Emergency and Disaster Management
- Articles, Reports, & Multimedia
- Statistics & Data
- Public Opinion
- Demographics
- APA 7th Edition
- Research Methods This link opens in a new window
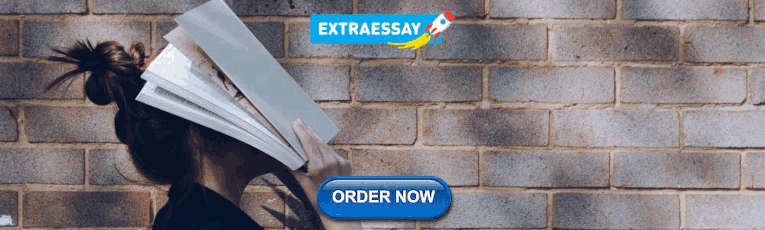
Literature Reviews
Annotated bibliographies, writing the literature review, matrix for organizing sources for literature reviews / annotated bibliographies, sample literature reviews.
A literature review is a synthesis of published information on a particular research topics. The purpose is to map out what is already known about a certain subject, outline methods previously used, prevent duplication of research, and, along these lines, reveal gaps in existing literature to justify the research project.
Unlike an annotated bibliography, a literature review is thus organized around ideas/concepts, not the individual sources themselves. Each of its paragraphs stakes out a position identifying related themes/issues, research design, and conclusions in existing literature.
An annotated bibliography is a bibliography that gives a summary of each article or book. The purpose of annotations is to provide the reader with a summary and an evaluation of the source. Each summary should be a concise exposition of the source's central idea(s) and give the reader a general idea of the source's content.
The purpose of an annotated bibliography is to:
- review the literature of a particular subject;
- demonstrate the quality and depth of reading that you have done;
- exemplify the scope of sources available—such as journals, books, websites and magazine articles;
- highlight sources that may be of interest to other readers and researchers;
- explore and organize sources for further research.
Further Reading:
- Annotated Bibliographies (Purdue OWL)
- How to Prepare an Annotated Bibliography (Cornell University)
" Literature Reviews: An Overview for Graduate Students " 2009. NC State University Libraries
Review the following websites for tips on writing a literature review:
Literature Reviews. The Writing Center, University of North Carolina at Chapel Hill.
Write a Literature Review: Virginia Commonwealth University.
- Matrix for Organizing Sources
Levac, J., Toal-Sullivan, D., & O`Sullivan, T. (2012). Household Emergency Preparedness: A Literature Review. Journal Of Community Health , 37 (3), 725-733. doi:10.1007/s10900-011-9488-x
Geale, S. K. (2012). The ethics of disaster management. Disaster Prevention and Management, 21 (4), 445-462. doi:http://dx.doi.org/10.1108/09653561211256152
- << Previous: Research Methods
- Next: ArcGIS >>
- Last Updated: Jan 26, 2024 10:52 AM
- URL: https://guides.library.georgetown.edu/edm

Investigating resilience in emergency management: An integrative review of literature
Affiliations.
- 1 Industrial and Systems Engineering, Texas A&M University, 101 Bizzel St., Colleg Station, Texas, 77843, USA.
- 2 Industrial and Systems Engineering, Texas A&M University, 101 Bizzel St., Colleg Station, Texas, 77843, USA; Center for Outcomes Research, Houston Methodist Hospital, 6565 Fannin St, Houston, TX, 77030, USA. Electronic address: [email protected].
- 3 Environmental and Occupational Heaelth, Texas A&M University, 212 Adriance Lab Road College Station, TX, 77843, USA.
- PMID: 32501246
- DOI: 10.1016/j.apergo.2020.103114
There is a growing need for resilience in dealing with unexpected events during disasters. The purpose of this review was to summarize and synthesize the literature that examined resilience in the context of emergency management (EM). Four groups of findings were synthesized: definitions, key dimensions, technical tools, and research settings employed in the research. First, definitions of resilience, improvisation, and adaptation were summarized and critically evaluated. Second, four key dimensions of EM resilience were identified: collective sensemaking, team decision making, harmonizing work-as-imagined and work-as-done, and interaction and coordination. Third, this review identified five prevalent technical tools used to enhance resilience in EM: mapmaking, event history logging, mobile communication applications, integrated information management system, and decision support tools. Fourth, two major design features of emergency simulations, incident scenarios and participant roles, are evaluated. For each finding, directions for future research efforts to improve resilience in EM are proposed.
Keywords: Emergency response; Resilience engineering; System safety; Systematic review.
Copyright © 2020 Elsevier Ltd. All rights reserved.
Publication types
- Systematic Review
- Disaster Planning / organization & administration*
- Emergencies / psychology*
- Emergency Medical Services / organization & administration*
- Ergonomics*
- Resilience, Psychological*
- Systems Analysis

Developing a Guide to Emergency Management at State Transportation Agencies (2020)
Chapter: chapter 2 literature review.
Below is the uncorrected machine-read text of this chapter, intended to provide our own search engines and external engines with highly rich, chapter-representative searchable text of each book. Because it is UNCORRECTED material, please consider the following text as a useful but insufficient proxy for the authoritative book pages.
6 CHAPTER 2 LITERATURE REVIEW As part of the Task 2 Literature Review, the research team conducted a focused review of recent domestic and international research on emergency management and emergency response within the all-hazards context. The team sought out recent resources and guidance to identify additional hazards to be considered and understand the impact on emergency response planning, if any, of those additional hazards. Another area of focus was compiling recent research on recovery and its relationship with response. Resilience and sustainability are other areas of focus for this Task. The 2015 Fundamentals report (2015) included resilience principles relevant for emergency management such as improving the ability to improvise during an event and adding redundancies to systems to improve availability and to reroute traffic through one or more parallel components. In particular, the research team sought out information on: ⢠Emergency Management and Federal/State/Local Requirements ⢠Nature and Degree of Hazards ⢠Emergency Management Programs and Planning ⢠Emergency Management Stakeholders and Roles ⢠Regional Communications and Collaboration ⢠Emergency Management Training and Exercises ⢠Resilience and Sustainability ⢠Federal Emergency Management Frameworks and Guidance Sources for the literature review include Google, Google Scholar, and the Transport Research International Documentation (TRID) - composed of Transportation Research Information Services (TRIS) Database and the OECDâs Joint Transport Research Centerâs International Transport Research Documentation (ITRD) Database - as the initial search engines for the online search of relevant research and resources. The team looked at both U.S. and non-U.S. results as part of the literature review. Transportation specific sources included the published research reports from the NCHRP and TCRP programs; Federal Highway Administration (FHWA), Federal Transit Administration (FTA), Volpe National Transportation Systems Center and other federal agencies; ITS America, AASHTO, APTA, and other transportation-related organizations. The literature review included in Appendix A provides a summary of current guidance and resources for emergency management as of the September 2017. Summary key findings are provided below. Key Findings ï· Since the publication of the 2010 Guide, four significant national level directives and executive orders have been issued, each one adding to the nationâs complementary goals pertaining to transportation security, infrastructure protection, system resiliency, and emergency management.
7 o PRESIDENTIAL POLICY DIRECTIVE 8: NATIONAL PREPAREDNESS (2011) strengthens security and resilience through five preparedness mission areas - Prevention, Protection, Mitigation, Response, and Recovery. o PRESIDENTIAL POLICY DIRECTIVE-21: CRITICAL INFRASTRUCTURE SECURITY AND RESILIENCE (2013) focuses on the need for secure critical infrastructure that is able to withstand and rapidly recover (resilient) from all hazards. o 2013 NATIONAL INFRASTRUCTURE PROTECTION PLAN: PARTNERING FOR CRITICAL INFRASTRUCTURE SECURITY AND RESILIENCE emphasizes the importance of resilience, the need to reduce all-hazards vulnerabilities and mitigate potential consequences of incidents or events that do occur. o EXECUTIVE ORDER 13636: IMPROVING CRITICAL INFRASTRUCTURE CYBERSECURITY (2013) provides a technology-neutral cybersecurity framework and means to promote and the adoption of cybersecurity practices. ï· Transportation agencies are in the process of understanding and incorporating the details of these policy directives and are wrestling with their impacts on ongoing security and emergency management functions. For example, in most states today, responsibility for infrastructure security may reside in emergency management or homeland security organizations. Because of this, the perception within some state DOTs may be that security is not a DOT core business function. (Because transit agencies have specific federal security requirements, this is not as great an issue in transit.) However, as NCHRP REPORT 793: INCORPORATING TRANSPORTATION SECURITY AWARENESS INTO ROUTINE STATE DOT OPERATIONS AND TRAINING noted, âThough state DOTs might not be directly responsible for patrolling state- owned infrastructure, DOTs do have the responsibility for controlling access to critical components, establishing coordination with law enforcement to ensure quick response to incidents, conducting infrastructure risk and vulnerability assessments, and taking action to mitigate the effects of those risks and vulnerabilities. As a result state DOTs do play a significant role in infrastructure security.â ï· Today there are even higher expectations for system performance and reliability and lower tolerance for delays. Small events pose threats of great consequences since the impact of any incident is magnified when a transportation network is operating at or past its capacity â as is the case in portions of many states as travel demand on their transportation networks grows. ï· Hazards have continued to evolve since the 2010 Guide was published. In more recent times transportation agencies have been experiencing more and more devastating events either due to natural causes (e.g., Superstorm Sandy, extensive Midwest flooding, powerful hurricanes), caused by unintentional human intervention (e.g., truck crashes and fires on the Oakland Bay Bridge and in the Boston tunnels, oil train derailments) or intentional acts (e.g. cyber attacks and armed assault including active-shooter incidents). Because todayâs transportation systems are integrated cyber and physical systems, there are greater cyber
8 risks than ever, including the risk of a cyber incident impacting not only data, but the control systems and physical infrastructure of transportation agencies. ï· There has been, and continues to be, significant deployment of new resources and rapidly developing technologies to support DOT activities such as ShakeCast, FloodCast and remote, in-situ, or portable monitoring/damage detection techniques employing sensors such as sonar, radar, satellite imagery, and unmanned aerial vehicles. ï· Traffic Incident Management (TIM) provides processes and procedures for responders (firefighters, emergency medical services (EMS), law enforcement, towing and recovery, safety patrols, transportation and maintenance crews, and 911 professionals) to work together as a team to clear incidents safely and quickly. The NIMS integrates best practices into a comprehensive framework for use by emergency management personnel at the local, state, and federal levels. The Incident Command System (ICS) provides the integration of facilities, equipment, personnel, procedures, and communications for emergencies. ï· Transportation agencies play a key role in evacuating people out of harmâs way. Recognizing the unique challenges posed by the disaster environment on mobility and the safe and secure movement of people and goods, the FHWA produced a primer series entitled ROUTES TO EFFECTIVE EVACUATIONS to improve evacuation planning and implementation by bringing to the emergency management community new ways of better using the transportation network before and during evacuations. ï· Two types of post-event assessments are now being conducted: one of structural integrity and another of network resilience. Structural integrity assessment evaluates the degradation state under an extreme event, whereas the resilience assessment evaluates the system or networkâs recovery following extreme events. Structural integrity assessment is well established. Network resilience is a more recent practice. ï· Post-event assessment and recovery has been enhanced by rapidly developing technologies providing digitized data acquisition, storage, and transmission along with structural diagnostics, i.e. monitoring of structures by sensitive instruments measuring temperature, displacement, acceleration, and other significant performance indicators during regular service. ï· A considerable amount of research and practice has been documented on recovery strategies. Thus far this information is very site-specific. Additional work will be required to develop metrics, rules-of-thumb, or other methods to make use of this knowledge for all aspects of transportation systems.
Transportation plays a critical role in emergency management. After all, state transportation agencies have large and distributed workforces, easy access to heavy equipment, and a robust communications infrastructure capable of sustained mobilization.
The TRB National Cooperative Highway Research Program's NCHRP Web-Only Document 267: Developing a Guide to Emergency Management at State Transportation Agencies is a supplemental report to NCHRP Research Report 931: A Guide to Emergency Management at State Transportation Agencies . It details how the guide was developed.
READ FREE ONLINE
Welcome to OpenBook!
You're looking at OpenBook, NAP.edu's online reading room since 1999. Based on feedback from you, our users, we've made some improvements that make it easier than ever to read thousands of publications on our website.
Do you want to take a quick tour of the OpenBook's features?
Show this book's table of contents , where you can jump to any chapter by name.
...or use these buttons to go back to the previous chapter or skip to the next one.
Jump up to the previous page or down to the next one. Also, you can type in a page number and press Enter to go directly to that page in the book.
To search the entire text of this book, type in your search term here and press Enter .
Share a link to this book page on your preferred social network or via email.
View our suggested citation for this chapter.
Ready to take your reading offline? Click here to buy this book in print or download it as a free PDF, if available.
Get Email Updates
Do you enjoy reading reports from the Academies online for free ? Sign up for email notifications and we'll let you know about new publications in your areas of interest when they're released.
Journal of Emergency Management
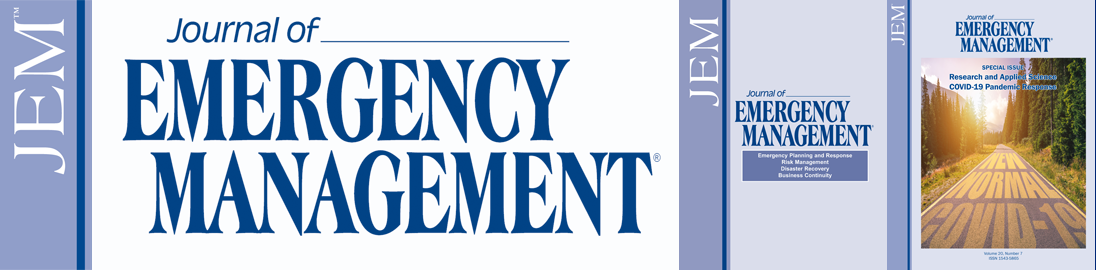
About the Journal
The most respected name in emergency management.
The Journal of Emergency Management is edited, written, and peer-reviewed by an internationally recognized team of the foremost, hands-on EM experts. They include top professionals from the public and private sectors who offer real-world experience and practical solutions and leading academics who provide perspective and analysis on the latest research and studies. Together, they bring you the most thorough, relevant, and useful source of information on emergency management.
The Most Important Thing You Can Do for Yourself and Your EM Team
Published bi-monthly, every issue of the journal of emergency management is peer-reviewed and packed with invaluable information and insight. topics include:.
- Emergency planning and response
- Disaster recovery and business continuity planning
- Emergency preparedness and response legislation
- Risk management
- Emergency management today, tomorrow, and in the future
- Severe weather, flood, and hurricane studies
- Emergency communications
- Continuity of operations and infrastructure protection
- Preparation and evacuation for the disabled
- Cross-training in emergency management
- And much more, including a special focus on EM training and higher education
Current Issue
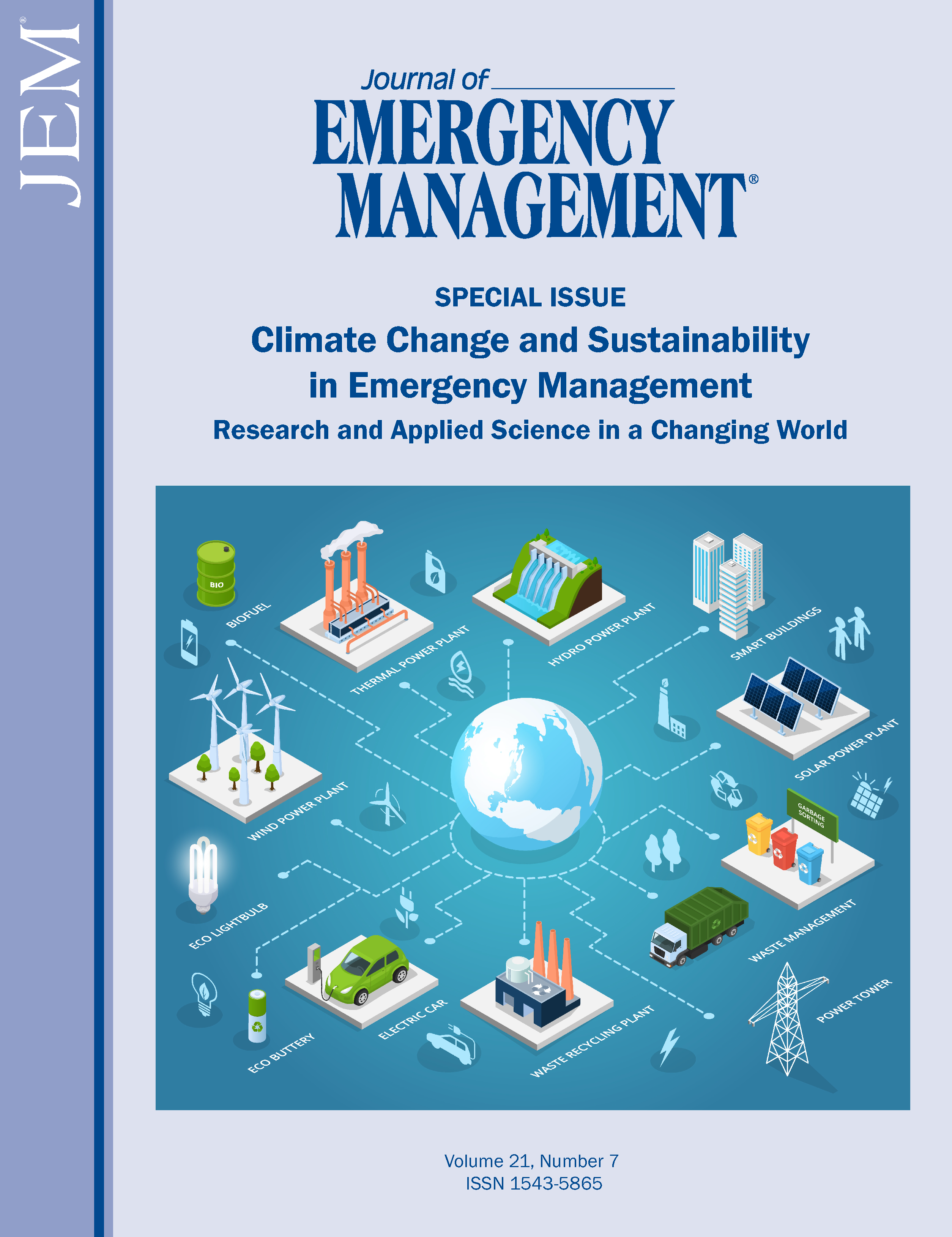
This special issue of the Journal of Emergency Management titled Climate Change and Sustainability in Emergency Management - Research and Applied Science in a Changing World focuses on the intersection of Climate Change, Sustainability, and the Emergency Management profession.
From the publisher
Leveraging technology in emergency management: an opportunity to improve compounding and cascading hazards linked to climate change, emergency management and sustainability: understanding the link between disaster and citizen participation for sustainability efforts and climate change.
- Requires Subscription or Fee PDF (USD 56)
Identifying and assessing corporate employment variables that influence community resilience: A novel model
A hegelian approach to resilient communities, ocean state rising: storm simulation and vulnerability mapping to predict hurricane impacts for rhode island’s critical infrastructure, covid-19 and climate change concerns: matters arising, designing user-centered decision support systems for climate disasters: what information do communities and rescue responders need during floods, manufactured housing communities and climate change: understanding key vulnerabilities and recommendations for emergency managers, rapid assessment of public interest in drought and its likely drivers in south africa, climate change and health: the case of mapping droughts and migration pattern in iran (2011-2016), complete issue, special issue on climate change and sustainability in emergency management, iaem plugged in - 2024.
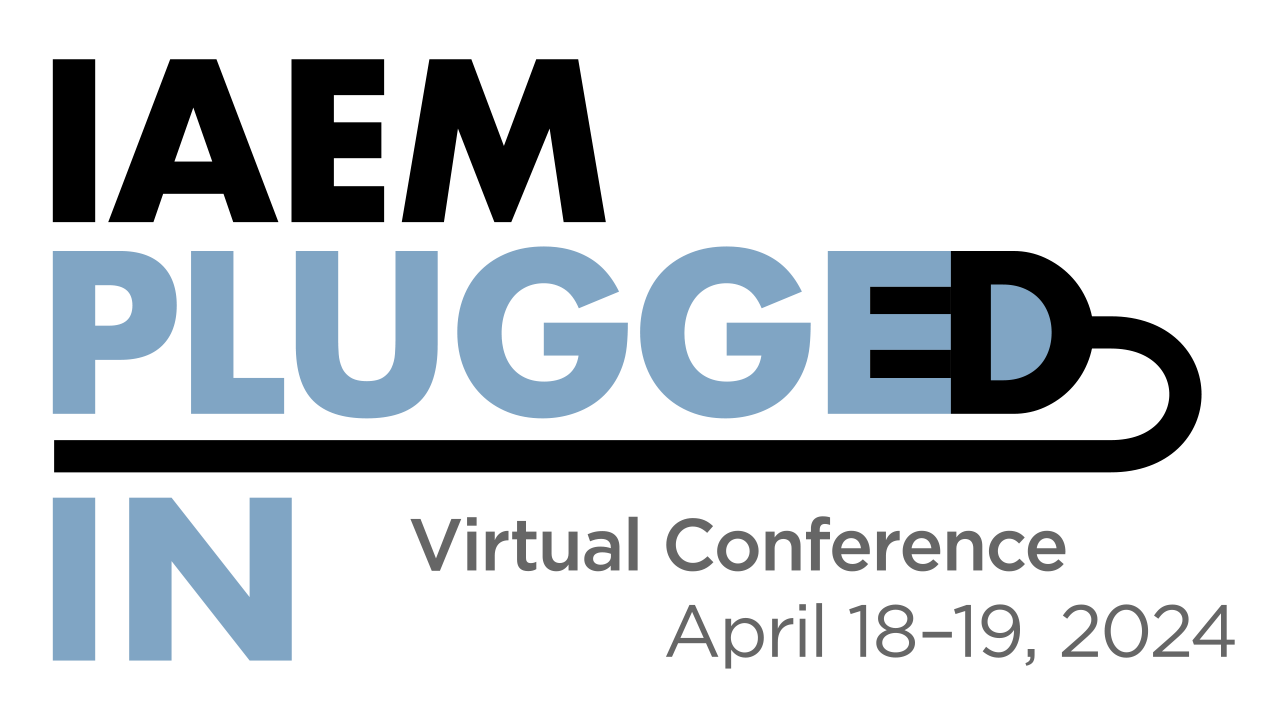
Subscription
Login to access subscriber-only resources.
Advertising Information Editorial Review Board Permissions and Licensing Special Issue - Turkey and Syria Earthquake Feb 2023 Call for Papers - Leaving Nobody Behind: Emergency Management in an Inclusive Way Call for Papers - Climate Change, Sustainability and Emergency Management JEM Stressors and Mental Health Survey Special Issues - COVID-19 Pandemic Response Special Issues - COVID-19 and Mental Health Special Issue - Puerto Rico and Hurricane Maria Webinars - Upcoming and Recorded User License Agreement
Search Scope All fields By Author Title Abstract Index terms Full Text
Information
- For Readers
- For Authors
- For Librarians
- Defining a risk-informed framework for whole-of-government lessons learned: A Canadian perspective 140
- Code of Ethics and Professional Standards of Conduct for Emergency Management Professionals 81
- Emergency management leadership in 2030: Shaping the next generation meta-leader 80
- Assessing the living environment of persons displaced following a strong earthquake sequence in Puerto Rico, 2020 32
- Volume 21, Number 6 27
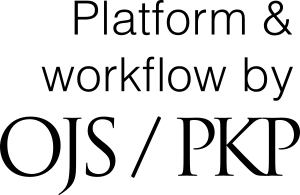
Application of computational intelligence technologies in emergency management: a literature review
- Published: 09 November 2017
- Volume 52 , pages 2131–2168, ( 2019 )
Cite this article
- Ning Chen 1 ,
- Wenjing Liu 2 ,
- Ruizhen Bai 3 &
- An Chen 3 , 4
9805 Accesses
38 Citations
Explore all metrics
Due to the frequently occurring disasters in the world, emergency management is an attractive research area aiming to stabilize the disasters and reduce the potential damage to human, facility and environment. The timely and effective emergency management is highly relied on the utilization of observable information and the integration of available resources. Computational intelligence is one of the fastest growing areas in the field of computer technology. Nowadays, big data has brought ever-increasing impact and challenge to effective data processing and intelligent decision-making. Computation intelligence technologies play a vital role during the lifecycle of emergency management in the context of big data. This review provides a comprehensive survey of state-of-the-art computation intelligence technologies widely applied in the emergency management, and summarizes the present-day emergency management systems in diverse industries. Finally, some promising future research directions and challenges are indicated.
Similar content being viewed by others
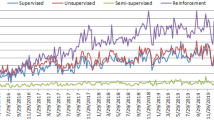
Machine Learning: Algorithms, Real-World Applications and Research Directions
Iqbal H. Sarker
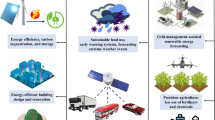
Artificial intelligence-based solutions for climate change: a review
Lin Chen, Zhonghao Chen, … Pow-Seng Yap
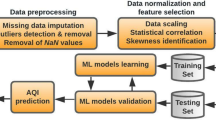
Air pollution prediction with machine learning: a case study of Indian cities
K. Kumar & B. P. Pande
Avoid common mistakes on your manuscript.
1 Introduction to emergency management
In the contemporary society, a variety of disasters take place more and more frequently. Inevitably, a considerable number of emergency events have posed a devastating threat to human life, environmental protection, social stability, and even political relationship of all countries around the world. The negative effects of disasters highlight the need to improve the emergency management capability and strengthen the security for all countries in the world.
Emergency management (EM) is served as a new discipline and there does not exist a unified definition (Ji et al. 2007 ). Some representative definitions in the literature are as follows:
Defined by the Federal Emergency Management Agency (FEMA) of USA, emergency management is the process of preparing for, mitigating, responding to, and recovering from an emergency when a disaster happens (Federal Emergency Management Agency 1999 ).
Emergency management disposes the disastrous events by implementing a serious of activities, including detection, preparation, planning, mitigation, response, and recovery (George et al. 2007 ).
Modern emergency management is a process to apply modern technologies and management methods to effectively monitor, response to, control, and process the emergency events, by integrating various social resources and analyzing scientifically the cause, development process and negative impact of events (Chen et al. 2009 ).
In short, emergency management is a complex and multifaceted task that involves a variety of management activities from managers and stakeholders, so as to prevent the occurrence of unexpected events, to control the social damages, and to eliminate the impacts caused by emergency events.
The frequent occurrence of natural and man-made disasters prompted all countries to continuously improve the emergency management process and mechanism. In the USA (Department of Homeland Security of USA 2017 ), congress approved Emergency Banking Act in 1933, which marked the beginning of emergency management. In 1967, the nation promoted the construction of emergency system. In 1979, FEMA was established as a federal emergency management organization. Later, the 911 terrorist attack and hurricane Katrina urged the US to issue the notable National Incident Management System (NIMS) in 2008.
Japan is one of the countries with massive disasters, especially earthquake, in the world. It has been equipped with specialized emergency management mechanism based on the science and technology in hazard mitigation. In 1993, the International Emergency Management Society (IEMS) was published (Rego 2001 ). In 1990s, the government designed an assessment system to evaluate the emergency management capability of government and provincials. Nowadays, Japan has built a multi-angle, multi-domain and multi-level coordination system from prime minister’s office to various levels of power.
Emergency management in China started lately compared with the developed countries (Chen et al. 2009 ). In the aftermath of the SARS in 2003, China initiated the development of emergency management to cope with all types of disasters, ranging from natural hazards, industrial accidents, epidemics, to terrorist attack. In 2007, China promulgated the Law of Emergency Response, which is the first overall and standardized document of emergency management. Afterwards, more and more special emergency plans have been introduced to meet the practical requirements. In 2015, the Anti-Terrorism Law was issued as a milestone of emergency management for China.
Over the years, unconventional emergency management (Chen et al. 2009 ) has gradually evolved as a focus of research. Unconventional emergency management is aimed to deal with the unexpected, unconventional emergencies (disasters, incidents, hazards) that happened frequently in the world. Unconventional emergency management is characterized by (1) no established rules; (2) a variety of constraints on time, information, and decision; (3) intangible and conflicting criteria. These properties pose significant challenges to intelligent data analysis and decision-support in emergency response. The objective of unconventional emergency decision-making is to make fast and effective decisions with the partial and incomplete information during the emergency response.
The evolution of an incident is composed of three stages in general, namely pre-incident, during-incident, and post-incident. Accordingly, emergency management refers to the process of three phases: (1) detect the early warning signs and predict the occurrence of potential incidents; (2) response to, control, and process the emergency events for the purpose of reducing the negative impact; (3) evaluate the loss caused by incidents and the execution of response, and recover from an emergency. In Chen et al. ( 2009 ), emergency management is described as a ‘4R’ process, namely reduction, readiness, response and recovery, where reduction is referred to pre-incident phase, readiness and response are referred to during-incident phase, and recovery is referred to post-incident phase. In each phase, the outcome of decision-making impacts significantly the evolution of incidents and the effectiveness of emergency management.
With the popularity of Internet, big data has become a challenging problem in the world and therefore brought ever-increasing impact with both benefits and negatives in a wide range of industries (Chen et al. 2012 ; McAfee and Brynjolfsson 2012 ). Big data is characterized by ‘4V’s (i.e., volume, variety, velocity, and veracity) that have brought great difficulties and challenges to traditional data understanding and analysis. Within the present-day emergency management systems, the immediate and accurate decision-making more and more relies on the capability of data analysis and processing especially in the face of big data. Therefore, there is an urgent need to enhance the computational intelligence functionality of emergency management, such as, to develop scalable and real-time algorithms for time-sensitive decisions, to integrate structured, unstructured, and semi-structured data, to deal with the imprecise and uncertain information, to extract dynamic patterns and outline the evolution of these patterns, to work in distributed environment, and to present the multi-scale, multi-level and multi-dimensional patterns through various visualization approaches (Amaye et al. 2016 ).
With the overwhelming increase of data, computational intelligence is regarded as a vital decision-supporting technique in many popular emergency management systems. So far, although both computational intelligence and emergency management have attracted considerable attention in their research areas, little effort Chen and Chen ( 2009 ) was devoted to the systemic literature review of computational intelligence technology application in emergency management. There is an urgent necessity to review the development of current emergency management systems from the computational intelligence view and identify the gap between computational intelligence and emergency management. Our work is deemed for this task contributing to the literature in the following issues. (1) It provides an extensive review of existing computational intelligence technologies broadly applied in emergency management. (2) It demonstrates the roles and functionalities of decision-supporting components embedded in real-world emergency management systems with the consideration of industrial specificity. (3) It analyzes the challenges to computational intelligence in the big data era when establishing an effective and applicable emergency management system, and provides some caveats and guidelines for future research.
The rest of the paper is organized as follows. Section 2 reviews state-of-the-art computational intelligence and related technologies, including decision tree, artificial neural networks, support vector machines, evolutionary computation algorithms, approximate reasoning approaches, association rule mining, case-based reasoning, clustering and visualization. Section 3 discusses the major topics in emergency management with the emphasis on the contribution of computational intelligence. The potential of computational intelligence is extensively addressed in different tasks of emergency management, including risk assessment and early warning evaluation, emergency service facility location, emergency supply allocation and route programming, crowd evacuation in emergencies, emergency response planning, emergency data preprocessing and visualization. In Sect. 4 , we propose a multi-level framework of intelligent emergency management system integrating a variety of sources and functionalities. Some real-world emergency management systems categorized by industries are introduced mainly focusing on the functionalities of computational intelligence technologies. Section 5 concludes the paper and highlights some interesting directions for future research.
2 Literature of computational intelligence technologies
Computational intelligence (CI) is a sub-discipline of artificial intelligence (AI), usually defined as a set of computational methodologies and approaches designed to solve a specific task. The concept of computational intelligence was proposed for the first time in Bezdek ( 1992 ) and later the 1st World Congress on Computational Intelligence (WCCI) was held in 1994. Nowadays, computational intelligence has become a hot and ongoing research subject of computer science and has a wide range of applications in real world. Computational intelligence has potential advantages over traditional modeling methods to address the difficult real-world problems which are characterized by complexity, uncertainty and stochastic process in nature. As an interdisciplinary subject, the methodologies and principles of computational intelligence come from multiple subjects including physics, chemistry, mathematics, biology, psychology, physiology, neuroscience, computer science, etc.
Conventional computing mostly involves the methods implemented manually by a set of programs and data structures, such as databases, word processors, and spreadsheet analysis. In contrast, computational intelligence involves the iterative learning from empirical data and eventually emulating an intelligent response to users. From the perspective of data, the learning can be categorized into supervised learning, unsupervised learning, and semi-supervised learning. Supervised learning is the machine learning task which analyzes the labeled data with desired input and output, and infers a mapping function that can be used on new data. Typical examples of supervised learning include face recognition, medical diagnosis, fault detection, generally producing a prediction in response to a query. The widely studied supervised learning problems are binary classification, multi-class classification, multi-label classification, ranking problems, and real-valued prediction (Jordan and Mitchell 2015 ). Unsupervised learning intends to discover the hidden patterns under specific assumptions about the structural properties of unlabeled data. A diverse array of clustering methods have been developed to detect the structure of clusters embedded in data. In addition, semi-supervised learning that falls between supervised learning and unsupervised learning makes use of unlabeled data along with labeled data in the context of supervised learning.
From a general perspective, computational intelligence tools mainly comprise neural computing, fuzzy logic computing, evolutionary computation, and other related intelligent computing methods. Neural computing typically refers to artificial neural network algorithms which simulate the human intelligence by a network structure of artificial neurons. Fuzzy logic computing imitates the imprecise concepts of human language and thought. Evolutionary computation algorithms that simulate the wisdom of nature include genetic algorithm, swarm intelligence algorithms (ant colony optimization algorithm, particle swarm optimization algorithm, etc.), immune algorithm, simulated annealing algorithm, Tabu search algorithm, and so forth. In addition, some other intelligent computing methods (such as decision tree, association rule mining, clustering and visualization) also have extensive utilization in emergency management. In this article, our efforts are directed to the application of these technologies in different emergency management tasks that cover the lifecycle of emergency and the corresponding management.
2.1 Decision trees
Classification is one of the most important tasks in emergency management, dealing with the likelihood of an incident’s occurrence, quantitative rating of the damage, assessment of emergency actions, and recognition of affected objects. Classification is typically a supervised learning process which learns the patterns that best fit the relation between independent features and target feature (i.e., class label) given a training data set, and then predicts the class of new data whose label is unknown. Among all different classification methods, decision tree (DT) is one of the fastest and easily interpreted algorithms. Decision tree infers a tree-shape structure in which the internal nodes define a test on the value of independent attributes, and the leaf nodes indicate the class of associated instances. It is constructed by recursively selecting the best feature and value that split the data into subsets (corresponding to the tree branches) until the stopping criterion is met. So far, different decision tree algorithms such as ID3, C4.5, CART, Random Forest, ADTree have been proposed differing in feature selection, tree pruning, and data structure to improve the generalization capability and scalability of decision trees. In a post-earthquake emergency building inspection system, an assessment model based on C4.5 decision tree was designed to evaluate the damage and usability of affected buildings (Gerbesioti et al. 2001 ). Decision trees have some advantages: (1) The learning process of decision trees is non-parametric without the requirement of other domain knowledge. (2) Decision trees can deal with both discrete and continuous data. (3) Decision trees have comparable accuracy with neural networks, but the computational cost is much lower. (4) The hierarchical tree model is simple and easily interpreted. Once the tree is constructed, the classification of new samples is operated by a series of test on the independent features. A set of classification rules can be easily derived by combining the tests along the path from the tree root to the leaf nodes. (5) Decision trees are able to discover the dominant variables that determine the target class. For example, Revillaromero et al. ( 2014 ) used random forest decision tree to recognize the potential factors that affected the remote sensing signal of Global Flood Detection System on the analysis of 322 river measurement locations in Africa, Asia, Europe, North America and South America.
2.2 Artificial neural networks
Artificial neural network (ANN) is an information processing paradigm inspired from the biological system to deal with nonlinear complex problems that are difficult for conventional computations. It is composed of a set of highly interconnected processing elements (i.e., artificial neurons) working in parallel for data computation through an adaptive learning approach. Artificial neural networks have different architectures accordingly used in various emergency management tasks. Generally, the ANN family consists of many variants including multi-layer perceptron (MLP), self-organizing map (SOM), learning vector quantization (LVQ) among others.
MLP where the connections between neurons do not form a direct circle is aimed to optimize the connection weights by back-propagation (BP) algorithm that minimizes the outcome error. MLP has remarkable ability to approximate any nonlinear relationship embedded in the real-world data. The prediction models based on ANNs usually demonstrate high accuracy and robustness to noise data. However, artificial neural networks suffer from some weaknesses, such as, explanation difficulty as a black-box algorithm, high computational cost, sensitivity to parameters, convergence to local minima, overfitting to the training data, handling only continuous data. MLP neural network has a wide range of applications in emergency management mostly focusing on prediction and evaluation tasks, such as the risk of incidents, vulnerability of facilities, and effectiveness of emergency response. It was ever used to evaluate the validity of electric power emergency management system (Zhang et al. 2010 ), city emergency management system (Jiang and Li 2012 ), and coal mine safety emergency management (Wen et al. 2013 ).
SOM where the neurons are set along a grid and connected through a neighborhood function is learned in an unsupervised manner. SOM is able to reduce the amount of data and simultaneously project the data onto a lower dimensional array, so that it is usually used for data clustering and visualization while dealing with big data. SOM was used to discover and visualize the spatial and temporal anomalies from the large amount of emergency calls in the Czech Republic (Klement and Snase 2010 ).
LVQ is a supervised variant of SOM designed for data classification. In Abpeykar and Ghatee ( 2014 ), a decision support system was implemented based on both unsupervised learning (including SOM, K-means, and hierarchical clustering) and supervised learning (including LVQ, SVM, and CART decision tree) for intelligent incident management in Tehran Niayesh tunnel.
2.3 Support vector machines
Support vector machines (SVMs) are supervised learning models that define a kernel function able to transform the data to a high-dimensional feature space where the data can be separated by linear models. SVMs search for an optimal hyper-plane that separates the different class samples with the maximal margin. The points closest to the hyper-plane (decision boundary) are called support vectors which in fact are the most difficultly classified samples. SVMs are typically categorized as a type of ANN in the sense they share the same form of model, whilst they differ in the selection of activation function and regularization. SVMs have demonstrated significant generalization performance when the underlying data is nonlinear and non-stationary, and therefore gained wide popularity in solving both regression and classification tasks. SVMs are designed for binary classification in nature, but they can also solve the multi-class classification problems through one-against-one or one-against-all strategy. SVMs were found effective in Emergency Rescue Evacuation Support System (ERESS) to detect the occurrence of a sudden incidence and generate an appropriate evacuation route so as to decrease the human damage in panic-type disasters (Higuchi et al. 2014 ; Mori et al. 2012 ).
2.4 Evolutionary computation algorithms
It is well known that many decision-making problems, such as the allocation optimization and route scheduling of emergency supplies, emergency facility location, and crowd evacuation, can be defined as an optimization solving problem. Evolutionary computation algorithms and recently emerged swarm intelligence methods are the widely employed strategies to solve optimization problems. The most commonly used evolutionary computation algorithms consist of genetic algorithm, Tabu search, simulated annealing, swarm intelligence.
The genetic algorithm (GA), proposed by Holland in the 1970, simulates the evolutionary process of natural selection to find the global solution to an optimization problem. It represents the solution in the form of chromosome string coded in a proper way. The evolutionary process starts from an initial population of solutions, and iteratively improves the solutions to achieve better fitness by selection, crossover and mutation operators. GA has promising advantages of self-organization, self-adaption, self-learning, fault tolerance, and implicit parallelization. It was proved an effective approach to solve the location and allocation optimization problems of emergency facilities (Chuan-Feng and Chao 2009 ; Donmez 2015 ).
Tabu search is a meta-heuristic random search method for mathematical optimization problems. It starts from an initial solution, and searches the neighbors for an improved solution (local search) with some relaxed rules. During the search process, a Tabu table which records the previously visited solutions is employed to avoid the convergence of local optimal solution. In Ren et al. ( 2012 ), a Tabu search heuristic algorithm was developed to solve the dynamic scheduling optimization problem for emergency rescue. In a similar way, simulated annealing (SA) is an improved local search algorithm based on the principle that a bad solution can be accepted with a certain probability so that the search has the opportunity to jump out the local optimal solutions and finally reach the global optimal solution. SA was used in a dynamic optimization model to calculate the optimal allocation of available resources to different operational areas (Fiedrich et al. 2000 ).
Swarm intelligence algorithms inspired by the swarm behavior of insects, birds, and other animals, attempt to find the optimal solution through the collective intelligence of the swarm. Swarm intelligence algorithms are remarkable in robustness, self-organization, distribution, simplicity, scalability, and especially appropriate for solving complex optimization problems in large data environment. In the implementation of multi-agent systems, swarm intelligence algorithms have shown the notable potential in improving the robustness, flexibility and adaptability of systems (Duan 2012 ). At present, the commonly applied swarm intelligence algorithms are ant colony algorithm (ACO), particle swarm optimization (PSO), bacterial foraging optimization (BFO), frog leading algorithm (FLA), artificial bee colony algorithm (ABC). The swarm intelligence algorithms are usually applied in stand-alone manner to optimization problems or combined with the prediction models to improve the prediction accuracy. In many studies, swarm intelligence algorithms are proved particularly practical to solve the multi-objective optimization problems involved in various emergency tasks. Ibri et al. ( 2010 ) combined ACO with Tabu search heuristic algorithm in the hope of improving the dispatching and covering optimization for emergency vehicle fleet management system. Wen et al. ( 2013 ) proposed an ACO algorithm to solve the resource location-allocation problem and route planning problem. Zhang et al. ( 2015 ) used PSO to simulate the individual and crowd movement in a fire and designed the best evacuation mechanism.
2.5 Approximate reasoning approaches
Unconventional emergency management decision making problems are usually characterized by insufficient risk identification, incomplete and inaccurate information, and uncertain decision-making environment to which the classic determinate decision models are no longer feasible (Sun et al. 2013 ). Fuzzy logic, rough set, and Bayesian theory belong to the soft computing and approximate reasoning approaches capable to address the imprecise, inconsistent, incomplete information and knowledge.
Fuzzy sets were introduced by Zadeh in 1965 as an extension of crisp sets. Different from crisp sets where an element belongs to a set definitely, fuzzy sets define an indefinite boundary that elements have a membership degree to the set by a real number between 0 and 1. Application of fuzzy logic can be found in many disciplines, such as computer science, control engineering, decision theory, expert systems. Fuzzy logic is of extreme interest in dealing with the uncertain problems, in particular the intricate process of inter-organizational problem solving. In emergency management, Fuzzy logic can assist decision makers to handle the complex decision making problems in uncertain environments in the form of linguistic concepts and rules (Guo et al. 2014 ). Dellorco has embedded the fuzzy perception and anxiety reasoning in a microscopic model of crowd evacuation able to depict the collective behavior of the crowd (Dellorco 2007 ). To evaluate the physical effects of non-lethal weapons, a fuzzy logic-based crowd injury model was proposed by using linguistic rules properly designed by the problem domain experts (Kugu et al. 2014 ).
Rough set approximates the imprecise concepts with a number of precise concepts. Given an imprecise concept A , the rough set is based on two approximation operators: lower approximation operator indicates the precise concepts contained in A , and upper approximation operator indicates the precise concepts whose intersection with A is non-empty. Rough set has potential in dealing with uncertain concepts, without the necessity to define the membership function as it was in fuzzy logic. The research on rough set theory is mainly focused on feature reduction, rule acquisition, and hybrid prediction model. Sun et al. ( 2013 ) developed a fuzzy rough set model (Dai and Tian 2013 ) to predict the emergency material demand and applied with success in earthquake emergency material demand forecasting. Xi and Sun implemented an urban emergency early warning system by the use of rough set theory to improve the prediction accuracy (Xi and Sun 2013 ). Fuzzy logic and rough set are usually combined with some modeling approaches (for example, a soft CBR model that combined fuzzy logic with case-based reasoning Krupka et al. 2009 ) to handle the vagueness and uncertainty during the knowledge description in emergency management.
Bayesian decision theory is a probability decision paradigm under incomplete information, by estimating the unknown state with subjective probability, modifying the probability by Bayesian rules, and making optimal decisions with respect to the expected value and corrected probability. A Bayesian decision framework for hurricane forecast is intended to address the complex decision making problems (mandatory evacuation, evacuation supplies location, etc.) with respect to an observed tropical cyclone with a tradeoff between the efficiency and accuracy (Taskin and Lodree 2011 ). Bayesian theory has notable benefit to describe the uncertainty of the relationship among decision makers, decision and decision alternatives. It was recently used to derive decision rules with the consideration of dynamic changes of the relation among the three factors. The effectiveness of the decision model was verified in a flood disaster emergency case (Wang and Luo 2015 ).
2.6 Association rule mining
Association rule (AR) mining is intended to discover the interesting correlation among variables in large databases. It was initially introduced to discover the relation among products (items) from the purchase data (transactions) of supermarkets and has extended to various types of databases and application areas. The present-day AR mining algorithms are able to discover binary association rules, quantitative association rules, multi-level association rules, and sequence rules. The strength of an association rule is measured in terms of support and confidence. The former indicates the possibility of the items (item sets) occurring in the transaction. The latter indicates the conditional possibility of some items (referred to the right part of rule) occurring in the transaction in the presence of other items (referred to the left part of rule) in the same transaction. In generally, AR mining consists of two steps: it firstly finds all large item sets which satisfy the support threshold, and then generates the association rules meeting the confidence threshold. In emergency management, AR mining is usually used in risk assessment of a particular incident or exploring the implicit relationship in emergency event analysis (Harms et al. 2004 ). It was applied to explore the spatial relationship between emergency locations and surrounding objects that contribute to emergency resource planning (Fan and Luo 2013 ), as well as the inner relationship of related parameters about drilling accidents (Yue and Xiao 2013 ).
2.7 Case-based reasoning
Case-based reasoning (CBR) is a popular and well researched method based on the concept that previous experience is able to provide suggestions to new problems by recalling the similar historical events and scenarios. The kernel of CBR is K-nearest neighbors (KNN) principle which measures the similarity of a new instance with the past samples using a properly-defined kernel function. CBR system is a ‘4R’ process: (1) Retrieval step searches the case base for the similar past samples; (2) Reuse step adapts the similar samples to the new situation and generates the solution; (3) Revise step validates the effectiveness of new solution in terms of some criteria; (4) Retain step adds the new sample and its solution to the case base if the validation is accepted. Despite the disadvantages (e.g., sensitivity to kernel function, high computational cost, and maintenance overhead of the case base) compared with the rule-based learning paradigm, CBR avoids the complicated model training phase and is applicable to a variety of data types. In emergency management, CBR is the most commonly used approach to generate the emergency response plan under the support of a well-managed plan database. An emergency response plan starts from the risk assessment that identifies the potential emergency scenario, then understands the resource requirements, and finally creates a response plan for all the facilities involved with the full use of available resources. A CBR-based model was realized to offer the rescue recommendations to the commander of a fire protection unit (Krupka et al. 2009 ). The decision is made based on previous cases, namely the guidelines in the fire protection manual, and modified with respect to the real situation and constraints. Later, Huang et al. ( 2012 ) applied natural language process technique that generates imperfect cases from raw information to a practical CBR system for incident reaction and treatment of emergency engineering. In Ma et al. ( 2014 ), CBR was introduced to automatically generate the traffic incident response plan. In this approach, Bayesian theory was adopted to predict the unknown values in order to enhance the accuracy of case retrieval. CBR was also embedded in a decision support system to generate the response and recovery measures to nuclear emergencies (Moehrle and Raskob 2015 ). However, the application of CBR in emergency management is mostly limited to specific disaster types like fire, hurricane, flood, where the domain knowledge could be easily obtained and structured.
2.8 Clustering and visualization
Clustering analysis is an unsupervised process to group the data into a number of homogeneous clusters that have maximal intra-class similarity and minimal inter-class similarity. The intrinsic similarity or distance measure is defined with respect to the data type and investigated problem. Clustering is a very useful approach when there is no pre-classified data. It is usually carried out in the pre-processing phase to generate compressed representatives of raw data so that the deep data analysis becomes easier especially for large, complex data set with many variables and a lot of internal structures. The use of clustering in emergency management mainly focuses on the high quality information acquisition from a mass of raw data with redundancy and noise. There are multiple approaches to clustering, including partitional clustering, hierarchical clustering, density-based clustering, grid clustering, concept clustering, self-organizing map and so on. In additional to the traditional data, social media (such as Twitter, Flickr and YouTube) has become a vital part with increasing popularity in emergency management. Sakai and Tamura ( 2014 ) utilized geotagged tweets to identify the bursty areas of emergency topics using a density-based spatiotemporal clustering algorithm. Clustering was used to generate the situational summaries by making use of the image and video streams from social media and recognize the sub-events that are valuable to emergency management response (Pohl et al. 2016 ).
Visualization offers a visual and interactive representation for users to understand the data. Visualization plays an important role in emergency management, such as to detect the distribution and property of the incident, facility and environment, to monitor the evolution of incidents and the process of emergency response, and to provide a user-friendly interface to emergency experts (Lu et al. 2013 ; Wu et al. 2013 ). When the data is in low dimension (< 3), the commonly used visualization techniques include histogram, box plot, scatter plot, dendrogram, heatmap, plot matrix, hyperbolic tree, parallel coordinates, tree mapping, cobweb, etc. For high dimensional data that are unable to be visualized in a straightforward way, dimensional reduction is an indispensable technique not only for data visualization but also for the subsequent classification or clustering. From the perspective of reduction strategy, dimension reduction can be divided into feature selection and feature construction. The former selects an optimal sub-set from original features by the use of embedding, packaging, or filtering approaches. The latter transforms the high-dimensional original data through a linear or nonlinear projection to a low-dimensional space where the new features are the composition of original ones. The well-known linear data projection methods include principal component analysis (PCA), linear discriminant analysis (LDA), independent component analysis (ICA), singular value decomposition (SVD). Linear methods are able to preserve the linear relation embedded in the data through a simple, fast transformation, but inapplicable to data with complicated and nonlinear structures. Nonlinear methods overcome the linear assumption of data structures and hence effectively explore the real embedded structures and reduce the generalization error of classification. The commonly used nonlinear methods include multidimensional scaling analysis (MDS), kernel mapping, non-negative matrix factorization, manifold learning. Wang et al. ( 2016 ) adopted a kernel mapping dimension reduction method in the pre-processing of big data for emergency management of power system.
3 Emergency management tasks
As a branch of artificial intelligence, computational intelligence technologies have the outstanding advantages of self-learning, self-organization, and self-adaptation, along with simpleness, generality and robustness. These technologies can greatly improve the ability and effectiveness of emergency management. Successful emergency management requires comprehensive emergency planning, preparedness, effective response and recovery. A variety of tasks are involved during the lifecycle of emergency management.
Risk analysis and warning helps the possibly-influenced area and people to defend, evacuate, and eliminate the disaster.
Resource management provides the shelter, food, water, relief, and other technical equipment.
Emergency training and exercising refers to the basic skill of people when they are facing the disaster, incident, hurt, and other mishap suddenness.
Debris removal focuses on the polluted residues that are harmful to the environment and resident.
Temporary housing provides the temporary houses and ancillary facilities for the victims of the hazard.
Emergency service facility location aims to set up a reliable and responsive emergency service network able to satisfy the service demand of the victims.
Supply distribution and route programming concern on the distribution planning and vehicle route programming of diverse emergency materials to the disaster area.
Evacuation planning studies the human behavior and logical patterns, crowd evacuation animation model and decision making after the occurrence of hazard.
Emergency planning management is responsible for implementing the effective planning of emergency response.
Life-support system restoration refers to the recovery and restoration throughout the lifecycle of the disaster.
In this section, we will illustrate some emergency management tasks that are studied extensively in modern emergency management and closely related to intelligent computing and decision making, with the emphasis on the computational intelligence technologies commonly used in each task.
3.1 Risk assessment and early warning evaluation
In emergency management, evaluation is one of critical and complex tasks. From the time dimension of view, emergency evaluation can be categorized into three types (Chen and Chen 2012 ): pre-disaster assessment, in-disaster assessment, and post-disaster assessment as shown in Fig. 1 . Particularly the pre-disaster assessment serves as the basis of disaster prevention and mitigation, mainly to predict the occurrence and severity of potential disasters, dispatch the early warning signs, evaluate the vulnerability of affected facilities, and assess the capacity of emergency management. Among them, risk assessment is an independent and scientific process, generally consisting of three typical steps: (1) hazard identification to recognize what can be likely to go wrong and result in casualties and damage; (2) exposure assessment to describe the likelihood that hazards occur in a qualitative and / or quantitative way; (3) consequence estimation to predict what might be the consequence caused by the hazards and their severity (Jacxsens et al. 2016 ).
Evaluation tasks during the lifecycle of emergency management
Emergency risk refers to the likelihood that an event could occur to a particular community or a society within a given time and inflict the loss of life and property (Chiou and Chen 2015 ). Reasonable assessment of risk and response is critical to suppress the deterioration of emergency. Huang ( 2013 ) provided some measurements of risk analysis and crisis response based on the information collected from Internet. According to different criteria, risks can be divided into various categories as showed in Table 1 .
According to risk severity, risks can be divided into five levels: extremely high, high, moderate, low and very low risks (Gao 2016 ). The category is based on the risk value but varies with the applied domains and the assessment methods. Take Chiou’s approach (Chiou and Chen 2015 ) as an example, the risk value depends on four parameters, namely mitigation, hazard, exposure, and resistance (MHER): \(\hbox {Risk} = (1\,-\,M)\,\times \,H\,\times \,E\,\times \,(1-R)\) , where the values of all parameters are normalized to [0, 1]. The risk severity is then defined as: “Extremely high” ( \(\hbox {Risk} \ge 0.7\) ), “High” ( \(0.7 > \hbox {Risk} \ge 0.5\) ), “Moderate” ( \(0.5 > \hbox {Risk} \ge 0.3\) ), “Low” ( \(0.3 > \hbox {Risk} \ge 0.2\) ), and “Very low” ( \(\hbox {Risk} < 0.2\) ). The assessment results lay foundations for further risk early warning. In other approaches, the warning degree was divided into red, yellow and blue warning (Liu et al. 2017 ) (or red, orange and yellow warning Zhang et al. 2014 ), representing the severity of risk from the high to low level.
The process of emergency assessment methods can be performed in a qualitative or quantitative way depending on the data available and the application. The qualitative assessment methods include Checklist, Delphi, Brain storm, Fault tree, Risk-matrix and so forth. This review focuses on the computational intelligence related assessment methods that typically belong to the quantitative scope. The broadly used assessment methods include decision tree, neural network, support vector machines, Bayesian theory, association rule mining, and so forth.
3.2 Emergency service facility location
Generally, a city is split up into several administrative districts, where a number of emergency service facilities (e.g., ambulances, fire engines, police patrol vehicles) are constructed in order to provide rescue materials to event scene. It should be noted that the emergency service facilities would be taken into operation for a long term once they were built, and the operation cost is highly relevant to the facility location. For the sake of utility maximization, it is of great value to design and optimize the distribution of emergency service facilities, which will directly affect whether the supply of emergency resources may achieve the maximum and optimal effect. Emergency service facility location can be identified as an optimization problem (Mahmud and Indriasari 2009 ) under the consideration of some requirements.
Time urgency: arrive at the affected spot within a given time;
Space full coverage: cover the entire area without rescue blind-spot;
Cost minimization: avoid the redundant sites and reduce the construction investment and operation cost;
Service difference: set up different emergency service sites concerning the diversity in disaster type and intensity;
Coordination efficiency: enhance the coordination among sites and undertake the rescue mission reasonably.
However, these requirements (normally called objectives) are hardly met simultaneously and even conflict with each other in reality. Therefore, only some objectives will be considered in real-world optimization problems. Among them, two optimization problems are commonly investigated: single-objective location-related decision-making problem and multi-objective location-related decision-making problem. In last decades, a lot of efforts have been devoted to constructing optimization models of emergency service facility location. There are four classical location models, namely P-median problem (PMP), P-center problem (PCP), location set covering problem (LSCP), and maximal covering location problem (MCLP). Table 2 compares the four problems in the terms of objective, advantages and disadvantages.
Emergency service facility location is characterized by an optimization problem in nature regardless of the format of models. At present, there are various types of optimization algorithms including linear programming and dynamic programming, local search algorithm, and computational intelligence techniques. Given a location problem, the optimization algorithm is determined concerning the complexity of problem, expected solution effectiveness, processing time limitation, data size and other considerations (Mahmud and Indriasari 2009 ).
Genetic algorithm (GA) is an optimal heuristic method which simulates the biological evolution process in nature. In Guan et al. ( 2013 ), GA algorithm was designed to solve the location-allocation models which satisfy the large-scale emergency requirement. Other applications of GA include subway emergency service facility location problem (Li et al. 2011 ) and risk-based optimization of emergency rescue facility locations (Zhao and Chen 2015 ). Genetic-simulated annealing algorithm (GA-SA) enriches the searching behavior in optimization process, and has strong capability of exploration in large search space (Ma et al. 2012 ). In this algorithm, GA algorithm controls the search direction, while SA algorithm makes contribution to local optimum convergence. Case study showed that the algorithm had strong practicability not only calculating the optimal location results, but also getting the possible affected points that are covered by the selected facilities (Li and Yeh 2005 ; Murray and Church 2004 ).
Swarm intelligence algorithms referring to a collective behavior of decentralized, self-organized systems, consist of a population of simple agents. There exist interactive activities between agent and agent, and between agents and their environments (Hinchey et al. 2007 ; Rubio-Largo et al. 2012 ). Different swarm intelligence algorithms, such as ant colony optimization, particle swarm optimization, artificial bee colony algorithm, bacterial foraging optimization algorithm, glowworm swarm optimization, have been extensively applied to emergency service facility location problem (Aydin and Murat 2013 ; Xu and Xu 2014 ).
3.3 Emergency supply allocation and route programming
In the process of emergency management, appropriate emergency supply allocation (or distribution) is an important and challenging task due to the dispersity of supply sources, limitation of transportation capacity, and uncertainty of supply-and-demand. To decrease the disasters’ related casualties and financial losses, a mass of emergency supplies and timely delivery are needed. However, in reality although all kinds of official and unofficial organizations have reserved an amount of relief materials, emergency supplies are usually insufficient. The study of emergency logistics, particularly the supply distribution and route programming, is always one of the most popular research fields in emergency management (Anaya-Arenas et al. 2014 ). Likewise, Holguín-Veras et al. ( 2012 ) focused on designing the relief distribution network such as the knowledge of demand, the periodicity of logistic activities and supporting system. Some studies from more concreted perspectives analyzed available supplies after disasters (Davis et al. 2013 ) or solved a practical transportation problem encountered by crisis managers in dealing with emergency situations (Berkoune et al. 2012 ). Vitoriano et al. ( 2015 ) proposed two intelligent decision aid models for humanitarian logistics to provide emergency relief.
Emergency supply distribution is intended to allocate all kinds of emergency supplies including food, clothes, tents, medical materials and specialized rescue equipment, to distribution centers in disaster-affected areas (Ozdamar et al. 2004 ; Yi and Ozdamar 2007 ). In emergency logistics, emergency supply distribution and routing programming are two continuous or inter-sectional activities. When the emergency alert is given, the authorities need to allocate all available resources, determine specific vehicles, and program the vehicle route to the destination. The objectives of emergency supply distribution and routing programming always concern the following restrictions: (1) cost minimization; (2) covering maximization; (3) minimization of transportation time between the supply centers and demand centers. Qiang proposed a vehicle scheduling model along with hill climbing method for better route selection and shorter allocation time in emergency logistics (Qiang 2012 ). A hybrid simulated annealing (HSA) with a Tabu list that converges fast to reasonable solutions was developed to solve the HP-hard location and routing scheduling problems (Mousavi and Tavakkoli-Moghaddam 2013 ). Particle swarm optimization and ant colony optimization algorithm have demonstrated the remarkable capacity in emergency supply distribution and route programming (Tian et al. 2011 ; Zhang et al. 2014 ).
3.4 Crowd evacuation in emergencies
When disasters occur, one of the urgent tasks is to organize the evacuation of a large number of population in the affected areas. Without a doubt, it is of practical significance to research on crowd evacuation under emergency conditions. A large quantity of researchers from various fields have investigated crowd evacuation in emergencies and recognized the factors hindering the evacuation (Gu et al. 2016 ; Jafer and Lawler 2016 ). These studies can be categorized into three types: crowd emergency evacuation theory, evacuation risk assessment and evacuation modeling, evacuation decision-making and simulation.
Crowd emergency evacuation theory basically concerns on the parameters associated with pedestrians’ movement, crowd behaviors and pedestrians’ behavior rules in evacuation. Compared with the pedestrians’ behaviors under normal conditions, their behaviors under emergency circumstances are much more chaotic and disordered (Haghani and Sarvi 2016 ).
Evacuation risks are mainly originated from the crowd risk and traffic evacuation risk. When a large crowd is gathered, stampedes are easily caused which bring high risk for crowd safety evacuation. Crowd-gathering risk aims to identify the reason that incidents occur in crowd by analyzing the crowd movement behaviors and individual psychological characters (Haghani and Sarvi 2016 ). Meantime, the road capacity and the number of pedestrians two are critical factors that influence the evacuation effect. For this problem, a cluster model was applied to evacuation risk assessment within the evacuation areas (Chen et al. 2009 ). In Chen et al. ( 2012 ), the researchers developed a critical cluster model (CCM) that integrated both pre-disaster factors (e.g., the vulnerability and accessibility of the road network) and post-disaster factors (e.g. the impact of disaster and evacuees’ behaviors) for evacuation risk assessment and visualization . A large quantity of literature focused on egress and evacuation modeling. Jafer and Lawler divided the evacuation models into five categories, namely random movement models, optimal movement models, directional movement models, patrol movement models, and herd movement models from the perspective of the movement and behavior property of crowd (Jafer and Lawler 2016 ). In another way, evacuation models was partitioned into mathematics models, human behavior and decision making models, software simulation models, integrated virtual crowd simulation models, and human-centered sensing models (Gonzalez et al. 2014 ). The mathematics-based models aim to derive quantitative crowd evacuation rules in emergencies so as to predict the crowd movement state and improve the evacuation strategies. These models can be categorized into micro-models (e.g., social force model, cellular automate) and macro-models (e.g., queue network model, fluid dynamic model). The commonly used optimization algorithms have shown the superiority in constructing optimal micro- and macro- evacuation models. The swarm intelligence algorithms were applied to improve the quality of emergency crowd evacuation (Chen and Lin 2009 ; Forcael et al. 2014 ). Also, simulated annealing algorithm was used as an optimal solution in crowd evacuation especially for predictable crisis (Afandizadeh et al. 2013 ; Sutliff et al. 2011 ).
Emergency crowd evacuation is typically a process that performs the movement of crowd from affected area to safe places. Accordingly, evacuation decision-making includes evacuation path choice, evacuation number allocation and resource allocation. Evacuation path choice is somewhat similar to routing programming that selects the optimal path which usually takes as objective the shortest distance, the shortest time or the most number of evacuation individuals. Once the evacuation paths have been decided, how many individuals should be allocated in every path is the next problem. What’s more, resources (relief staff, emergency evacuation vehicles, etc.) are important guarantee for smooth evacuation. A dynamic model was built by coordinating the logistics and evacuation operations for commodity dispatch and crowd evacuation in disaster response activities (Yi and Ozdamar 2007 ).
3.5 Emergency planning management
Although the disasters cannot be absolutely avoided no matter how advanced risk prediction models are used, there are many approaches to mitigate the consequence of disasters. Among them, emergency planning is of practical significance to avoid the blind and disorder response when the disasters actually occur. Emergency plan is an important preparation that helps government officials to make prompt and efficient emergency response (Wang et al. 2010 ). Emergency planning management is responsible for implementing the planning effectively in terms of clear objectives, comprehensive content, multi-level balance, coordination among various departments, unified and standardized lead tools (Zhou et al. 2010 ). A complete emergency planning consists of four elements: risk scenarios, emergency host organization, emergency resource, and emergency activity (Pilone et al. 2016 ). In China, since General Emergency Plan for National Public Emergency was generated in 2006, a number of emergency plans have been applied in different incidents. In this perspective, emergency plans can be partitioned into general emergency plans and specific plans. General emergency plans provide general procedures, principles and foundation for government in dealing with emergencies, determine the departments or individuals responsible for emergency disposal and define their responsibilities, and ensure the emergency resources. Specific emergency plans further determine the departments and individuals that participant in emergency disposal, as well as the resources required under the framework of general emergency plan. However, it’s worth to notice that emergency plan doesn’t provide the concrete action tasks for real operations because emergency circumstance is usually uncertain (Tian and Li 2012 ). There exists a gap between the generation and operation of an emergency plan so that applying an emergency plan would be more challenging than formulating it (Sun et al. 2014 ).
During the process of developing emergency plans, the typical and major events in history are helpful to generate a more comprehensive emergency plan. Nowadays, CBR is the mostly used approach for generating emergency planning. CBR is a reasoning approach to present solution based on the experience of solving similar problems in the past. Solution of a new problem can be generated by duplicating and modifying similar and successful case solutions existing in the case base. CBR is usually integrated with other modeling methods to achieve a better solution. For example, Li et al. ( 2013 ) utilized a rule-based reasoning (RBR) to boost the nearest neighborhood matching of CBR when generating a new emergency plan.
3.6 Emergency data preprocessing and visualization
In the emergency context, the preprocessing and visualization of data have an important effect on emergency management by well understanding the patterns of emergencies and related data (Amaye et al. 2016 ). Emergency data has various sources from social media, remote sensing, and geographical systems. Generally, they can be divided into six types:
Basic data: all kinds of social, economic and population data related to emergency management, regional dangerous sources, emergency refuge, emergency organizations, distribution of emergency supplies, emergency rescue force, etc.
Spatial information data: physical geographic data (e.g., landforms, rivers, vegetation, mountains lakes), basic geographic units (e.g., residential areas, traffic network, boundary, landmark buildings, airports, docks, stations), etc.
Reported data: monitoring and warning data, loss evaluation data, etc.
Models: risk prediction models, early alerting models, facility location models, route programming models, supply allocation models, crowd evacuation models, etc.
Emergency management knowledge: relevant concepts, theories and experience, etc.
Cases: emergency plans, etc.
The quality of real emergency data is usually not high with an amount of dirty data originating from multiple and disparate data sources (Jia et al. 2016 ). Moreover, there are inevitably redundant, missing, noisy, inconsistent and uncertain data. In order to avoid decision-making faults caused possibly by these data, it’s extremely necessary to preprocess the data before decision-making, including data cleaning, data transformation, data integration to disposal the missing value, delete the mutation data and combine the multi-source data.
Data visualization aims to present data in a graphical present pictorial way so as to depict the relationship embedded in the data intuitively and visually (Brigham 2016 ). The aforementioned data clustering and visualization techniques introduced in Sect. 2.8 are commonly used approaches to obtain more compact and accurate information from the raw data that contribute to the subsequent in-depth data analysis. Image recognition is another well-known technique to discover the potential disasters (e.g., the landslide disasters along railway by using SVM Wei 2013 ), or affected individuals (e.g., face-image classification by using FNN Dai et al. 2015 ) from the images not only saving a lot of human efforts but also improving the recognition accuracy.
In conclusion, computational intelligence has become one of the crucial decision-making techniques in emergency management. In Table 3 , the advanced computational intelligence technologies adopted in different emergency management tasks are summarized from the aspect of principle, advantages, and limitations.
4 Intelligence emergency management systems
Emergency management aims at maintaining the social stability, strengthening the national security and resilience for the threats that pose risk to life and property. Accordingly, the evolution of the emergency management system (EMS) determines the national emergency response capacity and efficiency. Emergency management systems with different functions have been proposed and applied with success in coping with devastating incidents. Emergency management relates to the whole spectrum of incidents, also known as a kind of system engineering. Hence, a complete emergency management system plays a vital role in improving efficiency and effectiveness of emergency management (Lee et al. 2012 ). The modern emergency management system is an intelligent decision support system, able to assist managers in making timely and effective response to emergencies.
Emergency management develops earlier and more mature in America compared with other countries. Department of Homeland Security (DHS) released the National Incident Management System to implement all-hazard emergency management which is applicable at all jurisdictional levels, including preparedness, communication and information system, resource management, command and management, ongoing management and maintenance (Department of Homeland Security of USA 2017 ). All the time, emergency management system has attracted the researches’ attention due to its robustness to cope with complex emergencies. Over the several decades, emergency management systems have become more and more mature in various aspects as shown in Table 4 . In this table, ‘Early’ refers to ‘before the big data era’ where the level of technologies and productivity was still underdeveloped. ‘Modern’ refers to ‘in the big data Era’, where cloud computing, big data and mobile Internet are three major themes with the development of Internet and computational intelligence technologies in the recent decades.
A variety of advanced techniques have been implemented within the emergency management systems. The 3S technologies, namely Remote sensing (RS), Geography information systems (GIS), and Global positioning systems (GPS), possess the function of collecting, processing and updating the spatial information and environmental information rapidly, accurately and reliably. They have been applied in the emergency management, such as disaster rescue management system (Yotsukura and Takahashi 2009 ), forest fire protection (Kalabokidis et al. 2012 ), environmental risk source management (Ma et al. 2013 ), and recovery analysis of damaged highway in earthquake (Liu et al. 2015 ). Some other soft technologies have been used in specific industries. Radio Frequency Identification (RFID) technology is used in intelligent inventory management system to real-time trace (Ozguven and Ozbay 2015 ). It can utilize management techniques to keep track of real-time commodities in the aftermath of a disaster. Furthermore, sensor network attracts the interest of both academic research and government, such as information communication technology, wireless sensor network, and internet of things (Quan and Zhu 2012 ; Zambrano et al. 2016 ).
In the era of big data, computational intelligence technologies are more and more considered vital in a wide range of domains. Inevitably, there is massive unstructured information during the process of emergency handling. Lee et al. ( 2012 ) presented an unstructured information management system (UIMS) to organize useful knowledge quickly and accurately. The UIMS can be implemented as a city emergency management system (CEMS), in which dynamic clustering was used to process the unstructured information. In recent years, cloud computing is provided as a service over the Internet. Palmieri presented a hybrid cloud architecture to compute and storage resources (Palmieria et al. 2016 ). Balis proposed modern environmental monitoring and decision support systems incorporating IT and cloud computing (Balis et al. 2016 ). How to introduce the newly-emerging technologies into the lifecycle of emergency management and realize emergency management system intelligent, real-time and interactive is still an urgent and on-going issue to both academic researchers and industrial practitioners.
4.1 A general framework of emergency management systems
Various emergency management systems and tools have been developed in different industries. Despite the specificity due to the industrial characteristics, they have some generalities. According to the requirement analysis of emergency management and core components of specific emergency management systems, we propose a general framework of intelligent emergency management system in Fig. 2 . It should be noted the real-world emergency management systems may be designed with some components and limited capabilities. The framework consists of data layer, network middleware, application layer and interaction layer. Semantic Web technologies are adopted to harmonize heterogeneous data from databases in data layer via data access and network middleware. Then, the heterogeneous data are processed in application layer. And finally, emergency management decision results are presented through interaction platform. In brief, the framework outlines the procedure of emergency management: data collection (data-layer), data analysis (network middleware and application-layer), and data dissemination (interaction-layer).
The data-layer is in charge of collecting and installing information from sensor devices located in various regions. During the disposing emergency events, information acquisition can determine the effectiveness of intelligent decision-making. For this purpose, the framework has the function of static information storage and dynamic information searching (Chen and Chen 2016 ; Sedighi 2008 ).
The network middleware is a channel of achieving application-layer communication usually composed of wireless sensor networks and local area networks.
The application-layer comprises eight sub-systems, which is the platform for decision support system to analyze, dispose emergency information and make decisions. Among them, prevention and preparation system is responsible for training commanders, making plans, public education and emergency drilling in day-to-day operations. Recover and reconstruction system is in charge of constructing lifeline engineering and providing psychological assistance for affected people after disasters. Legal guarantee system manages the laws, regulations and standards. Decision support system as the heart of an EMS, processes the emergency information to generate decisions for emergency commanders. Incident command system contains multi-agent coordination and resource management system.
The interaction-layer presents a variety of information under the help of visualization technologies. The social media and Web-based platform can communicate the real-time information to the public, and provide decision-making alternatives to the emergency commanders.
The general framework of intelligent emergency management system
4.2 Fire emergency management systems
Among all kinds of natural disasters, fire is one of the most frequent and widespread hazards that threaten major ecosystem and social development. Effective and ideal fire warning is an important task which can discover the potential risks and avoid the occurrence of some events.
In the past, most countries focused on fire suppression, such as Spain in the 1980s and 1990s of the last century (Munoz 2007 ). For example, CBR was applied widely in fire rescue service (Krupka et al. 2009 ) and knowledge management of fire response (Baisakhi et al. 2010 ). Kostas et al. ( 2012 ) developed a decision support system (DSS) using 3S technologies. Zheng et al. ( 2014 ) applied particle swarm optimization algorithm to classify population in fire evacuation. Zia et al. ( 2014 ) proposed a fire emergency management system in the Web-of-Objects (WoO) infrastructure through sensors and actuators. Generally, the existing fire management systems have ability of reviewing fuel behavior and weather conditions. Involving economic analysis in fire management will be a future research direction with practical significance (Robert et al. 2013 ).
4.3 Earthquake emergency management systems
Earthquake is sharp shocking of the earth’s crust within several seconds, which can lead to tremendous losses to lives and property. This disaster has abruptness characteristic and usually results in a chain of evolutional disasters, such as quake aftershocks and tsunami threats (Li and Li 2012 ). The formulation and implementation of emergency plan is indubitably an effective way to deal with the earthquake (Hou and Li 2008 ).
In some developed countries, earthquake early warning systems are applied in view of the time difference between receiving S and P waves (Zollo and Lancieri 2007 ). Tomohiro et al. ( 2011 ) designed an earthquake early warning system (EEWS) and real-time strong motion monitoring system (PSMS) for high-rise buildings. Kangi ( 2015 ) applied clustering technique to prepare the rescue and relief operations in earthquake damage system. A multi-objective stochastic programming model was constructed using particle swarm optimization algorithm for developing earthquake response plan (Mohammadi et al. 2016 ). Munib et al. ( 2016 ) designed prediction and warning systems using Wireless Sensor Networks and Information Communication Technologies (ICT). However, the effectiveness of warnings highly depends on the location of person and whether he (or she) can escape to a safe area. Despite the supporting systems, improving the emergency awareness and response capacity of the public is still an important prerequisite for successful earthquake rescue.
4.4 Environment emergency management systems
Environmental emergency refers to natural or man-made accidents that suddenly take place and cause serious environmental damage, such as water pollution, heavy metal pollution, ecological damage and species crisis. Such events usually occur suddenly and release a considerable number of contaminants within a short period of time.
It has been demonstrated that computational intelligence technologies have a great potential in environmental EMS. The application of CBR is mature in environment management (Chen et al. 2008 ). However, CBR-based systems suffered from the deficiency of cases and difficulty of case adaptation, therefore they were usually integrated with GA (Kerstin et al. 2013 ) and BP-ANN (Kostas and Stamoulis 2007 ; Liao et al. 2012 ) to achieve a better performance. Ma et al. ( 2013 ) developed an environmental risk source management system on GIS platform. Later, Chen et al. ( 2014 ) developed an environmental risk analysis system for petrochemical industry based on a browser/server model and Web-GIS technology. More recently, a two-stage optimization model was presented to identify the material warehouse locations and emergency material reserve schemes in pre-incident stage (Liu et al. 2016 ).
4.5 Transportation emergency management systems
Nowadays, the increasing number of vehicles poses great threats on traffic safety, such as the traffic congestion and illegal driving. Some developed countries have established stable traffic emergency management system and mechanism, such as, Freeway Incident Management System by US (Deng 2000 ), Vehicle Information and Communication System (VICS) by Japan (Rego 2001 ), and Traffic Incident Assistance System (TIAS) by Germany (Feng 2006 ). These systems greatly enhance the traffic safety and achieve abundant experience in transportation emergency management.
Some computational intelligence algorithms, including neural networks (Srinivasan et al. 2004 ), fuzzy logic (Kong and Xue 2006 ), wavelet analysis (Samant and Adeli 2000 ), Bayesian network (Zhang and Taylor 2006 ), hybridization algorithms of ANN and GA (Lee 2010 ), have shown their advantages on high detection accuracy and computational efficiency in transportation emergency management. Regarding the problem of heterogeneous information processing and environmental interaction in traffic accidents, Wang et al. ( 2012 ) developed a Cyber-Physical System (CPS) based on perceptual control theory. Alvear et al. ( 2013 ) applied decision tree to estimate the rescue and evacuation time of the traffic accidents occurred in road tunnels.
4.6 Flood emergency management systems
Flood is a common natural disaster with increasing frequency in the world and usually accompanied by destructive damage. It is of critical importance to establish an efficient flood emergency management system which is scientifically sound and technologically rigorous.
A Web heterogeneous node meta-mode (Chen et al. 2014 ) called Petrochemical Industry Environmental Risk Source Management System (PIERSMS) was developed to evaluate the environmental risk of the petrochemical industry in China. A Web-based decision support system ( www.flire-dss.eu ) Giorgos et al. ( 2016 ) was developed by Greece researchers for dynamic flood monitoring and warning. A chaotic PSO algorithm was designed to flood control operation (He et al. 2014 ). In order to protect against flood in built-up areas, Magdalena et al. ( 2015 ) developed a decision support system for emergency flood embankment stability. A flood controlling system was developed in combination of various algorithms including PSO, fuzzy logic, and GA (Hamed et al. 2016 ). In addition to flood controlling, the study of flood emergency has mostly focused on computer simulation (Liu et al. 2012 ), decision support (Horita et al. 2015 ) and flood vulnerability evaluation (Louis et al. 2016 ).
4.7 Nuclear emergency management systems
Nuclear accidents are caused by large nuclear facilities, which may result in radiation damage and radioactive contamination, even pollute the surrounding environment over a long period of time. For instance, the Chernobyl disaster in 1986 has been lingering until today. Radiological accidents are considered a major threat that requires effective prevention and rapid reaction (Benamrane and Boustras 2015 ).
An interactive computer support in decision conferencing was an early attempt for quick response to offsite emergency (Jyri et al. 2007 ). Later, a nuclear radiation release prediction system was implemented in nuclear emergency management (Benamrane et al. 2013 ). CBR was extensively applied in nuclear emergency management for creating response plan (Farah et al. 2011 ; Stella and Wolfgang 2015 ). Zhang et al. ( 2014 ) applied GA-SA algorithm to source term inversion of nuclear accident. Zhao et al. ( 2014 ) analyzed the accident scenarios by accident diagnosis model based on BP-ANN. Recently, Xie et al. ( 2016 ) developed a framework of the cross-domain integration processes using Space Mapping and Semantic Web. For Fukushima accident shocking nuclear management, Mahdi and Mohammad ( 2016 ) proposed a modern Accident Management Support Tool (AMST) that adopted ANN, fuzzy logic, heuristic methods and expert system.
4.8 Hurricane emergency management systems
Hurricanes are deep tropical cyclones, generally accompanied by strong wind and heavy rain. Timely forecast and evacuations before hurricane are fundamental to reduce the damage. Some representative hurricane emergency management systems have emerged in the past. Selda and Emmett ( 2010 ) presented inventory decisions for emergency supplies based on hurricane prediction. Yin et al. ( 2014 ) developed an agent-based modeling system for travel demand simulation. Taramelli et al. ( 2015 ) proposed a GIS-based approach for hurricane hazard and vulnerability assessment, able to judge the hazard (type, magnitude), identify the risk elements (type, location, data features), and assess the vulnerability. Gu et al. ( 2010 ) forecasted the tropical cyclone intensity based on PSO in conjunction with SVM. Dong and Pi ( 2014 ) established hurricane trajectory prediction model by association rules.
4.9 Integrated emergency management systems
The integrated emergency management systems are characterized by multi-agency, multi-objective, multi-layer and comprehensive aspects. They are usually designed as inter-departmental, cross-regional and cross-industrial taking into consideration the connection among disasters and coordination among departments. The most typical system is National Integrated Management System of USA, which can take response to both natural and man-made disasters coordinating individuals and federal government. The city emergency response systems aim to integrate the public security, transportation, communications, electric power, water conservancy, earthquake, civil air defense, municipal administration and other governmental departments into a unified operation system in order to achieve cross-regional, inter-departmental, and cross-industrial management to disasters. In China, a number of cities (e.g., Beijing, Shanghai, Nanning, Chongqing, Weihai, Liuzhou) have established the city emergency response systems which provide the guarantee for the public safety of the city (Pei et al. 2010 ; Wan and Song 2012 ). In Australia, some scholars have put forward a mobile-based emergency response system for intelligent m-government services with the attempt to enhance the interaction between multiple agencies in emergency situations (Amailef and Lu 2011 ). In United States, many cities such as San Francisco, Portland and Seattle have built comprehensive public security geographic information systems and emergency resource systems to achieve scientific allocation of personnel, equipment, supplies (Zhong 2011 ). In Japan, a cross-department and comprehensive urban crisis management institution has been established under the leadership of the mayor. For example, Tokyo set up a comprehensive disaster prevention department against various types of crisis in 2003 (Jin 2015 ). In England, a city risk management system based on the comprehensive risk registration was established in London, and a syndromic surveillance system was used to detect a bioterrorism attack in Scotland (Meyer et al. 2008 ).
In conclusion, computational intelligence technologies can discover the potential value of data by realizing the intelligent disposal of unexpected events. It is commonly believed that the CI-based emergency management can systematically help the commanders to identify the risk sources and provide response measures, especially under the circumstance of limited time, enormous pressure and information asymmetry. Table 5 summarizes the representative emergency management systems in specific industries with the focus on the adopted computational intelligence technologies and primary functions.
5 Conclusions and future remarks
Emergency management has become a major and growing discipline in the world. As an important innovation and development element in the global society, big data has a significant influence on emergency management (Wu et al. 2016 ). Under the background of big data era, the acquired data should be well utilized to meet the requirement of the timely and effective emergency management. Computational intelligence technologies able to deal with big data are intended to make the full use of all acquired data, construct more accurate models, so as to take the timely and effective emergency response, and eventually decrease the damage and stabilize the incidents. Over the recent years, it has been observed an increasing interest of integrating a diversity of computational intelligence technologies in emergency management for diverse tasks and industries. However, effective decision support still requires the in-depth integration of newly-emerging computational intelligence techniques. This paper presents a comprehensive survey of the state-of-the-art computational intelligence techniques commonly applied in the literature of emergency management considering the challenges of big data. In this research survey, we reviewed more than 170 papers published in scientific referred journals and related international conferences putting the emphasis on the computation intelligence technologies integrated in emergency management theory and systems. The computational intelligence techniques are discussed from the perspective of emergency management tasks and industries. It can be revealed that computational intelligence has become one of the critical aspects to develop an intelligent emergency management system especially in big data era.
There are some critical open issues necessary to be addressed. (1) The growth of unconventional incidents today highlights the ability to handle incomplete information, incompatible criteria under pressures and uncertainties. Although the importance of emergency management has been broadly recognized, applicable methods in the emergency management process still cannot fill the requirements. The research of unconventional emergency decision-making is ongoing to bring computational intelligence technologies and other related disciplines together. (2) The explosive growth of acquirable information provides a rich repository for evidence-based decision-making, whilst at the same time makes the decision-making more difficult and complicated. The traditional methods usually become infeasible when dealing with large-scale, high-dimensional, sparse, and incomplete data. The applicable techniques able to process the big data are of strong and urgent necessity for providing critical decision support in emergency management. For example, the scalability of decision-making algorithms is in particular important when the real-time response is of great value. The recently emerged research techniques designed for big data analysis (such as deep learning algorithms characterized by semi-supervised feature learning, and transfer of learning algorithms that utilize the knowledge learned in one context to another similar context) and the adaptation of these techniques specifically for emergency management are considered crucial issues in future research. One major concern of this research review is to promote the theoretical results of computational intelligence being applied to practical emergency management. On the other hand, computational intelligence is still a young and rapidly expanding field with new invented methods over the past two decades. Emergency management undoubtedly has a substantial impact on the development of computational intelligence driven by some practical problems across a range of emergency industries. (3) Emergency management is an active and developing research area due to the fact that it has broad applications closely related to the human safety. Although a variety of industry-oriented emergency management systems have been developed continuously, they share some general characteristics, such as the mechanism of incidents. A framework to describe the inherent mechanisms of different harmful incidents and clarify the universality and particularity of the evolving logic is required for successful approaches to emergency management. (4) It was found that a disaster may derive other types of disaster making the response more complicated and difficult. It is necessary to investigate the relation among disasters, and integrate all emergency sources in disaster responding. In this sense, the integrated emergency management system is a research direction of practical value for both academic and industry.
Abpeykar S, Ghatee M (2014) Supervised and unsupervised learning DSS for incident management in intelligent tunnel: a case study in Tehran Niayesh tunnel. Tunn Undergr Space Technol 42(11):293–306
Google Scholar
Afandizadeh S, Jahangiri A, Kalantari N (2013) Identifying the optimal configuration of one-way and two-way streets for contraflow operation during an emergency evacuation. Nat Hazards 69(3):1315–1133
Alvear D, Abreu O, Cuesta A et al (2013) Decision support system for emergency management: road tunnels. Tunnel Undergr Space Technol 34:13–21
Amailef K, Lu J (2011) A mobile-based emergency response system for intelligent M-government services. J Enterpr Inf Manag 24(4):338–358
Amaye A, Neville K, Pope A (2016) BigPromises: using organisational mindfulness to integrate big data in emergency management decision making. J Decis Syst 25(Suppl 1):76–84
Anaya-Arenas AM, Renaud J, Ruiz A (2014) Relief distribution networks: a systematic review. Ann Oper Res 223(1):53–79
MathSciNet MATH Google Scholar
Aydin N, Murat A (2013) A swarm intelligence based sample average approximation algorithm for the capacitated reliable facility location problem. Int J Prod Econ 145(1):173–183
Baisakhi C, Ghosh D, Maji RK et al (2010) Knowledge management with case-based reasoning applied on fire emergency handling. IEEE international conference on industrial informatics. IEEE Press, pp 708–713
Balis B, Brzoza R, Bubak M et al (2016) Holistic approach to management of IT infrastructure for environmental monitoring and decision support systems with urgent computing capabilities. Future Gener Comput Syst. https://doi.org/10.1016/j.future.2016.08.007
Basu S, Sharma M, Ghosh PS (2015) Metaheuristic applications on discrete facility location problems: a survey. Opsearch 52(3):530–561
Benamrane Y, Boustras G (2015) Atmospheric dispersion and impact modeling systems: how are they perceived as support tools for nuclear crises management? Saf Sci 71:48–55
Benamrane Y, Wybo JL, Armand P (2013) Chernobyl and Fukushima nuclear accidents: what has changed in the use of atmospheric dispersion modeling? J Environ Radioact 126:239–252
Berkoune D, Renaud J, Rekik M, Ruiz A (2012) Transportation in disaster response operations. Socio-Econ Plan Sci 46(1):23–32
Bezdek JC (1992) On the relationship between neural networks, pattern recognition and intelligence. Int J Approx Reason 6(2):85–107
Brigham TJ (2016) Feast for the eyes: an introduction to data visualization. Alexis Med Ref Serv Q 35(2):215–223
Chen N, Chen A (2012) Emergency evaluation model and method: a perspective overview. J Saf Crisis Manag 2(1):11–23
Chen L, Chen A (2016) Improve the efficiency of improvisation decision of emergency management-analysis on the explosion event of hazardous chemical products in Tianjin. Theor Explor 1:80–84
Chen Y, Lin Y (2009) Controlling the movement of crowds in computer graphics by using the mechanism of particle swarm optimization. Appl Soft Comput 9:1170–1176
Chen SH, Jakeman AJ, Norton JP (2008) Artificial intelligence techniques: an introduction to their use for modelling environmental systems. Math Comput Simul 78:379–400
Chen A, Chen N, Ni H (2009) Modern emergency management theory and method. Science Press, Beijing, pp 1–6
Chen Q, Chen X, Tang Q et al (2009) An evacuation risk assessment model for emergency traffic with consideration of urban hazard installations. Chin Sci Bull 54:1000–1006
Chen H, Chiang R, Storey VC (2012) Business intelligence and analytics: from big data to big impact. MIS Q 36(4):1165–1188
Chen X, Kwan MP, Li Q (2012) A model for evacuation risk assessment with consideration of pre- and post-disaster factors. Comput Environ Urban Syst 36(3):207–217
Chen NC, Wang K, Xiao CJ et al (2014) A heterogeneous sensor Web node meta-model for the management of a flood monitoring system. Environ Model Softw 54:222–237
Chen Q, Jia Q, Yuan ZW et al (2014) Environmental risk source management system for the petrochemical industry. Process Saf Environ Prot 92:251–260
Chen N, Chen A (2009) The role of data mining techniques in emergency management. In: Proceedings of 11th international conference on enterprise information systems (ICEIS09), 6–10 May 2009, Milan, Italy, pp 118–123
Chiou I, Chen C et al (2015) Methodology of disaster risk assessment for debris flows in a river basin. Stoch Env Res Risk Assess 29:775–792
Chuan-Feng H, Chao Z (2009) Genetic algorithm for solving problems in emergency management. In: International conference on natural computation, pp 259–264
Dai J, Tian H (2013) Fuzzy rough set model for set-valued data. Fuzzy Sets Syst 229:54–68
Dai K, Zhao J, Cao F (2015) A novel algorithm of extended neural networks for image recognition. Eng Appl Artif Intell 42:57–66
Davis LB, Samanlioglu F, Qu X, Root S (2013) Inventory planning and coordination in disaster relief efforts. Int J Prod Econ 141:561–573
Dellorco M (2007) Competitive egress behaviour: a fuzzy logic-inspired microscopic model. Int J Crit Infrastruct 3:408–429
Deng ZY (2000) The new trend of American highway traffic safety. J Foreign Highw 1:9–13
Department of Homeland Security of USA (2017) National Incident Management System, Federal Emergency Management System. https://www.dhs.gov/ . Accessed 17 Oct 2017
Dong X, Pi D (2014) Hurricane trajectory prediction method with pattern matching. J Chin Comput Syst 35(5):983–988
Donmez S (2015) A genetic algorithm based approach to provide solutions for emergency aid stations location problem and a case study for Pendik/İstanbul. J Homel Secur Emerg Manag 130(4):915–940
Duan JH (2012) Recent development of application of swarm intelligence in multi-agent system. Comput Sci 39(6):6–9
Fan B, Luo J (2013) Spatially enabled emergency event analysis using a multi-level association rule mining method. Nat Hazards 67(2):239–260
Farah J, Henriet J, Broggio D et al (2011) Development of a new CBR-based platform for human contamination emergency situations. Radiat Prot Dosimetry 144:564–570
Federal Emergency Management Agency (1999) Federal Response Plan (FRP)
Feng Q (2006) Enlightenment from management system of emergency response in Germany. City Disaster Reduct 2:17–19
Fiedrich F, Gehbauer F, Rickers U (2000) Optimized resource allocation for emergency response after earthquake disasters. Saf Sci 35(1–3):41–57
Forcael E, Gonzalez V, Orozco F et al (2014) Ant colony optimization model for Tsunamis evacuation routes. Comput-Aided Civil Infrastruct Eng 29(10):723–737
Gao J (2016) Analysis and assessment of the risk of snow and freezing disaster in China. Int J Disaster Risk Reduct 19:334–340
George H, Coppola DP, Bullock J (2007) Introduction to emergency management. Butterworth-Heinemann, Oxford, pp 2–5
Gerbesioti A, Delis V, Theodoridis Y (2001) Developing decision support tools for confronting seismic hazards. In: Proceeding of the 8th Panhellenic conference on informatics, 8–10 Nov 2001, Nicosia, Cyprus, pp 247–253
Giorgos K, Dimitris P, Nektarios C et al (2016) A web-based DSS for the management of floods and wildfires (FLIRE) in urban and periurban areas. Environ Model Softw 86:111–115
Gonzalez JJ, Radianti J, Granmo OC, Bouhmala N, Sarshar P (2014) Comparing different crowd emergency evacuation models based on human centered sensing criteria. Int J Inf Syst Crisis Response Manag 6(3):53–70
Gu J, Liu H, Sun Y (2010) Tropical cyclone intensity forecast experiment based on combination of particle swarm optimization and support vector machine. Meteorol Disaster Reduct Res 33(3):22–26
Gu Z, Liu Z, Shiwakoti N, Yang M (2016) Video-based analysis of school students’ emergency evacuation behavior in earthquakes. Int J Disaster Risk Reduct 18:1–11
Guan X, Ge C, Wang X et al (2013) Models and algorithms for hub and spoke locations for emergency service facilities in response to serious emergency incidents. Hum Ecol Risk Asses 19:553–565
Guo E, Zhang J, Ren X et al (2014) Integrated risk assessment of flood disaster based on improved set pair analysis and the variable fuzzy set theory in central Liaoning Province. China. Nat Hazards 74(2):947–965
Haghani M, Sarvi M (2016) Human exit choice in crowded built environments: investigating underlying behavioural differences between normal egress and emergency evacuations. Fire Saf J 85:1–9
Hamed ZS, Delbert H, Shalamu A et al (2016) Optimization of adaptive fuzzy logic controller using novel combined evolutionary algorithms, and its application in Diez Lagos flood controlling system, Southern New Mexico. Expert Syst Appl 43:154–164
Harms SK, Goddard S, Tadesse T, Goddard S, Waltman WJ (2004) Data mining in a geospatial decision support system for drought risk management. In: Proceedings of national conference on digital government research, pp 9–16
He Y, Xu Q, Yang S et al (2014) Reservoir flood control operation based on chaotic particle swarm optimization algorithm. Appl Math Model 38:4480–4492
Higuchi H, Fujimura J, Nakamura T et al (2014) Disaster detection by statistics and SVM for emergency rescue evacuation support system. In: 43rd international conference on parallel processing workshops (ICCPW), 9–12 September 2014, pp 349–354
Hinchey MG, Sterritt R, Rouff C (2007) Swarms and swarm intelligence. Computer 40(4):111–113
Holguín-Veras J, Jaller M, Wassenhove L et al (2012) On the unique features of post-disaster humanitarian logistics. J Oper Manag 30(7–8):494–506
Horita F, Albuquerque J, Degrossi LC et al (2015) Development of a spatial decision system for flood risk management Brazil that combines volunteered geographic information with wireless sensor networks. Comput Geosci 80:84–94
Hou JS, Li M (2008) The process of earthquake disaster emergency management in China. Recent Dev World Seismol 1:14–20
Huang C (2013) The measurement of effective knowledge in multiple Internet of intelligences and application in risk assessment. In: 4th international conference on risk analysis and crisis response, Istanbul, Turkey, 27–29 August 2013, Huang C, Kahraman C (eds) Intelligent systems and decision making for risk analysis and crisis response, 1832(10). CRC Press, pp 1–8
Huang C, Shen S, Huang Q (2012) An Approach of information processing for CBR in emergency management engineering. Syst Eng Proc 5:185–190
Ibri S, Drias H, Nourelfath M (2010) A parallel hybrid Ant-Tabu algorithm for integrated emergency vehicle dispatching and covering problem. Int J Innov Comput Appl 2(4):226–236
Jacxsens L, Uyttendaele M, Meulenaer BD (2016) Challenges in risk assessment: quantitative risk assessment. Proc Food Sci 6:23–30
Jafer S, Lawler R (2016) Emergency crowd evacuation modeling and simulation framework with cellular discrete event systems. Simulation 92(8):795–817
Ji L, Chi H, Chen A et al (2007) Emergency management. Higher Education Press, Beijing, pp 8–12
Jia C, Wang H, Wei L (2016) Research on visualization of multi-dimensional real-time traffic data stream based on cloud computing. Proc Eng 137:709–718
Jiang DM, Li ZB (2012) The evaluation of city emergency management system results based on BP neural network. Appl Mech Mater 263–266:3288–3291
Jiang T, Ren G, Zhao X (2013) Evacuation route optimization based on Tabu search algorithm and hill-climbing algorithm. Proc Soc Behav Sci 96(1):865–872
Jin LT (2015) Comparison of emergency management of public security incidents in mega cities abroad. J Shenyang Off 17(4):51–53
MathSciNet Google Scholar
Jordan MI, Mitchell TM (2015) Machine learning: trends, perspectives, and prospects. Science 349(6245):255–260
Jun Tian, Zhi Li (2012) Emergency tasks planning based on formal modeling of emergency plan and HTN planning system SHOP2. Intell Inf Manag 4:357–363
Jyri M, Raimo PH, Kari S (2007) Interactive computer support in decision conferencing: two cases on off-site nuclear emergency management. Decis Support Syst 42:2247–2260
Kalabokidis K, Xanthopoulos G, Moor P et al (2012) Decision support system for forest fire protection in the Euro-Mediterranean region. Eur J Forest Res 13(131):597–608
Kangi A (2015) Application of warning and rapid estimation of earthquake damage (WARED) system in unprepared cities cases study: Mashhad, north east of Iran. Int J Disaster Risk Reduct 13:139–144
Kerstin W, Ana C, Tomas M (2013) Parameter calibration framework for environmental emergency models. Simul Model Pract Theory 31:10–21
Klement P, Snase V (2010) SOM neural network—a piece of intelligence in disaster management. In: World congress on nature and biologically inspired computing, pp 872–877
Kong Y, Xue Anke (2006) Urban traffic incident detection based on fuzzy logic. In: IEEE conference on industrial electronics (IECON), IEEE, pp 772–775
Kostas DK, Stamoulis K (2007) Air pollution modelling with the aid of computational intelligence methods in Thessaloniki, Greece. Simul Model Pract Theory 15:1310–1319
Kostas K, Gavriil X, Peter M et al (2012) Decision support system for forest fire protection in the Euro-Mediterranean region. Eur J Forest Res 131(3):597–608
Krupka J, Kasparova M, Jirava P (2009) Case-based reasoning model in process of emergency management. Man-machine interactions. Springer, Berlin, Heidelberg, pp 77–84
Kugu E, Li J, Mckenzie FD et al (2014) Fuzzy logic approach and sensitivity analysis for agent-based crowd injury modeling. Simul Trans Soc Model Simul Int 1(1):1–18
Lee Y (2010) A computerized feature selection method using genetic algorithms to forecast freeway accident duration times. Comput-Aided Civil Infrastruct Eng 25:132–148
Lee WB, Wang Y, Wang WM et al (2012) An unstructured information management system (UIMS) for emergency management. Expert Syst Appl 39:12743–12758
Li Z (2011) Fuzzy clustering analysis of emergency rescue route. In: 2nd international conference on artificial intelligence, management science and electronic commerce (AIMSEC), pp 1148–1151
Li YJ, Li Z (2012) Great East Japan earthquake emergency evolution and contingency decision based on system engineering approach. Syst Eng Proc 5:254–259
Li X, Yeh AG (2005) Integration of genetic algorithms and GIS for optimal location search. Int J Geogr Inf Sci 19(5):581–601
Li Y, Li X, Wu Q, Chen L (2013) Emergency plan generating method based on CBR and RBR. Ordnance Ind Autom 32(5):31–35
Liao ZL, Mao XW, Phillip MH et al (2012) Adaptation methodology of CBR for environmental emergency preparedness system based on an Improved Genetic Algorithm. Expert Syst Appl 39:7029–7040
Liao ZL, Wang B, Xia XW et al (2012) Environmental emergency decision support system based on artificial neural network. Saf Sci 50:150–163
Liu YZ, Zhang WT, Cui XM (2012) Flood emergency management using hydrodynamic modelling. Proc Eng 28:750–753
Liu J, Liang G, Jiang JP et al (2016) A two-stage optimization model for emergency material reserve layout planning under uncertainty in response to environmental accidents. J Hazard Mater 310:30–39
Liu M, Wei J, Wang G, Wang F (2017) Water resources stress assessment and risk early warning–a case of Hebei Province China. Ecol Ind 73:358–368
Liu X, Chen A, Chi F et al (2015) Recoverability of broken highways in earthquake based-on remote sensing technology. In: Focus on China, pp 30–37
Li M, Wang Y, Sun C (2011) Research on the location allocation model for subway emergency service facilities under network operating conditions. In: Third Pacific-Asia conference on circuits, communications and system (PACCS). IEEE, pp 1–6
Louis GZ, Charles TS, Mustafa SA et al (2016) Flood vulnerability indices and emergency management planning in the Yazoo Basin, Mississippi. Int J Disaster Risk Reduct 18:89–99
Lu L, Zhang H (2014) A route planning method for micro UAV based on improved genetic algorithm. Appl Mech Mater 602–605:1348–1351
Lu J, Yang M, Zhang HX et al (2013) Power grid ice melting decision method based on rough set theory and principal component analysis. J Electr Power Sci Technol 28(3):90–95
Ma C, Li Y, He R, Qi B, Diao A (2012) Research on location problem of emergency service facilities based on genetic-simulated annealing algorithm. Int J Wirel Mobile Comput 5:206–211
Ma S, Zhang S, Chen Y et al (2013) Design and realization of a major environmental risk source management system. Proc Environ Sci 18:372–376
Ma Y, Zhang W, Lu J et al (2014) Automated generation of traffic incident response plan based on case-based reasoning and Bayesian theory. Discrete Dyn Nat Soc 6:1–7
Magdalena H, Michal L, Monika C et al (2015) A decision support system for emergency flood embankment stability. Proc Comput Sci 51:2957–2961
Mahdi S, Mohammad BG (2016) Accident management support tools in nuclear power plants: a post-Fukushima review. Prog Nucl Energy 92:1–14
Mahmud AR, Indriasari V (2009) Facility location models development to maximize total service area. Theor Empir Res Urban Manag 4(1):87–100
McAfee A, Brynjolfsson E (2012) Big data: the management revolution. Harvard Bus Rev 90(10):60–68
Meyer N, McMenamin J, Robertson C, Donaghy M, Allardice G, Cooper D (2008) A multi-data source surveillance system to detect a bioterrorism attack during the G8 summit in Scotland. Epidem Infect 136(7):876–885
Moehrle S, Raskob W (2015) Structuring and reusing knowledge from historical events for supporting nuclear emergency and remediation management. Eng Appl Artif Intell 46(PB):303–311
Mohammadi R, Ghomi S, Jolai F (2016) Prepositioning emergency earthquake response supplies: a new multi-objective particle swarm optimization algorithm. Appl Math Model 40:5183–5199
Mori K, Tsudaka K, Wada T et al (2012) Development of emergency rescue evacuation support system (ERESS) in Panic-type disasters: disaster detection algorithm by Buffering-SVM. Ieice technical report information networks 111:65–70
Mousavi SM, Tavakkoli-Moghaddam R (2013) A hybrid simulated annealing algorithm for location and routing scheduling problems with cross-docking in the supply chain. J Manuf Syst 32(2):335–347
Munib R, Soliha R, Seema M et al (2016) Implementation of ICT and wireless sensor networks for earthquake alert and disaster management in earthquake prone areas. Proc Comput Sci 85:92–99
Munoz RV (2007) Experiences in Spain of community based fire management. In: 4th international wildland fire conference, Sevilla
Murray AT, Church RL (2004) Applying simulated annealing to location-planning models. J Heurist 2(1):31–53
Ozdamar L, Ekinci E, Kucukyazici B (2004) Emergency logistics planning in natural disasters. Ann Oper Res 129(1):217–245
Ozguven EE, Ozbay K (2015) An RFID-based inventory management framework for emergency relief operations. Transp Res Part C 57:166–187
Palmieria F, Ficcob M, Pardi S et al (2016) A cloud-based architecture for emergency management and first responders localization in smart city environments. Comput Electr Eng 56:810–830
Pei YS, Zhang KJ, Liu XG (2010) Environmental risk management with the aid of city emergency response system in Nanning City. Proc Environ Sci 2(6):2012–2019
Pilone E, Mussini P, Demichela M, Camuncoli G (2016) Municipal emergency plans in Italy: requirements and drawbacks. Saf Sci 85:163–170
Pohl D, Bouchachia A, Hellwagner H (2016) Online indexing and clustering of social media data for emergency management. Neurocomputing 172(C):168–179
Qiang X (2012) Vehicle scheduling model for emergency logistics distribution with improved genetic algorithm. Int J Adv Comput Technol 4(18):315–323
Quan DU, Zhu SB (2012) Research on urban public safety emergency management early warning system based on technologies for the internet of things. Proc Eng 45:748–754
Rego AJ (2001) National disaster management information systems and networks: an Asian overview. In: Global disaster information network conference (GDIN)
Ren SK, Yuan ZP, Yu XU (2012) Dynamic scheduling optimization for emergency rescue: taking KX blowout accident as an example. Oper Res Manag Sci 21(3):1–7
Revillaromero B, Thielen J, Salamon P (2014) Evaluation of the satellite-based global flood detection system for measuring river discharge: influence of local factors. Hydrol Earth Syst Sci 18(11):4467–4484
Robert M, Armando GC, Elsa V (2013) The state of development of fire management decision support systems in America and Europe. For Policy Econ 29:45–55
Rubio-Largo A, Vega-Rodriguez MA, Gomez-Pulido JA et al (2012) A comparative study on multi-objective swarm intelligence for the routing and wavelength assignment problem. IEEE Trans Syst Man Cybern-Part C Appl Rev 42(6):1644–1655
Sakai T, Tamura K (2014) Identifying bursty areas of emergency topics in geotagged tweets using density-based spatio-temporal clustering algorithm. In: IEEE international workshop on computational intelligence and applications, pp 95–100
Samant A, Adeli H (2000) Feature extraction for traffic incident detection using wavelet transform and linear discriminant analysis. Comput-Aided Civil Infrastruct Eng 15:241–250
Sedighi M (2008) Use of geographical information system (GIS) in the cataloging of documents–a case study of earthquake documents collections. Emerald Insight 26:454–465
Selda T, Emmett JL (2010) Inventory decisions for emergency supplies based on hurricane count predictions. Int J Prod Econ 126:66–75
Simon TW, Zhu Y, Dourson ML, Beck NB (2016) Bayesian methods for uncertainty factor application for derivation of reference values. Regul Toxicol Pharmacol 80:9–24
Srinivasan D, Jin X, Cheu RL (2004) Evaluation of adaptive neural network models for freeway incident detection. IEEE Trans Intell Transp Syst 5(1):1–11
Stella M, Wolfgang R (2015) Structuring and reusing knowledge from historical events for supporting nuclear emergency and remediation management. Eng Appl Artif Intell 46:303–311
Sun B, Ma W, Zhao H (2013) A fuzzy rough set approach to emergency material demand prediction over two universes. Appl Math Model 37(10–11):7062–7070
Sun HY, Rao LL, Zhou K, Li S (2014) Formulating an emergency plan based on expectation-maximization is one thing, but applying it to a single case is another. J Risk Res 17(7):785–814
Sutliff WD, Dulaney AD, Davis WL (2011) The optimization of traffic signal timing for emergency evacuation using the simulated annealing algorithm. Transport 26(2):133–140
Taramelli A, Valentini E, Sterlacchini S (2015) A GIS-based approach for hurricane hazard and vulnerability assessment in the Cayman Islands. Ocean Coast Manag 108:116–130
Taskin S, Lodree EJ (2011) A Bayesian decision model with hurricane forecast updates for emergency supplies inventory management. J Oper Res Soc 62(6):1098–1108
Tian J, Ma WZ, Wang YL, Wang KL (2011) Emergency supplies distributing and vehicle routes programming based on particle swarm optimization. Syst Eng Theory Pract 31(5):898–906
Tomohiro K, Yoshiaki H, Masahiro M et al (2011) Application of an earthquake early warning system and a real-time strong motion monitoring system in emergency response in a high-rise building. Soil Dyn Earthq Eng 31:231–239
Villa V, Paltrinieri N, Khan F, Cozzani V (2016) Towards dynamic risk analysis: a review of the risk assessment approach and its limitations in the chemical process industry. Saf Sci 89:77–93
Vitoriano B, Rodríguez JT, Tirado G, Martíncampo FJ, Ortuño MT, Montero J (2015) Intelligent decision-making models for disaster management. Hum Ecol Risk Assess 21(5):1341–1360
Wan JJ, Song YH (2012) Evaluation of the city integrated emergency response system based on the analytic hierarchy process. Adv Mater Res 461:830–834
Wang XZ, Zhou J, Huang ZL et al (2016) A multilevel deep learning method for big data analysis and emergency management of power system. In: IEEE international conference on big data analysis. IEEE Press, pp 1–5
Wang J, Luo D (2015) Research on emergency decision-making model based on Bayesian decision network. Chin J Manag Sci 1:316–324
Wang YD, Tan GZ, Wang Y et al (2012) Perceptual control architecture for cyber-physical systems in traffic incident management. J Syst Architect 58:398–411
Wang H, Andrews SP, Ni D, Collura J (2010) Scenario-based analysis of transportation impacts in case of dam failure flood evacuation in Franklin County, Massachusetts. In: 89th annual meeting of the transportation research board, Washington, DC, pp 10–14
Wei X (2013) Landslide disaster image recognition algorithm based on SVM. Electr Meas Technol 36(8):65–70
Wen L, Wang HB, Cai WF (2013) Coal mine emergency management safety early warning research based on factor analysis and BP network. Sci Technol Ind 13(10):145–148
Wen R, Zhong S, Zhang B (2013) Multi-Objective Optimization of post-disaster emergency resources scheduling using multiple ant colony systems optimization algorithm. In: Proceedings of 8th international conference on bio-inspired computing: theories and applications (BIC-TA). Springer, Berlin, Heidelberg, pp 1047–1054
Wu A, Convertino G, Ganoe C et al (2013) Supporting collaborative sense-making in emergency management through geo-visualization. Int J Hum Comput Stud 71(71):4–23
Wu X, Ji D, Chen A (2016) Opportunities and challenges of the reform of China’s emergency management system in the age of big data. J Henan Polytech Univ 17(66):182–186
Xie T, Li CD, Wei YY et al (2016) Cross-domain integrating and reasoning spaces for offsite nuclear emergency response. Saf Sci 85:99–116
Xi Y, Sun F (2013) Intelligent urban emergency early warning system based on dynamic rough set and cloud computing. In: IEEE international conference on software engineering and service science, pp 701–704
Xu J, Xu X (2014) A fusion algorithm of swarm intelligence and its application in emergency services facility location. Comput Eng Sci 36(4):667–673
Yan R, Ma Z, Zhao Y, Kokogiannakis G (2016) A decision tree based data-driven diagnostic strategy for air handling units. Energy Build 133:37–45
Yasufuku K, Kashiwagi T, Abe H (2014) Development of arrangement system of evacuation facilities using genetic algorithm and evaluation the safety based on crowd movement. J Archit Plan 79(697):635–642
Yi W, Ozdamar L (2007) A dynamic logistics coordination model for evacuation and support in disaster response activities. Eur J Oper Res 179(3):177–1193
Yin WH, Pamela MT, Satish VU et al (2014) An agent-based modeling system for travel demand simulation for hurricane evacuation. Transp Res Part C 42:44–59
Yotsukura S, Takahashi T (2009) Framework of an emergency management system using different rescue simulators. Adv Robot 23:1233–1246
Yue J, Xiao Y (2013) Application of association rules mining in emergency management system. Netw Secur Technol Appl 1:56–58
Zambrano AM, Perez I, Palau C, Esteve M (2016) Technologies of internet of things applied to an earthquake early warning system. Future Gener Comput Syst 75:206–215
Zhang K, Taylor MAP (2006) Effective arterial road incident detection: a Bayesian network based algorithm. Transp Res Part C 14:403–417
Zhang Q, Zhang J, Wang C, Cui L, Yan D (2014) Risk early warning of maize drought disaster in Northwestern Liaoning Province. China. Nat Hazards 72(2):701–710
Zhang Y, Liang M, Li K et al (2014) Research of source term inversion based on genetic simulated annealing algorithms. Nucl Electron Detect Technol 34(4):451–473
Zhang Y, Dongxiao N, Liu J (2010) The evaluation of electric power emergency management mechanism based on BP neural network. In: IEEE international conference on computer science and information technology, pp 413–416
Zhang L, Li Y, Fei T et al (2014) Research of emergency logistics routing optimization based on particle swarm optimization. In: Proceedings of international conference on computer science and information technology. Springer, India, pp 4027–4030
Zhang W, Zhou Y, Tian W et al (2015) GIS-based indoor pedestrian evacuation simulation combining the particle swarm optimization and the floor field model, pp 1–4
Zhao M, Chen Q (2015) Risk-based optimization of emergency rescue facilities locations for large-scale environmental accidents to improve urban public safety. Nat Hazards 75(1):163–189
Zhao Y, Zhang L, Jie J (2014) Preliminary study on application of BP-neural network in AP1000 nuclear power plant accident diagnosis. Atomic Energy Sci Technol 48:480–484
Zheng Y, Ling H, Xue J et al (2014) Population classification in fire evacuation: a multi-objective particle swarm optimization approach. IEEE Trans Evol Comput 18(1):70–81
Zhong KB (2011) Progress and trend of urban emergency management information technology in the United States. Study Times, 17 Oct 2011
Zhou H, Jia L, YQin (2010) Railway emergency planning system and its implementation. In: Fourth international conference on information systems for crisis response and management, pp 368–374
Zia US, Safina SA, Chong I et al (2014) Web-of-objects (WoO)-based context aware emergency fire management systems for the internet of things. Sensors 14:2944–2966
Zollo A, Lancieri M (2007) Real-time estimation of earthquake magnitude for seismic early warning. In: Gasparini P, Manfredi G, Zschau J (eds) Earthquake early warning systems. Springer, Berlin, Heidelberg, pp 45–63
Download references
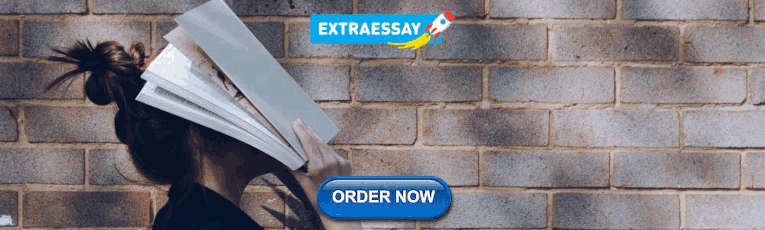
Acknowledgements
This work was supported by the National Social Science Foundation of China (Contact No. 16FGL001) and Scientific Research Foundation of Henan Polytechnic University (No. Y2017-1).
Author information
Authors and affiliations.
College of Computer Science and Technology, Henan Polytechnic University, No. 2001, Century Avenue, Jiaozuo, Henan, People’s Republic of China
School of Business, Jiangnan University, No. 1800, Lihu Avenue, Wuxi, Jiangsu, People’s Republic of China
Wenjing Liu
Institute of Policy and Management, University of the Chinese Academy of Sciences, Beijing, People’s Republic of China
Ruizhen Bai & An Chen
Safety and Emergency Management Research Center, Henan Polytechnic University, No. 2001, Century Avenue, Jiaozuo, Henan, People’s Republic of China
You can also search for this author in PubMed Google Scholar
Corresponding author
Correspondence to Ning Chen .
Ethics declarations
Conflict of interest.
The authors declare that they have no conflict of interest.
Rights and permissions
Reprints and permissions
About this article
Chen, N., Liu, W., Bai, R. et al. Application of computational intelligence technologies in emergency management: a literature review. Artif Intell Rev 52 , 2131–2168 (2019). https://doi.org/10.1007/s10462-017-9589-8
Download citation
Published : 09 November 2017
Issue Date : 01 October 2019
DOI : https://doi.org/10.1007/s10462-017-9589-8
Share this article
Anyone you share the following link with will be able to read this content:
Sorry, a shareable link is not currently available for this article.
Provided by the Springer Nature SharedIt content-sharing initiative
- Emergency management
- Computational intelligence
- Intelligent decision-making
- Find a journal
- Publish with us
- Track your research
- Case Report
- Open access
- Published: 06 April 2024
Effective Apixaban removal using hemoadsorption during emergent open-heart surgery: a case report and narrative literature review
- Vitor Mendes 1 ,
- Jaid Mamode 1 ,
- Jalal Jolou 1 ,
- Mourad Malki 1 ,
- Christoph Ellenberger 2 , 3 ,
- Mustafa Cikirikcioglu 1 , 3 &
- Christoph Huber 1 , 3
Journal of Cardiothoracic Surgery volume 19 , Article number: 185 ( 2024 ) Cite this article
Metrics details
The management of hemostasis in patients medicated with apixaban (Eliquis) undergoing emergency cardiac surgery is exceedingly difficult. The body’s natural elimination pathways for apixaban prove ineffective in emergency situations, and the impact of hemodialysis is limited. The application of Cytosorb ® may attenuate the concentration of apixaban, thereby facilitating the stabilization of these patients.
Case presentation
An 84-year-old man treated with apixaban, underwent emergency ascending aorta replacement surgery due to an acute type A aortic dissection. To address the challenges induced by apixaban, we integrated Cytosorb ® cartridge into the Cardiopulmonary bypass circuit. There was a 63.7% decrease in perioperative apixaban-specific anti-factor Xa activity. The patient’s postoperative course was favourable.
Hemoadsorption with Cytosorb ® may offers a safe and feasible approach for reducing apixaban concentration in emergency cardiac surgery, thereby mitigating the risk of hemorrhagic complications.
Peer Review reports
Cardiopulmonary bypass (CPB) is a technique employed in most cardiac surgery cases. In addition to the underlying disease and the procedure performed, CPB can lead to the development of Systemic Inflammatory Response Syndrome (SIRS), largely mediated by cytokines. Severe cases of SIRS are associated with a prolonged need for vasopressors and mechanical ventilation, and thus, an extended stay in the Intensive Care Unit (ICU) [ 1 ].
Hemoadsorption with Cytosorb ® (CytoSorbents Corporation, Monmouth Junction, NJ, USA) is a novel therapy that has garnered recent attention due to its association with relief from hyper-inflammatory responses in critically ill patients [ 2 ]. Cytosorb ® is currently approved by the Swiss Federal Statistical Office, as well as by the European Union and Food and Drug Administration, as an extracorporeal cytokine adsorber designed to mitigate the effects of cytokine storm that can result in inflammation and multiorgan dysfunction [ 3 , 4 ].
Hemoadsorption with Cytosorb ® can be easily administered by a trained perfusionist using an extracorporeal circuit. Its use is indicated in cases of complex cardiac surgery, infective endocarditis, and in patients with multiple comorbidities. Although initially designed to modulate the inflammatory response and eliminate cytokines and other inflammatory mediators from the blood, it has also demonstrated efficacy in removing drugs such as antiplatelet agents (e.g.,ticagrelor) and novels oral anticoagulants (NOACs) (e.g., apixaban) in emergent surgery cases [ 5 , 6 ].
Apixaban is a factor Xa (FXa) inhibitor used in various clinical conditions, including deep venous embolism and atrial fibrillation [ 7 ].
After administration, Apixaban is rapidly absorbed, reaching peak concentrations 3-4 hours following oral administration. Apixaban undergoes various elimination pathways, including metabolism, biliary excretion, and direct intestinal excretion. However, the primary route of elimination is through renal excretion, constituting approximately 27%. The half-life of Apixaban is 12 hours, but it is only achieved when the glomerular filtration rate is within normal range. This duration may be significantly extended in the presence of renal insufficiency [ 8 ].
Due to its high affinity for plasma proteins, hemodialysis exerts only a limited impact on apixaban elimination, approximately 20%. Consequently, conventional dialysis-based approaches are not recommended as an effective method for managing apixaban overdose [ 9 ]. In the context of cardiac surgery, bleeding is a common complication, and the use of Apixaban is linked to increased surgical morbidity and mortality.
In this paper, we present a case report of the adjuvant use of hemoadsorption with Cytosorb ® in a patient with multiple comorbidities and treated with apixaban, who underwent ascending aorta replacement surgery due to a dissecting pseudoaneurysm of the ascending aorta.
A 84-year-old man (76.5Kg/174cm), treated with apixaban (5mg twice a day) for new-onset atrial fibrillation and known to have heart failure with reduced ejection fraction (HFrEF) of 35% due to amyloidosis and metastatic prostate adenocarcinoma at bone level diagnosed 2 years previously, was admitted to our hospital with NYHA stage III dyspnea and decompensated heart failure.
Blood tests showed high D-dimer levels (4515 ng/ml) and thoracic computed tomography (CT) scan revealed an acute type A aortic dissection.
He was admitted for emergency ascending aorta replacement surgery.
Preoperative transthoracic echocardiography revealed a moderate aortic regurgitation (regurgitant volume- 22.4 ml), with an ascending aorta diameter of 7 cm and a reduced ventricular ejection fraction of 35-40%.
The EuroScore-II pre-operative mortality risk estimate was 24.2 %.
The CPB circuit included a roller pump, a membrane oxygenator (Affinity Fusion TM , Medtronic, Tolochenaz, Switzerland), a hard-shell reservoir (Affinity Fusion Cardiotomy/ venous Reservoir, Medtronic, Tolochenaz, Switzerland) with a 200 ml minimum operating level, a hemoconcentrator (Dideco DHF02, LivaNova, Sorin Group, Mirandola, Italia) and a cardioplegia device (Myotherm XP ® , Medtronic, Tolochenaz, Switzerland). A NaCl 0.9% purged CytoSorb ® cartridge was inserted between the recirculation line and the venous reservoir (Fig. 1 ). Hemoadsorption was performed throughout the entire CPB duration.
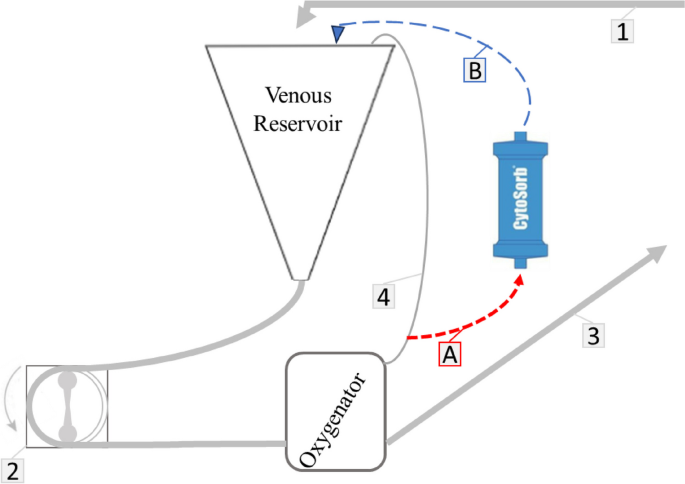
Diagram of Cytosorb ® on CPB circuit. 1-Venous line; 2-Roller pump; 3- Arterial line; 4- Recirculation line, A- Aspiration line, B- Infusion line
Preoperatively, the patient presented thrombocytopenia (platelets, 144 g/l), stage II acute kidney failure (serum creatinine, 107 µmol/l). Activated clotting time (ACT) was 195 seconds, Partial thromboplastin time was 33 seconds and apixaban-specific anti-factor Xa activity (AFXaA) was 121 ng/ml (Table 1 ).
After administration of 20,000 IU of non-fractioned heparin, the ACT reached 714 seconds. CPB was conducted in standard fashion (ph-stat) at normothermia. The ascending aorta was replaced with a 30 mm diameter Dacron tube. The duration of the surgical intervention was 5h53 with 109 min of CPB duration and 52 minutes of aortic cross-clamping.
After CPB weaning, heparin was reversed with 30,000 IU of protamine and final ACT was 161 seconds. Transoesophageal echocardiography showed a left ventricular ejection fraction of 40% and a discrete aortic regurgitation.
A total of 7 units of packed red blood cells, 2 unit of platelets, 8 units of fresh frozen plasma and 6 gramme of fibrinogen were administered to treat perioperative coagulopathy, as well as 3000 IU of prothrombin complex concentrate (Octaplex ® ) and 5 mg of recombinant factor VIIa (NovoSeven ® ).
The patient was transferred to ICU on 5 ug/kg/min of dobutamine and 0.35 ug/kg/min of norepinephrine. No adverse or any device-related side effects were documented during or after CytoSorb ® treatment.
Postoperative chest tube drainage volume at 24h was 280 ml. Dobutamine was discontinued on the end of the first postoperative day, and norepinephrine at the 5 th postoperative hour.
The patient was extubated 48h post-surgery, transferred to the ward on the 4 th postoperative day and discharged on the 21 st postoperative day.
Apixaban is commonly prescribed for the prevention of stroke in patients with non-valvular atrial fibrillation [ 7 ]. Patients medicated with apixaban who require emergency surgery face an increased risk of hemorrhagic complications. Andexanet-alfa serves as the antidote to apixaban [ 10 ]. In an emergency scenario, this drug can rapidly and almost completely reverse the anticoagulant effects of FXa inhibitors.
However, the effects of andexanet alfa in cardiac surgery and potential interactions with unfractionated heparin are not well-established. Additionally, andexanet-alfa comes at a high cost (US$24,750) [ 11 ].
In a cost-utility study aimed at assessing the inherent cost of hemoadsorption in patients treated with ticagrelor, it was found that the use of Cytosorb ® could save approximately GBP 4,000 within 30 days. This analysis encompassed the cartridge price, reduced consumption of blood products, and shorter time spent in an emergency surgical environment [ 5 ]. Economic factors and ease of use have favoured intraoperative hemoadsorption with Cytosorb ® for apixaban elimination in an emergency surgery context [ 5 ].
Hemoadsorption with Cytosorb ® can be easily integrated into a CPB circuit. In our case, the cartridge was inserted between the recirculation line and the venous reservoir (Fig. 1 ).
The fundamental principle is that the aspiration line should be connected to a high-pressure area of the circuit (after the arterial pump), and the ejection line should be connected to a low-pressure point in the circuit. This type of connection prevents veno-arterial shunting and gas embolism, allowing for a constant flow in the arterial part of the circuit.
The ideal flow rate through the Cytosorb ® cartridge is unknown; however, manufacturers recommend a flow rate between 100-700 ml/min [ 12 ]. Recirculation generated through the cartridge should be considered in the blood flow delivered to the patient. As for anticoagulation, it should be mandatory and comprehensive during therapy due to the extensive blood-cartridge contact surface (>40,000m 2 ) [ 13 ]. Since this therapy involves an extracorporeal circuit, this point may not be an issue. Nevertheless, it is important to note that partial anticoagulation is likely to result in rapid device thrombosis, greatly limiting its effectiveness [ 13 ].
To support our findings, we conducted a brief literature review (up until October 1, 2023) on the PubMed database. Only five articles describing interactions between Cytosorb ® and apixaban were identified. The key findings are summarized in Table 2 .
The retrieved studies encompassed various designs, including an experimental in vitro investigation and observational cases studies. Notably, an experimental study examined the reduction of apixaban concentration in an in vitro setting using Cytosorb®. Their results revealed a substantial decrease in apixaban levels after 30 minutes of adsorption, suggesting the potential of this device in reducing apixaban concentrations [ 14 ] .
Complementing these findings, observational case studies, presented instances of patients, particularly elderly individuals, undergoing emergent surgeries or dialysis while on apixaban therapy [ 6 , 15 , 17 ]. In each case, the utilization of Cytosorb® demonstrated a reduction in apixaban concentration, indicating its potential role in managing anticoagulation during critical interventions.
Furthermore, the case-control study investigated the impact of Cytosorb® on patients undergoing cardiac surgery while on concurrent apixaban therapy. Noteworthy outcomes include a significant reduction in bleeding events, lower postoperative volumes, and decrease requirements for DDAVP in the Cytosorb® group compared to controls. This study suggests a potential benefit of Cytosorb® in improving hemostatic outcomes during cardiac surgery in patients on apixaban [ 16 ].
While these findings collectively suggest a positive trend in the use of Cytosorb® in managing apixaban-associated scenarios, it is essential to acknowledge certain limitations within the existing literature, with the majority of studies being case reports. Moreover, the absence of randomized controlled trials introduces uncertainty regarding causality and necessitates caution in interpreting the observational effects.
However, our brief literature review indicates a growing body of evidence suggesting a potential benefit of Cytosorb® in reducing apixaban concentrations and improving hemostasis in patients undergoing surgeries or dialysis while on apixaban therapy.
It is known that the gold standard to measure plasma apixaban concentration, like other drugs, is mass-spectrometry. However, this technique is not viable for real-time use during emergency cardiac surgery. The anti-Xa test is a common method to measure apixaban concentration. Although is it known that during extracorporeal circulation, apixaban levels are not interpretable due to the indirect inhibition of FXa by heparin, with the administration of protamine, the values become valid, as after neutralization of heparin, the test can be interpreted as the apixaban activity.
In our patient, in line with the existing literature, AFXaA also decreased between the beginning and the end of the surgical intervention. A reduction of 63.7% was recorded between the patient’s admission to the operating room (AFXaA=121 ng/l) and admission to the intensive care unit (AFXaA=44 ng/l). This result can be supported by the postoperative 24h chest-tube-drainage, which was only 280 ml.
We also observed an improvement in liver markers (i.e, alkaline phosphatase and GGT) between the two assessment points, which is also supported by published literature [ 18 ].
Regarding limitations, it’s important to note that despite the rapid weaning of vasoactive drugs and the small volume recovered by drains, the stabilization of hemodynamics and coagulopathy cannot be solely attributed to the use of Cytosorb ® . Aggressive administration of blood products and coagulation factors including fresh plasma, fibrinogen, platelets, prothrombin complex concentrate and recombinant factor VIIa was also necessary.
The measurement of apixaban blood concentration should ideally have been conducted at various stages of the surgery to demonstrate the progressive reduction of this drug during therapy. However, the urgent nature of our case precluded the possibility of conducting this type of analysis.
Another limitation worth noting relates to the monitoring of ACT using the Hemochron system (Hemochron ® Signature Elite System, Werfen, Bedford, USA). Although this device provides a quick ACT result in a single measurement, its reliability in assessing the adequacy of heparin anticoagulation monitoring for CPB is questionable. The use of devices like the Hemostasis Management System Plus (HMS ® , Medtronic, Minneapolis, USA) with simultaneous dual measurements could address this issue; however, we do not have this equipment in our institution.
It is important to emphasize that, although the emergent nature, as well as the advanced age and health status, of our case may pose challenges for extended follow-up, we recognize the importance of considering the long-term implications of hemoadsorption in future research endeavours.
In conclusion, this case demonstrates that intraoperative hemoadsorption with Cytosorb ® may be a safe and feasible approach, increasing the elimination of apixaban in patients undergoing emergency cardiac surgery with CPB, resulting in a reduced risk of hemorrhagic complications.
Availability of data and materials
No datasets were generated or analysed during the current study.
Abbreviations
- Cardiopulmonary bypass
Systemic Inflammatory Response Syndrome
Intensive Care Unit
Novel Oral Anticoagulants
Heart failure with reduced ejection fraction
New York Heart Association
Computed Tomography
Activated Clotting Time
Apixaban-Specific anti-factor Xa activity
Continuous Renal Replacement Therapy
Paparella D. Cardiopulmonary bypass induced inflammation pathophysiology and treatment. An update Eur J Cardio-Thorac Surg. 2002;21(2):232–44.
Article CAS Google Scholar
Naruka V, Salmasi MY, Arjomandi Rad A, Marczin N, Lazopoulos G, Moscarelli M, et al. Use of Cytokine Filters During Cardiopulmonary Bypass: Systematic Review and Meta-Analysis. Heart Lung Circ. 2022;31(11):1493–503.
Article PubMed Google Scholar
CytoSorbents Corporation. CytoSorb® Assigned Dedicated Procedure Code in Switzerland. In:PRNewswire. 2018. Available from: https://cytosorbents.com/cytosorb-assigned-dedicated-procedure-code-in-switzerland/ . Cited 2023 Oct 9.
CytoSorbents Corporation. Health Canada Authorizes Cytosorb For Use In Hospitalized COVID-19 Patients. In:PRNewswire. 2021. Available from: https://cytosorbents.com/health-canada-authorizes-cytosorb-for-use-in-hospitalized-covid-19-patients/ . Cited 2023 Oct 9.
Javanbakht M, Trevor M, Rezaei Hemami M, Rahimi K, Branagan-Harris M, Degener F, et al. Ticagrelor Removal by CytoSorb® in Patients Requiring Emergent or Urgent Cardiac Surgery: A UK-Based Cost-Utility Analysis. Pharmacoecon Open. 2020;4(2):307–19.
Mendes V, Colombier S, Verdy F, Bechtold X, Schlaepfer P, Scala E, et al. Cytosorb ® hemoadsorption of apixaban during emergent cardio-pulmonary bypass: a case report. Perfusion. 2021;36(8):873–5.
Jiménez D, Yusen RD, Ramacciotti E. Apixaban: an Oral Direct Factor-Xa Inhibitor. Adv Ther. 2012;29(3):187–201.
Byon W, Garonzik S, Boyd RA, Frost CE. Apixaban: A Clinical Pharmacokinetic and Pharmacodynamic Review. Clin Pharmacokinet. 2019;58(10):1265–79.
Article PubMed PubMed Central Google Scholar
Poel T, de Rouw N, Péquériaux NCV, van de Kerkhof D, Windsant AMAV, van Marum RJ, et al. Effect of conventional hemodialysis on the apixaban plasma concentration. Hemodialysis International. 2024;28(1):72–6.
Connolly SJ, Crowther M, Eikelboom JW, Gibson CM, Curnutte JT, Lawrence JH, et al. Full Study Report of Andexanet Alfa for Bleeding Associated with Factor Xa Inhibitors. New England Journal of Medicine. 2019;380(14):1326–35.
Article CAS PubMed Google Scholar
Beik N, Reddy P, Sylvester KW, Connell NT, Giugliano RP, Piazza G, et al. Andexanet Alfa (Andexxa) Formulary Review. Critical Pathways in Cardiology: A Journal of Evidence-Based Medicine. 2019;18(2):66–71.
Article Google Scholar
Wei S, Zhang Y, Zhai K, Li J, Li M, Yang J, et al. CytoSorb in patients with coronavirus disease 2019: A rapid evidence review and meta-analysis. Front Immunol. 2023;31:14.
Google Scholar
Abraham P, Mendes V, Kirsch M, Schneider A. Hemoperfusion in Cardiac Surgery and ECMO. In: Ronco C, Bellomo R, editors. Contributions to Nephrology Adsorption: The New Frontier in Extracorporeal Blood Purification. S.Karger AG; 2023;180–91. Available from: https://karger.com/doi/10.1159/000527338
Røed-Undlien H, Schultz NH, Lunnan A, Husebråten IM, Wollmann BM, Molden E, et al. In Vitro Apixaban Removal By CytoSorb Whole Blood Adsorber: An Experimental Study. J Cardiothorac Vasc Anesth. 2022;36(6):1636–44.
Buonocore M, Rex S, Degezelle K, Meyns B. CytoSorb haemoadsorption for removal of apixaban—A proof-of-concept pilot case for a randomized controlled trial. J Clin Pharm Ther. 2022;47(12):2373–5.
Hassan K, Thielmann M, Easo J, Kamler M, Wendt D, Haidari Z, et al. Removal of Apixaban during Emergency Cardiac Surgery Using Hemoadsorption with a Porous Polymer Bead Sorbent. J Clin Med. 2022;11(19):5889.
Article CAS PubMed PubMed Central Google Scholar
Dalmastri V, Angelini A, Minerva V, Ballarini M, Grammatico F, Todeschini P, et al. Extracorporeal hemoadsorption therapy as a potential therapeutic option for rapid removal of Apixaban in high risk-surgical patients: a case report. J Med Case Rep. 2023;17(1):283.
Dhokia V, Madhavan D, Austin A, Morris CG. Novel use of Cytosorb TM haemadsorption to provide biochemical control in liver impairment. J Intensive Care Soc. 2019;20(2):174–81.
Download references
Open access funding provided by University of Geneva
Author information
Authors and affiliations.
Division of Cardiovascular Surgery, Department of Surgery, Geneva University Hospitals, 1205, Geneva, Switzerland
Vitor Mendes, Jaid Mamode, Jalal Jolou, Mourad Malki, Mustafa Cikirikcioglu & Christoph Huber
Department of Anesthesiology, Pharmacology Division of Anesthesiology, Intensive Care and Emergency Medicine, Geneva University Hospitals, Rue Gabrielle-Perret- Gentil 4, 1205, Geneva, Switzerland
Christoph Ellenberger
Faculty of Medicine, University of Geneva, Geneva, Switzerland
Christoph Ellenberger, Mustafa Cikirikcioglu & Christoph Huber
You can also search for this author in PubMed Google Scholar
Contributions
VM analysed and interpreted the patient data, and was a major contributor in the writing of the manuscript. VM, JM and MM were a major contribution to prepared Tables 1 and 2 and Fig. 1 . VM was the perfusionist operating the heart-lung machine for the patient and deploying Cytosorb therapy. JJ and MC were the cardiac surgeons operating the patient, and CE was the anesthesiologist in the operating room during the surgery. JJ, CE, MC and CH reviewed the manuscript and made appropriate changes. All authors read and approved the final manuscript.
Corresponding author
Correspondence to Vitor Mendes .
Ethics declarations
Ethics approval and consent to participate.
Since this is a case report, no ethical review board approval is required.
Consent for publication
Written informed consent was obtained from the patient for publication of this case report.
Competing interests
The authors declare no competing interests.
Additional information
Publisher’s note.
Springer Nature remains neutral with regard to jurisdictional claims in published maps and institutional affiliations.
Rights and permissions
Open Access This article is licensed under a Creative Commons Attribution 4.0 International License, which permits use, sharing, adaptation, distribution and reproduction in any medium or format, as long as you give appropriate credit to the original author(s) and the source, provide a link to the Creative Commons licence, and indicate if changes were made. The images or other third party material in this article are included in the article's Creative Commons licence, unless indicated otherwise in a credit line to the material. If material is not included in the article's Creative Commons licence and your intended use is not permitted by statutory regulation or exceeds the permitted use, you will need to obtain permission directly from the copyright holder. To view a copy of this licence, visit http://creativecommons.org/licenses/by/4.0/ . The Creative Commons Public Domain Dedication waiver ( http://creativecommons.org/publicdomain/zero/1.0/ ) applies to the data made available in this article, unless otherwise stated in a credit line to the data.
Reprints and permissions
About this article
Cite this article.
Mendes, V., Mamode, J., Jolou, J. et al. Effective Apixaban removal using hemoadsorption during emergent open-heart surgery: a case report and narrative literature review. J Cardiothorac Surg 19 , 185 (2024). https://doi.org/10.1186/s13019-024-02748-1
Download citation
Received : 08 December 2023
Accepted : 29 March 2024
Published : 06 April 2024
DOI : https://doi.org/10.1186/s13019-024-02748-1
Share this article
Anyone you share the following link with will be able to read this content:
Sorry, a shareable link is not currently available for this article.
Provided by the Springer Nature SharedIt content-sharing initiative
- Hemoadsorption
- Case report
Journal of Cardiothoracic Surgery
ISSN: 1749-8090
- General enquiries: [email protected]

An official website of the United States government
The .gov means it’s official. Federal government websites often end in .gov or .mil. Before sharing sensitive information, make sure you’re on a federal government site.
The site is secure. The https:// ensures that you are connecting to the official website and that any information you provide is encrypted and transmitted securely.
- Publications
- Account settings
Preview improvements coming to the PMC website in October 2024. Learn More or Try it out now .
- Advanced Search
- Journal List
- J Med Internet Res
- PMC10199396

User Compliance With the Health Emergency and Disaster Management System: Systematic Literature Review
Widia resti fitriani.
1 Faculty of Computer Science, Universitas Indonesia, Depok, Indonesia
Juliana Sutanto
2 Department Human Centred Computing, Faculty of Information Technology, Monash University, Melbourne, Australia
Putu Wuri Handayani
Achmad nizar hidayanto, associated data.
Hazard classification by WHO [ 36 ]. WHO: World Health Organization.
PRISMA 2020 checklist. PRISMA: Preferred Reporting Items for Systematic Reviews and Meta-Analyses.
Studies included in the final review.
Factors affecting user compliance with Health EDMS. EDMS: emergency and disaster management system.
All data analyzed during this study are included in this published paper and its Multimedia Appendices.
Health-related hazards have a detrimental impact on society. The health emergency and disaster management system (Health EDMS), such as a contact-tracing application, is used to respond to and cope with health-related hazards. User compliance with Health EDMS warnings is key to its success. However, it was reported that user compliance with such a system remains low.
Through a systematic literature review, this study aims to identify the theories and corresponding factors that explain user compliance with the warning message provided by Health EDMS.
The systematic literature review was conducted using Preferred Reporting Items for Systematic reviews and Meta-Analyses 2020 guidelines. The search was performed using the online databases Scopus, ScienceDirect, ProQuest, IEEE, and PubMed, for English journal papers published between January 2000 and February 2022.
A total of 14 papers were selected for the review based on our inclusion and exclusion criteria. Previous research adopted 6 theories when examining user compliance, and central to the research was Health EDMS. To better understand Health EDMS, based on the literature reviewed, we mapped the activities and features of Health EDMS with the key stakeholders involved. We identified features that require involvement from individual users, which are surveillance and monitoring features and medical care and logistic assistance features. We then proposed a framework showing the individual, technological, and social influencing factors of the use of these features, which in turn affects compliance with the warning message from Health EDMS.
Conclusions
Research on the Health EDMS topic increased rapidly in 2021 due to the COVID-19 pandemic. An in-depth understanding of Health EDMS and user compliance before designing the system is essential for governments and developers to increase the effectiveness of Health EDMS. Through a systematic literature review, this study proposed a research framework and identified research gaps for future research on this topic.
Introduction
Emergencies and disasters threaten the safety of human life and trigger acute feelings of stress, anxiety, and uncertainty [ 1 ]. The increasing number of disasters and the exposure of people and property to hazards have prompted an increased interest in and support for emergency and disaster management (EDM) policies and programs [ 2 ]. The terms “emergency management” and “disaster management” are often used interchangeably. Disaster management is the organization, planning, and application of measures to prepare for, respond to, and recover from disasters [ 3 , 4 ]. Meanwhile, emergency management is defined as a strategic management process to protect critical assets from hazards, save lives, minimize property or environmental damage, and reduce suffering [ 5 , 6 ]. During disaster or emergency management, there is a need to coordinate the efforts within and across organizations and securely exchange data and share information through a system [ 7 ].
Previous studies [ 8 - 11 ] have distinguished between the emergency management system (EMS) and the disaster management system (DMS). An EMS aims to handle emergency planning, control, and reduction with 3 essential components: hazard detection, emergency management, and public communication [ 8 , 9 ]. Meanwhile, a DMS consists of 3 application domains: monitoring, response, and forecasting the expected disasters [ 10 ]. In addition, a DMS includes the functionality of information sharing, search and rescue missions, and damage assessment [ 11 ]. In general, the functionality of the EMS and DMS follows the EDM phases, which are mitigation, preparedness, response, and recovery. This study uses the term “emergency and disaster management system” (EDMS) to cover all the EDM phases.
With the increasing risk of emergency and disaster, research related to EDMSs is constantly evolving for various hazard types, including health-related hazards. Health-related hazards, such as disease outbreaks (eg, COVID-19, avian influenza, and Ebola), are 1 of the most common hazardous events [ 9 ]. From 2012 to 2017, the World Health Organization (WHO) recorded more than 1200 outbreaks in 168 countries, including those due to new or re-emerging infectious diseases. In 2018, another 352 infectious disease events emerged. Several disease outbreaks are classified as Public Health Emergency for International Concern (PHEIC) by WHO, including the 2009 H1N1 influenza pandemic, Ebola (2013-2015 West African outbreak and 2018-2020 outbreak in the Democratic Republic of Congo), poliomyelitis (2014 to present), Zika (2016), and COVID-19 (2020 to now) [ 7 ]. From the ongoing COVID-19 pandemic, there have been more than 349 million cases of COVID-19 worldwide, including more than 5.5 million deaths as of January 31, 2022 [ 12 ]. Moreover, the International Monetary Fund estimates that the global economy shrunk by 4.4% in 2020 compared to 2019 due to the COVID-19 pandemic [ 13 ].
Health-related hazards have different characteristics from natural disasters and disasters caused by humans. When a natural disaster or human-induced disaster occurs, people are advised to evacuate to a safe place. In contrast, during a pandemic, people must stay at home or be quarantined to prevent disease transmission [ 14 ]. As infectious diseases spread quickly, they require fast and appropriate treatment [ 14 ]. Various media and information sources, such as mass media, text messages, social media, and notifications in the community, can be used to disseminate warnings [ 15 ]. During the COVID-19 pandemic, self-administered warning systems or applications, called contact-tracing applications (CTAs), have been widely used to disseminate alerts and recommendations [ 16 , 17 ]. A CTA is a form of the health emergency and disaster management system (Health EDMS) that is used to prevent, prepare for, respond to, and deal with emergencies and disasters that threaten public health [ 3 ]. Health EDMS can be applied to all stages of EDM, but the response stage requires user involvement the most. During the response phase, Health EDMS performs disease monitoring and tracing, provides medical care, and disseminates information and warnings [ 18 - 21 ]. Risk communication plays a vital role in attracting user attention and encouraging compliance to Health EDMS warnings.
Understanding individual behavior toward Health EDMS is essential because health EDM is not solely the government's responsibility [ 2 ]. Individuals are an integral part of the system as they are responsible for the health of themselves, their families, and their neighbors [ 2 ]. Previous studies [ 22 - 30 ] have identified the factors driving individuals' adoption of Health EDMS. In addition to these studies, 2 literature reviews [ 31 , 32 ] have summarized the determining factors of individuals' acceptance and adoption of Health EDMS. The first literature review compiled 25 studies of individuals' acceptance and adoption of a CTA. The second literature review conducted a systematic review of 21 national COVID-19 CTAs and verified CTA quality and public adoption [ 32 ]. These reviews have shown that engagement could increase adoption and that there is an association between higher application adoption and lower infection rates [ 32 ]. Several other literatures reviewed on the Health EDMS topic have discussed the privacy concerns [ 33 - 35 ] and the digital solutions for dealing with the COVID-19 outbreak [ 36 , 37 ].
The extant literature reviews focus on the acceptance and adoption of Health EDMS. Although they have offered valuable insights into the development of studies in the Health EDMS field, little attention has been paid to how effective the warning message provided by Health EDMS generates user compliance [ 38 ]. According to Han et al [ 38 ], Abdelhamid et al [ 39 ], and O’Malley et al [ 40 ], compliance with warning messages sent by Health EDMS is crucial to determining the EDMS's effectiveness. Users who comply with Health EDMS's warning message play an active role in preventing disease transmission [ 38 ]. However, the compliance rate is reported to be low in the context of health-related hazards [ 27 , 41 ]. Therefore, it is important to understand the factors affecting user compliance with Health EDMS's warning message. This paper addresses the following question: What theories and corresponding factors explain user compliance with the warning message provided by Health EDMS?
To answer this question, we reviewed concepts related to hazards, the EDM cycle, and EDMS features from previous research to understand the activities and stakeholders involved in EDM. After that, we mapped the EDMS feature into a CTA to find out which EDMS features have been implemented to deal with health-related hazards. We also analyzed the roles and authorization of stakeholders in each feature to find out which features involve user participation and compliance. Through this mapping, this research can make a specific contribution regarding user participation in Health EDMS and compliance with Health EDMS’s warning message.
Our systematic review followed the Preferred Reporting Items for Systematic Reviews and Meta-Analyses (PRISMA) 2020 method because it has clear, structured, transparent, and complete reporting of systematic reviews [ 42 ]. The target audience for this review was threefold: first, researchers who are interested in pursuing research on compliance with the warning message provided by Health EDMS; second, organizations and governments that need information about what related factors can influence the design and implementation of Health EDMS; and third, Health EDMS vendors or developers who would like to understand the sociotechnical factors affecting the compliance of warning messages prior to Health EDMS implementation.
This paper is organized into 5 sections. Section 1 describes the research background and explains the key concepts, and the research methodology is discussed in Section 2. Next, the results and discussion of this study are elaborated in Sections 3 and 4. The final section concludes this study.
Key Concepts
Before discussing emergencies and disasters, it is important to understand the definition and classification of hazards. A hazard is a process, phenomenon, or human activity that can harm people's lives and health, damage property, disrupt social and economic activities, and damage the environment [ 3 ]. The WHO classification of hazards generally consists of natural, human-induced, and environmental hazards, as provided in Multimedia Appendix 1 [ 36 ].
Hazards have the potential to create any scale of emergency or disaster. Emergency and disaster, at first glance, have a similar meaning, but there are fundamental differences between the two. An emergency can be defined as a severe disruption to the functioning of a community or society, causing human, material, economic, or environmental impacts, which can be overcome by the internal resources of the community and society itself [ 43 ]. However, a disaster is a severe disturbance that impacts communities and society and cannot be overcome solely by relying on internal resources [ 43 ]. In other words, a disaster is an event that causes significant damage or loss and thus requires resources beyond a community's capability and multiple agency responses [ 44 ]. Thus, an event is declared a disaster if there is a need for external assistance to address its impact [ 45 ]. Both emergencies and disasters must be managed to prevent or minimize their impacts.
Emergency and Disaster Management
To answer the research question, we need to understand the general concept of EDM. As previously mentioned, the terms “emergency management” and “disaster management” are often used interchangeably because they have considerable overlap [ 3 ]. The EDM cycle consists of 4 phases, namely mitigation, preparedness, response, and recovery [ 11 , 45 , 46 ], as shown in Figure 1 . The mitigation and preparedness phases are part of risk assessments before a hazardous event [ 45 ]. Mitigation is the application of actions to prevent a hazard from occurring or reduce its impact [ 18 ]. Although the impact of a hazard is often not wholly preventable, various strategies and actions can substantially reduce its scale or severity. Activities during the mitigation phase include engineering techniques and hazard-resistant construction, environmental and social policy development, and public awareness enhancement [ 3 ].

The EDM cycle. EDM: emergency and disaster management.
The second phase, preparedness, aims to prepare the community to respond to a hazard [ 18 ] by providing information, creating partnerships, developing plans, building resources, and creating procedures [ 47 ]. When a hazardous event occurs, response and recovery must be carried out [ 45 ]. The response actions directly before, during, or immediately after a hazardous event occurs can save lives, reduce health impacts, ensure public safety, and meet the basic needs of the affected people [ 3 ]. Activities during the response phase include evacuation, search and rescue, medical care, and temporary housing [ 3 , 18 ]. The response activities may also extend to the recovery stage [ 45 ]. Recovery is a long-term action taken after the immediate effects of a hazardous event have passed in order to stabilize society and return to normalcy [ 18 ]. Recovery also aims to restore or improve the livelihoods and health of the affected populations and restore the economic, physical, social, cultural, and environmental systems [ 3 ].
Each activity shown in Figure 1 requires cooperation and coordination among the various parties, including the community, governments, health institutions, other groups, and regional and international organizations [ 48 ]. The community includes at-risk populations, survivors, and community groups or organizations [ 48 ]. Governments at all levels include leaders and politicians, ministries and agencies, national disaster management agencies, emergency services (eg, fire department, police, ambulance), and military service [ 48 ]. The third stakeholder is health institutions, including the Ministry of Health and health authorities, health-related nongovernment organizations (NGOs), health care providers, hospitals and other health care facilities, the health workforce, and private sector health organizations and professionals [ 48 ]. Other groups include universities and research institutes, nongovernment and volunteer groups, media, social media, new media, community influencers, and the private sector [ 48 ]. Finally, regional and international organizations include WHO and international NGOs [ 48 ].
This research focuses on community stakeholders affected by health hazards. Individuals can contribute to community-level surveillance, household preparedness, first-aid training, and emergency response [ 48 ]. Their active engagement in all aspects of EDM activities is vital [ 48 ]. To encourage active participation from the community, EDM needs to implement effective risk communication to attract attention and encourage user compliance. A warning is a risk communication used to inform people about a hazard so that accidents, injuries, or unwanted consequences can be avoided [ 49 , 50 ]. The purpose of warning messages is to draw the attention of people at risk, to reduce the time it takes to understand the warning, and to guide people to take appropriate protective measures [ 51 , 52 , 53 ]. In this case, risk communication serves as a component of disaster control and strategies to reduce the health, economic, and psychosocial impacts of major disaster events [ 40 ]. Risk communication is vital in all EDM phases, especially the response phase. The next section maps EDM phases and activities to EDMS features.
Emergency and Disaster Management System
Various studies have used different terms to describe the EDMS features for each of the 4 EDM phases. The term “emergency management system” is used by the United Nations [ 4 ] and Landau [ 54 ], which refers to a system that works for emergency planning, control, and reduction. The term “disaster management system” is used by Hannan et al [ 55 ] and Currion et al [ 56 ] as a system that aims to manage relief operations, recovery, and rehabilitation. The term “emergency response system“ is used by Amailef and Lu [ 57 ] and refers to a system that supports communications, data gathering, data analysis, and decision-making during the response phase. Meanwhile, research by Edwards et al [ 58 ] and Malizia et al [ 59 ] uses the term “emergency notification system,” which refers to a system that aims to broadcast information to as many devices as possible. Other studies by Ouyang et al [ 60 ] and Bonaretti and Fischer-Preßler [ 61 ] use the term “early warning system,” which aims to disseminate warnings to a threatened population before or at an early stage of hazardous events. In the context of biological hazards that threaten public health, the terms “digital contact tracing” [ 14 , 62 ] and “contact-tracing application” [ 34 , 63 ] are used to refer to a system that monitors and tracks infection and provides immediate support and information during pandemics.
Tables 1 - -4 4 map the activities and system features for each EDM phase and the stakeholders involved according to previous studies. The stakeholders consist of the community, governments, health institutions, other groups, and regional and international organizations. Tables 1 - -4 4 also show the stakeholders' roles in each feature with the responsible, accountable, consulted, and informed (RACI) matrix. In this study, the RACI matrix was used to analyze stakeholders and their level of involvement and authorization in each feature of the EDMS. Because this research focused on community users, the RACI matrix showed which features require involvement from community users. From the 4 stages of EDM, 19 main activities and 42 EDMS features were identified. Among all the features identified, there were 27 features that require involvement from the community users that the other stakeholder groups should pay attention to in order to encourage user compliance with the warning messages.
System features and key stakeholders of an EDMS a for the mitigation (MT) activity.
a EDMS: emergency and disaster management system.
b Co: community.
c G: governments.
d H: health institutions.
e O: other groups.
f Ro: regional and international organizations.
g N/A: not applicable.
h A: accountable.
i C: consulted.
j I: informed.
k R: responsible.
System features and key stakeholders of an EDMS a for the recovery (RC) activity.
Based on Tables 1 - -4, 4 , community users can act as actors who are informed by or responsible for several features in an EDMS. Before a hazard occurs, community users can receive information about policies and strategies, potential hazards, and various types of education to increase their awareness of emergencies and disasters. When a hazard occurs, community users need information about the location of shelters, evacuation routes, and regular reports on ongoing disasters. During the response and recovery phases, community users play a more important role because in addition to obtaining information, they are also responsible for being actively involved in disaster management efforts. They must actively report disaster victims and missing persons, report tracing data and lab results, report damage to infrastructure, and request medical assistance and treatment. Community users who actively use EDMS features are encouraged to comply with the protocols and measures to deal with emergencies and disasters. Therefore, the design of these features is essential to drive user compliance.
System features and key stakeholders of an EDMS a for the preparedness (PR) activity.
i I: informed.
j C: consulted.
System features and key stakeholders of an EDMS a for the response (RS) activity.
g I: informed.
i R: responsible.
k N/A: not applicable.
Health Emergency and Disaster Management System
All the features analyzed in the previous section are used in a general EDMS dealing with all types of emergencies and disasters. To find out how these features have been used to address health-related disasters, we investigated their application to Health EDMS, an EDMS that is specifically used to respond to and cope with hazardous events that threaten public health. During the COVID-19 pandemic, Health EDMS was used by multiple countries to assist in contact tracing. Contact tracing is a control measure to prevent further disease transmission [ 70 ]. When someone tests positive for COVID-19, that person must be quarantined or self-isolated. After that, the contact-tracing process is carried out by identifying the person's close contacts and advising them to take precautionary self-isolation [ 71 ]. Close contact means direct face-to-face contact with an infected person or confirmed case [ 71 ]. Various countries have developed contact-tracing mechanisms with technology support. A CTA allows devices to communicate through Bluetooth technology, GPS, wireless technology, and sensors [ 71 ]. A CTA has features to automate contact-tracing activities and other features to disseminate information and provide medical care [ 70 ].
In this study, the discussion of Health EDMS will focus on a CTA because a CTA is a clear example of a technology application that deals with health emergencies and disasters. As a self-administered warning system, CTAs have been widely used in more than 50 countries [ 72 ]. A CTA is implemented when COVID-19 emerges in order to respond to and recover from the pandemic [ 16 ]. Therefore, the system features are geared more toward the response and recovery phases than the mitigation and preparedness phases. Table 5 displays a list of features for response and recovery phases in some CTAs implemented by different countries. The countries shown were selected based on the number of COVID-19 cases: high (the United States and India), medium (the United Kingdom and France), and low (Singapore and Switzerland) [ 73 ]. In addition to the number of cases, these countries were also chosen to represent the Americas, Europe, and Asia regions. The CTAs selected are available on Apple App Store and Google Play Store with the most significant number of users, free of cost, and launched and supported by the governments of selected countries [ 73 ]. The CTA features in the selected countries were based on the information provided by Alanzi [ 16 ] and Blasimme et al [ 17 ].
CTA a features.
a CTA: contact-tracing application.
b EDMS: emergency and disaster management system.
c NHS: National Health Service.
There are 6 CTAs reviewed in Table 5 . PathCheck SafePlace is a CTA developed in the United States that aims to integrate people and health departments to prevent the spread of COVID-19 by sharing information [ 16 ]. The application was developed by the Massachusetts Institute of Technology, TripleBlind, and a nonprofit organization called PathCheck Foundation [ 16 ]. Aarogya Setu is an official CTA launched by the Indian government with more than 1.4 million users [ 16 ]. NHS COVID19 is a CTA launched by the National Health Service (NHS) Test and Trace in the United Kingdom [ 16 ]. Although this application does not store personal information, it still collects location information for track and trace purposes [ 16 ]. TousAntiCovid is implemented in France using a centralized IT architecture where data are stored on centralized servers run by the national health authorities [ 17 ]. Users must enter their personal information to report a positive test, which triggers a notification to other users [ 17 ]. The health authorities in France send an exposure code to users via email and post [ 17 ]. In contrast to TousAntiCovid, SwissCovid implements a decentralized protocol like in other European countries, such as the United Kingdom [ 66 ]. Users must contact health authorities to activate an exposure code after receiving a notification of a positive test result [ 66 ]. Another application, TraceTogether, is an official CTA launched by the Singapore government [ 16 ]. This application emphasizes user privacy by using anonymous IDs and giving users the right to delete their data [ 16 ].
Based on Table 5 , at the most, the CTAs implemented 11 EDMS features in 5 EDM activities: response (RS)2, RS3, RS4, recovery (RC)3, and RC4. Most CTAs implemented all 3 features in RS2 to monitor and report the ongoing hazard; disseminate notifications and warnings; and provide timely, credible, and actionable information to the public. The CTAs also provide data visualization that enables users to monitor the ongoing status of the COVID-19 cases in their areas. Some CTAs (ie, PathCheck SafePlace, and Aarogya Setu, provide query resolution feature as part of RS3, allowing users to request help and ask questions through the applications [ 16 ].
Medical care provision (RS4) is 1 of the priority features in CTAs. CTAs provide automatic contact-tracing tools integrated with location mapping through GPS. Aarogya Setu integrates these tools with an electronic pass (e-pass) in transportation and public places [ 16 ]. CTAs also provide users with self-assessment tools to check their health status and exposure to COVID-19. Health institutions provide laboratory check reports to users through their handheld devices. Some CTAs(ie, PathCheck SafePlace and Aarogya Setu) also come with online consultation and appointment scheduling with health facilities [ 16 ]. The medical care and surveillance activities continue to the recovery phases in RC3 and RC4.
In the previous section, there were 27 EDMS features that involved the user community. Meanwhile, Table 5 shows that only 11 have been implemented in Health EDMS, mainly functioning to monitor and report ongoing hazards and provide medical care and logistical support. Health EDMS does not implement all the features at the mitigation and preparedness stages. Several features in the response and recovery phases are also not implemented because they are not in accordance with the scope of health-related hazards, such as shelter data management, reporting of victims and missing persons, and recording of infrastructure damage. In Health EDMS, all the measures need to be complied with by the users to make them effective in controlling the pandemic [ 38 , 40 ]. When surveillance and monitoring tools detect hazardous conditions, Health EDMS disseminates notifications and warnings to users. User compliance with notifications and warnings determines the success of Health EDMS. The following section discusses user compliance in the information system (IS) context.
Compliance refers to the agreement with the expectations stated in the rules, standards, proposals, requests, orders, or suggestions [ 74 ]. It is also defined as a relationship consisting of the power used by superiors to control subordinates and subordinates' orientation to this power [ 75 ]. Compliance can include approval as well as obedience [ 74 ]. In health care, compliance is conceptualized as a cognitive-motivational process of personal attitudes and intentions, behaviors, and the outcome of patient-practitioner interactions [ 76 ]. It also involves professional power over the patient [ 76 ]. Compliance is not based solely on an individual risk-benefit assessment but also involves relationships between commanders and followers and between organizations and members [ 38 ].
Research on compliance has been investigated in various fields, and theories regarding compliance also come from multiple domains, such as psychology, criminology, health, management, and organization [ 77 ]. In the organizational context, business dynamism and IT advancements encourage companies to frequently update their IS and its usage policy [ 78 ]. In this case, users need to maintain IS compliance behavior due to the interdependent nature of tasks that need to be accomplished through IS [ 78 ]. Social (trust and support) and performance (discipline and stretch) management influences collective compliance with IS [ 78 ]. Compliance also has been widely studied in IS security policy research [ 38 ]. Employees are willing to comply with IS security policy if they feel they have the capacity to carry out a security task, have a positive attitude in carrying it out, and see others performing the same security task [ 77 ]. When they encounter a security threat, they evaluate the threat and coping behavior to decide whether to comply [ 77 ]. Employee compliance with IS and its usage policy is critical to achieving IS objectives in supporting business operations [ 78 ].
Health EDMS differs from other ISs as it is used in life-critical and time-sensitive situations, often with limited resources. Immediate compliance with the health notifications and warnings is essential to save lives [ 38 ]. When a person receives a warning, the warning response can be sequenced as follows: listen to the warning, understand the content of the warning message, believe that the warning is credible and accurate, personalize the warning, confirm the warning, and respond by taking protective action [ 79 ]. Previous studies have shown that responses to warning messages are influenced by the type of hazard, the message and its source, the medium or channel through which the message is communicated, the characteristics of the recipient, and situational or environmental factors [ 51 , 79 ]. We conducted a systematic literature review to map the extant studies on user compliance with Health EDMS.
Search Strategy
A systematic literature review is a secondary study conducted to identify, evaluate, and interpret all available research relevant to a particular research question, topic area, or phenomenon of interest [ 80 ]. A systematic literature review summarizes the existing research insights, identifies research gaps, suggests areas for further investigation, or provides a framework to position new research activities [ 81 ]. Our systematic review was conducted using PRISMA 2020 guidelines [ 42 ]. The PRISMA checklist can be seen in Multimedia Appendix 2 .
The search was conducted using the online databases Scopus, ScienceDirect, ProQuest, IEEE, and PubMed. The keywords or search strings used to search the papers were (“emergency” OR “disaster” OR “response” OR “notification” OR “warning” OR “alert” OR “tracing”) AND (“system” OR “apps” OR “application”) AND (“compliance”). The search was conducted for journal papers published between January 2000 and February 2022.
Inclusion and Exclusion Criteria
The inclusion criteria were the review guidelines for study selection, as displayed in Table 6 : be published in a journal, have full text available, and be written in English. In addition, in accordance with the objectives of this study, the papers had to discuss compliance with the warning or alert from Health EDMS and focus on individual perceptions or behaviors on Health EDMS. Therefore, papers with related terms, such as “emergency management system,” “disaster management system,” “emergency notification system,” “emergency warning system,” “emergency response system,” and “contact-tracing application,” were also included in this review. We did not include the keywords “mobile health” or “mHealth” because these applications are not used specifically for health emergencies and disasters but are for everyday use, which was not the scope of this research. This study was limited to a review of previous research on compliance from a user perspective and did not include the technical design of Health EDMS.
Inclusion and exclusion criteria.
Study Selection
The study selection was carried out as follows:
- Step 1: The keyword or search string was searched in the aforementioned online databases. We limited the search to the abstract, title, or keyword fields. Duplicate records were removed.
- Step 2: The title and abstract of the identified papers were reviewed based on the inclusion criteria. Papers that did not meet inclusion criteria were removed.
- Step 3: The remaining papers were read in full to determine whether they met the inclusion criteria.
Data Items and Synthesis
The data extraction process aimed to identify relevant information from the included studies that pertained to our research question. This process included producing a Microsoft Excel data sheet consisting of key aspects related to the research aim. The following data were extracted from each publication: title, author(s), year of publication, name of the journal, country, topic, research question or objective, factor(s), method, recommendation, finding, and research gap. Each paper's full text was read, and the research data were entered into the Excel sheet. Once the extraction was completed, the Excel sheet was reviewed, and then the findings of the studies were analyzed to answer the research question. The results of the review are discussed in the next section.
Review Process
The selected electronic databases were searched following the previously explained search strategy. In total, 618 papers were retrieved. Next, duplicate papers were removed, resulting in 573 (92.7%) papers. The papers' titles and abstracts were reviewed by applying the inclusion criteria. After removing the papers that did not fulfill the inclusion criteria, we were left with 95 (16.6%) papers. Next, the papers' full texts were read to ensure they covered the predefined scope. This step resulted in 14 (14.7%) selected papers. A summary table of the characteristics of the included studies is provided in Multimedia Appendix 3 . Figure 2 shows the review process using PRISMA guidelines.

PRISMA flow diagram. EDMS: emergency and disaster management system; PRISMA: Preferred Reporting Items for Systematic Reviews and Meta-Analyses.
Study Characteristics
The included papers showed that Health EDMS research was conducted in the United States (n=5, 35.7%), the United Kingdom (n=2, 14.3%), China (n=2, 14.3%), Switzerland (n=1, 7.1%), France (n=1, 7.1%), Germany (n=1, 7.1%), South Korea (n=1, 7.1%), and Israel (n=1, 7.1%). Figure 3 shows the distribution of the papers by country.

Distribution of papers based on country.
From 2004 to 2022, 14 studies on user compliance with Health EDMS were found. There were relatively few studies before 2021. Research on Health EDMS compliance increased rapidly in 2021 (there were 7, 50.0%, studies) due to the COVID-19 pandemic. The COVID-19 pandemic has led to many studies on user compliance with CTAs, EDMSs for handling health-related emergencies and disasters.
Related to the sources of publications, 2 (14.3%) papers were published in the Journal of Medical Internet Research and 1 (7.1%) paper each was published in the following journals: MIS Quarterly , AIS Transactions on Replication Research , Applied Ergonomics , BMJ Open , Disaster Medicine and Public Health Preparedness , Disasters , Frontiers in Psychology , Human Factors , Humanities and Social Sciences Communications , International Journal of Disaster Risk Reduction , Public Health , and Public Relations Review . These papers used the quantitative research method (n=11, 78.6%), mixed method (n=1, 7.1%), and conceptual method (n=2, 14.3%). The papers that applied quantitative methods used surveys (n=7, 50.0%) and experiments (n=4, 28.6%) to collect data. The 1 (7.1%) paper that applied a mixed method used a combination of experiments (quantitative) and focus group discussions (qualitative). In addition, 2 (14.3%) conceptual papers developed arguments about concept associations.
Theoretical Lens of User Compliance with Health EDMS
The theories used in Health EDMS compliance research ( Table 7 ) include the protective action decision model (PADM), Etzioni's compliance theory, the Health Belief Model (HBM), protection motivation theory (PMT), social amplification of risk framework (SARF), extension of the technology acceptance model (TAM2), and the theory of planned behavior (TPB). Of the 14 papers in this review, 5 (35.7%) did not use any theory to analyze compliance factors, 2 (14.3) were conceptual papers [ 82 , 83 ], and the others applied selected concepts or themes from previous studies. To investigate compliance with Health EDMS, Freberg [ 84 ] examined the message source and message reliability; Albrecht et al [ 85 ] examined risk perception, risk preference, and social preference; and Panchal et al [ 86 ] examined security and privacy concerns, information content, usability, and knowledge of the application. Next, we elaborate on each of the theories used in Health EDMS compliance research.
Theoretical lenses to investigate compliance with Health EDMS a .
b PADM: protective action decision model.
c HBM: Health Belief Model.
d PMT: protection motivation theory.
e SARF: social amplification of risk framework.
f TAM2: extension of the technology acceptance model.
g TPB: theory of planned behavior.
Protective Action Decision Model
The PADM is a framework for managing the societal response to environmental hazards [ 51 ]. The PADM has been applied to 3 different areas: risk communication, evacuation modeling, and long-term hazard adjustment [ 51 ]. In the PADM, decision-making begins with environmental cues, social cues, and warnings [ 51 ]. Environmental cues are signs of threat obtained from the environment, such as sight, smell, or sound [ 51 ]. Social cues are signs obtained after observing the behavior of others [ 51 ]. A warning is a message sent from an information source through a channel to the recipient [ 51 ]. The 3 triggers encourage a pre–decision-making process involving exposure, attention, and comprehension [ 51 ]. Exposure measures whether people receive information, attention measures whether people care about the information, and comprehension measures whether people understand the information [ 51 ].
Furthermore, the pre–decision-making process generates perceptions of the environmental threats, alternative protective actions, and relevant stakeholders (government, other groups, and community) [ 51 ]. These perceptions provide the basis for protective action decision-making [ 51 ]. The dependent variable of the PADM is a behavioral response that is generally in the form of information seeking, protective responses, or emotion-focused coping [ 51 ]. The actual implementation of a response depends not only on people's intentions to act but also on the physical and social conditions that can hinder or facilitate the action [ 51 ]. The final stage in the PADM is the feedback loop [ 51 ]. People attempt to confirm or contradict every warning they receive, usually by searching for additional information from different sources and channels [ 51 ].
Stakeholder perceptions and protective action perceptions have been shown to influence compliance with warnings during the avian influenza A (H7N9) outbreak [ 87 ] and the COVID-19 pandemic [ 15 ]. Meanwhile, threat perception has not been shown to affect warning compliance based on COVID-19 research in Germany and China [ 15 , 52 ]. The use of protective measures can reduce threat appraisal, making people feel less likely to be infected. However, the duration of the pandemic, the extended and repeated warnings, and the familiar feeling of the disease resulted in information fatigue that undermined the perceived threat [ 52 ]. Among all the theories in Table 7 , the PADM is the only theory that focuses on the human response to threats [ 51 ]. The PADM also specifies prompts for action, which are important for encouraging engagement in health-related behaviors [ 87 ]. However, for slow-moving and long-term health-related hazards, the PADM has not been able to predict changes in threat perceptions.
Etzioni's Compliance Theory
Etzioni's compliance theory states that there are 2 parties to a compliance relationship: an actor who has power and another actor who responds to the power (subordinated actor) [ 91 ]. Power can be differentiated into 3 types according to the means used to make the subject comply [ 91 ]. Coercive power applies physical sanctions, force, and fear to control lower-level participants [ 91 ]. Remuneration power applies material resources and rewards to control others [ 91 ]. However, normative power is the provision and manipulation of symbolic rewards and deprivations [ 91 ]. The effectiveness of each power in obtaining the cooperation of subordinates depends on their involvement [ 91 ].
The subordinated actor can have 3 kinds of involvement: alienative, calculative, and moral [ 91 ]. Alienative involvement leads to intense negative orientation, while calculative involvement can lead to positive or negative orientation [ 91 ]. However, moral involvement, also known as commitment, leads to intensely positive orientation [ 91 ]. Moral involvement can be based on pure commitment (based on the internalization of norms) and social commitment (based on pressure from primary groups and their members) [ 91 ]. Subsequently, combining 3 types of power and 3 types of involvement produces 9 kinds of compliance [ 91 ]. However, 3 kinds of compliance are more frequently found in practice [ 91 ]. These are the relationships between coercive power and alienative involvement, remuneration power and calculative involvement, and normative power and moral involvement [ 91 ]. These compliance types are called congruent types [ 91 ]. This happens when the subordinate's involvement type is the same as the types that want to be generated by organization power [ 91 ]. The congruent types are more frequent because they are more effective to implement.
Etzioni's compliance theory has been adapted to campus emergencies [ 38 ] and health care–related emergencies [ 41 ]. Both studies were designed to accommodate emergency notifications sent by normative organizations, such as the campus and the government [ 38 , 41 ]. Normative power was adapted as the subjective norm, while coercive power and remuneration power were adapted as a perceived security threat and a perceived financial threat, respectively [ 38 , 41 ]. The results of the 2 studies are similar and show that subjective norms are the most important factor promoting immediate compliance in health-related hazard scenarios [ 38 , 41 ].
Health Belief Model
The HBM is a conceptual model for understanding why individuals do or do not perform various actions related to health behavior [ 92 ]. The HBM defines 6 different constructs. These constructs focus on individual representations of threat perceptions and evaluation of health behaviors [ 93 ]. Threat perception is defined as 2 central beliefs: perceived susceptibility to health problems and perceived severity of disease consequences [ 93 ]. Health behavioral evaluation also consists of 2 constructs: perceptions of the benefits or efficacy of the recommended health behaviors and perceptions related to the barriers to enacting these behaviors [ 93 ]. In addition, the HBM proposes that cues to action can activate health behaviors when individuals hold appropriate beliefs [ 92 ]. Cues to action can be individual perceptions of symptoms, social influences, and health education campaigns [ 93 ]. In a later version of the theory, individual health motivations are also included as constructs that drive health behavior actions [ 93 ].
The HBM has been used to analyze individual compliance with warnings to take recommended protective actions during the H7N9 [ 87 ] and COVID-19 [ 88 ] outbreaks. The perceived risk or perceived vulnerability positively influenced compliance intentions in the H7N9 outbreak [ 87 ]. Meanwhile, all HBM constructs except health motivation were used by Guillon and Kergall [ 88 ] and were shown to affect compliance to protective measures and CTAs during the COVID-19 pandemic. However, the HBM does not consider the effect of information on protective behavior, even though risk information has been seen as an essential driver of risk perception and behavioral response [ 87 ].
Protection Motivation Theory
In a hazardous event, the increased fear of the individual also increases their intention to take action [ 90 ]. The PMT describes the social and cognitive processes that underlie protective behavior [ 94 ]. The PMT proposes that people protect themselves based on threat appraisal and coping appraisal [ 94 ]. Threat appraisal refers to the perceived likelihood that the event will occur and have negative consequences [ 94 ]. Threat appraisal is a person's assessment of the estimated severity of the disease (perceived severity) and estimates the likelihood of contracting the disease (perceived susceptibility) [ 95 ]. In addition, the PMT also states that fear arousal indirectly affects attitudes and behavior changes through the perceived severity of danger [ 95 ]. However, coping appraisal consists of the individual's expectation that implementing the recommendations will eliminate the threat (response efficacy) and the belief that they can successfully carry out the recommended actions (self-efficacy) [ 95 ]. Thus, if individuals conclude that a threat will affect them, they will be more motivated to protect themselves and initiate or continue certain self-protective behaviors [ 94 ].
In Health EDMS compliance studies, the PMT has been used in campus emergency notification systems [ 90 ] and emergency alerts during the COVID-19 pandemic in South Korea [ 89 ]. However, the PMT constructs cannot explain rapid compliance with health advisory warnings on campus. Instead, the PMT can better explain compliance in fast-evolving scenarios, such as robberies, active shooters, and building fires. In the COVID-19 alert study, the PMT construct was used as a mediating factor between reading text messages and practicing preventive behaviors. Response efficacy was the only variable driving compliance to warnings, whereas the perceived risk was insignificant. Based on these 2 studies [ 89 , 90 ], the PMT constructs are less predictive of explaining compliance in slower-developing scenarios, such as health-related hazards.
Social Amplification of Risk Framework
SARF describes a dynamic process for understanding how risk is perceived when communicated to the community [ 15 ]. SARF conceptualizes that individual and social perceptions of risk and risk behavior can be shaped, enhanced, or attenuated when there is an interaction between hazardous events and psychological, social, institutional, and cultural processes [ 96 ]. In this case, the IS and the characteristics of the public response can form a social amplification that determines the nature and magnitude of the risk. ISs can amplify risk events in 2 ways: by intensifying or attenuating the signals that individuals and social groups receive about risk and by filtering out the many signals related to risk attributes and their importance [ 96 ].
Amplification occurs in 2 stages: when transferring information about the risk and when the community responds to the information [ 96 ]. Signals about risk are processed by individual and social amplification stations, including scientists communicating risk assessments, news media, cultural groups, and interpersonal networks [ 96 ]. Reinforced risk leads to a behavioral response, resulting in secondary impacts, such as financial loss, organizational change, and physical risk [ 96 ]. Communication is at the core of SARF, as individuals are most often exposed to risky information through the media or discussions with others [ 15 ]. In this manner, risk amplification allows different “amplification stations” to compete for public attention and influence how the public perceives and responds to risk [ 15 ].
SARF has been used to investigate compliance behavior in China during the COVID-19 pandemic [ 15 ]. One variable from SARF, namely information interaction, is used to examine how interactions between individuals can shape risk perceptions [ 15 ]. Information interaction significantly influences risk perception and preparedness intentions, while also serving as a mediator for warning [ 15 ]. Of the 2 stages of amplification in SARF, this study only analyzed amplification when the public responds to information [ 15 ]. Other SARF variables have not been found in Health EDMS compliance studies.
Extension of the Technology Acceptance Model
TAM2 extends the original TAM by including additional key determinants of perceived usefulness and usage intention constructs: social influence and cognitive instrumental process [ 97 ]. The social influence processes consist of subjective norms, voluntariness, and image [ 97 ]. Subjective norms are added as determinants considering that people can choose to perform a behavior if they believe their important references think they should [ 97 ]. TAM2 distinguishes between mandatory and voluntary use arrangements by adding voluntariness as a moderating variable [ 97 ]. In addition, TAM2 theorizes that social influences can affect how users judge the system's image (ie, whether the system can increase their status in a social system) [ 97 ]. The elevated status leads to increased power and influence that eventually provides a general basis for greater productivity (perceived usefulness) [ 97 ]. TAM2 also investigates how these determinants' effects change with increasing user experience over time with the target system [ 97 ]. As cognitive instrumental processes, TAM2 includes job relevance, output quality, and result demonstrability constructs. TAM2 shows that the influence of cognitive instrumental processes is not influenced by experience over time [ 97 ].
Research on CTAs in the United Kingdom found that most TAM2 constructs significantly affect user behavior to download the application and comply with notifications [ 27 ]. The trust factor was added and proved to be significant [ 27 ]. Compliance with the notification was fairly high, but there were issues surrounding trust and understanding of the application's features that hindered the adoption of the CTA [ 27 ]. Moreover, Dowthwaite et al [ 27 ] stated that users would likely delete the CTA when they are frustrated by a notification from the application (output quality) and do not understand how their data would be used (result demonstrability). Although TAM2 has described the factors that influence compliance with Health EDMS, it is still unclear what level of experience, output quality, and result demonstrability can positively affect compliance.
Theory of Planned Behavior
The TPB was first introduced as a development of the theory of reasoned action (TRA) and has been most widely adopted in the research on motivations for human behavior. The TPB explains that behavior is influenced by intention and intention is determined by 3 types of beliefs: attitudes, subjective norms, and perceived behavioral control [ 98 ]. Attitude is a comprehensive evaluation of the implementation of behavior [ 98 ]. Subjective norms are individual perceptions of other people's expectations considered important about certain behaviors [ 98 ]. Meanwhile, perceived behavioral control is an individual's perception of how easy or difficult it is to do something [ 98 ]. It can also be interpreted as the resources and opportunities available to a person to encourage them to perform a behavior [ 98 ]. The TPB has been applied in studies of ISs, organizations, and user populations.
The TPB is 1 of the most influential theories in disaster and emergency preparedness planning. The TPB has been used to analyze the factors that affect rapid compliance with emergency notifications in 7 emergency scenarios in a campus: robbery, active shooter, building fire, hazardous material, riot/violent protest, air quality advisory, and health advisory [ 90 ]. Attitude significantly affected compliance across all scenarios, while subjective norms significantly affected almost all scenarios except the active shooter [ 90 ]. However, perceived behavioral control was only significant in the air quality advisory scenario [ 90 ].
Factors Affecting User Compliance With Health EDMS
This section summarizes the factors affecting compliance with Health EDMS from the selected papers. People can comply immediately or verify and then comply after receiving a warning [ 38 ]. Verification is performed by contacting other people or seeking information from other media and channels [ 41 ]. In this case, various features of Health EDMS, such as warning and notification (RS2.2), interactive messaging (RS2.3), or video and media (RS2.3), can facilitate remote verification to increase compliance levels [ 41 ]. Other technological factors, such as system design, including communication channels, public education, usability, and information content, also affect warning compliance [ 78 ].
In addition, individual characteristics and social influence play a significant role in driving compliance [ 78 , 85 , 90 ]. The individual evaluates the situation to build their belief about whether compliance with an emergency message yields a valuable outcome [ 90 ]. They also consider their expectations from influential people in their lives, social pressures, and cultural norms about behavior [ 90 ]. The factors affecting compliance with Health EDMS can be categorized into 3 main groups: individual, technology, and social.
Sorensen [ 79 ] stated that warning compliance is influenced by sender and receiver factors, situational factors, and social contacts. Upon receiving the warning, individuals make decisions based on their judgment of the message source, their ability to understand the message and implement countermeasures, and the personal situation they experienced. The sender and receiver are included in the category of individual factors. Moreover, compliance results from interactions between users and other parties who provide warnings. In this study, the interaction is described in the form of social factors consisting of situational factors and social contact. Sorensen [ 79 ] also stated that system design can affect the response to warnings.
From the literature reviewed, our study identified 14 individual, 10 technological, and 4 social factors that influenced users' compliance with Health EDMS. Most of them were derived from the previously discussed behavior theories, while others were added by the researchers without referring to any particular theory. From individual factors, perceived risk and response efficacy are the most widely used predictors of compliance. From technological factors, the most frequently used factors are warning message characteristics. Moreover, subjective norms and stakeholder perception are the most researched social factors. A summary table of the individual, technological, and social factors influencing users' compliance with Health EDMS is provided in Multimedia Appendix 4 .
Principal Findings
EDM is 1 of the most challenging management tasks because decisions must be made in a short time, under a rapidly changing environment, with unique situations for each incident, and involve high operational costs [ 64 ]. In this case, an IS (EDMS) can be used to support decision-making. Each stage in EDM (ie, mitigation, preparedness, response, and recovery) requires a different type of EDMS feature in terms of the problem to be solved, the stakeholders involved, the need to provide real-time data, presentation of the data to users, and the technological sophistication required [ 64 ]. This research mapped EDMS features from various literatures into the main activities at each EDM stage. From the 4 stages of EDM, 19 main activities and 42 EDMS features were identified ( Tables 1 - -4). 4 ). This study also identified stakeholder roles for each feature using the RACI matrix, which will be helpful during EDMS development. The list of features presented in this study is expected to guide governments and system developers in designing a comprehensive and integrated EDMS.
This study mapped the EDMS features into CTA functionalities. Contact-tracing strategies have been implemented worldwide, with varying degrees of success [ 86 ]. In 2020, months after COVID-19 spread, CTAs were launched in more than 50 countries [ 72 ], both official and unofficial, to respond to the pandemic. This study found that at the most, a CTA implements 11 EDMS features for the response and recovery phases. For the response phase, a CTA provides features to monitor and report ongoing hazards, disseminate notifications and alerts, provide information to the public, manage request data, manage contact-tracing data, manage medical care, and manage laboratory test data. When the number of infected cases decreases and activities return to normal, a CTA can still be used in the recovery phase to support ongoing medical care and monitoring activities. An important feature that needs to be implemented in the recovery phase is the management of lessons learned data. The lessons learned can provide insight into the use of Health EDMS in the response stage [ 63 ], especially for new infectious diseases that have not been previously identified. This feature will be beneficial in determining future strategies and policies. A CTA was only implemented when the disease had spread, so there were no mitigation and preparedness functions. The system should also be equipped with these functions for further development to enable early strategic planning and build sufficient capacity for dealing with the health-related hazard.
Rapid compliance with warnings is vital to save lives. The features of Health EDMS can provide information and warnings that increase the sense of urgency and become a source of verification to improve compliance levels [ 41 ]. Through a systematic literature review using the PRISMA method, this study classified factors that determine compliance with Health EDMS into individual, technological, and social factors. Based on the analysis and synthesis of the literature, we proposed a research framework showing the relationship between the influencing factors of Health EDMS feature use and design and user compliance with Health EDMS ( Figure 4 ). The framework shows that individual, technological, and social factors influence the use and design of surveillance and reporting features, and medical care and logistical support features. Appropriate use of these Health EDMS features during health disaster events affects user compliance with Health EDMS.

Proposed research framework. EDMS: emergency and disaster management system.
In the previous explanation ( Table 5 ), 11 features have been implemented in Health EDMS, mainly for surveillance and reporting ongoing hazards and providing medical care and logistical support. Individual, technological, and social factors influence the use of these features. Individual factors are related to perceptions of health risks, experiences, attitudes, and perceptions of Health EDMS. The perceived probability and consequences of contracting a disease can encourage someone to take protective actions in Health EDMS [ 85 , 88 ]. Individuals who feel the threat of an illness will seek more information about it and will always be aware of warnings and notifications from the system. Therefore, individual factors influence the use of surveillance and monitoring features of Health EDMS. During the COVID-19 pandemic, the perception of risk also encouraged the public to carry out laboratory tests and self-quarantine to ensure they did not contract the disease or infect other people [ 88 ]. They were self-encouraged to take preventive measures, such as testing, medical treatment, and requesting logistical assistance through Health EDMS.
Technological factors cover all aspects of information and technical quality. System notifications and warnings can change how individuals think and feel about threats and risks. Health EDMS must provide sufficient information to users about their possible risk exposure and the actions they should take [ 86 ]. The information quality of the system will drive the use of both feature sets in Health EDMS (ie, surveillance and monitoring, and medical care and logistical support). In addition, if users believe that Health EDMS is easy to use, relevant to their needs, and protects their privacy, they will be encouraged to use the system [ 27 , 86 ].
In addition to technological and individual factors, the use and design of Health EDMS are influenced by social factors. Opinions from influential people, such as family, friends, or coworkers, encourage individuals to use Health EDMS [ 90 ], for example, seeking information about hazards and viewing daily case reports. Moreover, users who believe that Health EDMS stakeholders have the expertise to manage hazards will use Health EDMS as their primary source of information and warnings [ 15 ]. Users will also ask for medical and logistical assistance through Health EDMS if they believe the stakeholders can respond quickly and effectively [ 15 ]. In designing Health EDMS, social factors can be supported by allowing users to share information to social media or messaging applications. Health EDMS must also highlight the ability of the stakeholders to overcome the health hazards [ 15 ]. Frequent use of the surveillance and monitoring features as well as the medical and logistical assistance features of Health EDMS may increase compliance. User compliance analyzed in previous research is limited to the intention to comply, not actual compliance. Future research needs to examine actual compliance to produce stronger evidence on the causal factors and effective interventions in this area.
The proposed research framework was developed based on our analysis of theories and corresponding factors affecting user compliance with Health EDMS in the existing literature. Future research can empirically examine the framework with a specific health EDMS. Collaborations with CTA providers are needed to examine actual compliance. In addition to the proposed research framework, we proposed 2 research topics on Health EDMS that require further investigation. First, Health EDMS has only implemented features to handle the response and recovery phases of EDM. The mitigation and preparedness phases of EDM are not supported by Health EDMS. Health EDMS should not only be used to respond to and cope with disasters but also be used to prevent and prepare for health-related hazards. Nevertheless, unlike natural disasters or human-induced disasters, such as forest fires, the characteristics of future health-related hazards are unknown. How to design features for mitigation and preparedness of future health-related hazards is an interesting research topic. Second, considering that a CTA is a relatively new IS implemented for a specific pandemic case, the effectiveness of CTA features in increasing user compliance with warnings has not been widely analyzed. Instead of examining a CTA as a whole, future research should investigate each feature of a CTA in depth toward increasing user compliance.
Health EDMS has been implemented to cope with health-related hazards. However, the compliance rate with Health EDMS remains low [ 27 , 41 ]. This study reviewed previous research on user compliance with Health EDMS based on PRISMA reporting guidelines. Research on this topic increased rapidly in 2021 due to the COVID-19 pandemic. This study identified 7 theories researchers adopted when examining Health EDMS compliance behavior: PADM, Etzioni's compliance theory, HBM, PMT, SARF, TAM2, and TPB. This study also discussed the individual, technological, and social factors influencing the use of 2 Health EDMS features (ie, surveillance and reporting features, and medical care and logistical support features). During health-related hazards, community users play an important role in actively reporting disaster victims and missing persons, report tracing data and lab results, report damage to infrastructure, and request medical assistance and treatment. Community users who actively use the surveillance and reporting features as well as the medical care and logistical support features will be encouraged to comply with the protocols and measures to deal with the health disaster. An in-depth understanding of user compliance before designing a health EDMS is essential for governments and developers to increase the effectiveness of Health EDMS implementation.
Acknowledgments
This work is supported by the Penelitian Dasar Unggulan Perguruan Tinggi (PDUPT) grant from the Ministry of Education, Culture, Research, and Technology, Republic of Indonesia (contract number: NKB-905/UN2.RST/HKP.05.00/2022).
Abbreviations
Multimedia appendix 1, multimedia appendix 2, multimedia appendix 3, multimedia appendix 4, data availability.
Conflicts of Interest: None declared.
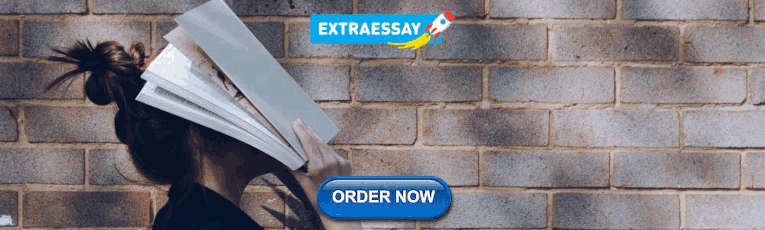
COMMENTS
The purpose of this review was to summarize and synthesize the literature that examined resilience in the context of emergency management (EM). Four groups of findings were synthesized: definitions, key dimensions, technical tools, and research settings employed in the research.
Focus on phases in disaster management literature. Looking back, moving forward: Reflections on future research ... Governance, and Public Management Review. Search terms were CRISIS or DISASTER, and the search engine used was Web of Science. The results show 330 articles in 2020; 333 in 2010, 111 in 2000; and 124 in 1990. ...
Through the literature review, we find that few scholars use bibliometrics and social network analysis to systematically study the field of emergency response. ... An effective emergency management information system can make accurate judgment and assessment of the disaster situation and vehicle location in the shortest time and provide ...
A literature review is a synthesis of published information on a particular research topics. The purpose is to map out what is already known about a certain subject, outline methods previously used, prevent duplication of research, and, along these lines, reveal gaps in existing literature to justify the research project.
It focused on incorporating emergency management knowledge that existed in the local level and on improving coping capacities to manage known risks ... For this literature review, ∼150 academic papers from 2012 to 2017 1 were identified from Google Scholar. This selection was based on the search term "disaster (prepar* ...
This is a systematic review of emergency management publications using the bibliometric analysis. The authors addressed the questions of how emergency management research has evolved in connections to previous literature, what are the fundamental elements of effective strategies, and what topics emerged as new trends in adapting to changing environments.
The purpose of this review was to summarize and synthesize the literature that examined resilience in the context of emergency management (EM). Four groups of findings were synthesized: definitions, key dimensions, technical tools, and research settings employed in the research. First, definitions of resilience, improvisation, and adaptation ...
With these words, a recent literature review on resilience in Emergency Management (Son et al., 2020) opens a discussion on cornerstones and future research streams of the field. In this research ...
The research of emergency management networks (EMNs) attracted much attention in recent years. However, it is lack of a systematic study to review these results. This paper aims to review publications related to EMNs based on a three-stage research framework. Then, the main research status, research methods and research topics were determined through scientometric analysis and qualitative ...
6 CHAPTER 2 LITERATURE REVIEW As part of the Task 2 Literature Review, the research team conducted a focused review of recent domestic and international research on emergency management and emergency response within the all-hazards context.
Systematic Literature Review. Based on the systematic research methodology (Ghobadi 2015) which is considered as a means of identifying, evaluating and interpreting all available research relevant to a particular research question, or topic area, or phenomenon of interest (Budgen and Brereton 2006), this study processes three phases of work, including planning, conducting, and reporting.
The Most Important Thing You Can Do for Yourself and Your EM Team. Published bi-monthly, every issue of the Journal of Emergency Management is peer-reviewed and packed with invaluable information and insight. Topics include: Emergency planning and response. Disaster recovery and business continuity planning. Emergency preparedness and response ...
A review of the "grey literature" in Google Scholar (Google Inc.; Mountain View, California USA) was conducted using the same search terms (Table 2). This literature review was also informed by a consideration of emergency services literature, policy, and non-peer-reviewed professional journals or publications and non-medical media.
A literature review and environmental scan were conducted to identify domestic activities, programs, or initiatives that exemplify the whole community approach. ... The findings also support and validate the six strategic themes outlined in FEMA's Whole Community Approach to Emergency Management. 11 The findings, like the whole community ...
DOI: 10.1016/J.IJDRR.2018.01.006 Corpus ID: 169380863; Social media applications and emergency management: A literature review and research agenda @article{Luna2018SocialMA, title={Social media applications and emergency management: A literature review and research agenda}, author={Sergio Luna and Michael J. Pennock}, journal={International Journal of Disaster Risk Reduction}, year={2018}, url ...
a literature review in response to Task Order 07EASPE000074 to identify promising risk communication approaches and messaging strategies that address the communication limitations or barriers facing vulnerable populations before, during, and after a public health emergency. Our review (Task 3) had three aims: 1.
So far, although both computational intelligence and emergency management have attracted considerable attention in their research areas, little effort Chen and Chen was devoted to the systemic literature review of computational intelligence technology application in emergency management. There is an urgent necessity to review the development of ...
The Department of Veterans Affairs (VA) is a key player in emergency management for both veterans and civilians. Unfortunately, limited evidence-based research findings exist regarding the role and experience of VA during disasters. The present study is a systematic literature review of 41 published, peer-reviewed articles regarding VA and ...
Healthcare Management: A Review of the Literature Naomi Alanis, MS, MHA, MBA, & Hao Wang, MD, PhD, Texas Christian University, Burnett School of Medicine References 1.Mueller B, Kinoshita T, Peebles A, Graber MA, Lee S: Artificial intelligence and machine learning in emergency medicine: a narrative review. Acute Med Surg 2022, 9(1):e740.
The management of hemostasis in patients medicated with apixaban (Eliquis) undergoing emergency cardiac surgery is exceedingly difficult. The body's natural elimination pathways for apixaban prove ineffective in emergency situations, and the impact of hemodialysis is limited. The application of Cytosorb® may attenuate the concentration of apixaban, thereby facilitating the stabilization of ...
Historical analysis of emergency management in the United States shows that the concept of emergency management has evolved over time, and primarily a disaster-driven approach has been adopted. Social media. The first social media application, SixDegrees.com, was introduced in 1997, reaching an estimate of 3.5 million registered users [40].
The term "emergency management system" is used by the United Nations and Landau , which refers to a system that works for emergency planning, control ... A systematic literature review is a secondary study conducted to identify, evaluate, and interpret all available research relevant to a particular research question, ...