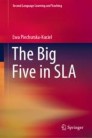
The Big Five in SLA pp 1–25 Cite as
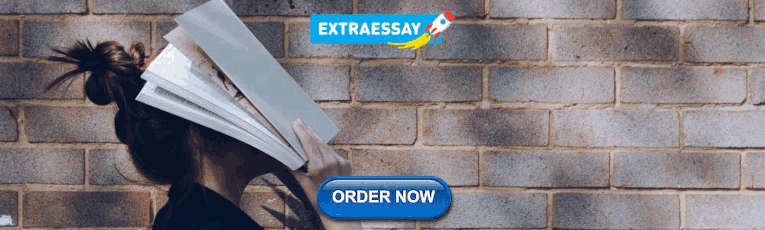
Personality: Definitions, Approaches and Theories
- Ewa Piechurska-Kuciel ORCID: orcid.org/0000-0002-6690-231X 3
- First Online: 04 November 2020
2191 Accesses
2 Citations
Part of the book series: Second Language Learning and Teaching ((SLLT))
The main objective of this chapter is to describe the concept of personality and approaches to researching it. For this reason, first a view on outlining the field of personality psychology in its present form, then the key term—personality—is discussed. The next section contains a synopsis of the main approaches to the study of personality, including psychoanalytic, learning and humanistic perspectives. The objective of the second part is to present the main theoretical directions in personality studies, which are divided into two basic trends. The first one is represented by type theories that focus on qualitative differences and discrete categories. The other direction is composed of trait theories that aim to formulate the latent structure of personality on the basis of statistical procedures, this has led to the development of the trait model adopted as the groundwork of this volume—the Big Five. The last section of this chapter is devoted to a general description of the most important theories exploring the development of personality across a lifespan (psychosexual, psychosocial, cognitive, and social cognitive).
This is a preview of subscription content, log in via an institution .
Buying options
- Available as PDF
- Read on any device
- Instant download
- Own it forever
- Available as EPUB and PDF
- Compact, lightweight edition
- Dispatched in 3 to 5 business days
- Free shipping worldwide - see info
- Durable hardcover edition
Tax calculation will be finalised at checkout
Purchases are for personal use only
Author information
Authors and affiliations.
Institute of Linguistics, Opole University, Opole, Opolskie, Poland
Ewa Piechurska-Kuciel
You can also search for this author in PubMed Google Scholar
Rights and permissions
Reprints and permissions
Copyright information
© 2020 The Editor(s) (if applicable) and The Author(s), under exclusive license to Springer Nature Switzerland AG
About this chapter
Cite this chapter.
Piechurska-Kuciel, E. (2020). Personality: Definitions, Approaches and Theories. In: The Big Five in SLA. Second Language Learning and Teaching. Springer, Cham. https://doi.org/10.1007/978-3-030-59324-7_1
Download citation
DOI : https://doi.org/10.1007/978-3-030-59324-7_1
Published : 04 November 2020
Publisher Name : Springer, Cham
Print ISBN : 978-3-030-59323-0
Online ISBN : 978-3-030-59324-7
eBook Packages : Education Education (R0)
Share this chapter
Anyone you share the following link with will be able to read this content:
Sorry, a shareable link is not currently available for this article.
Provided by the Springer Nature SharedIt content-sharing initiative
- Publish with us
Policies and ethics
- Find a journal
- Track your research
To read this content please select one of the options below:
Please note you do not have access to teaching notes, personality research in the 21st century: new developments and directions for the field.
Journal of Management History
ISSN : 1751-1348
Article publication date: 19 August 2022
Issue publication date: 6 April 2023
The purpose of this study is to systematically examine and classify the multitude of personality traits that have emerged in the literature beyond the Big Five (Five Factor Model) since the turn of the 21st century. The authors argue that this represents a new phase of personality research that is characterized both by construct proliferation and a movement away from the Big Five and demonstrates how personality as a construct has substantially evolved in the 21st century.
Design/methodology/approach
The authors conducted a comprehensive, systematic review of personality research from 2000 to 2020 across 17 management and psychology journals. This search yielded 1,901 articles, of which 440 were relevant and subsequently coded for this review.
The review presented in this study uncovers 155 traits, beyond the Big Five, that have been explored, which the authors organize and analyze into 10 distinct categories. Each category comprises a definition, lists the included traits and highlights an exemplar construct. The authors also specify the significant research outcomes associated with each trait category.
Originality/value
This review categorizes the 155 personality traits that have emerged in the management and psychology literature that describe personality beyond the Big Five. Based on these findings, this study proposes new avenues for future research and offers insights into the future of the field as the concept of personality has shifted in the 21st century.
- Personality
- Systematic literature review
Medina-Craven, M.N. , Ostermeier, K. , Sigdyal, P. and McLarty, B.D. (2023), "Personality research in the 21st century: new developments and directions for the field", Journal of Management History , Vol. 29 No. 2, pp. 276-304. https://doi.org/10.1108/JMH-06-2022-0021
Emerald Publishing Limited
Copyright © 2022, Emerald Publishing Limited
Related articles
We’re listening — tell us what you think, something didn’t work….
Report bugs here
All feedback is valuable
Please share your general feedback
Join us on our journey
Platform update page.
Visit emeraldpublishing.com/platformupdate to discover the latest news and updates
Questions & More Information
Answers to the most commonly asked questions here
Thank you for visiting nature.com. You are using a browser version with limited support for CSS. To obtain the best experience, we recommend you use a more up to date browser (or turn off compatibility mode in Internet Explorer). In the meantime, to ensure continued support, we are displaying the site without styles and JavaScript.
- View all journals
- My Account Login
- Explore content
- About the journal
- Publish with us
- Sign up for alerts
- Open access
- Published: 22 May 2020
Assessing the Big Five personality traits using real-life static facial images
- Alexander Kachur ORCID: orcid.org/0000-0003-1165-2672 1 ,
- Evgeny Osin ORCID: orcid.org/0000-0003-3330-5647 2 ,
- Denis Davydov ORCID: orcid.org/0000-0003-3747-7403 3 ,
- Konstantin Shutilov 4 &
- Alexey Novokshonov 4
Scientific Reports volume 10 , Article number: 8487 ( 2020 ) Cite this article
122k Accesses
41 Citations
347 Altmetric
Metrics details
- Computer science
- Human behaviour
There is ample evidence that morphological and social cues in a human face provide signals of human personality and behaviour. Previous studies have discovered associations between the features of artificial composite facial images and attributions of personality traits by human experts. We present new findings demonstrating the statistically significant prediction of a wider set of personality features (all the Big Five personality traits) for both men and women using real-life static facial images. Volunteer participants (N = 12,447) provided their face photographs (31,367 images) and completed a self-report measure of the Big Five traits. We trained a cascade of artificial neural networks (ANNs) on a large labelled dataset to predict self-reported Big Five scores. The highest correlations between observed and predicted personality scores were found for conscientiousness (0.360 for men and 0.335 for women) and the mean effect size was 0.243, exceeding the results obtained in prior studies using ‘selfies’. The findings strongly support the possibility of predicting multidimensional personality profiles from static facial images using ANNs trained on large labelled datasets. Future research could investigate the relative contribution of morphological features of the face and other characteristics of facial images to predicting personality.
Similar content being viewed by others
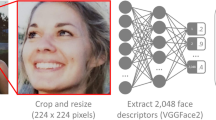
Facial recognition technology can expose political orientation from naturalistic facial images
Michal Kosinski
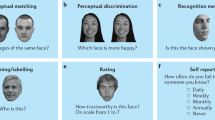
Individual differences and the multidimensional nature of face perception
David White & A. Mike Burton
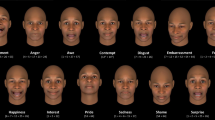
Professional actors demonstrate variability, not stereotypical expressions, when portraying emotional states in photographs
Tuan Le Mau, Katie Hoemann, … Lisa Feldman Barrett
Introduction
A growing number of studies have linked facial images to personality. It has been established that humans are able to perceive certain personality traits from each other’s faces with some degree of accuracy 1 , 2 , 3 , 4 . In addition to emotional expressions and other nonverbal behaviours conveying information about one’s psychological processes through the face, research has found that valid inferences about personality characteristics can even be made based on static images of the face with a neutral expression 5 , 6 , 7 . These findings suggest that people may use signals from each other’s faces to adjust the ways they communicate, depending on the emotional reactions and perceived personality of the interlocutor. Such signals must be fairly informative and sufficiently repetitive for recipients to take advantage of the information being conveyed 8 .
Studies focusing on the objective characteristics of human faces have found some associations between facial morphology and personality features. For instance, facial symmetry predicts extraversion 9 . Another widely studied indicator is the facial width to height ratio (fWHR), which has been linked to various traits, such as achievement striving 10 , deception 11 , dominance 12 , aggressiveness 13 , 14 , 15 , 16 , and risk-taking 17 . The fWHR can be detected with high reliability irrespective of facial hair. The accuracy of fWHR-based judgements suggests that the human perceptual system may have evolved to be sensitive to static facial features, such as the relative face width 18 .
There are several theoretical reasons to expect associations between facial images and personality. First, genetic background contributes to both face and personality. Genetic correlates of craniofacial characteristics have been discovered both in clinical contexts 19 , 20 and in non-clinical populations 21 . In addition to shaping the face, genes also play a role in the development of various personality traits, such as risky behaviour 22 , 23 , 24 , and the contribution of genes to some traits exceeds the contribution of environmental factors 25 . For the Big Five traits, heritability coefficients reflecting the proportion of variance that can be attributed to genetic factors typically lie in the 0.30–0.60 range 26 , 27 . From an evolutionary perspective, these associations can be expected to have emerged by means of sexual selection. Recent studies have argued that some static facial features, such as the supraorbital region, may have evolved as a means of social communication 28 and that facial attractiveness signalling valuable personality characteristics is associated with mating success 29 .
Second, there is some evidence showing that pre- and postnatal hormones affect both facial shape and personality. For instance, the face is a visible indicator of the levels of sex hormones, such as testosterone and oestrogen, which affect the formation of skull bones and the fWHR 30 , 31 , 32 . Given that prenatal and postnatal sex hormone levels do influence behaviour, facial features may correlate with hormonally driven personality characteristics, such as aggressiveness 33 , competitiveness, and dominance, at least for men 34 , 35 . Thus, in addition to genes, the associations of facial features with behavioural tendencies may also be explained by androgens and potentially other hormones affecting both face and behaviour.
Third, the perception of one’s facial features by oneself and by others influences one’s subsequent behaviour and personality 36 . Just as the perceived ‘cleverness’ of an individual may lead to higher educational attainment 37 , prejudice associated with the shape of one’s face may lead to the development of maladaptive personality characteristics (i.e., the ‘Quasimodo complex’ 38 ). The associations between appearance and personality over the lifespan have been explored in longitudinal observational studies, providing evidence of ‘self-fulfilling prophecy’-type and ‘self-defeating prophecy’-type effects 39 .
Fourth and finally, some personality traits are associated with habitual patterns of emotionally expressive behaviour. Habitual emotional expressions may shape the static features of the face, leading to the formation of wrinkles and/or the development of facial muscles.
Existing studies have revealed the links between objective facial picture cues and general personality traits based on the Five-Factor Model or the Big Five (BF) model of personality 40 . However, a quick glance at the sizes of the effects found in these studies (summarized in Table 1 ) reveals much controversy. The results appear to be inconsistent across studies and hardly replicable 41 . These inconsistencies may result from the use of small samples of stimulus faces, as well as from the vast differences in methodologies. Stronger effect sizes are typically found in studies using composite facial images derived from groups of individuals with high and low scores on each of the Big Five dimensions 6 , 7 , 8 . Naturally, the task of identifying traits using artificial images comprised of contrasting pairs with all other individual features eliminated or held constant appears to be relatively easy. This is in contrast to realistic situations, where faces of individuals reflect a full range of continuous personality characteristics embedded in a variety of individual facial features.
Studies relying on photographic images of individual faces, either artificially manipulated 2 , 42 or realistic, tend to yield more modest effects. It appears that studies using realistic photographs made in controlled conditions (neutral expression, looking straight at the camera, consistent posture, lighting, and distance to the camera, no glasses, no jewellery, no make-up, etc.) produce stronger effects than studies using ‘selfies’ 25 . Unfortunately, differences in the methodologies make it hard to hypothesize whether the diversity of these findings is explained by variance in image quality, image background, or the prediction models used.
Research into the links between facial picture cues and personality traits faces several challenges. First, the number of specific facial features is very large, and some of them are hard to quantify. Second, the effects of isolated facial features are generally weak and only become statistically noticeable in large samples. Third, the associations between objective facial features and personality traits might be interactive and nonlinear. Finally, studies using real-life photographs confront an additional challenge in that the very characteristics of the images (e.g., the angle of the head, facial expression, makeup, hairstyle, facial hair style, etc.) are based on the subjects’ choices, which are potentially influenced by personality; after all, one of the principal reasons why people make and share their photographs is to signal to others what kind of person they are. The task of isolating the contribution of each variable out of the multitude of these individual variables appears to be hardly feasible. Instead, recent studies in the field have tended to rely on a holistic approach, investigating the subjective perception of personality based on integral facial images.
The holistic approach aims to mimic the mechanisms of human perception of the face and the ways in which people make judgements about each other’s personality. This approach is supported by studies of human face perception, showing that faces are perceived and encoded in a holistic manner by the human brain 43 , 44 , 45 , 46 . Put differently, when people identify others, they consider individual facial features (such as a person’s eyes, nose, and mouth) in concert as a single entity rather than as independent pieces of information 47 , 48 , 49 , 50 . Similar to facial identification, personality judgements involve the extraction of invariant facial markers associated with relatively stable characteristics of an individual’s behaviour. Existing evidence suggests that various social judgements might be based on a common visual representational system involving the holistic processing of visual information 51 , 52 . Thus, even though the associations between isolated facial features and personality characteristics sought by ancient physiognomists have emerged to be weak, contradictory or even non-existent, the holistic approach to understanding the face-personality links appears to be more promising.
An additional challenge faced by studies seeking to reveal the face-personality links is constituted by the inconsistency of the evaluations of personality traits by human raters. As a result, a fairly large number of human raters is required to obtain reliable estimates of personality traits for each photograph. In contrast, recent attempts at using machine learning algorithms have suggested that artificial intelligence may outperform individual human raters. For instance, S. Hu and colleagues 40 used the composite partial least squares component approach to analyse dense 3D facial images obtained in controlled conditions and found significant associations with personality traits (stronger for men than for women).
A similar approach can be implemented using advanced machine learning algorithms, such as artificial neural networks (ANNs), which can extract and process significant features in a holistic manner. The recent applications of ANNs to the analysis of human faces, body postures, and behaviours with the purpose of inferring apparent personality traits 53 , 54 indicate that this approach leads to a higher accuracy of prediction compared to individual human raters. The main difficulty of the ANN approach is the need for large labelled training datasets that are difficult to obtain in laboratory settings. However, ANNs do not require high-quality photographs taken in controlled conditions and can potentially be trained using real-life photographs provided that the dataset is large enough. The interpretation of findings in such studies needs to acknowledge that a real-life photograph, especially one chosen by a study participant, can be viewed as a holistic behavioural act, which may potentially contain other cues to the subjects’ personality in addition to static facial features (e.g., lighting, hairstyle, head angle, picture quality, etc.).
The purpose of the current study was to investigate the associations of facial picture cues with self-reported Big Five personality traits by training a cascade of ANNs to predict personality traits from static facial images. The general hypothesis is that a real-life photograph contains cues about personality that can be extracted using machine learning. Due to the vast diversity of findings concerning the prediction accuracy of different traits across previous studies, we did not set a priori hypotheses about differences in prediction accuracy across traits.
Prediction accuracy
We used data from the test dataset containing predicted scores for 3,137 images associated with 1,245 individuals. To determine whether the variance in the predicted scores was associated with differences across images or across individuals, we calculated the intraclass correlation coefficients (ICCs) presented in Table 2 . The between-individual proportion of variance in the predicted scores ranged from 79 to 88% for different traits, indicating a general consistency of predicted scores for different photographs of the same individual. We derived the individual scores used in all subsequent analyses as the simple averages of the predicted scores for all images provided by each participant.
The correlation coefficients between the self-report test scores and the scores predicted by the ANN ranged from 0.14 to 0.36. The associations were strongest for conscientiousness and weakest for openness. Extraversion and neuroticism were significantly better predicted for women than for men (based on the z test). We also compared the prediction accuracy within each gender using Steiger’s test for dependent sample correlation coefficients. For men, conscientiousness was predicted more accurately than the other four traits (the differences among the latter were not statistically significant). For women, conscientiousness was predicted more accurately, and openness was predicted less accurately compared to the three other traits.
The mean absolute error (MAE) of prediction ranged between 0.89 and 1.04 standard deviations. We did not find any associations between the number of photographs and prediction error.
Trait intercorrelations
The structure of the correlations between the scales was generally similar for the observed test scores and the predicted values, but some coefficients differed significantly (based on the z test) (see Table 3 ). Most notably, predicted openness was more strongly associated with conscientiousness (negatively) and extraversion (positively), whereas its association with agreeableness was negative rather than positive. The associations of predicted agreeableness with conscientiousness and neuroticism were stronger than those between the respective observed scores. In women, predicted neuroticism demonstrated a stronger inverse association with conscientiousness and a stronger positive association with openness. In men, predicted neuroticism was less strongly associated with extraversion than its observed counterpart.
To illustrate the findings, we created composite images using Abrosoft FantaMorph 5 by averaging the uploaded images across contrast groups of 100 individuals with the highest and the lowest test scores on each trait. The resulting morphed images in which individual features are eliminated are presented in Fig. 1 .
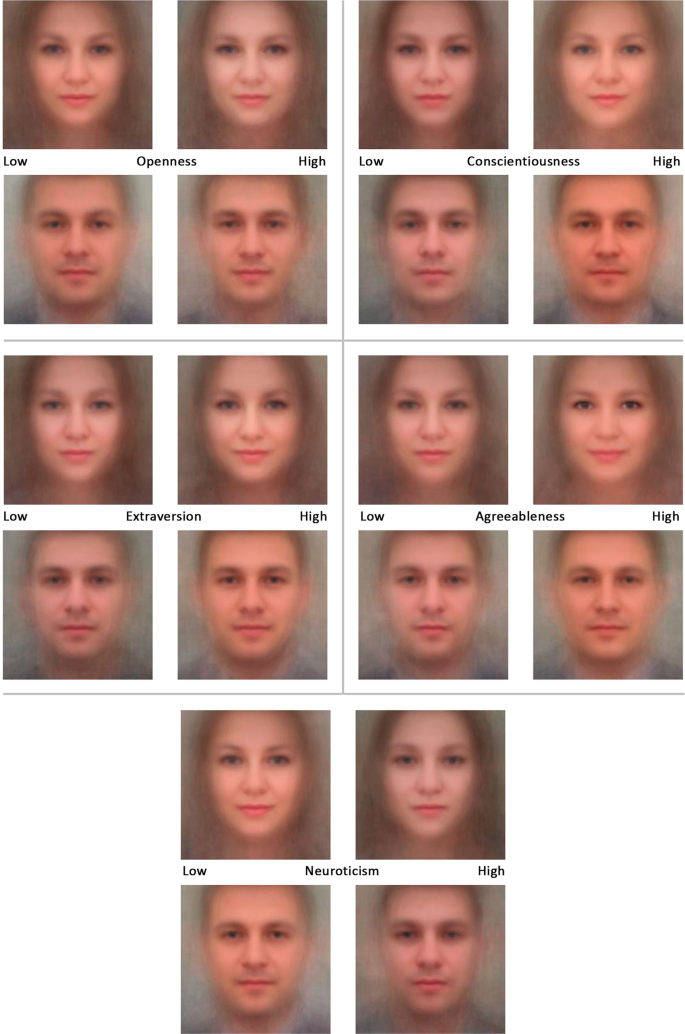
Composite facial images morphed across contrast groups of 100 individuals for each Big Five trait.
This study presents new evidence confirming that human personality is related to individual facial appearance. We expected that machine learning (in our case, artificial neural networks) could reveal multidimensional personality profiles based on static morphological facial features. We circumvented the reliability limitations of human raters by developing a neural network and training it on a large dataset labelled with self-reported Big Five traits.
We expected that personality traits would be reflected in the whole facial image rather than in its isolated features. Based on this expectation, we developed a novel two-tier machine learning algorithm to encode the invariant facial features as a vector in a 128-dimensional space that was used to predict the BF traits by means of a multilayer perceptron. Although studies using real-life photographs do not require strict experimental conditions, we had to undertake a series of additional organizational and technological steps to ensure consistent facial image characteristics and quality.
Our results demonstrate that real-life photographs taken in uncontrolled conditions can be used to predict personality traits using complex computer vision algorithms. This finding is in contrast to previous studies that mostly relied on high-quality facial images taken in controlled settings. The accuracy of prediction that we obtained exceeds that in the findings of prior studies that used realistic individual photographs taken in uncontrolled conditions (e.g., selfies 55 ). The advantage of our methodology is that it is relatively simple (e.g., it does not rely on 3D scanners or 3D facial landmark maps) and can be easily implemented using a desktop computer with a stock graphics accelerator.
In the present study, conscientiousness emerged to be more easily recognizable than the other four traits, which is consistent with some of the existing findings 7 , 40 . The weaker effects for extraversion and neuroticism found in our sample may be because these traits are associated with positive and negative emotional experiences, whereas we only aimed to use images with neutral or close to neutral emotional expressions. Finally, this appears to be the first study to achieve a significant prediction of openness to experience. Predictions of personality based on female faces appeared to be more reliable than those for male faces in our sample, in contrast to some previous studies 40 .
The BF factors are known to be non-orthogonal, and we paid attention to their intercorrelations in our study 56 , 57 . Various models have attempted to explain the BF using higher-order dimensions, such as stability and plasticity 58 or a single general factor of personality (GFP) 59 . We discovered that the intercorrelations of predicted factors tend to be stronger than the intercorrelations of self-report questionnaire scales used to train the model. This finding suggests a potential biological basis of GFP. However, the stronger intercorrelations of the predicted scores can be explained by consistent differences in picture quality (just as the correlations between the self-report scales can be explained by social desirability effects and other varieties of response bias 60 ). Clearly, additional research is needed to understand the context of this finding.
We believe that the present study, which did not involve any subjective human raters, constitutes solid evidence that all the Big Five traits are associated with facial cues that can be extracted using machine learning algorithms. However, despite having taken reasonable organizational and technical steps to exclude the potential confounds and focus on static facial features, we are still unable to claim that morphological features of the face explain all the personality-related image variance captured by the ANNs. Rather, we propose to see facial photographs taken by subjects themselves as complex behavioural acts that can be evaluated holistically and that may contain various other subtle personality cues in addition to static facial features.
The correlations reported above with a mean r = 0.243 can be viewed as modest; indeed, facial image-based personality assessment can hardly replace traditional personality measures. However, this effect size indicates that an ANN can make a correct guess about the relative standing of two randomly chosen individuals on a personality dimension in 58% of cases (as opposed to the 50% expected by chance) 61 . The effect sizes we observed are comparable with the meta-analytic estimates of correlations between self-reported and observer ratings of personality traits: the associations range from 0.30 to 0.49 when one’s personality is rated by close relatives or colleagues, but only from −0.01 to 0.29 when rated by strangers 62 . Thus, an artificial neural network relying on static facial images outperforms an average human rater who meets the target in person without any prior acquaintance. Given that partner personality and match between two personalities predict friendship formation 63 , long-term relationship satisfaction 64 , and the outcomes of dyadic interaction in unstructured settings 65 , the aid of artificial intelligence in making partner choices could help individuals to achieve more satisfying interaction outcomes.
There are a vast number of potential applications to be explored. The recognition of personality from real-life photos can be applied in a wide range of scenarios, complementing the traditional approaches to personality assessment in settings where speed is more important than accuracy. Applications may include suggesting best-fitting products or services to customers, proposing to individuals a best match in dyadic interaction settings (such as business negotiations, online teaching, etc.) or personalizing the human-computer interaction. Given that the practical value of any selection method is proportional to the number of decisions made and the size and variability of the pool of potential choices 66 , we believe that the applied potential of this technology can be easily revealed at a large scale, given its speed and low cost. Because the reliability and validity of self-report personality measures is not perfect, prediction could be further improved by supplementing these measures with peer ratings and objective behavioural indicators of personality traits.
The fact that conscientiousness was predicted better than the other traits for both men and women emerges as an interesting finding. From an evolutionary perspective, one would expect the traits most relevant for cooperation (conscientiousness and agreeableness) and social interaction (certain facets of extraversion and neuroticism, such as sociability, dominance, or hostility) to be reflected more readily in the human face. The results are generally in line with this idea, but they need to be replicated and extended by incorporating trait facets in future studies to provide support for this hypothesis.
Finally, although we tried to control the potential sources of confounds and errors by instructing the participants and by screening the photographs (based on angles, facial expressions, makeup, etc.), the present study is not without limitations. First, the real-life photographs we used could still carry a variety of subtle cues, such as makeup, angle, light facial expressions, and information related to all the other choices people make when they take and share their own photographs. These additional cues could say something about their personality, and the effects of all these variables are inseparable from those of static facial features, making it hard to draw any fundamental conclusions from the findings. However, studies using real-life photographs may have higher ecological validity compared to laboratory studies; our results are more likely to generalize to real-life situations where users of various services are asked to share self-pictures of their choice.
Another limitation pertains to a geographically bounded sample of individuals; our participants were mostly Caucasian and represented one cultural and age group (Russian-speaking adults). Future studies could replicate the effects using populations representing a more diverse variety of ethnic, cultural, and age groups. Studies relying on other sources of personality data (e.g., peer ratings or expert ratings), as well as wider sets of personality traits, could complement and extend the present findings.
Sample and procedure
The study was carried out in the Russian language. The participants were anonymous volunteers recruited through social network advertisements. They did not receive any financial remuneration but were provided with a free report on their Big Five personality traits. The data were collected online using a dedicated research website and a mobile application. The participants provided their informed consent, completed the questionnaires, reported their age and gender and were asked to upload their photographs. They were instructed to take or upload several photographs of their face looking directly at the camera with enough lighting, a neutral facial expression and no other people in the picture and without makeup.
Our goal was to obtain an out-of-sample validation dataset of 616 respondents of each gender to achieve 80% power for a minimum effect we considered to be of practical significance ( r = 0.10 at p < 0.05), requiring a total of 6,160 participants of each gender in the combined dataset comprising the training and validation datasets. However, we aimed to gather more data because we expected that some online respondents might provide low-quality or non-genuine photographs and/or invalid questionnaire responses.
The initial sample included 25,202 participants who completed the questionnaire and uploaded a total of 77,346 photographs. The final combined dataset comprised 12,447 valid questionnaires and 31,367 associated photographs after the data screening procedures (below). The participants ranged in age from 18 to 60 (59.4% women, M = 27.61, SD = 12.73, and 40.6% men, M = 32.60, SD = 11.85). The dataset was split randomly into a training dataset (90%) and a test dataset (10%) used to validate the prediction model. The validation dataset included the responses of 505 men who provided 1224 facial images and 740 women who provided 1913 images. Due to the sexually dimorphic nature of facial features and certain personality traits (particularly extraversion 1 , 67 , 68 ), all the predictive models were trained and validated separately for male and female faces.
Ethical approval
The research was carried out in accordance with the Declaration of Helsinki. The study protocol was approved by the Research Ethics Committee of the Open University for the Humanities and Economics. We obtained the participants’ informed consent to use their data and photographs for research purposes and to publish generalized findings. The morphed group average images presented in the paper do not allow the identification of individuals. No information or images that could lead to the identification of study participants have been published.
Data screening
We excluded incomplete questionnaires (N = 3,035) and used indices of response consistency to screen out random responders 69 . To detect systematic careless responses, we used the modal response category count, maximum longstring (maximum number of identical responses given in sequence by participant), and inter-item standard deviation for each questionnaire. At this stage, we screened out the answers of individuals with zero standard deviations (N = 329) and a maximum longstring above 10 (N = 1,416). To detect random responses, we calculated the following person-fit indices: the person-total response profile correlation, the consistency of response profiles for the first and the second half of the questionnaire, the consistency of response profiles obtained based on equivalent groups of items, the number of polytomous Guttman errors, and the intraclass correlation of item responses within facets.
Next, we conducted a simulation by generating random sets of integers in the 1–5 range based on a normal distribution (µ = 3, σ = 1) and on the uniform distribution and calculating the same person-fit indices. For each distribution, we generated a training dataset and a test dataset, each comprised of 1,000 simulated responses and 1,000 real responses drawn randomly from the sample. Next, we ran a logistic regression model using simulated vs real responses as the outcome variable and chose an optimal cutoff point to minimize the misclassification error (using the R package optcutoff). The sensitivity value was 0.991 for the uniform distribution and 0.960 for the normal distribution, and the specificity values were 0.923 and 0.980, respectively. Finally, we applied the trained model to the full dataset and identified observations predicted as likely to be simulated based on either distribution (N = 1,618). The remaining sample of responses (N = 18,804) was used in the subsequent analyses.
Big Five measure
We used a modified Russian version of the 5PFQ questionnaire 70 , which is a 75-item measure of the Big Five model, with 15 items per trait grouped into five three-item facets. To confirm the structural validity of the questionnaire, we tested an exploratory structural equation (ESEM) model with target rotation in Mplus 8.2. The items were treated as ordered categorical variables using the WLSMV estimator, and facet variance was modelled by introducing correlated uniqueness values for the items comprising each facet.
The theoretical model showed a good fit to the data (χ 2 = 147854.68, df = 2335, p < 0.001; CFI = 0.931; RMSEA = 0.040 [90% CI: 0.040, 0.041]; SRMR = 0.024). All the items showed statistically significant loadings on their theoretically expected scales (λ ranged from 0.14 to 0.87, M = 0.51, SD = 0.17), and the absolute cross-loadings were reasonably low (M = 0.11, SD = 0.11). The distributions of the resulting scales were approximately normal (with skewness and kurtosis values within the [−1; 1] range). To assess the reliability of the scales, we calculated two internal consistency indices, namely, robust omega (using the R package coefficientalpha) and algebraic greatest lower bound (GLB) reliability (using the R package psych) 71 (see Table 4 ).
Image screening and pre-processing
The images (photographs and video frames) were subjected to a three-step screening procedure aimed at removing fake and low-quality images. First, images with no human faces or with more than one human face were detected by our computer vision (CV) algorithms and automatically removed. Second, celebrity images were identified and removed by means of a dedicated neural network trained on a celebrity photo dataset (CelebFaces Attributes Dataset (CelebA), N > 200,000) 72 that was additionally enriched with pictures of Russian celebrities. The model showed a 98.4% detection accuracy. Third, we performed a manual moderation of the remaining images to remove images with partially covered faces, those that were evidently photoshopped or any other fake images not detected by CV.
The images retained for subsequent processing were converted to single-channel 8-bit greyscale format using the OpenCV framework (opencv.org). Head position (pitch, yaw, roll) was measured using our own dedicated neural network (multilayer perceptron) trained on a sample of 8 000 images labelled by our team. The mean absolute error achieved on the test sample of 800 images was 2.78° for roll, 1.67° for pitch, and 2.34° for yaw. We used the head position data to retain the images with yaw and roll within the −30° to 30° range and pitch within the −15° to 15° range.
Next, we assessed emotional neutrality using the Microsoft Cognitive Services API on the Azure platform (score range: 0 to 1) and used 0.50 as a threshold criterion to remove emotionally expressive images. Finally, we applied the face and eye detection, alignment, resize, and crop functions available within the Dlib (dlib.net) open-source toolkit to arrive at a set of standardized 224 × 224 pixel images with eye pupils aligned to a standard position with an accuracy of 1 px. Images with low resolution that contained less than 60 pixels between the eyes, were excluded in the process.
The final photoset comprised 41,835 images. After the screened questionnaire responses and images were joined, we obtained a set of 12,447 valid Big Five questionnaires associated with 31,367 validated images (an average of 2.59 images per person for women and 2.42 for men).
Neural network architecture
First, we developed a computer vision neural network (NNCV) aiming to determine the invariant features of static facial images that distinguish one face from another but remain constant across different images of the same person. We aimed to choose a neural network architecture with a good feature space and resource-efficient learning, considering the limited hardware available to our research team. We chose a residual network architecture based on ResNet 73 (see Fig. 2 ).
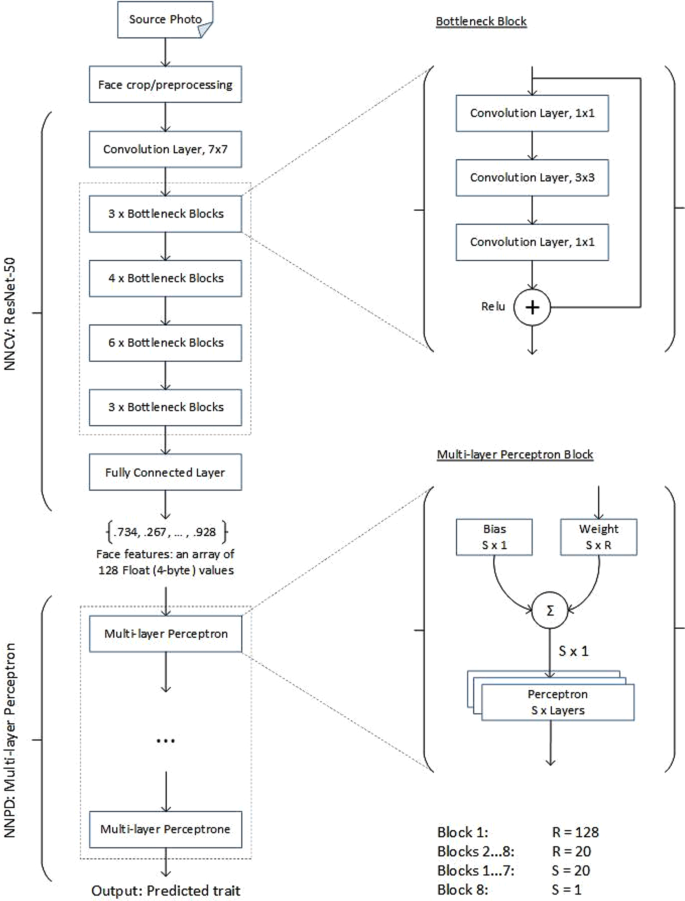
Layer architecture of the computer vision neural network (NNCV) and the personality diagnostics neural network (NNPD).
This type of neural network was originally developed for image classification. We dropped the final layer from the original architecture and obtained a NNCV that takes a static monochrome image (224 × 224 pixels in size) and generates a vector of 128 32-bit dimensions describing unique facial features in the source image. As a measure of success, we calculated the Euclidean distance between the vectors generated from different images.
Using Internet search engines, we collected a training dataset of approximately 2 million openly available unlabelled real-life photos taken in uncontrolled conditions stratified by race, age and gender (using search engine queries such as ‘face photo’, ‘face pictures’, etc.). The training was conducted on a server equipped with four NVidia Titan accelerators. The trained neural network was validated on a dataset of 40,000 images belonging to 800 people, which was an out-of-sample part of the original dataset. The Euclidean distance threshold for the vectors belonging to the same person was 0.40 after the training was complete.
Finally, we trained a personality diagnostics neural network (NNPD), which was implemented as a multilayer perceptron (see Fig. 2 ). For that purpose, we used a training dataset (90% of the final sample) containing the questionnaire scores of 11,202 respondents and a total of 28,230 associated photographs. The NNPD takes the vector of the invariants obtained from NNCV as an input and predicts the Big Five personality traits as the output. The network was trained using the same hardware, and the training process took 9 days. The whole process was performed for male and female faces separately.
Data availability
The set of photographs is not made available because we did not solicit the consent of the study participants to publish the individual photographs. The test dataset with the observed and predicted Big Five scores is available from the openICPSR repository: https://doi.org/10.3886/E109082V1 .
Kramer, R. S. S., King, J. E. & Ward, R. Identifying personality from the static, nonexpressive face in humans and chimpanzees: Evidence of a shared system for signaling personality. Evol. Hum. Behav . https://doi.org/10.1016/j.evolhumbehav.2010.10.005 (2011).
Walker, M. & Vetter, T. Changing the personality of a face: Perceived big two and big five personality factors modeled in real photographs. J. Pers. Soc. Psychol. 110 , 609–624 (2016).
Article Google Scholar
Naumann, L. P., Vazire, S., Rentfrow, P. J. & Gosling, S. D. Personality Judgments Based on Physical Appearance. Personal. Soc. Psychol. Bull. 35 , 1661–1671 (2009).
Borkenau, P., Brecke, S., Möttig, C. & Paelecke, M. Extraversion is accurately perceived after a 50-ms exposure to a face. J. Res. Pers. 43 , 703–706 (2009).
Shevlin, M., Walker, S., Davies, M. N. O., Banyard, P. & Lewis, C. A. Can you judge a book by its cover? Evidence of self-stranger agreement on personality at zero acquaintance. Pers. Individ. Dif . https://doi.org/10.1016/S0191-8869(02)00356-2 (2003).
Penton-Voak, I. S., Pound, N., Little, A. C. & Perrett, D. I. Personality Judgments from Natural and Composite Facial Images: More Evidence For A “Kernel Of Truth” In Social Perception. Soc. Cogn. 24 , 607–640 (2006).
Little, A. C. & Perrett, D. I. Using composite images to assess accuracy in personality attribution to faces. Br. J. Psychol. 98 , 111–126 (2007).
Kramer, R. S. S. & Ward, R. Internal Facial Features are Signals of Personality and Health. Q. J. Exp. Psychol. 63 , 2273–2287 (2010).
Pound, N., Penton-Voak, I. S. & Brown, W. M. Facial symmetry is positively associated with self-reported extraversion. Pers. Individ. Dif. 43 , 1572–1582 (2007).
Lewis, G. J., Lefevre, C. E. & Bates, T. Facial width-to-height ratio predicts achievement drive in US presidents. Pers. Individ. Dif. 52 , 855–857 (2012).
Haselhuhn, M. P. & Wong, E. M. Bad to the bone: facial structure predicts unethical behaviour. Proc. R. Soc. B Biol. Sci. 279 , 571 LP–576 (2012).
Valentine, K. A., Li, N. P., Penke, L. & Perrett, D. I. Judging a Man by the Width of His Face: The Role of Facial Ratios and Dominance in Mate Choice at Speed-Dating Events. Psychol. Sci . 25 , (2014).
Carre, J. M. & McCormick, C. M. In your face: facial metrics predict aggressive behaviour in the laboratory and in varsity and professional hockey players. Proc. R. Soc. B Biol. Sci. 275 , 2651–2656 (2008).
Carré, J. M., McCormick, C. M. & Mondloch, C. J. Facial structure is a reliable cue of aggressive behavior: Research report. Psychol. Sci . https://doi.org/10.1111/j.1467-9280.2009.02423.x (2009).
Haselhuhn, M. P., Ormiston, M. E. & Wong, E. M. Men’s Facial Width-to-Height Ratio Predicts Aggression: A Meta-Analysis. PLoS One 10 , e0122637 (2015).
Lefevre, C. E., Etchells, P. J., Howell, E. C., Clark, A. P. & Penton-Voak, I. S. Facial width-to-height ratio predicts self-reported dominance and aggression in males and females, but a measure of masculinity does not. Biol. Lett . 10 , (2014).
Welker, K. M., Goetz, S. M. M. & Carré, J. M. Perceived and experimentally manipulated status moderates the relationship between facial structure and risk-taking. Evol. Hum. Behav . https://doi.org/10.1016/j.evolhumbehav.2015.03.006 (2015).
Geniole, S. N. & McCormick, C. M. Facing our ancestors: judgements of aggression are consistent and related to the facial width-to-height ratio in men irrespective of beards. Evol. Hum. Behav. 36 , 279–285 (2015).
Valentine, M. et al . Computer-Aided Recognition of Facial Attributes for Fetal Alcohol Spectrum Disorders. Pediatrics 140 , (2017).
Ferry, Q. et al . Diagnostically relevant facial gestalt information from ordinary photos. Elife 1–22 https://doi.org/10.7554/eLife.02020.001 (2014).
Claes, P. et al . Modeling 3D Facial Shape from DNA. PLoS Genet. 10 , e1004224 (2014).
Carpenter, J. P., Garcia, J. R. & Lum, J. K. Dopamine receptor genes predict risk preferences, time preferences, and related economic choices. J. Risk Uncertain. 42 , 233–261 (2011).
Dreber, A. et al . The 7R polymorphism in the dopamine receptor D4 gene (<em>DRD4</em>) is associated with financial risk taking in men. Evol. Hum. Behav. 30 , 85–92 (2009).
Bouchard, T. J. et al . Sources of human psychological differences: the Minnesota Study of Twins Reared Apart. Science (80-.). 250 , 223 LP–228 (1990).
Article ADS Google Scholar
Livesley, W. J., Jang, K. L. & Vernon, P. A. Phenotypic and genetic structure of traits delineating personality disorder. Arch. Gen. Psychiatry https://doi.org/10.1001/archpsyc.55.10.941 (1998).
Bouchard, T. J. & Loehlin, J. C. Genes, evolution, and personality. Behavior Genetics https://doi.org/10.1023/A:1012294324713 (2001).
Vukasović, T. & Bratko, D. Heritability of personality: A meta-analysis of behavior genetic studies. Psychol. Bull. 141 , 769–785 (2015).
Godinho, R. M., Spikins, P. & O’Higgins, P. Supraorbital morphology and social dynamics in human evolution. Nat. Ecol. Evol . https://doi.org/10.1038/s41559-018-0528-0 (2018).
Rhodes, G., Simmons, L. W. & Peters, M. Attractiveness and sexual behavior: Does attractiveness enhance mating success? Evol. Hum. Behav . https://doi.org/10.1016/j.evolhumbehav.2004.08.014 (2005).
Lefevre, C. E., Lewis, G. J., Perrett, D. I. & Penke, L. Telling facial metrics: Facial width is associated with testosterone levels in men. Evol. Hum. Behav. 34 , 273–279 (2013).
Whitehouse, A. J. O. et al . Prenatal testosterone exposure is related to sexually dimorphic facial morphology in adulthood. Proceedings. Biol. Sci. 282 , 20151351 (2015).
Penton-Voak, I. S. & Chen, J. Y. High salivary testosterone is linked to masculine male facial appearance in humans. Evol. Hum. Behav . https://doi.org/10.1016/j.evolhumbehav.2004.04.003 (2004).
Carré, J. M. & Archer, J. Testosterone and human behavior: the role of individual and contextual variables. Curr. Opin. Psychol. 19 , 149–153 (2018).
Swaddle, J. P. & Reierson, G. W. Testosterone increases perceived dominance but not attractiveness in human males. Proc. R. Soc. B Biol. Sci . https://doi.org/10.1098/rspb.2002.2165 (2002).
Eisenegger, C., Kumsta, R., Naef, M., Gromoll, J. & Heinrichs, M. Testosterone and androgen receptor gene polymorphism are associated with confidence and competitiveness in men. Horm. Behav. 92 , 93–102 (2017).
Article CAS Google Scholar
Kaplan, H. B. Social Psychology of Self-Referent Behavior . https://doi.org/10.1007/978-1-4899-2233-5 . (Springer US, 1986).
Rosenthal, R. & Jacobson, L. Pygmalion in the classroom. Urban Rev . https://doi.org/10.1007/BF02322211 (1968).
Masters, F. W. & Greaves, D. C. The Quasimodo complex. Br. J. Plast. Surg . 204–210 (1967).
Zebrowitz, L. A., Collins, M. A. & Dutta, R. The Relationship between Appearance and Personality Across the Life Span. Personal. Soc. Psychol. Bull. 24 , 736–749 (1998).
Hu, S. et al . Signatures of personality on dense 3D facial images. Sci. Rep. 7 , 73 (2017).
Kosinski, M. Facial Width-to-Height Ratio Does Not Predict Self-Reported Behavioral Tendencies. Psychol. Sci. 28 , 1675–1682 (2017).
Walker, M., Schönborn, S., Greifeneder, R. & Vetter, T. The basel face database: A validated set of photographs reflecting systematic differences in big two and big five personality dimensions. PLoS One 13 , (2018).
Goffaux, V. & Rossion, B. Faces are ‘spatial’ - Holistic face perception is supported by low spatial frequencies. J. Exp. Psychol. Hum. Percept. Perform . https://doi.org/10.1037/0096-1523.32.4.1023 (2006).
Schiltz, C. & Rossion, B. Faces are represented holistically in the human occipito-temporal cortex. Neuroimage https://doi.org/10.1016/j.neuroimage.2006.05.037 (2006).
Van Belle, G., De Graef, P., Verfaillie, K., Busigny, T. & Rossion, B. Whole not hole: Expert face recognition requires holistic perception. Neuropsychologia https://doi.org/10.1016/j.neuropsychologia.2010.04.034 (2010).
Quadflieg, S., Todorov, A., Laguesse, R. & Rossion, B. Normal face-based judgements of social characteristics despite severely impaired holistic face processing. Vis. cogn. 20 , 865–882 (2012).
McKone, E. Isolating the Special Component of Face Recognition: Peripheral Identification and a Mooney Face. J. Exp. Psychol. Learn. Mem. Cogn . https://doi.org/10.1037/0278-7393.30.1.181 (2004).
Sergent, J. An investigation into component and configural processes underlying face perception. Br. J. Psychol . https://doi.org/10.1111/j.2044-8295.1984.tb01895.x (1984).
Tanaka, J. W. & Farah, M. J. Parts and Wholes in Face Recognition. Q. J. Exp. Psychol. Sect. A https://doi.org/10.1080/14640749308401045 (1993).
Young, A. W., Hellawell, D. & Hay, D. C. Configurational information in face perception. Perception https://doi.org/10.1068/p160747n (2013).
Calder, A. J. & Young, A. W. Understanding the recognition of facial identity and facial expression. Nature Reviews Neuroscience https://doi.org/10.1038/nrn1724 (2005).
Todorov, A., Loehr, V. & Oosterhof, N. N. The obligatory nature of holistic processing of faces in social judgments. Perception https://doi.org/10.1068/p6501 (2010).
Junior, J. C. S. J. et al . First Impressions: A Survey on Computer Vision-Based Apparent Personality Trait Analysis. (2018).
Wang, Y. & Kosinski, M. Deep neural networks are more accurate than humans at detecting sexual orientation from facial images. J. Pers. Soc. Psychol. 114 , 246–257 (2018).
Qiu, L., Lu, J., Yang, S., Qu, W. & Zhu, T. What does your selfie say about you? Comput. Human Behav. 52 , 443–449 (2015).
Digman, J. M. Higher order factors of the Big Five. J.Pers.Soc.Psychol . https://doi.org/10.1037/0022-3514.73.6.1246 (1997).
Musek, J. A general factor of personality: Evidence for the Big One in the five-factor model. J. Res. Pers . https://doi.org/10.1016/j.jrp.2007.02.003 (2007).
DeYoung, C. G. Higher-order factors of the Big Five in a multi-informant sample. J. Pers. Soc. Psychol. 91 , 1138–1151 (2006).
Rushton, J. P. & Irwing, P. A General Factor of Personality (GFP) from two meta-analyses of the Big Five: Digman (1997) and Mount, Barrick, Scullen, and Rounds (2005). Pers. Individ. Dif. 45 , 679–683 (2008).
Wood, D., Gardner, M. H. & Harms, P. D. How functionalist and process approaches to behavior can explain trait covariation. Psychol. Rev. 122 , 84–111 (2015).
Dunlap, W. P. Generalizing the Common Language Effect Size indicator to bivariate normal correlations. Psych. Bull. 116 , 509–511 (1994).
Connolly, J. J., Kavanagh, E. J. & Viswesvaran, C. The convergent validity between self and observer ratings of personality: A meta-analytic review. Int. J. of Selection and Assessment. 15 , 110–117 (2007).
Harris, K. & Vazire, S. On friendship development and the Big Five personality traits. Soc. and Pers. Psychol. Compass. 10 , 647–667 (2016).
Weidmann, R., Schönbrodt, F. D., Ledermann, T. & Grob, A. Concurrent and longitudinal dyadic polynomial regression analyses of Big Five traits and relationship satisfaction: Does similarity matter? J. Res. in Personality. 70 , 6–15 (2017).
Cuperman, R. & Ickes, W. Big Five predictors of behavior and perceptions in initial dyadic interactions: Personality similarity helps extraverts and introverts, but hurts “disagreeables”. J. of Pers. and Soc. Psychol. 97 , 667–684 (2009).
Schmidt, F. L. & Hunter, J. E. The validity and utility of selection methods in personnel psychology: Practical and theoretical implications of 85 years of research findings. Psychol. Bull. 124 , 262–274 (1998).
Brown, M. & Sacco, D. F. Unrestricted sociosexuality predicts preferences for extraverted male faces. Pers. Individ. Dif. 108 , 123–127 (2017).
Lukaszewski, A. W. & Roney, J. R. The origins of extraversion: joint effects of facultative calibration and genetic polymorphism. Pers. Soc. Psychol. Bull. 37 , 409–21 (2011).
Curran, P. G. Methods for the detection of carelessly invalid responses in survey data. J. Exp. Soc. Psychol. 66 , 4–19 (2016).
Khromov, A. B. The five-factor questionnaire of personality [Pjatifaktornyj oprosnik lichnosti]. In Rus. (Kurgan State University, 2000).
Trizano-Hermosilla, I. & Alvarado, J. M. Best alternatives to Cronbach’s alpha reliability in realistic conditions: Congeneric and asymmetrical measurements. Front. Psychol . https://doi.org/10.3389/fpsyg.2016.00769 (2016).
Liu, Z., Luo, P., Wang, X. & Tang, X. Deep Learning Face Attributes in the Wild. in 2015 IEEE International Conference on Computer Vision (ICCV) 3730–3738 https://doi.org/10.1109/ICCV.2015.425 (IEEE, 2015).
He, K., Zhang, X., Ren, S. & Sun, J. Deep Residual Learning for Image Recognition. in 2016 IEEE Conference on Computer Vision and Pattern Recognition (CVPR) 770–778 https://doi.org/10.1109/CVPR.2016.90 (IEEE, 2016).
Download references
Acknowledgements
We appreciate the assistance of Oleg Poznyakov, who organized the data collection, and we are grateful to the anonymous peer reviewers for their detailed and insightful feedback.
Contributions
A.K., E.O., D.D. and A.N. designed the study. K.S. and A.K. designed the ML algorithms and trained the ANN. A.N. contributed to the data collection. A.K., K.S. and D.D. contributed to data pre-processing. E.O., D.D. and A.K. analysed the data, contributed to the main body of the manuscript, and revised the text. A.K. prepared Figs. 1 and 2. All the authors contributed to the final version of the manuscript.
Corresponding authors
Correspondence to Alexander Kachur or Evgeny Osin .
Ethics declarations
Competing interests.
A.K., K.S. and A.N. were employed by the company that provided the datasets for the research. E.O. and D.D. declare no competing interests.
Additional information
Publisher’s note Springer Nature remains neutral with regard to jurisdictional claims in published maps and institutional affiliations.
Supplementary information
Supplementary information., rights and permissions.
Open Access This article is licensed under a Creative Commons Attribution 4.0 International License, which permits use, sharing, adaptation, distribution and reproduction in any medium or format, as long as you give appropriate credit to the original author(s) and the source, provide a link to the Creative Commons license, and indicate if changes were made. The images or other third party material in this article are included in the article’s Creative Commons license, unless indicated otherwise in a credit line to the material. If material is not included in the article’s Creative Commons license and your intended use is not permitted by statutory regulation or exceeds the permitted use, you will need to obtain permission directly from the copyright holder. To view a copy of this license, visit http://creativecommons.org/licenses/by/4.0/ .
Reprints and permissions
About this article
Cite this article.
Kachur, A., Osin, E., Davydov, D. et al. Assessing the Big Five personality traits using real-life static facial images. Sci Rep 10 , 8487 (2020). https://doi.org/10.1038/s41598-020-65358-6
Download citation
Received : 12 April 2019
Accepted : 28 April 2020
Published : 22 May 2020
DOI : https://doi.org/10.1038/s41598-020-65358-6
Share this article
Anyone you share the following link with will be able to read this content:
Sorry, a shareable link is not currently available for this article.
Provided by the Springer Nature SharedIt content-sharing initiative
This article is cited by
Unravelling the many facets of human cooperation in an experimental study.
- Victoria V. Rostovtseva
- Mikael Puurtinen
- Franz J. Weissing
Scientific Reports (2023)
Using deep learning to predict ideology from facial photographs: expressions, beauty, and extra-facial information
- Stig Hebbelstrup Rye Rasmussen
- Steven G. Ludeke
- Robert Klemmensen
Facial Expression of TIPI Personality and CHMP-Tri Psychopathy Traits in Chimpanzees (Pan troglodytes)
- Lindsay Murray
- Jade Goddard
- David Gordon
Human Nature (2023)
Seeing the darkness: identifying the Dark Triad from emotionally neutral faces
- Danielle Haroun
- Yaarit Amram
- Joseph Glicksohn
Current Psychology (2023)
Py-Feat: Python Facial Expression Analysis Toolbox
- Jin Hyun Cheong
- Eshin Jolly
- Luke J. Chang
Affective Science (2023)
By submitting a comment you agree to abide by our Terms and Community Guidelines . If you find something abusive or that does not comply with our terms or guidelines please flag it as inappropriate.
Quick links
- Explore articles by subject
- Guide to authors
- Editorial policies
Sign up for the Nature Briefing newsletter — what matters in science, free to your inbox daily.

Click through the PLOS taxonomy to find articles in your field.
For more information about PLOS Subject Areas, click here .
Loading metrics
Open Access
Peer-reviewed
Research Article
Personality types revisited–a literature-informed and data-driven approach to an integration of prototypical and dimensional constructs of personality description
Roles Conceptualization, Data curation, Formal analysis, Investigation, Methodology, Validation, Visualization, Writing – original draft
* E-mail: [email protected]
Affiliation Department of Psychology, Freie Universität Berlin, Berlin, Germany

Roles Conceptualization, Investigation, Methodology, Supervision, Validation, Writing – review & editing
Affiliation Department of Psychology, University of Duisburg-Essen, Duisburg Germany
Affiliation Personality Psychology and Psychological Assessment Unit, Helmut Schmidt University of the Federal Armed Forces Hamburg, Hamburg, Germany
- André Kerber,
- Marcus Roth,
- Philipp Yorck Herzberg
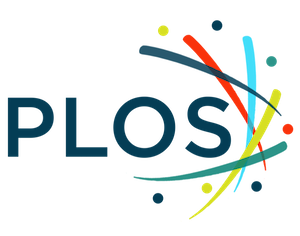
- Published: January 7, 2021
- https://doi.org/10.1371/journal.pone.0244849
- Peer Review
- Reader Comments
A new algorithmic approach to personality prototyping based on Big Five traits was applied to a large representative and longitudinal German dataset (N = 22,820) including behavior, personality and health correlates. We applied three different clustering techniques, latent profile analysis, the k-means method and spectral clustering algorithms. The resulting cluster centers, i.e. the personality prototypes, were evaluated using a large number of internal and external validity criteria including health, locus of control, self-esteem, impulsivity, risk-taking and wellbeing. The best-fitting prototypical personality profiles were labeled according to their Euclidean distances to averaged personality type profiles identified in a review of previous studies on personality types. This procedure yielded a five-cluster solution: resilient, overcontroller, undercontroller, reserved and vulnerable-resilient. Reliability and construct validity could be confirmed. We discuss wether personality types could comprise a bridge between personality and clinical psychology as well as between developmental psychology and resilience research.
Citation: Kerber A, Roth M, Herzberg PY (2021) Personality types revisited–a literature-informed and data-driven approach to an integration of prototypical and dimensional constructs of personality description. PLoS ONE 16(1): e0244849. https://doi.org/10.1371/journal.pone.0244849
Editor: Stephan Doering, Medical University of Vienna, AUSTRIA
Received: January 5, 2020; Accepted: December 17, 2020; Published: January 7, 2021
Copyright: © 2021 Kerber et al. This is an open access article distributed under the terms of the Creative Commons Attribution License , which permits unrestricted use, distribution, and reproduction in any medium, provided the original author and source are credited.
Data Availability: The data used in this article were made available by the German Socio-Economic Panel (SOEP, Data for years 1984-2015) at the German Institute for Economic Research, Berlin, Germany. To ensure the confidentiality of respondents’ information, the SOEP adheres to strict security standards in the provision of SOEP data. The data are reserved exclusively for research use, that is, they are provided only to the scientific community. To require full access to the data used in this study, it is required to sign a data distribution contract. All contact informations and the procedure to request the data can be obtained at: https://www.diw.de/en/diw_02.c.222829.en/access_and_ordering.html .
Funding: The author(s) received no specific funding for this work.
Competing interests: The authors have declared that no competing interests exist.
Introduction
Although documented theories about personality types reach back more than 2000 years (i.e. Hippocrates’ humoral pathology), and stereotypes for describing human personality are also widely used in everyday psychology, the descriptive and variable-oriented assessment of personality, i.e. the description of personality on five or six trait domains, has nowadays consolidated its position in modern personality psychology.
In recent years, however, the person-oriented approach, i.e. the description of an individual personality by its similarity to frequently occurring prototypical expressions, has amended the variable-oriented approach with the addition of valuable insights into the description of personality and the prediction of behavior. Focusing on the trait configurations, the person-oriented approach aims to identify personality types that share the same typical personality profile [ 1 ].
Nevertheless, the direct comparison of the utility of person-oriented vs. variable-oriented approaches to personality description yielded mixed results. For example Costa, Herbst, McCrae, Samuels and Ozer [ 2 ] found a higher amount of explained variance in predicting global functioning, geriatric depression or personality disorders for the variable-centered approach using Big Five personality dimensions. But these results also reflect a methodological caveat of this approach, as the categorical simplification of dimensionally assessed variables logically explains less variance. Despite this, the person-centered approach was found to heighten the predictability of a person’s behavior [ 3 , 4 ] or the development of adolescents in terms of internalizing and externalizing symptoms or academic success [ 5 , 6 ], problem behavior, delinquency and depression [ 7 ] or anxiety symptoms [ 8 ], as well as stress responses [ 9 ] and social attitudes [ 10 ]. It has also led to new insights into the function of personality in the context of other constructs such as adjustment [ 2 ], coping behavior [ 11 ], behavioral activation and inhibition [ 12 ], subjective and objective health [ 13 ] or political orientation [ 14 ], and has greater predictive power in explaining longitudinally measured individual differences in more temperamental outcomes such as aggressiveness [ 15 ].
However, there is an ongoing debate about the appropriate number and characteristics of personality prototypes and whether they perhaps constitute an methodological artifact [ 16 ].
With the present paper, we would like to make a substantial contribution to this debate. In the following, we first provide a short review of the personality type literature to identify personality types that were frequently replicated and calculate averaged prototypical profiles based on these previous findings. We then apply multiple clustering algorithms on a large German dataset and use those prototypical profiles generated in the first step to match the results of our cluster analysis to previously found personality types by their Euclidean distance in the 5-dimensional space defined by the Big Five traits. This procedure allows us to reliably link the personality prototypes found in our study to previous empirical evidence, an important analysis step lacking in most previous studies on this topic.
The empirical ground of personality types
The early studies applying modern psychological statistics to investigate personality types worked with the Q-sort procedure [ 1 , 15 , 17 ], and differed in the number of Q-factors. With the Q-Sort method, statements about a target person must be brought in an order depending on how characteristic they are for this person. Based on this Q-Sort data, prototypes can be generated using Q-Factor Analysis, also called inverse factor analysis. As inverse factor analysis is basically interchanging variables and persons in the data matrix, the resulting factors of a Q-factor analysis are prototypical personality profiles and not hypothetical or latent variable dimensions. On this basis, personality types (groups of people with similar personalities) can be formed in a second step by assigning each person to the prototype with whose profile his or her profile correlates most closely. All of these early studies determined at least three prototypes, which were labeled resilient, overcontroler and undercontroler grounded in Block`s theory of ego-control and ego-resiliency [ 18 ]. According to Jack and Jeanne Block’s decade long research, individuals high in ego-control (i.e. the overcontroler type) tend to appear constrained and inhibited in their actions and emotional expressivity. They may have difficulty making decisions and thus be non-impulsive or unnecessarily deny themselves pleasure or gratification. Children classified with this type in the studies by Block tend towards internalizing behavior. Individuals low in ego-control (i.e. the undercontroler type), on the other hand, are characterized by higher expressivity, a limited ability to delay gratification, being relatively unattached to social standards or customs, and having a higher propensity to risky behavior. Children classified with this type in the studies by Block tend towards externalizing behavior.
Individuals high in Ego-resiliency (i.e. the resilient type) are postulated to be able to resourcefully adapt to changing situations and circumstances, to tend to show a diverse repertoire of behavioral reactions and to be able to have a good and objective representation of the “goodness of fit” of their behavior to the situations/people they encounter. This good adjustment may result in high levels of self-confidence and a higher possibility to experience positive affect.
Another widely used approach to find prototypes within a dataset is cluster analysis. In the field of personality type research, one of the first studies based on this method was conducted by Caspi and Silva [ 19 ], who applied the SPSS Quick Cluster algorithm to behavioral ratings of 3-year-olds, yielding five prototypes: undercontrolled, inhibited, confident, reserved, and well-adjusted.
While the inhibited type was quite similar to Block`s overcontrolled type [ 18 ] and the well-adjusted type was very similar to the resilient type, two further prototypes were added: confident and reserved. The confident type was described as easy and responsive in social interaction, eager to do exercises and as having no or few problems to be separated from the parents. The reserved type showed shyness and discomfort in test situations but without decreased reaction speed compared to the inhibited type. In a follow-up measurement as part of the Dunedin Study in 2003 [ 20 ], the children who were classified into one of the five types at age 3 were administered the MPQ at age 26, including the assessment of their individual Big Five profile. Well-adjusteds and confidents had almost the same profiles (below-average neuroticism and above average on all other scales except for extraversion, which was higher for the confident type); undercontrollers had low levels of openness, conscientiousness and openness to experience; reserveds and inhibiteds had below-average extraversion and openness to experience, whereas inhibiteds additionally had high levels of conscientiousness and above-average neuroticism.
Following these studies, a series of studies based on cluster analysis, using the Ward’s followed by K-means algorithm, according to Blashfield & Aldenderfer [ 21 ], on Big Five data were published. The majority of the studies examining samples with N < 1000 [ 5 , 7 , 22 – 26 ] found that three-cluster solutions, namely resilients, overcontrollers and undercontrollers, fitted the data the best. Based on internal and external fit indices, Barbaranelli [ 27 ] found that a three-cluster and a four-cluster solution were equally suitable, while Gramzow [ 28 ] found a four-cluster solution with the addition of the reserved type already published by Caspi et al. [ 19 , 20 ]. Roth and Collani [ 10 ] found that a five-cluster solution fitted the data the best. Using the method of latent profile analysis, Merz and Roesch [ 29 ] found a 3-cluster, Favini et al. [ 6 ] found a 4-cluster solution and Kinnunen et al. [ 13 ] found a 5-cluster solution to be most appropriate.
Studies examining larger samples of N > 1000 reveal a different picture. Several favor a five-cluster solution [ 30 – 34 ] while others favor three clusters [ 8 , 35 ]. Specht et al. [ 36 ] examined large German and Australian samples and found a three-cluster solution to be suitable for the German sample and a four-cluster solution to be suitable for the Australian sample. Four cluster solutions were also found to be most suitable to Australian [ 37 ] and Chinese [ 38 ] samples. In a recent publication, the authors cluster-analysed very large datasets on Big Five personality comprising more than 1,5 million online participants using Gaussian mixture models [ 39 ]. Albeit their results “provide compelling evidence, both quantitatively and qualitatively, for at least four distinct personality types”, two of the four personality types in their study had trait profiles not found previously and all four types were given labels unrelated to previous findings and theory. Another recent publication [ 40 ] cluster-analysing data of over 270,000 participants on HEXACO personality “provided evidence that a five-profile solution was optimal”. Despite limitations concerning the comparability of HEXACO trait profiles with FFM personality type profiles, the authors again decided to label their personality types unrelated to previous findings instead using agency-communion and attachment theories.
We did not include studies in this literature review, which had fewer than 199 participants or those which restricted the number of types a priori and did not use any method to compare different clustering solutions. We have made these decisions because a too low sample size increases the probability of the clustering results being artefacts. Further, a priori limitation of the clustering results to a certain number of personality types is not well reasonable on the base of previous empirical evidence and again may produce artefacts, if the a priori assumed number of clusters does not fit the data well.
To gain a better overview, we extracted all available z-scores from all samples of the above-described studies. Fig 1 shows the averaged z-scores extracted from the results of FFM clustering solutions for all personality prototypes that occurred in more than one study. The error bars represent the standard deviation of the distribution of the z-scores of the respective trait within the same personality type throughout the different studies. Taken together the resilient type was replicated in all 19 of the mentioned studies, the overcontroler type in 16, the undercontroler personality type in 17 studies, the reserved personality type was replicated in 6 different studies, the confident personality type in 4 and the non-desirable type was replicated twice.
- PPT PowerPoint slide
- PNG larger image
- TIFF original image
Average Big Five z-scores of personality types based on clustering of FFM datasets with N ≥ 199 that were replicated at least once. Error bars indicate the standard deviation of the repective trait within the respective personality type found in the literature [ 5 , 6 , 10 , 22 – 25 , 27 – 31 , 33 – 36 , 38 , 39 , 41 ].
https://doi.org/10.1371/journal.pone.0244849.g001
Three implications can be drawn from this figure. First, although the results of 19 studies on 26 samples with a total N of 1,560,418 were aggregated, the Big Five profiles for all types can still be clearly distinguished. In other words, personality types seem to be a phenomenon that survives the aggregation of data from different sources. Second, there are more than three replicable personality types, as there are other replicated personality types that seem to have a distinct Big Five profile, at least regarding the reserved and confident personality types. Third and lastly, the non-desirable type seems to constitute the opposite of the resilient type. Looking at two-cluster solutions on Big Five data personality types in the above-mentioned literature yields the resilient opposed to the non-desirable type. This and the fact that it was only replicated twice in the above mentioned studies points to the notion that it seems not to be a distinct type but rather a combined cluster of the over- and undercontroller personality types. Further, both studies with this type in the results did not find either the undercontroller or the overcontroller cluster or both. Taken together, five distinct personality types were consistently replicated in the literature, namely resilient, overcontroller, undercontroller, reserved and confident. However, inferring from the partly large error margin for some traits within some prototypes, not all personality traits seem to contribute evenly to the occurrence of the different prototypes. While for the overcontroler type, above average neuroticism, below average extraversion and openness seem to be distinctive, only below average conscientiousness and agreeableness seemed to be most characteristic for the undercontroler type. The reserved prototype was mostly characterized by below average openness and neuroticism with above average conscientiousness. Above average extraversion, openness and agreeableness seemed to be most distinctive for the confident type. Only for the resilient type, distinct expressions of all Big Five traits seemed to be equally significant, more precisely below average neuroticism and above average extraversion, openness, agreeableness and conscientiousness.
Research gap and novelty of this study
The cluster methods used in most of the mentioned papers were the Ward’s followed by K-means method or latent profile analysis. With the exception of Herzberg and Roth [ 30 ], Herzberg [ 33 ], Barbaranelli [ 27 ] and Steca et. al. [ 25 ], none of the studies used internal or external validity indices other than those which their respective algorithm (in most cases the SPSS software package) had already included. Gerlach et al. [ 39 ] used Gaussian mixture models in combination with density measures and likelihood measures.
The bias towards a smaller amount of clusters resulting from the utilization of just one replication index, e.g. Cohen's Kappa calculated by split-half cross-validation, which was ascertained by Breckenridge [ 42 ] and Overall & Magee [ 43 ], is probably the reason why a three-cluster solution is preferred in most studies. Herzberg and Roth [ 30 ] pointed to the study by Milligan and Cooper [ 44 ], which proved the superiority of the Rand index over Cohen's Kappa and also suggested a variety of validity metrics for internal consistency to examine the construct validity of the cluster solutions.
Only a part of the cited studies had a large representative sample of N > 2000 and none of the studies used more than one clustering algorithm. Moreover, with the exception of Herzberg and Roth [ 30 ] and Herzberg [ 33 ], none of the studies used a large variety of metrics for assessing internal and external consistency other than those provided by the respective clustering program they used. This limitation further adds up to the above mentioned bias towards smaller amounts of clusters although the field of cluster analysis and algorithms has developed a vast amount of internal and external validity algorithms and criteria to tackle this issue. Further, most of the studies had few or no other assessments or constructs than the Big Five to assess construct validity of the resulting personality types. Herzberg and Roth [ 30 ] and Herzberg [ 33 ] as well, though using a diverse variety of validity criteria only used one clustering algorithm on a medium-sized dataset with N < 2000.
Most of these limitations also apply to the study by Specht et. al. [ 36 ], which investigated two measurement occasions of the Big Five traits in the SOEP data sample. They used only one clustering algorithm (latent profile analysis), no other algorithmic validity criteria than the Bayesian information criterion and did not utilize any of the external constructs also assessed in the SOEP sample, such as mental health, locus of control or risk propensity for construct validation.
The largest sample and most advanced clustering algorithm was used in the recent study by Gerlach et al. [ 39 ]. But they also used only one clustering algorithm, and had no other variables except Big Five trait data to assess construct validity of the resulting personality types.
The aim of the present study was therefore to combine different methodological approaches while rectifying the shortcomings in several of the studies mentioned above in order to answer the following exploratory research questions: Are there replicable personality types, and if so, how many types are appropriate and in which constellations are they more (or less) useful than simple Big Five dimensions in the prediction of related constructs?
Three conceptually different clustering algorithms were used on a large representative dataset. The different solutions of the different clustering algorithms were compared using methodologically different internal and external validity criteria, in addition to those already used by the respective clustering algorithm.
To further examine the construct validity of the resulting personality types, their predictive validity in relation to physical and mental health, wellbeing, locus of control, self-esteem, impulsivity, risk-taking and patience were assessed.
Mental health and wellbeing seem to be associated mostly with neuroticism on the variable-oriented level [ 45 ], but on a person-oriented level, there seem to be large differences between the resilient and the overcontrolled personality type concerning perceived health and well-being beyond mean differences in neuroticism [ 33 ]. This seems also to be the case for locus of control and self-esteem, which is associated with neuroticism [ 46 ] and significantly differs between resilient and overcontrolled personality type [ 33 ]. On the other hand, impulsivity and risk taking seem to be associated with all five personality traits [ 47 ] and e.g. risky driving or sexual behavior seem to occur more often in the undercontrolled personality type [ 33 , 48 ].
We chose these measures because of their empirically known differential associations to Big Five traits as well as to the above described personality types. So this both offers the opportunity to have an integrative comparison of the variable- and person-centered descriptions of personality and to assess construct validity of the personality types resulting from our analyses.
Materials and methods
The acquisition of the data this study bases on was carried out in accordance with the principles of the Basel Declaration and recommendations of the “Principles of Ethical Research and Procedures for Dealing with Scientific Misconduct at DIW Berlin”. The protocol was approved by the Deutsches Institut für Wirtschaftsforschung (DIW).
The data used in this study were provided by the German Socio-Economic Panel Study (SOEP) of the German institute for economic research [ 49 ]. Sample characteristics are shown in Table 1 . The overall sample size of the SOEP data used in this study, comprising all individuals who answered at least one of the Big-Five personality items in 2005 and 2009, was 25,821. Excluding all members with more than one missing answers on the Big Five assessment or intradimensional answer variance more than four times higher than the sample average resulted in a total Big Five sample of N = 22,820, which was used for the cluster analyses. 14,048 of these individuals completed, in addition to the Big Five, items relevant to further constructs examined in this study that were assessed in other years. The 2013 SOEP data Big Five assessment was used as a test sample to examine stability and consistency of the final cluster solution.
https://doi.org/10.1371/journal.pone.0244849.t001
The Big Five were assessed in 2005 2009 and 2013 using the short version of the Big Five inventory (BFI-S). It consists of 15 items, with internal consistencies (Cronbach’s alpha) of the scales ranging from .5 for openness to .73 for openness [ 50 ]. Further explorations showed strong robustness across different assessment methods [ 51 ].
To measure the predictive validity, several other measures assessed in the SOEP were included in the analyses. In detail, these were:
Patience was assessed in 2008 with one item: “Are you generally an impatient person, or someone who always shows great patience?”
Risk taking.
Risk-taking propensity was assessed in 2009 by six items asking about the willingness to take risks while driving, in financial matters, in leisure and sports, in one’s occupation (career), in trusting unknown people and the willingness to take health risks, using a scale from 0 (risk aversion) to 10 (fully prepared to take risks). Cronbach’s alpha was .82 for this scale in the current sample.
Impulsivity/Spontaneity.
Impulsivity/spontaneity was assessed in 2008 with one item: Do you generally think things over for a long time before acting–in other words, are you not impulsive at all? Or do you generally act without thinking things over for long time–in other words, are you very impulsive?
Affective and cognitive wellbeing.
Affect was assessed in 2008 by four items asking about the amount of anxiety, anger, happiness or sadness experienced in the last four weeks on a scale from 1 (very rare) to 5 (very often). Cronbach’s alpha for this scale was .66. The cognitive satisfaction with life was assessed by 10 items asking about satisfaction with work, health, sleep, income, leisure time, household income, household duties, family life, education and housing, with a Cronbach’s alpha of .67. The distinction between cognitive and affective wellbeing stems from sociological research based on constructs by Schimmack et al. [ 50 ].
Locus of control.
The individual attitude concerning the locus of control, the degree to which people believe in having control over the outcome of events in their lives opposed to being exposed to external forces beyond their control, was assessed in 2010 with 10 items, comprising four positively worded items such as “My life’s course depends on me” and six negatively worded items such as “Others make the crucial decisions in my life”. Items were rated on a 7-point scale ranging from “does not apply” to “does apply”. Cronbach’s alpha in the present sample for locus of control was .57.
Self-esteem.
Global self-esteem–a person’s overall evaluation or appraisal of his or her worth–was measured in 2010 with one item: “To what degree does the following statement apply to you personally?: I have a positive attitude toward myself”.
To assess subjective health, the 12-Item Short Form Health Survey (SF-12) was integrated into the SOEP questionnaire and assessed in 2002, 2004, 2006, 2008 and 2010. In the present study, we used the data from 2008 and 2010. The SF-12 is a short form of the SF-36, a self-report questionnaire to assess the non-disease-specific health status [ 52 ]. Within the SF-12, items can be grouped onto two subscales, namely the physical component summary scale, with items asking about physical health correlates such as how exhausting it is to climb stairs, and the mental component summary scale, with items asking about mental health correlates such as feeling sad and blue. The literature on health measures often distinguishes between subjective and objective health measures (e.g., BMI, blood pressure). From this perspective, the SF-12 would count as a subjective health measure. In the present sample, Cronbach’s alpha for the SF-12 items was .77.
Derivation of the prototypes
The first step was to administer three different clustering methods on the Big Five data of the SOEP sample: First, the conventional linear clustering method used by Asendorpf [ 15 , 35 , 53 ] and also Herzberg and Roth [ 30 ] combines the hierarchical clustering method of Ward [ 54 ] with the k-means algorithm [ 55 ]. This algorithm generates a first guess of personality types based on hierarchical clustering, and then uses this first guess as starting points for the k-means-method, which iteratively adjusts the personality profiles, i.e. the cluster means to minimize the error of allocation, i.e. participants with Big Five profiles that are allocated to two or more personality types. The second algorithm we used was latent profile analysis with Mclust in R [ 56 ], an algorithm based on probabilistic finite mixture modeling, which assumes that there are latent classes/profiles/mixture components underlying the manifest observed variables. This algorithm generates personality profiles and iteratively calculates the probability of every participant in the data to be allocated to one of the personality types and tries to minimize an error term using maximum likelihood method. The third algorithm was spectral clustering, an algorithm which initially computes eigenvectors of graph Laplacians of the similarity graph constructed on the input data to discover the number of connected components in the graph, and then uses the k-means algorithm on the eigenvectors transposed in a k-dimensional space to compute the desired k clusters [ 57 ]. As it is an approach similar to the kernel k-means algorithm [ 58 ], spectral clustering can discover non-linearly separable cluster formations. Thus, this algorithm is able, in contrast to the standard k-means procedure, to discover personality types having unequal or non-linear distributions within the Big-Five traits, e.g. having a small SD on neuroticism while having a larger SD on conscientiousness or a personality type having high extraversion and either high or low agreeableness.
Within the last 50 years, a large variety of clustering algorithms have been established, and several attempts have been made to group them. In their book about cluster analysis, Bacher et al. [ 59 ] group cluster algorithms into incomplete clustering algorithms, e.g. Q-Sort or multidimensional scaling, deterministic clustering, e.g. k-means or nearest-neighbor algorithms, and probabilistic clustering, e.g. latent class and latent profile analysis. According to Jain [ 60 ], cluster algorithms can be grouped by their objective function, probabilistic generative models and heuristics. In his overview of the current landscape of clustering, he begins with the group of density-based algorithms with linear similarity functions, e.g. DBSCAN, or probabilistic models of density functions, e.g. in the expectation-maximation (EM) algorithm. The EM algorithm itself also belongs to the large group of clustering algorithms with an information theoretic formulation. Another large group according to Jain is graph theoretic clustering, which includes several variants of spectral clustering. Despite the fact that it is now 50 years old, Jain states that k-means is still a good general-purpose algorithm that can provide reasonable clustering results.
The clustering algorithms chosen for the current study are therefore representatives of the deterministic vs. probabilistic grouping according to Bacher et. al. [ 59 ], as well as representatives of the density-based, information theoretic and graph theoretic grouping according to Jain [ 60 ].
Determining the number of clusters
There are two principle ways to determine cluster validity: external or relative criteria and internal validity indices.
External validity criteria.
External validity criteria measure the extent to which cluster labels match externally supplied class labels. If these external class labels originate from another clustering algorithm used on the same data sample, the resulting value of the external cluster validity index is relative. Another method, which is used in the majority of the cited papers in section 1, is to randomly split the data in two halves, apply a clustering algorithm on both halves, calculate the cluster means and allocate members of one half to the calculated clusters of the opposite half by choosing the cluster mean with the shortest Euclidean distance to the data member in charge. If the cluster algorithm allocation of one half is then compared with the shortest Euclidean distance allocation of the same half by means of an external cluster validity index, this results in a value for the reliability of the clustering method on the data sample.
As allocating data points/members by Euclidean distances always yields spherical and evenly shaped clusters, it will favor clustering methods that also yield spherical and evenly shaped clusters, as it is the case with standard k-means. The cluster solutions obtained with spectral clustering as well as latent profile analysis (LPA) are not (necessarily) spherical or evenly shaped; thus, allocating members of a dataset by their Euclidean distances to cluster means found by LPA or spectral clustering does not reliably represent the structure of the found cluster solution. This is apparent in Cohen’s kappa values <1 if one uses the Euclidean external cluster assignment method comparing a spectral cluster solution with itself. Though by definition, Cohen’s kappa should be 1 if the two ratings/assignments compared are identical, which is the case when comparing a cluster solution (assigning every data point to a cluster) with itself. This problem can be bypassed by allocating the members of the test dataset to the respective clusters by training a support vector machine classifier for each cluster. Support vector machines (SVM) are algorithms to construct non-linear “hyperplanes” to classify data given their class membership [ 61 ]. They can be used very well to categorize members of a dataset by an SVM-classifier trained on a different dataset. Following the rationale not to disadvantage LPA and spectral clustering in the calculation of the external validity, we used an SVM classifier to calculate the external validity criteria for all clustering algorithms in this study.
To account for the above mentioned bias to smaller numbers of clusters we applied three external validity criteria: Cohen’s kappa, the Rand index [ 62 ] and the Hubert-Arabie adjusted Rand index [ 63 ].
Internal validity criteria.
Again, to account for the bias to smaller numbers of clusters, we also applied multiple internal validity criteria selected in line with the the following reasoning: According to Lam and Yan [ 64 ], the internal validity criteria fall into three classes: Class one includes cost-function-based indices, e.g. AIC or BIC [ 65 ], whereas class two comprises cluster-density-based indices, e.g. the S_Dbw index [ 66 ]. Class three is grounded on geometric assumptions concerning the ratio of the distances within clusters compared to the distances between the clusters. This class has the most members, which differ in their underlying mathematics. One way of assessing geometric cluster properties is to calculate the within- and/or between-group scatter, which both rely on summing up distances of the data points to their barycenters (cluster means). As already explained in the section on external criteria, calculating distances to cluster means will always favor spherical and evenly shaped cluster solutions without noise, i.e. personality types with equal and linear distributions on the Big Five trait dimensions, which one will rarely encounter with natural data.
Another way not solely relying on distances to barycenters or cluster means is to calculate directly with the ratio of distances of the data points within-cluster and between-cluster. According to Desgraupes [ 67 ], this applies to the following indices: the C-index, the Baker & Hubert Gamma index, the G(+) index, Dunn and Generalized Dunn indices, the McClain-Rao index, the Point-Biserial index and the Silhouette index. As the Gamma and G(+) indices rely on the same mathematical construct, one can declare them as redundant. According to Bezdek [ 68 ], the Dunn index is very sensitive to noise, even if there are only very few outliers in the data. Instead, the authors propose several ways to compute a Generalized Dunn index, some of which also rely on the calculation of barycenters. The best-performing GDI algorithm outlined by Bezdek and Pal [ 68 ] which does not make use of cluster barycenters is a ratio of the mean distance of every point between clusters to the maximum distance between points within the cluster, henceforth called GDI31. According to Vendramin et al. [ 69 ], the Gamma, C-, and Silhouette indices are the best-performing (over 80% correct hit rate), while the worst-performing are the Point-Biserial and the McClain-Rao indices (73% and 51% correct hit rate, respectively).
Fig 2 shows a schematic overview of the procedure we used to determine the personality types Big Five profiles, i.e. the cluster centers. To determine the best fitting cluster solution, we adopted the two-step procedure proposed by Blashfield and Aldenfelder [ 21 ] and subsequently used by Asendorpf [ 15 , 35 , 53 ] Boehm [ 41 ], Schnabel [ 24 ], Gramzow [ 28 ], and Herzberg and Roth [ 30 ], with a few adjustments concerning the clustering algorithms and the validity criteria.
LPA = latent profile analysis, SVM = Support Vector Machine.
https://doi.org/10.1371/journal.pone.0244849.g002
First, we drew 20 random samples of the full sample comprising all individuals who answered the Big-Five personality items in 2005 and 2009 with N = 22,820 and split every sample randomly into two halves. Second, all three clustering algorithms described above were performed on each half, saving the 3-, 4-,…,9- and 10-cluster solution. Third, participants of each half were reclassified based on the clustering of the other half of the same sample, again for every clustering algorithm and for all cluster solutions from three to 10 clusters. In contrast to Asendorpf [ 35 ], this was implemented not by calculating Euclidean distances, but by training a support vector machine classifier for every cluster of a cluster solution of one half-sample and reclassifying the members of the other half of the same sample by the SVM classifier. The advantages of this method are explained in the section on external criteria. This resulted in 20 samples x 2 halves per sample x 8 cluster solutions x 3 clustering algorithms, equaling 960 clustering solutions to be compared.
The fourth step was to compute the external criteria comparing each Ward followed by k-means, spectral, or probabilistic clustering solution of each half-sample to the clustering by the SVM classifier trained on the opposite half of the same sample, respectively. The external calculated in this step were Cohen's kappa, Rand’s index [ 62 ] and the Hubert & Arabie adjusted Rand index [ 63 ]. The fifth step consisted of averaging: We first averaged the external criteria values per sample (one value for each half), and then averaged the 20x4 external criteria values for each of the 3-,4-…, 10-cluster solutions for each algorithm.
The sixth step was to temporarily average the external criteria values for the 3-,4-,… 10-cluster solution over the three clustering algorithms and discard the cluster solutions that had a total average kappa below 0.6.
As proposed by Herzberg and Roth [ 30 ], we then calculated several internal cluster validity indices for all remaining cluster solutions. The internal validity indices which we used were, in particular, the C-index [ 70 ], the Baker-Hubert Gamma index [ 71 ], the G + index [ 72 ], the Generalized Dunn index 31 [ 68 ], the Point-Biserial index [ 44 ], the Silhouette index [ 73 ], AIC and BIC [ 65 ] and the S_Dbw index [ 66 ]. Using all of these criteria, it is possible to determine the best clustering solution in a mathematical/algorithmic manner.
The resulting clusters where then assigned names by calculating Euclidean distances to the clusters/personality types found in the literature, taking the nearest type within the 5-dimensional space defined by the respective Big Five values.
To examine the stability and consistency of the final cluster solution, in a last step, we then used the 2013 SOEP data sample to calculate a cluster solution using the algorithm and parameters which generated the solution with the best validity criteria for the 2005 and 2009 SOEP data sample. The 2013 personality prototypes were allocated to the personality types of the solution from the previous steps by their profile similarity measure D. Stability then was assessed by calculation of Rand-index, adjusted Rand-index and Cohen’s Kappa for the complete solution and for every single personality type. To generate the cluster allocations between the different cluster solutions, again we used SVM classifier as described above.
To assess the predictive and the construct validity of the resulting personality types, the inversed Euclidean distance for every participant to every personality prototype (averaged Big Five profile in one cluster) in the 5-dimensional Big-Five space was calculated and correlated with further personality, behavior and health measures mentioned above. To ensure that longitudinal reliability was assessed in this step, Big Five data assessed in 2005 were used to predict measures which where assessed three, four or five years later. The selection of participants with available data in 2005 and 2008 or later reduced the sample size in this step to N = 14,048.
Internal and external cluster fit indices
Table 2 shows the mean Cohen’s kappa values, averaged over all clustering algorithms and all 20 bootstrapped data permutations.
https://doi.org/10.1371/journal.pone.0244849.t002
Whereas the LPA and spectral cluster solutions seem to have better kappa values for fewer clusters, the kappa values of the k-means clustering solutions have a peak at five clusters, which is even higher than the kappa values of the three-cluster solutions of the other two algorithms.
Considering that these values are averaged over 20 independent computations, there is very low possibility that this result is an artefact. As the solutions with more than five clusters had an average kappa below .60, they were discarded in the following calculations.
Table 3 shows the calculated external and internal validity indices for the three- to five-cluster solutions, ordered by the clustering algorithm. Comparing the validity criterion values within the clustering algorithms reveals a clear preference for the five-cluster solution in the spectral as well as the Ward followed by k-means algorithm.
https://doi.org/10.1371/journal.pone.0244849.t003
Looking solely at the cluster validity results of the latent profile models, they seem to favor the three-cluster model. Yet, in a global comparison, only the S_Dbw index continues to favor the three-cluster LPA model, whereas the results of all other 12 validity indices support five-cluster solutions. The best clustering solution in terms of the most cluster validity index votes is the five-cluster Ward followed by k-means solution, and second best is the five-cluster spectral solution. It is particularly noteworthy that the five-cluster K-means solution has higher values on all external validity criteria than all other solutions. As these values are averaged over 20 independent cluster computations on random data permutations, and still have better values than solutions with fewer clusters despite the fact that these indices have a bias towards solutions with fewer clusters [ 42 ], there seems to be a substantial, replicable five-component structure in the Big Five Data of the German SOEP sample.
Description of the prototypes
The mean z-scores on the Big Five factors of the five-cluster k-means as well as the spectral solution are depicted in Fig 2 . Also depicted is the five-cluster LPA solution, which is, despite having poor internal and external validity values compared to the other two solutions, more complicated to interpret. To find the appropriate label for the cluster partitions, the respective mean z-scores on the Big Five factors were compared with the mean z-scores found in the literature, both visually and by the Euclidean distance.
The spectral and the Ward followed by k-means solution overlap by 81.3%; the LPA solution only overlaps with the other two solutions by 21% and 23%, respectively. As the Ward followed by k-means solution has the best values both for external and internal validity criteria, we will focus on this solution in the following.
The first cluster has low neuroticism and high values on all other scales and includes on average 14.4% of the participants (53.2% female; mean age 53.3, SD = 17.3). Although the similarity to the often replicated resilient personality type is already very clear merely by looking at the z-scores, a very strong congruence is also revealed by computing the Euclidean distance (0.61). The second cluster is mainly characterized by high neuroticism, low extraversion and low openness and includes on average 17.3% of the participants (54.4% female; mean age 57.6, SD = 18.2). It clearly resembles the overcontroller type, to which it also has the shortest Euclidean distance (0.58). The fourth cluster shows below-average values on the factors neuroticism, extraversion and openness, as opposed to above-average values on openness and conscientiousness. It includes on average 22.5% of the participants (45% female; mean age 56.8, SD = 17.6). Its mean z-scores closely resemble the reserved personality type, to which it has the smallest Euclidean distance (0.36). The third cluster is mainly characterized by low conscientiousness and low openness, although in the spectral clustering solution, it also has above-average extraversion and openness values. Computing the Euclidean distance (0.86) yields the closest proximity to the undercontroller personality type. This cluster includes on average 24.6% of the participants (41.3% female; mean age 50.8, SD = 18.3). The fifth cluster exhibits high z-scores on every Big Five trait, including a high value for neuroticism. Computing the Euclidean distances to the previously found types summed up in Fig 1 reveals the closest resemblance with the confident type (Euclidean distance = 0.81). Considering the average scores of the Big Five traits, it resembles the confident type from Herzberg and Roth [ 30 ] and Collani and Roth [ 10 ] as well as the resilient type, with the exception of the high neuroticism score. Having above average values on the more adaptive traits while having also above average neuroticism values reminded a reviewer from a previous version of this paper of the vulnerable but invincible children of the Kauai-study [ 74 ]. Despite having been exposed to several risk factors in their childhood, they were well adapted in their adulthood except for low coping efficiency in specific stressful situations. Taken together with the lower percentage of participants in the resilient cluster in this study, compared to previous studies, we decided to name the 5 th cluster vulnerable-resilient. Consequently, only above or below average neuroticism values divided between resilient and vulnerable resilient. On average, 21.2% of the participants were allocated to this cluster (68.3% female; mean age 54.9, SD = 17.4).
Summarizing the descriptive statistics, undercontrollers were the “youngest” cluster whereas overcontrollers were the “oldest”. The mean age differed significantly between clusters ( F [4, 22820] = 116.485, p <0.001), although the effect size was small ( f = 0.14). The distribution of men and women between clusters differed significantly (c 2 [ 4 ] = 880.556, p <0.001). With regard to sex differences, it was particularly notable that the vulnerable-resilient cluster comprised only 31.7% men. This might be explained by general sex differences on the Big Five scales. According to Schmitt et al. [ 75 ], compared to men, European women show a general bias to higher neuroticism (d = 0.5), higher conscientiousness (d = 0.3) and higher extraversion and openness (d = 0.2). As the vulnerable-resilient personality type is mainly characterized by high neuroticism and above-average z-scores on the other scales, it is therefore more likely to include women. In turn, this implies that men are more likely to have a personality profile characterized mainly by low conscientiousness and low openness, which is also supported by our findings, as only 41.3% of the undercontrollers were female.
Concerning the prototypicality of the five-cluster solution compared to the mean values extracted from previous studies, it is apparent that the resilient, the reserved and the overcontroller type are merely exact replications. In contrast to previous findings, the undercontrollers differed from the previous findings cited above in terms of average neuroticism, whereas the vulnerable-resilient type differed from the previously found type (labeled confident) in terms of high neuroticism.
Stability and consistency
Inspecting the five cluster solution using the k-means algorithm on the Big Five data of the 2013 SOEP sample seemed to depict a replication of the above described personality types. This first impression was confirmed by the calculation of the profile similarity measure D between the 2005/2009 and 2013 SOEP sample cluster solutions, which yielded highest similarity for the undercontroler (D = 0.27) and reserved (D = 0.36) personality types, followed by the vulnerable-resilient (D = 0.37), overcontroler (D = 0.44) and resilient (D = 0.50) personality types. Substantial agreement was confirmed by the values of the Rand index (.84) and Cohen’ Kappa (.70) whereas the Hubert Arabie adjusted Rand Index (.58) indicated moderate agreement for the comparison between the kmeans cluster solution for the 2013 SOEP sample and the cluster allocation with an SVM classifier trained on the 2005 and 2009 kmeans cluster solution.
Predictive validity
In view of the aforementioned criticisms that (a) predicting dimensional variables will mathematically favor dimensional personality description models, and (b) using dichotomous predictors will necessarily provide less explanation of variance than a model using five continuous predictors, we used the profile similarity measure D [ 76 ] instead of dichotomous dummy variables accounting for the prototype membership. Correlations between the inversed Euclidean similarity measure D to the personality types and patience, risk-taking, spontaneity/impulsivity, locus of control, affective wellbeing, self-esteem and health are depicted in Table 4 .
https://doi.org/10.1371/journal.pone.0244849.t004
Patience had the highest association with the reserved personality type (r = .19, p < .001). The propensity to risky behavior, e.g. while driving (r = .17, p < .001), in financial matters (r = .17, p < .001) or in health decisions (r = .13, p < .001) was most highly correlated with the undercontroller personality type. This means that the more similar the Big-Five profile to the above-depicted undercontroller personality prototype, the higher the propensity for risky behavior. The average correlation across all three risk propensity scales with the undercontroller personality type is r = .21, with p < .001. This is in line with the postulations by Block and Block and subsequent replications by Caspi et al. [ 19 , 48 ], Robins et al. [ 1 ] and Herzberg [ 33 ] about the undercontroller personality type. Spontaneity/impulsivity showed the highest correlation with the overcontroller personality type (r = -.18, p<0.001). This is also in accordance with Block and Block, who described this type as being non-impulsive and appearing constrained and inhibited in actions and emotional expressivity.
Concerning locus of control, proximity to the resilient personality profile had the highest correlation with internal locus of control (r = .25, p < .001), and in contrast, the more similar the individual Big-Five profile was to the overcontroller personality type, the higher the propensity for external allocation of control (r = .22, p < .001). This is not only in line with Block and Block’s postulations that the resilient personality type has a good repertoire of coping behavior and therefore perceives most situations as “manageable” as well as with the findings by [ 33 ], but is also in accordance with findings regarding the construct and development of resilience [ 77 , 78 ].
Also in line with the predictions of Block and Block and replicating the findings of Herzberg [ 33 ], self-esteem was correlated the highest with the resilient personality profile similarity (r = .33, p < .001), second highest with the reserved personality profile proximity (r = .15, p < .001), and negatively correlated with the overcontroller personality type (r = -.27, p < .001).
This pattern also applies to affective and cognitive wellbeing as well as physical and mental health measured by the SF-12. Affective wellbeing was correlated the highest with similarity to the resilient personality type (r = .27, p < .001), and second highest with the reserved personality type (r = .23, p < .001). The overcontroller personality type, in contrast, showed a negative correlation with affective (r = -.16, p < .001) and cognitive (r = -21, p < .001) wellbeing. Concerning health, a remarkable finding is that lack of physical health impairment correlated the highest with the resilient personality profile similarity (p = -.23, p < .001) but lack of mental health impairment correlated the highest with the reserved personality type (r = -.15, p < .001). The highest correlation with mental health impairments (r = .11, p < .001), as well as physical health impairments (r = .16, p < .001) was with the overcontroller personality profile similarity. It is striking that although the undercontroller personality profile similarity was associated with risky health behavior, it had a negative association with health impairment measures, in contrast to the overcontroller personality type, which in turn had no association with risky health behavior. This result is in line with the link of internalizing and externalizing behavior with the overcontroller and undercontroller types [ 79 ], respectively. Moreover, it is also in accordance with the association of internalizing problems with somatic symptoms and/or symptoms of depressiveness and anxiety [ 80 ].
A further noteworthy finding is that these associations cannot be solely explained by the high neuroticism of the overcontroller personality type, as the vulnerable-resilient type showed a similar level of neuroticism but no correlation with self-esteem, the opposite correlation with impulsivity, and far lower correlations with health measures or locus of control. The vulnerable-resilient type showed also a remarkable distinction to the other types concerning the correlations to wellbeing. While for all other types, the direction and significance of the correlations to affective and cognitive measures of wellbeing were alike, the vulnerable-resilient type only had a significant negative correlation to affective wellbeing while having no significant correlation to measures of cognitive wellbeing.
To provide an overview of the particular associations of the Big Five values with all of the above-mentioned behavior and personality measures, Table 5 shows the bivariate correlations.
https://doi.org/10.1371/journal.pone.0244849.t005
Investigating the direction of the correlation and the relativity of each value to each other row-wise reveals, to some extent, a clear resemblance with the z-scores of the personality types shown in Fig 3 . Correlation profiles of risk taking, especially the facet risk-taking in health issues and locus of control, clearly resemble the undercontroller personality profile (negative correlations with openness and conscientiousness, positive but lower correlations with extraversion and openness). Patience had negative correlations with neuroticism and extraversion, and positive correlations with openness and conscientiousness, which in turn resembles the z-score profile of the reserved personality profile. Spontaneity/impulsivity had moderate to high positive correlations with extraversion and openness, and low negative correlations with openness and neuroticism, which resembles the inverse of the overcontroller personality profile. Self-esteem as well as affective and cognitive wellbeing correlations with the Big Five clearly resemble the resilient personality profile: negative correlations with neuroticism, and positive correlations with extraversion, openness, openness and conscientiousness. Inspecting the SF-12 health correlation, in terms of both physical and mental health, reveals a resemblance to the inversed resilient personality profile (high correlation with neuroticism, low correlation with extraversion, openness, openness and conscientiousness, as well as a resemblance with the overcontroller profile (positive correlation with neuroticism, negative correlation with extraversion).
https://doi.org/10.1371/journal.pone.0244849.g003
On the variable level, neuroticism had the highest associations with almost all of the predicted variables, with the exception of impulsivity, which was mainly correlated with extraversion and openness. It is also evident that all variables in question here are correlated with three or more Big Five traits. This can be seen as support for hypothesis that the concept of personality prototypes has greater utility than the variable-centered approach in understanding or predicting more complex psychological constructs that are linked to two or more Big Five traits.
The goal of this study was to combine different methodological approaches while overcoming the shortcomings of previous studies in order to answer the questions whether there are replicable personality types, how many of them there are, and how they relate to Big Five traits and other psychological and health-related constructs. The results revealed a robust five personality type model, which was able to significantly predict all of the psychological constructs in question longitudinally. Predictions from previous findings connecting the predicted variables to the particular Big Five dimensions underlying the personality type model were confirmed. Apparently, the person-centered approach to personality description has the most practical utility when predicting behavior or personality correlates that are connected to more than one or two of the Big Five traits such as self-esteem, locus of control and wellbeing.
This study fulfils all three criteria specified by von Eye & Bogat [ 81 ] regarding person-oriented research and considers the recommendations regarding sample size and composition by Herzberg and Roth [ 30 ]. The representative and large sample was analyzed under the assumption that it was drawn from more than one population (distinct personality types). Moreover, several external and internal cluster validity criteria were taken into account in order to validate the groupings generated by three different cluster algorithms, which were chosen to represent broad ranges of clustering techniques [ 60 , 82 ]. The Ward followed by K-means procedure covers hierarchical as well as divisive partitioning (crisp) clustering, the latent profile algorithm covers density-based clustering with probabilistic models and information theoretic validation (AIC, BIC), and spectral clustering represents graph theoretic as well as kernel-based non-linear clustering techniques. The results showed a clear superiority of the five-cluster solution. Interpreting this grouping based on theory revealed a strong concordance with personality types found in previous studies, which we could ascertain both in absolute mean values and in the Euclidean distances to mean cluster z-scores extracted from 19 previous studies. As no previous study on personality types used that many external and internal cluster validity indices and different clustering algorithms on a large data set of this size, the present study provides substantial support for the personality type theory postulating the existence of resilient, undercontroller, overcontroller, vulnerable-resilient and reserved personality types, which we will refer to with RUO-VR subsequently. Further, our findings concerning lower validity of the LPA cluster solutions compared to the k-means and spectral cluster solutions suggest that clustering techniques based on latent models are less suited for the BFI-S data of the SOEP sample than iterative and deterministic methods based on the k-means procedure or non-linear kernel or graph-based methods. Consequently, the substance of the clustering results by Specht et. al. [ 36 ], which applied latent profile analysis on the SOEP sample, may therefore be limited.
But the question, if the better validity values of the k-means and spectral clustering techniques compared to the LPA indicate a general superiority of these algorithms, a superiority in the field of personality trait clustering or only a superiority in clustering this specific personality trait assessment (BFI-S) in this specific sample (SOEP), remains subject to further studies on personality trait clustering.
When determining the longitudinal predictive validity, the objections raised by Asendorpf [ 53 ] concerning the direct comparison of person-oriented vs. variable-oriented personality descriptions were incorporated by using continuous personality type profile similarity based on Cronbach and Gleser [ 75 ] instead of dichotomous dummy variables as well as by predicting long-term instead of cross-sectionally assessed variables. Using continuous profile similarity variables also resolves the problem that potentially important information about members of the same class is lost in categorical personality descriptions [ 15 , 53 , 83 ]. Predictions regarding the association of the personality types with the assessed personality and behavior correlates, including risk propensity, impulsivity, self-esteem, locus of control, patience, cognitive and affective wellbeing as well as health measures, were confirmed.
Overcontrollers showed associations with lower spontaneity/impulsivity, with lower mental and physical health, and lower cognitive as well as affective wellbeing. Undercontrollers were mainly associated with higher risk propensity and higher impulsive behavior. These results can be explained through the connection of internalizing and externalizing behavior with the overcontroller and undercontroller types [ 5 – 7 , 78 ] and further with the connection of internalizing problems with somatic symptoms and/or symptoms of depressiveness and anxiety [ 79 ]. The dimensions or categories of internalizing and externalizing psychopathology have a long tradition in child psychopathology [ 84 , 85 ] and have been subsequently replicated in adult psychopathology [ 86 , 87 ] and are now basis of contemporary approaches to general psychopathology [ 88 ]. A central proceeding in this development is the integration of (maladaptive) personality traits into the taxonomy of general psychopathology. In the current approach, maladaptive personality traits are allocated to psychopathology spectra, such as the maladaptive trait domain negative affectivity to the spectrum of internalizing disorders. However, the findings of this study suggests that not specific personality traits are intertwined with the development or the occurrence of psychopathology but specific constellations of personality traits, in other words, personality profiles. This hypothesis is also supported by the findings of Meeus et al. [ 8 ], which investigated longitudinal transitions from one personality type to another with respect to symptoms of generalized anxiety disorder. Transitions from resilient to overcontroller personality profiles significantly predicted higher anxiety symptoms while the opposite was found for transitions from overcontroller to resilient personality profiles.
The resilient personality type had the strongest associations with external locus of control, higher patience, good health and positive wellbeing. This not only confirms the characteristics of the resilient type already described by Block & Block [ 18 ] and subsequently replicated, but also conveys the main characteristics of the construct of resilience itself. While the development of resiliency depends on the quality of attachment experiences in childhood and youth [ 89 ], resiliency in adulthood seems to be closely linked to internal locus of control, self-efficacy and self-esteem. In other words, the link between secure attachment experiences in childhood and resiliency in adulthood seems to be the development of a resilient personality trait profile. Seen the other way around, the link between traumatic attachment experiences or destructive environmental factors and low resiliency in adulthood may be, besides genetic risk factors, the development of personality disorders [ 90 ] or internalizing or externalizing psychopathology [ 91 ]. Following this thought, the p-factor [ 92 ], i.e. a general factor of psychopathology, may be an index of insufficient resilience. Although from the viewpoint of personality pathology, having a trait profile close to the resilient personality type may be an index of stable or good personality structure [ 93 ], i.e. personality functioning [ 94 ], which, though being consistently associated with general psychopathology and psychosocial functioning, should not be confused with it [ 95 ].
The reserved personality type had the strongest associations with higher patience as well as better mental health. The vulnerable-resilient personality type showed low positive correlations with spontaneity/impulsivity and low negative correlations with patience as well as health and affective wellbeing.
Analyzing the correlations of the dimensional Big Five values with the predicted variables revealed patterns similar to the mean z-scores of the personality types resilient, overcontrollers, undercontrollers and reserved. Most variables had a low to moderate correlation with just one personality profile similarity, while having at least two or three low to moderate correlations with the Big Five measures. This can be seen as support for the argument of Chapman [ 82 ] and Asendorpf [ 15 , 53 ] that personality types have more practical meaning in the prediction of more complex correlates of human behavior and personality such as mental and physical health, wellbeing, risk-taking, locus of control, self-esteem and impulsivity. Our findings further underline that the person-oritented approach may better be suited than variable-oriented personality descriptions to detect complex trait interactions [ 40 ]. E.g. the vulnerable-resilient and the overcontroller type did not differ in their high average neuroticism values, while differing in their correlations to mental and somatic health self-report measures. It seems that high neuroticism is far stronger associated to lower mental and physical health as well as wellbeing if it occurs together with low extraversion and low openness as seen in the overcontroller type. This differential association between the Big-Five traits also affects the correlation between neuroticism and self-esteem or locus of control. Not differing in their average neuroticism value, the overcontroller personality profile had moderate associations with low self-esteem and external locus of control while the vulnerable-resilient personality profile did only show very low or no association. Further remarkable is that the vulnerable-resilient profile similarity had no significant correlation with measures of cognitive wellbeing while being negatively correlated with affective wellbeing. This suggests that individuals with a Big-Five personality profile similar to the vulnerable-resilient prototype seem not to perceive impairments in their wellbeing, at least on a cognitive layer, although having high z-values in neuroticism. Another explanation for this discrepancy as well as for the lack of association of the vulnerable-resilient personality profile to low self-esteem and external locus of control though having high values in neuroticism could be found in the research on the construct of resilience. Personalities with high neuroticism values but stable self-esteem, internal locus of control and above average agreeableness and extraversion values may be the result of the interplay of multiple protective factors (e.g. close bond with primary caregiver, supportive teachers) with risk factors (e.g. parental mental illness, poverty). The development of a resilient personality profile with below average neuroticism values, on the other hand, may be facilitated if protective factors outweigh the risk factors by a higher ratio.
An interesting future research question therefore concerns to what extent personality types found in this study may be replicated using maladaptive trait assessments according to DSM-5, section III [ 96 ] or the ICD-11 personality disorder section [ 97 ] (for a comprehensive overview on that topic see e.g. [ 98 ]). As previous studies showed that both DSM-5 [ 99 ] and ICD-11 [ 100 ] maladaptive personality trait domains may be, to a large extent, conceptualized as maladaptive variants of Big Five traits, it is highly likely that also maladaptive personality trait domains align around personality prototypes and that the person-oriented approach may amend the research field of personality pathology [ 101 ].
Taken together, the findings of this study connect the variable centered approach of personality description, more precisely the Big Five traits, through the concept of personality types to constructs of developmental psychology (resiliency, internalizing and externalizing behavior and/or problems) as well as clinical psychology (mental health) and general health assessed by the SF-12. We could show that the distribution of Big Five personality profiles, at least in the large representative German sample of this study, aggregates around five prototypes, which in turn have distinct associations to other psychological constructs, most prominently resilience, internalizing and externalizing behavior, subjective health, patience and wellbeing.
Limitations
Several limitations of the present study need to be considered: One problem concerns the assessment of patience, self-esteem and impulsivity. From a methodological perspective, these are not suitable for the assessment of construct validity as they were assessed with only one item. A further weakness is the short Big Five inventory with just 15 items. Though showing acceptable reliability, 15 items are more prone to measurement errors than measures with more items and only allow a very broad assessment of the 5 trait domains, without information on individual facet expressions. A more big picture question is if the Big Five model is the best way to assess personality in the first place. A further limitation concerns the interpretation of the subjective health measures, as high neuroticism is known to bias subjective health ratings. But the fact that the vulnerable-resilient and the overcontroler type had similar average neuroticism values but different associations with the subjective health measures speaks against a solely neuroticism-based bias driven interpretation of the associations of the self-reported health measures with the found personality clusters. Another limitation is the correlation between the personality type similarities: As they are based on Euclidean distances and the cluster algorithms try to maximize the distances between the cluster centers, proximity to one personality type (that is the cluster mean) logically implies distance from the others. In the case of the vulnerable-resilient and the resilient type, the correlation of the profile similarities is positive, as they mainly differ on only one dimension (neuroticism). These high correlations between the profile similarities prevents or diminishes, due to the emerging high collinearity, the applicability of general linear models, i.e. regression to calculate the exact amount of variance explained by the profile similarities.
The latter issue could be bypassed by assessing types and dimensions with different questionnaires, i.e. as in Asendorpf [ 15 ] with the California Child Q-set to determine the personality type and the NEO-FFI for the Big Five dimensions. Another possibility is to design a new questionnaire based on the various psychological constructs that are distinctly associated with each personality type, which is probably a subject for future person-centered research.
Acknowledgments
The data used in this article were made available by the German Socio-Economic Panel (SOEP, Data for years 1984–2015) at the German Institute for Economic Research, Berlin, Germany. However, the findings and views reported in this article are those of the authors. To ensure the confidentiality of respondents’ information, the SOEP adheres to strict security standards in the provision of SOEP data. The data are reserved exclusively for research use, that is, they are provided only to the scientific community. All users, both within the EEA (and Switzerland) and outside these countries, are required to sign a data distribution contract.
- View Article
- PubMed/NCBI
- Google Scholar
- 21. Blashfield RK, Aldenderfer MS. The methods and problems of cluster analysis. Handbook of multivariate experimental psychology: Springer; 1988. p. 447–73. https://doi.org/10.1002/1097-4679(198801)44:1<47::aid-jclp2270440110>3.0.co;2-r pmid:3343363
- 48. Caspi A. Personality development across the life course (In Damon W.& Eisenberg N.(Eds.). Handbook of child psychology: Social, emotional, and personality development (Vol. 3, pp. 311–388). Hoboken. NJ: Wiley; 1998.
- 59. Bacher J, Pöge A, Wenzig K. Clusteranalyse: Anwendungsorientierte Einführung in Klassifikationsverfahren: Oldenbourg Verlag; 2010.
- 61. Cristianini N, Shawe-Taylor J. An introduction to support vector machines and other kernel-based learning methods: Cambridge university press; 2000.
- 66. Halkidi M, Vazirgiannis M, editors. Clustering validity assessment: Finding the optimal partitioning of a data set. Data Mining, 2001 ICDM 2001, Proceedings IEEE International Conference on; 2001: IEEE.
- 93. Kernberg OF. Borderline Personality Disorder and Borderline Personality Organization: Psychopathology and Psychotherapy. Handbook of Personality Disorders: Theory and Practice. Hoboken, NJ, US: John Wiley & Sons Inc; 2004. p. 92–119.
- 96. Diagnostic and statistical manual of mental disorders: DSM-5, (2013).
- 97. ICD-11 Clinical Descriptions and Diagnostic Guidelines for Mental and Behavioural Disorders. World Health Organisation, (2019).
- Research article
- Open access
- Published: 05 May 2020
Personality traits, emotional intelligence and decision-making styles in Lebanese universities medical students
- Radwan El Othman 1 ,
- Rola El Othman 2 ,
- Rabih Hallit 1 , 3 , 4 na1 ,
- Sahar Obeid 5 , 6 , 7 na1 &
- Souheil Hallit 1 , 5 , 7 na1
BMC Psychology volume 8 , Article number: 46 ( 2020 ) Cite this article
54k Accesses
30 Citations
16 Altmetric
Metrics details
This study aims to assess the impact of personality traits on emotional intelligence (EI) and decision-making among medical students in Lebanese Universities and to evaluate the potential mediating role-played by emotional intelligence between personality traits and decision-making styles in this population.
This cross-sectional study was conducted between June and December 2019 on 296 general medicine students.
Higher extroversion was associated with lower rational decision-making style, whereas higher agreeableness and conscientiousness were significantly associated with a higher rational decision-making style. More extroversion and openness to experience were significantly associated with a higher intuitive style, whereas higher agreeableness and conscientiousness were significantly associated with lower intuitive style. More agreeableness and conscientiousness were significantly associated with a higher dependent decision-making style, whereas more openness to experience was significantly associated with less dependent decision-making style. More agreeableness, conscientiousness, and neuroticism were significantly associated with less spontaneous decision-making style. None of the personality traits was significantly associated with the avoidant decision-making style. Emotional intelligence seemed to fully mediate the association between conscientiousness and intuitive decision-making style by 38% and partially mediate the association between extroversion and openness to experience with intuitive decision-making style by 49.82 and 57.93% respectively.
Our study suggests an association between personality traits and decision-making styles. The results suggest that EI showed a significant positive effect on intuitive decision-making style and a negative effect on avoidant and dependent decision-making styles. Additionally, our study underlined the role of emotional intelligence as a mediator factor between personality traits (namely conscientiousness, openness, and extroversion) and decision-making styles.
Peer Review reports
Decision-making is a central part of daily interactions; it was defined by Scott and Bruce in 1995 as «the learned habitual response pattern exhibited by an individual when confronted with a decision situation. It is not a personality trait, but a habit-based propensity to react in a certain way in a specific decision context» [ 1 ]. Understanding how people make decisions within the moral domain is of great importance theoretically and practically. Its theoretical value is related to the importance of understanding the moral mind to further deepen our knowledge on how the mind works, thus understanding the role of moral considerations in our cognitive life. Practically, this understanding is important because we are highly influenced by the moral decisions of people around us [ 2 ]. According to Scott and Bruce (1995), there are five distinct decision-making styles (dependent, avoidant, spontaneous, rational, intuitive) [ 1 ] and each individuals’ decision-making style has traits from these different styles with one dominant style [ 3 ].
The dependent decision-making style can be regarded as requiring support, advice, and guidance from others when making decisions. Avoidant style is characterized by its tendency to procrastinate and postpone decisions if possible. On the other hand, spontaneous decision-making style is hallmarked by making snap and impulsive decisions as a way to quickly bypass the decision-making process. In other words, spontaneous decision-makers are characterized by the feeling of immediacy favoring to bypass the decision-making process rapidly without employing much effort in considering their options analytically or relying on their instinct. Rational decision-making style is characterized by the use of a structured rational approach to analyze information and options to make decision [ 1 ]. In contrast, intuitive style is highly dependent upon premonitions, instinct, and feelings when it comes to making decisions driving focus toward the flow of information rather than systematic procession and analysis of information, thus relying on hunches and gut feelings. Several studies have evaluated the factors that would influence an individual’s intuition and judgment. Rand et al. (2016) discussed the social heuristics theory and showed that women and not men tend to internalize altruism _ the selfless concern for the well-being of others_ in their intuition and thus in their intuitive decision-making process [ 4 ]. Additionally, intuitive behavior honesty is influenced by the degree of social relationships with individuals affected by the outcome of our decision: when dishonesty harms abstract others, intuition promotion causes more dishonesty. On the contrary, when dishonesty harms concrete others, intuition promotion has no significant effect on dishonesty. Hence, the intuitive appeal of pro-sociality may cancel out the intuitive selfish appeal of dishonesty [ 5 ]. Moreover, the decision-making process and styles have been largely evaluated in previous literature. Greene et al. (2008) and Rand (2016) showed that utilitarian moral judgments aiming to minimize cost and maximize benefits across concerned individuals are driven by controlled cognitive process (i.e. rational); whereas, deontological moral judgments _where rights and duties supersede utilitarian considerations_ are dictated by an automatic emotional response (e.g. spontaneous decision-making) [ 6 , 7 ]. Trémolière et al. (2012) found that mortality salience makes people less utilitarian [ 8 ].
Another valuable element influencing our relationships and career success [ 9 ] is emotional intelligence (EI) a cardinal factor to positive patient experience in the medical field [ 10 ]. EI was defined by Goleman as «the capacity of recognizing our feelings and those of others, for motivating ourselves, and for managing emotions both in us and in our relationships» [ 11 ]. Hence, an important part of our success in life nowadays is dependent on our ability to develop and preserve social relationships, depict ourselves positively, and control the way people descry us rather than our cognitive abilities and traditional intelligence measured by IQ tests [ 12 ]. In other words, emotional intelligence is a subtype of social intelligence involving observation and analyses of emotions to guide thoughts and actions. Communication is a pillar of modern medicine; thus, emotional intelligence should be a cornerstone in the education and evaluation of medical students’ communication and interpersonal skills.
An important predictor of EI is personality [ 13 ] defined as individual differences in characteristic patterns of thinking, feeling and behaving [ 14 ]. An important property of personality traits is being stable across time [ 15 ] and situations [ 16 ], which makes it characteristic of each individual. One of the most widely used assessment tools for personality traits is the Five-Factor model referring to «extroversion, openness to experience, agreeableness, conscientiousness, neuroticism». In fact, personality traits have an important impact on individuals’ life, students’ academic performance [ 17 ] and decision-making [ 18 ].
Extroversion is characterized by higher levels of self-confidence, positive emotions, enthusiasm, energy, excitement seeking, and social interactions. Openness to experience individuals are creative, imaginative, intellectually curious, impulsive, and original, open to new experiences and ideas [ 19 ]. Agreeableness is characterized by cooperation, morality, sympathy, low self-confidence, high levels of trust in others, and tend to be happy and satisfied because of their close interrelationships [ 19 ]. Conscientiousness is characterized by competence, hard work, self-discipline, organization, strive for achievement and goal orientation [ 20 ] with a high level of deliberation making conscientious individuals capable of analyzing the pros and cons of a given situation [ 21 ]. Neuroticism is characterized by anxiety, anger, insecurity, impulsiveness, self-consciousness,and vulnerability [ 20 ]. High neurotic individuals have higher levels of negative affect, are easily irritated, and more likely to turn to inappropriate coping responses, such as interpersonal hostility [ 22 ].
Multiple studies have evaluated the impact of personality traits on decision-making styles. Narooi and Karazee (2015) studied personality traits, attitude to life, and decision-making styles among university students in Iran [ 23 ]. They deduced the presence of a strong relationship between personality traits and decision-making styles [ 23 ]. Riaz and Batool (2012) evaluated the relationship between personality traits and decision-making among a group of university students (Fig. 1 ). They concluded that «15.4 to 28.1% variance in decision-making styles is related to personality traits» [ 24 ]. Similarly, Bajwa et al. (2016) studied the relationship between personality traits and decision-making among students. They concluded that conscientiousness personality trait is associated with rational decision-making style [ 25 ]. Bayram and Aydemir (2017) studied the relationship between personality traits and decision-making styles among a group of university students in Turkey [ 26 ]. Their work yielded to multiple conclusion namely a significant association between rational and intuitive decision-making styles and extroversion, openness to experience, conscientiousness, and agreeableness personality traits [ 26 ]. The dependent decision-making style had a positive relation with both neuroticism and agreeableness. The spontaneous style had a positive relation with neuroticism and significant negative relation with agreeableness and conscientiousness. Extroversion personality traits had a positive effect on spontaneous style. Agreeableness personality had a positive effect on the intuitive and dependent decision-making style. Conscientiousness personality had a negative effect on avoidant and spontaneous decision-making style and a positive effect on rational style. Neuroticism trait had a positive effect on intuitive, dependent and spontaneous decision-making style. Openness to experience personality traits had a positive effect on rational style [ 26 ].
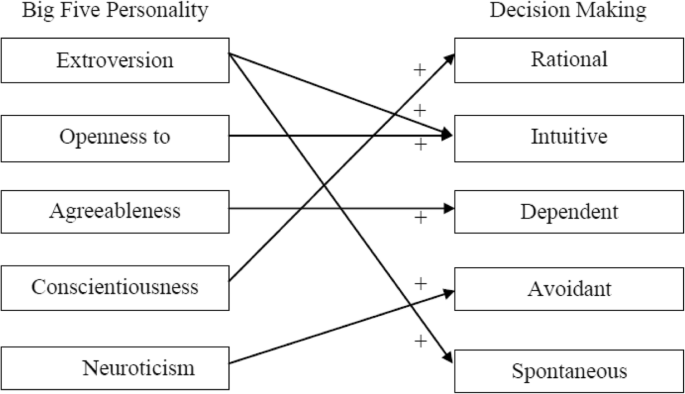
Schematic representation of the effect of the big five personality types on decision-making styles [ 24 ]
Furthermore, several studies have evaluated the relationship between personality traits and emotional intelligence. Dawda and Hart (2000) found a significant relationship between emotional intelligence and all Big Five personality traits [ 27 ]. Day and al. (2005) found a high correlation between emotional intelligence and extroversion and conscientiousness personality traits [ 28 ]. A study realized by Avsec and al. (2009) revealed that emotional intelligence is a predictor of the Big Five personality traits [ 29 ]. Alghamdi and al. (2017) investigated the predictive role of EI on personality traits among university advisors in Saudi Arabia. They found that extroversion, agreeableness, and openness to experience emerged as significant predictors of EI. The study also concluded that conscientiousness and neuroticism have no impact on EI [ 13 ].
Nonetheless, decision-making is highly influenced by emotion making it an emotional process. The degree of emotional involvement in a decision may influence our choices [ 30 ] especially that emotions serve as a motivational process for decision-making [ 31 ]. For instance, patients suffering from bilateral lesions of the ventromedial prefrontal cortex (interfering with normal processing of emotional signals) develop severe impairments in personal and social decision-making despite normal cognitive capabilities (intelligence and creativity); highlighting the guidance role played by emotions in the decision-making process [ 32 ]. Furthermore, EI affects attention, memory, and cognitive intelligence [ 33 , 34 ] with higher levels of EI indicating a more efficient decision-making [ 33 ]. In one study, Khan and al. concluded that EI had a significant positive effect on rational and intuitive decision-making styles and negative effect on dependent and spontaneous decision-making styles among a group of university students in Pakistan [ 35 ].
This study aims to assess the impact of personality traits on both emotional intelligence and decision-making among medical students in Lebanese Universities and to test the potential mediating role played by emotional intelligence between personality and decision-making styles in this yet unstudied population to our knowledge. The goal of the present research is to evaluate the usefulness of implementing such tools in the selection process of future physicians. It also aimed at assessing the need for developing targeted measures, aiming to ameliorate the psychosocial profile of Lebanese medical students, in order to have a positive impact on patients experience and on medical students’ career success.
Study design
This cross-sectional study was conducted between June and December 2019. A total of 296 participants were recruited from all the 7 faculties of medicine in Lebanon. Data collection was done through filling an anonymous online or paper-based self-administered English questionnaire upon the participant choice. All participants were aware of the purpose of the study, the quality of data collected and gave prior informed consent. Participation in this study was voluntary and no incentive was given to the participants. All participants were General medicine students registered as full-time students in one of the 7 national schools of medicine aged 18 years and above regardless of their nationality. The questionnaire was only available in English since the 7 faculties of medicine in Lebanon require a minimum level of good English knowledge in their admission criteria. A pilot test was conducted on 15 students to check the clarity of the questionnaire. To note that these 15 questionnaires related data was not entered in the final database. The methodology used in similar to the one used in a previous paper [ 36 ]
Questionnaire and variables
The questionnaire assessed demographic and health characteristics of participants, including age, gender, region, university, current year in medical education, academic performance (assessed using the current cumulative GPA), parental highest level of education, and health questions regarding the personal history of somatic, and psychiatric illnesses.
The personality traits were evaluated using the Big Five Personality Test, a commonly used test in clinical psychology. Since its creation by John, Donahue, and Kentle (1991) [ 37 ], the five factor model was widely used in different countries including Lebanon [ 38 ]; it describes personality in terms of five board factors: extroversion, openness to experience, agreeableness, conscientiousness and neuroticism according to an individual’s response to a set of 50 questions on a 5-point Likert scale: 1 (disagree) to 5 (agree). A score for each personality trait is calculated in order to determine the major trait(s) in an individual personality (i.e. the trait with the highest score). The Cronbach’s alpha values were as follows: total scale (0.885), extroversion (0.880), openness to experience (0.718), agreeableness (0.668), conscientiousness (0.640), and neuroticism (0.761).
Emotional intelligence was assessed using the Quick Emotional Intelligence Self-Assessment scale [ 38 ]. The scale is divided into four domains: «emotional alertness, emotional control, social-emotional awareness, and relationship management». Each domain is composed of 10 questions, with answers measured on a 5-point Likert scale: 0 (never) to 4 (always). Higher scores indicate higher emotional intelligence [ 38 ] (α Cronbach = 0.950).
The decision-making style was assessed using the Scott and Bruce General Decision-Making Style Inventory commonly used worldwide since its creation in 1995 for this purpose [ 1 ]. The inventory consists of 25 questions answered according to a 5-point Likert scale: 1 (strongly disagree) to 5 (strongly agree) intended to evaluate the importance of each decision-making style among the 5 styles proposed by Scott and Bruce: dependent, avoidant, spontaneous, rational and intuitive. The score for each decision-making style is computed in order to determine the major style for each responder (α Cronbach total scale = 0.744; α Cronbach dependent style = 0.925; α Cronbach avoidant style = 0.927; α Cronbach spontaneous style = 0.935; α Cronbach rational style = 0.933; α Cronbach intuitive style = 0.919).
Sample size calculation
The Epi info program (Centers for Disease Control and Prevention (CDC), Epi Info™) was employed for the calculation of the minimal sample size needed for our study, with an acceptable margin of error of 5% and an expected variance of decision-making styles that is related to personality types estimated by 15.4 to 28.1% [ 24 ] for 5531 general medicine student in Lebanon [ 39 ]. The result showed that 294 participants are needed.
Statistical analysis
Statistical Package for Social Science (SPSS) version 23 was used for the statistical analysis. The Student t-test and ANOVA test were used to assess the association between each continuous independent variable (decision-making style scores) and dichotomous and categorical variables respectively. The Pearson correlation test was used to evaluate the association between two continuous variables. Reliability of all scales and subscales was assessed using Cronbach’s alpha.
Mediation analysis
The PROCESS SPSS Macro version 3.4, model four [ 40 ] was used to calculate five pathways (Fig. 2 ). Pathway A determined the regression coefficient for the effect of each personality trait on emotional intelligence, Pathway B examined the association between EI and each decision-making style, independent of the personality trait, and Pathway C′ estimated the total and direct effect of each personality trait on each decision-making style respectively. Pathway AB calculated the indirect intervention effects. To test the significance of the indirect effect, the macro generated bias-corrected bootstrapped 95% confidence intervals (CI) [ 40 ]. A significant mediation was determined if the CI around the indirect effect did not include zero [ 40 ]. The covariates that were included in the mediation model were those that showed significant associations with each decision-making style in the bivariate analysis.
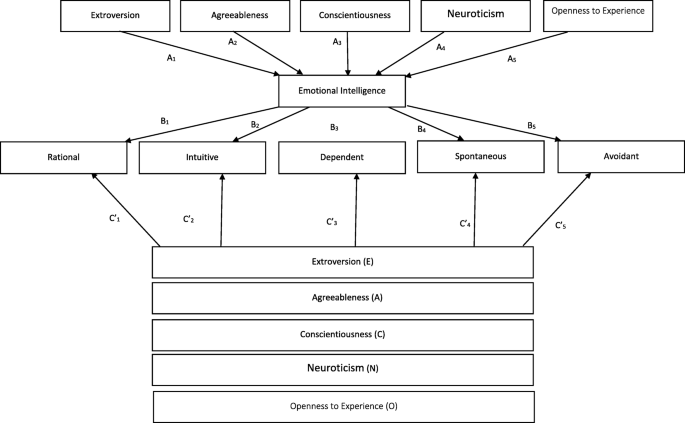
Summary of the pathways followed during the mediation analysis
Sociodemographic and other characteristics of the participants
The mean age of the participants was 22.41 ± 2.20 years, with 166 (56.1%) females. The mean scores of the scales used were as follows: emotional intelligence (108.27 ± 24.90), decision-making: rationale style (13.07 ± 3.17), intuitive style (16.04 ± 3.94), dependent style (15.53 ± 4.26), spontaneous style (13.52 ± 4.22), avoidant style (12.44 ± 4.11), personality trait: extroversion (21.18 ± 8.96), agreeableness (28.01 ± 7.48), conscientiousness (25.20 ± 7.06), neuroticism (19.29 ± 8.94) and openness (27.36 ± 7.81). Other characteristics of the participants are summarized in Table 1 .
Bivariate analysis
Males vs females, having chronic pain compared to not, originating from South Lebanon compared to other governorates, having an intermediate income compared to other categories, those whose mothers had a primary/complementary education level and those whose fathers had an undergraduate diploma vs all other categories had higher mean rationale style scores. Those fathers, who had a postgraduate diploma, had a higher mean intuitive style scores compared to all other education levels. Those who have chronic pain compared to not and living in South Lebanon compared to other governorates had higher dependent style scores. Those who have chronic pain compared to not, those who take medications for a mental illness whose mothers had a primary/complementary education level vs all other categories and those whose fathers had a postgraduate diploma vs all other categories had higher spontaneous style scores (Table 2 ).
Higher agreeableness and conscientiousness scores were significantly associated with higher rational style scores, whereas higher extroversion and neuroticism scores were significantly associated with lower rational style scores. Higher extroversion, openness and emotional intelligence scores were significantly associated with higher intuitive scores, whereas higher agreeableness, conscientiousness and neuroticism scores were significantly associated with lower intuitive style scores. Higher agreeableness and conscientiousness were associated with higher dependent style scores, whereas higher openness and emotional intelligence scores were significantly associated with lower dependent styles scores. Higher agreeableness, conscientiousness, neuroticism, and emotional intelligence scores were significantly associated with lower spontaneous style scores. Finally, higher extroversion, neuroticism and emotional intelligence scores were significantly associated with lower avoidant style scores (Table 3 ).
Post hoc analysis: rationale style: governorate (Beirut vs Mount Lebanon p = 0.022; Beirut vs South p < 0.001; Mount Lebanon vs South p = 0.004; South vs North p = 0.001; South vs Bekaa p = 0.047); monthly income (intermediate vs high p = 0.024); mother’s educational level (high school vs undergraduate diploma p = 0.048); father’s education level (undergraduate vs graduate diploma p = 0.01).
Intuitive style: father’s education level (high school vs postgraduate diploma p = 0.046).
Dependent style: governorate (Beirut vs Mount Lebanon p = 0.006; Beirut vs South p = 0.003);
Avoidant style: mother’s educational level (high school vs undergraduate diploma p = 0.008; undergraduate vs graduate diploma p = 0.004; undergraduate vs postgraduate diploma p = 0.001).
Mediation analysis was run to check if emotional intelligence would have a mediating role between each personality trait and each decision-making style, after adjusting overall covariates that showed a p < 0.05 with each decision-making style in the bivariate analysis.
Rational decision-making style (Table 4 , model 1)
Higher extroversion was significantly associated with higher EI, b = 0.91, 95% BCa CI [0.60, 1.23], t = 5.71, p < 0.001 (R2 = 0.31). Higher extroversion was significantly associated with lower rational decision-making even with EI in the model, b = − 0.06, 95% BCa CI [− 0.11, − 0.02], t = − 2.81, p = 0.003; EI was not significantly associated with rational decision-making, b = 0.02, 95% BCa CI [− 0.0003, 0.03], t = 1.93, p = 0.054 (R2 = 0.29). When EI was not in the model, higher extroversion was significantly associated with lower rational decision-making, b = − 0.05, 95% BCa CI [− 0.09, − 0.01], t = − 2.43, p = 0.015 (R2 = 0.28). The mediating effect of EI was 21.22%.
Higher agreeableness was not significantly associated with EI, b = − 0.05, 95% BCa CI [− 0.40, 0.31], t = − 0.26, p = 0.798 (R2 = 0.31). Higher agreeableness was significantly associated with higher rational decision-making style even with EI in the model, b = 0.07, 95% BCa CI [0.02, 0.11], t = 2.89, p = 0.004; EI was not significantly associated with the rational decision-making, b = 0.01, 95% BCa CI [− 0.0003, 0.03], t = 1.92, p = 0.055 (R2 = 0.29). When EI was not in the model, higher agreeableness was significantly associated with higher rational decision-making, b = 0.07, 95% BCa CI [0.02, 0.11], t = 2.86, p = 0.004 (R2 = 0.28). The mediating effect of EI was 0.10%.
Higher conscientiousness was significantly associated with higher EI, b = 1.40, 95% BCa CI [1.04, 1.76], t = 7.62, p < 0.001 (R2 = 0.31). Higher conscientiousness was significantly associated with the rational decision-making style even with EI in the model, b = 0.09, 95% BCa CI [0.04, 0.14], t = 3.55, p < 0.001; EI was not significantly associated with the rational decision-making, b = 0.01, 95% BCa CI [− 0.0003, 0.03], t = 1.93, p = 0.055 (R2 = 0.29). When EI was not in the model, conscientiousness was significantly associated with the rational decision-making style, b = 0.11, 95% BCa CI [0.07, 0.16], t = 4.76, p < 0.001 (R2 = 0.28). The mediating effect of EI was 22.47%.
Higher neuroticism was significantly associated with lower EI, b = − 0.50, 95% BCa CI [− 0.80, − 0.20], t = − 3.26, p = 0.001 (R2 = 0.31). Neuroticism was not significantly associated with rational decision-making style with EI in the model, b = − 0.09, 95% BCa CI [− 0.05, 0.03], t = − 0.43, p = 0.668; EI was not significantly associated with rational decision-making, b = 0.01, 95% BCa CI [− 0.0003, 0.03], t = 1.93, p = 0.055 (R2 = 0.29). When EI was not in the model, neuroticism was not significantly associated with the rational decision-making style, b = − 0.02, 95% BCa CI [− 0.06, 0.02], t = − 0.81, p = 0.418 (R2 = 0.28).
No calculations were done for the openness to experience personality traits since it was not significantly associated with the rational decision-making style in the bivariate analysis.
Intuitive decision-making style (Table 4 , model 2)
Higher extroversion was significantly associated with higher EI, b = 0.86, 95% BCa CI [0.59, 1.13], t = 6.28, p < 0.001 (R2 = 0.41). Higher extroversion was significantly associated with higher intuitive decision-making even with EI in the model, b = 0.05, 95% BCa CI [0.002, 0.11], t = 2.03, p = 0.043; EI was significantly associated with intuitive decision-making style, b = 0.03, 95% BCa CI [0.01, 0.05], t = 2.91, p = 0.003 (R2 = 0.21). When EI was not in the model, higher extroversion was significantly associated with higher intuitive decision-making, b = 0.08, 95% BCa CI [0.03, 0.13], t = 3.21, p = 0.001 (R2 = 0.18). The mediating effect of EI was 49.82%.
Higher agreeableness was significantly associated with EI, b = − 0.33, 95% BCa CI [− 0.65, − 0.02], t = − 2.06, p = 0.039 (R2 = 0.41). Higher agreeableness was significantly associated with lower intuitive decision-making style even with EI in the model, b = − 0.15, 95% BCa CI [− 0.21, − 0.10], t = − 5.16, p < 0.001; higher EI was significantly associated with higher intuitive decision-making, b = 0.03, 95% BCa CI [0.01, 0.05], t = 2.91, p = 0.004 (R2 = 0.21). When EI was not in the model, higher agreeableness was significantly associated with lower intuitive decision-making, b = − 0.17, 95% BCa CI [− 0.22, − 0.11], t = − 5.48, p < 0.001 (R2 = 0.18). The mediating effect of EI was 6.80%.
Higher conscientiousness was significantly associated with higher EI, b = 1.18, 95% BCa CI [0.85, 1.51], t = 7.06, p < 0.001 (R2 = 0.41). Higher conscientiousness was significantly associated with lower intuitive decision-making style even with EI in the model, b = − 0.10, 95% BCa CI [− 0.16, − 0.03], t = − 2.95, p = 0.003; higher EI was also significantly associated with higher intuitive decision-making, b = 0.03, 95% BCa CI [0.01, 0.05], t = 2.91, p = 0.004 (R2 = 0.21). When EI was not in the model, conscientiousness was not significantly associated with the intuitive decision-making style, b = − 0.06, 95% BCa CI [− 0.12, 0.0004], t = − 1.95, p = 0.051 (R2 = 0.18). The mediating effect of EI was 38%.
Higher openness to experience was significantly associated with higher EI, b = 1.44, 95% BCa CI [1.13, 1.75], t = 9.11, p < 0.001 (R2 = 0.41). Higher openness to experience was significantly associated with higher intuitive decision-making style with EI in the model, b = 0.08, 95% BCa CI [0.01, 0.14], t = 2.38, p = 0.017; higher EI was also significantly associated with intuitive decision-making style, b = 0.03, 95% BCa CI [0.01, 0.05], t = 2.91, p = 0.004 (R2 = 0.21). When EI was not in the model, higher openness to experience was significantly associated with intuitive decision-making style, b = 0.12, 95% BCa CI [0.06, 0.18], t = 4.22, p < 0.001 (R2 = 0.18). The mediating effect of EI was 57.93%.
No calculations were done for neuroticism personality trait since it was not significantly associated with the intuitive decision-making style in the bivariate analysis.
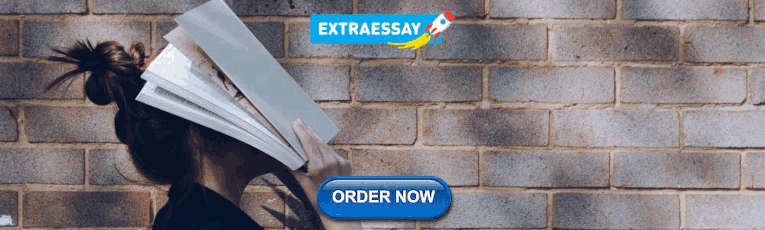
Dependent decision-making style (Table 4 , model 3)
Agreeableness was not significantly associated with EI, b = − 0.15, 95% BCa CI [− 0.49, 0.17], t = − 0.94, p = 0.345 (R2 = 0.32). Higher agreeableness was significantly associated with higher dependent decision-making style even with EI in the model, b = 0.29, 95% BCa CI [0.23, 0.34], t = 10.51, p < 0.001; higher EI was significantly associated with lower dependent decision-making, b = − 0.04, 95% BCa CI [− 0.06, − 0.02], t = − 4.50, p < 0.001 (R2 = 0.40). When EI was not in the model, higher agreeableness was significantly associated with higher dependent decision-making, b = 0.29, 95% BCa CI [0.24, 0.35], t = 10.44, p < 0.001 (R2 = 0.18). The mediating effect of EI was 2.38%.
Higher conscientiousness was significantly associated with higher EI, b = 1.04, 95% BCa CI [0.69, 1.38], t = 5.93, p < 0.001 (R2 = 0.32). Higher conscientiousness was significantly associated with higher dependent decision-making style even with EI in the model, b = 0.15, 95% BCa CI [0.09, 0.20], t = 4.88, p < 0.001; higher EI was also significantly associated with lower dependent decision-making, b = − 0.04, 95% BCa CI [− 0.06, − 0.02], t = − 4.50, p < 0.001 (R2 = 0.40). When EI was not in the model, higher conscientiousness was significantly associated with a higher dependent decision-making style, b = 0.10, 95% BCa CI [0.04, 0.16], t = 3.49, p < 0.001 (R2 = 0.36). The mediating effect of EI was 30.25%.
Higher openness to experience was significantly associated with higher EI, b = 1.37, 95% BCa CI [1.05, 1.69], t = 8.41, p < 0.001 (R2 = 0.32). Higher openness to experience was significantly associated with lower dependent decision-making style even with EI in the model, b = − 0.13, 95% BCa CI [− 0.19, − 0.08], t = − 4.55, p < 0.001; higher EI was also significantly associated with dependent decision-making style, b = − 0.04, 95% BCa CI [− 0.19, − 0.08], t = − 4.50, p < 0.001 (R2 = 0.40). When EI was not in the model, higher openness to experience was significantly associated with lower dependent decision-making style, b = − 0.19, 95% BCa CI [− 0.24, − 0.14], t = − 7.06, p < 0.001 (R2 = 0.36). The mediating effect of EI was 43.69%.
No calculations were done for neuroticism and extroversion personality traits since they were not significantly associated with the dependent decision-making style in the bivariate analysis.
Spontaneous decision-making style (Table 4 , model 4)
Agreeableness was not significantly associated with EI, b = 0.17, 95% BCa CI [− 0.19, 0.53], t = 0.91, p = 0.364 (R2 = 0.17). Higher agreeableness was significantly associated with lower spontaneous decision-making style even with EI in the model, b = − 0.10, 95% BCa CI [− 0.16, − 0.03], t = − 3.07, p = 0.002; EI was not significantly associated with spontaneous decision-making, b = − 0.01, 95% BCa CI [− 0.03, 0.01], t = − 0.71, p = 0.476 (R2 = 0.15). When EI was not in the model, higher agreeableness was significantly associated with lower spontaneous decision-making, b = − 0.10, 95% BCa CI [− 0.16, − 0.04], t = − 3.11, p = 0.002 (R2 = 0.15). The mediating effect of EI was 1.25%.
Higher conscientiousness was significantly associated with higher EI, b = 1.26, 95% BCa CI [0.88, 1.64], t = 6.56, p < 0.001 (R2 = 0.17). Higher conscientiousness was significantly associated with lower spontaneous decision-making style even with EI in the model, b = − 0.16, 95% BCa CI [− 0.23, − 0.09], t = − 4.51, p < 0.001; EI was not significantly associated with spontaneous decision-making style, b = − 0.01, 95% BCa CI [− 0.03, 0.01], t = − 0.71, p = 0.476 (R2 = 0.15). When EI was not in the model, higher conscientiousness was significantly associated with lower spontaneous decision-making style, b = − 0.17, 95% BCa CI [− 0.23, − 0.10], t = − 5.11, p < 0.001 (R2 = 0.15). The mediating effect of EI was 5.64%.
Neuroticism was not significantly associated with EI, b = − 0.22, 95% BCa CI [− 0.53, 0.08], t = − 1.43, p = 0.153 (R2 = 0.17). Higher neuroticism was significantly associated with lower spontaneous decision-making style even with EI in the model, b = − 0.11, 95% BCa CI [− 0.16, − 0.06], t = − 4.05, p < 0.001; EI was not significantly associated with spontaneous decision-making style, b = − 0.01, 95% BCa CI [− 0.03, 0.01], t = − 0.71, p = 0.476 (R2 = 0.15). When EI was not in the model, higher neuroticism was significantly associated with lower spontaneous decision-making style, b = − 0.11, 95% BCa CI [− 0.16, − 0.05], t = − 4.01, p < 0.001 (R2 = 0.15). The mediating effect of EI was 1.49%.
No calculations were done for openness to experience and extroversion personality traits since they were not significantly associated with the spontaneous decision-making style in the bivariate analysis .
Avoidant decision-making style (Table 4 , model 5)
Higher extroversion was significantly associated with higher EI, b = 0.88, 95% BCa CI [0.54, 1.21], t = 5.18, p < 0.001 (R2 = 0.15). Extroversion was not significantly associated with avoidant decision-making style even with EI in the model, b = − 0.01, 95% BCa CI [− 0.06, 0.05], t = − 0.27, p = 0.790; higher EI was significantly associated with avoidant decision-making style, b = − 0.04, 95% BCa CI [− 0.06, 0.03], t = − 4.79, p < 0.001 (R2 = 0.25). When EI was not in the model, extroversion was not significantly associated with avoidant decision-making style, b = − 0.05, 95% BCa CI [− 0.1, 0.08], t = − 1.69, p = 0.092 (R2 = 0.19).
Higher neuroticism was significantly associated with lower EI, b = − 0.59, 95% BCa CI [− 0.91, − 0.27], t = − 3.60, p < 0.001 (R2 = 0.15). Neuroticism was not significantly associated with avoidant decision-making style even with EI in the model, b = − 0.03, 95% BCa CI [− 0.09, 0.02], t = − 1.34, p = 0.182; higher EI was significantly associated with lower avoidant decision-making style, b = − 0.04, 95% BCa CI [− 0.06, − 0.03], t = − 4.79, p < 0.001 (R2 = 0.25). When EI was not in the model, neuroticism was not significantly associated with avoidant decision-making style, b = − 0.09, 95% BCa CI [− 0.06, 0.04], t = − 0.33, p = 0.739 (R2 = 0.19).
No calculations were done for openness to experience, agreeableness, and conscientiousness personality traits since they were not significantly associated with the avoidant decision-making style in the bivariate analysis.
This study examined the relationship between personality traits and decision-making styles, and the mediation role of emotional intelligence in a sample of general medicine students from different medical schools in Lebanon.
Agreeableness is characterized by cooperation, morality, sympathy, low self-confidence, high levels of trust in others and agreeable individuals tend to be happy and satisfied because of their close interrelationships [ 19 , 20 ]. Likewise, dependent decision-making style is characterized by extreme dependence on others when it comes to making decisions [ 1 ]. Our study confirmed this relationship similarly to Wood (2012) [ 41 ] and Bayram and Aydemir (2017) [ 26 ] findings of a positive relationship between dependent decision-making style and agreeableness personality trait and a negative correlation between this same personality trait and spontaneous decision-making style. In fact, this negative correlation can be explained by the reliance and trust accorded by agreeable individuals to their surroundings, making them highly influenced by others opinions when it comes to making a decision; hence, avoiding making rapid and snap decisions on the spur of the moment (i.e. spontaneous decision-making style); in order to explore the point of view of their surrounding before deciding on their own.
Conscientiousness is characterized by competence, hard work, self-discipline, organization, strive for achievement, and goal orientation [ 20 ]. Besides, conscientious individuals have a high level of deliberation making them capable of analyzing the pros and cons of a given situation [ 21 ]. Similarly, rational decision-makers strive for achievements by searching for information and logically evaluating alternatives before making decisions; making them high achievement-oriented [ 20 , 42 ]. This positive relationship between rational decision-making style and conscientiousness was established by Nygren and White (2005) [ 43 ] and Bajwa et al. (2016) [ 25 ]; thus, solidifying our current findings. Furthermore, we found that conscientiousness was positively associated with dependent decision-making; this relationship was not described in previous literature to our knowledge and remained statistically significant after adding EI to the analysis model. This relationship may be explained by the fact that conscientious individuals tend to take into consideration the opinions of their surrounding in their efforts to analyze the pros and cons of a situation. Further investigations in similar populations should be conducted in order to confirm this association. Moreover, we found a positive relationship between conscientiousness and intuitive decision-making that lost significance when EI was removed from the model. Thus, solidifying evidence of the mediating role played by EI between personality trait and decision-making style with an estimated mediation effect of 38%.
Extroversion is characterized by higher levels of self-confidence, positive emotions, enthusiasm, energy, excitement seeking, and social interactions. Similarly, intuitive decision-making is highly influenced by emotions and instinct. The positive relationship between extroversion and intuitive decision-making style was supported by Wood (2012) [ 41 ], Riaz et al. (2012) [ 24 ] and Narooi and Karazee (2015) [ 23 ] findings and by our present study.
Neuroticism is characterized by anxiety, anger, self-consciousness, and vulnerability [ 20 ]. High neurotic individuals have higher levels of negative affect, depression, are easily irritated, and more likely to turn to inappropriate coping responses, such as interpersonal hostility [ 22 ]. Our study results showed a negative relationship between neuroticism and spontaneous decision-making style.
Openness to experience individuals are creative, imaginative, intellectually curious, impulsive and original, open to new experiences and ideas [ 19 , 20 ]. One important characteristic of intuitive decision-making style is tolerance for ambiguity and the ability to picture the problem and its potential solution [ 44 ]. The positive relationship between openness to experience and intuitive decision-making style was established by Riaz and Batool (2012) [ 24 ] and came in concordance with our study findings. Additionally, our results suggest that openness personality trait is negatively associated with dependent decision-making style similar to previous findings [ 23 ]. Openness to experience individuals are impulsive and continuously seek intellectual pursuits and new experiences; hence, they tend to depend to a lesser extent on others’ opinions when making decisions since they consider the decision-making process a way to uncover new experiences and opportunities.
Our study results showed that EI had a significant positive effect on intuitive decision-making style. Intuition can be regarded as an interplay between cognitive and affective processes highly influenced by tactic knowledge [ 45 ]; hence, intuitive decision-making style is the result of personal and environmental awareness [ 46 , 47 , 48 ] in which individuals rely on the overall context without much concentration on details. In other words, they depend on premonitions, instinct, and predications of possibilities focusing on designing the overall plan [ 49 ] and take responsibility for their decisions [ 46 ]. Our study finding supports the results of Khan and al. (2016) who concluded that EI and intuitive decision-making had a positive relationship [ 35 ]. On the other hand, our study showed a negative relationship between EI and avoidant and dependent decision-making styles. Avoidant decision-making style is defined as a continuous attempt to avoid decision-making when possible [ 1 ] since they find it difficult to act upon their intentions and lack personal and environmental awareness [ 50 ]. Similarly to our findings, Khan and al. (2016) found that avoidant style is negatively influenced by EI [ 35 ]. The dependent decision-making style can be regarded as requiring support, advice, and guidance from others when making decisions. In other words, it can be described as an avoidance of responsibility and adherence to cultural norms; thus, dependent decision-makers tend to be less influenced by their EI in the decision-making process. Our conclusion supports Avsec’s (2012) findings [ 51 ] on the negative relationship between EI and dependent decision-making style.
Practical implications
The present study helps in determining which sort of decision is made by which type of people. This study also represents a valuable contribution to the Lebanese medical society in order to implement such variables in the selection methods of future physicians thus recruiting individuals with positively evaluated decision-making styles and higher levels of emotional intelligence; implying better communication skills and positively impacting patients’ experience. Also, the present study may serve as a valuable tool for the medical school administration to develop targeted measures to improve students’ interpersonal skills.
Limitations
Even though the current study is an important tool in order to understand the complex relationship between personality traits, decision-making styles and emotional intelligence among medical students; however, it still carries some limitations. This study is a descriptive cross-sectional study thus having a lower internal validity in comparison with experimental studies. The Scott and Bruce General Decision-Making Style Inventory has been widely used internationally for assessing decision-making styles since 1995 but has not been previously validated in the Lebanese population. In addition, the questionnaire was only available in English taking into consideration the mandatory good English knowledge in all the Lebanese medical schools; however, translation, and cross-language validation should be conducted in other categories of Lebanese population. Furthermore, self-reported measures were employed in the present research where participants self-reported themselves on personality types, decision-making styles and emotional intelligence. Although, all used scales are intended to be self-administered; however, this caries risk of common method variance; hence, cross-ratings may be employed in the future researches in order to limit this variance.
The results suggest that EI showed a significant positive effect on intuitive decision-making style and a negative effect on avoidant and dependent decision-making styles. In addition, our study showed a positive relationship between agreeableness and dependent decision-making style and a negative correlation with spontaneous decision-making style. Furthermore, conscientiousness had a positive relationship with rational and dependent decision-making style and extroversion showed a positive relationship with intuitive decision-making style. Neuroticism had a negative relationship with spontaneous style and openness to experience showed a positive relationship with intuitive decision-making style and a negative relationship with dependent style. Additionally, our study underlined the role of emotional intelligence as a mediation factor between personality traits and decision-making styles namely openness to experience, extroversion, and conscientiousness personality traits with intuitive decision-making style. Personality traits are universal [ 20 ]; beginning in adulthood and remaining stable with time [ 52 ]. Comparably, decision-making styles are stable across situations [ 1 ]. The present findings further solidify a previously established relationship between personality traits and decision-making and describes the effect of emotional intelligence on this relationship.
Availability of data and materials
All data generated or analyzed during this study are not publicly available to maintain the privacy of the individuals’ identities. The dataset supporting the conclusions is available upon request to the corresponding author.
Scott SG, Bruce RA. Decision-making style: the development and assessment of a new measure. Educ Psychol Meas. 1995;55(5):818–31.
Article Google Scholar
Capraro V, Everett JA, Earp BD. Priming intuition disfavors instrumental harm but not impartial beneficence. J Exp Soc Psychol. 2019;83:142–9.
Allwood CM, Salo I. Decision-making styles and stress. Int J Stress Manag. 2012;19(1):34.
Rand DG, Brescoll VL, Everett JA, Capraro V, Barcelo H. Social heuristics and social roles: intuition favors altruism for women but not for men. J Exp Psychol Gen. 2016;145(4):389–96.
Article PubMed Google Scholar
Kobis NC, Verschuere B, Bereby-Meyer Y, Rand D, Shalvi S. Intuitive honesty versus dishonesty: meta-analytic evidence. Perspect Psychol Sci. 2019;14(5):778–96.
Greene JD, Morelli SA, Lowenberg K, Nystrom LE, Cohen JD. Cognitive load selectively interferes with utilitarian moral judgment. Cognition. 2008;107(3):1144–54.
Rand DG. Cooperation, fast and slow: meta-analytic evidence for a theory of social heuristics and self-interested deliberation. Psychol Sci. 2016;27(9):1192–206.
Tremoliere B, Neys WD, Bonnefon JF. Mortality salience and morality: thinking about death makes people less utilitarian. Cognition. 2012;124(3):379–84.
Brown C, George-Curran R, Smith ML. The role of emotional intelligence in the career commitment and decision-making process. J Career Assess. 2003;11(4):379–92.
Clancy C. The importance of emotional intelligence. Nursing Management (2014+). 2014;21(8):15.
Goleman D. Working with emotional intelligence. New York: Bantam; 1998.
Google Scholar
Cotruş A, Stanciu C, Bulborea AA. EQ vs. IQ which is Most important in the success or failure of a student? Procedia Soc Behav Sci. 2012;46:5211–3.
Alghamdi NG, Aslam M, Khan K. Personality traits as predictor of emotional intelligence among the university teachers as advisors. Educ Res Int. 2017;2017:1–6 https://doi.org/10.1155/2017/9282565 .
Kazdin AE. Encyclopedia of psychology, vol. Vol 8. Washington, DC: American Psychological Association; 2000.
Bleidorn W, Klimstra TA, Denissen JJ, Rentfrow PJ, Potter J, Gosling SD. Personality maturation around the world: a cross-cultural examination of social-investment theory. Psychol Sci. 2013;24(12):2530–40.
Borkenau P, Riemann R, Spinath FM, Angleitner A. Genetic and environmental influences on person× situation profiles. J Pers. 2006;74(5):1451–80.
Hazrati-Viari A, Rad AT, Torabi SS. The effect of personality traits on academic performance: the mediating role of academic motivation. Procedia Soc Behav Sci. 2012;32:367–71.
Proceedings of the international conference on multiple academic disciplines. HanoiISBN: 978–1–943579-61-7 Hai Phong - . . Paper ID: VM714. 18–19, August: Vietnam (MAD17Vietnam Conference); 2017.
McCrae RR, John OP. An introduction to the five-factor model and its applications. J Pers. 1992;60(2):175–215.
McCrae R, Costa P Jr. Personality in adulthood. In: O envelhecimento. Uma abordagem psicológica . New York: Guilford; 1990. p. 2.
Johnson JA. Units of analysis for the description and explanation of personality. In R. Hogan, J. Johnson, & S. Briggs (Eds.) Handbook of personality psychology : Elsevier; 1997. p. 73–93.
Camps J, Stouten J, Euwema M. The relation between supervisors’ big five personality traits and employees’ experiences of abusive supervision. Front Psychol. 2016;7:112.
Article PubMed PubMed Central Google Scholar
Narooi ZS, Karazee F. Investigating the relationship among personality traits, decision-making styles, and attitude to life (Zahedan branch of Islamic Azad University as case study in Iran). Mediterr J Soc Sci. 2015;6(6 S6):311.
Riaz MN, Riaz MA, Batool N. Personality types as predictors of decision making styles. J Behav Sci. 2012;22(2):100–14.
Bajwa RS, Batool I, Asma M, Ali H, Ajmal A. Personality traits and decision making styles among university students (Pakistan). Pakistan J Life Soc Sci. 2016;14(1):38–41.
Bayram N, Aydemir M. Decision-making styles and personality traits. Int J Recent Adv Organizational Behaviour Decision Sci. 2017;3:905–15.
Dawda D, Hart SD. Assessing emotional intelligence: reliability and validity of the Bar-on emotional quotient inventory (EQ-i) in university students. Personal Individ Differ. 2000;28(4):797–812.
Day AL, Therrien DL, Carroll SA. Predicting psychological health: assessing the incremental validity of emotional intelligence beyond personality, type a behaviour, and daily hassles. Eur J Personality. 2005;19(6):519–36.
Avsec A, Takšić V, Mohorić T. The relationship of trait emotional intelligence with the big five in Croatian and Slovene university student samples. Psihološka obzorja/Horizons Psychol. 2009;18(3):99–110.
Spicer DP, Sadler-Smith E. An examination of the general decision making style questionnaire in two UK samples. J Manag Psychol. 2005;20(2):137–49.
Zeelenberg M, Pieters R. Feeling is for doing: a pragmatic approach to the study of emotions in decision-makingManuscript under review; 2005.
Bechara A, Damasio AR. The somatic marker hypothesis: a neural theory of economic decision. Games Econ Behavior. 2005;52(2):336–72.
Kustubayeva A, Bagayeva E. Emotional intelligence and decision making. RUDN J Psychol Pedagogics. 2011;S5:104–9.
Jaušovec N, Jaušovec K. Sex differences in brain activity related to general and emotional intelligence. Brain Cogn. 2005;59(3):277–86.
Khan E, Riaz M, Batool N, Riaz M. Emotional intelligence as a predictor of decision making styles among university students. J Appl Environ Biol Sci. 2016;6(4S):93–9.
Sarkis A. Hallit S. Hajj A. Lebanese students’ motivation in medical school: does it change throughout the years? A cross-sectional study. BMC Med Educ 20, 94 (2020). https://doi.org/10.1186/s12909-020-02011-w .
John OP, Donahue EM, Kentle RL. The big five inventory—versions 4a and 54. Berkeley: University of California, Berkeley, Institute of Personality; 1991.
Mohapel P. The quick emotional intelligence self-assessment: San Diego City College MESA Program; 2015.
Soueid M, Ghanem S, Hariri Z, Yamout N, Nehme R. Analysis of Lebanon's education sector: BankMed Market & Economic Research Divisionupdated Nov 10; cited 2015 Apr 6; 2014. Available from: http://www.bankmed.com.lb/BOMedia/subservices/categories/News/20150515170635891.pdf . Accessed 17 Jan 2020.
Hayes AF. Introduction to Mediation, Moderation, and Conditional Process Analysis: A Regression‐Based Approach. New York, NY: The Guilford Press.
Thomas E. Nygren, Rebecca J. White; 2005. Relating Decision Making Styles to Predicting Selfefficacy and a Generalized Expectationof Success and Failure. Article in Human Factors andErgonomics Society Annual Meeting Proceedings 49(3):432–34.
Baiocco R, Laghi F, D'Alessio M. Decision-making style among adolescents: relationship with sensation seeking and locus of control. J Adolesc. 2009;32(4):963–76.
Nygren TE, White RJ. Relating decision making styles to predicting selfefficacy and a generalized expectation of success and failure. Paper presented at: Proceedings of the Human Factors and Ergonomics Society Annual Meeting. 2005.
Bergstrand B. Situating the estimate: naturalistic decision-making as an alternative to analytical decision making in the Canadian forcesunpublished article. n. pag. http://www.cfcsc.dnd.ca/irc/nh/nh9798/0021.html ; 2001. p. 24.
Sinclair M, Ashkanasy NM. Intuition: myth or a decision-making tool? Manag Learn. 2005;36(3):353–70.
Harren VA. A model of career decision making for college students. J Vocat Behav. 1979;14(2):119–33.
Hablemitoglu S, Yildirim F. The relationship between perception of risk and decision making styles of Turkish university students: a descriptive study of individual differences. World Appl Sci J. 2008;4(2):214–24.
Singh R, Greenhaus JH. The relation between career decision-making strategies and person–job fit: a study of job changers. J Vocat Behav. 2004;64(1):198–221.
Miller CC, Ireland RD. Intuition in strategic decision making: friend or foe in the fast-paced 21st century? Acad Manag Perspect. 2005;19(1):19–30.
Phillips SD, Pazienza NJ, Ferrin HH. Decision-making styles and problem-solving appraisal. J Couns Psychol. 1984;31(4):497.
Avsec A. Do emotionally intelligent individuals use more adaptive decision-making styles? Stud Psychol. 2012;54(3):209.
Soldz S, Vaillant GE. The big five personality traits and the life course: a 45-year longitudinal study. J Res Pers. 1999;33(2):208–32.
Download references
Acknowledgements
We would like to thank all students who agreed to participate in this study.
Author information
Rabih Hallit, Sahar Obeid and Souheil Hallit are last co-authors.
Authors and Affiliations
Faculty of Medicine and Medical Sciences, Holy Spirit University of Kaslik (USEK), Jounieh, Lebanon
Radwan El Othman, Rabih Hallit & Souheil Hallit
Department of Pediatrics, Bahman Hospital, Beirut, Lebanon
Rola El Othman
Department of Infectious Disease, Bellevue Medical Center, Mansourieh, Lebanon
Rabih Hallit
Department of Infectious Disease, Notre Dame des Secours University Hospital Center, Byblos, Lebanon
Research and Psychology departments, Psychiatric Hospital of the Cross, P.O. Box 60096, Jal Eddib, Lebanon
Sahar Obeid & Souheil Hallit
Faculty of Arts and Sciences, Holy Spirit University of Kaslik (USEK), Jounieh, Lebanon
Sahar Obeid
INSPECT-LB: Institut National de Santé Publique, Epidémiologie Clinique et Toxicologie – Liban, Beirut, Lebanon
You can also search for this author in PubMed Google Scholar
Contributions
REO and REO were responsible for the data collection and entry and drafted the manuscript. SH and SO designed the study; SH carried out the analysis and interpreted the results; RH assisted in drafting and reviewing the manuscript; All authors reviewed the final manuscript and gave their consent; SO, SH and RH were the project supervisors.
Corresponding authors
Correspondence to Sahar Obeid or Souheil Hallit .
Ethics declarations
Ethics approval and consent to participate.
The Holy Spirit University of Kaslik – School of Medicine and Medical Sciences ethics committee approved the study protocol. A written consent was obtained from each student.
Consent for publication
Not applicable.
Competing interests
The authors have nothing to disclose.
Additional information
Publisher’s note.
Springer Nature remains neutral with regard to jurisdictional claims in published maps and institutional affiliations.
Rights and permissions
Open Access This article is licensed under a Creative Commons Attribution 4.0 International License, which permits use, sharing, adaptation, distribution and reproduction in any medium or format, as long as you give appropriate credit to the original author(s) and the source, provide a link to the Creative Commons licence, and indicate if changes were made. The images or other third party material in this article are included in the article's Creative Commons licence, unless indicated otherwise in a credit line to the material. If material is not included in the article's Creative Commons licence and your intended use is not permitted by statutory regulation or exceeds the permitted use, you will need to obtain permission directly from the copyright holder. To view a copy of this licence, visit http://creativecommons.org/licenses/by/4.0/ . The Creative Commons Public Domain Dedication waiver ( http://creativecommons.org/publicdomain/zero/1.0/ ) applies to the data made available in this article, unless otherwise stated in a credit line to the data.
Reprints and permissions
About this article
Cite this article.
El Othman, R., El Othman, R., Hallit, R. et al. Personality traits, emotional intelligence and decision-making styles in Lebanese universities medical students. BMC Psychol 8 , 46 (2020). https://doi.org/10.1186/s40359-020-00406-4
Download citation
Received : 27 January 2020
Accepted : 12 April 2020
Published : 05 May 2020
DOI : https://doi.org/10.1186/s40359-020-00406-4
Share this article
Anyone you share the following link with will be able to read this content:
Sorry, a shareable link is not currently available for this article.
Provided by the Springer Nature SharedIt content-sharing initiative
- Personality traits
- Decision-making
- Decision-making style
- Emotional intelligence
- Medical students
BMC Psychology
ISSN: 2050-7283
- General enquiries: [email protected]
" Effects of Achievement Motivation on Behavior ," Scott T. Rabideau
" heredity versus environment: twin, adoption, and family studies ," avi g. haimowitz, " social causes of depression ," gregory s. beattie, " pathways from childhood conduct problems to adult criminality ," tiffany l. panko, " expressed emotion as a precipitant of relapse in psychological disorders ," linda a. mcdonagh, " relations between social support and physical health ," corey m. clark, " decrease of deaf potential in a mainstreamed environment ," wyatte c. hall, " genetic and environmental influences on criminal behavior ," caitlin m. jones, " reactive attachment disorder: a disorder of attachment or of temperament " maureen e. wood, " multiple personality disorder: fact or fiction " alexandria k. cherry, " the evolution of human intelligence: increasing importance of domain-specific intelligence in the modern environment ," kyle r. skottke, " biological rhythms as a basis for mood disorders ," patrick c. marino, " the iq paradox resolved a critical analysis ," alexandria k. cherry, " evolutionary and biochemical explanations for a unique female stress response: tend-and-befriend ," lauren a. mccarthy, " effects of video games on aggressive thoughts and behaviors during development ," thomas a. kooijmans, " attachment and divorce: family consequences ," cristina e. eagan, " contributions and limitations of cattell's sixteen personality factor model ," heather m. fehringer, " the five-factor model of personality in the workplace ," sean p. neubert, " evolutionary versus social structural explanations for sex differences in mate preferences, jealousy, and aggression ," jennifer s. denisiuk, " the rise of civilization and the evolution of personality ," brian p. smith, " derivation of offensive selection from natural selection as it relates to sexual strategies ," laura r. thatcher, " factors contributing to the development of pathological gambling ," kory sinha, " the attachment system throughout the life course: review and criticisms of attachment theory ," erin j. lee, " an overview of beck's cognitive theory of depression in contemporary literature ," josiah p. allen, " effects of stress and psychological disorders on the immune system ," david b. beaton, " depression in children: what causes it and how we can help ," irina v. sokolova, " creative genius or psychotic a look at the strong positive correlation between creativity and psychoses ," jonathan s. byrd, " a critical review of eysenck's theory of psychoticism and how it relates to creativity ," shane k. porzio, " why women are more susceptible to depression: an explanation for gender differences ," christina m. mulé, " depression and suicide in older adults ," ratna roy, " eating disorders: more to them than meets the mouth ," jason j. zodda, " a brief review of creativity ," johanna e. dickhut, " defining schizophrenia: a testable model for schizophrenia incorporating homogeneous subtypes ," matthew p. rick, " ethological attachment theory: a great idea in personality " patricia pendry, " the dilemma of the only child ," alissa d. eischens, " intimate relationships: personality development through interaction during early life ," maren cardillo, " the five-factor model: emergence of a taxonomic model for personality psychology ," nathan c. popkins, " psychoanalysis: freud's revolutionary approach to human personality ," kristen m. beystehner, " behaviorism as a theory of personality: a critical look ," payal naik, " natural characteristics that influence environment: how physical appearance affects personality ," nathan c. popkins, " one intelligence or many alternative approaches to cognitive abilities ," han s. paik, " psychoanalysis: from theory to practice, past to present ," ethan r. plaut, " eysenck's pen model: its contribution to personality psychology ," kwangmin jang, " the evolution of evolutionary psychology: from sociobiology to evolutionary psychology ," melissa seltin, " can behaviorism still apply in the face of overwhelming opposition " eileen pizzurro, " reconciling the mental and the behavioral: an evaluation of behaviorism ," alissa d. eischens.
- Bipolar Disorder
- Therapy Center
- When To See a Therapist
- Types of Therapy
- Best Online Therapy
- Best Couples Therapy
- Best Family Therapy
- Managing Stress
- Sleep and Dreaming
- Understanding Emotions
- Self-Improvement
- Healthy Relationships
- Student Resources
- Personality Types
- Verywell Mind Insights
- 2023 Verywell Mind 25
- Mental Health in the Classroom
- Editorial Process
- Meet Our Review Board
- Crisis Support
Psychology Questions About Personality
Personality Psychology Research Topics
Kendra Cherry, MS, is a psychosocial rehabilitation specialist, psychology educator, and author of the "Everything Psychology Book."
:max_bytes(150000):strip_icc():format(webp)/IMG_9791-89504ab694d54b66bbd72cb84ffb860e.jpg)
Emily is a board-certified science editor who has worked with top digital publishing brands like Voices for Biodiversity, Study.com, GoodTherapy, Vox, and Verywell.
:max_bytes(150000):strip_icc():format(webp)/Emily-Swaim-1000-0f3197de18f74329aeffb690a177160c.jpg)
List of Personality Topics
- Before You Begin
- Starting Your Research
Personality is a popular subject in psychology, so it's no surprise that this broad area is rife with fascinating research topics. There are many psychology questions about personality that can be a great topic for a paper, or just help you get to know others a little better.
Are you looking for a great topic for a paper , presentation, or experiment for your personality psychology class? Here are just a few ideas that might help kick-start your imagination.
At a Glance
If you are writing a paper, doing an experiment, or just curious about why people do the things they do, exploring some different psychology questions about personality can be a great place to start. Topics you might choose to explore include different personality traits, personality tests, and how different aspects of personality influence behavior.
Possible Topics for Personality Psychology Research
The type of psychology questions about personality that you might want to explore depend on what you are interested in and what you want to know. Some topics you might opt to explore include:
Personality Traits
- How do personality traits relate to creativity? Are people with certain traits more or less creative? For your project, you might try administering scales measuring temperament and creativity to a group of participants.
- Are certain personality traits linked to prosocial behaviors ? Consider how traits such as kindness, generosity, and empathy might be associated with altruism and heroism .
- How does Type A behavior influence success in school? Are people who exhibit Type A characteristics more likely to succeed?
- Is there a connection between a person's personality type and the kind of art they like? For example, are extroverts more drawn to brighter colors or art that depicts people vs. abstract, non-representational art?
- Do people tend to choose pets based on their personality types? How do the personalities of dog owners compare to those of cat owners?
Personality Tests
- How do personality assessments compare? Consider comparing common assessments such as the Myers-Briggs Temperament Indicator , the Keirsey Temperament Sorter, and the 16PF Questionnaire.
- How reliable are personality test results? If you give someone the same test weeks later, will their results be the same?
Family and Relationships
- Do people tend to marry individuals with similar personalities? Do people who marry partners with personalities similar to their own have more satisfying relationships?
- What impact does birth order have on personality? Are first-born children more responsible, and are last-borns less responsible?
Personality and Behaviors
- Is there a connection between personality types and musical tastes ? Do people who share certain personality traits prefer the same types of music?
- Are people who participate in athletics more likely to have certain personality characteristics? Compare the personality types of athletes versus non-athletes.
- Are individuals with high self-esteem more competitive than those with low self-esteem? Do those with high self-esteem perform better than those who have lower self-esteem?
- Is there a correlation between personality type and the tendency to cheat on exams? Are people low in conscientiousness more likely to cheat? Are extroverts or introverts more liable to cheat?
- How do personality factors influence a person's use of social media? For example, are people high in certain traits more likely to use Facebook, Instagram, and Twitter? Are individuals who use social media frequently more or less extroverted?
You can also come up with questions about your own about different topics in personality psychology. Some that you might explore include:
- Big 5 personality traits
- The id, ego, and superego
- Psychosocial development
- Hierarchy of needs
- Myers-Briggs Type Indicator
- Personality disorders
What to Do Before You Begin Your Research
Once you find a suitable research topic, you might be tempted just to dive right in and get started. However, there are a few important steps you need to take first.
Most importantly, be sure to run your topic idea past your instructor. This is particularly important if you are planning to conduct an actual experiment with human participants.
In most cases, you will need to gain your instructor's permission and possibly submit your plan to your school's human subjects committee to gain approval.
How to Get Started With Your Research
Whether you are doing an experiment, writing a paper , or developing a presentation, background research should always be your next step.
Consider what research already exists on the topic. Look into what other researchers have discovered. By spending some time reviewing the existing literature, you will be better able to develop your topic further.
What This Means For You
Asking psychology questions about personality can help you figure out what you want to research or write about. It can also be a way to think about your own personality or the characteristics of other people. If you're stumped for an idea, consider talking to your instructor or think about some questions you've had about people in your own life.
Atherton OE, Chung JM, Harris K, et al. Why has personality psychology played an outsized role in the credibility revolution ? Personal Sci . 2021;2:e6001. doi:10.5964/ps.6001
American Psychological Association. Frequently asked questions about institutional review boards .
Leite DFB, Padilha MAS, Cecatti JG. Approaching literature review for academic purposes: The Literature Review Checklist . Clinics (Sao Paulo) . 2019;74:e1403. doi:10.6061/clinics/2019/e1403
By Kendra Cherry, MSEd Kendra Cherry, MS, is a psychosocial rehabilitation specialist, psychology educator, and author of the "Everything Psychology Book."

An official website of the United States government
The .gov means it’s official. Federal government websites often end in .gov or .mil. Before sharing sensitive information, make sure you’re on a federal government site.
The site is secure. The https:// ensures that you are connecting to the official website and that any information you provide is encrypted and transmitted securely.
- Publications
- Account settings
Preview improvements coming to the PMC website in October 2024. Learn More or Try it out now .
- Advanced Search
- Journal List
- Ups J Med Sci
- v.123(4); 2018 Dec

Personality disorder: a disease in disguise
Lisa ekselius.
Department of Neuroscience, Psychiatry, Uppsala University, Sweden
Personality disorders (PDs) can be described as the manifestation of extreme personality traits that interfere with everyday life and contribute to significant suffering, functional limitations, or both. They are common and are frequently encountered in virtually all forms of health care. PDs are associated with an inferior quality of life (QoL), poor health, and premature mortality. The aetiology of PDs is complex and is influenced by genetic and environmental factors. The clinical expression varies between different PD types; the most common and core aspect is related to an inability to build and maintain healthy interpersonal relationships. This aspect has a negative impact on the interaction between health-care professionals and patients with a PD. From being discrete and categorical disease entities in previous classification systems, the current concept of PD, reflected in the newly proposed ICD-11, is a dimensional description based on the severity of the disturbed functioning rather than on the type of clinical presentation. Insight about the characteristics of PDs among medical practitioners is limited, which is partly because persons do not seek health care for their PD, but instead for other medical issues which are obscured by their underlying personality problems. What needs to be emphasized is that PDs affect both the clinical presentation of other medical problems, and the outcome of these, in a negative manner and that the integrated effects of having a PD are a shortened life expectancy. Accordingly, PDs need to be recognized in clinical practice to a greater extent than previously.
Introduction
In everyday clinical practice persons who think, feel, behave, or relate to others differently than the average person are identified. This deviation from the norm is a central feature in all personality disorders (PDs). Although using slightly different formulations over the years, PDs are roughly characterized by ‘a pervasive pattern of thought, feeling and behaviour that characterize an individual’s unique lifestyle and mode of adaptation, which deviates markedly from the expectations of the individual’s culture’ ( 1 ). Such characteristics obviously create problems for those who bear them. PDs are likely to have an onset in adolescence or early adulthood, appear to be stable over time, and lead to impairment or distress ( 1 , 2 ).
This review, which is an overview on PDs and the core problems these ultimately lead to, is commenced with some background information about the concept of personality and on the attempts that have been made to understand and to describe different characteristics of personality, how these characteristics can be structured and understood, and about the deviations in normal personality that form the basis for the different types of PD. Above all, the paper focuses on problems met in primary and specialist health care. Such problems are common, and persons with PDs are known to be under-treated with respect to physical health ( 3 ) and are over-represented in the group categorized as the ‘difficult patient’ ( 4 , 5 ).
The present paper argues that all health-care professionals need basic knowledge about manifestations of different personality traits, above all in the form of manifest PDs, as we know that such pathology has a negative effect on the interaction between the patient and health-care professionals in terms of communication, clinical assessment, treatment, and outcome ( 6 ). The patients’ suffering is considerable, and generally they report a low QoL ( 7 , 8 ). Having a PD also infers a risk factor for premature mortality ( 9 , 10 ), which affects individuals and incurs a high cost to society ( 11 ).
A historic perspective of aberrant personalities
The large variation in the way individuals think, feel, and behave has been recognized throughout antiquity. The terms for these characteristics have been diverse. For instance, Confucius (551–479 BCE) used the combination of ‘blood and vital essence’. The Greek philosopher and naturalist Theophrastus (c. 371 to c. 287 BC) used the term ‘characters’, and in eighteenth-century France the Galenus–Hippocrates term ‘temperament’ was reinstituted. The term ‘personality’ has been used since the eighteenth century to label distinguishing qualities of a person ( 12 ).
Pathological personalities have also generated interest over the years. Since the fourth century BC, philosophers have been trying to understand what it is that makes ‘us’ what we are. Theophrastus, a scholar of Plato and Aristotle, was the first to publish a systematic description of the multifaceted nature of personality types ( 12 ). A few hundred years later, Aelius Galenus (130–200 AD) linked Hippocrates’ four humours to personality characteristics in his description of sanguine , phlegmatic , choleric , and melancholic temperaments. He proposed that each of these four body fluids held a combination of two properties split along two axes: temperature (hot/cold) and humidity (wet/dry). The humoral pathology system influenced the view among European doctors until the breakthrough of medical science in the nineteenth century.
In the early nineteenth century, Franz Joseph Gall (1758–1828), a German neuroanatomist, thought that some brain areas were associated with specific functions. He also thought that measurements of the skull represented differences in the individual’s personality ( 13 ).
Philippe Pinel (1745–1826), a French physician, was the first to include an aberrant personality in the nosology of psychiatry ( 14 ). Pinel introduced the term ‘ manie sans délire ’ (mania without delusion). During that time, the term ‘mania’ was employed to refer to states of agitation. Pinel described a few of his male patients who were disposed to bursts of irrational anger and impulsive violence in response to minor irritation. In the same intellectual environment Jean-Étienne Dominique Esquirol (1772–1840) introduced the concept monomanie raisonnante and the Englishman James Cowles Prichard (1786–1848) used the term moral insanity . These three physicians were obsessed by the practical question at that time whether psychiatry could explain abnormal behaviour in persons lacking acute psychiatric symptoms who had committed a violent crime ( 14 ).
During the late nineteenth and early twentieth century, several conceptual systems for normal and abnormal personalities emerged as the result of the work of European psychologists and psychiatrists (e.g. Ribot, Heymans, Lazursky, Schneider, and Sjöbring).
Théodule Ribot (1839–1916), a French psychologist, described normal and abnormal characters. He pointed out that a person’s character is stable from childhood into adult life. Ribot described three primary personality types: the sensitive, the active, and the apathetic, all three of which were divided into subtypes ( 15 ).
The Dutch scientist Gerard Heymans (1857–1930) applied empirical methods to the study of personality. He developed the Cube of Heymans, a description of a personality typology. He defined personality types in three dimensions: ‘activity level’, ‘emotionality’, and ‘primary versus secondary functioning’, with the last-mentioned dimension comparable to ‘extroversion/introversion’. These three dimensions are represented on the x -, y -, and z -axes of the Heymans cube, where all combinations of the three dimensions defined eight personality types ( 16 ).
The contributions of Aleksandr Lazursky (1874–1917), a Russian psychologist, were not widespread because most of his publications were in Russian and because of the political climate of the time. His major contribution was the description of the ‘endopsychic’ and ‘exopsychic’ aspects of personality. The endopsychic components represent the psychological functions (e.g. perception, memory, attention, thinking) that are mainly inborn; the exopsychic components are the consequence of the interaction with the outside world. The interplay between these two aspects of personality determines how a person functions in an integrated social context ( 17 ).
The German psychiatrist Kurt Schneider (1887–1967) focused on diagnostic issues that included concepts of ‘psychopathy’, which he had broadly equated to PDs. Based on his clinical views ( 18 ), he vaguely defined abnormal personality as a statistical deviation from the norm. He proposed 10 psychopathic personalities, all of which are very similar to those in the current classifications of PDs in the DSM-5 and ICD-10 ( 19 ).
Henrik Sjöbring (1879–1956), a Swedish psychiatrist, suggested four constitution factors of the personality: capacity (intelligence), validity (psychic energy), stability (balance in keynote), and solidity (firmness, tardiness, tenacity). By these variables, all persons can be categorized as either normal, super-, or sub-: e.g. subcapable (unintelligent), subvalid (lack of psychic energy), normosolid, superstable, and so on ( 20 ).
The first modern attempt to determine the structure of human personality was credited to the English scientist Sir Francis Galton (1882–1911). He used a lexical approach to the dimensions of personality based on the assumption that those personality characteristics important to a group of people will eventually be represented in their language ( 21 ). This work was continued by several others ( 22 ), and the lexical hypothesis constitutes the basis for how current approaches describe personality dimensions. It is also important to mention the work of the psychologists Raymond Bernard Cattell (1905–1998) ( 23 ) and Gordon Willard Allport (1897–1967) ( 24 ) who independently used advanced statistics (e.g. factor analysis) to discern dimensions of personality.
Modern concepts of personality disorder and personality
Before discussing this issue, it needs to be re-emphasized that the description of personalities is based purely on observations, or rather expressions, of the individual’s way to think, feel, behave, or relate. As a corollary, it follows that PDs are diagnoses based on symptoms described by the persons themselves, by persons in their surroundings, or are objectively observed in study situations. This circumstance accounts for why the validity and reliability of the current diagnostic instruments lack optimality ( 25 ).
Current knowledge on pathological personalities is primarily based on studies from four perspectives, all of which are necessary to create an in-depth template of what characterizes personality pathology.
The first perspective is the clinical picture, i.e. the integrated presentation of the clinical symptoms that are either expressed or witnessed. This perspective is what constitutes the basis for the clinical structured diagnosis according to classification systems. The second perspective entails a determination of underlying dysfunctional personality traits as well as dysfunctional limitations on capacity and functionality in the brain’s cognitive, emotional, and impulse control systems. The third perspective relates to the brain’s biological systems and their functions; this third perspective has highly benefited from the rapid development of brain imaging techniques ( 26 ). The fourth perspective denotes the underlying genetic contribution to the above-mentioned phenomena ( 27 ), which is currently approached in whole-genome association studies ( 28 ).
Not unexpectedly, studies have shown that the aetiology of personality pathology is complex. Overwhelming evidence supports the idea that an interaction between genetic and environmental factors is necessary for the development of human personality. The relation between the dimensions of normal personality and PD is not clear, however. Even if a PD has been viewed as an overexpression of personality traits to the extent that they lead to clinically significant distress or impairment, it has recently been demonstrated that a moderate-to-sizable proportion of the genetic influence underlying PD is not shared with the domain constructs of normative personality ( 29 ).
Based on the hypothesis that the domains of dysfunction in PDs are linked to specific neural circuits, neuroimaging techniques have been used over the past decade to examine the neural integrity of these circuits in personality-disordered individuals. Currently, the literature is flooded with information acquired through this approach. Most studies are done to explore borderline PD ( 30 ). In general, the studies have thus far demonstrated deviations in neuronal circuitry in areas previously found to be active in the symptomatology that characterizes the specific type of PD. Even if the results of such studies contribute to an understanding of underlying physiological processes, they are not yet ready to be used in clinical practice.
Several studies have examined the effects of being exposed to childhood adversities and the risk to develop PD. Just to mention one such study, we recently showed that exposure to cumulative childhood adversity was incrementally associated with a diagnosis of PD in young adulthood ( 31 ). Furthermore, childhood or adolescent psychiatric disorders have been suggested to trigger a chain of behaviours and responses that foster the more persistent psychopathology of a PD ( 32 , 33 ).
To determine the importance of genetic and environmental factors in early childhood in personality pathology the relationship between vulnerability to child abuse and antisocial personality patterns in adulthood was investigated ( 34 ). It was shown that individuals with a gene polymorphism that resulted in a low activity in monoamine oxidase A (MAOA) were more vulnerable to developing an antisocial personality pattern than those who had high activity in the MAOA gene, given that they had been exposed to child abuse. This gene–environment interaction has subsequently been confirmed ( 35 ). Moreover, a similar interaction for the effects of child maltreatment on antisocial behaviour has also been shown for other genes ( 36 , 37 ).
There is thus reason to consider genetic and environmental factors as interacting systems of crucial importance in the development of functional and dysfunctional personality traits.
Classification of personality disorders
The differences in the types of aberration in thought, feeling, and behaviour have been the basis for the classification of different PDs. The characteristics described by Galenus, and later by e.g. Pinel and Schneider, are very similar to contemporary classification systems. What today are referred to as PDs were earlier called ‘pathological personalities’ or ‘ persona pathologica ’ and were found under that heading in earlier versions of the ICD (up to ICD-8). These diagnoses were used rarely, in part because of their stigmatizing connotations.
Up to now, classification of PDs has been based on fulfilling a specified number of defined and ‘specific’ criteria for each PD, resulting in a categorical description; if a defined number of these criteria were met, a disorder was acknowledged, else not.
Over time, there has been an intraprofessional dispute on whether the classification of PDs should be based on the defined and specific characteristics or on the severity of the functional aberration. Historically, and currently, in the ICD-10 (which is from 1992) and in the current American DSM-5 ( 38 ) (from 2013) classification is based on types of symptom, i.e. characteristics of the clinical presentation, represented by the abovementioned ‘specific’ criteria for each PD. ICD-10 describes nine discrete and specific (as well as one unspecified) types of PD ( Table 1 ). The DSM-5 ( 38 ) identifies 10 PDs of similar structure. The DSM system has gone one step further in classification by grouping the different disorders in three clusters based on some overall common features. To illustrate, cluster A contains odd and eccentric personalities, cluster B includes dramatic, impulsive, emotional personalities, and cluster C fearful and anxious personalities.
Personality disorders in the ICD-10 ( 2 ).
A basic feature common for the different classification systems is that the aberration must be severe enough to cause a functional impairment in everyday life. This is the ‘general criterion’ for all PDs and overrides other perspectives. In other words, even the observance of very odd behaviour or feelings is not enough for a clinical diagnosis of a PD unless it can be ascertained that they lead to impairment or distress in everyday life.
Currently, there is somewhat of a paradigm shift in that more and more arguments speak for the relevance of a dimensional classification of PD based on the severity of symptoms rather than on the specific characteristics ( 19 ). Studies have, thus, shown that the conceptualization of PDs into discrete categories results in an insufficient description of the problem. Rather, it seems that within each discrete PD category the level of dysfunction is dimensional and dependent on the number of criteria fulfilled ( 39 ). Furthermore, only about half of all individuals with diagnosable PD fulfil criteria for a specific PD and are thus given a diagnosis of unspecified PD ( 40 ). In addition, the expression of different symptoms evolves continuously across the lifespan ( 41 ).
General description of personality disorders in ICD-11
The new ICD-11 classification system, which was released by WHO in June 2018 ( 42 ), means a radical change in the classification of PDs and will impact all aspects of health care, as well as influence the way PDs are seen ( 43 ). After 1–2 years of adaptive work, ICD-11 is expected to be operative internationally in 2020–2021. In ICD-11 PDs have been classified based on the perspectives mentioned above, i.e. according to the severity of suffering, and are divided into three severity groups: mild , moderate , or severe . The degree of severity is determined by the extent of problems in interpersonal relationships or the ability and willingness to perform expected social and occupational roles, or both (see Table 2 for a full description).
Personality disorders in the forthcoming ICD-11.
Excerpt from reference ( 41 ) with permission from WHO.
A new feature in ICD-11 is the introduction of the term ‘Personality Difficulty’, which refers to pronounced personality characteristics that may affect treatment or health services but do not rise to the level of severity to merit a diagnosis of PD.
Dimensions of personality disorders in ICD-11
ICD-11 has, thus, wiped out all type-specific categories of PD apart from the main one, the presence of PD itself. Instead, the type of clinical manifestation is added as a specific ‘post-coordination’ code describing the clinical characteristics in the form of six different personality domains. Factor analytic strategies have supported five domains, although clinical reasoning has suggested six domains ( 44–46 ). These six clinically derived personality domains do not fully correspond with the different specific PD types in the earlier ICD-10 ( Table 2 ).
The domain traits are not inherently pathological, but rather represent a profile of underlying personality structure ( 19 ). They apply equally to individuals without any PD and to those with severe disorder, but in PD they show where the focus of the disorder is apparent. In severe disorder, several domain traits are likely to be associated with the disorder ( 47 ). To describe personality functioning, as many domains as necessary can be applied.
The descriptions of the six different domain traits below are slightly and only linguistically modified from those in the original ICD-11.
Negative affectivity in personality disorder
The core aspect of negative affectivity is the tendency to experience a broad range of negative emotions. Common manifestations, not all of which may be present in everyone at a given time, include experiencing a variety of negative emotions with a frequency and intensity out of proportion to the situation: emotional lability and poor emotion regulation, negativistic attitudes, low self-esteem, low self-confidence, and mistrustfulness.
Patients fulfilling criteria for this disorder were classified as anxious/avoidant in previous classifications.
Detachment in personality disorder
The core aspect of the detachment domain is the tendency to maintain interpersonal (social detachment) and emotional distance (emotional detachment). Common manifestations, not all of which may be present in everyone at a given time, include social detachment (avoidance of social interactions, lack of friendships, and avoidance of intimacy) and emotional detachment (reserve, aloofness, and limited emotional expression and experience).
This disorder type is like the schizoid type of PD described in ICD-10.
Dissocial or antisocial personality disorder
Dissocial or antisocial PD is characterized by a gross disparity between behaviour and the prevailing social norms as well as by a callous unconcern for the feelings of others. Moreover, this type of PD can be described by a number of other attributes, including a gross and persistent attitude of irresponsibility and disregard for social norms, rules, and obligations; an incapacity to maintain enduring relationships, although having no difficulty in establishing them; very low tolerance to frustration and a low threshold for discharge of aggression, including violence; an incapacity to experience guilt or to profit from experience, particularly punishment; and finally, a marked proneness to blame others or to offer plausible rationalizations for the behaviour that has brought the person into conflict with society.
Persons with a dissocial PD often have an early criminal record and exhibit conduct problems in childhood or adolescence. The construct of a dissocial PD largely overlaps characteristics of the concept of psychopathy, which is not a term used to define a psychiatric disorder ( 48 ).
Disinhibition in personality disorder
The core aspect of disinhibition traits is the tendency to act rashly based on immediate external or internal stimuli (i.e. sensations, emotions, thoughts) without consideration of the consequences. Common manifestations—not all of which may be present in everyone at a given time—include impulsivity, distractibility, irresponsibility, recklessness, and lack of planning.
Patients fulfilling the criteria for this disorder in previous classifications were classified as histrionic, narcissistic, or borderline.
Anankastia in personality disorder
Anankastic PD (or obsessive-compulsive PD) is characterized by a narrow focus on orderliness and perfectionism and on right and wrong, although it also implies a need to control one’s own behaviour and the behaviour of others, as well as the need to control one’s environment to ensure conformity to these standards. Common manifestations, not all of which may be present in a given individual at a given time, can include conscientiousness (e.g. concern with social rules, obligations, and norms of right and wrong, scrupulous attention to detail, rigid, systematic, day-to-day routines, obsessiveness about hyper-scheduling, emphasis on organization, orderliness, and neatness) and emotional and behavioural constraint (e.g. rigid control over emotional expression, stubbornness and inflexibility, risk-avoidance, perseveration, and deliberativeness).
Borderline personality disorder
The criteria for this disorder are very similar to those for disinhibition. It was included in the new ICD-11 at a very late stage of the process ( 45 ). The classification may be applied to individuals whose pattern of personality disturbance is characterized by a pervasive pattern of instability of interpersonal relationships, self-image, and affects, as well as marked impulsivity, as indicated by many of the following behavioural patterns: frantic efforts to avoid real or imagined abandonment; a pattern of unstable and intense interpersonal relationships; identity disturbance, manifested in markedly and persistently unstable self-image or sense of self; a tendency to act rashly in states of high negative affect, leading to potentially self-damaging behaviours; recurrent episodes of self-harm; emotional instability due to marked reactivity of mood; chronic feelings of emptiness; inappropriate intense anger or difficulty controlling anger; and transient dissociative symptoms or psychotic-like features in situations of high affective arousal. The condition involves anxiety without an identifiable connection to concrete stimuli and, among other things, has been called ‘annihilation anxiety’, ‘pan-anxiety’, or ‘global anxiety’. The term ‘emptiness depression’ describes general feelings of despair and hopelessness with dominance of depressive thoughts.
Borderline PD (in ICD-10 ‘emotionally unstable PD’) is a dominating diagnosis in out- and inpatient psychiatric care ( 9 , 10 ). Clinical expressions are more evident in younger ages and tend to decrease with advancing age.
Borderline PD is associated with high mortality by suicide ( 9 , 10 , 49 ). There is also a high risk to die prematurely because of impulsive risk-taking, as well as succumbing to violence from others ( 9 , 10 ). Recurrent suicidal threats or attempts, when combined with fears of abandonment, are strongly indicative of the diagnosis ( 50 ). Even if these characteristics make borderline pattern PD easy to identify, the diagnosis is often overlooked. A key reason for this neglect is the perception that the overemotional, sometimes theatrical, and self-injurious behaviours are signs of wilfulness and manipulations rather than signs of an illness ( 51 ).
Borderline PD occasionally includes depressive and anxiety symptoms and mild irritability. In general, many persons with borderline PD describe recurrent occasions with panic anxiety, which may lead to suspicion of a primary panic disorder or generalized anxiety disorder. Likewise, experienced social discomfort and fears can arouse suspicion of primary social anxiety disorder. Finally, recent studies have suggested that attention deficit hyperactivity disorder (ADHD), bipolar disorder, and borderline PD have similar origins or share common pathological mechanisms ( 52 , 53 ).
Prevalence and longitudinal perspective
PDs are common in the general population. A recent overview ( 54 ), based on 13 studies conducted in the USA and Europe, reported prevalence figures varying from 3.9% to 15.5%. In the WHO World Mental Health Survey, carried out in 13 countries, the prevalence rate was 6.1% ( 55 ). The large variation in prevalence may be due to differences in sampling, diagnostic methods, and study settings. Furthermore, there may be differences in culture regarding significant personality pathology.
Persons in contact with the health-care system exhibit higher prevalence of PDs as compared with those not in contact. In fact, one-fourth of patients in primary care ( 56 ) and about half of those in psychiatric outpatient facilities fulfil the criteria for a PD ( 57 ).
PDs are equally common or more common in men ( 54 ) in the general population. In clinical settings, however, PDs are more often recognized in women, probably due to the higher rates of help-seeking behaviour in women ( 9 , 10 ).
Stability over time has long been a basic concept both in the description of personality and of PDs. Supporting this concept is the observation that there is rank-order stability over time in the expression of personality symptoms ( 58 ). On the other hand, an exaggeration in some personality traits over the life course and a decline in others have been observed ( 41 , 59 ). Furthermore, it has recently been shown that drugs affecting serotonin uptake can modulate personality traits ( 60 , 61 ). There is less support for the idea that a PD should be regarded as stable over time. Actually, modern research has shown that, although a maladaptive personality can be recognized early in life, it evolves continuously across the lifespan and is more plastic than previously believed ( 41 ). In addition, in the case of coexisting mental disorders, their contribution to the clinical picture will vary over time with the state of these disorders. In other words, even if personality traits are largely constant across time, there is a tendency that symptoms in persons with a PD change more over time than those without a PD. This change is often in the direction of improvement ( 62 , 63 ).
Diagnosis, differential diagnosis, and psychiatric comorbidity
Establishing a formal diagnosis of a PD is an issue for specialist psychiatry, where it must be regarded as a time-process function. The patient history must cover the life perspective to understand the current clinical landscape in context and against a background of the individual’s unique developmental history.
General and permanent problems in work, studies, and relationships are often primary and obvious observations. Difficulties in interpersonal relations are often visible already at the first patient encounter. Those difficulties justify a step-by-step deepening of the formal diagnostic work while initiating treatment efforts. Enhanced personal knowledge will also provide a more nuanced image of the patient’s problems as well as adaptive resources.
Accounts of the current problem and the patient’s current life situation are a natural starting point when collecting data on the clinical history of the patient. Special attention must be given to the risks of suicide and violence. The clinical history should be expanded in a piecemeal manner on appropriate occasions.
During the diagnostic process, it often becomes clear that the patient presents with criteria for several disorders, both within and outside the PD spectrum. Such comorbidity is common ( 64 , 65 ); it is seen across the whole spectrum of PDs and other mental disorders and in general represents a broader pattern of symptoms as well as a more severe condition. This is reflected in the observation that the total number of fulfilled criteria for any PD is related to the observed dysfunction and to the reported QoL ( 54 ). Comorbidity between PDs and other mental disorders contributes significantly to functional impairment ( 64 ) while also increasing the risk of early mortality ( 9 , 10 ).
Because of this characteristic, it is not uncommon that the person who fulfils criteria for a diagnosis of PD will seek health care for another mental disorder, a fact that might be misleading during the diagnostic process. Not too infrequently, there are rapid onset depressive or anxiety states that motivate the care episode during which the coexisting problems related to a comorbid PD are apparent. A more pressing issue is comorbidity of a more enduring character. For example, a coexisting ADHD can obscure the clinical symptoms of borderline PD. Conversely, a severe and prolonged eating disorder may dominate over an underlying personality pathology.
When the symptomatic picture of PD is complex and partially overlapping between different diagnoses, it is seldom possible to distinguish between different underlying pathologies. The differential diagnostic procedure will therefore be more about evaluating the relative influence of the various demonstrable expressed symptoms on the severity of functioning.
To optimize diagnostic accuracy self-assessment tools, semi-structured interviews and personality inventories can be used. The SCID-II is such an interview support for personality diagnosis according to the DSM-IV and DSM-5 ( 66 ).
Personality disorders and health
The long-term negative effects of having a PD are significant ( 9 , 10 , 41 , 54 ). Furthermore, because a PD is often overlooked diagnostically, the potential risks for the bearer may go undetected. A certain proportion of those who fulfil the criteria for a diagnosis of PD will ultimately have psychiatric care, while almost everyone eventually comes in contact with primary care or specialized somatic care. Given that personality-related problems lead to varying degrees of lack of adaptivity in interaction with other people, there are often complications in the contacts with health care and social services.
Personality traits are well known to impact health-related QoL ( 8 ) and outcome in health care. The negative consequence of having a high degree of neuroticism has been extensively studied ( 67 ). The consequence of having a PD is, however, only well studied in psychiatric care, where a multitude of studies have shown that having a comorbid PD represents a more severe condition with a worse prognosis as stated above.
There has been less focus on somatic care. Still, it has been shown that having a PD is related to higher rates of pain, greater use of analgesics, and more primary care, taken together suggesting an increase in somatic morbidity ( 68 ). It has also been shown that the outcome of treatment for somatic ill health is usually worse in the presence of a PD ( 68 ). For instance, having a PD was found to increase the risk of stroke ( 69 ) and coronary artery disease ( 70 , 71 ). Furthermore, having any PD is strongly associated with severe occupational outcomes, including disability benefits, regardless of disability diagnosis ( 72–74 ).
The most studied PD in this respect is borderline PD. Persons with this PD tend to be impulsive, and where self-harm is common they have an increased tendency to seek health care ( 75 ). Borderline PD, however, is related to an increased risk for several health problems, and consequently for a greater consumption of health care ( 76–79 ).
Based on previous knowledge that individuals with a PD have a higher mortality rate and a shorter life expectancy compared with the general population ( 69 , 80–83 ), we recently assessed to what extent this was related to type of PD or cause of death ( 9 , 10 ). Data from nationwide Swedish hospital registers with a follow-up of 25 years were used. Overall, all-cause standardized mortality ratios (SMRs) were found to increase in all clusters of PDs for natural and unnatural causes of death. The overall SMR was 6.1 in women and 5.0 in men, figures in line with those previously reported for anorexia nervosa, with higher rates in cluster B and unspecified/other PDs. The increased mortality ratio was seen for all somatic causes of death, reflecting the impact of having a PD on somatic health and wellbeing. The SMR for suicide was as high as 34.5 in women and 16.0 in men for cluster B disorders. Somatic and psychiatric comorbidity increased SMRs further. This excess mortality was also observed for most patients diagnosed with PDs not severe enough to lead to hospitalization ( 9 ). This observation contradicts the idea that only those persons with a PD severe enough to motivate inpatient treatment are burdened by an increased risk in mortality. This has important practical implications in that most patients with clinical problems linked to a PD are only treated as outpatients. On the other hand, the risk of death in those only treated as outpatients was clearly less than in those who received inpatient care, supporting the view of a difference in clinical severity between these groups ( 47 ).
Aspects on handling and treatment
Given the impact of PDs on treatment outcomes in somatic and mental health care, a clinical pattern suggesting the existence of such a disorder should be identified in primary or somatic specialist care ( 84 ). Treatment is a concern for specialist psychiatry, however. A well-developed liaison psychiatry, a subspecialty of psychiatry, is particularly suitable.
Even if treatment modalities are not the topic of this survey, some basic principles must be addressed. Because PDs are deeply ingrained ways of thinking and behaving that evolved as the personality developed, they are considered difficult to treat. In recent years several studies have emerged that have, to some extent, changed this concept ( 85–88 ). In general, there are many challenges and no simple solution in the treatment of PDs. At the same time, because fundamental problems of PDs are related to interpersonal relations, a structured and stable relationship between the patient and the clinician is the basis for any successful approach. This ‘therapeutic alliance’ looms as the strongest predictor of successful outcome of any treatment attempt. The most difficult challenge for the clinician is to achieve this goal. In fact, the pre-existing quality of the patient’s relationships, rather than the type of PD, is the single factor that most affects the quality of this alliance ( 89 ). The strength of this alliance is crucial not only to obtain anamnestic information about the true history of the problems encountered but also to motivate and maintain adherence to treatment.
Although there is only a paucity of randomized controlled studies on the effect of psychotherapy in PD ( 90 ), the few studies published suggest that it should be the core treatment ( 91 ), leading to individual benefit and a reduction in care costs ( 92 ).
Currently, no pharmaceuticals are registered for use in PDs. Any attempt to apply a pharmacological approach is therefore an issue for the psychiatric specialist. Such an approach should aim to reduce or eliminate specific symptoms seen in other psychiatric disorders, where there is evidence that the drug in question is efficient. Irrespective of which drug is used, the clinical effect should be closely monitored.
The underlying hypothesis when attempting psychotropic pharmaceuticals in the treatment of PDs is the assumption that the features and attributes associated with the clinical expression are linked to biochemical abnormalities and thus can be regulated by psychotropic drugs. For most specific PDs, studies on the putative benefit of pharmaceuticals are lacking, and where studies have been done the results are at best modest ( 93 ). Despite this limitation, most psychiatrists can testify about patients with PDs who are prescribed many drugs, often over a long time, and without information about the expected or obtained benefit, or how the treatment was followed up. Such polypharmacy, particularly in combination with poor documentation, can put patients at risk of adverse drug events (side effects) and interactions. In other words, drugs should never be the first-line treatment but may be justified as a supplement to other treatment forms in specific situations.
PD frequently goes undetected, in the shade of other health problems or diseases. PD is a predictor of worse health, premature death, and more serious life issues. It constitutes a challenge to health-care professionals and, above all, a burden for the patient, the family, and society. PD involves deviations in cognition, affectivity, interpersonal functioning, and/or impulse control. Deepened knowledge requires intellectual approaches based on sociodemographic, as well as epidemiological and advanced genetic and imaging techniques.
There is a clinical shift from an earlier focus on the characteristics of discrete PD entities to an awareness of the common features of different PDs, the suffering of patients, and the many problems they face in interpersonal relationships and daily life. The new ICD-11 classification aims to improve the description of the severity of problems encountered by patients.
Knowledge of the clinical aspects of PDs in general health care, vigilance to symptoms of PD, and appropriate diagnosis are all essential for optimal support to affected patients.
Disclosure statement
No potential conflict of interest was reported by the authors.
Note on the contributor
Lisa Ekselius, MD, PhD, is a Full Professor of Psychiatry at Uppsala University and a Senior Consultant in Psychiatry at the Uppsala University Hospital. Her research is focused on issues related to personality and personality disorders. These include the epidemiology of personality disorders, but also the contribution of personality traits to the expression of somatic as well as psychiatric disorders, and to the vulnerability to recover from major body trauma.
REVIEW article
Twenty years of research on borderline personality disorder: a scientometric analysis of hotspots, bursts, and research trends.
- 1 Department of Psychology, School of Humanities and Social Sciences, Anhui Agricultural University, Hefei, China
- 2 College of Computing & Informatics, Drexel University, Philadelphia, PA, United States
- 3 Department of Psychology, School of Education, China University of Geosciences, Wuhan, China
- 4 Department of Information Management, Anhui Vocational College of Police Officers, Hefei, China
Borderline personality disorder (BPD), a complex and severe psychiatric disorder, has become a topic of considerable interest to current researchers due to its high incidence and severity of consequences. There is a lack of a bibliometric analysis to visualize the history and developmental trends of researches in BPD. We retrieved 7919 relevant publications on the Web of Science platform and analyzed them using software CiteSpace (6.2.R4). The results showed that there has been an overall upward trend in research interest in BPD over the past two decades. Current research trends in BPD include neuroimaging, biological mechanisms, and cognitive, behavioral, and pathological studies. Recent trends have been identified as “prevention and early intervention”, “non-pharmacological treatment” and “pathogenesis”. The results are like a reference program that will help determine future research directions and priorities.
1 Introduction
Borderline personality disorder (BPD) is a complex and severe psychiatric disorder characterized by mood dysregulation, interpersonal instability, self-image disturbance, and markedly impulsive behavior (e.g., aggression, self-injury, suicide) ( 1 ). In addition, people with BPD may have chronic, frequent, random feelings of emptiness, fear, and so on. These symptoms often lead them to use unhealthy coping mechanisms in response to negative emotions, such as alcohol abuse ( 2 ). BPD has a long course, which makes treatment difficult and may have a negative impact on patients’ quality of life ( 3 ). Due to its clinical challenge, BPD is by far the most studied category of personality disorder ( 4 ). This disorder is present in 1−3% of the general population as well as in 10% of outpatients, 15−20% of inpatients, and 30−60% of patients with a diagnosed personality disorder, and has a suicide rate of up to 10% ( 5 , 6 ). Families of individuals with serious mental illness often experience distress, and those with relatives diagnosed with BPD tend to carry a heavier burden compared to other mental illnesses ( 7 , 8 ). As early as the 20th century, scholars began describing BPD and summarizing its symptoms. However, there was some debate regarding the precise definition of BPD.
In the past few decades, the research community has made remarkable progress in the study of BPD, equipping us with a wider range of perspectives and tools for understanding this intricate condition. However, numerous challenges still remain to be tackled by researchers. Diagnosing BPD is inherently challenging and often more difficult than anticipated. The symptoms of BPD are complex, diverse, and often overlap with those of other mental health conditions. For example, individuals with BPD may experience extreme mood swings similar to those observed in individuals with bipolar disorder ( 9 ); At the same time, they may also be entrenched in long-term depression, making it easy for doctors to initially misdiagnose them with depression ( 10 ). Because these symptoms overlap and interfere with each other, doctors often face the risk of misdiagnosing or overlooking the condition during initial diagnosis. Therefore, researchers are working to develop more accurate and comprehensive diagnostic tools and methods.
According to the “Neuro-behavioral Model” proposed by Lieb ( 1 ), the process of BPD formation is very complex and is determined by the interaction of several factors. The interaction between different factors can be complex and dynamic. Genetic factors and adverse childhood experiences may contribute to emotional disorders and impulsivity, leading to dysfunctional behaviors and inner conflicts. These, in turn, can reinforce emotional dysregulation and impulsivity, exacerbating the preexisting conditions. Genetic factors are an important factor in the development of BPD ( 11 ). Psychosocial factors, including adverse childhood experiences, have also been strongly associated with the development of BPD ( 12 ). Emotional instability and impulsive behavior are even more common in patients with BPD ( 13 ). The current study is based on the “Neuro-behavioral Model” and conducts a literature review of previous scientific research on BPD through bibliometric analysis to reorganize the influencing factors. Through large-sample data analysis, the association between BPD and other diseases is explored, which contributes to further refining this theory’s explanation of the common neurobiological mechanisms among various mental illnesses.
It is worth noting that with the development of BPD, some scholars have conducted bibliometrics studies on BPD to provide insights into this academic field. To date, the current study has identified two published bibliometric studies on the field: One is Ilaria M. A. Benzi and her colleagues’ 2020 metrological analysis of the literature in the field of BPD pathology for the period 1985−2020 ( 14 ). The other is a bibliometric analysis by Taylor Reis and his colleagues of the growth and development of research on personality disorders between 1980 and 2019 ( 15 ). Ilaria M. A. Benzi and her colleagues integrated and sorted out the research results of borderline personality pathology, and revealed the research results and development stages in this field through the method of network and cluster analysis. The results of the study clearly demonstrate that the United States and European countries are the main contributors, that institutional citations are more consistent, and that BPD research is well developed in psychiatry and psychology. At the same time, the development of research in borderline personality pathology is demonstrated from the initial development of the construct, through studies of treatment effects, to the results of longitudinal studies. Taylor Reis and his colleagues used a time series autoregressive moving average model to analyze publishing trends for different personality disorders to reveal their historical development patterns, and projected the number of publications for the period 2024 to 2029. The study finds a trend towards diversity in the research and development of personality disorders, with differences in publication rates for different types of personality disorders, and summarizes the reasons that influence these differences. This may ultimately determine which personality disorders will remain in future psychiatric classifications. These studies have provided valuable insights into the evolution of BPD, focusing primarily on its pathology or a broader personality disorder perspective. While basic bibliometric analyses of these studies have been conducted, there is a need for more in-depth investigations of specific trends in the evolution of BPD and a clearer delineation of emerging research foci. Therefore, in order to enhance the current study, this study extends the analysis to 2022 and utilizes a comprehensive structural variation analysis of the literature using scientometric methods. Building on previous bibliometric studies, we expect to provide new insights and additions to research in this area. At the same time, the research trends and hot topics in the field of BPD are further explored. In addition, several cocitation-based analyses are also carried out in order to better understand citation performance.
2.1 Objectives
One of our goals was to understand the current status and progress of researches on BPD, and to summarize the latest developments and research findings in BPD, such as new treatment methods and disease mechanisms. Through the intuitive presentation of knowledge graphs and other images or data, we aimed to provide clinical practice and research guidance for clinicians, researchers, and policymakers.
Our second goal was to help identify future research directions and priorities, and provide more scientific and systematic research guidance for researchers. For example, by identifying hotspots and associations in certain research areas, we can determine the fields and issues that require further investigations, thus providing clearer directions and focus for researches. Additionally, through bibliometric analysis, we can provide researchers with more targeted and practical research strategies and methods, improving research efficiency and the quality of research outcomes.
2.2 Search strategy and data collection
The selection of appropriate methods and tools in the process of analyzing research information is crucial. Web of Science (WOS) is a popular database for bibliometric analysis that includes numerous respectable and high-impact academic journals. In addition, data information, such as references and citations, is more extensive than other academic databases ( 16 ). Data collection took place on the date of May 10, 2023. The search strategy included the following: topic=“Neuro-behavioral Model” or “borderline characteristics” or “borderline etiology” or “borderline personality disorder”, database selected=WOS Core Collection, time span=2003−2022, index=Science Citation Index Expanded (SCI-EXPENDED) and Social Sciences Citation Index (SSCI). The “Neuro-behavioral Model” serves as a theoretical framework that is useful for explaining the development and pathophysiology of BPD; “borderline characteristics” can describe the related symptoms and features of BPD; “borderline etiology” helps to understand the factors that contribute to the development of BPD; “borderline personality disorder” is the most commonly used terms in relevant research. Using these as keywords in title searches can help researchers find researches related to BPD more accurately, facilitating deeper understanding of the characteristics, pathophysiology, etiology, and other aspects of BPD. In the current study, we focused only on two types of literature: articles and review articles, and limited the language to English. After removing all literature unrelated to BPD, a total of 7919 records met the criteria. They were exported in record and reference formats, and saved in plain text file format.
2.3 Data analysis and tools
Bibliometrics was first proposed by Alan Pritchard in 1969, as a method that combines data visualization to analyze publications statistically and quantitatively in specific fields and journals ( 17 ). Bibliometric analysis is a good way to analyze the trend of knowledge structure and research activities in scientific fields over time, and has been widely used in various fields since it was first used ( 18 ). Scientometrics is the application of bibliometrics in scientific fields, and it focuses on the quantitative characteristics and features of science and scientific researches ( 19 ). Compared to traditional literature review studies, visualized knowledge graphs can accurately identify key articles from many publications, comprehensively and systematically combing existing research in a field ( 20 ).
Currently, two important academic indicators are included in research. The impact factor (IF) is used as an indicator of a publication’s impact to assess the quality and importance of the publication ( 21 ). However, some researchers believe that IF has defects such as inaccuracy and misuse ( 22 ). Although many researchers have proposed to replace the impact factor with other indicators, IF is still one of the most effective ways to measure the impact of a journal ( 23 ). The IF published in the 2021 Journal Citation Reports were used. Another indicator is the H-index, which is an important measure of a scholar’s academic achievements. Some researchers consider it as a correction or supplement to the traditional IF ( 24 ).
All data were imported into CiteSpace (6.2.R4) and Scimago Graphica (1.0.30) for analysis. CiteSpace was used to obtain collaboration networks and impact networks. Scimago Graphica was used to construct a network graph of country collaboration. CiteSpace is a Java-based software developed in the context of scientometrics and data visualization ( 25 ). It combines scientific knowledge mapping with bibliometric analysis to determine the progress and current research frontiers in a particular field, as well as predict the development trends in that field ( 26 ). Scimago Graphica is a no-code tool. It can not only perform visualization analysis on communication data but also explore exploratory data ( 27 ). Currently, it is used for visual analysis of national cooperation relationships, displaying the geographic distribution of countries and publication trends.
3.1 Analysis of publication outputs, and growth trend prediction
Annual publications can provide an overview of the evolution of a research area and its progress ( 28 ). We retrieved 7919 articles from the WOS database on BPD between 2003 and 2022, including 6834 research articles and 1085 reviews ( Figure 1 ). As of the search date, these articles had received a total of 289,958 citations, equating to an average of 14,498 citations per year. Over the past two decades, the number of research articles published on BPD has shown a fluctuating upward trend. In addition, citations to these publications have increased significantly. A polynomial curve fit of the literature on BPD clearly indicates a strong correlation between the year of publication and the number of publications ( R 2 = 0.973). The number of research articles on BPD has indeed fluctuated and increased over the past two decades. This observation does, to some extent, indicate an upward trend, probably due to increasing interest in BPD. However, there are other factors to consider as well. For example, the accumulation of data or technological advances, government policies and corporate investment may also affect the direction of BPD research development.
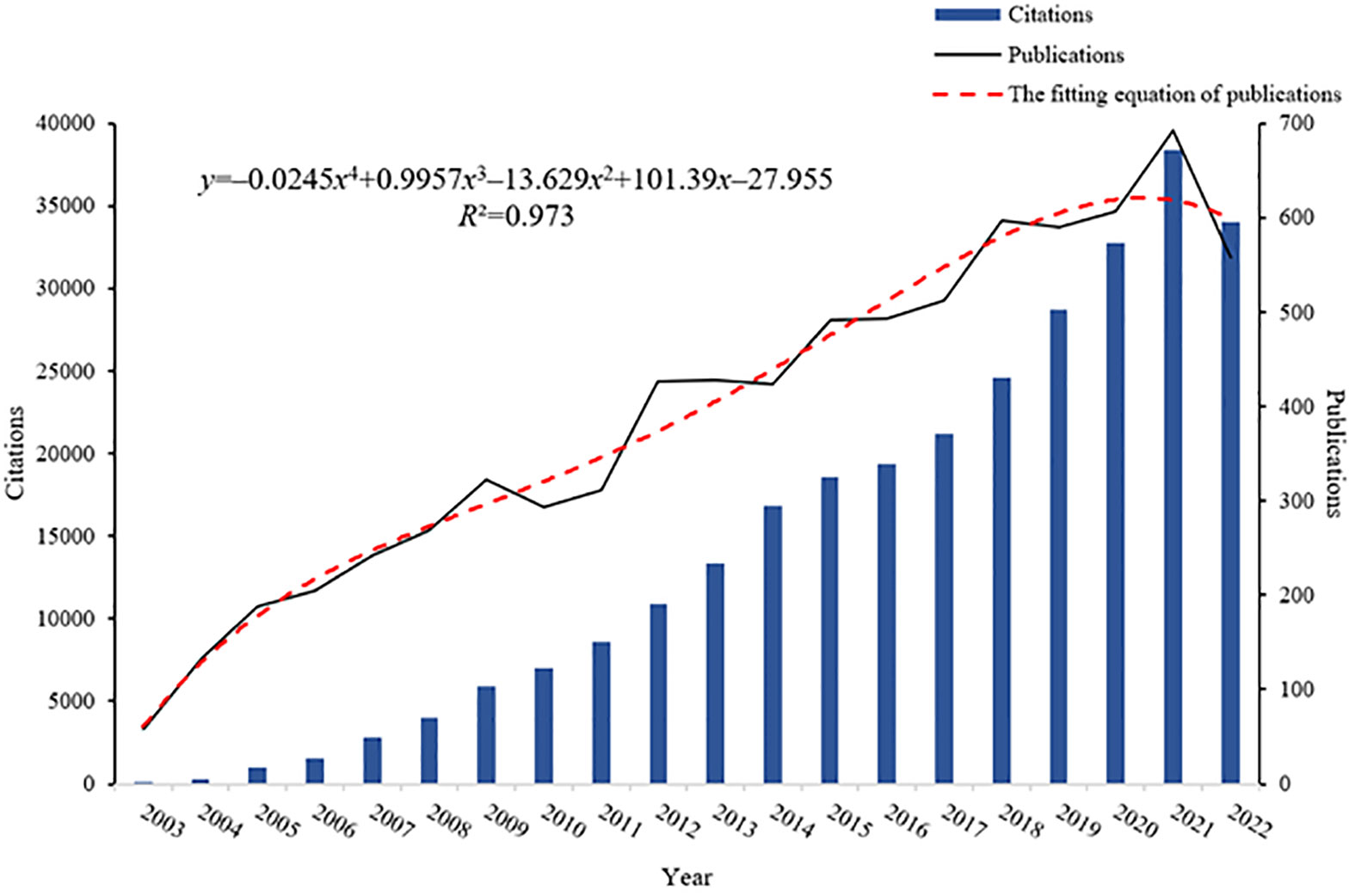
Figure 1 Annual publications, citation counts, and the fitting equation for annual publications in BPD.
3.2 Analysis of co-citation references: clusters and timeline of research
Co-cited references, which are cited by multiple papers concurrently, are considered a crucial knowledge base in any given field ( 28 ). In the current study, CiteSpace clustering was utilized to identify common themes within BPD-related literature. Figure 2 presented a co-citation network of highly cited references between 2003 and 2022, comprising 1163 references. A time slice of 1 was used, with the g -index was set at k =25, which resulted in the identification of 14 clusters representing distinct research themes in BPD. The significant cluster structure is denoted by a modularity value ( Q value) of 0.7974, and the high confidence level in the clusters by an average profile value ( S value) of 0.9176.
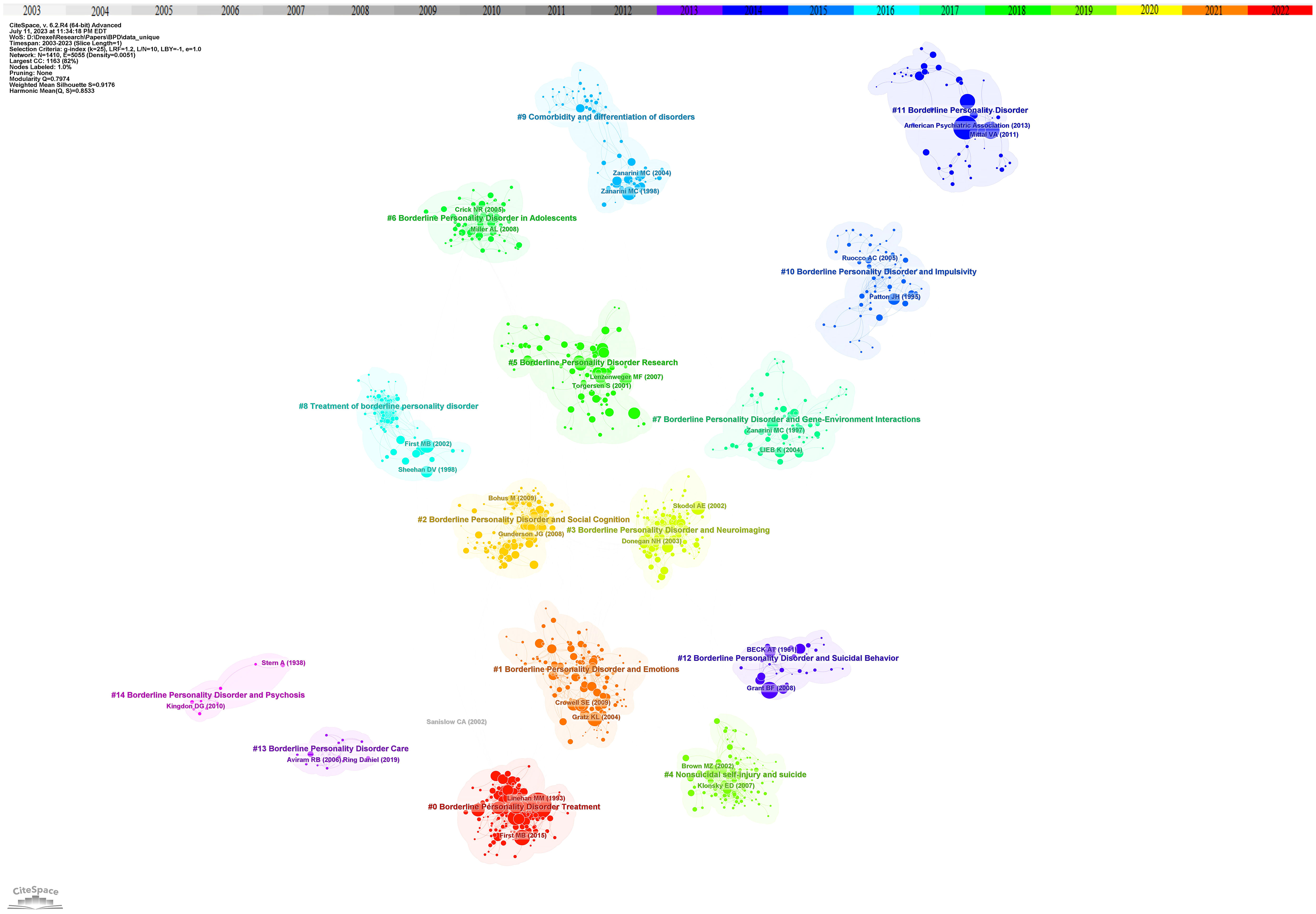
Figure 2 Reference co-citation network with cluster visualization in BPD. Trend 1 clinical researches, sub-trend clinical characteristics includes clusters #1, #2, #4, #10, #12; biological mechanisms include clusters #3, #7; nursing treatments includes clusters #0, #8, #13. Trend 2 associations and complications includes clusters #5, #6, #9, #11, #14.
Cluster analysis is performed through CiteSpace. Related clusters are classified into the same trend based on the knowledge of related fields and whether the clusters show similar trends. At the same time, based on the analysis of time series, to identify the movement of one cluster to another. Based on the cluster map of co-cited references on BPD, several different research trends were identified. The first major research trend is clinical research on BPD, which in turn consists of three sub-trends: clinical characterization of BPD, biological mechanisms, and nursing treatment. Of the data obtained, the earliest research on the clinical characterization of BPD began in 1992 with cluster #12, “borderline personality disorder and suicidal behavior” ( S =0.979; 1992). Paul H. Soloff and his colleagues conducted a comparative study of suicide attempts between major depressives and patients with BPD. The aim of this study was to develop more effective intervention strategies for suicide prevention ( 29 ). This cluster was further developed in cluster #4, “nonsuicidal self-injury and suicide” ( S =0.96; 2004). Thomas A. Widiger and Timothy J. Trull proposed a more flexible dimension-based categorization model to overcome the previous drawbacks of personality disorder categorization ( 30 ). Next in cluster #10 “borderline personality disorder and impulsivity” ( S =0.93; 2000), Jim H. Patton and his colleagues revised the Barratt Impulsivity Scale to measure impulsivity to facilitate practical clinical research ( 31 ). Related research continues to evolve into cluster #1 “borderline personality disorder and emotions” ( S =0.87; 2007) and cluster #2 “borderline personality disorder and social cognition” ( S =0.911; 2009), researchers have focused on understanding the causal relationship between BPD traits and factors such as social environment, emotion regulation, and interpersonal evaluative bias, as well as their potential impact ( 32 , 33 ). In the sub-trend of biological mechanisms, two main clusters are involved: cluster #7 “borderline personality disorder and gene-environment interactions” ( S =0.871; 2002) and cluster #3 “borderline personality disorder and neuroimaging” ( S =0.938; 2007). In the related cluster, researchers have found a relationship between BPD and genetic and environmental factors ( 34 ). Researchers have also utilized various external techniques to explore the degree of correlation between the risk of developing BPD and its biological mechanisms, aiming to reveal the complex mechanisms that influence the emergence and development of BPD ( 35 ). In nursing treatment, cluster #8 “treatment of borderline personality disorder “ ( S =0.968; 2001), Silvio Bellino and his colleagues systematically analyzed the current publications on BPD pharmacotherapy research and summarized relevant clinical trials and findings ( 36 ). However, due to the complexity of BPD, there is still a lack of information on the exact efficacy of pharmacotherapy in BPD, and therefore pharmacotherapy remains an area of ongoing development and research. This trend continues to be developed in cluster #0 “borderline personality disorder treatment” ( S =0.887; 2006), which emphasizes the development of novel pharmacotherapies for BPD. Cluster #13 “borderline personality disorder care” ( S =0.997; 2013) mainly focuses on the comprehensive care of people with borderline personality disorder and the education of patients and families. The goal is to improve patients’ quality of life, reduce self-injury and suicidal behavior, and promote full recovery.
The second major research trend is association and comorbidity. This trend first began in cluster #9 “comorbidity and differentiation of disorders” ( S =0.946; 1999). Mary C Zanarini and his colleagues explored the comorbidity of BPD with other psychiatric disorders on Axis I ( 37 ). Cluster #14 “borderline personality disorder and psychosis” ( S =0.966; 2003) also explored symptoms associated with BPD ( 38 ). This trend continues, with researchers studying BPD research in cluster #11 “borderline personality disorder” ( S =0.935; 2004) and cluster #5 “borderline personality disorder research” ( S =0.881; 2007) ( 39 , 40 ). In addition, cluster #6 “borderline personality disorder in adolescents” ( S =0.894; 2011) points out that the focus of BPD research is increasingly shifting towards adolescents ( 41 ).
Figure 3 showed the time span and research process of the developmental evolution of these different research themes. The temporal view reveals the newest and most active clusters, namely #0 “dialectical behavior therapy”, #1 “daily life”, and #2 “social cognition”, which have been consistently researched for almost a decade. Cluster #0 “dialectical behavior therapy” has the largest number and the longest duration, lasting almost 10 years. Similarly, this article by Rebekah Bradley and Drew Westen on understanding the psychodynamic mechanisms of BPD from the perspective of developmental psychopathology has the largest node ( 34 ).
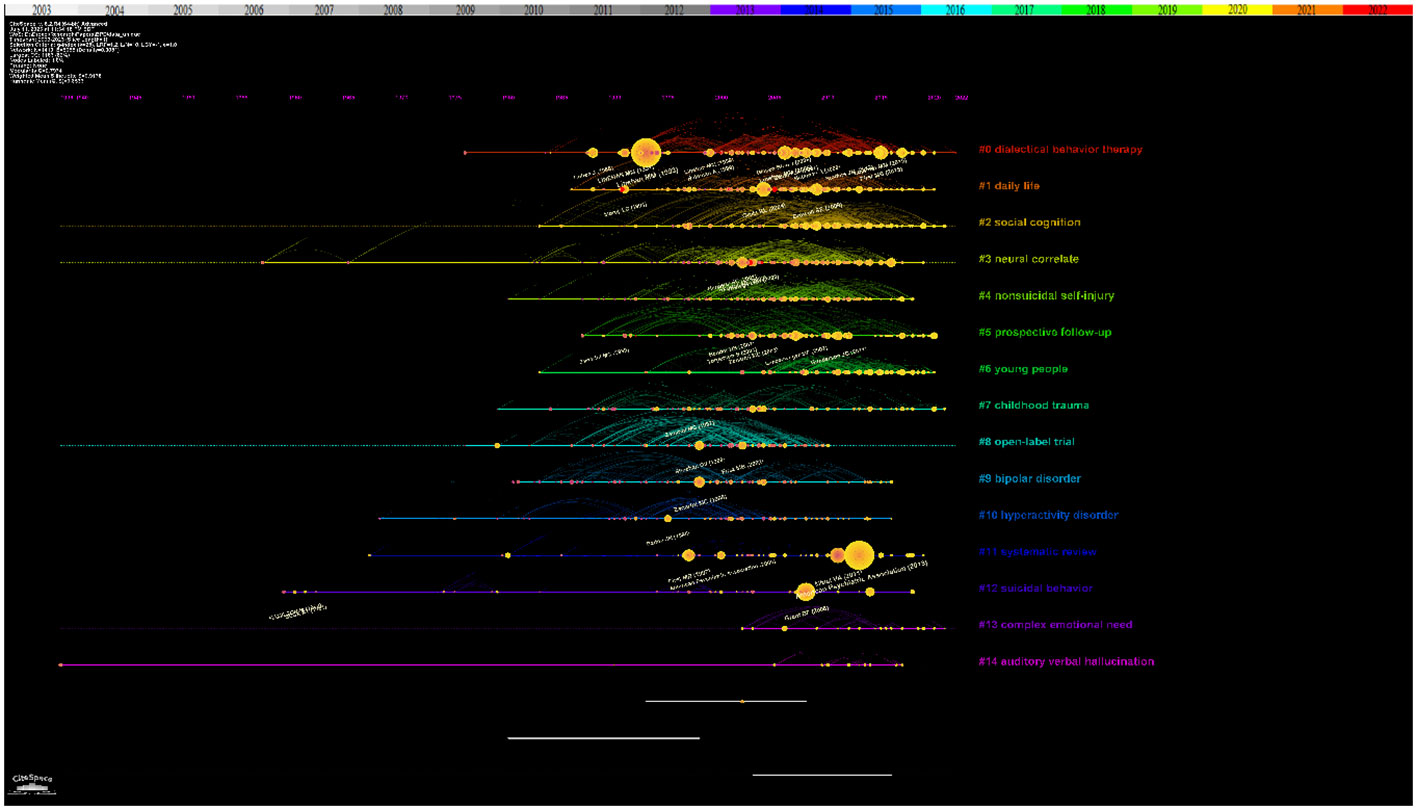
Figure 3 Reference co-citation network with timeline visualization in BPD.
3.3 Most cited papers
The top 10 highly cited papers on BPD research were presented in Table 1 . The most cited paper, by Marsha M. Linehan and colleagues, focus on the treatment of suicidal behavior in BPD ( 42 ). The transition between suicidal and non-suicidal self-injurious behavior in individuals with BPD has attracted researchers’s attention, mainly in cluster #4 “nonsuicidal self-injury and suicide” ( 52 ). The second is the experimental study by Josephine Giesen-Bloo and his colleagues on the psychotherapy of BPD ( 43 ). In cluster #0 “borderline personality disorder treatment” and Cluster #8 “treatment of borderline personality disorder”, researchers strive to find non-pharmacological approaches with comparable or enhanced therapeutic effects. This was followed by Sheila E. Crowell and her colleagues’ study of the biological developmental patterns of BPD ( 44 ). Research on the biological mechanisms and other contributing factors of BPD, including #7 “borderline personality disorder and gene-environment interactions” have been closely associated with the development of BPD ( 53 ).
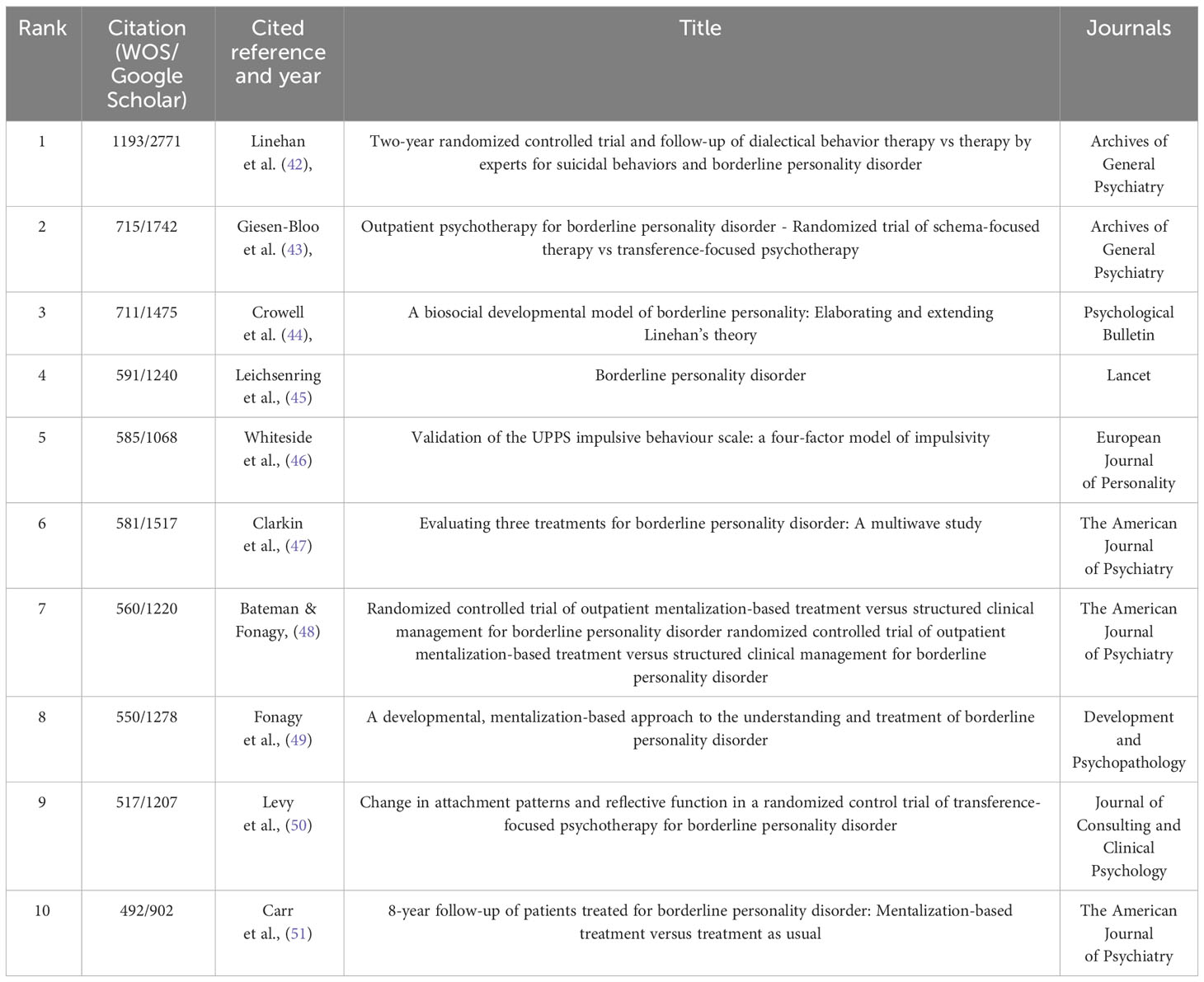
Table 1 Top 10 cited references that published BPD researches.
3.4 Burst analysis and transformative papers
The “citation explosion” reflects the changing research focus of a field over time and indicates that certain literature has been frequently cited over time. Figure 4 showed the top 9 references with the highest citation intensity. The three papers with the greatest intensity of outbursts during the period 2003−2022 are: The first is the American Psychiatric Association’s Diagnostic and Statistical Manual of Mental Disorders ( 54 ). In the second article, Vijay A. Mittal and Elaine F. Walker discuss key issues surrounding dyspraxia, tics, and psychosis that are likely to appear in an upcoming edition of the Diagnostic and Statistical Manual of Mental Disorders ( 39 ). In addition, Ioana A. Cristea and colleagues conducted a systematic review and meta-analysis to evaluate the effectiveness of psychotherapy for borderline personality disorder ( 55 ).
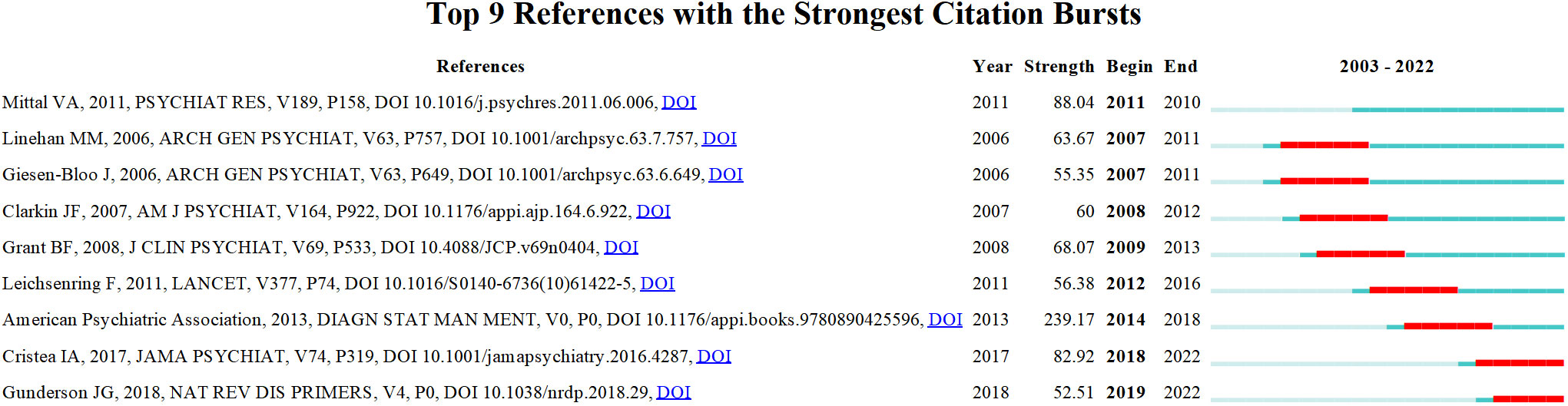
Figure 4 References with the strongest occurrence burst on BPD researches. Article titles correspond from top to bottom: Mittal VA et al. Diagnostic and Statistical Manuel of Mental Disorders; Linehan MM et al. Two-year randomized controlled trial and follow-up of dialectical behavior therapy vs therapy by experts for suicidal behaviors and borderline personality disorder; Giesen-Bloo J et al. Outpatient psychotherapy for borderline personality disorder: Randomized trial of schema-focused therapy vs transference-focused psychotherapy; Clarkin Jf et al. Evaluating three treatments for borderline personality disorder: A multiwave study; Grant BF et al. Prevalence, correlates, disability, and comorbidity of DSM-IV borderline personality disorder: Results from the Wave 2 National Epidemiologic Survey on Alcohol and Related Conditions; Leichsenring F et al. Borderline personality disorder; American Psychiatric Association, DSM-5 Task Force. Diagnostic and statistical manual of mental disorders: DSM-5™ (5th ed.); Cristea IA et al. Efficacy of psychotherapies for borderline personality disorder: A systematic review and meta-analysis; Gunderson JG et al. Borderline personality disorder.
Structural variation analysis can be understood as a method of measuring and studying structural changes in the field, mainly reflecting the betweenness centrality and sigma of the references. The high centrality of the reference plays an important role in the connection between the preceding and following references and may help to identify critical points of transformation, or intellectual turning points. Sigma values, on the other hand, are used to measure the novelty of a study, combining a combination of citation burst and structural centrality ( 56 ). Table 2 listed the top 10 structural change references that can be considered as landmark studies connecting different clusters. The top three articles with high centrality are the studies conducted by Milton Z. Brown and his colleagues on the reasons for suicide attempts and non-suicidal self-injury in BPD women ( 57 ); the research by Nelson H. Donegan and his colleagues on the impact of amygdala on emotional dysregulation in BPD patients ( 59 ); and the fMRI study by Sabine C. Herpertz and her colleagues on abnormal amygdala function in BPD patients ( 61 ). In addition, publications with high sigma values are listed. They are Larry J. Siever and Kenneth L. Davis on psychobiological perspectives on personality disorders ( 58 ); Ludger Tebartz van Elst and his colleagues on abnormalities in frontolimbic brain functioning ( 60 ); and Marsha M. Linehan on therapeutic approaches in BPD research ( 62 ). These works are recognized as having transformative potential and may generate some new ideas.
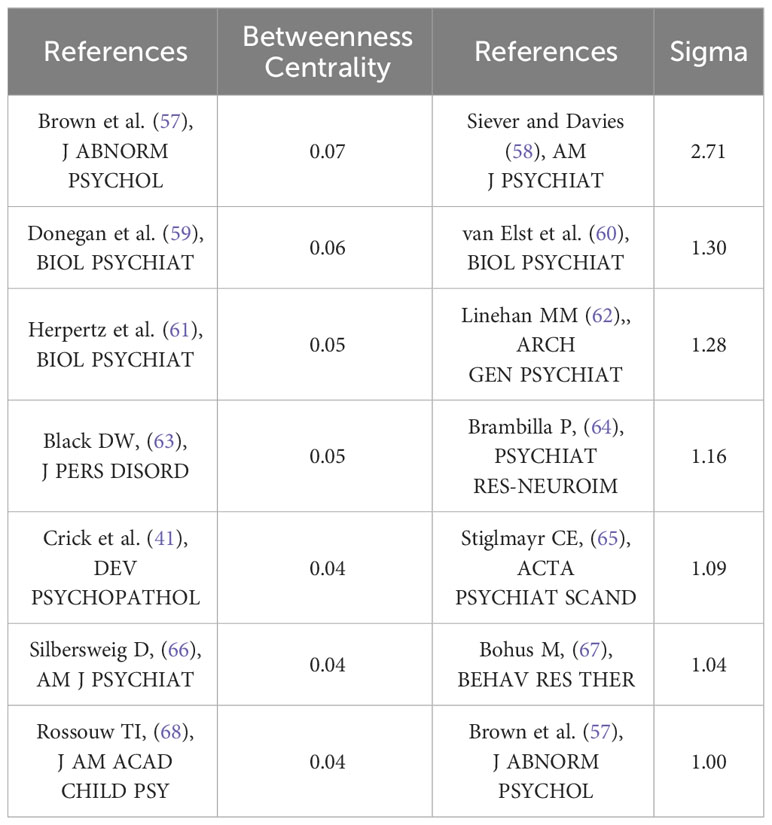
Table 2 Top 7 betweenness centrality and stigma references.
3.5 Analysis of authors and co-authors
Figure 5 showed a map of the co-authorship network over the last two decades. In total, 10 different clusters are shown, each of which gathers co-authors around the same research topic. For example, the main co-authors of cluster #0 “remission” are Christian Schmahl, Martin Bohus, Sabine C. Herpertz, Timothy J. Trull and Stefan Roepke. More recently, the three authors with the greatest bursts of research have been Mary C. Zanarini, Erik Simonsen, and Carla Sharp. As shown in Table 3 , the three most published authors are Martin Bohus (145 publications; 1.83%; H-index=61), Mary C. Zanarini (144 publications; 1.82%; H-index=80) and Christian Schmahl (142 publications; 1.79%; H-index=54).
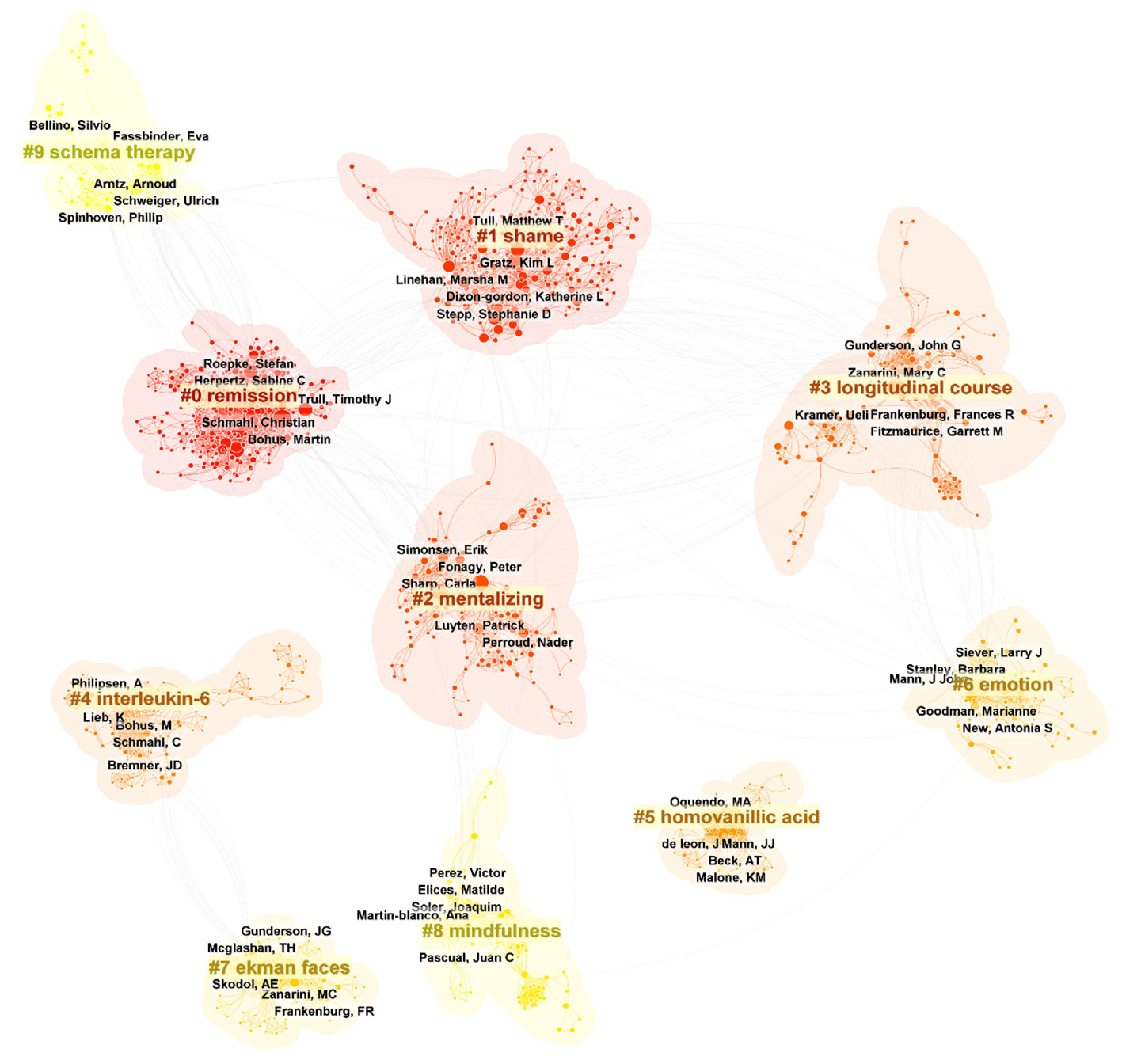
Figure 5 Top 10 clusters of coauthors in BPD (2003–2023). Selection Criteria: Top 10 per slice. Clusters labeled by keywords. The five authors with the highest number of publications in each cluster were labeled.
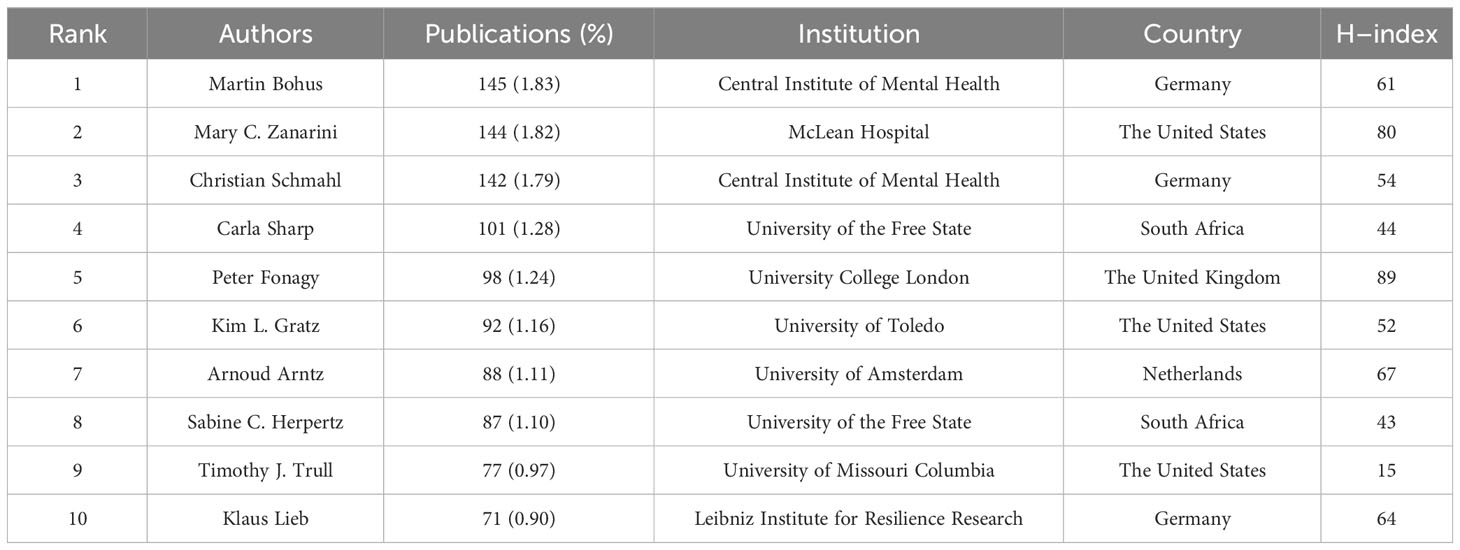
Table 3 Top 10 authors that published BPD researches.
3.6 Analysis of cooperation networks across countries
The top 10 countries in terms of number of publications in the BPD are added in Table 4 . With 3,440 published papers, or nearly 43% of all BPD research papers, the United States is the leading contributor to BPD research. This is followed by Germany (1196 publications; 15.10%) and the United Kingdom (1020 publications; 9.32%). Centrality refers to the degree of importance or centrality of a node in a network and is a measure of the importance of a node in a network ( 69 ). In Table 4 the United States is also has the highest centrality (0.43). Figure 6 shows the geographic collaboration network of countries in this field, with 83 countries contributing to BPD research, primarily from the United States and Europe.
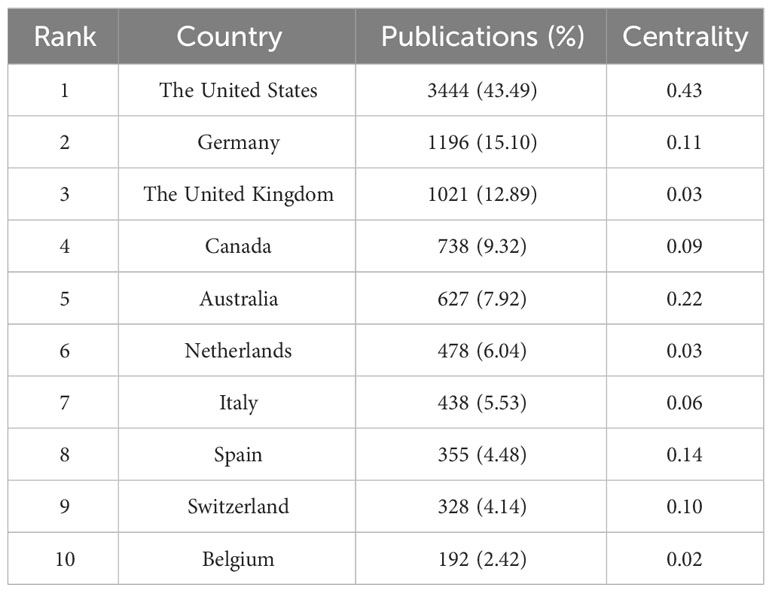
Table 4 Top 10 countries that published BPD researches.
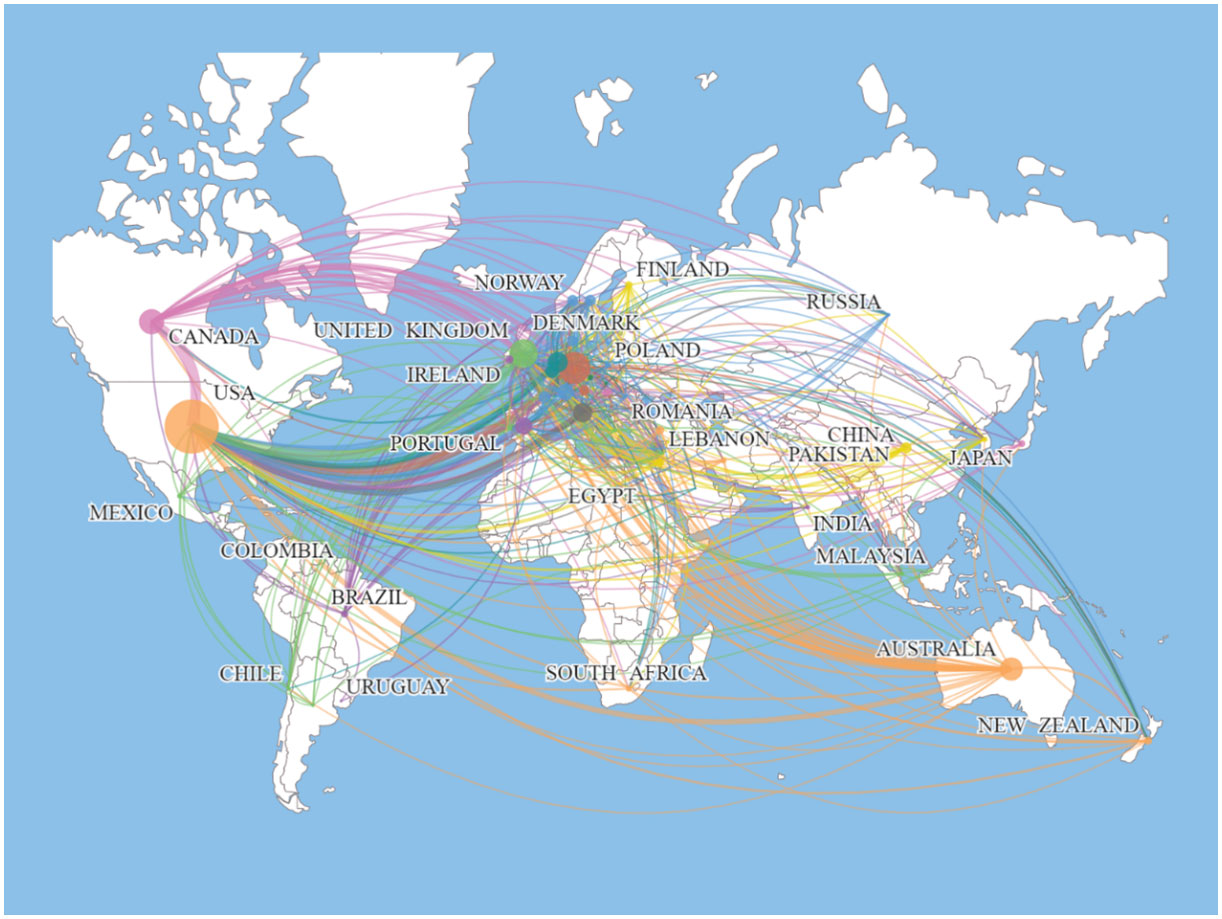
Figure 6 Map of the distribution of countries/regions engaged in BPD researches.
3.7 Analysis of the co-author’s institutions network
Table 5 listed the top 10 institutions ranked by the number of publications. The current study shows that Research Libraries Uk is the institution with the highest number of publications, with 766 publications (9.67%). The subsequent institutions are Harvard University and Ruprecht Karls University Heidelberg with 425 (5.37%) and 389 (4.91%) publications respectively. As can be seen from Table 4 , six of the top 10 institutions in terms of number of publications are from the United States. In part, this reflects the fact that the United States institutions are at the forefront of the BPD field and play a key role in it.
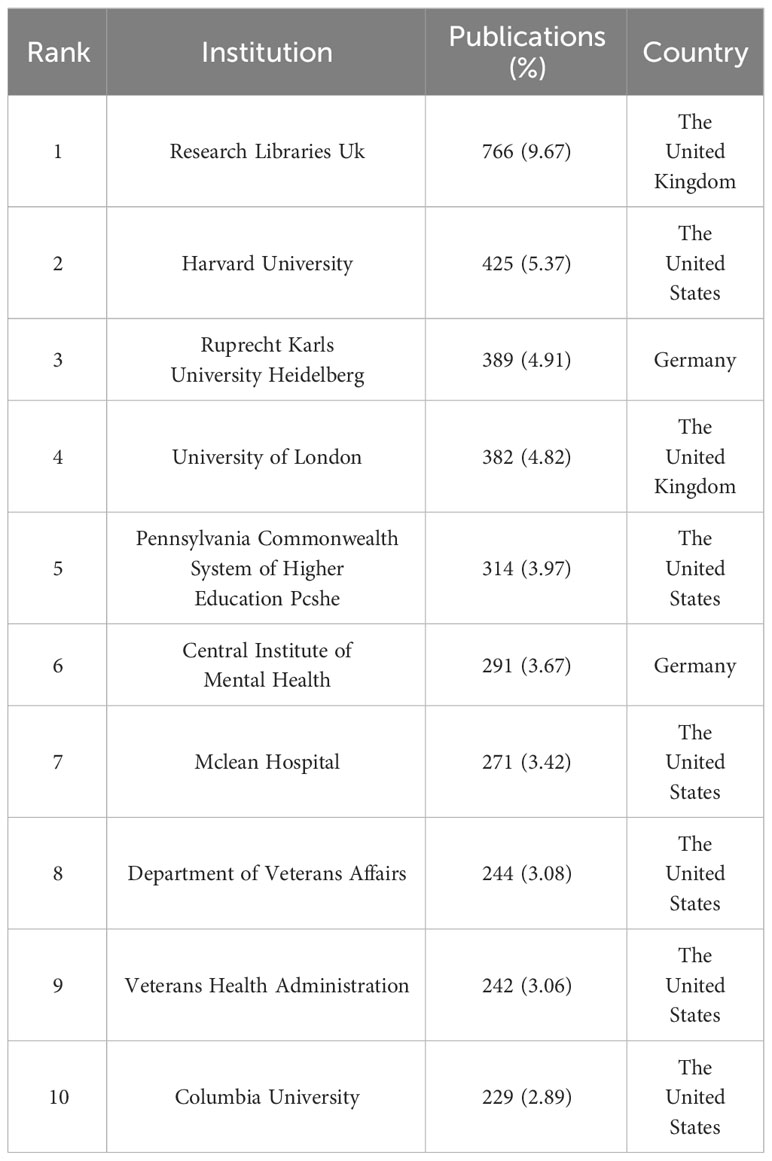
Table 5 Top 10 institutions that published BPD researches.
3.8 Analysis of journals and cited journals
If the more papers are published in a particular journal and at the same time it has a high number of citations, then it can be considered that the journal is influential ( 70 ). The top 10 journals in the field of BPD in terms of number of publications are listed in Table 6 . Journal of Personality Disorders from the Netherlands published the most literature on BPD with 438 (5.53%; IF=3.367) publications. This was followed by two journals from the United States: Psychiatry Research and Personality Disorders Theory Research and Treatment , with 269 (3.40%, IF=11.225) and 232 (2.93%; IF=4.627) publications, respectively. Among the top 10 journals in terms of number of publications published, Psychiatry Research has the highest impact factor.
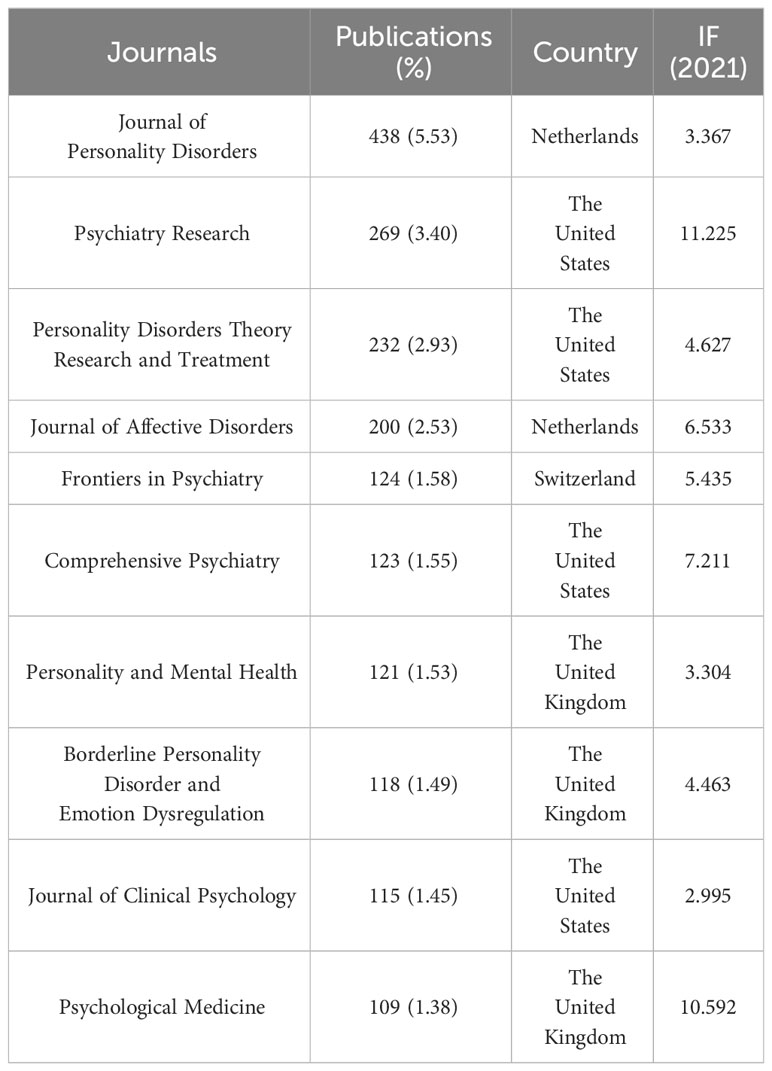
Table 6 Top 10 journals that published BPD researches.
3.9 Analysis of keywords and keywords co-occurrence
Keyword co-occurrence analysis can help researchers to understand the research hotspots in a certain field and the connection between different research topics. As shown in Figure 7 , all keywords can be categorized into 9 clusters: cluster #0 “diagnostic interview”, cluster #1 “diagnostic behavior therapy”, cluster #3 “social cognition”, cluster #4 “emotional regulation”, cluster #5 “substance use disorders “, cluster #6 “posttraumatic stress disorder”, cluster #7 “suicide” and cluster #8 “double blind”. These keywords have all been important themes in BPD research during the last 20 years.
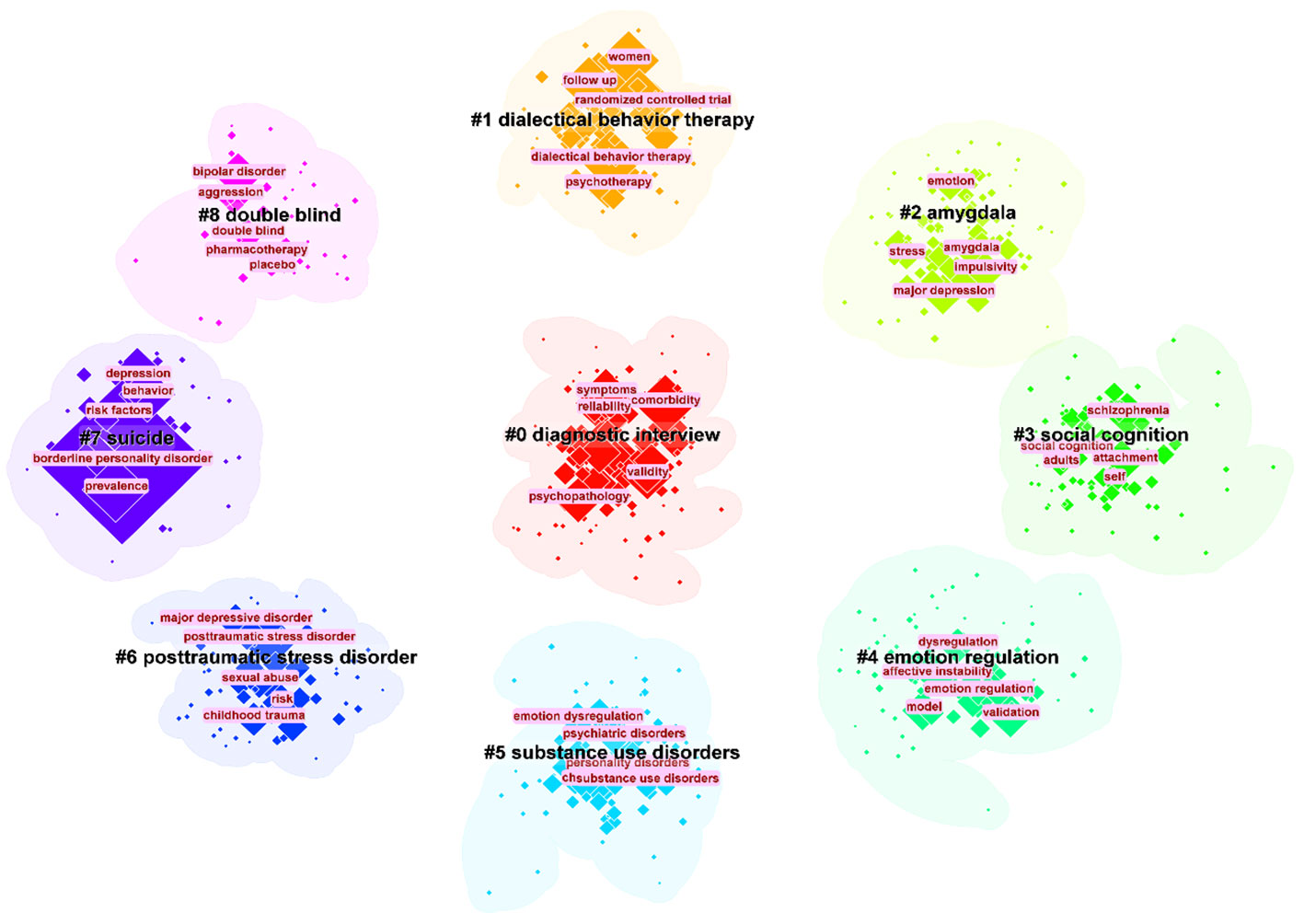
Figure 7 The largest 9 clusters of co-occurring keywords. The top 5 most frequent keywords in each cluster are highlighted.
Keyword burst is used to identify keywords with a significant increase in the frequency of occurrence in a topic or domain, helping to identify emerging concepts, research hotspots or keyword evolutions in a specific domain ( 71 ). Figure 8 presented the top 32 keywords with the strongest citation bursts in BPD from 2003−2023. Significantly, the keywords “positron emission tomography” (29.63), “major depression” (27.93), and “partial hospitalization” (27.1) had the highest intensity of outbreaks.
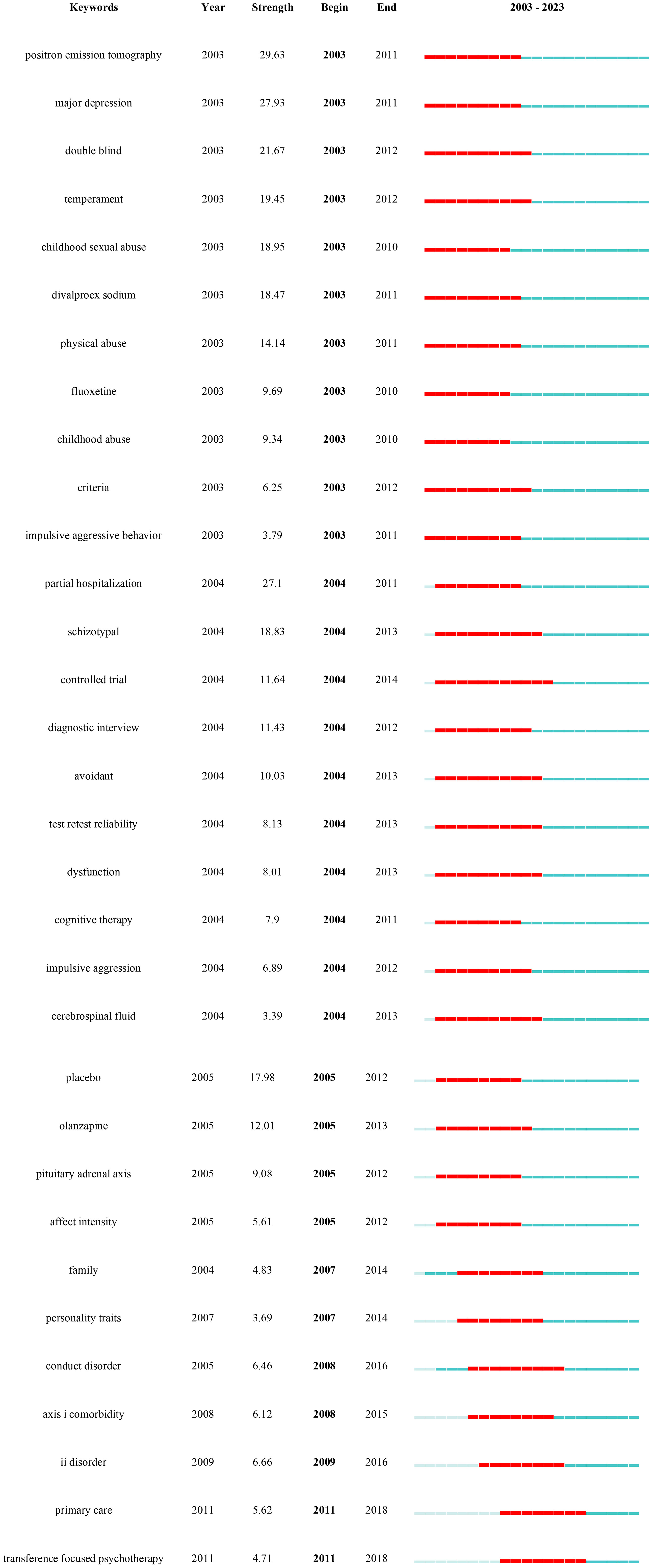
Figure 8 Keywords with the strongest occurrence burst on BPD researches.
4 Discussion
4.1 application of the “neuro-behavioral model” to bpd research.
In this study, we chose specific search terms, particularly “Neuro-behavioral Model”, to efficiently collect and analyze BPD research literature related to this emerging framework. This choice of keyword helped narrow the research scope and ensure its relevance to our objectives. However, it may have excluded some studies using different terminology, thus limiting comprehensiveness. In addition, the ‘Neuro-behavioral Model’, as an interdisciplinary field, encompasses a wide range of connotations and extensions, which also poses challenges to our research. This undoubtedly adds to the complexity of the study, yet it enhances our understanding of the field’s diversity.
4.2 Summary of the main findings
This current study utilized CiteSpace and Scimago Graphic software to conduct a comprehensive bibliometric analysis of the research literature on BPD. The study presented the current status of research, research hotspots, and research frontiers in BPD over the past 20 years (2003–2022) through knowledge mapping. The scientific predictions of future trends in BPD provided by this study can guide researchers interested in this field. This study also uses bibliometrics analysis method to show the knowledge structure and research results in the field of BPD, as well as the scientific prediction of the future trend of BPD research.
4.3 Identification of research hotspots
Previous studies have indicated an increasing trend in the number of papers focused on BPD, with the field gradually expanding into various areas. The first major research trend involves clinical studies on BPD. This includes focusing on emotional recognition difficulties in BPD patients, as well as studying features related to suicide attempts and non-suicidal self-injury. Clinical recognition and confirmation of BPD remains low, mainly related to the lack of clarity of its biological mechanisms ( 72 ). The nursing environment for BPD patients plays an important role in the development of the condition, which has become a focus of research. Researchers are also exploring the expansion of treatment options from conventional medication to non-pharmacological approaches, particularly cognitive-behavioral therapy. Another major research trend involves the associations and complications of BPD, including a greater focus on the adolescent population to reduce the occurrence of BPD starting from adolescence. Additionally, many researchers are interested in the comorbidity of BPD with various clinical mental disorders.
4.4 Potential trends of future research on BPD
Based on the results of the above studies and the results of the research trends in the table of details of the co-citation network clusters in 2022 ( Table 7 ), several predictions are made for the future trends in the field of BPD. In Table 7 , there were some trends related to previous studies, including #1”dialectical behavior therapy”, #7 “dialectical behavior therapy” ( 73 ), #5 “mentalization” ( 74 ), and #9 “non-suicidal self-injury” ( 75 ). The persistence of these research trends is evidence that they have been a complex issue in this field and a focus of researchers. The recently emerged turning point paper provides a comprehensive assessment about BPD, offering practical information and treatment recommendations ( 76 ). New research is needed to improve standards and suggest more targeted and cost-effective treatments.
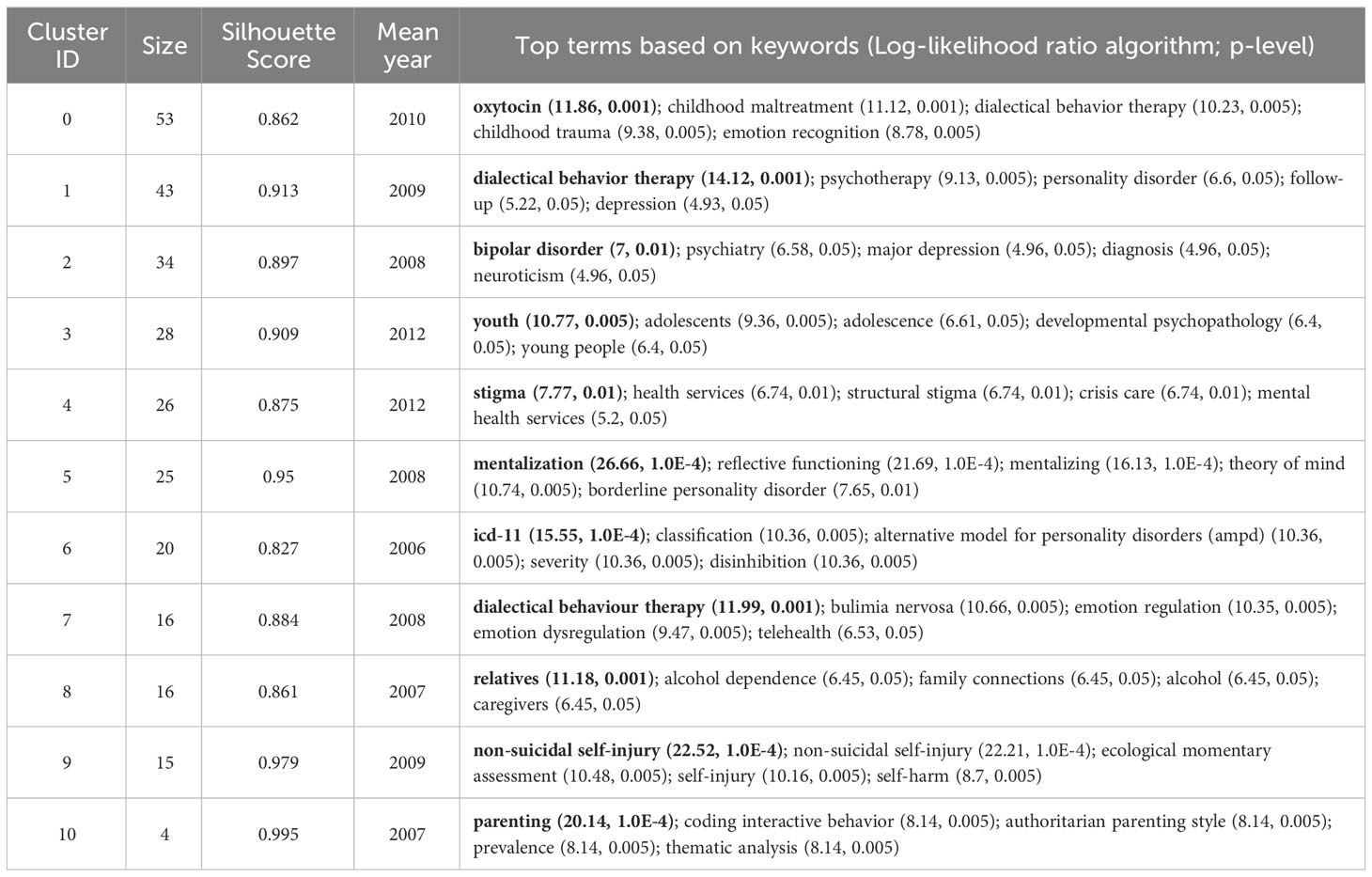
Table 7 The references co-citation network cluster detail (2022).
BPD symptoms in adolescents have been shown to respond to interventions with good results, so prevention and intervention for BPD is warranted ( 77 ). This trend can be observed in #3 “youth” ( 78 ). Mark F. Lenzenweger and Dante Cicchetti summarized the developmental psychopathology approach to BPD, one of the aims of which is to provide information for the prevention of BPD ( 79 ). Prevention and early intervention of BPD has been shown to provide many benefits, including reduced occurrence of secondary disorders, improved psychosocial functioning, and reduced risk of interpersonal conflict ( 80 ). However, there are differences between individuals, and different prevention goals are recommended for adolescents at risk for BPD. Therefore, prevention and early intervention for BPD has good prospects for the future.
The etiology of BPD is closely related to many factors, and its pathogenesis is often ignored by clinicians. The exploration of risk factors has been an important research direction in the study. Some studies have found that BPD is largely the product of traumatic childhood experiences, which may lead to negative psychological effects on children growing up ( 81 ). It has also been found that the severity of borderline symptoms in parents is positively associated with poor parenting practices ( 82 ). Future researches need to know more about the biological-behavioral processes of parents in order to provide targeted parenting support and create a good childhood environment.
Because pharmacotherapy is only indicated for comorbid conditions that require medication, psychotherapy has become one of the main approaches to treating BPD. The increasingly advanced performance and availability of contemporary mobile devices can help to take advantage of them more effectively in the context of optimizing the treatment of psychiatric disorders. The explosion of COVID-19 is forcing people to adapt to online rather than face-to-face offline treatment ( 83 ). The development of this new technology will effectively advance the treatment of patients with BPD. Although telemedicine has gained some level of acceptance by the general public, there are some challenges that have been reported, so further research on the broader utility of telemedicine is needed in the future.
4.5 The current study compares with a previous bibliometric review of BPD
As mentioned earlier, there have been previous bibliometric studies conducted by scholars in the field of BPD. This paper focuses more on BPD in personality disorders than the extensive study of personality disorders as a category by Taylor Reis et al. ( 15 ). The results of both studies show an increasing trend in the number of publications in the field of BPD, suggesting positive developments in the field. Taylor Reis et al. focused primarily on quantifying publications on personality disorders and did not delve into other specific aspects of BPD. Ilaria M.A. Benzi et al. focused on a bibliometric analysis of the pathology of BPD ( 14 ). They give three trends for the future development of BPD pathology: first, the growing importance of self-injurious behavior research; second, the association of attention deficit hyperactivity disorder with BPD and the influence of genetics and heritability on BPD; and third, the new focus on the overlap between fragile narcissism and BPD. The study in this paper also concludes that there are three future development directions for BPD: first, the prevention and early intervention of BPD; second, the non-pharmacological treatment of BPD; and third, research into the pathogenesis of BPD. Owing to variations in research backgrounds and data sources, the outcomes presented in the two studies diverge significantly. Nevertheless, both contributions hold merit in advancing the understanding of BPD. In addition to this, this paper also identifies trends in BPD over the past 20 years: the first trend is the clinical research of BPD, which is specifically subdivided into three sub-trends; the second trend is association and comorbidity. The identification of these trends is important for understanding the disorder, improving diagnosis and treatment, etc. Structural variant analysis also features prominently in the study. The impact of literature in terms of innovativeness is detected through in-depth mining and analysis of large amounts of literature data. This analysis is based on research in the area of scientific creativity, especially the role and impact of novel reorganizations in creative thinking. Structural variation analysis is precisely designed to find and reveal embodiments of such innovative thinking in scientific literature, enabling researchers to more intuitively grasp the dynamics and cutting-edge advances in the field of science.
5 Limitations
However, it must be admitted that our study has some limitations. The first is the limited nature of data resources. The data source for our study came from only one database, WOS. Second, the limitation of article type. Search criteria are limited to papers and reviews in SCI and SSCI databases. Third, the effect of language type. In the current study, only English-language literature could be included in the analysis, which may lead us to miss some important studies published in other languages. Fourth, limitations of research software. Although this study used well-established and specialized software, the results obtained by choosing different calculation methods may vary. Finally, the diversity of results interpretation. The results analyzed by the software are objective, but there is also some subjectivity in the interpretation and analysis of the research results. While we endeavor to be comprehensive and accurate in our research, the choice of search terms inevitably introduces certain limitations. Using “Neuro-behavioral Model” as the search term enhances the study’s relevance, but it may also cause us to miss significant studies in related areas. This limits the generalizability and replicability of our results. Furthermore, the inherent complexity and diversity of neurobehavioral models might introduce subjectivity and bias in our interpretation and application of the literature. Although we endeavored to reduce bias via multi-channel validation and cross-referencing, we cannot entirely eliminate its potential impact on our findings.
6 Conclusion
Overall, a comprehensive scientometrics analysis of BPD provides a comprehensive picture of the development of this field over the past 20 years. This in-depth examination not only reveals research trends, but also allows us to understand which areas are currently hot and points the way for future research efforts. In addition, this method provides us with a framework to evaluate the value of our own research results, which helps us to more precisely adjust the direction and strategy of research. More importantly, this in-depth analysis reveals the depth and breadth of BPD research, which undoubtedly provides valuable references for researchers to have a deeper understanding of BPD, and also provides a reference for us to set future research goals. In short, this scientometrics approach gives us a window into the full scope of BPD research and provides valuable guidance for future research.
Author contributions
YL: Data curation, Formal analysis, Investigation, Methodology, Software, Visualization, Writing – original draft, Writing – review & editing. CC: Conceptualization, Data curation, Formal analysis, Investigation, Methodology, Project administration, Resources, Software, Supervision, Validation, Visualization, Writing – original draft, Writing – review & editing. YZ: Validation, Visualization, Writing – review & editing. NZ: Conceptualization, Data curation, Formal analysis, Investigation, Methodology, Project administration, Software, Supervision, Validation, Visualization, Writing – original draft, Writing – review & editing. SL: Conceptualization, Data curation, Formal analysis, Funding acquisition, Investigation, Methodology, Project administration, Resources, Software, Supervision, Validation, Visualization, Writing – original draft, Writing – review & editing.
The author(s) declare that financial support was received for the research, authorship, and/or publication of this article. SL is supported by the Outstanding Youth Program of Philosophy and Social Sciences in Anhui Province (2022AH030089) and the Starting Fund for Scientific Research of High-Level Talents at Anhui Agricultural University (rc432206).
Conflict of interest
The authors declare that the research was conducted in the absence of any commercial or financial relationships that could be construed as a potential conflict of interest.
Publisher’s note
All claims expressed in this article are solely those of the authors and do not necessarily represent those of their affiliated organizations, or those of the publisher, the editors and the reviewers. Any product that may be evaluated in this article, or claim that may be made by its manufacturer, is not guaranteed or endorsed by the publisher.
1. Lieb K, Zanarini MC, Schmahl C, Linehan MM, Bohus M. Borderline personality disorder. Lancet (2004) 364:453–61. doi: 10.1016/S0140-6736(04)16770-6
PubMed Abstract | CrossRef Full Text | Google Scholar
2. Chugani CD, Byrd AL, Pedersen SL, Chung T, Hipwell AE, Stepp SD. Affective and sensation-seeking pathways linking borderline personality disorder symptoms and alcohol-related problems in young women. J Pers Disord . (2020) 34:420–31. doi: 10.1521/pedi_2018_32_389
3. Bagge CL, Stepp SD, Trull TJ. Borderline personality disorder features and utilization of treatment over two years. J Pers Disord . (2005) 19:420–39. doi: 10.1521/pedi.2005.19.4.420
4. Paris J. Suicidality in borderline personality disorder. Medicina . (2019) 55:223. doi: 10.3390/medicina55060223
5. Lenzenweger MF, Lane MC, Loranger AW, Kessler RC. DSM-IV personality disorders in the National Comorbidity Survey Replication. Biol Psychiatry . (2007) 62:553–64. doi: 10.1016/j.biopsych.2006.09.019
6. Skodol AE, Gunderson JG, Pfohl B, Widiger TA, Livesley WJ, Siever LJ. The borderline diagnosis I: Psychopathology, comorbidity, and personality structure. Biol Psychiatry . (2002) 51:936–50. doi: 10.1016/s0006-3223(02)01324-0
7. Bailey RC, Grenyer BF. Burden and support needs of carers of persons with borderline personality disorder: A systematic review. Harvard Rev Psychiatry . (2013) 21:248–58. doi: 10.1097/HRP.0b013e3182a75c2c
CrossRef Full Text | Google Scholar
8. Weimand BM, Hedelin B, Sällström C, Hall-Lord M-L. Burden and health in relatives of persons with severe mental illness: A Norwegian cross-sectional study. Issues Ment Health Nursing . (2010) 31:804–15. doi: 10.3109/01612840.2010.520819
9. Saccaro LF, Schilliger Z, Dayer A, Perroud N, Piguet C. Inflammation, anxiety, and stress in bipolar disorder and borderline personality disorder: A narrative review. Neurosci Biobehav Rev . (2021) 127:184−192. doi: 10.1016/j.neubiorev.2021.04.017
10. Dixon-Gordon KL, Laws H. Emotional variability and inertia in daily life: Links to borderline personality and depressive symptoms. J Pers Disord . (2021) 35:162−171. doi: 10.1521/pedi_2021_35_504
11. Torgersen S. Genetics of patients with borderline personality disorder. Psychiatr Clinics North A . (2000) 23:1–9. doi: 10.1016/S0193-953X(05)70139-8
12. Quenneville AF, Kalogeropoulou E, Küng AL, Hasler R, Nicastro R, Prada P, et al. Childhood maltreatment, anxiety disorders and outcome in borderline personality disorder. Psychiatry Res . (2020) 284:112688. doi: 10.1016/j.psychres.2019.112688
13. Antoine SM, Fredborg BK, Streiner D, Guimond T, Dixon-Gordon KL, Chapman AL, et al. Subgroups of borderline personality disorder: A latent class analysis. Psychiatry Res . (2023) 323:115131. doi: 10.1016/j.psychres.2023.115131
14. Benzi IMA, Di Pierro R, De Carli P, Cristea IA, Cipresso P. All the faces of research on borderline personality pathology: Drawing future trajectories through a network and cluster analysis of the literature. J Evidence-Based Psychotherapies . (2020) 20:3–30. doi: 10.24193/jebp.2020.2.9
15. Reis T, Gekker M, Land MGP, Mendlowicz MV, Berger W, Luz MP, et al. The growth and development of research on personality disorders: A bibliometric study. Pers Ment Health . (2022) 16:290–9. doi: 10.1002/pmh.1540
16. Singh VK, Singh P, Karmakar M, Leta J, Mayr P. The journal coverage of Web of Science, Scopus and Dimensions: A comparative analysis. Scientometrics . (2021) 126:5113–42. doi: 10.1007/s11192-021-03948-5
17. Pritchard A. Statistical bibliography or bibliometrics. J Documentation . (1969) 25:348.
Google Scholar
18. Price DJ. Networks of scientific papers. Science . (1965) 149:510–5. doi: 10.1126/science.149.3683.510
19. Sabe M, Chen C, Perez N, Solmi M, Mucci A, Galderisi S, et al. Thirty years of research on negative symptoms of schizophrenia: A scientometric analysis of hotspots, bursts, and research trends. Neurosci Biobehav Rev . (2023) 144:104979. doi: 10.1016/j.neubiorev.2022.104979
20. Shen Z, Ji W, Yu S, Cheng G, Yuan Q, Han Z, et al. Mapping the knowledge of traffic collision reconstruction: A scientometric analysis in CiteSpace, VOSviewer, and SciMAT. Sci Justice . (2023) 63:19–37. doi: 10.1016/j.scijus.2022.10.005
21. Wu H, Wang Y, Tong L, Yan H, Sun Z. Global research trends of ferroptosis: A rapidly evolving field with enormous potential. Front Cell Dev Biol . (2021) 9:646311. doi: 10.3389/fcell.2021.646311
22. Brody S. Impact factor: Imperfect but not yet replaceable. Scientometrics . (2013) 96:255–7. doi: 10.1007/s11192-012-0863-x
23. Kaldas M, Michael S, Hanna J, Yousef GM. Journal impact factor: A bumpy ride in an open space. J Invest Med . (2020) 68:83–7. doi: 10.1136/jim-2019-001009
24. Schubert A, Glänzel W. A systematic analysis of Hirsch-type indices for journals. J Informetrics . (2007) 1:179–84. doi: 10.1016/j.joi.2006.12.002
25. Chen C. CiteSpace II: Detecting and visualizing emerging trends and transient patterns in scientific literature. J Am Soc Inf Sci Technol . (2006) 57:359–77. doi: 10.1002/asi.20317
26. Cheng K, Guo Q, Shen Z. Bibliometric analysis of global research on cancer photodynamic therapy: Focus on nano-related research. Front Pharmacol . (2022) 13:927219. doi: 10.3389/fphar.2022.927219
27. Hassan-Montero Y, De-Moya-Anegón F, Guerrero-Bote VP. SCImago Graphica: A new tool for exploring and visually communicating data. Profesional la Información . (2022) 31:e310502. doi: 10.3145/EPI
28. Wang Y, Li D, Jia Z, Hui J, Xin Q, Zhou Q, et al. A bibliometric analysis of research on the links between gut microbiota and atherosclerosis. Front Cardiovasc Med . (2022) 9:941607. doi: 10.3389/fcvm.2022.941607
29. Soloff PH, Lynch KG, Kelly TM, Malone KM, Mann JJ. Characteristics of suicide attempts of patients with major depressive episode and borderline personality disorder: a comparative study. Am J Psychiatry . (2000) 157:601–8. doi: 10.1176/appi.ajp.157.4.601
30. Widiger TA, Trull TJ. Plate tectonics in the classification of personality disorder: shifting to a dimensional model. Am Psychol . (2007) 62:71–83. doi: 10.1037/0003-066X.62.2.71
31. Patton JH, Stanford MS, Barratt ES. Factor structure of the Barratt impulsiveness scale. J Clin Psychol . (1995) 51:768–74. doi: 10.1002/1097-4679(199511)51:6<768::aid-jclp2270510607>3.0.co;2-1
32. Bartow S, Stopsack M, Grabe HJ, Meinke C, Spitzer C, Kronmüller K, et al. Interpersonal evaluation bias in borderline personality disorder. Behav Res Ther . (20092009) 47:359–65. doi: 10.1016/j.brat.2009.02.003
33. Cheavens JS, Rosenthal MZ, Daughters SB, Nowak J, Kosson D, Lynch TR, et al. An analogue investigation of the relationships among perceived parental criticism, negative affect, and borderline personality disorder features: The role of thought suppression. Behav Res Ther . (2005) 43:257–68. doi: 10.1016/j.brat.2004.01.006
34. Bradley R, Westen D. The psychodynamics of borderline personality disorder: a view from developmental psychopathology. Dev Psychopathol . (2005) 17:927–57. doi: 10.1017/s0954579405050443
35. Tzourio-Mazoyer N, Landeau B, Papathanassiou D, Crivello F, Etard O, Delcroix N, et al. Automated anatomical labeling of activations in SPM using a macroscopic anatomical parcellation of the MNI MRI single-subject brain. NeuroImage . (2002) 15:273–89. doi: 10.1006/nimg.2001.0978
36. Bellino S, Rinaldi C, Bozzatello P, Bogetto F. Pharmacotherapy of borderline personality disorder: a systematic review for publication purpose. Curr Medicinal Chem . (2011) 18:3322–9. doi: 10.2174/092986711796504682
37. Zanarini MC, Frankenburg FR, Dubo ED, Sickel AE, Trikha A, Levin A. Axis I comorbidity of borderline personality disorder. Am J Psychiatry . (1998) 155:1733–9. doi: 10.1176/ajp.155.12.1733
38. Yee L, Korner AJ, McSwiggan S, Meares RA, Stevenson J. Persistent hallucinosis in borderline personality disorder. Compr Psychiatry . (2005) 46:147–54. doi: 10.1016/j.comppsych.2004.07.032
39. Mittal VA, Walker EF. Diagnostic and statistical manual of mental disorders. Psychiatry Res . (2011) 189:158–9. doi: 10.1016/j.psychres.2011.06.006
40. Torgersen S, Kringlen E, Cramer V. The prevalence of personality disorders in a community sample. Arch Gen Psychiatry . (2001) 58:590–6. doi: 10.1001/archpsyc.58.6.590
41. Crick NR, Murray-Close D, Woods K. Borderline personality features in childhood: A short-term longitudinal study. Dev Psychopathol . (2005) 17:1051–70. doi: 10.1017/S0954579405050492
42. Linehan MM, Comtois KA, Murray AM, Brown MZ, Gallop RJ, Heard HL, et al. Two-year randomized controlled trial and follow-up of dialectical behavior therapy vs therapy by experts for suicidal behaviors and borderline personality disorder. Arch Gen Psychiatry . (2006) 63:757–66. doi: 10.1001/archpsyc.63.7.757
43. Giesen-Bloo J, van Dyck R, Spinhoven P, van Tilburg W, Dirksen C, van Asselt T, et al. Outpatient psychotherapy for borderline personality disorder: Randomized trial of schema-focused therapy vs transference-focused psychotherapy. Arch Gen Psychiatry . (2006) 63:649–58. doi: 10.1001/archpsyc.63.6.649
44. Crowell SE, Beauchaine TP, Linehan MM. A biosocial developmental model of borderline personality: Elaborating and extending Linehan's theory. psychol Bulletin . (2009) 135:495–510. doi: 10.1037/a0015616
45. Leichsenring F, Leibing E, Kruse J, New AS, Leweke F. Borderline personality disorder. Lancet (2011) 377(9759):74–84. doi: 10.1016/S0140-6736(10)61422-5
46. Whiteside SP, Lynam DR, Miller JD, Reynolds SK. Validation of the UPPS impulsive behaviour scale: A four-factor model of impulsivity. Eur J Pers (2005) 19(7):559–74. doi: 10.1002/per.556
47. Clarkin JF, Levy KN, Lenzenweger MF, Kernberg OF. Evaluating three treatments for borderline personality disorder: A multiwave study. Am J Psychiatry (2007) 164(6):922–8. doi: 10.1176/ajp.2007.164.6.922
48. Bateman A, Fonagy P. Randomized controlled trial of outpatient mentalization-based treatment versus structured clinical management for borderline personality disorder. Am J Psychiatry (2009) 166(12):1355–64. doi: 10.1176/appi.ajp.2009.09040539
49. Fonagy P, Luyten P. A developmental, mentalization-based approach to the understanding and treatment of borderline personality disorder. Dev Psychopathol (2009) 21(4):1355–81. doi: 10.1017/S0954579409990198
50. Levy KN, Meehan KB, Kelly KM, Reynoso JS, Weber M, Clarkin JF, et al. Change in attachment patterns and reflective function in a randomized control trial of transference-focused psychotherapy for borderline personality disorder. J Consulting Clin Psychol (2006) 74(6):1027–40. doi: 10.1037/0022-006X.74.6.1027
51. Carr DS, Francis A. Childhood familial environment, maltreatment and borderline personality disorder symptoms in a non-clinical sample: A cognitive behavioural perspective. Clin Psychol (2009) 13(1):28–37. doi: 10.1080/13284200802680476
52. Selby EA, Kranzler A, Fehling KB, Panza E. Nonsuicidal self-injury disorder: The path to diagnostic validity and final obstacles. Clin Psychol Review . (2015) 38:79–91. doi: 10.1016/j.cpr.2015.03.003
53. Skoglund C, Tiger A, Rück C, Petrovic P, Asherson P, Hellner C, et al. Familial risk and heritability of diagnosed borderline personality disorder: A register study of the Swedish population. Mol Psychiatry . (2021) 26:999–1008. doi: 10.1038/s41380-019-0442-0
54. American Psychiatric Association, DSM-5 Task Force. Diagnostic and statistical manual of mental disorders: DSM-5™ . 5th ed. Washington: American Psychiatric Publishing, Inc., (2013). doi: 10.1176/appi.books.9780890425596
55. Cristea IA, Gentili C, Cotet CD, Palomba D, Barbui C, Cuijpers P. Efficacy of psychotherapies for borderline personality disorder: A systematic review and meta-analysis. JAMA Psychiatry . (2017) 74:319–28. doi: 10.1001/jamapsychiatry.2016.4287
56. Chen C. Predictive effects of structural variation on citation counts. J Am Soc Inf Sci Technol . (2012) 63:431–49. doi: 10.1002/asi.21694
57. Brown MZ, Comtois KA, Linehan MM. Reasons for suicide attempts and non-suicidal self-injury in women with borderline personality disorder. J Abnormal Psychol . (2002) 111:198–202. doi: 10.1037//0021-843x.111.1.198
58. Siever LJ, Davis KL. A psychobiological perspective on the personality disorders. Am J Psychiatry . (1991) 148:1647–58. doi: 10.1176/ajp.148.12.1647
59. Donegan NH, Sanislow CA, Blumberg HP. Amygdala hyperreactivity in borderline personality disorder: Implications for emotional dysregulation. Biol Psychiatry . (2003) 54:1284–93. doi: 10.1016/S0006-3223(03)00636-X
60. Tebartz van Elst L, Hesslinger B, Thiel T, Geiger E, Haegele K, Lemieux L, et al. Frontolimbic brain abnormalities in patients with borderline personality disorder: A volumetric magnetic resonance imaging study. Biol Psychiatry . (2003) 54:163–71. doi: 10.1016/s0006-3223(02)01743-2
61. Herpertz SC, Dietrich TM, Wenning B, Krings T, Erberich SG, Willmes K, et al. Evidence of abnormal amygdala functioning in borderline personality disorder: A functional MRI study. Biol Psychiatry . (2001) 50:292–8. doi: 10.1016/S0006-3223(01)01075-7
62. Linehan MM. Cognitive-behavioral treatment of borderline personality disorder . New York: Guilford Press (1993).
63. Black DW, Blum N, Pfohl B, Hale N. Suicidal behavior in borderline personality disorder: Prevalence, risk factors, prediction, and prevention. J Pers Disord (2004) 18(3):226–39. doi: 10.1521/pedi.18.3.226.35445
64. Brambilla P, Soloff PH, Sala M, Nicoletti MA, Keshavan MS, Soares JC. Anatomical MRI study of borderline personality disorder patients. Psychiatry Res (2004) 131(2):125–33. doi: 10.1016/j.pscychresns.2004.04.003
65. Stiglmayr CE, Grathwol T, Linehan MM, Ihorst G, Fahrenberg J, Bohus M. Aversive tension in patients with borderline personality disorder: A computer-based controlled field study. Acta Psychiatrica Scandinavica (2005) 111(5):372–9. doi: 10.1111/j.1600-0447.2004.00466.x
66. Silbersweig D, Clarkin JF, Goldstein M, Kernberg OF, Tuescher O, Levy KN, et al. Failure of frontolimbic inhibitory function in the context of negative emotion in borderline personality disorder. Am J Psychiatry (2007) 164(12):18321841. doi: 10.1176/appi.ajp.2007.06010126
67. Bohus M, Haaf B, Stiglmayr C, Pohl U, Böhme R, Linehan M. Evaluation of inpatient dialectical-behavioral therapy for borderline personality disorder–a prospective study. Behav Res Ther (2000) 38(9):875887. doi: 10.1016/s0005-7967(99)00103-5
68. Rossouw TI, Fonagy P. Mentalization-based treatment for self-harm in adolescents: a randomized controlled trial. J Am Acad Child Adolesc Psychiatry (2012) 51(12):13041313. doi: 10.1016/j.jaac.2012.09.018
69. Zhang S, Wang S, Liu R. A bibliometric analysis of research trends of artificial intelligence in the treatment of autistic spectrum disorders. Front Psychiatry . (2022) 13:967074. doi: 10.3389/fpsyt.2022.967074
70. Dzikowski P. A bibliometric analysis of born global firms. J Business Res . (2018) 85:281–94. doi: 10.1016/j.jbusres.2017.12.054
71. Chen C, Ibekwe-SanJuan F, Hou J. The structure and dynamics of cocitation clusters: A multiple-perspective cocitation analysis. J Am Soc Inf Sci Technol . (2010) 61:1386–409. doi: 10.1002/asi.21309
72. Gunderson JG, Herpertz SC, Skodol AE, Torgersen S, Zanarini MC. Borderline personality disorder. Nat Rev Dis Primers . (2018) 4:18029. doi: 10.1038/nrdp.2018.29
73. Tan MYL, McConnell B, Barlas J. Application of Dialectical Behaviour Therapy in treating common psychiatric disorders: Study protocol for a scoping review. BMJ Open . (2002) 12:e058565. doi: 10.1136/bmjopen–2021–058565
74. Quevedo Y, Booij L, Herrera L, Hernández C, Jiménez JP. Potential epigenetic mechanisms in psychotherapy: A pilot study on DNA methylation and mentalization change in borderline personality disorder. Front Hum Neurosci . (2022) 16:955005. doi: 10.3389/fnhum.2022.955005
75. Uhlmann C, Tschöke S, Steinert T, Schmid P. Can you see and hear how bad I feel right now? Self–injury and suicidal communication in borderline personality disorder-A secondary analysis. Psychiatrische Praxis . (2022) 49:329–33. doi: 10.1055/a–1820–0438
76. Bohus M, Stoffers-Winterling J, Sharp C, Krause-Utz A, Schmahl C, Lieb K. Borderline personality disorder. Lancet (2022) 398:1528–40. doi: 10.1016/S0140–6736(21)00476–1
77. Schuppert HM, Giesen-Bloo J, van Gemert TG, Wiersema HM, Minderaa RB, Emmelkamp PMG, et al. Effectiveness of an emotion regulation group training for adolescents—A randomized controlled pilot study. Clin Psychol Psychother . (2009) 16:467–78. doi: 10.1002/cpp.637
78. American Psychiatric Association. Diagnostic and statistical manual of mental disorders, text revision . 4th ed. Washington: American Psychiatric Association (2000).
79. Lenzenweger MF, Cicchetti D. Toward a developmental psychopathology approach to borderline personality disorder. Dev Psychopathol . (2005) 17:893–8. doi: 10.1017/S095457940505042X
80. Chanen AM, Jovev M, McCutcheon LK, Jackson HJ, McGorry PD. Borderline personality disorder in young people and the prospects for prevention and early intervention. Curr Psychiatry Rev . (2008) 4:48–57. doi: 10.2174/157340008783743820
81. Pohl S, Steuwe C, Mainz V, Driessen M, Beblo T. Borderline personality disorder and childhood trauma: Exploring the buffering role of self-compassion and self-esteem. J Clin Psychol . (2021) 77:837–45. doi: 10.1002/jclp.23070
82. Bartsch DR, Roberts R, Proeve M. Relationships between parental borderline symptom severity, empathy, parenting styles and child psychopathology. Clin Psychol . (2022) 26:211–21. doi: 10.1080/13284207.2022.2031947
83. Lakeman R, Hurley J, Campbell K, Hererra C, Leggett A, Tranter R, et al. High fidelity dialectical behaviour therapy online: Learning from experienced practitioners. Int J Ment Health Nursing . (20222022) 31:1405–16. doi: 10.1111/inm.13039
Keywords: neuro-behavioral model, borderline personality disorder, BPD, bibliometric, Scimago Graphica
Citation: Liu Y, Chen C, Zhou Y, Zhang N and Liu S (2024) Twenty years of research on borderline personality disorder: a scientometric analysis of hotspots, bursts, and research trends. Front. Psychiatry 15:1361535. doi: 10.3389/fpsyt.2024.1361535
Received: 12 January 2024; Accepted: 19 February 2024; Published: 01 March 2024.
Reviewed by:
Copyright © 2024 Liu, Chen, Zhou, Zhang and Liu. This is an open-access article distributed under the terms of the Creative Commons Attribution License (CC BY) . The use, distribution or reproduction in other forums is permitted, provided the original author(s) and the copyright owner(s) are credited and that the original publication in this journal is cited, in accordance with accepted academic practice. No use, distribution or reproduction is permitted which does not comply with these terms.
*Correspondence: Shen Liu, [email protected] ; Chaomei Chen, [email protected] ; Na Zhang, [email protected]
Disclaimer: All claims expressed in this article are solely those of the authors and do not necessarily represent those of their affiliated organizations, or those of the publisher, the editors and the reviewers. Any product that may be evaluated in this article or claim that may be made by its manufacturer is not guaranteed or endorsed by the publisher.
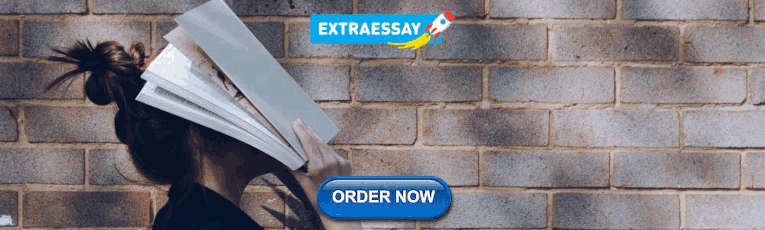
IMAGES
VIDEO
COMMENTS
Abstract. This review paper on the current status of the studies on personality traits, aimed at summarizing the progress achieved in the study of personality traits and examining the evidences ...
Personality traits can be defined as broad patterns of thoughts, feelings, and behaviors (Lucas & Donnellan, 2011).Early empirical research on personality mainly focused on the structure, measurement, and consequences of traits (e.g., Digman, 1990).Stability and change in traits were less common topics, largely because traits were regarded as highly stable once people reach adulthood (McCrae ...
Emphasizing experimental and descriptive research, the Journal of Research in Personality presents articles that examine important issues in the field of personality and in related fields basic to the understanding of personality. The subject matter includes treatments of genetic, physiological, …. View full aims & scope.
This paper presents an innovative theoretical model on how personality develops. ... L. B. in Handbook of Personality Theory and Research (eds John, O. & Robins, R. W.) 259-283 (Guilford, 2021).
1. Current conceptualization of personality. The Five Factor Model (FFM) of personality has guided research and theory building for almost three decades (John, Naumann, & Soto, 2008).FFM, also known as the Big Five model, contends that the construct of personality includes Basic Tendencies or traits that are biologically-based, as well as Characteristic Adaptations that result from dynamic ...
Journal of Personality and Social Psychology ® publishes original papers in all areas of personality and social psychology and emphasizes empirical reports, but may include specialized theoretical, methodological, and review papers.. The journal is divided into three independently edited sections. Attitudes and Social Cognition publishes articles concerning attitudinal and social cognitive ...
Latest Research and Reviews The process and mechanisms of personality change Personality is relatively stable over long timescales but remains malleable to some degree.
Personality psychology, which seeks to study individual differences in thoughts, feelings, and behaviors that persist over time and place, has experienced a renaissance in the last few decades. It has also not been reviewed as a field in the Annual Review of Psychology since 2001. In this article, we seek to provide an update as well as a meta-organizational structure to the field. In ...
1.1.1 Definitions of Personality. People differ from each other in their behaviour, cognition and emotions, which makes them unique and very special. Their individual differences lay the founda-tion for an understanding of personality as the popular conception of a person as a. whole entity (Brunas-Wagstaff, 1998).
This review categorizes the 155 personality traits that have emerged in the management and psychology literature that describe personality beyond the Big Five. Based on these findings, this study proposes new avenues for future research and offers insights into the future of the field as the concept of personality has shifted in the 21st century.
Another widely studied indicator is the facial width to height ratio (fWHR), which has been linked to various traits, such as achievement striving 10, deception 11, dominance 12, aggressiveness 13 ...
A new algorithmic approach to personality prototyping based on Big Five traits was applied to a large representative and longitudinal German dataset (N = 22,820) including behavior, personality and health correlates. We applied three different clustering techniques, latent profile analysis, the k-means method and spectral clustering algorithms. The resulting cluster centers, i.e. the ...
Personality research has had a contentious history, and there are still vestiges of doubt about the importance of personality traits. ... Preparation of this paper was supported by National Institute of Aging Grants AG19414 and AG20048; National Institute of Mental Health Grants MH49414, MH45070, MH49227; United Kingdom Medical Research Council ...
In the field of personality type research, one of the first studies based on this method was conducted by Caspi and Silva , who applied the SPSS Quick Cluster algorithm to behavioral ratings of 3-year-olds, ... Another method, which is used in the majority of the cited papers in section 1, is to randomly split the data in two halves, apply a ...
The Future of the Structural Modeling of Personality. Janek Musek, in The General Factor of Personality, 2017. Toward Methodological Convergence in Personality Research. In the future, the structural research in personality psychology needs to be more integrated with regard to the assessment methods and the methods of data analysis. Unfortunately, in psychology we are faced with a variety of ...
Personality traits, individual innovativeness and satisfaction with life is a research article that examines how these three factors interact and influence each other. The article uses a sample of Spanish university students and applies various statistical methods to test the hypotheses. The article is published in the Journal of Innovation and Knowledge, a peer-reviewed journal that explores ...
Personality. Personality refers to the enduring characteristics and behavior that comprise a person's unique adjustment to life, including major traits, interests, drives, values, self-concept, abilities, and emotional patterns. Various theories explain the structure and development of personality in different ways, but all agree that ...
This study aims to assess the impact of personality traits on emotional intelligence (EI) and decision-making among medical students in Lebanese Universities and to evaluate the potential mediating role-played by emotional intelligence between personality traits and decision-making styles in this population. This cross-sectional study was conducted between June and December 2019 on 296 general ...
2005 2004 2003 1997-1998. This on-line repository provides a publication outlet for 47 theoretical and review papers on the science of personality. Some papers present original theories that take account of existing research and have testable consequences; other papers describe and evaluate an existing personality research program.
List of Personality Topics. You can also come up with questions about your own about different topics in personality psychology. Some that you might explore include: Big 5 personality traits. The id, ego, and superego. Psychosocial development. Hierarchy of needs. Myers-Briggs Type Indicator. Personality disorders.
The present paper argues that all health-care professionals need basic knowledge about manifestations of different personality traits, above all in the form of manifest PDs, as we know that such pathology has a negative effect on the interaction between the patient and health-care professionals in terms of communication, clinical assessment ...
3.3 Most cited papers. The top 10 highly cited papers on BPD research were presented in Table 1.The most cited paper, by Marsha M. Linehan and colleagues, focus on the treatment of suicidal behavior in BPD ().The transition between suicidal and non-suicidal self-injurious behavior in individuals with BPD has attracted researchers's attention, mainly in cluster #4 "nonsuicidal self-injury ...
The paper suggests that personality traits important for financial decisions may differ from those relevant in other economic domains, and it discusses the implications of this difference for understanding investment behavior. ... Research is her life's work and by combing her scientific grounding in quantitative investment management with a ...