How To Get A Quant Job Once You Have A PhD
In this article we are going to discuss an issue that repeatedly crops up via the QuantStart mailbox, namely how to get a quant job once you have a PhD . There's a lot of confusion around this topic because quite a few people who currently work in academia and want to make the shift believe that it is quite straightforward to "walk into" a high-paying financial role. While this may have been true 10-15 years ago, the reality of the current job market is such that quant roles are now highly competitive and candidates need to stand out if they are to get the best jobs.
Firstly we'll discuss what sort of candidates you will be competing against when considering going for interview. Secondly, we'll discuss how to make an honest assessment of your PhD and what you got out of it that might be relevant to quantitative finance roles. Finally, we'll consider whether it is necessary to return to school in order to train up in a quant-specific qualification.
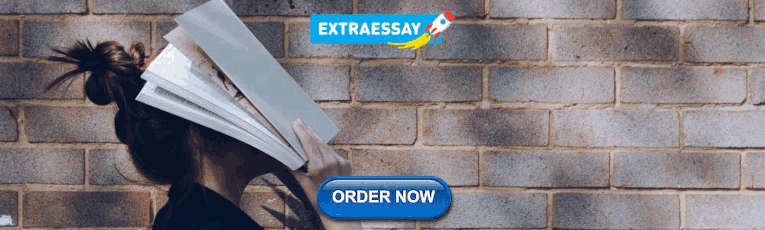
The Competition
I've made it rather clear on QuantStart that the competition for some of the top quantitative trading researcher roles can be extremely tough. In the UK the best roles tend to be filled well upstream of any "front door" interview process. Usually extremely bright academics in mathematics, physics, computer science, economics or mathematical finance are head-hunted for a particular skill set, such as deep expertise on market microstructure, insight into high-frequency trading algorithms, novel stochastic calculus techniques for certain derivatives pricing regimes or extensive statistical machine learning knowledge that applies to datasets used by such funds.
When such quant researcher roles ARE opened up to the public they will often state that they are looking for "only the best and brightest", which in the UK usually means "Top Five" universities (Cambridge, Oxford, Imperial College, LSE and UCL). In the US this will mean high-end Ivy League institutions. The adverts will often state that they want to see evidence of consistent Mathematical Olympiad prizes and an extensive publication list in a relevant field.
While this is certainly true of the top roles, there are plenty of other (very well paying and prestigious) jobs that also need filling. Bear in mind that there are only so many Mathematical Olympiad winners, after all! Thus one should not be disheartened when seeing numerous adverts asking for such qualifications. There are plenty of smaller funds and boutique outfits that do not have the resources to aggressively hunt for the ultimate talent and so will be more than willing to employ bright PhDs who might not necessarily have an Olympiad track record.
Honestly Assess Your PhD
The first task to carry out when applying for quant roles is an honest assessment of your PhD and what you achieved with it . Primarily you need to consider the level of mathematical ability you were able to attain as well as your computational programming skill.
Quant roles in the derivative pricing space, known traditionally as the "quant analyst" or "financial engineer", require a reasonable amount of mathematical sophistication. Specifically, expertise in stochastic calculus, probability and measure theory. These are topics usually taught in an undergraduate mathematics course, but can form a component of taught graduate school PhDs. In addition they require a good understanding of scientific programming usually in C++, Python or MatLab. Since the role of a quant analyst is often to code up an implementation of a particular algorithm from a research paper, under heavy deadlines, it is quite naturally suited to those with PhDs of this type.
Quant roles in the algorithmic trading and quant hedge fund world are almost exclusively going to require novel methods for generating "alpha" (i.e. excess return above a benchmark). Usually this is accomplished via time series analysis and econometrics, but more recently statistical machine learning techniques have been applied, as have methods related to sentiment analysis. Some of the best quant funds make extensive use of even more advanced graduate level mathematics in the realms of algebraic geometry, number theory and information theory. Hence anything highly mathematically, statistically or physically oriented is likely to be of interest to a top quant hedge fund.
As for computer scientists and strong scientific software developers, generally there is always work available for quantitative developer roles. Although you will be competing against those with industry experience in rigourous software engineering. Hence "academic code" of the "20,000 line single-file of Fortran" variety might be a bit of a hindrance! Make sure to brush up on the more modern software development methodologies such as OOP , Agile , etc.
I want to discuss specific PhD fields as well, to give you an idea of where you might consider focusing your efforts based on what you have previously studied:
- Pure Mathematics - The top funds generally hire the pure mathematicians from esoteric realms such as algebraic geometry and information theory. Banks will also take individuals who study stochastic calculus to a high level for their derivatives research teams.
- Mathematical Finance - Portfolio optimisation and derivatives pricing are two common themes studied in mathematical finance PhDs. You will often have collaborated with banks during your PhD, so it is unlikely your job prospects will be slim! If you are struggling, it can be very helpful to contact department heads as they will often have a strong network.
- Theoretical Physics - Funds will be very interested in your ability to model physical phenomena, either through direct or statistical approaches. Some theoretical physics areas are highly mathematical (Cosmology, String Theory, Quantum Field Theory etc) and so the advice given to theoretical physics PhDs is similar to pure mathematicians.
- Computational Physics/Engineering - The main skillset taught here is how to take an algorithm and produce a robust scientific computing implementation, perhaps in a parallelised fashion. This is an extremely useful skill for quant work both in banks and funds, especially for developing infrastructure. Make sure however to brush up on core topics such as statistics and stochastic calculus prior to interview.
- Statistics/Econometrics - Statisticians and theoretical econometricians will be in good demand from technical quant funds, especially in the Commodity Trading Advisor (CTA)/Managed Futures space. The time series modelling will be highly appropriate here.
- Computer Science/Machine Learning - Many funds are now making extensive use of machine learning and optimisation tools, which are the natural domain of the theoretical computer scientist and, more recently, the "data scientist". Familiarity with statistical machine learning and Bayesian methods will be highly attractive.
- Bioinformatics - Bioinformaticians also make extensive use of machine learning tools on "big data" sets. For interview you will want to emphasise your familiarity with such tools and your programming capability. Depending upon your background you may need to brush up on your (pure) mathematics for interview questions.
- Economics/Finance - Economics and Finance PhDs do not always teach you the mathematical maturity necessary for pure quant work, but it really depends on the project. You will need to be honest with yourself about where you lie on the mathematical spectrum. In addition you will need to consider your programming ability.
Heading Back To School
An extremely common question that I receive in the QuantStart mailbag is whether to return to school for finance-specific training subsequent to a PhD.
I've previously documented my views on Masters in Financial Engineering (MFE) programs as related to quantitative trading . In essence I believe that MFEs are not hugely suitable for quantitative trading research work, but they are a good entry point into investment banking quant work.
If your PhD was not heavy on quantitative or programming work, but you have a sufficiently mature mathematical background, then it can make good sense to take a MFE assuming that you can afford to fund the course. A MFE at a top-tier school will provide you with a solid network of other candidates (and thus people who might later help you secure a role), a relatively healthy recruitment position upon graduation and a useful skillset for investment banking derivatives pricing work.
I would advise against returning to school if you have a strong quantitative PhD as you simply won't need the additional qualifications and you should be able to pick up the necessary interview material yourself, albeit with a lot of study.
If you have a PhD in a non-quantitative field and your background is not sufficiently mathematical, then you should definitely consider that you will likely need to return to school if you truly want to work in quantitative finance. In particular you will need to study an undergraduate degree that has a strong quantitative component such as Mathematics or Physics as these two degrees will generally let you transition into other quantitative fields easily.
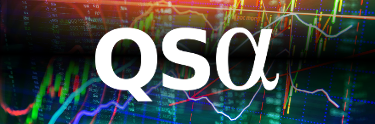
Join the QSAlpha research platform that helps fill your strategy research pipeline, diversifies your portfolio and improves your risk-adjusted returns for increased profitability.
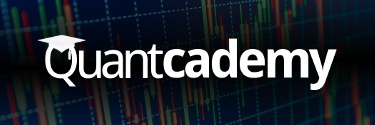
The Quantcademy
Join the Quantcademy membership portal that caters to the rapidly-growing retail quant trader community and learn how to increase your strategy profitability.
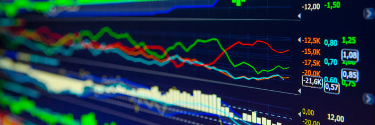
Successful Algorithmic Trading
How to find new trading strategy ideas and objectively assess them for your portfolio using a Python-based backtesting engine.
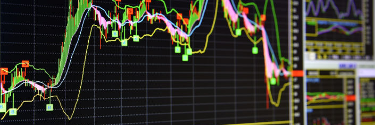
Advanced Algorithmic Trading
How to implement advanced trading strategies using time series analysis, machine learning and Bayesian statistics with R and Python.
- Search Search Please fill out this field.
What Do Quantitative Analysts Do?
Where do quant analysts work.
- Skills and Education
The Right Career for You?
The bottom line.
- Career Advice
Quants: The Rocket Scientists of Wall Street
Quantitative analysts are professionals who understand the complex mathematical models that price financial securities and are able to enhance them to generate profits and reduce risk. As financial securities have become increasingly complex, demand has grown steadily for quantitative analysts , often called simply "quants," or even the colloquially affectionate "quant geeks."
Because of the challenging nature of the work—which needs to blend mathematics, finance, and computer skills effectively—quant analysts are in great demand and able to command very high salaries. Here's a look at what they do, where they work, how much they earn, and what knowledge is required, to help you decide whether this may be the career for you.
Key Takeaways
- Quantitative analysts, or quants, combine their skills in finance, math, and computer software to analyze and predict the markets, creating complex models that can be used to price and trade securities.
- They tend to work in investment banks and for hedge funds, although insurance companies, commercial banks, and financial software and information providers may also hire them.
- Quants work in major financial centers in the U.S. and in London and Asia, among other places across the globe.
- Firms often look for candidates who have a master's degree or a Ph.D. in a quantitative subject, such as mathematics, economics, finance, or statistics.
- Compensation can be in the low-to-middle six figures.
Quantitative analysts design and implement complex models that allow financial firms to price and trade securities. They are employed primarily by investment banks and hedge funds , but sometimes also by commercial banks, insurance companies, and management consultancies; in addition to financial software and information providers.
Quants who work directly with traders , providing them with pricing or trading tools, are often referred to as " front-office " quants. In the " back office ," quants validate the models, conduct research, and create new trading strategies . For banks and insurance companies, the work is focused more on risk management than trading strategies. Front-office positions are typically more stressful and demanding but are better compensated.
The high demand for quants is driven by multiple trends:
- The rapid growth of hedge funds and automated trading systems
- The increasing complexity of both liquid and illiquid securities
- The need to give traders, accountants, and sales reps access to pricing and risk models
- The ongoing search for market-neutral investment strategies
Quantitative analyst positions are found almost exclusively in major financial centers with trading operations . In the United States, that would be New York and Chicago, and areas where hedge funds tend to cluster, such as Boston, Massachusetts and Stamford, Connecticut. Across the Atlantic, London dominates; in Asia, many quants are working in Hong Kong, Singapore, Tokyo, and Sydney, among other regional financial centers.
Despite the heavy concentration in those cities, quants are found all over the world—after all, many global firms analyze and/or trade complex securities, which creates demand for the quant's brainpower and abilities. But the problem that a quant working in Houston or San Francisco faces is that changing employers most likely would mean changing cities, whereas a quant working in Manhattan should be able to interview for and find a job within a mile or two of their previous one.
What Do Quants Earn?
Compensation in the field of finance tends to be very high, and quantitative analysis follows this trend. It is not uncommon to find positions with posted salaries of $250,000 or more, and when you add in bonuses, a quant could earn $500,000+ per year. As with most careers, the key to landing the high-paying jobs is a resume filled with experience, including with well-known employers, as well as reliance on recruiting firms and professional networking for opportunities.
The highest-paid positions are with hedge funds or other trading firms, and part of the compensation depends on the firm's earnings, also known as the profit and loss (P&L) . At the other end of the pay scale, an entry-level quant position may earn only $125,000 or $150,000, but this type of position provides a fast learning curve and plenty of room for future growth in both responsibilities and salary.
Also, some of the lower-paid quant positions likely would be primarily quant developers, which is more of a software-development position where the individual is not required to have as much math and financial expertise. An excellent quant developer could certainly earn $250,000, but that's about as high as the compensation package generally would go.
Despite the high pay level, some quants do complain that they are "second-class citizens" on Wall Street and don't earn the multimillion-dollar salaries that top hedge fund managers or investment bankers command. As you can see, financial success is always relative.
Estimated total pay of a quantitative analyst in the U.S. Google is among the 10 highest paying companies for a quant, offering an annual salary of $279,284.
Quants Skills and Education
Financial knowledge.
Many financial securities, such as options and convertibles , are easy to understand conceptually but are very difficult to model precisely. Because of this hidden complexity, the skills most valued in a quant are those related to mathematics and computation rather than finance. It is a quant's ability to structure a complex problem that makes them valuable, not their specific knowledge of a company or market.
A quant should understand the following mathematical concepts:
- Calculus (including differential, integral, and stochastic)
- Linear algebra and differential equations
- Probability and statistics
Key financial topics include:
- Portfolio theory
- Equity and interest rate derivatives , including exotics
- Credit-risk products
Some quants will specialize in specific products, such as commodities , foreign exchange (Forex) or asset-backed securities .
Computer Competency
Software skills are also critical to job performance. C++ is typically used for high-frequency trading applications, and offline statistical analysis would be performed in MATLAB, SAS, S-PLUS or a similar package. Pricing knowledge may also be embedded in trading tools created with Java, .NET or VBA , and are often integrated with Excel. Monte Carlo techniques are essential. A majority of the work is also realized in Python, as scripting-type languages are good for running lots of data and multiple scenarios.
Education and Certifications
Most firms look for at least a master's degree or preferably a Ph.D. in a quantitative subject, such as mathematics, economics, finance, or statistics. Master's degrees in financial engineering or computational finance are also effective entry points for quant careers. Generally, an MBA is not enough by itself to obtain a quant position, unless the applicant also has a very strong mathematical or computational skill set in addition to some solid experience in the real world.
While most financial certifications, such as the Chartered Financial Analyst (CFA) designation likely wouldn't add much value to a prospective quant's resume, one that may is the Certificate in Quantitative Finance (CQF) —which you may earn globally via distance learning in a six-month intensive program.
Clearly, you need to have "the right stuff" to be a quantitative analyst. It requires both the intellectual ability to master complex and abstract mathematical domains and a willingness to tackle challenges that can seem insurmountable—all while under considerable pressure—which only a select few can do.
But that also doesn't mean that everyone who has the ability to be a quant should become one. The financial problems that quants face are very abstract and narrow. Unlike fundamental or qualitative analysts , quants don't read annual reports , meet with management, visit operations, prepare roadshows, or talk to shareholders . Most of their time is spent working with computer code and numbers on a screen.
Individuals with strong analytical skills are valuable in many different areas of finance, such as economic and financial analysis , for example. Having to compete against the best and brightest quants every single day may not be the quickest path through the ranks, especially for those with broader skills and interests and a desire to manage.
Another career issue to consider is that many Ph.D. quants who come from academic environments find they miss the research environment. Instead of being able to study a problem for several months, when supporting a trading desk you need to find solutions in days or hours. This usually precludes making any breakthroughs in the field.
Do Quants Get Paid Well?
Yes, quants tend to command high salaries, in part because they are in demand. Hedges funds and other trading firms generally offer the highest compensation. Entry-level positions may earn only $125,000 or $150,000, but there is usually room for future growth in both responsibilities and salary.
How Hard Is Quant Finance?
It take advanced-level skills in finance, math, and computer programming to get into quantitative trading , and the competition for a first job can be fierce. Once someone has landed a job, it then requires long working hours, innovation, and comfort with risk to succeed.
Do You Need a Ph.D. to Be a Quant?
Having a Ph.D. in a subject like math, finance, economics or statistics can be a definite plus for anyone wanting to become a quant. But a master's degree in computational finance or financial engineering can also be the ticket to a career as a quantitative analyst.
Success in quantitative analysis is largely based on knowledge, talent, merit, and dedication instead of the ability to sell, network, or play politics. The quants who work in the field are there because they can do the job well—an environment that many find remarkably refreshing—and they are justly rewarded for their work.
Bureau of Labor Statistics. " Financial Analysts ."
Open Quant. " The Various Types of Quants and Quant Employers ."
Bureau of Labor Statistics. " Financial Analysts: Work Environment ."
Glassdoor. " Quantitative Analyst Salaries ."
Bureau of Labor Statistics. " Financial Analysts: Pay ."
Glassdoor. " How Much Does a Quantitative Analyst Make? "
Duke University Career Center. " Quantitative Analysis ."
Bureau of Labor Statistics. " Financial Analysts: How to Become One ."
Certificate in Quantitative Finance. " Who Is It For? "
:max_bytes(150000):strip_icc():format(webp)/GettyImages-1448674736-6de8fd3502b9493887440279ce4dc25c.jpg)
- Terms of Service
- Editorial Policy
- Privacy Policy
- Your Privacy Choices
The new hottest PhDs at multi-strategy hedge funds
If you want to attract the attention of a top multi-strategy hedge fund now, it may help to have a PhD. Not a PhD in fluid mechanics like in the recent past, but a PhD in weather forecasting. Big hedge funds today love big meteorologists.
As the Financial Times reported yesterday, Citadel has assembled a team of at least 20 of weather scientists within its commodities trading business . In a world where both the weather and energy demand and supply are increasingly unpredictable, those weather scientists were critical to driving the fund's 38% returns last year. Joanna Welsh, Citadel's chief risk officer, says weather has become an important factor in Citadel's modelling of future scenarios: "We can see what might happen to supply and demand in commodities with a regular price shock, say, or with a price shock and a weather move, or with both and an infrastructure problem as well,” she told Risk Magazine in January.
If you're a meteorologist who wants to work for Citadel, where the average pay in London was $1.3m in 2020 (the last year for which figures are available), the good news is that you still have options. The hedge fund is currently looking for a PhD level weather expert to conduct "applied research into atmospheric predictability, data analysis and visualization, operational weather prediction and communication of weather signals." The resulting research will be used by the fund's natural gas, power and agricultural traders.
Only the most accomplished meteorologists need apply: Citadel specifies that applicants must have "superb educational attainment."
The meteorologists hedge funds hire
What sorts of backgrounds are popular? When Citadel built its weather team in 2018, there were (possibly apocryphal) stories of its recruiters touring the Met Office in Exeter, seeking to poach UK government weather scientists for vastly higher pay.
Citadel's weather team is run by UK-based Nicholas Klingaman, a former principal research fellow at the National Centre for Atmospheric Science in Reading. It's US weather lead is Heather M. Archambault, who joined from the National Oceanic and Atmospheric Administration in Princeton and who has a PhD from the University of Albany, where Citadel is understood to fish for much of its US meteorological talent.
Citadel isn't the only fund hitting-up meteorological talent. Balyasny has been there too. The fund hired Alexander Goldstein from Credit Suisse in March 2021 and Ann Shelly from Citadel (and previously the Met Office) in July 2022. Shelly, who lives in Cornwall and likes surfing, is a specialist in tropical weather.
One headhunter, who helps hedge funds hire meteorologists and other commodities professionals, says many funds are finding it necessary to be flexible on location. "A lot of meteorologists are outdoor junkies," he says. "They are earthy type people, and they want to be hybrid or remote."
With extreme weather events increasing, he says it's not just the big hedge funds that want meteorology PhDs. "A lot of the new hedge funds and commodities houses also want weather forecasting talent," he says. "There are huge profits to be made in areas like gas and power trading if you can predict a weather event before it happens."
How much will you earn as a PhD level meteorologist in a fund? Ross Gregory, senior partner and head of commodities at New York-City based Proco Commodities, says pay is on a par with that for senior analysts in hedge funds who work closely with portfolio managers. "You're probably looking at a $200k salary and three or four times that in bonus, depending upon performance."
Click here to create a profile on eFinancialCareers. Make yourself visible to recruiters hiring for top jobs in technology and finance.
Have a confidential story, tip, or comment you’d like to share? Contact: [email protected] in the first instance. Whatsapp/Signal/Telegram also available (Telegram: @SarahButcher)
Bear with us if you leave a comment at the bottom of this article: all our comments are moderated by human beings. Sometimes these humans might be asleep, or away from their desks, so it may take a while for your comment to appear. Eventually it will – unless it’s offensive or libelous (in which case it won’t.)
Sign up to Morning Coffee!
The essential daily roundup of news and analysis read by everyone from senior bankers and traders to new recruits.
Boost your career
"My daughter is very unhappy with her Goldman Sachs bonus"
"I worked 80 hour weeks to deliver a platform for a hedge fund. Then they fired me"
The last bank paying $600k to 23-year-olds
Goldman Sachs' potential next CEO has 5 children & a wife who's best friends with Gwyneth
Reflections of a banking MD: "20 years of 70-90 hour weeks; six million air miles"
Citigroup made another major JPMorgan hire without anyone noticing
Deutsche Bank hired a new head of credit trading from Morgan Stanley
Evercore is still hiring but it's paying slightly better now, too
Bank of Singapore fired up to 40 people for medical insurance claims
Citi’s cheap happy junior bankers in Malaga are not so grateful

Related articles
"If you're a commodities trader in a bank, leaving is a no-brainer"
- Search Menu
- Browse content in Arts and Humanities
- Browse content in Archaeology
- Anglo-Saxon and Medieval Archaeology
- Archaeological Methodology and Techniques
- Archaeology by Region
- Archaeology of Religion
- Archaeology of Trade and Exchange
- Biblical Archaeology
- Contemporary and Public Archaeology
- Environmental Archaeology
- Historical Archaeology
- History and Theory of Archaeology
- Industrial Archaeology
- Landscape Archaeology
- Mortuary Archaeology
- Prehistoric Archaeology
- Underwater Archaeology
- Urban Archaeology
- Zooarchaeology
- Browse content in Architecture
- Architectural Structure and Design
- History of Architecture
- Residential and Domestic Buildings
- Theory of Architecture
- Browse content in Art
- Art Subjects and Themes
- History of Art
- Industrial and Commercial Art
- Theory of Art
- Biographical Studies
- Byzantine Studies
- Browse content in Classical Studies
- Classical History
- Classical Philosophy
- Classical Mythology
- Classical Literature
- Classical Reception
- Classical Art and Architecture
- Classical Oratory and Rhetoric
- Greek and Roman Epigraphy
- Greek and Roman Law
- Greek and Roman Papyrology
- Greek and Roman Archaeology
- Late Antiquity
- Religion in the Ancient World
- Digital Humanities
- Browse content in History
- Colonialism and Imperialism
- Diplomatic History
- Environmental History
- Genealogy, Heraldry, Names, and Honours
- Genocide and Ethnic Cleansing
- Historical Geography
- History by Period
- History of Emotions
- History of Agriculture
- History of Education
- History of Gender and Sexuality
- Industrial History
- Intellectual History
- International History
- Labour History
- Legal and Constitutional History
- Local and Family History
- Maritime History
- Military History
- National Liberation and Post-Colonialism
- Oral History
- Political History
- Public History
- Regional and National History
- Revolutions and Rebellions
- Slavery and Abolition of Slavery
- Social and Cultural History
- Theory, Methods, and Historiography
- Urban History
- World History
- Browse content in Language Teaching and Learning
- Language Learning (Specific Skills)
- Language Teaching Theory and Methods
- Browse content in Linguistics
- Applied Linguistics
- Cognitive Linguistics
- Computational Linguistics
- Forensic Linguistics
- Grammar, Syntax and Morphology
- Historical and Diachronic Linguistics
- History of English
- Language Acquisition
- Language Evolution
- Language Reference
- Language Variation
- Language Families
- Lexicography
- Linguistic Anthropology
- Linguistic Theories
- Linguistic Typology
- Phonetics and Phonology
- Psycholinguistics
- Sociolinguistics
- Translation and Interpretation
- Writing Systems
- Browse content in Literature
- Bibliography
- Children's Literature Studies
- Literary Studies (Asian)
- Literary Studies (European)
- Literary Studies (Eco-criticism)
- Literary Studies (Romanticism)
- Literary Studies (American)
- Literary Studies (Modernism)
- Literary Studies - World
- Literary Studies (1500 to 1800)
- Literary Studies (19th Century)
- Literary Studies (20th Century onwards)
- Literary Studies (African American Literature)
- Literary Studies (British and Irish)
- Literary Studies (Early and Medieval)
- Literary Studies (Fiction, Novelists, and Prose Writers)
- Literary Studies (Gender Studies)
- Literary Studies (Graphic Novels)
- Literary Studies (History of the Book)
- Literary Studies (Plays and Playwrights)
- Literary Studies (Poetry and Poets)
- Literary Studies (Postcolonial Literature)
- Literary Studies (Queer Studies)
- Literary Studies (Science Fiction)
- Literary Studies (Travel Literature)
- Literary Studies (War Literature)
- Literary Studies (Women's Writing)
- Literary Theory and Cultural Studies
- Mythology and Folklore
- Shakespeare Studies and Criticism
- Browse content in Media Studies
- Browse content in Music
- Applied Music
- Dance and Music
- Ethics in Music
- Ethnomusicology
- Gender and Sexuality in Music
- Medicine and Music
- Music Cultures
- Music and Religion
- Music and Media
- Music and Culture
- Music Education and Pedagogy
- Music Theory and Analysis
- Musical Scores, Lyrics, and Libretti
- Musical Structures, Styles, and Techniques
- Musicology and Music History
- Performance Practice and Studies
- Race and Ethnicity in Music
- Sound Studies
- Browse content in Performing Arts
- Browse content in Philosophy
- Aesthetics and Philosophy of Art
- Epistemology
- Feminist Philosophy
- History of Western Philosophy
- Metaphysics
- Moral Philosophy
- Non-Western Philosophy
- Philosophy of Science
- Philosophy of Language
- Philosophy of Mind
- Philosophy of Perception
- Philosophy of Action
- Philosophy of Law
- Philosophy of Religion
- Philosophy of Mathematics and Logic
- Practical Ethics
- Social and Political Philosophy
- Browse content in Religion
- Biblical Studies
- Christianity
- East Asian Religions
- History of Religion
- Judaism and Jewish Studies
- Qumran Studies
- Religion and Education
- Religion and Health
- Religion and Politics
- Religion and Science
- Religion and Law
- Religion and Art, Literature, and Music
- Religious Studies
- Browse content in Society and Culture
- Cookery, Food, and Drink
- Cultural Studies
- Customs and Traditions
- Ethical Issues and Debates
- Hobbies, Games, Arts and Crafts
- Lifestyle, Home, and Garden
- Natural world, Country Life, and Pets
- Popular Beliefs and Controversial Knowledge
- Sports and Outdoor Recreation
- Technology and Society
- Travel and Holiday
- Visual Culture
- Browse content in Law
- Arbitration
- Browse content in Company and Commercial Law
- Commercial Law
- Company Law
- Browse content in Comparative Law
- Systems of Law
- Competition Law
- Browse content in Constitutional and Administrative Law
- Government Powers
- Judicial Review
- Local Government Law
- Military and Defence Law
- Parliamentary and Legislative Practice
- Construction Law
- Contract Law
- Browse content in Criminal Law
- Criminal Procedure
- Criminal Evidence Law
- Sentencing and Punishment
- Employment and Labour Law
- Environment and Energy Law
- Browse content in Financial Law
- Banking Law
- Insolvency Law
- History of Law
- Human Rights and Immigration
- Intellectual Property Law
- Browse content in International Law
- Private International Law and Conflict of Laws
- Public International Law
- IT and Communications Law
- Jurisprudence and Philosophy of Law
- Law and Politics
- Law and Society
- Browse content in Legal System and Practice
- Courts and Procedure
- Legal Skills and Practice
- Primary Sources of Law
- Regulation of Legal Profession
- Medical and Healthcare Law
- Browse content in Policing
- Criminal Investigation and Detection
- Police and Security Services
- Police Procedure and Law
- Police Regional Planning
- Browse content in Property Law
- Personal Property Law
- Study and Revision
- Terrorism and National Security Law
- Browse content in Trusts Law
- Wills and Probate or Succession
- Browse content in Medicine and Health
- Browse content in Allied Health Professions
- Arts Therapies
- Clinical Science
- Dietetics and Nutrition
- Occupational Therapy
- Operating Department Practice
- Physiotherapy
- Radiography
- Speech and Language Therapy
- Browse content in Anaesthetics
- General Anaesthesia
- Neuroanaesthesia
- Browse content in Clinical Medicine
- Acute Medicine
- Cardiovascular Medicine
- Clinical Genetics
- Clinical Pharmacology and Therapeutics
- Dermatology
- Endocrinology and Diabetes
- Gastroenterology
- Genito-urinary Medicine
- Geriatric Medicine
- Infectious Diseases
- Medical Toxicology
- Medical Oncology
- Pain Medicine
- Palliative Medicine
- Rehabilitation Medicine
- Respiratory Medicine and Pulmonology
- Rheumatology
- Sleep Medicine
- Sports and Exercise Medicine
- Clinical Neuroscience
- Community Medical Services
- Critical Care
- Emergency Medicine
- Forensic Medicine
- Haematology
- History of Medicine
- Browse content in Medical Dentistry
- Oral and Maxillofacial Surgery
- Paediatric Dentistry
- Restorative Dentistry and Orthodontics
- Surgical Dentistry
- Browse content in Medical Skills
- Clinical Skills
- Communication Skills
- Nursing Skills
- Surgical Skills
- Medical Ethics
- Medical Statistics and Methodology
- Browse content in Neurology
- Clinical Neurophysiology
- Neuropathology
- Nursing Studies
- Browse content in Obstetrics and Gynaecology
- Gynaecology
- Occupational Medicine
- Ophthalmology
- Otolaryngology (ENT)
- Browse content in Paediatrics
- Neonatology
- Browse content in Pathology
- Chemical Pathology
- Clinical Cytogenetics and Molecular Genetics
- Histopathology
- Medical Microbiology and Virology
- Patient Education and Information
- Browse content in Pharmacology
- Psychopharmacology
- Browse content in Popular Health
- Caring for Others
- Complementary and Alternative Medicine
- Self-help and Personal Development
- Browse content in Preclinical Medicine
- Cell Biology
- Molecular Biology and Genetics
- Reproduction, Growth and Development
- Primary Care
- Professional Development in Medicine
- Browse content in Psychiatry
- Addiction Medicine
- Child and Adolescent Psychiatry
- Forensic Psychiatry
- Learning Disabilities
- Old Age Psychiatry
- Psychotherapy
- Browse content in Public Health and Epidemiology
- Epidemiology
- Public Health
- Browse content in Radiology
- Clinical Radiology
- Interventional Radiology
- Nuclear Medicine
- Radiation Oncology
- Reproductive Medicine
- Browse content in Surgery
- Cardiothoracic Surgery
- Gastro-intestinal and Colorectal Surgery
- General Surgery
- Neurosurgery
- Paediatric Surgery
- Peri-operative Care
- Plastic and Reconstructive Surgery
- Surgical Oncology
- Transplant Surgery
- Trauma and Orthopaedic Surgery
- Vascular Surgery
- Browse content in Science and Mathematics
- Browse content in Biological Sciences
- Aquatic Biology
- Biochemistry
- Bioinformatics and Computational Biology
- Developmental Biology
- Ecology and Conservation
- Evolutionary Biology
- Genetics and Genomics
- Microbiology
- Molecular and Cell Biology
- Natural History
- Plant Sciences and Forestry
- Research Methods in Life Sciences
- Structural Biology
- Systems Biology
- Zoology and Animal Sciences
- Browse content in Chemistry
- Analytical Chemistry
- Computational Chemistry
- Crystallography
- Environmental Chemistry
- Industrial Chemistry
- Inorganic Chemistry
- Materials Chemistry
- Medicinal Chemistry
- Mineralogy and Gems
- Organic Chemistry
- Physical Chemistry
- Polymer Chemistry
- Study and Communication Skills in Chemistry
- Theoretical Chemistry
- Browse content in Computer Science
- Artificial Intelligence
- Computer Architecture and Logic Design
- Game Studies
- Human-Computer Interaction
- Mathematical Theory of Computation
- Programming Languages
- Software Engineering
- Systems Analysis and Design
- Virtual Reality
- Browse content in Computing
- Business Applications
- Computer Security
- Computer Games
- Computer Networking and Communications
- Digital Lifestyle
- Graphical and Digital Media Applications
- Operating Systems
- Browse content in Earth Sciences and Geography
- Atmospheric Sciences
- Environmental Geography
- Geology and the Lithosphere
- Maps and Map-making
- Meteorology and Climatology
- Oceanography and Hydrology
- Palaeontology
- Physical Geography and Topography
- Regional Geography
- Soil Science
- Urban Geography
- Browse content in Engineering and Technology
- Agriculture and Farming
- Biological Engineering
- Civil Engineering, Surveying, and Building
- Electronics and Communications Engineering
- Energy Technology
- Engineering (General)
- Environmental Science, Engineering, and Technology
- History of Engineering and Technology
- Mechanical Engineering and Materials
- Technology of Industrial Chemistry
- Transport Technology and Trades
- Browse content in Environmental Science
- Applied Ecology (Environmental Science)
- Conservation of the Environment (Environmental Science)
- Environmental Sustainability
- Environmentalist Thought and Ideology (Environmental Science)
- Management of Land and Natural Resources (Environmental Science)
- Natural Disasters (Environmental Science)
- Nuclear Issues (Environmental Science)
- Pollution and Threats to the Environment (Environmental Science)
- Social Impact of Environmental Issues (Environmental Science)
- History of Science and Technology
- Browse content in Materials Science
- Ceramics and Glasses
- Composite Materials
- Metals, Alloying, and Corrosion
- Nanotechnology
- Browse content in Mathematics
- Applied Mathematics
- Biomathematics and Statistics
- History of Mathematics
- Mathematical Education
- Mathematical Finance
- Mathematical Analysis
- Numerical and Computational Mathematics
- Probability and Statistics
- Pure Mathematics
- Browse content in Neuroscience
- Cognition and Behavioural Neuroscience
- Development of the Nervous System
- Disorders of the Nervous System
- History of Neuroscience
- Invertebrate Neurobiology
- Molecular and Cellular Systems
- Neuroendocrinology and Autonomic Nervous System
- Neuroscientific Techniques
- Sensory and Motor Systems
- Browse content in Physics
- Astronomy and Astrophysics
- Atomic, Molecular, and Optical Physics
- Biological and Medical Physics
- Classical Mechanics
- Computational Physics
- Condensed Matter Physics
- Electromagnetism, Optics, and Acoustics
- History of Physics
- Mathematical and Statistical Physics
- Measurement Science
- Nuclear Physics
- Particles and Fields
- Plasma Physics
- Quantum Physics
- Relativity and Gravitation
- Semiconductor and Mesoscopic Physics
- Browse content in Psychology
- Affective Sciences
- Clinical Psychology
- Cognitive Psychology
- Cognitive Neuroscience
- Criminal and Forensic Psychology
- Developmental Psychology
- Educational Psychology
- Evolutionary Psychology
- Health Psychology
- History and Systems in Psychology
- Music Psychology
- Neuropsychology
- Organizational Psychology
- Psychological Assessment and Testing
- Psychology of Human-Technology Interaction
- Psychology Professional Development and Training
- Research Methods in Psychology
- Social Psychology
- Browse content in Social Sciences
- Browse content in Anthropology
- Anthropology of Religion
- Human Evolution
- Medical Anthropology
- Physical Anthropology
- Regional Anthropology
- Social and Cultural Anthropology
- Theory and Practice of Anthropology
- Browse content in Business and Management
- Business Strategy
- Business Ethics
- Business History
- Business and Government
- Business and Technology
- Business and the Environment
- Comparative Management
- Corporate Governance
- Corporate Social Responsibility
- Entrepreneurship
- Health Management
- Human Resource Management
- Industrial and Employment Relations
- Industry Studies
- Information and Communication Technologies
- International Business
- Knowledge Management
- Management and Management Techniques
- Operations Management
- Organizational Theory and Behaviour
- Pensions and Pension Management
- Public and Nonprofit Management
- Strategic Management
- Supply Chain Management
- Browse content in Criminology and Criminal Justice
- Criminal Justice
- Criminology
- Forms of Crime
- International and Comparative Criminology
- Youth Violence and Juvenile Justice
- Development Studies
- Browse content in Economics
- Agricultural, Environmental, and Natural Resource Economics
- Asian Economics
- Behavioural Finance
- Behavioural Economics and Neuroeconomics
- Econometrics and Mathematical Economics
- Economic Systems
- Economic History
- Economic Methodology
- Economic Development and Growth
- Financial Markets
- Financial Institutions and Services
- General Economics and Teaching
- Health, Education, and Welfare
- History of Economic Thought
- International Economics
- Labour and Demographic Economics
- Law and Economics
- Macroeconomics and Monetary Economics
- Microeconomics
- Public Economics
- Urban, Rural, and Regional Economics
- Welfare Economics
- Browse content in Education
- Adult Education and Continuous Learning
- Care and Counselling of Students
- Early Childhood and Elementary Education
- Educational Equipment and Technology
- Educational Strategies and Policy
- Higher and Further Education
- Organization and Management of Education
- Philosophy and Theory of Education
- Schools Studies
- Secondary Education
- Teaching of a Specific Subject
- Teaching of Specific Groups and Special Educational Needs
- Teaching Skills and Techniques
- Browse content in Environment
- Applied Ecology (Social Science)
- Climate Change
- Conservation of the Environment (Social Science)
- Environmentalist Thought and Ideology (Social Science)
- Natural Disasters (Environment)
- Social Impact of Environmental Issues (Social Science)
- Browse content in Human Geography
- Cultural Geography
- Economic Geography
- Political Geography
- Browse content in Interdisciplinary Studies
- Communication Studies
- Museums, Libraries, and Information Sciences
- Browse content in Politics
- African Politics
- Asian Politics
- Chinese Politics
- Comparative Politics
- Conflict Politics
- Elections and Electoral Studies
- Environmental Politics
- European Union
- Foreign Policy
- Gender and Politics
- Human Rights and Politics
- Indian Politics
- International Relations
- International Organization (Politics)
- International Political Economy
- Irish Politics
- Latin American Politics
- Middle Eastern Politics
- Political Methodology
- Political Communication
- Political Philosophy
- Political Sociology
- Political Behaviour
- Political Economy
- Political Institutions
- Political Theory
- Politics and Law
- Public Administration
- Public Policy
- Quantitative Political Methodology
- Regional Political Studies
- Russian Politics
- Security Studies
- State and Local Government
- UK Politics
- US Politics
- Browse content in Regional and Area Studies
- African Studies
- Asian Studies
- East Asian Studies
- Japanese Studies
- Latin American Studies
- Middle Eastern Studies
- Native American Studies
- Scottish Studies
- Browse content in Research and Information
- Research Methods
- Browse content in Social Work
- Addictions and Substance Misuse
- Adoption and Fostering
- Care of the Elderly
- Child and Adolescent Social Work
- Couple and Family Social Work
- Developmental and Physical Disabilities Social Work
- Direct Practice and Clinical Social Work
- Emergency Services
- Human Behaviour and the Social Environment
- International and Global Issues in Social Work
- Mental and Behavioural Health
- Social Justice and Human Rights
- Social Policy and Advocacy
- Social Work and Crime and Justice
- Social Work Macro Practice
- Social Work Practice Settings
- Social Work Research and Evidence-based Practice
- Welfare and Benefit Systems
- Browse content in Sociology
- Childhood Studies
- Community Development
- Comparative and Historical Sociology
- Economic Sociology
- Gender and Sexuality
- Gerontology and Ageing
- Health, Illness, and Medicine
- Marriage and the Family
- Migration Studies
- Occupations, Professions, and Work
- Organizations
- Population and Demography
- Race and Ethnicity
- Social Theory
- Social Movements and Social Change
- Social Research and Statistics
- Social Stratification, Inequality, and Mobility
- Sociology of Religion
- Sociology of Education
- Sport and Leisure
- Urban and Rural Studies
- Browse content in Warfare and Defence
- Defence Strategy, Planning, and Research
- Land Forces and Warfare
- Military Administration
- Military Life and Institutions
- Naval Forces and Warfare
- Other Warfare and Defence Issues
- Peace Studies and Conflict Resolution
- Weapons and Equipment

The Oxford Handbook of Hedge Funds
Douglas Cumming, JD, PhD, CFA, is the DeSantis Distinguished Professor of Professor of Finance and Entrepreneurship at the College of Business, Florida Atlantic University in Boca Raton, Florida. Douglas is also a Visiting Professor of Finance at Birmingham Business School, University of Birmingham, UK. Douglas has published over 200 articles in leading refereed academic journals (including 38 in Financial Times top 50 journals) in finance, management, and law and economics, such as the Academy of Management Journal , Economic Journal , Journal of Business , Journal of Financial and Quantitative Analysis , Journal of Financial Economics , Review of Financial Studies , Journal of International Business Studies , and the Journal of Empirical Legal Studies . His work has been cited over 20,000 times according to Google Scholar. He is the Managing Editor-in-Chief of the Review of Corporate Finance (2021–current) and British Journal of Management (2020–current). Douglas has published 21 academic books. Douglas’ work has been reviewed in numerous media outlets, including The Economist , The New York Times , the Chicago Tribune , the Wall Street Journal , the Globe and Mail , Canadian Business , the National Post , and The New Yorker .
Sofia Johan, LLB, LLM, PhD, is an Associate Professor of Finance at the College of Business, Florida Atlantic University and a Phil Smith Fellow at The Phil Smith Center for Free Enterprise at the College of Business. Also, Sofia is a Chair in Entrepreneurial Finance at the University of Aberdeen, Scotland and an Extramural Research Fellow at Tilburg Law and Economics Center in the Netherlands. Sofia has published over 75 articles in leading journals, such as the Academy of Management Journal , Journal of Financial Economics , Journal of Financial and Quantitative Analysis , and Journal of International Business Studies , and has been cited over 4000 times. Sofia is a Coeditor of Venture Capital: An International Journal of Entrepreneurial Finance (2021), and an Associate Editor of the British Journal of Management, Emerging Markets Review (2018–), and Finance Research Letters . Sofia has published six academic books, including The Oxford Handbook of Hedge Funds (2021), Crowdfunding: Fundamental Cases , Facts, and Insights (2019), and The Oxford Handbook of IPOs (2018).
Geoffrey Wood is DanCap Private Equity Chair and Professor, and Head of DAN Management at Western University in Canada, and Visiting Professor at Trinity College, Dublin.
- Cite Icon Cite
- Permissions Icon Permissions
The Oxford Handbook of Hedge Funds provides a comprehensive look at the hedge fund industry from a global perspective. The chapters are organized into five main parts. After the introductory chapter in Part I, Part II begins in Chapter 2 with an analysis of the main factors that have affected the operation of hedge funds. Chapter 3 explains the concept of hedge fund flows. Chapter 4 examines hedge fund manager fees and contracts. Part III focuses on different types of hedge fund strategies. The broad array of strategies are summarized in Chapter 5. Chapter 6 empirically examines the performance of hedge fund strategies. Chapter 7 compares the strategies of hedge funds to private equity funds. Chapter 8 examines hedge fund herding. Chapter 9 examines hedge fund commodity trading advisors and leverage. Chapter 10 examines financial technology in hedge fund strategies. In Part IV, hedge fund activism in the US is examined in Chapter 11. The US and international literature on hedge fund activism is reviewed in different perspectives in Chapters 12 and 13. Case studies are provided in Chapter 14. The impact of activism on large company innovation is discussed in Chapter 15. In Part V, Chapter 16 examines whether hedge funds may engage in misreporting and fraud. Chapter 17 reviews work on hedge fund misconduct and detection. Chapter 18 discusses compliance among hedge funds. Chapter 19 examines theoretical approaches to hedge fund regulation. Chapter 20 examines optimal taxation. Chapter 21 examines hedge funds from a political economy context.
Signed in as
Institutional accounts.
- Google Scholar Indexing
- GoogleCrawler [DO NOT DELETE]
Personal account
- Sign in with email/username & password
- Get email alerts
- Save searches
- Purchase content
- Activate your purchase/trial code
Institutional access
- Sign in with a library card Sign in with username/password Recommend to your librarian
- Institutional account management
- Get help with access
Access to content on Oxford Academic is often provided through institutional subscriptions and purchases. If you are a member of an institution with an active account, you may be able to access content in one of the following ways:
IP based access
Typically, access is provided across an institutional network to a range of IP addresses. This authentication occurs automatically, and it is not possible to sign out of an IP authenticated account.
Sign in through your institution
Choose this option to get remote access when outside your institution. Shibboleth/Open Athens technology is used to provide single sign-on between your institution’s website and Oxford Academic.
- Click Sign in through your institution.
- Select your institution from the list provided, which will take you to your institution's website to sign in.
- When on the institution site, please use the credentials provided by your institution. Do not use an Oxford Academic personal account.
- Following successful sign in, you will be returned to Oxford Academic.
If your institution is not listed or you cannot sign in to your institution’s website, please contact your librarian or administrator.
Sign in with a library card
Enter your library card number to sign in. If you cannot sign in, please contact your librarian.
Society Members
Society member access to a journal is achieved in one of the following ways:
Sign in through society site
Many societies offer single sign-on between the society website and Oxford Academic. If you see ‘Sign in through society site’ in the sign in pane within a journal:
- Click Sign in through society site.
- When on the society site, please use the credentials provided by that society. Do not use an Oxford Academic personal account.
If you do not have a society account or have forgotten your username or password, please contact your society.
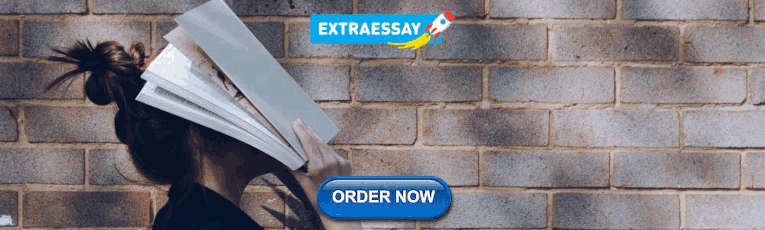
Sign in using a personal account
Some societies use Oxford Academic personal accounts to provide access to their members. See below.
A personal account can be used to get email alerts, save searches, purchase content, and activate subscriptions.
Some societies use Oxford Academic personal accounts to provide access to their members.
Viewing your signed in accounts
Click the account icon in the top right to:
- View your signed in personal account and access account management features.
- View the institutional accounts that are providing access.
Signed in but can't access content
Oxford Academic is home to a wide variety of products. The institutional subscription may not cover the content that you are trying to access. If you believe you should have access to that content, please contact your librarian.
For librarians and administrators, your personal account also provides access to institutional account management. Here you will find options to view and activate subscriptions, manage institutional settings and access options, access usage statistics, and more.
Our books are available by subscription or purchase to libraries and institutions.
- About Oxford Academic
- Publish journals with us
- University press partners
- What we publish
- New features
- Open access
- Rights and permissions
- Accessibility
- Advertising
- Media enquiries
- Oxford University Press
- Oxford Languages
- University of Oxford
Oxford University Press is a department of the University of Oxford. It furthers the University's objective of excellence in research, scholarship, and education by publishing worldwide
- Copyright © 2024 Oxford University Press
- Cookie settings
- Cookie policy
- Privacy policy
- Legal notice
This Feature Is Available To Subscribers Only
Sign In or Create an Account
This PDF is available to Subscribers Only
For full access to this pdf, sign in to an existing account, or purchase an annual subscription.
S T R E E T OF W A L L S
Important mathematical topics for quants, math topics for quant interviews.
Do you know the difference in meaning between “stochastic” and “statistic”? Does Black-Scholes (BS) stand for “Boring Stuff” in your mind? Does the thought of explaining how to calculate an R-squared value make you wish you had reviewed your econometrics textbook before arriving at your interview?
Most Hedge Funds that are looking for Quants will not expect you to have strong knowledge of finance developed yet, unless you’ve worked in the field before. Nonetheless, there are some basic concepts you should be familiar with; even if you haven’t taken a finance class; we have detailed those below. Knowing some elementary financial concepts shows an initiative to get yourself up to speed in your desired industry.
Mathematical Finance Topics
What is black-scholes (b-s) walk me through the derivation..
B-S is a mathematical formula that is used to determine the price of a European call option (or European put option) on a financial instrument. The model assumes that asset price movements follow geometric Brownian motion with constant drift and volatility. Brownian motion originally referred to the microscopic motions of a small particle floating in a liquid; if stock prices move in a similar way, then the random increments or price changes will be normally distributed with an infinitesimal variance.

The basis of Black-Scholes is Ito’s Lemma, which explains the process of stochastic behavior. If a variable x follows an Ito process ( dx = a ( x , t ) + b ( x , t ) dW ) then Ito’s Lemma shows that a function G , of x and t , follows the process:
If f is the price of a call option on stock S , and f is a function of S and t , then via Ito’s Lemma:
The above formula is key to the derivation of the B-S equation, which incorporates the constant price variation (volatility) of the stock, the time value of money, the option’s strike price and the option’s time to expiry. Black-Scholes for a non-dividend paying stock depends on the construction of a riskless portfolio, where positions are taken in bonds (cash), the underlying stock and options. If one holds –1 units (i.e., sold short one unit) of a derivative f plus df/ds shares, the change in the value of this portfolio Π over time Δt is equal to:
If the stock price can be modeled as above:
then via Ito’s Lemma 1) and 3) above:
Notice how the above equation (the change in the portfolio value) does not involve dW. During time increment Δt , the portfolio is not subject to random movement—it must earn the riskless rate. Because
using 2) and 4) in conjunction with 5) above yields:
What are the assumptions made in Black-Scholes?
- There is no arbitrage opportunity (i.e., there is no way to make a riskless profit).
- It is possible to borrow and lend cash at the same constant risk-free interest rate.
- It is possible to buy and sell stocks in any amount (including fractional amounts); there is no restraint on short selling.
- There are no transaction costs (the market is frictionless).
- Stock prices follow geometric Brownian motion with constant drift and constant volatility.
- Note: The original B-S equation assumed no dividend payments. However, dividends can be taken into account by subtracting them from the risk-free rate, assuming that both the dividend and the risk-free rate are continuously compounding.
What are the “Greeks” in Black-Scholes? What are Vanna and Volga?
The “Greeks” are the model outputs from Black-Scholes, known as Greeks due to their mathematical notation with Greek letters. They provide information about the sensitivity of outputs of the model to changes in the inputs into the model.
- Delta: Measures the rate of change in the option value with respect to changes in the underlying asset’s price (the first derivative of the option price with respect to the underlying price). It loosely equals the probability that the option finishes in-the-money. Delta for a call ranges from 0 to 1, and from -1 to 0 for puts.
- Gamma: Measures the rate of change of the option delta with respect to the change in the underlying asset price (the second derivative of the option price with respect to the underlying price). Even if the underlying asset price remains unchanged, the option delta for an in-the-money option increases as expiration nears; the opposite is true for an out-of-the-money option. The gamma of an option indicates how the delta of an option will change for a one-point move in the underlying asset. In other words, the Gamma shows the option delta’s sensitivity to market price changes. Gamma is important for maintaining a delta neutral position.
- Vega: Measures the sensitivity of the option to changes in implied volatility. It equals the first derivative of the option price with respect to the volatility of the underlying asset. Vega is typically expressed as the amount of money per underlying share that the option’s value will gain or lose as volatility rises or falls by 1%. Vega is most sensitive when the option is at-the-money and tapers off either side as the market trades above/below the strike. Some option trading strategies that are particularly Vega-sensitive are straddles, where a profit can be made when volatility increases or decreases without a move in the underlying asset. Vega falls as an option moves to become in-the-money or out-of-the money.
- Theta: Theta measures how fast the premium of an option decays with time or how much value an option’s price will diminish per day—including non-business days (all other factors being constant). Note that it is not possible to hedge the passage of time. The nearer the expiration date, the higher the Theta. Option trading strategies that are particularly Theta-sensitive include Calendar Spreads, where traders maintain a net positive Theta by buying longer-dated options and selling shorter-dated options, profiting when the underlying stock remains within a tight range.
- Rho: Rho measures the sensitivity of a stock option’s price to a change in interest rates; typically, changes in interest rates over short time periods have very little effect on options where the underlying asset is not rate sensitive (equities instead of bonds, for example). Call options rise in value when interest rates rise; the opposite is true for puts. Rho increases as time to expiration becomes longer.
What is delta hedging? Are there scenarios where it’s not profitable?
Delta hedging is the process of setting or keeping the delta of a portfolio consisting of assets and options on those assets as close to zero as possible, typically by buying or selling some amount of the asset such that the total exposure from the asset plus the option equals zero. Generally speaking, when one purchases options and delta hedges, one wants future realized volatility to be higher than the implied volatility at which the option was purchased.
When a position is delta-hedged, the daily P&L on a delta-hedged option position equals:
where “Other” includes the P&L from financing the underlying position as well as P&L due to changes in interest rates and dividend expectations.

where ΔS is the change in the underlying stock price, Δt is the fraction of time elapsed, Δσ is the change in implied volatility, and Γ , Θ , and ν refer to Gamma, Theta and Vega, respectively.
Typically, an investor who sells options (and is short gamma) and is delta hedging should make money if future realized volatility is lower than the volatility that the option was priced with (its implied volatility). So what’s going on? If implied volatility is constant and interest rates are assumed to equal zero, then
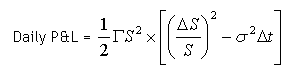
The second half of the above equation reflects the difference between an asset’s 1-day squared return (its variance) and the 1-day implied variance. When the difference between these two variances equals zero, the daily P&L equals zero as well. However, the Gamma ( Γ ) of an option does not remain constant through time or through asset price moves. Gamma reaches its maximum value (at a given time) when an option is at-the-money. (However, if overall volatility is high, gamma tends to be stable across all strike prices, as the time value of deeply in/out-of-the-money options is already quite substantial.) For a given price, Gamma increases as the time to expiry decreases.
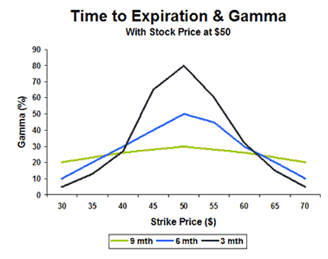
While over the lifetime of an option, a trader who is short Gamma may find that realized volatility is below the implied volatility over which the option was sold, the P&L of a delta-hedged portfolio is path-dependent.
A short option holder who is Delta hedging may be “up” in P&L during the early period of the trade, only to lose money at the very end when asset prices oscillate around the strike in the final months, causing Gamma to rise. If realized volatility remained low, higher Gamma would be a good thing, but an environment of rising volatility in tandem with higher Gamma will lead to losses. Because the daily P&L of the option position was weighted by a volatility spread that was negative (realized > implied), the final P&L “drowned,” even though the realized volatility over the entire history of the option was below its initial implied level.
What is realized volatility and how is it calculated?
Realized volatility is the historical annualized standard deviation of an asset’s log returns. It is calculated by 1) taking the standard deviation of the log returns of an asset and 2) multiplying this value by the square root of the frequency of the data.
For example, with weekly stock prices:
Frequency of data: Weekly, or 52 weeks in a year.
The volatility of the above data set = (Std Deviation of log returns) × Sqrt(52)
Note: With daily price data, it is assumed that there are 252 trading (or business) days in a year, so that the frequency adjustment would be Sqrt(252).
How is realized volatility different from implied volatility?
Realized volatility is based entirely on an asset price’s past behavior over some calculated period; implied volatility is an input into the Black-Scholes model that is based on an asset’s assumed volatility over the option period. Note that future volatility may or may not resemble past volatility.
How much should I pay for an option when the future volatility of the underlying is zero?
When the implied volatility of an asset is zero, the future price is known with certainty—there is not a distribution around a mean value. Based on the ability to replicate the payoff of an asset risklessly using cash and options, under the risk-neutral measure of Black-Scholes, the asset is expected to grow exactly at the risk-free rate for the period; one may take this future value, subtract the exercise (strike) price, and discount the future gain to the present value using the same risk-free rate.
What is a variance swap?
A variance swap is an instrument that allows investors to trade future realized (or historical) volatility against current implied volatility. Only variance—the square of volatility—can be replicated with a static hedge. The final payment = Variance Notional Amount × (Final Realized Volatility 2 – Strike Price 2 ). Unlike the delta-hedged example above, a long variance position will always benefit when realized volatility is higher than implied at inception, and conversely for a short position.
How is a forward FX rate calculated?
An exchange rate is a ratio: the price of one country’s currency expressed in another country’s currency. Unlike equities, where all equity prices worldwide can decline, this is not true for the currencies; if the dollar has depreciated against the pound, then the pound by definition will have strengthened (or appreciated) against the dollar.
A forward FX rate is an arbitrage free rate at a future point in time T that takes into account the riskless rate of return that can be earned in each country in local currency over the interval T . For example: If today’s exchange rate is 100 units of foreign currency per 1 USD, and 1-year interest rates are 10% abroad and 5% in the U.S., then one would have either $1.05 in the U.S. after one year or 110 foreign currency units after one year. In order to avoid a situation where one could earn riskless profits, the 1-year forward exchange rate will equal 110 foreign currency units per $1.05, or 104.76 units of foreign currency per 1 USD. In this case, an investor would be indifferent between borrowing in foreign currency at today’s spot rate and earning a higher interest rate abroad, since the rate at which he can contractually exchange the foreign currency for his home currency is lower by the ratio of the current interest rate differential.
What is the difference between a forward contract and a futures contract? Under what cases are the prices of the two contracts the same?
A forward contract is an agreement between two parties, in which a buyer agrees to buy a quantity of an asset at a specific price from the seller at a future date. In a forward transaction, no actual cash changes hands. Forward contracts are traded over-the-counter (OTC), i.e., not on an exchange. Both the buyer and seller are bound by the contractual terms, where the price remains fixed. Because a forward is not exchange-traded, there may be a lack of liquidity; moreover, each party is subject to non-performance by the other—either buyer or seller may fail to honor her end of the contract.
A futures contract is an agreement to buy or sell an asset at a certain time in the future (the delivery date) at a pre-set price. The future date is called the delivery date or final settlement date. The pre-set price is called the futures price. The price of the underlying asset on the delivery date is called the settlement price. The futures price converges towards the settlement price (or future spot price) on the delivery date. Terms are standardized, rather than customized. Futures are traded on organized exchanges, and use a clearing house to provide protection against non-performance to both the buyer and the seller. The price of the futures contract can change prior to delivery; in order to guarantee that neither party defaults on the obligation, both participants must settle daily price changes as per the contract values (daily margin) in addition to posting an initial margin.
There is a difference between forward and futures prices when interest rates are stochastic—in other words, when they vary unpredictably over time. If an asset is positively correlated with interest rates, then the holder of a long futures contract will profit if the asset price rises—both from the fact that the direction of the market move is positive for the long futures holder, but also because the daily margin posted from the market move can be invested at a higher rate than the prior day’s rate. When asset prices are correlated with interest rates, futures prices will typically be higher than forward prices.
What is a lognormal distribution?
A lognormal distribution is a distribution of values that are positively skewed (versus the symmetric distribution of a normal distribution). In a lognormal distribution, values may not go below zero but can have unlimited positive potential. Variables that are distributed lognormally include stock prices and (typically) interest rates.
What is a correlation coefficient? How is it calculated?
A correlation coefficient is a measure of the interdependence of two random variables and ranges in value from –1 to +1, indicating perfect negative correlation at –1, absence of correlation at zero, and perfect positive correlation at +1. It is calculated as the covariance of the two variables, divided by the square root of the product of each variable’s variance (i.e., the product of each variable’s standard deviation). Note that an equity Beta for stock x is the covariance of the stock x with the market divided by the variance of the market.
What is the difference between a permutation and combination?
If order does matter, compute occurrences using the formula for a permutation (permutation = position). If the order doesn’t matter, use the formula for a combination.
When items may only be selected once (i.e., there is no repetition) and the order of selection matters , the number of possible outcomes equals: n ! ÷ ( n – r )! where n is the number of items to choose from, and r items are chosen. This is a permutation.
When items may only be selected once (i.e., there is no repetition) and the order of selection doesn’t matter, the number of possible outcomes equals: n ! ÷ [ r ! × ( n – r )!] where n is the number of items to choose from, and r items are chosen. This combination is typically written using the following shorthand: n C r . Combinations are symmetric, so choosing 3 balls out of 16 or choosing 13 balls out of 16 results in the same number of combinations.
What is the probability that I flip this penny 5 times, it will come up heads at least 2 times?
The trick here is the phrase “at least 2.” This means: what is the probability that of 5 flips, I will end up with 2 or 3 or 4 or 5 heads? Think of this as the inverse to the problem: what is the probability that of 5 flips, I see a head only once or no times at all?
The probability out of 5 flips of getting 0 heads:
= (probability of getting 5 tails) = (0.5) 5 = 3.125%
The probability out of 5 flips of getting 1 head:
=(probability of getting 4 tails) × (probability of getting 1 head) × 5 C 1 = (0.5) 4 × (0.5) 1 × 5 C 1 = (0.5) 4 × (0.5) 1 × 5! ÷ (1! × 4!) = 15.625%
The probability of getting 0 or 1 heads in 5 flips = 3.125% + 15.625% = 18.750%.
The probability of getting 2 or more heads in 5 flips = 1 – 18.75% = 81.25%.
What is a Monte Carlo simulation?
A Monte Carlo simulation is a computerized mathematical procedure for sampling random outcomes for a given process. It provides a range of possible outcomes (and their associated probabilities) rather than a discrete point estimate of a given outcome. Monte Carlo simulation performs risk analysis by building models of possible results by substituting a range of values—a probability distribution —for any factor that has inherent uncertainty. It calculates results over and over, each time using a different set of random values chosen from the probability functions. During a Monte Carlo simulation, values are sampled at random from the input probability distributions. Each set of results from that sample is recorded; the values comprise a probability distribution of possible outcomes. Depending upon the number of uncertainties and the ranges specified for them, a Monte Carlo simulation could involve thousands or tens of thousands of recalculations.
Monte Carlo simulation provides a number of advantages over deterministic, or “single-point estimate” analysis:
1) Probabilistic Results. Results show not only what could happen, but also how likely each outcome is.
2) Sensitivity Analysis. In a Monte Carlo simulation, it’s easy to see which inputs had the biggest effect on line results. In deterministic models, it’s difficult to model different combinations of values for different inputs to see the effects of truly different scenarios.
3) Correlation of Inputs. In a Monte Carlo simulation, it’s possible to model relationships among input variables.
Monty Hall problem: Assume that I tell you that a prize is behind one of three doors. If you pick a door (say Door #2), and I tell you that the prize is not behind another door (Door #3, for example), and I give you the option of remaining with your first pick (Door #2) versus switching to Door #1, should you switch? Why?
This question has been asked for decades; the answer is much simpler than many think. The probability that a prize is behind Door 1 is 1/3; the probability that the prize is behind Door 2 is 1/3; and similarly, the probability that the prize is behind Door 3 is 1/3.
Before any additional information is given, the key is that the probability that the prize is behind Door 1 OR Door 3 is 1/3 + 1/3 = 2/3. If I then tell you that the prize is NOT behind Door 3, then the probability that the prize is behind Door 1 is now 2/3 (since I’ve just told you that there is a 0% probability that the prize is behind Door 3).
If the probability that the prize is behind Door 2 is 1/3, but the probability that the prize is behind Door 1 is now 2/3 (with the new information that the prize is NOT behind Door 3), then you should switch your choice of doors to Door 1; switching from Door 2 to Door 1 doubles your chance of winning (raises the probability from 1/3 to 2/3).
Birthday problem: What’s the probability that in a room full of k people, at least 2 people will have the same birthday?
Each person has 365 “possible” birthdays (ignoring leap years); in a room of k people, the total number of possible birthdays is 365 k .
In order for at least 2 people to have the same birthday, this means that 2 or 3 or 4….have the same birthday, which is the “inverse” or complement of none of the k birthdays in the group being the same. This latter event is a permutation; if John is born on January 1 and Jeff is born on December 31, this is a different outcome than John being born on December 31 and Jeff being born on January 1 (i.e., position matters.) The total number of ways to choose k different birthdays from 365 elements (with no repetitions) is 365! ÷ (365 – k )!. So for a room full of k people, the probability that at least 2 have the same birthday is:
1 – [365! ÷ (365 – k )!] ÷ 365 k
When k = 15, the probability is 25.3%; when k = 50, the probability rises to 97.0%!
What is Bayes’ Theorem and when is it used?
Bayes’ theorem gives the relationship between the probabilities of Event A and Event B, and the conditional probability of Event A occurring given the occurrence of Event B, written P (A|B).
For example, assume a drug test is correct 99% of the time, meaning that when someone has used the drug, they test positive for the drug 99% of the time; when someone hasn’t used the drug, they test negative 99% of the time. If 2% of the population uses the drug, what is the probability that someone who has tested positive actually does use the drug?
Prob(drug user) = 2% Prob(test positive | drug user) = 99% Prob(test positive | not drug user) = 1%
So we have:
Prob(drug user |test positive) = [Prob(test positive |drug user) × Prob(drug user)] ÷ {[Prob(test positive |drug user) × Prob(drug user)] + [Prob (test positive |not drug user) × Prob(not drug user)]}
Prob(drug user |test positive) = [99% × 2%] / [(99% × 2%) + (1% × 98%)] = 66.9%
So for a positive test, there’s only a 2/3 chance that the test-taker is a drug user.
Econometrics
What is an r 2 statistic.
An R 2 statistic is a measure of goodness-of-fit, also known as the coefficient of determination. It is the proportion of variability in a data set that is accounted for by the chosen model. In a simple linear regression ( y = mx + b ), the R 2 statistic is the percentage of variability in ‘ y ’ that can be explained by movements in ‘ x .’ Note that in a linear regression, R 2 equals the square of the sample correlation coefficient between the outcomes ( y ) and their predicted values, and can be thought of as a percentage from 1 to 100.
What is a random walk? Is it stationary or non-stationary?
A random walk is a path that consists of taking successive random steps. Often, the “steps” taken by a stock price are assumed to follow a random walk. Random walks are not stationary, i.e., where you “are” in the walk depends on where you were immediately prior to your last step. Non-stationary behaviors can include trends, cycles, random walks or combinations of the three. Note that while non-stationary data cannot be modeled or forecasted, the data can usually be transformed so that they can be modeled.
In a pure random walk ( Y t = Y t -1 + ε t ), the value or location at time t will be equal to the last period value plus a stochastic (non-systematic) component that is a white noise, which means ε t is independent and identically distributed with mean of 0 and variance equal to σ². The random walk is a non-mean reverting process that can move away from the mean either in a positive or negative direction. Put another way, the means, variances and co-variances of the walk change over time. Another characteristic of a random walk is that the variance evolves over time and goes to infinity as time goes to infinity; therefore, a random walk cannot be predicted.
What happens if you create a regression based on 2 variables that each are continuously increasing with time?
If you attempt to model two series that are both time-dependent (say, consumption and income), you will get a spurious regression, i.e., a model with a high R 2 , but poor predictive properties. This is because both series are non-stationary. With non-stationary variables, one needs to transform them into stationary series; the easiest way to do this is by differencing, or looking at changes in the series. Changes in a non-stationary series are usually stationary.
What to do if two series are non-stationary: Rather than differencing each series, one may be able to create a better model by finding a cointegrating relationship. With cointegration, the aim is to detect any common stochastic trends in the underlying data; whereas the two series may not be stationary, the difference between two non-stationary series may itself, be stationary.
If I have a regression between x and y, what test statistics should I look at to determine whether I have a good model?
- R 2 : See above. Explains how much of the movement in the independent variable can be explained by the dependent variables chosen.
- t-Statistic : In a least squares regression, the t -statistic is the estimated regression coefficient of a given independent variable divided by its standard error. If the t -statistic is more than 2 (i.e., the coefficient is at least twice as large as the standard error), one can conclude that the variable in question has a significant impact on the dependent variable.
- H 0 : All non-constant coefficients in the regression equation are zero (i.e., the model has no explanatory power).
- H a : At least one of the non-constant coefficients in the regression equation is non-zero.
The F -test tests the joint explanatory power of the variables, while the t tests test their explanatory power individually. One rejects the null hypothesis when the F statistic is greater than its critical value.
- Durbin-Watson statistic: the Durbin–Watson ( d ) statistic measures the presence of autocorrelation in the residuals. The value of d always lies between 0 and 4. A value of 2 indicates no autocorrelation. If the Durbin–Watson statistic is substantially less than 2, there is evidence of positive serial correlation.
- Akaike Information Criterion: From among a set of models, the Akaike Information Criterion (AIC) suggests the preferred model as the one with the minimum AIC value. The AIC = 2 k – 2 × ln( L ) where k is the number of parameters in the statistical model, and L is the maximized value of the likelihood function for the estimated model. The AIC rewards goodness of fit, but also includes a penalty that is an increasing function of the number of estimated parameters, which discourages overfitting.
If I have data from 1912-2012, what’s the danger if I build a model forecasting values in 2012 using all of the historical data?
Fitting a model with all available data is called in-sample testing. A model can be constructed that may perform exceptionally well during a selected period of history, but structural changes over time may mean that a model that worked well in the past may not work well in the future. Rather than back-testing a model using in-sample data, create a model that uses some historical data and test how well the model works when applied to data that wasn’t used to construct the model. While back-testing can provide valuable information regarding a model’s potential, back-testing alone often produces deceptive results.
Why should I care about residuals in a regression?
If a plot of residuals versus time does not look like white noise, the model is likely misspecified. Correlation of residuals is known as autocorrelation, and can be checked by calculating the Durbin-Watson statistic (above). With autocorrelation of residuals, the estimated regression coefficients are still unbiased but may no longer have the minimum variance among all unbiased estimates (they are inefficient); moreover, confidence intervals may be underestimated and estimation of the test statistics for the F-test (and so significance) may also be underestimated, potentially leading to the conclusion that the set of explanatory variables is not significant as a whole.
Funding Sources
An offer of admission to the Ph.D. program includes an offer of financial support from the Department of Mathematics. Applicants need not complete any special form in order to be considered for financial support.
First Year Students
Admitted students are offered a departmental fellowship for their first year, so that they have no teaching assistant duties then.
Continuing Graduate Students
Continuing graduate students in the Department of Mathematics are generally awarded Teaching Assistantships (TAs) or in some cases Research Assistantships (RAs), though a few fellowships also exist. Financial support is renewed for students making satisfactory progress towards their degrees, up to a total of five years, with the type of award varying.
Financial Support
The department provides full financial support -- including tuition, individual medical insurance, and monthly stipend -- for up to five years, assuming students are making satisfactory progress toward the degree. For students with outside awards such as external fellowships, the department will supplement such awards to bring funding up to the level of our standard package. While students are guaranteed full support during the 9-month academic year, the level of summer support varies, depending on how much of the summer students remain at MIT and the level that they choose to work during that time.
For more detailed information regarding the cost of attendance, including specific costs for tuition and fees, books and supplies, housing and food as well as transportation, please visit the SFS website .
International Students
Because of visa requirements of the Department of Homeland Security, international students with full TA or RA appointments are not eligible to pursue any additional work during the regular semester. International students are eligible to work on campus (not off-campus) during MIT's Independent Activity Period and the summer term. International students who wish to pursue employment off-campus must apply for Optional Practical Training or Academic Training ; see the International Students Office for more details.
Students are discouraged from accepting additional employment during the fall or spring semester if it would involve more than 3-4 hours per week beyond their TA and RA duties. Employment opportunities both inside and outside the department may be available during the summer.
More information on summer support may be found on the Summer Support Page .
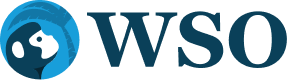
- Recently Active
- Top Discussions
- Best Content
By Industry
- Investment Banking
- Private Equity
- Hedge Funds
- Real Estate
- Venture Capital
- Asset Management
- Equity Research
- Investing, Markets Forum
- Business School
- Fashion Advice
- Hedge Fund Forum HF
Hedge Fund Mathematics

- Share on Facebook
- Share on Twitter
- Share on LinkedIn
- Share via Email
I'm currently working in ER but would like to move to HF as I feel that I have more interest in PM rather than research.
As some of my colleagues has commented and I have searched on WSO and find that various quant skills are a plus for HF. Coming from a Physics major, I have no problem with algebra or calculus. However, what "specific" mathematics are important to say a long/short equity or a market neutral HF, eg. hypothesis testing? normal distribution? probability? Regression? Non-linear equations or calculus?
Hedge Fund Math
The type of math you will regularly use, in Hedge Funds, depends heavily on what kind of fund you are dealing with.
User @DeepLearning - Hedge Fund - Equity Hedge - Quant agrees:
Depends on the type of hedge fund. Machine Learning, Statistics, Time Series, Markov Models are all used heavily. Also just straight up linear regressions with regularization, coefficient constraints, etc. is used heavily. For quantitative hedge funds, good, useful and clean data is usually more powerful than having a more sophisticated model. While quantitative hedge funds do use a good amount of advanced mathematics, they spend probably more effort in data collection/cleaning. If you are using a unique and useful data but only performing basic regression techniques, you are probably going to get better performance than a fund that is using non-unique (but still useful) data but using sophisticated models. Many PhD mathematicians are surprised to find that they spend most of their time applying undergraduate mathematics in creative ways rather than using the full extent of their graduate math knowledge.
Read More About Starting A Career In Hedge Funds
- Hedge Fund Careers: Getting A Hedge Fund Job Out Of Undergrad And Beyond
- To Hedge Fund Or Not To Hedge Fund
- Private Equity Vs Hedge Funds: 4 Key Differences
Looking to Break into the Hedge Fund World?
Want to land at an elite hedge fund use our HF Interview Prep Course which includes 814 questions across 165 hedge funds. The WSO Hedge Fund Interview Prep Course has everything you’ll ever need to land the most coveted jobs on the buyside.
Hedge Fund Interview Course

Probability, stochastic process, ordinary and partial diff equations, analysis. Etcetcetc. You want to be well versed in all aspects of math, maybe get a masters in financial math or financial engineering its up to you

YourWorstEnemy: Probability, stochastic process, ordinary and partial diff equations, analysis. Etcetcetc. You want to be well versed in all aspects of math, maybe get a masters in financial math or financial engineering its up to you
Why awp thinks this is hilarious? Was it a joke and these things are not actually needed?

Long/short isn't too quant, probably not even going into stochastics. As a physics major, you should be fine, just brush up on prob/stats.

Thanks guys for the input! Really appreciate the help!

Wouldn't this really depend on the strategy of the fund? I'm guessing most value investing funds for example simply rely on financial analysis, which is basic algebra at best. Renaissance technologies on the other hand probably has some serious mathematicians up in there.

Nyctola: Wouldn't this really depend on the strategy of the fund? I'm guessing most value investing funds for example simply rely on financial analysis, which is basic algebra at best. Renaissance technologies on the other hand probably has some serious mathematicians up in there.
You need to be able to solve 48÷2(9+3).

Quant in Hedge Fund ( Originally Posted: 09/15/2010 )
People always say that Hedge Fund likes people who's good at math. I'm wondering what do you do in HF that makes you really need to be comfortable in math? What are some jobs in HF involved a lot of math but doesn't require a PHD?

Capital markets are full of data which attracts mathematicians. Specific mathematic areas that are popular in finance include:
Statistical Inference: what is the strength of the relationship that I am observing? Bayesian Probability: given a set of priors, what is the likelihood of X happening? Signal Processing Linear Algebra and Regressions: Financial models are essentially multi-factor models. Optimization: Portfolio construction and risk management
Financial Engineering is a huge academic and professional movement with nearly unlimited applications in financial markets . While having a PhD is a plus, there are certainly many, many jobs where a facility with math is beneficial/essential.
Let me know if you want more specifics.

for a real answer to your question: basically none
I disagree. Lots of MSFE from Columbia and NYU are scattered all over NYC hedge funds. These are two years programs.
I dont believe you Kevin, your profile is not on the page anymore! I only believe Daniel and Ryan now. tzz

I’m breaking my head trying to decide my major: Is pure math the best option to prepare for working for hedge funds? ( Originally Posted: 10/12/2017 )
Or is economics /finance/accounting better? Is pure math a useless degree as many people say? Or is it a very good one to work in Wall Street as other say? IM VEEEERY CONFUSED! Please help me

I think coupling pure math with econ /finance and possibly computer science minor is your best bet, or going somewhere with undergraduate financial engineering if you 're SURE that's what you want to do.

https://www.quantnet.com/mfe-programs-rankings/

Math/ CS with finance/accounting electives is ideal.

Mathematics for hedge funds ( Originally Posted: 03/20/2018 )
What kind of mathematics/statistics Phds are hedge funds most interested about? Stochastic calculus, PDE, Machine learning, Stochastic control, Time series, financial econometrics etc ?
Sit autem est sapiente earum omnis quia molestiae. Quisquam enim sed beatae cum accusamus.
Corrupti quae neque molestiae earum perferendis culpa voluptas. Et esse dolorum similique. Aut eum sed asperiores.
See All Comments - 100% Free
WSO depends on everyone being able to pitch in when they know something. Unlock with your email and get bonus: 6 financial modeling lessons free ($199 value)
or Unlock with your social account...
Want to Vote on this Content?! No WSO Credits?
Already a member? Login
Trending Content
Career Resources
- Financial Modeling Resources
- Excel Resources
- Download Templates Library
- Salaries by Industry
- Investment Banking Interview Prep
- Private Equity Interview Prep
- Hedge Fund Interview Prep
- Consulting Case Interview Prep
- Resume Reviews by Professionals
- Mock Interviews with Pros
- WSO Company Database
WSO Virtual Bootcamps
- Apr 27 Foundations Bootcamp 10:00AM EDT
- May 04 Venture Capital Bootcamp 10:00AM EDT
- May 11 Financial Modeling & Valuation Bootcamp May 11 - 12 10:00AM EDT
- May 18 Investment Banking Interview Bootcamp 10:00AM EDT
- Jun 01 Private Equity Interview Bootcamp 10:00AM EDT
Career Advancement Opportunities
April 2024 Hedge Fund
Overall Employee Satisfaction
Professional Growth Opportunities
Total Avg Compensation
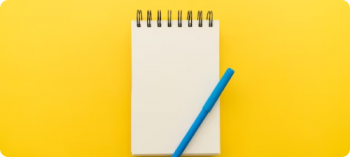
“... there’s no excuse to not take advantage of the resources out there available to you. Best value for your $ are the...”
Leaderboard
- Silver Banana
- Banana Points
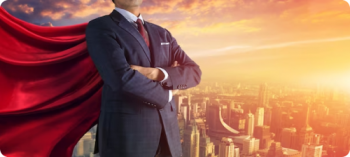
“... I believe it was the single biggest reason why I ended up with an offer...”
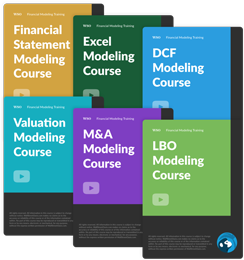
Get instant access to lessons taught by experienced private equity pros and bulge bracket investment bankers including financial statement modeling, DCF, M&A, LBO, Comps and Excel Modeling.
or Want to Sign up with your social account?
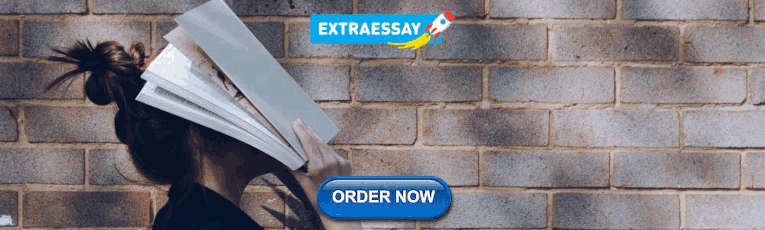
IMAGES
VIDEO
COMMENTS
Doctoral students. Jeff Cheeger. James Harris Simons (born 25 April 1938) is an American hedge fund manager, investor, mathematician, and philanthropist. [3] He is the founder of Renaissance Technologies, a quantitative hedge fund based in East Setauket, New York. He and his fund are known to be quantitative investors, using mathematical models ...
Some of the best quant funds make extensive use of even more advanced graduate level mathematics in the realms of algebraic geometry, number theory and information theory. Hence anything highly mathematically, statistically or physically oriented is likely to be of interest to a top quant hedge fund.
Ever heard of a guy who turned math into $30,700,000,000 on Wall Street? Meet Jim Simons, the brain behind Renaissance Technologies, a financial magic shop that's been making waves since 1982.
Renaissance Technologies LLC, also known as RenTech or RenTec, is an American hedge fund based in East Setauket, New York, on Long Island, which specializes in systematic trading using quantitative models derived from mathematical and statistical analysis.Their signature Medallion fund is famed for the best record in investing history. Renaissance was founded in 1982 by James Simons, a ...
In fact, some banks offer entry-level quants with PhDs from top universities base salaries as high as $125k and hedge funds offer up to $175k base salary. Exceptional entry-level PhD quants can receive total compensation packages, including sign-on bonuses, worth up to $400k, according to recruiting firm Options Group.
Transition Pure Math PhD to Hedge Fund. Pure Math PhD to HF. Monkey 40 O. Subscribe. Hello everyone, I'm a pure math PhD student in my first summer of the program. I have no interest in academia and would like to make the transition to working at a HF when I graduate. Obviously quant related jobs would be the most straight forward transition ...
Blend your math, finance, and computer skills to command the high salary of a quantitative analyst. ... They tend to work in investment banks and for hedge funds, although insurance companies ...
As demand for quants to work in banks and hedge funds increased in recent years, so the number of Masters courses in quantitative finance proliferated wildly. A year into the COVID pandemic, however, it seems the quant Masters boom may be turning into the quant masters bust. Headhunters in London and New York say they are awash in applications from juniors with masters and bachelors degrees ...
Junior Quantitative Trader. GTS. New York, NY 10022. ( Midtown area) 5 Av/53 St. $125,000 - $225,000 a year. Full-time. 2+ years of experience working on a prop trading, quantitative trading or electronic trading desk (investment bank, hedge fund, etc.). Posted 11 days ago ·.
Something that I've read is that in regards to linear models, anyone can create them but a lot of hedge funds hire PhDs in math/ statistics/ physics because they are able to quickly and accurately decide which variables will be the most significant. 3. Reply. proverbialbunny.
Renaissance's flagship Medallion Fund has been the best performing hedge fund in the world for the past 20 years. One of the key creative minds at Renaissance, Robert Frey, Stony Brook Applied Mathematics PhD 1986, returned to Stony Brook in 2005 after early retirement at Renaissance to develop a Quantitative Finance program in the Stony Brook ...
you can do pure math phd as long as you do it at elite institute. One way to get hired is to get academic position at elite institute after your phd and work for a few years. That credentials alone would land you at lucrative quant position. 6. Reply.
The best performing hedge funds in the world, like renaissance technologies, hire mathematicians and scientists over finance degrees, and will throw out resumes if they ever worked on Wall Street. Reply reply ... Math PhD isn't smth unique, there are plenty of unemployed Math PhD holders, it gives zero value and zero prestige -- people look for ...
The new hottest PhDs at multi-strategy hedge funds. If you want to attract the attention of a top multi-strategy hedge fund now, it may help to have a PhD. Not a PhD in fluid mechanics like in the recent past, but a PhD in weather forecasting. Big hedge funds today love big meteorologists. As the Financial Times reported yesterday, Citadel has ...
Abstract. The Oxford Handbook of Hedge Funds provides a comprehensive look at the hedge fund industry from a global perspective. The chapters are organized into five main parts. After the introductory chapter in Part I, Part II begins in Chapter 2 with an analysis of the main factors that have affected the operation of hedge funds.
Only variance—the square of volatility—can be replicated with a static hedge. The final payment = Variance Notional Amount × (Final Realized Volatility 2 - Strike Price 2 ). Unlike the delta-hedged example above, a long variance position will always benefit when realized volatility is higher than implied at inception, and conversely for ...
Financial Support. The department provides full financial support -- including tuition, individual medical insurance, and monthly stipend -- for up to five years, assuming students are making satisfactory progress toward the degree. For students with outside awards such as external fellowships, the department will supplement such awards to ...
You may very well deal with more applied math in an Econ PhD than you might in a theoretical Math PhD. Sorry Blue. If you don't like math, you're not going to have much fun getting a PhD that will get you a job at a bank or hedge fund. That said, I hate unapplied math, but I find it really fun when talking about the economy, computer algorithms ...
Hedge Fund Math. The type of math you will regularly use, in Hedge Funds, depends heavily on what kind of fund you are dealing with. User @DeepLearning - Hedge Fund - Equity Hedge - Quant agrees: ... What are some jobs in HF involved a lot of math but doesn't require a PHD? Reply. Quote. Report. Other . KevinNYC. Senior Orangutan. 417 HF. 12y.