data acquisition system Recently Published Documents
Total documents.
- Latest Documents
- Most Cited Documents
- Contributed Authors
- Related Sources
- Related Keywords
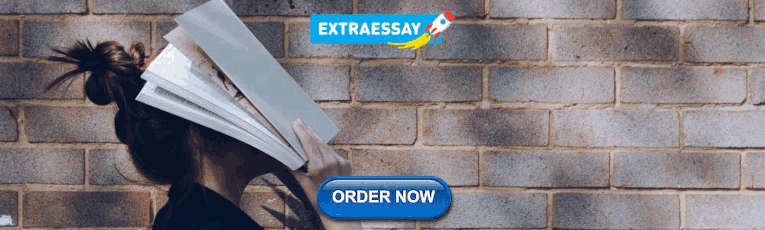
Automation of monitoring of drying parameters in hybrid solar-electric dryer for agricultural products
ABSTRACT Increasing the efficiency of solar dryers with ensuring that the system remains accessible to all users can be achieved with their automation through low-cost and easy-to-use technique sensors. The objective was to develop, implement and evaluate an automatic system for monitoring drying parameters in a hybrid solar-electric dryer (HSED). Initially, an automated data acquisition system for collecting the parameters of sample mass, air temperature, and relative air humidity was developed and installed. The automatic mass data acquisition system was calibrated in the hybrid solar-electric dryer. The automated system was validated by comparing it with conventional devices for measuring the parameters under study. The data obtained were subjected to analysis of variance, Tukey test and linear regression at p ≤ 0.05. The system to turn on/off the exhaust worked efficiently, helping to reduce the errors related to the mass measurement. The GERAR Mobile App showed easy to be used since it has intuitive icons and compatibility with the most used operating systems for mobile devices. The responses in communication via Bluetooth were fast. The use of Arduino, a low-cost microcontroller, to automate the monitoring activity allowed estimating the mass of the product and collecting the drying air temperature and relative air humidity data through the DHT22. This sensor showed a good correlation of mass and air temperature readings between the automatic and conventional system, but low correlation for relative air humidity. In general, the automatic data acquisition system monitored in real time the parameters for drying agricultural products in the HSED.
A Low-Cost Multi-Sensor Data Acquisition System for Fault Detection in Fused Deposition Modelling
Fused deposition modelling (FDM)-based 3D printing is a trending technology in the era of Industry 4.0 that manufactures products in layer-by-layer form. It shows remarkable benefits such as rapid prototyping, cost-effectiveness, flexibility, and a sustainable manufacturing approach. Along with such advantages, a few defects occur in FDM products during the printing stage. Diagnosing defects occurring during 3D printing is a challenging task. Proper data acquisition and monitoring systems need to be developed for effective fault diagnosis. In this paper, the authors proposed a low-cost multi-sensor data acquisition system (DAQ) for detecting various faults in 3D printed products. The data acquisition system was developed using an Arduino micro-controller that collects real-time multi-sensor signals using vibration, current, and sound sensors. The different types of fault conditions are referred to introduce various defects in 3D products to analyze the effect of the fault conditions on the captured sensor data. Time and frequency domain analyses were performed on captured data to create feature vectors by selecting the chi-square method, and the most significant features were selected to train the CNN model. The K-means cluster algorithm was used for data clustering purposes, and the bell curve or normal distribution curve was used to define individual sensor threshold values under normal conditions. The CNN model was used to classify the normal and fault condition data, which gave an accuracy of around 94%, by evaluating the model performance based on recall, precision, and F1 score.
Linear Array Photodiode and Data Acquisition System Development for Multi-Point Line FLDI Measurements
Progress in hxr diagnostics at golem and compass tokamaks.
Abstract Scintillation detectors are widely used for hard X-ray spectroscopy and allow us to investigate the dynamics of runaway electrons in tokamaks. This diagnostic tool proved to be able to provide information about the energy or the number of runaway electrons. Presently it has been used for runaway studies at the GOLEM and the COMPASS tokamaks. The set of scintillation detectors used at both tokamaks was significantly extended and improved. Besides NaI(Tl) (2 × 2 inch) scintillation detectors, YAP(Ce) and CeBr3 were employed. The data acquisition system was accordingly improved and the data from scintillation detectors is collected with appropriate sampling rate (≈300 MHz) and sufficient bandwidth (≈100 MHz) to allow a pulse analysis. Up to five detectors can currently simultaneously monitor hard X-ray radiation at the GOLEM. The same scintillation detectors were also installed during the runaway electron campaign at the COMPASS tokamak. The aim of this contribution is to report progress in diagnostics of HXR radiation induced by runaway electrons at the GOLEM and the COMPASS tokamaks. The data collected during the 12th runaway electron campaign (2020) at COMPASS shows that count rates during typical low-density runaway electron discharges are in a range of hundreds of kHz and detected photon energies go up to 10 MeV (measured outside the tokamak hall). Acquired data from experimental campaigns from both machines will be discussed.
Motorsport Data Acquisition System and Live Telemetry using FPGA based CAN controller
Abstract The paper describes the design and working of a motorsport data acquisition, logging, live telemetry, and display system developed using the Controller Area Network (CAN) communication protocol as the backbone of the arrangement. The main controller of the CAN system is the myRIO which was programmed using LabVIEW. A Formula One car hosts over a hundred sensors during each of its races. The data acquisition/logging system, although does not directly affect the car’s performance, is indispensable when it comes to the testing and design phase of the car. Designers can validate their assumptions and calculations, real-time data during testing can be a safety indicator and it provides insight to the driver about the performance of the vehicle. The FPGA-based controller for CAN is designed for data acquisition and live telemetry system with the interest of the formula car team in mind. The design choices were made to improve and deliver a more effective system than the pre-existing ones. All choices of controllers, sensors, formatting were custom made for the requirements of the team. All programmable devices were coded individually to suit the system and the graphical user interface was designed internally. Data acquired by the proposed system helps in making sure that the car achieves the goals that were envisioned when it was designed.
The Data Acquisition System for the ATLAS Tile Calorimeter Phase-II Upgrade Demonstrator
Development of a data acquisition system for monitoring environmental parameters of laboratories, development of distributed data acquisition system, design and implementation of the farm-level data acquisition system for the behavior analysis of livestocks, computerised calibration system for radiosonde pressure sensors.
A computer bast1d data acquisition system has been designed for the calibration of pressure sensors used in MK III Indian radiosondes. The system can calibrate 64 baroswitchesin one cycle and gives a tabular printout of pressure values at various contact numbers. This replaces the analog type of recording system, which was in use for the last few decades. The present system gives better accuracy and resolution and avoids human judgement and errors.
Export Citation Format
Share document.
Thank you for visiting nature.com. You are using a browser version with limited support for CSS. To obtain the best experience, we recommend you use a more up to date browser (or turn off compatibility mode in Internet Explorer). In the meantime, to ensure continued support, we are displaying the site without styles and JavaScript.
- View all journals
Data acquisition articles from across Nature Portfolio
Data acquisition is the process of converting measurements, such as temperature, pressure, relative humidity, light, resistance, current, power, speed and vibration, into digital numeric values that can be manipulated by a computer. During data acquisition physical measurements are converted into a voltage that is in turn transformed into a binary number by a digital-to-analog converter.
Latest Research and Reviews
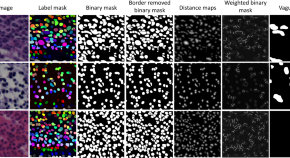
NuInsSeg: A fully annotated dataset for nuclei instance segmentation in H&E-stained histological images
- Amirreza Mahbod
- Christine Polak
- Isabella Ellinger
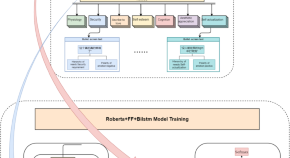
Sentiment analysis of video danmakus based on MIBE-RoBERTa-FF-BiLSTM
- Jianbo Zhao
- Huailiang Liu
- Shanzhuang Zhang
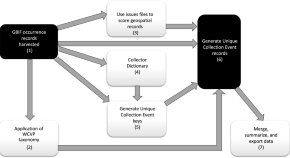
A new R package to parse plant species occurrence records into unique collection events efficiently reduces data redundancy
- Pablo Hendrigo Alves de Melo
- Nadia Bystriakova
- Alexandre K. Monro
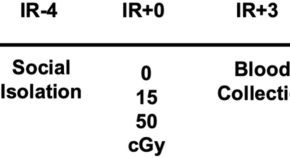
Sexual dimorphism during integrative endocrine and immune responses to ionizing radiation in mice
- Marissa Burke
- Amber M. Paul
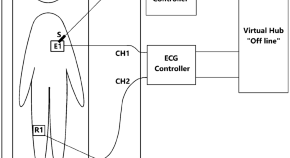
Synchronous recording of magnetocardiographic and electrocardiographic signals
- Kazimierz Pȩczalski
- Judyta Sobiech
- Maryla Zajdel
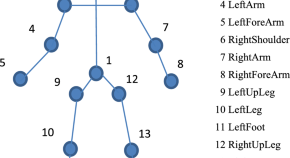
Table tennis motion recognition based on the bat trajectory using varying-length-input convolution neural networks
- Yuanshi Ren
News and Comment
Artificial intelligence can improve patients’ experience in decentralized clinical trials.
- Kevin A. Thomas
- Łukasz Kidziński
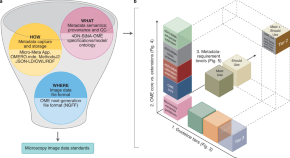
Towards community-driven metadata standards for light microscopy: tiered specifications extending the OME model
Rigorous record-keeping and quality control are required to ensure the quality, reproducibility and value of imaging data. The 4DN Initiative and BINA here propose light Microscopy Metadata Specifications that extend the OME Data Model, scale with experimental intent and complexity, and make it possible for scientists to create comprehensive records of imaging experiments.
- Mathias Hammer
- Maximiliaan Huisman
- Caterina Strambio-De-Castillia
Efficient cellular annotation of histopathology slides with real-time AI augmentation
In recent years, a steady swell of biological image data has driven rapid progress in healthcare applications of computer vision and machine learning. To make sense of this data, scientists often rely on detailed annotations from domain experts for training artificial intelligence (AI) algorithms. The time-consuming and costly process of collecting annotations presents a sizable bottleneck for AI research and development. HALS (Human-Augmenting Labeling System) is a collaborative human-AI labeling workflow that uses an iterative “review-and-revise” model to improve the efficiency of this critical process in computational pathology.
- James A. Diao
- Richard J. Chen
- Joseph C. Kvedar
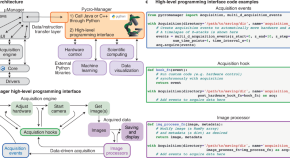
Pycro-Manager: open-source software for customized and reproducible microscope control
- Henry Pinkard
- Nico Stuurman
- Laura Waller
Data curation during a pandemic and lessons learned from COVID-19
Detailed, accurate data related to a disease outbreak enable informed public health decision making. Given the variety of data types available across different regions, global data curation and standardization efforts are essential to guarantee rapid data integration and dissemination in times of a pandemic.
- Moritz U. G. Kraemer
- Samuel V. Scarpino
- John S. Brownstein
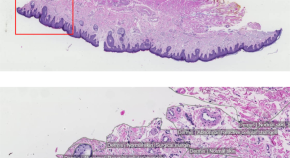
Key insights in the AIDA community policy on sharing of clinical imaging data for research in Sweden
Development of world-class artificial intelligence (AI) for medical imaging requires access to massive amounts of training data from clinical sources, but effective data sharing is often hindered by uncertainty regarding data protection. We describe an initiative to reduce this uncertainty through a policy describing a national community consensus on sound data sharing practices.
- Joel Hedlund
- Anders Eklund
- Claes Lundström
Quick links
- Explore articles by subject
- Guide to authors
- Editorial policies

Advertisement
A Sensor Data Acquisition System for Smart Agriculture
- Original Research
- Published: 30 August 2023
- Volume 4 , article number 667 , ( 2023 )
Cite this article
- Disha Garg 1 &
- Mansaf Alam ORCID: orcid.org/0000-0002-6293-7005 1
171 Accesses
Explore all metrics
The agricultural economy of India depends heavily on agricultural production for its economic structure. Crop monitoring and timely interventions can be critical in improving agriculture outcomes. Because of the criticality of the agriculture domain and the relevance of analytical solutions for improving its operational effectiveness, this research paper presents a sensor data acquisition framework for acquiring data from sensors mounted on the robot which is an unmanned aerial vehicles (UAVs) technology. The proposed work uses Internet of Things (IoT)-based sensors for data acquisition by deploying robot-mounted sensors on crop fields. These robots traverse the crop and collect real-time parameters, i.e., temperature, humidity, moisture, and bacteria, which are then pushed to the cloud and subsequently used for analysis. The dataset is analyzed using exploratory analysis to determine the statistical values of the observed parameters. The results of the analysis are published on the Twitter platform using ThingSpeak which is an IoT analytics service that allows us to aggregate, visualize, and analyze live data streams in the cloud. The obtained results demonstrate the ability of the system and its possibility to be utilized as a commercial agriculture practice for farmers/users.
This is a preview of subscription content, log in via an institution to check access.
Access this article
Price includes VAT (Russian Federation)
Instant access to the full article PDF.
Rent this article via DeepDyve
Institutional subscriptions
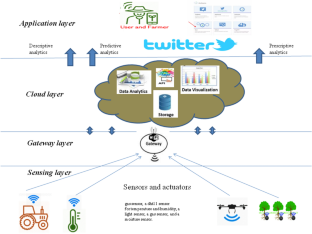
Similar content being viewed by others
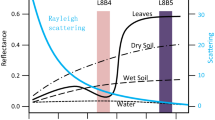
A commentary review on the use of normalized difference vegetation index (NDVI) in the era of popular remote sensing
Sha Huang, Lina Tang, … Guofan Shao
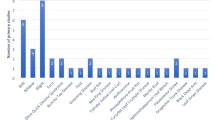
Plant disease detection using drones in precision agriculture
Ruben Chin, Cagatay Catal & Ayalew Kassahun
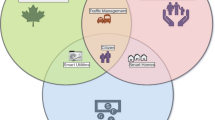
Smart cities: the role of Internet of Things and machine learning in realizing a data-centric smart environment
Amin Ullah, Syed Myhammad Anwar, … Tanzila Saba
Code or Data Availability
The data and code used in this study are available on request from the corresponding author.
Rajeswari S, Suthendran K, Rajakumar K. A smart agricultural model by integrating IoT, mobile and cloud-based big data analytics. In: Proceedings of 2017 International Conference on Intelligent Computing and Control I2C2 2017. IEEE, 2018. p. 1–5.
Khanna A, Kaur S. Evolution of internet of things (IoT) and its significant impact in the field of precision agriculture. Comput Electron Agric. 2019;157:218–31.
Article Google Scholar
Ruan J, et al. A life cycle framework of green IoT-based agriculture and its finance, operation, and management issues. IEEE Commun Mag. 2019;57(3):90–6.
Bu F, Wang X. A smart agriculture IoT system based on deep reinforcement learning. Futur Gener Comput Syst. 2019;99:500–7.
Tzounis A, Katsoulas N, Bartzanas T, Kittas C. Internet of Things in agriculture, recent advances and future challenges. Biosyst Eng. 2017;164:31–48.
Roopaei M, Rad P, Choo KKR. Cloud of things in smart agriculture: Intelligent irrigation monitoring by thermal imaging. IEEE Cloud Computing. 2017;4(1):10–5.
Freeman PK, Freeland RS. Agricultural UAVs in the U.S.: potential, policy, and hype. Remote Sens Appl Soc Environ. 2015;2:35–43.
Google Scholar
Barry T. Drones over homeland: expansion of scope and lag in governance. Brown J World Aff. 2013;19(2):65–80.
Wang N, Suomalainen J, Bartholomeus H, Kooistra L, Masiliūnas D, Clevers JGPW. Diurnal variation of sun-induced chlorophyll fluorescence of agricultural crops observed from a point-based spectrometer on a UAV. Int J Appl Earth Obs Geoinf. 2021;96: 102276.
Zamora-Izquierdo MA, Santa J, Martínez JA, Martínez V, Skarmeta AF. Smart farming IoT platform based on edge and cloud computing. Biosys Eng. 2019;177:4–17.
Khan S, Liu X, Shakil KA, Alam M. Big data technology-enabled analytical solution for quality assessment of higher education systems. Int J Adv Comput Sci Appl. 2019;10(6):292–304.
Garg D, Khan S, Alam M. Integrative use of iot and deep learning for agricultural applications. Lect Notes Electr Eng. 2020;605:521–31.
Tseng FH, Cho HH, Te Wu H. Applying big data for intelligent agriculture-based crop selection analysis. IEEE Access. 2019;7:116965–74.
Jinbo C, Xiangliang C, Han-Chi F, Lam A. Agricultural product monitoring system supported by cloud computing. Clust Comput. 2019;22(4):8929–38.
Gill SS, Buyya R, Chana I. IoT based agriculture as a cloud and big data service: The beginning of digital India. J Organ End User Comput. 2017;29(4):1–23.
Alonso RS, Sittón-Candanedo I, García Ó, Prieto J, Rodríguez-González S. An intelligent Edge-IoT platform for monitoring livestock and crops in a dairy farming scenario. Ad Hoc Netw. 2020;98:102047.
Adafruit. The Magic of NeoPixels | AdafruitNeoPixelÜberguide | Adafruit Learning System. https://learn.adafruit.com/adafruit-neopixel-uberguide . Accessed 25 Mar 2022
Simulink. MATLAB-MathWorks-MATLAB&Simulink. https://www.mathworks.com/products/matlab.html . Accessed 25 Mar 2022
Yang J, Sharma A, Kumar R. IoT-based framework for smart agriculture. Int J Agric Environ Inform Syst. 2021;12(2):1–14.
Bhojwani Y, Singh R, Reddy R, Perumal B. Crop selection and IoT based monitoring system for precision agriculture. In: 2020 International Conference on Emerging Trends in Information Technology and Engineering (ic-ETITE). IEEE; 2020. p. 1–11.
Ramachandran V, Ramalakshmi R, Srinivasan S. An automated irrigation system for smart agriculture using the internet of things. In: 2018 15th International conference on control, automation, robotics and vision (ICARCV). IEEE; 2018. p. 210–5.
Chapter Google Scholar
Ramaprasad SS, Kumar BS, Lebaka S, Prasad PR, Kumar KS, Manohar GN. Intelligent Crop Monitoring and Protection System in Agricultural fields Using IoT. In: 2019 4th International Conference on Recent Trends on Electronics, Information, Communication & Technology (RTEICT). IEEE; 2019. p. 1527–31.
Heble S, Kumar A, Prasad KVD, Samirana S, Rajalakshmi P, Desai UB. A low power IoT network for smart agriculture. In: 2018 IEEE 4th World Forum on Internet of Things (WF-IoT). IEEE; 2018. p. 609–14.
Reche A, Sendra S, Díaz JR, Lloret J. A smart M2M deployment to control the agriculture irrigation. In: Ad-hoc Networks and Wireless: ADHOC-NOW 2014 International Workshops, ETSD, MARSS, MWaoN, SecAN, SSPA, and WiSARN, Benidorm, Spain, June 22–27, 2014, Revised Selected Papers 13. Berlin Heidelberg: Springer; 2015. p. 139–51.
Chaudhary DD, Nayse SP, Waghmare LM. Application of wireless sensor networks for greenhouse parameter control in precision agriculture. Int J Wirel Mobile Netw. 2011;3(1):140–9.
Liqiang Z, Shouyi Y, Leibo L, Zhen Z, Shaojun W. A crop monitoring system based on wireless sensor network. Procedia Environ Sci. 2011;11:558–65.
Kaur G, Upadhyaya P, Chawla P. Comparative analysis of IoT-based controlled environment and uncontrolled environment plant growth monitoring system for hydroponic indoor vertical farm. Environ Res. 2023;222:115313.
Koteswara Rao M, Satish Kumar M, Jaijaivenkataramana M, SaiSowjanya C. ESP32 based irrigation system. In: Intelligent cyber physical systems and internet of things: ICoICI 2022. Cham: Springer International Publishing; 2023. p. 465–74.
Dorthi K, Narasimha Reddy S, Pitta S. Smart water management system in agriculture using internet of things. In: Smart Intelligent Computing and Applications, Volume 2: Proceedings of Fifth International Conference on Smart Computing and Informatics (SCI 2021). Singapore: Springer Nature Singapore; 2022. p. 235–41.
Garg D, Alam M. Smart agriculture: a literature review. J Manag Anal. 2023;10:1–57.
Shah SIH, Peristeras V, Magnisalis I. DaLiF: a data lifecycle framework for data-driven governments. J Big Data. 2021;8(1):1–44.
Obasanya TD, Oluwafemi IB, Bello OO, Lawal TA. An internet of things-based irrigation and tank monitoring system. Int J Inf Commun Technol. 2022;11(1):65–75.
Srilakshmi A, Geetha K, Harini D. MAIC: a proficient agricultural monitoring and alerting system using IoT in cloud platform. In: Inventive Communication and Computational Technologies: Proceedings of ICICCT 2019. Singapore: Springer; 2020. p. 805–18.
Jha, K., Doshi, A., Patel, P., & Shah, M. A comprehensive review on automation in agriculture using artificial intelligence. Artif Intell Agric. 2019;2:1–12.
Kumar KA, Aju D. An Internet of Thing based agribot (IOT-agribot) for precision agriculture and farm monitoring. Int J Educ Manag Eng. 2020;10(4):33–9.
Kaushal M, Wani SP. Nanosensors: frontiers in precision agriculture. Nanotechnol Agric Paradigm. 2017;279–291.
Mousavi SR, Rezaei M. Nanotechnology in agriculture and food production. J Appl Environ Biol Sci. 2011;1(10):414–9.
Cui S, Ling P, Zhu H, Keener HM. Plant pest detection using an artificial nose system: a review. Sensors. 2018;18(2):378.
Jijina CK, Raju G. Social media and farmers. Int J Res Eng Technol. 2016;5(19):20–5.
Mills J, Reed M, Skaalsveen K, Ingram J. The use of Twitter for knowledge exchange on sustainable soil management. Soil Use Manag. 2019;35(1):195–203.
Kushwaha HL, Sinha J, Khura T, Kushwaha DK, Ekka U, Purushottam M, Singh N. Status and scope of robotics in agriculture. Int Conf Emerg Technol Agric Food Eng. 2016;12:163.
Botta A, Cavallone P, Baglieri L, Colucci G, Tagliavini L, Quaglia G. A review of robots, perception, and tasks in precision agriculture. Appl Mech. 2022;3(3):830–54.
Vidoni R, Bietresato M, Gasparetto A, Mazzetto F. Evaluation and stability comparison of different vehicle configurations for robotic agricultural operations on side-slopes. Biosys Eng. 2015;129:197–211.
Download references
Acknowledgements
The authors wish to thank the editors and anonymous reviewers for their insightful and helpful feedback in advance.
The authors declare that no funds, grants, or other supports were received during the preparation of this manuscript.
Author information
Authors and affiliations.
Department of Computer Science, Jamia Millia Islamia, New Delhi, India
Disha Garg & Mansaf Alam
You can also search for this author in PubMed Google Scholar
Contributions
Both authors contributed to the study’s conception and design. MA: helped in the original draft, laboratory experiments, data collection, and visualization, supervision. DG: contributed to the investigation, formal analysis, editing, material preparation, and data analysis. All authors read and approved the final manuscript.
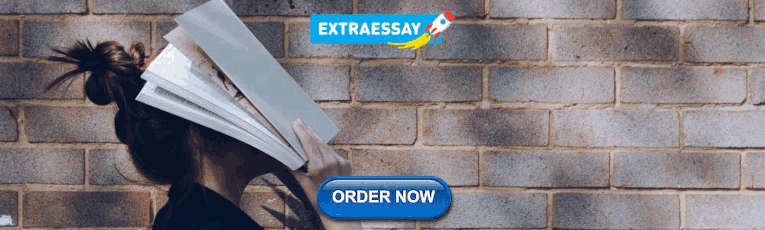
Corresponding author
Correspondence to Mansaf Alam .
Ethics declarations
Conflict of interest.
The authors have no conflicts of interest.
Additional information
Publisher's note.
Springer Nature remains neutral with regard to jurisdictional claims in published maps and institutional affiliations.
This article is part of the topical collection “Research Trends in Communication and Network Technologies” guest edited by Anshul Verma, Pradeepika Verma and Kiran Kumar Pattanaik.
Rights and permissions
Springer Nature or its licensor (e.g. a society or other partner) holds exclusive rights to this article under a publishing agreement with the author(s) or other rightsholder(s); author self-archiving of the accepted manuscript version of this article is solely governed by the terms of such publishing agreement and applicable law.
Reprints and permissions
About this article
Garg, D., Alam, M. A Sensor Data Acquisition System for Smart Agriculture. SN COMPUT. SCI. 4 , 667 (2023). https://doi.org/10.1007/s42979-023-02085-5
Download citation
Received : 25 February 2023
Accepted : 26 June 2023
Published : 30 August 2023
DOI : https://doi.org/10.1007/s42979-023-02085-5
Share this article
Anyone you share the following link with will be able to read this content:
Sorry, a shareable link is not currently available for this article.
Provided by the Springer Nature SharedIt content-sharing initiative
- Cloud computing
- Internet of things
- Smart agriculture
- Unmanned aerial vehicle (UAV)
- Find a journal
- Publish with us
- Track your research
This paper is in the following e-collection/theme issue:
Published on 29.3.2024 in Vol 26 (2024)
Usability of Health Care Price Transparency Data in the United States: Mixed Methods Study
Authors of this article:

Original Paper
- Negar Maleki 1 , PhD ;
- Balaji Padmanabhan 2 , PhD ;
- Kaushik Dutta 1 , PhD
1 School of Information Systems and Management, Muma College of Business, University of South Florida, Tampa, FL, United States
2 Decision, Operations & Information Technologies Department, Robert H. Smith School of Business, University of Maryland, College Park, MD, United States
Corresponding Author:
Negar Maleki, PhD
School of Information Systems and Management
Muma College of Business
University of South Florida
4202 E Fowler Avenue
Tampa, FL, 33620
United States
Phone: 1 8139742011
Email: [email protected]
Background: Increasing health care expenditure in the United States has put policy makers under enormous pressure to find ways to curtail costs. Starting January 1, 2021, hospitals operating in the United States were mandated to publish transparent, accessible pricing information online about the items and services in a consumer-friendly format within comprehensive machine-readable files on their websites.
Objective: The aims of this study are to analyze the available files on hospitals’ websites, answering the question—is price transparency (PT) information as provided usable for patients or for machines?—and to provide a solution.
Methods: We analyzed 39 main hospitals in Florida that have published machine-readable files on their website, including commercial carriers. We created an Excel (Microsoft) file that included those 39 hospitals along with the 4 most popular services—Current Procedural Terminology (CPT) 45380, 29827, and 70553 and Diagnosis-Related Group (DRG) 807—for the 4 most popular commercial carriers (Health Maintenance Organization [HMO] or Preferred Provider Organization [PPO] plans)—Aetna, Florida Blue, Cigna, and UnitedHealthcare. We conducted an A/B test using 67 MTurkers (randomly selected from US residents), investigating the level of awareness about PT legislation and the usability of available files. We also suggested format standardization, such as master field names using schema integration, to make machine-readable files consistent and usable for machines.
Results: The poor usability and inconsistent formats of the current PT information yielded no evidence of its usefulness for patients or its quality for machines. This indicates that the information does not meet the requirements for being consumer-friendly or machine readable as mandated by legislation. Based on the responses to the first part of the experiment (PT awareness), it was evident that participants need to be made aware of the PT legislation. However, they believe it is important to know the service price before receiving it. Based on the responses to the second part of the experiment (human usability of PT information), the average number of correct responses was not equal between the 2 groups, that is, the treatment group (mean 1.23, SD 1.30) found more correct answers than the control group (mean 2.76, SD 0.58; t 65 =6.46; P <.001; d =1.52).
Conclusions: Consistent machine-readable files across all health systems facilitate the development of tools for estimating customer out-of-pocket costs, aligning with the PT rule’s main objective—providing patients with valuable information and reducing health care expenditures.
Introduction
From 1970 to 2020, on a per capita basis, health care expenditures in the United States have increased sharply from US $353 per person to US $12,531 per person. In constant 2020 dollars, the increase was from US $1875 in 1970 to US $12,531 in 2020 [ 1 ]. The significant rise in health care expenses has put policy makers under enormous pressure to find ways to contain these expenditures. Price transparency (PT) in health care is 1 generally proposed strategy for addressing these problems [ 2 ] and has been debated for years [ 3 ]. Some economists believe that PT in health care will cut health care prices in the same way it has in other industries, while others argue that owing to the specific characteristics of the health care market, PT would not ameliorate rising health care costs. Price elasticity also does not typically apply in health care, since, if a problem gets severe, people will typically seek treatment regardless of cost, with the drawback that individuals learn of their health care costs after receiving treatment [ 4 ]. Complex billing processes, hidden insurer-provider contracts, the sheer quantity of third-party payers, and substantial quality differences in health care delivery are other unique aspects of health care that complicate the situation considerably.
The Centers for Medicare & Medicaid Services (CMS) mandated hospitals to post negotiated rates, including payer-specific negotiated costs, for 300 “shoppable services” beginning in January 2021. The list must include 70 CMS-specified services and an additional 230 services each hospital considers relevant to its patient population. Hospitals must include each third-party payer and their payer-specific fee when negotiating multiple rates for the same care. The data must be displayed simply, easily accessible (without requiring personal information from the patient), and saved in a machine-readable manner [ 5 ]. These efforts aim to facilitate informed patient decision-making, reduce out-of-pocket spending, and decrease health care expenditures. Former Secretary of Health and Human Services, Alex Azar, expressed a vision of hospital PT when declaring the new legislation “a patient-centered system that puts you in control and provides the affordability you need, the options and control you want, and the quality you deserve. Providing patients with clear, accessible information about the price of their care is a vital piece of delivering on that vision” [ 6 ].
Despite the legislation, it is not clear if people are actually engaging in using PT tools. For example, in 2007, New Hampshire’s HealthCost website was established, providing the negotiated price and out-of-pocket costs for 42 commonly used services by asking whether the patient is insured or their insurer and the zip code to post out-of-pocket costs in descending order. Mehrotra et al [ 7 ] examined this website over 3 years to understand how often and why these tools have mainly been used. Their analysis suggested that despite the growing interest in PT, approximately 1% of the state’s population used this tool. Low PT tool usage was also seen in other studies [ 8 - 10 ], suggesting that 3% to 12% of individuals who were offered the tool used it during the study period, and in all studies, the duration was at least 12 months. Thus, offering PT tools does not in itself lead to decreased total spending, since few people who have access to them use them to browse for lower-cost services [ 7 , 11 ].
In a recent paper, researchers addressed 1 possible reason for low engagement—lack of awareness. They implemented an extensive targeted online advertising campaign using Google Advertisements to increase awareness and assessed whether it increased New Hampshire’s PT website use. Their findings suggested that although lack of awareness is a possible reason for the low impact of PT tools in health care spending, structural factors might affect the use of health care information [ 12 ]. Individuals may not be able to exactly determine their out-of-pocket expenses from the information provided.
Surprisingly, there is little research on the awareness and usability of PT information after the current PT legislation went into effect. A recent study [ 13 ] highlighted the nonusability of existing machine-readable files for employers, policy makers, researchers, or consumers, and this paper adds to this literature by answering the question—is PT information as provided usable for patients or machines? Clearly, if it is of value to patients, it can be useful; the reason to take the perspective of machines was to examine whether this information as provided might also be useful for third-party programs that can extract information from the provided data (to subsequently help patients through other ways of presenting this information perhaps). We address this question through a combination of user experiments and data schema analysis. While there are recent papers that have also argued that PT data have deficiencies [ 13 , 14 ], ours is the first to combine user experiments with analysis of data schema from several hospitals in Florida to make a combined claim on value for patients and machines. We hope this can add to the discourse on PT and what needs to be done to extract value for patients and the health care system as a whole.
Impact of PT Tools
The impact of PT tools on consumers and health care facilities has been investigated in the literature. Some studies showed that consumers with access to PT tools are more likely to reduce forgone needed services over time. Moreover, consumers who use tools tend to find the lowest service prices [ 8 , 15 - 17 ]. A few studies investigated the impact of PT tools on the selection of health care facilities. They illustrated that some consumers tend to change health care facilities pursuing lower prices, while some others prefer to stay with expensive ones, although they are aware of some other facilities that offer lower prices [ 9 , 18 ]. Finally, some research studied the impact of PT tools on cost and showed that some consumers experienced no effect, while others experienced decreases in average consumer expenses [ 8 , 17 , 18 ]. However, the impact of PT tools on health care facilities is inconclusive, meaning different studies concluded different effects. Some stated that PT tools decrease the prices of imaging and laboratory services, while others said that although public charge disclosure lowers health care facility charges, the final prices remained unchanged [ 17 , 18 ].
Legislation Related Works
In a study, researchers considered 20 leading US hospitals to assess provided chargemasters to understand to what extent patients can obtain information from websites to determine the out-of-pocket costs [ 19 ]. Their findings showed that although all hospitals provided chargemasters on their websites, they rarely offered transparent information, making it hard for patients to determine out-of-pocket costs. Their analysis used advanced diagnostic imaging services to assess hospitals’ chargemasters since these are the most common services people look for. Mehrotra et al [ 7 ] also mentioned that the most common searches belonged to outpatient visits, magnetic resonance imaging (MRI), and emergency department visits. To this end, we used “MRI scan of the brain before and after contrast” as one of the shoppable services in our analysis. Another study examined imaging services in children’s hospitals (n=89), restricting the analysis to hospitals (n=35) that met PT requirements—published chargemaster rates, discounted cash prices, and payer-negotiated prices in a machine-readable file, and published costs for 300 common shoppable medical services in a consumer-friendly format. Their study revealed that, in addition to a broad range of imaging service charges, most hospitals lack the machine-readable file requirement [ 20 ].
Arvisais-Anhalt et al [ 21 ] identified 11 hospitals with available chargemasters in Dallas County to compare the prices of a wide range of available services. They observed significant variations for a laboratory test: partial thromboplastin time, a medication: 5 mg tablet of amlodipine, and a procedure: circumcision. Reddy et al [ 22 ] focus on New York State to assess the accessibility and usability of hospitals’ chargemasters from patients’ viewpoint. They found that 189 out of 202 hospitals had a locatable chargemaster on their home page. However, only 37 hospitals contain the Current Procedural Terminology (CPT) code, which makes those without the CPT code unusable due to the existence of many different descriptions for the same procedure; for example, an elective heart procedure had 34 entries. We add to this considerable literature by examining a subset of Florida hospitals.
In a competitive market, higher-quality goods and services require higher prices [ 23 ]. Based on this, Patel et al [ 24 ] examined the relationship between the Diagnosis-Related Group (DRG) chargemaster and quality measures. Although prior research found no convincing evidence that hospitals with greater costs also delivered better care [ 25 ], they discovered 2 important quality indicators that were linked to standard charges positively and substantially—mortality rate and readmission rates—which both are quality characteristics that are in line with economic theory. Moreover, Patel et al [ 24 ] studied the variety of one of the most commonly performed services (vaginal delivery) as a DRG code, which motivated us to select “Vaginal delivery without sterilization or D&C without CC/MCC” as another shoppable service in our analysis.
Ethical Considerations
All data used in this study, including the secondary data set obtained from hospitals’ websites and the data collected during the user experiment, underwent a thorough anonymization process. The study was conducted under protocols approved by the University of South Florida institutional review board (STUDY004145: “Effect of price transparency regulation (PTR) on the public decisions”) under HRP-502b(7) Social Behavioral Survey Consent. This approval encompassed the use of publicly available anonymized secondary data from hospitals’ websites, as well as a user experiment aimed at assessing awareness of the PT rule and the usability of hospitals’ files. No individual-specific data were collected during the experiment, which solely focused on capturing subjects’ awareness and opinions regarding the PT rule and associated files. At the onset of the experiment, participants were provided with a downloadable consent form and were allowed to withdraw their participation at any time. Survey participants were offered a US $2 reward, and their involvement was entirely anonymous.
Data Collection
According to CMS, “Starting January 1, 2021, each hospital operating in the United States will be required to provide clear, accessible pricing information online about the items and services they provide in two ways: 1- As a comprehensive machine-readable file with all items and services. 2- In a display of shoppable services in a consumer-friendly format.” As stated, files available on hospitals’ websites should be consumer-friendly, so the question of whether these files are for users arises. On the other hand, as stated, files should be machine-readable, so again the question of whether these files are for machines arises. Below we try to answer both questions in detail, respectively.
Value for Users: User Experiments
When a public announcement is disseminated, its efficacy relies on ensuring widespread awareness and facilitating practical use during times of necessity. Previous research on PT announcements has highlighted the challenges faced by patients in accurately estimating out-of-pocket expenses. However, a fundamental inquiry arises—are individuals adequately informed about the availability of tools that enable them to estimate their out-of-pocket costs for desired services? To address this, we conducted a survey to assess public awareness of PT legislation. The survey encompassed a range of yes or no and multiple-choice questions aimed at gauging participants’ familiarity with the PT rule in health care and their entitlement to obtain cost information prior to receiving a service. Additionally, we inquired about participants’ knowledge of resources for accessing pricing information and whether they were aware of the PT rule. Furthermore, we incorporated follow-up questions to ensure that the survey responses were not provided arbitrarily, thereby securing reliable and meaningful outcomes.
Moreover, considering the previously established evidence of subpar usability associated with the currently available files, we propose streamlining the existing files and developing a user-friendly and comprehensive document for conducting an A/B test. This test aims to evaluate which file better facilitates participants in accurately estimating their out-of-pocket costs. In collaboration with Florida Blue experts during biweekly meetings throughout the entire process outlined in this paper, the authors determined the optimal design for the summary table. This design, which presents prices in a more user-friendly format, enhancing overall participant comprehension, was used during the A/B testing. Participants were randomly assigned to either access the hospitals’ files or a meticulously constructed summary table, manually created in Excel, prominently displaying cost information (Please note that all files, including the hospitals’ files and our Excel file, are made available in the same format [Excel] on a cloud-based platform to eliminate any disparities in accessing the files. This ensures equitable ease of finding, downloading, and opening files, as accessing the hospitals’ files typically requires significant effort.). The experiment entailed presenting 3 distinct health-related scenarios and instructing participants to locate the price for the requested service. Subsequently, participants were asked to provide the hospital name, service price, insurer name, and insurance plan. Additionally, we sought feedback on the perceived difficulty of finding the requested service and their priority for selecting hospitals [ 26 ], followed by Likert scale questions to assess participants’ evaluation of the provided file’s efficacy in facilitating price retrieval.
The experiments were conducted to investigate the following questions: (1) Are the individuals aware of the PT legislation? and (2) Is the information provided usable for patients? To evaluate the usability of files found on websites, we selected 2 prevalent services based on existing literature and 2 other services recommended as high-demand ones by Florida Blue experts, Table 1 . Furthermore, meticulous efforts were made to ensure that both the control and treatment groups encountered identical circumstances, thus allowing for a systematic examination of the disparities solely attributable to variations in data representation.
a DRG: Diagnosis-Related Group.
b D&C: dilation and curettage.
c CC/MCC: complication or comorbidity/major complication or comorbidity.
d CPT: Current Procedural Terminology.
e MRI: magnetic resonance imaging.
Participants
A total of 67 adults (30 female individuals; mean 41.43, SD 12.39 years) were recruited on the Amazon Mechanical Turk platform, with no specific selection criteria other than being located in the United States.
We focused on 75 main hospitals (ie, the main hospital refers to distinguish a hospital from smaller clinics or specialized medical centers within the same health system) in the state of Florida. When we searched their websites for PT files (machine-readable files), only 89% (67/75) of hospitals included machine-readable files. According to the PT legislation, these files were supposed to contain information about 300 shoppable services. However, only 58% (39/67) of hospitals included information such as insurer prices in their files. Therefore, for the rest of the analysis, we only included the 39 hospitals that have the required information in their machine-readable files on their websites. We created an Excel file that included those 39 hospitals along with the 4 services—CPT 45380, 29827 and 70553 and DRG 807—mentioned in the literature ( Table 1 ) for 4 popular (suggested by Florida Blue experts) commercial carriers (Health Maintenance Organization [HMO] or Preferred Provider Organization [PPO] plans)—Aetna, Florida Blue, Cigna, and UnitedHealthcare.
Participants were recruited for the pilot and randomly assigned by the Qualtrics XM platform to answer multiple-choice questions and fill in blanks based on the given scenarios. First, participants responded to questions regarding the awareness of PT and then were divided into 2 groups randomly to answer questions regarding the usability of hospital-provided PT information. One group was assigned hospitals’ website links (control group), while the other group was given an Excel file with the same information provided in files on hospitals’ websites, but in a manner that was designed to allow easier comparison of prices across hospitals ( Multimedia Appendix 1 ). Participants were given 3 scenarios that asked them to find a procedure’s price based on their hospital and insurer selection to compare hospital-provided information with Excel. We provide some examples of hospitals’ files and our Excel file in Multimedia Appendix 1 and the survey experiment questions in Multimedia Appendix 2 .
Value for Machines: Schema Integration—Machine-Readable Files Representation
Through meticulous investigation of machine-readable files from 39 hospitals, we discovered that these files may vary in formats such as CSV or JSON, posing a challenge for machines to effectively manage the data within these files. Another significant obstacle arises from the lack of uniformity in data representation across these files, rendering them unsuitable for machine use without a cohesive system capable of processing them collectively. Our analysis revealed that hospitals within a single health system exhibit consistent data representation, although service prices may differ (we include both the same and different chargemaster prices in our study), while substantial disparities in data representation exist between hospitals affiliated with different health systems.
Moving forward, we will use the terms “data representation” and “schema” interchangeably, with “schema” denoting its database management context. In this context, a schema serves as a blueprint outlining the structure, organization, and relationships of data within a database system. It encompasses key details such as tables, fields, data types, and constraints that define the stored data. To systematically illustrate schema differences among hospitals associated with different health systems, we adopted the methodology outlined in reference [ 27 ] for schema integration, which offers a valid approach for comparing distinct data representations. The concept of schema integration encompasses four common categories: (1) identical: hospitals within the same health system adhere to this concept as their representations are identical; (2) equivalent: while hospitals in health system “A” may present different representations from those in health system “B,” they possess interchangeable columns; (3) compatible: in cases where hospitals across different health systems are neither identical nor equivalent, the modeling constructs, designer perception, and integrity constraints do not contradict one another; and (4) incompatible: in situations where hospitals within different health systems demonstrate contradictory representations, distinct columns exist for each health system due to specification incoherence.
Our analysis focused on health systems in Florida that encompassed a minimum of 4 main hospitals, using the most up-to-date data available on their respective websites. Within this scope, we identified 8 health systems with at least 4 main hospitals, of which 88% (7/8) of health systems had published machine-readable files on their websites. Consequently, our analysis included 65% (36/55) of hospitals that possessed machine-readable files available on their websites. To facilitate further investigation by interested researchers, we have made the analyzed data accessible on a cloud-based platform. During our analysis, we meticulously extracted the schema of each health system by closely scrutinizing the hospitals associated with each health system, capturing key details such as tables, fields, and data types. Subsequently, we compiled a comprehensive master field name table trying to have the same data type and field names that make it easier for machines to retrieve information. We elaborate on the master field names table in greater detail within the results section.
Value for Users
Question 1 (pt awareness).
Based on the responses, it is evident that participants need to be made aware of the PT legislation. Among the participants, 64% (49/76) reported that they had not heard about the legislation. However, they believe it is important to know the service price before receiving it—response charts are provided in Multimedia Appendix 3 .
Question 2 (Human Usability of PT Information)
Based on the responses to scenarios, the average number of correct responses is not equal between the 2 groups, that is, the treatment group (mean 1.23, SD 1.30) found more correct answers than the control group (mean 2.76, SD 0.58; t 65 =6.46; P <.049; d =1.52). The t tests (2-tailed) for the other questions in the experiment are in Multimedia Appendix 4 .
These suggest that current files on hospitals’ websites are not consumer-friendly, and participants find it challenging to estimate out-of-pocket costs for a desired service. For this reason, in addition to making the files easier to use, this information should also include thorough documentation that explains what each column represents, up to what amount an insurer covers for a specific service, or the stated price covers up to how many days of a particular service, that is, “contracting method.” For example, based on consulting with one of the senior network analysts of Florida Blue, some prices for a service like DRG 807 are presented as per diem costs, and based on the current information on these files, it cannot be recognizable without having comprehensive documentation for them.
Value for Machines
After carefully reviewing all machine-readable file schemas, we create a master field name table, including the available field names in machine-readable files ( Table 2 ). According to Table 2 , the first column represents master field names that we came up with, and the following columns each represent hospitals within a health system. The “✓” mark shows that hospitals within a health system have identical field names as we consider as master field names and the “written” cells show equivalent field names, meaning that hospitals within that health system use different field names—we write what they use in their representation—while the content is equivalent to what we select as the master field name. The “❋” mark means that although hospitals within health system #2 provide insurer names and plans in their field names, some codes make those columns unusable for machines to recognize them the same as master field names. We also include the type of field names for all representations in parentheses.
a As noted previously, since we focus on the health system level instead of the hospital level, our schema does not have hospital-level information; however, it would be beneficial to add hospital information to the table.
b ✓: it means the given master field name in that row appears on the given health system file in that column.
c str: shows “string” as the data type.
d int: shows “integer” as the data type.
e CPT: Current Procedural Terminology.
f HCPCS: Health care Common Procedure Coding System.
g Not applicable.
h Apr: all patients refined.
i DRG: Diagnosis-Related Group.
j Ms: Medicare severity.
k CDM: charge description master.
l UB: uniform billing.
m float: it shows “float” as the data type.
n ❋: it means that although hospitals within health system #2 provide insurer names and plans in their field names, some codes make those columns unusable for machines to recognize them the same as master field names.
We did reverse engineering and drew entity-relationship diagrams (ERDs) for each hospital based on their data representation. However, as hospitals within the same health system have the same ERDs, we only include 1 ERD for each health system ( Figure 1 ). According to Figure 1 , although hospitals have tried to follow an intuitive structure, we can still separate them into three groups: (1) group I: all hospitals within this group have several columns for different insurers. As shown in the ERDs, we decided to have a separate entity, called “Insurance” for this group; (2) group II: all hospitals within this group have many sheets, and each sheet belongs to a specific insurer with a specific plan. As shown in the ERDs, we decided to create an “Insurance_Name” entity for this group’s ERD to show the difference in data representation; and (3) group III: all hospitals within this system have a “payer” column which includes the names of insurers without their plans. As shown in the ERDs, we decided to put this column as an attribute in the “Service” entity, and do not have an “Insurance” entity for this group’s ERD.
In conclusion, although most hospitals have adopted group I logic for data representation, for full similarity, a standard representation with the same intuitive field names (like what we suggest as the master field name; Table 2 ) should be proposed so that it can cover all systems’ data representations and be used as machine-readable file, for at least machine benefits. Mainly, standardization in the format and semantics of the provided data can help substantially in making the data more machine friendly.

Comparison With New CMS Guidelines
Recently, CMS has published guidelines regarding the PT legislation [ 28 ]. The most recent CMS guideline is a step forward in ensuring standardization but is still only recommended and is not mandatory. These guidelines exhibit overlaps with our fields in Table 2 , with slight differences attributed to granularities. Our observation reveals that hospitals within the same health system adopt a uniform schema. Therefore, our suggested schema operates on the granularity of health systems rather than individual hospitals.
The recent CMS guidelines allocate 24% (6/25) of field names specifically to hospital information, encompassing details such as “Hospital Name,” “Hospital File Date,” “Version,” “Hospital Location,” “Hospital Financial Aid Policy,” and “Hospital Licensure Information.” These details, absent in current hospital files, are crucial for informed decision-making. As noted previously, since we focus on the health system level instead of the hospital level, our schema does not have hospital-level information; however, it would be beneficial to add hospital information to the tables.
Our analysis reveals that the 11 field names in Table 2 align with the field names in the new CMS guidelines, demonstrating a substantial overlap of 58% (11/19). The corresponding CMS field names (compatible with our schema) include “Item or Service Description (Description or CDM Service Description),” “Code (Code),” “Code Type (Code Type),” “Setting (Patient Class),” “Gross Charge (Gross Charge),” “Discounted Cash Price (Discounted Cash Price),” “Payer Name (Insurer Name),” “Plan Name (Insurer Plan),” “Payer Specific Negotiated Charge: Dollar Amount (Price),” “De-identified Minimum Negotiated Charge (Min Negotiated Rate),” and “De-identified Maximum Negotiated Charge (Max Negotiated Rate).” Additionally, both our schema and the new CMS guidelines propose data types for each field name.
In our schema, which represents current hospitals’ files, there are 5 field names absent in the new CMS guidelines “Revenue Description,” “Revenue Code,” “Package/Line Level,” “Procedure ID,” and “Self Pay.” Conversely, the new CMS guidelines introduce 8 additional field names “Billing Class,” “Drug Unit of Measurement,” “Drug Type of Measurement,” “Modifiers,” “Payer Specific Negotiated Charge: Percentage,” “Contracting Method,” “Additional Generic Notes,” and “Additional Payer-Specific Notes.” We regard these new field names as providing further detailed information and enhancing consumer decision-making. If hospitals within a health system adopt consistent formats and can map their formats to the new CMS guidelines clearly in a mapping document they also provide, this can be more useful than the current optional guideline that is suggested.
In summary, since our analysis is based on the current data schema that hospitals have in place, we believe the schema we put out is easier to implement with minimal change to what the hospitals are currently doing. However, given the recent CMS guidelines, we recommend adding 8 additional fields as well as hospital-specific information.
Implications
The PT legislation aims to enable informed decision-making, reduce out-of-pocket expenses, and decrease overall health care expenditures. This study investigates the usage of current files by individuals and machines. Our results, unfortunately, suggest that PT data—as currently reported—appear to be neither useful for patients nor machines, raising important questions as to what these appear to be achieving today. Moreover, the findings indicate that even individuals with basic computer knowledge struggle with the usability of these files, highlighting the need for significant revisions to make them consumer-friendly and accessible to individuals of all technical proficiency levels. Additionally, inconsistencies in data representation between hospitals affiliated with different health systems pose challenges for machines, necessitating schema design improvements and the implementation of a standardized data representation. By addressing these concerns, PT legislation can achieve consistency and enhance machine readability, thus improving its effectiveness in promoting informed decision-making and reducing health care costs.
Although the official announcement of PT legislation is recent, prior studies [ 15 - 17 ] have attempted to evaluate the usability of PT, while subsequent studies [ 19 - 22 ] have examined the effectiveness of PT tools following the announcement. However, despite the introduction of PT rules, it appears that the usability of these files has not undergone significant improvements, indicating the necessity for proactive measures from responsible executives to ensure the effectiveness of this legislation. Our analysis of this matter emphasizes 2 primary factors—a lack of awareness among stakeholders and the challenges associated with using files due to inconsistencies in their format and representation.
As of April 2023, the CMS has issued over 730 warning notices and 269 requests for Corrective Action Plans. A total of 4 hospitals have faced Civil Monetary Penalties for noncompliance, and these penalties are publicly disclosed on the CMS website. The remaining hospitals subjected to comprehensive compliance reviews have either rectified their deficiencies or are actively engaged in doing so. While we acknowledge these efforts to comply with PT rules, our research revealed a notable disparity in data representation among hospitals affiliated with different health systems. Consequently, we focused on schema design and proposed the implementation of a master field name that encompasses a comprehensive data representation derived from an analysis of 36 hospitals. Standardizing the data representation across all health systems’ machine-readable files will effectively address concerns about consistency. Therefore, significant modifications are required for the PT legislation to enhance machine readability and provide clearer guidance on the design and structure of the files’ schema. If the hospital-provided information is consistent and of high quality, PT tools provided by health insurers may be able to estimate an individual’s total expenses more accurately.
Limitations
Our objective was to have an equal number in both groups. However, in the case of the group tasked with obtaining information from the hospitals’ websites, most did not finish the task and dropped out without completing it. This occurred because the task of retrieving the cost from the hospitals’ websites in its current form is complex, as indicated by feedback from some participants. Only 19% (13/67) completed the task in that group (control group). Although this is a limitation of the study, it also highlights the complexity of obtaining cost information from hospitals’ websites in the current form. In the treatment group, 81% (54 out of 67) of participants completed the task of retrieving the data, and the completion percentage was much higher.
Conclusions
Due to the poor usability and inconsistency of the formats, we, unfortunately, did not find evidence that the PT rule as implemented currently is useful to consumers, researchers, or policy makers (despite the legislation’s goals that files are “consumer-friendly” and “machine-readable”). As 1 solution, we suggest a master field name for the data representation of machine-readable files to make them consistent, at least for the machines. Building tools that enable customers to estimate out-of-pocket costs is facilitated by having consistent machine-readable files across all health systems, which can be considered as future work for researchers and companies to help the PT rule reach its main goal, which is providing useful information for patients and reducing health care expenditures. In addition, another worthwhile approach to reducing some of the exorbitant health care costs in the United States would be to integrate clinical decision support tools into the providers’ workflow, triggered by orders for medications, diagnostic testing, and other billable services. In this regard, Bouayad et al [ 29 ] conducted experiments with physicians to demonstrate that PT, when included as part of the system they interact with, such as clinical decision support integrated into electronic health record systems, can significantly aid in cost reduction. This is a promising direction for practice but needs to be implemented carefully to avoid unanticipated consequences, such as scenarios where cost is incorrectly viewed as a proxy for quality, or where the use of this information introduces new biases for physicians and patients.
Conflicts of Interest
None declared.
Example of Excel format of hospitals’ files and our created Excel file.
Survey questions and experiment scenarios.
Participants’ responses chart regarding price transparency awareness.
The t test analysis regarding human usability of price transparency information based on participants’ responses.
- McGough M, Winger A, Rakshit S, Amin K. How has U.S. spending on healthcare changed over time? Health System Tracker. 2022. URL: https://www.healthsystemtracker.org/chart-collection/u-s-spending-healthcare-changed-time/ [accessed 2024-03-13]
- Christensen HB, Floyd E, Maffett M. The only prescription is transparency: the effect of charge-price-transparency regulation on healthcare prices. Manag Sci. 2020;66(7):2861-2882. [ CrossRef ]
- Muir MA, Alessi SA, King JS. Clarifying costs: can increased price transparency reduce healthcare spending? UC Hastings Research Paper No. 38 (SSRN). Feb 26, 2013.:319-367. [ FREE Full text ] [ CrossRef ]
- Reinhardt UE. Health care price transparency and economic theory. JAMA. 2014;312(16):1642-1643. [ CrossRef ] [ Medline ]
- CY 2020 hospital Outpatient Prospective Payment System (OPPS) policy changes: hospital price transparency requirements (CMS-1717-F2). CMS.gov. 2020. URL: https://tinyurl.com/mrafxtvd [accessed 2024-03-13]
- Secretary Azar statement on proposed rule for hospital price transparency. HHS.gov. 2020. URL: https://tinyurl.com/yc4dx2vx [accessed 2024-03-13]
- Mehrotra A, Brannen T, Sinaiko AD. Use patterns of a state health care price transparency web site: what do patients shop for? Inquiry. 2014;51:0046958014561496. [ FREE Full text ] [ CrossRef ] [ Medline ]
- Desai S, Hatfield LA, Hicks AL, Sinaiko AD, Chernew ME, Cowling D, et al. Offering a price transparency tool did not reduce overall spending among California public employees and retirees. Health Aff (Millwood). 2017;36(8):1401-1407. [ FREE Full text ] [ CrossRef ] [ Medline ]
- Sinaiko AD, Joynt Maddox KE, Rosenthal MB. Association between viewing health care price information and choice of health care facility. JAMA Intern Med. 2016;176(12):1868-1870. [ FREE Full text ] [ CrossRef ] [ Medline ]
- Desai S, Hatfield LA, Hicks AL, Chernew ME, Mehrotra A. Association between availability of a price transparency tool and outpatient spending. JAMA. 2016;315(17):1874-1881. [ FREE Full text ] [ CrossRef ] [ Medline ]
- Sinaiko AD, Rosenthal MB. Examining a health care price transparency tool: who uses it, and how they shop for care. Health Aff (Millwood). 2016;35(4):662-670. [ FREE Full text ] [ CrossRef ] [ Medline ]
- Desai SM, Shambhu S, Mehrotra A. Online advertising increased New Hampshire residents' use of provider price tool but not use of lower-price providers. Health Aff (Millwood). 2021;40(3):521-528. [ FREE Full text ] [ CrossRef ] [ Medline ]
- Kona M, Corlette S. Hospital and insurer price transparency rules now in effect but compliance is still far away. Health Affairs Forefront. 2022. URL: hhttps://tinyurl.com/3x6ymxf2 [accessed 2024-03-13]
- Wheeler C, Taylor R. New year, new CMS price transparency rule for hospitals. Health Affairs Forefront. 2021. URL: https://www.healthaffairs.org/content/forefront/new-year-new-cms-price-transparency-rule-hospitals [accessed 2024-03-13]
- Chernew M, Cooper Z, Larsen-Hallock E, Morton FS. Are health care services shoppable? Evidence from the consumption of lower-limb MRI scans. National Bureau of Economic Research. 2021. URL: https://www.nber.org/papers/w24869 [accessed 2024-03-13]
- Mehrotra A, Dean KM, Sinaiko AD, Sood N. Americans support price shopping for health care, but few actually seek out price information. Health Aff (Millwood). 2017;36(8):1392-1400. [ FREE Full text ] [ CrossRef ] [ Medline ]
- Brown ZY. Equilibrium effects of health care price information. Rev Econ Stat. 2019;101(4):699-712. [ FREE Full text ] [ CrossRef ]
- Wu SJ, Sylwestrzak G, Shah C, DeVries A. Price transparency for MRIs increased use of less costly providers and triggered provider competition. Health Aff (Millwood). 2014;33(8):1391-1398. [ CrossRef ] [ Medline ]
- Glover M, Whorms DS, Singh R, Almeida RR, Prabhakar AM, Saini S, et al. A radiology-focused analysis of transparency and usability of top U.S. hospitals' chargemasters. Acad Radiol. 2020;27(11):1603-1607. [ CrossRef ] [ Medline ]
- Hayatghaibi SE, Alves VV, Ayyala RS, Dillman JR, Trout AT. Transparency and variability in pricing for pediatric outpatient imaging in US children's hospitals. JAMA Netw Open. 2022;5(3):e220736. [ FREE Full text ] [ CrossRef ] [ Medline ]
- Arvisais-Anhalt S, McDonald S, Park JY, Kapinos K, Lehmann CU, Basit M. Survey of hospital chargemaster transparency. Appl Clin Inform. 2021;12(2):391-398. [ FREE Full text ] [ CrossRef ] [ Medline ]
- Reddy S, Daly G, Baban S, Kadesh A, Block AE, Grimes CL. Accessibility and usability of hospital chargemasters in New York state. J Gen Intern Med. 2022;37(8):2130-2131. [ FREE Full text ] [ CrossRef ] [ Medline ]
- Robinson JC. Hospital quality competition and the economics of imperfect information. Milbank Q. 1988;66(3):465-481. [ Medline ]
- Patel KN, Mazurenko O, Ford E. Analysis of hospital quality measures and web-based chargemasters, 2019: cross-sectional study. JMIR Form Res. 2021;5(8):e26887. [ FREE Full text ] [ CrossRef ] [ Medline ]
- Batty M, Ippolito B. Mystery of the chargemaster: examining the role of hospital list prices in what patients actually pay. Health Aff (Millwood). 2017;36(4):689-696. [ FREE Full text ] [ CrossRef ] [ Medline ]
- Muhlestein DB, Wilks CEA, Richter JP. Limited use of price and quality advertising among American hospitals. J Med Internet Res. 2013;15(8):e185. [ FREE Full text ] [ CrossRef ] [ Medline ]
- Batini C, Lenzerini M, Navathe SB. A comparative analysis of methodologies for database schema integration. ACM Comput Surv. 1986;18(4):323-364. [ FREE Full text ] [ CrossRef ]
- Voluntary hospital price transparency machine-readable file sample format data dictionary (version 1.1). CMS.gov. URL: https://www.cms.gov/files/document/hospital-price-transparency-machine-readable-data-dictionary-tall.pdf [accessed 2024-03-13]
- Bouayad L, Padmanabhan B, Chari K. Can recommender systems reduce healthcare costs? the role of time pressure and cost transparency in prescription choice. MIS Q. 2020;44(4):1859-1903. [ CrossRef ]
Abbreviations
Edited by S He; submitted 07.07.23; peer-reviewed by KN Patel, R Marshall, G Deckard; comments to author 03.12.23; revised version received 21.01.24; accepted 26.02.24; published 29.03.24.
©Negar Maleki, Balaji Padmanabhan, Kaushik Dutta. Originally published in the Journal of Medical Internet Research (https://www.jmir.org), 29.03.2024.
This is an open-access article distributed under the terms of the Creative Commons Attribution License (https://creativecommons.org/licenses/by/4.0/), which permits unrestricted use, distribution, and reproduction in any medium, provided the original work, first published in the Journal of Medical Internet Research, is properly cited. The complete bibliographic information, a link to the original publication on https://www.jmir.org/, as well as this copyright and license information must be included.
- Get 7 Days Free
International Paper Co
International paper: potential acquisition of ds smith would bolster company’s global footprint.
/s3.amazonaws.com/arc-authors/morningstar/8d9c3e2f-1859-4b26-84b3-0ec4daf3bde3.jpg)
London-based DS Smith announced on March 26 that it was engaged in discussions with International Paper concerning a potential takeover bid. It is currently reported that International Paper made an all-share offer, where DS Smith shareholders would receive 0.1285 shares of International Paper for every share of DS Smith they own. This offer would value DS Smith at $7.22 billion, which would represent a sizable premium for the company. We are maintaining our $41 fair value estimate until an official agreement is reached.
Free Trial of Morningstar Investor
Get our analysts’ objective, in-depth, and continuous investment coverage of IP so you can make buy / sell decisions free of market noise.
Sponsor Center
Data Acquisition System for Abrasive Wheel Manufacturing Industry
Ieee account.
- Change Username/Password
- Update Address
Purchase Details
- Payment Options
- Order History
- View Purchased Documents
Profile Information
- Communications Preferences
- Profession and Education
- Technical Interests
- US & Canada: +1 800 678 4333
- Worldwide: +1 732 981 0060
- Contact & Support
- About IEEE Xplore
- Accessibility
- Terms of Use
- Nondiscrimination Policy
- Privacy & Opting Out of Cookies
A not-for-profit organization, IEEE is the world's largest technical professional organization dedicated to advancing technology for the benefit of humanity. © Copyright 2024 IEEE - All rights reserved. Use of this web site signifies your agreement to the terms and conditions.
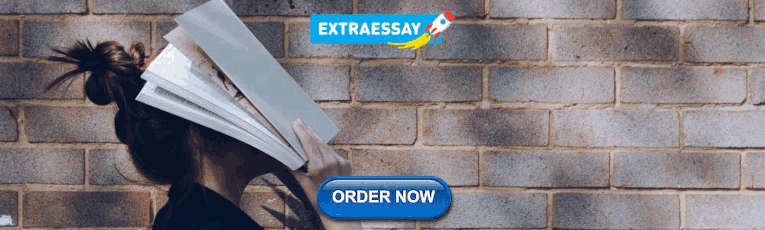
IMAGES
VIDEO
COMMENTS
The data acquisition system was accordingly improved and the data from scintillation detectors is collected with appropriate sampling rate (≈300 MHz) and sufficient bandwidth (≈100 MHz) to allow a pulse analysis. Up to five detectors can currently simultaneously monitor hard X-ray radiation at the GOLEM.
This paper centers on the design and installation of a robust photovoltaic (PV)-based microgrid data acquisition system (DAS) that can monitor different PV systems simultaneously.
The Data Acquisition (DAQ) systems are so fundamental that plays important role in all walks of science and technology and indeed in our everyday lives. At the heart of the DAQ system is data ...
Explore the latest full-text research PDFs, articles, conference papers, preprints and more on DATA ACQUISITION SYSTEMS. Find methods information, sources, references or conduct a literature ...
RSS Feed. Data acquisition is the process of converting measurements, such as temperature, pressure, relative humidity, light, resistance, current, power, speed and vibration, into digital numeric ...
A data acquisition system was proposed and validated through a real application using the prototype built, monitoring variables in a photovoltaic system such as voltage and current to analyze the behavior of the solar panels. ... Feature papers represent the most advanced research with significant potential for high impact in the field. A ...
Data acquisition systems have been used in various applications in our world. Numerous techniques and algorithms have been employed to achieve high quality acquisition. Each application has its own philosophy and data acquisition structure. Hence, in this paper, a survey on data acquisition systems has been presented. Different DAQ categories have been introduced. A comparison study between ...
The present work proposes a low-cost data acquisition system, based on Raspberry-Pi, with a high sampling frequency capacity in the recording of up to three channels. ... provides an outlook for future research directions and describes possible research applications. Feature papers are submitted upon individual invitation or recommendation by ...
About this book. This book describes the fundamentals of data acquisition systems, how they enable users to sample signals that measure real physical conditions and convert the resulting samples into digital, numeric values that can be analyzed by a computer. The author takes a problem-solving approach to data acquisition, providing the tools ...
This paper examines data acquisition (DAQ) and monitoring systems designed and implemented for PV systems. DAQ and monitoring applications for PV systems are presented from a different perspective according to the methods existing in the literature on PV monitoring systems, and the presented method provides a reference for further studies. 2.
The big data collected from IoT (Internet of Things) devices have difficulty sending to the cloud server due to the finite resources. To get over such limitation, edge computing is suggested. In this paper, we propose data acquisition device (DAQ), executing data collecting and computing functions, programmed to conduct the target works like sending message to the other DAQ device and ...
Finally, a set of multi-modal data real-time acquisition system is developed, which has the characteristics of high localization rate, low cost, easy expansion and stable performance and lays a foundation for the subsequent research on multi-modal data fusion technology. ... Cite this paper. Cao, L. et al. (2022). Research on Multi-modal Sensor ...
Proposed System. This research paper proposes a sensor-based data acquisition system, which can mount sensors onto robots. For our system, robots have been used. This can produce a comprehensive dataset for improving crop production by facilitating farmer decisions with analysis based on data.
A remote data acquisition system based on CC3200 is proposed. Based on the fact that most of the current acquisition systems are unable to carry out remote data acquisition and monitoring, the system presents an efficient and stable solution for remote data acquisition. The system includes the sensing layer, the network layer and the remote application layer. The system completed the local ...
A UNIT OF BHABHA ATOMIC RESEARCH CENTRE, DEPARTMENT OF ATOMIC ENERGY, ... DEVELOPMENT OF A DATA ACQUISITION & CONTROL (DAQ) SYSTEM USING TCP/MODBUS PROTOCOL" submitted to the Variable Energy Cyclotron Centre, Kolkata is a record of an original work done by me under the guidance of Mr. Tamal Bhattacharya, Head, Cryogenic Instrumentation
A general-purpose digital data acquisition system (GDDAQ) has been developed at Peking University. This GDDAQ, composed of 16-channel Digital Pulse Processor Pixie-16 modules from XIA LLC, is a versatile, flexible, and easily expandable data acquisition system for nuclear physics research in China. The software used by this GDDAQ is based on ...
facility data systems. Data systems of this scale must be versatile enough to meet the wide range of data acquisition and measurement requirements from a diverse set of customers and test programs, but also must minimize design changes to maintain reliability and serviceability. This paper presents an overview
In this research paper, authors have proposed a real-time Data Acquisition System for measuring the pressure of seawater using a piezo-resistive pressure sensor. A survey is presented on various ...
Abstract. The presented work was conducted to test the hypothesis that a Raspberry Pi Model B single board computer can be applied as a riding dynamics data acquisition system for use on human powered vehicles. The research of the subject was followed by the development, which led to a working prototype of such a data acquisition system.
The objective of this paper is to build the control system and data acquisition system for the RFP called KTX which is in the construction in University of science and technology of China. The data acquisition system and the control system includes three ingredients: the master control system, the data acquisition and storage system, the plasma ...
Research that uses these edited public data relies on limited information on MENA people's racial identification. To address this limitation, we obtained unedited race responses in the nationally representative American Community Survey from 2005-2019 to better understand how people of MENA ancestry report their race. ... This paper examines ...
Therefore, this paper proposes a DAQ system, based on low cost hardware, IoT principles and open source and freely available data mining tools, what enables its widespread usage in educational ...
Background: Increasing health care expenditure in the United States has put policy makers under enormous pressure to find ways to curtail costs. Starting January 1, 2021, hospitals operating in the United States were mandated to publish transparent, accessible pricing information online about the items and services in a consumer-friendly format within comprehensive machine-readable files on ...
This paper presents the construction of a low-cost data acquisition system (DAS) prototype based on Raspberry Pi-2 microcomputer. The prototype is designed to operate as a standalone system without the need for an additional personal computer (PC). It performs the data acquisition, online plotting and data logging, simultaneously. This is a general-purpose setup, as it is capable of reading ...
Morningstar is an investment research company offering mutual fund, ETF, and stock analysis, ratings, and data, and portfolio tools. Discover actionable insights today.
Using big data analysis algorithm, this paper discusses the source of data acquisition, points out the technical characteristics of data source, and explains the data acquisition methods and requirements. Clear the purpose of big data analysis, build data analysis system, describe the process of data analysis. There are four steps in big data analysis: data acquisition, data storage, data ...
This paper presents the development of a user-friendly weight data management system utilizing data acquisition methods. The system was designed to tackle prominent industrial challenges faced by Ocean Abrasive, an abrasive wheel manufacturing factory: (i) misinterpretation of weight or nameof grain (raw material) arising from handwritten entry in paper cards, (ii) no data logging (of weight ...