Have a language expert improve your writing
Run a free plagiarism check in 10 minutes, generate accurate citations for free.
- Knowledge Base
Methodology
- Random Assignment in Experiments | Introduction & Examples
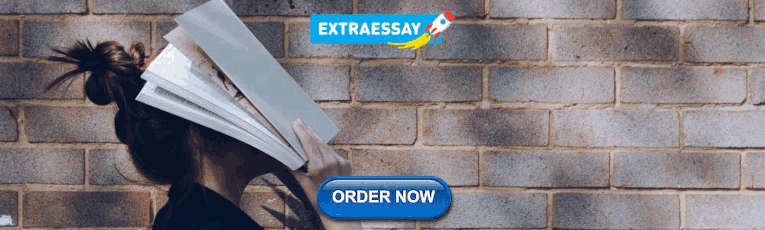
Random Assignment in Experiments | Introduction & Examples
Published on March 8, 2021 by Pritha Bhandari . Revised on June 22, 2023.
In experimental research, random assignment is a way of placing participants from your sample into different treatment groups using randomization.
With simple random assignment, every member of the sample has a known or equal chance of being placed in a control group or an experimental group. Studies that use simple random assignment are also called completely randomized designs .
Random assignment is a key part of experimental design . It helps you ensure that all groups are comparable at the start of a study: any differences between them are due to random factors, not research biases like sampling bias or selection bias .
Table of contents
Why does random assignment matter, random sampling vs random assignment, how do you use random assignment, when is random assignment not used, other interesting articles, frequently asked questions about random assignment.
Random assignment is an important part of control in experimental research, because it helps strengthen the internal validity of an experiment and avoid biases.
In experiments, researchers manipulate an independent variable to assess its effect on a dependent variable, while controlling for other variables. To do so, they often use different levels of an independent variable for different groups of participants.
This is called a between-groups or independent measures design.
You use three groups of participants that are each given a different level of the independent variable:
- a control group that’s given a placebo (no dosage, to control for a placebo effect ),
- an experimental group that’s given a low dosage,
- a second experimental group that’s given a high dosage.
Random assignment to helps you make sure that the treatment groups don’t differ in systematic ways at the start of the experiment, as this can seriously affect (and even invalidate) your work.
If you don’t use random assignment, you may not be able to rule out alternative explanations for your results.
- participants recruited from cafes are placed in the control group ,
- participants recruited from local community centers are placed in the low dosage experimental group,
- participants recruited from gyms are placed in the high dosage group.
With this type of assignment, it’s hard to tell whether the participant characteristics are the same across all groups at the start of the study. Gym-users may tend to engage in more healthy behaviors than people who frequent cafes or community centers, and this would introduce a healthy user bias in your study.
Although random assignment helps even out baseline differences between groups, it doesn’t always make them completely equivalent. There may still be extraneous variables that differ between groups, and there will always be some group differences that arise from chance.
Most of the time, the random variation between groups is low, and, therefore, it’s acceptable for further analysis. This is especially true when you have a large sample. In general, you should always use random assignment in experiments when it is ethically possible and makes sense for your study topic.
Receive feedback on language, structure, and formatting
Professional editors proofread and edit your paper by focusing on:
- Academic style
- Vague sentences
- Style consistency
See an example
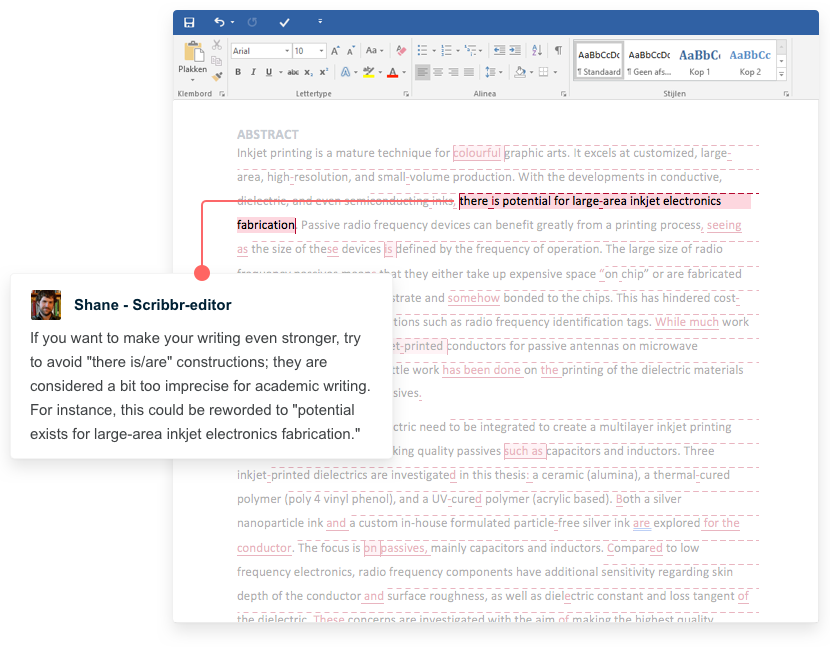
Random sampling and random assignment are both important concepts in research, but it’s important to understand the difference between them.
Random sampling (also called probability sampling or random selection) is a way of selecting members of a population to be included in your study. In contrast, random assignment is a way of sorting the sample participants into control and experimental groups.
While random sampling is used in many types of studies, random assignment is only used in between-subjects experimental designs.
Some studies use both random sampling and random assignment, while others use only one or the other.
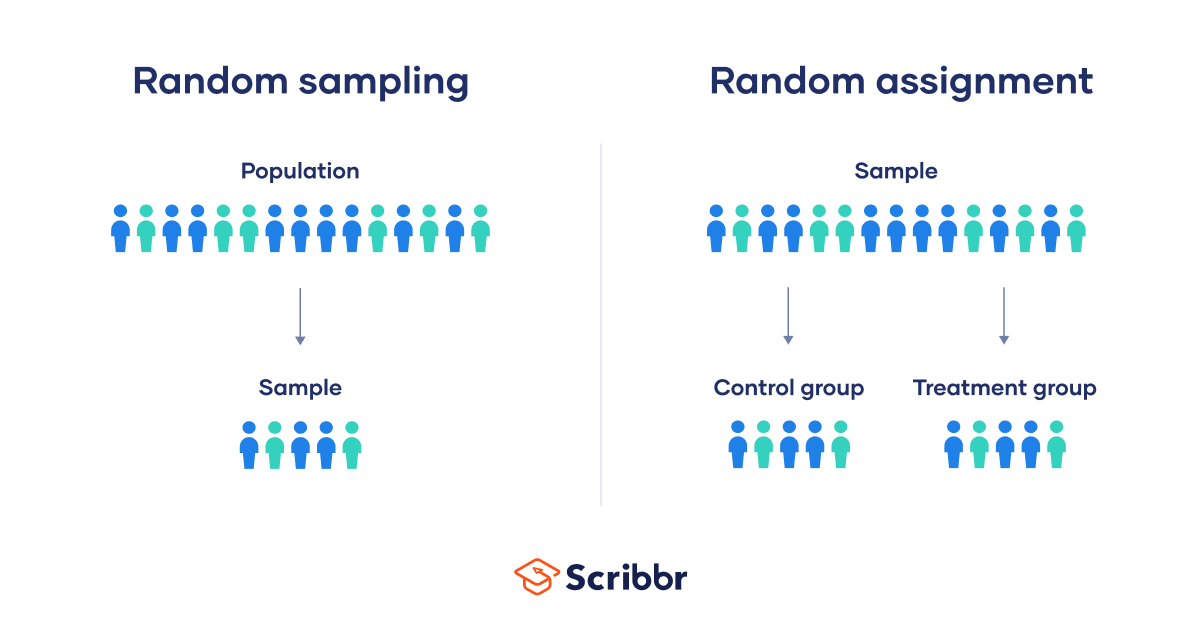
Random sampling enhances the external validity or generalizability of your results, because it helps ensure that your sample is unbiased and representative of the whole population. This allows you to make stronger statistical inferences .
You use a simple random sample to collect data. Because you have access to the whole population (all employees), you can assign all 8000 employees a number and use a random number generator to select 300 employees. These 300 employees are your full sample.
Random assignment enhances the internal validity of the study, because it ensures that there are no systematic differences between the participants in each group. This helps you conclude that the outcomes can be attributed to the independent variable .
- a control group that receives no intervention.
- an experimental group that has a remote team-building intervention every week for a month.
You use random assignment to place participants into the control or experimental group. To do so, you take your list of participants and assign each participant a number. Again, you use a random number generator to place each participant in one of the two groups.
To use simple random assignment, you start by giving every member of the sample a unique number. Then, you can use computer programs or manual methods to randomly assign each participant to a group.
- Random number generator: Use a computer program to generate random numbers from the list for each group.
- Lottery method: Place all numbers individually in a hat or a bucket, and draw numbers at random for each group.
- Flip a coin: When you only have two groups, for each number on the list, flip a coin to decide if they’ll be in the control or the experimental group.
- Use a dice: When you have three groups, for each number on the list, roll a dice to decide which of the groups they will be in. For example, assume that rolling 1 or 2 lands them in a control group; 3 or 4 in an experimental group; and 5 or 6 in a second control or experimental group.
This type of random assignment is the most powerful method of placing participants in conditions, because each individual has an equal chance of being placed in any one of your treatment groups.
Random assignment in block designs
In more complicated experimental designs, random assignment is only used after participants are grouped into blocks based on some characteristic (e.g., test score or demographic variable). These groupings mean that you need a larger sample to achieve high statistical power .
For example, a randomized block design involves placing participants into blocks based on a shared characteristic (e.g., college students versus graduates), and then using random assignment within each block to assign participants to every treatment condition. This helps you assess whether the characteristic affects the outcomes of your treatment.
In an experimental matched design , you use blocking and then match up individual participants from each block based on specific characteristics. Within each matched pair or group, you randomly assign each participant to one of the conditions in the experiment and compare their outcomes.
Sometimes, it’s not relevant or ethical to use simple random assignment, so groups are assigned in a different way.
When comparing different groups
Sometimes, differences between participants are the main focus of a study, for example, when comparing men and women or people with and without health conditions. Participants are not randomly assigned to different groups, but instead assigned based on their characteristics.
In this type of study, the characteristic of interest (e.g., gender) is an independent variable, and the groups differ based on the different levels (e.g., men, women, etc.). All participants are tested the same way, and then their group-level outcomes are compared.
When it’s not ethically permissible
When studying unhealthy or dangerous behaviors, it’s not possible to use random assignment. For example, if you’re studying heavy drinkers and social drinkers, it’s unethical to randomly assign participants to one of the two groups and ask them to drink large amounts of alcohol for your experiment.
When you can’t assign participants to groups, you can also conduct a quasi-experimental study . In a quasi-experiment, you study the outcomes of pre-existing groups who receive treatments that you may not have any control over (e.g., heavy drinkers and social drinkers). These groups aren’t randomly assigned, but may be considered comparable when some other variables (e.g., age or socioeconomic status) are controlled for.
Here's why students love Scribbr's proofreading services
Discover proofreading & editing
If you want to know more about statistics , methodology , or research bias , make sure to check out some of our other articles with explanations and examples.
- Student’s t -distribution
- Normal distribution
- Null and Alternative Hypotheses
- Chi square tests
- Confidence interval
- Quartiles & Quantiles
- Cluster sampling
- Stratified sampling
- Data cleansing
- Reproducibility vs Replicability
- Peer review
- Prospective cohort study
Research bias
- Implicit bias
- Cognitive bias
- Placebo effect
- Hawthorne effect
- Hindsight bias
- Affect heuristic
- Social desirability bias
In experimental research, random assignment is a way of placing participants from your sample into different groups using randomization. With this method, every member of the sample has a known or equal chance of being placed in a control group or an experimental group.
Random selection, or random sampling , is a way of selecting members of a population for your study’s sample.
In contrast, random assignment is a way of sorting the sample into control and experimental groups.
Random sampling enhances the external validity or generalizability of your results, while random assignment improves the internal validity of your study.
Random assignment is used in experiments with a between-groups or independent measures design. In this research design, there’s usually a control group and one or more experimental groups. Random assignment helps ensure that the groups are comparable.
In general, you should always use random assignment in this type of experimental design when it is ethically possible and makes sense for your study topic.
To implement random assignment , assign a unique number to every member of your study’s sample .
Then, you can use a random number generator or a lottery method to randomly assign each number to a control or experimental group. You can also do so manually, by flipping a coin or rolling a dice to randomly assign participants to groups.
Cite this Scribbr article
If you want to cite this source, you can copy and paste the citation or click the “Cite this Scribbr article” button to automatically add the citation to our free Citation Generator.
Bhandari, P. (2023, June 22). Random Assignment in Experiments | Introduction & Examples. Scribbr. Retrieved April 2, 2024, from https://www.scribbr.com/methodology/random-assignment/
Is this article helpful?
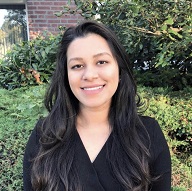
Pritha Bhandari
Other students also liked, guide to experimental design | overview, steps, & examples, confounding variables | definition, examples & controls, control groups and treatment groups | uses & examples, what is your plagiarism score.
Your session is about to expire
Clinical trial basics: randomization in clinical trials, introduction.
Clinical trials represent a core pillar of advancing patient care and medical knowledge. Clinical trials are designed to thoroughly assess the effectiveness and safety of new drugs and treatments in the human population. There are 4 main phases of clinical trials, each with its own objectives and questions, and they can be designed in different ways depending on the study population, the treatment being tested, and the specific research hypotheses. The “gold standard” of clinical research are randomized controlled trials (RCTs), which aim to avoid bias by randomly assigning patients into different groups, which can then be compared to evaluate the new drug or treatment. The process of random assignment of patients to groups is called randomization.
Randomization in clinical trials is an essential concept for minimizing bias, ensuring fairness, and maximizing the statistical power of the study results. In this article, we will discuss the concept of randomization in clinical trials, why it is important, and go over some of the different randomization methods that are commonly used.
What does randomization mean in clinical trials?
Randomization in clinical trials involves assigning patients into two or more study groups according to a chosen randomization protocol (randomization method). Randomizing patients allows for directly comparing the outcomes between the different groups, thereby providing stronger evidence for any effects seen being a result of the treatment rather than due to chance or random variables.
What is the main purpose of randomization?
Randomization is considered a key element in clinical trials for ensuring unbiased treatment of patients and obtaining reliable, scientifically valuable results. [1] Randomization is important for generating comparable intervention groups and for ensuring that all patients have an equal chance of receiving the novel treatment under study. The systematic rule for the randomization process (known as “sequence generation”) reduces selection bias that could arise if researchers were to manually assign patients with better prognoses to specific study groups; steps must be taken to further ensure strict implementation of the sequence by preventing researchers and patients from knowing beforehand which group patients are destined for (known as “allocation sequence concealment”). [2]
Randomization also aims to remove the influence of external and prognostic variables to increase the statistical power of the results. Some researchers are opposed to randomization, instead supporting the use of statistical techniques such as analysis of covariance (ANCOVA) and multivariate ANCOVA to adjust for covariate imbalance after the study is completed, in the analysis stage. However, this post-adjustment approach might not be an ideal fit for every clinical trial because the researcher might be unaware of certain prognostic variables that could lead to unforeseen interaction effects and contaminate the data. Thus, the best way to avoid bias and the influence of external variables and thereby ensure the validity of statistical test results is to apply randomization in the clinical trial design stage.
Randomized controlled trials (RCTs): The ‘gold standard’
Randomized controlled trials, or RCTs, are considered the “gold standard” of clinical research because, by design, they feature minimized bias, high statistical power, and a strong ability to provide evidence that any clinical benefit observed results specifically from the study intervention (i.e., identifying cause-effect relationships between the intervention and the outcome).[3] A randomized controlled trial is one of the most effective studies for measuring the effectiveness of a new drug or intervention.
How are participants randomized? An introduction to randomization methods
Randomization includes a broad class of design techniques for clinical trials, and is not a single methodology. For randomization to be effective and reduce (rather than introduce) bias, a randomization schedule is required for assigning patients in an unbiased and systematic manner. Below is a brief overview of the main randomization techniques commonly used; further detail is given in the next sections.
Fixed vs. adaptive randomization
Randomization methods can be divided into fixed and adaptive randomization. Fixed randomization involves allocating patients to interventions using a fixed sequence that doesn’t change throughout the study. On the other hand, adaptive randomization involves assigning patients to groups in consideration of the characteristics of the patients already in the trial, and the randomization probabilities can change over the course of the study. Each of these techniques can be further subdivided:
Fixed allocation randomization methods:
- Simple randomization : the simplest method of randomization, in which patient allocation is based on a single sequence of random assignments.
- Block randomization : patients are first assigned to blocks of equal size, and then randomized within each block. This ensures balance in group sizes.
Stratified randomization : patients are first allocated to blocks (strata) designed to balance combinations of specific covariates (subject’s baseline characteristics), and then randomization is performed within each stratum.
Adaptive randomization methods:
- Outcome-adaptive/results-adaptive randomization : involves allocating patients to study groups in consideration of other patients’ responses to the ongoing trial treatment. Minimization: involves minimizing imbalance amongst covariates by allocating new enrollments as a function of prior allocations
Below is a graphic summary of the breakdown we’ve just covered.
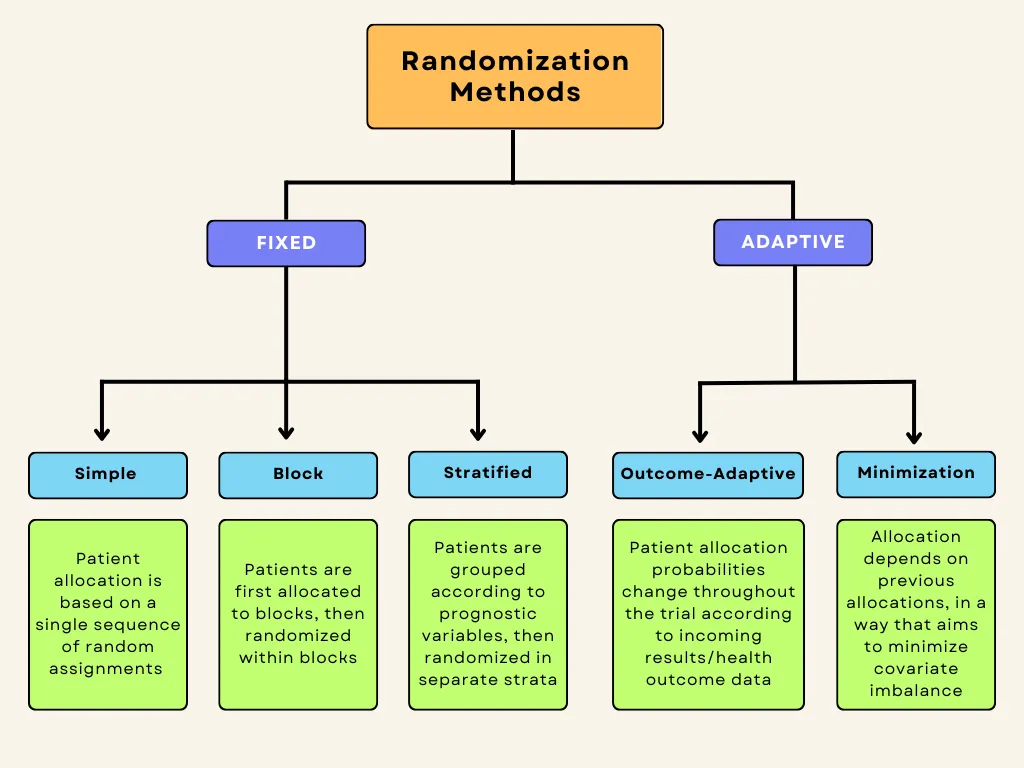
Fixed-allocation randomization in clinical trials
Here, we will discuss the three main fixed-allocation randomization types in more detail.
Simple Randomization
Simple randomization is the most commonly used method of fixed randomization, offering completely random patient allocation into the different study groups. It is based on a single sequence of random assignments and is not influenced by previous assignments. The benefits are that it is simple and it fulfills the allocation concealment requirement, ensuring that researchers, sponsors, and patients are unaware of which patient will be assigned to which treatment group. Simple randomization can be conceptualized, or even performed, by the following chance actions:
- Flipping a coin (e.g., heads → control / tails → intervention)
- Throwing a dice (e.g., 1-3 → control / 4-6 → intervention)
- Using a deck of shuffled cards (e.g., red → control / black → intervention)
- Using a computer-generated random number sequence
- Using a random number table from a statistics textbook
There are certain disadvantages associated with simple randomization, namely that it does not take into consideration the influence of covariates, and it may lead to unequal sample sizes between groups. For clinical research studies with small sample sizes, the group sizes are more likely to be unequal.
Especially in smaller clinical trials, simple randomization can lead to covariate imbalance. It has been suggested that clinical trials enrolling at least 1,000 participants can essentially avoid random differences between treatment groups and minimize bias by using simple randomization. [4] On the other hand, the risks posed by imbalances in covariates and prognostic factors are more relevant in smaller clinical trials employing simple randomization, and thus, other methods such as blocking should be considered for such trials.
Block Randomization
Block randomization is a type of “constrained randomization” that is preferred for achieving balance in the sizes of treatment groups in smaller clinical trials. The first step is to select a block size. Blocks represent “subgroups” of participants who will be randomized in these subgroups, or blocks. Block size should be a multiple of the number of groups; for instance, if there are two groups, the block size can be 4, 6, 8, etc. Once block size is determined, then all possible different combinations (permutations) of assignment within the block are identified. Each block is then randomly assigned one of these permutations, and individuals in the block are allocated according to the specific pattern of the permuted block.[5]
Let’s consider a small clinical trial with two study groups (control and treatment) and 20 participants. In this situation, an allocation sequence based on blocked randomization would involve the following steps:
1. The researcher chooses block size: In this case, we will use a block size of 4 (which is a multiple of the number of study groups, 2).
2. All 6 possible balanced combinations of control (C) and treatment (T) allocations within each block are shown as follows:
3. These allocation sequences are randomly assigned to the blocks, which then determine the assignment of the 4 participants within each block. Let’s say the sequence TCCT is selected for block 1. The allocation would then be as follows:
- Participant 1 → Treatment (T)
- Participant 2 → Control (C)
- Participant 3 → Control (C)
- Participant 4 → Treatment (T)
We can see that blocked randomization ensures equal (or nearly equal, if for example enrollment is terminated early or the final target is not quite met) assignment to treatment groups.
There are disadvantages to blocked randomization. For one, if the investigators/researchers are not blinded (masked), then the condition of allocation concealment is not met, which could lead to selection bias. To illustrate this, let’s say that two of four participants have enrolled in block 2 of the above example, for which the randomly selected sequence is CCTT. In this case, the investigator would know that the next two participants for the current block would be assigned to the treatment group (T), which could influence his/her selection process. Keeping the investigator masked (blinded) or utilizing random block sizes are potential solutions for preventing this issue. Another drawback is that the determined blocks may still contain covariate imbalances. For instance, one block might have more participants with chronic or secondary illnesses.
Despite these drawbacks, block randomization is simple to implement and better than simple randomization for smaller clinical trials in that treatment groups will have an equal number of participants. Blinding researchers to block size or randomizing block size can reduce potential selection bias. [5]
Stratified Randomization
Stratified randomization aims to prevent imbalances amongst prognostic variables (or the patients’ baseline characteristics, also known as covariates) in the study groups. Stratified randomization is another type of constrained randomization, where participants are first grouped (“stratified”) into strata based on predetermined covariates, which could include such things as age, sex, comorbidities, etc. Block randomization is then applied within each of these strata separately, ensuring balance amongst prognostic variables as well as in group size.
The covariates of interest are determined by the researchers before enrollment begins, and are chosen based on the potential influence of each covariate on the dependent variable. Each covariate will have a given number of levels, and the product of the number of levels of all covariates determines the number of strata for the trial. For example, if two covariates are identified for a trial, each with two levels (let’s say age, divided into two levels [<50 and 50+], and height [<175 cm and 176+ cm]), a total of 4 strata will be created (2 x 2 = 4).
Patients are first assigned to the appropriate stratum according to these prognostic classifications, and then a randomization sequence is applied within each stratum. Block randomization is usually applied in order to guarantee balance between treatment groups in each stratum.
Stratified randomization can thus prevent covariate imbalance, which can be especially important in smaller clinical trials with few participants. [6] Nonetheless, stratification and imbalance control can become complex if too many covariates are considered, because an overly large number of strata can lead to imbalances in patient allocation due to small sample sizes within individual strata,. Thus, the number of strata should be kept to a minimum for the best results – in other words, only covariates with potentially important influences on study outcomes and results should be included. [6] Stratified randomization also reduces type I error, which describes “false positive” results, wherein differences in treatment outcomes are observed between groups despite the treatments being equal (for example, if the intervention group contained participants with overall better prognosis, it could be concluded that the intervention was effective, although in reality the effect was only due to their better initial prognosis and not the treatment). [5] Type II errors are also reduced, which describe “false negatives,” wherein actual differences in outcomes between groups are not noticed. The “power” of a trial to identify treatment effects is inversely related to these errors, which are related to variance between groups being compared; stratification reduces variance between groups and thus theoretically increases power. The required sample size decreases as power increases, which can also be used to explain why stratification is relatively more impactful with smaller sample sizes.
A major drawback of stratified randomization is that it requires identification of all participants before they can be allocated. Its utility is also disputed by some researchers, especially in the context of trials with large sample sizes, wherein covariates are more likely to be balanced naturally even when using simpler randomization techniques. [6]
Adaptive randomization in clinical trials
Adaptive randomization describes schemes in which treatment allocation probabilities are adjusted as the trial progresses.In adaptive randomization,allocation probabilities can be altered in order to either minimize imbalances in prognostic variables (covariate-adaptive randomization, or “minimization”), or to increase the allocation of patients to the treatment arm(s) showing better patient outcomes (“response-adaptive randomization”). [7] Adaptive randomization methods can thus address the issue of covariate imbalance, or can be employed to offer a unique ethical advantage in studies wherein preliminary or interim analyses indicate that one treatment arm is significantly more effective, maximizing potential therapeutic benefit for patients by increasing allocation to the most-effective treatment arm.
One of the main disadvantages associated with adaptive randomization methods is that they are time-consuming and complex; recalculation is necessary for each new patient or when any treatment arm is terminated.
Outcome-adaptive (response-adaptive) randomization
Outcome-adaptive randomization was first proposed in 1969 as “play-the-winner” treatment assignments. [8] This method involves adjusting the allocation probabilities based on the data and results being collected in the ongoing trial. The aim is to increase the ratio of patients being assigned to the more effective treatment arm, representing a significant ethical advantage especially for trials in which one or more treatments are clearly demonstrating promising therapeutic benefit. The maximization of therapeutic benefit for participants comes at the expense of statistical power, which is one of the major drawbacks of this randomization method.
Outcome-adaptive randomization can decrease power because, by increasing the allocation of participants to the more-effective treatment arm, which then in turn demonstrates better outcomes, an increasing bias toward that treatment arm is created. Thus, outcome-adaptive randomization is unsuitable for long-term phase III clinical trials requiring high statistical power, and some argue that the high design complexity is not warranted as the benefits offered are minimal (or can be achieved through other designs). [8]
Covariate-adaptive randomization (Minimization)
Minimization is a complex form of adaptive randomization which, similarly to stratified randomization, aims to maximize the balance amongst covariates between treatment groups. Rather than achieving this by initially stratifying participants into separate strata based on covariates and then randomizing, the first participants are allocated randomly and then each new allocation involves hypothetically allocating the new participant to all groups and calculating a resultant “imbalance score.” The participant will then be assigned in such a way that this covariate imbalance is minimized (hence the name minimization). [9]
A principal drawback of minimization is that it is labor-intensive due to frequent recalculation of imbalance scores as new patients enroll in the trial. However, there are web-based tools and computer programs that can be used to facilitate the recalculation and allocation processes. [10]
Randomization in clinical trials is important as it ensures fair allocation of patients to study groups and enables researchers to make accurate and valid comparisons. The choice of the specific randomization schedule will depend on the trial type/phase, sample size, research objectives, and the condition being treated. A balance should be sought between ease of implementation, prevention of bias, and maximization of power. To further minimize bias, considerations such as blinding and allocation concealment should be combined with randomization techniques.
Other Trials to Consider
Dolutegravir 5 mg Dispersible Tablets
Pegylated interferon alpha2a, order: period a, period b, period c, pet/ct imaging with [18f]ctt1057 followed by [68ga]ga-psma-11 or vice versa, registry for flow diversion, online therapy with coaching, telemedicine, 900 +/- 15nm/ 830 +/- 15nm/ 770 +/- 9nm, waterpipe regular smokers, popular categories.
Tymlos Clinical Trials
Paid Clinical Trials in Cincinnati, OH
Paid Clinical Trials in Omaha, NE
Paid Clinical Trials in Meridian, ID
Paid Clinical Trials in New York
Paid Clinical Trials in Tennessee
Paid Clinical Trials in New Mexico
Paid Clinical Trials in Alaska
Paid Clinical Trials in Wyoming
Forteo Clinical Trials
Popular guides.
- Open access
- Published: 16 August 2021
A roadmap to using randomization in clinical trials
- Vance W. Berger 1 ,
- Louis Joseph Bour 2 ,
- Kerstine Carter 3 ,
- Jonathan J. Chipman ORCID: orcid.org/0000-0002-3021-2376 4 , 5 ,
- Colin C. Everett ORCID: orcid.org/0000-0002-9788-840X 6 ,
- Nicole Heussen ORCID: orcid.org/0000-0002-6134-7206 7 , 8 ,
- Catherine Hewitt ORCID: orcid.org/0000-0002-0415-3536 9 ,
- Ralf-Dieter Hilgers ORCID: orcid.org/0000-0002-5945-1119 7 ,
- Yuqun Abigail Luo 10 ,
- Jone Renteria 11 , 12 ,
- Yevgen Ryeznik ORCID: orcid.org/0000-0003-2997-8566 13 ,
- Oleksandr Sverdlov ORCID: orcid.org/0000-0002-1626-2588 14 &
- Diane Uschner ORCID: orcid.org/0000-0002-7858-796X 15
for the Randomization Innovative Design Scientific Working Group
BMC Medical Research Methodology volume 21 , Article number: 168 ( 2021 ) Cite this article
24k Accesses
34 Citations
14 Altmetric
Metrics details
Randomization is the foundation of any clinical trial involving treatment comparison. It helps mitigate selection bias, promotes similarity of treatment groups with respect to important known and unknown confounders, and contributes to the validity of statistical tests. Various restricted randomization procedures with different probabilistic structures and different statistical properties are available. The goal of this paper is to present a systematic roadmap for the choice and application of a restricted randomization procedure in a clinical trial.
We survey available restricted randomization procedures for sequential allocation of subjects in a randomized, comparative, parallel group clinical trial with equal (1:1) allocation. We explore statistical properties of these procedures, including balance/randomness tradeoff, type I error rate and power. We perform head-to-head comparisons of different procedures through simulation under various experimental scenarios, including cases when common model assumptions are violated. We also provide some real-life clinical trial examples to illustrate the thinking process for selecting a randomization procedure for implementation in practice.
Restricted randomization procedures targeting 1:1 allocation vary in the degree of balance/randomness they induce, and more importantly, they vary in terms of validity and efficiency of statistical inference when common model assumptions are violated (e.g. when outcomes are affected by a linear time trend; measurement error distribution is misspecified; or selection bias is introduced in the experiment). Some procedures are more robust than others. Covariate-adjusted analysis may be essential to ensure validity of the results. Special considerations are required when selecting a randomization procedure for a clinical trial with very small sample size.
Conclusions
The choice of randomization design, data analytic technique (parametric or nonparametric), and analysis strategy (randomization-based or population model-based) are all very important considerations. Randomization-based tests are robust and valid alternatives to likelihood-based tests and should be considered more frequently by clinical investigators.
Peer Review reports
Various research designs can be used to acquire scientific medical evidence. The randomized controlled trial (RCT) has been recognized as the most credible research design for investigations of the clinical effectiveness of new medical interventions [ 1 , 2 ]. Evidence from RCTs is widely used as a basis for submissions of regulatory dossiers in request of marketing authorization for new drugs, biologics, and medical devices. Three important methodological pillars of the modern RCT include blinding (masking), randomization, and the use of control group [ 3 ].
While RCTs provide the highest standard of clinical evidence, they are laborious and costly, in terms of both time and material resources. There are alternative designs, such as observational studies with either a cohort or case–control design, and studies using real world evidence (RWE). When properly designed and implemented, observational studies can sometimes produce similar estimates of treatment effects to those found in RCTs, and furthermore, such studies may be viable alternatives to RCTs in many settings where RCTs are not feasible and/or not ethical. In the era of big data, the sources of clinically relevant data are increasingly rich and include electronic health records, data collected from wearable devices, health claims data, etc. Big data creates vast opportunities for development and implementation of novel frameworks for comparative effectiveness research [ 4 ], and RWE studies nowadays can be implemented rapidly and relatively easily. But how credible are the results from such studies?
In 1980, D. P. Byar issued warnings and highlighted potential methodological problems with comparison of treatment effects using observational databases [ 5 ]. Many of these issues still persist and actually become paramount during the ongoing COVID-19 pandemic when global scientific efforts are made to find safe and efficacious vaccines and treatments as soon as possible. While some challenges pertinent to RWE studies are related to the choice of proper research methodology, some additional challenges arise from increasing requirements of health authorities and editorial boards of medical journals for the investigators to present evidence of transparency and reproducibility of their conducted clinical research. Recently, two top medical journals, the New England Journal of Medicine and the Lancet, retracted two COVID-19 studies that relied on observational registry data [ 6 , 7 ]. The retractions were made at the request of the authors who were unable to ensure reproducibility of the results [ 8 ]. Undoubtedly, such cases are harmful in many ways. The already approved drugs may be wrongly labeled as “toxic” or “inefficacious”, and the reputation of the drug developers could be blemished or destroyed. Therefore, the highest standards for design, conduct, analysis, and reporting of clinical research studies are now needed more than ever. When treatment effects are modest, yet still clinically meaningful, a double-blind, randomized, controlled clinical trial design helps detect these differences while adjusting for possible confounders and adequately controlling the chances of both false positive and false negative findings.
Randomization in clinical trials has been an important area of methodological research in biostatistics since the pioneering work of A. Bradford Hill in the 1940’s and the first published randomized trial comparing streptomycin with a non-treatment control [ 9 ]. Statisticians around the world have worked intensively to elaborate the value, properties, and refinement of randomization procedures with an incredible record of publication [ 10 ]. In particular, a recent EU-funded project ( www.IDeAl.rwth-aachen.de ) on innovative design and analysis of small population trials has “randomization” as one work package. In 2020, a group of trial statisticians around the world from different sectors formed a subgroup of the Drug Information Association (DIA) Innovative Designs Scientific Working Group (IDSWG) to raise awareness of the full potential of randomization to improve trial quality, validity and rigor ( https://randomization-working-group.rwth-aachen.de/ ).
The aims of the current paper are three-fold. First, we describe major recent methodological advances in randomization, including different restricted randomization designs that have superior statistical properties compared to some widely used procedures such as permuted block designs. Second, we discuss different types of experimental biases in clinical trials and explain how a carefully chosen randomization design can mitigate risks of these biases. Third, we provide a systematic roadmap for evaluating different restricted randomization procedures and selecting an “optimal” one for a particular trial. We also showcase application of these ideas through several real life RCT examples.
The target audience for this paper would be clinical investigators and biostatisticians who are tasked with the design, conduct, analysis, and interpretation of clinical trial results, as well as regulatory and scientific/medical journal reviewers. Recognizing the breadth of the concept of randomization, in this paper we focus on a randomized, comparative, parallel group clinical trial design with equal (1:1) allocation, which is typically implemented using some restricted randomization procedure, possibly stratified by some important baseline prognostic factor(s) and/or study center. Some of our findings and recommendations are generalizable to more complex clinical trial settings. We shall highlight these generalizations and outline additional important considerations that fall outside the scope of the current paper.
The paper is organized as follows. The “ Methods ” section provides some general background on the methodology of randomization in clinical trials, describes existing restricted randomization procedures, and discusses some important criteria for comparison of these procedures in practice. In the “ Results ” section, we present our findings from four simulation studies that illustrate the thinking process when evaluating different randomization design options at the study planning stage. The “ Conclusions ” section summarizes the key findings and important considerations on restricted randomization procedures, and it also highlights some extensions and further topics on randomization in clinical trials.
What is randomization and what are its virtues in clinical trials?
Randomization is an essential component of an experimental design in general and clinical trials in particular. Its history goes back to R. A. Fisher and his classic book “The Design of Experiments” [ 11 ]. Implementation of randomization in clinical trials is due to A. Bradford Hill who designed the first randomized clinical trial evaluating the use of streptomycin in treating tuberculosis in 1946 [ 9 , 12 , 13 ].
Reference [ 14 ] provides a good summary of the rationale and justification for the use of randomization in clinical trials. The randomized controlled trial (RCT) has been referred to as “the worst possible design (except for all the rest)” [ 15 ], indicating that the benefits of randomization should be evaluated in comparison to what we are left with if we do not randomize. Observational studies suffer from a wide variety of biases that may not be adequately addressed even using state-of-the-art statistical modeling techniques.
The RCT in the medical field has several features that distinguish it from experimental designs in other fields, such as agricultural experiments. In the RCT, the experimental units are humans, and in the medical field often diagnosed with a potentially fatal disease. These subjects are sequentially enrolled for participation in the study at selected study centers, which have relevant expertise for conducting clinical research. Many contemporary clinical trials are run globally, at multiple research institutions. The recruitment period may span several months or even years, depending on a therapeutic indication and the target patient population. Patients who meet study eligibility criteria must sign the informed consent, after which they are enrolled into the study and, for example, randomized to either experimental treatment E or the control treatment C according to the randomization sequence. In this setup, the choice of the randomization design must be made judiciously, to protect the study from experimental biases and ensure validity of clinical trial results.
The first virtue of randomization is that, in combination with allocation concealment and masking, it helps mitigate selection bias due to an investigator’s potential to selectively enroll patients into the study [ 16 ]. A non-randomized, systematic design such as a sequence of alternating treatment assignments has a major fallacy: an investigator, knowing an upcoming treatment assignment in a sequence, may enroll a patient who, in their opinion, would be best suited for this treatment. Consequently, one of the groups may contain a greater number of “sicker” patients and the estimated treatment effect may be biased. Systematic covariate imbalances may increase the probability of false positive findings and undermine the integrity of the trial. While randomization alleviates the fallacy of a systematic design, it does not fully eliminate the possibility of selection bias (unless we consider complete randomization for which each treatment assignment is determined by a flip of a coin, which is rarely, if ever used in practice [ 17 ]). Commonly, RCTs employ restricted randomization procedures which sequentially balance treatment assignments while maintaining allocation randomness. A popular choice is the permuted block design that controls imbalance by making treatment assignments at random in blocks. To minimize potential for selection bias, one should avoid overly restrictive randomization schemes such as permuted block design with small block sizes, as this is very similar to alternating treatment sequence.
The second virtue of randomization is its tendency to promote similarity of treatment groups with respect to important known, but even more importantly, unknown confounders. If treatment assignments are made at random, then by the law of large numbers, the average values of patient characteristics should be approximately equal in the experimental and the control groups, and any observed treatment difference should be attributed to the treatment effects, not the effects of the study participants [ 18 ]. However, one can never rule out the possibility that the observed treatment difference is due to chance, e.g. as a result of random imbalance in some patient characteristics [ 19 ]. Despite that random covariate imbalances can occur in clinical trials of any size, such imbalances do not compromise the validity of statistical inference, provided that proper statistical techniques are applied in the data analysis.
Several misconceptions on the role of randomization and balance in clinical trials were documented and discussed by Senn [ 20 ]. One common misunderstanding is that balance of prognostic covariates is necessary for valid inference. In fact, different randomization designs induce different extent of balance in the distributions of covariates, and for a given trial there is always a possibility of observing baseline group differences. A legitimate approach is to pre-specify in the protocol the clinically important covariates to be adjusted for in the primary analysis, apply a randomization design (possibly accounting for selected covariates using pre-stratification or some other approach), and perform a pre-planned covariate-adjusted analysis (such as analysis of covariance for a continuous primary outcome), verifying the model assumptions and conducting additional supportive/sensitivity analyses, as appropriate. Importantly, the pre-specified prognostic covariates should always be accounted for in the analysis, regardless whether their baseline differences are present or not [ 20 ].
It should be noted that some randomization designs (such as covariate-adaptive randomization procedures) can achieve very tight balance of covariate distributions between treatment groups [ 21 ]. While we address randomization within pre-specified stratifications, we do not address more complex covariate- and response-adaptive randomization in this paper.
Finally, randomization plays an important role in statistical analysis of the clinical trial. The most common approach to inference following the RCT is the invoked population model [ 10 ]. With this approach, one posits that there is an infinite target population of patients with the disease, from which \(n\) eligible subjects are sampled in an unbiased manner for the study and are randomized to the treatment groups. Within each group, the responses are assumed to be independent and identically distributed (i.i.d.), and inference on the treatment effect is performed using some standard statistical methodology, e.g. a two sample t-test for normal outcome data. The added value of randomization is that it makes the assumption of i.i.d. errors more feasible compared to a non-randomized study because it introduces a real element of chance in the allocation of patients.
An alternative approach is the randomization model , in which the implemented randomization itself forms the basis for statistical inference [ 10 ]. Under the null hypothesis of the equality of treatment effects, individual outcomes (which are regarded as not influenced by random variation, i.e. are considered as fixed) are not affected by treatment. Treatment assignments are permuted in all possible ways consistent with the randomization procedure actually used in the trial. The randomization-based p- value is the sum of null probabilities of the treatment assignment permutations in the reference set that yield the test statistic values greater than or equal to the experimental value. A randomization-based test can be a useful supportive analysis, free of assumptions of parametric tests and protective against spurious significant results that may be caused by temporal trends [ 14 , 22 ].
It is important to note that Bayesian inference has also become a common statistical analysis in RCTs [ 23 ]. Although the inferential framework relies upon subjective probabilities, a study analyzed through a Bayesian framework still relies upon randomization for the other aforementioned virtues [ 24 ]. Hence, the randomization considerations discussed herein have broad application.
What types of randomization methodologies are available?
Randomization is not a single methodology, but a very broad class of design techniques for the RCT [ 10 ]. In this paper, we consider only randomization designs for sequential enrollment clinical trials with equal (1:1) allocation in which randomization is not adapted for covariates and/or responses. The simplest procedure for an RCT is complete randomization design (CRD) for which each subject’s treatment is determined by a flip of a fair coin [ 25 ]. CRD provides no potential for selection bias (e.g. based on prediction of future assignments) but it can result, with non-negligible probability, in deviations from the 1:1 allocation ratio and covariate imbalances, especially in small samples. This may lead to loss of statistical efficiency (decrease in power) compared to the balanced design. In practice, some restrictions on randomization are made to achieve balanced allocation. Such randomization designs are referred to as restricted randomization procedures [ 26 , 27 ].
Suppose we plan to randomize an even number of subjects \(n\) sequentially between treatments E and C. Two basic designs that equalize the final treatment numbers are the random allocation rule (Rand) and the truncated binomial design (TBD), which were discussed in the 1957 paper by Blackwell and Hodges [ 28 ]. For Rand, any sequence of exactly \(n/2\) E’s and \(n/2\) C’s is equally likely. For TBD, treatment assignments are made with probability 0.5 until one of the treatments receives its quota of \(n/2\) subjects; thereafter all remaining assignments are made deterministically to the opposite treatment.
A common feature of both Rand and TBD is that they aim at the final balance, whereas at intermediate steps it is still possible to have substantial imbalances, especially if \(n\) is large. A long run of a single treatment in a sequence may be problematic if there is a time drift in some important covariate, which can lead to chronological bias [ 29 ]. To mitigate this risk, one can further restrict randomization so that treatment assignments are balanced over time. One common approach is the permuted block design (PBD) [ 30 ], for which random treatment assignments are made in blocks of size \(2b\) ( \(b\) is some small positive integer), with exactly \(b\) allocations to each of the treatments E and C. The PBD is perhaps the oldest (it can be traced back to A. Bradford Hill’s 1951 paper [ 12 ]) and the most widely used randomization method in clinical trials. Often its choice in practice is justified by simplicity of implementation and the fact that it is referenced in the authoritative ICH E9 guideline on statistical principles for clinical trials [ 31 ]. One major challenge with PBD is the choice of the block size. If \(b=1\) , then every pair of allocations is balanced, but every even allocation is deterministic. Larger block sizes increase allocation randomness. The use of variable block sizes has been suggested [ 31 ]; however, PBDs with variable block sizes are also quite predictable [ 32 ]. Another problematic feature of the PBD is that it forces periodic return to perfect balance, which may be unnecessary from the statistical efficiency perspective and may increase the risk of prediction of upcoming allocations.
More recent and better alternatives to the PBD are the maximum tolerated imbalance (MTI) procedures [ 33 , 34 , 35 , 36 , 37 , 38 , 39 , 40 , 41 ]. These procedures provide stronger encryption of the randomization sequence (i.e. make it more difficult to predict future treatment allocations in the sequence even knowing the current sizes of the treatment groups) while controlling treatment imbalance at a pre-defined threshold throughout the experiment. A general MTI procedure specifies a certain boundary for treatment imbalance, say \(b>0\) , that cannot be exceeded. If, at a given allocation step the absolute value of imbalance is equal to \(b\) , then one next allocation is deterministically forced toward balance. This is in contrast to PBD which, after reaching the target quota of allocations for either treatment within a block, forces all subsequent allocations to achieve perfect balance at the end of the block. Some notable MTI procedures are the big stick design (BSD) proposed by Soares and Wu in 1983 [ 37 ], the maximal procedure proposed by Berger, Ivanova and Knoll in 2003 [ 35 ], the block urn design (BUD) proposed by Zhao and Weng in 2011 [ 40 ], just to name a few. These designs control treatment imbalance within pre-specified limits and are more immune to selection bias than the PBD [ 42 , 43 ].
Another important class of restricted randomization procedures is biased coin designs (BCDs). Starting with the seminal 1971 paper of Efron [ 44 ], BCDs have been a hot research topic in biostatistics for 50 years. Efron’s BCD is very simple: at any allocation step, if treatment numbers are balanced, the next assignment is made with probability 0.5; otherwise, the underrepresented treatment is assigned with probability \(p\) , where \(0.5<p\le 1\) is a fixed and pre-specified parameter that determines the tradeoff between balance and randomness. Note that \(p=1\) corresponds to PBD with block size 2. If we set \(p<1\) (e.g. \(p=2/3\) ), then the procedure has no deterministic assignments and treatment allocation will be concentrated around 1:1 with high probability [ 44 ]. Several extensions of Efron’s BCD providing better tradeoff between treatment balance and allocation randomness have been proposed [ 45 , 46 , 47 , 48 , 49 ]; for example, a class of adjustable biased coin designs introduced by Baldi Antognini and Giovagnoli in 2004 [ 49 ] unifies many BCDs in a single framework. A comprehensive simulation study comparing different BCDs has been published by Atkinson in 2014 [ 50 ].
Finally, urn models provide a useful mechanism for RCT designs [ 51 ]. Urn models apply some probabilistic rules to sequentially add/remove balls (representing different treatments) in the urn, to balance treatment assignments while maintaining the randomized nature of the experiment [ 39 , 40 , 52 , 53 , 54 , 55 ]. A randomized urn design for balancing treatment assignments was proposed by Wei in 1977 [ 52 ]. More novel urn designs, such as the drop-the-loser urn design developed by Ivanova in 2003 [ 55 ] have reduced variability and can attain the target treatment allocation more efficiently. Many urn designs involve parameters that can be fine-tuned to obtain randomization procedures with desirable balance/randomness tradeoff [ 56 ].
What are the attributes of a good randomization procedure?
A “good” randomization procedure is one that helps successfully achieve the study objective(s). Kalish and Begg [ 57 ] state that the major objective of a comparative clinical trial is to provide a precise and valid comparison. To achieve this, the trial design should be such that it: 1) prevents bias; 2) ensures an efficient treatment comparison; and 3) is simple to implement to minimize operational errors. Table 1 elaborates on these considerations, focusing on restricted randomization procedures for 1:1 randomized trials.
Before delving into a detailed discussion, let us introduce some important definitions. Following [ 10 ], a randomization sequence is a random vector \({{\varvec{\updelta}}}_{n}=({\delta }_{1},\dots ,{\delta }_{n})\) , where \({\delta }_{i}=1\) , if the i th subject is assigned to treatment E or \({\delta }_{i}=0\) , if the \(i\) th subject is assigned to treatment C. A restricted randomization procedure can be defined by specifying a probabilistic rule for the treatment assignment of the ( i +1)st subject, \({\delta }_{i+1}\) , given the past allocations \({{\varvec{\updelta}}}_{i}\) for \(i\ge 1\) . Let \({N}_{E}\left(i\right)={\sum }_{j=1}^{i}{\delta }_{j}\) and \({N}_{C}\left(i\right)=i-{N}_{E}\left(i\right)\) denote the numbers of subjects assigned to treatments E and C, respectively, after \(i\) allocation steps. Then \(D\left(i\right)={N}_{E}\left(i\right)-{N}_{C}(i)\) is treatment imbalance after \(i\) allocations. For any \(i\ge 1\) , \(D\left(i\right)\) is a random variable whose probability distribution is determined by the chosen randomization procedure.
Balance and randomness
Treatment balance and allocation randomness are two competing requirements in the design of an RCT. Restricted randomization procedures that provide a good tradeoff between these two criteria are desirable in practice.
Consider a trial with sample size \(n\) . The absolute value of imbalance, \(\left|D(i)\right|\) \((i=1,\dots,n)\) , provides a measure of deviation from equal allocation after \(i\) allocation steps. \(\left|D(i)\right|=0\) indicates that the trial is perfectly balanced. One can also consider \(\Pr(\vert D\left(i\right)\vert=0)\) , the probability of achieving exact balance after \(i\) allocation steps. In particular \(\Pr(\vert D\left(n\right)\vert=0)\) is the probability that the final treatment numbers are balanced. Two other useful summary measures are the expected imbalance at the \(i\mathrm{th}\) step, \(E\left|D(i)\right|\) and the expected value of the maximum imbalance of the entire randomization sequence, \(E\left(\underset{1\le i\le n}{\mathrm{max}}\left|D\left(i\right)\right|\right)\) .
Greater forcing of balance implies lack of randomness. A procedure that lacks randomness may be susceptible to selection bias [ 16 ], which is a prominent issue in open-label trials with a single center or with randomization stratified by center, where the investigator knows the sequence of all previous treatment assignments. A classic approach to quantify the degree of susceptibility of a procedure to selection bias is the Blackwell-Hodges model [ 28 ]. Let \({G}_{i}=1\) (or 0), if at the \(i\mathrm{th}\) allocation step an investigator makes a correct (or incorrect) guess on treatment assignment \({\delta }_{i}\) , given past allocations \({{\varvec{\updelta}}}_{i-1}\) . Then the predictability of the design at the \(i\mathrm{th}\) step is the expected value of \({G}_{i}\) , i.e. \(E\left(G_i\right)=\Pr(G_i=1)\) . Blackwell and Hodges [ 28 ] considered the expected bias factor , the difference between expected total number of correct guesses of a given sequence of random assignments and the similar quantity obtained from CRD for which treatment assignments are made independently with equal probability: \(E(F)=E\left({\sum }_{i=1}^{n}{G}_{i}\right)-n/2\) . This quantity is zero for CRD, and it is positive for restricted randomization procedures (greater values indicate higher expected bias). Matts and Lachin [ 30 ] suggested taking expected proportion of deterministic assignments in a sequence as another measure of lack of randomness.
In the literature, various restricted randomization procedures have been compared in terms of balance and randomness [ 50 , 58 , 59 ]. For instance, Zhao et al. [ 58 ] performed a comprehensive simulation study of 14 restricted randomization procedures with different choices of design parameters, for sample sizes in the range of 10 to 300. The key criteria were the maximum absolute imbalance and the correct guess probability. The authors found that the performance of the designs was within a closed region with the boundaries shaped by Efron’s BCD [ 44 ] and the big stick design [ 37 ], signifying that the latter procedure with a suitably chosen MTI boundary can be superior to other restricted randomization procedures in terms of balance/randomness tradeoff. Similar findings confirming the utility of the big stick design were recently reported by Hilgers et al. [ 60 ].
Validity and efficiency
Validity of a statistical procedure essentially means that the procedure provides correct statistical inference following an RCT. In particular, a chosen statistical test is valid, if it controls the chance of a false positive finding, that is, the pre-specified probability of a type I error of the test is achieved but not exceeded. The strong control of type I error rate is a major prerequisite for any confirmatory RCT. Efficiency means high statistical power for detecting meaningful treatment differences (when they exist), and high accuracy of estimation of treatment effects.
Both validity and efficiency are major requirements of any RCT, and both of these aspects are intertwined with treatment balance and allocation randomness. Restricted randomization designs, when properly implemented, provide solid ground for valid and efficient statistical inference. However, a careful consideration of different options can help an investigator to optimize the choice of a randomization procedure for their clinical trial.
Let us start with statistical efficiency. Equal (1:1) allocation frequently maximizes power and estimation precision. To illustrate this, suppose the primary outcomes in the two groups are normally distributed with respective means \({\mu }_{E}\) and \({\mu }_{C}\) and common standard deviation \(\sigma >0\) . Then the variance of an efficient estimator of the treatment difference \({\mu }_{E}-{\mu }_{C}\) is equal to \(V=\frac{4{\sigma }^{2}}{n-{L}_{n}}\) , where \({L}_{n}=\frac{{\left|D(n)\right|}^{2}}{n}\) is referred to as loss [ 61 ]. Clearly, \(V\) is minimized when \({L}_{n}=0\) , or equivalently, \(D\left(n\right)=0\) , i.e. the balanced trial.
When the primary outcome follows a more complex statistical model, optimal allocation may be unequal across the treatment groups; however, 1:1 allocation is still nearly optimal for binary outcomes [ 62 , 63 ], survival outcomes [ 64 ], and possibly more complex data types [ 65 , 66 ]. Therefore, a randomization design that balances treatment numbers frequently promotes efficiency of the treatment comparison.
As regards inferential validity, it is important to distinguish two approaches to statistical inference after the RCT – an invoked population model and a randomization model [ 10 ]. For a given randomization procedure, these two approaches generally produce similar results when the assumption of normal random sampling (and some other assumptions) are satisfied, but the randomization model may be more robust when model assumptions are violated; e.g. when outcomes are affected by a linear time trend [ 67 , 68 ]. Another important issue that may interfere with validity is selection bias. Some authors showed theoretically that PBDs with small block sizes may result in serious inflation of the type I error rate under a selection bias model [ 69 , 70 , 71 ]. To mitigate risk of selection bias, one should ideally take preventative measures, such as blinding/masking, allocation concealment, and avoidance of highly restrictive randomization designs. However, for already completed studies with evidence of selection bias [ 72 ], special statistical adjustments are warranted to ensure validity of the results [ 73 , 74 , 75 ].
Implementation aspects
With the current state of information technology, implementation of randomization in RCTs should be straightforward. Validated randomization systems are emerging, and they can handle randomization designs of increasing complexity for clinical trials that are run globally. However, some important points merit consideration.
The first point has to do with how a randomization sequence is generated and implemented. One should distinguish between advance and adaptive randomization [ 16 ]. Here, by “adaptive” randomization we mean “in-real-time” randomization, i.e. when a randomization sequence is generated not upfront, but rather sequentially, as eligible subjects enroll into the study. Restricted randomization procedures are “allocation-adaptive”, in the sense that the treatment assignment of an individual subject is adapted to the history of previous treatment assignments. While in practice the majority of trials with restricted and stratified randomization use randomization schedules pre-generated in advance, there are some circumstances under which “in-real-time” randomization schemes may be preferred; for instance, clinical trials with high cost of goods and/or shortage of drug supply [ 76 ].
The advance randomization approach includes the following steps: 1) for the chosen randomization design and sample size \(n\) , specify the probability distribution on the reference set by enumerating all feasible randomization sequences of length \(n\) and their corresponding probabilities; 2) select a sequence at random from the reference set according to the probability distribution; and 3) implement this sequence in the trial. While enumeration of all possible sequences and their probabilities is feasible and may be useful for trials with small sample sizes, the task becomes computationally prohibitive (and unnecessary) for moderate or large samples. In practice, Monte Carlo simulation can be used to approximate the probability distribution of the reference set of all randomization sequences for a chosen randomization procedure.
A limitation of advance randomization is that a sequence of treatment assignments must be generated upfront, and proper security measures (e.g. blinding/masking) must be in place to protect confidentiality of the sequence. With the adaptive or “in-real-time” randomization, a sequence of treatment assignments is generated dynamically as the trial progresses. For many restricted randomization procedures, the randomization rule can be expressed as \(\Pr(\delta_{i+1}=1)=\left|F\left\{D\left(i\right)\right\}\right|\) , where \(F\left\{\cdot \right\}\) is some non-increasing function of \(D\left(i\right)\) for any \(i\ge 1\) . This is referred to as the Markov property [ 77 ], which makes a procedure easy to implement sequentially. Some restricted randomization procedures, e.g. the maximal procedure [ 35 ], do not have the Markov property.
The second point has to do with how the final data analysis is performed. With an invoked population model, the analysis is conditional on the design and the randomization is ignored in the analysis. With a randomization model, the randomization itself forms the basis for statistical inference. Reference [ 14 ] provides a contemporaneous overview of randomization-based inference in clinical trials. Several other papers provide important technical details on randomization-based tests, including justification for control of type I error rate with these tests [ 22 , 78 , 79 ]. In practice, Monte Carlo simulation can be used to estimate randomization-based p- values [ 10 ].
A roadmap for comparison of restricted randomization procedures
The design of any RCT starts with formulation of the trial objectives and research questions of interest [ 3 , 31 ]. The choice of a randomization procedure is an integral part of the study design. A structured approach for selecting an appropriate randomization procedure for an RCT was proposed by Hilgers et al. [ 60 ]. Here we outline the thinking process one may follow when evaluating different candidate randomization procedures. Our presented roadmap is by no means exhaustive; its main purpose is to illustrate the logic behind some important considerations for finding an “optimal” randomization design for the given trial parameters.
Throughout, we shall assume that the study is designed as a randomized, two-arm comparative trial with 1:1 allocation, with a fixed sample size \(n\) that is pre-determined based on budgetary and statistical considerations to obtain a definitive assessment of the treatment effect via the pre-defined hypothesis testing. We start with some general considerations which determine the study design:
Sample size ( \(n\) ). For small or moderate studies, exact attainment of the target numbers per group may be essential, because even slight imbalance may decrease study power. Therefore, a randomization design in such studies should equalize well the final treatment numbers. For large trials, the risk of major imbalances is less of a concern, and more random procedures may be acceptable.
The length of the recruitment period and the trial duration. Many studies are short-term and enroll participants fast, whereas some other studies are long-term and may have slow patient accrual. In the latter case, there may be time drifts in patient characteristics, and it is important that the randomization design balances treatment assignments over time.
Level of blinding (masking): double-blind, single-blind, or open-label. In double-blind studies with properly implemented allocation concealment the risk of selection bias is low. By contrast, in open-label studies the risk of selection bias may be high, and the randomization design should provide strong encryption of the randomization sequence to minimize prediction of future allocations.
Number of study centers. Many modern RCTs are implemented globally at multiple research institutions, whereas some studies are conducted at a single institution. In the former case, the randomization is often stratified by center and/or clinically important covariates. In the latter case, especially in single-institution open-label studies, the randomization design should be chosen very carefully, to mitigate the risk of selection bias.
An important point to consider is calibration of the design parameters. Many restricted randomization procedures involve parameters, such as the block size in the PBD, the coin bias probability in Efron’s BCD, the MTI threshold, etc. By fine-tuning these parameters, one can obtain designs with desirable statistical properties. For instance, references [ 80 , 81 ] provide guidance on how to justify the block size in the PBD to mitigate the risk of selection bias or chronological bias. Reference [ 82 ] provides a formal approach to determine the “optimal” value of the parameter \(p\) in Efron’s BCD in both finite and large samples. The calibration of design parameters can be done using Monte Carlo simulations for the given trial setting.
Another important consideration is the scope of randomization procedures to be evaluated. As we mentioned already, even one method may represent a broad class of randomization procedures that can provide different levels of balance/randomness tradeoff; e.g. Efron’s BCD covers a wide spectrum of designs, from PBD(2) (if \(p=1\) ) to CRD (if \(p=0.5\) ). One may either prefer to focus on finding the “optimal” parameter value for the chosen design, or be more general and include various designs (e.g. MTI procedures, BCDs, urn designs, etc.) in the comparison. This should be done judiciously, on a case-by-case basis, focusing only on the most reasonable procedures. References [ 50 , 58 , 60 ] provide good examples of simulation studies to facilitate comparisons among various restricted randomization procedures for a 1:1 RCT.
In parallel with the decision on the scope of randomization procedures to be assessed, one should decide upon the performance criteria against which these designs will be compared. Among others, one might think about the two competing considerations: treatment balance and allocation randomness. For a trial of size \(n\) , at each allocation step \(i=1,\dots ,n\) one can calculate expected absolute imbalance \(E\left|D(i)\right|\) and the probability of correct guess \(\Pr(G_i=1)\) as measures of lack of balance and lack of randomness, respectively. These measures can be either calculated analytically (when formulae are available) or through Monte Carlo simulations. Sometimes it may be useful to look at cumulative measures up to the \(i\mathrm{th}\) allocation step ( \(i=1,\dots ,n\) ); e.g. \(\frac{1}{i}{\sum }_{j=1}^{i}E\left|D(j)\right|\) and \(\frac1i\sum\nolimits_{j=1}^i\Pr(G_j=1)\) . For instance, \(\frac{1}{n}{\sum }_{j=1}^{n}{\mathrm{Pr}}({G}_{j}=1)\) is the average correct guess probability for a design with sample size \(n\) . It is also helpful to visualize the selected criteria. Visualizations can be done in a number of ways; e.g. plots of a criterion vs. allocation step, admissibility plots of two chosen criteria [ 50 , 59 ], etc. Such visualizations can help evaluate design characteristics, both overall and at intermediate allocation steps. They may also provide insights into the behavior of a particular design for different values of the tuning parameter, and/or facilitate a comparison among different types of designs.
Another way to compare the merits of different randomization procedures is to study their inferential characteristics such as type I error rate and power under different experimental conditions. Sometimes this can be done analytically, but a more practical approach is to use Monte Carlo simulation. The choice of the modeling and analysis strategy will be context-specific. Here we outline some considerations that may be useful for this purpose:
Data generating mechanism . To simulate individual outcome data, some plausible statistical model must be posited. The form of the model will depend on the type of outcomes (e.g. continuous, binary, time-to-event, etc.), covariates (if applicable), the distribution of the measurement error terms, and possibly some additional terms representing selection and/or chronological biases [ 60 ].
True treatment effects . At least two scenarios should be considered: under the null hypothesis ( \({H}_{0}\) : treatment effects are the same) to evaluate the type I error rate, and under an alternative hypothesis ( \({H}_{1}\) : there is some true clinically meaningful difference between the treatments) to evaluate statistical power.
Randomization designs to be compared . The choice of candidate randomization designs and their parameters must be made judiciously.
Data analytic strategy . For any study design, one should pre-specify the data analysis strategy to address the primary research question. Statistical tests of significance to compare treatment effects may be parametric or nonparametric, with or without adjustment for covariates.
The approach to statistical inference: population model-based or randomization-based . These two approaches are expected to yield similar results when the population model assumptions are met, but they may be different if some assumptions are violated. Randomization-based tests following restricted randomization procedures will control the type I error at the chosen level if the distribution of the test statistic under the null hypothesis is fully specified by the randomization procedure that was used for patient allocation. This is always the case unless there is a major flaw in the design (such as selection bias whereby the outcome of any individual participant is dependent on treatment assignments of the previous participants).
Overall, there should be a well-thought plan capturing the key questions to be answered, the strategy to address them, the choice of statistical software for simulation and visualization of the results, and other relevant details.
In this section we present four examples that illustrate how one may approach evaluation of different randomization design options at the study planning stage. Example 1 is based on a hypothetical 1:1 RCT with \(n=50\) and a continuous primary outcome, whereas Examples 2, 3, and 4 are based on some real RCTs.
Example 1: Which restricted randomization procedures are robust and efficient?
Our first example is a hypothetical RCT in which the primary outcome is assumed to be normally distributed with mean \({\mu }_{E}\) for treatment E, mean \({\mu }_{C}\) for treatment C, and common variance \({\sigma }^{2}\) . A total of \(n\) subjects are to be randomized equally between E and C, and a two-sample t-test is planned for data analysis. Let \(\Delta ={\mu }_{E}-{\mu }_{C}\) denote the true mean treatment difference. We are interested in testing a hypothesis \({H}_{0}:\Delta =0\) (treatment effects are the same) vs. \({H}_{1}:\Delta \ne 0\) .
The total sample size \(n\) to achieve given power at some clinically meaningful treatment difference \({\Delta }_{c}\) while maintaining the chance of a false positive result at level \(\alpha\) can be obtained using standard statistical methods [ 83 ]. For instance, if \({\Delta }_{c}/\sigma =0.95\) , then a design with \(n=50\) subjects (25 per arm) provides approximately 91% power of a two-sample t-test to detect a statistically significant treatment difference using 2-sided \(\alpha =\) 5%. We shall consider 12 randomization procedures to sequentially randomize \(n=50\) subjects in a 1:1 ratio.
Random allocation rule – Rand.
Truncated binomial design – TBD.
Permuted block design with block size of 2 – PBD(2).
Permuted block design with block size of 4 – PBD(4).
Big stick design [ 37 ] with MTI = 3 – BSD(3).
Biased coin design with imbalance tolerance [ 38 ] with p = 2/3 and MTI = 3 – BCDWIT(2/3, 3).
Efron’s biased coin design [ 44 ] with p = 2/3 – BCD(2/3).
Adjustable biased coin design [ 49 ] with a = 2 – ABCD(2).
Generalized biased coin design (GBCD) with \(\gamma =1\) [ 45 ] – GBCD(1).
GBCD with \(\gamma =2\) [ 46 ] – GBCD(2).
GBCD with \(\gamma =5\) [ 47 ] – GBCD(5).
Complete randomization design – CRD.
These 12 procedures can be grouped into five major types. I) Procedures 1, 2, 3, and 4 achieve exact final balance for a chosen sample size (provided the total sample size is a multiple of the block size). II) Procedures 5 and 6 ensure that at any allocation step the absolute value of imbalance is capped at MTI = 3. III) Procedures 7 and 8 are biased coin designs that sequentially adjust randomization according to imbalance measured as the difference in treatment numbers. IV) Procedures 9, 10, and 11 (GBCD’s with \(\gamma =\) 1, 2, and 5) are adaptive biased coin designs, for which randomization probability is modified according to imbalance measured as the difference in treatment allocation proportions (larger \(\gamma\) implies greater forcing of balance). V) Procedure 12 (CRD) is the most random procedure that achieves balance for large samples.
Balance/randomness tradeoff
We first compare the procedures with respect to treatment balance and allocation randomness. To quantify imbalance after \(i\) allocations, we consider two measures: expected value of absolute imbalance \(E\left|D(i)\right|\) , and expected value of loss \(E({L}_{i})=E{\left|D(i)\right|}^{2}/i\) [ 50 , 61 ]. Importantly, for procedures 1, 2, and 3 the final imbalance is always zero, thus \(E\left|D(n)\right|\equiv 0\) and \(E({L}_{n})\equiv 0\) , but at intermediate steps one may have \(E\left|D(i)\right|>0\) and \(E\left({L}_{i}\right)>0\) , for \(1\le i<n\) . For procedures 5 and 6 with MTI = 3, \(E\left({L}_{i}\right)\le 9/i\) . For procedures 7 and 8, \(E\left({L}_{n}\right)\) tends to zero as \(n\to \infty\) [ 49 ]. For procedures 9, 10, 11, and 12, as \(n\to \infty\) , \(E\left({L}_{n}\right)\) tends to the positive constants 1/3, 1/5, 1/11, and 1, respectively [ 47 ]. We take the cumulative average loss after \(n\) allocations as an aggregate measure of imbalance: \(Imb\left(n\right)=\frac{1}{n}{\sum }_{i=1}^{n}E\left({L}_{i}\right)\) , which takes values in the 0–1 range.
To measure lack of randomness, we consider two measures: expected proportion of correct guesses up to the \(i\mathrm{th}\) step, \(PCG\left(i\right)=\frac1i\sum\nolimits_{j=1}^i\Pr(G_j=1)\) , \(i=1,\dots ,n\) , and the forcing index [ 47 , 84 ], \(FI(i)=\frac{{\sum }_{j=1}^{i}E\left|{\phi }_{j}-0.5\right|}{i/4}\) , where \(E\left|{\phi }_{j}-0.5\right|\) is the expected deviation of the conditional probability of treatment E assignment at the \(j\mathrm{th}\) allocation step ( \({\phi }_{j}\) ) from the unconditional target value of 0.5. Note that \(PCG\left(i\right)\) takes values in the range from 0.5 for CRD to 0.75 for PBD(2) assuming \(i\) is even, whereas \(FI(i)\) takes values in the 0–1 range. At the one extreme, we have CRD for which \(FI(i)\equiv 0\) because for CRD \({\phi }_{i}=0.5\) for any \(i\ge 1\) . At the other extreme, we have PBD(2) for which every odd allocation is made with probability 0.5, and every even allocation is deterministic, i.e. made with probability 0 or 1. For PBD(2), assuming \(i\) is even, there are exactly \(i/2\) pairs of allocations, and so \({\sum }_{j=1}^{i}E\left|{\phi }_{j}-0.5\right|=0.5\cdot i/2=i/4\) , which implies that \(FI(i)=1\) for PBD(2). For all other restricted randomization procedures one has \(0<FI(i)<1\) .
A “good” randomization procedure should have low values of both loss and forcing index. Different randomization procedures can be compared graphically. As a balance/randomness tradeoff metric, one can calculate the quadratic distance to the origin (0,0) for the chosen sample size, e.g. \(d(n)=\sqrt{{\left\{Imb(n)\right\}}^{2}+{\left\{FI(n)\right\}}^{2}}\) (in our example \(n=50\) ), and the randomization designs can then be ranked such that designs with lower values of \(d(n)\) are preferable.
We ran a simulation study of the 12 randomization procedures for an RCT with \(n=50\) . Monte Carlo average values of absolute imbalance, loss, \(Imb\left(i\right)\) , \(FI\left(i\right)\) , and \(d(i)\) were calculated for each intermediate allocation step ( \(i=1,\dots ,50\) ), based on 10,000 simulations.
Figure 1 is a plot of expected absolute imbalance vs. allocation step. CRD, GBCD(1), and GBCD(2) show increasing patterns. For TBD and Rand, the final imbalance (when \(n=50\) ) is zero; however, at intermediate steps is can be quite large. For other designs, absolute imbalance is expected to be below 2 at any allocation step up to \(n=50\) . Note the periodic patterns of PBD(2) and PBD(4); for instance, for PBD(2) imbalance is 0 (or 1) for any even (or odd) allocation.
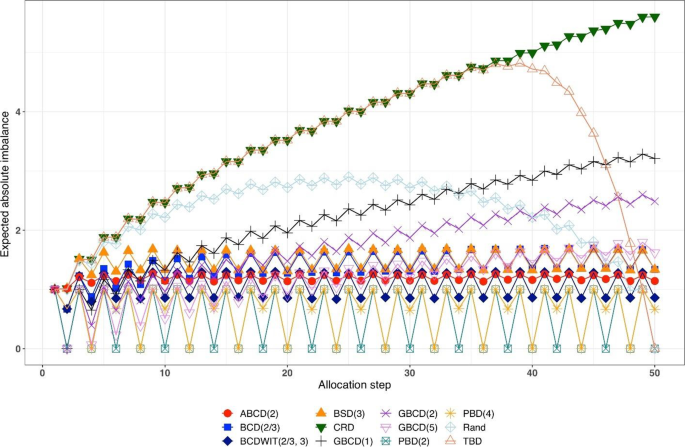
Simulated expected absolute imbalance vs. allocation step for 12 restricted randomization procedures for n = 50. Note: PBD(2) and PBD(4) have forced periodicity absolute imbalance of 0, which distinguishes them from MTI procedures
Figure 2 is a plot of expected proportion of correct guesses vs. allocation step. One can observe that for CRD it is a flat pattern at 0.5; for PBD(2) it fluctuates while reaching the upper limit of 0.75 at even allocation steps; and for ten other designs the values of proportion of correct guesses fall between those of CRD and PBD(2). The TBD has the same behavior up to ~ 40 th allocation step, at which the pattern starts increasing. Rand exhibits an increasing pattern with overall fewer correct guesses compared to other randomization procedures. Interestingly, BSD(3) is uniformly better (less predictable) than ABCD(2), BCD(2/3), and BCDWIT(2/3, 3). For the three GBCD procedures, there is a rapid initial increase followed by gradual decrease in the pattern; this makes good sense, because GBCD procedures force greater balance when the trial is small and become more random (and less prone to correct guessing) as the sample size increases.
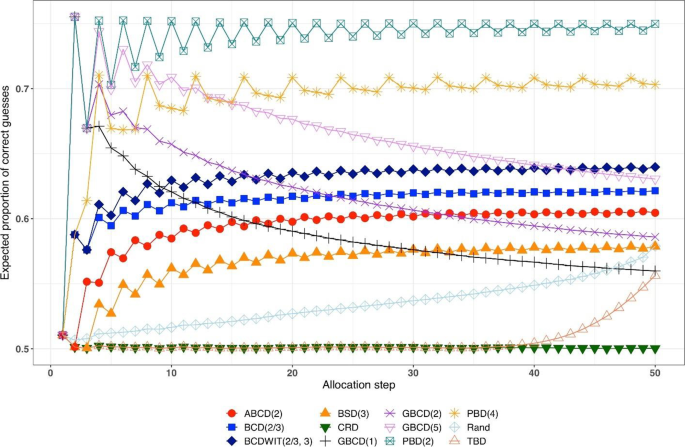
Simulated expected proportion of correct guesses vs. allocation step for 12 restricted randomization procedures for n = 50
Table 2 shows the ranking of the 12 designs with respect to the overall performance metric \(d(n)=\sqrt{{\left\{Imb(n)\right\}}^{2}+{\left\{FI(n)\right\}}^{2}}\) for \(n=50\) . BSD(3), GBCD(2) and GBCD(1) are the top three procedures, whereas PBD(2) and CRD are at the bottom of the list.
Figure 3 is a plot of \(FI\left(n\right)\) vs. \(Imb\left(n\right)\) for \(n=50\) . One can see the two extremes: CRD that takes the value (0,1), and PBD(2) with the value (1,0). The other ten designs are closer to (0,0).
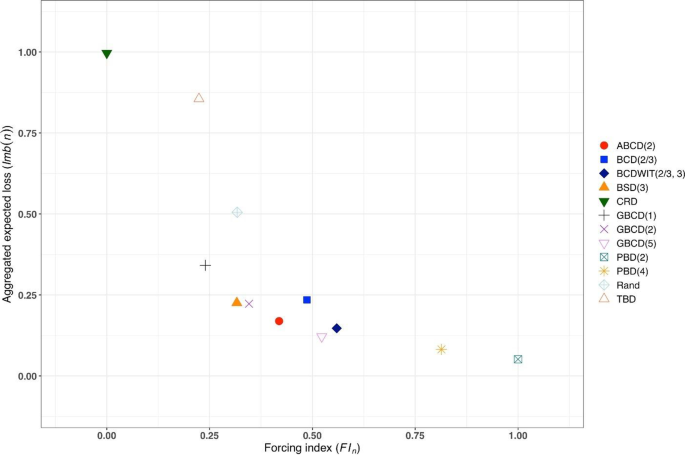
Simulated forcing index (x-axis) vs. aggregate expected loss (y-axis) for 12 restricted randomization procedures for n = 50
Figure 4 is a heat map plot of the metric \(d(i)\) for \(i=1,\dots ,50\) . BSD(3) seems to provide overall best tradeoff between randomness and balance throughout the study.
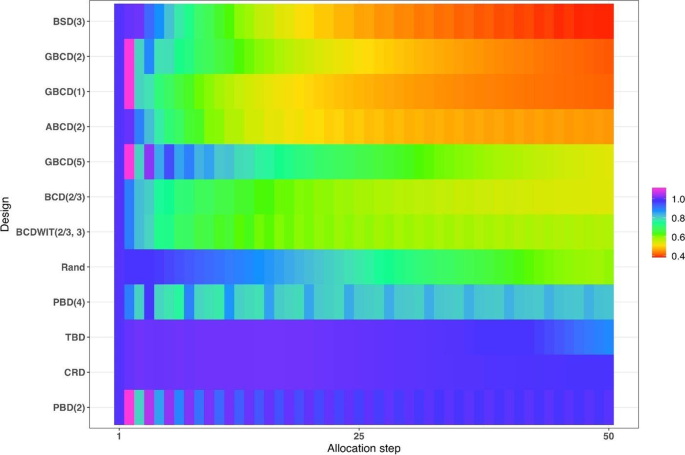
Heatmap of the balance/randomness tradeoff \(d\left(i\right)=\sqrt{{\left\{Imb(i)\right\}}^{2}+{\left\{FI(i)\right\}}^{2}}\) vs. allocation step ( \(i=1,\dots ,50\) ) for 12 restricted randomization procedures. The procedures are ordered by value of d(50), with smaller values (more red) indicating more optimal performance
Inferential characteristics: type I error rate and power
Our next goal is to compare the chosen randomization procedures in terms of validity (control of the type I error rate) and efficiency (power). For this purpose, we assumed the following data generating mechanism: for the \(i\mathrm{th}\) subject, conditional on the treatment assignment \({\delta }_{i}\) , the outcome \({Y}_{i}\) is generated according to the model
where \({u}_{i}\) is an unknown term associated with the \(i\mathrm{th}\) subject and \({\varepsilon }_{i}\) ’s are i.i.d. measurement errors. We shall explore the following four models:
M1: Normal random sampling : \({u}_{i}\equiv 0\) and \({\varepsilon }_{i}\sim\) i.i.d. N(0,1), \(i=1,\dots ,n\) . This corresponds to a standard setup for a two-sample t-test under a population model.
M2: Linear trend : \({u}_{i}=\frac{5i}{n+1}\) and \({\varepsilon }_{i}\sim\) i.i.d. N(0,1), \(i=1,\dots ,n\) . In this model, the outcomes are affected by a linear trend over time [ 67 ].
M3: Cauchy errors : \({u}_{i}\equiv 0\) and \({\varepsilon }_{i}\sim\) i.i.d. Cauchy(0,1), \(i=1,\dots ,n\) . In this setup, we have a misspecification of the distribution of measurement errors.
M4: Selection bias : \({u}_{i+1}=-\nu \cdot sign\left\{D\left(i\right)\right\}\) , \(i=0,\dots ,n-1\) , with the convention that \(D\left(0\right)=0\) . Here, \(\nu >0\) is the “bias effect” (in our simulations we set \(\nu =0.5\) ). We also assume that \({\varepsilon }_{i}\sim\) i.i.d. N(0,1), \(i=1,\dots ,n\) . In this setup, at each allocation step the investigator attempts to intelligently guess the upcoming treatment assignment and selectively enroll a patient who, in their view, would be most suitable for the upcoming treatment. The investigator uses the “convergence” guessing strategy [ 28 ], that is, guess the treatment as one that has been less frequently assigned thus far, or make a random guess in case the current treatment numbers are equal. Assuming that the investigator favors the experimental treatment and is interested in demonstrating its superiority over the control, the biasing mechanism is as follows: at the \((i+1)\) st step, a “healthier” patient is enrolled, if \(D\left(i\right)<0\) ( \({u}_{i+1}=0.5\) ); a “sicker” patient is enrolled, if \(D\left(i\right)>0\) ( \({u}_{i+1}=-0.5\) ); or a “regular” patient is enrolled, if \(D\left(i\right)=0\) ( \({u}_{i+1}=0\) ).
We consider three statistical test procedures:
T1: Two-sample t-test : The test statistic is \(t=\frac{{\overline{Y} }_{E}-{\overline{Y} }_{C}}{\sqrt{{S}_{p}^{2}\left(\frac{1}{{N}_{E}\left(n\right)}+\frac{1}{{N}_{C}\left(n\right)}\right)}}\) , where \({\overline{Y} }_{E}=\frac{1}{{N}_{E}\left(n\right)}{\sum }_{i=1}^{n}{{\delta }_{i}Y}_{i}\) and \({\overline{Y} }_{C}=\frac{1}{{N}_{C}\left(n\right)}{\sum }_{i=1}^{n}{(1-\delta }_{i}){Y}_{i}\) are the treatment sample means, \({N}_{E}\left(n\right)={\sum }_{i=1}^{n}{\delta }_{i}\) and \({N}_{C}\left(n\right)=n-{N}_{E}\left(n\right)\) are the observed group sample sizes, and \({S}_{p}^{2}\) is a pooled estimate of variance, where \({S}_{p}^{2}=\frac{1}{n-2}\left({\sum }_{i=1}^{n}{\delta }_{i}{\left({Y}_{i}-{\overline{Y} }_{E}\right)}^{2}+{\sum }_{i=1}^{n}(1-{\delta }_{i}){\left({Y}_{i}-{\overline{Y} }_{C}\right)}^{2}\right)\) . Then \({H}_{0}:\Delta =0\) is rejected at level \(\alpha\) , if \(\left|t\right|>{t}_{1-\frac{\alpha }{2}, n-2}\) , the 100( \(1-\frac{\alpha }{2}\) )th percentile of the t-distribution with \(n-2\) degrees of freedom.
T2: Randomization-based test using mean difference : Let \({{\varvec{\updelta}}}_{obs}\) and \({{\varvec{y}}}_{obs}\) denote, respectively the observed sequence of treatment assignments and responses, obtained from the trial using randomization procedure \(\mathfrak{R}\) . We first compute the observed mean difference \({S}_{obs}=S\left({{\varvec{\updelta}}}_{obs},{{\varvec{y}}}_{obs}\right)={\overline{Y} }_{E}-{\overline{Y} }_{C}\) . Then we use Monte Carlo simulation to generate \(L\) randomization sequences of length \(n\) using procedure \(\mathfrak{R}\) , where \(L\) is some large number. For the \(\ell\mathrm{th}\) generated sequence, \({{\varvec{\updelta}}}_{\ell}\) , compute \({S}_{\ell}=S({{\varvec{\updelta}}}_{\ell},{{\varvec{y}}}_{obs})\) , where \({\ell}=1,\dots ,L\) . The proportion of sequences for which \({S}_{\ell}\) is at least as extreme as \({S}_{obs}\) is computed as \(\widehat{P}=\frac{1}{L}{\sum }_{{\ell}=1}^{L}1\left\{\left|{S}_{\ell}\right|\ge \left|{S}_{obs}\right|\right\}\) . Statistical significance is declared, if \(\widehat{P}<\alpha\) .
T3: Randomization-based test based on ranks : This test procedure follows the same logic as T2, except that the test statistic is calculated based on ranks. Given the vector of observed responses \({{\varvec{y}}}_{obs}=({y}_{1},\dots ,{y}_{n})\) , let \({a}_{jn}\) denote the rank of \({y}_{j}\) among the elements of \({{\varvec{y}}}_{obs}\) . Let \({\overline a}_n\) denote the average of \({a}_{jn}\) ’s, and let \({\boldsymbol a}_n=\left(a_{1n}-{\overline a}_n,...,\alpha_{nn}-{\overline a}_n\right)\boldsymbol'\) . Then a linear rank test statistic has the form \({S}_{obs}={{\varvec{\updelta}}}_{obs}^{\boldsymbol{^{\prime}}}{{\varvec{a}}}_{n}={\sum }_{i=1}^{n}{\delta }_{i}({a}_{in}-{\overline{a} }_{n})\) .
We consider four scenarios of the true mean difference \(\Delta ={\mu }_{E}-{\mu }_{C}\) , which correspond to the Null case ( \(\Delta =0\) ), and three choices of \(\Delta >0\) which correspond to Alternative 1 (power ~ 70%), Alternative 2 (power ~ 80%), and Alternative 3 (power ~ 90%). In all cases, \(n=50\) was used.
Figure 5 summarizes the results of a simulation study comparing 12 randomization designs, under 4 models for the outcome (M1, M2, M3, and M4), 4 scenarios for the mean treatment difference (Null, and Alternatives 1, 2, and 3), using 3 statistical tests (T1, T2, and T3). The operating characteristics of interest are the type I error rate under the Null scenario and the power under the Alternative scenarios. Each scenario was simulated 10,000 times, and each randomization-based test was computed using \(L=\mathrm{10,000}\) sequences.
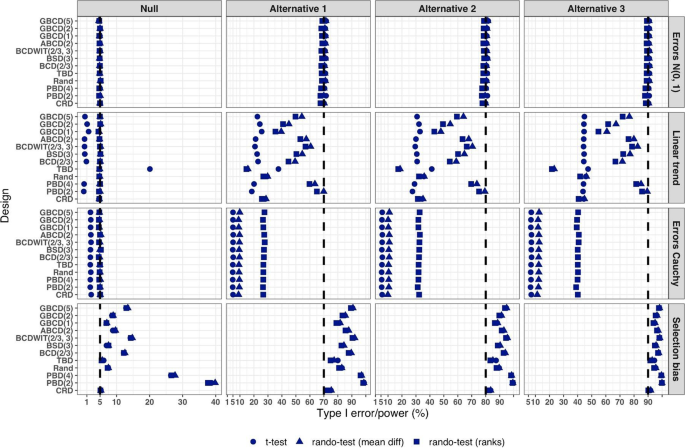
Simulated type I error rate and power of 12 restricted randomization procedures. Four models for the data generating mechanism of the primary outcome (M1: Normal random sampling; M2: Linear trend; M3: Errors Cauchy; and M4: Selection bias). Four scenarios for the treatment mean difference (Null; Alternatives 1, 2, and 3). Three statistical tests (T1: two-sample t-test; T2: randomization-based test using mean difference; T3: randomization-based test using ranks)
From Fig. 5 , under the normal random sampling model (M1), all considered randomization designs have similar performance: they maintain the type I error rate and have similar power, with all tests. In other words, when population model assumptions are satisfied, any combination of design and analysis should work well and yield reliable and consistent results.
Under the “linear trend” model (M2), the designs have differential performance. First of all, under the Null scenario, only Rand and CRD maintain the type I error rate at 5% with all three tests. For TBD, the t-test is anticonservative, with type I error rate ~ 20%, whereas for nine other procedures the t-test is conservative, with type I error rate in the range 0.1–2%. At the same time, for all 12 designs the two randomization-based tests maintain the nominal type I error rate at 5%. These results are consistent with some previous findings in the literature [ 67 , 68 ]. As regards power, it is reduced significantly compared to the normal random sampling scenario. The t-test seems to be most affected and the randomization-based test using ranks is most robust for a majority of the designs. Remarkably, for CRD the power is similar with all three tests. This signifies the usefulness of randomization-based inference in situations when outcome data are subject to a linear time trend, and the importance of applying randomization-based tests at least as supplemental analyses to likelihood-based test procedures.
Under the “Cauchy errors” model (M3), all designs perform similarly: the randomization-based tests maintain the type I error rate at 5%, whereas the t-test deflates the type I error to 2%. As regards power, all designs also have similar, consistently degraded performance: the t-test is least powerful, and the randomization-based test using ranks has highest power. Overall, under misspecification of the error distribution a randomization-based test using ranks is most appropriate; yet one should acknowledge that its power is still lower than expected.
Under the “selection bias” model (M4), the 12 designs have differential performance. The only procedure that maintained the type I error rate at 5% with all three tests was CRD. For eleven other procedures, inflations of the type I error were observed. In general, the more random the design, the less it was affected by selection bias. For instance, the type I error rate for TBD was ~ 6%; for Rand, BSD(3), and GBCD(1) it was ~ 7.5%; for GBCD(2) and ABCD(2) it was ~ 8–9%; for Efron’s BCD(2/3) it was ~ 12.5%; and the most affected design was PBD(2) for which the type I error rate was ~ 38–40%. These results are consistent with the theory of Blackwell and Hodges [ 28 ] which posits that TBD is least susceptible to selection bias within a class of restricted randomization designs that force exact balance. Finally, under M4, statistical power is inflated by several percentage points compared to the normal random sampling scenario without selection bias.
We performed additional simulations to assess the impact of the bias effect \(\nu\) under selection bias model. The same 12 randomization designs and three statistical tests were evaluated for a trial with \(n=50\) under the Null scenario ( \(\Delta =0\) ), for \(\nu\) in the range of 0 (no bias) to 1 (strong bias). Figure S1 in the Supplementary Materials shows that for all designs but CRD, the type I error rate is increasing in \(\nu\) , with all three tests. The magnitude of the type I error inflation is different across the restricted randomization designs; e.g. for TBD it is minimal, whereas for more restrictive designs it may be large, especially for \(\nu \ge 0.4\) . PBD(2) is particularly vulnerable: for \(\nu\) in the range 0.4–1, its type I error rate is in the range 27–90% (for the nominal \(\alpha =5\) %).
In summary, our Example 1 includes most of the key ingredients of the roadmap for assessment of competing randomization designs which was described in the “ Methods ” section. For the chosen experimental scenarios, we evaluated CRD and several restricted randomization procedures, some of which belonged to the same class but with different values of the parameter (e.g. GBCD with \(\gamma =1, 2, 5\) ). We assessed two measures of imbalance, two measures of lack of randomness (predictability), and a metric that quantifies balance/randomness tradeoff. Based on these criteria, we found that BSD(3) provides overall best performance. We also evaluated type I error and power of selected randomization procedures under several treatment response models. We have observed important links between balance, randomness, type I error rate and power. It is beneficial to consider all these criteria simultaneously as they may complement each other in characterizing statistical properties of randomization designs. In particular, we found that a design that lacks randomness, such as PBD with blocks of 2 or 4, may be vulnerable to selection bias and lead to inflations of the type I error. Therefore, these designs should be avoided, especially in open-label studies. As regards statistical power, since all designs in this example targeted 1:1 allocation ratio (which is optimal if the outcomes are normally distributed and have between-group constant variance), they had very similar power of statistical tests in most scenarios except for the one with chronological bias. In the latter case, randomization-based tests were more robust and more powerful than the standard two-sample t-test under the population model assumption.
Overall, while Example 1 is based on a hypothetical 1:1 RCT, its true purpose is to showcase the thinking process in the application of our general roadmap. The following three examples are considered in the context of real RCTs.
Example 2: How can we reduce predictability of a randomization procedure and lower the risk of selection bias?
Selection bias can arise if the investigator can intelligently guess at least part of the randomization sequence yet to be allocated and, on that basis, preferentially and strategically assigns study subjects to treatments. Although it is generally not possible to prove that a particular study has been infected with selection bias, there are examples of published RCTs that do show some evidence to have been affected by it. Suspect trials are, for example, those with strong observed baseline covariate imbalances that consistently favor the active treatment group [ 16 ]. In what follows we describe an example of an RCT where the stratified block randomization procedure used was vulnerable to potential selection biases, and discuss potential alternatives that may reduce this vulnerability.
Etanercept was studied in patients aged 4 to 17 years with polyarticular juvenile rheumatoid arthritis [ 85 ]. The trial consisted of two parts. During the first, open-label part of the trial, patients received etanercept twice weekly for up to three months. Responders from this initial part of the trial were then randomized, at a 1:1 ratio, in the second, double-blind, placebo-controlled part of the trial to receive etanercept or placebo for four months or until a flare of the disease occurred. The primary efficacy outcome, the proportion of patients with disease flare, was evaluated in the double-blind part. Among the 51 randomized patients, 21 of the 26 placebo patients (81%) withdrew because of disease flare, compared with 7 of the 25 etanercept patients (28%), yielding a p- value of 0.003.
Regulatory review by the Food and Drug Administrative (FDA) identified vulnerability to selection biases in the study design of the double-blind part and potential issues in study conduct. These findings were succinctly summarized in [ 16 ] (pp.51–52).
Specifically, randomization was stratified by study center and number of active joints (≤ 2 vs. > 2, referred to as “few” or “many” in what follows), with blocked randomization within each stratum using a block size of two. Furthermore, randomization codes in corresponding “few” and “many” blocks within each study center were mirror images of each other. For example, if the first block within the “few” active joints stratum of a given center is “placebo followed by etanercept”, then the first block within the “many” stratum of the same center would be “etanercept followed by placebo”. While this appears to be an attempt to improve treatment balance in this small trial, unblinding of one treatment assignment may lead to deterministic predictability of three upcoming assignments. While the double-blind nature of the trial alleviated this concern to some extent, it should be noted that all patients did receive etanercept previously in the initial open-label part of the trial. Chances of unblinding may not be ignorable if etanercept and placebo have immediately evident different effects or side effects. The randomized withdrawal design was appropriate in this context to improve statistical power in identifying efficacious treatments, but the specific randomization procedure used in the trial increased vulnerability to selection biases if blinding cannot be completely maintained.
FDA review also identified that four patients were randomized from the wrong “few” or “many” strata, in three of which (3/51 = 5.9%) it was foreseeable that the treatment received could have been reversed compared to what the patient would have received if randomized in the correct stratum. There were also some patients randomized out of order. Imbalance in baseline characteristics were observed in age (mean ages of 8.9 years in the etanercept arm vs. that of 12.2 years in the placebo arm) and corticosteroid use at baseline (50% vs. 24%).
While the authors [ 85 ] concluded that “The unequal randomization did not affect the study results”, and indeed it was unknown whether the imbalance was a chance occurrence or in part caused by selection biases, the trial could have used better alternative randomization procedures to reduce vulnerability to potential selection bias. To illustrate the latter point, let us compare predictability of two randomization procedures – permuted block design (PBD) and big stick design (BSD) for several values of the maximum tolerated imbalance (MTI). We use BSD here for the illustration purpose because it was found to provide a very good balance/randomness tradeoff based on our simulations in Example 1 . In essence, BSD provides the same level of imbalance control as PBD but with stronger encryption.
Table 3 reports two metrics for PBD and BSD: proportion of deterministic assignments within a randomization sequence, and excess correct guess probability. The latter metric is the absolute increase in proportion of correct guesses for a given procedure over CRD that has 50% probability of correct guesses under the “optimal guessing strategy”. Footnote 1 Note that for MTI = 1, BSD is equivalent to PBD with blocks of two. However, by increasing MTI, one can substantially decrease predictability. For instance, going from MTI = 1 in the BSD to an MTI of 2 or 3 (two bottom rows), the proportion of deterministic assignments decreases from 50% to 25% and 16.7%, respectively, and excess correct guess probability decreases from 25% to 12.5% and 8.3%, which is a substantial reduction in risk of selection bias. In addition to simplicity and lower predictability for the same level of MTI control, BSD has another important advantage: investigators are not accustomed to it (as they are to the PBD), and therefore it has potential for complete elimination of prediction through thwarting enough early prediction attempts.
Our observations here are also generalizable to other MTI randomization methods, such as the maximal procedure [ 35 ], Chen’s designs [ 38 , 39 ], block urn design [ 40 ], just to name a few. MTI randomization procedures can be also used as building elements for more complex stratified randomization schemes [ 86 ].
Example 3: How can we mitigate risk of chronological bias?
Chronological bias may occur if a trial recruitment period is long, and there is a drift in some covariate over time that is subsequently not accounted for in the analysis [ 29 ]. To mitigate risk of chronological bias, treatment assignments should be balanced over time. In this regard, the ICH E9 guideline has the following statement [ 31 ]:
“...Although unrestricted randomisation is an acceptable approach, some advantages can generally be gained by randomising subjects in blocks. This helps to increase the comparability of the treatment groups, particularly when subject characteristics may change over time, as a result, for example, of changes in recruitment policy. It also provides a better guarantee that the treatment groups will be of nearly equal size...”
While randomization in blocks of two ensures best balance, it is highly predictable. In practice, a sensible tradeoff between balance and randomness is desirable. In the following example, we illustrate the issue of chronological bias in the context of a real RCT.
Altman and Royston [ 87 ] gave several examples of clinical studies with hidden time trends. For instance, an RCT to compare azathioprine versus placebo in patients with primary biliary cirrhosis (PBC) with respect to overall survival was an international, double-blind, randomized trial including 248 patients of whom 127 received azathioprine and 121 placebo [ 88 ]. The study had a recruitment period of 7 years. A major prognostic factor for survival was the serum bilirubin level on entry to the trial. Altman and Royston [ 87 ] provided a cusum plot of log bilirubin which showed a strong decreasing trend over time – patients who entered the trial later had, on average, lower bilirubin levels, and therefore better prognosis. Despite that the trial was randomized, there was some evidence of baseline imbalance with respect to serum bilirubin between azathioprine and placebo groups. The analysis using Cox regression adjusted for serum bilirubin showed that the treatment effect of azathioprine was statistically significant ( p = 0.01), with azathioprine reducing the risk of dying to 59% of that observed during the placebo treatment.
The azathioprine trial [ 88 ] provides a very good example for illustrating importance of both the choice of a randomization design and a subsequent statistical analysis. We evaluated several randomization designs and analysis strategies under the given time trend through simulation. Since we did not have access to the patient level data from the azathioprine trial, we simulated a dataset of serum bilirubin values from 248 patients that resembled that in the original paper (Fig. 1 in [ 87 ]); see Fig. 6 below.
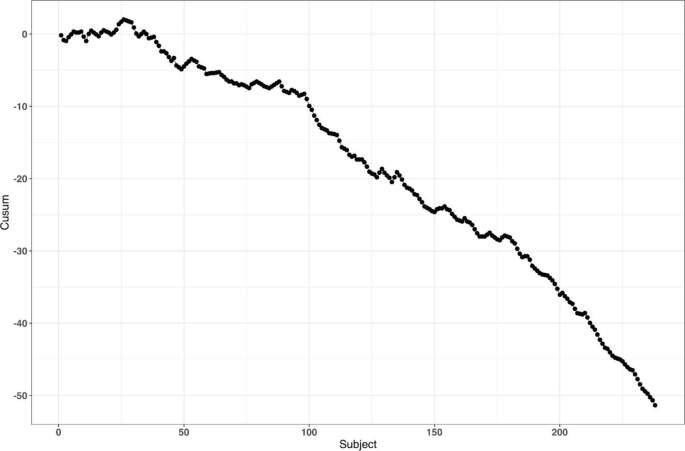
reproduced from Fig. 1 of Altman and Royston [ 87 ]
Cusum plot of baseline log serum bilirubin level of 248 subjects from the azathioprine trial,
For the survival outcomes, we use the following data generating mechanism [ 71 , 89 ]: let \({h}_{i}(t,{\delta }_{i})\) denote the hazard function of the \(i\mathrm{th}\) patient at time \(t\) such that
where \({h}_{c}(t)\) is an unspecified baseline hazard, \(\log HR\) is the true value of the log-transformed hazard ratio, and \({u}_{i}\) is the log serum bilirubin of the \(i\mathrm{th}\) patient at study entry.
Our main goal is to evaluate the impact of the time trend in bilirubin on the type I error rate and power. We consider seven randomization designs: CRD, Rand, TBD, PBD(2), PBD(4), BSD(3), and GBCD(2). The latter two designs were found to be the top two performing procedures based on our simulation results in Example 1 (cf. Table 2 ). PBD(4) is the most commonly used procedure in clinical trial practice. Rand and TBD are two designs that ensure exact balance in the final treatment numbers. CRD is the most random design, and PBD(2) is the most balanced design.
To evaluate both type I error and power, we consider two values for the true treatment effect: \(HR=1\) (Null) and \(HR=0.6\) (Alternative). For data analysis, we use the Cox regression model, either with or without adjustment for serum bilirubin. Furthermore, we assess two approaches to statistical inference: population model-based and randomization-based. For the sake of simplicity, we let \({h}_{c}\left(t\right)\equiv 1\) (exponential distribution) and assume no censoring when simulating the data.
For each combination of the design, experimental scenario, and data analysis strategy, a trial with 248 patients was simulated 10,000 times. Each randomization-based test was computed using \(L=\mathrm{1,000}\) sequences. In each simulation, we used the same time trend in serum bilirubin as described. Through simulation, we estimated the probability of a statistically significant baseline imbalance in serum bilirubin between azathioprine and placebo groups, type I error rate, and power.
First, we observed that the designs differ with respect to their potential to achieve baseline covariate balance under the time trend. For instance, probability of a statistically significant group difference on serum bilirubin (two-sided P < 0.05) is ~ 24% for TBD, ~ 10% for CRD, ~ 2% for GBCD(2), ~ 0.9% for Rand, and ~ 0% for BSD(3), PBD(4), and PBD(2).
Second, a failure to adjust for serum bilirubin in the analysis can negatively impact statistical inference. Table 4 shows the type I error and power of statistical analyses unadjusted and adjusted for serum bilirubin, using population model-based and randomization-based approaches.
If we look at the type I error for the population model-based, unadjusted analysis, we can see that only CRD and Rand are valid (maintain the type I error rate at 5%), whereas TBD is anticonservative (~ 15% type I error) and PBD(2), PBD(4), BSD(3), and GBCD(2) are conservative (~ 1–2% type I error). These findings are consistent with the ones for the two-sample t-test described earlier in the current paper, and they agree well with other findings in the literature [ 67 ]. By contrast, population model-based covariate-adjusted analysis is valid for all seven randomization designs. Looking at the type I error for the randomization-based analyses, all designs yield consistent valid results (~ 5% type I error), with or without adjustment for serum bilirubin.
As regards statistical power, unadjusted analyses are substantially less powerful then the corresponding covariate-adjusted analysis, for all designs with either population model-based or randomization-based approaches. For the population model-based, unadjusted analysis, the designs have ~ 59–65% power, whereas than the corresponding covariate-adjusted analyses have ~ 97% power. The most striking results are observed with the randomization-based approach: the power of unadjusted analysis is quite different across seven designs: it is ~ 37% for TBD, ~ 60–61% for CRD and Rand, ~ 80–87% for BCD(3), GBCD(2), and PBD(4), and it is ~ 90% for PBD(2). Thus, PBD(2) is the most powerful approach if a time trend is present, statistical analysis strategy is randomization-based, and no adjustment for time trend is made. Furthermore, randomization-based covariate-adjusted analyses have ~ 97% power for all seven designs. Remarkably, the power of covariate-adjusted analysis is identical for population model-based and randomization-based approaches.
Overall, this example highlights the importance of covariate-adjusted analysis, which should be straightforward if a covariate affected by a time trend is known (e.g. serum bilirubin in our example). If a covariate is unknown or hidden, then unadjusted analysis following a conventional test may have reduced power and distorted type I error (although the designs such as CRD and Rand do ensure valid statistical inference). Alternatively, randomization-based tests can be applied. The resulting analysis will be valid but may be potentially less powerful. The degree of loss in power following randomization-based test depends on the randomization design: designs that force greater treatment balance over time will be more powerful. In fact, PBD(2) is shown to be most powerful under such circumstances; however, as we have seen in Example 1 and Example 2, a major deficiency of PBD(2) is its vulnerability to selection bias. From Table 4 , and taking into account the earlier findings in this paper, BSD(3) seems to provide a very good risk mitigation strategy against unknown time trends.
Example 4: How do we design an RCT with a very small sample size?
In our last example, we illustrate the importance of the careful choice of randomization design and subsequent statistical analysis in a nonstandard RCT with small sample size. Due to confidentiality and because this study is still in conduct, we do not disclose all details here except for that the study is an ongoing phase II RCT in a very rare and devastating autoimmune disease in children.
The study includes three periods: an open-label single-arm active treatment for 28 weeks to identify treatment responders (Period 1), a 24-week randomized treatment withdrawal period to primarily assess the efficacy of the active treatment vs. placebo (Period 2), and a 3-year long-term safety, open-label active treatment (Period 3). Because of a challenging indication and the rarity of the disease, the study plans to enroll up to 10 male or female pediatric patients in order to randomize 8 patients (4 per treatment arm) in Period 2 of the study. The primary endpoint for assessing the efficacy of active treatment versus placebo is the proportion of patients with disease flare during the 24-week randomized withdrawal phase. The two groups will be compared using Fisher’s exact test. In case of a successful outcome, evidence of clinical efficacy from this study will be also used as part of a package to support the claim for drug effectiveness.
Very small sample sizes are not uncommon in clinical trials of rare diseases [ 90 , 91 ]. Naturally, there are several methodological challenges for this type of study. A major challenge is generalizability of the results from the RCT to a population. In this particular indication, no approved treatment exists, and there is uncertainty on disease epidemiology and the exact number of patients with the disease who would benefit from treatment (patient horizon). Another challenge is the choice of the randomization procedure and the primary statistical analysis. In this study, one can enumerate upfront all 25 possible outcomes: {0, 1, 2, 3, 4} responders on active treatment, and {0, 1, 2, 3, 4} responders on placebo, and create a chart quantifying the level of evidence ( p- value) for each experimental outcome, and the corresponding decision. Before the trial starts, a discussion with the regulatory agency is warranted to agree upon on what level of evidence must be achieved in order to declare the study a “success”.
Let us perform a hypothetical planning for the given study. Suppose we go with a standard population-based approach, for which we test the hypothesis \({H}_{0}:{p}_{E}={p}_{C}\) vs. \({H}_{0}:{p}_{E}>{p}_{C}\) (where \({p}_{E}\) and \({p}_{C}\) stand for the true success rates for the experimental and control group, respectively) using Fisher’s exact test. Table 5 provides 1-sided p- values of all possible experimental outcomes. One could argue that a p- value < 0.1 may be viewed as a convincing level of evidence for this study. There are only 3 possibilities that can lead to this outcome: 3/4 vs. 0/4 successes ( p = 0.0714); 4/4 vs. 0/4 successes ( p = 0.0143); and 4/4 vs. 1/4 successes ( p = 0.0714). For all other outcomes, p ≥ 0.2143, and thus the study would be regarded as a “failure”.
Now let us consider a randomization-based inference approach. For illustration purposes, we consider four restricted randomization procedures—Rand, TBD, PBD(4), and PBD(2)—that exactly achieve 4:4 allocation. These procedures are legitimate choices because all of them provide exact sample sizes (4 per treatment group), which is essential in this trial. The reference set of either Rand or TBD includes \(70=\left(\begin{array}{c}8\\ 4\end{array}\right)\) unique sequences though with different probabilities of observing each sequence. For Rand, these sequences are equiprobable, whereas for TBD, some sequences are more likely than others. For PBD( \(2b\) ), the size of the reference set is \({\left\{\left(\begin{array}{c}2b\\ b\end{array}\right)\right\}}^{B}\) , where \(B=n/2b\) is the number of blocks of length \(2b\) for a trial of size \(n\) (in our example \(n=8\) ). This results in in a reference set of \({2}^{4}=16\) unique sequences with equal probability of 1/16 for PBD(2), and of \({6}^{2}=36\) unique sequences with equal probability of 1/36 for PBD(4).
In practice, the study statistician picks a treatment sequence at random from the reference set according to the chosen design. The details (randomization seed, chosen sequence, etc.) are carefully documented and kept confidential. For the chosen sequence and the observed outcome data, a randomization-based p- value is the sum of probabilities of all sequences in the reference set that yield the result at least as large in favor of the experimental treatment as the one observed. This p- value will depend on the randomization design, the observed randomization sequence and the observed outcomes, and it may also be different from the population-based analysis p- value.
To illustrate this, suppose the chosen randomization sequence is CEECECCE (C stands for control and E stands for experimental), and the observed responses are FSSFFFFS (F stands for failure and S stands for success). Thus, we have 3/4 successes on experimental and 0/4 successes on control. Then, the randomization-based p- value is 0.0714 for Rand; 0.0469 for TBD, 0.1250 for PBD(2); 0.0833 for PBD(4); and it is 0.0714 for the population-based analysis. The coincidence of the randomization-based p- value for Rand and the p- value of the population-based analysis is not surprising. Fisher's exact test is a permutation test and in the case of Rand as randomization procedure, the p- value of a permutation test and of a randomization test are always equal. However, despite the numerical equality, we should be mindful of different assumptions (population/randomization model).
Likewise, randomization-based p- values can be derived for other combinations of observed randomization sequences and responses. All these details (the chosen randomization design, the analysis strategy, and corresponding decisions) would have to be fully specified upfront (before the trial starts) and agreed upon by both the sponsor and the regulator. This would remove any ambiguity when the trial data become available.
As the example shows, the level of evidence in the randomization-based inference approach depends on the chosen randomization procedure and the resulting decisions may be different depending on the specific procedure. For instance, if the level of significance is set to 10% as a criterion for a “successful trial”, then with the observed data (3/4 vs. 0/4), there would be a significant test result for TBD, Rand, PBD(4), but not for PBD(2).
Summary and discussion
Randomization is the foundation of any RCT involving treatment comparison. Randomization is not a single technique, but a very broad class of statistical methodologies for design and analysis of clinical trials [ 10 ]. In this paper, we focused on the randomized controlled two-arm trial designed with equal allocation, which is the gold standard research design to generate clinical evidence in support of regulatory submissions. Even in this relatively simple case, there are various restricted randomization procedures with different probabilistic structures and different statistical properties, and the choice of a randomization design for any RCT must be made judiciously.
For the 1:1 RCT, there is a dual goal of balancing treatment assignments while maintaining allocation randomness. Final balance in treatment totals frequently maximizes statistical power for treatment comparison. It is also important to maintain balance at intermediate steps during the trial, especially in long-term studies, to mitigate potential for chronological bias. At the same time, a procedure should have high degree of randomness so that treatment assignments within the sequence are not easily predictable; otherwise, the procedure may be vulnerable to selection bias, especially in open-label studies. While balance and randomness are competing criteria, it is possible to find restricted randomization procedures that provide a sensible tradeoff between these criteria, e.g. the MTI procedures, of which the big stick design (BSD) [ 37 ] with a suitably chosen MTI limit, such as BSD(3), has very appealing statistical properties. In practice, the choice of a randomization procedure should be made after a systematic evaluation of different candidate procedures under different experimental scenarios for the primary outcome, including cases when model assumptions are violated.
In our considered examples we showed that the choice of randomization design, data analytic technique (e.g. parametric or nonparametric model, with or without covariate adjustment), and the decision on whether to include randomization in the analysis (e.g. randomization-based or population model-based analysis) are all very important considerations. Furthermore, these examples highlight the importance of using randomization designs that provide strong encryption of the randomization sequence, importance of covariate adjustment in the analysis, and the value of statistical thinking in nonstandard RCTs with very small sample sizes and small patient horizon. Finally, in this paper we have discussed randomization-based tests as robust and valid alternatives to likelihood-based tests. Randomization-based inference is a useful approach in clinical trials and should be considered by clinical researchers more frequently [ 14 ].
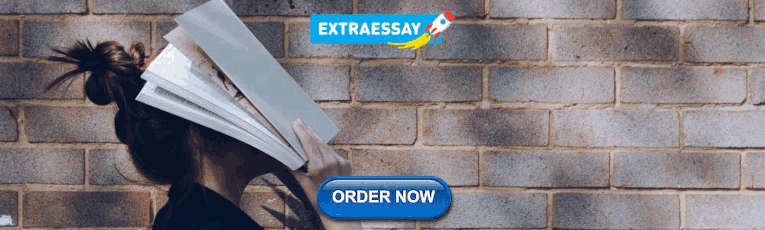
Further topics on randomization
Given the breadth of the subject of randomization, many important topics have been omitted from the current paper. Here we outline just a few of them.
In this paper, we have focused on the 1:1 RCT. However, clinical trials may involve more than two treatment arms. Extensions of equal randomization to the case of multiple treatment arms is relatively straightforward for many restricted randomization procedures [ 10 ]. Some trials with two or more treatment arms use unequal allocation (e.g. 2:1). Randomization procedures with unequal allocation ratios require careful consideration. For instance, an important and desirable feature is the allocation ratio preserving property (ARP). A randomization procedure targeting unequal allocation is said to be ARP, if at each allocation step the unconditional probability of a particular treatment assignment is the same as the target allocation proportion for this treatment [ 92 ]. Non-ARP procedures may have fluctuations in the unconditional randomization probability from allocation to allocation, which may be problematic [ 93 ]. Fortunately, some randomization procedures naturally possess the ARP property, and there are approaches to correct for a non-ARP deficiency – these should be considered in the design of RCTs with unequal allocation ratios [ 92 , 93 , 94 ].
In many RCTs, investigators may wish to prospectively balance treatment assignments with respect to important prognostic covariates. For a small number of categorical covariates one can use stratified randomization by applying separate MTI randomization procedures within strata [ 86 ]. However, a potential advantage of stratified randomization decreases as the number of stratification variables increases [ 95 ]. In trials where balance over a large number of covariates is sought and the sample size is small or moderate, one can consider covariate-adaptive randomization procedures that achieve balance within covariate margins, such as the minimization procedure [ 96 , 97 ], optimal model-based procedures [ 46 ], or some other covariate-adaptive randomization technique [ 98 ]. To achieve valid and powerful results, covariate-adaptive randomization design must be followed by covariate-adjusted analysis [ 99 ]. Special considerations are required for covariate-adaptive randomization designs with more than two treatment arms and/or unequal allocation ratios [ 100 ].
In some clinical research settings, such as trials for rare and/or life threatening diseases, there is a strong ethical imperative to increase the chance of a trial participant to receive an empirically better treatment. Response-adaptive randomization (RAR) has been increasingly considered in practice, especially in oncology [ 101 , 102 ]. Very extensive methodological research on RAR has been done [ 103 , 104 ]. RAR is increasingly viewed as an important ingredient of complex clinical trials such as umbrella and platform trial designs [ 105 , 106 ]. While RAR, when properly applied, has its merit, the topic has generated a lot of controversial discussions over the years [ 107 , 108 , 109 , 110 , 111 ]. Amid the ongoing COVID-19 pandemic, RCTs evaluating various experimental treatments for critically ill COVID-19 patients do incorporate RAR in their design; see, for example, the I-SPY COVID-19 trial ( https://clinicaltrials.gov/ct2/show/NCT04488081 ).
Randomization can also be applied more broadly than in conventional RCT settings where randomization units are individual subjects. For instance, in a cluster randomized trial, not individuals but groups of individuals (clusters) are randomized among one or more interventions or the control [ 112 ]. Observations from individuals within a given cluster cannot be regarded as independent, and special statistical techniques are required to design and analyze cluster-randomized experiments. In some clinical trial designs, randomization is applied within subjects. For instance, the micro-randomized trial (MRT) is a novel design for development of mobile treatment interventions in which randomization is applied to select different treatment options for individual participants over time to optimally support individuals’ health behaviors [ 113 ].
Finally, beyond the scope of the present paper are the regulatory perspectives on randomization and practical implementation aspects, including statistical software and information systems to generate randomization schedules in real time. We hope to cover these topics in subsequent papers.
Availability of data and materials
All results reported in this paper are based either on theoretical considerations or simulation evidence. The computer code (using R and Julia programming languages) is fully documented and is available upon reasonable request.
Guess the next allocation as the treatment with fewest allocations in the sequence thus far, or make a random guess if the treatment numbers are equal.
Byar DP, Simon RM, Friedewald WT, Schlesselman JJ, DeMets DL, Ellenberg JH, Gail MH, Ware JH. Randomized clinical trials—perspectives on some recent ideas. N Engl J Med. 1976;295:74–80.
Article CAS PubMed Google Scholar
Collins R, Bowman L, Landray M, Peto R. The magic of randomization versus the myth of real-world evidence. N Engl J Med. 2020;382:674–8.
Article PubMed Google Scholar
ICH Harmonised tripartite guideline. General considerations for clinical trials E8. 1997.
Hernán MA, Robins JM. Using big data to emulate a target trial when a randomized trial is not available. Am J Epidemiol. 2016;183(8):758–64.
Article PubMed PubMed Central Google Scholar
Byar DP. Why data bases should not replace randomized clinical trials. Biometrics. 1980;36:337–42.
Mehra MR, Desai SS, Kuy SR, Henry TD, Patel AN. Cardiovascular disease, drug therapy, and mortality in Covid-19. N Engl J Med. 2020;382:e102. https://www.nejm.org/doi/10.1056/NEJMoa2007621 .
Mehra MR, Desai SS, Ruschitzka F, Patel AN. Hydroxychloroquine or chloroquine with or without a macrolide for treatment of COVID-19: a multinational registry analysis. Lancet. 2020. https://www.sciencedirect.com/science/article/pii/S0140673620311806?via%3Dihub .
Mehra MR, Desai SS, Kuy SR, Henry TD, Patel AN. Retraction: Cardiovascular disease, drug therapy, and mortality in Covid-19. N Engl J Med. 2020. https://doi.org/10.1056/NEJMoa2007621 . https://www.nejm.org/doi/10.1056/NEJMc2021225 .
Medical Research Council. Streptomycin treatment of pulmonary tuberculosis. BMJ. 1948;2:769–82.
Article Google Scholar
Rosenberger WF, Lachin J. Randomization in clinical trials: theory and practice. 2nd ed. New York: Wiley; 2015.
Google Scholar
Fisher RA. The design of experiments. Edinburgh: Oliver and Boyd; 1935.
Hill AB. The clinical trial. Br Med Bull. 1951;7(4):278–82.
Hill AB. Memories of the British streptomycin trial in tuberculosis: the first randomized clinical trial. Control Clin Trials. 1990;11:77–9.
Rosenberger WF, Uschner D, Wang Y. Randomization: The forgotten component of the randomized clinical trial. Stat Med. 2019;38(1):1–30 (with discussion).
Berger VW. Trials: the worst possible design (except for all the rest). Int J Person Centered Med. 2011;1(3):630–1.
Berger VW. Selection bias and covariate imbalances in randomized clinical trials. New York: Wiley; 2005.
Book Google Scholar
Berger VW. The alleged benefits of unrestricted randomization. In: Berger VW, editor. Randomization, masking, and allocation concealment. Boca Raton: CRC Press; 2018. p. 39–50.
Altman DG, Bland JM. Treatment allocation in controlled trials: why randomise? BMJ. 1999;318:1209.
Article CAS PubMed PubMed Central Google Scholar
Senn S. Testing for baseline balance in clinical trials. Stat Med. 1994;13:1715–26.
Senn S. Seven myths of randomisation in clinical trials. Stat Med. 2013;32:1439–50.
Rosenberger WF, Sverdlov O. Handling covariates in the design of clinical trials. Stat Sci. 2008;23:404–19.
Proschan M, Dodd L. Re-randomization tests in clinical trials. Stat Med. 2019;38:2292–302.
Spiegelhalter DJ, Freedman LS, Parmar MK. Bayesian approaches to randomized trials. J R Stat Soc A Stat Soc. 1994;157(3):357–87.
Berry SM, Carlin BP, Lee JJ, Muller P. Bayesian adaptive methods for clinical trials. Boca Raton: CRC Press; 2010.
Lachin J. Properties of simple randomization in clinical trials. Control Clin Trials. 1988;9:312–26.
Pocock SJ. Allocation of patients to treatment in clinical trials. Biometrics. 1979;35(1):183–97.
Simon R. Restricted randomization designs in clinical trials. Biometrics. 1979;35(2):503–12.
Blackwell D, Hodges JL. Design for the control of selection bias. Ann Math Stat. 1957;28(2):449–60.
Matts JP, McHugh R. Analysis of accrual randomized clinical trials with balanced groups in strata. J Chronic Dis. 1978;31:725–40.
Matts JP, Lachin JM. Properties of permuted-block randomization in clinical trials. Control Clin Trials. 1988;9:327–44.
ICH Harmonised Tripartite Guideline. Statistical principles for clinical trials E9. 1998.
Shao H, Rosenberger WF. Properties of the random block design for clinical trials. In: Kunert J, Müller CH, Atkinson AC, eds. mODa 11 – Advances in model-oriented design and analysis. Springer International Publishing Switzerland; 2016. 225–233.
Zhao W. Evolution of restricted randomization with maximum tolerated imbalance. In: Berger VW, editor. Randomization, masking, and allocation concealment. Boca Raton: CRC Press; 2018. p. 61–81.
Bailey RA, Nelson PR. Hadamard randomization: a valid restriction of random permuted blocks. Biom J. 2003;45(5):554–60.
Berger VW, Ivanova A, Knoll MD. Minimizing predictability while retaining balance through the use of less restrictive randomization procedures. Stat Med. 2003;22:3017–28.
Zhao W, Berger VW, Yu Z. The asymptotic maximal procedure for subject randomization in clinical trials. Stat Methods Med Res. 2018;27(7):2142–53.
Soares JF, Wu CFJ. Some restricted randomization rules in sequential designs. Commun Stat Theory Methods. 1983;12(17):2017–34.
Chen YP. Biased coin design with imbalance tolerance. Commun Stat Stochastic Models. 1999;15(5):953–75.
Chen YP. Which design is better? Ehrenfest urn versus biased coin. Adv Appl Probab. 2000;32:738–49.
Zhao W, Weng Y. Block urn design—A new randomization algorithm for sequential trials with two or more treatments and balanced or unbalanced allocation. Contemp Clin Trials. 2011;32:953–61.
van der Pas SL. Merged block randomisation: A novel randomisation procedure for small clinical trials. Clin Trials. 2019;16(3):246–52.
Zhao W. Letter to the Editor – Selection bias, allocation concealment and randomization design in clinical trials. Contemp Clin Trials. 2013;36:263–5.
Berger VW, Bejleri K, Agnor R. Comparing MTI randomization procedures to blocked randomization. Stat Med. 2016;35:685–94.
Efron B. Forcing a sequential experiment to be balanced. Biometrika. 1971;58(3):403–17.
Wei LJ. The adaptive biased coin design for sequential experiments. Ann Stat. 1978;6(1):92–100.
Atkinson AC. Optimum biased coin designs for sequential clinical trials with prognostic factors. Biometrika. 1982;69(1):61–7.
Smith RL. Sequential treatment allocation using biased coin designs. J Roy Stat Soc B. 1984;46(3):519–43.
Ball FG, Smith AFM, Verdinelli I. Biased coin designs with a Bayesian bias. J Stat Planning Infer. 1993;34(3):403–21.
BaldiAntognini A, Giovagnoli A. A new ‘biased coin design’ for the sequential allocation of two treatments. Appl Stat. 2004;53(4):651–64.
Atkinson AC. Selecting a biased-coin design. Stat Sci. 2014;29(1):144–63.
Rosenberger WF. Randomized urn models and sequential design. Sequential Anal. 2002;21(1&2):1–41 (with discussion).
Wei LJ. A class of designs for sequential clinical trials. J Am Stat Assoc. 1977;72(358):382–6.
Wei LJ, Lachin JM. Properties of the urn randomization in clinical trials. Control Clin Trials. 1988;9:345–64.
Schouten HJA. Adaptive biased urn randomization in small strata when blinding is impossible. Biometrics. 1995;51(4):1529–35.
Ivanova A. A play-the-winner-type urn design with reduced variability. Metrika. 2003;58:1–13.
Kundt G. A new proposal for setting parameter values in restricted randomization methods. Methods Inf Med. 2007;46(4):440–9.
Kalish LA, Begg CB. Treatment allocation methods in clinical trials: a review. Stat Med. 1985;4:129–44.
Zhao W, Weng Y, Wu Q, Palesch Y. Quantitative comparison of randomization designs in sequential clinical trials based on treatment balance and allocation randomness. Pharm Stat. 2012;11:39–48.
Flournoy N, Haines LM, Rosenberger WF. A graphical comparison of response-adaptive randomization procedures. Statistics in Biopharmaceutical Research. 2013;5(2):126–41.
Hilgers RD, Uschner D, Rosenberger WF, Heussen N. ERDO – a framework to select an appropriate randomization procedure for clinical trials. BMC Med Res Methodol. 2017;17:159.
Burman CF. On sequential treatment allocations in clinical trials. PhD Thesis Dept. Mathematics, Göteborg. 1996.
Azriel D, Mandel M, Rinott Y. Optimal allocation to maximize the power of two-sample tests for binary response. Biometrika. 2012;99(1):101–13.
Begg CB, Kalish LA. Treatment allocation for nonlinear models in clinical trials: the logistic model. Biometrics. 1984;40:409–20.
Kalish LA, Harrington DP. Efficiency of balanced treatment allocation for survival analysis. Biometrics. 1988;44(3):815–21.
Sverdlov O, Rosenberger WF. On recent advances in optimal allocation designs for clinical trials. J Stat Theory Practice. 2013;7(4):753–73.
Sverdlov O, Ryeznik Y, Wong WK. On optimal designs for clinical trials: an updated review. J Stat Theory Pract. 2020;14:10.
Rosenkranz GK. The impact of randomization on the analysis of clinical trials. Stat Med. 2011;30:3475–87.
Galbete A, Rosenberger WF. On the use of randomization tests following adaptive designs. J Biopharm Stat. 2016;26(3):466–74.
Proschan M. Influence of selection bias on type I error rate under random permuted block design. Stat Sin. 1994;4:219–31.
Kennes LN, Cramer E, Hilgers RD, Heussen N. The impact of selection bias on test decisions in randomized clinical trials. Stat Med. 2011;30:2573–81.
PubMed Google Scholar
Rückbeil MV, Hilgers RD, Heussen N. Assessing the impact of selection bias on test decisions in trials with a time-to-event outcome. Stat Med. 2017;36:2656–68.
Berger VW, Exner DV. Detecting selection bias in randomized clinical trials. Control Clin Trials. 1999;25:515–24.
Ivanova A, Barrier RC, Berger VW. Adjusting for observable selection bias in block randomized trials. Stat Med. 2005;24:1537–46.
Kennes LN, Rosenberger WF, Hilgers RD. Inference for blocked randomization under a selection bias model. Biometrics. 2015;71:979–84.
Hilgers RD, Manolov M, Heussen N, Rosenberger WF. Design and analysis of stratified clinical trials in the presence of bias. Stat Methods Med Res. 2020;29(6):1715–27.
Hamilton SA. Dynamically allocating treatment when the cost of goods is high and drug supply is limited. Control Clin Trials. 2000;21(1):44–53.
Zhao W. Letter to the Editor – A better alternative to the inferior permuted block design is not necessarily complex. Stat Med. 2016;35:1736–8.
Berger VW. Pros and cons of permutation tests in clinical trials. Stat Med. 2000;19:1319–28.
Simon R, Simon NR. Using randomization tests to preserve type I error with response adaptive and covariate adaptive randomization. Statist Probab Lett. 2011;81:767–72.
Tamm M, Cramer E, Kennes LN, Hilgers RD. Influence of selection bias on the test decision. Methods Inf Med. 2012;51:138–43.
Tamm M, Hilgers RD. Chronological bias in randomized clinical trials arising from different types of unobserved time trends. Methods Inf Med. 2014;53:501–10.
BaldiAntognini A, Rosenberger WF, Wang Y, Zagoraiou M. Exact optimum coin bias in Efron’s randomization procedure. Stat Med. 2015;34:3760–8.
Chow SC, Shao J, Wang H, Lokhnygina. Sample size calculations in clinical research. 3rd ed. Boca Raton: CRC Press; 2018.
Heritier S, Gebski V, Pillai A. Dynamic balancing randomization in controlled clinical trials. Stat Med. 2005;24:3729–41.
Lovell DJ, Giannini EH, Reiff A, et al. Etanercept in children with polyarticular juvenile rheumatoid arthritis. N Engl J Med. 2000;342(11):763–9.
Zhao W. A better alternative to stratified permuted block design for subject randomization in clinical trials. Stat Med. 2014;33:5239–48.
Altman DG, Royston JP. The hidden effect of time. Stat Med. 1988;7:629–37.
Christensen E, Neuberger J, Crowe J, et al. Beneficial effect of azathioprine and prediction of prognosis in primary biliary cirrhosis. Gastroenterology. 1985;89:1084–91.
Rückbeil MV, Hilgers RD, Heussen N. Randomization in survival trials: An evaluation method that takes into account selection and chronological bias. PLoS ONE. 2019;14(6):e0217964.
Article CAS Google Scholar
Hilgers RD, König F, Molenberghs G, Senn S. Design and analysis of clinical trials for small rare disease populations. J Rare Dis Res Treatment. 2016;1(3):53–60.
Miller F, Zohar S, Stallard N, Madan J, Posch M, Hee SW, Pearce M, Vågerö M, Day S. Approaches to sample size calculation for clinical trials in rare diseases. Pharm Stat. 2017;17:214–30.
Kuznetsova OM, Tymofyeyev Y. Preserving the allocation ratio at every allocation with biased coin randomization and minimization in studies with unequal allocation. Stat Med. 2012;31(8):701–23.
Kuznetsova OM, Tymofyeyev Y. Brick tunnel and wide brick tunnel randomization for studies with unequal allocation. In: Sverdlov O, editor. Modern adaptive randomized clinical trials: statistical and practical aspects. Boca Raton: CRC Press; 2015. p. 83–114.
Kuznetsova OM, Tymofyeyev Y. Expansion of the modified Zelen’s approach randomization and dynamic randomization with partial block supplies at the centers to unequal allocation. Contemp Clin Trials. 2011;32:962–72.
EMA. Guideline on adjustment for baseline covariates in clinical trials. 2015.
Taves DR. Minimization: A new method of assigning patients to treatment and control groups. Clin Pharmacol Ther. 1974;15(5):443–53.
Pocock SJ, Simon R. Sequential treatment assignment with balancing for prognostic factors in the controlled clinical trial. Biometrics. 1975;31(1):103–15.
Hu F, Hu Y, Ma Z, Rosenberger WF. Adaptive randomization for balancing over covariates. Wiley Interdiscipl Rev Computational Stat. 2014;6(4):288–303.
Senn S. Statistical issues in drug development. 2nd ed. Wiley-Interscience; 2007.
Kuznetsova OM, Tymofyeyev Y. Covariate-adaptive randomization with unequal allocation. In: Sverdlov O, editor. Modern adaptive randomized clinical trials: statistical and practical aspects. Boca Raton: CRC Press; 2015. p. 171–97.
Berry DA. Adaptive clinical trials: the promise and the caution. J Clin Oncol. 2011;29(6):606–9.
Trippa L, Lee EQ, Wen PY, Batchelor TT, Cloughesy T, Parmigiani G, Alexander BM. Bayesian adaptive randomized trial design for patients with recurrent glioblastoma. J Clin Oncol. 2012;30(26):3258–63.
Hu F, Rosenberger WF. The theory of response-adaptive randomization in clinical trials. New York: Wiley; 2006.
Atkinson AC, Biswas A. Randomised response-adaptive designs in clinical trials. Boca Raton: CRC Press; 2014.
Rugo HS, Olopade OI, DeMichele A, et al. Adaptive randomization of veliparib–carboplatin treatment in breast cancer. N Engl J Med. 2016;375:23–34.
Berry SM, Petzold EA, Dull P, et al. A response-adaptive randomization platform trial for efficient evaluation of Ebola virus treatments: a model for pandemic response. Clin Trials. 2016;13:22–30.
Ware JH. Investigating therapies of potentially great benefit: ECMO. (with discussion). Stat Sci. 1989;4(4):298–340.
Hey SP, Kimmelman J. Are outcome-adaptive allocation trials ethical? (with discussion). Clin Trials. 2005;12(2):102–27.
Proschan M, Evans S. Resist the temptation of response-adaptive randomization. Clin Infect Dis. 2020;71(11):3002–4. https://doi.org/10.1093/cid/ciaa334 .
Villar SS, Robertson DS, Rosenberger WF. The temptation of overgeneralizing response-adaptive randomization. Clinical Infectious Diseases. 2020; ciaa1027; doi: https://doi.org/10.1093/cid/ciaa1027 .
Proschan M. Reply to Villar, et al. Clinical infectious diseases. 2020; ciaa1029; doi: https://doi.org/10.1093/cid/ciaa1029 .
Donner A, Klar N. Design and Analysis of Cluster Randomization Trials in Health Research. London: Arnold Publishers Limited; 2000.
Klasnja P, Hekler EB, Shiffman S, Boruvka A, Almirall D, Tewari A, Murphy SA. Micro-randomized trials: An experimental design for developing just-in-time adaptive interventions. Health Psychol. 2015;34:1220–8.
Article PubMed Central Google Scholar
Download references
Acknowledgements
The authors are grateful to Robert A. Beckman for his continuous efforts coordinating Innovative Design Scientific Working Groups, which is also a networking research platform for the Randomization ID SWG. We would also like to thank the editorial board and the two anonymous reviewers for the valuable comments which helped to substantially improve the original version of the manuscript.
None. The opinions expressed in this article are those of the authors and may not reflect the opinions of the organizations that they work for.
Author information
Authors and affiliations.
National Institutes of Health, Bethesda, MD, USA
Vance W. Berger
Boehringer Ingelheim Pharma GmbH & Co. KG, Biberach, Germany
Louis Joseph Bour
Boehringer-Ingelheim Pharmaceuticals Inc, Ridgefield, CT, USA
Kerstine Carter
Population Health Sciences, University of Utah School of Medicine, Salt Lake City UT, USA
Jonathan J. Chipman
Cancer Biostatistics, University of Utah Huntsman Cancer Institute, Salt Lake City UT, USA
Clinical Trials Research Unit, University of Leeds, Leeds, UK
Colin C. Everett
RWTH Aachen University, Aachen, Germany
Nicole Heussen & Ralf-Dieter Hilgers
Medical School, Sigmund Freud University, Vienna, Austria
Nicole Heussen
York Trials Unit, Department of Health Sciences, University of York, York, UK
Catherine Hewitt
Food and Drug Administration, Silver Spring, MD, USA
Yuqun Abigail Luo
Open University of Catalonia (UOC) and the University of Barcelona (UB), Barcelona, Spain
Jone Renteria
Department of Human Development and Quantitative Methodology, University of Maryland, College Park, MD, USA
BioPharma Early Biometrics & Statistical Innovations, Data Science & AI, R&D BioPharmaceuticals, AstraZeneca, Gothenburg, Sweden
Yevgen Ryeznik
Early Development Analytics, Novartis Pharmaceuticals Corporation, NJ, East Hanover, USA
Oleksandr Sverdlov
Biostatistics Center & Department of Biostatistics and Bioinformatics, George Washington University, DC, Washington, USA
Diane Uschner
You can also search for this author in PubMed Google Scholar
- Robert A Beckman
Contributions
Conception: VWB, KC, NH, RDH, OS. Writing of the main manuscript: OS, with contributions from VWB, KC, JJC, CE, NH, and RDH. Design of simulation studies: OS, YR. Development of code and running simulations: YR. Digitization and preparation of data for Fig. 5 : JR. All authors reviewed the original manuscript and the revised version. The authors read and approved the final manuscript.
Corresponding author
Correspondence to Oleksandr Sverdlov .
Ethics declarations
Ethics approval and consent to participate.
Not applicable.
Consent for publication
Competing interests, additional information, publisher's note.
Springer Nature remains neutral with regard to jurisdictional claims in published maps and institutional affiliations.
Supplementary Information
Additional file 1: figure s1.
. Type I error rate under selection bias model with bias effect ( \(\nu\) ) in the range 0 (no bias) to 1 (strong bias) for 12 randomization designs and three statistical tests.
Rights and permissions
Open Access This article is licensed under a Creative Commons Attribution 4.0 International License, which permits use, sharing, adaptation, distribution and reproduction in any medium or format, as long as you give appropriate credit to the original author(s) and the source, provide a link to the Creative Commons licence, and indicate if changes were made. The images or other third party material in this article are included in the article's Creative Commons licence, unless indicated otherwise in a credit line to the material. If material is not included in the article's Creative Commons licence and your intended use is not permitted by statutory regulation or exceeds the permitted use, you will need to obtain permission directly from the copyright holder. To view a copy of this licence, visit http://creativecommons.org/licenses/by/4.0/ . The Creative Commons Public Domain Dedication waiver ( http://creativecommons.org/publicdomain/zero/1.0/ ) applies to the data made available in this article, unless otherwise stated in a credit line to the data.
Reprints and permissions
About this article
Cite this article.
Berger, V., Bour, L., Carter, K. et al. A roadmap to using randomization in clinical trials. BMC Med Res Methodol 21 , 168 (2021). https://doi.org/10.1186/s12874-021-01303-z
Download citation
Received : 24 December 2020
Accepted : 14 April 2021
Published : 16 August 2021
DOI : https://doi.org/10.1186/s12874-021-01303-z
Share this article
Anyone you share the following link with will be able to read this content:
Sorry, a shareable link is not currently available for this article.
Provided by the Springer Nature SharedIt content-sharing initiative
- Randomization-based test
- Restricted randomization design
BMC Medical Research Methodology
ISSN: 1471-2288
- General enquiries: [email protected]
Protection of Random Assignment
- First Online: 14 October 2021
Cite this chapter
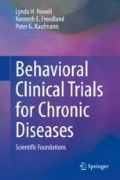
- Lynda H. Powell 4 ,
- Peter G. Kaufmann 5 &
- Kenneth E. Freedland 6
496 Accesses
Existence of an alternative explanation for the benefit of a treatment is a confounder. It is a nuisance “passenger” variable that rides along with treatment and undermines the ability to make causal inferences. This chapter focuses on why random assignment is so powerful and should be protected. It presents a history of attempts to answer the question of whether or not a treatment works, and the arrival at random assignment as the best way to make causal inferences about the benefits of a treatment. It defines confounding as an error of interpretation and the essential role of avoiding it by protecting the random assignment. It then goes on to illustrate ways to protect random assignment in the design, conduct, and analyses of a trial, with particular attention to the central role of identifying a patient-centered target population, recruiting it, retaining it, and insuring that all randomized participants are included in the evaluation of trial results.
- Confounding
- Random assignment
- Recruitment
- Intent-to-treat analyses
- Per-protocol analyses
- Post-randomization exclusions
- Target population
- Patient-centered target population
- pre-contemplators
- Inconclusive trial
- Responder-only analyses
- On-treatment analyses
“Daniel and his three companions were young Israelites who were taken to serve in the palace of the king of Babylon because they were of noble royal family, without physical defect, handsome, versed in wisdom, and competent. Daniel determined he would not defile himself with the King’s food or wine. He asked the overseer: ‘Please test us for 10 days and let us be given some vegetables to eat and water to drink. Then let our appearance be compared to the appearance of youths who are eating the King’s choice food.’ At the end of 10 days, their appearance seemed better and they were fatter than any of the youths who had been eating the King’s food. So the overseer let them continue to eat vegetables and drink water instead of what the king provided.” Bible, Old Testament, Book of Daniel 1:16
This is a preview of subscription content, log in via an institution to check access.
Access this chapter
- Available as PDF
- Read on any device
- Instant download
- Own it forever
- Available as EPUB and PDF
- Durable hardcover edition
- Dispatched in 3 to 5 business days
- Free shipping worldwide - see info
Tax calculation will be finalised at checkout
Purchases are for personal use only
Institutional subscriptions
Bull JP (1959) The historical development of clinical therapeutic trials. J Chron Dis 10:218–248
PubMed Google Scholar
Armitage P (1982) The role of randomization in clinical trials. Stat Med 1:345–352
Van Helmont JB (1662) Oriatrike or Physik Refined. In Debus AG (1968) The chemical dream of the renaissance. Heffer, London
Google Scholar
Peirce CS, Jastrow J (1884) Fifth memoir: on small differences of sensation. Ntl Acad Sci 3:73–83
Yule G (1924) The function of statistical method in scientific investigation. Industrial Health Research Board Report 28. His Majesty’s Stationery Office, London
Eliot MM (1925) The control of rickets: preliminary discussion of the demonstration in New Haven. JAMA 85:656–663
Hill AB (1952) The clinical trial. New Engl J Med 247:113–119
Hill AB (1953) Observation and experiment. New Engl J Med 248:995–1001
Sinclair HM (1951) Nutritional surveys of population groups. New Engl J Med 245:39–47
Mill JS (1843) A system of logic ratiocinative and inductive. Being a connected view of the principles of evidence and the methods of scientific investigation. Book I. In Robson JM (ed). The collected works of John Stuart Mill (1974). University of Toronto Press, Toronto
Hill AB (1965) The environment and disease: association or causation. Proc Roy Soc Med 58:295–300
Wang D, Bakhai A (2006) Clinical trials: a practical guide to design, analysis, and reporting. Remedica, London
Domanski M, McKinlay S (2009) Successful randomized trials. A handbook for the 21st century. Lippincott Williams & Wilkins, Philadelphia
Friedman LM, Furberg CD, DeMets D, Reboussin DH, Granger CB (2015) Fundamentals of clinical trials, 5th edn. Springer, Cham
Rothman KJ, Greenland S, Lash TL (2008) Modern epidemiology, 3rd edn. Lippincott Williams & Wilkins, Philadelphia
Szklo M, Nieto FJ (2019) Epidemiology: beyond the basics, 4th edn. Jones & Bartlett Learning, Burlington
Hennekens CH, Buring JE, Mayrent SL (1987) Epidemiology in medicine. Little Brown, Boston
Susser M (1973) Causal thinking in the health sciences: Concepts and strategies of epidemiology. Oxford University Press, New York
Fisher RA (1951) The design of experiments, 6th edn. Hafner, New York
Shadish WR, Cook TD, Campbell DT (2002) Experimental and quasi-experimental designs for generalized causal inference. Houghton Mifflin, Boston
Byar DP, Simon RM, Friedewald WT, Schlesselman JJ, DeMets D, Ellenberg JH, Gail MH, Ware JH (1976) Randomized clinical trials--perspectives on some recent ideas. N Engl J Med 295:74–80
Moher D, Hopewell S, Schulz KF, Montori V, Gotzche PC, Devereaux PJ, Elbourne D, Egger M, Altman DG (2010) CONSORT 2010 explanation and elaboration: updated guidelines for reporting parallel group randomised trials. BMJ 340:c869. https://doi.org/10.1136/bmj.c869
Mosteller F, Gilbert JP, McPeek B (1980) Reporting standards and research strategies for controlled trials. Control Clin Trials 1:37–58
Schulz KF, Chalmers I, Hayes RJ, Altman DG (1995) Empirical evidence of bias. Dimensions of methodological quality associated with estimates of treatment effects in controlled trials. JAMA 273:408–412
CONSORT Group (2010) CONSORT checklist. www.consort-statement.org
Schulz KF, Altman DG, Moher D, CONSORT Group (2010) CONSORT 2010 statement: updated guidelines for reporting parallel group randomized trials. Ann Intern Med 152:726–732
Zwarenstein M, Treweek S, Gagnier JJ, Altman DG, Tunis S, Haynes B, Oxman AD, Moher D, and for the CONSORT and Pragmatic Trials in Healthcare (Practihc) groups (2008) Improving the reporting of pragmatic trials: an extension of the CONSORT statement. BMJ 337:a2390. https://doi.org/10.1136/bmj.a2390
Schulz KF (1995) Subverting randomization in controlled trials. JAMA 274:1456–1458
Kraemer HC (2015) A source of false findings in published research studies: adjusting for covariates. JAMA Psychiatry 72:961–962
Pocock SJ, Assmann SE, Enos LE, Kasten LE (2002) Subgroup analysis, covariate adjustment and baseline comparisons in clinical trial reporting: current practice and problems. Stat Med 21:2917–2930
Schulz KF, Grimes DA, Altman DG, Hayes RJ (1996) Blinding and exclusions after allocation in randomised controlled trials: survey of published parallel group trials in obstetrics and gynaecology. BMJ 312:742–744
PubMed PubMed Central Google Scholar
Detry MA, Lewis RJ (2014) The intention-to-treat principle: how to assess the true effect of choosing a medical treatment. JAMA 312:85–86
Freedman B (1987) Equipoise and the ethics of clinical research. N Eng J Med 317:141–145
Green SB, Byar DP (1984) Using observational data from registries to compare treatments: the fallacy of omnimetrics. Stat Med 3:361–373
Hollon SD, Wampold BE (2009) Are randomized controlled trials relevant to clinical practice? Can J Psychiatry 54:637–643
Cook TD, Campbell DT (1979) Quasi-experimentation: Design and analysis issues for field settings. Houghton Mifflin, Boston
Glasgow RE, Lichtenstein E, Marcus AC (2003) Why don’t we see more translation of health promotion research to practice? Rethinking the efficacy-to-effectiveness transition. Am J Public Health 93:1261–1267
Areán PA, Kraemer HC (2013) High-quality psychotherapy research: From conception to piloting to national trials. Oxford University Press, New York
Brownell KD, Wadden TA (1992) Etiology and treatment of obesity: understanding a serious, prevalent, and refractory disorder. J Consult Clin Psychol 60:505–517
Prochaska JO, DiClemente CC, Norcross JC (1992) In search of how people change: Applications to addictive behaviors. Am Psychol 47:1102–1114
Hall SM, Tsoh JY, Prochaska JJ, Eisendrath S, Rossi JS, Redding CA, Rosen AB, Meisner M, Humfleet GL, Gorecki JA (2006) Treatment for cigarette smoking among depressed mental health outpatients: a randomized clinical trial. Am J Public Health 96:1808–1814
Prochaska JJ, Hall SE, Delucchi K, Hall SM (2014) Efficacy of initiating tobacco dependence treatment in inpatient psychiatry: a randomized controlled trial. Am J Public Health 104:1557–1565
Prochaska JJ, Hall SE, Hall SM (2009) Stage-tailored tobacco cessation treatment in inpatient psychiatry. Psychiatr Serv 60:848. https://doi:10.1176/appi.ps.60.6.848
Prochaska JJ, Velicer WF, Prochaska JO, Delucchi K, Hall SM (2006) Comparing intervention outcomes in smokers treated for single versus multiple behavioral risks. Health Psychol 25:380–388
The Steering Committee of the Physicians Health Study Research Group (1988) Preliminary report: findings from the aspirin component of the ongoing Physicians’ Health Study. N Engl J Med 318:262–264
Coronary Drug Project Research Group (1980) Influence of adherence to treatment and response of cholesterol on mortality in the Coronary Drug Project. N Engl J Med 303:1038–1041
Adamson J, Cockayne S, Puffer S, Torgerson DJ (2006) Review of randomised trials using the post-randomised consent (Zelen’s) design. Contemp Clin Trials 27:305–319
Fabricatore AN, Wadden TA, Moore RH, Butryn ML, Gravallese EA, Erondu NE, Heymsfield SB, Nguyen AM (2009) Attrition from randomized controlled trials of pharmacological weight loss agents: a systematic review and analysis. Obes Rev 10:333–341
Lang JM (1990) The use of a run-in to enhance compliance. Stat Med 9:87–93
Kong W, Langlois MF, Kamga-Ngandé C, Gagnon C, Brown C, Baillargeon JP (2010) Predictors of success to weight-loss intervention program in individuals at high risk for type 2 diabetes. Diabetes Res Clin Pract 90:147–153
Teixeira PJ, Going SB, Houtkooper LB, Cussler EC, Metcalfe LL, Blew RM, Sardinha LB, Lohman TG (2004) Pretreatment predictors of attrition and successful weight management in women. Int J Obes Relat Metab Disord 28:1124–1133
Czajkowski SM, Powell LH, Adler N, Naar-King S, Reynolds KD, Hunter CM, Laraia B, Olster DH, Perna FM, Peterson JC, Epel E, Boyington JE, Charlson ME (2015) From ideas to efficacy: the ORBIT model for developing behavioral treatments for chronic diseases. Health Psychol 34:971–982
Bailey JV, Pavlou M, Copas A, McCarthy OL, Carswell K, Rait G, Hart G, Nazareth I, Free CJ, French R, Murray E (2013) The Sexunzipped trial: optimizing the design of online randomized controlled trials. J Med Internet Res 15:e278. https://doi.org/10.2196/jmir.2668
Boyd A, Tilling K, Cornish R, Davies A, Humphries K, Macleod J (2015) Professionally designed information materials and telephone reminders improved consent response rates: evidence from an RCT nested within a cohort study. J Clin Epidemiol 68:877–887
Dickson S, Logan J, Hagen S, Stark D, Glazener C, McDonald AM, McPherson G (2013) Reflecting on the methodological challenges of recruiting to a United Kingdom-wide, multi-centre, randomised controlled trial in gynaecology outpatient settings. Trials 14:389. https://doi.org/10.1186/1745-6215-14-389
Gupta A, Calfas KJ, Marshall SJ, Robinson TN, Rock CL, Huang JS, Epstein-Corbin M, Servetas C, Donohue MC, Norman GJ, Raab F, Merchant G, Fowler JH, Griswold WG, Fogg BJ, Patrick K (2015) Clinical trial management of participant recruitment, enrollment, engagement, and retention in the SMART study using a Marketing and Information Technology (MARKIT) model. Contemp Clin Trials 42:185–195
Hadidi N, Buckwalter K, Lindquist R, Rangen C (2012) Lessons learned in recruitment and retention of stroke survivors. J Neurosci Nurs 44:105–110
Hartlieb KB, Jacques-Tiura AJ, Naar-King S, Ellis DA, Jen KL, Marshall S (2015) Recruitment strategies and the retention of obese urban racial/ethnic minority adolescents in clinical trials: the FIT families project, Michigan, 2010–2014. Prev Chronic Dis 12:E22. https://doi.org/10.5888/pcd12.140409
Johnson DA, Joosten YA, Wilkins CH, Shibao CA (2015) Case study. Community engagement and clinical trial success: outreach to African American women. Clin Transl Sci 8:388–390
Blake K, Holbrook JT, Antal H, Shade D, Bunnell HT, McCahan SM, Wise RA, Pennington C, Garfinkel P, Wysocki T (2015) Use of mobile devices and the internet for multimedia informed consent delivery and data entry in a pediatric asthma trial: study design and rationale. Contemp Clin Trials 42:105–118
Cermak SA, Stein Duker LI, Williams ME, Lane CJ, Dawson ME, Borreson AE, Polido JC (2015) Feasibility of a sensory-adapted dental environment for children with autism. Am J Occup Ther 69:6903220020. https://doi.org/10.5014/ajot.2015.013714
Giuffrida A, Torgerson DJ (1997) Should we pay the patient? Review of financial incentives to enhance patient compliance. BMJ 315:703–707
Brown SD, Lee K, Schoffman DE, King AC, Crawley LM, Kiernan M (2012) Minority recruitment into clinical trials: experimental findings and practical implications. Contemp Clin Trials 33:620–623
Kiernan M, Phillips K, Fair JM, King AC (2000) Using direct mail to recruit Hispanic adults into a dietary intervention: an experimental study. Ann Behav Med 22:89–93
Batliner T, Fehringer KA, Tiwari T, Henderson WG, Wilson A, Brega AG, Albino J (2014) Motivational interviewing with American Indian mothers to prevent early childhood caries: study design and methodology of a randomized control trial. Trials 15:125. https://doi.org/10.1186/1745-6215-15-125
Article PubMed PubMed Central Google Scholar
Clark F, Pyatak EA, Carlson M, Blanche E, Vigen C, Hay J, Mallinson T, Blanchard J, Unger JB, Garber SL, Diaz J, Florindez L, Atkins M, Rubayi S, Azen SP, PUPS Study Group (2014) Implementing trials of complex interventions in community settings: the USC-Rancho Los Amigos Pressure Ulcer Prevention Study (PUPS). Clin Trials 11:218–229
Cruz TH, Davis SM, FitzGerald CA, Canaca GF, Keane PC (2014) Engagement, recruitment, and retention in a trans-community, randomized controlled trial for the prevention of obesity in rural American Indian and Hispanic children. J Prim Prev 35:135–149
Jimenez DE, Reynolds CF 3rd, Alegría M, Harvey P, Bartels SJ (2015) The Happy Older Latinos are Active (HOLA) health promotion and prevention study: study protocol for a pilot randomized controlled trial. Trials 6:579. https://doi.org/10.1186/s13063-015-1113-3
Koziol-McLain J, Vandal AC, Nada-Raja S, Wilson D, Glass NE, Eden KB, McLean C, Dobbs T, Case J (2015) A web-based intervention for abused women: the New Zealand isafe randomised controlled trial protocol. BMC Public Health 15:56. https://doi.org/10.1186/s12889-015-1395-0
Bakari M, Munseri P, Francis J, Aris E, Moshiro C, Siyame D, Janabi M, Ngatoluwa M, Aboud S, Lyamuya E, Sandström E, Mhalu F (2013) Experiences on recruitment and retention of volunteers in the first HIV vaccine trial in Dar es Salam, Tanzania - the phase I/II HIVIS 03 trial. BMC Public Health 13:1149. https://doi.org/10.1186/1471-2458-13-1149
Goldberg JH, Kiernan M (2005) Innovative techniques to address retention in a behavioral weight-loss trial. Health Educ Res 20:439–447
National Commission for the Protection of Human Subjects of Biomedical Behavioral Research (1978) The Belmont report: ethical principles and guidelines for the protection of human subjects of research. ERIC Clearinghouse, Bethesda
Moseley JB, O’Malley K, Petersen NJ, Menke TJ, Brody BA, Kuykendall DH, Hollingsworth JC, Ashton CM, Wray NP (2002) A controlled trial of arthroscopic surgery for osteoarthritis of the knee. N Engl J Med 347:81–88
Hays JL, Hunt JR, Hubbell FA, Anderson GL, Limacher MC, Allen C, Rossouw JE (2003) The Women’s Health Initiative recruitment methods and results. Ann Epidemiol 13:S18–S77
Kaptchuk TJ, Friedlander E, Kelley JM, Sanchez MN, Kokkotou E, Singer JP, Kowalczykowski M, Miller FG, Kirsch I, Lembo AJ (2010) Placebos without deception: a randomized controlled trial in irritable bowel syndrome. PLoS One 5:e15591. https://doi.org/10.1371/journal.pone.0015591
Crichton GE, Howe PR, Buckley JD, Coates AM, Murphy KJ, Bryan J (2012) Long-term dietary intervention trials: critical issues and challenges. Trials 13:111. https://doi.org/10.1186/1745-6215-13-111
Hulley SB, Cummings SR, Browner WS, Grady DG, Newman TB (2013) Designing clinical research, 4th edn. Lippincott Williams & Wilkins, Philadelphia
Siddiqi AE, Sikorskii A, Given CW, Given B (2008) Early participant attrition from clinical trials: role of trial design and logistics. Clin Trials 5:328–335
Idoko OT, Owolabi OA, Odutola AA, Ogundare O, Worwui A, Saidu Y, Smith-Sanneh A, Tunkara A, Sey G, Sanyang A, Mendy P, Ota MO (2014) Lessons in participant retention in the course of a randomized controlled clinical trial. BMC Res Notes 7:706. https://doi.org/10.1186/1756-0500-7-706
Rucker-Whitaker C, Flynn KJ, Kravitz G, Eaton C, Calvin JE, Powell LH (2006) Understanding African-American participation in a behavioral intervention: results from focus groups. Contemp Clin Trials 27:274–286
Gross D, Fogg L (2004) A critical analysis of the intent-to-treat principle in prevention research. J Primary Prevention 25:475–489
Feinstein AR (1991) Intent-to-treat policy for analyzing randomized trials: statistical distortions and neglected clinical challenges. In: Cramer JA, Spilker B (eds) Patient compliance in medical practice and clinical trials. Raven, New York
Sheiner LB, Rubin DB (1995) Intention-to-treat analysis and the goals of clinical trials. Clin Pharmacol Ther 57:6–15
Knowler WC, Barrett-Connor E, Fowler SE, Hamman RF, Lachin JM, Walker EA, Nathan DM, Diabetes Prevention Program Research Group (2002) Reduction in the incidence of type 2 diabetes with lifestyle intervention or metformin. N Engl J Med 346:393–403
Diabetes Prevention Program Research Group (1999) The Diabetes Prevention Program. Design and methods for a clinical trial in the prevention of type 2 diabetes. Diabetes Care 22:623–634
Diabetes Prevention Program Research Group (2000) The Diabetes Prevention Program: baseline characteristics of the randomized cohort. Diabetes Care 23:1619–1629
Frasure-Smith N, Prince R (1985) The Ischemic Heart Disease Life Stress Monitoring Program. Impact on mortality. Psychosom Med 47:431–445
Frasure-Smith N, Prince R (1989) Long-term follow-up of the Ischemic Heart Disease Life Stress Monitoring Program. Psychosom Med 51:485–513
Powell LH (1989) Unanswered questions in the Ischemic Heart Disease Life Stress Monitoring Program. Psychosom Med 51:479–484
Frasure-Smith N, Lespérance F, Prince RH, Verrier P, Garber RA, Juneau M, Wolfson C, Bourassa MG (1997) Randomised trial of home-based psychosocial nursing intervention for patients recovering from myocardial infarction. Lancet 350:473–479
O’Connor CM, Whellan DJ, Lee KL, Keteyian SJ, Cooper LS, Ellis SJ, Leifer ES, Kraus WE, Kitzman DW, Blumenthal JA, Rendall DS, Miller NH, Fleg JL, Schulman KA, McKelvie RS, Zannad F, Piña IL, HF-ACTION Investigators (2009) Efficacy and safety of exercise training in patients with chronic heart failure: HF-ACTION randomized controlled trial. JAMA 301:1439–1450
Keteyian SJ, Leifer ES, Houston-Miller N, Kraus WE, Brawner CA, O’Connor CM, Whellan DJ, Cooper LS, Fleg JL, Kitzman DW, Cohen-Solal A, Blumenthal JA, Rendall DS, Piña IL, HF-ACTION Investigators (2012) Relation between volume of exercise and clinical outcomes in patients with heart failure. J Am Coll Cardiol 60:1899–1905
Yancy CW, Jessup M, Bozkurt B, Butler J, Casey DE Jr, Drazner MH, Fonarow GC, Geraci SA, Horwich T, Januzzi JL, Johnson MR, Kasper EK, Levy WC, Masoudi FA, McBride PE, McMurray JJ, Mitchell JE, Peterson PN, Riegel B, Sam F, Stevenson LW, Tang WH, Tsai EJ, Wilkoff BL, American College of Cardiology Foundation, American Heart Association Task Force on Practice Guidelines (2013) 2013 ACCF/AHA guideline for the management of heart failure: a report of the American College of Cardiology Foundation/American Heart Association Task Force on Practice Guidelines. J Am Coll Cardiol 62:e147–e239
Centers for Medicare and Medicaid Services (2014) Decision memo for cardiac rehabilitation programs - chronic heart failure (CAG-00437N). US Department of Health & Human Services. http://www.cms.gov/medicare-coverage-database/details/nca-decision-memo.aspx?
McCambridge J, Kypri K, Elbourne D (2014) In randomization we trust? There are overlooked problems in experimenting with people in behavioral intervention trials. J Clin Epidemiol 67:247–253
Ashley EA (2015) The precision medicine initiative: a new national effort. JAMA 313:2019–2020
Khoury MJ, Evans JP (2015) A public health perspective on a national precision medicine cohort: balancing long-term knowledge generation with early health benefit. JAMA 313:2117–2118
Ma J, Rosas LG, Lv N (2016) Precision lifestyle medicine: a new frontier in the science of behavior change and population health. Am J Prev Med 50:395–397
Brewin CR, Bradley C (1989) Patient preferences and randomised clinical trials. Br Med J 299:313–315
Download references
Author information
Authors and affiliations.
Department of Preventive Medicine, Rush University Medical Center, Chicago, IL, USA
Lynda H. Powell
College of Nursing, Villanova University, Villanova, PA, USA
Peter G. Kaufmann
Department of Psychiatry, Washington University in St. Louis, St. Louis, MO, USA
Kenneth E. Freedland
You can also search for this author in PubMed Google Scholar
Rights and permissions
Reprints and permissions
Copyright information
© 2021 Springer Nature Switzerland AG
About this chapter
Powell, L.H., Kaufmann, P.G., Freedland, K.E. (2021). Protection of Random Assignment. In: Behavioral Clinical Trials for Chronic Diseases. Springer, Cham. https://doi.org/10.1007/978-3-030-39330-4_8
Download citation
DOI : https://doi.org/10.1007/978-3-030-39330-4_8
Published : 14 October 2021
Publisher Name : Springer, Cham
Print ISBN : 978-3-030-39328-1
Online ISBN : 978-3-030-39330-4
eBook Packages : Behavioral Science and Psychology Behavioral Science and Psychology (R0)
Share this chapter
Anyone you share the following link with will be able to read this content:
Sorry, a shareable link is not currently available for this article.
Provided by the Springer Nature SharedIt content-sharing initiative
- Publish with us
Policies and ethics
- Find a journal
- Track your research

Learn About Randomization
Clinical trial randomization.
Clinical trials are research studies "in which one or more human subjects are prospectively assigned to one or more interventions (which may include placebo or other control) to evaluate the effects of those interventions on health-related biomedical or behavioral outcomes," as defined by the NIH .
A key determinant of randomized clinical trial quality is the process by which subjects (or "participants") are assigned an intervention (or "treatment group").
Reducing predictability with randomization
Treatment groups must comprise similar participants so that the comparison of treatments is unbiased. It is imperative to avoid selection bias by ensuring that participants are not assigned to a group based on identifiable health characteristics. To this end, participants are assigned to groups randomly. All randomization procedures are intended to reduce selection bias, but they differ in how effectively they achieve this goal. Therefore, the method of randomization chosen must aim to reduce the predictability of assignment to each treatment group .
Maintaining balance over time
According to a trial's design requirements, each treatment group ideally will have been assigned a certain number of participants by each time point. If treatments are assigned randomly, without restriction, it could happen by chance that one treatment group is assigned too frequently, while another group is assigned too infrequently. Even if the treatment groups end in balance, it may still happen that at some point during the trial the imbalance reaches unacceptable levels. The method of randomization chosen must ensure that, as the randomization proceeds, the expected number of participants are assigned to each treatment group and that balance is maintained over time .
MTI Randomization Procedures
A maximally tolerated imbalance (MTI) procedure is an ideal way to randomly assign participants to treatment groups in a clinical trial. As the name suggests, an MTI is a quantity which specifies how unbalanced the group sizes can ever become during the randomization sequence. In other words, as each participant is randomized, the difference between any two treatment groups never exceeds the MTI.
MTI procedures have an important advantage compared to other methods. While randomization procedures in general maintain balance across treatment groups, MTI procedures do so with reduced predictability of group assignment. This is a crucial consideration, as the ability to predict treatment group must be minimized. MTI procedures are designed for that purpose.
Choosing an MTI
MTI procedures don't have a defined value for the MTI, so a value must be chosen by the investigator when planning the randomization process. A small MTI will maintain a tight group balance but suffer from increased group predictability. Conversely, a larger MTI decreases group predictability but is susceptible to greater group imbalance at any given time. For large trials, a larger MTI may be appropriate, and small trials might employ a smaller MTI. The choice of the MTI is up to the investigator. – If you are using the randomization tool on this site, a default MTI value is provided.
As to the computational calculation of the randomization sequence, a variety of MTI procedures are available: the maximal procedure [1] , the asymptotic maximal procedure [2] , the big stick procedure [3] , Chen's procedure [4] , and the block urn design [5] . See Table II of [6] for a graphical illustration of how these MTI procedures compare. The choice of procedure is up to the investigator. – If you are using the randomization tool on this site, the default procedure is the asymptotic maximal procedure.
Ultimately, the choice of MTI value and MTI procedure are left to the investigator.
Example of MTI randomization
Suppose participants are randomized into two groups, one treatment and one control. The investigator chooses an MTI of 3. At no point in time can the imbalance between treatment groups exceed 3.
If at any particular time during the trial we see, for example, 20 subjects allocated to treatment A and 23 subjects allocated to treatment B, and if the MTI value is 3, then we have reached the MTI boundary. Therefore, any MTI procedure will ensure that the next allocation (44 th subject) is to treatment A, so as to avoid violating the MTI condition.
Maximal procedure
Among MTI procedures, the maximal procedure is often considered best at reducing the overall predictability of treatment assignment. It does this by constructing all possible ways participants might be randomized into groups, and constructing a course of randomization that is just as likely as any other course.
In a maximal procedure the probability of assignment to a treatment depends on the current imbalance between groups and on the number of participants remaining to be randomized (i.e., how many allocations remain in the randomization sequence).
Asymptotic maximal procedure
The asymptotic maximal procedure is based upon the same principles as the maximal procedure but is simpler in design and implementation. In the asymptotic maximal procedure, the probability of group assignment depends only on the imbalance between the group sizes. The asymptotic maximal procedure uses fixed allocation probabilities, so that it is more easily described to clinical colleagues than the maximal procedure.
As trial size increases, the asymptotic maximal procedure becomes more like a maximal procedure.
Chen's procedure
Like the maximal and asymptotic maximal procedures, Chen's procedure applies a balance-forcing probability when treatment group sizes are unbalanced. Unlike the maximal and asymptotic maximal procedures, Chen's procedure ignores the extent of imbalance and considers only whether groups are or are not balanced.
Big stick procedure
The big stick procedure is in essence a special case of Chen's procedure. In the big stick procedure, the MTI constrains imbalance between treatment groups, but the probability of treatment assignment is otherwise independent of whether the treatment has more or fewer participants than expected by that point in time.
Block urn design
Another method is the block urn design, which in some ways is analogous to permuted blocks (see Suboptimal Methods below), but much improved in that the blocks "reset" under certain conditions, reducing the overall predictability of treatment group assignments.
Suboptimal Methods
Unrestricted randomization.
In concept, unrestricted randomization is similar to tossing a fair coin for each participant assignment, with no consideration given to the number of participants currently assigned to each treatment group. An obvious concern with unrestricted randomization is the risk of large group imbalances and, relatedly, chronological bias [7] . Chronological bias means that, even if final group sizes are their intended size, it may happen that a treatment is assigned too frequently during one period of time and too infrequently at another time. This becomes a problem when there are time trends, because then, through a common association with time, treatment assignment and some predictor of success will become confounded. Moreover, it is also impractical to use unrestricted randomization in the way intended [8] . These issues render unrestricted randomization less than ideal.
Permuted block randomization
Given the limitations of unrestricted randomization and the need to maintain group balance, many investigators employ a method called permuted block randomization. As the name suggests, treatment group assignment order is arranged within small blocks and participants are assigned accordingly within each block of treatments. By the end of each block, an exact and known number of participants have been assigned to each treatment group, and a new block begins. A major disadvantage to blocked randomization is its high group assignment predictability and susceptibility to selection bias, and so it is not preferred when better methods are available [9] .
As an example, consider 2 treatment groups, A and B, where each group will have the same number of subjects. If the block size is 4, treatments can be arranged six different ways: AABB, ABAB, ABBA, BAAB, BABA, and BBAA. At the start of randomization, one of these blocks is chosen randomly. If after the first 3 participants are randomized the pattern ABB is observed, then it is known for certain that the next participant will be assigned to treatment A. In other words, the 4 th allocation is deterministic for treatment A. In this case, even the 2 nd allocation is predictable, though not deterministic.
Even if block procedures employ varied block size (such as randomly alternating between blocks of 4 and 6) in an attempt (generally unsuccessful) to eliminate deterministic assignments at the end of each block, permuted blocks force balance between treatment groups so frequently that the overall predictability of group assignment is much higher than the preferred MTI procedures.
- Berger, VW, Ivanova, A, Deloria-Knoll, M. Minimizing Predictability while Retaining Balance through the Use of Less Restrictive Randomization Procedures. Statistics in Medicine . 2003;22(19):3017-3028. doi:10.1002/sim.1538
- Zhao, W, Berger, VW, Yu, Z. The Asymptotic Maximal Procedure for Subject Randomization in Clinical Trials. Statistical Methods in Medical Research . 2018;27(7):2142-2153. doi:10.1177/0962280216677107
- Soares, JF, We, CFL. Some Restricted Randomization Rules in Sequential Designs. Communications in Statistics Theory and Methods . 1983;12:2017-2034. doi:10.1080/03610928308828586
- Chen, YP. Biased Coin Design With Imbalance Intolerance. Communications in Statistics Stochastic Models . 1999;15:953-975. doi:10.1080/15326349908807570
- Zhao, W, Weng, Y. Block Urn Design -- A New Randomization Algorithm for Sequential Trials with Two or More Treatments and Balanced or Unbalanced Allocation. Contemporary Clinical Trials . 2011;32(6):953-961. doi:10.1016/j.cct.2011.08.004
- Berger, VW, Bejleri, K, Agnor, R. Comparing MTI Randomization Procedures To Blocked Randomization. Statistics In Medicine . 2016;35:685-694. doi:10.1002/sim.6637
- Matts, JP, McHugh, RB. Conditional Markov Chain Design for Accrual Clinical Trials. Biometrical Journal . 1983;25:563-577. doi:10.1002/bimj.19830250608
- Berger, VW. The Alleged Benefits of Unrestricted Randomization in "Randomization, Masking, and Allocation Concealment". Boca Raton: Vance W. Berger, Editor, CRC Press, Chapman and Hall; 2018.
- Berger, VW. Allocation Concealment and Blinding: When Ignorance is Bliss. Medical Journal of Australia . 2005;183(3):165. doi:10.5694/j.1326-5377.2005.tb06974.x
Random allocation in controlled clinical trials: a review
Affiliation.
- 1 Ladoke Akintola University of Technology.
- PMID: 24934553
- DOI: 10.18433/j3sw36
Purpose: An allocation strategy that allows for chance placement of participants to study groups is crucial to the experimental nature of randomised controlled trials. Following decades of the discovery of randomisation considerable erroneous opinion and misrepresentations of its concept both in principle and practice still exists. In some circles, opinions are also divided on the strength and weaknesses of each of the random allocation strategies. This review provides an update on various random allocation techniques so as to correct existing misconceptions on this all important procedure.
Methods: This is a review of literatures published in the Pubmed database on concepts of common allocation techniques used in controlled clinical trials.
Results: Allocation methods that use; case record number, date of birth, date of presentation, haphazard or alternating assignment are non-random allocation techniques and should not be confused as random methods. Four main random allocation techniques were identified. Minimisation procedure though not fully a random technique, however, proffers solution to the limitations of stratification at balancing for multiple prognostic factors, as the procedure makes treatment groups similar in several important features even in small sample trials.
Conclusions: Even though generation of allocation sequence by simple randomisation procedure is easily facilitated, a major drawback of the technique is that treatment groups can by chance end up being dissimilar both in size and composition of prognostic factors. More complex allocation techniques that yield more comparable treatment groups also have certain drawbacks. However, it is important that whichever allocation technique is employed, unpredictability of random assignment should not be compromised.
Publication types
- Research Support, Non-U.S. Gov't
- Random Allocation*
- Randomized Controlled Trials as Topic

An official website of the United States government
The .gov means it’s official. Federal government websites often end in .gov or .mil. Before sharing sensitive information, make sure you’re on a federal government site.
The site is secure. The https:// ensures that you are connecting to the official website and that any information you provide is encrypted and transmitted securely.
- Publications
- Account settings
Preview improvements coming to the PMC website in October 2024. Learn More or Try it out now .
- Advanced Search
- Journal List
- J Hum Reprod Sci
- v.4(1); Jan-Apr 2011
This article has been retracted.
An overview of randomization techniques: an unbiased assessment of outcome in clinical research.
Department of Biostatics, National Institute of Animal Nutrition & Physiology (NIANP), Adugodi, Bangalore, India
Randomization as a method of experimental control has been extensively used in human clinical trials and other biological experiments. It prevents the selection bias and insures against the accidental bias. It produces the comparable groups and eliminates the source of bias in treatment assignments. Finally, it permits the use of probability theory to express the likelihood of chance as a source for the difference of end outcome. This paper discusses the different methods of randomization and use of online statistical computing web programming ( www.graphpad.com /quickcalcs or www.randomization.com ) to generate the randomization schedule. Issues related to randomization are also discussed in this paper.
INTRODUCTION
A good experiment or trial minimizes the variability of the evaluation and provides unbiased evaluation of the intervention by avoiding confounding from other factors, which are known and unknown. Randomization ensures that each patient has an equal chance of receiving any of the treatments under study, generate comparable intervention groups, which are alike in all the important aspects except for the intervention each groups receives. It also provides a basis for the statistical methods used in analyzing the data. The basic benefits of randomization are as follows: it eliminates the selection bias, balances the groups with respect to many known and unknown confounding or prognostic variables, and forms the basis for statistical tests, a basis for an assumption of free statistical test of the equality of treatments. In general, a randomized experiment is an essential tool for testing the efficacy of the treatment.
In practice, randomization requires generating randomization schedules, which should be reproducible. Generation of a randomization schedule usually includes obtaining the random numbers and assigning random numbers to each subject or treatment conditions. Random numbers can be generated by computers or can come from random number tables found in the most statistical text books. For simple experiments with small number of subjects, randomization can be performed easily by assigning the random numbers from random number tables to the treatment conditions. However, in the large sample size situation or if restricted randomization or stratified randomization to be performed for an experiment or if an unbalanced allocation ratio will be used, it is better to use the computer programming to do the randomization such as SAS, R environment etc.[ 1 – 6 ]
REASON FOR RANDOMIZATION
Researchers in life science research demand randomization for several reasons. First, subjects in various groups should not differ in any systematic way. In a clinical research, if treatment groups are systematically different, research results will be biased. Suppose that subjects are assigned to control and treatment groups in a study examining the efficacy of a surgical intervention. If a greater proportion of older subjects are assigned to the treatment group, then the outcome of the surgical intervention may be influenced by this imbalance. The effects of the treatment would be indistinguishable from the influence of the imbalance of covariates, thereby requiring the researcher to control for the covariates in the analysis to obtain an unbiased result.[ 7 , 8 ]
Second, proper randomization ensures no a priori knowledge of group assignment (i.e., allocation concealment). That is, researchers, subject or patients or participants, and others should not know to which group the subject will be assigned. Knowledge of group assignment creates a layer of potential selection bias that may taint the data.[ 9 ] Schul and Grimes stated that trials with inadequate or unclear randomization tended to overestimate treatment effects up to 40% compared with those that used proper randomization. The outcome of the research can be negatively influenced by this inadequate randomization.
Statistical techniques such as analysis of covariance (ANCOVA), multivariate ANCOVA, or both, are often used to adjust for covariate imbalance in the analysis stage of the clinical research. However, the interpretation of this post adjustment approach is often difficult because imbalance of covariates frequently leads to unanticipated interaction effects, such as unequal slopes among subgroups of covariates.[ 1 ] One of the critical assumptions in ANCOVA is that the slopes of regression lines are the same for each group of covariates. The adjustment needed for each covariate group may vary, which is problematic because ANCOVA uses the average slope across the groups to adjust the outcome variable. Thus, the ideal way of balancing covariates among groups is to apply sound randomization in the design stage of a clinical research (before the adjustment procedure) instead of post data collection. In such instances, random assignment is necessary and guarantees validity for statistical tests of significance that are used to compare treatments.
TYPES OF RANDOMIZATION
Many procedures have been proposed for the random assignment of participants to treatment groups in clinical trials. In this article, common randomization techniques, including simple randomization, block randomization, stratified randomization, and covariate adaptive randomization, are reviewed. Each method is described along with its advantages and disadvantages. It is very important to select a method that will produce interpretable and valid results for your study. Use of online software to generate randomization code using block randomization procedure will be presented.
Simple randomization
Randomization based on a single sequence of random assignments is known as simple randomization.[ 3 ] This technique maintains complete randomness of the assignment of a subject to a particular group. The most common and basic method of simple randomization is flipping a coin. For example, with two treatment groups (control versus treatment), the side of the coin (i.e., heads - control, tails - treatment) determines the assignment of each subject. Other methods include using a shuffled deck of cards (e.g., even - control, odd - treatment) or throwing a dice (e.g., below and equal to 3 - control, over 3 - treatment). A random number table found in a statistics book or computer-generated random numbers can also be used for simple randomization of subjects.
This randomization approach is simple and easy to implement in a clinical research. In large clinical research, simple randomization can be trusted to generate similar numbers of subjects among groups. However, randomization results could be problematic in relatively small sample size clinical research, resulting in an unequal number of participants among groups.
Block randomization
The block randomization method is designed to randomize subjects into groups that result in equal sample sizes. This method is used to ensure a balance in sample size across groups over time. Blocks are small and balanced with predetermined group assignments, which keeps the numbers of subjects in each group similar at all times.[ 1 , 2 ] The block size is determined by the researcher and should be a multiple of the number of groups (i.e., with two treatment groups, block size of either 4, 6, or 8). Blocks are best used in smaller increments as researchers can more easily control balance.[ 10 ]
After block size has been determined, all possible balanced combinations of assignment within the block (i.e., equal number for all groups within the block) must be calculated. Blocks are then randomly chosen to determine the patients’ assignment into the groups.
Although balance in sample size may be achieved with this method, groups may be generated that are rarely comparable in terms of certain covariates. For example, one group may have more participants with secondary diseases (e.g., diabetes, multiple sclerosis, cancer, hypertension, etc.) that could confound the data and may negatively influence the results of the clinical trial.[ 11 ] Pocock and Simon stressed the importance of controlling for these covariates because of serious consequences to the interpretation of the results. Such an imbalance could introduce bias in the statistical analysis and reduce the power of the study. Hence, sample size and covariates must be balanced in clinical research.
Stratified randomization
The stratified randomization method addresses the need to control and balance the influence of covariates. This method can be used to achieve balance among groups in terms of subjects’ baseline characteristics (covariates). Specific covariates must be identified by the researcher who understands the potential influence each covariate has on the dependent variable. Stratified randomization is achieved by generating a separate block for each combination of covariates, and subjects are assigned to the appropriate block of covariates. After all subjects have been identified and assigned into blocks, simple randomization is performed within each block to assign subjects to one of the groups.
The stratified randomization method controls for the possible influence of covariates that would jeopardize the conclusions of the clinical research. For example, a clinical research of different rehabilitation techniques after a surgical procedure will have a number of covariates. It is well known that the age of the subject affects the rate of prognosis. Thus, age could be a confounding variable and influence the outcome of the clinical research. Stratified randomization can balance the control and treatment groups for age or other identified covariates. Although stratified randomization is a relatively simple and useful technique, especially for smaller clinical trials, it becomes complicated to implement if many covariates must be controlled.[ 12 ] Stratified randomization has another limitation; it works only when all subjects have been identified before group assignment. However, this method is rarely applicable because clinical research subjects are often enrolled one at a time on a continuous basis. When baseline characteristics of all subjects are not available before assignment, using stratified randomization is difficult.[ 10 ]
Covariate adaptive randomization
One potential problem with small to moderate size clinical research is that simple randomization (with or without taking stratification of prognostic variables into account) may result in imbalance of important covariates among treatment groups. Imbalance of covariates is important because of its potential to influence the interpretation of a research results. Covariate adaptive randomization has been recommended by many researchers as a valid alternative randomization method for clinical research.[ 8 , 13 ] In covariate adaptive randomization, a new participant is sequentially assigned to a particular treatment group by taking into account the specific covariates and previous assignments of participants.[ 7 ] Covariate adaptive randomization uses the method of minimization by assessing the imbalance of sample size among several covariates.
Using the online randomization http://www.graphpad.com/quickcalcs/index.cfm , researcher can generate randomization plan for treatment assignment to patients. This online software is very simple and easy to implement. Up to 10 treatments can be allocated to patients and the replication of treatment can also be performed up to 9 times. The major limitations of this software is that once the randomization plan is generated, same randomization plan cannot be generated as this uses the seed point of local computer clock and is not displayed for further use. Other limitation of this online software Maximum of only 10 treatments can be assigned to patients. Entering the web address http://www.graphpad.com/quickcalcs/index.cfm on address bar of any browser, the page of graphpad appears with number of options. Select the option of “Random Numbers” and then press continue, Random Number Calculator with three options appears. Select the tab “Randomly assign subjects to groups” and press continue. In the next page, enter the number of subjects in each group in the tab “Assign” and select the number of groups from the tab “Subjects to each group” and keep number 1 in repeat tab if there is no replication in the study. For example, the total number of patients in a three group experimental study is 30 and each group will assigned to 10 patients. Type 10 in the “Assign” tab and select 3 in the tab “Subjects to each group” and then press “do it” button. The results is obtained as shown as below (partial output is presented)
Another randomization online software, which can be used to generate randomization plan is http://www.randomization.com . The seed for the random number generator[ 14 , 15 ] (Wichmann and Hill, 1982, as modified by McLeod, 1985) is obtained from the clock of the local computer and is printed at the bottom of the randomization plan. If a seed is included in the request, it overrides the value obtained from the clock and can be used to reproduce or verify a particular plan. Up to 20 treatments can be specified. The randomization plan is not affected by the order in which the treatments are entered or the particular boxes left blank if not all are needed. The program begins by sorting treatment names internally. The sorting is case sensitive, however, so the same capitalization should be used when recreating an earlier plan. Example of 10 patients allocating to two groups (each with 5 patients), first the enter the treatment labels in the boxes, and enter the total number of patients that is 10 in the tab “Number of subjects per block” and enter the 1 in the tab “Number of blocks” for simple randomization or more than one for Block randomization. The output of this online software is presented as follows.
The benefits of randomization are numerous. It ensures against the accidental bias in the experiment and produces comparable groups in all the respect except the intervention each group received. The purpose of this paper is to introduce the randomization, including concept and significance and to review several randomization techniques to guide the researchers and practitioners to better design their randomized clinical trials. Use of online randomization was effectively demonstrated in this article for benefit of researchers. Simple randomization works well for the large clinical trails ( n >100) and for small to moderate clinical trials ( n <100) without covariates, use of block randomization helps to achieve the balance. For small to moderate size clinical trials with several prognostic factors or covariates, the adaptive randomization method could be more useful in providing a means to achieve treatment balance.
Source of Support: Nil
Conflict of Interest: None declared.
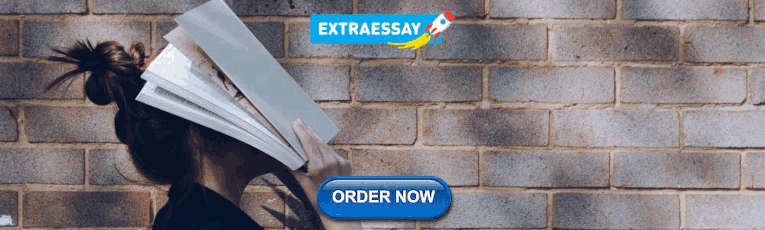
IMAGES
VIDEO
COMMENTS
Implementation of randomization in clinical trials is due to A. Bradford Hill who designed the first randomized clinical trial evaluating the use of ... systematic design such as a sequence of alternating treatment assignments has a major fallacy: an investigator, knowing an upcoming treatment assignment in a sequence, may enroll a patient who ...
Example: Random assignment. In your study, you have two groups: a control group that receives no intervention. an experimental group that has a remote team-building intervention every week for a month. You use random assignment to place participants into the control or experimental group.
The process of random assignment of patients to groups is called randomization. Randomization in clinical trials is an essential concept for minimizing bias, ensuring fairness, and maximizing the statistical power of the study results. In this article, we will discuss the concept of randomization in clinical trials, why it is important, and go ...
Randomized controlled trial is widely accepted as the best design for evaluating the efficacy of a new treatment because of the advantages of randomization (random allocation). Randomization eliminates accidental bias, including selection bias, and provides a base for allowing the use of probability theory.
Various research designs can be used to acquire scientific medical evidence. The randomized controlled trial (RCT) has been recognized as the most credible research design for investigations of the clinical effectiveness of new medical interventions [1, 2].Evidence from RCTs is widely used as a basis for submissions of regulatory dossiers in request of marketing authorization for new drugs ...
Summary: The major steps in the planning and conduction of a randomized clinical trial (RCT) include the definition of the study population, the random assignment of treatments, the choice of the measures of treatment effects, the duration of the experiment, the assessment of the tolerability and safety of the treatment, and the choice of ...
Random sampling is a process for obtaining a sample that accurately represents a population. Random assignment uses a chance process to assign subjects to experimental groups. Using random assignment requires that the experimenters can control the group assignment for all study subjects. For our study, we must be able to assign our participants ...
The primary goal of comparative clinical trials is to provide comparisons of treatments with maximum precision and validity. 4 One critical component of clinical trials is random assignment of participants into groups. Randomizing participants helps remove the effect of extraneous variables (eg, age, injury history) and minimizes bias ...
The essential rationale for protecting random assignment is to obtain a conclusive result. An inconclusive result occurs when there is an alternative explanation for the outcome of a trial [ 95 ]. The risk of confounding is great in the presence of the background noise that surrounds most trials in humans.
Clinical trials are research studies "in which one or more human subjects are prospectively assigned to one or more interventions (which may include placebo or other control) to evaluate the effects of those interventions on health-related biomedical or behavioral outcomes," as defined by the NIH. A key determinant of randomized clinical trial quality is the process by which subjects (or ...
Random assignment or random placement is an experimental technique for assigning human participants or animal subjects to different groups in an experiment (e.g., a treatment group versus a control group) using randomization, such as by a chance procedure (e.g., flipping a coin) or a random number generator. This ensures that each participant or subject has an equal chance of being placed in ...
Abstract. Purpose: An allocation strategy that allows for chance placement of participants to study groups is crucial to the experimental nature of randomised controlled trials. Following decades of the discovery of randomisation considerable erroneous opinion and misrepresentations of its concept both in principle and practice still exists.
The major purpose of random assignment in a clinical trial is to: a. help ensure that study subjects are representative of the general population b. facilitate double blinding (masking) c. facilitate the measurement of outcome variables d. ensure that the study groups have comparable baseline characteristics e. reduce selection bias in the allocation of treatment
Abstract. Randomized clinical trial (RCT) is the gold standard study for the evaluation of health interventions and is considered the second level of evidence for clinical decision making. However, the quality of the evidence produced by these studies is dependent on the methodological rigor employed at every stage of their execution.
Cumulative Incidence Ratio=80/90=0.89. Study with Quizlet and memorize flashcards containing terms like 1. The major purpose of random assignment in a clinical trial is to:, 2. Based on the evidence given, the claim is incorrect because:, 3. The purpose of a double blind or double masked study is to: and more.
The major purpose of random assignment in a clinical trial is to: A. Help ensure that study subjects are representative of the general population B. Facilitate double blinding (masking) C. Facilitate the measurement of outcome variables D. Ensure that the study groups have comparable baseline characteristics E. Reduce selection bias in the allocation of treatment
The purpose of this paper is to introduce the randomization, including concept and significance and to review several randomization techniques to guide the researchers and practitioners to better design their randomized clinical trials. Use of online randomization was effectively demonstrated in this article for benefit of researchers.
1) The major purpose of random assignment in a clinical trial is to:a. Help ensure that study subjects are representative of the general populationb. Facilitate double blinding (masking)c. Facilitate the measurement of outcome variablesd. Ensure that the study groups have comparable baseline characteristicse.
The major purpose of a random assignment in a clinical trial is to: a. Help ensure that study subjects are representative of the general population b. Reduce selection bias in subject allocation to treatment c. Facilitate double blinding d. Facilitate measurement of outcome variables The purpose of a double-blind study is to: a.
The major purpose of random assignment in a clinical trial is to: ... Ch. 7,8,9. Share. Terms in this set (20) reduce selection bias in the allocation of treatment. The major purpose of random assignment in a clinical trial is to: may be incorrect because no control or comparison group was involved.
1) e Reduce the allocatio bias in …. The major purpose of random assignment in a clinical trial is to: Help ensure that study subjects are representative of the general population Facilitate double blinding (masking) Facilitate the measurement of outcome variables Ensure that the study groups have comparable baseline characteristics Reduce ...
The major purpose of random assignment in a clinical trial is to: a. help ensure that study subjects are representative of the general population b. facilitate double blinding c. facilitate the measurement of outcome variables d. ensure that the study groups have comparable baseline characteristics e. reduce selection bias in the allocation of treatment