
An official website of the United States government
The .gov means it’s official. Federal government websites often end in .gov or .mil. Before sharing sensitive information, make sure you’re on a federal government site.
The site is secure. The https:// ensures that you are connecting to the official website and that any information you provide is encrypted and transmitted securely.
- Publications
- Account settings
Preview improvements coming to the PMC website in October 2024. Learn More or Try it out now .
- Advanced Search
- Journal List
- Elsevier - PMC COVID-19 Collection

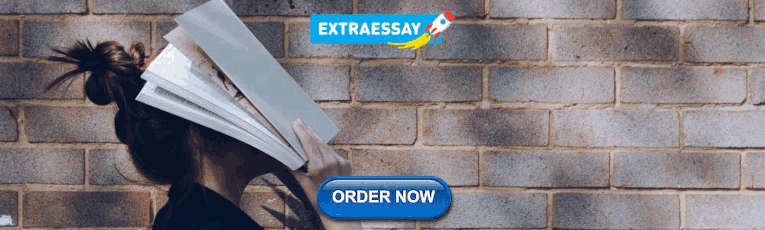
Analysis of the COVID-19 impacts on employment and unemployment across the multi-dimensional social disadvantaged areas
This is the study of economic impacts in the context of social disadvantage. It specifically considers economic conditions in regions with pre-existing inequalities and examines labor market outcomes in already socially vulnerable areas. The economic outcomes remain relatively unexplored by the studies on the COVID-19 impacts. To fill the gap, we study the relationship between the pandemic-caused economic recession and vulnerable communities in the unprecedented times. More marginalized regions may have broader economic damages related to the pandemic. First, based on a literature review, we delineate areas with high social disadvantage. These areas have multiple factors associated with various dimensions of vulnerability which existed pre-COVID-19. We term these places “ multi-dimensional social disadvantaged areas ”. Second, we compare employment and unemployment rates between areas with high and low disadvantage. We integrate geospatial science with the exploration of social factors associated with disadvantage across counties in Tennessee which is part of coronavirus “red zone” states of the US southern Sunbelt region. We disagree with a misleading label of COVID-19 as the “great equalizer”. During COVID-19, marginalized regions experience disproportionate economic impacts. The negative effect of social disadvantage on pandemic-caused economic outcomes is supported by several lines of evidence. We find that both urban and rural areas may be vulnerable to the broad social and economic damages. The study contributes to current research on economic impacts of the COVID-19 outbreak and social distributions of economic vulnerability. The results can help inform post-COVID recovery interventions strategies to reduce COVID-19-related economic vulnerability burdens.
1. Introduction: social disadvantage
Pandemics create severe disruptions to a functioning society. The economic and social disruptions intersect in complex ways and affect physical and mental health and illness ( Wu et al, 2020 ). Additionally, loss of jobs, wages, housing, or health insurance, as well as disruption to health care, hospital avoidance, postponement of planned medical treatment increase mortality, e.g., premature deaths ( Kiang et al., 2020 ; Petterson et al., 2020 ). The COVID-19, misleadingly labelled the “great equalizer” implies everyone is equally vulnerable to the virus, and that the economic activity of almost everyone is similarly impacted regardless of social status ( Jones & Jones, 2020 ). We set out to answer whether economic vulnerability is equally distributed during the COVID-19-caused economic recession or whether is it based on structural disadvantages? Is the social distribution of economic vulnerability magnified in regions with pre-existing social disparities, thus, creating new forms of inequalities? Knowledge of what areas experience the greater economic burden will help identify the most economically vulnerable communities relevant to post-COVID recovery interventions ( Qian and Fan, 2020 ).
Current studies on the impacts of COVID-19 largely focus on medical aspects including the COVID diagnosis and treatment ( Cai et al., 2020 ; Kass et al., 2020 ; O’Hearn et al., 2021 ; Price-Haywood et al., 2020 ). Non-medical urban research primarily concentrates on the impact of COVID on cities by studying factors related to environmental quality including meteorological parameters, and air and water quality ( Sharifi and Khavarian-Garmsir, 2020 ). COVID-related socio-economic impacts on cities are relatively less well studied, especially during the later stages of the recession.
Many pre-pandemic disparities unfold during COVID-19. To illustrate, residents of Black and Latino communities are suffering disproportionately higher unemployment rates, greater mortality due to the COVID-19 ( Thebault, Tran, & Williams, 2020 ; Wade, 2020 ), higher hospitalizations ( O’Hearn et al., 2021 ) and financial troubles. In contrast, some attributes make persons and communities more resilient. In China’s context, these include higher worker education and family economic status, membership in Communist Party, state-sector employment, and other traditional markers. These factors protect people from the pandemic-related financial stress and diminish its adverse economic effects ( Qian and Fan, 2020 ). Building on these recent studies on economic impacts, this social justice research focuses on areas with pre-existing social disadvantages. We study the role of social disadvantage and its impact on labor market during the COVID.
The distribution of economic vulnerability may potentially be related to COVID-19 conditions including those of economic burdens for people living in the pandemic epicenters ( Creţan and Light, 2020 ). Similarly, socio-economic disruptions create “a characteristic mosaic pattern in the region” ( Krzysztofik et al., 2020 , p. 583). The disruptions are strongly correlated with the spatial distribution of the COVID-19-related health effects. This study is set in Tennessee which is part of coronavirus “red zone” states of the US southern Sunbelt region. It is among the U.S. states with the highest rates of cases per capita, with 137,829 cases per 1 million people, or the 6th highest as of August 13, 2021 ( Worldometers, 2020 ; https://www.worldometers.info/coronavirus/country/us/ ). The study seeks to explore the impacts of social disadvantage on economy. The impact is measured by employment and unemployment in unprecedented times in the US context of prolonged disruptions to the health system, society, and economy intersecting in complex ways ( Kiang et al., 2020 ). We answer the following questions: (1) Do communities with high social disadvantage already burdened pre-COVID-19 by the lack of income, healthcare access, lacking resources, have less jobs available during the COVID-19 pandemic? (2) Do these areas simultaneously experience higher unemployment compared with other areas in the context of the pandemic?
The paper is organized as follows: Section 1 introduces the topic, provides the background information on social disadvantage and a brief description of the study implementation. It further discusses the links between employment and unemployment, and coronavirus, respectively, and introduces the study area. Section 2 describes in detail materials and methods used in the study. Section 3 provides the theory and calculations. Section 4 reports the results, and Section 5 offers a discussion. Finally, the paper concludes with conclusions found in Section 6 .
1.1. Background
Certain socio-economic and demographic conditions burden some communities more than others including racial and ethnic minorities, lower-income groups, and rural residents. The conditions include lacking economic opportunities and other inequalities ( Petterson et al., 2020 ) caused by social environment. Prior to the pandemic, it was challenging to live in areas with high social disadvantage where residents already have increased vulnerability to poor health due to greater psychosocial stress such as discrimination, unhealthy behaviors, and poorer health status ( Hajat et al., 2015 ). This is true for poor, marginalized communities elsewhere as spatial segregation of disadvantaged and marginalized communities decreases life opportunities for their members who have limited relationships with broader communities ( Méreiné-Berki et al., 2021 ). Within the context of studying disadvantaged urban communities, a recent work by Creţan et al. (2020) focused on the everyday manifestations of contemporary stigmatization of the urban poor using the case study of the Roma people who have been historically subject to state discrimination, ghettoization, inadequate access to education, housing, and the labor market for many decades in the past in multicultural urban societies of Central and Eastern Europe. The inequalities may persist and even increase if left unaddressed during pandemics ( Wade, 2020 ) leading to stark COVID-19-related health and economic disparities. Indeed, during the COVID-19, economic impacts of the pandemic disproportionately affect marginalized groups. The impact of coronavirus was harsh for those people as many of the already existing disparities unfold during COVID-19: black communities in the United States are disproportionately affected by higher death rates due to the COVID-19 virus ( Thebault et al., 2020 ), unemployment, and financial stress. Other growing COVID-19 research similarly suggests that elsewhere outside of the United States, areas that were disadvantaged prior to the pandemic with high rates of poverty and unemployment tended to be affected the strongest by the COVID-19 with the largest concentration of cases, while other spatially segregated ethnicity-based communities (e.g., the Roma) that have been vulnerable decades prior to COVID-19, saw an increase in the existing discrimination and stigmatization experiencing greater marginalization even during the current COVID-19 pandemic period ( Crețan & Light, 2020 ).
To achieve greater economic stability, and secure a dynamic labor market, countries in the global north and south for several decades have been increasing service employment much of which is low wage. The recent book Corona and Work around the Globe ( Eckert and Hentschke, 2020 ) describes the tremendous impact of the pandemic on human life and livelihoods as it sheds light on various experiences of workers during COVID-19 in various countries. Among the dramatically different cases worldwide, Germany which for decades has been promoting the low-wage sector to combat unemployment, provides a good example. The official approach to handling a disease differed substantially depending on whether the infected individuals were working people from the low- or upper-wage sector of the economy: applying a strict lockdown to the entire high-rise building where ethnic workers lived and preventing them from going to work in the former case and granting permission to work from home in the latter ( Mayer-Ahuja, 2020 ). The plight of the agricultural migrant workers who come to Germany from Eastern and Southeastern Europe, subjected during the pandemic to low wages or no payments and poor working and living conditions, however, is shared among the workers of low-wage sector across all countries who are more likely to get infected due to higher exposure and direct contact, but often experience unfair treatment based on ethnicity, migration and class status.
In yet another case set in the U.K., disadvantaged households have experienced intensified disadvantage during the COVID-19 as they could not access vital necessities, already stretched for resources pre-COVID-19. As provision of services or employment was discontinued due to their closure, disadvantaged households had significant impacts on their income level, mental health and wellbeing, education, nutrition, and domestic violence. In the absence of the key support of public institutions including schools, community centers, and social services, care for the most vulnerable members such as elderly, children, the disabled, have been absorbed by households ( Bear et al., 2020 ).
Another aspect experienced by workers during the pandemic is the total loss of earnings which is especially harsh in places with precarious employment even under normal circumstances. Informal workers in India who represent the vast majority of working population (over 93%), with no social security benefits and absent job security, experienced prolonged periods of time of no work due to lockdown and suspended transport services preventing them from getting to their workplaces, many on the verge of starvation ( Banerjee, 2020 ). This study looks into this aspect of COVID-19 economic impacts and confirms the findings of the growing COVID-19 research.
However, not only the poorest and marginalized people, but also marginalized regions are more likely to suffer from broader social and economic damages related to the pandemic compared with more privileged areas ( Creţan and Light, 2020 ; Krzysztofik et al., 2020 ). When disadvantages combine, it may lead to environment-driven COVID-19-related disparities in health. Besides a direct health effect, disadvantaged communities are disproportionally experiencing other side effects of COVID-19 such as negative labor market outcomes including forced unemployment, loss of income and social isolation. Studies found the extreme vulnerability of cities and urban areas exposed during the global pandemic ( Batty, 2020 ; Gössling et al., 2020 ). We argue that rural areas may be equally vulnerable to the broad range of social and economic damages if there is a spatial concentration of factors related to various dimensions of vulnerability.
This study is situated in the context of social disadvantage. Prior studies developed the methodology of the delineation of disadvantaged residential communities proxied by low-income workers ( Antipova, 2020 ). Disadvantaged low-income workers can be defined as those with inadequate access to material and social resources in the study area. However, this is a narrow approach which uses only a single dimension of a disadvantage, that of worker low earnings and misses other social inequality indicators. Accordingly, an approach adopted in this study identifies areas where socio-economic and demographic attributes each associated with multiple dimensions of social disadvantage are spatially co-locating. Spatial segregation of disadvantaged and marginalized communities decreases life opportunities for their members who have limited relationships with wider communities ( Méreiné-Berki et al., 2021 ). We identify these attributes based on a thorough literature review. Thus, we simultaneously consider multiple factors associated with disadvantage capturing a multi-dimensional social disadvantage. To meet the objective, we integrate geospatial science with the exploration of predictive geographic and social factors associated with disadvantage across counties in TN. The geospatial analysis includes point interpolation within the Geographic Information System (GIS) environment for the generation of a surface from a sample of social disadvantage values. This allowed us to visualize the spatial extent of disadvantaged communities. The focus is on labor market outcomes which are important indicators of society well-being. We study the association between pre-existing inequalities and COVID-19-related employment and unemployment rates. Thus, we identify the role of social disadvantage on labor market conditions in the context of the ongoing pandemic-caused economic recession.
Prior research determined the key metrics of social disadvantage. Conditions contributing to various aspects of disadvantage include poverty, occupations with low earnings, low rent, segregation and discrimination-related residential concentrations of minorities, and exposure to poor air quality ( Bullard, 2000 ). The recent COVID-19-related literature focuses on the separate effect of minorities, Hispanics, crowded households, dense areas, obesity, poverty, air pollution exposure and identifies those as important COVID-19 health risk factors ( Finch & Hernández Finch, 2020 ; Golestaneh et al., 2020 ; Han et al., 2020 ; Millett et al., 2020 ). These community-level variables result in neighborhood disadvantage comprising sub-standard housing quality, crowded conditions, poverty- and violence-caused stress which combined increase the risk of disease and other negative outcomes in life among socially disadvantaged groups ( Malhotra et al., 2014 ). The demographic and socio-economic attributes selected to represent the various aspects of social disadvantage in this research include minorities and ethnicities, poverty, housing crowdedness, educational attainment, underlying population health conditions, and pre-COVID-19 unemployment which may collectively drive a greater vulnerability to the COVID-19 infection and mortality as well as loss in employment and higher unemployment. It is challenging to isolate the separate effects of the multiple risk factors. By “critically analyzing the theoretically intended meaning of a concept” ( Song et al., 2013 ), a composite variable can be created to logically represent a multi-dimensional social disadvantage .
The following subsection briefly describes study implementation. First, we locate areas of disadvantage where multiple factors associated with various aspects of disadvantage co-locate spatially and term these places “multi-dimensional social disadvantaged areas”. Then, we examine how employment and unemployment were impacted in these already socially vulnerable areas. We map geographical inequalities in employment and unemployment rates during the period of COVID-19-related economic recession. For the first objective, we identify socially disadvantaged counties within TN which is part of coronavirus “red zone” states of the US southern Sunbelt region applying consistent criteria. For the second objective, we compare employment and unemployment outcomes between areas with high and low disadvantage.
1.1.1. Employment and coronavirus
This subsection discusses the role of employment and how it was impacted by the COVID-19-caused economic recession. The literature recognizes the complex interrelationship between employment and overall health and well-being. Negative COVID-19 impacts on urban economy include loss of citizens' income, while movement restrictions and ‘stay home’ measures adversely impacted tourism and hospitality and small- and medium sized businesses due to the closure of markets, food outlets and social spaces ( Wilkinson et al., 2020 ).
Millions of essential or blue-collar workers are still doing their jobs out of necessity and because they cannot telecommute and work jobs that cannot be done from home and have higher exposure to the virus. Some racial groups disproportionally have jobs that do not allow them to work from home and where social distancing is a challenge. Prior studies find that workplaces of low-income individuals tend to be close to their residential spaces, and disproportionately concentrated in lower-wage industries such as hospitality and retail services ( Antipova, 2020 ). These industries commonly represent essential services experiencing higher exposure to the COVID virus through workplaces. At the same time, minorities and lower-income groups often live in inner-ring suburbs with older housing and aging infrastructure ( Antipova, 2020 ) in multiunit structures and in multigenerational households which inhibit the ability to practice social distancing increasing the risks of disease occurrence and deaths ( Qualls et al., 2017 ). In addition, minorities and lower-income groups have fewer options for protecting both their health and economic well-being ( Gould and Wilson, 2020 ). Nearly two-thirds of Hispanic people (64.5%) considered at high risk for coronavirus live with at least one person who is unable to work from home, compared to 56.5% of black and less than half (47%) of white Americans, according to a recent study ( Selden and Berdahl, 2020 ).
Despite the pandemic-induced layoffs, job hires have occurred by major retailers such as Walmart and e-commerce giant Amazon, and takeout and delivery-based services such as Domino’s Pizza and Papa John’s which may become permanent positions. These workplaces may match the job skill sets of low-income residents of vulnerable communities. However, oftentimes many low-income workers benefitted less, even when jobs were created during the COVID-19. To illustrate, big technology companies (i.e., communication services: Netflix, Tencent, Facebook, T-Mobile; information technology: Microsoft, Nvidia, Apple, Zoom Video, PayPal, Shopify; consumer discretionary: Amazon, Tesla, Alibaba, etc.) prospered in the pandemic with the financial success measured by equity value added ( Financial Times, 2020 ). Workers who lost jobs in low-income segment such as hospitality sector may be hired by retailers such as Kroger or CVS. However, many others from the communities with high social disadvantage may not have a skill set needed at technology firms that benefit from the working from home trend and hire skilled workers including software engineers and product designers. Cross-industry employment shifts plays a minor role in total job creation, while employer-specific factors primarily account for job reallocation ( Barrero et al., 2020 ).
1.1.2. Unemployment and coronavirus
This subsection discusses how unemployment was impacted by the COVID-19-caused economic recession. An economic recession occurs when there is a substantial drop in overall economic activity diffused throughout the economy for longer than a few months. While past recessions were driven by an inherently economic or financial shock, the current recession is caused by a public health crisis ( Weinstock, 2020 ). COVID-19 caused a drop in consumer demand across all industrial sectors resulting in economic recession and massive unemployment where not only hourly workers but salaried professionals lost their jobs ( Petterson et al., 2020 ). A range of factors contributed to the spatial variation in economic damage including the share of jobs in industries delivering non-essential services to in-person customers ( Dey and Loewenstein, 2020 ), declines in personal consumption caused by individual fears of contracting COVID-19 ( Goolsbee and Syverson, 2020 ), and the implementation of social policies including stay-at-home orders and business shutdowns ( Gupta et al., 2020 ).
Unemployment rate is defined as a percentage of unemployed workers in the total labor force. The rate is published monthly by the Bureau of Labor Statistics (BLS) which uses both the establishment data (captured by the Current Employment Statistics program) and household surveys (Current Population Survey) to generate the labor market data ( Bureau of Labor Statistics (BLS), 2020b ). A person is unemployed if they were not employed during the survey’s reference week and who had actively searched for a job in the 4-week period ending with the reference week, and were presently available for work ( BLS, 2020b ).
Caused by the COVID-19, the unemployment rate reached a peak in April 2020 at 14.7% nationwide, an unprecedented joblessness amount since employment data collection started in 1948. It exceeded the previous peaks during the Great Recession and after ( Falk et al., 2020 ). The official unemployment rate may have been over 20%, since the actual level of joblessness could have been understated due to local unemployment rate measurement errors ( Coibion et al., 2020 ). In addition, the unemployment rate was understated due to a geographically widespread misclassification of those who was not at work but considered employed and non-inclusion of labor force non-participants who still counted as employed ( Bureau of Labor Statistics (BLS), 2020a ). Further, the COVID-19 caused the rapid rate of change in unemployment at the national level challenging accurate forecast of the monthly unemployment rate ( Weinstock, 2020 ).
Overall, current unemployment (using the most recently available county-level data at the time of writing for December 2020) is still elevated and is almost twice as high as it was back in February 2020 which represented the business cycle peak with the peak of payroll employment. March 2020 was the first month of the subsequent current economic recession as declared by The National Bureau of Economic Research (NBER, 2020) caused by the COVID-19 pandemic which turned out the worst downturn after the Great Recession. As Fig. 1 shows using the Current Population Survey data (Series ID: LNS14000000) from the BLS, during the prior recessions the unemployment rate rose gradually reaching its peak, and in the pandemic-caused recession it increased unprecedentedly to its peak over one month, from March 2020 to April 2020 by 10.3% (from 3.5% in February 2020 to 4.4% in March 2020 to 14.7% in April). After that, the rate declined as workers continued to return to work to 6.3% in December 2020.

U.S. Historical unemployment rate for workers 16 years and over, January 1948 to December 2020, % (seasonally adjusted).
Some communities can absorb the impact of economic downturns due to more favorable economic and social factors protecting residents from adversity. Yet other communities are witnessing the effect of rising unemployment in the time of COVID-19. Loss of income and livelihood has further effects: as wages drop, more people are forced into poverty while simultaneously people's health is impacted. Unemployment impacts all-cause mortality. Fig. 2 presents the dynamics of unemployment distribution across counties in TN for the selected months. Shown are pre-COVID-19 unemployment rates as of August 2019 ( Fig. 2 a), followed by May 2020 ( Fig. 2 b) where even the lowest levels of unemployment exceed the highest rates of the pre-pandemic period even in wealthy counties around Nashville (seen in the legend entries), August 2020 ( Fig. 2 c), and September 2020 ( Fig. 2 d). The overall unemployment abates somewhat during the later stage, and the general spatial pattern resembles that of the pre-COVID-19 period with higher unemployment concentrated in the southwestern corner of the state around Memphis.

Dynamics of unemployment rate across counties in TN for selected months: (a) August 2019, (b) May 2020; (c) August 2020; (d) September 2020.
1.1.3. Study area
Tennessee is home to large cities including Nashville (the county seat), Memphis, Knoxville and Chattanooga. Despite urban diversified economy, there was a steep decline in the number of international and domestic tourists impacting urban economy. Among cities listed above, Memphis, located in Shelby County, is a shrinking city with a declining population base. Urban shrinkage makes cities more vulnerable due to very negative impacts on urban economy. Shrinking cities are characterized by higher unemployment rates, depopulation (as people with higher economic and social status leave elsewhere), and a higher share of older people (increasing a share of individuals with underlying health conditions) ( Haase et al., 2014 ; Hartt 2019 ; Hoekveld 2012 ; Krzysztofik et al., 2020 ). The shrinking cities have higher exposure to extreme socioeconomic phenomena, including financial stress due to the decreases in the city’s budget. Decreasing budget in its turn has further urban development implications since implementation of some plans deemed of lesser priority such as environmental and cultural may be delayed and cancelled altogether ( Kunzmann, 2020 ; Sharifi and Khavarian-Garmsir, 2020 ).
Tennessee is one of the US southern Sunbelt states which had infection surges since summer 2020 due to the aggressive push for economy opening by then-President Trump administration. The pandemic has affected unemployment for every state in the United States ( Falk et al., 2020 ). Fig. 3 portrays selected industries impacted by the economic recession in Tennessee using seasonally adjusted data on employees on nonfarm payrolls for November 2019 (as a base period), September–November 2020. Unemployment rates concentrate disproportionately in sectors providing in-person non-essential services where some demographic groups are overrepresented. This results in substantially higher unemployment rates for those workers ( Cortes and Forsythe, 2020 ; Fairlie, 2020 ). Accordingly, it can be seen in Fig. 3 that in Tennessee, among the reported industries, leisure and hospitality has suffered the most, followed by jobs in government, education and health services, professional and business services, and trade, transportation, utilities. There was a slight increase in jobs in financial activities from 2019 to 2020 ( Bureau of Labor Statistics (BLS), 2020a ). The hardest hit industries tend to employ demographic groups such as women, minorities, low-income workers, and younger workers who have experienced greater job losses ( Murray and Olivares, 2020 ).

Employees on nonfarm payrolls by selected industry sector, seasonally adjusted, in TN.
2. Materials and methods
In the absence of fine-scale monthly data on employment and unemployment, we sourced county-level data from the Bureau of Labor Statistics (BLS) to track monthly changes in employment and unemployment in Tennessee (retrieved from https://www.bls.gov/lau/ ). Labor force data were extracted from this official primary source.
We used a comparative assessment approach to analyze the COVID-19-based labor market outcomes including the rates of COVID-19-related employment and unemployment attributable to social disadvantage conditions. For this, we stratify data based on community disadvantage status, and combine data in a comparative assessment framework. We proceed and identify disadvantaged communities using the methodology described below. Next, we test the hypothesis that in areas with high social disadvantage where more essential workers are more likely to reside, the unemployment is higher while employment opportunities are lower by comparing unemployment and employment rates within these communities to those of more privileged communities.
3. Theory/calculation
We focus on the areas where the multiple risk factors identified in the recent literature co-locate spatially and term these places “ multi-dimensional social disadvantaged areas ”. We carried out a rigorous literature review of the variables to stand in for social disadvantage in this research. The following demographic and socio-economic factors have been selected to represent community’s vulnerability: (1) Minorities and ethnicity; (2) Crowded households; (3) Poverty; (4) Education; (5) Underlying medical conditions (obesity); and (6) Unemployment. For the 1st variable, minorities and ethnicity , we used percent minority population and Hispanic ethnicity as studies commonly use race and ethnicity as vulnerability metrics (as explained in Section 2 Background information). For the 2nd variable, crowded households , we used percent households that are multigenerational as an indicator of crowdedness, and thus, indicating area’s disadvantage with a high share of such households. For the 3rd variable, poverty , we chose percent of households below 100% of federal poverty level which is also known as the poverty line. It is an economic measure of income. The poverty guidelines are updated annually by the US Department of Health and Human Services to indicate the minimum income needed by a family for housing, food, clothing, transportation, and other basic necessities and to determine eligibility for certain welfare benefits. This measure was used because less affluent and less privileged households have fewer means and less access to various resources to cope with the effects of financial crises ( Pfeffer et al., 2013 ). Low-income households may be especially vulnerable to wage losses during the outbreak ( Qian and Fan, 2020 ). For the 4th variable, education , we used percent of population with less than high school diploma since lower educational attainment is an indicator of poverty and thus captures social disadvantage, while workers with better education have higher economic resilience when challenged with a large-scaled social shock ( Cutler et al., 2015 ; Kalleberg, 2011 ). For the 5th variable, underlying medical conditions , we used percent population with obesity as the top risk for COVID-19-related hospitalization. Supported by several lines of evidence, both domestically and internationally, obesity may predispose to more severe COVID-19 outcomes ( O’Hearn et al., 2021 ). Finally, for the 6th variable, unemployment , unemployment rate (averaged from August 2019 to January 2020 to adjust for seasonality) was used as a marker of overall vulnerability as it is linked to overall mortality. Further, regions with higher unemployment are more susceptible to business-cycle fluctuations, and thus, are more socially and economically vulnerable.
These socio-economic and demographic attributes (minority population, Hispanic ethnicity, federal poverty level, crowded households, adult obesity, lower educational attainment, and unemployment) have been used in this research to create a composite variable to represent a multi-dimensional social disadvantage (also referred to as vulnerability). Due to different variances in the original variables, we standardized them to prevent a disproportionate impact which may be caused by any one original variable with a large variance. The z-score transformation was applied by averaging the original variables and computing z scores with a mean of 0 and values ranging from negative to positive numbers ( Song et al., 2013 ).
Thus, the original variables were converted to z-scores to preserve the distribution of the raw scores and to ensure the equal contributions of the original variables. Next, we created a composite variable capturing a multi-dimensional social disadvantage. It was calculated by summing standardized z-scores of the original risk factors. The higher value can be interpreted as higher disadvantage while the lower value means more privileged communities. Based on the frequency distribution of values of the composite variable, we established a cut-off value for the composite variable to designate communities with high or low exposure to social disadvantage. We used the following method to determine the cut-off value of the composite variable. The values greater than 3.38 correspond to 1 standard deviation above the mean (or, the 88th percentile in the value distribution) indicating communities in the top 12 percent of social disadvantage and therefore, a higher share of factors contributing to disadvantage. This value was used to differentiate communities according to their disadvantage status. We identified twelve counties with high social disadvantage (N high = 12), and other counties represent more privileged communities (N low = 83). To test whether the taken approach correctly identifies disadvantaged communities, we conducted a Wilcoxon two-sample test for the variables of interest ( Table 1 ). We report the results of the estimates in the following section. The above socio-economic and demographic population characteristics come from the 2018 American Community Survey (ACS) 5-year data, an annual nationwide survey conducted by the US Census Bureau, available for various geographic units and applied for areal units within the study area ( U. S. Census Bureau, 2020 ).
Descriptive statistics.
The basic descriptive demographic and socio-economic characteristics of the TN population are shown in Table 1 . It includes the summaries for communities with high and low social disadvantage allowing to compare the variables of interest between these communities. The following variables are reported: percent African American, percent Hispanic, median income, percent of people over 25 years who are less than high school graduates, estimated percent of obese adults, percent households below 100% of federal poverty level, and percent of multi-generation households. The factors comprising social disadvantage were statistically significantly different than those extant in more privileged counties. Compared with the general TN population, the disadvantaged cohort was generally more likely to be of non-Hispanic Black race; more impoverished; with less educational attainment, more obese, and had more households with crowded conditions.
To visualize social disadvantage and show how it varies across the space, we used our sample of social disadvantage measurements and created a surface of social disadvantage within the study area using the Geographic Information System (GIS). The interpolated surface was derived from an Inverse Distance Weighted technique ( Watson and Philip, 1985 ). Fig. 4 presents the surface illustrating that both urban and rural counties in Tennessee are subject to social disadvantage.

Social disadvantage within the study area.
We examined how unemployment changed from August 2019 to December 2020. Currently, all counties have substantially higher unemployment compared with that prior to COVID. Fig. 5 presents the results of the Nonparametric One-Way ANOVA test showing the distribution of Wilcoxon scores for unemployment rate for all counties in Tennessee combined, regardless of social disadvantage status, for 17 months. A statistically significant difference is found for unemployment rates between the pre-COVID period and the period since April 2020, with current unemployment rates although decreased but still significantly higher compared with those prior to the recession.

Nonparametric One-Way ANOVA and distribution of Wilcoxon scores for unemployment rate for all counties combined for 17 months (August 2019–October 2020), regardless of social disadvantage status.
We compared employment and unemployment rates for Tennessee counties stratified by the type of social disadvantage separately for each month. Fig. 6 presents the average employment and unemployment rates by community disadvantage from August 2019 to December 2020 in a graphical form. The results of the non-parametric Wilcoxon test for employment and unemployment rates are presented in Table 2 . Pre-COVID and before the unemployment peak in April 2020, communities with high social disadvantage consistently had less jobs and greater unemployment, which we tested statistically and found a significant difference for both outcomes of the labor market between communities by their disadvantage status ( Table 2 ). Shown in Table 2 , in April and May 2020, during the peak of unemployment and immediately after, unemployment rates observed in both types of communities were high with no statistical difference. In June, the differences again became prominent, when there were more jobs available in more advantaged areas and employment rate remained consistently greater in areas with less disadvantage. Also in June, unemployment rate remained consistently greater in areas with higher disadvantage. This month saw the greater difference in both outcomes since the COVID-19 than pre-pandemic (supported by higher p-values). Compared with all TN population, residents of disadvantaged counties had less jobs available and were more likely to be unemployed during all periods except for April and May.

Mean employment and unemployment stratified by community disadvantage status.
Wilcoxon Two-Sample Test: Distribution of Wilcoxon scores in employment and unemployment rates by community disadvantage status by month (August 2019–December 2020).
We examined the percent change in both labor market outcomes. Fig. 7 presents the percent change in mean employment ( Fig. 7 a), and mean unemployment by community disadvantage ( Fig. 7 b). The percent change in employment and unemployment was relatively small in both types of community during the pre-COVID period. However, the overall fluctuations in both conditions were greater in communities with high social disadvantage (evidenced by a greater range between ups and downs for disadvantaged communities shown with the black-colored symbols). On the other hand, employment and unemployment were more stable in more privileged communities (shown with the grey-colored symbols in the Fig. 7 ). During the unemployment peak in April 2020, the change in percent employment was −11.5 points from the previous month even in more advantaged counties, while the unemployment in April increased by 10.42 percentage points in disadvantaged counties.

Percent change in (a) mean employment; (b) mean unemployment by community disadvantage.
We show how various factors of social disadvantage intersect and combined impact economic vulnerability measured by unemployment rate. Fig. 8 reports the link between unemployment and social disadvantage pre-COVID (unemployment rate was averaged over August 2019–January 2020 in Fig. 8 a), and during COVID (unemployment rate for November 2020 is shown in Fig. 8 b). During the COVID pandemic, its impact is even stronger as evidenced by a greater slope of the line of fit, larger coefficients, and a greater R-squared value ( Fig. 8 b). The strong relationship between these factors of social disadvantage and economic outcomes in COVID-19 might inform post-COVID recovery intervention strategies to reduce COVID-19-related economic vulnerability burdens. For example, in the light of findings on socio-economic and demographic subpopulations at a higher risk for economic damages, prioritization of economic relief distribution might be based on community disadvantage status targeting individuals from areas with existing inequalities to increase economic resilience of marginalized communities.

Unemployment and Social disadvantage: (a) pre-COVID (averaged August 2019–January 2020); (b) during COVID (November 2020).
5. Discussion
Current studies on the impacts of COVID-19 tend to focus on medical aspects while non-medical urban research mostly analyzes the role of environmental quality. To better understand the full effects of pandemics on communities and minimize the various impacts as well as to improved response, other aspects need to be examined. This includes studying less researched themes including socio-economic impacts consisting of both social impacts and social factors making individuals and communities less resilient and more vulnerable to the effects of the COVID. Additionally, economic impacts of the pandemic-caused recession so far remain relatively underexplored and need to be investigated ( Sharifi and Khavarian-Garmsir, 2020 ).
Communities are often severely segregated along wealth and social lines in developing and developed world ( Wilkinson et al., 2020 ). We study the role of social factors and the impact of the COVID on labor market conditions in Tennessee. Specifically, we studied the impacts of social environment on employment and unemployment through the concept of a multi-dimensional social disadvantage by using geospatial science.
A recent study identified factors which can make a community more vulnerable to the pandemic’s effects using as a case study the province of Silesia in Poland, one of the largest industrial and mining regions in Europe. Specialized functions such as mining-oriented industries, large care centers, polycentricity, and urban shrinkage make communities most at risk due to very negative impacts on urban economy ( Krzysztofik et al., 2020 ). Since vulnerability is always very context-specific, we found a combination of different causal factors of social disadvantage captured by a composite variable making communities most at risk during the COVID reflected in broader social and economic outcomes. In creating a composite variable to capture social disadvantage logically and meaningfully, the following variables were used: % African American, % Hispanic, % below 100% federal poverty level, % population with less than high school diploma (an indicator of poverty), % multi-generation households (an indicator of crowdedness), % estimated obese adults reporting to be obese with the BMI 30 or greater, % unemployed. The proposed method can be generalized beyond the study area and used as a tool by policy makers using consistent criteria for the delineation of areas carrying a greater risk for the more severe impact by the pandemic due to co-existence and co-location of the multi-dimensional social disadvantage factors which are more likely to experience further socio-economic disruptions.
Current urban research on COVID economic impacts found that some cities are more vulnerable than others and are most at risk. Cities with an undiversified economic structure with industries where a large number of workers are shoulder-to-shoulder share cramped spaces for a prolonged time and where social distancing is challenging (e.g., meat-packing and poultry processing plants), cities relying on tourism as well as cities that have large care centers, polycentric cities, and shrinking cities are the most vulnerable to negative impacts on urban economy. The urban hotel market, city tax revenues, citizens' income, tourism and hospitality, small- and medium sized firms, urban food supply chain, and migrant workers are all impacted ( Krzysztofik et al., 2020 ). Other recent studies similarly concluded that the COVID has revealed the extreme vulnerability of cities and urban areas disrupting tourism and affecting supply chains in cities ( Batty, 2020 ; Gössling et al., 2020 ). We support this statement but also find that rural areas can experience a broad range of social and economic damages related to COVID.
Before and during the COVID-19 period, money laundering, limitations of economic development, environmental pollution and uncontrolled deforestation, population displacement, institutional incompetence, and corruption of political elites have been debated including corruption and conflagration in Bucharest before the pandemic ( Creţan & O’Brien, 2020 ), as well as other contestations on selling masks and different medical products highlighted in different countries during the pandemic period. Following catalytic events, the affected community may respond to long-held concerns with demands to address these problems bringing about important changes to the systems. Marginalized stigmatized minorities may effectively overcome discriminatory laws, higher poverty and other constraints and influence public opinion and politics in their favor through collective action via various strategies including protests against corruption and the inaction of the political leaders in Romania in 2015 forcing the resignation of the Government, and protests in the US in the aftermath of police violence against black people have been documented ( Creţan & O’Brien, 2020 ; Fryer, 2019 ). During the COVID-19, the non-payment of wages and poor working and living conditions caused seasonal workers in Germany to protest against this unfair treatment, however, generating low coverage in the national press ( Mayer-Ahuja, 2020 ).
6. Conclusions
Some socio-economic and demographic conditions consistently and significantly impact some communities more often than others, particularly based on ethnic minority status, low income, and rural location. The conditions include systemic issues such as fragmented health care system (within which some individuals do not get health care in a timely fashion), racism and structural disparities in education, income, wealth, a consistent lack of economic opportunity, environmental factors, transportation and housing ( Petterson et al., 2020 ). These factors interact in complex ways resulting in persisting social environment-driven health and other inequalities which if left unaddressed will only increase.
Respectively, among policies goals across the Global North enhancing wellbeing and social mobility for disadvantaged and marginalized families, creating socially mixed, heterogeneous neighborhoods (that is, desegregation) is promoted to avoid spatial segregation based on racial and ethnic membership and class while supporting social cohesion ( Méreiné-Berki et al., 2021 ). Importantly, a marginalized community is not a homogeneous group as the lived experience of disadvantage within the communities is variegated: respectively, policies to improve socio-spatial integration and addressing the various causes of extreme poverty including social, economic, and cultural that improve social equity have been suggested since desegregation on its own is insufficient (( Méreiné-Berki et al., 2021 ). Sustainable planning may mitigate consequences of urban sprawl noted in the urban studies literature including urban blight which is the greatest in poorest areas entrapping the low-income residents in the inner city where they have only limited regional mobility and access to job opportunities at the urban edge. Understanding the links between a development of a metropolitan-wide blight remediation strategy toward a sustainable urban form and welfare enhancing among the disadvantaged populations needs to be further investigated.
During public health crises, the importance of the central role of the community has been highlighted especially when some state-based social services may be less available due to lockdown. Rather than inventing new solutions, voluntary informal social networks that have been generated by communities utilize local assets and resources ( Bear et al., 2020 ). Community-based initiatives may rely on the voluntary sector, faith- and charities-based organizations, and social enterprises for various services including help with visiting housebound people, or using them as a distribution hub for food distribution to families in need.
In conclusion, in this study, we situated the research on economic impacts of the COVID in the broader context of social disadvantage with findings both domestically and from other countries in line with those in our study. The earlier misleading view of the global epidemic representing a systematic disadvantage that may affect and limit everyone’s economic activity, with any socioeconomic status or from any geographic location, was rejected. Our finding indicates that certain factors may increase people's vulnerability to the financial stress related to COVID-19. We find support that the social distribution of economic vulnerability is magnified in regions with pre-existing social disparities, creating new forms of disparity ( Qian and Fan, 2020 ).
This work was supported by the UTHSC/UofM SARS-CoV-2/COVID-19 Research CORNET (Collaboration Research Network) Award.
CRediT authorship contribution statement
Anzhelika Antipova: Conceptualization, Formal analysis, Methodology, Visualization, Writing – original draft, Writing – review & editing.
Declaration of competing interest
The author declares no conflict of interest.
- Antipova A. Analysis of Commuting Distances of Low-Income Workers in Memphis Metropolitan Area. TN. Sustainability. 2020; 12 (3):1209. doi: 10.3390/su12031209. [ CrossRef ] [ Google Scholar ]
- Banerjee S. In: Corona and work around the Globe. Eckert A., Hentschke F., editors. De Gruyter; Berlin, Boston: 2020. Skill, informality, and work in pandemic times: Insights from India; pp. III–IX. 2020. [ CrossRef ] [ Google Scholar ]
- Barrero J.M., Bloom N., Davis S.J. COVID-19 also a reallocation shock. 2020. https://bfi.uchicago.edu/wp-content/uploads/BFI_WP_202059.pdf Working paper NO. 2020-59. At:
- Batty M. The coronavirus crisis: What will the post-pandemic city look like? Environ. Plan. B: Urban Anal. City Sci. 2020; 47 (4):547–552. [ Google Scholar ]
- Bear L., James D., Simpson N., Alexander E., Bhogal J.K., Bowers R.E., Cannell F., Lohiya A.G., Koch I., Laws M., Lenhard J.F., Long N.J., Pearson A., Samanani F., Wuerth M., Vicol O., Vieira J., Watt C., Whittle C., Zidaru-Barbulescu T. In: Corona and work around the Globe. Eckert A., Hentschke F., editors. De Gruyter; Berlin, Boston: 2020. Changing care networks in the United Kingdom; pp. VVIII–VVX. [ CrossRef ] [ Google Scholar ]
- Bullard R. Westview Press; 2000. Dumping in Dixie: Race, class, and environmental quality, third edition: Bullard, Robert D.: 9780813367927: Amazon.com: Books. [ Google Scholar ]
- Bureau of Labor Statistics (BLS) Economic news release. State employment and unemployment —NOVEMBER 2020. December 18, 2020. 2020. https://www.bls.gov/news.release/laus.nr0.htm USDL-20-2267. At:
- Bureau of Labor Statistics (BLS) Frequently asked questions: The impact of the coronavirus (COVID-19) pandemic on the Employment Situation for April 2020. 2020. https://www.bls.gov/cps/employment-situation-covid19-faq-april-2020.pdf May 8, 2020. At:
- Cai Q., et al. Obesity and COVID-19 severity in a designated hospital in shenzhen, China. Diabetes Care. 2020; 43 (7):1392–1398. [ PubMed ] [ Google Scholar ]
- Coibion O., Gorodnichenko Y., Weber M. NBER working paper No. 27017. 2020. Labor markets during the COVID-19 crisis: A preliminary view. April 2020. [ Google Scholar ]
- Cortes G.M., Forsythe E. Upjohn Institute Working Paper; May 2020. The heterogeneous labor market impacts of the Covid-19 pandemic. [ PMC free article ] [ PubMed ] [ Google Scholar ]
- Creţan R., Light D. COVID-19 in Romania: Transnational labour, geopolitics, and the Roma ‘outsiders. Eurasian Geography and Economics. 2020; 61 (4–5):559–572. [ Google Scholar ]
- Creţan R., Málovics G., Méreiné-Berki B. On the perpetuation and contestation of racial stigma: Urban Roma in a disadvantaged neighbourhood of Szeged. Geographica Pannonica. 2020; 24 (4):294–310. [ Google Scholar ]
- Creţan R., O’Brien T. Corruption and conflagration: (in)justice and protest in bucharest after the colectiv fire. Urban Geography. 2020; 41 (3):368–388. doi: 10.1080/02723638.2019.1664252. [ CrossRef ] [ Google Scholar ]
- Cutler D.M., Huang W., Lleras-Muney A. When does education matter? The protective effect of education for cohorts graduating in bad times. Social Science & Medicine. 2015; 127 :63–73. 2015. [ PMC free article ] [ PubMed ] [ Google Scholar ]
- Dey M., Loewenstein M.A. How many workers are employed in sectors directly affected by COVID-19 shutdowns, where do they work, and how much do they earn? Monthly Labor Review. April 2020 https://www.bls.gov/opub/mlr/2020/article/covid-19-shutdowns.htm [ Google Scholar ]
- Eckert A., Hentschke F. Andreas Eckert and Felicitas Hentschke. De Gruyter; Berlin, Boston: 2020. Introduction: Corona and work around the Globe". Corona and work around the Globe; pp. XVII–XXII. 2020. [ CrossRef ] [ Google Scholar ]
- Fairlie R. NBER working paper No. 27309, June 2020. 2020. The impact of covid-19 on small business owners: Evidence of early-stage losses from the April 2020 current population survey. [ Google Scholar ]
- Falk G., Carter J.A., Nicchitta I.A., Nyhof E.C., Romero P.D. Unemployment rates during the COVID-19 pandemic. 2020. https://fas.org/sgp/crs/misc/R46554.pdf Brief. Nov. 2020. Prepared by the Congressional Research Service (CRS). CRS Report R46554. At:
- Financial Times “Prospering in the pandemic: The top 100 companies,” 18 June. 2020. https://www.ft.com/content/844ed28c-8074-4856-bde0-20f3bf4cd8f0 At:
- Finch W.H., Hernández Finch M.E. Poverty and covid-19: Rates of incidence and deaths in the United States during the first 10 Weeks of the pandemic. Front. Sociol. 2020; 5 :1–10. June. [ PMC free article ] [ PubMed ] [ Google Scholar ]
- Fryer R.G.J. An empirical analysis of racial differences in police use of force. Journal of Political Economy. 2019; 127 (3):1210–1261. [ Google Scholar ]
- Golestaneh L., et al. The association of race and COVID-19 mortality. EClinicalMedicine. 2020; 25 :100455. [ PMC free article ] [ PubMed ] [ Google Scholar ]
- Goolsbee A., Syverson C. NBER working paper No. 27432. June 2020. Fear, lockdown, and diversion: Comparing drivers of pandemic economic decline 2020. [ PMC free article ] [ PubMed ] [ Google Scholar ]
- Gössling S., Scott D., Hall C.M. Pandemics, tourism and global change: A rapid assessment of COVID-19. Journal of Sustainable Tourism. 2020:1–20. [ Google Scholar ]
- Gould E., Wilson V. 2020. Black workers face two of the most lethal preexisting conditions for coronavirus — racism and economic inequality. [ Google Scholar ]
- Gupta S., et al. NBER working paper No. 2780. May 2020. Effects of social distancing policy on labor market outcomes. [ PMC free article ] [ PubMed ] [ Google Scholar ]
- Haase A., Rink D., Grossmann K., Bernt M., Mykhnenko V. Conceptualizing urban shrinkage. Environment and Planning A. 2014; 46 (7):1519–1534. doi: 10.1068/a46269. [ CrossRef ] [ Google Scholar ]
- Hajat A., Hsia C., O’Neill M.S. Vol. 2. Springer; 2015. Socioeconomic disparities and air pollution exposure: A global review; pp. 440–450. (Current environmental health reports). 4. 01-Dec. [ PMC free article ] [ PubMed ] [ Google Scholar ]
- Han J., Meyer B.D., Sullivan J.X. 2020. Income and poverty in the COVID-19 pandemic. [ Google Scholar ]
- Hartt M. The prevalence of prosperous shrinking cities. Annals of the Association of American Geographers. 2019; 109 (5):1651–1670. doi: 10.1080/24694452.2019.1580132. [ CrossRef ] [ Google Scholar ]
- Hoekveld J.J. Time-space relations and the differences between shrinking regions. Built Environment. 2012; 38 (2):179–195. doi: 10.2148/benv.38.2.179. [ CrossRef ] [ Google Scholar ]
- Jones B.L., Jones J.S. Gov. Cuomo is wrong, covid-19 is anything but an equalizer. Washington Post. 2020 https://www.washingtonpost.com/outlook/2020/04/05/gov-cuomo-is-wrong-covid-19-is-anything-an-equalizer/ Accessed from. [ Google Scholar ]
- Kalleberg A.L. Russell Sage Foundation; New York, NY: 2011. Good jobs, bad jobs: The rise of polarized and precarious employment systems in the United States, 1970s-2000s. 2011. [ Google Scholar ]
- Kass D.A., Duggal P., Cingolani O. Obesity could shift severe COVID-19 disease to younger ages. Lancet. 2020; 395 (10236):1544–1545. [ PMC free article ] [ PubMed ] [ Google Scholar ]
- Kiang M.V., Irizarry R.A., Buckee C.O., Balsari S. Every body counts: Measuring mortality from the COVID-19 pandemic. Annals of Internal Medicine. Sep. 2020:M20–M3100. [ PMC free article ] [ PubMed ] [ Google Scholar ]
- Krzysztofik R., Kantor-Pietraga I., Spórna T. Spatial and functional dimensions of the COVID-19 epidemic in Poland. Eurasian Geography and Economics. 2020; 61 (4–5):573–586. 10.1080/15387216.2020.1783337. [ Google Scholar ]
- Kunzmann K.R. Smart cities after covid-19: Ten narratives. disP - Plan. Rev. 2020; 56 (2):20–31. [ Google Scholar ]
- Malhotra K., Baltrus P., Zhang S., Mcroy L., Immergluck L.C., Rust G. Geographic and racial variation in asthma prevalence and emergency department use among Medicaid-enrolled children in 14 southern states. Journal of Asthma. 2014; 51 (9):913–921. Nov. [ PMC free article ] [ PubMed ] [ Google Scholar ]
- Mayer-Ahuja N. In: Corona and work around the Globe. Eckert A., Hentschke F., editors. De Gruyter; Berlin, Boston: 2020. Solidarity’ in times of Corona? Of migrant Ghettos, low-wage heroines, and empty public coffers; pp. XVII–XXII. 2020. [ CrossRef ] [ Google Scholar ]
- Méreiné-Berki B., Málovics G., Crețan R. “You become one with the place”: Social mixing, social capital, and the lived experience of urban desegregation in the Roma community. Cities. 2021; 117 :103302. [ Google Scholar ]
- Millett G.A., et al. Assessing differential impacts of COVID-19 on black communities. Annals of Epidemiology. 2020; 47 :37–44. [ PMC free article ] [ PubMed ] [ Google Scholar ]
- Murray S., Olivares E. 2020. Job losses during the onset of the COVID-19 pandemic: Stay-at-home orders, industry composition, and administrative capacity (June 18, 2020) https://ssrn.com/abstract=3633502 Available at SSRN: [ CrossRef ] [ Google Scholar ]
- O’Hearn M., Liu J., Cudhea F., Micha R., Mozaffarian D. Coronavirus disease 2019 hospitalizations attributable to cardiometabolic conditions in the United States: A comparative risk assessment analysis. Journal of the American Heart Association. 2021 doi: 10.1161/JAHA.120.019259. https://www.ahajournals.org/doi/abs/10.1161/JAHA.120.019259 [ PMC free article ] [ PubMed ] [ CrossRef ] [ Google Scholar ]
- Petterson S., Westfall J.M., Miller B.F. Well Being Trust; 2020. Projected deaths of despair from COVID-19. Well being trust. [ Google Scholar ]
- Pfeffer T., Danziger S., Schoeni R.F. Wealth disparities before and after the great recession. The Annals of the American Academy of Political and Social Science. 2013; 650 (1):98–123. 2013. [ PMC free article ] [ PubMed ] [ Google Scholar ]
- Price-Haywood E.G., et al. Hospitalization and mortality among black patients and white patients with Covid-19. New England Journal of Medicine. 2020; 382 (26):2534–2543. [ PMC free article ] [ PubMed ] [ Google Scholar ]
- Qian Y., Fan W. Research in social stratification and mobility. JAI Press; 2020. Who loses income during the COVID-19 outbreak? Evidence from China. [ Google Scholar ]
- Qualls N., et al. Community mitigation guidelines to prevent pandemic influenza — United States, 2017. MMWR. Recomm. Reports. Apr. 2017; 66 (1):1–34. [ PMC free article ] [ PubMed ] [ Google Scholar ]
- Selden T.M., Berdahl T.A. COVID-19 and racial/ethnic disparities in health risk, employment, and household composition. Health Affairs. Sep. 2020; 39 (9):1624–1632. [ PubMed ] [ Google Scholar ]
- Sharifi A., Khavarian-Garmsir A.R. The COVID-19 pandemic: Impacts on cities and major lessons for urban planning, design, and management. The Science of the Total Environment. 2020; 749 :1–3. 2020. [ PMC free article ] [ PubMed ] [ Google Scholar ]
- Song M.K., Lin F.C., Ward S.E., Fine J.P. Composite variables: When and how. Nursing Research. Jan. 2013; 62 (1):45–49. [ PMC free article ] [ PubMed ] [ Google Scholar ]
- The National Bureau of Economic Research https://www.nber.org/cycles.html At:
- Thebault Reis, Tran Andrew Ba, Williams Vanessa. “African Americans are at higher risk of deathfrom coronavirus”, The Washington Post, 07-Apr-2020. The Washington Post. 2020 https://www.washingtonpost.com/nation/2020/04/07/coronavirus-is-infecting-killing-black-americans-an-alarmingly-high-rate-post-analysis-shows/ [ Google Scholar ]
- U. S. Census Bureau 2018 American Community Survey (ACS 2018 5-year) 2020. https://www.census.gov/programs-surveys/acs/data.html [Online]. Available:
- Wade L. An unequal blow. Science. 2020; 368 (6492):700–703. [ PubMed ] [ Google Scholar ]
- Watson D.F., Philip G.M. A refinement of Inverse distance weighted interpolation. Geo-Processing. 1985; 2 :315–327. [ Google Scholar ]
- Weinstock L.R. Prepared by the congressional research service (CRS). CRS report IN11460. 2020. COVID-19: How quickly will unemployment recover? https://crsreports.congress.gov/product/pdf/IN/IN11460 AT: [ Google Scholar ]
- Wilkinson A., Ali H., Bedford J., Boonyabancha S., Connolly C., Conteh A., Dean L., Decorte F., Dercon B., Dias S., Dodman D., Duijsens R., D’Urzo S., Eamer G., Earle L., Gupte J., Frediani A.A., Hasan A., Hawkins K.…Whittaker L. Local response in health emergencies: Key considerations for addressing the COVID-19 pandemic in informal urban settlements. Environment and Urbanization. 2020; 32 (2):503–522. https://journals.sagepub.com/doi/full/10.1177/ [ PMC free article ] [ PubMed ] [ Google Scholar ]
- Worldometers COVID-19 coronavirus pandemic. 2020. https://www.worldometers.info/coronavirus/country/us/ At:
- Wu D., Yu L., Yang T., Cottrell R., Peng S., Guo W., Jiang S. The Impacts of Uncertainty Stress on Mental Disorders of Chinese College Students: Evidence From a Nationwide Study. Frontiers in Psychology. 2020; 11 :243. [ PMC free article ] [ PubMed ] [ Google Scholar ]

- SUGGESTED TOPICS
- The Magazine
- Newsletters
- Managing Yourself
- Managing Teams
- Work-life Balance
- The Big Idea
- Data & Visuals
- Reading Lists
- Case Selections
- HBR Learning
- Topic Feeds
- Account Settings
- Email Preferences
A Crisis of Long-Term Unemployment Is Looming in the U.S.
- Ofer Sharone

How biases trap qualified job seekers in a cycle of rejection — and how to help them break free.
The stigma of long-term unemployment can be profound and long-lasting. As the United States eases out of the Covid-19 pandemic, it needs better approaches to LTU compared to the Great Recession. But research shows that stubborn biases among hiring managers can make the lived experiences of jobseekers distressing, leading to a vicious cycle of diminished emotional well-being that can make it all but impossible to land a role. Instead of sticking with the standard ways of helping the LTU, however, a pilot program that uses a wider, sociologically-oriented lens can help jobseekers understand that their inability to land a gig isn’t their fault. This can help people go easier on themselves which, ultimately, can make it more likely that they’ll find a new position.
Covid-19 has ravaged employment in the United States, from temporary furloughs to outright layoffs. Currently, over 4 million Americans have been out of work for six months or more , including an estimated 1.5 million workers in white-collar occupations, according to my calculations. Though the overall unemployment rate is down from its peak last spring, the percent of the unemployed who are long-term unemployed (LTU) keeps increasing and is currently at over 40%, a level of LTU comparable to the Great Recession but otherwise unseen in the U.S. in over 60 years.

- Ofer Sharone is an expert on long-term unemployment and the author of the book Flawed System/Flawed Self: Job Searching and Unemployment Experiences (University of Chicago Press). Sharone received his PhD in sociology from the University of California Berkeley, his JD from Harvard Law School, and is currently an associate professor of sociology at the University of Massachusetts Amherst.
Partner Center
- Search Menu
- Advance articles
- Featured articles
- Virtual Issues
- Browse content in B - History of Economic Thought, Methodology, and Heterodox Approaches
- Browse content in B4 - Economic Methodology
- B49 - Other
- Browse content in C - Mathematical and Quantitative Methods
- Browse content in C0 - General
- C01 - Econometrics
- Browse content in C1 - Econometric and Statistical Methods and Methodology: General
- C10 - General
- C11 - Bayesian Analysis: General
- C12 - Hypothesis Testing: General
- C13 - Estimation: General
- C14 - Semiparametric and Nonparametric Methods: General
- C15 - Statistical Simulation Methods: General
- Browse content in C2 - Single Equation Models; Single Variables
- C21 - Cross-Sectional Models; Spatial Models; Treatment Effect Models; Quantile Regressions
- C22 - Time-Series Models; Dynamic Quantile Regressions; Dynamic Treatment Effect Models; Diffusion Processes
- C23 - Panel Data Models; Spatio-temporal Models
- C26 - Instrumental Variables (IV) Estimation
- Browse content in C3 - Multiple or Simultaneous Equation Models; Multiple Variables
- C31 - Cross-Sectional Models; Spatial Models; Treatment Effect Models; Quantile Regressions; Social Interaction Models
- C33 - Panel Data Models; Spatio-temporal Models
- C34 - Truncated and Censored Models; Switching Regression Models
- C35 - Discrete Regression and Qualitative Choice Models; Discrete Regressors; Proportions
- C36 - Instrumental Variables (IV) Estimation
- Browse content in C4 - Econometric and Statistical Methods: Special Topics
- C41 - Duration Analysis; Optimal Timing Strategies
- C44 - Operations Research; Statistical Decision Theory
- Browse content in C5 - Econometric Modeling
- C50 - General
- C51 - Model Construction and Estimation
- C52 - Model Evaluation, Validation, and Selection
- C53 - Forecasting and Prediction Methods; Simulation Methods
- C55 - Large Data Sets: Modeling and Analysis
- C57 - Econometrics of Games and Auctions
- Browse content in C6 - Mathematical Methods; Programming Models; Mathematical and Simulation Modeling
- C61 - Optimization Techniques; Programming Models; Dynamic Analysis
- C62 - Existence and Stability Conditions of Equilibrium
- C63 - Computational Techniques; Simulation Modeling
- C67 - Input-Output Models
- Browse content in C7 - Game Theory and Bargaining Theory
- C70 - General
- C72 - Noncooperative Games
- C73 - Stochastic and Dynamic Games; Evolutionary Games; Repeated Games
- C78 - Bargaining Theory; Matching Theory
- Browse content in C8 - Data Collection and Data Estimation Methodology; Computer Programs
- C83 - Survey Methods; Sampling Methods
- Browse content in C9 - Design of Experiments
- C90 - General
- C91 - Laboratory, Individual Behavior
- C92 - Laboratory, Group Behavior
- C93 - Field Experiments
- Browse content in D - Microeconomics
- Browse content in D0 - General
- D01 - Microeconomic Behavior: Underlying Principles
- D02 - Institutions: Design, Formation, Operations, and Impact
- D03 - Behavioral Microeconomics: Underlying Principles
- Browse content in D1 - Household Behavior and Family Economics
- D11 - Consumer Economics: Theory
- D12 - Consumer Economics: Empirical Analysis
- D13 - Household Production and Intrahousehold Allocation
- D14 - Household Saving; Personal Finance
- D15 - Intertemporal Household Choice: Life Cycle Models and Saving
- D18 - Consumer Protection
- Browse content in D2 - Production and Organizations
- D21 - Firm Behavior: Theory
- D22 - Firm Behavior: Empirical Analysis
- D23 - Organizational Behavior; Transaction Costs; Property Rights
- D24 - Production; Cost; Capital; Capital, Total Factor, and Multifactor Productivity; Capacity
- D25 - Intertemporal Firm Choice: Investment, Capacity, and Financing
- D29 - Other
- Browse content in D3 - Distribution
- D30 - General
- D31 - Personal Income, Wealth, and Their Distributions
- Browse content in D4 - Market Structure, Pricing, and Design
- D40 - General
- D41 - Perfect Competition
- D42 - Monopoly
- D43 - Oligopoly and Other Forms of Market Imperfection
- D44 - Auctions
- D47 - Market Design
- Browse content in D5 - General Equilibrium and Disequilibrium
- D50 - General
- D51 - Exchange and Production Economies
- D52 - Incomplete Markets
- D53 - Financial Markets
- D57 - Input-Output Tables and Analysis
- D58 - Computable and Other Applied General Equilibrium Models
- Browse content in D6 - Welfare Economics
- D60 - General
- D61 - Allocative Efficiency; Cost-Benefit Analysis
- D62 - Externalities
- D63 - Equity, Justice, Inequality, and Other Normative Criteria and Measurement
- D64 - Altruism; Philanthropy
- Browse content in D7 - Analysis of Collective Decision-Making
- D70 - General
- D71 - Social Choice; Clubs; Committees; Associations
- D72 - Political Processes: Rent-seeking, Lobbying, Elections, Legislatures, and Voting Behavior
- D73 - Bureaucracy; Administrative Processes in Public Organizations; Corruption
- D74 - Conflict; Conflict Resolution; Alliances; Revolutions
- D78 - Positive Analysis of Policy Formulation and Implementation
- Browse content in D8 - Information, Knowledge, and Uncertainty
- D80 - General
- D81 - Criteria for Decision-Making under Risk and Uncertainty
- D82 - Asymmetric and Private Information; Mechanism Design
- D83 - Search; Learning; Information and Knowledge; Communication; Belief; Unawareness
- D84 - Expectations; Speculations
- D85 - Network Formation and Analysis: Theory
- D86 - Economics of Contract: Theory
- Browse content in D9 - Micro-Based Behavioral Economics
- D90 - General
- D91 - Role and Effects of Psychological, Emotional, Social, and Cognitive Factors on Decision Making
- D92 - Intertemporal Firm Choice, Investment, Capacity, and Financing
- Browse content in E - Macroeconomics and Monetary Economics
- Browse content in E0 - General
- E03 - Behavioral Macroeconomics
- Browse content in E1 - General Aggregative Models
- E10 - General
- E12 - Keynes; Keynesian; Post-Keynesian
- E13 - Neoclassical
- E17 - Forecasting and Simulation: Models and Applications
- Browse content in E2 - Consumption, Saving, Production, Investment, Labor Markets, and Informal Economy
- E20 - General
- E21 - Consumption; Saving; Wealth
- E22 - Investment; Capital; Intangible Capital; Capacity
- E23 - Production
- E24 - Employment; Unemployment; Wages; Intergenerational Income Distribution; Aggregate Human Capital; Aggregate Labor Productivity
- E25 - Aggregate Factor Income Distribution
- E26 - Informal Economy; Underground Economy
- E27 - Forecasting and Simulation: Models and Applications
- Browse content in E3 - Prices, Business Fluctuations, and Cycles
- E30 - General
- E31 - Price Level; Inflation; Deflation
- E32 - Business Fluctuations; Cycles
- Browse content in E4 - Money and Interest Rates
- E40 - General
- E41 - Demand for Money
- E42 - Monetary Systems; Standards; Regimes; Government and the Monetary System; Payment Systems
- E43 - Interest Rates: Determination, Term Structure, and Effects
- E44 - Financial Markets and the Macroeconomy
- E49 - Other
- Browse content in E5 - Monetary Policy, Central Banking, and the Supply of Money and Credit
- E50 - General
- E51 - Money Supply; Credit; Money Multipliers
- E52 - Monetary Policy
- E58 - Central Banks and Their Policies
- Browse content in E6 - Macroeconomic Policy, Macroeconomic Aspects of Public Finance, and General Outlook
- E60 - General
- E61 - Policy Objectives; Policy Designs and Consistency; Policy Coordination
- E62 - Fiscal Policy
- E65 - Studies of Particular Policy Episodes
- Browse content in E7 - Macro-Based Behavioral Economics
- E70 - General
- E71 - Role and Effects of Psychological, Emotional, Social, and Cognitive Factors on the Macro Economy
- Browse content in F - International Economics
- Browse content in F1 - Trade
- F10 - General
- F11 - Neoclassical Models of Trade
- F12 - Models of Trade with Imperfect Competition and Scale Economies; Fragmentation
- F13 - Trade Policy; International Trade Organizations
- F14 - Empirical Studies of Trade
- F15 - Economic Integration
- F16 - Trade and Labor Market Interactions
- F17 - Trade Forecasting and Simulation
- F18 - Trade and Environment
- F19 - Other
- Browse content in F2 - International Factor Movements and International Business
- F22 - International Migration
- F23 - Multinational Firms; International Business
- Browse content in F3 - International Finance
- F30 - General
- F31 - Foreign Exchange
- F32 - Current Account Adjustment; Short-Term Capital Movements
- F33 - International Monetary Arrangements and Institutions
- F34 - International Lending and Debt Problems
- F36 - Financial Aspects of Economic Integration
- Browse content in F4 - Macroeconomic Aspects of International Trade and Finance
- F40 - General
- F41 - Open Economy Macroeconomics
- F42 - International Policy Coordination and Transmission
- F43 - Economic Growth of Open Economies
- F44 - International Business Cycles
- Browse content in F5 - International Relations, National Security, and International Political Economy
- F50 - General
- F53 - International Agreements and Observance; International Organizations
- Browse content in F6 - Economic Impacts of Globalization
- F60 - General
- F63 - Economic Development
- F64 - Environment
- F65 - Finance
- Browse content in G - Financial Economics
- Browse content in G0 - General
- G01 - Financial Crises
- G02 - Behavioral Finance: Underlying Principles
- Browse content in G1 - General Financial Markets
- G10 - General
- G11 - Portfolio Choice; Investment Decisions
- G12 - Asset Pricing; Trading volume; Bond Interest Rates
- G13 - Contingent Pricing; Futures Pricing
- G14 - Information and Market Efficiency; Event Studies; Insider Trading
- G15 - International Financial Markets
- G18 - Government Policy and Regulation
- Browse content in G2 - Financial Institutions and Services
- G20 - General
- G21 - Banks; Depository Institutions; Micro Finance Institutions; Mortgages
- G22 - Insurance; Insurance Companies; Actuarial Studies
- G23 - Non-bank Financial Institutions; Financial Instruments; Institutional Investors
- G24 - Investment Banking; Venture Capital; Brokerage; Ratings and Ratings Agencies
- G28 - Government Policy and Regulation
- Browse content in G3 - Corporate Finance and Governance
- G30 - General
- G31 - Capital Budgeting; Fixed Investment and Inventory Studies; Capacity
- G32 - Financing Policy; Financial Risk and Risk Management; Capital and Ownership Structure; Value of Firms; Goodwill
- G33 - Bankruptcy; Liquidation
- G34 - Mergers; Acquisitions; Restructuring; Corporate Governance
- G38 - Government Policy and Regulation
- Browse content in G4 - Behavioral Finance
- G40 - General
- G41 - Role and Effects of Psychological, Emotional, Social, and Cognitive Factors on Decision Making in Financial Markets
- Browse content in H - Public Economics
- Browse content in H0 - General
- H00 - General
- Browse content in H1 - Structure and Scope of Government
- H11 - Structure, Scope, and Performance of Government
- Browse content in H2 - Taxation, Subsidies, and Revenue
- H20 - General
- H21 - Efficiency; Optimal Taxation
- H23 - Externalities; Redistributive Effects; Environmental Taxes and Subsidies
- H24 - Personal Income and Other Nonbusiness Taxes and Subsidies; includes inheritance and gift taxes
- H25 - Business Taxes and Subsidies
- H26 - Tax Evasion and Avoidance
- Browse content in H3 - Fiscal Policies and Behavior of Economic Agents
- H30 - General
- H31 - Household
- Browse content in H4 - Publicly Provided Goods
- H41 - Public Goods
- Browse content in H5 - National Government Expenditures and Related Policies
- H50 - General
- H51 - Government Expenditures and Health
- H52 - Government Expenditures and Education
- H53 - Government Expenditures and Welfare Programs
- H55 - Social Security and Public Pensions
- H56 - National Security and War
- Browse content in H6 - National Budget, Deficit, and Debt
- H60 - General
- H63 - Debt; Debt Management; Sovereign Debt
- Browse content in H7 - State and Local Government; Intergovernmental Relations
- H71 - State and Local Taxation, Subsidies, and Revenue
- H75 - State and Local Government: Health; Education; Welfare; Public Pensions
- Browse content in H8 - Miscellaneous Issues
- H81 - Governmental Loans; Loan Guarantees; Credits; Grants; Bailouts
- Browse content in I - Health, Education, and Welfare
- Browse content in I0 - General
- I00 - General
- Browse content in I1 - Health
- I10 - General
- I11 - Analysis of Health Care Markets
- I12 - Health Behavior
- I13 - Health Insurance, Public and Private
- I14 - Health and Inequality
- I15 - Health and Economic Development
- I18 - Government Policy; Regulation; Public Health
- Browse content in I2 - Education and Research Institutions
- I20 - General
- I21 - Analysis of Education
- I22 - Educational Finance; Financial Aid
- I23 - Higher Education; Research Institutions
- I24 - Education and Inequality
- I25 - Education and Economic Development
- I26 - Returns to Education
- I28 - Government Policy
- Browse content in I3 - Welfare, Well-Being, and Poverty
- I30 - General
- I31 - General Welfare
- I32 - Measurement and Analysis of Poverty
- I38 - Government Policy; Provision and Effects of Welfare Programs
- I39 - Other
- Browse content in J - Labor and Demographic Economics
- Browse content in J0 - General
- J00 - General
- J01 - Labor Economics: General
- J08 - Labor Economics Policies
- Browse content in J1 - Demographic Economics
- J10 - General
- J11 - Demographic Trends, Macroeconomic Effects, and Forecasts
- J12 - Marriage; Marital Dissolution; Family Structure; Domestic Abuse
- J13 - Fertility; Family Planning; Child Care; Children; Youth
- J14 - Economics of the Elderly; Economics of the Handicapped; Non-Labor Market Discrimination
- J15 - Economics of Minorities, Races, Indigenous Peoples, and Immigrants; Non-labor Discrimination
- J16 - Economics of Gender; Non-labor Discrimination
- J17 - Value of Life; Forgone Income
- Browse content in J2 - Demand and Supply of Labor
- J20 - General
- J21 - Labor Force and Employment, Size, and Structure
- J22 - Time Allocation and Labor Supply
- J23 - Labor Demand
- J24 - Human Capital; Skills; Occupational Choice; Labor Productivity
- Browse content in J3 - Wages, Compensation, and Labor Costs
- J30 - General
- J31 - Wage Level and Structure; Wage Differentials
- J32 - Nonwage Labor Costs and Benefits; Retirement Plans; Private Pensions
- J33 - Compensation Packages; Payment Methods
- J38 - Public Policy
- Browse content in J4 - Particular Labor Markets
- J41 - Labor Contracts
- J42 - Monopsony; Segmented Labor Markets
- J44 - Professional Labor Markets; Occupational Licensing
- Browse content in J5 - Labor-Management Relations, Trade Unions, and Collective Bargaining
- J50 - General
- J52 - Dispute Resolution: Strikes, Arbitration, and Mediation; Collective Bargaining
- Browse content in J6 - Mobility, Unemployment, Vacancies, and Immigrant Workers
- J60 - General
- J61 - Geographic Labor Mobility; Immigrant Workers
- J62 - Job, Occupational, and Intergenerational Mobility
- J63 - Turnover; Vacancies; Layoffs
- J64 - Unemployment: Models, Duration, Incidence, and Job Search
- J65 - Unemployment Insurance; Severance Pay; Plant Closings
- J68 - Public Policy
- Browse content in J7 - Labor Discrimination
- J71 - Discrimination
- Browse content in J8 - Labor Standards: National and International
- J81 - Working Conditions
- J82 - Labor Force Composition
- J83 - Workers' Rights
- Browse content in K - Law and Economics
- Browse content in K0 - General
- K00 - General
- Browse content in K1 - Basic Areas of Law
- K14 - Criminal Law
- Browse content in K3 - Other Substantive Areas of Law
- K31 - Labor Law
- K33 - International Law
- K35 - Personal Bankruptcy Law
- Browse content in K4 - Legal Procedure, the Legal System, and Illegal Behavior
- K40 - General
- K41 - Litigation Process
- K42 - Illegal Behavior and the Enforcement of Law
- Browse content in L - Industrial Organization
- Browse content in L0 - General
- L00 - General
- Browse content in L1 - Market Structure, Firm Strategy, and Market Performance
- L10 - General
- L11 - Production, Pricing, and Market Structure; Size Distribution of Firms
- L12 - Monopoly; Monopolization Strategies
- L13 - Oligopoly and Other Imperfect Markets
- L14 - Transactional Relationships; Contracts and Reputation; Networks
- L15 - Information and Product Quality; Standardization and Compatibility
- Browse content in L2 - Firm Objectives, Organization, and Behavior
- L20 - General
- L22 - Firm Organization and Market Structure
- L23 - Organization of Production
- L25 - Firm Performance: Size, Diversification, and Scope
- Browse content in L3 - Nonprofit Organizations and Public Enterprise
- L31 - Nonprofit Institutions; NGOs; Social Entrepreneurship
- Browse content in L4 - Antitrust Issues and Policies
- L41 - Monopolization; Horizontal Anticompetitive Practices
- L42 - Vertical Restraints; Resale Price Maintenance; Quantity Discounts
- L43 - Legal Monopolies and Regulation or Deregulation
- Browse content in L5 - Regulation and Industrial Policy
- L50 - General
- L51 - Economics of Regulation
- Browse content in L6 - Industry Studies: Manufacturing
- L60 - General
- L62 - Automobiles; Other Transportation Equipment; Related Parts and Equipment
- L63 - Microelectronics; Computers; Communications Equipment
- Browse content in L7 - Industry Studies: Primary Products and Construction
- L71 - Mining, Extraction, and Refining: Hydrocarbon Fuels
- Browse content in L8 - Industry Studies: Services
- L81 - Retail and Wholesale Trade; e-Commerce
- L82 - Entertainment; Media
- Browse content in L9 - Industry Studies: Transportation and Utilities
- L93 - Air Transportation
- L94 - Electric Utilities
- L96 - Telecommunications
- Browse content in M - Business Administration and Business Economics; Marketing; Accounting; Personnel Economics
- Browse content in M0 - General
- M00 - General
- Browse content in M1 - Business Administration
- M11 - Production Management
- M14 - Corporate Culture; Social Responsibility
- Browse content in M2 - Business Economics
- M21 - Business Economics
- Browse content in M3 - Marketing and Advertising
- M31 - Marketing
- M37 - Advertising
- Browse content in M5 - Personnel Economics
- M50 - General
- M51 - Firm Employment Decisions; Promotions
- M52 - Compensation and Compensation Methods and Their Effects
- M54 - Labor Management
- M55 - Labor Contracting Devices
- Browse content in N - Economic History
- Browse content in N1 - Macroeconomics and Monetary Economics; Industrial Structure; Growth; Fluctuations
- N10 - General, International, or Comparative
- Browse content in N2 - Financial Markets and Institutions
- N20 - General, International, or Comparative
- Browse content in N3 - Labor and Consumers, Demography, Education, Health, Welfare, Income, Wealth, Religion, and Philanthropy
- N31 - U.S.; Canada: Pre-1913
- N32 - U.S.; Canada: 1913-
- N34 - Europe: 1913-
- Browse content in N4 - Government, War, Law, International Relations, and Regulation
- N42 - U.S.; Canada: 1913-
- N43 - Europe: Pre-1913
- N44 - Europe: 1913-
- N45 - Asia including Middle East
- Browse content in N9 - Regional and Urban History
- N90 - General, International, or Comparative
- N92 - U.S.; Canada: 1913-
- N94 - Europe: 1913-
- Browse content in O - Economic Development, Innovation, Technological Change, and Growth
- Browse content in O1 - Economic Development
- O10 - General
- O11 - Macroeconomic Analyses of Economic Development
- O12 - Microeconomic Analyses of Economic Development
- O13 - Agriculture; Natural Resources; Energy; Environment; Other Primary Products
- O14 - Industrialization; Manufacturing and Service Industries; Choice of Technology
- O15 - Human Resources; Human Development; Income Distribution; Migration
- O16 - Financial Markets; Saving and Capital Investment; Corporate Finance and Governance
- O17 - Formal and Informal Sectors; Shadow Economy; Institutional Arrangements
- O18 - Urban, Rural, Regional, and Transportation Analysis; Housing; Infrastructure
- Browse content in O2 - Development Planning and Policy
- O23 - Fiscal and Monetary Policy in Development
- Browse content in O3 - Innovation; Research and Development; Technological Change; Intellectual Property Rights
- O30 - General
- O31 - Innovation and Invention: Processes and Incentives
- O32 - Management of Technological Innovation and R&D
- O33 - Technological Change: Choices and Consequences; Diffusion Processes
- O34 - Intellectual Property and Intellectual Capital
- O38 - Government Policy
- Browse content in O4 - Economic Growth and Aggregate Productivity
- O40 - General
- O41 - One, Two, and Multisector Growth Models
- O42 - Monetary Growth Models
- O43 - Institutions and Growth
- O44 - Environment and Growth
- O47 - Empirical Studies of Economic Growth; Aggregate Productivity; Cross-Country Output Convergence
- Browse content in O5 - Economywide Country Studies
- O51 - U.S.; Canada
- O55 - Africa
- Browse content in P - Economic Systems
- Browse content in P0 - General
- P00 - General
- Browse content in P2 - Socialist Systems and Transitional Economies
- P26 - Political Economy; Property Rights
- Browse content in Q - Agricultural and Natural Resource Economics; Environmental and Ecological Economics
- Browse content in Q1 - Agriculture
- Q15 - Land Ownership and Tenure; Land Reform; Land Use; Irrigation; Agriculture and Environment
- Q16 - R&D; Agricultural Technology; Biofuels; Agricultural Extension Services
- Browse content in Q3 - Nonrenewable Resources and Conservation
- Q33 - Resource Booms
- Browse content in Q4 - Energy
- Q41 - Demand and Supply; Prices
- Q43 - Energy and the Macroeconomy
- Browse content in Q5 - Environmental Economics
- Q51 - Valuation of Environmental Effects
- Q53 - Air Pollution; Water Pollution; Noise; Hazardous Waste; Solid Waste; Recycling
- Q54 - Climate; Natural Disasters; Global Warming
- Q55 - Technological Innovation
- Q56 - Environment and Development; Environment and Trade; Sustainability; Environmental Accounts and Accounting; Environmental Equity; Population Growth
- Q58 - Government Policy
- Browse content in R - Urban, Rural, Regional, Real Estate, and Transportation Economics
- Browse content in R1 - General Regional Economics
- R10 - General
- R11 - Regional Economic Activity: Growth, Development, Environmental Issues, and Changes
- R12 - Size and Spatial Distributions of Regional Economic Activity
- R13 - General Equilibrium and Welfare Economic Analysis of Regional Economies
- R15 - Econometric and Input-Output Models; Other Models
- Browse content in R2 - Household Analysis
- R21 - Housing Demand
- R23 - Regional Migration; Regional Labor Markets; Population; Neighborhood Characteristics
- Browse content in R3 - Real Estate Markets, Spatial Production Analysis, and Firm Location
- R31 - Housing Supply and Markets
- R33 - Nonagricultural and Nonresidential Real Estate Markets
- Browse content in R4 - Transportation Economics
- R41 - Transportation: Demand, Supply, and Congestion; Travel Time; Safety and Accidents; Transportation Noise
- R48 - Government Pricing and Policy
- Browse content in R5 - Regional Government Analysis
- R51 - Finance in Urban and Rural Economies
- Browse content in Z - Other Special Topics
- Browse content in Z1 - Cultural Economics; Economic Sociology; Economic Anthropology
- Z10 - General
- Z12 - Religion
- Z13 - Economic Sociology; Economic Anthropology; Social and Economic Stratification
- Author Guidelines
- Submission Site
- Open Access
- About The Review of Economic Studies
- Editorial Board
- Advertising and Corporate Services
- Self-Archiving Policy
- Dispatch Dates
- Journals on Oxford Academic
- Books on Oxford Academic
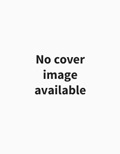
Article Contents
- < Previous
Growth and Unemployment
- Article contents
- Figures & tables
- Supplementary Data
Philippe Aghion, Peter Howitt, Growth and Unemployment, The Review of Economic Studies , Volume 61, Issue 3, July 1994, Pages 477–494, https://doi.org/10.2307/2297900
- Permissions Icon Permissions
This paper analyses the effects of growth on long-run unemployment using a search model of equilibrium unemployment where growth arises explicitly from the introduction of new technologies that require labour reallocation for their implementation.
The analysis uncovers and compares between two competing effects of growth on unemployment. The first is a capitalisation effect, whereby an increase in growth raises the capitalised returns from creating jobs and consequently reduces the equilibrium rate of unemployment. The second is a creative destruction effect whereby an increase in growth reduces the duration of a job match, thereby raising the equilibrium level of unemployment both directly, by raising the job separation rate, and indirectly, by discouraging the creation of job vacancies.
Email alerts
Citing articles via.
- Recommend to your Library
- Journals Career Network
Affiliations
- Online ISSN 1467-937X
- Print ISSN 0034-6527
- Copyright © 2024 Review of Economic Studies Ltd
- About Oxford Academic
- Publish journals with us
- University press partners
- What we publish
- New features
- Open access
- Institutional account management
- Rights and permissions
- Get help with access
- Accessibility
- Advertising
- Media enquiries
- Oxford University Press
- Oxford Languages
- University of Oxford
Oxford University Press is a department of the University of Oxford. It furthers the University's objective of excellence in research, scholarship, and education by publishing worldwide
- Copyright © 2024 Oxford University Press
- Cookie settings
- Cookie policy
- Privacy policy
- Legal notice
This Feature Is Available To Subscribers Only
Sign In or Create an Account
This PDF is available to Subscribers Only
For full access to this pdf, sign in to an existing account, or purchase an annual subscription.
Academia.edu no longer supports Internet Explorer.
To browse Academia.edu and the wider internet faster and more securely, please take a few seconds to upgrade your browser .
Enter the email address you signed up with and we'll email you a reset link.
- We're Hiring!
- Help Center
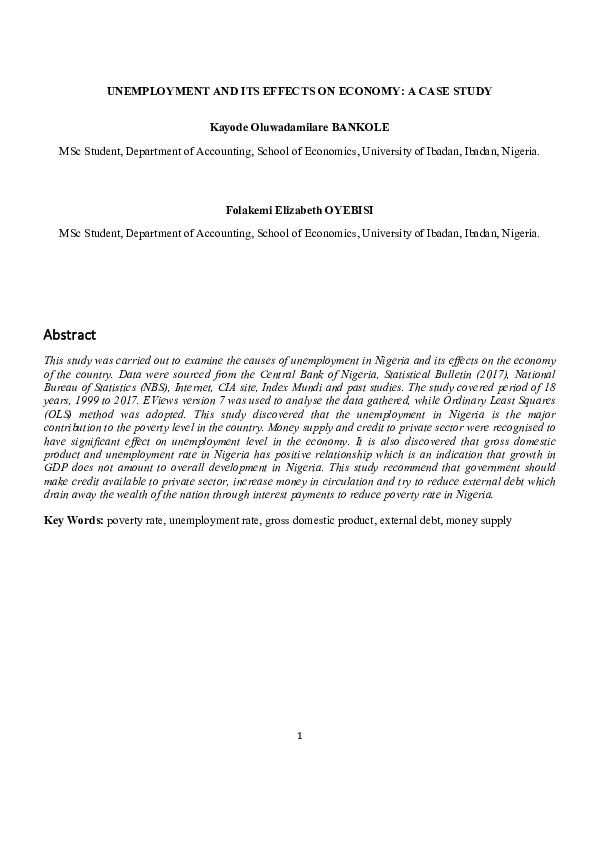
UNEMPLOYMENT AND ITS EFFECTS ON ECONOMY: A CASE STUDY

This study was carried out to examine the causes of unemployment in Nigeria and its effects on the economy of the country. Data were sourced from the Central Bank of Nigeria, Statistical Bulletin (2017), National Bureau of Statistics (NBS), Internet, CIA site, Index Mundi and past studies. The study covered period of 18 years, 1999 to 2017. EViews version 7 was used to analyse the data gathered, while Ordinary Least Squares (OLS) method was adopted. This study discovered that the unemployment in Nigeria is the major contribution to the poverty level in the country. Money supply and credit to private sector were recognised to have significant effect on unemployment level in the economy. It is also discovered that gross domestic product and unemployment rate in Nigeria has positive relationship which is an indication that growth in GDP does not amount to overall development in Nigeria. This study recommend that government should make credit available to private sector, increase money in circulation and try to reduce external debt which drain away the wealth of the nation through interest payments to reduce poverty rate in Nigeria.
RELATED TOPICS
- We're Hiring!
- Help Center
- Find new research papers in:
- Health Sciences
- Earth Sciences
- Cognitive Science
- Mathematics
- Computer Science
- Academia ©2024
Race and underemployment in the US labor market
Subscribe to the economic studies bulletin, ryan nunn , ryan nunn assistant vice president for applied research in community development - federal reserve bank of minneapolis @ryandnunn jana parsons , and jana parsons former research analyst - the hamilton project @janamkparsons jay shambaugh jay shambaugh under secretary for international affairs - u.s. department of the treasury @jaycshambaugh.
August 1, 2019
Each month a new reading of the unemployment rate helps us assess the health of the labor market. However, as many have pointed out , the unemployment rate is in some ways a narrow measure of the labor market that misses important aspects of labor market distress. A broader indicator of labor market weakness called the under employment rate—and in Bureau of Labor Statistics jargon referred to as the U-6 unemployment rate—takes into account some of this additional distress. Examining both unemployment and underemployment is useful for analyzing different aspects of the labor market, and, as shown below, it can reveal dramatic racial disparities.
In addition to the number of unemployed (those without a job and actively seeking work), the underemployment rate captures the number of people who work part time but would rather have a full-time job (called “part time for economic reasons”) and those who want and can take a job but have not looked for work in the past four weeks (called “marginally attached”). These groups make sense to include in a measure of underemployment because while they are not unemployed in the formal sense, they would work more if the option presented itself. While the unemployed are often those most ready to take new jobs, workers who are marginally attached and part time for economic reasons also stand ready to take full-time employment when employers are hiring.
At its peak in the wake of the Great Recession, the underemployment rate was 17.1 percent in October 2009, indicating that more than one in six people were experiencing some sort of labor market hardship (see figure 1). This was far above the 10.0 percent unemployment rate at the time and demonstrates the wide swath of individuals who were in labor market distress in the aftermath of the Great Recession. Since then the underemployment rate has steadily declined, and is now below its prerecession low, but it did not fall below its prerecession low for nearly a year after the unemployment rate did. In addition, at 7.2 percent in June 2019, the underemployment rate is nearly double the June 2019 unemployment rate of 3.7 percent. This makes clear that while a relatively small percentage of people are both out of work and currently searching for a job, there is still a considerable amount of underutilized labor and many people for whom the labor market is not providing adequate opportunities.

In 2010, after the Great Recession had officially ended, the average annual unemployment rate for black workers was 16.0 percent, compared to 12.5 and 8.7 percent for Hispanics and whites, respectively. The black unemployment rate is typically about twice as high as the white unemployment rate. Economists Cajner, Radler, Ratner, and Vidangos find that observable characteristics such as education, age, and marital status explain relatively little of the gap. Instead, black workers have a much higher risk of losing their jobs, accounting for much of the unemployment rate gap.
While unemployment rates have fallen substantially since 2010, racial disparities still exist today: in the first half of 2019 the unemployment rate was 6.6 percent for blacks, 4.4 percent for Hispanics, and 3.3 percent for whites. Moreover, as Andre Perry notes , national estimates of the unemployment rate mask the wide geographic differences in the labor market experiences of minorities.
Like the standard unemployment rate, the underemployment rate reveals very different labor market outcomes for black, Hispanic, and white workers (see figure 2). Below, we highlight a few of the distinct insights the underemployment rate provides about the varied labor market experiences of American workers.
- White workers have lower underemployment rates than black or Hispanic workers at all points in the business cycle. In fact, the peak white underemployment rate in the wake of the Great Recession was only slightly higher than the prerecession low for black underemployment.
- For black workers, the underemployment rate is strikingly high: black underemployment reached 24.9 percent in April 2011, well after the peak of the black unemployment rate at 16.8 percent in March 2010. That is, for more than a year after the black unemployment rate began to fall, the underemployment rate continued to rise.
- The Hispanic underemployment rate rose 12 percentage points from December 2007 to December 2009, compared to 10 percentage points for blacks and just under 7 percentage points for whites.
- This was predominantly driven by increases in unemployment and the number of those working part time for economic reasons (i.e., those who would like full-time jobs but cannot find them) rather than changes in the number of marginally attached workers.
- Much of the gap between white and Hispanic involuntary part-time work can be explained by observable characteristics like education, industry, and occupation.

The large gaps in underemployment between white and non-white workers seen in figure 2 are driven by differing within-race gender gaps, as shown in figure 3. In December 2018, at 6.3 percentage points, the gap between black and white male underemployment is substantially larger than the 4.6 percentage point black–white gap for women. But the white–Hispanic gap is based on the opposite pattern: the underemployment gap for women (4.7 percentage points) is larger than the gap for men (2.8 percentage points).

The unemployment rate is an incredibly valuable summary statistic for the labor market. That statistic is effective in capturing changes in labor market conditions, making it a valuable basis for assessing cyclical patterns—particularly at the start of downturns. But as shown above, the unemployment rate misses the full extent of labor market distress. This omission is especially important during economic downturns and for black and Hispanic workers. The underemployment rate is also helpful for determining the amount of labor market slack that remains once the unemployment rate has fallen to a low level.
A focus on the unemployment rate alone would have suggested that the labor market experience of black workers was improving in 2010 when the underemployment measure showed deterioration, and an exclusive focus on unemployment would also have missed the particularly sharp deterioration of conditions for Hispanic workers in 2007–8. Examining patterns in underemployment is a partial remedy. However, the Bureau of Labor Statistics does not release a seasonally-adjusted monthly series for individual racial groups making underemployment rates less useful for real-time analysis to understand turning points in the business cycle. Still, much can be learned from these data to better understand how well the labor market is functioning for all workers.
Related Content
Ryan Nunn, Jana Parsons, Jay Shambaugh
June 6, 2019
Heather Boushey, Ryan Nunn, Jay Shambaugh
May 16, 2019
Claudia Sahm
Economic Studies
The Hamilton Project
April 4, 2024
April 3, 2024
Benjamin H. Harris, Liam Marshall
April 2, 2024
Cosmopolitan Civil Societies: An Interdisciplinary Journal
Vol. 13, No. 2 2021
Article (Refereed)
Covid-19 Pandemic and Unemployment in Malaysia: A Case Study from Sabah
Janice L. H. Nga, Wijaya Kamal Ramlan, Shafinaz Naim
Universiti Malaysia Sabah
Corresponding author: Janice Nga, Universiti Malaysia Sabah, Jalan UMS, 88400 Kota Kinabalu, Sabah, Malaysia. [email protected]
DOI: http://dx.doi.org/10.5130/ccs.v13.i2.7591
Article History: Received 06/02/2021; Revised 13/05/2021; Accepted 15/06/2021; Published 19/07/2021
Covid-19 not only exposed the vulnerability of most industries especially industries that relies on air travel and tourism but resulted in the exponential increase of unemployment in Malaysia. At the same time, online business or trade and ‘GIG’ economy increased exponentially. The sudden and unexpected loss of jobs had dire consequences for many people. This paper examines how policies enacted during the Covid-19 pandemic affected unemployment in Malaysia by focussing on the situation in Sabah, one of the three remaining partners in the formation of Malaysia. It draws on data from the Family, Women and Youth Survey conducted online towards the end of 2020, as well as secondary data. The study shows that hardship has been faced by many people, especially those previously in professional roles, and those who are younger. The widespread damage to the economy, and to social cohesion, will require significant collaboration between business and industry, the government and the people.
Covid-19; Employment; Unemployment; Malaysia; Sabah
Introduction
Covid-19 was initially a localised health crisis, but it expanded into a global health crisis with severe economic impact, with the speed and scale of which the world has never seen before. Economists have yet to comprehensively measure the effects of the pandemic since the chain of reaction is still progressing and spreading around the world in the form of 2 nd and 3 rd waves ( Chan & King 2020 ) or even 4 th waves ( Ravindran 2021 ; Whyte 2021 ). Covid-19 not only exposed the fundamental flaws in all industries in Malaysia, with the exception of industries in glove-making and pharmaceuticals, but also exposed the fragility of employment. Many companies and industry players have adopted unpaid leave and layoffs to reduce their operational costs so that the company could have sufficient liquidity to tide over this difficulty. Some of these former employees managed to survive in the challenging situation. However, some of them could not withstand the loss of their jobs and choose to commit suicide instead. Blustein et al. claim that unemployment has damaging effects at both individual and community levels, in the aspects of psychological, economic, and social well-being ( Blustein et al. 2020 ; Ferreira et al. 2015 ). The lockdown or Movement Control Orders (MCO) initiated by the government to flatten the infection rate also added to the depression of being retrenched as feelings of isolation also accompanied the lockdown.
The initial shock from the pandemic distinguished the Covid-19 recession as one of the worst ever pandemics ( Ferguson et al. 2020 ). While previous economic hard times, like recessions, were mainly caused by a series of unfortunate economic or financial policies, by 2020, the adversely impacted labour market was severely disrupted, triggered by the emergence of a new virus, worldwide. By the second quarter of 2020, millions of employees were moved into a temporary job loss category. The record-high in temporary unemployment percentage dwarfed previous recessions, which typically begin with an increase in permanent layoffs ( Elsby et al. 2010 ). The path of job vacancies has also been unusual: while vacancies fell throughout the first half of 2020, the drop was much smaller than is typical observed in most recessions due to the ever-increasing popularity of the ‘Gig’ economy ( Umar et al. 2020 ; Lim 2021 ). In fact, vacancies at the lowest point were similar in 2015, when the labour market shrank ( Altig et al. 2020 ). While the Beveridge curve – the negative relationship between vacancies and unemployment – usually ‘loops around’ during and after a recession, the rise in the unemployment rate was much greater than the related decrease in work vacancies in the early months of the Covid-19 recession.
Pandemic and Unemployment
Economic hard times caused by historical pandemics will enable us to establish a long-term impact on health derived directly from the current pandemic’s mortality trends. But what about the ramifications due to the disruption of everyday economic life when people become unemployed? The rapid, severe, and widespread economic disruption caused by the implementation of the MCO to reduce the daily infection spike caused by the spread of the Covid-19 virus is one of its most prominent features in this pandemic. This pandemic may have less in common with other pandemics such as H1N1 swine flu 2009-2010, or the Spanish Flu 1918 in this regard ( Newman 2020 ). For example, the 1918 pandemic’s immediate economic damage pales in comparison to the damage triggered by Covid-19 currently. While this pandemic can provide an insight into long-term social and psychological health effects (direct effect), we need to look elsewhere to consider the long-term consequences of pandemics because of economic downturns, particularly for employment ( Blustein et al. 2020 ; Su et al. 2021 ).
According to the definition of the International Labour Office (ILO), employment comprises of “work performed for others in exchange for pay or profit” ( ILO 2013 ). With that, “employed persons include those who, in their present job, were ‘not at work’ for a short duration but maintained a job attachment during their absence” ( OECD 2020a ). The ILO has reported that there are trends of income inequalities in terms of gender and age. However, different regions may vary in the level of differences for these criteria in the access to employment. For instance, the female labour force participation rate globally is 47% as compared to 74% for their male counterparts ( ILO 2020 ). It should also be pointed out, that the youth face challenges in the labour market such as the growing trend of temporary employment. Unemployment involves individuals with the expected total duration of absence of more than three months or where there is no or unknown expected date for returning to work, and these individuals do not receive any portion of salary or wage from their employers ( OECD 2020a ).
Theoretical modelling of the interaction between pandemics and economic dynamics has been examined by Eichenbaum et al. (2020) . This theoretical model suggests that reductions in consumption and work will slow down the spread of the Covid-19. Unfortunately, these measures also increase the severity of economic recession. It is reported that offline consumption in China dropped by an average of 32% hitting hard across the board ( Chen et al. 2020 ). This reduction in offline consumption cost China over 1.2%of its 2019 GDP in the twelve-week period of initial lockdown, giving a significant estimate of the impact and magnitude faced. Other countries have reported experiencing similar situations ( Chen et al. 2020 ).
As Covid-19 continues to spread across the globe, the expected decline in consumption has since become a reality with unemployment reaching nearly 10 million in March 2020 ( Wolfers 2020 ). Martin et al. (2020) claimed that three months of lockdown during the pandemic recorded an increase of about 17.1 to 25.9% in the poverty rate in San Francisco Bay Area with significant decreases in household saving and consumption. Long recovery times have further exacerbated the slowdown of overall economic activity by low household consumption due to changes in consumption behaviours. Sobieralski (2020) projected that job loss in the airline industry during times of air travel instability, due to most countries restricting or closing their borders to curb the spread, was approximately 7%of the airline workforce. Although air travel was not completely halted, the Covid-19 pandemic caused most airlines to ground their planes and retrench a major portion of their workforce.
The Covid-19 pandemic also impacted other economic activities around the world. De Vito and Gómez (2020) reported the fear of lockdown resulted in a change in consumer demand for products and services. This situation has affected the production and service supply chain at domestic and international levels. The pandemic has caused job losses that led to a reduction in consumer demand and resulted in a global economic recession ( OECD 2020b ). Investment activities were also affected as investors tried to discount the liquidity risk in stock prices. In this regard, the liquidity of listed firms across 26 countries showed a drop of 50%in sales for a full operating firm, with a partial operating firm facing up to a 75% drop in sales ( De Vito & Gómez 2020 ). It was suggested that government fiscal policies, tax deferrals and bridge loans are the most practical policies to overcome the issue of a massive problem with cash flow, a cash crunch.
Pandemic, Unemployment and Mental Health
Unemployment may be generally viewed as an economic issue, but its impacts are actually greater than that as it affects mental health, stress levels and consequently an individual’s quality of life, as well as having an impact on community. As it is, staying employed during economic hard times is one of the most challenging things in life, and when unemployment is prevalent, finding work is doubly difficult. Unemployment is regarded as one of the major discrete and objective life events that necessitate some social or psychological adjustment on the part of the individual in the stress literature (Wheaton 2009). The stress process model ( Pearlin 1981 , 1989 ) is a common explanation for the relationship between unemployment and mental health that is significant in the present scenario.
Although the theoretical model in its original study was based on the effects of stress by people with Parkinson’s disease, its findings are useful and applicable in explaining the stress caused by unemployment due to the Covid-19 pandemic. Pearlin claims that being unemployed increases the likelihood of experiencing stress-inducing factors such as a lack of resources, limited opportunities, and low self-esteem, as well as limiting access to privileges and security ( Pearlin 1989 ). That is, unemployment is likely to result in a variety of losses, including social ties and economic stability, and these losses may be stressful in and of themselves ( Pearlin 1981 ). As a result, unemployment raises the risk of poor mental health. Achdut and Refaeli (2020) through a hierarchical linear model demonstrate that contextual stressors related with the Covid-19 outbreak such as worries and fears of family members being infected, and financial strain might further reinforce the strain caused by unemployment. Additional stress would be in the form of lack of social services for mental health that most government in third world countries are hard pressed to provide. This situation compounds the problem.
Methodology
Primary and secondary data were utilised in this paper. Primary data were collected through two separate sets of questionnaires conducted by the Family, Women and Youth Survey (see Tey 2020 ). The survey was conducted online between 13 November and 5 December 2020. Data were collected from 2503 respondents from all districts in Sabah. The data were collected using stratified sampling method to achieve a representative sample. In that, the number of respondents for each district was pre-determined proportional to the population size, and stratified according to gender, ethnicity, and age of the respondents. Secondary data were mainly sourced from various official agencies and organisations especially online publications and reports.
This paper is structured with an introduction of the background concerning the issue and relates it to theoretical discourses. It continues with methodological and limitations issues, and a discussion on employment in the airlines industry as the most vulnerable industry under threat. While the airline and hotel industries which makes up the bulk of the hospitality part in the tourism sector, were directly affected in this pandemic, many other downstream industries felt the brunt as well. Thus, the general employment situation needs to be examined to assess the impact of the pandemic. For that purpose, this paper includes a discussion about employment in Malaysia as a whole, utilising secondary data. It then scales the discussion to the employment situation in Sabah based on findings from the primary data collected and corroborated to the secondary data. Sabah is selected as case study because of the importance of the tourism sector in its economy, ranking third in the hierarchy of contributing sectors to the economy of Sabah.
Limitations
While this paper attempts to make a first inventory about the Covid-19 pandemic effects on the employment sector, it does have its limitations. Arguably, it is still relatively early to make any determination about long term effects, whether from national government policies or from global contributions. Currently as the pandemic rages on throughout the world, many companies are trying various attempts and measures to survive, with or without laying off staff. Some experts have warned that with the emergence of variants, Covid-19 may become endemic. However, it is hoped that the vaccination process currently underway, worldwide, will put a halt to the spread and in due time, reverse the damage done. Nevertheless, this paper will be a valuable contribution if and when we find ourselves in a post-pandemic era.
The Vulnerable Airline Industry
According to Sobieralski (2020) , the travel restrictions put in place by governments have greatly affected the movement of peoples around the world, while having a negative impact on numerous downstream industries such as the tourism, hospitality and the transportation industries. The issue of flight cancellations and capacity reductions have made the airline industry fight for survival. Some locally grown airlines, for example Malindo Air, had thrown in the towel due to various cash flow problems over the one-year lockdown period, resulting in the majority of its staff losing their jobs, with only 1000 staff remaining employed ( Ram 2020 ; Birruntha 2020 ). For airlines, a reduction in the workforce was unavoidable. It was estimated that nearly 7% of the airlines’ workforce was trimmed with an upper limit of over 13% with major airline workers being most impacted while regional and low-cost workers were the least affected ( Sobieralski 2020 ). The work a person didalso plays a part here. An airline employee who handled flight operations and passengers was the most affected while management employees were relatively little impacted during this period. It is projected that the recovery of this industry will take around 4 to 6 years to return to pre-Covid-19 situation provided government support via plans and financial assistance are forthcoming.
As airlines are the most directly and immediately affected by the travel restrictions put in place by governments, employment in the airline industry is the focus of discussion here. At the beginning of the pandemic, airlines management opted for unpaid leave and pay cuts for its employees. Unpaid leave was seen as a temporary measure to help airlines reduce the cost burden, but it could not reduce the pressure for the potential risk of unemployment to employees. During the period of unpaid leave, the most affected employees were aircrew members, who were not able to work from home like other employees because the nature of their jobs has been serving passengers on flight or in the airport. During the MCO, they had to survive with their existing savings, and they may have needed to find other ways of making ends meet.
As the airline industry could not operate normally during this period, some aircrew members who had been flying for many years chose to start their own businesses to earn an income to supplement their daily needs. The first MCO in Malaysia was imposed from 18 March 2020. According to a report in Malay Mail , a pilot named Johan MD Rosnan took the unpaid leave program from the beginning of the MCO ( Zikri 2020 ). Since the international borders of most countries and regions closed, this pilot, who flew international flights, has not had any job assigned to him. It is fortunate that Johan, his wife, and his friends had started a plan prior the announcement of the MCO to sell their homemade rainbow bread and coffee bread. As there had been an increase in sales orders for the bread, he decided to open a physical store before MCO in Johor to earn income during the pandemic period. This bakery business not only helped him significantly financially during the pandemic, but it has also helped him psychologically. Since the MCO brought a lot of financial pressure to everyone, his business has helped to keep him busy.
Similar situations were found in Sabah as well. An example is that of stewardess Wendy Radin who had her last flight in April 2020, and is now a full-time financial planning consultant in unit trust sales ( Inus 2021 ). She shared her experience and recruited others to the industry to earn some income to support their family, including those who could not afford to invest themselves. Investment in unit trusts has been encouraging when the government announced the i-sinar programme. This programme was aimed at those who were affected during the MCO and it allowed eligible depositors of the Employees’ Provident Fund (EPF) to withdraw a certain amount from their respective Account 1 with a ceiling of RM10,000 for those depositors with RM100,000 or below; and maximum 10% or RM60,000 for those have more than RM100,000 in their Account 1 ( Azman 2021 ). There were success stories for some but not for all.
The AirAsia Group Berhad has gone through several cycles of layoff. The first cycle of layoffs in Air Asia involved more than 333 employees to reduce operational cost under difficult circumstances ( Aroff 2020 ; Bernama 2020 ). The 333 comprised 111 cabin crew members, 172 pilots and 50 engineers across Malaysia. From that number, it was reported 50 of the laid off staff were based in Sabah ( Micheal 2020 ). The majority of those terminated in Sabah has been working for 10 to 15 years in Air Asia. It was claimed that those involved had not received any information about the layoffs prior to the announcement. The sudden news of layoffs had caused the affected employees to panic and feel helpless. This number was a significant proportion because it represented about one sixth of the total laid off staff. Hence, it reflected the importance of Air Asia’s presence in the air travel industry, especially in Sabah. With tourism being one of the dominant sectors in Sabah, the pandemic has severely affected the Sabah economy. Tourism is the third largest contributor to the economic sector in Sabah prior to the pandemic, representing 23% of its total labour force with RM8 billion (USD 1.85 billion) generated in 2018 ( Chan & King 2020 ). Thus, the repercussion to be felt as the pandemic progressed throughout the year 2020 were severe.
Retrenchment brings people into financial hardship, especially those with families to support. As the breadwinner of the family, the loss of a job means the loss of one’s self-identity, besides losing the only source of income for the family, resulting in mental and psychological stress. According to another news report, the stress of losing a job had forced a former pilot to jump to his death from an apartment in Kuala Lumpur ( Bernama 2020 ). This 36-year-old man, a former pilot with local airline, had taken such drastic action due to the pressure of unemployment.
One might ask, in relation to the retrenchment exercise, whether, prior to the airlines laying off its employees, they conducted an exit interview with the personnel involved. If the action of exit interviews were undertaken, the airlines could identify vulnerable employees and taken immediate steps to remediate the situation. In general, companies need to address some or all of the problems and elements related to layoff. As companies plan and communicate the layoffs, it would be useful to maintain a record of the actions taken to help the employee adjust to the new situation. The elements involved in planning layoff can be divided into three sections which are layoff pre-planning, layoff notification planning, and post-layoff notification planning. In the context of Covid-19, there are questions over whether these processes were followed. Were the unfortunate employees prepared mentally and emotionally to face this new challenge in their life? For instance, what kind of package or program would be offered to the laid off employees or to employees offered early-retirement? This kind of package or program offered may include subsistence e.g. their daily needs like money, financial advice, but the most important issues were emotional and mental support. Did the airline offer any kind of psychological need for their employees? These processes would help the airline determine if the employee had enough resources to face retrenchment. Unfortunately, the evidence is that these processes were lacking as many terminated staff claimed that they were given short notices i.e. 2 days, and offered unfair compensation ( Micheal 2020 ).
There is evidence from several sources that there was a significant need for psychological support for people facing hardship at this time. According to the police records, from 18 March 2020 (when the MCO started) to 9 June 2020 there were 78 suicides across the country that reflects a spike as compared to 64 suicides reported in the same period for the previous year 2019 ( Astro Ulagam 2020 ). A study conducted by the Malaysian think tank centre in April also found that 45% of 1084 Malaysians surveyed experienced anxiety and depression during the partial lockdown ( Astro Ulagam 2020 ). Referring to the Department of Statistic Malaysia (DOSM), the unemployment rate in Malaysia for May rose to 5.3%, and 826,100 Malaysians were unemployed that month. Losing a job can easily make people lose the will to live. Although unemployment can lead people to a dead end, it may also bring a new opportunity to learn new things such as new business ventures.
The National Health and Morbidity Survey 2019 found that the prevalence of depression in Sabah was 4.0%, higher than the national prevalence of depression at 2.3%. It also revealed that Bumiputera Sabah, the indigenous people of Sabah, ranked highest among all ethnicities with score of 5.2% for depression ( IPH 2020 ). Thus, the consequences of mental threat due to the Covid-19 pandemic posed a significant threat to people in Sabah especially the indigenous people. Recognising the potential threats and risks, mental health psychosocial support teams were established in every district throughout Sabah at the early stages of the pandemic. However, information about accessing this support was somewhat lacking.
The survival of airlines is dependent on travelling passengers. As such, tourist arrivals is an important factor to examine. While there are other means to reach Sabah such as by land (from other parts of Borneo Island) and sea (from West Malaysia), air travel remains the popular method of travel, especially for international tourists, that accounted for 95% of the total arrivals ( Chan & King 2020 ). Thus, it is a relevant indicator and a useful reference point to explain the situation in Sabah and its relationship to the employment situation in Sabah. In other words, employment is explained through the data of visitor arrivals that is directly indicative of the vitality on the airlines and hospitality industry, subsectors of the tourism sector that forms a major part of the Sabah economy, and other industries that service this sector indirectly.
In Sabah, the total tourist arrivals experienced a drastic reduction for the year 2020 due to the Covid-19 pandemic and its corresponding measures to curb the spread such as border closure and movement control orders (MCO) of which there were various kinds: Conditional Movement Control Order (CMCO), Recovery Movement Control Order (RMCO), Enhanced Movement Control Order (EMCO), Targeted Enhanced Movement Control Order (TEMCO), Administrative Enhanced Movement Control Order (AEMCO), and Full Movement Control Order (FMCO) or ‘Total Lockdown’ that each has a separate set of standard operating procedures ( Povera & Harun 2020 ). Currently, the FMCO has completed its first phase 1st -14th of June 2021 and has been continued with its second phase of implementation from the 15th-28th of June 2021 that may be further extended if necessary.
As early as January 2020, Sabah was the first state in the country to ban all flights from Wuhan as part of its preventive measures ( Bernama 2020 ). A few days after this ban was imposed, the Government of Sabah took another bold step to ban all China-Sabah flights on 30 January 2020, a decision that was opposed by several quarters (FMT 2020; MATTA 2020 ). The concern raised may have been due to the fact that tourists from China comprised 42.3% of the total foreign tourists in 2019. The decision was a serious blow to revenue from the tourism sector and its related subsectors. The ban on China-Sabah flights has affected 127 weekly flights involving airlines including AirAsia (60), China Southern Airlines (21), Malindo Air (20), Malaysia Airlines (9), Xiamen Air (7), Shanghai Airlines (7) and Loong Air (3). It is clear that Air Asia has played a dominant role in Sabah tourism, being the main air carrier for Chinese tourists.
As a result, Sabah had managed to remain free from the Covid-19 while other places in Malaysia started to record cases of Covid-19. Sabah maintained its record at zero cases until 12 March 2020 when its first case of the Covid-19 was reported ( Kee 2020 ). The patient zero for Sabah had attended a religious gathering at Sri Petaling, Kuala Lumpur. Over time, further restrictions were imposed as the situation changed. By the middle of March 2020, special approval from the Government of Sabah was required for non-Sabahan Malaysians to enter Sabah, with the provision of medical certificates and polymerase chain reaction (PCR) swab test results, while all foreign tourists and visitors were banned from entering Sabah ( Geraldine 2020 ).
Source: Sabah Tourism Board (STB), 2021
By comparing the data available from the year 2019, the total tourist arrivals in Sabah decreased 76.7% from 4.2 million in 2019 to less than a million in 2020 i.e. from 4,195,903 (2019) and 977,460 (2020) respectively ( Sabah Tourism Board 2021 ). Total international tourist arrivals observed a greater decline than domestic arrivals. There was a reduction of 87.7% for international tourist arrivals from 1,469,475 in 2019 to 180,284 in 2020; while domestic arrivals decreased 70.8% from 2,726,428 to 797,176 for the same period. Prior to the pandemic, total tourist arrivals in Sabah have been rising steadily over time ( Table 1 ). When MCO was declared on the 18 th of March 2020, the decline became noticeable. For the year 2020, tourist arrivals from January to March represented 71.3% of total visitors for the year while April to December the proportion recorded was 28.7%. It was particularly obvious for international tourists with 97.5% of them visiting Sabah during the period from January to March 2020 while only 2.5% of the total international visitors arrived during the remaining months of the year ( Sabah Tourism Board 2021 ). This indicates a vast shrinkage in foreign arrivals, bordering on an almost total absence in Sabah from April 2020 onwards. While we understand the situation of the tourism sector in Sabah, we must now explore this situation with regards to the general employment situation in Malaysia.
Employment Situation in Malaysia
In Malaysia, the Covid-19 pandemic has affected the labour market. Job losses and reduction in working hours implemented by employers as cost-cutting measures were observed in almost all industries affected by unemployment for the year 2020 at 711,000, increased from 508,200 for year 2019 or unemployment rate increases to 4.5% (2020) from 3.3% (2019) as reported by the Department of Statistics Malaysia (2020).
It has been observed that the ratio of labour force participation for male is higher than female for all ranges of age. While these data might be a decade old as the census in Malaysia is taken on a 10-year cycle (Census 2010, as shown in Table 2 ) they are useful as a benchmark.
Source: Department of Statistics Malaysia (DOSM)
The major motivations for people to work are ranked in order as follow: to sustain cost of living, seeking attention, addressing pressure from one’s surrounding peers, family and relatives, making one feeling comfortable and having peace of mind by working. For youth aged 20-29 years old, the main factor for them to work is to sustain their cost of living or cover their daily expenses (39%), follow by seeking attention (26%), addressing pressure from their peers, family and relatives (22%), feeling comfortable (8%) and obtain peace of mind (5%). This ranking order of factors are consistent with other age groups, as well as for both genders and regardless of whether one is with or without dependent (see Table 3 ).
Source: Credit Counselling & Debt Management Agency (AKPK)
Adapted by: Institute of Youth Research Malaysia (IYRES)
The year 2020 was a challenging year for most countries around the world. Besides the airline industry, many industries in Malaysia were also affected by the pandemic. Figure 1 shows the loss of employment (LOE) in Malaysia for the year 2020. LOE in Malaysia refers to individuals who are insured with the Employment Insurance System (EIS), who had lost their job, but it does not include compulsory retirement, voluntary resignation, expiry of a fixed-term contract, and retrenchment due to misconduct. While it does not include all individuals affected by the pandemic such as those that did not sign up for the EIS, it is a significant point of reference to examine the impact in due course. The implementation of the Movement Control Order (since the18 March 2020) has resulted the number of LOE to drastically increasing in tandem, peaking in June and July 2020 as can be seen in Figure 1 .
Figure 1. Monthly Loss of Employment in Malaysia, 2018 – 2020
Source: EIAS, 2020
The data also indicated that most industries were affected by the MCO, with the top three most affected industries being the manufacturing industry, followed by the wholesale and retail industries, and finally the accommodation and food and beverage sectors. Many other industries underwent a similar retrenchment process but to a lesser extent as shown in Figure 2 . The main causes for these losses were downsizing, constituting 28% of total LOE, followed by business closure (25%), Voluntary/Mutual Separation Schemes (23%) (VSS/MSS), company financial problems (15%) and other issues (9%) as shown in Figure 2 .
Figure 2. Loss of Employment in Malaysia by Industry and Causes, 2020
In terms of occupations affected by the LOE, professionals top the list, follow by technicians and associate professionals, various categories of managers, service and sales workers, plant & machine operators and assemblers, and finally clerical support workers and others (see Figure 3 ).
Figure 3. Loss of Employment in Malaysia by Major Occupation Category, 2020
It is important to note that most LOE involved those earning a relatively lower income. It shows a tendency for lower salary earners to face a greater risk of having their employment terminated in a challenging economy. As such 40.1% were those earning below RM2,000, while 62.2% of the LOEs have an income less than RM3,000 or 78.1% were those below RM4,000. Those with relatively higher incomes were not spared from being laid off. Table 4 demonstrates the income levels of LOE in the year 2020 that may well reflect the income disparity and further increase the gap between the rich and the poor, worsened over time by the Covid-19 pandemic.
Figure 4. Loss of Employment by Wage Level in Malaysia, 2020
Employment Situation in Sabah
The case study for Sabah was examined and compared with the situation at the national and international levels. Further data requested from Social Security Organization (SOCSO) in Malaysia, revealed that 74.6% of those suffering loss of employment (LOE) in Sabah are workers earning below RM2,000 ( SOCSO 2021 ). While the trend is consistent with the LOE trend in Malaysia that lower income earners are the major casualties in employment, the situation in Sabah is relatively more severe than the situation in Malaysia as a whole (40.1%, refer Table 4 above). LOE over time in Sabah was found consistent with the trend of LOE in West Malaysia, peaking in June and July 2020 (see Figure 1 and Figure 5 ).
Figure 5. Monthly Loss of Employment in Sabah, 2019 – 2020
Source: SOCSO, 2021
The main reasons for job losses in Sabah were due to business closure (17.9%), financial problems of companies (17.4%), downsizing (15.2%), other issues (10.2%), partial closure (6.4%), VSS/MSS (5.5%), and several other categories that were below 4% ( SOCSO 2021 ). In comparison with the overall Malaysian situation, the businesses were hard hit and relatively more difficult to sustain under the circumstances due to the fact that many companies in Sabah were relatively more engaged in economic activities pertaining to the tourism sector or have a higher business overhead cost in Sabah. With the implementation of MCOs to curb the spread of the disease, this overhead cost became unmanageable ( Idris 2021 ). It was also interesting to note that the LOE on VSS/MSS in Sabah was relatively much lower in Sabah as compared to Malaysia as a whole (23%). This disparity may well reflect that fewer people have the option to ‘select’ or ‘choose’ the circumstances under which they would become unemployed, or rather this was not an option available to them. Although these may be unsubstantiated claims, this situation is well known anecdotally. As such, it would be interesting to establish its extent via further investigation into the wellbeing of workers as well as industry players in due course.
On the type of industries that top the list for LOE in Sabah was accommodation and food and beverages (21.6%), wholesale and retail (17.2%), administrative and support service (16.3%), construction (15.1%), manufacturing (4.6%), real estate (4.5%) while other industries accounted for less than 4% in each of the categories ( SOCSO 2021 ). The industry based LOE composition in Sabah was very different from the West Malaysia situation where manufacturing sector had the highest LOE. This corroborates the fact that tourism plays a dominant role in the economy of Sabah.
In following section, some relevant primary data from the Family, Women and Youth Survey, conducted online between 13 November and 5 December 2020, were analysed. While these data did not specify the tourism sector, it is useful considering the main sector affected by loss of employment were mainly those related to tourism directly or indirectly as discussed above. Table 5 shows the distribution of respondents under unmarried and married sample categories that cover all districts in Sabah.
Source: Tey et al. 2020
From the data collected from 2503 respondents at the end of 2020, 779 respondents (31%) reported that they were forced out of their jobs, comprising 438 female respondents and 341 male respondents. In terms of marital status, 443 unmarried respondents and 336 married respondents had lost employment due to the implementation of MCO (see Table 6 ). The findings demonstrated that more unmarried respondents had their jobs terminated as compared to married respondents. Unmarried respondents are relatively younger that married respondents on average. Hence, this is also in line with the claim that younger workers are facing greater risks of losing their job than more experienced workers. Besides, it may well be that marital status has been one of the factors considered by employer in retrenchment. However, this claim needs further verification.
The survey also investigated the loss of businesses as a consequence of implementing the MCO. A total of 582 respondents or about one quarter of respondents reported that they have loss businesses. Detailed figures on loss of businesses due to MCO are shown in Table 7 . These primary data were consistent with the analysis of the secondary data presented in earlier section.
With the imposition of MCO, it is noticed that majority of the respondents or more than two-third of respondents experienced a reduction in income (see Table 8 ). Thus, we can extrapolate that whether it is the loss of businesses or employment, most respondents were impacted financially under MCO. This claim is consistent when comparing the loss of employment for year 2019 and 2020 in Sabah, a significant increase that LOE in 2020 has doubled from year 2019 or 104.2%, i.e. from 2123 cases to 4344 that was recorded by SOCSO ( SOCSO 2021 ).
The pandemic of Covid-19 has affected working hours and the earnings of workers around the world. The International Labour Organization (ILO) has compiled the list of industries affected and urgent government policy is needed to address the situation. This crisis is expected to affect 6.7% of working hours around the world, which is equivalent to 195 million full-time workers ( ILO 2020 ). Around 81% of the global workforce of 3.3 billion was exposed partially or fully by this pandemic. Thus, comprehensive policies are needed to focus on, but not limited to, the following four aspects: supporting enterprises, employment, and incomes; protecting workers in the workplace; stimulating the economy and jobs; and using social dialogue between government, workers, and employers to find the solutions.
As Malaysia enters a new phase of the Covid-19 wave, it is unknown where the peak is as new highs are reported daily. Thus, it is important to prepare the community in facing the new challenges, financially and psychologically by evaluating and revisiting the strategies employed over the last one year. If the pandemic is prolonged and continue to impact the bread and butter of the people, fatigue will set in, resulting in the Malaysian society putting aside the current Standard Operating Procedures (SOP) in the Covid-19 prevention strategies or measures. We have seen this happen in countries like Indonesia and India, where livelihood has trumped Covid 19 prevention SOPs.
Indecisiveness in policy, political uncertainty and politicians behaving as if they are above the law in complying with SOP, will result in the erosion of the people’s trust as the situation of Covid-19 and economy continues to worsen over time. With uncertainty ahead and the declaration of emergency, the ruling PN-government may have been able to avoid a ‘vote of confidence’ in parliament, however, the ruling government cannot prevent the declining of ‘voice of confidence’ in the government.
With the news of the availability of vaccines for the COVID19 after successfully passing the mandatory clinical trials, being made available in Malaysia, the situation is looking favourable. However, effective measures to address the economic issues are still lacking. It is generally accepted that the people’s confidence in the government is closely related to the well-being of the economy. As shown in the survey conducted in Sabah and in the secondary data obtained from government agencies at the national level in Malaysia, the findings pointed to one common ground i.e. the hardship faced by the majority of Malaysians in the time of this pandemic Covid-19. Government roles and engagement with various communities and industries are essential especially when it involves retrenchment, loss of employment and/or loss of businesses in due course. Supports and measures for employers and employees need to be emphasised to overcome the challenges in this difficult time for all.
Acknowledgments
The support of the Research Management Centre of Universiti Malaysia Sabah and GRRI-HOSEI (Grant No. GKP 0020-2018) is gratefully acknowledged. The authors would like to thank SOCSO for additional data provision and UNFPA through IF066-2019 (UMS-TLK2019) for sharing primary data.
Achdut, N. & Refaeli, T. 2020, ‘Unemployment and psychological distress among young people during the COVID-19 Pandemic: Psychological resources and risk factors’, International Journal of Environmental Research and Public Health , vol. 17, no. 3, 7163. https://doi.org/10.3390/ijerph17197163
Altig, D., Baker, S., Barrero, J.M., Bloom, N., Bunn, P. Chen, S., ... & Thwaites, G. 2020, ‘Economic uncertainty before and during the COVID-19 pandemic’ University of Chicago Becker Friedman Institute for Economics Working Paper No. 2020-88. https://doi.org/10.2139/ssrn.3638649
Aroff, N. 2020, ‘AirAsia terminates 111 flight attendants, 172 pilots, 50 engineers’, Astro Awani , 4 June 2020. Accessed 22 January 2021, https://www.astroawani.com/berita-malaysia/airasia-terminates-111-flight-attendants-172-pilots-50-engineers-245696 .
Astro Ulagam 2020, ‘Ex-pilot Driven to Suicide After Losing Job, Say Cops’, 5 August 2020. Accessed 21 January 2021, https://astroulagam.com.my/news/article/158680/ex-pilot-driven-to-suicide-after-losing-job-say-cops
Azman, N. H. 2021, Malaysians using i-Sinar for investment, The Malaysian Reserve , 24 March 2021. Accessed 8 June 2021, https://themalaysianreserve.com/2021/03/24/malaysians-using-i-sinar-for-investment/
Bernama 2020, ‘AirAsia may lay off employees’, Free Malaysia Today , 4 June 2020. Accessed 22 January 2021, https://www.freemalaysiatoday.com/category/nation/2020/06/04/airasia-may-lay-off-employees-to-pare-down-operations/
Bernama 2020, ‘Coronavirus outbreak: All flights from Sabah to Wuhan suspended’, Bernama.com , 26 January 2020. Accessed 30 January 2020, https://bernama.com/en/general/news_covid-19.php?id=1809388
Bernama 2020, ‘Pilot commits suicide in Serdang after losing job’ , The Star , 5 August 2020. Accessed 22 January 2021, https://www.thestar.com.my/news/nation/2020/08/05/pilot-commits-suicide-in-serdang-after-losing-job
Birruntha, S. 2020, ‘Malindo crew ‘signing off’’, The Malaysian Reserve, 2 November 2020. Accessed 29 April 2021, https://themalaysianreserve.com/2020/11/02/malindo-crew-members-signing-off/
Blustein, D. L., Duffy, R., Ferreira, J. A., Cohen-Scali, V., Cinamon, R. G. & Allan, B. A. 2020, ‘Unemployment in the time of COVID-19: A research agenda’, Journal of Vocational Behavior , vol. 119, article 103436. https://doi.org/10.1016/j.jvb.2020.103436
Capbay 2020, ‘COVID-19 – Is Malaysia in economic trouble?’, CapBay , 12 March 2020. Accessed 25 December 2020, https://capbay.com/covid-19-outbreak-is-the-malaysian-economy-in-trouble/
Chan, J. K. L. & King, V. T. 2020 ‘The Coronavirus pandemic and tourism in Southeast Asia: Case material from Malaysia. Journal of Humanities and Social Sciences Research , vol. 2, no. S, pp. 87-102. https://horizon-jhssr.com/view-issue.php?id=26
Chen, H., Qian, W. & Wen, Q. 2020, ‘The impact of the COVID-19 pandemic on consumption: learning from high frequency transaction data’, Luohan Academy Working Paper No.LHA20201003, May 2020. https://www.luohanacademy.com/frontiers/00d2c3eacfa38705 . https://doi.org/10.2139/ssrn.3568574
De Vito, A. & Gomez, J. P. 2020. Estimating the COVID-19 Cash Crunch: Global Evidence and Policy. Journal of Accounting and Public Policy , vol. 39, no. 2, article 106741. https://doi.org/10.1016/j.jaccpubpol.2020.106741
DOSM-Department of Statistics Malaysia 2021, Press Release: Labour Force Survey Report Malaysia 2020, 22 April 2021. Accessed 26 April 2021, https://www.dosm.gov.my/v1/index.php?r=column/pdfPrev&id=dTF2dkJpcUFYUWRrczhqUHVpcDRGQT09
EIAS (Employment Information and Analysis Services) 2020, Interim Report 2020 – Employment Insurance System, Employment Insurance System Office, Social Security Organization (SOCSO), Putrajaya. https://eiscentre.perkeso.gov.my/wp-content/uploads/2021/01/EIS-REPORT-2020-31-December-2020.pdf
Eichenbaum, M. S., Rebelo, S. and Traband, M. 2020, The Macroeconomics of Epidemics, National Bureau of Economic Research, Working Paper 26882. Accessed 26 April 2021, https://www.nber.org/system/files/working_papers/w26882/w26882.pdf
Elsby, M. W., Hobijn, B. & Şahin, A. 2010, ‘The labor market in the Great Recession’, Brookings Papers on Economic Activity , vol. 41, pp. 1-69. https://doi.org/10.1353/eca.2010.0001
Ferguson, N. M., Laydon, D., Nedjati-Gilani, G., Imai, N., Ainslie, K., ... & Ghani, A.C. 2020, Impact of Non-pharmaceutical Interventions (NPIs) to Reduce COVID-19 Mortality and Healthcare Demand, Report 9, Imperial College London COVID-19 Response Team. DOI: https://doi.org/10.25561/77482
Ferreira, J. A., Reitzle, M., Lee, B., Freitas, R. A., Santos, E. R., Alcoforado, L. & Vondracek, F. W. 2015, ‘Configurations of unemployment, reemployment, and psychological well-being: A longitudinal study of unemployed individuals in Portugal’, Journal of Vocational Behavior , vol. 91, pp. 54-64. https://doi.org/10.1016/j.jvb.2015.09.004
Free Malaysia Today (FMT) 2020, ‘Former CM questions Sabah govt over China travel ban’, Free Malaysia Today , 31 January 2020. Accessed 3 February 2020, https://www.freemalaysiatoday.com/category/nation/2020/01/31/former-cm-questions-sabah-govt-over-china-travel-ban/
Geraldine, A. 2020, ‘Sabah govt imposes immigration restriction order’, New Straits Times , 17 March 2020. Accessed 20 March 2020, https://www.nst.com.my/news/nation/2020/03/575489/sabah-govt-imposes-immigration-restriction-order
Idris, R. 2021 The Sabah Economic Model: A General Overview, Universiti Malaysia Sabah, 11 January 2021. Accessed 12 February 2021, https://www.ums.edu.my/v5/ms/featured-1/10268-the-sabah-economic-model-a-general-overview
ILO 2013, ‘Resolution concerning statistics of work, employment and labour underutilization’, 19th International Conference of Labour Statisticians (ICLS) , Geneva. Accessed 25 January 2021, https://www.ilo.org/wcmsp5/groups/public/---dgreports/---stat/documents/normativeinstrument/wcms_230304.pdf
ILO 2020 Covid-19 Causes Devastating Losses in Working Hours and Employment, Press Release, 7 April 2020. Accessed 26 April 2021, https://www.ilo.org/global/about-the-ilo/newsroom/news/WCMS_740893/lang--en/index.htm
Institute for Public Health (IPH), National Institutes of Health, Ministry of Health Malaysia. 2020, National Health and Morbidity Survey (NHMS) 2019: Vol. I: NCDs – Non-Communicable Diseases: Risk Factors and other Health Problems, IPH, Shah Alam. http://iku.moh.gov.my/images/IKU/Document/REPORT/NHMS2019/Report_NHMS2019-NCD_v2.pdf
Inus, K. 2021 ‘Air stewardess makes the best of her time on the ground’, The Star , 22 March 2021. Accessed 10 June 2021, https://www.thestar.com.my/news/nation/2021/03/22/air-stewardess-makes-the-best-of-her-time-on-the-ground
IYRES 2020, Institute of Youth Research Malaysia – Malaysia Youth Data Bank System . IYRES, Putrajaya. Accessed 21 January 2021, https://ydata.iyres.gov.my
Kee, A. 2020, ‘Sabah records first Covid-19 case in Tawau’, The Borneo Post , 12 March 2020. Accessed 13 March 2020, https://www.theborneopost.com/2020/03/12/sabah-records-first-covid-19-case-in-tawau/
Lim, R. 2021, ‘Gig economy on the rise’, The Star , 18 April 2021. Accessed 8 June 2021, https://www.thestar.com.my/news/nation/2021/04/18/gig-economy-on-the-rise
Malaysian Association of Tour and Travel Agents (MATTA) 2020, China-Sabah Flights Suspension a Bold Move, says MATTA. Press Release, 31 January 2020. Accessed 3 February 2020, https://www.matta.org.my/press-release/11664-china-sabah-flights-suspension-a-bold-move-says-matta
Martin, A., Markhvida, M., Hallegatte, S. & Walsh, B. 2020, ‘Socio-economic impacts of COVID-19 on household consumption and poverty’, Economics of Disasters and Climate Change , vol. 4, pp. 453-479. https://doi.org/10.1007/s41885-020-00070-3
Micheal, S. M. 2020, ‘50 Laid-off AirAsia Staff Clock Out for Last Time’, Daily Express , 13 June 2020. Accessed 30 May 2021, https://www.dailyexpress.com.my/news/154213/50-laid-off-airasia-staff-clock-out-for-last-time/
Newman, T. 2020, ‘Comparing Covid-19 with previous pandemics’, Medical News Today , 19 April 2020. Accessed 29 April 2021, https://www.medicalnewstoday.com/articles/comparing-covid-19-with-previous-pandemics
OECD 2020a, Employment and Unemployment Statistics During the COVID-19 crisis . Accessed 25 January 2021, https://www.oecd.org/sdd/labour-stats/OECD-employment-and-unemployment-statistics-during-the-COVID-19-crisis.pdf
OECD 2020b, OECD Economic Outlook - Interim Report March 2020: Coronavirus: The World Economy at Risk. Accessed 26 April 2021, https://www.oecd.org/economic-outlook/march-2020/
Pearlin, L. I. 1989, ‘The sociological study of stress’, Journal of Health and Social Behavior , vol. 30, no. 3, pp. 241-256. https://doi.org/10.2307/2136956
Pearlin, L. I., Menaghan, E. G., Lieberman, M. A. & Mullan, J. T. 1981, ‘The stress process’, Journal of Health and Social Behavior , vol. 22, no. 4. pp. 337-356. https://doi.org/10.2307/2136676
Povera, A. & Harun, H. N. 2020, ‘Ismail Sabri explains SOPs for CMCO, EMCO and TEMCO’, New Straits Times , 10 October 2020. Accessed 6 June 2021, https://www.nst.com.my/news/nation/2020/10/631180/ismail-sabri-explains-sops-cmco-emco-and-temco
Ram, A. 2020, ‘Thousands of Malindo staff sign off after the airline’s latest retrenchment exercise’. Says , 2 November 2020. Accessed 29 April 2021, https://says.com/my/news/thousands-of-malindo-staff-sign-off-after-the-airline-s-latest-retrenchment-exercise
Ravindran, A. 2021, ‘Malaysia’s fourth Covid-19 wave inevitable: Epidemiologists’, Code Blue , 19 April 2021. Accessed 30 May 2021, https://codeblue.galencentre.org/2021/04/19/malaysias-fourth-covid-19-wave-inevitable-epidemiologists/
Sabah Tourism Board 2021, Sabah Tourism Statistics . Accessed 25 May 2021, https://www.sabahtourism.com/statistics/
Sobieralski, J. B. 2020, ‘COVID-19 and airline employment: Insights from historical uncertainty shocks to the industry’, Transportation Research Interdisciplinary Perspectives , vol. 5, article 100123. https://doi.org/10.1016/j.trip.2020.100123
Social Security Organization (SOCSO) 2021, Data on Sabah, Year 2020 and Year 2019 (Unpublished), Received on 9 June 2021 and 11 June 2021 respectively .
Su, C. W., Dai, K., Ullah, S. & Andlib, Z. 2021, ‘COVID-19 Pandemic and unemployment dynamics in European economies’, Economic Research-Ekonomska Istraživanja . https://doi.org/10.1080/1331677X.2021.1912627
Tey, N. P., Chan, J. K. L., Lai, S. L., Nga, J. L. H. & Eboy, O. V. 2020 Family, Women and Youth Survey in Sabah, 2020. (Draft Report). UNFPA Project No. IF066-2019 (UMS-TLK2019).
Umar, M., Xu, Y. & Mirza, S. S. 2020, ‘The Impact of Covid-19 on Gig economy’, Economic Research-Ekonomska Istraživanja . https://doi.org/10.1080/1331677X.2020.1862688
Wheaton, B. & Montazer, S. 2020, “Stressors, stress, and distress’, in Scheid, T. and Brown, T. (eds.), A Handbook for the Study of Mental Health: Social Contexts, Theories, and Systems . Eds. Cambridge University Press, Cambridge, pp. 171–199. https://doi.org/10.1017/CBO9780511984945.013
Whyte, J. 2021, Has the U.S. Entered a Fourth Wave? 12 April 2021. Accessed 30 May 2021, https://www.webmd.com/coronavirus-in-context/video/eric-topol-fourth-wave
Wolfers, J. 2020. ‘The Unemployment Rate Is Probably Around 13 Percent’, New York Times, The Upshot, 16 April 2020. Accessed 26 April 2021, https://www.nytimes.com/2020/04/03/upshot/coronavirus-jobless-rate-great-depression.html
Zikri, A. 2020, ‘How Malaysia Airline employees cope with MCO and Covid-19 pandemic’, Malay Mail, 11 June 2020. Accessed 1 January 2021, https://www.malaymail.com/news/life/2020/ 06/11/how-malaysian-airline-employees-cope-with-mco-and-covid-19-pandemic/18744 21
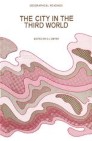
The City in the Third World pp 159–168 Cite as
Unemployment in Less Developed Countries: A Case Study of a Poor District of Tehran
- William H. Bartsch
22 Accesses
Part of the book series: The Geographical Readings series
Unemployment is today considered perhaps the most serious of the problems afflicting less developed countries and one that is believed to be steadily worsening as the gap between the rapidly rising numbers pressing for work and new employment opportunities being created widens 1 . Yet surveys conducted in recent years in these countries do not indicate levels of unemployment much higher than found in many developed countries today or any perceptible trend towards significant increases 2 . Even in the urban areas, where such unemployment is regarded to be most serious, very high rates are not reported 3 .
This is a preview of subscription content, log in via an institution .
Unable to display preview. Download preview PDF.
Growing concern for the employment situation in less developed countries has characterised recent policy statements by the United Nations and its specialised agencies, including the fao, the World Bank, and the ilo. In response to what it regards as a growing threat, the ilo in 1969 launched its World Employment Programme aimed at accelerating the rate of employment-creation, and within this context in 1970 carried out the first of a number of planned country missions (to Colombia) for developing comprehensive strategies to overcome the employment problem in less developed countries. A similar concern with the unemployment problem was expressed by the Pearson Commission in its report, Partners in Development , which termed the problem ‘urgent’. Both the World Bank and the oecd Development Centre are devoting considerable research effort during the Second Development Decade to analysis of unemployment in ldcs, reflecting the rising concern with the problem.
Google Scholar
For instance, unemployment (excluding the seasonally unemployed) as a percentage of the economically-active population in Iran in November 1966 was only 3–7 per cent, according to the census of that date, as compared with 2–6 per cent in the census of November 1956. Annual surveys of unemployment in the United Arab Republic, Korea, Philippines, and Taiwan during the 1960s show rates varying from 1–5 to 8–4 per cent only, with no trend towards increase. See David Turnham . The Employment Problem in Less Developed Countries: A Review of Evidence’, Unpublished-Roneo oecd Development Centre, Paris, June 1970, p. 56.
Iranian urban unemployment as reported by censuses increased only slightly between 1956 and 1966, or from 4–5 per cent to 4–9 per cent. Rates for the urban areas of Chile in 1968, Panama in 1963–4, Venezuela in 1969, Ceylon in 1968, India in 1961–2, Malaya in 1965, and Philippines in 1965, were 6, 10–4, 7–9, 15–0, 3–2, 9–8, and 11–6 per cent, respectively. See Turnham . Op. cit. , pp. 58–60.
In his recent study of conditions in southeast Asia, Asian Drama , London 1968, Gunnar Myrdal is also highly critical of the use of Western definitions and concepts of unemployment in less developed countries. See pp. 1020, 1024–5. Similarly, the oecd regards the concept of unemployment used in industrial countries as ‘inappropriate’ for most ldcs. See oecd. Development Assistance: 1970 Review , Paris December 1970, p. 117.
A situation noted, among others, by Myrdal . Op. cit. , pp. 1020 and 1120; Jan L. Sadie . ‘Labor Supply and Employment in Less Developed Countries,’ The Annals of the American Academy of Political and Social Science , January 1967, pp. 127–8; and International Labour Office . Employment and Economic Growth , Geneva 1964, p. 124.
Of 944 employed men in two of the slum communities subsequently razed (Behjatabad and Salsabil), 84–5 per cent were reported by surveys of the Ministry of Development and Housing in 1965 as earning 100 rials ($1–33) or less a day when minimum needs for food alone in that year for a family of four in Tehran were estimated at 137 rials ($1–83) by a Ministry of Labour investigation. The supplementary earnings of the considerable number of wives and children in the two communities who were also working (396 persons) may be assumed to have brought family incomes up to a subsistence level.
All figures for Tehran City and its District 7 quoted in this article derive from data in Plan Organisation, Iranian Statistical Centre, National Census of Population and Housing , November 1966, Volume 10, Tehran Shahrestan, Tehran, August 1967.
The writer is currently engaged in a study of the relationship between head of household income and labour force participation rates in less developed countries, using data for the urban areas of Iran. In Ku-ye 9 Aban, the 1967 survey also showed that 4–6 per cent of boys under the age of 10 were economically active, a real poverty indicator.
For instance, the Ku-ye 9 Aban day maid employed by the writer’s Iranian parents-in-law asked them to lend her 1000 rials ($13–33) so that her unemployed husband could buy a stock of watermelons and start a street sales business.
About half of the wage employed males in the two slum districts surveyed in 1965 (see Note 6) were construction workers, typically subject to day-to-day employment and discharge on completion of the work assignment.
See, for instance, the views of the ilo on this subject in United Nations . Handbook of Household Surveys , Studies in Methods, Series F, No. 10, New York 1964, p. 83. Useful guidelines in determining whether a respondent should be classified unemployed or not are also included in this Handbook , p. 77.
For an example of how such data might be shown in tabular form, see William H. Bartsch and Lothar E. Richter . ‘An Outline of Rural Manpower Assessment and Planning in Developing Countries,’ International Labour Review , 103 , No. 1 , January 1971, Table 1 , p. 74.
Indeed, the Indian Government has already initiated action along these lines, setting up recently a Committee of Experts on Unemployment Estimates, reporting to its Planning Commission, charged with making recommendations for improving the methodology currently in use for surveying unemployment.
Download references
You can also search for this author in PubMed Google Scholar
Editor information
Copyright information.
© 1974 Macmillan Publishers Limited
About this chapter
Cite this chapter.
Bartsch, W.H. (1974). Unemployment in Less Developed Countries: A Case Study of a Poor District of Tehran. In: Dwyer, D.J. (eds) The City in the Third World. The Geographical Readings series. Palgrave, London. https://doi.org/10.1007/978-1-349-86177-4_10
Download citation
DOI : https://doi.org/10.1007/978-1-349-86177-4_10
Publisher Name : Palgrave, London
Print ISBN : 978-0-333-15409-0
Online ISBN : 978-1-349-86177-4
eBook Packages : Palgrave Social & Cultural Studies Collection Social Sciences (R0)
Share this chapter
Anyone you share the following link with will be able to read this content:
Sorry, a shareable link is not currently available for this article.
Provided by the Springer Nature SharedIt content-sharing initiative
- Publish with us
Policies and ethics
- Find a journal
- Track your research

- Create new account
- Reset your password

Gendered Impact on Unemployment: A Case Study of India during the COVID-19 Pandemic
India witnessed one of the worst coronavirus crises in the world. The pandemic induced sharp contraction in economic activity that caused unemployment to rise, upheaving the existing gender divides in the country. Using monthly data from the Centre for Monitoring Indian Economy on subnational economies of India from January 2019 to May 2021, we find that a) unemployment gender gap narrowed during the COVID-19 pandemic in comparison to the pre-pandemic era, largely driven by male unemployment dynamics, b) the recovery in the post-lockdown periods had spillover effects on the unemployment gender gap in rural regions, and c) the unemployment gender gap during the national lockdown period was narrower than the second wave.
Introduction
The coronavirus disease (COVID-19) has adversely impacted labour markets all around the world. According to the International Labour Organization, the working hours lost in 2020 were equal to 255 million full-time jobs, which translated into labour income losses worth US$3.7 trillion (International Labour Organization 2021). Due to the existing gender inequalities, women were more vulnerable to the economic impact of COVID-19 (Madgavkar et al. 2020). The sudden closure of schools and daycare centres due to the Great Lockdown exacerbated the burden of unpaid care on women (Collins et al. 2020; Power 2020; Czymara et al. 2020; Seck et al. 2021). Women also disproportionately represented the accommodation, food services, and retail and wholesale trade sectors, which were worst-hit by the COVID-19 pandemic (Alon et al. 2020; Adams-Prassl et al. 2020; Bonacini et al. 2021). In most countries, women often work in these sectors without any work protection or job guarantee (United Nations Women 2020), leading them to loose their livelihoods faster than men while also dealing with their deteriorating mental health. India is an interesting case study with one of the lowest female labour force participation rates (LFPRs) globally to analyse how the COVID-19 pandemic exacerbated the pre-existing gender disparities in unemployment. According to the World Bank data, India’s female LFPRs was approximately 21% in 2019, the lowest among the BRICS nations (Brazil, Russia, India, China, and South Africa) and 26 percentage points lower than the global average. An even more troubling fact is that women’s LFPRs has been falling since the mid-2000s (Ghai 2018; Andres et al. 2017; Sarkar et al. 2019). Since the onset of the pandemic, women in India have been increasingly dropping out of the labour force. As seen in Figure 1, the greater female labour force, which comprises unemployed females who are active and inactive job seekers, has been lower than the pre-pandemic average since April 2020. The number of unemployed women actively looking for jobs has also been lower than the pre-pandemic average barring the months of April, May, and December in 2020. On the contrary, the number of women who are unemployed but inactive in their job search has risen drastically, albeit with minor fluctuations, during this period (Figure 2). A recent survey by Deloitte (2021) identified that the burden of household chores and responsibility for childcare and family dependents increased exponentially for women worldwide and more so in India due to the pandemic. The surveyed women mentioned increase in work and caregiving responsibilities as the main reasons for considering leaving the workforce.
Figure 1 : Percent Change in Female Greater Labour Force and Unemployed Active Job Seekers Compared to the Pre-pandemic Average

Source: Centre for Monitoring Indian Economy April 2020 - May 2021
Figure 2: Percent Change in Female Unemployed and Inactive Job Seekers Compared to the Pre-pandemic Average

Figure 3: Unemployment Rate in India (Percent)

Source: Centre for Monitoring Indian Economy Jan 2020 - May 2021
This study analyses the effect of the COVID-19 pandemic on the gender unemployment gap from its onset until the second wave using the subnational-level monthly data from the Centre for Monitoring Indian Economy (CMIE). The gender unemployment gap is defined as the difference between male and female unemployment rates ( Albanesi and Şahin 2018 ). We assess the gender unemployment gap during the COVID-19 pandemic compared to the pre-pandemic era using a difference-in-differences (DID) model. A preliminary investigation of the gender unemployment gap based on the raw data reveals that the gap declined in the lockdown period compared to the pre-lockdown period (Figure 3). We find the gender gap to widen during the second wave, albeit smaller than the pre-pandemic level.
Although a large number of national-level studies were conducted on the impact of the COVID-19 pandemic on unemployment (Estupinan and Sharma 2020; Estupinan et al. 2020; Bhalotia et al. 2020; Chiplunkar et al. 2020; Afridi et al. 2021; Deshpande 2020; Desai et al. 2021), this study is among the very first to assess the impact of the second wave of COVID-19 on the unemployment gender gap in India. A previous study found the rise in male unemployment during the lockdown period contributing to a smaller gender gap (Zhang et al. 2021). In this study, we take one step further to assess the effect of the second COVID-19 wave on the unemployment gender gap in India.
The remainder of the article is organised as follows. In Sections 2 and 3, we present the data sources and some facts on the unemployment trend in India. The effects of first and second COVID-19 waves on unemployment disaggregated by gender are discussed in Section 4. Section 5 delves into the gendered impact on unemployment dynamics across urban and rural regions. The concluding remarks are presented in Section 6.
Data and Methodology
In this study, we use the subnational-level monthly employment data from the CMIE from the period of
January 2019 to May 2021 . Starting from January 2016, the CMIE has been conducting household surveys in India on a triennial basis, covering the periods of January to April, May to August, and September to December. This is the only nationally representative employment data in the absence of official government data (Abraham and Shrivastava 2019) and has been used by several employment studies on India (Beyer et al. 2020; Deshpande 2020; Deshpande and Ramachandran 2020).
The employment data are classified into three categories—the number of persons employed, the number of persons unemployed and actively seeking jobs, and the number of persons unemployed and not actively seeking jobs. The sum of these three categories constitutes the greater labour force. The data are also disaggregated by gender (male and female) and residence (rural and urban).[1] For the analysis, we focus on five time periods as indicated in Table 1.
Table 1: Time Periods

For state[2] i at time t, we construct the unemployment rate as given below:
Unemployment rate = Number of persons unemployed and seeking jobs/Greater labour force (1)
Stylised Facts on Unemployment
This section describes some stylised facts based on the subnational unemployment data from February 2019 to May 2021. To this end, we estimate the regression model below:
where Unemp it is the unemployment rate of state i in time t . To see the unemployment dynamics over the period of study, we use a binary variable Month s that takes the value one for month s and 0, otherwise. The model takes into consideration the impact of past unemployment rates, represented by Unemp it −1. Additionally, the state fixed effects δ i are included to account for unobserved, time-invariant state-level characteristics that may potentially confound our estimates.
Figure 4: Trends in Unemployment Rate

Our coefficient of interest is β 1 s which depicts the time trend in unemployment. The results from the model estimation are shown in Figure 4, in which we can see the dynamics of aggregate unemployment in India from February 2019 to May 2021. The vertical axis pertains to coefficient β 1 s , and the horizontal axis corresponds to the respective months. In Figure 4, the aggregate unemployment rate is found to be relatively stable during the pre-pandemic era. This trend faces an overhaul during the national lockdown (April–May 2020) with a structural upward shift in the unemployment rate. The shock to the unemployment rate does not persist as economic recovery during the post-lockdown period enables unemployment to fall steadily from June 2020 onwards. The unemployment rate becomes stable from January to March 2020 as the country returned to a sense of normalcy with the continued resumption of economic activity.[3] However, the economic impact from the onset of the second wave of the COVID-19 pandemic caused the unemployment rate to rise again in April and May 2021.
Next, we estimate Equation (3) separately for the female and male unemployment rates to assess the gender differential impacts of the COVID-19 pandemic on unemployment in India.[4]
where binary variable Quarter s takes the value one for quarter s in the time period of our sample. The model also accounts for lagged unemployment effects through Unemp it −1.
Figure 5: Trends in Unemployment Rate by Gender

Figure 5 shows that a stark gender gap in the unemployment rate (distance between the red and blue lines) exists in the pre-pandemic era as the male unemployment rate is consistently lower than that of the female. Figure 5 also shows that the gender gap dynamics are primarily driven by male unemployment. The sharp rise in male unemployment during the national lockdown causes the gender gap to close in Q2 2020. The post-lockdown recovery (Q3–Q4 2020) is found to have a favourable impact on male unemployment, causing gender gap to revert to the pre-pandemic levels. Although both males and females lost jobs during the onset of the second wave (Q2 2021), the gender gap narrowed as males are found to lose more jobs in absolute terms.
Figure 6: Trends in Urban and Rural Unemployment Rate by Gender

Figure 6 shows the estimates of β 1 s (see Equation [3]) for urban and rural unemployment in Panels (a) and (b), respectively. During the national lockdown, the sharp rise in male unemployment is more evident in urban areas than rural. In fact, the national lockdown period dynamics in aggregate male and female unemployment in Figure 5 largely resemble the effects seen in the urban region (see Figure 6, Panel [a]). The post-lockdown recovery suits male unemployment, both in rural and urban areas. Female unemployment remains stable in rural areas during the pandemic.
Figure 7: Trends in Regional Unemployment Rate by Gender

7 c

The subsample regression estimates of β 1 s pertaining to the north, east, west and south regions are shown in Figure 7. All regions witnessed a rise in male unemployment during the national lockdown period. On the contrary, the female unemployment dynamics differ between regions. During the national lockdown period, female unemployment rose in the west and south regions (Panels [c] and [d] in Figure 7). The north region shows an interesting anomaly (Panel [a] in Figure 7). Contrary to other regions, female unemployment dipped steeply in the north during the national lockdown period. East region alone did not
experience any strong movements in female unemployment throughout the pandemic (Panel [b] in Figure 7).
Impact of COVID-19 on Unemployment
Section 3 discussed how the overall unemployment and unemployment gender gap witnessed structural breaks during the COVID-19 pandemic. To further investigate the gender aspect of the COVID-19 unemployment dynamics in India, we begin our empirical exercise by examining the unemployment changes during the COVID-19 pandemic compared to the pre-pandemic era. We use the following model:
where Period 1 , Period 2 , Period 3 , and Period 4 pertain to lockdown, post-lockdown, post-lockdown normalcy, and second wave time periods, respectively. Besides the overall unemployment, we also estimate Equation (4) for male and female unemployment separately. The results are shown in Table 2. We can see from Column (1) of Table 2 that the overall unemployment rate ( β 11 ) witnessed an increase of 0.066 (statistically significant at one percent level) during the lockdown period in comparison to the pre-pandemic period. This effect was primarily driven by the rise in the male unemployment that shot up by 0.082 during the lockdown period (Column [3]).
The uneven distributional effects of the post-lockdown recovery are seen from β 12 estimates. Male unemployment rose by 0.01, while female unemployment fell by 0.036 in comparison to the pre-pandemic era. The fall in female unemployment does not necessarily indicate that the overall labour conditions improved for women during this period. Equation (1) shows that the unemployment rate is driven by two components. Figure 1 validates that the female unemployment rate fell over time due to the decline in the number of unemployed females actively seeking jobs being higher than the decline in the female labour force.[5]
β 14 estimate in Column (1) indicates that the total unemployment rose by 0.019 (statistically significant at 10 percent level) during the second wave compared to the pre-pandemic period. A comparison between β 14 and β 11 estimates reveals an interesting policy highlight that the second wave’s impact on unemployment was smaller than the nationwide lockdown. Finally, the rise in unemployment during the second wave is primarily driven by male unemployment.
Table 2: Impact of COVID-19 on Unemployment

Note: *** p<0.01, ** p<0.05, and * p<0.1. The robust standard errors are in parentheses.
Unemployment Gender Gap in Urban and Rural Regions
This section delves further into the gendered impact of lockdown on the unemployment dynamics across urban and rural regions. As defined in Section 1, the unemployment gender gap measures the difference between female and male unemployment rates. To identify the effect of the first and second COVID-19 waves on the unemployment gender gap, we estimate the regression model below:
where Female is a binary variable that takes the value 1 for female unemployment and 0, otherwise.
Table 3 shows the estimation results of Equation (5). We discuss the coefficient estimates that are found to be significant. The significant β 1 coefficient reiterates that the unemployment gender gap was an existential problem in India even before the COVID-19 pandemic. The β 31 estimates reveal that the urban region dynamics drove the narrow unemployment gender gap during the lockdown period. Although the magnitude of the narrowing gap during the lockdown did not persist to the post-lockdown period ( β 32 ), rural regions experienced a narrow unemployment gender gap (marginally significant at 10%). This trend continues even in the post-lockdown normalcy period ( β 33 ) as the unemployment gender gap is narrower than the pre-pandemic level by 0.047 in the rural region. This highlights the possibility that the post-lockdown recovery process had a spillover effect on the unemployment gender gap in rural regions. Finally, β 34 estimates show that the narrowing gender gap trend persists only in the urban region during the second wave.
Table 3: Impact of COVID-19 on Unemployment across Urban and Rural Regions during the post-lockdown and post-lockdown normalcy periods.

This article analyses the impact of the COVID-19 pandemic vis-à-vis the pre-pandemic period on the gender unemployment gap. Our findings indicate that the gender gap in unemployment narrowed during the COVID-19 pandemic, primarily driven by male unemployment dynamics. Interestingly, we find that female unemployment declined during the post-lockdown period. Such a decline was likely driven by women dropping out of the labour force rather than a dip in the absolute number of unemployed persons. Further, the region-wide subsample analysis finds the unemployment gender gap in urban regions to narrow across all periods of the COVID-19 era. In contrast, the rural regions witness narrowing gender gap during the post-lockdown normalcy. This indicates that the rural regions’ unemployment gender gap witnessed spillover effects from recovery associated with the economic reopening. Finally, the narrow gender gap (compared to the pre-pandemic level) is smaller during the second wave.
There is a looming uncertainty whether the impending third wave will further narrow the gender unemployment gap at the expense of increasing male unemployment and females being pushed out of the workforce. Further research is required with a more extended period of assessment and focussed on household-level data to understand the difference in the impact of COVID-19 on the gender unemployment gap across the different parts of the country and income strata.
[1] The data are not available for Jammu and Kashmir, Andaman and Nicobar Islands, Arunachal Pradesh, Dadra and Nagar Haveli, Daman and Diu, Lakshadweep, Manipur, Mizoram, Nagaland, and Sikkim. Hence, the main analysis focuses on only 26 subnational economies.
[2] The terms “state” and “subnational economy” are used interchangeably throughout the article.
[3] According to the official data, power consumption grew by 10.2% in January 2021; the highest growth rate in three months, which was indicative of higher commercial and industrial demand (Press Trust of India 2021).
[4] In order to obtain the unemployment dynamics on a quarterly basis, Equation (2) is revised to Equation (3) with dummies pertaining to quarter instead of month.
[5] This reason is also validated by CMIE who found the female labour participation in urban regions to fall to 7.2% in October 2020, the lowest since the organisation started measuring this indicator in 2016 (Centre for Monitoring Indian Economy 2020).
Abraham, Rosa and Anand Shrivastava (2019): “How Comparable Are India’s Labour Market Surveys? An Analysis of NSS, Labour Bureau, and CMIE Estimates,’’ Azim Premji University CSE Working Paper 2019-03.
Adams-Prassl, et al (2020): “Work Tasks That Can be Done From Home: Evidence on Variation Within & Across Occupations and Industries,” CEPR Discussion Paper No. DP14901.
Afridi, Farzana, Kanika Mahajan and Nikita Sangwan (2021): “Employment Guaranteed? Social Protection during a Pandemic,” Oxford Open Economics, Vol 1, https://doi.org/10.1093/ooec/odab003 .
Albanesi, Stephania and Ayşegül Şahin (2018): “The Gender Unemployment Gap,” Review of Economic Dynamics, Vol 30, pp 47–67.
Alon, Titan, et al (2020): “The Impact of COVID-19 on Gender Equality,” Technical Report w26947, National Bureau of Economic Research, Cambridge, MA.
Andres, Luis A, et al (2017): “ Precarious Drop: Re- Assessing Patterns of Female Labor Force Participation in India ,” Technical Report 5595-1149, Vol 8024, World Bank, Washington DC.
Beyer, Robert C.M., Tarun Jain and Sonalika Sinha (2020): “Lights Out? COVID-19 Containment Policies and Economic Activity,” Policy Research Working Papers , The World Bank.
Bhalotia, Shania, Swati Dhingra and Fjolla Kondirolli (2020): “City of Dreams no More: The Impact of Covid-19 on Urban Workers in India,” Centre for Economic Performance, https://cep.lse.ac.uk/pubs/download/cepcovid-19-008.pdf.
Bonacini, Luca, Giovanni Gallo and Sergio Scicchitano (2021): “Working from Home and Income Inequality: Risks of A ‘New Normal’ With COVID-19,” Journal of Population Economics , Vol 34, No 1, pp 303–60.
Centre for Monitoring Indian Economy (2020): “Female Workforce Shrinks in Economic Shocks.” www.cmie.com/kommon/bin/sr.php?kall=warticle&dt=20201214124829&msec=703&ver=pf
Chiplunkar, Gaurav, Erin Kelley and Gregory Lane (2020): “Which Jobs Are Lost during a Lockdown? Evidence from Vacancy Postings in India,” SSRN Journal. https://papers.ssrn.com/sol3/papers.cfm?abstract_id=3659916
Collins, Caitlyn, et al (2020): “COVID-19 and the Gender Gap in Work Hours,” Gender, Work & Organization , Vol 28, pp 101–12, https://doi.org/10.1111/gwao.12506 .
Czymara, Chritian S., Alexander Langenkamp and Tomás Cano (2020): “Cause For Concerns: Gender Inequality In Experiencing The COVID-19 Lockdown In Germany,” European Societies , Vol 23, Sup 1, pp 1–14.
Deloitte (2021): “Women @ Work: A Global Outlook India Findings,” https://www2.deloitte.com/content/dam/Deloitte/in/Documents/about-deloitte/in-women-at-work-india-outlook-report-noexp.pdf.
Desai, Sonalde, Neerad Deshmukh and Santanu Pramanik (2021): “Precarity in a Time of Uncertainty:
Gendered Employment Patterns during the Covid-19 Lockdown in India,” Feminist Economics , Vol 27, Nos 1–2, pp 152–72.
Deshpande, Ashwini (2020): “The COVID-19 Pandemic and Gendered Division of Paid and Unpaid Work: Evidence from India,” IZA – Institute of Labor Economics.
Deshpande, Ashwini and Rajesh Ramachandran (2020): “Is Covid-19 ‘The Great Leveler’?” The
Critical Role of Social Identity in Lockdown-induced Job Losses , GLO Discussion Paper 622, Global Labor Organization (GLO).
Estupinan, Xavier and Mohit Sharma (2020): “Job and Wage Losses in Informal Sector due to the COVID-19 Lockdown Measures in India,” SSRN Electronic Journal .
Estupinan, Xavier, et al (2020): “Impact of COVID-19 Pandemic on Labor Supply and Gross Value Added in India,” SSRN Electronic Journal .
Ghai, Surbhi (2018): “The Anomaly of Women’s Work And Education In India,” Working Paper 368, Indian Council for Research on International Economic Relations (ICRIER), New Delhi.
International Labour Organization (2021): “ILO Monitor: COVID-19 and the World of Work. Seventh
Edition,” Updated Estimates and Analysis. Int Labour Organ .
Madgavkar, Anu, et al (2020): “COVID19 and Gender Equality: Countering The Regressive Effects,” McKinsey Global Institute, https://www.mckinsey.com/featured-insights/future-of-work/covid-19-and-gender-equality-countering-the-regressive-effects .
Power, Kate (2020): “The COVID-19 Pandemic has Increased the Care Burden of Women and
Families,” Sustainability: Science, Practice and Policy , Vol 16, No 1, pp 67–73.
Press Trust of India (2021): “India’s Power Consumption Grows At 3-Month High Rate Of 10.2% In January,” Business Standard India , https://www.business-standard.com/article/current-affairs/india-s-power-consumption-grows-at-3-month-high-rate-of-10-2-in-january-121020100353_1.html.
Sarkar, Sudipa, Soham Sahoo, and Stephan Klasen (2019): “Employment Transitions Of Women In
India: A Panel Analysis,” World Development , Vol 115, pp 291–309.
Seck, Papa A., et al (2021): “Gendered Impacts of COVID-19 in Asia and the Pacific: Early Evidence on Deepening Socioeconomic Inequalities in Paid and Unpaid Work,” Feminist Economics , Vol 27, Nos 1–2, pp 117–32.
United Nations Women (2020): “From Insights to Action: Gender Equality in the Wake of COVID-19,” Technical report, https://www.unwomen.org/sites/default/files/Headquarters/Attachments/Sections/Library/Publications/2020/Gender-equality-in-the-wake-of-COVID-19-en.pdf.
Zhang, Xuyao, et al (2021): Annual Competitivness Analysis and Gendered Impact of the COVID-19 Pandemic on Unemployment in the Sub-National Economies of India , Singapore: Asia Competitiveness Institute, Lee Kuan Yew School of Public Policy, National University of Singapore.
Image Courtesy; Canva

In light of the triple talaq judgment that has now criminalised the practice among the Muslim community, there is a need to examine the politics that guide the practice and reformation of personal....

- About Engage
- For Contributors
- About Open Access
- Opportunities
Term & Policy
- Terms and Conditions
- Privacy Policy
- Style Sheet
Circulation
- Refund and Cancellation
- User Registration
- Delivery Policy
Advertisement
- Why Advertise in EPW?
- Advertisement Tariffs
Connect with us

320-322, A to Z Industrial Estate, Ganpatrao Kadam Marg, Lower Parel, Mumbai, India 400 013
Phone: +91-22-40638282 | Email: Editorial - [email protected] | Subscription - [email protected] | Advertisement - [email protected]
Designed, developed and maintained by Yodasoft Technologies Pvt. Ltd.
Economics 201
Case of the day: the great depression, the broad outline.
The Great Depression of the 1930s has affected the study of macroeconomics more than any other event in history. Indeed, the founding of macroeconomics as a separate discipline largely coincided with attempts to explain the Great Depression. It wasn't until the 1970s and 1980s that mainstream macroeconomics emerged from being dominated by theories of recession and depression.
The most common association that the general public has with the Great Depression is the crash of the stock market that occurred in October of 1929. Stock prices did fall dramatically on the day of the crash and continued in a general downward trend for several years. However, the Great Depression was much more than a crash in financial markets. Although the crash had an impact on the Depression, it was less a cause of the Depression than a co-symptom of a collection of underlying problems.
Both because of its magnitude as a social phenomenon and because of its impact on the development of macroeconomics, it is important to understand some basic facts about what happened during the Great Depression. In the words of Milton Friedman and Anna Schwartz, from their Monetary History of the United States , "The contraction from 1929 to 1933 was by far the most severe business-cycle contraction during the near-century of U.S. history we cover [1867-1960] and it may well have been the most severe in the whole of U.S. history. Though sharper and more prolonged in the United States than in most other countries, it was worldwide in scope and ranks as the most severe and widely diffused international contraction of modern times. U.S. net national product in current prices fell by more than one-half from 1929 to 1933; net national product in constant prices, by more than one-third; implicit prices [a broad index of prices across the economy], by more than one-quarter; and monthly wholesale prices, by more than one-third."
The most commonly cited indicator of business cycles, the unemployment rate, rose from 3.2% of the labor force in 1929 to 24.9% in 1933. Unemployment fell to 14.3% in 1937, but then increased again to 19.0% in 1938 and was still 9.9% in 1941 before the military boom associated with World War II brought it down to 1.9% in 1943. Real gross national product (output of goods and services) per person did not until 1940 recover to the level it had achieved in 1929. As the quote from Friedman and Schwartz indicates, prices were falling steadily through the 1930s as well. Table 1 summarizes some major macroeconomic statistics for the period 1925 to 1940.
Table 1
Source: Historical Statistics of the United States, Colonial Times to 1970 (Washington, DC: United States Bureau of the Census, 1975).
Financial markets, banks, and the real economy
The stock-market problems—stock prices lost about three-quarters of their value between October 1929 and 1933—had significant repercussions for the banking system, which in turn affected the economy. At that time, it was common for investors to borrow from banks to purchase stocks "on the margin," using the value of the stocks as collateral on the loans. (Such margin loans are now much more carefully restricted.) A sudden collapse in stock prices reduced the value of the collateral, causing banks to make "margin calls," in which investors were asked to either repay their loans or provide additional collateral. In order to try to obtain enough liquidity to do one of these things, many investors attempted to sell their stock, which of course drove stock prices down even more rapidly in a self-reinforcing spiral.
Since many customers were not able to get enough liquidity to make their margin calls, defaults on bank loans soared, leading to a loss in confidence in the banking system. Meanwhile, as the economy soured, other bank loans were beginning to look less safe as well, raising the prospect of significant bank failures. At that time, bank deposits were not insured, so that if a bank was destined to fail, those depositors who were able to get their money out before it actually closed would be paid in full, while others would get their money back only in part and only after a delay (because the bank's remaining assets would have to be sold to determine how much could be paid). This gave depositors a very strong incentive to race to the withdrawal window to be first to get their money, starting a "run on the bank" any time a bank was thought to be in difficulty.
However, banks—then as now—keep only a small amount of depositors' money in the form of reserves (vault cash and readily available deposits at the Federal Reserve Banks). The rest is lent to customers to earn interest or used to purchase interest-bearing securities. A bank run can use up a bank's reserves very quickly, forcing it to either (1) sell off some of its loans and securities very quickly to get cash, (2) borrow cash from someone, perhaps the Federal Reserve, or (3) close down, at least temporarily. The securities (and even loans) of an individual bank may be quite "liquid" (commonly traded, so it is easy to find a willing buyer) during normal times, but when a whiff of crisis is in the air there are far more sellers in the market than buyers. If a bank is forced to sell into a frightened market, they may get very low "fire-sale" prices for the assets they are able to sell. If they have to sell assets at a loss, this worsens the bank's financial situation and may push it over the edge into insolvency, where the bank's liabilities exceed the value of its assets—the bank may fail.
When the Federal Reserve System was founded in 1913, its principal mission was to provide an "elastic currency" by acting as a "lender of last resort" for banks, lending to them through the "discount window" by purchasing their loans and securities for cash when the banks faced possible runs and needed liquidity. However, during the bank crises of the Great Depression the Fed put such strict rules on the kinds of assets that it would buy that emergency borrowing from the Fed failed to avert bank runs. Between 1929 and 1933, bank failures were so wide-spread that the number of commercial banks operating in the United States fell by over one-third.
The extraordinarily high rate of unemployment of the economy's labor resources was mirrored by underutilization of its capital. Industrial production declined by about half from 1929-33, leaving many factories, mines, and shops shut down and many others operating at far below their capacity. The economy had entered a vicious circle: aggregate demand for goods and services was so low that firms could not sell as much output as they could produce under full utilization of resources. Because production and employment were so low, households' incomes contracted severely so that they could not afford to buy many goods, which in turn kept aggregate demand depressed.
The apparent failure of the market system to coordinate households' and firms' economic decisions in an efficient way directly contradicted the fundamental efficiency implications of classical economics, which was based on the perfectly competitive model of general equilibrium. Moreover, the source of this coordination failure did not seem to lie in any of the classical causes of market failure such as externalities, public goods, or monopoly. Rather, the price system seemed to be ineffective at providing the appropriate signals to clear the markets for products and resources. Markets did not seem to clear; demand was apparently not equal to supply.
Explaining the nature of this failure was the task attempted by John Maynard Keynes, a brilliant but maverick British economist, in The General Theory of Employment, Interest and Money , published in 1936. Keynes focused on the key role played by aggregate demand in determining the overall level of economic activity in an economy. The refinement of models based on Keynes's insights and of alternative models exploring the relationships among economic aggregates led to the development of the discipline of macroeconomics.
Modern macroeconomists fall into two broad categories. Neoclassical macroeconomists think of the world as being primarily well explained by the competitive, market-clearing model. Keynesian (or New Keynesian) macroeconomists emphasize the imperfections of the market and the difficulties that may attend convergence to market clearing. Although there is much on which these two groups agree, they tend often to support different strategies for macroeconomic policy. Neoclassical economists usually favor a laissez-faire approach, relying on the self-correcting mechanism of the market to restore the economy to equilibrium. Keynesians tend to support a more active role for the government in managing aggregate demand either directly through fiscal policy (government spending and taxation levels) or monetary policy (using changes in the quantity of money to affect liquidity, interest rates, and hence aggregate demand).
The differences are highlighted in an amusing way by this video .
Questions for analysis
1. Macroeconomists classify variables as "procyclical" if they tend to be positively related to real output across the business cycle, in other words, if they usually rise in booms and fall in recessions. Variables that move in the opposite direction are called "countercyclical." Based on the evidence in Table 1, would you say that inflation was procyclical or countercyclical during the period shown? What about nominal interest rates? The unemployment rate?
2. Describe the pattern of nominal and real interest rates during the Great Depression. (Recall that the real interest rate is approximately the nominal rate minus the rate of inflation.) Why can't nominal interest rates ever be negative? What bound does this put on real interest rates when there is substantial deflation? Is it possible that this could prevent the loanable-funds market from clearing?
3. Can you explain why long-term interest rates moved down less in the Great Depression than short-term rates? The rates shown in Table 1 are rates on loans to extremely creditworthy borrowers: the U.S. government and the largest corporations. How do you think the "spread" between these rates and those of less reliable borrowers changed during the Great Depression?
4. If you operated a bank that had not (yet) experienced a run during the Great Depression, how would you change your lending and reserve-holding policies to try to prepare yourself for that possibility? Would these changes in lending, in turn, contribute further to the depression in aggregate demand?
- Skip to main content
- Keyboard shortcuts for audio player
Construction boom helps fuel job gains in March

Scott Horsley

Hiring accelerated in the U.S. in March, adding 303,000 jobs, according to a report from the Bureau of Labor Statistics. The unemployment rate dipped to 3.8%, staying under 4% for more than two full years. People walk past a Home Depot in San Rafael, Calif. Justin Sullivan/Getty Images hide caption
Hiring accelerated in the U.S. in March, adding 303,000 jobs, according to a report from the Bureau of Labor Statistics. The unemployment rate dipped to 3.8%, staying under 4% for more than two full years. People walk past a Home Depot in San Rafael, Calif.
Construction companies helped to hammer together another strong month for the U.S. job market.
The Labor Department reported Friday that construction firms added 39,000 jobs in March — a remarkably strong showing in the face of high interest rates. Hiring was also robust in health care, restaurants and local government. The unemployment rate dipped to 3.8%, even as more than 400,000 people joined or rejoined the workforce.
"Today's release was a blockbuster jobs report and indicates that recession is not arriving anytime soon," said Anirban Basu, chief economist for the Associated Builders and Contractors (ABC). He noted that construction job gains in March were about twice as high as the monthly average over the last year.
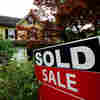
You'll need more than $100,000 in income to afford a typical home, studies show
The ABC's most recent Construction Confidence Index found nearly 48% of builders expect to add jobs in the next six months, while only about 11% expect job cuts.
That's surprising at a time when borrowing costs are so high. Mortgage rates are hovering around 6.8%, according to Freddie Mac . Of the jobs added in March, about 14,000 were in residential construction, 19,000 were in non-residential construction, and 6,000 were in civil engineering projects.
"We are seeing an uptick in project opportunities for our folks," said Ryan Odendahl, CEO of Kwest Group, a civil and industrial construction firm based in Perrysburg, Ohio. "We're doing everything from a large road construction project to bike path and park-style projects."
Kwest has about 340 employees and is looking to hire more. About 20% of the company's employees are military veterans, which Odendahl calls a good training ground.
"A squad leader is a foreman with different skills," Odendahl said. "The ability to work outside. The ability to change quickly and to handle adversity that happens on projects every day."
The unemployment rate for construction workers was 5.4% in March — higher than the national average but down from 5.6% a year ago.
"Young people are starting to see the opportunity, both from an earnings potential and a growth potential that the construction industry offers," Odendahl said. "We need to do a better job as an industry of telling some of the great things that are going on in construction. We are competing with all of the other industries in the economy for people."
Overall, U.S. employers added 303,000 jobs in March — significantly more than forecasters had expected. The strength of the job market has allowed the Federal Reserve to take its time in cutting interest rates.
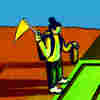
This year, colleges must choose between fast financial aid offers, or accurate ones
"We don't need to be in an hurry to cut," Fed chairman Jerome Powell said recently at the Federal Reserve Bank of San Francisco. "We can wait and become more confident that in fact inflation is coming down to 2%."
The Fed will be watching closely when March inflation numbers are released next week. Average wages in March were up 4.1% — almost certainly outpacing the rise in prices.
- construction
- Unemployment
- Federal Reserve
The Century Foundation The Century Foundation The Century Foundation
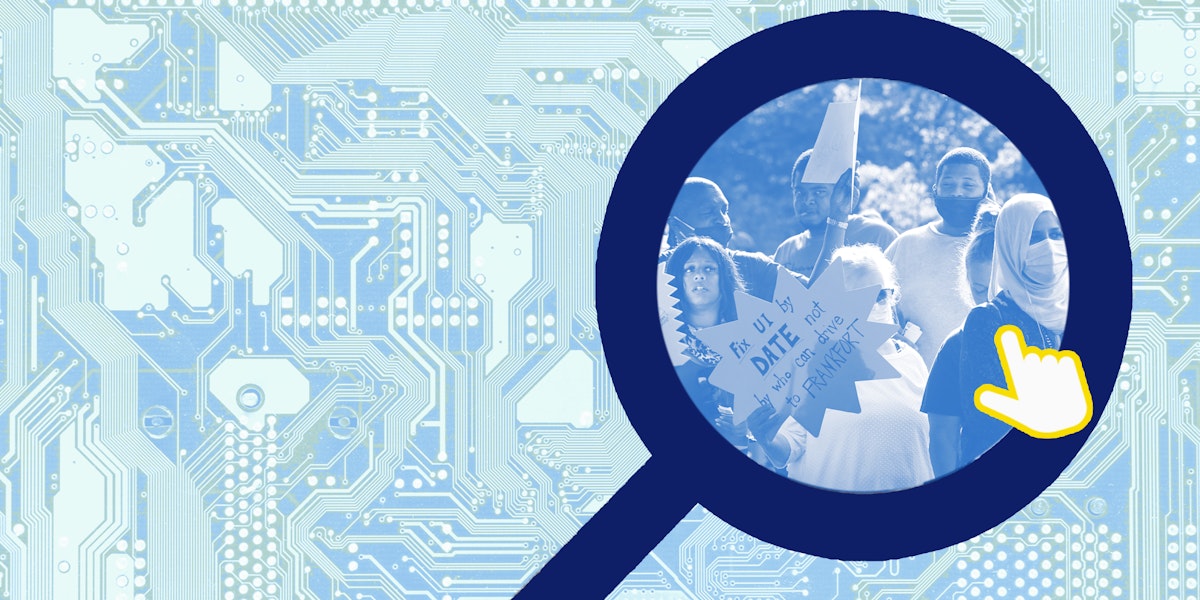
Centering Workers—How to Modernize Unemployment Insurance Technology
- Download (EN) (EN)
Executive Summary
This report, jointly authored by The Century Foundation, the National Employment Law Project, and Philadelphia Legal Assistance, presents the findings of an intensive study of state efforts to modernize their unemployment insurance (UI) benefit systems. This is the first report to detail how UI modernization has altered the customer experience. It offers lessons drawn from state modernization efforts and recommends user-friendly design and implementation methods to help states succeed in future projects.
While the need for better systems was apparent even before the COVID-19 pandemic struck, that crisis has illuminated the challenges with the existing UI infrastructure. This report includes specific recommendations that can inform the federal and state response to the unprecedented volume of unemployment claims during the pandemic, as well as ideas for longer-term reforms.
What Is Benefits Modernization?
Benefits modernization is the process of moving the administration of unemployment benefits from legacy mainframe systems to a modern application technology that supports web-based services. Many of these mainframe systems were programmed with COBOL, a long-outdated computer language. A few states began to upgrade their systems in the early 2000s, with the pace picking up after targeted federal funds were made available to support modernization in 2009.
Unfortunately, many of the initial modernization projects encountered significant problems. Some were abandoned altogether, while others were poorly implemented. Too often, workers paid the price through inaccessible systems, delayed payments, and even false fraud accusations. The COVID-19 pandemic, which led to an unprecedented spike in unemployment claims, has further exposed weaknesses in these systems.
To date, fewer than half of states have modernized their unemployment benefits systems. Several have plans to modernize or are already in the midst of modernizing. The guidance in this report is meant for them, as well as for modernized states that are looking to improve their systems.
Research Methods
The findings and recommendations in this report are grounded in interviews with officials from more than a dozen states and in-depth case studies of modernization in Maine, Minnesota, and Washington, conducted from October 2018 to January 2020. The case studies involved many hours of in-person discussions with agency leadership and staff, focus groups with unemployed workers, and interviews with legal services organizations, union officials, and other stakeholders.
The report provides lessons for states no matter which pathway to modernization they choose. In fact, the three states featured in the case studies took notably different approaches. Minnesota was one of the first to modernize in 2007, and while it used a private vendor, the code remains the property of the state agency. Washington contracted directly for a proprietary commercial off-the-shelf (COTS) system which rolled out in 2017. Maine also modernized in 2017, but as part of a consortium, meaning that it shares system and maintenance expenses with two other states (Mississippi and Rhode Island).
To complement the interviews and case studies, this report presents new data analysis , suggesting that while timeliness in processing claims and paying benefits improved in many states after they modernized, denial rates went up for workers seeking benefits and the quality of decisions declined.
The report also examines the growing use of artificial intelligence and predictive analytics in unemployment insurance. It concludes that, while some of these tools can improve operations and potentially help workers better understand program requirements, major concerns about fairness, accuracy, and due process remain.
Recommendations
The single strongest recommendation in this report is for states to place their customers at the center of a modernization project, from start to finish. The biggest mistake states made was failing to involve their customers—workers and employers—at critical junctures in the modernization process. This led to systems touted as convenient and accessible, but which claimants often found challenging and unintuitive. Customer-centered design and user experience (UX) testing are widely accepted best practices in the private sector, and should be a core part of any UI modernization effort.
More specifically, the report provides recommendations for states at each of the three major stages of a modernization project.
At the planning stage:
- setting a realistic timetable and to avoid rushing implementation;
- embedding talented agency staff in a modernization effort and getting their buy-in every step of the way;
- asking customers what they need;
- being willing to revamp the agency’s business processes along with the technology; and
- identifying key conditions up front in an RFP (for agencies using outside vendors).
At the design stage:
- getting user feedback from a broad range of stakeholders;
- allowing plenty of “sandbox” time for agency staff; and
- building in a set of key features that will help customers and reduce the burden on agency staff.
At the implementation stage:
- not going live in the November–March period, when seasonal claims rise;
- considering rolling out pieces of a new system in stages;
- training and supporting staff on an ongoing basis;
- staffing up call centers and deploying additional staff to career centers;
- having a robust community engagement plan;
- expecting bugs and having a process in place to fix them; and
- providing for ongoing feedback from customers and front-line staff.
The pandemic has revealed how critically important unemployment insurance is to workers, their families, and the broader economy. By following the steps outlined in this report, states can build stronger unemployment systems that deliver the services and benefits their customers need.
What States Can Do Right Now
The release of this report coincides with the emergence of one of the greatest challenges the unemployment insurance system has ever faced: the COVID-19 pandemic. More than 10 percent of the workforce filed initial claims for unemployment in a three-week period in March and April, and job losses continued to rise thereafter.
State systems have been overwhelmed by the basic task of accepting claims, and workers are frustrated. Luckily, there are immediate steps states can take to improve access, even within outdated systems. Some states are already moving to implement these reforms, and others should follow their lead as quickly as possible.
Michigan is a good example of a state that has turned around a poorly engineered system into one that is serving workers well during the pandemic. While the original system had been modernized, the new system was designed with a faulty algorithm that inaccurately flagged workers for fraud and cut off benefits at every decision point. A new governor appointed a claimant representative to head the agency, and less than a year later, the system faced the massive challenge posed by the COVID-19 outbreak. The new leadership identified places in the system that placed an unnecessary hold on benefits and turned off those chokepoints. As a result, the agency became the second-fastest in new benefit processing among the ten states with the largest numbers of claims, 1 and one of the first states to stand up the new Pandemic Unemployment Assistance benefit and the Pandemic Unemployment Compensation benefit authorized by the CARES Act.
Our recommendations for what states can do now come from our study of best practices at the state level. While states are unlikely to be able to fully replace their UI systems in the midst of this crisis, they can and must improve their technology. Here are six key areas for immediate improvement.
First, unemployed workers need 24/7 access to online and mobile services. We live in a country where you can shop online at any hour of the day. Filing for unemployment shouldn’t be restricted to nine to five on weekdays.
Second, unemployment websites and applications must be mobile-optimized. More people have mobile phones than desktop or laptop computers, and public access to computers has vanished in an era of social distancing. Low-wage workers and workers of color are particularly likely to rely on their phones for Internet access. While more than 80 percent of white adults report owning a desktop or laptop, fewer than 60 percent of Black and Latinx adults do. States must also allow workers and employers to email documents or upload them from their phones.
Third, states should update their password reset protocols. In some states, workers must be mailed a new password; in others, staff cannot process claims because they are busy answering phone calls about password resets. Technology exists for states to implement secure password reset protocols that do not require action by the agency, which saves time for everyone.
Fourth, states can use call-back and chat technology to deal with the unprecedented volume. These short-term fixes could become part of a permanent solution. “Call back” systems return a worker’s phone call instead of making them wait on hold. Chat-bots, live chats, and thought bubbles can define terms and answer simple questions for workers filing online.
Fifth, states should adopt a triage business model. Many of the questions coming into the call centers relate to passwords or claim status. Using a triage model, states can quickly train staff to handle this part of the volume, and leave more challenging questions to more experienced staff.
Finally, civil rights laws require that states translate their websites and applications into Spanish and other commonly spoken languages. Right now, an unemployed worker with limited English skills may have no choice but to file an application over the phone with an interpreter. With so many seeking help, workers may never get through on the phone or can get stuck on hold for hours. Translating online materials would not only ensure equal access, but also be more efficient.
Even if these measures take a number of weeks or months to implement, the investment would be well worth it. The employment crisis triggered by the pandemic has highlighted gaping holes in accessing unemployment, but it has also created an opportunity. We can build twenty-first-century systems nimble enough to handle disasters and designed to meet the needs of customers who are depending on access to unemployment insurance in this traumatic time.
Introduction
Unemployment insurance (UI) is the nation’s primary social program for jobless workers and their families. The federal–state UI program was established by the Social Security Act of 1935, providing critical income support for workers contending with a national unemployment rate of approximately 30 percent during the Great Depression. More recently, UI was credited with reducing poverty, empowering workers, and stabilizing the economy during the Great Recession. 2 The benefits available through these programs keep workers economically stable during periods of job loss, often meaning the difference between losing access to vital job search resources like a phone or a car, or basic necessities like a roof over one’s head and food on the table.
While the research for this report was conducted before the COVID-19 crisis erupted, the current economic crisis has put into sharp focus the need for strong unemployment systems. Between March 14 and April 25, 30 million Americans—one-fifth of the workforce that is covered by unemployment insurance programs—filed an initial application for unemployment benefits. These workers experienced overwhelmed phone lines and websites, and most importantly, excruciating delays in receiving benefits. As the crisis erupted in March, only 14 percent of the 11.7 million jobless workers who filed claims received a UI payment. A recent survey revealed that for every ten workers who were able to file for unemployment insurance, three to four additional workers tried to apply but could not get through UI systems to make a claim, and two additional people did not try because it was too difficult. 3
This report explains efforts by states to modernize unemployment benefits systems—many of which still to this day rely on 1980s technologies such as mainframe computers running on COBOL programming language—and explores ways for these new systems to realize their potential to be more customer-friendly and scalable to challenges like the ones states face today. In looking at challenges and best practices among states, we also identify actions states can take right now, even before modernization, to improve the experience of workers and the performance of UI systems.
Most importantly, as states and the federal government look to rebuild the backbone of this crucial safety net anew, our report suggests a new customer-driven approach geared to meet the needs of jobless workers reaching out for help during the long economic recovery ahead and future economic crises. As those new systems are built, the practical realities of workers’ lives must be kept front and center. Our analysis finds, for example, that states that modernized their benefits systems were more likely to deny benefits because they have imposed more stringent online verification of work search activities that workers struggle to navigate. While these rules have been temporarily eased in many states during the COVID-19 crisis, they could become a major factor as the economy opens up.
While a few states began to modernize in the early 2000s, this trend picked up around the time of the Great Recession, as states began looking for technological improvements that could streamline business processes, reduce costs, and provide better security and privacy protocols for the massive amount of data maintained by the UI system. These UI benefit modernization projects involved moving state unemployment benefits and appeals systems from a “legacy” mainframe-based system to an application technology that supports web-based services. They gained significant traction as states received targeted federal funds in 2009. These upgrades were necessary for security; one state secretary of labor described the mainframe system as held together by “bubblegum and duct tape.” 4 The upgraded systems also offered customers the potential for more convenient online filing, notification of claims progress, and appeal filing.
Some early modernization efforts were unsuccessful. As of 2016, 26 percent of projects had failed and been discarded; 38 percent were past due, over budget, or lacking critical features and requirements; and 13 percent were still in progress. System failures can have disastrous human consequences. UI systems that are poorly planned and lack critical user testing limit claimants’ ability to access benefits, and sometimes cut them off from benefits entirely. Many states experienced significant lock-out issues when claimants had no easy way to reset their online account passwords. 5 Florida cut off all points of access to its system for anyone not using the online tools, creating significant barriers for workers with language access, computer literacy, or broadband access issues. Systems that incorporated automated decision-making processes generated tens of thousands of incorrect fraud determinations that put workers into massive debt, drove them to bankruptcy, and cut off future access to unemployment benefits. 6
However, the last few years have shown great improvement by states, with more states implementing full systems while at the same time controlling costs. Some states learned from past challenges and made improvements in time for challenges presented by COVID-19.
This report is the first to detail how UI modernization has altered claimant experiences. It shares the findings from interviews with officials from more than a dozen states and in-depth case studies of modernization in Maine, Minnesota, and Washington. It also analyzes publicly available UI data to run a comparative analysis of state UI programs at various stages of modernization. The report then draws on those interviews, case studies, and data analysis to present a set of recommendations for states to follow in their modernization projects.
States that have modernized acknowledge that the process is challenging and never perfect, but many have sought to learn from these experiences to build user-centric systems with positive outcomes for workers. Our hope is that this report will aid all states in doing so.
The Role of Unemployment Insurance
UI serves several key policy goals. Most obviously, it provides income stabilization for individuals who are involuntarily unemployed through a cash benefit. This stabilization extends to the local economy generally, but is critically important during times of economic downturn. The UI system also promotes attachment to the workforce and provides job search assistance and standards to prevent workers from accepting new work that is not suitable to their skills and to avoid downward pressure on wages.
Generally, during periods of recession, Congress has acted to temporarily extend benefits for workers after they exhaust their standard twenty-six weeks of state UI. During the Great Recession, state and federal UI payments totaled over $600 billion, keeping 11 million workers above the poverty line. 7 Economists Alan Blinder and Mark Zandi examined the effect these payments made in the recovery, and found that every dollar in benefits paid generated $1.61 in local economic development. 8 Similarly, the CARES Act of 2020 added thirteen weeks of benefits, temporarily increased payouts by $600 per week, and expanded eligibility to new categories of workers, delivering an estimated $250 billion in support to workers and the broader economy.
Maintaining the federal–state UI program is a macroeconomic balancing act. During periods of economic growth, UI agencies build up their trust funds to prepare to stabilize the workforce and economy during periods of economic recession. However, while the level of funding in a state’s UI trust fund is an important indicator of a state’s recession readiness, just as important—perhaps even more so—is a state’s ability to make benefits available to workers accurately, efficiently, and in a timely manner during a recession. As we evaluate the effect of modernizing IT systems, it is critical that we recognize access to benefits as an important countercyclical tool.
Who Does the System Serve?
UI systems have two primary customers: workers and employers. While worker benefits are the most visible part of the system, employers also interact with the UI system from both a tax and a benefit perspective.
While every state operates its program differently, in general, employers are charged for UI benefits based on their former employees’ experience with the system. This taxation system is referred to as “experience rating.” State UI taxes (assessed per the State Unemployment Tax Act, or SUTA) are levied on whatever the state sets as a Taxable Wage Base—which can vary from the first $7,000 of income to the first $46,800 each worker earns. The UI tax structure gives employers an interest in whether or not workers receive benefits, as benefit receipt can put employers on the hook for higher taxes. Employers are also included in initial investigations into benefit eligibility, receive notices about eligibility determinations, appeal determinations, and often participate in administrative hearings that address the reason a worker is unemployed.
Workers have historically been assessed for eligibility on two bases: financial eligibility and separation eligibility. Financial eligibility is based on how much money a worker earned during the qualifying base period and how often they earned money. Separation eligibility addresses why the worker is unemployed—that is, whether they left their job for a qualifying reason, such as through a layoff, a discharge without cause, or quitting for good reason.
Workers also have continuing eligibility requirements that require them to file weekly or biweekly certifications to receive benefits in which they must report any earnings, show they are able to work, and inform the state they are still unemployed. Additionally, workers’ continuing eligibility may be challenged based on their availability for work and effort made to find suitable employment. In order to interact with the system, workers must file initial claims, communicate with the agency representatives, file continuing claims, receive notices about eligibility, appeal determinations, and participate in administrative hearings.
The states are obligated under federal law to serve customers in a manner that also ensures due process. Specifically, the Social Security Act of 1935, which created the UI program, requires that the states provide for “methods of administration . . . reasonably calculated to insure the full payment of compensation “when due” and for a “fair hearing.” 9 These provisions necessitate fair but also rapid and accurate administration of the program so that workers are able to receive benefits within a few weeks of losing work. Failing to conform to these requirements can trigger the loss of a tax credit to employers (per the Federal Unemployment Tax Act, or FUTA) of 5.4 percent of the first $7,000 in worker pay. The U.S. Department of Labor’s Employment and Training Administration (ETA) plays a critical role enforcing these federal safeguards and ensuring compliance by the states.
Racial Equity Implications of the UI System
The evidence suggests that institutional racism plays a significant role not only in unemployment but also in access to UI systems. If we look at the racial equity implications of modernizing UI systems, we see a compelling reason to center the experiences of Black and brown workers.
Higher Unemployment Rates
Black and Latinx workers face labor market obstacles and exclusions due to hiring discrimination rates that have remained unchanged over the past twenty-five years. 10 The unemployment rate for Black workers across almost every level of education has remained double that of white workers for nearly forty years. 11 And in the ten largest majority-Black cities, the unemployment rate of Black residents was 3.9 to 10.8 percent higher than that of white residents. 12
Lower UI Benefits Despite facing higher rates of unemployment, evidence shows that Black and Latinx workers do not receive UI benefits at the same rate as white workers. In 2010, following the Great Recession, non-Latinx Black unemployed workers had the lowest rates of receiving UI benefits at only 23.8 percent, compared to 33.2 percent of non-Latinx white unemployed workers; meanwhile, only 29.2 percent of Latinx unemployed workers received benefits. 13 Between April 27 and May 10, 2020, over 71.5 percent of Black unemployed women did not receive unemployment benefits, compared to just 54 percent for white unemployed women.
Racial Wealth Gap Applying for unemployment insurance during normal times can be a complicated and arduous process; add in a built-in waiting week policy in many states, and workers are often left struggling with a gap in income. With somewhere from half to 74 percent of all workers reporting they live paycheck to paycheck, a wait for a UI check—or no check at all—can be painful. 14 This is particularly true if a worker doesn’t have wealth to fall back on. Being able to wait for unemployment benefits is a luxury afforded to those with savings and wealth; and unsurprisingly, this nation’s racial inequities have created a racial wealth gap.
America’s laws and policies have deprived people of color of an equitable share of the nation’s wealth. The typical net worth of a white family is nearly ten times that of a Black family and seven times that of a Latinx family. 15 And more than 25 percent of Black households have zero or negative wealth, compared to less than 10 percent of white households. 16 Without wealth to fall back on, workers of color are even more harmed by inefficient and ineffective systems.
Racial Wage Gap
Racial wage gaps mean lower earnings, resulting in smaller UI benefits. Black men earn just 73 cents—and Latinx men earn just 69 cents—for every dollar earned by a white man. 17 And while the overall gender wage gap means that for every dollar earned by a white man, the average white woman just makes 79 cents, women of color earn even less: 62 cents for Black women, 57 cents for Native American women, and 54 cents for Latinx women. 18
Digital and Mobile Divide
How people access information regarding and apply for unemployment benefits is also impacted by race. More people have mobile phones than desktop or laptop computers, 19 and unemployment websites and applications that are not mobile-responsive disproportionately place a burden on workers of color. Twenty-five percent of Latinx and 23 percent of Black adults, compared to just 12 percent of white adults, are entirely smartphone dependent and do not use broadband at home. 20 While more than 80 percent of white adults report owning a desktop or laptop, fewer than 60 percent of Black and Latinx adults do. 21 And when it comes to job searches, 55 percent of Black and Latinx workers, compared to just 37 percent of white, use their smartphone to get information about a job; and when applying for jobs, Black and Latinx workers are more than twice as likely than white workers to apply for a job using their mobile device. 22 Ensuring all workers can navigate the UI system requires access to mobile-friendly programs.
Biased Algorithms
According to the research institute Data & Society, “algorithms can be incredibly complicated and can create surprising new forms of risk, bias, and harm.” 23 Algorithmic systems can have bias in multiple places, including biases introduced through data input or by the algorithm creator. 24 One of the problems with algorithmic systems is that they often make decisions that result in different outcomes based on protected attributes, including race, even if these attributes are not formally entered into the decision-making process. 25 For example, evidence shows a racial impact in medical algorithms that ignore social determinants of health or result in Black people needing to be sicker than white people before being offered additional medical help. 26 Without external auditing systems that assess how the data is processed, these biases are allowed to go unchecked. 27
Due to the layers of institutional racism faced by Black, Indigenous, and workers of color, we must work alongside worker leaders and organizations to create a racially just, inclusive, and truly accessible unemployment insurance system. A modernized unemployment insurance system should center the experiences of workers of color and collect data on race and ethnicity, to ensure states are adequately meeting the needs of all workers.
Administrative Funding
Critical to the viability of state UI programs is access to adequate funding for administration of the tax, benefits, and appeals systems. The federal government funds the administration of the state UI programs, including eligibility determinations, tax collections from employers, and the appeals process. State systems have been chronically underfunded, and the resulting search for efficient technology solutions is one of the principal motivators behind benefit modernization projects.
Administrative grants are tied to the amount of unemployment insurance claims paid out by the state and therefore drop when there are improvements in the economy and declines in UI recipiency. As a result, federal grants for the administration of unemployment insurance declined by 30 percent from 1999 to 2019 on an inflation-adjusted basis. 28
These funding levels during this period were barely enough for states to manage the basic staff needed to operate their UI programs, let alone upgrade and maintain unemployment insurance technology. The approximately $2 billion in annual federal funds available to states before the COVID-19 crisis left state UI programs with threadbare staffs that struggled to address the sudden and major surge of claims. In particular, states lacked flexible technology that could quickly incorporate law changes, bandwidth to process claims, and enough trained staff to ramp up call center and adjudication operations that require interactions with claimants. While the federal government provided an additional $2 billion in state UI administrative funding under the Families First Coronavirus Response Act, which is allowing the states to increase staffing and pursue short-term technology improvements to respond to COVID claims, the “boom and bust” cycle of federal funding is inconsistent with the needs of the states for stable levels of funding to sustain their UI programs.
Federal regulations stipulate that states should deliver a first payment to at least 87 percent of eligible applicants within fourteen or twenty-one days from their initial claim for benefits (the difference is that those states with a waiting week are given 21 days to make a payment). 29 The national average of first payment timeliness dropped below the national standard in 2008, which was understandable, given that the surge of unemployment in the early years of the Great Recession came before states got more administrative dollars from the formula. However, timeliness did not recover, even when UI claims fell to their lowest level in fifty years. Throughout our interviews, state officials consistently complained about having to juggle staffing to meet all their requirements, including taking claims, and cited low administrative funding as a reason for driving more claimants to online initial applications.
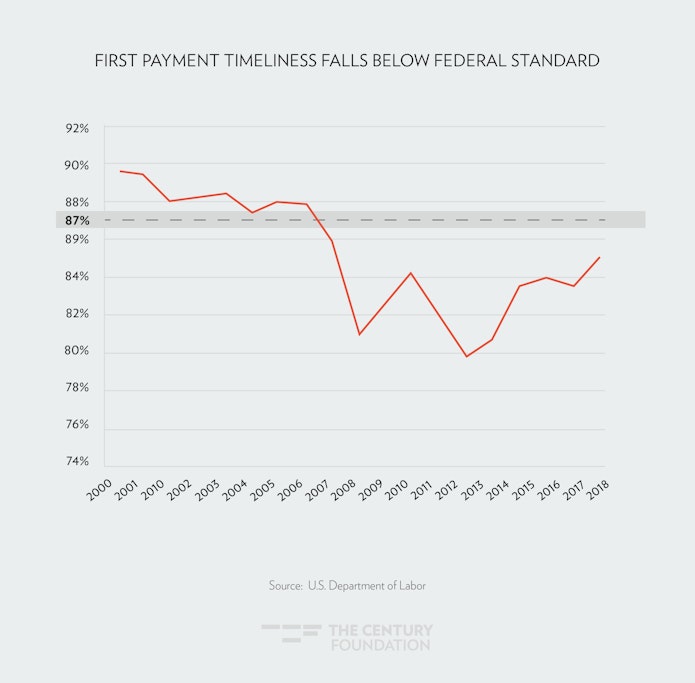
As a result, states have been forced to look for supplemental funding. Between 2007 and 2016, the National Association of State Workforce Agencies reported a 115 percent national increase in state supplemental spending for UI administration. As of 2017, seventeen states and territories reported the use of a state administrative tax to supplement administrative costs; nine states reported using general revenue, while twelve states said they used other sources for administrative funding. 30 The federal government has periodically, but not consistently, provided additional funding that has supported benefits modernization projects, as discussed further below (see “The Federal Role”).
Benefit Modernization: Where Are We Now?
Since the early 2000s, states have been working toward upgrading their UI technology. Driven by concerns about data security and privacy, administrative costs, and efficiency, states have tried a variety of methods to either wholesale replace their systems or gradually improve their components. Most have done so through contracts with private vendors, sometimes as part of a consortium, where system and maintenance expenses are shared across a group of states, but the product itself is customized to some degree to each participating state’s needs. At least one state, Idaho, handled its modernization project entirely in house. 31 Consortium models hold the potential to generate even more financial savings if other states join the consortium later, but states still have to navigate governance issues.
For many years, modernization projects trended in the red—encountering significant cost overruns and schedule delays, with several states actually pulling their projects. As noted in the introduction to this report, as of 2016, 26 percent of projects had failed and been discarded; 38 percent were past due, over budget, or lacking critical features and requirements; and 13 percent were still in progress. 32 However, the past few years have shown great improvement, with more states implementing final systems while controlling costs.
As of 2019, twenty-two states had completed modernization projects for their UI benefits systems and twenty-one states completed modernization projects for their UI tax collection systems (sixteen states have completed both). Thirteen states reported having UI modernization projects in development, 33 including two—Ohio and Montana—that are re-modernizing after an early 2000s implementation.
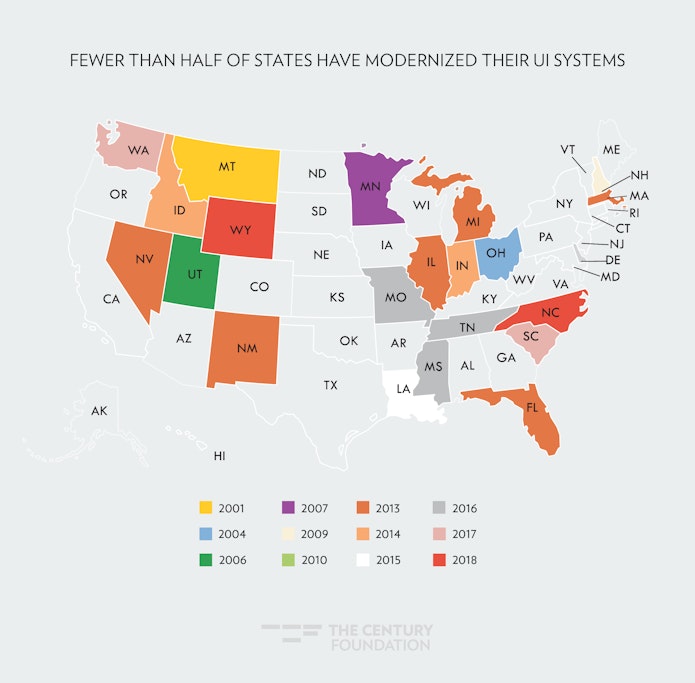
The completed modernization projects have encountered significant problems, including numerous delays, issues with testing, data conversion errors between legacy and new systems, data loss and security issues, and poor training of staff who interact with claimants. For example, Massachusetts’ modernized system, built by Deloitte, was $6 million over budget and, after rolling out two years late, was riddled by implementation problems. 34 Call center wait times doubled, and there were 100–300 claimant complaints per week, owing in large part to a major increase in system-generated questionnaires to claimants, which delayed claims processing. 35
While Tennessee’s system was developed by a different vendor, Geographic Unemployment Solutions, they experienced some of the same problems as Massachusetts: the system auto-generated numerous non-critical questions about applications that had to be cleared by staff, and the backlog for responding to user questions about claims stretched to eighty-two days after the system was rolled out in May 2016. Data conversion problems between the legacy and new system caused delays in payments, all during an implementation that a legislative audit later concluded was rushed. 36
In several other states, implementation has been rushed to meet external deadlines, leading to situations like that in Maine and Washington (described in depth in the case studies) where the state’s system was not ready for a surge of claimant questions, glitches caused the system to go down after launch, and repeat problems with core elements like passwords could not be solved. 37
Florida’s CONNECT system was riddled with timeliness and accuracy problems when it launched, including more than 400,000 claims documents that were stuck in an “unidentified” queue and unable to be processed. 38 The implementation of the new system coincided with a new requirement that claimants report five employer contacts per week, which could only be reported online through the new system. As described in a previous NELP report, “the number of workers disqualified because DEO [the Florida Department of Economic Opportunity] found they were not ‘able and available for work’ or not ‘actively seeking work’ more than doubled in the year following the launch of CONNECT, even though weekly claims declined by 20 percent in that same year.” 39 The U.S. Department of Labor Civil Rights Division found that this aspect of the system had a discriminatory effect on Limited English Proficient claimants who struggled the most. 40 New Mexico’s modernization implementation also faced a civil rights complaint from a legal service organization based on multiple language access problems, including an elderly Spanish-speaking farm worker who was told he could file online in a site that was only in English. 41
The U.S. Department of Labor (DOL) has issued guidance advising states to move away from phone-based work-search verification and instead move to online collection of work search activities through a case management system. 42 But as was demonstrated in Florida, verifying details of job-seeking efforts (such as details of job applications submitted) online can be a hurdle for many claimants who have difficulty navigating online systems, like those with limited English proficiency.
The DOL guidance on work search is one aspect of the department’s focus on “program integrity;” that is, reducing the incidence of payments made in error. There have been several supplemental federal appropriations related to program integrity, which have been used to fund modernized systems featuring new models to detect improper payments and assess fraud. In one such instance gone badly awry, the state of Michigan used an automatic determination process that falsely charged more than 20,000 claimants with improperly collecting unemployment benefits (such as by both working and collecting UI benefits). 43
This report intends to help modernizing states learn from the challenges these early-adopting states faced. It is clear from the research done for this report that UI modernization is maturing, with several states joining forces in consortia in an attempt to reduce the costs of development and maintenance of their system. Moreover, states are benefiting from products that have been developed by vendors specifically for UI and that have been road-tested in these early applications, and can be deployed more smoothly. Still other states have designed their own unemployment insurance technology systems, and, as in the case of Idaho, are making their technologies and expertise available to other states. Taken together, these advances put the UI system in position to improve the outcomes of UI modernization, both among those states that have yet to modernize and who are looking to improve their systems. At the same time, it is important to learn from the ways that COVID-19 pandemic has tested and stressed these systems, and how states have responded.
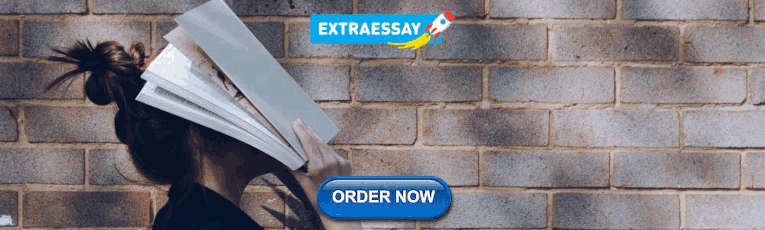
Sign up for updates.
The federal role.
While each state UI agency is responsible for its own benefit modernization project, the federal government plays a critical role in funding the projects and oversight to ensure compliance with the legal safeguards requiring fair and timely processing of benefits. Congress has also played a monitoring role, assisted by research provided by the U.S. Government Accountability Office (GAO), which issued a series of reports addressing the severe staffing, phone claims system, and IT challenges that compromised access to benefits during the Great Recession. 44
Funding Federal funds have been critical to many benefit modernization projects. During the Great Recession, Congress authorized the release of federal trust fund dollars (called “Reed Act” distributions, which are based on each state’s share of FUTA revenues) to the states to stabilize the solvency of their state trust funds, expand benefits, and support UI administration. In 2009, as part of the American Recovery and Reinvestment Act, Congress passed the UI Modernization Act, which created an incentive program for the states to expand UI benefits for low-wage and part-time workers, many of whom are women, while also helping the states make critical investments in IT, staffing, and other UI administration needs. From 2009 to 2011, 39 states claimed about $4.5 billion in incentives to improve UI systems, including improving technology. 45
In addition, in recent years, DOL has made varying amounts of supplemental funding available to support state consortia and state IT needs. However, that funding has been limited and typically comes with a number of strings attached, such as mandates that the systems are designed to identify UI overpayments and assurances that the projects would be completed with other sources of funding, if necessary to cover the full cost.
Oversight DOL’s Employment and Training Administration (ETA) has set some standards in response to the growing reliance of states on technology to process UI benefits. Of particular significance, in 2015, ETA and DOL’s Civil Rights Center issued state guidance entitled “State Responsibilities for Ensuring Access to Unemployment Insurance Benefits,” 46 which relied on the federal UI and civil rights laws to clarify where technology can compound problems of access to UI benefits facing many unemployed workers. On May 11, 2020, DOL updated this 2015 guidance in response to the COVID-19 pandemic, pointing out to states the requirement that they translate vital written, oral, and electronic information into languages spoken by a significant portion of the eligible or affected population, as defined by Department of Justice guidelines. 47 Moreover, states must provide access to people with disabilities, including online enrollment systems that incorporate modern accessibility standards for deaf, blind, and otherwise disabled applicants.
This comprehensive interpretation of federal law provides a template for states to ensure full and fair access to benefits when modernizing their IT systems. The federal guidance clarifies that the “use of a website or web-based technology as the sole or primary way for individuals to obtain information about UI benefits or to file UI claims may have the effect of denying or limited access to members of protected groups in violation of Federal nondiscrimination law.” 48 It goes on to caution that the state UI agencies “must also take reasonable steps to ensure that, if technology or other issues discussed in this [guidance] interfere with claimants’ access, they have established alternative methods of access, such as telephonic and/or in-person options.” 49
On a separate track, in 2018, DOL rolled out a “pre-implementation planning checklist” for states to follow, which candidly recognizes that “recent efforts by states in launching new IT systems have resulted in unexpected disruptions in service to customers, delays in payment of benefits, and the creation of processing delays.” 50 Before going “live” with a new UI benefit or tax IT system, the states are required to submit a report to DOL indicating that they have reviewed and addressed each element of the checklist, 51 which covers all phases of the process, including functionality and testing of the system, customer access and usability, policies and procedures, implementation preparation, call center operations readiness, vendor support, communications, training and other core functions and activities. 52
The checklist was developed with the assistance of the Information Technology Support Center (ITSC), which is operated by the national organization of state UI and workforce agency administrators (called the National Association of State Workforce Agencies, or NASWA). ITSC also provides UI IT modernization resources online and other services funded by DOL grants, which are available only to NASWA members. ITSC also provides consulting services at the initial planning phases of a state’s UI IT project.
To date, only a limited number of states have launched new systems that require submission of the planning documents. ITSC is not responsible for reviewing the pre-implementation planning documents to evaluate their compliance with the checklist. And given its limited resources and expertise in UI IT planning and implementation, ETA is likely to defer to the judgement of the states in evaluating the planning documents.
Recommendations for States
This section presents our full list of recommendations to states on how to plan, design, and implement a UI benefits modernization project. These recommendations are grounded in interviews with officials from more than a dozen states; in-depth case studies of UI modernization projects in Maine, Minnesota, and Washington; and analysis of data on UI system performance from all fifty states. (See the remainder of this report for these materials.) This list of recommendations presents:
- best practices, as identified in our interviews and case studies of state UI modernization projects;
- lessons learned about missteps to avoid; and
- best practices from beyond UI, looking at both the public and private sectors.
These recommendations will be most applicable to states that have not yet modernized, or who are in the midst of modernization. However, they may also be helpful to states that have already modernized but are looking to improve their systems.
We recognize that state UI agencies operate with limited human, financial, and technological resources. Nonetheless, we believe the recommendations presented here are achievable even within those constraints, particularly if federal funds are available to bolster state efforts.
We have structured our recommendations to follow each of the three major stages of modernization: planning, design, and implementation. Our single strongest recommendation is to place customers at the center of the project, from start to finish. The biggest mistake we saw states make was failing to involve workers at critical junctures in the modernization process. This led to systems touted as convenient and accessible, but which claimants often found challenging and unintuitive. Customer-centered design and user experience (UX) testing are widely accepted best practices in the private sector, and should be a core part of any UI modernization effort.
Stage 1: Planning
Recommendation 1.1. Set a realistic timetable. Many state officials interviewed for this project said they regretted allowing too little time and having to rush implementation. Allow ample time for planning and design, including user testing and refinement, before you roll out a new system.
Recommendation 1.2. Get buy-in from agency staff. Modernization projects demand full commitment and cooperation across all agency divisions. It may be challenging to divert talented staff away from their daily responsibilities, but to succeed, you have to embed them in the modernization effort and get their buy-in every step of the way. Experienced staff can draw on their institutional knowledge to inform operational change management. Staff with coding experience can help ease the data migration from legacy systems. Seek their input early, to inform your RFP (if you are using an outside vendor) and make sure you don’t omit anything critical.
Recommendation 1.3. Ask customers what they need. As part of your initial needs assessment, reach out to unemployed workers and employers, and ask them what they would like to see in a modernized UI system. Agency staff will provide valuable input, but there is no substitute for going straight to your customers.
Recommendation 1.4. Be willing to revamp your business process. As you plan your modernization project, don’t be held back by your current business process. Assume it can and will change, rather than designing your new system around processes that may no longer make sense in a new environment.
Recommendation 1.5. Identify key conditions in your RFP. If you are using an outside vendor, making these three conditions clear in your RFP will help you negotiate a contract that sets you up for success.
1.5.1. Retain control of the go-live date. You don’t want to be rushed by a vendor into rolling out a new system before you are confident that you are ready.
1.5.2. Allow for extensive usability testing by your staff and customers. Some vendors only test out a product with their own software engineers, a narrow approach known as user acceptance testing (UAT). Make sure you have the opportunity for broader user experience (UX) testing, to gather input from workers and employers in your state.
1.5.3. Provide for tech support after the go-live date. Even the best-planned modernization project will not roll out perfectly. Require the vendor to train your staff in advance so they can handle issues as they arise and make changes to the system. In addition, make sure the vendor remains available to you after rollout without further costs.
Stage 2: The Design Process
Recommendation 2.1. Get user feedback from a broad range of stakeholders. Create ample opportunities throughout the design process for workers and employers to try out features of your system, and tell you what makes sense to them and what doesn’t. There are others who will use the system frequently and should be involved in testing as well. They include labor unions, legal aid organizations, community groups, and other social service agencies. Be sure to compensate members of the public for their time and transportation costs when you invite them to participate in focus groups or other consultations.
Pennsylvania and Massachusetts Listen to Stakeholders
Two states have now created mechanisms for stakeholder involvement and feedback in these projects. In 2017, Pennsylvania’s legislature created the “Benefit Modernization Advisory Committee” in the bill that provided funding for Pennsylvania’s new project. 53 The small committee consists of employer, labor, technologist, and claimant representatives, along with three agency staff members who will use the new system. By law, the committee:
– meets at least quarterly with project and agency leadership;
– receives monthly updates on the project;
– monitors the implementation and deployment of the project, providing feedback and both formal and informal recommendations; and
– submits a yearly report of the project’s process and the committee’s project recommendations to the legislature.
In 2020, Massachusetts’ legislature created a similar advisory council in the funding mechanism for upgrades to the modernized system that was deployed in 2013, and includes the council in the bid selection process. 54 The advisory council has similar responsibilities to Pennsylvania’s committee, but had broader stakeholder involvement, creates greater project transparency, and requires feedback from vulnerable communities:
[T]he advisory council shall solicit input on the criteria utilized for the selection of the bid evaluation from low-wage unemployed workers, people with disabilities who use assistive technology, community-based organizations that advocate for people with limited English proficiency, people of color, recipients of unemployment benefits and individuals with technological expertise in systems designed to maximize user accessibility and inclusiveness. 55
Recommendation 2.2. Allow plenty of “sandbox” time for agency staff. Create both structured and unstructured opportunities for staff to experiment with features of the system as it is being developed and recommend improvements.
Recommendation 2.3. Build in key features that help customers and reduce the burden on agency staff. While the precise design of your system should be guided by the needs of your customers and staff, there are a set of features that we recommend building into any system (and writing into your vendor contract).
2.3.1. Create a substantive, accessible claimant portal. Customers should be able to access a portal where they can perform all essential tasks: filing an initial claim, continuing claim, or appeal; checking on the status of a claim or appeal; completing fact-finding questionnaires; uploading documents and evidence, and reviewing correspondence.
2.3.2. Go for a professional look. Your website should present your agency as the professional operation that it is. The interface should look like the private sector websites customers are used to encountering; if it doesn’t, customers may be less likely to trust it, resulting in higher call center volume and more paper applications.
2.3.3. Make your website mobile-optimized. More people have mobile phones than desktop or laptop computers. 56 Low-wage workers and workers of color are particularly likely to rely on their phones for Internet access. 57 While more than 80 percent of white adults report owning a desktop or laptop, fewer than 60 percent of Black and Latinx adults do. 58 Some states had already been planning to optimize their sites for mobile access before the COVID-19 pandemic struck; Connecticut, for example, quickly made that change afterwards. There’s no need to build an app; just make sure your website reformats automatically for mobile devices.
2.3.4. Design a sensible password reset process. At the start of the pandemic, a major source of delay in filing unemployment claims was the overwhelming number of people getting locked out of their accounts. Technology exists to implement secure password reset protocols that do not require the mailing of a new password or other action by agency staff. Using those protocols saves time and frustration for everyone.
2.3.5. Make online and mobile systems available 24/7. In an era when online commerce and banking happens at all hours, workers expect similar access to the UI system. Allowing claims to be filed at any time also reduces pressure on the system when demand surges, by spreading out the claims.
2.3.6. Automatically save incomplete applications, and provide a warning before timing out. Too many systems kick customers out before they have completed their claim. Auto-save can prevent them from having to start all over again if they leave their applications open on their screens for too long while searching for information. Providing a warning before a customer is timed out is an added safeguard, but doesn’t substitute for auto-save.
2.3.7. Allow customers to choose email or texting as a communications method. It is unrealistic to expect that customers will constantly log back into the system to check for updates. Push information out through email or text, and allow customers to choose which method works best for them. Minimize the use of mailed documents; if it is required by law, make that clear to customers.
2.3.8. Permit customers to email in or upload documents from a computer or mobile device. The system should be designed to allow photographs and scans of documents to be easily submitted. This is how mobile banking works; UI should be no different.
2.3.9. Avoid automated decision-making. Technology can streamline many aspects of UI, but allowing computers to make decisions is inconsistent with the requirements of due process. As a safeguard against AI-driven error, several of the states we studied required a staff member’s involvement before a claim could be denied.
2.3.10. Use plain language and smart questioning. Use simple, non-bureaucratic language. It may help to gather information from customers through a series of straightforward questions; a vendor we interviewed suggested thinking of the claims process as an interview, rather than a form to fill out. For example, many people use the terms “laid off” and “fired” interchangeably, so posing a series of simple questions about why they lost their job could provide better information and thus reduce errors.
2.3.11. Translate the application and other online materials into Spanish and other commonly spoken languages. Civil rights laws require translation of materials to ensure equal access. Translating materials also can save states money, by reducing demand for interpretive services at call centers. Washington provides an entirely Spanish-language version of its website and application, for example.
2.3.12. Minimize the paperwork burdens associated with work search. If your state directs claimants to register for worksearch on a state government website to search for a job, look for a way to integrate the two systems. Provide consistent guidance about what is required and avoid unduly burdening claimants. You want people spending their time looking for work, not assembling extensive documentation that your system lacks the capacity to review. Rely instead on the Reemployment Services and Eligibility Assessment (RESEA) program for verification, as needed.
2.3.13. Coordinate technology with other state agencies. If your state plans to move to a single sign-on system, make sure the password mechanism you create can be easily integrated into that new system. If your state handles appeals in a centralized manner, make sure UI claimants can tap into information about their appeals through the UI portal.
2.3.14. Provide a view-only option for non-UI staff. Workers often go to career centers and other public agencies seeking help with UI. Constituent services representatives in legislators’ offices also receive UI inquiries. Allowing public employees to log in and see what’s happening with a claim—but not to make changes that interfere with UI processing—is a best practice that Pennsylvania has adopted.
Designing a Modern Website
Websites today include a variety of features customers are used to seeing, all of which would enhance the functioning of a UI website. They include:
– Chatbots (to answer frequently asked questions)
– Live chat (if you have the staff capacity)
– Calendaring
– Drop-down menus
– Progress bars (to track the steps in an application)
– Hover text (to pull up the definition of a term, for example)
– Select-all options (to file a batch appeal, for example)
– Expansive character limits for text fields
– Dark/dim versions
Stage 3: Implementation
Recommendation 3.1. Don’t go live during the busy season. It’s best to avoid the November–March period, so you are not struggling to adjust to a new system at a time when seasonal claims surge.
Recommendation 3.2. Consider rolling out pieces of the new system in stages. Going live with just one element, like the appeals process or Disaster Unemployment Assistance (DUA) claims, gives you a chance to see how the new system is working and make refinements, before everyone has to use it. Idaho and Washington have taken this approach.
Recommendation 3.3. Train and support your staff before going live, and on an ongoing basis. Modernization means making big changes, not only to your computer systems but likely to your business process as well. Your staff, including those on the front lines in call centers and career centers, should feel well prepared for those changes and supported throughout the transition. Maine and Wisconsin reported that call center locations that received the most practice with the new system were the best prepared for the rollout. Minnesota also provided guidance to its staff on how to respond in an empathetic manner to claimants struggling with their new system.
Recommendation 3.4. Staff up your call centers and deploy staff to career centers before going live. Call center usage spikes dramatically when a new system is rolled out, as does the number of people seeking assistance with UI at career centers. Minnesota put additional staff on the phones and Maine placed staff at career centers before going live with the new system, to help manage the demand. If you don’t anticipate being able to answer calls without substantial delays, add a call-back option, as Washington did.
Recommendation 3.5. Have a robust community engagement plan. Your rollout shouldn’t catch the community by surprise. Reach out in advance to stakeholders, educate them about the new system, and ask them to help spread the word. Tap into the same group you asked for help in testing your system before rollout (see Recommendation 2.1, above).
Recommendation 3.6. Expect lots of bugs and have a clear process in place to fix them. If you run into major problems, hold the claims, as Maine did, rather than adjudicating them, as Michigan did. Putting claims on hold during a system malfunction prevents a lot of hardship for workers, and unnecessary workload for staff who process appeals and reversals.
Recommendation 3.7. Provide for ongoing feedback from your customers and front-line staff. Washington, for example, used customer surveys to inform its decisions about business process changes. New Mexico did a particularly thorough job with the usability surveys it sent to claimants and employers. Be sure to dig deeper than just asking customers for their overall level of satisfaction with the experience. Provide the opportunity for feedback at every stage, not just at the end of a transaction, by which point customers may have forgotten exactly what language they found confusing or where they got stuck. Create a mechanism for staff to provide suggestions for improvements as well, and follow up in a timely manner.
Initial Lessons from States
In the early stages of the research for this report, the authors communicated with UI agency officials from a diverse group of about twenty states, of which over a dozen agreed to be interviewed to share the lessons learned from their experience. 59 Specifically, the state officials were asked to share features of their modernized systems they are most proud of, and what they would do differently if they were just starting the process. Most of the states interviewed had completed the transition to modernized benefits processing, and the remaining states were fairly far along in their development.
Highlights from these initial interviews are presented below, structured to follow the three stages of a modernization process: planning, design, and implementation.
1. Planning Lessons from State Interviews
- Funding is key. As one state official emphatically put it, “FUNDING these projects is the greatest obstacle of all!” Many states relied primarily on the one-time large infusion of flexible “Reed Act” funding resulting from the 2009 Recovery Act, while others (e.g., Colorado, Utah, and Washington) accessed dedicated state funding or special tax assessments to support their programs. Some agencies have sought out a specific funding mechanism. For example, Pennsylvania currently has an employee-side tax, and at the UI agency’s request, the legislature directed some of the revenue from that tax to fund its current modernization effort.
- State consortia can be challenging to form, but useful. Most states reported serious challenges forming UI IT consortia due to restrictions on federal consortia funding for such consortia, changing priorities of state political leaders, and other factors. As a result, many UI IT modernization efforts were significantly delayed or altogether abandoned in some cases. However, some states have valued and benefited from the formation of consortia to share UI IT infrastructure, expertise, and costs (including the Mississippi/Maine consortium and the Idaho/Vermont/North Dakota consortium).
- Strong teams representing all functions should be involved in the planning process. Several states (e.g., Utah, Washington, Vermont) emphasized the importance of assembling strong teams that represent all the major functions of the agency to be involved in the planning, design, and implementation processes, and develop the expertise in the new systems. The team members should also play a central role providing the training of the system to front-line staff, both before the system is launched and on a continuous basis thereafter, while also regularly engaging the staff to solicit feedback on the system.
2. Design Lessons from State Interviews
- New internal staffing structures and business practices may be needed. Some states (e.g., Minnesota, New Mexico) emphasized the importance of creating internal staffing structures to more efficiently and effectively process and adjudicate UI claims. These “unified integrated” systems break down traditional agency staffing silos (e.g., creating separate units that handle initial UI claims, adjudications, and overpayments) and instead apply UI staff where they are most needed (e.g., responding by phone to resolve more complicated UI adjudication issues).
- Investments in internal IT systems and expertise can be valuable. Several of the states interviewed (e.g., Iowa, Utah, Minnesota, Washington) have worked with commercial off- the- shelf technologies, developed open-source technologies, or invested significantly to develop internal IT staffing and expertise in order to rely less on established vendors and provide greater flexibility to manage their UI IT systems.
- Data conversion should begin as early as possible. UI agency officials also recommended that states taking on new UI IT modernization efforts begin the process of data conversion from their legacy systems as early as possible and conduct extensive testing of the converted data.
- Too little usability testing was done. With some exceptions, such as usability studies in New Mexico, the interviews with UI officials clarified that there has been limited feedback solicited directly from workers or worker advocates to evaluate the usability of the new UI IT systems for all workers, but especially for the large proportion of unemployed workers whose first language is not English or who have limited computer literacy. Where outreach has been conducted, it has often taken place at the end of the planning and development process, when the opportunity to inform key decisions is more limited.
3. Implementation Lessons from State Interviews
- Rolling out new systems is challenging and can take a long time. As one state official put it, UI IT modernization is a “roller coaster” ride, fraught with funding, vendor, staffing, and automation challenges. Many of the states interviewed started the process in the early 2000s, and only recently launched their automated benefits systems. Several states also experienced challenging launches of their new systems, which required major adjustments. Accordingly, most UI agency officials strongly advised that states provide for extensive lead time and testing of the technology (e.g., Wyoming tested 1,800 cases with staff before they were put in a live environment), organize the staff to ensure that “all hands are on deck” while rolling out the new system, and perhaps most importantly, wait to launch the new system during low-volume periods (e.g., during the summer months when fewer people are applying for benefits).
- Modernization can improve UI access. Uniformly, state UI officials emphasized the significant role that new automated and IT reforms have played in improving communication with UI claimants, with twenty-four-hour access in many cases to a range of online services and direct access to information on the history and status of the individual’s claim. Indeed, the rate of online initial and continued claims filing has increased in most states that modernized. Some states (e.g., Colorado and Mississippi) have provided mobile-ready platforms, which helps workers in more rural communities that do not have reliable broadband access. New Mexico has also developed “personas” to help the platform accommodate particular groups of workers seeking to navigate the online claims systems by anticipating their needs and providing them with “pop ups” and other features that provide clarifying information. Several states (e.g., New Mexico and Mississippi) are also providing or developing “single service sign-on” systems, which allow workers to readily access not just UI benefits, but also job service and reemployment services.
- UI access “pain points” remain. Due to administrative funding limitations and other pressures, several states indicated that they are reducing access to phone-claims services, which have been critical to many workers who have more challenging claims or have experienced barriers to navigating online systems. Many states also reported that the new automated systems have generated a greater number of eligibility, disqualification, and overpayment issues, which often produce multiple unfavorable determinations that the claimant is required to respond to separately. Where possible, certain states have taken steps to intervene in a timely fashion in these cases, and at least one state (Vermont) has adopted a policy to merge these multiple determinations into a single notice and determination.
Case Study Findings
This section presents findings from detailed case studies of UI modernization in Maine, Minnesota, and Washington. All three states had completed UI IT modernization projects at the time of data collection. Their UI IT projects were generally regarded as successful in terms of current program outcomes or public response—though no modernization effort was without its unique challenges. They also represent states with different populations, economies, and labor forces, as shown in Table 1.
Maine, Minnesota, and Washington took different approaches to UI IT modernization. Minnesota was one of the earliest states to modernize their benefits system, which went live in 2007. Though the online platform—the Minnesota Unemployment Insurance (UI) Program—was created by private vendors BearingPoint and Deloitte, the code is the property of the state. Minnesota’s Department of Employment and Economic Development (DEED) maintains the code and shares it freely with other state agencies that request it. While building their online system, Minnesota’s UI agency was also engaged in updating their business practices. They accomplished this by reviewing call center management, scripts, and training, among other things.
Washington interviewed several technology vendors for their project. After conducting extensive market research, Washington’s Employment Security Department (ESD) ultimately negotiated a contract with Fast Enterprises that obligated the vendor to provide ongoing system support and maintenance for its commercial off-the-shelf (COTS) product. Though agency employees reported that they have a lot of oversight over the system, it is a proprietary system owned by Fast Enterprises. Unlike Minnesota, Washington’s modernization project, which was rolled out in 2017, included no operational change management.
Maine is the only case study state that is part of a consortium, meaning that it shares system and maintenance expenses with two other states (Mississippi and Rhode Island). The Maine–Mississippi–Rhode Island consortium—ReEmployUSA—uses software developed by Tata Consulting Services (TCS), a subsidiary of the multinational conglomerate Tata Group. Maine’s personalized UI system is called ReEmployME and was rolled out in 2017. Maine made reviewing agency business processes a feature of its modernization project. The consortium owns the supporting code, though TCS provides ongoing application support.
The case studies presented in this section provide a description of our research methods as well as our findings on each state’s UI IT project and its successes and challenges, with attention given to how modernization has impacted claimants.
Minnesota’s UI agency (the Department of Employment and Economic Development, or DEED) takes pride in its business philosophy and quality of services. It was the first state to modernize both its UI tax collection and benefit payment systems. Before the COVID crisis, Minnesota was one of the few states that regularly met the federal trust fund solvency standard. Furthermore, Minnesota does well on official performance measurements set by the Department of Labor—in 2018, Minnesota’s recipiency rate was ranked fifth highest and their average weekly benefit amount ($462.61) was ranked third highest among all state UI programs. Both measurements improved following modernization. Minnesota has been a national leader, developing and maintaining IT and claims processing systems that have been shared widely with other states. They are one of the top states in major elements of administrative performance, such as first-payment timeliness, overpayments, nonmonetary time lapse, and nonmonetary quality, a fact they are proud of. The feedback provided in focus groups by worker advocates, summarized below, generated helpful recommendations to further improve upon the Minnesota system.
Planning in Minnesota Minnesota began planning its Unemployment Insurance Technology Initiative Project (UITIP) in 2003. Prior to UITIP, unemployment insurance was a paper-based process. During interviews, agency officials indicated that evaluating business practices and eliminating system complexities were compatible project goals.
The hallmark of the modernization project was a triage system to better manage call center operations. Minnesota’s business reorganization was intended to ensure that the majority of claims were able to be efficiently processed with minimal staff time, leaving staff to spend time on claims with more difficult issues. Under the triage system, call center staff are trained to handle common issues while transferring any exceptional scenarios to a smaller group of subject matter experts (SMEs). Project leaders observed call center staff and analyzed existing datasets to identify successful practices that could be built into the online system. These operational adjustments helped reduce work backlogs and call center wait times. Even during the Great Recession, wait times were less than three minutes, on average.
Minnesota had one of the quickest modernization processes and was able to roll out its system after sixteen months. In retrospect, the agency believed that having the project run by UI experts, rather than technology experts, was critical to its success.
The code that powers Minnesota’s online UI system was developed by third-party vendors. It was purchased by the state so that they would have full intellectual control to make any necessary or desired modifications.
Stakeholder Feedback on Planning in Minnesota
- Stakeholders were not really consulted during the planning stages of the project. While
- Minnesota has an Unemployment Insurance Advisory Council, it had grown to over forty members around the time of modernization and was not engaged with the modernization project.
Design in Minnesota Minnesota’s online application was designed with common scenarios in mind. Rules-based routing populates relevant questionnaires for applicants, expediting fact-finding stages. The system automatically detects and flags issues that require further fact-finding and generates relevant questionnaires for the applicant. The design approach from DEED was to “meet claimants where they are” rather than having to chase them down for information later. DEED reviewed prior claims data to determine the decision trees and drop-down options in the application and questionnaires. For weekly work search questions, DEED consulted with an outside academic to identify which questions actually cut to the heart of what it means to “search for work.”
The online self-service options available to claimants were completely new, since no online access was available prior to the project. At the time of our interview, the online system had a forty-five-minute “time-out” for initial applications, with limited autosave functionality. Claimants and employers, or employer representatives, are able to file claims as well as appeals online. They can also pick their hearing date and time, request interpretation, add witnesses, and add representatives. However, while prior to the UITIP, parties could file a single appeal that covered several issues, the new online appeals system requires a separate appeal for every issue. The system does not permit a party to select multiple issues to be addressed in a single appeal. While DEED still attempts to “batch” many of these appeals on the back end, that process is not automated and there is no way for parties to mark multiple appeals as “related” to ensure batching.
All credibility determinations are made by DEED staff, and no overpayment determinations are made without an actual person involved. The backend functionality “pushes” flagged issues to adjudicators, with the oldest issue flagged as top priority, to help streamline claim processing. Still, the system primarily relies on the ability of claimants to answer detailed questionnaires, which were written at a high literacy level.
The two-stage design project included user testing. During the first phase, the agency assembled focus groups composed of employer customers and third-party administrators. These user groups were shown prototypes of the self-service system and asked for feedback. The second phase invited employers, third-party administrators (TPAs), and current UI applicants to participate in focus group discussions. Feedback from the focus groups helped the agency make improvements to website navigation and wording prior to the official launch. 60
Stakeholder Feedback on Design in Minnesota
- Hours were limited. Focus group participants were frustrated by the website’s limited service hours. While most Internet users expect 24/7 access to online services, Minnesota’s online UI system is only available from 6:00 AM to 6:00 PM, Monday through Friday. Claimants explained that they found this timeframe inconvenient, especially when they were also actively looking for work.
- Navigation was difficult. Workers also noted that navigating the website was challenging, given the text-heavy design of many of the webpages. The online application generated long questionnaires that required close and careful reading, often resulting in claimants “timing out” of the system and losing their progress. Most participants revealed that they resorted to drafting their answers in a word-processing application to copy and paste into the application.
- There was no advocate access point. Legal aid attorneys reported that the system was designed without an online access point for representatives in which they could file appeals or review documents to help claimants understand agency communications (unlike employer accounts, which have an access point for TPAs). Having an access point into the system would legitimize their role as claimant representatives, one attorney said, and make the new system more transparent for claimants and advocates alike.
Implementation in Minnesota
UITIP went live in 2007, and despite its careful planning, Minnesota immediately experienced several issues with the new system. First, the website was rolled out prior to the 2008 recession, and during the winter months, when many seasonal workers were filing. The agency was down to just thirty full-time employees at that time, which was inadequate for handling the volume of applications received. The call center was inundated with panicked questions about the online application, many of which were users who had forgotten their password or been locked out of their account. Additionally, staff were also struggling to adjust to the new triage system. Some call center staff still treated UI as a “case system” in which every call warranted close, personal examination. Still others treated phone assignments as punishment.
Minnesota’s UI IT modernization project was delivered on time and on budget, though it took time and training for the new program to achieve its high performance metrics post-modernization. When we interviewed DEED staff, approximately 90 percent of UI applications were received online. However, agency leadership affirmed that the core of their UI operations would always be the call center. DEED operates the only dedicated call center training room within the department. New call center workers receive empathy training and attend emotional intelligence seminars to improve customer service prior to taking calls. Training also prepares staff to handle technological questions, as they use the administrative version of the online application for data entry. Call center workers can even see what information claimants have input into the system to assist them with their application. Moreover, the state thinks of their triage system as a model for other states. Along with computer modernization, the state put in a new business process in which calls are first answered by generalists who can answer basic questions, and then are routed to different staff members who are specialists for more difficult questions about benefits and legal issues. DEED staff see this as an adoption of call center models used in the private sector, and see themselves as being in the business of providing service.
However, the original build tied the frontend presentation layer—the user interface—closely to the backend business logic, which prevents the agency from making significant changes to what the customer sees or even offering different language versions of the online application, without changing the code for the entire system. It also prevents changes that would improve the visual appeal of the application and self-service options. DEED is planning to separate the presentation layer so that it can make such changes in the future. Since go-live, DEED has created Spanish, Hmong, and Somali language videos to help claimants through the filing process, but acknowledges that these resources are not a satisfactory replacement for supporting multiple other-language versions of the online application.
Stakeholder Feedback on Implementation in Minnesota
- System is still paper-based. Focus group participants were surprised by the amount of mailed paperwork they still received given the introduction of a fully online option. Even though the new system allows applicants to file entirely online, questionnaires can generate issues that require further information from applications that must be mailed or faxed to the agency. Furthermore, the system does not allow claimants to attach supporting documents to their online application or to send them via email.
- Password reset problems occurred. One of the inevitable consequences of most UI modernization projects—a spike in technical support questions related to resetting passwords—continues to be handled by DEED entirely via snail mail. Claimants that get locked out of their online accounts must call DEED to request a password reset. During focus groups, participants who experienced this issue explained that they lost a week of potential benefits while waiting for their new password to arrive by mail.
- Workers were generally pleased with the website access and call center help. Participants acknowledged that while Minnesota’s online system replicates certain pre-modernization access barriers, the website is convenient—especially for younger workers. One participant explained that he finished his online application in less than ten minutes. He said that most of the questions were easy to understand, even if they weren’t designed to address his particular situation. Focus group participants generally were pleased with DEED’s call center. They reported that call times could occasionally be long, but most of the staff were considerate and empathetic.
- Triage system was imperfect. Though call center support was expedient, it was not always helpful. A Minneapolis participant explained that it was difficult to get a “straight answer” to a “straightforward question” about his eligibility. Other participants echoed this sentiment, admitting that certain call center staff didn’t have the authority to answer any complex inquiries—an actual feature of the triage system. Another Minneapolis participant suggested that call center workers weren’t “worried about giving me the wrong answer,” but “worried about giving me an answer that I’m going to use later [during] an appeal.”
- Claimants experienced increased disqualification and appeal problems. Legal aid attorneys raised concerns about the new appeals system and the requirement that claimants file separate appeals for each individual issue. The modernization project resulted in an increase in determinations and disqualifications for their clients, especially concerning wage reporting and continuing eligibility requirements. Attorneys reported that clients often missed appealing an issue and did not understand separate appeals were necessary. The new online system also failed to clearly demarcate at what point an appeal was considered “filed,” and many claimants missed going to the final screen and therefore never timely filed their appeals.
- Department was responsive to identified problems. Legal services attorneys reported that technical issues they identified in the system after it went live did lead to later positive changes. For example, a prominent button that would dismiss existing appeals was fixed to prevent claimants from clicking it in error, and a notification was added to alert applicants about the thirty-minute time-out window.
- System was not built for all workers. Legal aid attorneys explained that they represented some of the most vulnerable worker populations, and that their clients experienced language and technological barriers, as well as complex appeals, in the new system. Post-modernization, it became more common to address a client’s multiple issues across multiple hearings (in the past, all issues could usually be resolved in one hearing).
With funding provided by the state, Washington’s UI agency (the Employment Security Department, or ESD) has made substantial improvements to a system that, like other states, struggled when it was first launched in 2017. Agency officials actively engaged and trained ESD staff at all levels to continuously upgrade the new system, and collected extensive data to closely monitor its performance. Washington also offers a positive range of user self-service tools to help workers navigate the system, and a website with more design features than the others we studied. Like Minnesota, Washington consistently does well on key measures of performance—in 2018, Washington’s recipiency rate was ranked thirteenth highest among all the states in 2018, and their average weekly benefit ($467 in 2018) was ranked fourth highest among all the state UI programs. It also maintained a trust fund balance that exceeded the federal solvency standard before the COVID-19 pandemic hit. Washington’s worker advocates have also been exceptionally effective, and together with the workers who participated in the project’s focus groups, they identified key priorities to ensure that the state’s most vulnerable workers can readily access UI benefits.
Washington’s performance, as compared to other states, has been impacted by modernization. The agency experienced a dip in the quality of nonmonetary determinations after modernization, from above the national standard of 75 percent quality to below after modernization. The state agency attributed the problem to an overreliance on the computerized tools and insufficient proactive fact finding, something they are training on and reported seeing some better results beyond the study period. As will be described below, most states, including Washington, experienced an increase in the denial rate after modernization and Washington went from the states least likely to issue a denial to an average state on denial rates. In the agency’s opinion this is because claims were more likely to be accurately decided at first, rather than having to be reworked and redone. This aside, modernization has coincided with tighter monitoring of work-search activities which are the underlying source of this increase in denials.
Planning in Washington
In 2010, ESD requested state funds to help leverage existing federal funds for modernization. The legacy mainframe system, called GUIDE, required thousands of patches and several ancillary systems to manage added agency functions, like the Reemployment Trade Adjustment Assistance (RTAA) program. It was a difficult request to make during an economic recession, but the state legislature was responsive and made a significant investment in improving the system. To its credit, the legislature has continued to invest state dollars in the administration and IT needs of the program.
ESD issued a request for proposals aimed at procuring a commercial off-the-shelf (COTS) product to support their UI IT project. After interviewing six vendors, ESD and Washington’s Attorney General signed a contract with Fast Enterprises. Agency officials were satisfied with terms of the contract. They reported that they enlisted several experts from the many Washington-based technology industries to help with gap analysis, feasibility study, and training prior to contract negotiations. Consequently, ESD had full control over the go-live date.
The contract also obligated Fast Enterprises to provide system maintenance and security. Fast Enterprises sent their own staff to support ESD during and after the unemployment tax and benefits (UTAB) modernization project. ESD reported being pleased with the support provided by the Fast Enterprises staff, and viewed their involvement positively. Unsurprisingly, our interviews at ESD included several Fast Enterprises employees now working on the twelfth version of the system. ESD found it difficult to keep high quality developers in house and up to speed with FAST, especially given the private sector competition for programmers in the state.
The Fast Enterprises core product had previously been implemented in Michigan. To learn and improve upon Michigan’s experience, ESD project members spent time speaking with Michigan officials about pain points and lessons learned.
As part of the project planning team, ESD pulled staff from all of its units to make sure it had the right people at the table, especially those who would be on the front line of the system implementation. One takeaway from ESD was that “if you do not feel the pain of missing those workers in your daily operation of the system, you did not choose the right people for the project.” ESD also learned that it was best to only make major decisions with a large team while authorizing some project team members to make smaller decisions. ESD also engaged some users in testing of the system through its local workforce centers toward the end of the project, but found that at that point it was too late to make any real changes to the product prior to implementation.
Stakeholder Feedback on Planning in Washington
As in Minnesota, worker advocates in Washington were mostly unaware of the benefit modernization project until implementation. Employers were included in the project only through some initial testing, when ESD brought sixty employers in for beta testing to help identify easy fixes to the system.
Design in Washington
Fast Enterprises already had a core product, so it was able to code the core architecture changes for UTAB quickly. However, like any commercial off-the-shelf product, this meant that the core design of the system was outside of Washington’s control and there was little leeway to make customizations.
One of the highlights of the UTAB design is the range of user self-service tools, which allow claimants more advanced access than the mainframe. For example, prior to UTAB, if a claimant missed a weekly certification, they could only reopen the claim by speaking with ESD staff on the phone. With UTAB, claimants can now reopen their claims online and are able to file for up to four weeks of backdating. Additional highlights include:
- Claimants can send messages through the online portal to ESD, and can attach documentation to be added to their claim.
- Claimants can file appeals through the system, and the system will pre-fill information based on previous data entries.
- All determinations are available to view online.
At the time of our interviews, all elements of UTAB were mobile responsive except for the initial claim application. The initial application has a fifteen minute auto logout, and no autosave feature, although claimants can save their application at the end of each page. Unlike most other systems, the initial application does not ask for significant separation information. Instead, after the application is filed, the system may flag a separation issue and then add a questionnaire to the eServices portal.
One major design change with the initial system was a change to the notices of determination. Prior to UTAB, all determinations were free form, and while there were some templates, adjudicators had full control over the content. Adjudicators commented that the new system “took a lot of thinking” out of the determinations and instead put in place a check-box system. Adjudicator indicated that independent analysis of facts felt more difficult and were limited in what would appear on the determination because they could not write in a free form manner. A state court later ruled these determinations insufficient to meet due process requirements, and ESD updated its system, although adjudicators still lack much of the flexibility they had prior to UTAB.
However, after reviewing the implementation of the FAST system in Michigan, Washington was extra careful about integrating any automated decision-making into its system. For fraud, every decision is evaluated by a human representative. While certain types of eligibility issues can “go presumptive” based on the burden of proof if one party does not respond, the only automated decision-making occurs with weekly job search reporting. This may explain the substantial increase in disqualifications for worksearch in the state, and the denial rate for these reasons more than doubled after modernization, from 2 percent of all weeks claimed to 5 percent of all weeks claimed.
Stakeholder Feedback on Design in Washington
- Many of the Seattle workers explained that it was helpful to see all their information listed out in one place, a record of ESD communications, and the amount of benefits money still available to them.
- Workers found it helpful to be able to appeal in the same system they saw their determinations.Others struggled to navigate eServices:
- Many workers struggled with the system timeouts. For appeals, workers said when they timed out they lost everything they had written. For weekly claims, they often timed out while trying to answer the weekly work search questions, which were quite long and cumbersome. If they did not affirmatively hit save somewhere in the claim, they would lose everything they had entered.
- Workers reported that the eServices portal was not intuitive and many encountered problems trying to find their determinations or other important information.
- Workers noted that sometimes there were multiple claim years available on eServices, but that it was difficult to navigate between them and find the current information.
- Claimant advocates had no access to eServices. As in Minnesota, claimant advocates were frustrated by their lack of access to the system, which made it difficult for them to see eligibility decisions or help claimants navigate the problems identified above.
- ESD determinations were unclear. The change in determination structure had a major impact on workers. Workers reported receiving one line decisions telling them they had been disqualified for benefits, but without any context or description of the facts. Some stated they did not even know what issue was involved in their disqualification. Advocates reported similar problems and that administrative law judges could not rule on the determinations in many of these cases due to the lack of information.
- Out of eleven focus group participants in Sunnyside, Washington, only one was able to use eServices. The migrant workers primarily used the telephone services to contact ESD. Most did not have access to a computer and could not navigate eServices on their phones. A few who tried could not manage to finish the initial application online and had to call. Despite this, workers reported feeling continuously pressured by ESD to use eServices.
Implementation in Washington
Project development was delayed and system changes were creating some frustrations and concerns about job security. Because the agency was not satisfied with the testing and system readiness, the original launch date had to be reset multiple times. Washington’s UI website was ultimately launched in January 2017.
Like Minnesota, Washington’s go-live came during a period of higher unemployment claims related to seasonal industries. Agency staff present at the time recall severe performance issues related to the launch, reflected in Washington’s official performance statistics from 2017. That year, the state was only able to deliver initial payments to 72.6 percent of eligible claimants within twenty-one days—short of the 87 percent federal standard. In 2018, the state’s performance had improved to 81.8 percent.
One of the primary issues during the launch was system overload. Call volume spiked dramatically, resulting in only about a 20 percent chance for connection. Panicked claimants experienced busy signals, dropped calls, and system error messages due to higher-than-expected web traffic. 61 Although the rollout was marred by public complaints, ESD and Fast Enterprises staff recalled how the launch helped them redouble their efforts to make the project a success. The agency hired thirty more agents to answer the phones. Even with minimal training, they were able to meet agency benchmarks for call handling times 93 percent of the time and cut wait times by 67 percent. By 2018, the call center connection rate had surged to 99 percent. Washington stood out as a state that collected a large amount of data about the functioning of its modernized systems, and used that data to make improvements.
Since go-live, ESD has expanded telephone hours to increase access and has done empathy training with all of its staff. ESD has also partnered with local workforce centers and twenty-three of these centers across the state have ESD staff placed on site. ESD explained that many of the (primarily migrant) agricultural workers in the center of the state had limited computer skills and relied on workforce staff for assistance with their UI claims.
A 2019 claimant survey that garnered over 16,000 responses found that 85 percent of claimants were using eServices and 62 percent were using the call center. Using the analytical tools within eServices, staff analyzed whether they could safely reduce support for call center operations; specifically, their interactive voice response (IVR) system (an automated routing system for filing weekly claims by telephone). With approximately 33 percent of claimants using the IVR system to file weekly claims, ESD felt that transitioning away from these services would negatively affect claimants and the state’s recipiency rate.
One other major learning experience from ESD implementation was around staff training on the new system. Fast Enterprises had done limited “just-in-time” training prior to the original go-live date. Delaying the launch twice left staff underprepared for the actual launch. Furthermore, the system training environment didn’t use actual data or bring together all elements of the system in a holistic way. During our interviews, ESD staff recognized that they had not prioritized training during contract negotiations. As a result, ESD had to significantly increase its own staff training and engagement. ESD staff recommend that states embed training staff in the development phases of the project, host expanded morning Q&A sessions during implementation, provide space for training in live environments, and plan for retraining three to six months after implementation.
While state did have a usability vendor, the agency reported not having enough time to implement many of the suggestions. ESD made efforts to gather feedback on its system after implementation. A feedback form embedded within the eServices portal has led to further operational tweaks and a dramatic reduction in claimant complaints.
Stakeholder Feedback on Implementation in Washington
- Claimants could not get through on the phone. Workers confirmed that after go-live the phone systems were overwhelmed. Many experienced long wait times or could not get through. Advocates reported that their clients who lacked access to computers, were not technologically literate, or had language barriers were unable to file or navigate their unemployment cases when they could not get through on the phones. However, all focus group participants said they still had to rely on the call center even with the addition of eServices. For most participants, the call center was their primary means of securing UI benefits and a critical resource for when they had questions or issues.
- The Office of Administrative Hearings (OAH) did not modernize. In Washington, all administrative appeals are centralized in OAH, which is separate from ESD. OAH did not have a modernization project, so although customers received electronic notices from ESD, they only received paper notices from OAH. This led to many workers missing their hearings because they did not know to look for mailed notices. It also caused issues on the back end. Advocates reported much longer wait times for benefits to be released after winning a hearing with OAH and that instead of having one hearing scheduled to cover multiple appeals for a single client, there were multiple hearings scheduled. ESD plans on improving the process so they are more aligned with OAH in the future, and electronic delivery is being turned on as of 2020.
- Migrant workers lacked trust in the system. Many of the Sunnyside focus group participants’ first or only language was Spanish. While noting that eservices were available in Spanish, the workers overwhelmingly preferred filing using the phone and always called ESD with their questions. However, their interactions with ESD representatives often made them feel like ESD was looking for ways to disqualify them or accuse them of fraud. This was especially true for worksearch. Given the limited number of warehouses in their town, many workers had no way to report three work searches per week. They were also afraid that if they applied to other, less well-paid work, they would have to accept an offer and miss their opportunity to return to their warehouse job when recalled.
Maine’s Bureau of Unemployment Compensation completed its benefits modernization project in 2017. The agency is part of the ReEmployUSA consortium, which uses software developed by Tata Consulting Services (TCS). Maine’s UI benefits website—ReEmployME—had a difficult launch that received significant public attention. However, recent changes in leadership have led to a renewed openness and focus on enhancing access to services and improving the system to be more user-friendly, as reflected in the state’s participation as a case study in this project. For example, to expand access to the system, the new leadership prioritized increasing the staff in the state’s local one-stop career centers to provide increased direct, in-person services to workers. They also reengaged with key stakeholder groups representing workers, reestablishing crucial lines of communication. The state’s UI program remains above the national average in its recipiency rate (ranked twenty-first among all states in 2018) and is one of the only states that saw benefit denial rates drop after modernization. In 2019, the state’s average weekly benefit ($351) replaced 52 percent of the state’s average weekly wage, which was above the national average of 45 percent. The worker advocates and claimants who participated in the focus groups raised several access concerns, which are summarized below, while applauding the direct service they received from the agency when they were reached by phone.
Table 6 reveals other positive impacts following benefits modernization—notably, the state’s overpayment rate went from one of the highest in the nation (seventeenth) prior to modernization to one of the lowest (forty-fourth). Despite these successes, Maine has struggled to improve its rankings and meet national performance standards post-modernization.
Planning in Maine After Maine moved its system off the mainframe in 2000, the state began planning for modernization of its remaining legacy design in 2013. The Bureau of Unemployment Compensation decided to proceed with the consortium model, noting that it would take less staff and funding resources, and appreciating the shared governance and systemwide updates. Maine joined Mississippi’s ReEmployUSA consortium for a system designed by TCS.
The original ReEmployUSA consortium states included Mississippi, Maine, and Rhode Island; Connecticut and Oklahoma will join the consortium but are still in project development. 62 Mississippi went live with its system in early 2017 and led the product development as the original user, having received a grant several years earlier to convert into an online rules based system. The U.S. Department of Labor awarded the consortium a $90 million development grant to help Maine and Rhode Island integrate Mississippi’s UI IT system framework under Mississippi’s leadership. 63
The ReEmployUSA consortium is cost-effective because it leverages existing technology and eliminates the need for state agencies to design their online platform from scratch. Maine was also able to operate through Mississippi’s procurement process, and all steps were reviewed by legal counsel for both states. The consortium then developed a Memorandum of Understanding for the development stage of the project, and the consortium itself owns the code for the project. Mississippi also sent some of its UI staff to Maine to assist with the project and provide feedback on the Mississippi experience.
Stakeholder Feedback on Planning in Maine
Similar to the other projects in the case study, no nongovernmental stakeholders were included in planning of the system before it was launched in 2017.
Design in Maine The consortium model comes with certain limits on design. Similar to a commercial off-the-shelf (COTS) system, many of the core components were predetermined based on the Mississippi system, otherwise considered the “core product.” Maine did perform a gap analysis of Maine and Mississippi law to determine any changes that were necessary to conform to Maine’s UI law. Maine found that there was an 85 percent code match for benefits and appeals, but the gap analysis took more time than was originally expected.
In large part because Maine’s core system was developed in the late 2000s as part of the Mississippi project, the presentation layer does not reflect modern design and dynamism of Washington’s system, which went live around the same time. Neither the initial application nor the weekly certification systems are mobile-responsive. When the system went live it also had a five-minute timeout for both types of filing, and only some autosave features for the weekly certifications. However, the weekly certifications are designed in an easy-to-understand way for wage reporting, which is a frequent challenge for many claimants to understand clearly.
The portal provides a way for parties to view determinations and appeal online. The portal also includes an internal messaging system. While there is no way in the portal to upload documentation, UI claim examiners often provide their email address so that claimants can send them documents.
There is no automated decision-making in Maine’s system design. Maine’s unemployment law requires scheduled fact-finding by telephone on potentially disqualifying issues. Fact-finding notices are still mailed and provide parties with a scheduled time for the interview.
Stakeholder Feedback on Design in Maine
- Smartphone usability. While Maine provides for smartphone access, which is a positive feature of the system, the focus groups reported that navigating ReEmployME on smartphones was difficult, especially when trying to access different tabs or parts of the online portal.
- Many reported that the website often crashed or would be unavailable.
- Claimants could not go back and change answers easily if they made a mistake, because the system did not include a “back” button. Instead, they would have to log out and back in to make changes if they accidentally hit the wrong radio button.
- Claimants had trouble using the drop-down menus, and did not understand why there was not an “other” with an opportunity to explain in many sections, given the broad scope of workers’ situations.
- Several claimants commented that the dependents section constantly froze and kicked them out of the application.
- Claimants consistently timed out of the application if they received a phone call or text message, or had to go look something up.
- The “old look” design of the system. Many claimants complained about the “old look” of ReEmployME, with some referring to it as a “Windows 95,” which made the system feel unwelcoming to them.
- Workers did not receive notifications. No workers reported receiving electronic alerts when new documents were added to their portal or new information was available. Many expressed concern that they would miss important documents or deadlines. Claimants newer to unemployment also did not understand why the system did not send them a weekly prompt to file their certification.
- Workers were confused by extra paperwork. Claimants did not understand why they still received extra paperwork many weeks after the date of action. Some reported it felt like they were often asked to fill out paper forms with information they had already filled out online. Other times, they only received certain vital information by mail, like fact-finding appointments or hearings, when they felt they should be able to see those notices online. One problematic example was a union worker who took out-of-state gigs, and would receive a return-to-work form in the mail to his home address that required action on his part, rather than electronically where he could actually see it while he was away.
- Hearing files were still sent by mail and not accessible online. Similarly, claimants only received the file of paperwork for their appeal hearings by mail and had no way to access the information online.
Implementation in Maine Maine went live with its ReEmployME benefits system in early December 2017. Similar to many other states, Maine’s decision to implement its system right before seasonal unemployment hit and the state experienced its highest quarter of unemployment led to additional challenges. The state felt forced to implement the project due to a hard date related to the funding of the project and was facing a loss of grant money.
Maine workers experienced well-documented and significant problems with the new system immediately upon its implementation. Workers had difficulties filing initial claims online and logging work search efforts, experienced arbitrary cutoffs at the end of the calendar year, and waited on hold for hours to get help by phone (sometimes only to be disconnected before they got assistance). 64 Thousands of claimants were locked out of their ReEmployME accounts after too many login attempts, after having been incorrectly instructed that they could use their usernames and passwords from the old system. Furthermore, most front-line agency staff did not have the authority to unlock an account and reset a password. The resulting delays prevented many claimants from filing a successful claim within the mandated fourteen-day window. 65
The online work search documentation required for weekly certifications proved particularly challenging for claimants, who found it difficult to navigate and reported that it could only be completed on Sundays (to cover the previous week). 66 State legislators had to step in with a bill allowing work search histories to be filed by phone or in person. 67
While the rollout was problematic, some of Maine’s responses to system issues provide a roadmap for how to protect workers from losing benefits. In particular, the state Department of Labor (which administers the UI program through its Bureau of Unemployment Compensation) gave claimants months to fix work search records, rather than disqualifying them based on failure to submit. Despite the widely reported problems with work search, the department said that very few claimants actually lost benefits. The department also provided leniency for claimants who missed filing claims because of login errors or other problems with the system and waived its backdating requirements.
The Department of Labor observed that claimants who lacked computer skills experienced the most challenges. Many claimants struggled with signing into the system and would forget their passwords. As in Minnesota, the most frequent type of call after implementation was from claimants who were locked out of the system. Based on this experience, Department staff recommended that other states seeking to modernize do targeted education on the password reset process as part of implementation. They also suggested that for new projects, states should roll out the registration system and portal early to give claimants a chance to register and explore.
Many of the issues were less about the actual technology than the business practices and staffing of the Department of Labor at the time ReEmployME was launched. For example, while the department had the technology for skills-based routing of telephone calls, they lacked the staff to implement it. The department also did not have enough staff at the time to support the call center hours. Similarly, the new system was designed to pick up eligibility issues that may have been missed by the legacy system, leading to an increase in fact-finding, which required additional claim examiner time. Finally, during the implementation the department reported several critical staff transitions.
The agency did make some efforts to prepare for the rollout. The Department of Labor created a Training and Support Unit that gathered information from the vendor, Mississippi, and a separate consulting firm that they hired. There were refresher training sessions offered throughout the project and after implementation. Importantly, the department gave its staff time “in the sandbox”—known otherwise as some freeform time to explore the new system. However, they found that structured exploration was the most effective. During this time, agency staff were given assignments to complete, such as practice adjudications, so they could understand how the system would work practically for them.
The Department of Labor also brought in an outside company that specializes in teaching staff how to manage different customer interactions with empathy. Staff rated this training highly, and the department now gives out a quarterly empathy award to staff. Department leadership recommended that, looking forward, they would recommend a “soft rollout” with stakeholders to get feedback before having the entire system go live. The general impression of the agency was that they were on the road to a successful rollout but the rush to go live led to unnecessary problems.
When new leadership took over in 2019, they took a hard look at changes that were necessary to improve ReEmployME and the Department of Labor’s business practices. To address access issues, the department now pays 50 percent of Employment Services staff salaries for the help they provide to UI claimants at local workforce centers and created a video tutorial to teach the staff how to use ReEmployME. During “mud season,” the spring thaw that slows down the state’s logging economy, the department set up mobile labs to help loggers access ReEmployME.
Maine is somewhat limited in the updates it can make to ReEmployME based on its position within the consortium. At the time of our interview, Maine had only recently finalized the operational memorandum of understanding with the consortium, and all changes to the core product must be agreed upon by all consortium members. When a change request comes from a UI director, they must first justify the business case for the change, followed by TCS evaluating the cost, time, and feasibility of such a change. However, one change that was noted during interviews was that Maine recently increased the timeout period on its web applications to fifteen minutes.
Stakeholder Feedback on Implementation in Maine
Claimants confirmed the implementation problems that were covered by the media at the time of implementation. Additionally, they and advocates noted:
- Work search was difficult to fill out on smartphones. Even those who attempted to answer the new work search questions online struggled. Trying to fill in detailed information was time-intensive and claimants felt the system should be able to at least automatically populate their previous entries. Additionally, several mentioned that the system required them to enter a zip code for an employer, which they often did not know. Finally, claimants did not understand why the certification was not integrated with the state job search website they were registered on.
- Department staff could not be reached by phone. The difficulty in reaching help through the telephone system was “demoralizing” and made claimants feel like the system “was not there to help them, and that it did not want them to collect benefits.” People waited for hours on the phone and many still rarely got through. They felt like they spent time waiting on the phone that they could have spent searching for work. Sometimes they would call and just get a message telling them to use the online services. New aspects of the system, like a screen that showed there were “issues” with their claim, without more information, made the inability to talk to department staff even more frustrating.
- Lack of access was compounded by the workforce centers. Workers thought they could go to workforce centers for help, but were typically turned away or told there was nothing that could be done for them there. Thus, the expanded workforce center services prioritized by the new leadership is helpful to addressing this concern.
- Claimants whose calls got through to the department staff were extremely well treated. Claimants said they thought the department representatives were empathetic and very helpful on the phone. The representative would “give them plenty of time, almost as if they felt you had earned that time with them.” Workers always felt like they got the right information from the call center and were relieved to speak with someone about their case.
Data Analysis
The adoption of modernized unemployment insurance systems has occurred at a period when the UI system is reaching fewer unemployed workers than ever before. The percentage of all jobless workers receiving a state unemployment insurance payment has dropped from 43.7 percent in 2001 to just 27.8 percent in 2018. 68 This metric, termed the unemployment insurance recipiency rate, reached an all-time low of 25.7 percent in 2013. Moreover, going into the COVID-19 crisis, fewer jobless workers were even filing an application. Indeed, the share of jobless workers surveyed by the Census Bureau that filed a UI application dropped in half, from 51 percent in 2006 to just 23 percent in 2016. 69
In examining the decline in recipiency from 2004 to 2017, economist Wayne Vroman points out not only that fewer workers are applying for benefits, but also that the increasing number of administrative disqualifications among those who do apply has driven recipiency down even further. 70 As described below, the ways in which modernized UI systems collect, analyze, and adjudicate information submitted by claimants can increase the number of administrative denials. Modernization, however, has nothing to do with another major reason why unemployment insurance recipiency declined, which is the decline in the value of benefits. Responding to increased costs during the Great Recession, nine states reduced their basic unemployment insurance package to fewer than twenty-six weeks. 71
This analysis closely looks at differences between states on administrative measures, such as the portion of workers being denied benefits. Figure 3 plots the increasing numbers of modernized states and the percent of all UI submitted applications that are denied. The national UI denial rate has steadily increased as the number of states modernizing their benefit systems has grown each year. Modernization directly impacts the way in which applications are adjudicated, so there is a much more plausible connection between modernization and denial rates, and this change appears to be correlated with more denials.
Indeed, most national and state agency officials we interviewed expressed little surprise that modernization has been associated with an increasing share of denial rates. Rather than viewing it as a negative sign of reduced claimant access to benefits, these leaders saw it as a positive sign that modernized systems were more accurately determining benefits eligibility. In their view, modernized systems were able to identify problems with benefit claims sooner through more effective fact-finding and prevent claims that may later be determined to be inaccurate, or even fraudulent.
While state officials also expressed hope that modernization might impact overpayment rates, the national overpayment rate changed little from 2012 to 2019, from 10.8 to 10.2 percent of benefits. 72 Overpayments are a major area of concern among UI officials, as the Office of Management and Budget has identified UI as one of the federal programs with the highest level of improper payments. 73
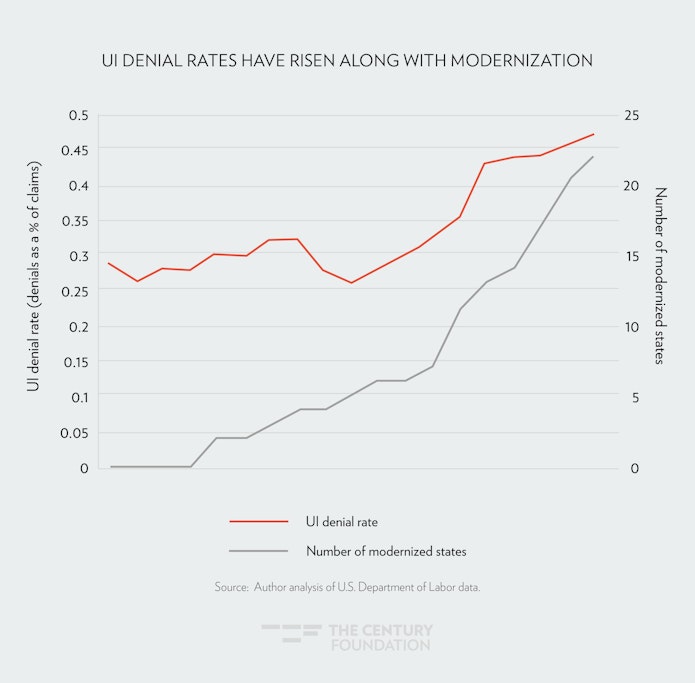
Comparing Modernized and Non-Modernized States
To understand the impact of modernization on access to benefits and the operations of the UI system, we delved into the differences in unemployment insurance data between modernized and non-modernized states. Table 7 compares key UI program indicators between two groups of states: modernized and non-modernized. Specifically, this analysis groups the states that have modernized their programs by 2018 (twenty-two states) and those who had not modernized (twenty-eight states and the District of Columbia). The analysis employed a t-test to see whether observed differences between modernized and non-modernized states were large enough to be statistically significant, or simply within the normal range of differences between states. 74
This analysis shows a systematic connection between modernization and the increasing rates of denials of those who apply for benefits, but not a statistically significant difference in state recipiency rates. In other words, modernization has presented additional challenges for those who make the effort to apply for benefits. Specifically, the data in Table 7 suggests that:
- Denial rates are statistically different between modernized and non-modernized states. Once the analysis is limited to those workers who have applied for UI benefits, the impacts of modernization are stark. Among modernized states, the number of unemployment insurance denials increased by 16.7 percent from 2002 to 2018. Among non-modernized states, the trend is nearly the opposite—denials decreased by 16.5 percent from 2002 to 2018. This difference is statistically significant at the 95 percent level.
- Denials relating to work search and availability to work are driving a wedge between the states. The increase in denial rates among modernized states is driven by one type of denials—nonseparation denials. Nonseparation denials typically occur when an unemployed worker is found to have failed to meet the law’s requirement for being able and available for work and searching for a job. 75 Nonseparation denials include cases when a worker fails to comply with ongoing eligibility requirements for UI like certifying their weekly work search activities or failing to report to a required appointment with a job counselor. Modernized systems have brought significant changes to the determinations of eligibility for these questions, including the ability to ask more detailed questions to claimants about their availability to work and more regularly request names connected with job search activities. As we learned from the stakeholder feedback in our case studies, these online systems can be more difficult to navigate than the phone-based systems that they replaced.
- Denials do not appear to have reduced overpayments. The officials we interviewed about modernization stated that the increased number of nonmonetary denials represented an improvement in issue detection, and that many of these cases would have been found to be overpayments after the fact. In other words, modernized systems better track issues such as working while collecting unemployment at the time they occur, rather than detecting them through data cross-matches that only surface after the infraction. However, our data does not find a systematic difference in overpayments between modernized and non-modernized states, so there is not evidence for the claim that increased denials have reduced overpayments.
Modernization Impacts on Timely, Accurate Payment of Benefits
Typically, new information technology implementations disrupt the business processes of an organization. For UI modernization, this impact can be measured by an analysis of federal performance standards, which regulate if states are deciding and paying UI benefits in a timely and accurate basis.
Table 8 displays a detailed analysis of the change in UI performance measures from the year before modernization to the year after modernization in that particular state. This analysis compares the rate of change at the time of each individual state’s modernization to the national average during the same time period. A state earns a green indicator of its UI performance if it has improved as compared to the national average and a red indicator if it has declined.
Modernization clearly impacts the quality of nonmonetary determinations, as sixteen out of the twenty states analyzed did worse than the national average. In addition, just over half of modernized states also experienced a decline in their ability to move through all the steps of the determination process and deliver a payment on time. Future modernizing states should be aware that changes to businesses processes that came along with modernization can slow state payment times during and can lead to declines in quality.
Moreover, sixteen states have a red indicator when it comes to the average age of appeals, meaning that modernization caused states to take a longer time deciding appeals of benefits. In future modernizations, states should be vigilant to the possibility of unacceptably long appeals timelines and take swift action to shorten them.
AI and Predictive Analytics
As state agencies upgrade their technological capabilities, more vendors have joined the market, offering tools that can automate functions previously performed by agency staff. This follows a national trend that has already impacted the public benefit system, especially state Medicaid and SNAP programs. A lot remains unknown about the types of automated decision-making, predictive analytics, and artificial intelligence currently in use by state unemployment agencies. While some of these tools can improve the functioning of the agencies, and potentially assist workers in better understanding their UI reporting requirements, major concerns about fairness, accuracy, and due process remain.
One of the driving forces behind modernization efforts has been pressure from the U.S. Department of Labor and state legislatures for UI agencies to address improper payments. Although many improper payments are not the result of fraudulent behavior, an entire cottage industry of vendors has developed to provide tools that identify and prevent fraud.
Fraud Detection and Risk Assessment Tools
Fraud detection and risk assessment tools aggregate and analyze data from different cross-match sources or databases and tools that use predictive analytics to detect potential fraudulent behavior or risk. They offer some limited advantages, but mostly raise concerns.
First, the limited advantages. For most of the past fifty years, unemployment systems have had access to different cross-matches with statewide and national databases that have provided information for claims investigations and determination of benefit eligibility. These cross-matches were often analyzed individually by staff, an arduous and time-consuming task. Now, many states have the capability to automatically input this new information into claims and issue fact-finding documentation or determinations, which should catch improper payments at an earlier stage.
However, the aggregation of data more generally can be problematic if the underlying data is not good data. Acting on aggregated inaccurate data only creates more barriers to benefits for workers, and more work for the agency. Furthermore, given the vast inequality in our country and the surveillance that results from using public resources, many low-income and minority workers have significantly more publicly accessible data about them than other workers applying for benefits.
Additionally, some vendors are offering “risk assessment” models or “discovery” tools that will aggregate cross-matches and other data to determine a “score” of fraud risk by the claimant, including FAST Enterprises in Washington. Risk assessment tools have proven constitutionally problematic when used in other contexts. Advocates have raised concerns about—and litigated—the use of risk assessment algorithms in bail-setting in criminal cases. Advocates have also recognized the dangers of discriminatory bias in assessment scores in the child abuse and neglect cases, which aggregate data that is inherently biased, especially against individuals of color. 76
While human bias has always existed in these decision-making processes, the introduction of this technology threatens to permanently cement that bias. These aggregated scores carry with them a sense of infallibility, because of course the computer analysis is more reliable than human analysis. A state actor may quickly rely on the output as a gold standard. However, some researchers have found that the “scores” and predictions from these systems are no more reliable than a coin flip. 77
An important question in these systems is what weighting the agency chooses to give the various cross-matches or data that is input into the assessment score. An outside evaluator can help states determine the fairness and accuracy of a scoring or weighting algorithm before it is deployed.
Automated Decision-making
Running a UI system requires thousands of discrete tasks, and one goal of modernized systems has been to increase efficiency. One method of increasing efficiencies is to automate more of the decisions and notices in the system. Automation has always existed in UI systems; for example, almost all initial determinations on financial eligibility are automated based on the wage reporting in the system for the claimant. Traditionally, other eligibility determinations, both separation and nonseparation, have always had a human touch, meaning that a UI representative is evaluating the facts and determining the outcome.
Vendors in the UI space are pushing technology that will remove these human touches. When information is collected, the system will issue a decision based on the programmed algorithm or analysis. Vendor websites specifically advertise that their systems can act “on behalf of” adjudicators.
The states that participated in our case studies took a careful approach to questions of automated decision-making:
- In Minnesota, the agency structured its decision-making on the idea that “humans do human things, machines do machine stuff—but the humans can always override the machines.” The Minnesota system flags and identifies eligibility issues on its own, which increases operational efficiency but does eliminate some flexibility in claim taking. But on the actual eligibility determinations, there is always a human touch, especially on credibility, which can often be the dispositive factor in UI decisions.
- In Washington, the agency was very careful about automation, as its vendor, FAST Enterprises, had been involved in the Michigan modernization project that resulted in state and federal litigation about its automated decision making. Leadership visited Michigan to better understand the pain points so they would not be replicated in Washington. Notably, the agency ensured that every fraud determination in Washington had a human touch, no decisions were “auto-determined.” While most eligibility determinations were not automated, the back-end adjudication system had almost the same effect at the time the project went live. It created a check box system that left little room for flexibility or individual analysis in each decision, and while determinations before were multiple pages long, the new ones barely had any facts or reasoning. Adjudications were initially left with little choice in how the determinations were written, but ESD later improved the back-end system.Finally, the agency did auto-determine work search compliance. As explained above, this report raises significant concerns about the lack of flexibility in work search questions on continuing claims and auto-determining eligibility based on those questions raises significant access issues.
- In Maine, the state UI statute provides protection against any form of computer decision-making. The statute requires that, prior to any disqualification based on new information during a continuing claim, a fact-finding telephone interview must be scheduled with the parties. 78
However, many concerns remain about how other states are using automated decision-making, especially in the work-search and overpayment contexts.
Another AI-driven technology in use in at least one state is “nudging”—the use of predictive analytics to apply targeted behavioral psychology to change the conduct of users. The basic premise is promising: states can use aggregated historical data to identify factors, not necessarily personal characteristics, that may pose challenges for claimants to successfully and correctly complete initial and continuing claims. Unlike other forms of AI, which can be used to proactively disqualify claimants, nudging in this fashion does not prescribe immediate negative consequences. A target of nudging should not be in a worse position than a claimant who does not receive any nudges.
At this point, the focus of nudging technology in UI has been to prevent improper payments. New Mexico, working with Deloitte, developed a series of nudging tools and messages aimed at improving compliance in areas where high numbers of improper payments were reported, primarily on continuing claims filing. New Mexico also used nudging and risk assessment models to identify worker misclassification.
Deloitte was able to statistically control which groups of claimants received which messages. Although neither Deloitte or New Mexico did any user testing or focus groups to develop the text of the messages, they collected data after implementation and continued to update and narrow the messages based on what wording was effective. New Mexico found that personalizing the messages worked best, while scare tactics and legalese failed to have an impact. However, overall there was not a clear indication that the nudging tactics had a significant impact on improper payments, in part due to message fatigue. New Mexico also abandoned an early effort to use nudging during initial claims after not seeing any improvement in claimant response, and also shared a concern that too much nudging during initial claims might dissuade claimants from thinking they are eligible for benefits. Importantly, to ensure fair consideration of every claim, no agency claim examiners or interviewers are able to see in the system who received a message as a result of the nudging analytics.
Nudging, especially when the technology has been outsourced to a private vendor, raises similar concerns to other uses of algorithms and automation in unemployment insurance systems: what data is being used and how is it being evaluated? New Mexico described the algorithm factors used to identify risk as designed and maintained by Deloitte. While they can see the scores, they cannot see the underlying characteristics or weighting upon which the scores are based. The state referred to the nudging technology as “proprietary” to Deloitte. Black box technology, especially when controlled by a private entity, raises due process concerns, both procedural and substantive, for claimants whose eligibility may be affected by the decision-making.
State Responsibility for Effects of AI and Predictive Analytics
While these technologies may improve efficiencies for state agencies, their use should be centered on improving outcomes for the end users of the unemployment system. An efficient system that improperly delays or denies benefits, or incorrectly assesses fraud, runs contrary to the due process rights of claimants and federal law governing fairness in the administration of unemployment systems. 79
Recent litigation in Michigan by claimant-plaintiffs alleging due process violations from the state’s automated unemployment fraud detection system, known as the Michigan Integrated Data Automated System (MiDAS), highlights the fact that state officials are not shielded from liability solely because an algorithmic system created by a private vendor caused the rights deprivation. As the federal District Court in the case described:
MiDAS was developed to search for discrepancies in the records of unemployment compensation recipients, automatically determine whether the claimants committed fraud, and execute collection proceedings, which included intercepting tax refunds and garnishing wages. Auto-adjudication is a process that starts with the automated generation of a flag, then leads to the automated generation of questionnaires, then to an automated determination based on logic trees, followed by an automated generation of a notice of fraud determination, then automated collection activity. 80
In the case, claimant-plaintiffs allege that the defendants, including several agency officials and the private vendor, “worked together with the state to design, maintain, operate, and implement the robo-fraud-detection and adjudication system . . . [which] labeled them fraudsters, and then assessed and collected fines and penalties, all without notice and an opportunity to be heard.” 81 Similar claims were made in an ongoing state court case alleging due process violations under Michigan’s constitution. 82 State officials sought qualified immunity in the federal case, but the Sixth Circuit Court of Appeals rejected the defendants’ “invitation to allow state actors to evade liability by utilizing new technologies to effectuate unconstitutional conduct.” 83 The court refused to let the state officials “hide behind MiDAS,” finding that “MiDAS did not create itself,” as the officials implemented and oversaw the project and enforced the false fraud determinations it “automatically rendered.” 84
State UI agency officials should heed the warning from the federal courts that the use of technology created by a private vendor does not create an automatic legal shield when that technology violates the rights of claimants. But these problems can be avoided if states follow the types of recommendations in this report, which place the user experience at the center of the planning, design, and implementation of modernized benefit systems. As a multitude of vendors flood the market with different fraud detection software, it is vital for states to concretely understand the underlying technology and the data and algorithms it relies upon, and to take proactive measures to ensure that claimants, already navigating these difficult systems, are not unduly harmed.
Conclusion: The Road Ahead
The surge in unemployment claims in 2020, and the failure to meet customer service standards, has caused a reckoning among states and will undoubtedly accelerate modernization efforts. States should heed the lessons of those that have come before them, by basing immediate and long-term changes on solid user testing and bedrock principles of claimant access. Too often, modernization efforts have had negative impacts of claimant access, and the future of the UI system depends on a different approach.
Appendix: Research Methods
The case studies of modernization in Maine, Minnesota, and Washington were conducted from October 2018 to January 2020. Each involved many hours of in-person discussions with UI agency leadership and staff, focus groups with unemployed workers, and interviews with legal services organizations, union officials, and other stakeholders.
Site Selection
As discussed earlier, Maine, Minnesota, and Washington were selected as case study sites because they showcased different approaches to UI IT modernization projects. They also represent states with different populations, economies, and labor forces. Following our conversations with several state agencies (see Initial Lessons from States section), we developed additional selection criteria that led us to feature these three states in our final report. These criteria included evaluating the strength of the agency’s business processes; evaluating the agency’s ability to respond to problems and public critiques following the go-live; and identifying a strong presence of legal aid services, labor organizations, and worker advocacy groups invested in improving the claimant side of the state’s unemployment insurance program.
Our final criteria turned out to be critical to determining our case study states: agency management had to be willing participants, which entailed agency staff working in policy and leadership, adjudication, claims taking, appeals, and IT committing to one or two full days of interview meetings and system demonstrations with our research team. While agency leaders were often enthusiastic about our project, it was challenging for them to divert staff away from daily operations for extended periods; early discussions with South Carolina, Mississippi, New Mexico, and Utah did not result in a case study for these reasons.
Our research team had strong existing relationships with many UI agency leaders from previous research on unemployment insurance and through NASWA, which helped us secure commitments from agency leadership in Maine, Minnesota, and Washington. Our agency partners had deep knowledge of the industry and suggested suitable states to choose for our case study research. They also were also helpful with initiating introductions for us when our research team did not have existing relationships.
Focus Group Recruitment
We recognize there are demographic limitations to our final site selection. Minnesota, Maine, and Washington are northern states with seasonal industries and labor forces. They have significantly less diverse populations—6.8 percent of Minnesota residents, 4.3 percent of Washington residents, and just 1.6 percent of Maine residents are Black or African American, compared to 13.4 percent of the total U.S. population. Similarly, 5.5 percent of Minnesota residents, 12.9 percent of Washington residents, and 1.7 percent of Maine residents are Latinx or Hispanic, compared to 18.3 percent of the national population. However, Minnesota and Washington had slightly denser American Indian and Alaska Native populations—1.4 percent and 1.9 percent, respectively—compared to the entire nation. 85
We attended to these demographic limitations by holding focus groups in an urban center as well as a rural township in each state. We coordinated focus group recruitment with legal aid advocates, labor organizations, and social-justice-oriented community groups in each state. Many of these organizations expressly provided services to BIPOC (Black, Indigenous, People of Color) communities, immigrants, and low-income workers. They reached out to their former or current clients to encourage focus group participation. The Century Foundation also ran Facebook advertisements in each state. The Facebook advertisements linked respondents to a short online screening survey designed and hosted by the research team, which collected self-reported demographic information from respondents. These combined efforts helped us select and plan for diverse focus group formations.
Participation in focus groups was limited to individuals who had filed for unemployment insurance in a case study state post-modernization. Participants did not have to have received unemployment insurance to be eligible. Though not always possible, we encouraged participation of individuals who had experience filing for unemployment insurance using both the old and the new system. Unless participants were directly recruited by our partnering organization, research team members contacted all respondents via email to confirm their eligibility. If there was more interest in a focus group than there were available seats, the research team extended invitations to individuals who self-reported as BIPOC first. For each focus group, we planned for up to twelve participants though typical attendance was between six to nine. Table 9 shows a breakdown of focus group participation.
All participants received a $100 per diem. Additionally, we gave all participants a free meal, since many of the focus groups took place after regular business hours and during dinnertime. Focus group conversations ran for approximately two hours and were attended by one moderator and one dedicated notetaker. When it was feasible, focus group conversations were also audio recorded, after obtaining signed and verbal consent from all participants.
Partnering with local organizations to recruit focus group participants was both strategic and mutually beneficial. Legal aid services in Portland, Seattle, and Minneapolis offered to host focus groups. A social-justice-oriented community organization in Sunnyside and a labor temple in Brewer also hosted focus groups. Organizations that volunteered to host our focus groups were given a donation of $500 to show our gratitude for their work.
Interview Methods
Our interview instrument had ten open-ended questions that were designed to analyze the institutional and procedural changes that accompanied UI IT projects. The questions were as follows:
- What are the three biggest ways modernization changed your business process?
- What are the three biggest ways modernization changed the claimant experience?
- Describe the claimants who have the easiest time accessing benefits today, and those who experience the greatest challenges. Would your answer have been different in any way before modernization?
- How has modernization changed the initial application experience for the state? For the claimant?
- How did modernization change the call center experience for claimants and staff at the time the new system was launched, and on an ongoing basis?
- How has modernization changed the continuing claims experience for the state? For the claimant?
- How has modernization changed the appeals process for the state? For the claimant?
- Did you get input from claimants or employers before implementation, and is your system set up to make continuous improvements ?
- What advice would you give another state that is considering modernizing?
- How would you describe the ideal UI system (in terms of how it operates, not the tax/benefit structure)?
These questions were shared with state agencies prior to the research team’s visit. By sharing the questions beforehand, agency management was able to identify team members with specialized knowledge in areas related to policy and leadership, claims taking, adjudication, and appeals. We also noted our interest in securing a system demonstration and meetings with agency staff from the IT department working in system design, updates, and data management. In Maine and Washington, this included conversations with external IT consultants from Tata Consulting Services and Fast Enterprises.
Sharing our research goals with agency leaders beforehand helped them create structured itineraries for our visit that made the best use of everyone’s time. Many of our interviews included visual presentations, handouts of UI data trends, and other prepared resources for our benefit. On average, we spent one to one-and-a-half full business days with state agencies in scheduled meetings across several agency departments.
At least three research team members were present during each agency interview. The interview format was necessarily semi-structured to allow agency staff to schedule opportune meetings that would not disrupt their daily operations. Though our questions were asked out of order depending on the availability of departmental staff, we secured meetings and system demonstrations that covered all our interest areas at each state. Furthermore, we always had scheduled time to debrief with agency staff that allowed them to ask questions about our project and research goals. All research team members took notes, although there was always one research team member dedicated to taking detailed notes. Meetings with state agencies were not recorded.
Our research questions did not require much modification to be relevant to other stakeholders, such as legal aid services and labor unions. These semi-structured interviews were shorter (usually two to three hours) and took place after the research team’s visits to state agencies. Questions probed whether worker advocates had played a role in the design and implementation of UI IT projects. Asking the same ten questions that we asked the state agencies proved very illuminating, since worker advocates’ interactions with claimants sometimes changed after modernization. They were able to identify specific aspects of the new system that were challenging for their clients. They also suggested innovative approaches to designing an ideal UI system.
Our strategy for choosing case study sites included assessing whether there were established community organizations with a vested interest in strengthening unemployment insurance. Given our team members’ backgrounds and expertise, we found making these connections and coordinating interviews relatively straightforward—community leaders and legal aid lawyers were interested and enthusiastic about our project. Research team members reached out to their existing connections with legal aid services and labor unions in each state to set up interviews. These working meetings also became the basis for participant recruitment for our focus groups, as several organizations represented clients that were appealing UI agency decisions.
Focus Group Methods
Focus groups are group interview settings that emphasize the importance of shared experiences and interest in specific topics. 86 Our research team identified unemployment insurance claimants early on as key stakeholders with vested interests in the design and outcome of UI IT modernization projects. Despite this, claimants are rarely consulted during or even after projects. Consequently, there is a dearth of knowledge about whether claimants find online UI systems to be as efficient, convenient, and beneficial as agencies claim them to be.
Focus group questions are necessarily semi-structured to allow discussion to flow freely. Our focus group questions included a variety of closed-ended and open-ended questions to encourage participation by all. Questions followed the procedural steps of applying for unemployment insurance. We began by asking participants about their experience filling out the initial application and filing weekly claims online. Next, we asked participants about how certain processes (for example, fulfilling the work search requirement) were impacted by moving these processes online. We asked participants to reflect on whether UI modernization changed other program services, such as call center and WorkForce center operations. Finally, we asked participants who had experienced issues or filed appeals to talk about how these processes were handled by the new system.
In each focus group, the moderator and notetaker began the discussion with personal introductions and a description of the research project. Participants were given consent forms to sign that explained the terms of their participation. Participants were asked to respect others’ privacy by not sharing stories outside of the group, and refrain from interrupting when others were speaking. To encourage camaraderie within the group, discussion started with introductions and an ice-breaker activity—each participant shared their story about applying for unemployment and rated their experience with the state UI program. In larger focus groups, participants were asked to use a nametag or name card to allow moderators or participants to refer to everyone by name.
Participants’ ratings of the UI program highlighted how the new system worked for some but not all claimants. Even participants that rated their experience with the UI agency highly were sympathetic towards participants who had struggled with their application. There was a natural agreement with most focus groups that applying for unemployment insurance was needlessly complex and embarrassing, which elicited rich discussions about how online systems helped or hindered claimants.
With the moderator asking questions and follow-ups, facilitating equal participation, and occasionally redirecting conversation, the second research team member was free to take detailed notes during the conversation. Transcriptions from recorded focus groups were generated automatically using Otter AI.
Narrative Analysis
After interviews and focus group meetings, research team members debriefed to extrapolate the themes of the day. This included comparing notes, parsing out answers to research questions, and identifying items that required direct follow-up. Our notes from interviews and focus groups, audio recordings and transcripts, and agency-generated data and presentations made up the bulk of materials analyzed for the purpose of this report.
Our objective was to identify how UI modernization impacted the claimant experience. Identifying claimant outcomes that are particular to UI IT systems requires isolating these issues from processes and norms related to UI systems generally. To accomplish this, we asked state agencies, worker advocacy organizations, and claimants themselves to share stories—or narratives—to relate personal experience to institutional change.
Narrative analysis is a group of methods for interpreting texts telling a common story from different angles. 87 It is a suitable analytical method for our study given the time elapsed between system go-lives and the time of our interviews. It focuses analysis away from what happened to how people make sense out of what happened and to what effect. 88 Agency staff explaining how modernization changed UI told stories about how project decision-making was linked to political actors and events, institutional values, and even the changing seasons. Similarly, claimants’ interactions with unemployment systems were closely tied to personal stories about experiencing job loss as well as other hardships.
Narrative analysis “is an approach to the analysis of qualitative data that emphasizes the stories that people employ to account for events,” Alan Bryman explains. 89 It is a method that illuminates overarching narratives through comparison; for example, three separate agencies explaining what went into their decision to go-live in the middle of winter. It is a method that humanizes contexts that would otherwise seem diametrically opposed; for example, finding that agency officials and claimants share many of the same values in system design.
Narrative analysis grounded the research team’s analysis. Analyzing our research products generated concrete recommendations for states during planning, design, and implementation stages of modernization projects. While these recommendations are tailored to include claimants during each stage, they are also responsive to the needs of agency employees, worker advocacy organizations, and other key stakeholders in building a UI system that works for everyone.
- Mark Bocchetti, “States navigate jobless flood at different speeds,” Roll Call, (April 28, 2020), https://www.rollcall.com/2020/04/28/states-navigate-jobless-flood-at-different-speeds/ .
- George Wentworth, “Our Country Has Forgotten the Lessons of the Great Recession,” The Hill , December 22, 2017 https://www.nelp.org/commentary/our-country-has-forgotten-the-lessons-of-the-great-recession/
- Ben Zipperer and Elise Gould, “Unemployment filing failures,” Economic Policy Institute , April 28, 2020, https://www.epi.org/blog/unemployment-filing-failures-new-survey-confirms-that-millions-of-jobless-were-unable-to-file-an-unemployment-insurance-claim/.
- Kathy Manderino, Secretary of the Pennsylvania Department of Labor and Industry, “Written Testimony before the House Labor and Industry Committee Regarding the Department of Labor and Industry Unemployment Compensation System,” Pennsylvania State Legislature, Harrisburg, Pennsylvania, March 1, 2017, https://www.legis.state.pa.us/WU01/LI/TR/Transcripts/2017_0021_0001_TSTMNY.pdf.
- Lou Ansaldi, NASWA/ITSC, “UI IT Modernization Overview,” presentation at the NASWA UI Directors Conference, Orlando, Florida, November 8, 2017.
- Paul Egan, “Judge blasts state agency as court OKs faulty computer system lawsuit,” Detroit Free Press , January 3, 2019, https://www.freep.com/story/news/local/michigan/2019/01/03/unemployment-insurance-agency-michigan/2474723002/ ; Steve Gray and Casey Farrington, “Opinion: Undoing the harm of MiDAS’ fraud designations,” The Detroit News , October 16, 2018, https://www.detroitnews.com/story/opinion/2018/10/16/opinion-undoing-harm-midas-fraud-designations/1649803002/ .
- William Carrington, “Unemployment Insurance in the Wake of the Recent Recession,” Congressional Budget Office, November 2012, http://www.cbo.gov/sites/default/files/cbofiles/attachments/11-28-UnemploymentInsurance_0.pdf.
- Alan S. Blinder and Mark Zandi, “The Financial Crisis: Lessons for the Next One,” Center of Budget and Policy Priorities, October 15, 2015, https://www.cbpp.org/research/economy/the-financial-crisis-lessons-for-the-next-one.
- 42 U.S.C. Sections 503(a)(1), (3); 20 C.F.R. Parts 640, 650.
- Lincoln Quillian, Devah Pager, Ole Hexel, Arnfinn H. Midtbøen, “The persistence of racial discrimination in hiring,” Proceedings of the National Academy of Sciences, September 2017, https://www.pnas.org/content/early/2017/09/11/1706255114#ref-17 ;Erik Sherman, “Hiring Bias Blacks And Latinos Face Hasn’t Improved In 25 Years,” Forbes, September 16, 2017, https://www.forbes.com/sites/eriksherman/2017/09/16/job-discrimination-against-blacks-and-latinos-has-changed-little-or-none-in-25-years/#3830954451e3.
- Olugbenga Ajilore, “On the Persistence of the Black-White Unemployment Gap,” Center for American Progress, February 24, 2020, https://www.americanprogress.org/issues/economy/reports/2020/02/24/480743/persistence-black-white-unemployment-gap /; Jhacova Williams and Valerie Wilson, “Black workers endure persistent racial disparities in employment outcomes,” Economic Policy Institute, August 27, 2019, https://www.epi.org/publication/labor-day-2019-racial-disparities-in-employment/.
- Andre M. Perry, “Black workers are being left behind by full employment,” The Brookings Institution, June 26, 2019, https://www.brookings.edu/blog/the-avenue/2019/06/26/black-workers-are-being-left-behind-by-full-employment/.
- Austin Nichols and Margaret Simms, “Racial and Ethnic Differences in Receipt of Unemployment Insurance Benefits during the Great Recession,” Urban Institute, June 2012, https://www.urban.org/sites/default/files/publication/25541/412596-Racial-and-Ethnic-Differences-in-Receipt-of-Unemployment-Insurance-Benefits-During-the-Great-Recession.PDF.
- Nicole Lyn Pesce, “A shocking number of Americans are living paycheck to paycheck,” MarketWatch , January 11, 2020, https://www.marketwatch.com/story/a-shocking-number-of-americans-are-living-paycheck-to-paycheck-2020-01-07.
- Kriston McIntosh, Emily Moss, Ryan Nunn, and Jay Shambaugh, “Examining the Black-white wealth gap,” The Brookings Institution, February 27, 2020, https://www.brookings.edu/blog/up-front/2020/02/27/examining-the-black-white-wealth-gap/; Danyelle Solomon and Darrick Hamilton, “The Coronavirus Pandemic and the Racial Wealth Gap,” Center for American Progress, March 19, 2020, https://www.americanprogress.org/issues/race/news/2020/03/19/481962/coronavirus-pandemic-racial-wealth-gap/ .
- Janelle Jones, “The racial wealth gap,” Economic Policy Institute, February 13, 2017, https://www.epi.org/blog/the-racial-wealth-gap-how-african-americans-have-been-shortchanged-out-of-the-materials-to-build-wealth/.
- Eileen Patten, “Racial, gender wage gaps persist in U.S. despite some progress,” Pew Research Center, July 1, 2016, https://www.pewresearch.org/fact-tank/2016/07/01/racial-gender-wage-gaps-persist-in-u-s-despite-some-progress/.
- “Quantifying America’s Gender Wage Gap by Race/Ethnicity,” National Partnership for Women and Families, March 2020, https://www.nationalpartnership.org/our-work/resources/economic-justice/fair-pay/quantifying-americas-gender-wage-gap.pdf .
- “Mobile Fact Sheet,” Pew Research Center, June 12, 2019, https://www.pewresearch.org/internet/fact-sheet/mobile/.
- Andrew Perrin and Erica Turner, “Smartphones help blacks, Hispanics bridge some—but not all—digital gaps with whites,” Pew Research Center, August 20, 2019, https://www.pewresearch.org/fact-tank/2019/08/20/smartphones-help-blacks-hispanics-bridge-some-but-not-all-digital-gaps-with-whites/.
- Monica Anderson, “Racial and ethnic differences in how people use mobile technology,” Pew Research Center, April 30, 2015, https://www.pewresearch.org/fact-tank/2015/04/30/racial-and-ethnic-differences-in-how-people-use-mobile-technology.
- Robyn Caplan, Joan Donovan, Lauren Hanson, and Jeanna Matthews, “Algorithmic Accountability: A Primer,” Data and Society Research Institute, April 18, 2018, https://datasociety.net/wp-content/uploads/2018/04/Data_Society_Algorithmic_Accountability_Primer_FINAL-4.pdf.
- “Understanding Algorithms,” Data and Society Research Institute, April 18, 2018, https://datasociety.net/wp-content/uploads/2018/04/Data_Society_Understanding_Algorithms_Explainer_FINAL-web.pdf.
- Caplan, Donovan, Hanson and Matthews, “Algorithmic Accountability”; Heidi Ledford, “Millions of black people affected by racial bias in health-care algorithms,” Nature Research , October 24, 2019, https://www.nature.com/articles/d41586-019-03228-6.
- Caplan, Donovan, Hanson and Matthews, “Algorithmic Accountability.”
- Email from Tom Stengle, U.S. Department of Labor, author’s calculations
- U.S. Department of Labor “UI Performs Core Measures,” accessed August 1, 2020 https://oui.doleta.gov/unemploy/pdf/Core_Measures.pdf .
- “State Supplemental Funding Survey,” National Association of State WorkforceAgencies, March 31, 2017 https://www.naswa.org/system/files/document/fy_2016_supplemental_report.pdf.
- Internet Unemployment System, Idaho Department of Labor, https://labor.idaho.gov/dnn/iUS , accessed June 26, 2020.
- Ansaldi, “UI IT Modernization Overview.”
- Information Technology Support Center, Status of State UI IT Modernization Projects, September 2019 http://www.itsc.org/Documents/Status%20of%20State%20UI%20IT%20Modernization%20Projects.pdf
- Megan Woolhouse and Beth Healy, “None Admit Fault on Troubled Jobless Benefits System,” Boston Globe, October 28, 2013, https://www.bostonglobe.com/business/2013/10/28/state-senators-question-deloitte-labor-chief-troubled-unemployment-benefits-system/8HrEnVobsloB9tNiILb7nJ/story.html. Auditor general reports have identified problems with modernized UI systems. See, e.g., Tennessee Comptroller of the Treasury, Single Audit Report, Division of State Audit, for the Year Ended June 30, 2016, March 22, 2017, 372, http://controller.finance.tennessee.edu/wp-content/uploads/sites/7/2017/03/2016_TN_Single_Audit.pdf (hereafter “Tennessee Report”); Louisiana Legislative Auditor, Financial Audit Services Management Letter, December 14, 2016, 2, http://app.lla.state.la.us/PublicReports.nsf/0/75F71BBD2CDA081686258089006513D9/$FILE/00011DB2.pdf (hereafter “Louisiana Report”); Office of the Auditor General, Report Summary of Performance Audit: Michigan Integrated Data System (MiDAS), February 2016, 1, https://audgen.michigan.gov/wp-content/uploads/2016/06/rs641059315.pdf (Michigan).
- Monica Halas, consulting attorney, and Hajar Hasani and Larisa Zehr, legal interns, Northeastern University School of Law, “UI Online: The Problem, the Legal Framework and Solutions,” Greater Boston Legal Services.
- Tennessee Report, 372.
- David Gutman, “State’s new unemployment- benefits website can’t handle traffic at launch,” The Seattle Times , January 3, 2017, https://www.seattletimes.com/seattle-news/politics/states-new-unemployment-benefits-website-crashes-right-after-launch/.
- Michael Van Sickler, “State audit highly critical of Florida’s unemployment system CONNECT,” Tampa Bay Times , February 27, 2015, https://www.tampabay.com/news/politics/gubernatorial/state-audit-highly-critical-of-floridas-unemployment-system-connect/2219504/#.
- George Wentworth and Claire McKenna, “Ain’t No Sunshine: Fewer than one in eight unemployed workers in Florida is receiving unemployment insurance,” National Employment Law Project, September 21, 2015, https://www.nelp.org/publication/aint-no-sunshine-florida-unemployment-insurance/.
- Miami Workers Center v. Florida Department of Economic Opportunity, Division of Workforce Services (CRC Complaint No. 12-FL-048).
- Bruce Krasnow, “Group files civil rights claim vs. New Mexico,” The New Mexican, August 15, 2013, https://www.santafenewmexican.com/news/local_news/group-files-civil-rights-claim-vs-new-mexico/article_f14e0688-ecbd-5a2f-9ddc-8eefdc89ac9e.htm.
- U.S. Department of Labor, “Model Unemployment Insurance Work Search Legislation,” Training and Employment Notice 17-19, February 10, 2020, https://wdr.doleta.gov/directives/attach/TEN/TEN_17-19.pdf.
- Jonathan Oosting, “Lawsuit over false fraud fiasco revived by Michigan Supreme Court,” The Detroit News , April 5, 2019, https://www.detroitnews.com/story/news/local/michigan/2019/04/05/supreme-court-revives-false-fraud-lawsuit-against-michigan/3377047002/.
- “Information Technology: Department of Labor Could Further Facilitate Modernization of States’ Unemployment Insurance Systems,” U.S. Government Accountability Office, GAO-12-957, September 2012; “Unemployment Insurance: States’ Customer Service Challenges and DOL’s Related Assistance,” U.S. Government Accountability Office, GAO-16-430, May 2016.
- “Modernizing the Unemployment Insurance Program: Federal Incentives Pave the Way for State Reforms,” National Employment Law Project, May 2012, https://www.nelp.org/wp-content/uploads/2015/03/ARRA_UI_Modernization_Report.pdf .
- Unemployment Insurance Program Letter 2-16, October 1, 2015, https://oui.doleta.gov/dmstree/uipl/uipl2k16/uipl_0216.pdf.
- Ibid., change 1.
- Unemployment Insurance Program Letter No. 11-18, August 17, 2018, 2, https://wdr.doleta.gov/directives/corr_doc.cfm?DOCN=9114.
- Phone interview with Ellen Golombeck, Deputy Director, National Association of State Workforce Agencies, October 10, 2018.
- 43 P.S. § 781.4(h)(6) (Pa.).
- “An Act Financing the General Governmental Infrastructure of the Commonwealth,” The Acts and Resolves of Massachusetts , chapter 151, 2020, https://malegislature.gov/Laws/SessionLaws/Acts/2020/Chapter151.
- “Mobile Fact Sheet,” Pew Research Center, June 12, 2019, https://www.pewresearch.org/internet/fact-sheet/mobile/ (showing that 96 percent of U.S. adults own a cell phone and 81 percent own a smartphone, compared to 74 percent who own a desktop or laptop computer).
- Monica Anderson and Madhumitha Kumar, “Digital divide persists even as lower-income Americans may gains in tech adoption,” Pew Research Center, May 7, 2019, https://www.pewresearch.org/fact-tank/2019/05/07/digital-divide-persists-even-as-lower-income-americans-make-gains-in-tech-adoption/ (showing that only 54 percent of adults with incomes below $30,000 have computers and 71 percent have smartphones, while ownership rates for the two types of technology are similar among middle-income and higher-income adults).
- UI agency officials from the following states were interviewed for this project: Colorado, Iowa, Kansas, Maine, Minnesota, Mississippi, New Mexico, Pennsylvania, Utah, Vermont, Washington, and Wyoming.
- State of Minnesota, Office of Enterprise Technology.
- “Status of State UI IT Modernization Projects,” NASWA Information Technology Support Center, updated September 2019 http://www.itsc.org/Documents/Status%20of%20State%20UI%20IT%20Modernization%20Projects.pdf.
- Theo Douglas, “Efficiency in Numbers as States Take Unemployment Insurance to the Cloud,” GT: Government Technology , November 6, 2017, https://www.govtech.com/computing/Efficiency-in-Numbers-as-States-Take-Unemployment-Insurance-to-the-Cloud.html.
- Colin Ellis, “How Maine bungled rollout of new jobless claims system,” Portland Press Herald , March 11, 2018, https://www.pressherald.com/2018/03/11/state-bungled-rollout-of-new-unemployment-claims-system/.
- Robert N. Charette, “Maine’s New Unemployment System Frustrates the Public and State Workers Alike,” IEEE Spectrum , March 23, 2018, https://spectrum.ieee.org/riskfactor/computing/it/maines-new-unemployment-system-frustrates-the-public-and-state-workers-alike.
- Colin Ellis, “Lawmakers still seeking fix for Maine unemployment filing system,” Portland Press Herald , March 7, 2018, https://www.centralmaine.com/2018/03/07/state-legislators-still-seeking-fix-for-states-unemployment-filing-system/ .
- “Application and Recipiency CY 1988–2019,” U.S. Department of Labor, Employment and Training Administration, updated November 1, 2019, https://oui.doleta.gov/unemploy/large_carousel.asp?slide=0.
- Stephen A. Wandner and Andrew Stettner, “Why are many jobless workers not applying for benefits?” Monthly Labor Review , June 2000, https://www.bls.gov/opub/mlr/2000/06/art2full.pdf , and Wayne Vroman, “Unemployment insurance recipients and nonrecipients in the CPS,” Monthly Labor Review , October 2009, https://www.impaqint.com/sites/default/files/project-reports/Vromen_UI.pdf.
- Wayne Vroman, “Unemployment Insurance Benefits Performance since the Great Recession,” Urban Institute, February 27, 2018, https://www.urban.org/research/publication/unemployment-insurance-benefits.
- Andrew Stettner, “Unemployment Trust Fund Recovery Is Helping Employers, not Workers,” The Century Foundation, December 7, 2017, https://tcf.org/content/report/unemployment-trust-fund-recovery-helping-employers-not-workers/ .
- “Unemployment Insurance Benefit Payment Integrity,” United States Department of Labor, Employment and Training Administration, https://oui.doleta.gov/unemploy/improp_payrate.asp.
- Office of Management and Budget, “High-Priority Programs and Programs over $100M in Monetary Loss, 2019,” https://www.paymentaccuracy.gov/payment-accuracy-high-priority-programs/ accessed August 1, 2020.
- While this does not show that modernization is a causal factor, it does show whether the difference is large enough that modernization could be the cause.
- Separation denials are related to the reason a worker lost their job and whether it counts as an eligible reason under UI law; everything else is a nonseparation.
- Virginia Eubanks, Virginia. Automating Inequality : How High-Tech Tools Profile, Police, and Punish the Poor (New York: St. Martin’s Press, 2018).
- “Impoverished Algorithms: Misguided Governments, Flawed Technologies, and Social Control,” 46 Fordham Urb. L.J. 364, 379.
- See 26 M.R.S. § 1194.
- Cahoo v. SAS Inst. Inc., 2020 U.S. Dist. LEXIS 145817, *4 (E.D. Mich. Aug. 11, 2020).
- See Bauserman v. Unemployment Ins. Agency, 2019 Mich. App. LEXIS 7683 (Mich. 2019).
- Cahoo v. SAS Analytics Inc ., 912 F.3d 887, 904 (6th Cir. Mich. 2019).
- “Quick Facts: Minnesota, Washington, Maine, United States Population Estimates July 1, 2019,” United States Census Bureau, https://www.census.gov/quickfacts/fact/table/MN,WA,ME,US/PST045219.
- Alan Bryman, Social Research Methods, 5th Edition (Oxford: Oxford University Press, 2016), 501.
- Catherine Kohler Riessman, Narrative Analysis (Newbury Park, Calif.: Sage, 1993), 11.
- Bryman, Social Research Methods , 589. Emphasis added.
- Ibid., 590.
Tags: unemployment , unemployment insurance
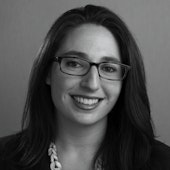
Julia Simon-Mishel, Contributor
Julia Simon-Mishel is the supervising attorney of the Unemployment Compensation Unit at Philadelphia Legal Assistance (PLA), where she represents low-wage workers in unemployment matters. She has represented over 700 clients and has an active appellate practice pursuing impact unemployment cases in Pennsylvania courts. She serves by appointment on Pennsylvania’s Unemployment Compensation Benefit Modernization Advisory Committee and has testified by invitation before the Pennsylvania legislature many times on issues affecting her clients. During the COVID-19 pandemic, she has spearheaded community outreach and education about unemployment programs in Pennsylvania, working closely with social service organizations, community groups, legal services organizations, and local officials to help tens of thousands of workers access benefits. Prior to joining PLA, she clerked for the Honorable Norma L. Shapiro of the Eastern District of Pennsylvania. She was recently named one of the American Bar Association’s “One the Rise: Top 40 Young Lawyers” and a Super Lawyers “Rising Star” in Pennsylvania. She graduated magna cum laude from the University of Pennsylvania Law School and Brandeis University.
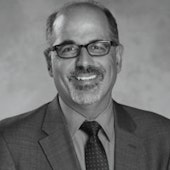
Maurice Emsellem, Contributor
Maurice Emsellem is director of the Fair Chance program at NELP. He joined NELP in 1991, after working for the Legal Aid Society in New York City. At NELP, Maurice has worked on collaborations with organizers and advocates that have successfully modernized state unemployment insurance programs, created employment protections for workfare workers, and reduced unfair barriers to employment of people with criminal records in state laws and in city hiring practices. He has testified before Congress and numerous state legislatures, promoting innovative policy reforms. He was a Soros Justice Senior Fellow in 2004 and a Stanford Public Interest Law Mentor in 2003.
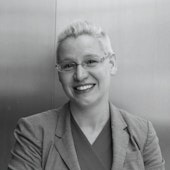
Michele Evermore, Interim Director of Disability Economic Justice and Senior Fellow
Michele Evermore is the interim director of disability economic justice and senior fellow at The Century Foundation, where she focuses on policy for improving the nation’ social insurance programs.
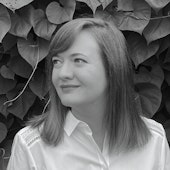
Ellen LeClere, Contributor
Ellen LeClere joined NELP as an independent researcher in January 2019. She received her PhD in information studies at the University of Wisconsin–Madison in December 2019, where she was awarded a prestigious Mellon Wisconsin Fellowship for her dissertation research. In addition to unemployment insurance modernization, her research projects and expertise includes work in archives and records management, information ethics, science and technology studies, and labor issues. She is currently a data specialist for the Association of Flight Attendants–CWA.
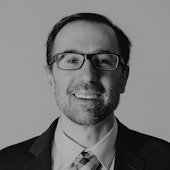
Andrew Stettner, Former Director of Workforce Policy and Senior Fellow
Andrew Stettner was the director of workforce policy and senior fellow at The Century Foundation, focusing on modernizing workforce protections and social insurance programs.
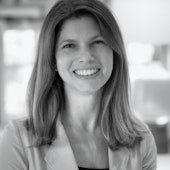
Martha Coven, Editor
Martha Coven is a consultant with two decades of federal policy experience in the executive branch, legislative branch, and nonprofit sector, including six years in the Obama White House. She was associate director for education, income maintenance, and labor in the Office of Management and Budget, where she oversaw a large share of the federal budget, and a special assistant to the president at the Domestic Policy Council, where she advised on poverty and workforce issues and developed the administration’s plan for reducing childhood obesity.
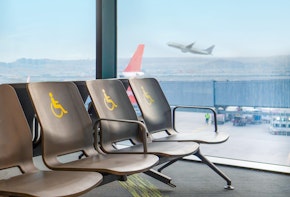
Americans Agree: Air Travel Should Be More Accessible for Disabled Passengers
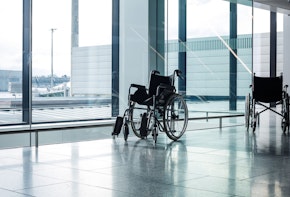
Trips Not Taken, Money Not Made: Inaccessible Air Travel Hurts Disabled Travelers and Airlines Alike
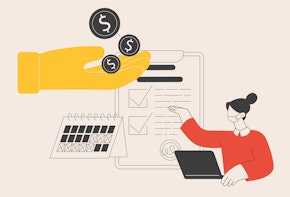
States Still Challenged in Making Timely Unemployment Payments to Laid Off Workers
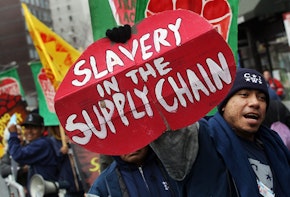
Creating a Stronger Voice for Workers
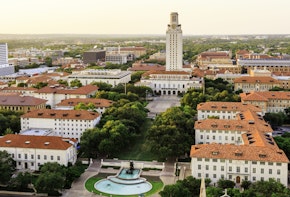
Anti-DEI Bill in Texas Is a Warning for Other States
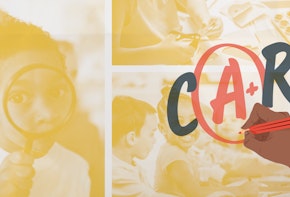
Care Matters: A 2024 Report Card for Policies in the States
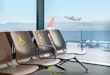
Will the US unemployment rate continue at historic lows?
US job growth has continued at a steady clip in the months since the COVID-19 pandemic, but experts are looking for warning signs.

All eyes will be on Friday’s US unemployment numbers to see how many jobs were added in March and whether the unemployment rate continues to stay in its historically low range or if it is time for the alarm bells to start ringing.
Job growth in the United States has continued at a steady clip in the months since the early days of the COVID-19 pandemic, when businesses came to a sudden stop.
Keep reading
India’s electoral bonds laundry: ‘corrupt’ firms paid parties, got cleansed, the take: layoffs are decimating the media industry. who profits, are modi’s economic policies benefitting all indians, diplomatic spat erupts between poland and israel after wck killings in gaza.
“In the aftermath of the pandemic as things started to pick back up, there was a real struggle to find people to work and companies had to raise how much you paid to get people,” said Matt Colyar, an economist at Moody’s Analytics.
There are a number of factors behind that, including restrictions on the number of foreigners entering the country during the COVID-19 pandemic and baby boomers dropping out of the workforce for fear of the pandemic, creating nearly a shortage of some two million workers aged 55 and older .
As business ground to a halt as a result of the pandemic, nearly 22 million jobs were lost. A lot of the hiring since then has been about refilling those roles, said Dan North, senior economist at Allianz Trade, adding: “It’s not like those jobs went away.”
Since the start of the pandemic, the US economy has lost 21,888,000 jobs and has added 27,387,000, according to government data. “You could argue that the economy has created only 5,499,000 new jobs,” said North.
But jobs are being created, nonetheless. While employment fell by 243,000 jobs in December 2020, following seven consecutive months of increases, the labour market has consistently added jobs each month since then, taking the US economy on a 38-month streak of monthly job gains.
If payroll employment is shown to have risen in March in Friday’s monthly jobs report, which is released at 8:30am local (12:30 GMT), then it will be a 39-month streak.
Healthcare and state sector driving jobs
While jobs in the leisure and hospitality sectors are still catching up to pre-pandemic levels, two sectors that are driving job growth are healthcare and state and local government, experts said.
“Healthcare in the US has always been under-supplied in terms of labour so a strong growth in that sector is a good thing,” said Bernard Yaros, lead US economist at Oxford Economics. “Our hospitals and health clinics should be fully staffed, especially given an ageing population.”
Hiring for government jobs is still focused on filling jobs that were lost during the pandemic, said Yaros. That sector was a late starter because of the government’s inability to match private sector salaries in order to attract talent, he said. But now that hiring is slowing down in the private sector, jobs in the state sector have seen solid growth, he added.
A lot of the hiring is also being driven by a rebound in immigration since 2023 – both legal and undocumented – that has allowed the economy to continue adding more than 200,000 jobs a month, said Yaros.
“When there’s an increase in labour supply through immigration, it allows for strong growth. But that doesn’t lead to inflation because you have more people looking for work so employers don’t have to raise wages [as much] to attract workers”, Yaros said.
However, hiring in most other sectors remains volatile and mixed, he added.
‘Starting to see some disruption’
“Underneath the shiny headlines, we are starting to see some disruption,” said North.
On Tuesday, the Job Openings and Labor Turnover Survey, or JOLTS report, from the US Department of Labor showed there were 1.36 vacancies for every unemployed person in February, down from 1.43 in January. The decline indicates a rise in unemployment.
According to the data, layoffs reached 1.7 million in February, up from 1.6 million in January. Job openings are down 11 percent year-on-year and job quits – the number of workers resigning from their jobs, likely for better opportunities, said North – have returned to pre-COVID levels, indicating that wage increases will not be as fast-paced or high as they have been.
Unemployment numbers, while still at historic lows, are slowly starting to creep up, hitting 3.9 percent last month, up from 3.7 percent for each of the three months prior.
While the unemployment rate has been below 4 percent for just over two years in a row – the longest such stretch since the late 1960s – the mood is starting to change. In a March consumer confidence survey by The Conference Board, consumers said that jobs are harder to get and that they expect their incomes to decrease over the next six months.
The question now is if, or when, unemployment numbers will break through 4 percent.
“If it goes up to 4.1 percent next month, everyone will start talking about the Sahm rule,” said North, referring to former Federal Reserve economist Claudia Sahm, who invented a measure that examines how fast the unemployment rate is rising to determine if it is an indication of a recession.
While most economists agree that the chances of the US economy slipping into a recession have receded, a rise in the unemployment rate will slow down economic growth.
All of this feeds into decisions that the Fed will have to take on whether to cut interest rates, and how quickly. The benchmark overnight interest rate is in the 5.25 percent to 5.5 percent range, where it has been since July to curb a 40-year high inflation spike. While inflation has come down since then and is hovering around 3.2 percent as of the end of February, the latest data available, that’s still higher than the Fed target of 2 percent.
In such a scenario, a robust job market – and a healthy spending ability alongside – will have the Fed looking for signs of a rise in inflation, delaying interest cuts.
But a slowdown in hiring – and a rise in unemployment, ultimately – could bring the prospect of interest rate cuts. The data on Friday will offer some clues.
Euro zone inflation unexpectedly eases, boosting rate cut case
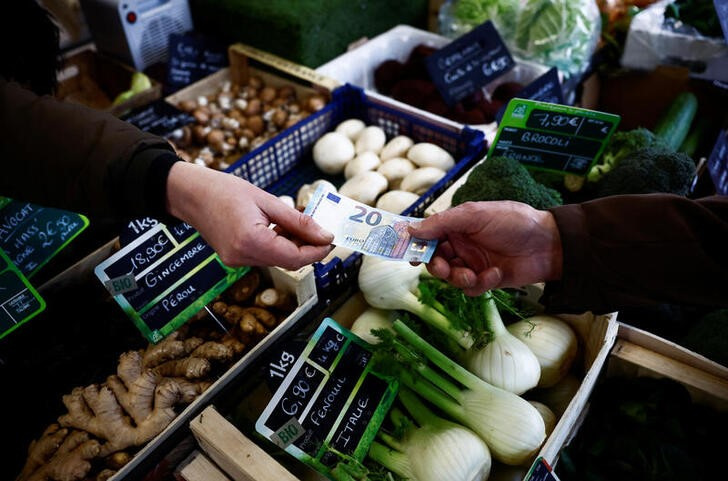
Get a look at the day ahead in European and global markets with the Morning Bid Europe newsletter. Sign up here.
Reporting by Balazs Koranyi; Editing by Toby Chopra
Our Standards: The Thomson Reuters Trust Principles. , opens new tab
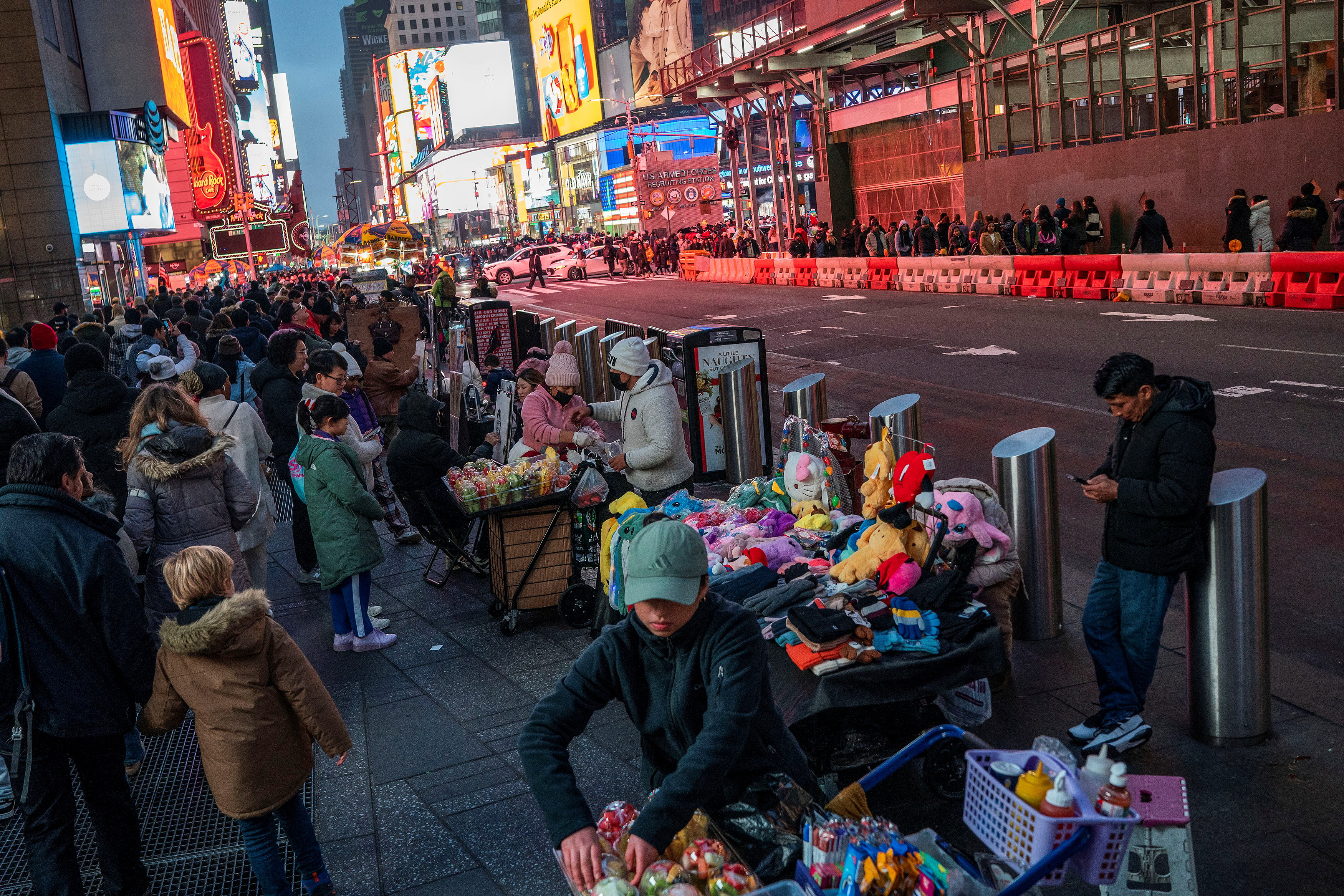
Hot U.S. jobs data tempers June Fed rate cut bets
Stocks on Wall Street rallied on Friday as the dollar and bond yields rose after another blowout U.S. jobs report suggested the Federal Reserve may delay cutting interest rates while it awaits further inflation data.
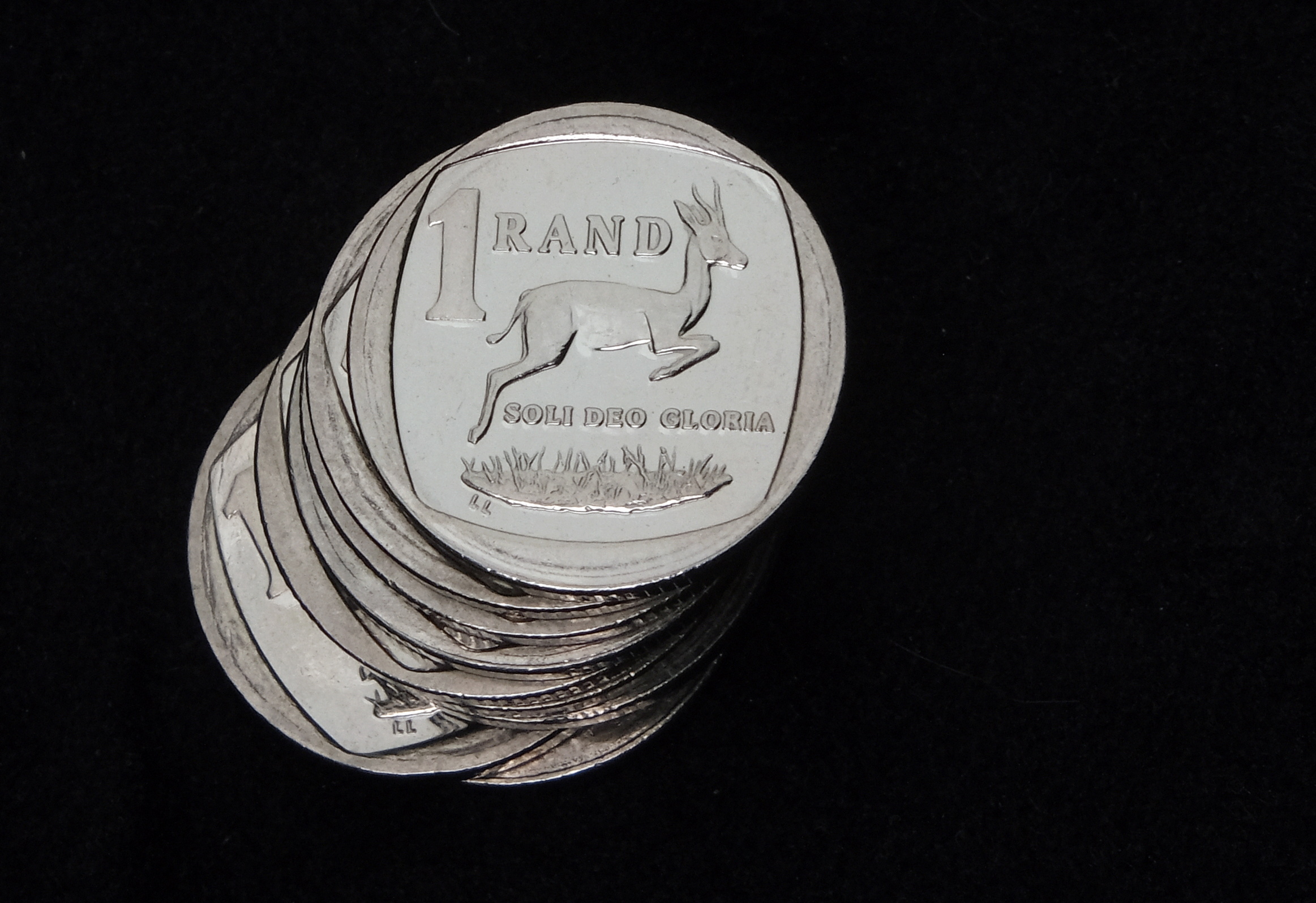
Small Study Suggests Ozempic Relative May Slow Parkinson's
Small Study Suggests Ozempic Relative May Slow Parkinson's
By Robin Foster HealthDay Reporter

THURSDAY, April 4, 2024 (HealthDay News) -- Could a medication similar to the blockbuster weight-loss drugs Ozempic and Wegovy slow the ravages of Parkinson's disease?
A new, small study suggests it could: Over the course of a year, a group of French researchers followed 156 people with early Parkinson’s who were randomly given lixisenatide, a GLP-1 receptor agonist made by Sanofi, or a placebo.
What did they discover? Parkinson’s symptoms like tremor, stiffness, slowness and balance got worse in those taking the placebo but not in those taking the drug.
Experts said the findings are a good starting point for future research on the drug's powers against the movement disorder.
U.S. Cities With the Most Homelessness

It is not a slam dunk, but it is “nibbling at the edges of disease modification,” Dr. Michael Okun , a Parkinson’s disease expert at the University of Florida who was not part of the study, told the New York Times.
Dr. Hyun Joo Chu , from the National Institute of Neurological Disorders and Stroke, said the study was “very important,” but she cautioned the early research was only designed to test a hypothesis.
“There are many, many examples of very promising Phase 2 trials,” she told the Times. “People get very excited, and then it doesn’t pan out.”
Not only that, but more than half of the patients suffered from nausea and vomiting, possibly because the researchers started with the highest dose instead of gradually increasing the dosage. When a third of patients had side effects that became intolerable, the investigators halved their dose.
For the researchers, led by Dr. Wassilios Meissner of the University of Bordeaux and Dr. Olivier Rascol of the University of Toulouse, it wasn't that far-fetched to think a GLP-1 drug might slow Parkinson’s.
Studies have found that people with type 2 diabetes are at increased risk for Parkinson’s disease, Rascol told the Times . But that increased risk drops in those who take a GLP-1 drug to treat their diabetes.
He added that studies of brain tissue from deceased Parkinson’s patients have revealed abnormalities related to insulin resistance, which is what GLP-1 drugs treat.
While the researchers said they want to do a larger and longer study, Sanofi withdrew the drug in the United States and has started withdrawing it worldwide. The move was made for business reasons, a company spokesman told the Times .
More information
The National Institute on Aging has more on Parkinson's disease .
SOURCE: New England Journal of Medicine , April 4, 2024; New York Times
Copyright © 2024 HealthDay . All rights reserved.
Join the Conversation
Tags: Parkinson's disease
America 2024

Health News Bulletin
Stay informed on the latest news on health and COVID-19 from the editors at U.S. News & World Report.
Sign in to manage your newsletters »
Sign up to receive the latest updates from U.S News & World Report and our trusted partners and sponsors. By clicking submit, you are agreeing to our Terms and Conditions & Privacy Policy .
You May Also Like
The 10 worst presidents.
U.S. News Staff Feb. 23, 2024

Cartoons on President Donald Trump
Feb. 1, 2017, at 1:24 p.m.

Photos: Obama Behind the Scenes
April 8, 2022

Photos: Who Supports Joe Biden?
March 11, 2020
The Dark Clouds Looming Over the Eclipse
Cecelia Smith-Schoenwalder April 5, 2024

Blowout: Jobs Gains Defy Expectations
Tim Smart April 5, 2024

‘Unity Ticket’ a No-Go for No Labels
Cecelia Smith-Schoenwalder April 4, 2024

Biden Ramps Up Pressure on Israel

Powell: Rate Cuts Still Likely in 2024
Tim Smart April 3, 2024

EXPLAINER: Rare Human Case of Bird Flu
Cecelia Smith-Schoenwalder April 3, 2024

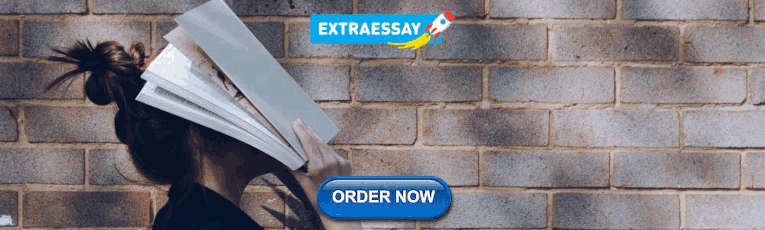
IMAGES
COMMENTS
They found that in the stores that experienced a minimum wage hike, workers on average worked fewer hours per week, were less likely to qualify for benefits, and had less-consistent schedules ...
In April 2020, the unemployment rate reached 14.8%—the highest rate observed since data collection began in 1948. In July 2021, unemployment remained higher (5.4%) than it had been in
Shown are pre-COVID-19 unemployment rates as of August 2019 (Fig. 2 a), followed by May 2020 (Fig. 2 b) where even the lowest levels of unemployment exceed the highest rates of the pre-pandemic period even in wealthy counties around Nashville (seen in the legend entries), August 2020 (Fig. 2 c), and September 2020 (Fig. 2 d). The overall ...
The stigma of long-term unemployment can be profound and long-lasting. As the United States eases out of the Covid-19 pandemic, it needs better approaches to LTU compared to the Great Recession.
of the unemployment rate since then has puzzled many. Despite high numbers of weekly initial claims, the unemployment rate started to decline rather quickly and had declined by 7 percentage points, to 6.7 percent, in December 2020. Figure 1 presents the actual path of the unemployment rate and monthly consensus expectations over time since the ...
Moreover, this effect is larger the lower the local unemployment rate was at the time of past unemployment episodes, confirming the stigmatization effect on US workers. ... The expected r varies from − 0.004 to − 0.056 in the case of studies focused on employment outcomes, and from − 0.006 to − 0.071 when the labor market outcome ...
The analysis uncovers and compares between two competing effects of growth on unemployment. The first is a capitalisation effect, whereby an increase in growth raises the capitalised returns from creating jobs and consequently reduces the equilibrium rate of unemployment. The second is a creative destruction effect whereby an increase in growth ...
A replication case study of forecasting real GDP, the unemployment rate and the impact of leading economic indicators John Guerard1*, Dimitrios Thomakos2 and Foteini Kyriazi2 Abstract: We test and report on time series modelling and forecasting using several US. Leading economic indicators (LEI) as an input to forecasting real US. GDP and
UNEMPLOYMENT AND ITS EFFECTS ON ECONOMY: A CASE STUDY Kayode Oluwadamilare BANKOLE MSc Student, Department of Accounting, School of Economics, University of Ibadan, Ibadan, Nigeria. ... George & Oseni (2012) studied the impact of electricity power on unemployment rates in Nigeria. The study covers the period of 1970 to 2005. They found that ...
In 2010, after the Great Recession had officially ended, the average annual unemployment rate for black workers was 16.0 percent, compared to 12.5 and 8.7 percent for Hispanics and whites ...
Case study No. 1: Providing unemployment insurance and 13 non-contributory income protection for all in Coresia ... In 2015, the unemployment rate stood at 4.7 per cent, but time-related underemployment was estimated at 10.8 per cent, especially among the rural youth. Rural
Automatic time series modelling of the unemployment rate using leading economic indicators (LEI) Another widely studied time series is the (US) unemployment rate. Montgomery, Zarnowitz, Tsay and Tiao (Montgomery et al., Citation 1998) modelled the quarterly unemployment rate for the 1948 to 1993 period and reached several very interesting ...
Referring to the Department of Statistic Malaysia (DOSM), the unemployment rate in Malaysia for May rose to 5.3%, and 826,100 Malaysians were unemployed that month. Losing a job can easily make people lose the will to live. ... The case study for Sabah was examined and compared with the situation at the national and international levels.
Unemployment is today considered perhaps the most serious of the problems afflicting less developed countries and one that is believed to be steadily worsening as the gap between the ... A Case Study of a Poor District of Tehran ... or from 4-5 per cent to 4-9 per cent. Rates for the urban areas of Chile in 1968, Panama in 1963-4 ...
Following the Box-Jenkins method, the formulated model for forecasting the unemployment rate is SARIMA (6, 1, 5) × (0, 1, 1)4 with a coefficient of determination of 0.79.
India is an interesting case study with one of the lowest female labour force participation rates (LFPRs) globally to analyse how the COVID-19 pandemic exacerbated the pre-existing gender disparities in unemployment. According to the World Bank data, India's female LFPRs was approximately 21% in 2019, the lowest among the BRICS nations ...
The most commonly cited indicator of business cycles, the unemployment rate, rose from 3.2% of the labor force in 1929 to 24.9% in 1933. Unemployment fell to 14.3% in 1937, but then increased again to 19.0% in 1938 and was still 9.9% in 1941 before the military boom associated with World War II brought it down to 1.9% in 1943.
Unemployment hysteresis, where joblessness persists even after economic improvement, reveals the lasting impact of initial downturns. We delve into this puzzle in Morocco from 1999Q1 to 2019Q3, using four methods to examine the non-accelerating inflation rate of unemployment (NAIRU) time series for unit root causes.
The unemployment rate for construction workers was 5.4% in March — higher than the national average but down from 5.6% a year ago. Overall, U.S. employers added 303,000 jobs in March ...
Three Different Case Study States: Population: Unemployment Rate. 2018: Major Industries: Maine: 1.34 million (ranking 42nd in population nationwide) 3.24%: ... Participation in focus groups was limited to individuals who had filed for unemployment insurance in a case study state post-modernization. Participants did not have to have received ...
This study examines the non-linear effects of artificial intelligence on unemployment in both developed countries and developing markets over the period 2000-to 2019. The paper uses a panel smooth transition regression (PSTR) model with individual-specific effects. Key findings from this paper can be summarised below.
The U.S. labor market is having a strong start to 2023, adding 504,000 nonfarm payrolls in January, and 311,000 in February. Both figures surpassed analyst expectations by a wide margin, and in January, the unemployment rate hit a 53-year low of 3.4%. With the recent release of February's numbers, unemployment is now reported at a slightly ...
It is a persistent problem that has various. social and economic consequences, including poverty, crime, and inequality. According to the latest available data from the National Statistical Office ...
Unemployment numbers, while still at historic lows, are slowly starting to creep up, hitting 3.9 percent last month, up from 3.7 percent for each of the three months prior. While the unemployment ...
April 4, 2024 10:36 am. . The number of new applications for unemployment benefits unexpectedly increased by 9,000 to 212,000 last week, the highest level since January. The reading marks the ...
Euro zone inflation unexpectedly eases, boosting rate cut case. By Balazs Koranyi. April 3, 2024 9:00 AM UTC Updated ago. ... Still, unemployment is holding at a record low 6.5%, separate Eurostat ...
The study involved married couples age 60 and older without younger people living in the home. Using saliva samples and questionnaires, rates of illness were tracked over two fall-winter periods ...
The only previous case of bird flu in a person occurred in Colorado in 2022 in someone who was directly exposed to poultry. Cecelia Smith-Schoenwalder April 3, 2024 Key Takeaways From 4 Primaries