in models with interactions between gender or social status and victimization. However, the author suggested that the effects of internet use and knowledge of risks were mediated by confidence in one’s abilities. Concerning technical skills, few studies have been investigating if the perception of low technical skills is related with a higher fear of online crime. For instance, Virtanen ( 2017 ) found a negative relationship between these variables, although the author observed that being in the group with the lowest confidence in one’s abilities was not a direct predictor of fear. Finally, Abdulai ( 2020 ) observed that knowledge of cybercrime was not a predictor of fear of becoming a victim of credit/debit card fraud.
Current focus
The present study has the main objective of identifying risk factors both for OIT victimization and fear of OIT (including fear and perceived risk of victimization). Despite some knowledge on the factors associated with several forms of online victimization, there have been relatively few empirical studies on the factors that affect the likelihood of online identity theft. Additionally, existing research rarely addresses all the core dimensions of RAT (online exposure, target suitability and online guardianship). Thus, it is unclear what online activities increase the risk of online identity theft victimization and who are the most targeted individuals in terms of personal characteristics. Furthermore, while the victimization of OIT has been studied in the last years, to what extent and why individuals feel more fearful of OIT has rarely been the focus of scientific research.
Therefore, our investigation is crucial and innovative since: (a) operationalizes all the core concepts included in RAT (online exposure, target suitability and online guardianship) to fully test its prediction of OIT victimization, (b) examines not only the victimization but also the fear and perceived risk of being a victim of OIT; (c) compares the importance of both individual variables and contextual variables both for victimization and fear of this form of victimization.
Data for the current study were collected in 2017 through an online self-report anonymous survey built to explore the variables related to RAT that influenced both the victimization, the perceived risk and the fear of OIT. For that purpose, an email containing the objective of the study and the link of the survey was sent by the University of Porto services to invite students and staff (teaching and non-teaching) to participate in this study. In total, 831 individuals answered the questionnaire.
Dependent variables
In the current study three dependent variables were considered: the OIT victimization, the fear of OIT and the perception of victimization risk concerning the previous referred crime. To measure OIT victimization, respondents were asked: “how many times someone has appropriated, via Internet, personal and financial data without prior consent and knowledge and used them improperly during your lifetime” . Responses varied between 0 to more than 5 times. Lifetime OIT victimization was recoded to a dummy variable (0 = no victimization, 1 = victimization). Fear of OIT was operationalized through an adapted scale of Hille et al. ( 2015 ). This scale conceptualized fear of OIT as having two main dimensions: fear of financial losses and fear of reputational damage. A four-point Likert scale was used to rate the following items varying from 1 (not afraid) to 4 (very afraid): “how fearful do you feel if” … (1) somebody steal your personal and financial data via online? (2) somebody use your personal and financial data via online? (3) somebody damage your reputation based on the illegitimate use of your personal and financial data online?
Concerning the risk perception of victimization related to OIT it was asked the participants to rate in a scale of 1 (not likely) to 5 (very likely) the following items: “ how likely do you think that…” (1) somebody could steal your personal and financial data via online during the next 12 months? (2) somebody could use your personal and financial data via online during the next 12 months? (3) somebody could damage your reputation based on the illegitimate use of your personal and financial data online during the next 12 months? Therefore, perceived risk of OIT was adapted from scale of fear of OIT built by Hille et al. ( 2015 ) and from previous literature that conceptualizes perceived risk as the estimated likelihood of being a victim of crime in the future (e.g., Guedes et al. 2018 ).
Individual characteristics
In order to study how sociodemographic dimensions influenced the dependent variables, insofar as these may be related to patterns of Internet usage and victimization experiences, four individual characteristics of respondents were included: (a) gender (0 = male, 1 = female), (b) age (in years), (c) perceived socioeconomic status (1 = low, 2 = average, 3 = high) and (d) levels of education (1 = up to 4th years of education to 5 = postgraduate studies). Furthermore, a measure of fear of crime offline was included to understand if fear of identity theft online (concrete fear) was influenced by a more general fear of being victimized. The operationalization was based on previous studies and a two item’s measure was undertaken: (a) “How safe do you feel when walking alone in your residential area after dark?” , ( b) How safe do you feel being alone in your home after dark?”.
Three theoretical dimensions from RAT were measured: online exposure to motivated offenders, target suitability, and capable guardianship.
Online exposure
Departing from one of the aims of the present study, analyzing the relationship between exposure and victimization, fear and perceived risk of OIT, respondents were asked a set of questions to measure their online routines. Thus, to examine Internet routine activities, two different measures were used. The first was assessed by the single item “how much time, per day, in average, do you spend online?”, measured in number of hours. Additionally, in an effort to examine specific online routine activities, the frequency of specific online routines activities was rated by participants in a scale of 1 (never) to 5 (always). Following Reyns ( 2013 ), participants were asked “how often do you use the Internet for the ensuing purposes” : (1) online banking or managing finances, (2) e-mail or instant messaging, (3) watching television or listening to the radio, (4) reading online newspapers or news websites, (5) participating in chat rooms or other forums, (6) reading or writing blogs, (7) downloading music, films, or podcasts, (8) social networking (e.g., Facebook, Myspace), (9) for work or study, or (10) buying goods or services (shopping). After performing a factor analysis, the items were aggregated in three main routine activities: (1) financial routines (items 1 and 10), (2) work routines (items 2, and 9), and (3) leisure routines (items 3, 5, 6 and 7). Finally, to understand the types of payments used by respondents, we asked if they used the following forms: home banking, PayPal, credit card, pay safe card, MB Net. Respondents had two response options: ‘yes’ (coded as 1) and ‘no’ (coded as 0).
Target suitability
One of the factors that can increase individual’s likelihood of being victimized (both online and offline) is his or her attractiveness as a potential target (Reyns and Henson 2015 ). In the present study, based on previous works such as those of Paternoster (2011) and Reyns ( 2015 ), online target suitability was measured through the following question: “In the past 12 months have you” : (1) communicated with strangers online, (2) provided personal information to somebody online, (3) opened any unfamiliar attachments to e-mails that they received, (4) clicked on any of the web-links in the emails that they received, (5) opened any file or attachment they received through their instant messengers, (6) clicked on a pop-up message, or (7) visited risky websites . Participants had two response options: ‘yes’ (coded as 1) and ‘no’ (coded as 0). After performing a factor analysis, responses were computed in three indexes: (a) interaction with unknown people (items 1, 2), (b) open dubious links (items 3, 4, 5), and c) visit risky on-line contents (items 6, 7).
Online capable guardianship
Theoretically, higher guardianship might be related to lower levels of victimization in general and victimization of OIT in particular, especially in individuals who protect their personal information through multiple security behaviors. Moreover, it is expected that individuals who fear and perceived more risk of OIT are more prone to adopt these types of security measures. In the present study, to measure the capable guardianship, 13 items based on Williams ( 2016 ) and Ngo and Paternoster’s ( 2011 ) works were used. It was asked participants “For security reasons do you…” (1) avoid online banking, (2) avoid online shopping, (3) use only one computer, (4) use e-mail spam filter, (5) change security settings, (6) use different passwords for different sites, (7) avoid opening emails from people you do not know, (8) visits only trusted websites, (9) has installed and updated antivirus software, (10) installed and upgraded antispyware software, (11) has installed and updated software or hardware firewall, (12) participate in public education workshops on cybercrime, or (13) visits websites aimed at public education on cybercrime. The answers to items were dichotomized (0 = no, 1 = yes) and after a factor analysis they were combined in summated scales corresponding to four types of guardianship: (a) avoiding behaviors (items 1 and 2); (b) Protective software/hardware (items 9, 10, 11); (3) Protective behaviors (items 4, 5, 6); 4) Information (items 12, 13). Additionally, a complementary question to measure the online capable guardianship was included. Therefore, participants had to rate their perception of computer skills ranged between low, medium and high.
Data analysis
Concerning the lifetime OIT victimization, given the dichotomous nature of the variable (0 = no; 1 = yes), binary logistic regression was performed comprising the individual variables but also the variables related to their routine activities.
Moreover, linear regression analysis was executed to analyze the effects of individual and routine activities variables on the dependent variables: fear of crime and risk perception of victimization. In the first model, the individual variables were included to assess their importance in the explanation of each dependent variable (fear and risk). In the second (full) model, contextual variables were added.
A total sample of 831 subjects completed the online survey. Sixty-six percent were females with a mean age of 27 years. The sociodemographic characteristics of the sample and the descriptive results of the studied variables are presented in Table Table1 1 .
Descriptive results of the studied variables
OIT victimization
Table Table2 2 presents the results of the logistic regression when lifetime OIT victimization prevalence was regressed on online exposure, target suitability, capable guardianship, and individual variables. Although the model does not reach statistical significance ( p = 0.068) and Nagelkerk R 2 are relatively modest (0.059) four independent variables reached statistical significance. Considering online exposure, the use of credit card form of payment was significantly related to OIT victimization. Specifically, those who do not used these forms of payment had lower odds (34%) of becoming an OIT victim. In the same direction, the use of paysafecard as form of payment was also significantly ( p = 0.05) related to OIT victimization, suggesting that those who do not use this form of on-line payment had lower odds (59%) of becoming victim. None of the other forms of payment and routine activities (financial, work, and leisure) predicted OIT victimization. In what concerns the variables related to target suitability, only one was significantly related to OIT victimization—visit risky contents ( B = 0.283, OR 1.328)—i.e. those who reported to visit risky contents present higher odds (33%) of becoming an OIT victim. None of the studied variables related to capable guardianship were associated with OIT victimization. Considering the individual variables, gender was significantly associated with OIT victimization, with males being less likely (35%) than females of suffering an OIT victimization. Age, perceived socioeconomic status and education were not related to OIT victimization (Table (Table2 2 ).
Logistic regression of lifetime prevalence of OIT victimization on online exposure, target suitability, capable guardianship and individual variables
Bold values indicate statistical significance
* p ≤ 0.05; ** p ≤ 0.01; *** p ≤ 0.001
Fear and risk of OIT
To test the correlates of fear of OIT and risk perception of being a victim of OIT, hierarchical regression analysis was performed (Table (Table3). 3 ). For each dependent variable, two models were tested. The first model includes the individual variables (gender, age, SES, education, OIT victimization and general fear of crime) to control the effects of these variables on the dependent variables. In the second model (full model) the routine activities variables were added (online exposure, target suitability and capable guardianship variables).
Hierarchical linear regression models predicting fear and risk of online identity theft (OIT)
In what concerns fear of OIT, the first model explains 10.8% of the variance. Concretely, gender, SES and general fear of crime are significant predictors of fear of OIT. Thus, females ( B = 0.186), and individuals with low SES ( B = − 0.142) present higher levels of fear of OIT. Interestingly, subjects who report more fear of crime in general also reported more fear of OIT ( B = 0.257). The model II explains 16.4% of the variance. Interaction with strangers, avoiding behaviors and computer skills were the variables significantly associated with fear of OIT. Concretely, subjects who reported more interaction with strangers are less fearful ( B = − 0.176), and those who reported to adopt more avoiding behaviors reported higher levels of fear of OIT ( B = 0.145). Finally subjects with higher computer skills are less fearful ( B = − 114). In the full model the effect of the individual variables is not affected substantially with exception of gender which do not reaches statistical significance but maintains the same direction of the association ( B = 0.105, p = 0.086), and the levels of education which reaches significance ( B = 0.084) with fear of OIT.
Regarding perceived risk of OIT victimization, the first model explains 5.3% of the variance. Concretely, gender ( B = 0.107), age ( B = 0.009), and educational levels ( B = 0.009) are positively associated with perceived risk. Moreover, SES is negatively associated with risk perception ( B = − 0.158). Thus, females, older subjects, those with higher levels of education and low SES perceived more risk of being victims of OIT. The full model (model II) explains 8.6% of the variance. It is observed that financial routines ( B = 0.077), open dubious links ( B = 0.091), and avoiding behaviors ( B = 0.080) are positively related with perceived risk. Inversely, computer skills are negatively correlated ( B = − 0.131) with perceived risk. In the full model the effect of the individual variables is not affected substantially with exception of gender which loses the statistical significance.
The current study tested the risk factors or victimization, fear and perceived risk of online identity theft. Concretely, it tried to uncover how RAT and individual variables were important to the explanation of the above referred dependent variables. Using a sample of 831 college students and staff of the University of Porto, the results suggest that RAT can be partially applied, however, other predictors were stronger in explaining these dependent variables.
Concerning the first dependent variable of the present study—victimization—, it was possible to observe a prevalence of 20% of individuals who reported to be a victim of OIT. Taken into consideration that greater exposure to online activities could be associated with higher likelihood of OIT victimization, it could be expected that individuals who spend more time online would be more victimized. In the present study, we found that time spent online does not appear to impact the risk of being victim of OIT. Nevertheless, this result follows prior studies in different cybercrime victimizations (e.g., Ngo and Paternoster 2011 ; Reyns and Henson 2015 ; Holt and Bossler 2013 ; Ngo et al. 2020 ).
Although the model did not reach statistical significance, it was found that two forms of payment (credit card and paysafecard), reflecting online exposure, and one type of suitable target (visit risky contents) were related to victimization of OIT. Furthermore, none of the measures of capable guardianship explained victimization. Concretely, it was observed that those who did not use credit card as a form of payment had lower odds (34%) of becoming an OIT victim. This result shows that not using credit card might be seen as a protective factor and is consistent with the RAT since individuals who pay on-line and use this type of payment need to insert their credit card details. Therefore, they are more exposed to potential offenders who wish to illegally obtain financial information. Besides the implication of the need to use more secure forms of online payments (e.g., PayPal), this result is especially important since the COVID-19 pandemic had necessarily increased internet usage and online purchasing. Consequently, if no security measures are adopted, these shifts in online behaviors might be exploited by fraudsters and increase victimizations levels. Even though the data from this study was collected before the pandemic, multiple reports and studies showed that cybercrime increased during and after COVID-19 pandemic. Therefore, in future studies it would be relevant to understand the development of routine activities adopted by individuals and how these are influencing the levels of cybervictimization.
Contrary to prior studies such as Reyns and Henson ( 2015 ) and Burnes et al. ( 2020 ), banking and purchasing online did not contribute to an increased risk of OIT. When analyzing differences between men and women, a few explanations can be presented to understand this result. Firstly, men reported higher mean levels of financial routines ( X = 2.61) than women ( X = 2.32, p < 0.001). Moreover, men search for more information to protect themselves online and present higher perception of technical skills ( X = 2.21) than females ( X = 1.77, p < 0.001). Therefore, the fact they have more confident in their online skills might influence the relationship between financial activities and OIT victimization. Further research needs better address the relationship between this type of exposure, gender and increased likelihood of victimization of OIT.
Next, one dimension of target suitability in this study, namely visiting risky contents, had an impact in the increased likelihood of victimization. This outcome was expressive since the composite measure including ‘clicked on a pop-up message’ and ‘visited risky websites’ amplified the likelihood of becoming a victim of OIT in 34%. Therefore, risky behaviors such as visiting not very well-known websites might increase the likelihood of OIT victimization. Results concerning the impact of target suitability have been produced mixed-results. For instance, while Leukfeldt and Yar ( 2016 ) found that only one measure of online activity (targeted browsing) had an effect on OIT victimization, Ngo and Paternoster ( 2011 ) observed that click/open links decreased the likelihood of being a victim of computer virus. In Reyns and Henson’s ( 2015 ) study, only posting personal information online was significative in explaining OIT. Nevertheless, our finding shows that increasing technical skills and sensitization on the type of websites visited by individuals might be important to avoid OIT victimization.
Concerning the impact of sociodemographic variables, the only relevant variable was gender. Interestingly, and contrary to what was expecting, males were less likely (36%) than females of being victim of OIT. In fact, this results it at odds with what was found by Holt and Turner ( 2012 ) and Reyns ( 2013 ) which observed higher levels of victimization in males. In turn, Anderson ( 2006 ) observed that females had a 20% higher likelihood of being a victim of OIT and 50% of being a victim of other frauds when comparing to men. One possible explanation for our result is the fact that the higher perception of technical skills reported by men contributed to the decreased possibility of being victimized comparing to women. However, as it was previous mentioned, since the model did not reach statistical significance, it is necessary to analyze the data with precaution. In future studies, it would be important to include other factors that might contribute to the explanation of OIT victimization. For instance, one of the most relevant risk factors that may impact identity theft is the online deviance (Ngo and Paternoster 2011 ; Holt and Turner 2012 ). Previous studies showed that individuals who reported either malicious software infections (Boss and Holt 2009 ) or harassment (Holt and Bossler 2009 ) also reported participation in online deviance. A great deal of research is needed to expand our understanding of RAT, cybercrime and OIT victimization.
We now turn our attention to the results concerning fear and risk of OIT. Although online victimization has been extendedly tested, the same has not been observed in fear and risk perception of OIT. Research has been showing that higher levels of risk perception decrease the likelihood of online purchase intentions (Reisig et al. 2009 ). Moreover, fear of OIT is decreasing consumer trust and confidence in using Internet to conduct business (Roberts et al. 2013 ). Our results attribute partially support to RAT but contribute with novel insights to the understanding of fear and risk perception of OIT.
First, fear was not explained by previous victimization of OIT, confirming the existence of mixed results concerning the relationship between fear and victimization. Instead, and in accordance with Roberts et al. ( 2013 ) the strongest predictor of fear of OIT was general fear of crime. This finding means that individuals who score higher on general fear of crime will present greater fear online. One can argue that dispositional factors such as general fear might be more important than daily internet routines to the understanding of fear of OIT. Studying the relationship between fear of crime, dispositional fear and personality dimensions, Guedes et al. ( 2018 ) found a positive correlation between dispositional fear and fear of crime. This result is in accordance with Gabriel and Greve’s ( 2003 ) theoretical work who argued that fear of concrete situations is related with a more general tendency of experiencing fear. Interestingly, general fear of crime in our sample did not explain risk perception of OIT, suggesting that fear and risk are two different dimensions. Accordingly, while fear of crime is emotional in nature, risk perception is a cognition. In fact, fear of crime is not a perception of the environment, but instead an emotional reaction to the perceived environment (Warr 2000 ), involving a set of different emotions towards the possibility of victimization (Jackson and Gouseti 2012 ). On the other hand, perceived risk is usually conceptualized as a cognitive judgment or an estimate of the risk of victimization (e.g., Ferraro and LaGrange 1987 ). Even though the emotional fear of crime and perceived risk might be highly correlated (e.g., Mesch 2000 ), different predictors explain each type of the dimensions (e.g., Rountree and Land 1996 ; Guedes et al. 2018 ). Therefore, the current results might shed some light to the debate of the multidimensionality of fear of crime.
The second important result is related to the applicability of RAT in the explanation of both fear and risk perception. Concerning fear of OIT, none of the online exposure dimensions of RAT explained fear, as in Henson et al. ( 2013 ). In turn, subjects who reported more interactions with strangers were less fearful. This result can be explained by the fact that interacting with strangers, reflecting the target suitability, might be a routine of individuals who perceive less risk of victimization which, in turn, is positively correlated with fear. Given this intriguing result, more research is needed to understand the impact of target suitability on fear of OIT. Other finding was that those who reported to adopt more avoiding behaviors presented higher levels of fear. Concretely, avoiding online banking and shopping online were predictors of fear of OIT. It is plausible to affirm that these online guardianship dimensions are also proxy measures of the behavioral dimension of fear of crime which, in turn, is generally related either to emotional and cognitive dimensions of the fear of crime in a larger sense. Liska et al. ( 1988 ) observed that fear and constrained social behavior were part of a positive escalating loop, where fear constrained social behavior which, in turn, increased fear. It would be interesting to apply the same model to fear of OIT. Furthermore, as in Virtanen ( 2017 ), we found that subjects with higher computer skills were less fearful and perceived lower risk of victimization. According to Jackson ( 2004 ), worry about crime is related to the seriousness of the consequences of victimization, the likelihood of the event occurring and the ability to control its occurrence. Therefore, one can argue that augmented levels of perception of technical skills might increase the feelings of control over the risk of being victim of OIT. The confidence in individual’s own technical skills was also a predictor of risk perception of OIT, showing the importance of this variable in the present study. Under the applicability of RAT to perceived risk of victimization, it is also important to note that different predictors, comparing to fear, explained risk perception. In fact, one measure of online exposure (financial activities) is positively related to higher levels of perceived risk of victimization. Moreover, open dubious links increase perceived risk of OIT but not the fear of this type of crime. Therefore, these results shed light to the importance of implementing preventive actions and increased knowledge of the risks of online activities since, as it was previously mentioned, higher risk perception of OIT is related to lower levels of online purchase intentions.
Lastly, the present study also intended to analyze the impact of sociodemographic variables on both fear and risk perception of victimization online. Generally, the best predictor of fear of crime offline is gender (Hale 1996 ). However, in the final model of the present study, gender lost its significance in the explanation of fear of OIT. This result is in accordance with others such as Yu ( 2014 ), who observed no statistical significative differences between women and men for OIT, fraud and virus. Moreover, gender was not a predictor of fear of OIT in Roberts et al. ( 2013 ) research. Instead, our findings suggest that education and socioeconomic status are predictors not only of fear but also of risk of OIT. While more educated individuals presented higher levels of fear and risk perception, as in accordance with Akdemir ( 2020 ), lower socioeconomic status predicted both fear and risk of OIT, as in Virtanen ( 2017 ) and Brands and Wilsem ( 2019 ). This result suggests that those individuals are most affected by property-focused victimization and predict more difficulties in dealing with potential costs of that victimization.
Finally, it is important to acknowledge the limitations of the present study. Our data stems from a convenience sample of university population, that includes students and staff of the same educational environment, not allowing generalization to a larger population. While a more diverse and representative population would permit a more diversified experiences and contexts, the positive aspect is that they are a population that uses computer and internet daily. Moreover, previous studies have also utilized samples of college students (e.g., Bossler and Holt 2009 ; Holt and Bossler 2009 ).
Future research can expand to other types of online victimization and increase the list of online activities with the objective of identifying common and specific risk/protective factors associated with cybervictimization. Since in our study individual computer skills were an important predictor of fear and risk of OIT, we suggest a deeper exploration of this variable as a mediator between online exposure and victimization. Finally, qualitative studies on this topic will be very useful to explore the (in)security experiences of the individuals, their online routines, and subjective perception of the risks to which they are exposed and in what activities and contexts. Another important limitation of the present study is the fact that it only relies on individual online activities to understand the victimization of online identity theft. In future studies it would be important to measure if the identity theft victimization occurred due to a data breach which put in risk an individual’s personal/financial information stored by a company or a government. For instance, Burnes et al. ( 2020 ) showed that individuals reporting breached personal information from a company or government were more likely to experience multiple forms of identity theft.
Given the costs associated with cybercrime, and the increasing use and importance of the internet in our daily lives, the identification of risk and protective factors for cybervictimization and fear of cybercrime will be very relevant for prevention strategies.
Declarations
On behalf of all authors, the corresponding author states that there is no conflict of interest.
Publisher's Note
Springer Nature remains neutral with regard to jurisdictional claims in published maps and institutional affiliations.
Contributor Information
Inês Guedes, Email: tp.pu.otierid@sedeugi .
Margarida Martins, Email: [email protected] .
Carla Sofia Cardoso, Email: tp.pu.otierid@osodracc .
- Anderson KB. Who are the victims of identity theft? The effect of demographics. Journal of Public Policy and Marketing. 2006; 25 (2):160–171. doi: 10.1509/jppm.25.2.160. [ CrossRef ] [ Google Scholar ]
- Abdulai M. Examining the effect of victimization experience on fear of cybercrime: University student’s experience of credit/debit card fraud. International Journal of Cyber Criminology. 2020; 14 (1):157–1754. [ Google Scholar ]
- Akdemir N. Examining the impact of fear of cybercrime on internet users’ behavioral adaptations, privacy calculus and security intentions. International Journal of Eurasia Social Sciences. 2020; 11 (40):606–648. doi: 10.35826/ijoess.2737. [ CrossRef ] [ Google Scholar ]
- Alshalan A. Cyber-crime fear and victimization: An analysis of a national survey. Mississippi: Mississippi State University; 2006. [ Google Scholar ]
- Bellah J. Training: Identity theft. Law and Order. 2001; 49 (10):222–226. [ Google Scholar ]
- Bossler AM, Holt TJ. On-line activities, guardianship, and malware infection: An examination of routine activities theory. International Journal of Cyber Criminology. 2009; 3 (1):974–2891. [ Google Scholar ]
- Brands & Wilsem Connected and fearful? Exploring fear of online financial crime, Internet behaviour and their relationship. European Journal of Criminology. 2019; 00 :1–12. [ Google Scholar ]
- Burnes D, DeLiema M, Langton L. Risk and protective factors of identity theft victimization in the United States. Preventive Medicine Reports. 2020; 17 :1–8. doi: 10.1016/j.pmedr.2020.101058. [ PMC free article ] [ PubMed ] [ CrossRef ] [ Google Scholar ]
- Capeller W. Not such a neat net: Some comments on virtual criminality. Social & Legal Studies. 2001; 10 (2):229–242. doi: 10.1177/a017404. [ CrossRef ] [ Google Scholar ]
- Choi K. An empirical assessment of an integrated theory of computer crime victimisation. International Journal of Cyber Criminology. 2008; 2 (1):308–333. [ Google Scholar ]
- Cohen LE, Felson M. Social change and crime rate trends: A routine activity approach. American Sociological Review. 1979; 44 :588–608. doi: 10.2307/2094589. [ CrossRef ] [ Google Scholar ]
- Copes, H., and L. Vieraitis, L. 2012. identity theft. In The Oxford handbook of crime and public policy , ed. M. Tonry. 10.1093/oxfordhb/9780199844654.001.0001
- Eck JE, Clarke RV. classifying common police problems: A routine activity approach. Crime Prevention Studies. 2003; 16 :7–39. [ Google Scholar ]
- Ferraro K, LaGrange R. The measurement of fear of crime. Sociological Inquiry. 1987; 57 (1):70–97. doi: 10.1111/j.1475-682X.1987.tb01181.x. [ CrossRef ] [ Google Scholar ]
- Gabriel U, Greve W. The psychology of fear of crime: Conceptual and methodological Perspectives. British Journal of Criminology. 2003; 43 (1):600–614. doi: 10.1093/bjc/43.3.600. [ CrossRef ] [ Google Scholar ]
- Garrison CP, Ncube M. A longitudinal analysis of data breaches. Information Management & Computer Security. 2011; 19 (4):216–230. doi: 10.1108/09685221111173049. [ CrossRef ] [ Google Scholar ]
- Golladay K, Holtfreter K. The consequences of identity theft victimization: An examination of emotional and physical health outcomes. Victims & Offenders. 2017; 12 (5):741–760. doi: 10.1080/15564886.2016.1177766. [ CrossRef ] [ Google Scholar ]
- Grabosky P. Virtual criminality: Old wine in new bottles? Social & Legal Studies. 2001; 10 (2):243–249. doi: 10.1177/a017405. [ CrossRef ] [ Google Scholar ]
- Guedes I, Domingos S, Cardoso C. Fear of crime, personality and trait emotions: An empirical study. European Journal of Criminology. 2018; 15 (6):658–679. doi: 10.1177/1477370817749500. [ CrossRef ] [ Google Scholar ]
- Hale C. Fear of crime: A review of the literature. International Review of Victimology. 1996; 4 :79–150. doi: 10.1177/026975809600400201. [ CrossRef ] [ Google Scholar ]
- Harrell E, Langton L. Victims of identity theft, 2012 (NCJ 243779) Washington: Bureau of Justice Statistics; 2013. [ Google Scholar ]
- Harrell, E. 2015. Victims of identity theft , 2014, Bureau of Justice Statistics, NCJ 248991.
- Harrell, E. 2019. Victims of identity theft , 2016: Bulletin.
- Henson B, Reyns BW, Fisher BS. Fear of crime online? Examining the effect of risk, previous victimization, and exposure on fear of online interpersonal victimization. Journal of Contemporary Criminal Justice. 2013; 29 (4):475–497. doi: 10.1177/1043986213507403. [ CrossRef ] [ Google Scholar ]
- Hille P, Walsh G, Cleveland M. Consumer fear of online identity theft: Scale development and validation. Journal of Interactive Marketing. 2015; 30 :1–19. doi: 10.1016/j.intmar.2014.10.001. [ CrossRef ] [ Google Scholar ]
- Holt TJ, Bossler AM. Examining the applicability of lifestyle-routine activities theory for cybercrime victimization. Deviant Behavior. 2009; 30 (1):1–25. doi: 10.1080/01639620701876577. [ CrossRef ] [ Google Scholar ]
- Holt TJ, Bossler AM. Examining the relationship between routine activities and malware infection indicators. Journal of Contemporary Criminal Justice. 2013; 29 (4):420–436. doi: 10.1177/1043986213507401. [ CrossRef ] [ Google Scholar ]
- Holt TJ, Turner M. Examining risks and protective factors of on-line identity theft. Deviant Behavior. 2012; 33 (4):308–323. doi: 10.1080/01639625.2011.584050. [ CrossRef ] [ Google Scholar ]
- Holt TJ, van Wilsem J, van de Weijer S, Leukfeldt R. Testing an integrated self-control and routine activities framework to examine malware infection victimization. Social Science Computer Review. 2020; 38 (2):187–206. doi: 10.1177/0894439318805067. [ CrossRef ] [ Google Scholar ]
- Jackson J. Experience and expression: Social and cultural significance in the fear of crime. British Journal of Criminology. 2004; 44 (6):946–966. doi: 10.1093/bjc/azh048. [ CrossRef ] [ Google Scholar ]
- Jackson, J., and I. Gouseti (eds). 2012. Fear of crime. In The encyclopedia of theoretical criminology . Hoboken, NJ: Wiley-Blackwell.
- Jordan G, Leskovar R, Marič M. Impact of fear of identity theft and perceived risk on online purchase intention. Organizacija. 2018; 51 (2):146–155. doi: 10.2478/orga-2018-0007. [ CrossRef ] [ Google Scholar ]
- Keane C. Fear of crime in Canada: An examination of concrete and formeless fear of victimization. Canadian Journal of Criminology. 1992; 34 (2):215–224. doi: 10.3138/cjcrim.34.2.215. [ CrossRef ] [ Google Scholar ]
- Koops BJ, Leenes RE. ID theft, ID fraud and/or ID-related crime-definitions matter. Datenschutz Und Datensicherheit. 2006; 30 (9):553–556. doi: 10.1007/s11623-006-0141-2. [ CrossRef ] [ Google Scholar ]
- Lee S, Choi K, Choi S, Englander E. A test of structural model for fear of crime in social networking sites. International Journal of Cybersecurity Intelligence Cybercrime. 2019; 2 (2):5–22. doi: 10.52306/02020219SVZL9707. [ CrossRef ] [ Google Scholar ]
- Leukfeldt ER, Yar M. Applying routine activity theory to cybercrime: A theoretical and empirical analysis. Deviant Behavior. 2016; 37 (3):263–280. doi: 10.1080/01639625.2015.1012409. [ CrossRef ] [ Google Scholar ]
- Li Y, Yazdanmehr A, Wang J, Rao HR. Responding to identity theft: A victimization perspective. Decision Support Systems. 2019; 121 :13–24. doi: 10.1016/j.dss.2019.04.002. [ CrossRef ] [ Google Scholar ]
- Liska AE, Sanchirico A, Reed MD. Fear of crime and constrained behavior: Specifying and estimating a reciprocal effects model. Social Forces. 1988; 66 (3):827–837. doi: 10.2307/2579577. [ CrossRef ] [ Google Scholar ]
- Marcum C, Higgins G, Ricketts M. Potential factors of online victimization of youth: An examination of adolescent online behaviors utilizing routine activity theory. Deviant Behavior. 2010; 31 (5):381–410. doi: 10.1080/01639620903004903. [ CrossRef ] [ Google Scholar ]
- Martin KD, Borah A, Palmatier RW. Data privacy: Effects on customer and firm performance. Journal of Marketing. 2017; 81 (1):36–58. doi: 10.1509/jm.15.0497. [ CrossRef ] [ Google Scholar ]
- Mesch GS. Perceptions of risk, lifestyle activities, and fear of crime. Deviant Behavior. 2000; 21 (1):47–62. doi: 10.1080/016396200266379. [ CrossRef ] [ Google Scholar ]
- McNeeley S. Lifestyle-routine activities and crime events. Journal of Contemporary Criminal Justice. 2015; 31 (1):30–52. doi: 10.1177/1043986214552607. [ CrossRef ] [ Google Scholar ]
- Ngo F, Paternoster R. Cybercrime victimization: An examination of individual and situational level factors. International Journal of Cyber Criminology. 2011; 5 (1):773–793. [ Google Scholar ]
- Ngo F, Piquero A, LaPrade J, Duong B. Victimization in cyberspace: Is it how long we spend online, what we do online, or what we post online? Criminal Justice Review. 2020; 45 (4):430–451. doi: 10.1177/0734016820934175. [ CrossRef ] [ Google Scholar ]
- Piquero NL. White-collar crime is crime: Victims hurt just the same. Criminology & Pub. Pol'y. 2018; 17 :595. doi: 10.1111/1745-9133.12384. [ CrossRef ] [ Google Scholar ]
- Pratt TC, Holtfreter K, Reisig MD. Routine online activity and Internet fraud targeting: Extending the generality of routine activity theory. Journal of Research in Crime and Delinquency. 2010; 47 :267–296. doi: 10.1177/0022427810365903. [ CrossRef ] [ Google Scholar ]
- Rader N, May D, Goodrum S. An empirical assessment of the ‘threat of victimization’: Considering fear of crime, perceived risk, avoidance, and defensive behaviors. Sociological Spectrum: MId-Shouth Sociological Association. 2007; 27 (5):475–505. doi: 10.1080/02732170701434591. [ CrossRef ] [ Google Scholar ]
- Randa R. The influence of the cyber-social environment on fear of victimization: Cyber bullying and school. Security Journal. 2013; 26 :331–348. doi: 10.1057/sj.2013.22. [ CrossRef ] [ Google Scholar ]
- Reid LW, Konrad M. The gender gap in fear of crime: Assessing the interactive effects of gender and perceived risk on fear of crime. Sociological Spectrum. 2004; 24 (4):399–425. doi: 10.1080/02732170490431331. [ CrossRef ] [ Google Scholar ]
- Reisig MD, Pratt TC, Holtfreter K. Perceived risk of internet theft victimisation: Examining the effects of social vulnerability and financial impulsivity. Criminal Justice and Behavior. 2009; 36 (4):369–384. doi: 10.1177/0093854808329405. [ CrossRef ] [ Google Scholar ]
- Reyns BW. Online routines and identity theft victimization: Further expanding routine activity theory beyond direct-contact offenses. Journal of Research in Crime and Delinquency. 2013; 50 (2):216–238. doi: 10.1177/0022427811425539. [ CrossRef ] [ Google Scholar ]
- Reyns BW. A routine activity perspective on online victimisation: Results from the Canadian General Social Survey. Journal of Financial Crime. 2015; 22 (4):396–411. doi: 10.1108/JFC-06-2014-0030. [ CrossRef ] [ Google Scholar ]
- Reyns BW, Henson B. The thief with a thousand faces and the victim with none: Identifying determinants for online identity theft victimization with routine activity theory. International Journal of Offender Therapy and Comparative Criminology. 2015; 60 (10):1119–1139. doi: 10.1177/0306624X15572861. [ PubMed ] [ CrossRef ] [ Google Scholar ]
- Reyns BW, Henson B, Fisher BS. Being pursued online: Applying cyberlifestyle–routine activities theory to cyberstalking victimization. Criminal Justice and Behavior. 2011; 38 :1149–1169. doi: 10.1177/0093854811421448. [ CrossRef ] [ Google Scholar ]
- Reyns BW, Randa R. Victim reporting behaviors following identity theft victimization: Results from the National Crime Victimization Survey. Crime & Delinquency. 2017; 63 (7):814–838. doi: 10.1177/0011128715620428. [ CrossRef ] [ Google Scholar ]
- Roberts LD, Indermaur D, Spiranovic C. Fear of cyber-identity theft and related fraudulent activity. Psychiatry, Psychology, & Law. 2013; 20 :315–328. doi: 10.1080/13218719.2012.672275. [ CrossRef ] [ Google Scholar ]
- Rountree W, Land K. Perceived risk versus fear of crime: Empirical evidence of conceptually distinct reactions in survey data. Social Forces. 1996; 74 (4):1353–1376. doi: 10.2307/2580354. [ CrossRef ] [ Google Scholar ]
- Skogan W, Maxfield M. Coping with crime: Individual and neighborhood reactions. Beverly Hills: Sage; 1981. [ Google Scholar ]
- Smith LN, Hill GD. Perceptions of crime seriousness and fear of crime. Sociological Focus. 1991; 24 (4):315–327. doi: 10.1080/00380237.1991.10570598. [ CrossRef ] [ Google Scholar ]
- Tatham, M. 2018. “Identity Theft Statistics.” Experian. https://www.experian.com/blogs/ask-experian/identity-theft-statistics/ .
- Tseloni A, Zarafonitou C. Fear of crime and victimization: A multivariate analyses of competing measurements. European Journal of Criminology. 2008; 5 (4):387–409. doi: 10.1177/1477370808095123. [ CrossRef ] [ Google Scholar ]
- Van Wilsem J. Hacking and harassment—Do they have something in common? Comparing risk factors for online victimization. Journal of Contemporary Criminal Justice. 2013; 29 (4):437–453. doi: 10.1177/1043986213507402. [ CrossRef ] [ Google Scholar ]
- Virtanen S. Fear of cybercrime in Europe: Examining the effects of victimization and vulnerabilities. Psychiatry, Psychology and Law. 2017; 24 (3):323–338. doi: 10.1080/13218719.2017.1315785. [ PMC free article ] [ PubMed ] [ CrossRef ] [ Google Scholar ]
- Wang W, Yuan Y, Archer N. A Contextual framework for combating identity theft. IEEE Security and Privacy. 2006; 4 (2):30–38. doi: 10.1109/MSP.2006.31. [ CrossRef ] [ Google Scholar ]
- Warr M. Fear of victimization: Why are women and the elderly more afraid? Social Science Quarterly. 1984; 65 (6):81–702. [ Google Scholar ]
- Warr M. Fear of crime in the United States: Avenues for research and policy. Measurement and Analysis of Crime and Justice. 2000; 4 :451–489. [ Google Scholar ]
- Williams M. Guardians upon high: An application of routine activities theory to online identity theft in Europe at the country and individual level. British Journal of Criminology. 2016; 56 :21–48. doi: 10.1093/bjc/azv011. [ CrossRef ] [ Google Scholar ]
- Yar M. The Novelty of ‘Cybercrime’: An Assessment in Light of Routine Activity Theory. European Journal of Criminology. 2005; 2 (4):407–427. doi: 10.1177/147737080556056. [ CrossRef ] [ Google Scholar ]
- Yu S. Fear of cybercrime among college students in the United States: An exploratory study. International Journal of Cyber Criminology. 2014; 8 (1):36. [ Google Scholar ]
- Ziegler R, Mitchell D. Aging and fear of crime: An experimental approach to an apparent paradox. Experimental Aging Research. 2003; 29 (2):173–187. doi: 10.1080/03610730303716. [ PubMed ] [ CrossRef ] [ Google Scholar ]

An official website of the United States government
Here’s how you know
The .gov means it’s official. Federal government websites often end in .gov or .mil. Before sharing sensitive information, make sure you’re on a federal government site.
The site is secure. The https:// ensures that you are connecting to the official website and that any information you provide is encrypted and transmitted securely.
View all Consumer Alerts
Get Consumer Alerts
Credit, Loans, and Debt
Learn about getting and using credit, borrowing money, and managing debt.
View Credit, Loans, and Debt
Jobs and Making Money
What to know when you're looking for a job or more education, or considering a money-making opportunity or investment.
View Jobs and Making Money
Unwanted Calls, Emails, and Texts
What to do about unwanted calls, emails, and text messages that can be annoying, might be illegal, and are probably scams.
View Unwanted Calls, Emails, and Texts
Identity Theft and Online Security
How to protect your personal information and privacy, stay safe online, and help your kids do the same.
View Identity Theft and Online Security
- Search Show/hide Search menu items Items per page 20 50 100 Filters Fulltext search
What to do when someone steals your identity
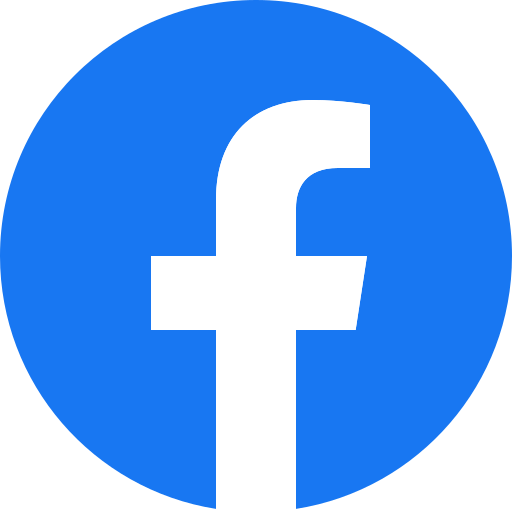
Did someone use your personal information to open up a new mobile account or credit card? Or maybe buy stuff with one of your existing accounts? Or did they file for unemployment or taxes in your name? That’s identity theft.
If any of this happened to you, the FTC wants to help you stop the damage and start recovering. Learn more by watching this video:
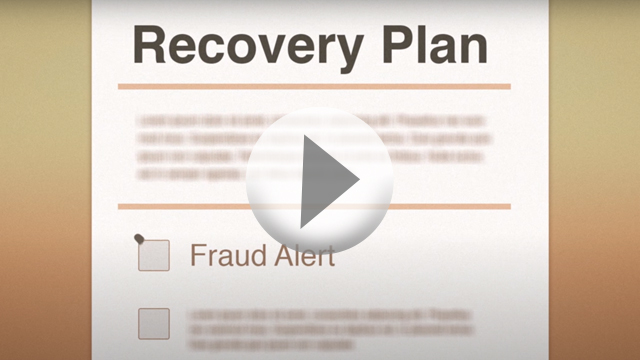
Not sure whether someone has stolen your identity? Check out these clues that someone is using your information . If your information has been compromised, find the next steps to take . But all roads lead to IdentityTheft.gov , so start there to get your recovery plan.
Read Our Privacy Act Statement
It is your choice whether to submit a comment. If you do, you must create a user name, or we will not post your comment. The Federal Trade Commission Act authorizes this information collection for purposes of managing online comments. Comments and user names are part of the Federal Trade Commission’s (FTC) public records system, and user names also are part of the FTC’s computer user records system. We may routinely use these records as described in the FTC’s Privacy Act system notices . For more information on how the FTC handles information that we collect, please read our privacy policy .
Read Our Comment Policy
The purpose of this blog and its comments section is to inform readers about Federal Trade Commission activity, and share information to help them avoid, report, and recover from fraud, scams, and bad business practices. Your thoughts, ideas, and concerns are welcome, and we encourage comments. But keep in mind, this is a moderated blog. We review all comments before they are posted, and we won’t post comments that don’t comply with our commenting policy. We expect commenters to treat each other and the blog writers with respect.
- We won’t post off-topic comments, repeated identical comments, or comments that include sales pitches or promotions.
- We won’t post comments that include vulgar messages, personal attacks by name, or offensive terms that target specific people or groups.
- We won’t post threats, defamatory statements, or suggestions or encouragement of illegal activity.
- We won’t post comments that include personal information, like Social Security numbers, account numbers, home addresses, and email addresses. To file a detailed report about a scam, go to ReportFraud.ftc.gov.
We don't edit comments to remove objectionable content, so please ensure that your comment contains none of the above. The comments posted on this blog become part of the public domain. To protect your privacy and the privacy of other people, please do not include personal information. Opinions in comments that appear in this blog belong to the individuals who expressed them. They do not belong to or represent views of the Federal Trade Commission.
Laini September 25, 2020 I've had identify theft about 15 years ago so I couldn't even file my taxes without having to jump through hoops,to this day I think it's still being used.
Bibi 07 September 25, 2020 July 2020, I placed a 12 month fraud alert w EXPERIAN who informed me they are notifying the 2 other bureaus who will send me directly a confirmation. But I only received 1 from TRANSUNION, none from EQUIFAX. I sent a letter to EQUIFAX mid Aug but did NOT hear from them as of today- almost 6 weeks- i tried to call = it appears the phone lines are busy.. a robot has a long menu of questions, and says : all out associates are busy... pl. wait for x minutes ( up to 30 minutes) or,, hung up . I also noticed if ever one is available, most are not nice: I asked for her name and she said you do not need it, just answer my questions... or " do it on line" or hung up-- MY QUESTION: How often I have to follow up with Equifax and why we only have those 3 ? Many of my friends , some are senior citizens, had the same problems and are AFRAID to call one of these 3, bec some in the staff are not customer- oriented- 2 even cried after they said they were insulted.. sad to see.
In reply to July 2020, I placed a 12 by Bibi 07
The credit bureau you contact to place the fraud alert is required to contact the other two bureaus. Experian is required to contact Equifax and TransUnion.
Although you did not get confirmation from Equifax, it should have gotten a notice from Experian. You don't need to keep asking Equifax to send confirmation.
Joseph A Di Enno September 25, 2020 Yeah, I know what it's like to have your identity stolen. I am still going through it. The person that stole my identity seems to be one step ahead of me. They stole it before 2007 and had a license, again in 2012 here we are 2020. I messed up and did not complete the recovery plan.
swipeidea September 30, 2020 If someone uses your personal information this is a big crime. But nowadays using other personal information to open up a new mobile account or virtual credit card generate occurs frequently.
Harry M October 01, 2020 When the unemployment compensation program first started I was had someone apply in my name without my knowledge. He has managed to get hold of some personal info like SSN and I think he stole the BOA credit card they sent me. I filled out a fraud report but am frustrated at every turn in finding out anything at all about the case, if there is one. I even gave them a name. What can I do. I have called every number and got the run around -
In reply to When the unemployment by Harry M
Did you report this at www.IdentityTheft.gov? That is the federal government website for reporting identity theft and theft of unemployment benefits. You can create an Identity Theft Report and send copies of the Report to businesses, like credit card companies, where a thief opened an account in your name.
You can also put a credit freeze on your credit report. A credit freeze makes it harder for someone to get access to your credit report and open new credit. This FTC article tells more about a credit freeze and how to place a credit freeze.
Shehryar Joiya October 08, 2020 keep sharing this type of informative content.I actually like the content very much.This is a very informative content. b it.ly/ 3jF2 2 mI
Ann in AZ October 12, 2020 My grandson is a victim of ID theft. He accessed his credit report to see what he needed to do to begin building credit in anticipation of buying a house. He was horrified that there were multiple collections accounts on his file. The file had 4 or 5 alias names. This is a 23 year old young man who has never applied for credit. His credit file was established when he was only 13 years old. I’m helping him dispute all of the incorrect information, but it is an intimidating and overwhelming process. Wish him luck as we move through this process.
cottonm39 October 20, 2020 My local social security office today me I have to send my ID they said know copies or anything and I have not gotten my ID Back yet.
JTAR October 16, 2020 Someone applied for a US SBA loan and Ohio unemployment compensation with my SS. I discovered this when Discover/Experian notified me and, a few days later, when I received a USPS letter from ODJFS with my 'new' PIN number. (I never had a PIN number, because I never applied for unemployment.) Anyhow, I placed a fraud alert and freeze with all 3 credit-reporting agencies, filed a police report locally, and completed the online FTC forms on Sep 29. Everyone except the FTC followed up with e-mails and a USPS letter of verification. Why didn't the FTC?
In reply to Someone applied for a US SBA by JTAR
If you report a problem to www.FTC.gov/Complaint and you give your email, you should get an email response.
If somoene used your Social Security number to get benefits, you should file an identity theft report at www.IdentityTheft.gov. There is a special link on the first page for people to report unemployment benefits identity theft. You can create an FTC Identity Theft Report and use that to correct problems caused by the theft. You can enter information that goes into a secure online database that is shared with law enforcement, and create an account that allows you to come back and add details about the theft and get information about other steps to take.
Crossout@1 February 17, 2021 I had my identity hacked now I can’t file my taxes because I can’t verify my identity when all the information is correct
GloBugHouse240 April 08, 2021 I am have a hard time with my background check they my driver's license is not in my name and my address is wrong on my driver's license
Don't use mary… June 07, 2021 I was with this guy that isn’t a legal citizen and he stole my identity and I want something done about it to him

An official website of the United States government
Here’s how you know
The .gov means it’s official. Federal government websites often end in .gov or .mil. Before sharing sensitive information, make sure you’re on a federal government site.
The site is secure. The https:// ensures that you are connecting to the official website and that any information you provide is encrypted and transmitted securely.
Take action
- Report an antitrust violation
- File adjudicative documents
- Find banned debt collectors
- View competition guidance
- Competition Matters Blog
New HSR thresholds and filing fees for 2024
View all Competition Matters Blog posts
We work to advance government policies that protect consumers and promote competition.
View Policy
Search or browse the Legal Library
Find legal resources and guidance to understand your business responsibilities and comply with the law.
Browse legal resources
- Find policy statements
- Submit a public comment
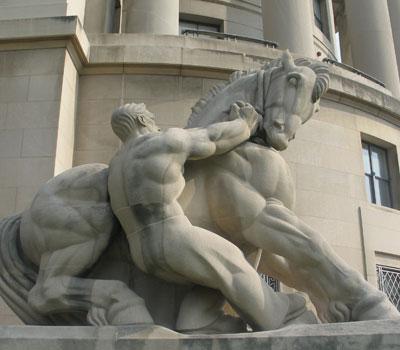
Vision and Priorities
Memo from Chair Lina M. Khan to commission staff and commissioners regarding the vision and priorities for the FTC.
Technology Blog
Approaches to address ai-enabled voice cloning.
View all Technology Blog posts
Advice and Guidance
Learn more about your rights as a consumer and how to spot and avoid scams. Find the resources you need to understand how consumer protection law impacts your business.
- Report fraud
- Report identity theft
- Register for Do Not Call
- Sign up for consumer alerts
- Get Business Blog updates
- Get your free credit report
- Find refund cases
- Order bulk publications
- Consumer Advice
- Shopping and Donating
- Credit, Loans, and Debt
- Jobs and Making Money
- Unwanted Calls, Emails, and Texts
- Identity Theft and Online Security
- Business Guidance
- Advertising and Marketing
- Credit and Finance
- Privacy and Security
- By Industry
- For Small Businesses
- Browse Business Guidance Resources
- Business Blog
Servicemembers: Your tool for financial readiness
Visit militaryconsumer.gov
Get consumer protection basics, plain and simple
Visit consumer.gov
Learn how the FTC protects free enterprise and consumers
Visit Competition Counts
Looking for competition guidance?
- Competition Guidance
News and Events
Latest news, ftc issues report to congress on collaboration with state attorneys general.
View News and Events
Upcoming Event
Chair khan speaking at 2024 aba spring meeting enforcers roundtable.
View more Events
Sign up for the latest news
Follow us on social media
--> --> --> --> -->
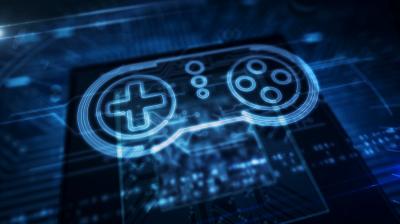
Playing it Safe: Explore the FTC's Top Video Game Cases
Learn about the FTC's notable video game cases and what our agency is doing to keep the public safe.
Latest Data Visualization
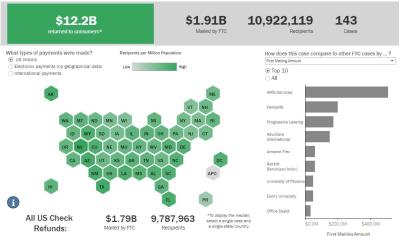
FTC Refunds to Consumers
Explore refund statistics including where refunds were sent and the dollar amounts refunded with this visualization.
About the FTC
Our mission is protecting the public from deceptive or unfair business practices and from unfair methods of competition through law enforcement, advocacy, research, and education.
Learn more about the FTC
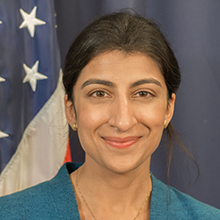
Meet the Chair
Lina M. Khan was sworn in as Chair of the Federal Trade Commission on June 15, 2021.
Chair Lina M. Khan
Looking for legal documents or records? Search the Legal Library instead.
- Cases and Proceedings
- Premerger Notification Program
- Merger Review
- Anticompetitive Practices
- Competition and Consumer Protection Guidance Documents
- Warning Letters
- Consumer Sentinel Network
- Criminal Liaison Unit
- FTC Refund Programs
- Notices of Penalty Offenses
- Advocacy and Research
- Advisory Opinions
- Cooperation Agreements
- Federal Register Notices
- Public Comments
- Policy Statements
- International
- Military Consumer
- Consumer.gov
- Bulk Publications
- Data and Visualizations
- Stay Connected
- Commissioners and Staff
- Bureaus and Offices
- Budget and Strategy
- Office of Inspector General
- Careers at the FTC
FTC Sends $1.2 Million in Refunds to Consumers Harmed by Deceptive Investment Claims
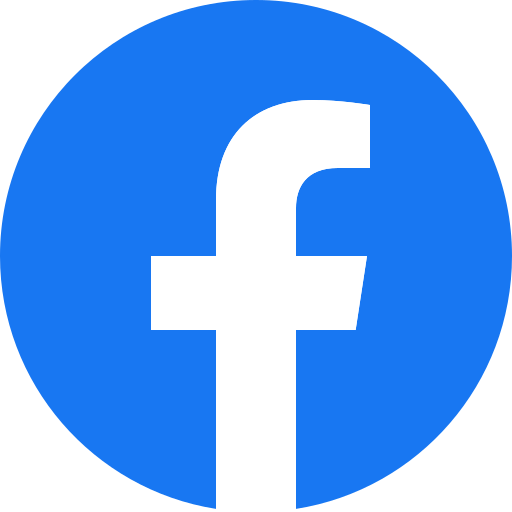
- Consumer Protection
- Bureau of Consumer Protection
The Federal Trade Commission is sending $1.2 million in refunds to consumers who paid for the advice of supposed experts based on deceptive claims of substantial investment profits.
The FTC sued Wealthpress in January 2023 along with two of its owners, Roger Scott and Conor Lynch, alleging that the company used deceptive claims of likely profits to sell consumers investment advising services—often touting that the services’ recommendations were based on a specific “algorithm” or “strategy” created by a purported expert. The company charged consumers hundreds or even thousands of dollars for access to these services but could not show that services they offered purchasers were likely to reap substantial profits. Indeed, many consumers lost substantial amounts of money in attempting to follow the services’ advice.
The defendants in the case agreed to a settlement that required them to pay more than $1.2 million in monetary relief along with $500,000 in civil penalties. The settlement also prohibits them from making any claims about earnings without having written evidence to back those claims up.
The FTC is sending payments to 19,857 consumers. Most consumers will get a check in the mail. Recipients should cash their checks within 90 days, as indicated on the check. Eligible consumers who did not have an address on file will receive a PayPal payment, which should be redeemed within 30 days.
Consumers who have questions about their payment should contact the refund administrator, JND Legal Administration, at 877-231-0641 or visit the FTC website to view frequently asked questions about the refund process. The Commission never requires people to pay money or provide account information to get a refund.
The Commission’s interactive dashboards for refund data provide a state-by-state breakdown of refunds in FTC cases. In 2023, FTC actions led to $324 million in refunds to consumers across the country.
The Federal Trade Commission works to promote competition and to protect and educate consumers . The FTC will never demand money, make threats, tell you to transfer money, or promise you a prize. You can learn more about consumer topics and report scams, fraud, and bad business practices online at ReportFraud.ftc.gov . Like the FTC on Facebook , follow us on Twitter , get consumer alerts , read our blogs , and subscribe to press releases for the latest FTC news and resources.
Press Release Reference
Contact information, media contact.
Jay Mayfield Office of Public Affairs 202-326-2656
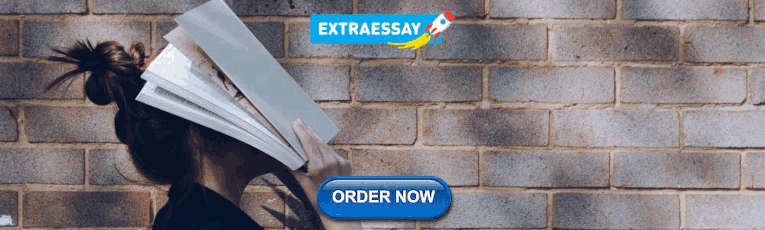
IMAGES
VIDEO
COMMENTS
Case Study #3: Facebook Security Scam. While the first two examples were intended as (relatively) harmless pranks, this next instance of social media fraud was specifically designed to separate social media users from their money. In 2012, a scam involving Facebook developed as an attempt to use social media to steal financial information from ...
Identity thieves know this and look for ways — both high-tech, like lifting our passwords, or low-tech, like stealing our mail — to get their hands on our money and personal information. Since identity theft can happen to anyone, here are some ways to keep your money and personal information safe. Protect documents that have personal ...
Phishing Scams and How to Spot Them. Phishing is a type of online scam that targets consumers by sending them an e-mail that appears to be from a well-known source - an internet service provider, a bank, or a mortgage company, for example. It asks the consumer to provide personal identifying information. Then a scammer uses the information to ...
This paper explores the extent and nature of this problem. Using data from depth interviews with 15 online fraud victims, 6 focus groups with a further 48 online fraud victims and interviews with 9 professional stakeholders involved in combating this problem. The paper explores why victims fall for online scams.
The Federal Trade Commission received more than 2.1 million fraud reports from consumers in 2020, according to newly released data, with imposter scams remaining the most common type of fraud reported to the agency. Online shopping was the second-most common fraud category reported by consumers, elevated by a surge of reports in the early days ...
Spam, online scams and frauds, identity theft and issues related to online purchases are a serious issue in the online world. Navigating the Web while avoiding these threats can be a challenging task. ... to obtain legal documents such as a driver's licence, health care, social insurance number and passport. This was the case for Gerber Guzman ...
The present study aims at understanding what factors contribute to the explanation of online identity theft (OIT) victimization and fear, using the Routine Activity Theory (RAT). Additionally, it tries to uncover the influence of factors such as sociodemographic variables, offline fear of crime, and computer perception skills. Data for the present study were collected from a self-reported ...
Question: CASE STUDY: WHAT THE? — Online scams and identity Theft Transcript "Well, I downloaded a lot of stuff to my computer—heaps of stuff actually: music, programs, and games. I guess in the back of my mind I've always been worried about security but I didn't think I could do anything about it, or anything that bad could happen to me.
Common Types of Fraud and Scam: IDENTITY THEFT A scammer assumes your identity and uses your personal information, bank account or credit card details to make unauthorized transactions and purchases, whether online or in person. They usually obtain a victim's personal information by posing as someone from an authority
March 7, 2024. When you log into your bank or credit card account, you might get a text message or email with a verification code. You then enter it at the login screen to confirm it's really you. That's a form of two-factor authentication that adds a layer of security to your account — and keeps would-be scammers and hackers out. The ...
Here are the 7 most common scams online stores are vulnerable to. 1. Email Account Phishing. Most people are familiar with email phishing scams, so let's start there. These are as old as the internet itself, so many of you have likely already opened an email from a stranger asking for sensitive account information.
And you also know that taking steps to avoid identity theft can make a big difference. But if someone steals your identity, here's the first thing you do: go to IdentityTheft.gov. To get you started, watch this video on how IdentityTheft.gov helps you report and recover from identity theft. If your identity is still all yours, you can find ...
This paper offers an overview of romance scams and identity fraud at dating sites, developing a typology of cyber criminals and analyzing each type along the dimensions of criminal techniques ...
They are the most vulnerable demographic to ID theft. Once there is a fraud attempt on any family member, or someone close to you who has been a victim, take urgent measures to secure your account. 11. Nakeisha Hall - the IRS Staff. Many identity theft cases involve the IRS.
Identity theft: I lost $6028 when scammers stole my identity. Scammers can gain access to your private information through unlocked mailboxes or discarded personal documents such as utility bills, insurance renewals or health care records. The ACCC acknowledges the Traditional Custodians of the lands across Australia on which we live and work.
Last year ID theft happened more than 223,000 times, up 18% on the year before, according to Cifas, the counter fraud organisation which runs the National Fraud Database and works with police and ...
In this paper, researchers have examined. online fraud cases through web applications and how fraudulent websites affect financial lo ss. Researchers also highlighted. the current situation ...
A text scam, meanwhile, is a form of phishing attack launched using mobile messages. A successful phishing attack can lead to identity theft, which is among Filipinos' primary concerns as well.
Abstract. Identity theft victimization is associated with serious physical and mental health morbidities. The problem is expanding as society becomes increasingly reliant on technology to store and transfer personally identifying information. Guided by lifestyle-routine activity theory, this study sought to identify risk and protective factors ...
Commonwealth of Australia. 3 Introduction The focus of this case study is how to protect yourself from online scams and identity theft. Students can undertake this activity by reading the handout and case study transcript, or in conjunction with viewing the video on the CyberNetrix CD-ROM. Prior learning Students need to have knowledge of ...
Online identity theft. Identity theft is a term used to classify numerous offenses including fraudulent use of personal information for criminal purposes without individuals' consent (Reyns 2013).Harrell (2015, p.2) defines identity theft as the "unauthorized use or attempted use of an existing account (such as credit/debit card, savings, telephone, online), the unauthorized use or ...
The credit bureau you contact to place the fraud alert is required to contact the other two bureaus. Experian is required to contact Equifax and TransUnion. Although you did not get confirmation from Equifax, it should have gotten a notice from Experian. You don't need to keep asking Equifax to send confirmation.
Identity theft refers to crimes where an individual's personal, financial, or medical information is stolen and misused. Victims of identity theft can suffer significant monetary loss, as was the case with the financial fraud committed by Bernie Madoff, costing victims an estimated $50 billion. Trust in institutions like banks, hospitals, and ...
Cybercrime, encompassing activities such as identity theft, cyberbullying, and fraud, has had a significant impact on individuals and their communities.. The potential threat of cybercrime has created a sense of vulnerability and fear among individuals.Trust in online platforms and communication channels has been eroded, impacting both personal and professional interactions.
The defendants in the case agreed to a settlement that required them to pay more than $1.2 million in monetary relief along with $500,000 in civil penalties. The settlement also prohibits them from making any claims about earnings without having written evidence to back those claims up. The FTC is sending payments to 19,857 consumers.