- How It Works
- PhD thesis writing
- Master thesis writing
- Bachelor thesis writing
- Dissertation writing service
- Dissertation abstract writing
- Thesis proposal writing
- Thesis editing service
- Thesis proofreading service
- Thesis formatting service
- Coursework writing service
- Research paper writing service
- Architecture thesis writing
- Computer science thesis writing
- Engineering thesis writing
- History thesis writing
- MBA thesis writing
- Nursing dissertation writing
- Psychology dissertation writing
- Sociology thesis writing
- Statistics dissertation writing
- Buy dissertation online
- Write my dissertation
- Cheap thesis
- Cheap dissertation
- Custom dissertation
- Dissertation help
- Pay for thesis
- Pay for dissertation
- Senior thesis
- Write my thesis
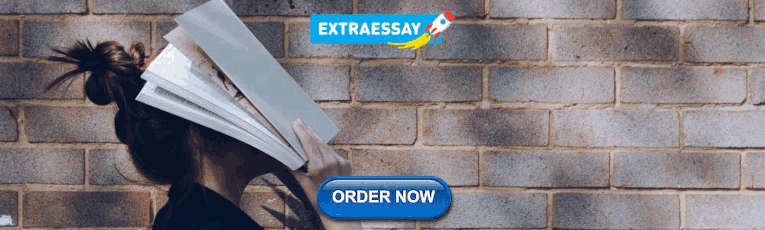
174 Film Research Paper Topics To Inspire Your Writing
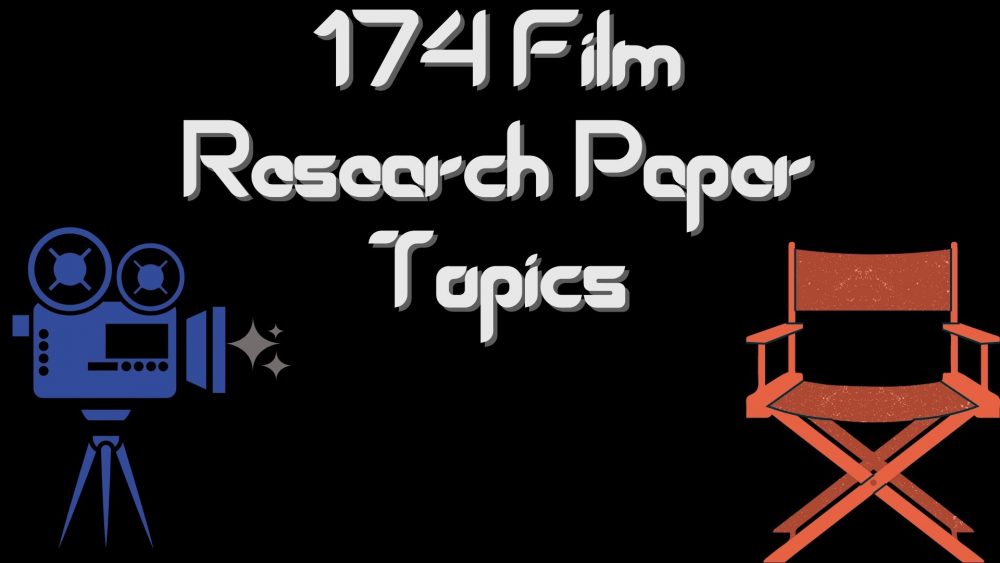
Also known as a moving picture or movie, the film uses moving images to communicate or convey everything from feelings and ideas to atmosphere and experiences. The making of movies, as well as the art form, is known as cinematography (or cinema, in short). The film is considered a work of art. The first motion pictures were created in the late 1880s and were shown to only one person at a time using peep show devices. By 1985, movies were being projected on large screens for large audiences.
Film has a rich and interesting history, as well as a bright future given the current technological advancements. This is why many professors will really appreciate it if you write a research paper on movies. However, to write a great paper, you need a great topic.
In this blog post, we will give you our latest list of 174 film research paper topics. They should be excellent for 2023 and should get you some bonus points for originality and creativity. As always, our topics are 100% free to use as you see fit. You can reword them in any way you like and you are not required to give us any credit.
Writing Good Film Research Paper
Before we get to the film topics for research papers in our list, you need to learn how you can write the best possible film research paper. It’s not overly complicated, don’t worry. Here are some pointers to get you started:
Start as early as possible Start your project with an outline that will keep you focused on what’s important Spend some time to find a great topic (or just use one of ours) Research every angle of the topic Spend some time composing the thesis statement Always use information from reliable sources Make sure you cite and reference properly Edit and proofread your work to make it perfect. Alternatively, you can rely on our editors and proofreaders to help you with this.
Now it’s time to pick your topic. We’ve made things easy for you, so all you have to do is go through our neatly organized list and select the topic you like the most. If you already know something about the topic, writing the paper shouldn’t take you more than 1 or 2 days, however if you have no desire to spend a lot of time on your assignment, thesis writing help from our professionals is on its way. Pick your topic now:
Easy Film Research Topics
We know most students are not too happy about spending days working on their research papers. This is why we have compiled a list of easy film research topics just for our readers:
- What was the Electrotachyscope?
- Research the history of film
- Describe the first films ever made
- Talk about the Kinetoscope
- Who were Auguste and Louis Lumière?
- An in-depth look at film during World War I
- Talk about the evolution of sound in motion pictures
- Most popular movie actors of all time
- The life and works of Charles Chaplin
- The life and works of Sergei Mikhailovich Eisenstein
- Discuss the Mutoscope device
- Talk about the introduction of natural color in films
Film Topics To Write About In High School
If you are a high school student, you probably want some topics that are not overly complicated. Well, the good news is that we have plenty of film topics to write about in high school. Check them out below:
- An in-depth analysis of sound film
- Research the shooting of Le Voyage dans la Lune
- Talk about the Technicolor process
- Research the film industry in India
- The growing popularity of television
- Discuss the most important aspects of film theory
- The drawbacks of silent movies
- Cameras used in 1950s movies
- The most important cinema movie of the 1900s
- Research the montage of movies in the 1970s
- The inception of film criticism
- Discuss the film industry in the United States
Interesting Film Paper Topics
Are you looking for the most interesting film paper topics so that you can impress your professor and your fellow students? We are happy to say that you have arrived at just the right place. Here are our latest ideas:
- Are digital movies much different from films?
- Research the evolution of cinematography
- Research the role of movies in Indian culture
- The principles of a cinema camera
- Technological advancements in the film industry
- The use of augmented reality in movies
- Talk about the role of film in American culture
- An in-depth look at the production cycle of a film
- The role of the filming crew on the set
- Latest cameras for cinematography
- An in-depth look at the distribution of films
- How are animated movies made?
Controversial Movie Topics
Why would you be afraid to write your paper on a controversial topic? Perhaps you didn’t know that most professors really appreciate the effort and the innovative ideas. Below, you can find a whole list of controversial movie topics for students:
- An in-depth look at Cannibal Holocaust
- Controversies behind Fifty Shades of Gray
- A Clockwork Orange: the banned movie
- All Quiet on the Western Front: a controversial war movie
- Discuss The Texas Chain Saw Massacre movie
- Apocalypse Now: one of the most banned movies
- Brokeback Mountain and the controversies surrounding it
- Talk about The Last Temptation of Christ
- The Birth of a Nation: the movie that was banned in America
Movie Topics Ideas For College
As you probably know already, college students should choose topics that are a bit more complex than those picked by high school students. The good news is that we have compiled a list of the best movie topics ideas for college students below:
- Methods to bring your sketches to life
- Discuss problems with documentary filming
- War movies and their impact on society
- What does a director actually do on the set?
- Talk about state-sanctioned movies in China
- Research cinematography in North Korea
- Talk about psychological reactions to films
- Research the good versus evil theme
- African Americans in the 1900s cinematography in the US
- Discuss the creation of sound for films
Hottest Film Topics To Date
Our writers and editors did their best to compile a list of the hottest film topics to date. You can safely pick any of the topics below and write your essay or research paper on it. You should be able to find plenty of information online about each and every topic:
- The life and works of Alfred Hitchcock
- Talk about racial discrimination in war movies
- The psychology behind vampire movies
- The life and works of Samuel L. Jackson
- Classic opera versus modern movie soundtracks
- Hollywood versus Bollywood
- The life and works of tom Hanks
- Research the Frankenstein character
- Major contributions by women in cinematography
- The life and works of Harrison Ford
- The 3 most popular topics for a moving picture
Good Movie Topics For 2023
We know, you probably want some topics that relevant today. You want to talk about something new and exciting. Well, we’ve got a surprise for you. This list of good movie topics for 2023 has just been added to the blog post, and you can use it for free:
- The life and works of Will Smith
- Why do people love movie monsters?
- Talk about the popularity of fan movies
- The life and works of Morgan Freeman
- Gender inequality in UK films
- Research movies that were produced because of video games
- The life and works of Anthony Hopkins
- The importance of the Golden Raspberry Award
- Outer space: the future of cinematography
- Compare today’s filming techniques to those in the 1950s
- The importance of winning a Golden Globe Award
Fascinating Film Topics
Are you looking for some of the most fascinating film topics one can ever find online? Our experts have outdone themselves this time. Check out our list of ideas below and choose the topic you like the most:
- Talk about the development of Star Wars
- Talk about spaghetti western movies
- Discuss the filming of Pride and Prejudice
- Research fantasy films
- The most popular movie genre in 2023
- What makes a movie a blockbuster?
- Filming for the Interstellar movie
- Peculiarities of Bollywood cinema
- Talk about the era of Hitchcock
- Discuss the role of motion pictures in society
- Talk about Neo-realism in Italian movies
- Research the filming of A Fistful of Dollars
The History Of Film Topics
Writing about the history of film and cinematography can be a good way to earn some bonus points from your professor. However, it’s not an easy thing to do. Fortunately, we have a list of the history of film topics right here for you, so you don’t have to waste any time searching:
- Research the first ever motion picture
- Discuss the idea behind moving images
- Research the Pioneer Era
- Talk about the introduction of sound in movies
- Talk about the Silent Era
- Who created the first ever movie?
- Discuss the Golden Era of cinematography
- The era of changes in 2023
- The rise of Hollywood cinematography
- Discuss the first color movie
- Research the first horror movie
- Discuss the phrase “No one person invented cinema”
Famous Cinematographers Topics
You can, of course, write your next research paper on the life and works of a famous or popular cinematographer. You have plenty to choose from. However, we’ve already selected the best famous cinematographers topics for you right here:
- The life and works of Sir Roger Deakins
- Research the cinematographer Vittorio Storaro
- An in-depth look at Bill Pope
- Research the cinematographer Gordon Willis
- The life and works of Wally Pfister
- An in-depth look at Robert Burks
- Research the cinematographer Stanley Cortez
- The life and works of Conrad Hall
- An in-depth look at Rodrigo Prieto
- The life and works of Claudio Miranda
- Emmanuel Lubezki
- An in-depth look at Jack Cardiff
- Research the cinematographer Michael Ballhaus
- The life and works of Kazuo Miyagawa
Famous Films Topic Ideas
The easiest and fastest way to write an essay or research paper about movies is to write about a famous movie. Take a look at these famous films topic ideas and start writing your paper today:
- Research A Space Odyssey
- Research the movie Seven Samurai
- Cinematography techniques in There Will Be Blood
- Discuss the film The Godfather
- An in-depth look at La Dolce Vita
- Research the movie Citizen Kane
- Cinematography techniques in Goodfellas
- An in-depth look at the Aliens series
- Cinematography techniques in Singin’ in the Rain
- Research the movie Mulholland Drive
- An in-depth look at In The Mood For Love
- Research the movie City Lights
The Future Of Movies Topic Ideas
Did you ever wonder what the movies of the future will look like? We can guarantee that your professor has thought about it. Surprise him by writing your paper on one of these the future of movies topic ideas:
- The future of digital films
- Discuss animation techniques of the future
- The future of cinematography cameras
- How do you view the actors of the future?
- Will digital releases eliminate the need for DVDs?
- The role of streaming services in the future
- Talk about the direct-to-consumer distribution concept
- Is cinematography a good career for the future?
- Will movie theaters disappear?
- Virtual reality in future films
- The rise of Pixar Studios
Awesome Cinema Topic Ideas
Our experts have just finished completing this section of the topics list. Here, you will find some of the most awesome cinema topic ideas. These should all work great in 2023, so give them a try today:
- The concept of the Road Movie
- Review the film “Donnie Brasco”
- The popularity of musical movies
- A comprehensive history of cinematography
- Discuss the A Beautiful Mind movie
- Compare watching movies now and in the 1990s
- Talk about film narrative
- The importance of the main characters in a movie
- The process of selecting the right actor for the role
- Well-known produces in the United States
- The most popular actors in 2023
- Research Nazi propaganda films
Simple Cinema Essay Ideas
If you want to write about cinematography but don’t want to spend too much time researching the topic, you could always choose one of our simple cinema essay ideas. New ideas are added to this list periodically:
- Discuss the concept of limited animation
- War movies during World War II
- The importance of James Bond for Americans
- What is docufiction?
- The traits of a filmophile
- The success of early crime movies
- An in-depth look at Hanna-Barbera
- The transition from VHS tape to DVD
- Best comedy movies ever made
- Discuss the Film Noir genre
- What is a Blaxploitation?
- The best samurai film ever produced
Movies And The Internet Topics
- How does piracy affect the movie industry?
- An in-depth look at Netflix
- Research the top 3 movie streaming websites
- Compare and contrast Netflix and Amazon Prime
- Should movies be shared for free online?
- The effects of online streaming on piracy
- Is pirating movies illegal everywhere?
- Illegal downloads of movies in North Korea
- Piracy: a form of film preservation
- The most pirated movies of the 21st century
- Research the best ways to stop film piracy
- The economic impact of movie piracy in the United States
Rely On The Best Thesis Writing Service
Are you preparing to start working on your thesis? Or perhaps you just need some help with a research paper or an essay related to films and the movie industry. Our thesis writing service is exactly what you have been looking for! We have the writers and the experts you need if you want to do a great job on your next academic writing project. And remember, you will get assistance fast and cheap from a team of ENL writers, editors and proofreaders. We are a reliable academic writing agency with years of industry experience, so collaborating with us is 100% secure.
Over the years, we have helped thousands of high school, college and university students with their writing projects. We have always delivered the high quality professors were expecting from their students. In other words, our customers get top grades in 99.6% of the cases. We have an expert for every class, so you don’t have to worry about your project being too complex for us.
If you are a student who is searching for top quality custom content online, custom dissertation writing online from our expert helpers. We have just added new offers and discounts, so don’t forget to ask our customer support specialist about them. Get in touch with us now and save!
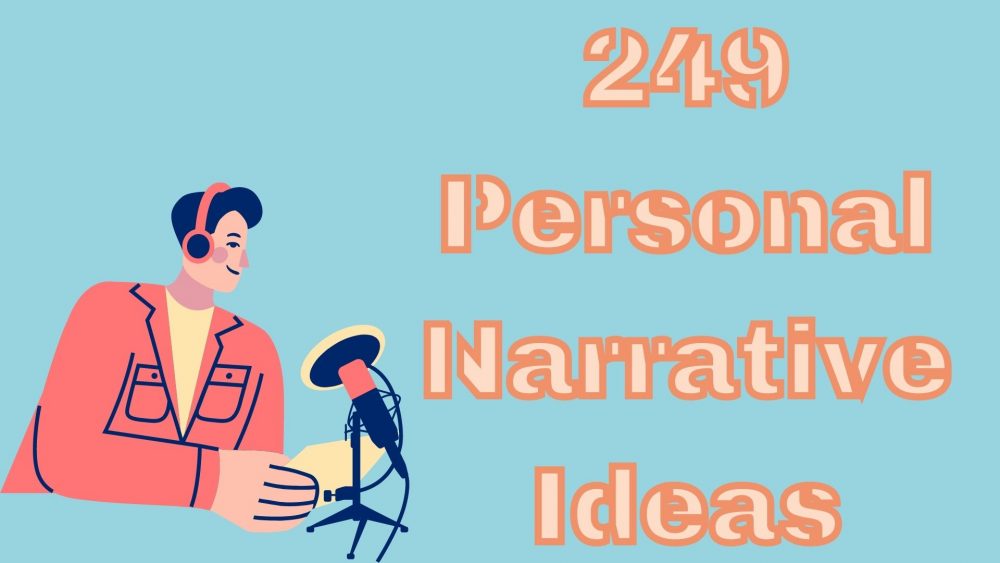
Leave a Reply Cancel reply
Your email address will not be published. Required fields are marked *
Comment * Error message
Name * Error message
Email * Error message
Save my name, email, and website in this browser for the next time I comment.
As Putin continues killing civilians, bombing kindergartens, and threatening WWIII, Ukraine fights for the world's peaceful future.
Ukraine Live Updates
Thank you for visiting nature.com. You are using a browser version with limited support for CSS. To obtain the best experience, we recommend you use a more up to date browser (or turn off compatibility mode in Internet Explorer). In the meantime, to ensure continued support, we are displaying the site without styles and JavaScript.
- View all journals
- My Account Login
- Explore content
- About the journal
- Publish with us
- Sign up for alerts
- Open access
- Published: 13 May 2020
Using data science to understand the film industry’s gender gap
- Dima Kagan ORCID: orcid.org/0000-0002-8216-8776 1 ,
- Thomas Chesney 2 &
- Michael Fire 1
Palgrave Communications volume 6 , Article number: 92 ( 2020 ) Cite this article
24k Accesses
15 Citations
28 Altmetric
Metrics details
- Complex networks
- Cultural and media studies
Data science can offer answers to a wide range of social science questions. Here we turn attention to the portrayal of women in movies, an industry that has a significant influence on society, impacting such aspects of life as self-esteem and career choice. To this end, we fused data from the online movie database IMDb with a dataset of movie dialogue subtitles to create the largest available corpus of movie social networks (15,540 networks). Analyzing this data, we investigated gender bias in on-screen female characters over the past century. We find a trend of improvement in all aspects of women‘s roles in movies, including a constant rise in the centrality of female characters. There has also been an increase in the number of movies that pass the well-known Bechdel test, a popular—albeit flawed—measure of women in fiction. Here we propose a new and better alternative to this test for evaluating female roles in movies. Our study introduces fresh data, an open-code framework, and novel techniques that present new opportunities in the research and analysis of movies.
Similar content being viewed by others
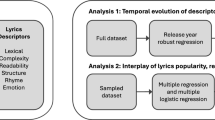
Song lyrics have become simpler and more repetitive over the last five decades
Emilia Parada-Cabaleiro, Maximilian Mayerl, … Eva Zangerle
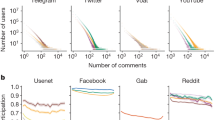
Persistent interaction patterns across social media platforms and over time
Michele Avalle, Niccolò Di Marco, … Walter Quattrociocchi
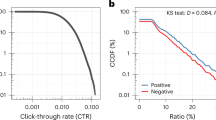
Negativity drives online news consumption
Claire E. Robertson, Nicolas Pröllochs, … Stefan Feuerriegel
Introduction
The film industry is one of the strongest branches of the media, reaching billions of viewers worldwide (MPAA, 2018 ; UNIC, 2017 ). Now more than ever, the media has a major influence on our daily lives (Silverstone, 2003 ), significantly influencing how we think (Entman, 1989 ), what we wear (Wilson and MacGillivray, 1998 ), and our self-image (Polce-Lynch et al., 2001 ). In particular, the representation of women in media has an enormous influence on society. As just one example, a new study shows that “women who regularly watch The X-Files are more likely to express interest in STEM, major in a STEM field in college, and work in a STEM profession than other women in the sample” (Fox, 2018 ).
Movies are the fulfillment of the vision of the movie director, who controls all aspects of the filming. It is well known that movie directors are primarily white and male (Smith et al., 2017 ). With such a gender bias, it is not surprising that there is a male gender dominance in movies (Smith and Choueiti, 2010 ; Ramakrishna et al., 2017 ). Studies from the past two decades have confirmed that women in the film industry are both underrepresented (University, 2017 ; Lauzen, 2018b ) and portrayed stereotypically (Wood, 1994 ). A recent study found that the underrepresentation is so sizeable that there are twice as many male speaking characters as female in the average movie (Lauzen, 2018a ).
While the gender gap in the film industry is a well-known issue (Lauzen, 2018a ; Rose, 2018 ; Cohen, 2017 ; Lauzen, 2018b ; Wood, 1994 ), there is still much value in researching this topic. Most previous gender studies can be categorized into two types: the first type offers simple statistics from the data to emphasize the gender gap (Lauzen, 2018b ); and the second type introduces more advanced analytical methods, yet generally uses only a small amount of data (Agarwal et al., 2015 ; Garcia et al., 2014 ).
In this study, we present Subs2Network , a novel algorithm to construct a movie character’s social network. We demonstrate possible utilizations of Subs2Network by employing the latest data science tools to comprehensively analyze gender in movies (see Fig. 1 Footnote 1 ). This is the largest study to date that uses social network analysis (SNA) to investigate the gender gap problem in the film industry and how it evolved.
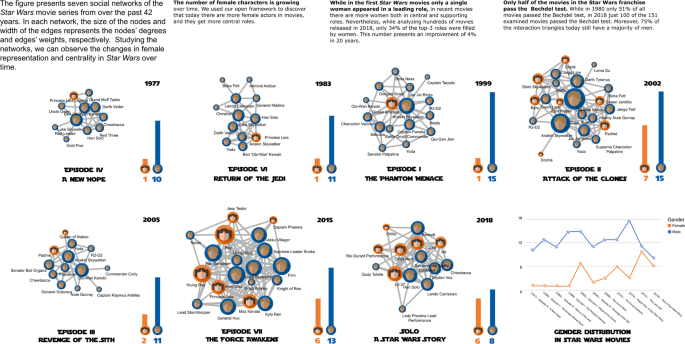
The evolution of female representation in the Star Wars movies series.
The study’s primary goals are to answer the following four questions:
Question 1: Are there movie genres that do not exhibit a gender gap?
Question 2: What do characters’ relationships reveal about gender, and how has this changed over time?
Question 3: Are women receiving more central movie roles today than in the past?
Question 4: How has the fairness of female representation in movies changed over the years?
To answer these questions, we first analyzed movie subtitles using text-processing algorithms and a list of movie characters’ names (see Fig. 2 ). We then developed Subs2Network to construct a movie character’s social network. We created an open-source code framework to collect and analyze movie data, and we used this framework to construct the largest open movie social network dataset that exists today.
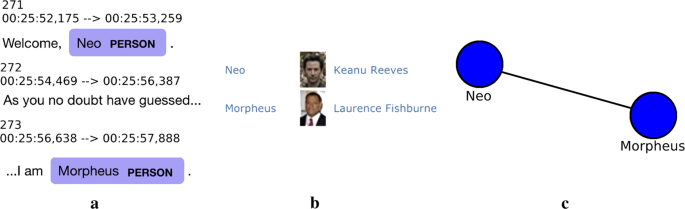
Turning subtitles into a network, step by step: a perform named entity recognition on the subtitles; b match the entities to the movie characters; and c link the characters and increase the edge weight by one.
Using the constructed movie social networks, we extracted dozens of topological features that characterized each movie. By analyzing these features, we could observe the gender gap across movie genres and over the last 99 years. Moreover, by utilizing the dataset, we developed a machine-learning classifier, which is able to assess, how fairly women are represented in movies (i.e., if a movie passes the Bechdel test (Bechdel, 1985 )).
Our results demonstrate that in most movie genres there is a statistically significant difference between men and women in centrality features like betweenness and closeness . These differences indicate that men are getting more central roles in movies than women (see Fig. 2a, b , and section “Results”). Another sign of the underrepresentation of women in movies is found by analyzing interactions among three characters: only 3.57% of the interactions are among three women, while 40.74% are among three men. These results strengthen previous studies‘ results that women play fewer central roles (Agarwal et al., 2015 ; Lauzen, 2018b ), and indicates that on average women have more minor roles. Our results highlight how and where gender bias manifests in the film industry and provides an automatic way to evaluate it over time.
The key contributions presented in this paper are fivefold:
A novel algorithm (see section “Methods and experiments”) which utilizes movie subtitles and character lists to automatically construct a movie’s social network (see section “Constructing movie social networks” and Fig. 2 ).
The largest open movie social network dataset, 21 times larger than the previous dataset (Kaminski et al., 2018 ) (see section “Datasets”). Our dataset contains 15,540 dynamic networks of movies (937 of these networks are networks of biographic movies, which have information about real-world events).
An open-source framework for movie analysis. The code contains a framework to generate additional social networks of movies, facilitating research by creating and analyzing larger amounts of data than ever before.
A machine-learning classifier that can predict if a movie passes the Bechdel test (see section “Constructing the Bechdel test classifier”) and can evaluate the change in gender bias in thousands of movies over several decades (see section “Results”).
Our new and alternative automated Bechdel test to measure female representation in movies. This new test overcomes the weaknesses of the original Bechdel test.
Our study demonstrates that inequality is still widespread in the film industry. In movies of 2018, a median of 30% women and a mean of 33% were found in each movie’s top-10 most central roles. That being said, there is evidence that the gender gap is improving (see Fig. 3 ).
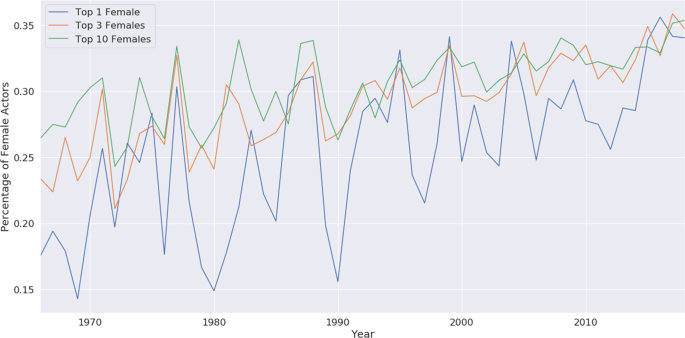
The change in the percentage of women in top 1, 3, and 10 most central roles over time.
The remainder of this paper is organized as follows: In section “Related work”, we present an overview of relevant studies. In section “Methods and experiments”, we describe the datasets, methods, algorithms, and experiments used throughout this study. In section “Results”, we present our results. Then, in section “Discussion”, we discuss the obtained results. Lastly, in section “Conclusions”, we present our conclusions from this study and offer future research directions.
Related work
Movie social networks.
In the past decade, the study of social networks has gained massive popularity. Researchers have discovered that SNA techniques can be used in many domains that do not have explicit data with a network structure. One such domain is the film industry. Researchers have applied SNA to analyze movies, gaining not only new insights about specific movies but also about the film industry in general. For example, using social networks makes it possible to empirically analyze social ties between movie characters.
In 2009, Weng et al. ( 2009 ) presented RoleNet, a method to convert a movie into a social network. The RoleNet algorithm builds a network by connecting links between characters that appear in the same scene. RoleNet is based on using image processing for scene detection and face recognition to find character appearances. Weng et al. evaluated their method on 10 movies and three TV shows. The method was used to perform semantic analysis of movies, find communities, detect leading roles, and determinine story segmentation.
In 2012, Park et al. ( 2012 ) developed Character-net, another method to convert movies to networks. Character-net builds the social network based on dialog between characters, using script–subtitle alignment to extract who speaks to whom in the scene. Park et al. ( 2012 ) evaluated their method on 13 movies. Similar to RoleNet, Character-net was used to detect leading roles and to cluster communities.
In 2014, Agarwal et al. ( 2014 ) presented a method for parsing screenplays by utilizing machine-learning algorithms instead of using regular expressions. Their study showed that the parsed screenplay can be used to create a social network of character interactions. In 2015, Tran and Jung ( 2015 ) developed the CoCharNet, a method which adds weight to a link in the interaction network, where the weight is a function of the number of times two characters appear together. Tran and Jung used CoCharNet to evaluate the importance of characters in movies. They demonstrated that network centrality features such as closeness centrality, betweenness centrality, and weighted degree can be used to classify minor and main characters in a movie. For instance, they detected the main characters using closeness centrality with a precision of 74.16%.
In 2018, Lv et al. ( 2018 ) developed an algorithm to improve the accuracy of creating social networks of movies. They presented StoryRoleNet, which combines video and subtitle analysis to build a more accurate movie social network. The subtitles were used to add additional links that the video analysis might miss. Similar to RoleNet and Character-net, Lv et al. ( 2018 ) used the movie social networks to cluster communities and to detect important roles. They evaluated the StoryRoleNet method on three movies and one TV series, for which they manually created baseline networks (Lv et al., 2018 ).
Also in 2018, a dataset from Moviegalaxies (Kaminski et al., 2018 ) Footnote 2 was released. Moviegalaxies is a website that displays social networks of movie characters. The dataset contains 773 movie social networks that were constructed based on movie scripts. However, Moviegalaxies did not disclose the exact methods which were used for the construction of the networks.
Evaluating the gender gap
In recent years, there have been many studies that attempt to evaluate the gender gap between males and females across various domains (Jia et al., 2016 ; Larivière et al., 2013 ; Lauzen, 2018b ; Wagner et al., 2015 ). For example, in 2018 the World Bank evaluated that the costs of gender bias are vast; gender inequality results in an estimated $160.2 trillion loss in human capital wealth (Worldbank, 2018 ).
Over the years, researchers have discovered many manifestations of the gender gap in our society. Larivière et al. ( 2013 ) discovered that scientific articles with women in dominant author positions receive fewer citations. Wagner et al. ( 2015 ) observed that men and women are covered equally on Wikipedia, but they also discovered that women on Wikipedia are portrayed differently from men. Jia et al. ( 2016 ) found that in online newspapers, women are underrepresented both in text and images.
The state of women in the film industry is similar to other domains: women are underrepresented and badly portrayed (Lauzen, 2018b ; Wood, 1994 ). The Boxed In 2017–18 report (Lauzen, 2018b ) observed a 2% decline in female major characters across all platforms, compared to the previous year.
To tackle the underrepresentation of women in movies in 1985, the cartoonist Alison Bechdel published a test in her comic strip Dykes to Watch Out For to assess how fairly women are presented in filmed media. The Bechdel–Wallace test (Bechdel, 1985 ) (denoted as the Bechdel test ) has three rules that a movie has to pass to be considered “women friendly”:
It has to have at least two women in it.
The women have to talk to each other.
The women must talk about something besides a man.
To Bechdel’s surprise, the media adopted her joke, and today it is a standard for female representation in movies (Douglas, 2017 ; Morlan, 2014 ; Hickey, 2014 ; Shift7, 2018 ; O’Hare, 2017 ). Today the Bechdel test is considered to be the mainstream benchmark for assessing the fairness of female representation in movies and today only 57% of current movies pass this test. Additionally, it is currently the only test that has available labeled data for about 8000 (Fest, 2019 ) out of 516,726 movies available on IMDb (IMDb, 2019b ).
The Bechdel test is also used by researchers. In recent years, studies have utilized the test to evaluate gender bias in movies. In 2014, Garcia et al. ( 2014 ) quantified the Bechdel test and also applied it to social media. They joined YouTube trailers, movie scripts, and Twitter data, which resulted in 704 trailers for 493 movies and 2970 Twitter shares. Garcia et al. created a social network of dialogues for these movies. Additionally, they constructed a network of dialogues between Twitter users who discussed the trailers. They mapped dialogues between men who were referring to women and between women who were referring to men. This mapping was used to calculate the Bechdel score. They found that trailers of movies which are male biased are more popular. Also, they discovered that Twitter dialogues have a similar bias to movie dialogues (Garcia et al., 2014 ).
In 2015, Agarwal et al. ( 2015 ) studied the differences between movies that pass and fail the Bechdel test. Similar to Garcia et al., Agarwal et al. also constructed social networks using screenplays. They created a classifier to automate the Bechdel test, which was trained on 367 movies and evaluated on 90. In the evaluation, they discovered that network-based features perform better than linguistic features. Additionally, they discovered that movies that fail the Bechdel test tend to have women in less central roles (Agarwal et al., 2015 ). With this being said, the Bechdel test has several major flaws. The test does not take into account if women are represented stereotypically (Waletzko, 2017 ). Additionally, there are movies that are considered feminist but do not pass the test (Florio, 2019 ). Moreover, the test is considered to be a low threshold since a film can pass the test with a single line of dialogue between two women (Shift7, 2018 ).
In 2017, Ramakrishna et al. ( 2017 ) utilized screenplays to study the differences in the portrayal of characters in movies. For the analysis, they used 945 screenplays. Mainly they performed linguistical analysis to capture gender stereotypes. They discovered that movies with female directors have less gender-biased casts. Also, they found that female characters use more positive language than males. Additionally, they constructed social networks from the screenplays and performed centrality analysis. The networks in the study were constructed. For the construction of the networks they used a method that was originally developed for converting books into social networks. In the same year, Sap et al. ( 2017 ) used connotation frames to study gender bias in films. They performed their analysis on 7772 movie screenplays, discovering that men were portrayed to have more authority than women. Additionally, they studied the relationship between connotation frames and the Bechdel test. Surprisingly, they found that movies where female characters speak with high agency are less likely to pass the Bechdel test.
Graph features and named entity recognition
Data science tools and techniques have evolved rapidly in the past couple of years (Donoho, 2015 ). In this study, we primarily utilized data science algorithms from the domains of natural language processing (NLP) and SNA to computationally analyze movie content, movie social network structure, and how movie features change over time.
Namely, we used NLP to extract character names from the movie subtitles by utilizing named entity extraction (NER) algorithms (Nadeau and Sekine, 2007 ). We used both Stanford Named Entity Recognizer (Finkel et al., 2005 ) and spaCy Python Package (Honnibal and Montani, 2017 ) to find where characters appear in the text.
To match characters’ names in the subtitles with characters’ full names, we utilized FuzzyWuzzy (Fuzzywuzzy, 2019 ), a Python package for fuzzy string matching. Specifically, we used FuzzyWuzzy’s WRatio (Fuzzywuzzy, 2018 ), a method for measuring the similarity between strings. WRatio uses several different preprocessing methods that rebuild the strings and compare them using Levenshtein distance (Levenshtein, 1966 ). Also, WRatio takes into account the ratio between the string lengths.
After extracting the movie characters, we constructed the movie social networks and used various graph centrality algorithms, such as closeness, betweenness, degree centrality, and PageRank (Brandes and Erlebach, 2005 ) to identify the most central characters in each constructed movie network.
Methods and experiments
Constructing movie social networks.
One of this study’s primary goals was to develop a straightforward algorithm that would construct the social network of character interaction within a given movie. We achieved this goal by utilizing movie subtitles Footnote 3 and a list of movie character names. Namely, given a movie, we constructed the movie social network G := 〈 V , E 〉, where V is the network’s vertices set, and E is the set of links among the network’s vertices. Each vertex v ∈ V is defined to be a character in the movie. Each link e := ( u , v , w ) ∈ E is defined as the interaction between two movie characters u and v , w times. For a movie with a given subtitle text and a given character list, we constructed the movie’s social network using the following steps (see Fig. 2 ):
First, we detected when each character appeared in the subtitles. To extract the characters from the subtitles we used NER, extracting all the entities which were labeled as a person or an organization. Additionally, for each entity, we stored the time the entity appeared in the movie.
Next, we matched the entities found in the subtitles with the character list. It worth mentioning that it is not possible to map one-to-one between the characters in the character list and the characters extracted from the subtitle. For example, in the movie The Dark Knight , Bruce Wayne was referred to as “Bruce Wayne” 3 times, as “Bruce” 16 times, and as “Wayne” 20 times.
To address the matching problem, we proposed the following mapping heuristic (see Algorithm 1). First, we split all the roles into first and last names and linked them to the actor and the character’s full name (line 2). Then, if there was only one character with a certain first or last name (one-to-one match), we linked to the character all its occurrences in the subtitles (lines 3–5). However, if we had several characters with the same first or last name, we did not always know who was referred to in the text. For example, in the movie Back to The Future there are three characters with the last name McFly; where only “McFly” was mentioned in the text, we could not determine which character was referenced. Another challenge we encountered was when only part of the character’s name was used. For instance, in the movie The Godfather , the main character is Don Vito Corleone, but he was never mentioned once by his full name because he usually was referred to as “Don Corleone.” Moreover, there are other Corleone family members in the movie. To overcome this challenge, we used WRatio to compare strings and match parts of a name to the full name. Using WRatio , we chose the highest matching character that received a score higher than Threshold (line 6).
In fact, we were able to overcome many of these problems by using hearing-impaired subtitles. In many hearing-impaired subtitles, the name of the speaking character is part of the text. This property allowed us to avoid most the problems we described earlier and gain additional information. For instance, the movie The Matrix has a scene in which Morpheus calls Neo, and we can know this only because of the tag [PHONE RINGS]. Afterward, there is an annotation “MORPHEUS:” which tells us that Morpheus is the one calling. Without this annotation, we could not know who is on the other end of the line (see Fig. 4 ).
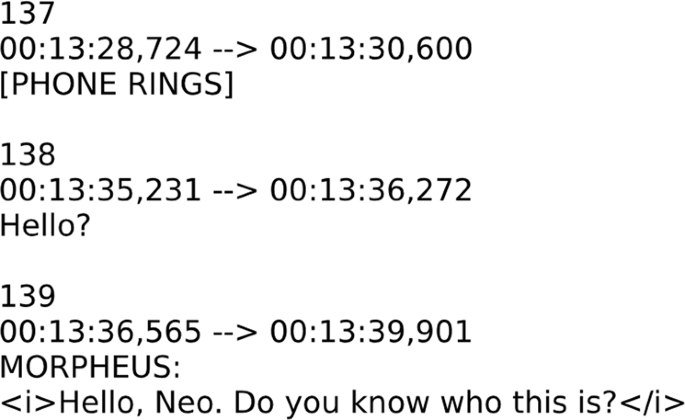
The textual format of subtitles in the SubRip format with additional data for hear-impaired. For example, the speaking charachter name, sounds in a textual fromat, etc.
Using the matched characters, we created a link between characters u and v if they appeared in the movie in a time interval less than threshold t seconds ( t was defined as 60). For each such appearance, we increased the weight w between u and v by one. Since in subtitles we do not have an indication of when each scene begins and ends, we used a heuristic to model the interaction between characters. We assumed that two characters who appear one after another in a short period of time probably relate. For example, in Fig. 2 we have part of the subtitles from the movie The Matrix . Morpheus introduces himself to Neo, and we know that Morpheus and Neo are talking within an interval of 5 s. Since, 5 s was smaller than the threshold, we increased the link weight between Morpheus and Neo by one.
To reduce the number of false positive edges, we filtered all the edges with weight lower than w min ( w min was defined as 3). There were two main reasons for the formation of edges that did not exist in the movie. The first case was when we matched an entity to the wrong character. The second case happened when in the interval of t seconds there was more than one scene. These kinds of false positive links add noise to the graph. Most of these links have a very low weight; hence, filtering edges with weight lower than w min helps remove false positive links.
Evaluations of constructed networks
In addition to constructing movie social networks, we also empirically quantified the quality of these networks. Evaluating movie networks is a challenging task. Creating a perfect ground truth is a manual and unscalable process. It requires spending several hours for each movie to manually create ground truth networks. In previous studies (Weng et al., 2009 ; Park et al., 2012 ; Tran and Jung, 2015 ; Lv et al., 2018 ), manually labeling of movies has been done at a very small scale with only several movies (see section “Related work”). Another option is to use the IMDb or TMDB datasets character lists as a ground truth to evaluate only the network nodes. However, these lists contain mostly unnamed characters that are impossible to detect, for example, Guard #2. To solve this issue we could try using name datasets to filter these lists, but we will lose many characters that have foreign names or characters with unreal names like Batman, Superman, etc. To evaluate the quality of the constructed networks without the presented issues, we compared them to other publicly available movie network datasets. Since it is challenging to manually annotate movies, most of the studies only compared their networks to a handful of manually annotated ground truth networks (see section “Related work”).
In this study, to the best of our knowledge, we performed the first large-scale, fully automatic comparison between movie networks. For the comparison, we used a dataset published in 2018 by Kaminski et al. ( 2018 ) (denoted as ScriptNetwork ); this is the only other publicly available movie social network dataset. The ScriptNetwork dataset is based on screenplays and can be considered as much easier content to parse than subtitles. Screenplays have additional information such as the exact name of the character who speaks in the scene even if this character is unnamed. For example, freckled kid is a character in the X-Men (2000) screenplay; unnamed characters like freckled kid are almost impossible to detect in regular texts like books or subtitles. Screenplays can be considered very close to the ground truth. However, screenplays sometimes have big differences with the final movie. For instance, in many screenplays, there are missing and even additional characters (see section “Discussion”).
To evaluate Subs2Network -constructed networks, we performed two types of evaluations:
Central character analysis : We tested if the most central roles in Subs2Network are actually the most central roles in the movie. As a ground truth, we used the IMDb ranking list similarly to Tran and Jung ( 2015 ). The IMDb characters list is ordered the same way as movie credits, which are ordered alphabetically or by the order of appearance (IMDb, 2019a ). For the evolution, we filtered out all the movies where the credits were in alphabetical order, which was only 1%. The actor rank in the credits is considered to be a direct indication of the actor’s power and prestige (Rossman et al., 2010 ). Furthermore, it is very rare for an actor not in the top-10 credited roles to be nominated for an Academy Award (Rossman et al., 2010 ). In other words this indicates that in most movies the credit order has a significance, and the top-10 movie credits are likely to include most of the central characters.
We tested if the top-5 and top-10 ranked nodes (characters) at Subs2Network are the top-5 and top-10 ranked on IMDb. Additionally, we performed the same test on networks constructed from screenplays (Kaminski et al., 2018 ). Our motivation behind this experiment was to verify that Subs2Network’s networks contain the most significant characters in the movie.
Network coverage : We tested if the edges in Subs2Network are the same edges as in other movie networks. For each movie, we created two sub-graphs containing the characters that exist in both networks. Then we calculated the edge coverage in the created sub-graphs. Given two graphs G and H , we define the edge coverage as \({\mathrm {{Coverage}}}_H(G) = \frac{{|E_G\, \cap \,E_H|}}{{|E_H|}}\) . We calculated Coverage Subs2Network ( ScriptNetwork ) and Coverage ScriptNetwork ( Subs2Network ).
In addition to using the Kaminski et al. ( 2018 ) dataset for the network evaluation, we also constructed a small dataset of 15 character co-appearance networks utilizing Amazon X-Ray (Stiffler and Sampaco, 2018 ). The movies in the dataset were selected randomly from the Amazon Prime TV main page, Footnote 4 which includes the most popular movies in the platform. The dataset was constructed semi-automatically in the following way: given a movie, we define the movie ’ s social network graph G xray := 〈 V xray , E xray 〉. Similar to Subs2Network , each character in the movie is represented as a vertex v ∈ V xray . Edges are defined as two characters that appear in the same scene according to Amazon X-Ray data. Namely, the set of movie edges E xray is defined to be \(E_{\mathrm {{xray}}}: = \{ (u,v,w)|u,v \in V_{\mathrm {{xray}}}\}\) , where w is the number of scenes in which u and v appeared in the same scene. Additionally, as with Subs2Network , we filtered all the edges with weights lower than 3. Similarly to our comparison with the Kaminski et al. ( 2018 ) dataset, we also calculated Network Coverage. Additionally, we used the fact that Amazon X-Ray is based on the finished movie, which includes additional data such as the time the character appeared in the movie. By utilizing G xray , we analyzed how well Subs2Network contains characters by their screen time. To this end, we calculated the total screen time (denoted as screen( v )) of each character in the X-Ray dataset and divided the characters into deciles according to their screen time. Lastly, we calculated for each decile, d i , i = 1..10, the percentage of characters that were detected by the Subs2Network algorithm, out of all the characters that were detected by Amazon X-Ray and had screen time in the d i decile. Namely, for each d i , we calculated \({CharCover}(d_i) = \frac{{|V_{Subs2Network}\, \cap\, \{ v \in V_{\mathrm {{xray}}}| {screen}(v)\, \in \,d_i\} |}}{{\{ v\, \in \,V_{\mathrm {{xray}}}| {screen}(v)\, \in \,d_i\} }}\) .
To evaluate and test our movie social network construction algorithm described above on real-world data, we assembled large-scale datasets of movie subtitles and movie character lists. In addition, we collected movie character lists from the IMDb (Internet Movie Database) website Footnote 5 and movie subtitles from 15,540 movies. Furthermore, we also used data from Bechdel test scores of 4658 movies. In the following subsections, we describe in detail the datasets we used.
IMDb dataset
To collect movie and actor data, we used IMDb, which is an online site that contains information related to movies, TV series, video games, etc. (IMDb, 2019b ). IMDb data is contributed by users worldwide. It contains 5,487,394 titles from which 505,380 are full-length movies (IMDb, n.d. ). In this study, we used the official IMDb dataset. Footnote 6 From the IMDb dataset, which contains only a subset of the IMDb database, we mainly used movies’ titles, crews, and ratings data.
Subtitle dataset
To inspect gender bias in movies, we decided to extract information out of subtitles. Subtitles are freely and widely available online on numerous sites. For instance, OpenSubtitles.org Footnote 7 alone hosts more than 500,000 English subtitles (opensubtitles.org, 2019 ) that were manually created by the community. We collected the subtitles using Subliminal Footnote 8 , a Python library for searching and downloading subtitles. Subliminal downloads subtitles from multiple sources, and using an internal scoring method, it decides which subtitles are the best for a specific movie. Using Subliminal, we downloaded subtitles for 15,540 movies.
Bechdel test dataset
Bechdel test data is available at Bechdel Test Movie List Footnote 9 , which is a community-operated website where people can label movies’ Bechdel scores. Using the Bechdel Test Movie List API, we downloaded a dataset that contains 7871 movies with labeled Bechdel scores, from which only 7322 are full-length movies.
Even for humans, it is a challenging task to determine if a movie actually passes the Bechdel test; Bechdeltest.com has a comments section where users discuss the scores and their disagreements (Agarwal et al., 2015 ). For example, according to Bechdeltest.com, the movie The Dark Knight Rises failed the test. However, by taking a closer look at the community comments, Footnote 10 we noticed users arguing regarding the test results, which are hard to determine.
Dataset preprocessing
The most critical part of building a social network of characters’ interaction is mapping correctly between the characters in subtitles and the characters in the character list. The IMDb character data includes data on even the most minor roles such as a nurse, guard, and thug #1. These nameless minor characters are almost impossible to map correctly to their subtitle appearances. Usually, they just add false positive edges and do not add additional information.
To clean the data from nameless characters, we created a blacklist of minor characters (for a detailed explanation of the blacklist construction process see Section S. 1 ). Additionally, to validate the characters’ names we used TMDb (The Movie Database) Footnote 11 , another community-built movie database. For each character, we matched the IMDb and TMDb data by the actor name. Then, we compared the lengths of the character names and kept the longer one. The usage of the longer names captures more variations of the name and helped us match more occurrences of the character in the subtitles. For example, in the film The Godfather (1972) James Caan portrays Sonny Corleone. Not surprisingly, on IMDb he is called Sonny Corleone, but on TMDB he is named Santino Sonny Corleone. In the film, he is addressed 12 times as Santino. By using the longer name, we can map these instances to the character.
Analyzing movie social networks to identify gender bias
Network features
To study gender bias in movies, we calculated five types of features: vertex features, network features, movie features, gender representation features, and actor features. Through the study, we analyzed how these features change over time. Additionally, we used these features to construct machine-learning classifiers. To create a ground truth for actors’ gender, we had to determine whether each actor was male or female. For most of the characters, we extracted the gender from IMDb similarly to Danescu et al. Danescu-Niculescu-Mizil and Lee ( 2011 ). IMDb has an attribute of “actor” or “actress,” which allowed us to identify gender. As we mentioned earlier, the IMDb dataset is only partial, so to overcome this issue we used a dataset that maps the first name to the gender. Footnote 12 In the rest of this section, we supply the definitions of these features.
Vertex features : For a given v ∈ V , a neighborhood is defined as a set of v friends, Γ( v ). Following are the formal definitions of the vertex-based features:
Total Weight : The total weight of all the edges, which represents the number of character v appearances in the movie, \({\mathrm {{Total}}}_{\mathrm {w}}(v) = \mathop {\sum}\nolimits_{\{ (v,u,w)|\left( {(v,u,w) \in E} \right.\} } w\) .
Closeness Centrality : The inverse value of the total distance to all the nodes in the graph. It is based on the idea that a node closer to other nodes is more central, \(C_{\mathrm {c}}(v) = \frac{1}{{\mathop {\sum}\nolimits_{v \in V} d (v,u)}}\) Brandes and Erlebach ( 2005 ), where d ( v , u ) is the shortest distance between v and u .
Betweenness Centrality : Represents the number of times that a node is a part of the shortest path between two nodes Brandes and Erlebach ( 2005 ). A junction (node) that is part of more paths is more central, \(C_{\mathrm {b}}(v) = \mathop {\sum}\nolimits_{s,t \in V} {\frac{{\sigma (s,t|v)}}{{\sigma (s,t)}}}\) Brandes and Erlebach ( 2005 ), where v ≠ s ≠ t , σ ( s , t ) is the number of those paths passing through some node v .
Degree Centrality: A node that has a higher degree is considered more central, \(C_{\mathrm {d}}(v) = \frac{{|{\mathrm{\Gamma }}(v)|}}{{|V|\, - \,1}}\) Brandes and Erlebach ( 2005 ).
Clustering : Measures link formation between neighboring nodes, \(C(v) = \frac{{2T(v)}}{{|{\mathrm{\Gamma }}(v)|(|{\mathrm{\Gamma }}(v)|\, - \,1)}}\) (Saramäki et al., 2007 ), where T ( v ) is defined as the number of triangles through vertex v where a triangle is a closed triplet (three vertices that each connect to the other two).
Pagerank: A node centrality measure that takes into account the number and the centrality of the nodes pointing to the current node Brandes and Erlebach ( 2005 ).
Edge Number —the number of edges in the network | E |.
Vertex Number —the number of vertices in the network | V |.
Number of Cliques —the number of maximal cliques in the network Brandes and Erlebach ( 2005 ).
Statistical Network Features —set of features which are based on the vertex features. From these features, we calculate statistical features for the entire network. We calculate the mean, median, standard deviation, minimum, maximum, first quartile, and third quartile.
Gender representation features
Triangles with N women : The number of triangles that contain N females and 3- N males, where N ∈ 1, 2, 3.
Percent of triangles with N women : The percent of triangles that contain N females and 3- N males, where N ∈ 1, 2, 3.
Females in Top-10 roles : The number of females in top-10 roles ordered by PageRank.
Male count: The number of male actors in the movie.
Female count : The number of female actors in the movie.
Movie features:
Release Year —the year when the movie was first aired.
Movie Rating —the rating the movie has on IMDb.
Runtime —the movie total runtime in minutes.
Genres —the movie genre by IMDb.
Number of Votes —number of votes by which the rating was calculated on IMDb.
Actor features:
Actor Birth Year —the year the actor was born.
Actor Death Year —the year the actor died.
Actor Age Filming —the age of the actor when the movie was released ( \(Release\,Year - Actor\,Birth\,Year\) ).
Network feature analysis
To examine the state of the gender gap, in movies generally and by genre in particular, we analyzed only the most popular movies (movies which had more than n votes on IMDb). We analyzed only the most popular movies since they have better, more correct data, and more importantly, better represent the mainstream media. To decide on n , we observed the distribution of movies by year. We found a right-tailed distribution and decided that n = 2000 should be a large enough number. To answer our first research question—if there are genres that do not show a gender gap (see section “Introduction”)—we calculated vertex and actor features (see section “Network features”) for all the roles. Next, we split the data by gender and movie genre. Finally, we utilized a Mann–Whitney U (Mann and Whitney, 1947 ) test on these features to check if there are statistical differences between the male and female roles in different genres.
To study relationships in movies, and to answer our second question regarding what relationships reveal about gender, we calculated all the relationship triangles in the network and grouped them by the number of women in each triangle. Afterward, we segmented the triangles by genres and how they changed over time.
To investigate the role of centrality by gender, our third research question regarding the centrality of female roles, we calculated PageRank for the nodes in all our movie networks. We analyzed the number of men and women in the top-10 characters in movies and examined how this number has changed over the years.
Constructing the Bechdel test classifier
As we described in section “Related work”, the Bechdel test is used to assess how fairly women are represented in a movie. The test has three criteria:
Are there at least two named women in the movie?
Do the women talk to each other?
Do the women talk about something other than men?
These criteria are hierarchical; hence, if a movie passes the last test, it has passed all of the tests.
To train the classifier, we extracted all the network, vertex, and gender representation features (see section “Network features”). For testing the trained model, we used the 1000 newest movies in the Bechdel test dataset. Footnote 13 The rest of the movies were used as the training set. As for the classifier, we used Random Forest with max depth 5 to avoid overfitting. For the classifier evaluation, we used AUC. This measure presents how many of the results the classifier is confident it classified correctly. Additionally, we compared our results to the results of Agarwal et al. ( 2015 ).
To answer the fourth research question regarding the fairness of female representation, we analyzed the change in the average probability of a movie passing the Bechdel test over time. Additionally, using the Random Forest feature importance, we inspected which feature was the most important for the Bechdel test classification. Finally, we analyzed the change over time by genre.
Alternative test
The Bechdel test has several major shortcomings; for instance, a movie passes the test if it consists of only one sentence between two women who do not speak about a man. For instance, American Pie 2 , which by no means can be considered to be a movie that fairly presents women, passes the Bechdel test in such a way. To offer solutions to the problems with the Bechdel test (see section “Discussion”), we propose a new gender equality test. We believe that a good test can be created by comparing the number of interactions according to each gender. Hence, we propose an interaction test that compares the total degree of male and female nodes. By utilizing over 15,000 movie social networks in our datasets, we observed that in only 16.7% of movies do female characters have an equal or higher total degree than male characters. Moreover, in 55.8% of analyzed movies, the total degree of male characters is at least twice as high as female characters. We think that a good rule of thumb for a movie should be \(0.8\, < \,\frac{ {{TotalDegree}_{\mathrm {F}}}}{{ {TotalDegree}_{\mathrm {M}}}}\, < \,1.2\) . The Gender Degree Ratio test is neither male nor female-biased; it is a gender equality test.
To evaluate the ability of the proposed test to distinguish between gender-biased and gender-equal movies, first we calculated the Gender Degree Ratio for all the movies in our dataset. Next, we performed significance tests between groups of movies with and without gender bias. Before performing the significance tests, we performed a Shapiro–Wilk test on the Gender Degree Ratio scores of our dataset to test if they distributed normally. To create the gender-biased and gender-equal movie lists, we utilized the three following movie lists:
The 100 best feminist films of all time (Rothkopf, 2018 ): From this list we had 67 movies in our dataset (see Section S. 2 ). We used this list to test if feminist movies get higher Gender Degree Ratio scores than the general population of movies.
100 Must see movies: The Essential Men’s Movie Library (McKay and McKay, 2019 )—from this list we had 79 movies in our dataset (see Section S. 2 ). This goal of using this list was to see if our test would give lower scores to male-centric movies than to the general population.
17 Blockbuster movies that surprisingly pass the Bechdel test (Allen, 2019 )—this list contains movies where women are not presented fairly but still pass the Bechdel test. From this list we had 15 in our dataset (see Section S. 2 ). The goal of testing these movies was to validate that they should fail the proposed test.
For the first two lists, we performed a significance test and compared their scores with the general population of movies. Additionally, the third list was used to test if the Gender Degree Ratio dealt with the shortcomings of the Bechdel test, specifically whether a movie with poor female representation yet passed the Bechdel test would fail our suggested ratio test.
To analyze the gender gap in the film industry, we analyzed subtitles of movies that had at least 1000 votes on IMDb. This resulted in a dataset containing 15,540 movies, which is a dataset 20 times bigger than the largest movie dataset currently available (Kaminski et al., 2018 ).
First, we analyzed the gender gap, in general, and by genres, in particular (see Tables S 1 and S 2 ). We found that the genres with the largest number of features that are distributed similarly between men and women are film-noir, history, horror, music, musical, mystery, and war. In these genres, 9 out of 10 features distribute similarly; only the clustering coefficient distributes differently between men and women. In terms of features, Total Weight and Weighted Betweenness are the features that distribute most similarities between the genders, with 15 out of 21 genres distributing the same. On the other side of the scale, Age Filming is the feature that distributes least similarly, with 0 out of 21 genres distributing similarly.
Second, to examine relationships among characters, we analyzed relationship triangles in the networks. We found that most triangles have three men, and triangles with three women are the least common (see Table 1 ). Out of 21 genres, in 8 genres the most common type of triangle is 3 men (without any women) and in all the others it is 2 men and a woman. According to the results, Romance is the genre with the most interaction among women and War is the genre where women have the least interaction. Inspecting the change in the number of triangles over time (see Fig. 5 ), we can observe that in many genres there is an equalizing improvement over the years, but there are genres like Sport without a big change.
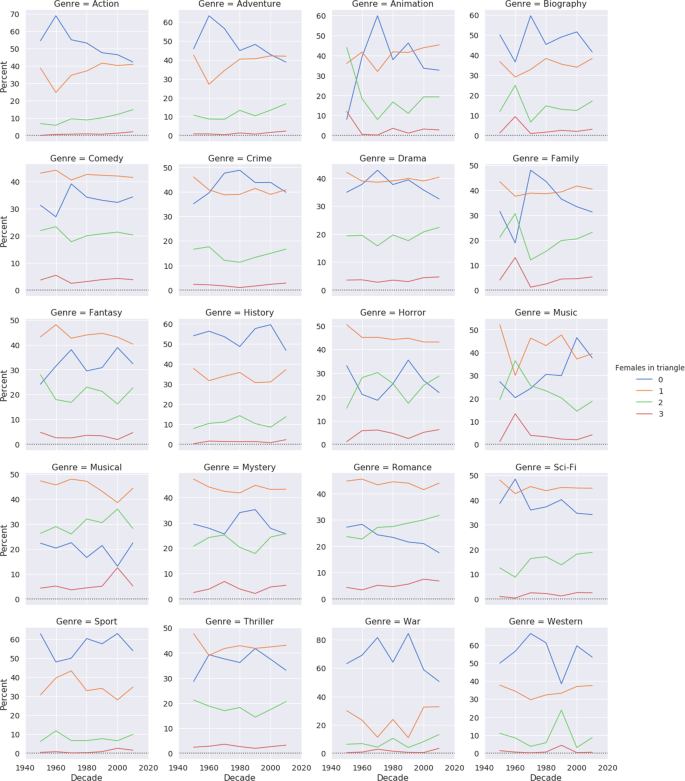
The change in the number of females in relationship triangles for each decaded for different genres.
Third, we analyzed how characters are ranked in terms of centrality (see Table 2 ). We found that among central roles, there are considerably more men than women. For example, men have about twice the roles that ranked in the top-10 most central roles than women. In all top-10 most central roles, the female percentage is the same except for the most central role.
Fourth, we analyzed the gender composition of the top-10 central roles in movies (see Fig. 6 ). We discovered that most of the movies have more men in central roles than women. Moreover, from the data, we can observe that there are almost no movies with no men and 10 women in the top-10 roles. Also, there are a considerable number of movies where the majority of the top-10 most central roles are men.
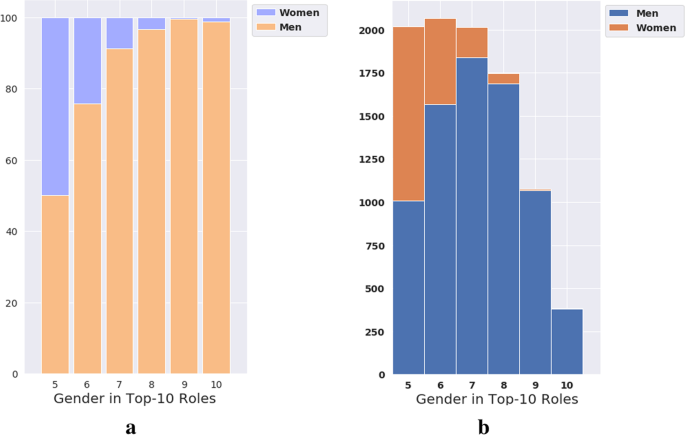
The distribution of movies by gender of the top-10 most central characters where: a The percentage of movies where out of top-10 role N are of a specific gender. b The number of movies where out of top-10 role N are of a specific gender.
Fifth, we wanted to observe how the percentage of women in top 1, 3 and 10 most central roles has evolved over time. We analyzed the change in this metric over almost from 1965 up to today Footnote 14 (see Fig. 3 ).
It can be seen from the network that there is a constant rise in the number of women in top-10 most central roles.
Sixth, to create an automatic classifier that can assess the fairness of female representation in movies, we created the Bechdel test classifier. Our classifier achieved an AUC of 0.81. We also inspected which feature was more important (see Table 3 ). Seven of 10 features were triangle-based features. Moreover, all the features in the table are a subset of the Gender Representation Features (see section “Network features”).
Next, we trained our automated Bechdel test classifier on all the labeled data and calculated the average probability of the classifier by decade on all the unlabeled data (see Fig. 7 ). We can see that there is a trend of growth. Also, we examined how the probability changed by genres (see Fig. 8 ). Comparing our results to Agarwal et al. ( 2015 ) (see Table 4 ), we found that our classifier performs better than Agarwal’s in terms of F1 score.
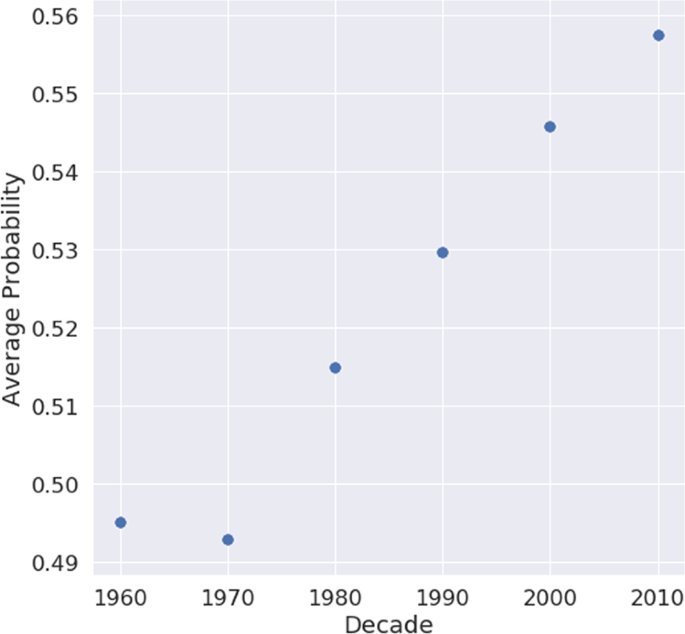
Trend line of the average probability of passing the Bechdel test in the past 60 years by decade.
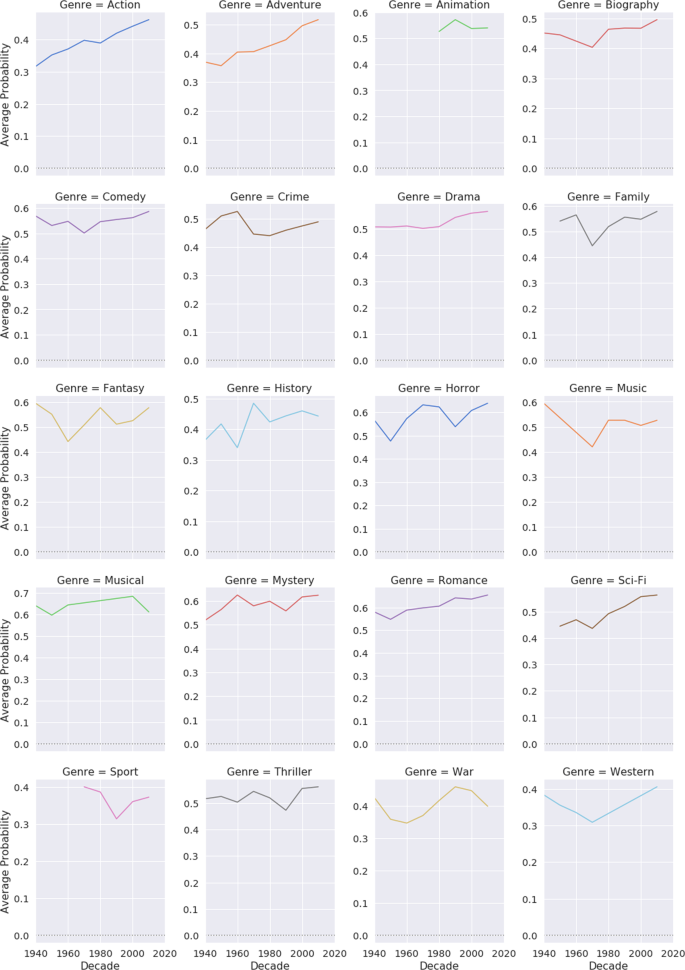
The average probability of a movie passing the Bechdel test by decade and genre.
Afterward, we analyzed the quality of the constructed social networks by comparing Subs2Network with the ScriptNetwork -released networks (Kaminski et al., 2018 ). We observed that the Subs2Network dataset contains 628 out of the 773 networks that appear in the ScriptNetwork dataset. On average, Subs2Network had more central characters than ScriptNetwork from the top-10 most central characters (see Table 5 ); for instance, in the top-10 characters Subs2Network matched 6.06 characters while ScriptNetwork matched 5.35 characters. In terms of edge coverage, we found that Subs2Network covered 65.4% of the edges in ScriptNetwork networks and ScriptNetwork covered 65.1% of the edges in Subs2Network networks. Additionally, we compared Subs2Network with networks we generated based on manually extracted Amazon X-Ray movie data. We observed that Subs2Network matched X-Ray nodes and edges at 79.6% and 54.5%, respectively. Additionally, when analyzing character matching by screen time, we found that we could detect main characters with a high accuracy of up to 96.4% (see Fig. 9 ).
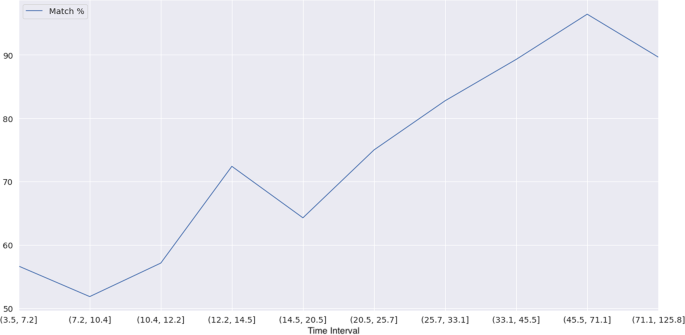
The percent of character that are overlapping between Amazon X-Ray and Subs2Network where the x axis is the screen time of the charcters.
Finally, we analyzed the Gender Degree Ratio test. We found that the average score of all the movies in the dataset was 0.6, meaning there were only 6 female interactions for every 10 male interactions. In fact, we found that today only 12% of all movies pass the gender degree ratio test by having scores between 0.8 and 1.2 (see Fig. 10 ). For instance, Resident Evil: Retribution and The Age of Innocence pass the test with scores of 1.06 and 0.94, respectively. On the other hand, Armageddon and Batman Begins fail the test with scores of 0.2 and 0.24, respectively. To check if the proposed test can distinguish between gender-biased and non-biased movies, we performed significance tests on two groups of movies. First, by performing the Shapiro–Wilk test, we observed that the movie scores were not from a normal distribution. Since the data was not normally distributed, we performed the Mann-Whitney- U test and found that list 1 (feminist movie list) distributed differently from the general population ( μ = 1.26, p -value = 6.7 × 10 −15 ). Also, we discovered that list 2 (male-biased movie list) scores also distributed differently from the general population ( μ = 0.34, p- value = 8.5 × 10 −07 ). Regarding the movies that surprisingly passed the Bechdel test, only the movie Grease passed the Gender Degree Ratio test.
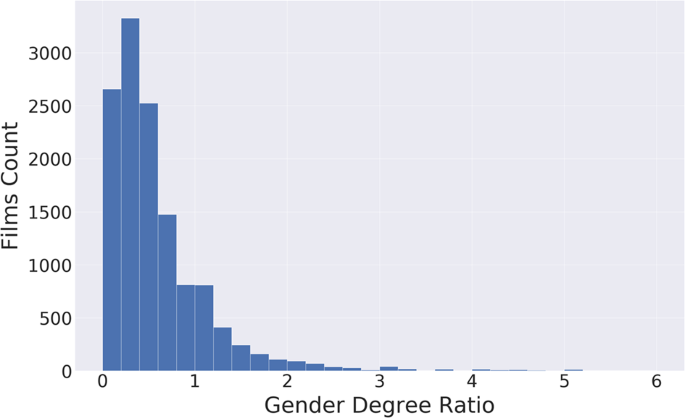
The number of movie and the ratio of between female and male characters.
In this study, we present a method that converts movie subtitles into social networks, and we analyze these networks to study gender disparities in the film industry. Using this method, we created the largest available corpus of movie character social networks. The method and the corpus are available for use by other researchers to study additional movies and even TV shows, and it has the potential to revolutionize the study of filmed media.
When looking at relationship triangles, we can see that in 77% of all triangles men are in the majority. In an equal society, we would expect to find that the number of triangles with three men, with three women, and with two men and two women would be the same. However, we discovered that, on average, there are 11.4 times more triangles with three men than with three women, and almost twice as many triangles with two men than two women. At a deeper level of granularity, we can see a difference in the number of triangles between different movie genres. The Romance genre has the highest number of triangles that have two and three women. On the other side of the scale, 90.6% of triangles in the War genre have a majority of men. This result makes sense intuitively. By looking at Fig. S. 1 , we can see that genres with a higher percentage of movies that pass the Bechdel test also have a higher percentage of triangles with a majority of women.
In terms of centrality (see Table 2 ), we can see that men have more central roles than women. We expected to find more females in less central roles, but the percentage of females distributes evenly in the top-10 most central roles. We believe that these results correspond to the total percentage of women in the dataset, which is 32.3% and is very similar to previous studies of Lauzen ( 2018a ) and Sap et al. ( 2017 ). This number is still lower than the total percentage of female roles in IMDb, which is 37.2%.
We also analyzed how many roles in a movie’s top-10 most central roles are those of women. Unsurprisingly, there is a dominance of movies with a majority of men. For instance, all Lord of the Rings movies have 10 men in the top-10 roles. We found only 5 films where all top-10 roles were female, and each of these featured only women (one of these films is called The Women , another movie Caged is about a women’s prison, and the movie The Trouble with Angels is about a girls’ school).
There is also the issue of what is considered fair. Mencarini ( 2014 ) states that fairness in gender context varies between cultures and historical periods. Sometimes women perceive their life as fair from a gender equality perspective while actually it is very low, and sometimes it is exactly the contrary. In a film context, some may argue that it is fair for war movies to have almost no women, while others will argue that it is not fair since women have taken part in all wars. Since fairness is subjective to measure, we used the Bechdel test, which is defined as “the basic measure to see if women are fairly represented in the film” (Fest, 2019 ). Centrality and fairness can sound very similar in the context of films, but they are two different notions. A character can be very central and very stereotypical at the same time. For example, Cinderella is the protagonist (most central character) in her story, but she is cooking and cleaning all day, and her life becomes better only when a rich and handsome prince arrives.
We also presented an automated Bechdel test classifier that can help assess the fairness of how women are presented in movies. We trained our model on data collected from bechdeltest.com, and we have indications that our model is even more accurate than the above presented results. We found that many movies on bechdeltest.com are misclassified. For example, The Young Offenders passes the test on bechdeltest.com (although the site does state this result is ‘dubious’), but our work classifies it as a fail. The reverse is true for the movie Never Let Go . Based on these observations, we believe that our classifier can automatically classify movies with high confidence in the classification. Moreover, while the Bechdel test is certainly a useful and important test, it fails to account for many parameters such as the centrality of the characters, repression, etc. Basically, if there is a movie with only two women who appear in one scene and talk about something other than men for 2 seconds, then the movie will pass the traditional Bechdel test. However, this is the only test that has data that can be used to train a classifier. Our classifier partially tackles this problem since it calculates a score of how strongly the movie passes the test.
To deal with the issues of the Bechdel test, we proposed a new test based on the ratio of the number of female interactions to the number of male interactions in a movie. We found that only 12% of all movies passed our Gender Ratio test (see Fig. 10 ), revealing how dominant gender disparities continue to be in the film industry. As anticipated, we found in our test that feminist movies received higher scores than the average movie. Additionally, we discovered that movies that passed the Bechdel test but did not have good female representation failed the Gender Ratio test, just as we had hoped. These results indicate that our proposed test dealt with some of the major problems of the Bechdel test and has the ability to differentiate between films with good and bad female representation. However, the test is not perfect and does not take into account context. For instance, we can see that Grease passed our test even though women in the film were presented stereotypically.
In future work, we are planning to perform statistical tests to compare the distributions of the degrees of male and female nodes and present a more accurate test. Creating a more accurate assessment of how women are truly represented in films requires manually watching thousands of movies and labeling data, which is impossible with the current research limitations. In the future, we plan to develop a more advanced method based on deep learning to create a better algorithm that will be able to create a much more accurate assessment of movie gender equality, taking into account additional parameters such as the context of the movie.
We also calculated the average probability of passing the Bechdel test for all the movies in our dataset that do not have a Bechdel test score. Afterward, we inspected the change in the average probability of movies passing the test over a long period of time and by different genres. In almost all genres there is a trend of improvement, and there is a correlation between relationship triangles and the Bechdel score. Looking at Fig. 8 , we see that historically war movies have the lowest probability of passing the Bechdel test.
There are many factors that affect our method’s accuracy. The most critical factor is the quality of both the subtitles and the cast information from IMDb. In movies where the name of the character in the subtitles does not correspond to IMDb data, the actor cannot be linked to a character. During our study, we stumbled upon subtitles with spelling mistakes and other inconsistencies. Also, in some movies like superhero movies, we did not know how to link the different identities of a character with names such as “Captain America,” that potentially could be filtered because it looks like a nameless character. In addition, nameless characters like “Street Pedestrian” sometimes eluded our cleaning process. There is a balance between cleaning the IMDb data too much and not enough. We observed that more accurate networks were in movies that had hearing-impaired subtitles since they have additional data and are less affected by the NER accuracy. Some of these limitations will be addressed in future research. Additionally, there are many different improvements that can done to increase the accuracy of the networks; for instance, it is possible to use co-reference resolution, train an NER for subtitles, etc.
One of the biggest challenges of this study was to evaluate the quality of the constructed movie networks. For the evaluation, we compared the networks created by our algorithm with the networks created by screenplay analysis and by Amazon X-Ray. Screenplays have easier content to analyze than subtitles, and they contain plenty of structured information, such as character names, scenes, etc. However, there are also some shortcomings in using screenplays. First, only a small fraction of movies have screenplays available online. Currently, the Internet Movie Script Database (IMSDb) Footnote 15 has only 1198 scripts, while there are hundreds of thousands of movies’ subtitles available online. Moreover, many publicly available screenplays are drafts and have major differences from the actual movies. For instance, the Minority Report Footnote 16 screenplay used by Kaminski et al. is completely different from the movie; almost all the characters’ names are different. Another example can be found in the X-Men (2000) movie where the character Beast appears in the screenplay. However, due to over-budget concerns, Beast was cut from the movie. From inspecting screenplays, we discovered many additional examples of extra, missing, and renamed characters. These problems show that comparing subtitles to screenplays is like comparing apples to oranges. The comparison indicates that there is a similarity between the networks, but it cannot be used as a precise measure of accuracy.
In addition to using screenplays to evaluate the constructed networks, we also used networks that were generated based on Amazon X-Ray. Unlike the screenplays, Amazon X-Ray is based on the finished movie and offers a more accurate representation of the movie’s social network. Using the X-Ray based networks, we found that even though sub2network is based on much less data than the X-Ray based networks, the networks are very similar. This similarity indicates that our graphs represent the essence of the movie. The biggest limitation in using X-Ray to generate movie social networks is that the full X-Ray dataset is not publicly available, and must be extracted manually.
There is no doubt that the presented method is not perfect. For instance, in the film Star Wars: Episode VI—Return of the Jedi (see Fig. 1 ), Princess Leia never meets Obi Wan Kenobi. Obi Wan Kenobi only talks with Luke about her, which created an edge in the graph. Nonetheless, from the network evaluation, we learn that the constructed networks represent the movie and have enough correct data to supply insights. Moreover, it is possible to perform many calibrations and parameter tunings to improve the method ’ s accuracy; for instance, we can manually select better subtitles to get more accurate networks. Such calibrations are out of the scope of this study, but in future studies we will explore such options.
Besides utilizing subtitles and screenplays, there are other possible ways to analyze movie content. The first option is to analyze movie videos as Weng et al. ( 2009 ) did. The problem with video analysis is that it is an expensive process which requires high computational power, especially when the plan is to analyze thousands of full-length movies. Moreover, most movies are copyrighted and not freely available online. The second option is to use speech recognition to extract information, which is what Park et al. ( 2012 ). However, this option has similar drawbacks.
Conclusions
Data science can provide great insights into many problems, including the gender gap in movies. In this work, we created a massive dataset of movie character interactions to present the largest-to-date SNA of gender disparities in the film industry. We constructed this dataset by fusing data from multiple sources, and then we analyzed the movie gender gap by examining multiple parameters over the past century.
Our results demonstrate that a gender gap remains in nearly all genres of the film industry. For instance, 3.5 times more relationship triangles in movies have a majority of men. In terms of top-10 most central movie roles, again there is a majority of men. However, we also saw an improvement in equality over the years. Today, women have more important movie roles than in the past, and our Bechdel test classifier quantifies this improvement over time by calculating a movie’s overall score. In a future study, we plan to analyze TV series, actors’ careers, and directors’ careers in a similar in-depth manner. We also plan to implement the tests that were proposed in (Walt et al., 2017 ) as well as develop new tests to gain further insight into how genders are represented in the film industry.
Data availability
The code and datasets generated during and analysed during the current study are available in the on the project’s website ( http://data4good.io/dataset.html#Movie-Dynamics ) and repository ( https://github.com/data4goodlab/subs2network ).
The Star Wars icons were created by Filipe de Carvalho and are licensed under CC BY-NC 4.0)
http://www.moviegalaxies.com
Many of the used movies’ subtitles were created by crowd-sourcing, i.e., by people who volunteered to create the subtitle.
American Beauty, Back to the Future, Back to the Future Part II, Funny People, Gladiator, Inglourious Basterds, Jurassic Park, Knight and Day, Marley & Me, Public Enemies, Serenity, Street Kings, Terminator 2 Judgment Day, The Godfather, The Godfather Part II.
https://www.imdb.com/
https://www.imdb.com/interfaces/
https://www.opensubtitles.org
https://github.com/Diaoul/subliminal
https://bechdeltest.com/ . Note the site uses the Bechdel test variation where women have to have names.
https://bechdeltest.com/view/3437/the_dark_knight_rises/
https://www.themoviedb.org
http://www.ise.bgu.ac.il/faculty/fire/computationalgenealogy/first_names.html
Similarly to Agarwal et al. ( 2015 ) this about 20%.
The bechdeltest.com data is mostly based on newer movies and there is too much noise in the graph for movies before 1965.
https://www.imsdb.com/
https://www.imsdb.com/scripts/Minority-Report.html
Agarwal A, Balasubramanian S, Zheng J, Dash S (2014) Parsing screen-plays for extracting social networks from movies, in ‘Proceedings of the 3rd Workshop on Computational Linguistics for Literature (CLFL)’, Associationfor Computational Linguistics, Gothenburg, Sweden, pp. 50–58
Agarwal A, Zheng J, Kamath S, Balasubramanian S, Dey SA (2015) Key female characters in film have more to talk about besides men: automating the Bechdel test. In: Proceedings of the 2015 Conference of the North American Chapter of the Association for Computational Linguistics: Human Language Technologies. pp. 830–840
Allen J (2019) 17 blockbuster movies that surprisingly pass bechdel test. https://thewhisp.mommyish.com/entertainment/movies/blockbuster-movies-pass-bechdel-test-surprising/ . Accessed on 14 Jan 2020
Bechdel A (1985) The rule. Dykes to Watch Out For Ithaca, New York: Firebrand Books
Brandes U, Erlebach T (2005) Network analysis. Lecture notes in computer science, vol. 3418
Cohen A (2017) Women and hollywood sexism in the film industry problem. https://www.refinery29.com/en-us/2017/10/175956/melissa-silverstein-women-hollywood-gender-inequality . Accessed on 17 Dec 2018
Danescu-Niculescu-Mizil C, Lee L (2011) Chameleons in imagined conversations: a new approach to understanding coordination of linguistic style in dialogs. In: Proceedings of the 2nd Workshop on Cognitive Modeling and Computational Linguistics. Association for Computational Linguistics. pp. 76–87
Donoho D (2015) 50 years of data science. http://courses.csail.mit.edu/18.337/2015/docs/50YearsDataScience.pdf
Douglas N (2017) The bechdel test, and other media representation tests, explained. https://lifehacker.com/the-bechdel-test-and-other-media-representation-tests-1819324045 . Accessed 23 Jan 2019
Entman RM (1989) How the media affect what people think: an information processing approach. J Politics 51(2):347–370
Article Google Scholar
Fest BT (2019) About|Bechdel test fest. http://bechdeltestfest.com/about/ . Accessed 21 Nov 2019
Finkel JR, Grenager T, Manning C (2005) Incorporating non-local information into information extraction systems by Gibbs sampling. In: Proceedings of the 43rd annual meeting on Association for Computational Linguistics. Association for Computational Linguistics, pp. 363–370. https://doi.org/10.3115/1219840.1219885
Florio A (2019) 22 movies that don’t pass the bechdel test but are still pretty darn feminist. https://www.bustle.com/p/22-movies-that-dont-pass-the-bechdel-test-but-are-still-pretty-darn-feminist-16961528 . Accessed 2 Jul 2019
Fox C (2018) The scully effect: I want to believe in stem. https://seejane.org/wp-content/uploads/x-files-scully-effect-report-geena-davis-institute.pdf . Accessed 15 Nov 2019
Fuzzywuzzy (2018) Fuzzywuzzy wratio function code. https://github.com/seatgeek/fuzzywuzzy/blob/df5b67a32d7ddaf2e86fe1247b6ff7e3b57e0805/fuzzywuzzy/fuzz.py#L224 . Accessed 17 Feb 2019
Fuzzywuzzy (2019) Fuzzy string matching in python. https://github.com/seatgeek/fuzzywuzzy . Accessed 4 Feb 2019
Garcia D, Weber I, Garimella VRK (2014) Gender asymmetries in reality and fiction: the Bechdel test of social media. In: ‘ICWSM’, pp. 131–140
Hickey W (2014) The dollar-and-cents case against hollywood’s exclusion of women | fivethirtyeight. https://fivethirtyeight.com/features/the-dollar-and-cents-case-against-hollywoods-exclusion-of-women/ . Accessed 23 Jan 2019
Honnibal M, Montani I (2017) spacy 2: natural language understanding with bloom embeddings, convolutional neural networks and incremental parsing, in press
IMDb (2019a) How are cast credits ordered? why don’t the main stars appear at the top of the cast? https://help.imdb.com/article/contribution/filmography-credits/how-are-cast-credits-ordered-why-don-t-the-main-stars-appear-at-the-top-of-the-cast/G39K5N4YYV2QJ4GR?ref_=helpsect_pro_3_4# . Accessed 22 Nov 2019
IMDb (2019b) Press room—imdb. https://www.imdb.com/pressroom/?ref_=helpms_ih_gi_whatsimdb . Accessed 15 Dec 2018
IMDb (n.d.) Press room—imdb. https://www.imdb.com/pressroom/stats/ . Accessed 17 Dec 2018
Jia S, Lansdall-Welfare T, Sudhahar S, Carter C, Cristianini N (2016) Women are seen more than heard in online newspapers. PLoS ONE 11(2):e0148434
Kaminski J, Schober M, Albaladejo R, Zastupailo O, Hidalgo C (2018) Moviegalaxies-social networks in movies. Harvard Dataverse
Larivière V, Ni C, Gingras Y, Cronin B, Sugimoto CR (2013) Bibliometrics: global gender disparities in science. Nat News 504(7479):211
Lauzen M (2018a) It’s a man’s (celluloid) world: portrayals of female characters in the 100 top films of 2017. Center for the Study of Women in Television and Film
Lauzen MM (2018b) Boxed in 2017–18: Women on screen and behind the scenes in television. Technical report, San Diego State University
Levenshtein VI (1966) Binary codes capable of correcting deletions, insertions, and reversals. Sov Phys dokl 10:707–710
Lv J, Wu B, Zhou L, Wang H (2018) Storyrolenet: social network construction of role relationship in video. IEEE Access 6:25958–25969
Mann HB, Whitney DR (1947) On a test of whether one of two random variables is stochastically larger than the other. The Annals of Mathematical Statistics 18(1):50–60
Article MathSciNet Google Scholar
McKay B, McKay K (2019) 100 must see movies: the essential men’s movie library. https://www.artofmanliness.com/articles/100-must-see-movies/ . Accessed 2 Jan 2020
Mencarini L (2014) Gender equity. Springer, Dordrecht, pp. 2437–2438
Google Scholar
Morlan K (2014) Comic-con vs. the bechdel test. https://web.archive.org/web/20150316161800/http://www.sdcitybeat.com/sandiego/article-13243-comic-con-vs-the-bechdel-test.html . Accessed 25 Jan 2019
MPAA (2018) Theme report 2017. https://www.mpaa.org/wp-content/uploads/2018/04/MPAA-THEME-Report-2017_Final.pdf . Accessed 5 Jan 2019
Nadeau D, Sekine S (2007) A survey of named entity recognition and classification. Lingvist Investig 30(1):3–26
O’Hare J (2017) Oscars 2017: half of the best picture nominees fail this test for gender equality. https://www.globalcitizen.org/en/content/oscars-best-picture-bechdel-test/ . Accessed 23 Jan 2019
opensubtitles.org (2019) Subtitles—download movie and TV series subtitles. https://www.opensubtitles.org/en/statistics . Accessed 15 Dec 2018
Park S-B, Oh K-J, Jo G-S (2012) Social network analysis in a movie using character-net. Multimed Tools Appl 59(2):601–627
Polce-Lynch M, Myers BJ, Kliewer W, Kilmartin C (2001) Adolescent self-esteem and gender: exploring relations to sexual harassment, body image, media influence, and emotional expression. J Youth Adolesc 30(2):225–244
Ramakrishna A, Martínez VR, Malandrakis N, Singla K, Narayanan S (2017) Linguistic analysis of differences in portrayal of movie characters. In: Proceedings of the 55th annual meeting of the association for computational linguistics, vol 1: Long papers. pp. 1669–1678
Rose S (2018) One female director for every 22 men: Hollywood’s stark diversity problem | film | the guardian. https://www.theguardian.com/film/2018/jan/04/hollywood-diversity-sees-no-improvement-in-2017-report-finds . Accessed 16 Dec 2018
Rossman G, Esparza N, Bonacich P (2010) I’d like to thank the academy, team spillovers, and network centrality. Am Sociol Rev 75(1):31–51
Rothkopf J (2018) 100 best feminist movies you need to watch. https://www.timeout.com/newyork/movies/best-feminist-movies-of-all-time . Accessed 21 Jan 2020
Sap M, Prasettio MC, Holtzman A, Rashkin H, Choi Y (2017) Connotation frames of power and agency in modern films. In: Proceedings of the 2017 conference on empirical methods in natural language processing. pp. 2329–2334
Saramäki J, Kivelä M, Onnela J-P, Kaski K, Kertesz J (2007) Generalizations of the clustering coefficient to weighted complex networks. Phys Rev E 75(2):027105
Article ADS Google Scholar
Shift7 (2018) Female-led films outperform at box office for 2014–2017. https://shift7.com/media-research . Accessed 23 Jan 2019
Silverstone R (2003) Television and everyday life. Routledge
Smith SL, Choueiti M (2010) Gender disparity on screen and behind the camera in family films; the executive report
Smith S, Pieper K, Choueiti M (2017) Inclusion in the director’s chair? gender, race, & age of film directors across 1,000 films from 2007–2016. Media, Diversity, & Social Change Initiative
Stiffler L, Sampaco S (2018) Amazon x-ray lets viewers take a deeper dive into shows, as 2018’s most popular are revealed—geekwire. https://www.geekwire.com/2018/amazon-x-ray-lets-viewers-take-deeper-dive-shows-2018s-popular-revealed/ . Accessed 28 Jan 2020
Tran QD, Jung JE (2015) Cocharnet: extracting social networks using character co-occurrence in movies. J Univ Comput Sci 21(6):796–815
UNIC (2017) Unic anual report 2018. https://www.unic-cinemas.org/fileadmin/user_upload/wordpress-uploads/2017/06/UNIC_AR2018_online.pdf . Accessed 5 Jan 2019
University SDS (2017) Women remain underrepresented in hollywood, study shows. https://phys.org/news/2017-09-women-underrepresented-hollywood.html . Accessed 7 Dec 2018
Wagner C, Garcia D, Jadidi M, Strohmaier M (2015), It’s a man’s wikipedia? Assessing gender inequality in an online encyclopedia. In: The International Conference on Web and Social Media. pp. 454–463
Waletzko A (2017) Why the Bechdel test fails feminism|huffpost. https://www.huffpost.com/entry/why-the-bechdel-test-fails-feminism_b_7139510 . Accessed 2 Sept 2019
Walt H, Koeze E, Dottle R, Wezerek G (2017) Creating the next bechdel test | fivethirtyeight. https://projects.fivethirtyeight.com/next-bechdel/. Accessed 16 Jan 2019
Weng C-Y, Chu W-T, Wu J-L (2009) Rolenet: movie analysis from the perspective of social networks. IEEE Trans Multimed 11(2):256–271
Wilson JD, MacGillivray MS (1998) Self-perceived influences of family, friends, and media on adolescent clothing choice. Fam Consum Sci Res J 26(4):425–443
Wood JT (1994) Gendered media: the influence of media on views of gender. Gendered lives: communication, gender and culture. pp. 231–244
Worldbank T (2018) Unrealized potential: the high cost of gender inequality in earnings. https://www.worldbank.org/en/topic/gender/publication/unrealized-potential-the-high-cost-of-gender-inequality-in-earnings . Accessed 9 Dec 2018
Download references
Acknowledgements
We would like to thank Carol Teegarden for editing and proofreading this article to completion. Also, we thank Mandy Henner, Sergey Korotchenko, and Ariel Plotkin for their help.
Author information
Authors and affiliations.
Department of Software and Information Systems Engineering, Ben-Gurion University of the Negev, Beer-Sheva, Israel
Dima Kagan & Michael Fire
Nottingham University Business School, Nottingham, UK
Thomas Chesney
You can also search for this author in PubMed Google Scholar
Corresponding authors
Correspondence to Dima Kagan , Thomas Chesney or Michael Fire .
Ethics declarations
Competing interests.
The authors declare no competing interests.
Additional information
Publisher’s note Springer Nature remains neutral with regard to jurisdictional claims in published maps and institutional affiliations.
Supplementary information
Supplemental material, rights and permissions.
Open Access This article is licensed under a Creative Commons Attribution 4.0 International License, which permits use, sharing, adaptation, distribution and reproduction in any medium or format, as long as you give appropriate credit to the original author(s) and the source, provide a link to the Creative Commons license, and indicate if changes were made. The images or other third party material in this article are included in the article’s Creative Commons license, unless indicated otherwise in a credit line to the material. If material is not included in the article’s Creative Commons license and your intended use is not permitted by statutory regulation or exceeds the permitted use, you will need to obtain permission directly from the copyright holder. To view a copy of this license, visit http://creativecommons.org/licenses/by/4.0/ .
Reprints and permissions
About this article
Cite this article.
Kagan, D., Chesney, T. & Fire, M. Using data science to understand the film industry’s gender gap. Palgrave Commun 6 , 92 (2020). https://doi.org/10.1057/s41599-020-0436-1
Download citation
Received : 25 September 2019
Accepted : 11 March 2020
Published : 13 May 2020
DOI : https://doi.org/10.1057/s41599-020-0436-1
Share this article
Anyone you share the following link with will be able to read this content:
Sorry, a shareable link is not currently available for this article.
Provided by the Springer Nature SharedIt content-sharing initiative
This article is cited by
Hollywood caught in two worlds the impact of the bechdel test on the international box office performance of cinematic films.
- Johann Valentowitsch
Marketing Letters (2023)
Quick links
- Explore articles by subject
- Guide to authors
- Editorial policies

Ask Yale Library
My Library Accounts
Find, Request, and Use
Help and Research Support
Visit and Study
Explore Collections
Film Studies Research Guide: Research Topics
- Film Reviews
- Films & Videos
- Screenplays/Filmscripts
- Archives & Institutes
- Critical Approaches & Problems
- Directors, Actors, Writers, etc.
- Filmmaking, Producing, etc.
- Genres, Styles, Categories, Series
- History and/of Film
- International Cinema
- Literature & Film
- Movie Business & Studios
- Music & Sound in Movies
- Social & Other Aspects
- Themes, Subjects & Characters
- Annuals & Directories
- Bibliographies & Filmographies
- Biographies, Credits & Plots
- Dictionaries & Encyclopedias
- Festivals & Awards
- Guides & Companions to Films
- Organizations & Associations
Topics in Film Studies
What's here.
This section of the Film Studies Research Guide provides assistance in many of the particular subjects in Film Studies. The pages discuss particular issues and list key resources on those topics.
You can get to the topical pages from the main navigation bar above or from the links below. The links are listed alphabetically.
- Critical Approaches and Problems: Theory, Methodology, Philosophy and Aesthetics
- Literature & Film
- The Movie Business & Studios
- Music & Sound in Movies
- Social & Other Aspects of Filmmaking
- Themes, Subjects and Characters
Director, Yale Film Archive

- << Previous: Archives & Institutes
- Next: Animation >>
- Last Updated: Mar 24, 2024 11:29 PM
- URL: https://guides.library.yale.edu/film

Site Navigation
P.O. BOX 208240 New Haven, CT 06250-8240 (203) 432-1775
Yale's Libraries
Bass Library
Beinecke Rare Book and Manuscript Library
Classics Library
Cushing/Whitney Medical Library
Divinity Library
East Asia Library
Gilmore Music Library
Haas Family Arts Library
Lewis Walpole Library
Lillian Goldman Law Library
Marx Science and Social Science Library
Sterling Memorial Library
Yale Center for British Art
SUBSCRIBE TO OUR NEWSLETTER
@YALELIBRARY

Yale Library Instagram
Accessibility Diversity, Equity, and Inclusion Giving Privacy and Data Use Contact Our Web Team
© 2022 Yale University Library • All Rights Reserved
217 Film Research Paper Topics & Ideas
18 January 2024
last updated
Film research paper topics provide a rich, multifaceted canvas for critical analysis. One can explore genre theory and its evolution, scrutinizing the symbiotic relationship between society and film genres, such as sci-fi, horror, or romance. Another fruitful area lies in auteur theory, assessing the unique stylistic fingerprints of directors, like Kubrick, Hitchcock, or Miyazaki. Delving into film adaptations provides an opportunity to study narrative transformation across different media. Studying representation in film, be it racial, gender, or cultural, opens a lens into societal norms and biases. In turn, there is the exploration of film technologies and their influence on the cinematic experience. Film criticism and its role in shaping public perception can also be an intriguing topic. With every cinematic element providing a potential research topic, film studies truly cater to diverse academic interests.
Best Film Research Paper Topics
- Impacts of Technological Advancements on the Animation Film Industry
- Portrayal of Mental Health in Contemporary Cinema
- Cultural Stereotypes in Global Film Industry
- Feminist Theory Analysis in Alfred Hitchcock’s Films
- Violence and its Effect on Teenagers in Action Films
- Representation of History in Steven Spielberg’s Movies
- Examination of Homosexuality in Bollywood Cinema
- Depiction of Science and Technology in Science Fiction Films
- Philosophical Themes Explored in the Matrix Trilogy
- Influence of Film Noir on Modern Thrillers
- Comic Book Adaptations: Success and Failure Factors
- Cinema’s Role in Promoting Environmental Awareness
- Portrayal of AI and Robotics in Films: A Comparative Study
- Evolution of Special Effects in the Film Industry
- Relationship Between Music and Narrative in Film
- Examination of Sociopolitical Contexts in Iranian Cinema
- Impacts of Hollywood on Global Film Cultures
- Aesthetic Evolution in French New Wave Cinema
- Exploring Symbolism in Stanley Kubrick’s Films
- Influence of the Silent Era on Modern Film Techniques
- Alien Depictions: Reflection of Societal Fears in Film
- Use of Dreams and Subconscious in David Lynch’s Films
- Examination of Masculinity in Clint Eastwood’s Westerns
- Evolution of Animation: From Disney to Studio Ghibli
- Exploring Religion and Spirituality in Indian Cinema
Easy Film Research Paper Topics
- Interpreting Magic Realism in Guillermo del Toro’s Films
- Analysis of Adaptation Theory in Book-to-Film Transitions
- Modern Film Criticism: Influence of Online Review Platforms
- Exploration of Absurdism in Coen Brothers’ Films
- Social Media Portrayal in Contemporary Film
- Influence of Film on Public Perception of Historical Events
- Analysis of Horror Tropes in Japanese Cinema
- Portrayal of Childhood and Growing Up in Animated Films
- Impacts of Censorship Policies on Film Creativity
- Narrative Techniques in Quentin Tarantino’s Films
- The Role of Fashion and Costume in Period Films
- Ethical Considerations in Documentary Filmmaking
- Representation of Post-Apocalyptic Themes in Cinema
- Exploring Cultural Identity in African Cinema
- Analysis of Musical Scores in Film Noir
- Examination of Adaptation of Video Games Into Films
- Portrayal of Space Travel in Science Fiction Films
- Evolution of Stop Motion Techniques in Cinema
- Cultural Interpretations of Love and Romance in Films
- Examination of Dystopian Themes in Animated Films
- Analyzing the Concept of Anti-Heroes in Film
- Exploring Satire and Parody in Comedy Films
- Portrayal of Race and Ethnicity in Hollywood Cinema
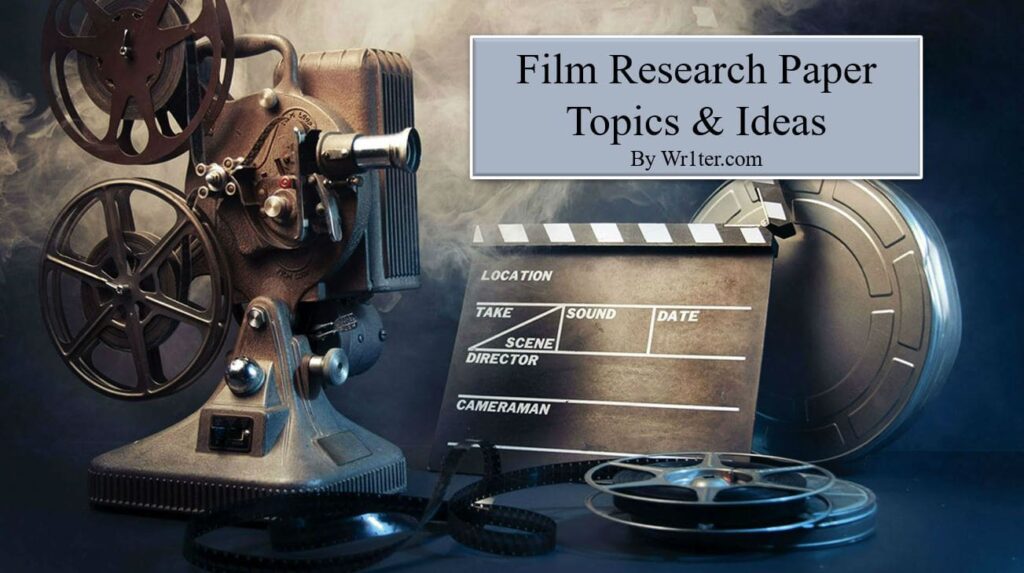
Interesting Film Research Paper Topics
- Depiction of Cybercrime in Contemporary Cinema
- Influence of German Expressionism on Tim Burton’s Aesthetic
- Use of Color and Lighting in Guillermo del Toro’s Films
- Examination of LGBTQ+ Representation in Hollywood Cinema
- Roles of Politics in the Cuban Film Industry
- Portrayal of Disability in Modern Films
- Treatment of Time Travel in Science Fiction Films
- Analyzing the Evolution of Cinematography Techniques
- Cultural Influences in South Korean Cinema
- Roles of Nostalgia in Recreating Period Pieces
- Importance of Film Score in Creating Atmosphere
- Analysis of Propaganda Techniques in North Korean Cinema
- Representation of Women in Action Films
- Ethical Implications of Animal Use in Film Production
- Impacts of Streaming Platforms on Film Distribution
- Evolution of Film Censorship: A Comparative Study
- Examination of Familial Relationships in Animated Films
- Interpretation of Surrealism in Luis Buñuel’s Films
- Examination of Biopics: Historical Accuracy vs. Dramatic License
- Impacts of Film Festivals on Independent Cinema
- Exploring Existentialism in Ingmar Bergman’s Films
Film Research Paper Topics About Students
- Influence of Silent Cinema on Modern Filmmaking Techniques
- Portrayal of Social Media’s Impact on Adolescents in Contemporary Movies
- Bollywood vs. Hollywood: A Comparative Study of Storytelling Styles
- Representation of Mental Health in Animation Movies
- Foreign Language Films: Enhancing Global Cultural Understanding among Students
- The Role of Women in Classic Film Noir: A Critical Analysis
- Analysis of Auteur Theory in Modern Independent Cinema
- Evaluating the Accuracy of Historical Dramas: A Fact vs. Fiction Study
- Roles of Music in Creating Emotional Impact: A Study on Film Scores
- Racial Stereotyping in Blockbuster Movies: A Comprehensive Study
- Interpreting Symbolism and Metaphor in Fantasy Genre Films
- Exploring Subliminal Messages in Advertising and Product Placement in Films
- Understanding the Social Impact of LGBTQ+ Representation in Cinema
- Examining the Evolution of Special Effects in the Film Industry
- Influence of Japanese Anime on Western Animation Styles
- Significance of Set Design in Creating Realistic Period Films
- Ethics in Documentary Filmmaking: Truth vs. Storytelling
- Roles of Cinematography in Enhancing Narratives in Films
- Impacts of Sci-Fi Films on Popular Science Understanding Among Students
- Subtext and Satire: The Power of Political Commentary in Movies
- Narrative Techniques in Autobiographical and Biographical Films
- Artistic Censorship: Its Impact on Creative Freedom in International Cinema
Film Research Paper Topics Made by Students
- Transformation of Comic Books to Silver Screen: A Historical Analysis
- Gender Representation in Oscar-Winning Films Over the Decades
- The Evolution of Horror Films: From Psycho to Paranormal
- Motion Capture Technology: Changing the Landscape of Animation Films
- Examination of Propaganda in World War II Era Cinema
- Unpacking the Influence of Music Scores in Emotional Storytelling
- Analyzing Film Noir: The Aesthetics of Grit and Shadows
- Impacts of Streaming Platforms on Traditional Movie Theatres
- Silent Era to Talkies: How Did Sound Revolutionize Cinema?
- Special Effects Techniques: The Making of Modern Sci-Fi Movies
- The Hero’s Journey: Exploring Mythological Themes in Films
- Ethical Dilemmas in Documentaries: A Study on Bias and Objectivity
- Dissecting the Psychological Depth of Christopher Nolan’s Films
- Censorship in Films: A Comparative Study Between Countries
- The Role of the Auteur in Independent Filmmaking
- How Disney Reinvents Fairy Tales: A Feminist Perspective
- Bollywood vs. Hollywood: Contrasting Storytelling Techniques
- Exploration of Coming-of-Age Themes in Teenage Films
- Stereotyping in Movies: Assessing the Consequences on Society
- Roles of Cinematography in Creating a Film’s Atmosphere
Film Research Paper Topics About Popular Movies
- Influences of Classic Literature on “The Lord of the Rings” Trilogy
- Propaganda and War-Time Politics in “Casablanca”
- Exploring Social Alienation in “Taxi Driver”
- Cinematography Techniques Used in “Citizen Kane”
- Implicit Racism Portrayed in “Gone with the Wind”
- Animation Evolution: A Study on the “Toy Story” Series
- Gender Stereotypes in Disney Princess Films
- Symbolism and Surrealism in “Pan’s Labyrinth”
- Cult Status and Cultural Impact of “Pulp Fiction”
- Examination of Crime and Morality in “The Godfather”
- “Fight Club” and the Commentary on Consumerism
- Psychological Analysis of the Protagonist in “A Clockwork Orange”
- Role of Music in the Narrative of the “Star Wars” Saga
- Concept of Love in Richard Linklater’s “Before” Trilogy
- “The Shining” and Its Divergence From the Original Novel
- “Inception” and the Philosophy of Dream Interpretation
- The Relevance of “1984” in the Age of Mass Surveillance
- Science and Fiction: A Study on “Interstellar”
- Decoding the Metaphor of “The Matrix”
- “The Dark Knight”: A Modern Take on Heroism and Villainy
- Biblical Themes in Darren Aronofsky’s “Noah”
- Investigating Historical Accuracy in “Schindler’s List”
Film Research Paper Topics on History
- The Impact of World War II on Hollywood: Propaganda and Patriotism
- The Rise of Film Noir: Exploring the Dark Side of Post-War America
- Cultural Significance of Epic Historical Films: From “Gone with the Wind” to “Gladiator”
- Uncovering Hidden Histories: Films That Shed Light on Forgotten Events
- The Representation of Ancient Civilizations in Hollywood: Myths and Realities
- The Birth of Cinema: Exploring the Early Pioneers and Their Historical Films
- Propaganda in Film During the Cold War: From East to West
- The Role of Women in Historical Films: Portrayals and Progressions
- Depicting the Civil Rights Movement on the Silver Screen: From “Selma” to “The Help”
- The Historical Accuracy of Biographical Films: Balancing Fact and Fiction
- The Representation of Colonialism in Film: Perspectives and Power Dynamics
- The Cinematic Portrayal of World War I: From “All Quiet on the Western Front” to “1917”
- Political Upheaval and Film: Exploring Revolutionary Movements on Screen
- The Historical Evolution of War Films: From Silent Era to Modern Blockbusters
- The Representation of Indigenous Peoples in Historical Films: Stereotypes and Subversions
- Holocaust’s Theme in Movies: Documenting Trauma and Commemorating History
- The Role of Historical Films in Shaping Collective Memory: Remembering the Past
- Film and the Civil Rights Movement: Documenting Activism and Progress
- The Portrayal of Historical Figures: Heroes, Villains, and Complex Characters
Research Paper Topics on Music in Films
- Musical Transformations: Exploring the Evolution of Film Scores
- Melodic Narrative: The Role of Music in Conveying Storytelling Elements in Films
- Harmonic Innovations: Examining the Impact of Experimental Music in Cinematic Soundtracks
- Rhythm and Emotion: Analyzing the Connection Between Beat and Mood in Film Music
- Melancholic Melodies: Investigating the Use of Music to Evoke Sadness in Movies
- Orchestral Powerhouses: Unveiling the Influence of Symphonic Scores in Epic Films
- Sonic Identity: The Significance of Musical Themes in Establishing Character Presence in Movies
- Vocal Expressions: Exploring the Role of Singing in Enhancing Cinematic Narratives
- Cinematic Soundscapes: Investigating the Use of Ambient Music in Establishing Atmosphere
- Cultural Harmonies: Examining the Representation of Different Music Genres in Film Scores
- Experimental Soundtracks: Analyzing the Use of Avant-Garde Music in Artistic Films
- Jazzy Tones: Unveiling the Influence of Jazz Music in Enhancing the Cinematic Experience
- Musical Archetypes: Exploring the Portrayal of Heroes and Villains through Music in Films
- Electronic Ambience: Investigating the Role of Techno and Electronic Music in Movie Soundtracks
- Musical Narrative Arcs: Analyzing the Structure and Development of Musical Scores in Films
- Emotional Resonance: Examining the Connection Between Music and Audience Response in Movies
- Historical Harmonies: Unveiling the Role of Period Music in Depicting Different Eras in Film
- Musical Cues: Exploring the Use of Leitmotifs in Creating Musical Associations in Cinema
- Cross-Cultural Fusion: Investigating the Incorporation of World Music in Film Scores
- Genre-Bending Soundtracks: Analyzing the Influence of Non-Traditional Music in Different Film Genres
Horror Film Research Paper Topics
- Evolution of Horror Cinema: From Silent Movies to CGI Monsters
- The Role of Sound Design and Score in Creating Horror Atmosphere
- Psychoanalysis and Fear: The Hidden Messages in Classic Horror Films
- Ghost Stories in Film: Cultural Differences in Horror Narratives
- Horror Tropes and Their Social Commentary: A Deep Dive
- Relevance of Classic Monsters in Modern Horror Films
- The Impact of Globalization on Horror Film Narratives
- Found Footage Films: The Realism in Fear and Dread
- Women in Horror: Representation and Character Development
- Dissecting Cinematic Techniques in Iconic Horror Scenes
- Psychological Horror vs. Slasher Films: A Comparative Study
- Portrayal of Mental Illness in Horror Movies: Is It Responsible?
- Exorcism and Religion: The Unholy Alliance in Horror Films
- Horror Comedy: The Unique Balance of Scares and Laughs
- Adaptation of Horror Literature into Film: Successes and Failures
- Body Horror: Physical Mutation as a Symbol of Inner Turmoil
- Dark Tourism in Horror Films: Spooky Locations and Their Histories
- Post-Apocalyptic Horror Films: Reflecting Societal Anxieties
- Creature Features: The Significance of Non-Human Antagonists
- Examining the Unsettling Nature of Uncanny Valley in Horror Movies
- Interplay of Light and Darkness in Horror Cinematography
- Reception Studies: How Do Different Cultures Respond to Horror Films?
- Queer Representation in the Horror Genre: Progress and Challenges
Film Research Paper Topics About Monster Movies
- Evolution of Monster Depictions in Cinema: A Historical Analysis
- Cultural Implications of Monster Symbols in Japanese Kaiju Films
- Transcending Fear: Psychoanalytic Theory in Monster Movies
- Dissecting the Female Monster: Gender Dynamics in Horror Films
- Monsters as Metaphors: Environmental Themes in Monster Cinema
- The Gaze of the Other: Racial and Ethnic Subtexts in Monster Films
- Unveiling Monstrosity: The Role of Cinematography in Monster Reveals
- CGI vs. Practical Effects: Creating Convincing Monsters in Modern Cinema
- How Do Score and Sound Design Enhance the Fright Factor in Monster Movies?
- Parallels Between Classical Mythology and Contemporary Monster Films
- The Lure of the Lovecraftian: Cosmic Horror in Monster Movies
- Alien Invaders: The Intersection of Monster and Science Fiction Genres
- Transformation and Fear: The Role of Werewolves in Cinema
- Gothic Influence on the Evolution of Vampire Movies
- The Horror of the Familiar: Domesticity as a Setting in Monster Films
- Monstrosity Reimagined: Postmodern Approaches in Monster Cinema
- Archetypes and Stereotypes: Monster Character Analysis in Film
- Sequels and Series: Examining the Longevity of Monster Movie Franchises
- Deconstructing Zombie Cinema: Metaphors of Disease and Decay
- Audience Reactions and Expectations: A Study on Monster Movie Reception
- Silent Era to Sound: The Influence on Early Monster Movies
- Comedy in the Midst of Horror: Analyzing Humor in Monster Films
To Learn More, Read Relevant Articles
484 sports research topics & good ideas, 295 criminal justice research topics & ideas.
- UNC Libraries
- Subject Research
- Film & Cinema Research
- Finding Articles / Journals
Film & Cinema Research: Finding Articles / Journals
- Find a Film
Film Journals
E-journals search, sample search terms, article searching tips.
- Databases & Reviews
- Film Terms & More
- Citing Film
- Watch Online
- UNC Film Studies
Criticism & Reviews
A Film Review is generally an article that is published in an online or print newspaper, magazine, or scholarly work that describes and evaluates a film. A review often offers an opinion or focuses on making a recommendation.
Film Criticism is generally written by an expert in film studies or film scholar. The criticism often presents the film within a specific context (theoretical, social, political or historical) while drawing on a larger dialog and positioning their argument within the field.

Looking for more Journals and articles for your research? Just look by selecting E-journals underneath the search fill in box on the UNC Libraries' main page.

From here you can search for Journals using your subject

or search using keywords to find the journal using the Search fill in box.

You can also use the Search articles (without finding the journal first) using the article search box in the top right corner.

- Motion pictures -- Aesthetics
- Motion pictures--United States—Aesthetics
- Motion pictures--Plots, themes, etc.
- Motion pictures--Reviews
- Film Criticism
Media Librarian

Using Find@ UNC

- << Previous: Find a Film
- Next: Databases & Reviews >>
- Last Updated: Mar 27, 2024 10:37 AM
- URL: https://guides.lib.unc.edu/filmresearch
Search & Find
- E-Research by Discipline
- More Search & Find
Places & Spaces
- Places to Study
- Book a Study Room
- Printers, Scanners, & Computers
- More Places & Spaces
- Borrowing & Circulation
- Request a Title for Purchase
- Schedule Instruction Session
- More Services
Support & Guides
- Course Reserves
- Research Guides
- Citing & Writing
- More Support & Guides
- Mission Statement
- Diversity Statement
- Staff Directory
- Job Opportunities
- Give to the Libraries
- News & Exhibits
- Reckoning Initiative
- More About Us

- Search This Site
- Privacy Policy
- Accessibility
- Give Us Your Feedback
- 208 Raleigh Street CB #3916
- Chapel Hill, NC 27515-8890
- 919-962-1053

An official website of the United States government
The .gov means it’s official. Federal government websites often end in .gov or .mil. Before sharing sensitive information, make sure you’re on a federal government site.
The site is secure. The https:// ensures that you are connecting to the official website and that any information you provide is encrypted and transmitted securely.
- Publications
- Account settings
Preview improvements coming to the PMC website in October 2024. Learn More or Try it out now .
- Advanced Search
- Journal List
- Sensors (Basel)

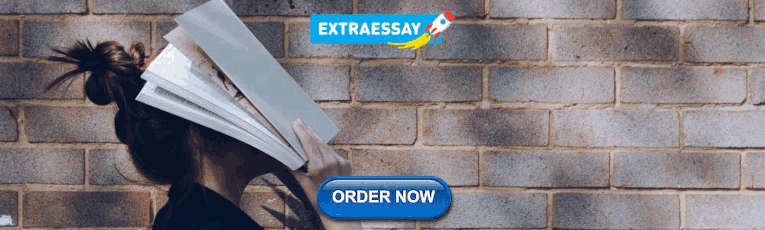
Movie Recommender Systems: Concepts, Methods, Challenges, and Future Directions
Sambandam jayalakshmi.
1 Department of Computer Science and Engineering, Vel Tech Multi Tech Dr. Rangarajan Dr. Sakunthala Engineering College, Chennai 600 062, India; gro.hcetitlumhcetlev@imhskalayaj (S.J.); gro.hcetitlumhcetlev@nhsenag (N.G.)
Narayanan Ganesh
Robert Čep.
2 Department of Machining, Assembly and Engineering Metrology, Faculty of Mechanical Engineering, VSB-Technical University of Ostrava, 708 00 Ostrava, Czech Republic
Janakiraman Senthil Murugan
3 Department of Computer Science and Engineering, Vel Tech High Tech Dr. Rangarajan Dr. Sakunthala Engineering College, Chennai 600 062, India; [email protected]
Associated Data
Not applicable.
Movie recommender systems are meant to give suggestions to the users based on the features they love the most. A highly performing movie recommendation will suggest movies that match the similarities with the highest degree of performance. This study conducts a systematic literature review on movie recommender systems. It highlights the filtering criteria in the recommender systems, algorithms implemented in movie recommender systems, the performance measurement criteria, the challenges in implementation, and recommendations for future research. Some of the most popular machine learning algorithms used in movie recommender systems such as K -means clustering, principal component analysis, and self-organizing maps with principal component analysis are discussed in detail. Special emphasis is given to research works performed using metaheuristic-based recommendation systems. The research aims to bring to light the advances made in developing the movie recommender systems, and what needs to be performed to reduce the current challenges in implementing the feasible solutions. The article will be helpful to researchers in the broad area of recommender systems as well as practicing data scientists involved in the implementation of such systems.
1. Introduction
Modern technology has revolutionized the volume, variety, and velocity at which data are generated. Digitalization of day-to-day experiences has led to the big data era. However, the enormous data have also led to the problem of information overload. Information overload may be defined as the state of being overwhelmed by the sheer volume of data presented to an average human for processing and decision making. Data mining methods can aid in obtaining and processing the relevant data and deal with the issue of information overload. Perhaps the most widely exploited tool among data mining methods is recommender systems.
Recommender systems work by assessing the available information about the likely patterns of the users and making suggestions from the information available [ 1 ]. The suggestions from the recommender systems help the system users find what is most suitable for them. Recommender systems are designed to ease product or service searches based on the least information available about the features [ 2 ]. A combination of various factors is used to assess the correlations in patterns and user characteristics to determine the best product suggestions for the customers [ 3 ].
The development of recommender systems depends on the field of application. The major application is in e-commerce websites where they suggest to the users the products or services based on the information available such as past search, age, gender, and other preferences [ 4 ]. They are also applied in job search platforms where the website suggests to a candidate the best possible positions fit for the skills. Since various industries have moved from an age of little available data to the era of big data, the junk information available is so much that it can delay the decision-making process. The recommender systems are typically made to ease the information search over the online systems so that the users find a more convenient way to connect to their preferences [ 5 ].
One of the applications of recommender systems is suggesting movies to watch to customers based on their preferences data. Movie recommender systems work by assessing the characteristic features of the users to make endorsements to the customers on what is best suited for them. It works by assessing the age, the previous preferences, gender, the content, context, and other demographic data to propose the movies. It checks the similarity among the users and items in the system to determine what could best fit the new user [ 6 ]. For example, a child will most likely receive recommendations for movies that children watch such as cartoons and animations based on the best similarity index for the children. Apart from that, children of various ages have different types of cartoons/animations to watch, and the recommender systems will propose the best depending on what other children of the same age are watching.
Movie recommender systems have helped the users overcome the chunk of information online to find only what is suited for them [ 7 ]. They use data mining techniques that match the similarities and help users find what is best suited for them [ 8 ]. Various criteria determine how the recommender systems work. The criteria are based on machine learning or deep learning algorithms that are used in matching the similarities before the suggestions are made. The algorithms achieve different levels of accuracy and require different computational times to retrieve the suggestions. Various computational algorithms have been proposed and used to increase the efficiency of recommender systems e [ 9 ]. However, each algorithm has its advantages and disadvantages; these make using the systems meet various needs based on their strengths. To reduce the limitations of each, the algorithms may be combined so that they perform better in making the recommendations [ 10 ].
This review paper aims to assess the challenges of recommender systems and make propositions to increase the accuracy of the systems. It assesses the recommendation approaches, the evaluation criteria of their efficiency, the challenges of these approaches, and possible solutions. A systematic literature review is conducted to determine the findings of the operational characteristics of the various recommendation approaches used and the performance criteria. The author aims to suggest the best solutions to make the approaches work better to achieve the operational expectations of the users.
The rest of the paper is arranged as follows: Section 2 details the methodology followed in this article. Section 3 describes different types of recommendation systems. Section 4 highlights some of the most popular machine learning algorithms used in movie recommender systems. Section 5 details the commonly used metaheuristic algorithms in movie recommendation tasks. Model metrics used for verifying the accuracy of recommendation systems are discussed in Section 6 . Some common problems with recommendation systems are discussed in detail in Section 7 . A critical discussion is presented in Section 8 . Section 9 presents the concluding remarks and the limitations of the study.
2. Review Methodology
This section describes the method used in obtaining information for the literature review. Peer-reviewed sources were used to gather information about movie recommender systems. The databases used were EBSCO Academic Search Premier, ScienceDirect, IEEE Library, ResearchGate, SpringerLink, and the ACM Portal. Google Scholar was also used to find leads to specific aspects of recommender systems for review.
Search Descriptors: Some of the keywords used in finding information about the movie recommender systems were “movie recommender systems”, “movie personalization”, “algorithms used in movie recommender systems”, “filtering techniques in movie recommender systems”, and “machine learning model metrics and measurement criteria”.
Inclusion Criteria: The inclusion criteria were papers that had information about recommender systems, the information had to be from published peer-reviewed sources. The paper abstracts were read to verify the validity of their information for use in this study. The exclusion criteria were papers that had grey literature on recommendation systems. The inclusion criteria for the articles and the methodology steps are summarized in Table 1 and Figure 1 .

Steps in conducting the systematic review.
Selection criteria for including sources in this review.
3. Movie Recommendation Systems
Movie recommendation work by filtering out data that is irrelevant and including only that which have matching characteristics or features [ 11 ]. As highlighted earlier, the world has moved from an era of scarcity of data online to an exponential growth in data. The systems work by manipulating the data to make sure it is efficient to drive data-driven decisions. In the jungle of available information about products, the systems need to evaluate what fits a certain customer and what does not. The systems go further in target and retargeting marketing to increase product viewership and hence increase the chance of the customers purchasing [ 12 ].
It is important for the developers to come up with systems that have higher performance characteristics and efficiency in matching the similarities in customer wants to seal the product sales or movie viewership [ 7 ]. The major types of filtering methods are collaborative filtering, content-based filtering, context-based filtering, and hybrid filtering.
3.1. Collaborative Filtering
Collaborative filtering works by matching the similarities in items and users. It looks at the characteristics of the users and the characteristics of the items the users have watched or searched for before [ 13 ]. In general, latent features obtained from rating matrices are looked at. In movie recommender systems, the recommendations are made based on the user information and what other people with similar user information are watching. For example, collaborative filtering in movie recommender systems picks the user demographic characteristics such as age, gender, and ethnicity [ 14 ]. Through these features, movie recommendations are made that match other people with similar demographic characteristics and previous user search history. Collaborative filtering suffers from a cold start if the user has not input any information, or the information is too little for any accurate clustering. In these cases, it does not know what to suggest [ 15 ]. The accuracy of the suggestion is also limited because people with similar demographic characteristics may not have similar preferences [ 16 ].
3.2. Content-Based Filtering
In contrast to collaborative filtering, content-based techniques employ user and item feature vectors to make recommendations. The fundamental differences between the two approaches are that content-based systems recommend items based on content features (no need for data about other users; recommendations about niche items, etc.) whereas collaborative filtering is based on user behaviour only and recommends items based on users with similar patterns (no domain knowledge; serendipity, etc.). A content-based filtering method works by making movie proposals to the user based on the content in the movies. It recognizes that clustering in the collaborative filtering recommendations may not match the preferences of the users [ 7 ]. The tastes and preferences of people with similar demographic characteristics are very different; what person X likes may not be similar to what person Y likes to watch. To solve this problem, content-based filtering algorithms give recommendations based on the contents of the movies [ 17 ]. In movie recommendations, some of the contents are the key characters and the genre of the movie.
3.3. Context-Based Filtering
This filtering technology is an improvement of the collaborative filtering method. It assumes that if person A and person B hold the same opinion on issue X, it is most likely that the same people will hold the same opinion/preference/thinking on a different issue Z. For example, if both people are attracted to Christmas movies from Netflix, it is most likely that they will still like Christmas movies by Showmax. The context-based filtering method recommends items with similar features or characteristics because the applications have just been extended to a different context [ 12 ]. It makes the same suggestions though the contexts are different. In most cases, web browsers import bookmarks and other settings when one upgrades from one browser to the next. This represents a change in context, since most of the settings and other items are imported into the new context, and the data available are used in making useful suggestions. Similarly, movie recommender systems may make a similar recommendation based on data from the previous context [ 18 ]. It is worth mentioning here about context-aware recommender systems (CARS), where the concept of context is well defined [ 19 , 20 , 21 , 22 ]. CARS acclimatize to the exact condition in which the recommended item will be used [ 23 , 24 , 25 , 26 ]. In this respect, CARS could avoid recommending a very long film to a user after a stressful day at work or suggest a romantic film if he/she is in the company of his/her partner.
3.4. Hybrid Filtering
This is a filtering technique that applies the concepts of all the other algorithms. It combines both collaborative filtering, content-based filtering, and context-based filtering to overcome the challenges of each method [ 10 ]. It is superior because it achieves higher performance in making the suggestions and also a faster computational time [ 11 ]. For instance, collaborative filtering may lack information about domain dependencies while content-based filtering lacks information about the preferences of the people [ 6 , 9 , 27 ]. A combination of these overcomes these challenges since user behaviour data and the content data are used to come up with recommendations.
4. Machine Leaning Algorithms for Movie Recommendation Systems
These are the algorithms that are used in filtering information and data mining so that the desired outcomes can be achieved. It is essential to understand the working of the information filtering methods so that the right algorithm is selected for the specific task in recommender systems [ 28 ].
4.1. K-Means Clustering
This is one of the simplest collaborative filtering approaches that categorizes the users based on their interests [ 29 ]. It is common for someone who wants to purchase an item to ask someone who has already purchased the product for their opinion. There is a higher chance that the influence of the current owner will affect the preferences and the tastes of the potentially new owner. Similarly, the algorithm compares the interesting features that can be associated with individuals that are classified to be within a group [ 30 ].
K -means clustering uses interests that are common among the users such as age, gender, movie time, history of the previous movies watched, etc. K -means clustering aims to group the features into clusters that represent the characteristics of the group [ 31 ]. If the classification is based on age, the probable K -means clustering will use children, teens, youth, and adult clustering methods. If a client falls within any of these age groups, movies are recommended based on what other people within that age group do. If the clustering depends on age, the closer an age is to the centroid age, the better the classification recommendation. The steps in the classification are measuring the similarity between the user and item features, selection of the neighbours, computing the prediction, and suggesting it [ 14 ].
4.1.1. Measurement of the Similarities
The first step is finding the similarity in the user features that the new user has with the previous system users. The algorithm always has the basic classifications for a beginning, where the user can give inputs and the predictions can be made [ 32 ]. Common features in finding the similarities are age, previous history, and geographical locations. Other recommender systems in movie theatres, including the price, the time to watch the movies, etc., are used in coming up with the means (centroids) for clustering. The distance from the centroids can be based on a Pearson correlation, cosine-based similarities, or an adjustment of the cosine-based similarity. The calculation of the similarity may be item-based or user-based. Item-based computation finds the similarities based on the features in the movies that similar people liked. If it is user-based, the calculation of the centroids is based on the demographic features of the user [ 15 ].
The computation of the similarities between items or users is shown in the mathematical equations below:
The equation above computes the correlation between the user and the item; it computes the closeness of the value to the centroid value. It is assumed that the two items i ∩ j are the correlated features (items or users); the value r j ¯ is the centroid feature, while the value r i is the value of the new user or new feature to be compared through correlation [ 33 ].
4.1.2. Selection of the Neighbours
There is always a consideration when developing the algorithm. The key metrics are the accuracy to obtain and the running time of the algorithm. To increase the accuracy of an algorithm, a large number of neighbours, which increases the computational time of the algorithm, is required. If a smaller computational time is needed, accuracy will be compromised [ 34 ]. To strike a balance, the selection may be threshold-based or use the top-N technique. The threshold technique will run only a specific number (sample number that meets the threshold value) of assessments of the neighbours and predict if that threshold is reached. For example, if the population is 1000, the system will run a prediction from 100 samples and predict out of the 100 samples [ 35 ]. In the top-N technique, only the top number of similarities (N) is run rather than the whole population of neighbours. For example, it will select only the top 10 for suggestions based on the nearest neighbours rather than assessing the whole population [ 36 ].
4.1.3. Prediction Computation
The computation of the subsequent predictions is based on the closest neighbours found in the system database. The prediction is obtained by the formula below:
The prediction or the nearest neighbour to the centroid ( K -mean) is made. In the equation above, the K -means is represented by r u ¯ while the correlation of the other variable on the right-hand side of the equation gives the nearest neighbour, both used in making the suggestion prediction [ 27 ].
4.1.4. Limitations of K -Means Clustering
- Cold-Start Problem : This is a prediction problem that happens with a new user to the system. There is very little information about the user; hence it is difficult for the system to make any predictions until the user starts feeding some information that can be correlated to and suggestions made based on the user or previous item characteristics. The negative impact is the system accuracy is greatly reduced [ 6 ]. It is because of this problem that new and excellent movies are not recommended to users, or new users do not find what is best for them.
- Sparsity in the dataset : The recommendation system involves assessing a large amount of data in the movie database. The users only look for a few items in the database; they are not able to use and assess a significant portion of the database to effectively evaluate the features. Apart from that, the users do not rate the movies they watched in the system. It becomes hard for the system to determine if the user liked the movie they watched, or they never liked it because they never left any rating. The negative impact is leaving some of the best movies not recommended in the large dataset since they have not been rated by the user. Moreover, the threshold/top-N techniques leave out the best matching suggestions [ 37 ].
- Scalability : One of the challenges cited in the selection of the neighbours was balancing the computational time and the accuracy of the system. The K -means filtering technique is accurate when the database has a small number of movies to recommend or few users [ 38 ]. However, with an increase in the number of users and the number of movies, the computational time or the threshold number of items increases; therefore, the computational time increases [ 39 ]. To overcome this disadvantage, computation and training of the algorithm are performed offline so that when the systems are back online, recommendations are made easily [ 40 ].
The K -means filtering algorithm is the most basic collaborative filtering technique. It is from this technique that other filtering concepts are developed. The computation technique to arrive at the predictions may not be the same; the mode of working mimics the K -means nearest neighbour [ 41 ]. The other algorithms are developed to overcome the K -means clustering limitations.
4.2. Principal Component Analysis K-Means
This is a content-based movie filtering technique that improves on the K -means clustering technique. The major components in the movie are used to classify the movies before recommendations can be made to the customers. The K -means algorithm calculates the closeness of a feature to the centroid using the distance from the mean point. However, the principal component analysis creates a covariance matrix to calculate the eigenvectors and eigenvalues [ 42 ]. Therefore, it widens the scalability to find better comparisons to make the movie suggestions [ 43 ]. To illustrate this, assume a K -means algorithm computes the similarity of a single feature at a time. This implies the computational time and accuracy are compromised. However, using PCA, a covariance matrix of various features is created; hence the scalability is increased and computed faster. If there are similarities that fall within the matrix, they can be found easily, and its eigenvector is computed. Suggestions close to such an eigenvector are then made to recommend the movies [ 44 ].
Steps in Conducting Principal Component Analysis
Structure of the tuples.
Where r i is the movie rating, u i is the user characteristics, and i i is the item characteristics (movie characteristics). A and B will give a 2D matrix of dimension m × n matrix, while C will give a 3D matrix of dimension m × n × o .
- Calculation of the covariance matrix: A covariance matrix of the dimension of the data formulated in the previous step is computed.
- Calculation of the eigenvectors and eigenvalues : The covariance matrix calculated will be a square matrix of the dimension of the data. It is used to compute the eigenvalues and eigenvectors which characterize the data. The computed eigenvectors are sorted in decreasing order according to the eigenvalues; a future vector is constructed [ 45 ].
4.3. Principal Component Analysis Self-Organizing Maps (PCA-SOM)
Self-organizing maps (SOMs) is a technique based on neural networks; it is an unsupervised learning technique, and there is no need for intervention of humans during the learning phase. It is vital in clustering data without knowing the class memberships in the input data [ 46 ]. The self-organizing feature map (SOFM) is known for detecting the features inherent in particular items which is important for the features in the movie recommender systems. SOM also uses topology-preserving mapping, which implies that the algorithm preserves the relative distance between all the points in the initial dataset [ 47 ]. Therefore, it effectively achieves the objective of transforming the arbitrary dimensions into a 1D or 2D discrete map. PCA is integrated with SOM because it is easier for the PCA to convert the matrices generated by SOM to eigenvectors and eigenvalues for ranking in the order of significance [ 48 ]. The steps in working out PCA-SOM are listed below:
- Obtain data without any rankings or classifications;
- Data modelling;
- SOM classified the data using unsupervised learning to bring together that which has similarities in features;
- PCA takes over from the classification achieved by SOM, checks the principal components, and comes up with further classifications of the dataset;
- The decision to make the suggestion.
Initialization: Once the data are obtained, random values are chosen for the weight of the initial vectors. The weights of the vectors represent the neurons in the data, and their values are also computed [ 49 ].
Sampling: A known sample x is drawn from the input space with a known probability. This is the activation pattern that is applied to the lattice. This pattern maps the x dimension to be proportional to the m-pattern in the new lattice [ 49 ].
Similarity Matching: The best matching is found at time step-n using the minimum Euclidean distance between the neuron centroid.
Updating: The synaptic weight of the neurons is adjusted using the formula below:
where η n is the learning rate, h j i x n is the neighbourhood function of i x winner neuron. These two are dynamic to obtain the optimum results.
Apply PCA: After the synaptic weights are derived from the minimum Euclidian distance from the formula above, the PCA process in creating the eigenvalues and eigenvectors is used further in processing the data to obtain a more accurate estimation [ 50 ].
Decision: After similarities are matched, the suggestions are made.
The great features of SOM that make it a good tool in recommender systems are:
Insights into the input space: The method uses unsupervised learning to classify the data by weight vectors and give output in a feature map. The cold starting is significantly reduced [ 51 ]. The user can then input data in light of the initial output features shown.
Topological arrangement: The feature map of SOM works by mapping the field of the input pattern to a spatial location in the output grid [ 52 ].
Density Matching: Once the input is fed into the system, any alterations in input distribution are equally represented in the output grid so that there will be a good representation of the highest density areas with the most matches and lower density areas with fewer matches [ 49 ].
Feature Selection: The SOM algorithm selects the best attributes for the non-linear distribution in the input data so that it can effectively match the similarities to the grids [ 50 ].
4.3.1. Advantages
Since it is based on unsupervised learning, it automatically updates the features and functions [ 53 ]. It is flexible to new input because it learns by itself. It is suitable for unidentified new inputs, for example, new movies that have no ratings or new users where there is no data about them. The new movies may be recommended when the system extracts their features, and the new users will not experience a cold start because they have somewhere to begin on the output feature map [ 54 ]. It is also faster in computation since it easily organizes complex data and makes a good representation of the mapping for easy interpretation.
4.3.2. Disadvantages
The major drawback is that feature classification may not be according to the expected output; therefore, the unsupervised learning classification algorithms have to be initialized often to maintain the relevance of the clustering [ 55 ].
5. Metaheuristic Algorithms for Movie Recommendation Systems
Metaheuristic algorithms are high-level methods or heuristics which have been developed to search, create, or select a heuristic that may produce a satisfactory solution for optimization problems. Metaheuristics find wide usage in almost all aspects of optimization problems. For example, metaheuristics have been used in design optimization [ 56 , 57 , 58 , 59 ], process optimization [ 60 , 61 , 62 , 63 ], structural optimization [ 64 , 65 ], knapsack problems [ 66 , 67 ], workflow scheduling [ 68 ], image segmentation [ 69 , 70 , 71 ], etc.
5.1. Genetic Algorithm
This is a hybrid filtering algorithm that uses the improved K -means clustering and is combined with the genetic algorithm (GA). It uses the PCA technique to partition the high dimensional space into clusters hence reducing the complexity of computations when making intelligent recommendations. The method has higher performance characteristics; hence it makes better recommendations. The steps of the recommendation system are outlined below:
5.1.1. Data Preprocessing Using PCA
The first step is processing the data, extracting it from the original high dimensional space into a linear relatively low space with denser features that carry the information. The PCA feature extraction technique has been very effective. It combines the data represented by the principal component with the highest eigenvalue with the significant information after ranking them. The components with lower significance are ignored but components with higher significance are given prominence. After the linear reduction, only a selected number of components from the rank is fed to the GA-KM algorithm for classification.
5.1.2. Enhanced K -Means Clustering Optimization by Generic Algorithms (GA-KM)
The objective is to make sure that the users/neighbours with like-minded interests or features are grouped. Therefore, it performs it in two stages which are K -means clustering and GA algorithms.
5.1.3. K -Means Clustering
The technique, as discussed, centres its clusters around centroids based on the linear distance from the central feature. The correlation of distance from the central point determines the similarity index. If it is too similar, there is convergence; if there is a high dissimilarity, then the dataset is sparse. As discussed, it suffers a cold start, and its first centroid may be based on the local optimum rather than the global optimum. The steps in K -means clustering are selecting the centroids, assigning objects to the closest clusters, computing the sum of squared distances from the members in the cluster, and checking for convergence in the computed objects. The procedure for computation is similar to that discussed.
5.1.4. Genetic Algorithm
This mimics biological evolution as explained by Darwin’s theory of evolution. The algorithm uses the population of individuals as chromosomes; the chromosomes represent possible solutions to the evolution problem [ 29 ]. Each of the chromosomes contains the genes with the survival ability. Therefore, through natural selection, the chromosomes with the highest quality genes have the highest chance of survival and are fit for reproduction for the next generation. The iterations are based on selection, crossover, and mutation. Selection picks just a proportion of the genes to breed for the next generation. Crossover swaps two parent chromosomes to be recombined into the offspring. Mutation randomly alters the value of a gene to produce offspring. The processes extend the diversity of the offspring. The processes end when the fitness conditions in the environment/context are met.
The GA algorithm is used to prevent premature convergence in the K -means algorithms. The centroids in K -means are considered the chromosomes; the fitness function to evaluate the quality of the solution is:
The fitness value is the sum of the distances of the inner points to the cluster centres. The values are minimized to find the optimal partitions. To find the optimal partitions, the three generic operators precede the construction of the offspring based on the survival fitness principles; convergence occurs when the fitness criterion is satisfied [ 72 ]. The pseudocode of the algorithm is summarized below:
- Initialization
Parameter initialization: Set the maximum iterations, population size, cluster numbers, probability crossover, probability mutation, and fitness function to minimize the total distance of every sample to its nearest centre;
Population initialization: Randomly generate the initial population for each of the k -centers.
Selection operation;
Cross-over operation;
Mutation operations;
Obtain the initial k -centres with optimal fitness values;
K -means optimization: generate new clusters with k -centres.
When tested with the MovieLens dataset, the algorithm has better performance features especially in reducing the cold-start problem [ 29 ].
5.2. Firefly Algorithm
The algorithm is also bio-inspired from the fireflies and combines it with a fuzzy C -means clustering technique. In the natural world, the fireflies are pulled to the brightest firefly using the light signal. Each firefly pulls the other, but the brightest has the highest attractiveness, and other fireflies are clustered around it. Similarly, the algorithm centres its suggestion on features of the users with the highest attractiveness (highest user ratings) [ 55 ]. If a movie has the highest rating from many users, the movie recommender system will make subsequent recommendations based on movies rated highest by users with similar characteristics [ 73 ]. The algorithm for the recommender system is highlighted below:
- All the fireflies are unisexual, and every firefly pulls to another firefly.
- The attractiveness of a firefly depends on its brightness, and the other fireflies will be pulled closer to the brighter one (feature reduction using the firefly algorithm).
- This brightness is related to a primary function in the FCM.
- FCM allocates memberships and utilizes them to show data elements from one cluster to another.
- The FCM separates a finite set of elements X = X 1 … . X n from the memberships into a set of c fuzzy clusters; hence it comes up with a list of cluster centres C = C 1 … C 2 . The partition matrix W = W i 0 , 1 ; i = 1 … n ; j = 1 … c expresses the degree to which each element X i is placed into a cluster C j . The aim is to reduce the objective function to optimal. C a r g ∑ i = 1 C ∑ j = 1 C W i j M X i − C j 2 (6)
- Then the fuzzy C -means clustering f l m m = 1 ∑ K = 1 C x i − c m x i − c k 2 n − 1 (7)
The recommender system efficiency and performance are generally higher than the traditional K -means clustering.
5.3. Artificial Bee Colony
This is a bioinspired algorithm that makes recommendations based on the workings of the bees in finding flowers for the best nectar [ 74 ]. The bees are mainly divided into two groups. Scouting bees go out to scout flowers with the best nectar, and the employee bees follow after the best flowers have been found [ 53 ]. It is worth noting that several scouting bees are sent out and come back with information to the hive regarding the quality of the nectar found. The employee bees will filter out the low-quality nectar from the information and follow the scout bee to the source of the best nectar. Similarly, the artificial bee colony in recommender systems works as an improvement of the K -means clustering algorithm [ 75 ]. In the K -means algorithm, an assumption is made that the data is based on a centroid where the closeness of the feature to the centroid feature determines the recommendation. In an artificial bee colony, there are many centroids (just as there are many flowers), and information from or to these centroids will bring a variety. From this variety, the user may choose what is best suited for them; henceforth, the recommendation system will bring recommendations close to the centroid chosen [ 76 ]. It is a good method to solve the sparsity, scalability and cold-starting problem. The user will choose the best suited feature from the first random set of options available. Subsequent recommendations will depend on the K -means around that particular choice. The steps are summarized below:
- Initialize the system users and movies in a matrix;
- Use the K -means clustering to find several centroids of various product features. This finds several centroids for clustering;
- Selection of the nearest clusters;
- Calculation of the estimated rating values from the user history;
- Use the artificial bee colony to select the closest to user likes based on ratings and features;
- Reclassification of the users for further iterations;
- Coming up with the recommendations.
ABC determines the community of vectors that explore the similarities in the neighbours. The objective function is then continually reduced when narrowing down to the nearest possibilities [ 77 ]. The aim is to minimize the objective function below:
The objective function is controlled by succeeding iterations determined by the detecting vector z → as below:
From the succeeding iterations, the points with the most similar features are selected, and the system recommends the movies to the user [ 78 ]. For instance, an initial allocation of the centroids may be classified as horror movies, thrillers, comedy, or thrillers. If the client selects thriller movies, a further classification may be Hollywood thriller, Bollywood thriller, etc. If the client selects any from these, the subsequent recommendations will be based on this particular centroid [ 74 ]. As seen, it has an advantage because there are always initial centroids that the user can select that further narrow down the selection. Optimization is reduced by the detecting vector to optimize the suggestion to the most viable suggestions [ 79 ].
5.4. Cuckoo Search
The cuckoo search algorithm is a combination of K -means clustering and the use of Levy’s flight function. In this process, the K -means algorithm divides the MovieLens Dataset into different clusters. This is performed using randomly selected centroids [ 14 ]. Measures such as the Euclidian distance and cosines are used to find the distance between centroids, and the features and/or users are reassigned to the closest cluster with similar characteristics.
The cuckoo search algorithm gets its inspiration from the cuckoo bird. The cuckoo bird does not sit on eggs to hatch; rather, it searches for the best nest with optimal conditions and lays eggs for the host bird to sit on to hatch [ 80 ]. If the host bird identifies the egg, it may throw it away or abandon the whole nest. Similarly, in the recommender algorithm, if the centroid does not present the optimal solution, the centroid is abandoned for a new iteration until no re-assignment happens. The pseudocode for the recommender system is outlined in the procedure below:
5.4.1. K -Means Clustering
- Initialize the number of k clusters;
- Random selection of centroids using K -means clustering;
- While no centroid is changed, assign each data point to the closest centroid and calculate the new centroids;
- Assign data points to the closest cluster mean.
5.4.2. Cuckoo Search Algorithm
- Begin the fitness function f x , X i = x 1 , x 2 … ;
- Initialize the random population of n host nests (centroids from K -means);
- Calculate the fitness function value for each nest;
- Find the ith cuckoo randomly by Levy flights, and calculate its fitness, Fi ;
- Select a nest (centroid);
- If ( F j > F i ) ; replace j with the new solution;
- The unqualified nests (centroids) are abandoned and new ones built by Levy flights function;
- The best solutions found are ranked and suggested to the client.
The cuckoo search algorithm has higher precision, recall, and a lower MAE than the PCA- K -means, hence higher performance characteristics. The computations can also be performed offline so that recommendations are made when the system is online, making it faster to make suggestions to the user.
5.5. Grey Wolf Optimizer
This is a recommendation system that is based on mimicking the leadership and hunting tactics of the grey wolfs [ 81 ]. The algorithm first conducts feature selection using the grey wolf optimizer (GWO) method, before clustering using the FCM method [ 82 ]. The algorithm pseudocode is listed below:
- Load the GWO culture;
- Initialize the coefficient points r, Q and R;
- The appropriateness of each explorer is estimated X α X β X δ ;
- Carry out iterations to determine the appropriateness of entire explorer negotiators;
- Return X α representing the position of centroids by GWO;
- Randomly select the cluster centres based on fuzzy means;
- Load the fuzzy clustering formula matrix and estimate f l m m as in the formula below: f l m m = 1 ∑ K = 1 C x i − c m x i − c k 2 n − 1 (10)
- Determine midpoints B (k) = [ c m ] with F (k) ; continue with iterations until ||F( k + 1) − F( k ))) < ε;
- Return to the newly formed cluster centres and make recommendations based on the cluster centres.
This recommender system has a relatively better performance.
5.6. Other Metaheuristic Algorithms
Some researchers have used other metaheuristic algorithms to develop movie recommender systems. For example, Papneja et al. [ 83 ] developed a movie recommendation using a whale optimization algorithm. Tripathi et al. [ 84 ] hybridized a map-reduce-based tournament along with a WOA to achieve a superior recommendation experience.
6. Model Metrics
Various aspects have to be measured apart from the accuracy to make sure that the algorism makes the right predictions. For example, the algorithms may be highly accurate but have too much logarithmic loss. Accuracy is not the only metric to determine the performance efficiency of a model. The metrics are discussed below:
6.1. Mean Absolute Error (MAE)
This is the average difference between the predicted values and the original values. In our case, it is the average difference between the choice of the movie by the customer (user) from the suggestion made (prediction). It gives the variation between the suggestion and what the customer chose. The only disadvantage is it does not give the direction of the error [ 85 ]. Generally, a low mean absolute error is desirable. The mathematical formula for MAE is shown below:
where n s is the number of samples, y ¯ i is the predicted suggestion, and y i is the true feature that the user picks/wants.
6.2. Mean Squared Error (MSE)
This gives the square of the MAE (the square of the average difference between the original values and the predicted values). The advantage is that it makes the large errors more pronounced so that the model focuses on the large errors and their causes [ 86 ]. In addition, it is easier to model the linear programming models in the computation of the slope using the mean absolute error since the differences will be clearer. The formula for the MSE is shown below:
6.3. Log Loss
This is a cross-entropy loss given by probability estimates. It is used in neural networks and recommender system optimizations. It calculates the probability of the suggestions rather than giving only discrete predictions, especially during the ranking of the suggestions [ 35 , 87 ].
6.4. Confusion Matrix
This is one of the most used metrics in determining the accuracy of a model. It is mainly used for classification problems, especially when the outputs expected should have more classifications [ 34 ]. The various characteristics of the confusion matrix are shown in Table 3 .
Characteristics of a confusion matrix.
As noted earlier, the movies are clustered based on the features or the users. In clustering, the true represents the actual classification of the movie, while the predicted gives the predicted classification of the movie before recommendation [ 88 ]. For example, a movie may be classified as a comedy when it is a thriller movie. The user may choose to think it is a comedy because of the characters only to find it is a thriller movie. The movie may be classified as a thriller, and it is a thriller; hence the users get what they want. Such variations happen in the movie classification; hence there is a need for accurate predictions.
True Positive: This points to a case in the recommender system where the actual suggestion was positive, and the client selection of the movie was positive, i.e., the system suggests what the client needed. An example is when the movie is classified as a comedy when the client needed comedy and selects it [ 89 ].
True Negative: This happens when the actual classification is negative, and the prediction is also negative. In our movie recommendation example, if the movie is not a comedy and our algorithm does not classify it as comedy, this output is termed as true negative [ 90 ].
False Positive: This happens when the actual classification is negative, but the system predicts it as positive [ 91 ]. In the movie recommendation system example, the actual case may be the movie is not a comedy; yet the prediction algorithm classifies it as comedy. The actual movie is not a comedy, hence negative, but the prediction is comedy, hence the term positive.
False Negative: This happens when the actual data is true (positive), but the prediction is negative (false) [ 87 ]. The actual classification is true; yet the system predicts it as negative. In the movie recommender systems, the actual movie is a comedy, but the system predicts not comedy.
6.5. Precision
This assesses how many of the true positives are true positives. It gives a fraction of the true positive predictions to the total positive predictions [ 92 ]. The mathematical format is shown below:
6.6. Recall/Sensitivity
This is used as the fraction of true values from the total true values [ 35 ]. In our recommender systems, it gives a fraction of what is classified as comedy out of a total of what is comedy. The fraction of the true values out of the total true values is mathematically modelled as below. Note that false negative is true, but the algorithm classified it as else:
Precision focuses on capturing the classifications correctly, while recall focuses on whether the system is able to capture the features we want though they may not be correctly captured [ 34 ].
6.7. Accuracy
This is the fraction of correct predictions with the total predictions. The accuracy is mathematically modelled as below:
Accuracy as a metric should be used only when the data are balanced and have various classes [ 93 ]. It should not be used as a metric when the data are skewed (have a majority of only one class). For example, if the data are made of 100 movies, and only 5 movies are comedies, the rest are different genres such as thrillers. If the algorithm wrongly predicts all the movies as thrillers, it will be 95% accurate because 95% are thrillers, and only five are comedies. However, from a rational standpoint, the algorithm failed to classify comedy movies. In a recommendation system, the client would think that none of the movies in the datasets is comedy and not watch; yet there are five top-rated comedy movies. Therefore, accuracy should be used when the dataset is well-balanced.
This is simply the harmonic mean of precision and recall. It shows how precise the system was and how it never missed significant instances. If the F 1 score is high, the model performance is high [ 94 ]. The mathematical formula for precision is shown below:
6.9. Computational Time
This is the time that the algorithm takes to come up with the final solution in the prediction. If the systems take long, they may be unreliable if the users want an immediate response before they select the movies to watch. The algorithms should ensure that the best results are found within the shorted period possible [ 53 , 95 ]. A high-performing system returns the most efficient results within a relatively short period. It increases its reliability and dependency. Sometimes the data to be analyzed may be too large to give immediate results. To overcome this limitation, the computations are performed when the system is offline so that the output is shown when it is back online for effective prediction suggestions [ 96 ].
7. Problems Associated with Movie Recommender Systems
7.1. cold start.
The best target audience for a recommendation always depends on the previous user characteristics and the features of the products they watched. A comparison is always made on the characteristics of the user was and the features of the movie and the rating given to the movie. However, in some instances, there are no user characteristics that can be used for a recommendation if the user is new, and nothing is known about them [ 97 ]. Sometimes the user may not be new but has used a different device when accessing the movies’ websites hence there are no stored cookies that can trace the user history.
A cold-start problem occurs when the recommender system is not able to make any suggestions to the user because the user is new or there is no information available about the user [ 98 ]. The problem is common in collaborative filtering which uses only user details to make recommendations of the best movie. The problem is overcome by using content-based filtering, context-based filtering and hybrid filtering. In content-based filtering, the movies are classified by the features such as the main characters, the genre etc. The new user will select any genre based on the content. A context-based filter is based on some of the user information derived from the device such as location, and the operating system, and correlates them with what other users from similar contexts are using. In hybrid filtering, the content, context, and user characteristics are used, therefore, if the recommender system does not have any information about the user, it will use the content and context to make the first recommendations [ 99 ]. The subsequent recommendations will depend on the hints of information available.
7.2. Accuracy
If the database for the recommender systems has few movies, the system will have higher accuracy. If the database is large, there tends to be a lower accuracy because the pool of information searched is too large. To counter the problem, the K -means algorithm reduces the computational time by restricting the computation to a certain number of iterations or selecting only the top-N number of movies for recommendations [ 100 ]. However, if some of the movies have never been rated, they are likely to be biased in the searches [ 101 ].
To increase the system accuracy in making the recommendations, some of the algorithms have employed sophisticated search criteria that will conduct a thorough search and match the product features to user and item characteristics [ 102 ]. In addition, two or more algorithms are combined to allow the perfect user and feature analysis and come up with the desired output. Some of the classification processes such as the PCA-SOM conducts the logarithmic computations offline so that they give recommendations easily when they are online. It reduces the computational time and increases the recommender system accuracy. In modern recommender systems, cold-start problems and the accuracy is solved by having a dialog box where the users can type in the features they need, and recommendations will be given according to what matches the search words [ 103 ].
7.3. Diversity
New movies in the recommender systems rarely appear among those that are suggested to the users. The new excellent movies may end up not being watched because of the lack of being rated by the users. Some of the excellent movies also may not be rated, leaving the recommendation system blank about whether the movies are great for a specific class of watchers or not. To overcome some of these challenges for new movies and movies that are not rated by the users, the diversity aspect is introduced by the recommender systems. In diversification, the new movies or unrated movies are given priority so that they can be noticed by the users [ 104 ]. If they are pleasing, they will be rated and watched more. From this information, the recommender systems will make subsequent decisions on whether to recommend the movie or archive it. From the number of watches, it will also classify the movie according to the features or user characteristics [ 102 ]. Diversity is often used to recommend new debut movies to increase their marketability and presence. It increases the diversity of the user to try out new features or new products.
7.4. Scalability
While sparsity and diversity aim to increase the chances of movies with new features appearing in top searches, scalability aims to solve the problem of increased computational time and increase the performance of the recommender system. Scalability ensures that there is a balance obtained between accuracy and computational time. If it is necessary, some of the classification computations are performed beforehand so that by the time the user comes to select an item to watch, the system makes an almost immediate recommendation with high levels of efficiency [ 105 ].
7.5. Sparsity
Sparsity in the movie data relates to the large volume of movie data in the system, but the users only utilize a few of the features or resources. It is common in K -means clustering where the data is interpolated linearly and gives fewer perspectives to the non-linear data [ 106 ]. Recommendation systems may sometimes be biased by only suggesting the most rated or the most liked movies based on a limited assessment of all the possible cluster features. By using the top-N theory to make the recommendation, those that do not meet the threshold of these can find better algorithms that consider the sparsity of information available [ 107 ]. Some of the methods such as PCA-SOM map all the features on a lattice; hence the user can find most of the features. WOA also widens the scope of the search by using both linear and orthogonal systems to find the desirable features and make recommendations that are sparser and more diverse. Generally, implementing systems that consider non-linearly related data is efficient [ 108 , 109 ].
8. Discussions
The current movie recommendation systems have to work in contexts where there is so much data to be considered before making recommendations. Both user and context information are so varied that the accuracy and precision of the systems are brought to real tests. For example, most of the user information is shared through social media platforms to generate interest in the movies. The MovieLens dataset was created approximately 20 years ago when there was little or no developments in the use of social media where users share movie information to create interest. However, current technologies need to analyze the content, context. and user characteristics in social media platforms to recommend the right movies to the customers. Some companies have taken steps to integrate analytics in their recommender system algorithms. They ask the customer to connect to their social media accounts such as Twitter, YouTube, and Meta not only for advertising but also to analyze the activity of the user on these social media accounts to recommend the best movies for them. Through connecting to these platforms, they analyze the previous history of the user and recommend appropriate movies. This significantly reduces the cold-start problem since new user information can be obtained.
Context-based filtering is gaining traction in the movie recommender systems. It has been adequately used in product recommendations on e-commerce platforms., for example, the most discounted products during black Fridays, the holiday products during Christmas seasons, etc. Movie recommender systems that integrate time stamps to recommend the best movies in various contexts should be studied and developed. For example, it will help recommend movies for children learning during the day and children lullaby movies when it is time to sleep at night.
There are various advances in the use of blockchain technology, and some of these applications may affect the efficacy of algorithms in movie recommender systems. Blockchain technology enhances user privacy through user data encryption; yet collaborative filtering depends on the availability of user information so that it can match the features and characteristics before making recommendations. If user information is concealed by the blockchain systems, the algorithms have to use advanced methods to prevent a decline in the accuracies such as the use of context and content-based filtering.
9. Conclusions
In this article, movie recommender systems have been described and classified. The various types of recommender systems are introduced and discussed. Special emphasis is given to explain in detail the various machine learning and metaheuristic algorithms commonly deployed in movie recommendation research. The various model metrics that summarize the quality of the model are discussed at length. The problems associated with movie recommender systems are also summarized in a structured way and discussed. A total of 77 articles strictly on the area of movie recommender systems are included in the study, and their major conclusions are presented. In addition, 32 other related articles on metaheuristics and recommender systems (not for movies) are also introduced in various sections to present a coherent and meaningful review. One of the limitations of the study is that the Scopus and Web of Science databases were not directly used for selecting the articles for review. In contrast, EBSCO Academic Search Premier, ScienceDirect, IEEE Library, ResearchGate, SpringerLink and the ACM Portal were used for the literature search. Nevertheless, more than 80% of the reviewed papers were found to be indexed in Scopus while more than 60% were available in the Web of Science database.
Funding Statement
This research received no external funding.
Author Contributions
Conceptualization, S.J., N.G. and R.Č.; data curation, S.J., N.G. and J.S.M.; formal analysis, S.J., N.G., R.Č. and J.S.M.; investigation, J.S.M.; methodology, S.J., N.G. and R.Č.; supervision, R.Č.; writing—original draft, S.J., N.G. and J.S.M.; writing—review and editing, R.Č. All authors have read and agreed to the published version of the manuscript.
Institutional Review Board Statement
Informed consent statement, data availability statement, conflicts of interest.
The authors declare no conflict of interest.
Publisher’s Note: MDPI stays neutral with regard to jurisdictional claims in published maps and institutional affiliations.
- Artist Spotlight
- Best New Music
- Short Film Selection
- Thoughts on Film
- MBFW Russia
- Sustainable Fashion
- Watch Spotlight
- Art & Photography
Album Review: Vampire Weekend, ‘Only God Was Above Us’
Charli xcx releases new songs ‘club classics’ and ‘b2b’, finom unveil video for new song ‘as you are’, iron & wine releases new song ‘anyone’s games’, special ops: lioness: daniel richtman reveals it will renewed for a second season, narcos return in 2024: latest news, rumors, & potential new series, review: love lies bleeding, the handmaid’s tale season 6: latest news, rumours & release date, take flight with the 2024 trend: delicate beauty of butterfly jewelry, the best british watch brands, tissot unveils their new pr516 collection, michael kors fall/winter 2024 runway show, types of best casino bonuses in 2024: no deposit, free play & more, blox fruits codes for march 2024: exp boosts, money & reset stats, best valorant crosshair codes used by pros in 2024, 8 books we’re excited to read in april 2024, author spotlight: alexandra tanner, ‘worry’, author spotlight: toby lloyd, ‘fervor’, revealing resistance: the art of protest in claire zou’s mask creations, interview: joe taveras, bridging cultures through art: jiawei fu’s narrative of communication and connection, film research paper topics: tips & ideas to use as inspiration.
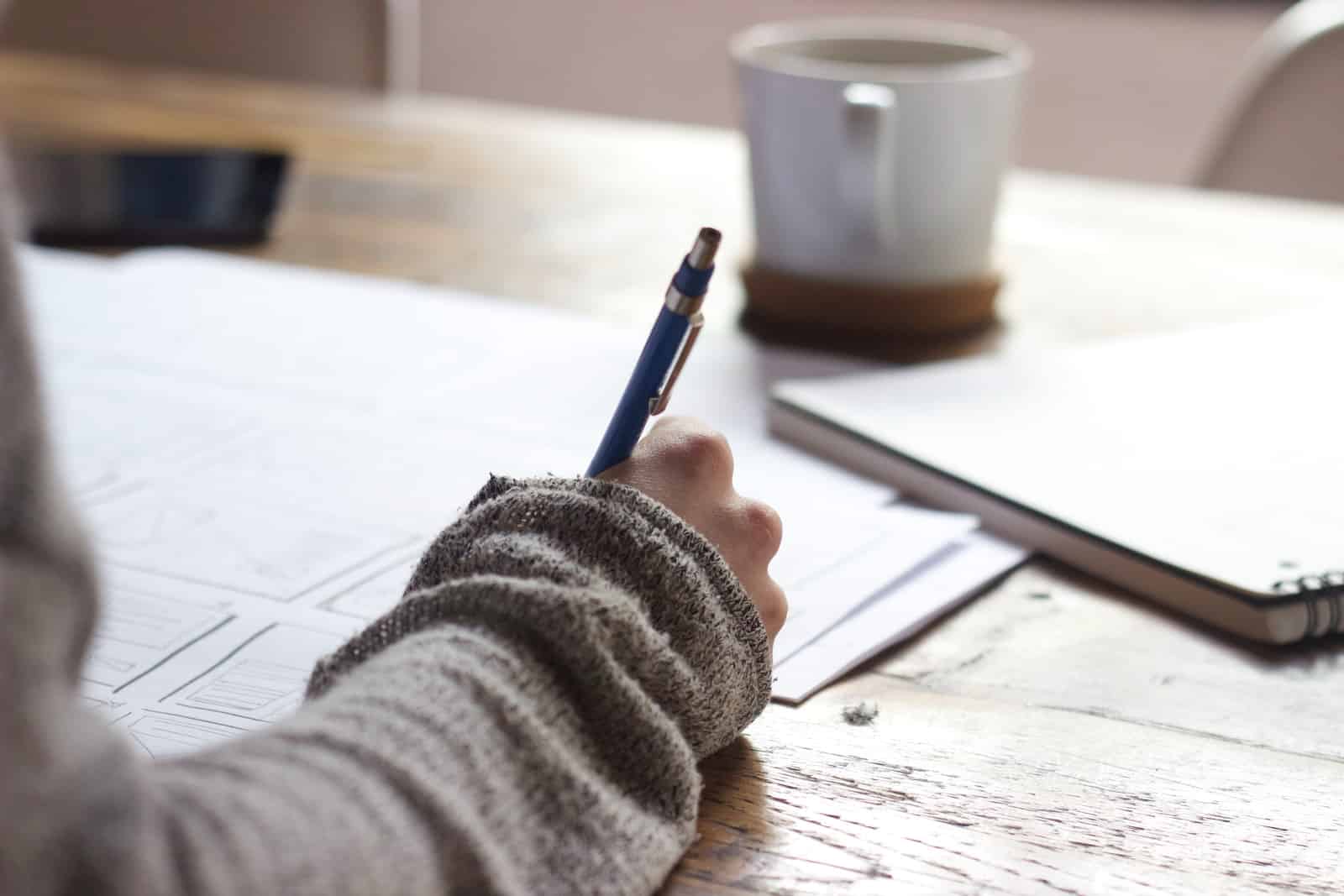
Film making has become part and parcel of current human life. This makes Film Research Paper Topics , Tips & Ideas to Use as Inspiration an affluent area of making money and even highly examinable in the schools. As such, as a veteran or aspiring filmmaker, researcher or student in that field, it is commendable to always have at your fingertips various current ideas to use as inspirations when working on such a topic. Consequently, this article seeks to keep you at par with the various current film research paper topics, tips & and ideas for inspiration. There are various film research paper topics by WriteMyEssays.info that the history of film making and research suggest to be very easy to work with as a beginner or student. Given the vast history of filmmaking, there are so many research topics and below is a list of the most relevant.
If you are searching for an essay writer on film topics you’ll need the best essay writing service company.
Film Research Paper Topics
There are various film research paper topics that the history of film making and research suggest to be very easy to work with as a beginner or student. Given the vast history of filmmaking, there are so many research topics and below is a list of the most relevant.
Science fiction movies
The digital era tends to appreciate science fiction movies so much. This gives this film research topic a wide range of markets, making it almost naturally acceptable. Science fiction movies as a film research paper topic also allow you to structure your script to your liking, provided it has a flow and is understandable.
African-Americans in the American Cinema
Writing about African-Americans has always been a marketable film research paper topic from time immemorial. This is given the diversity in the treatment and reception of African-Americans across the American continent. The success and rise to positions of power and leadership by the African-Americans, the rise in the anti-racism campaigns , the factual existence of people who still consider blacks as slaves and various topics surrounding the African-Americans allow you to structure your script as you so wish.
Women’s role and contribution in the film industry and society in general
Women have drastically evolved from their previous submissive nature into measuring up with their male counterparts in societal duties. Notably, in the film industry included, women have risen to the occasion to take up leadership and other roles in society and other fronts of life. This makes women in themselves a highly marketable film research paper topic.
The evolution of filmmaking
Like every other thing, filmmaking has evolved with technology over the decades. This is an exciting and highly marketable film research paper topic. The human mind tends to appreciate the knowledge of history and evolution of a field that has since become part and parcel of human life.
The Importance of Representation in Movie
The human brain tends to want to understand everything, especially the things that have since become a part of human life, like filmmaking. Consequently, the importance of representation in movies is a topic that tends to spike a debate anywhere across the globe. This makes it a highly marketable film research paper topic.
Tips & Ideas to Use as Inspiration
As a person working in the filmmaking industry, you need to know the tips and amp and ideas and to use them as inspiration when writing a particular film. When choosing an inspiration, it is commendable to go with a topic you are passionate about to make it easily navigable for you. Below is a list of ideas that tend to carry so much inspiration. This site also will help you find out more creative ideas.
- Your background may form a perfect inspiration as you perfectly understand it.
- The culture and heritage of a particular group of people don’t necessarily have to be ethnic.
- Deserted areas tend to be good inspirations as the human mind is naturally curious and would want to know what goes on there.
- Former old film .
- A day in one’s life.
What are the current trends in filmmaking and research? The answers to this question tend to solve a good percentage of your problems in the film industry.
4 Most Iconic Watches Used in Movies
Rare carat: best diamond website, how to write a good english literature essay, sustainable clothes, success stories of famous pin up casino players, arts in one place..
All of our content is free, if you would like to subscribe to our newsletter or even make a small donation, click the button below.
People are Reading
The 10 best films of 2023, interview: thr33som3s, album review: vagabon, ‘sorry i haven’t called’, author spotlight: jem calder, reward system.

Other Popular Stories
UrbanAsian.com
View Profile
For press, you can contact me at [email protected]
Predicting Movie Success Using Regression Techniques
- Conference paper
- First Online: 30 September 2020
- Cite this conference paper
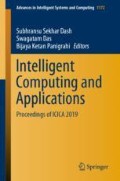
- Faaez Razeen 17 ,
- Sharmila Sankar 17 ,
- W. Aisha Banu 17 &
- Sandhya Magesh 17
Part of the book series: Advances in Intelligent Systems and Computing ((AISC,volume 1172))
1103 Accesses
2 Citations
Hollywood is the largest and most profitable movie industry in the world. In 2018 alone, it generated a massive global box office of over $42 billion. A single production company with multiple movies may benefit greatly from knowing which movies are likely to succeed—it would help them focus their resources on the required advertisement and promotion campaigns. Furthermore, theaters would get a preference on which movies to run for a longer duration based on its success rate. Large-scale investments come with large risks. Using machine learning to predict revenues may help investors mitigate these risks. The algorithms in this paper aim to recognize historical patterns in the movie industry to try and predict the success of upcoming movies using a variety of machine learning algorithms. The success metric used is the box office, i.e., the commercial success of a film in terms of overall money earned. The results show that it is indeed possible to predict revenue with a considerable amount of accuracy, with better results than a majority of the papers that were reviewed.
- Machine learning
- Movie revenue
This is a preview of subscription content, log in via an institution to check access.
Access this chapter
- Available as PDF
- Read on any device
- Instant download
- Own it forever
- Available as EPUB and PDF
- Compact, lightweight edition
- Dispatched in 3 to 5 business days
- Free shipping worldwide - see info
Tax calculation will be finalised at checkout
Purchases are for personal use only
Institutional subscriptions
N. Quader, M. Gani, D. Chaki, M. Ali, A machine learning approach to predict movie box-office success, in 2017 20th International Conference of Computer and Information Technology (ICCIT) (2018) (online). https://doi.org/10.1109/iccitechn.2017.8281839
S. Yoo, R.K. Kanter, D.C. Cummings, A. Maas, Predicting Movie Revenue from IMDb Data (2011)
Google Scholar
N. Vr, M. Pranav, P.B.S. Babu, A. Lijiya, Predicting movie success based on IMDB data. Int. J. Bus. Intell. 03 , 365–368 (2014) (online). https://doi.org/10.20894/ijbi.105.003.002.004
T. Liu, X. Ding, Y. Chen, C. Haochen, M. Guo, Predicting movie Box-office revenues by exploiting large-scale social media content. Multimed. Tools Appl. 75 , 1–20 (2014) (online). https://doi.org/10.1007/s11042-014-2270-1
C. Jernbäcker, P. Shahrivar, Predicting Movie Success Using Machine Learning Techniques (Stockholm, Sweden, 2017)
S. Asur, B. Huberman, Predicting the future with social media, in Proceedings—2010 IEEE/WIC/ACM International Conference on Web Intelligence, WI 2010 , vol. 1 (2010) (online). https://doi.org/10.1109/WI-IAT.2010.63
H. Afzal, Prediction of movies popularity using machine learning techniques. Int. J. Comput. Sci. Netw. Secur. 16 , 127–131 (2016)
L. Pal, L. H. Bui, R. Mody, Predicting Box Office Success: Do Critical Reviews Really Matter?
M. Mestyán, T Yasseri, J. Kertész, Early prediction of movie box office success based on Wikipedia activity big data. PLoS ONE 8 , e71226 (2013) (online). https://doi.org/10.1371/journal.pone.0071226
R.L. Wasserstein, N.A. Lazar, The ASA statement on p -values: context, process, and purpose. Am. Stat. 70 (2), 129–133 (2016) (online). https://doi.org/10.1080/00031305.2016.1154108
F. Pedregosa, G. Varoquaux, A. Gramfort, V. Michel, B. Thirion, O. Grisel, M. Blondel, P. Prettenhofer, R. Weiss, V. Dubourg, J. Vanderplas, A. Passos, D. Cournapeau, M. Brucher, M. Perrot, E. Duchesnay, Scikit-learn: machine learning in Python. J. Mach. Learn. Res. 12 , 2825–2830 (2011)
Download references
Author information
Authors and affiliations.
B.S. Abdur Rahman Crescent Institute of Science and Technology, Chennai, India
Faaez Razeen, Sharmila Sankar, W. Aisha Banu & Sandhya Magesh
You can also search for this author in PubMed Google Scholar
Corresponding author
Correspondence to Faaez Razeen .
Editor information
Editors and affiliations.
Dpt of Electrical and Electronics Engg, Government College of Engineering, Keonjhar, India
Subhransu Sekhar Dash
Electronics and Communication Sciences, Indian Statistical Institute, Kolkata, West Bengal, India
Swagatam Das
Indian Institute of Technology, New Delhi, Delhi, India
Bijaya Ketan Panigrahi
Rights and permissions
Reprints and permissions
Copyright information
© 2021 Springer Nature Singapore Pte Ltd.
About this paper
Cite this paper.
Razeen, F., Sankar, S., Banu, W.A., Magesh, S. (2021). Predicting Movie Success Using Regression Techniques. In: Dash, S.S., Das, S., Panigrahi, B.K. (eds) Intelligent Computing and Applications. Advances in Intelligent Systems and Computing, vol 1172. Springer, Singapore. https://doi.org/10.1007/978-981-15-5566-4_59
Download citation
DOI : https://doi.org/10.1007/978-981-15-5566-4_59
Published : 30 September 2020
Publisher Name : Springer, Singapore
Print ISBN : 978-981-15-5565-7
Online ISBN : 978-981-15-5566-4
eBook Packages : Intelligent Technologies and Robotics Intelligent Technologies and Robotics (R0)
Share this paper
Anyone you share the following link with will be able to read this content:
Sorry, a shareable link is not currently available for this article.
Provided by the Springer Nature SharedIt content-sharing initiative
- Publish with us
Policies and ethics
- Find a journal
- Track your research
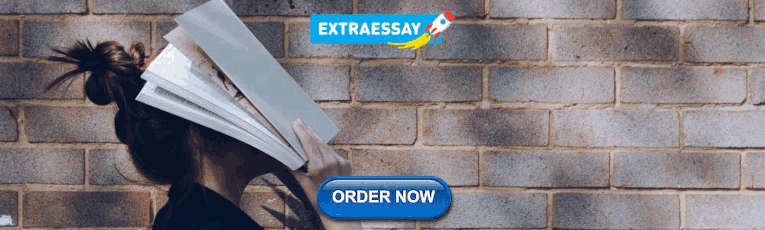
IMAGES
VIDEO
COMMENTS
First, Rudolf Arnheim developed since the 1920s a psychology of artistic film form. Second, although not visible as a coherent psychology of the film, laboratory research on issues in visual ...
Here are some captivating film research paper topics on music. The Evolution of Film Scores: From Silent Cinema to the Digital Age. The Role of Music in Establishing Film Genres. Iconic Film Composers: The Musical Styles of John Williams and Ennio Morricone. The Impact of Jazz on Film Noir Soundtracks.
This research focuses on the potential of pro-social, "humanistic" impact of films and their effectiveness in solving topical social issues. The studies reveal the influence of films on people's beliefs and opinions, stereotypes and attitudes. Movies can have a significant impact on gender and ethnic stereotypes [ 21, 22 ], change attitudes ...
Research the film industry in India. The growing popularity of television. Discuss the most important aspects of film theory. The drawbacks of silent movies. Cameras used in 1950s movies. The most important cinema movie of the 1900s. Research the montage of movies in the 1970s. The inception of film criticism.
Shevy (2007) distinguished two common empirically tested theoretical frameworks focusing on the specifics of music's potential to convey meaning: the Congruence-Association Model (e.g., Cohen, 1993, 2010, 2013) and the cognitive schema theory (e.g., Boltz, 2001).The Congruence-Association Model provides a comprehensive but more general and technical explanation of how the film audience ...
1997. High-Class Moving Pictures: Lyman H. Howe and the Forgotten Era of Traveling Exhibition, 1880-1920: Lyman H. Howe and the Forgotten Era of Traveling Exhibition, 1880-1920. 1991. Hip Hop on Film: Performance Culture, Urban Space, and Genre Transformation in the 1980s. 2013.
This paper investigates the key factors that contribute to the success of movies. By using sentiment and survival analysis, this study classified 1,038 movies according to the customer comments and movie characteristics and compared the number of screening days, the primary measure of success of movies, between the groups. Based on the analysis of film reviews (i.e., positive, negative, and ...
In conclusion, film adaptation is a captivating and complex process that bridges the. realms of literature and cinema. This thesis aims to delve into the artistry and. challenges of adapting ...
In addition, we collected movie character lists from the IMDb (Internet Movie Database) website Footnote 5 and movie subtitles from 15,540 movies. Furthermore, we also used data from Bechdel test ...
With or without cognitive scientists' endorsement, popular films related to cognitive science frequently dramatize our research and often attract curious minds to our discipline. A review of cognitive science student associations and undergraduate clubs finds that nearly all of them host 'movie nights' to entertain and recruit new members.
This section of the Film Studies Research Guide provides assistance in many of the particular subjects in Film Studies. The pages discuss particular issues and list key resources on those topics. You can get to the topical pages from the main navigation bar above or from the links below.
217 Film Research Paper Topics & Ideas. Film research paper topics provide a rich, multifaceted canvas for critical analysis. One can explore genre theory and its evolution, scrutinizing the symbiotic relationship between society and film genres, such as sci-fi, horror, or romance. Another fruitful area lies in auteur theory, assessing the ...
As a research approach, documentary can be categorized within the genre of filmmaking research or screen production research, as it is sometimes known, which is considered a more comprehensive way to acknowledge all forms of audio-visual media and include all stages of production (e.g. screenwriting, editing, visual effects, etc.) (Kerrigan & Batty, 2015).
A Film Review is generally an article that is published in an online or print newspaper, magazine, or scholarly work that describes and evaluates a film. A review often offers an opinion or focuses on making a recommendation.. Film Criticism is generally written by an expert in film studies or film scholar.The criticism often presents the film within a specific context (theoretical, social ...
The paper concludes with a discussion of the methodological implications of studying readings of films seen in the past and stress the need to contextualize those with the wider context of cinema ...
The paper abstracts were read to verify the validity of their information for use in this study. The exclusion criteria were papers that had grey literature on recommendation systems. ... emphasis is given to explain in detail the various machine learning and metaheuristic algorithms commonly deployed in movie recommendation research. The ...
Science fiction movies as a film research paper topic also allow you to structure your script to your liking, provided it has a flow and is understandable. African-Americans in the American Cinema. Writing about African-Americans has always been a marketable film research paper topic from time immemorial. This is given the diversity in the ...
5.1 Movie Rating Prediction. In our research study, we analyse the movie review sentiment using the VADER and TextBlob lexicon. Using both the lexicon, we performed the same process and predicted the movie rating of our selected movies. In Table 6, we have given ten examples of movies.
This paper discusses the prowess CF algorithm and its applications for Movie Recommendation System (MRS). It gives a brief overview of collaborative filtering consisting of two major approaches: user-based approach and Item-based approaches. ... Beel J, Gipp B, Langer S, Breitinger C (2016) Research-paper recommender systems: a literature ...
The present research attempts to extend the applicability of the idea of fit, which was till now largely confined to sponsorship and subjects it to the exploration of finding a fit between brands and specific events, in particular, movies.Because the link between country of origin of the entertainment event (national/international) and brand, placement is a relevant area of speculation, the ...
Movies are an interesting production and new techniques continue to emerge every day. To complete an ideal research paper on movies, the writer must show their understanding of the movies and provide a discussion that is supported by facts. A personal opinion on the movies is also welcomed as it offers a new perspective regarding the movie.
The Hero's Journey Research Paper. 1458 Words6 Pages. The Similarities of Adolescence and Skywalker's Journey The Star Wars Trilogy consists of three movies, A New Hope, The Empire Strikes Back, and The Return of the Jedi, created between 1977 and 1983. George Lucas, the creator of Star Wars, influenced many with his science fiction films, as ...
The algorithms in this paper aim to recognize historical patterns in the movie industry to try and predict the success of upcoming movies using a variety of machine learning algorithms. The success metric used is the box office, i.e., the commercial success of a film in terms of overall money earned.