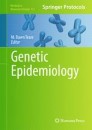
Genetic Epidemiology pp 227–237 Cite as
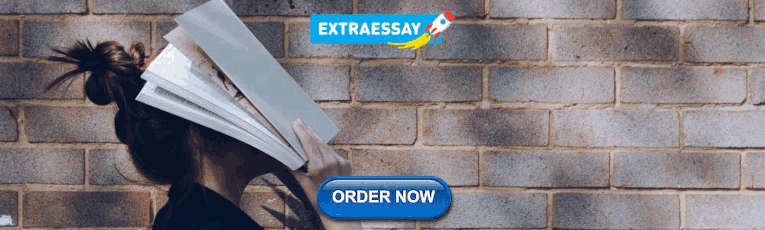
The Genetic Epidemiology of Obesity: A Case Study
- Laura M. Johnson 2
- First Online: 20 November 2010
3356 Accesses
3 Citations
Part of the book series: Methods in Molecular Biology ((MIMB,volume 713))
Obesity (OMIM #601665) is a disease where excessive stores of body fat impact negatively on health. The first law of thermodynamics dictates that energy cannot be created or destroyed so if energy is taken into the body, but not transformed to ATP for metabolic work or dissipated as heat, it will be stored as fat. Therefore, the ultimate cause of obesity is a long-term positive energy imbalance [energy intake (EI) exceeds energy expenditure (EE)]. Despite this simple explanation, there is no single reason why EI may exceed EE meaning that the proximate causes of obesity are multi-factorial in origin involving a complex interplay of genetic, behavioural, and environmental influences on metabolism, diet, and activity.
This is a preview of subscription content, log in via an institution .
Buying options
- Available as PDF
- Read on any device
- Instant download
- Own it forever
- Available as EPUB and PDF
- Compact, lightweight edition
- Dispatched in 3 to 5 business days
- Free shipping worldwide - see info
- Durable hardcover edition
Tax calculation will be finalised at checkout
Purchases are for personal use only
Springer Nature is developing a new tool to find and evaluate Protocols. Learn more
World Health Organization (WHO) (2008) Obesity. In: World Health Organization (WHO), editor. Health topics. Geneva: World Health Organisation (WHO).
Google Scholar
Ellis KJ (2000) Human body composition: in vivo methods. Physiol Rev 80: 649–680.
PubMed CAS Google Scholar
Heitmann BL, Erikson H, Ellsinger BM, Mikkelsen KL, Larsson B (2000) Mortality associated with body fat, fat-free mass and body mass index among 60-year-old Swedish men-a 22-year follow-up. The study of men born in 1913. Int J Obes Relat Metab Disord 24: 33–37.
Article PubMed CAS Google Scholar
Allison DB, Zhu SK, Plankey M, Faith MS, Heo M (2002) Differential associations of body mass index and adiposity with all-cause mortality among men in the first and second National Health and Nutrition Examination Surveys (NHANES I and NHANES II) follow-up studies. Int J Obes Relat Metab Disord 26: 410–416.
Deurenberg P, Weststrate JA, Seidell JC (1991) Body mass index as a measure of body fatness: age- and sex-specific prediction formulas. Br J Nutr 65: 105–114.
Kyle UG, Schutz Y, Dupertuis YM, Pichard C (2003) Body composition interpretation. Contributions of the fat-free mass index and the body fat mass index. Nutrition 19: 597–604.
Article PubMed Google Scholar
Demerath EW, Schubert CM, Maynard LM, Sun SS, Chumlea WC, et al. (2006) Do changes in body mass index percentile reflect changes in body composition in children? Data from the Fels Longitudinal Study. Pediatrics 117: e487–e495.
Prentice AM, Jebb SA (2001) Beyond body mass index. Obes Rev 2: 141–147.
Allison DB, Kaprio J, Korkeila M, Koskenvuo M, Neale MC, et al. (1996) The heritability of body mass index among an international sample of monozygotic twins reared apart. Int J Obes Relat Metab Disord 20: 501–506.
Korkeila M, Kaprio J, Rissanen A, Koskenvuo M (1991) Effects of gender and age on the heritability of body mass index. Int J Obes 15: 647–654.
Price RA, Gottesman II (1991) Body fat in identical twins reared apart: roles for genes and environment. Behav Genet 21: 1–7.
Slattery ML, Bishop DT, French TK, Hunt SC, Meikle AW, et al. (1988) Lifestyle and blood pressure levels in male twins in Utah. Genet Epidemiol 5: 277–287.
Vogler GP, Sorensen TI, Stunkard AJ, Srinivasan MR, Rao DC (1995) Influences of genes and shared family environment on adult body mass index assessed in an adoption study by a comprehensive path model. Int J Obes Relat Metab Disord 19: 40–45.
Rice T, Bouchard C, Perusse L, Rao DC (1995) Familial clustering of multiple measures of adiposity and fat distribution in the Quebec Family Study: a trivariate analysis of percent body fat, body mass index, and trunk-to-extremity skinfold ratio. Int J Obes Relat Metab Disord 19: 902–908.
Rice T, Daw EW, Gagnon J, Bouchard C, Leon AS, et al. (1997) Familial resemblance for body composition measures: the HERITAGE Family Study. Obes Res 5: 557–562.
Faith MS, Pietrobelli A, Nunez C, Heo M, Heymsfield SB, et al. (1999) Evidence for independent genetic influences on fat mass and body mass index in a pediatric twin sample. Pediatrics 104: 61–67.
Rice T, Despres JP, Daw EW, Gagnon J, Borecki IB, et al. (1997) Familial resemblance for abdominal visceral fat: the HERITAGE family study. Int J Obes Relat Metab Disord 21: 1024–1031.
Nguyen TV, Howard GM, Kelly PJ, Eisman JA (1998) Bone mass, lean mass, and fat mass: same genes or same environments? Am J Epidemiol 147: 3–16.
Rankinen T, Zuberi A, Chagnon YC, Weisnagel SJ, Argyropoulos G, et al. (2006) The human obesity gene map: the 2005 update. Obesity (Silver Spring) 14: 529–644.
Article Google Scholar
Saunders CL, Chiodini BD, Sham P, Lewis CM, Abkevich V, et al. (2007) Meta-analysis of genome-wide linkage studies in BMI and obesity. Obesity 15: 2263–2275.
Bouchard C, Perusse L, Rice T, Rao DC (1998) The Genetics of Human Obesity. In: Bray GA, Bouchard C, James WPT, editors. Handbook of obesity. New York: Marcel Dekker. pp. 157–190.
Farooqi IS, O’Rahilly S (2005) Monogenic obesity in humans. Annu Rev Med 56: 443–458.
Farooqi IS, Keogh JM, Yeo GS, Lank EJ, Cheetham T, et al. (2003) Clinical spectrum of obesity and mutations in the melanocortin 4 receptor gene. N Engl J Med 348: 1085–1095.
Geller F, Reichwald K, Dempfle A, Illig T, Vollmert C, et al. (2004) Melanocortin-4 receptor gene variant I103 is negatively associated with obesity. Am J Hum Genet 74: 572–581.
Heid IM, Vollmert C, Hinney A, Doring A, Geller F, et al. (2005) Association of the 103I MC4R allele with decreased body mass in 7937 participants of two population based surveys. J Med Genet 42: e21.
Young EH, Wareham NJ, Farooqi S, Hinney A, Hebebrand J, et al. (2007) The V103I polymorphism of the MC4R gene and obesity: population based studies and meta-analysis of 29 563 individuals. Int J Obes (Lond) 31: 1437–1441.
Article CAS Google Scholar
Loos RJ, Lindgren CM, Li S, Wheeler E, Zhao JH, et al. (2008) Common variants near MC4R are associated with fat mass, weight and risk of obesity. Nat Genet 40: 768–775.
Frayling TM, Timpson NJ, Weedon MN, Zeggini E, Freathy RM, et al. (2007) A common variant in the FTO gene is associated with body mass index and predisposes to childhood and adult obesity. Science 316(5826): 889–894. DOI: 10.1126/science.1141634.
Peeters A, Beckers S, Verrijken A, Roevens P, Peeters P, et al. (2008) Variants in the FTO gene are associated with common obesity in the Belgian population. Mol Genet Metab 93: 481–484.
Wardle J, Carnell S, Haworth CM, Farooqi IS, O’Rahilly S, et al. (2008) Obesity-associated genetic variation in FTO is associated with diminished satiety. J Clin Endocrinol Metab 93(9): 3640–3643.
Hinney A, Nguyen TT, Scherag A, Friedel S, Bronner G, et al. (2007) Genome wide association (GWA) study for early onset extreme obesity supports the role of fat mass and obesity associated gene (FTO) variants. PLoS One 2: e1361.
Andreasen CH, Stender-Petersen KL, Mogensen MS, Torekov SS, Wegner L, et al. (2008) Low physical activity accentuates the effect of the FTO rs9939609 polymorphism on body fat accumulation. Diabetes 57: 95–101.
Lopez-Bermejo A, Petry CJ, Diaz M, Sebastiani G, de Zegher F, et al. (2008) The association between the FTO gene and fat mass in humans develops by the postnatal age of two weeks. J Clin Endocrinol Metab 93: 1501–1505.
Speakman JR, Rance KA, Johnstone AM (2008) Polymorphisms of the FTO gene are associated with variation in energy intake, but not energy expenditure. Obesity (Silver Spring) 16(8): 1961–1965.
Price RA, Li WD, Zhao H (2008) FTO gene SNPs associated with extreme obesity in cases, controls and extremely discordant sister pairs. BMC Med Genet 9: 4.
Hunt SC, Stone S, Xin Y, Scherer CA, Magness CL, et al. (2008) Association of the FTO gene with BMI. Obesity (Silver Spring) 16: 902–904.
Li H, Wu Y, Loos RJ, Hu FB, Liu Y, et al. (2008) Variants in the fat mass- and obesity-associated (FTO) gene are not associated with obesity in a Chinese Han population. Diabetes 57: 264–268.
Scuteri A, Sanna S, Chen WM, Uda M, Albai G, et al. (2007) Genome-wide association scan shows genetic variants in the FTO gene are associated with obesity-related traits. PLoS Genet 3: e115.
Horikoshi M, Hara K, Ito C, Shojima N, Nagai R, et al. (2007) Variations in the HHEX gene are associated with increased risk of type 2 diabetes in the Japanese population. Diabetologia 50: 2461–2466.
Ohashi J, Naka I, Kimura R, Natsuhara K, Yamauchi T, et al. (2007) FTO polymorphisms in oceanic populations. J Hum Genet 52: 1031–1035.
Gerken T, Girard CA, Tung YC, Webby CJ, Saudek V, et al. (2007) The obesity-associated FTO gene encodes a 2-oxoglutarate-dependent nucleic acid demethylase. Science 318: 1469–1472.
Fredriksson R, Hagglund M, Olszewski PK, Stephansson O, Jacobsson JA, et al. (2008) The obesity gene, FTO, is of ancient origin, up-regulated during food deprivation and expressed in neurons of feeding-related nuclei of the brain. Endocrinology 149: 2062–2071.
Wahlen K, Sjolin E, Hoffstedt J (2008) The common rs9939609 gene variant of the fat mass- and obesity-associated gene FTO is related to fat cell lipolysis. J Lipid Res 49: 607–611.
Kurokawa N, Young EH, Oka Y, Satoh H, Wareham NJ, et al. (2008) The ADRB3 Trp64Arg variant and BMI: a meta-analysis of 44 833 individuals. Int J Obes (Lond) 32(8): 1240–1249.
Masud S, Ye S (2003) Effect of the peroxisome proliferator activated receptor-gamma gene Pro12Ala variant on body mass index: a meta-analysis. J Med Genet 40: 773–780.
Ottman R (1996) Gene–environment interaction: definitions and study designs. Prev Med 25: 764–770.
Ochoa MC, Marti A, Azcona C, Chueca M, Oyarzabal M, et al. (2004) Gene-gene interaction between PPAR gamma 2 and ADR beta 3 increases obesity risk in children and adolescents. Int J Obes Relat Metab Disord 28(Suppl 3): S37–S41.
Hsueh W-C, Cole SA, Shuldiner AR, Beamer BA, Blangero J, et al. (2001) Interactions between variants in the β3-adrenergic receptor and peroxisome proliferator-activated receptor-γ2 genes and obesity. Diab Care 24(4): 672–677.
Johnson L, van Jaarsveld CHM, Emmett PM, Rogers IM, Hattersley AT, et al. (2009) Dietary energy density affects fat mass in early adolescence and is not modified by FTO variants. PLoS One 4: e4594.
Marti A, Martinez-Gonzalez MA, Martinez JA (2008) Interaction between genes and lifestyle factors on obesity. Proc Nutr Soc 67: 1–8.
Robitaille J, Despres JP, Perusse L, Vohl MC (2003) The PPAR-gamma P12A polymorphism modulates the relationship between dietary fat intake and components of the metabolic syndrome: results from the Quebec Family Study. Clin Genet 63: 109–116.
Memisoglu A, Hu FB, Hankinson SE, Manson JE, De Vivo I, et al. (2003) Interaction between a peroxisome proliferator-activated receptor γ gene polymorphism and dietary fat intake in relation to body mass. Hum Mol Genet 12: 2923–2929. DOI: 10.1093/hmg/ddg318.
Meirhaeghe A, Helbecque N, Cottel D, Amouyel P (1999) β2-Adrenoceptor gene polymorphism, body weight, and physical activity. Lancet 353: 896.
Boks MP, Schipper M, Schubart CD, Sommer IE, Kahn RS, et al. (2007) Investigating gene environment interaction in complex diseases: increasing power by selective sampling for environmental exposure. Int J Epidemiol 36: 1363–1369.
Hunter DJ (2005) Gene–environment interactions in human diseases. Nat Rev Genet 6: 287–298.
Download references
Author information
Authors and affiliations.
Cancer Research UK Health Behaviour Research Centre, Department of Epidemiology and Public Health, University College London, London, UK
Laura M. Johnson
You can also search for this author in PubMed Google Scholar
Corresponding author
Correspondence to Laura M. Johnson .
Editor information
Editors and affiliations.
School of Health and Related Research, Health Services Research, University of Sheffield, Regent Street 30, Sheffield, S1 4DA, United Kingdom
M. Dawn Teare
Rights and permissions
Reprints and permissions
Copyright information
© 2011 Springer Science+Business Media, LLC
About this protocol
Cite this protocol.
Johnson, L.M. (2011). The Genetic Epidemiology of Obesity: A Case Study. In: Teare, M. (eds) Genetic Epidemiology. Methods in Molecular Biology, vol 713. Humana Press, Totowa, NJ. https://doi.org/10.1007/978-1-60327-416-6_17
Download citation
DOI : https://doi.org/10.1007/978-1-60327-416-6_17
Published : 20 November 2010
Publisher Name : Humana Press, Totowa, NJ
Print ISBN : 978-1-60327-415-9
Online ISBN : 978-1-60327-416-6
eBook Packages : Springer Protocols
- Publish with us
Policies and ethics
- Find a journal
- Track your research
The genetic epidemiology of obesity: a case study
Affiliation.
- 1 Cancer Research UK Health Behaviour Research Centre, Department of Epidemiology and Public Health, University College London, London, UK. [email protected]
- PMID: 21153623
- DOI: 10.1007/978-1-60327-416-6_17
Obesity (OMIM #601665) is a disease where excessive stores of body fat impact negatively on health. The first law of thermodynamics dictates that energy cannot be created or destroyed so if energy is taken into the body, but not transformed to ATP for metabolic work or dissipated as heat, it will be stored as fat. Therefore, the ultimate cause of obesity is a long-term positive energy imbalance [energy intake (EI) exceeds energy expenditure (EE)]. Despite this simple explanation, there is no single reason why EI may exceed EE meaning that the proximate causes of obesity are multi-factorial in origin involving a complex interplay of genetic, behavioural, and environmental influences on metabolism, diet, and activity.
- Body Mass Index
- Chromosome Mapping
- Genome-Wide Association Study
- Models, Genetic
- Molecular Epidemiology / methods*
- Obesity / epidemiology*
- Obesity / etiology
- Obesity / genetics*
Europe PMC requires Javascript to function effectively.
Either your web browser doesn't support Javascript or it is currently turned off. In the latter case, please turn on Javascript support in your web browser and reload this page.
Search life-sciences literature (43,961,101 articles, preprints and more)
- Free full text
- Citations & impact
- Similar Articles
The genetics of obesity: from discovery to biology.
Author information, affiliations, orcids linked to this article.
- Yeo GSH | 0000-0001-8823-3615
- Loos RJF | 0000-0002-8532-5087
Nature reviews. Genetics , 23 Sep 2021 , 23(2): 120-133 https://doi.org/10.1038/s41576-021-00414-z PMID: 34556834 PMCID: PMC8459824
Abstract
Free full text .

The genetics of obesity: from discovery to biology
Ruth j. f. loos.
1 Novo Nordisk Foundation Center for Basic Metabolic Research, University of Copenhagen, Copenhagen, Denmark
2 Charles Bronfman Institute for Personalized Medicine, Icahn School of Medicine at Mount Sinai, New York, NY USA
3 Mindich Child Health and Development Institute, Icahn School of Medicine at Mount Sinai, New York, NY USA
4 Department of Environmental Medicine and Public Health, Icahn School of Medicine at Mount Sinai, New York, NY USA
Giles S. H. Yeo
5 MRC Metabolic Diseases Unit, University of Cambridge Metabolic Research Laboratories, Wellcome-MRC Institute of Metabolic Science, Addenbrooke’s Hospital, Cambridge, UK
- Associated Data
The prevalence of obesity has tripled over the past four decades, imposing an enormous burden on people’s health. Polygenic (or common) obesity and rare, severe, early-onset monogenic obesity are often polarized as distinct diseases. However, gene discovery studies for both forms of obesity show that they have shared genetic and biological underpinnings, pointing to a key role for the brain in the control of body weight. Genome-wide association studies (GWAS) with increasing sample sizes and advances in sequencing technology are the main drivers behind a recent flurry of new discoveries. However, it is the post-GWAS, cross-disciplinary collaborations, which combine new omics technologies and analytical approaches, that have started to facilitate translation of genetic loci into meaningful biology and new avenues for treatment.
- Introduction
Obesity is associated with premature mortality and is a serious public health threat that accounts for a large proportion of the worldwide non-communicable disease burden, including type 2 diabetes, cardiovascular disease, hypertension and certain cancers 1 , 2 . Mechanical issues resulting from substantially increased weight, such as osteoarthritis and sleep apnoea, also affect people’s quality of life 3 . The impact of obesity on communicable disease, in particular viral infection 4 , has recently been highlighted by the discovery that individuals with obesity are at increased risk of hospitalization and severe illness from COVID-19 (refs 5 – 7 ).

Although changes in the environment have undoubtedly driven the rapid increase in prevalence, obesity results from an interaction between environmental and innate biological factors. Crucially, there is a strong genetic component underlying the large interindividual variation in body weight that determines people’s response to this ‘obesogenic’ environment . Twin, family and adoption studies have estimated the heritability of obesity to be between 40% and 70% 9 , 10 . As a consequence, genetic approaches can be leveraged to characterize the underlying physiological and molecular mechanisms that control body weight.
Classically, we have considered obesity in two broad categories (Fig. 2 ): so-called monogenic obesity , which is inherited in a Mendelian pattern, is typically rare, early-onset and severe and involves either small or large chromosomal deletions or single-gene defects; and polygenic obesity (also known as common obesity), which is the result of hundreds of polymorphisms that each have a small effect. Polygenic obesity follows a pattern of heritability that is similar to other complex traits and diseases. Although often considered to be two distinct forms, gene discovery studies of monogenic and polygenic obesity have converged on what seems to be broadly similar underlying biology. Specifically, the central nervous system (CNS) and neuronal pathways that control the hedonic aspects of food intake have emerged as the major drivers of body weight for both monogenic and polygenic obesity. Furthermore, early evidence shows that the expression of mutations causing monogenic obesity may — at least in part — be influenced by the individual’s polygenic susceptibility to obesity 11 .

Key features of monogenic and polygenic forms of obesity .
In this Review, we summarize more than 20 years of genetic studies that have characterized the molecules and mechanisms that control body weight, specifically focusing on overall obesity and adiposity, rather than fat distribution or central adiposity. Although most of the current insights into the underlying biology have been derived from monogenic forms of obesity, recent years have witnessed several successful variant-to-function translations for polygenic forms of obesity. We also explore how the ubiquity of whole-exome sequencing (WES) and genome sequencing has begun to blur the line that used to demarcate the monogenic causes of obesity from common polygenic obesity. Syndromic forms of obesity, such as Bardet–Biedl, Prader–Willi, among many others 12 , are not reviewed here. Although obesity is often a dominant feature of these syndromes, the underlying genetic defects are often chromosomal abnormalities and typically encompass multiple genes, making it difficult to decipher the precise mechanisms directly related to body-weight regulation. Finally, as we enter the post-genomic era, we consider the prospects of genotype-informed treatments and the possibility of leveraging genetics to predict and hence prevent obesity.
- Gene discovery approaches
The approaches used to identify genes linked to obesity depend on the form of obesity and genotyping technology available at the time. Early gene discovery studies for monogenic forms of obesity had a case-focused design: patients with severe obesity, together with their affected and unaffected family members, were examined for potential gene-disrupting causal mutations via Sanger sequencing. By contrast, genetic variation associated with common forms of obesity have been identified in large-scale population studies, either using a case–control design or continuous traits such as BMI. Gene discovery for both forms of obesity was initially hypothesis driven; that is, restricted to a set of candidate genes that evidence suggests have a role in body-weight regulation. Over the past two decades, however, advances in high-throughput genome-wide genotyping and sequencing technologies, combined with a detailed knowledge of the human genetic architecture, have enabled the interrogation of genetic variants across the whole genome for their role in body-weight regulation using a hypothesis-generating approach.
Gene discovery for monogenic obesity
Many of the candidate genes and pathways linked to body-weight regulation were initially identified in mice, such as the obese ( ob ) 13 and diabetes ( db ) 14 mouse lines, in which severe hyperphagia and obesity spontaneously emerged. Using reverse genetics , the ob gene was shown to encode leptin, a hormone produced from fat, and it was demonstrated that leptin deficiency resulting from a mutation in the ob gene caused the severe obesity seen in the ob/ob mouse 15 (Fig. 3 ). Shortly after the cloning of ob , the db gene was cloned and identified as encoding the leptin receptor (LEPR) 16 . Reverse genetics was also used to reveal that the complex obesity phenotype of Agouti ‘lethal yellow’ mice is caused by a rearrangement in the promoter sequence of the agouti gene that results in ectopic and constitutive expression of the agouti peptide 17 , 18 , which antagonizes the melanocortin 1 and 4 receptors (MC1R and MC4R) 19 , 20 . This finding linked the melanocortin pathway to body-weight regulation, thereby unveiling a whole raft of new candidate genes for obesity.

Genes identified for monogenic obesity in a given year are shown on the left. Discoveries made for polygenic obesity are shown on the right, including a cumulative count of newly discovered loci per year and by ancestry. Although candidate gene and genome-wide linkage studies became available in the late 1990s, findings were limited, and these study designs are not as frequently used as genome-wide association studies.
Once the genes for leptin and its receptor were identified, they became candidate genes for human obesity, and in 1997 the first humans with congenital leptin deficiency were identified 21 . This discovery was rapidly followed by the report of humans with mutations in the gene encoding the leptin receptor ( LEPR ) 22 , as well as in genes encoding multiple components of the melanocortin pathway, including PCSK1 (ref. 23 ), MC4R 24 – 26 and POMC 27 – 29 , all of which were found to result in severe early-onset obesity (Table 1 ).
Overview of all genes implicated in severe and early-onset obesity
AMPK, AMPK-activated kinase; bHLH. basic helix–loop–helix; GWAS, genome-wide association study.
Advances in high-throughput DNA sequencing led to candidate gene screening being replaced by WES, an unbiased approach that allows all coding sequences to be screened for mutations. However, it rapidly became clear that, whereas candidate gene studies yielded few mutations, WES identified too many potential obesity-associated variants such that the noise often masked the true causative mutations. However, with improved algorithms to predict the pathogenicity of mutations, as well as a rapidly expanding toolkit of functional assays, it has become easier to filter the likely pathogenic mutations. Several success stories have been reported in which WES has identified novel pathways and genes linked to obesity, such as the class 3 semaphorins (SEMA3A–G), which have been shown to direct the development of certain hypothalamic neurons, including those expressing pro-opiomelanocortin (POMC) 30 (see ‘Other neuronal circuits and molecules linked to severe obesity’).
Most monogenic obesity mutations have been identified in cohorts of patients with severe and early-onset (<10 years old) obesity. Additionally, as monogenic obesity often demonstrates a recessive inheritance pattern 31 , consanguinity in populations has further increased the chance of identifying mutations, owing to greater chances of homozygosity of deleterious mutations 32 . For example, studies have reported that mutations in the genes encoding leptin, LEPR and MC4R explain 30% of cases of severe obesity in children from a consanguineous Pakistani population 33 , and single-gene defects more broadly account for nearly 50% 34 .
Gene discovery for polygenic obesity
The discovery of genes that influence polygenic obesity, which is common in the general population, started off slowly with candidate gene studies and genome-wide linkage studies . The candidate gene approach was first applied in the mid-1990s and aimed to validate genes identified through human and animal models of extreme obesity for a role in common obesity (Fig. 3 ). Common variants in such candidate genes were tested for association with obesity risk, BMI or other body composition traits. Over the subsequent 15 years, hundreds of genes were studied as candidates, but variants in only six ( ADRB3 (ref. 35 ), BDNF 36 , CNR1 (ref. 37 ), MC4R 38 , PCSK1 (ref. 39 ) and PPARG 40 ) showed reproducible association with obesity outcomes. The genome-wide linkage approach made its entrance into the field towards the end of the 1990s (Fig. 3 ). Genome-wide linkage studies rely on the relatedness of individuals and test whether certain chromosomal regions co-segregate with a disease or trait across generations. Even though more than 80 genome-wide linkage studies identified >300 chromosomal loci with suggestive evidence of linkage with obesity traits, few loci were replicated and none was successfully fine-mapped to pinpoint the causal gene or genes 41 . Ultimately, candidate gene and genome-wide linkage studies, constrained by small sample sizes, sparse coverage of genetic variation across the genome and lack of replication, only had a marginal impact on the progression of gene discovery for common obesity outcomes.
However, the pace of gene discovery for common diseases accelerated with the advent of genome-wide association studies (GWAS) (Fig. 3 ). The first GWAS for obesity traits were published in 2007 and identified a cluster of common variants in the first intron of the FTO locus that was convincingly associated with BMI 42 , 43 . Many more GWAS followed and, to date, nearly 60 GWAS have identified more than 1,100 independent loci associated with a range of obesity traits 44 (Supplementary Tables 1 , 2 ).
Even though BMI is widely used, it is considered a crude proxy of overall adiposity because it does not distinguish between lean and fat mass 46 . Therefore, GWAS have been performed for more refined obesity traits, such as body fat percentage 47 , 48 , fat-free mass 49 , imaging-derived adipose tissue 50 , circulating leptin levels 51 and LEPR levels 52 . In addition, two GWAS have focused on persistent healthy thinness, assuming that genes that determine resistance to weight gain may also inform obesity prevention and weight loss maintenance 53 , 54 . Although GWAS of more refined and alternative obesity outcomes are generally much smaller than those for BMI, the phenotypes are often a more accurate representation of body-weight regulation and, as such, the loci identified tend to more often point to relevant biological pathways that underlie obesity.
GWAS have typically focused on biallelic, common genetic variation (MAF >5%), but have also been used to screen for the role of copy number variants (CNVs) in obesity. So far, only a few CNVs have been identified that have a convincing association with BMI, such as the 1p31.1 45-kb deletion near NEGR1 (ref. 68 ), which encodes a cell-adhesion molecule expressed in the brain 69 ; the 16p12.3 21-kb deletion upstream of GPRC5B 70 , which may modulate insulin secretion 71 ; the 10q11.22 CNV in PPYR1 (also known as NPY4R ) 72 , which encodes a potent anti-obesity agent known to inhibit food intake 73 ; and the 1p21.1 multi-allele CNV encompassing AMY1A 74 , which produces salivary α-amylase, a key enzyme in starch digestion 75 .
To determine the role of other types of variation in obesity, alternative genome-wide screens have been performed. For example, the impact of low-frequency and rare protein-coding variants has been tested using exome sequencing and exome array data 76 – 79 . It was speculated that low-frequency (MAF 1–5%) and rare (MAF <1%) variants would have larger effects than common variants, and thus be easier to detect. Nevertheless, even large-scale studies identified only a few robust associations for rare coding variants. For example, exome-wide screening based on array data from more than 400,000 individuals identified p.Tyr35Ter (rs13447324) in MC4R ; p.Arg190Gln (rs139215588) and p.Glu288Gly (rs143430880) in GIPR , which stimulates insulin secretion and mediates fat deposition 80 ; p.Arg95Ter (rs114285050) in GRP151 , which modulates habenular function that controls addiction vulnerability 81 ; and p.Arg769Ter (rs533623778) in PKHD1L1 , which has been involved in cancer development 77 , 78 . A recent study that leveraged WES data for more than 600,000 individuals identified 16 genes for which the burden of rare nonsynonymous variants was associated with BMI, including five brain-expressed G protein-coupled receptors ( CALCR , MC4R , GIPR , GPR151 and GPR75 ) 79 .
As obesity is a complex, multifactorial condition, some GWAS have integrated demographic factors (such as sex and age 82 ) and environmental factors (such as physical activity 83 , diet 84 or smoking 85 ) into their analyses. Despite sample sizes of more than 200,000 individuals, these genome-wide gene-by-environment (G×E) interaction analyses remain challenging and so far only 12 loci have been identified, the effects of which on obesity are attenuated or exacerbated by non-genetic factors. Nevertheless, the G×E interaction between the FTO locus and a healthy lifestyle has been robustly replicated. Specifically, increased physical activity or a healthy diet can attenuate the effect of the FTO locus on obesity risk by 30–40% 86 , 87 .
The increasing availability of large-scale cohorts and biobanks, such as the UK Biobank , the Million Veterans Project , All of Us , Biobank Japan and 23andMe , combined with ongoing work by the GIANT consortium, will boost sample sizes further to easily exceed 4 million participants in meta-analyses, expediting the discovery of many more obesity-associated loci. However, translation of GWAS-identified loci into new biological insights remains a major challenge.
- From genes to biology
Despite the difficulties in validating causative mutations and variants, genetic studies into both rare and common obesity over the past two decades have revealed two surprisingly cogent, overarching biological messages: first, the leptin–melanocortin pathway is a key appetitive control circuit 31 , 88 (Fig. 4 ); and second, genes that are either enriched or exclusively expressed within the brain and CNS have a central role in obesity 89 .

Pro-opiomelanocortin (POMC)-expressing neurons and agouti-related protein (AGRP)-expressing neurons within the arcuate nucleus of the hypothalamus (ARC) act to sense circulating leptin (LEP) levels, which reflect fat mass. These neurons signal to melanocortin 4 receptor (MC4R)-expressing neurons in the paraventricular nucleus of the hypothalamus (PVN), which controls appetite, thus linking long-term energy stores to feeding behaviour. Binding of class 3 semaphorins (SEMA3) to their receptors NRP and PLXNA influences the projection of POMC neurons to the PVN. Binding of brain-derived neurotrophic factor (BDNF) to its receptor neurotrophic receptor tyrosine kinase 2 (NTRK2) is thought to be an effector of leptin-mediated synaptic plasticity of neurons, including those in the ARC and PVN. The transcription factor SIM1 is crucial for the proper development of the PVN. +, agonist; −, antagonist; LEPR, leptin receptor; MRAP2, melanocortin receptor accessory protein 2; MSH, melanocyte-stimulating hormone; SH2B1, SH2B adaptor protein 1.
The leptin–melanocortin pathway and MC4R
Leptin is a key hormone secreted by adipocytes, which circulates at levels in proportion to fat mass 90 . Leptin also responds to acute changes in energy state, as its levels decrease with food deprivation and are restored during re-feeding. Administration of leptin to fasted mice abrogates many of the neuroendocrine consequences of starvation, suggesting that the normal biological role of leptin is to initiate the starvation response 91 . Leptin signals through the LEPR, which exists in several different isoforms. However, obesity-related effects of leptin are predominantly mediated by a long isoform that contains an intracellular domain (LEPRb), which is expressed in various regions of the CNS 90 .
Within the arcuate nucleus (ARC) of the hypothalamus, LEPRb is found on two populations of neurons at the heart of the melanocortin pathway, one of which expresses POMC and the other agouti-related protein (AGRP) 92 (Fig. 4 ). POMC is post-translationally processed by prohormone convertases to produce several biologically active moieties, including β-lipotrophin and β-endorphin, and, crucially, the melanocortin peptides adrenocorticotrophin (ACTH) and α-, β- and γ-melanocyte-stimulating hormone (MSH) 93 . The ARC POMC neurons project to MC4R neurons within the paraventricular nucleus (PVN) where melanocortin peptides signal to decrease food intake 92 . By contrast, AGRP acts as an endogenous antagonist of MC4R to increase food intake 92 , 94 . MC3R is another centrally expressed receptor that binds to both melanocortin peptides and AGRP; however, as mice with targeted deletions in the gene are not obese but instead have altered fat to lean mass ratio, MC3R is less likely to be related to food intake and more likely to be involved in nutrient partitioning 95 , 96 .
We can state with confidence that the fine balance of melanocortinergic agonism and AGRP antagonism of MC4R, in response to peripheral nutritional cues such as leptin, plays a central part in influencing appetitive drive 92 . The genetic evidence clearly supports this contention, with mutations in most genes of the melanocortin pathway resulting in hyperphagia and severe obesity in both humans and mice 31 , 88 . In fact, the vast majority of single-gene disruptions causing severe early-onset obesity in humans fall within this pathway, including LEPR , POMC , AGRP , MCR4R , PCSK1 (ref. 23 ), SH2B1 (ref. 97 ), PHIP 98 , MRAP2 (ref. 99 ) and SIM1 (ref. 100 ) (Fig. 4 ; Table 1 ). Mutations in MC4R in particular, are the most common single-gene defect leading to hyperphagia and obesity. Pathogenic mutations in MC4R are found in up to 5% of cases of severe childhood obesity 101 and up to 0.3% of the general population 101 , 102 . Of note, the degree of receptor dysfunction, as measured by in vitro assays, can predict the amount of food eaten at a test meal by an individual harbouring that particular mutation 101 . Thus MC4R does not act in a binary on/off manner, but as a rheostat; put simply, the melanocortin pathway is a ‘tunable’ system. In addition to regulating food intake, it also regulates food preference, with individuals who carry mutations in MC4R showing a preference for food with higher fat content 103 .
The importance of the melanocortin pathway in regulating feeding behaviour is highlighted by the identification of naturally occurring mutations in pathway genes in a wide range of different species where the appropriate selection pressure has been present (Table 1 ). For example, studies have found that 20–25% of Labrador retrievers, which are known to be more food-motivated than other dog breeds, carry a 14-bp deletion in POMC that disrupts the β-MSH and β-endorphin coding sequences and is associated with greater food motivation and increased body weight 104 . Also, certain breeds of pig have been shown to carry MC4R missense mutations that are associated with fatness, growth and food intake traits 105 . MC4R mutations even contribute to the adaptation and survival of blind Mexican cavefish to the nutrient-poor conditions of their ecosystem 106 .
Other neuronal circuits and molecules linked to severe obesity
It is now clear that in addition to engaging classical neuropeptide–receptor systems within the brain, leptin also rapidly modifies synaptic connections between neurons 107 , and that this structural plasticity is crucial to its downstream functions. One of the ways in which this plasticity is thought to be achieved is via brain-derived neurotrophic factor (BDNF) signalling to its receptor TrkB. BDNF is widely expressed in the CNS where it plays an important part in neuronal development 108 , 109 . In the hippocampus, BDNF contributes to synaptic plasticity and long-term potentiation associated with memory and learning 110 . However, evidence has emerged that implicates BDNF and TrkB in the regulation of mammalian eating behaviour and energy balance 111 . BDNF is downregulated by nutritional deprivation and upregulated by leptin within the ventromedial nucleus (VMN) of the hypothalamus 112 , although this regulation is probably indirect, as very few VMN BDNF neurons express the LEPR 113 (Fig. 4 ) and some evidence indicates that it acts at least in part downstream of melanocortin signalling 112 . Crucially, genetic disruption of BDNF 114 , 115 and TrkB 112 , 116 in both humans and mice results in hyperphagia and severe obesity.
Another group of neuronal proteins important in the development of neuronal circuitry and linked to energy balance are the class 3 semaphorins (SEMA3A–G). A study in humans found that 40 rare loss-of-function variants in SEMA3A–G and their receptors (PLXNA1–4, NRP1 and NRP2) were significantly enriched in 982 individuals with severe obesity compared with 4,449 controls 30 . Disruption of several of these genes in zebrafish caused increased somatic growth and/or adiposity, and experiments with mouse hypothalamic explants suggest that SEMA3 signalling via NRP2 receptors drives the development of POMC projections from the ARC to the PVN 30 . However, given that these results are from a single study, more data are required to confirm the exact role of class 3 semaphorins in energy homeostasis.
Insights from genetic loci linked to common obesity
Unlike candidate gene studies, GWAS make no a priori assumptions about the underlying biology that links genetic variants to a disease of interest. While this agnostic approach allows for new biological insights, the vast majority of GWAS-identified variants map to the non-coding parts of genes or to regions between genes. As such, they do not directly disrupt the protein-coding regions, but instead overlap with regulatory elements that influence expression of genes in close proximity or even over long distances.
However, even if the causative genes are unknown, pathway, tissue and functional enrichment analyses based on the genes located in the GWAS loci can provide insights into potential mechanisms. Since the very first GWAS for BMI 68 , 117 , such analyses have pointed to the CNS being a key player in body-weight regulation, consistent with insights from human and animal models of extreme obesity. Recent analyses that include the latest BMI-associated loci, combined with updated multi-omics databases and advanced computational tools, have further refined these observations. In addition to the hypothalamus and pituitary gland (which are both known appetite regulation sites), other brain areas have been highlighted, including the hippocampus and the limbic system (which are involved in learning, cognition and emotion) and the insula and the substantia nigra (which are related to addiction and reward) 58 , 89 , 118 , 119 . The enrichment of immune-related cells (such as lymphocytes and B cells) and adipose tissue was found to be weaker 58 .

FTO contains nine exons (depicted by blue rectangles) and the body mass index (BMI)-associated SNP identified in genome-wide association studies (depicted by a red ×) maps to intron 1. IRX3 and RPGRIP1L have both been proposed to be the causal genes for obesity within the locus and to act on body weight through distinct mechanisms. HFD, high-fat diet.
Besides the FTO locus, functional follow-up analyses have been performed for only a few obesity-associated GWAS loci. For example, early studies identified a cluster of variants just downstream of TMEM18 (refs 68 , 117 ). TMEM18 encodes a poorly characterized transmembrane protein that is highly conserved across species and widely expressed across tissues, including in several regions of the brain 135 , 136 . Tmem18 deficiency in mice results in a higher body weight owing to increased food intake, whereas Tmem18 overexpression reduces food intake and limits weight gain 136 . A knockdown experiment in Drosophila melanogaster suggests that TMEM18 affects carbohydrate and lipid levels by disrupting insulin and glucagon signalling 137 .
Two other GWAS loci for which functional analyses have been performed are located just upstream of CADM1 (ref. 82 ) and in CADM2 (ref. 70 ), genes that encode cell-adhesion proteins of the immunoglobulin superfamily and mediate synaptic assembly in the CNS 138 . The BMI-increasing alleles at each locus are associated with increased expression of CADM1 and CADM2 in the hypothalamus 139 , 140 . Deficiency of either Cadm1 or Cadm2 in mice results in a lower body weight and increased insulin sensitivity, glucose tolerance and energy expenditure without any change in food intake 139 , 140 . Conversely, increased neuronal expression of either Cadm1 or Cadm2 is associated with elevated body weight 139 , 140 . Furthermore, CADM1 is expressed in POMC neurons and Cadm1 deficiency leads to an increase in the number of excitatory synapses, suggestive of an increased synaptic plasticity 140 . Cadm2 -deficient mice exhibit increased locomotor activity and higher core body temperature 139 .
Another GWAS locus, just upstream of NEGR1 , harbours two deletions associated with increased obesity risk 68 , 117 , 141 . These deletions do not overlap with the coding sequence of NEGR1 , but encompass a conserved transcription factor-binding site for NKX6.1 , a potent transcriptional repressor 68 , 141 . Loss of binding of NKX6.1 leads to higher NEGR1 expression 141 , which is consistent with the observation that BMI-increasing alleles (that is, deletions) at this locus are associated with higher NEGR1 expression in the brain. Similar to CADM1 and CADM2, NEGR1 is a cell-adhesion molecule of the immunoglobulin superfamily that is expressed in several regions of the brain and has been shown to have a role in brain connectivity 69 , 142 , a process believed to be important in obesity 143 . NEGR1 deficiency in mice was shown to result in lower body weight, mainly due to reduced lean mass, mediated by lower food intake 144 . However, two other functional studies, one in mice and one in rats, found that knockdown of Negr1 expression resulted in the opposite phenotype — increased body weight and food intake 145 , 146 . While NEGR1 deficiency in mice was found to impair core behaviours, so far, findings and proposed mechanisms are not fully aligned 69 , 147 – 149 .
Taken together, functional follow-up analyses for these loci are slowly expanding our understanding of the pathophysiology that drives weight gain. However, many more obesity-associated loci are waiting to be translated into new biological insights. A major hurdle in translating GWAS loci into plausible candidate genes and appropriate paradigms for functional research is the annotation of the associated variants in a locus. Defining the regulatory function of the non-coding variants, identifying their putative effector transcripts and determining their tissues of action remains an ongoing challenge. The advent of high-throughput genome-scale technologies for mapping regulatory elements, combined with comprehensive multi-omics databases, advanced computational tools and the latest genetic engineering and molecular phenotyping approaches, is poised to speed up the translation of GWAS loci into meaningful biology 150 .
Converging results from monogenic and polygenic forms of obesity
Gene discovery is often dichotomized by allele frequency and disease prevalence; that is, mutations are sought for monogenic forms of obesity and common variants for polygenic obesity (Fig. 2 ). However, it is increasingly recognized that monogenic and polygenic forms of obesity are not discrete entities. Instead, they lie on a spectrum and share — at least in part — the same biology. As GWAS have continued to discover more obesity-associated loci, an increasing number of these loci harbour genes that were first identified for extreme and early-onset obesity in humans or animal models, including MC4R 151 , 152 , BDNF 117 , SH2B1 (refs 68 , 117 ), POMC 70 , LEP 51 , 153 , LEPR 52 , 154 , NPY 155 , SIM1 (ref. 155 ), NTRK2 (ref. 58 ), PCSK1 (ref. 154 ) and KSR2 (ref. 77 ). In fact, most of these genes encode components of the leptin–melanocortin and BDNF–TrkB signalling pathways (Table 1 ). Thus, whereas genetic disruption of components of these pathways results in severe obesity, genetic variants in or near these same genes that have more subtle effects on their expression will influence where an individual might sit in the normal distribution of BMI.
Although most genes have been first identified for extreme forms of obesity, a locus harbouring ADCY3 was first identified in GWAS for common obesity 77 , and ADCY3 was subsequently confirmed as having a role in extreme obesity 63 , 64 . ADCY3 encodes an adenylate cyclase that catalyses the synthesis of cAMP, an important second messenger in signalling pathways. There is some evidence that ADCY3 (adenylate cyclase) colocalizes with MC4R at the primary cilia of PVN neurons 67 and that cilia are required specifically on MC4R-expressing neurons for the control of energy homeostasis 156 . In mice, disruption of Adcy3 or Mc4r in the cilia of these neurons impairs melanocortin signalling, resulting in hyperphagia and obesity 67 .
As more GWAS loci are reported, we expect that findings across different lines of obesity research will continue to converge, providing accumulating evidence for new biology.
- From genes to clinical care
Genetic insights from gene discovery efforts are increasingly being used in the context of precision medicine in ways that directly affect health. Knowing a patient’s genotype may enable a more precise diagnosis of the type of obesity, which in turn allows the prescription of personalized treatment or prevention strategies. Furthermore, knowing an individual’s genetic susceptibility to obesity early in life may help to more accurately predict those most at risk of gaining weight in the future.
Use of genotype information in treatment of obesity
When a disease is caused by a single mutation and the environmental contribution is limited, as is the case for some forms of extreme and early-onset obesity, a genetic test can be instrumental in correctly diagnosing patients. Although no standard genetic testing panel is currently available for extreme and early-onset obesity, some clinics, research centres and pharmaceutical companies sequence well-known candidate genes to identify the functional mutation that may be the cause of a patient’s excess body weight. Such a genetic diagnosis can lessen the feelings of guilt and blame for the patient, and alleviate social stigma and discrimination. Importantly, a genetic diagnosis can inform disease prognosis and, in some cases, it will determine treatment. To date, there are two treatments for obesity that are tailored to patient genotype.
The prototype of genotype-informed treatment for obesity is the administration of recombinant human leptin in patients who are leptin-deficient owing to mutations in the LEP gene 157 , 158 . Although congenital leptin deficiency is exceptionally rare (only 63 cases have been reported to date 28 ), leptin replacement therapy has been remarkably beneficial for these patients by substantially reducing food intake, body weight and fat mass, and normalizing endocrine function 157 , 158 . It has literally transformed their lives.
The second genotype-informed treatment for obesity is setmelanotide, a selective MC4R agonist that was recently approved by the FDA for rare monogenic obesity conditions including LEPR, PCSK1 and POMC deficiency 159 . Setmelanotide acts as a substitute for the absent MSH in patients with POMC deficiency owing to mutations in POMC or PCSK1 , and in patients with LEPR deficiency owing to mutations in LEPR , which is essential for POMC function 160 – 162 . Daily subcutaneous injection of setmelanotide results in substantial weight loss and in reduction of hunger 160 – 162 . After a 1-year treatment with setmelanotide in phase III trials, patients with POMC deficiency lost on average 25.6% of their initial weight, with 80% of patients achieving at least a 10% weight loss 162 . The adverse effects of setmelanotide treatment are minor, and include hyperpigmentation, nausea and/or vomiting, penile erection and injection site reactions. Weight loss in patients with LEPR deficiency was less pronounced; on average, they lost 12.5% of their initial weight, with only 45% of patients achieving at least a 10% weight loss 162 . The difference in weight loss between the two patient groups may be because POMC deficiency directly affects the production of MC4R ligands (α-MSH and β-MSH), whereas LEPR deficiency affects signalling upstream of POMC 162 . As such, setmelanotide may be able to completely restore MC4R signalling in POMC deficiency, but only partially in LEPR deficiency. Even though the average weight loss in POMC-deficient patients was twice that in LEPR-deficient patients, the reduction in hunger was substantially larger in LEPR-deficient patients (−43.7%) than in POMC-deficient patients (−27.1%) 162 . The reasons for the discrepancy between weight loss and reduction in hunger remain to be studied in greater depth. It has been estimated that in the USA, >12,800 individuals carry mutations in the melanocortin pathway for whom setmelanotide may be more effective for weight loss than any other treatment 163 . Although 12,800 carriers represent only a fraction (0.004%) of the adult population in the USA, and not all of these mutation carriers are overweight or obese, for the patients for whom setmelanotide is effective, it may end a lifelong battle to lose weight 163 . In patients without genetic defects, neither setmelanotide nor leptin administration have, to date, demonstrated a substantial effect on weight loss 164 , 165 .
These two genotype-informed treatments show how insight into the underlying biological mechanisms can guide the development of molecules and medications that restore impaired pathways, at least in monogenic forms of obesity caused by deficiency of one protein. Nevertheless, there remain substantial obstacles in the transition from conventional to precision medicine for monogenic obesity, which would require the adoption of systematic WES for individuals suspected to be carriers of deleterious mutations, and eventually even standardized screening at birth. We are clearly a long way from such a scenario at present.
Use of genotype information in prediction of obesity
Despite these strong associations with BMI and obesity, the predictive performance of the PGS BMI is weak, which is unsurprising given its limited explained variance. For example, using the same PGS BMI and data from the UK Biobank, we estimate that the area under the receiver operating characteristic curve (AUC ROC ) is only 0.64 to predict obesity. This means that the probability that an individual with obesity has a higher PGS BMI than an individual without obesity is 0.64. However, for a PGS to have clinical utility, the AUC ROC needs to be much higher (>0.80). In addition, we calculated the extent to which a PGS BMI ≥90th percentile correctly classifies individuals with obesity (Fig. 6 ). We found that such a predictive test (PGS BMI ≥90th percentile) has a positive predictive value of 0.43, meaning that of those who were predicted to develop obesity, only 43% actually developed obesity. Its sensitivity is 0.19, which means that of the individuals who developed obesity, only 19% had been correctly classified by the PGS BMI . Given that the current treatment options for obesity are low risk, or even generally beneficial, the high false-positive rate is less concerning than the low sensitivity, as some at-risk individuals may miss the opportunity for early prevention.

The outcome is illustrated for a polygenic score (PGS) that assumes that individuals with a score in the highest decile (≥90th percentile (pct)) will develop obesity, has a positive predictive value of 0.4 and a sensitivity of 0.19. Of ten individuals with a high score classified by the PGS as ‘with obesity’, four will be classified correctly but the other six will be misclassified and will not develop obesity — a positive predictive value of 0.4. Likewise, 17 of the 90 individuals with a score <90th pct who are predicted to not develop obesity, will develop obesity. Thus, only four of the 21 individuals who developed obesity were correctly classified by the PGS — a sensitivity of 0.19. Misclassified individuals are indicated by the red boxes, individuals correctly classified as ‘with obesity’ are indicated by a blue box. Adapted with permission from ref. 170 , Elsevier.
Thus, the current PGS BMI has a high rate of misclassification and does not reliably predict who is at risk of developing obesity and who is not. The predictive ability of PGSs are expected to improve as GWAS increase in sample size and algorithms to calculate the scores become more refined. Nevertheless, given the importance of socio-demographic, lifestyle and clinical risk factors in the aetiology of obesity, it is unlikely that a PGS BMI will ever be able to accurately predict obesity on its own. Instead, effective prediction models will have to include genetic and non-genetic factors, including a broad spectrum of demographic, environmental, clinical and possibly molecular markers, as well.
- Conclusions and future perspectives
What initially began as two apparently distinct approaches, one studying rare Mendelian causes of extreme obesity, and the other exploring complex polygenic influences of population body-weight distribution, have eventually converged on the central role of the brain in regulating body weight. In particular, both approaches have highlighted the roles of the leptin–melanocortin pathway and TrkB–BDNF signalling. Perhaps it seems obvious now, but it was by no means certain that, just because genetic disruption of a pathway resulted in a severe phenotype, polymorphisms within that same pathway would produce a more subtle and nuanced result.
The GWAS approach is hypothesis-free, with the promise to reveal new genes that point to new biology and pathways. However, for the vast majority of the >1,000 GWAS-identified loci, we do not know which genes are causal, what cells, tissues and organs they act in to affect body weight, and we do not understand the underlying mechanisms. The translation from variant to function is a well-known challenge 167 , but with increasing availability of new omics data, high-throughput technologies and advanced analytical approaches, there is an unprecedented opportunity to speed up the translation of hundreds of GWAS loci.
Sample size remains a major driver for gene discovery. In an ongoing collaboration that combines data from more than 3 million individuals of diverse ancestry from the GIANT consortium, the UK Biobank and 23andMe, the number of BMI-associated GWAS loci is set to double. Also, a recent WES effort of more than 640,000 individuals has demonstrated that rare mutations are discoverable when sample sizes are sufficiently large 79 . However, alternative study designs, a focus on more refined phenotypes or a focus on population subgroups (that is, more homogeneous groups of individuals with similar outcomes) could further add to gene discovery.
Translation of only a few dozen of the GWAS-identified loci could tremendously improve our insights into the biology of obesity and possibly reveal new therapeutic targets. It would also take us a little closer to the ‘holy grail’ — the ability to move away from a failed ‘one-size-fits-all’ strategy, and towards true precision medicine for obesity, metabolic disease and other diet-related illnesses.
- Supplementary information
- Acknowledgements
R.J.F.L. is supported by funding from Novo Nordisk Foundation (NNF Laureate Award) and the US National Institutes of Health (R01DK110113; R01DK107786; R01HL142302; R01 DK124097). G.S.H.Y. is supported by the Medical Research Council (MRC Metabolic Diseases Unit (MC_UU_00014/1)). The authors thank M. Guindo Martinez for her help with creating data for Fig. 3 and Supplementary Tables 1 and 2.
- Author contributions
The authors contributed equally to all aspects of the article.
- Competing interests
The authors declare no competing interests.
Peer review information
Nature Reviews Genetics thanks D. Meyre, T. Fall and the other, anonymous, reviewer(s) for their contribution to the peer review of this work.
Publisher’s note
Springer Nature remains neutral with regard to jurisdictional claims in published maps and institutional affiliations.
Related links
23andMe: https://research.23andme.com/collaborate/
All of Us: https://allofus.nih.gov/
Biobank Japan: http://jenger.riken.jp/en/
Genetic Investigation for ANthropometric Traits (GIANT) consortium: https://portals.broadinstitute.org/collaboration/giant/index.php/Main_Page
Million Veterans Project: https://www.research.va.gov/mvp/
NCD Risk Factor Collaboration (NCD RisC): https://www.ncdrisc.org/data-visualisations.html
UK Biobank: https://www.ukbiobank.ac.uk/
- Contributor Information
Ruth J. F. Loos, Email: [email protected] .
Giles S. H. Yeo, Email: ku.ca.mac@2yhsg .
The online version contains supplementary material available at 10.1038/s41576-021-00414-z.
Full text links
Read article at publisher's site: https://doi.org/10.1038/s41576-021-00414-z
Citations & impact
Impact metrics, citations of article over time, alternative metrics.

Smart citations by scite.ai Smart citations by scite.ai include citation statements extracted from the full text of the citing article. The number of the statements may be higher than the number of citations provided by EuropePMC if one paper cites another multiple times or lower if scite has not yet processed some of the citing articles. Explore citation contexts and check if this article has been supported or disputed. https://scite.ai/reports/10.1038/s41576-021-00414-z
Article citations, maternal dietary fat during lactation shapes single nucleus transcriptomic profile of postnatal offspring hypothalamus in a sexually dimorphic manner in mice..
Huang Y , Wang A , Zhou W , Li B , Zhang L , Rudolf AM , Jin Z , Hambly C , Wang G , Speakman JR
Nat Commun , 15(1):2382, 16 Mar 2024
Cited by: 1 article | PMID: 38493217 | PMCID: PMC10944494
Protein-truncating variants in BSN are associated with severe adult-onset obesity, type 2 diabetes and fatty liver disease.
Zhao Y , Chukanova M , Kentistou KA , Fairhurst-Hunter Z , Siegert AM , Jia RY , Dowsett GKC , Gardner EJ , Lawler K , Day FR , Kaisinger LR , Tung YL , Lam BYH , Chen HC , Wang Q , Berumen-Campos J , Kuri-Morales P , Tapia-Conyer R , Alegre-Diaz J , [...] Perry JRB
Nat Genet , 56(4):579-584, 04 Apr 2024
Cited by: 0 articles | PMID: 38575728 | PMCID: PMC11018524
Relation between Polygenic Risk Score, Vitamin D Status and BMI-for-Age z Score in Chinese Preschool Children.
Peng L , Liu T , Han C , Shi L , Chen C , Zhao J , Feng J , Wang M , Zhuo Q , Huo J , Li Y , Gong Z
Nutrients , 16(6):792, 11 Mar 2024
Cited by: 0 articles | PMID: 38542703 | PMCID: PMC10975108
Physical Activity and Incident Obesity Across the Spectrum of Genetic Risk for Obesity.
Brittain EL , Han L , Annis J , Master H , Hughes A , Roden DM , Harris PA , Ruderfer DM
JAMA Netw Open , 7(3):e243821, 04 Mar 2024
Cited by: 0 articles | PMID: 38536175 | PMCID: PMC10973894
Unexpected identification of obesity-associated mutations in LEP and MC4R genes in patients with anorexia nervosa.
Rajcsanyi LS , Zheng Y , Herpertz-Dahlmann B , Seitz J , de Zwaan M , Herzog W , Ehrlich S , Zipfel S , Giel K , Egberts K , Burghardt R , Föcker M , Antel J , Fischer-Posovszky P , Hebebrand J , Hinney A
Sci Rep , 14(1):7067, 25 Mar 2024
Cited by: 0 articles | PMID: 38528040 | PMCID: PMC10963783
Data behind the article
This data has been text mined from the article, or deposited into data resources.
BioStudies: supplemental material and supporting data
- http://www.ebi.ac.uk/biostudies/studies/S-EPMC8459824?xr=true
SNPs (Showing 11 of 11)
- (1 citation) dbSNP - rs56214831
- (1 citation) dbSNP - rs10868215
- (1 citation) dbSNP - rs10487505
- (1 citation) dbSNP - rs6545975
- (1 citation) dbSNP - rs925946
- (1 citation) dbSNP - rs11208659
- (1 citation) dbSNP - rs6907240
- (1 citation) dbSNP - rs7498665
- (1 citation) dbSNP - rs17782313
- (1 citation) dbSNP - rs6235
- (1 citation) dbSNP - rs6545814
Similar Articles
To arrive at the top five similar articles we use a word-weighted algorithm to compare words from the Title and Abstract of each citation.
Current review of genetics of human obesity: from molecular mechanisms to an evolutionary perspective.
Albuquerque D , Stice E , Rodríguez-López R , Manco L , Nóbrega C
Mol Genet Genomics , 290(4):1191-1221, 08 Mar 2015
Cited by: 92 articles | PMID: 25749980
From monogenic to polygenic obesity: recent advances.
Hinney A , Vogel CI , Hebebrand J
Eur Child Adolesc Psychiatry , 19(3):297-310, 03 Feb 2010
Cited by: 101 articles | PMID: 20127379 | PMCID: PMC2839509
A current snapshot of common genomic variants contribution in psychiatric disorders.
Santoro ML , Moretti PN , Pellegrino R , Gadelha A , Abílio VC , Hayashi MA , Belangero SI , Hakonarson H
Am J Med Genet B Neuropsychiatr Genet , 171(8):997-1005, 03 Aug 2016
Cited by: 3 articles | PMID: 27486013
Methods for the Analysis and Interpretation for Rare Variants Associated with Complex Traits.
Weissenkampen JD , Jiang Y , Eckert S , Jiang B , Li B , Liu DJ
Curr Protoc Hum Genet , 101(1):e83, 08 Mar 2019
Cited by: 5 articles | PMID: 30849219 | PMCID: PMC6455968
Review Free full text in Europe PMC
Obesity and eating behavior from the perspective of twin and genetic research.
Silventoinen K , Konttinen H
Neurosci Biobehav Rev , 109:150-165, 01 Feb 2020
Cited by: 27 articles | PMID: 31959301
Funding
Funders who supported this work.
Biotechnology and Biological Sciences Research Council (1)
Grant ID: BB/S017593/1
31 publication s
Medical Research Council (6)
Enabling technologies.
Professor Giles Yeo, University of Cambridge
Grant ID: MC_UU_00014/5
263 publication s
Mechanisms in Disorders of Energy Balance
Grant ID: MC_UU_00014/1
66 publication s
Grant ID: MC_UU_12012/5
471 publication s
Professor Stephen O'Rahilly, University of Cambridge
Grant ID: MC_UU_12012/1
151 publication s
Grant ID: MC_UU_12012/5/B
598 publication s
Molecular characterization in human neurons of genes associated with severe obesity identified from consanguineous pedigrees.
Grant ID: MR/S026193/1
26 publication s
NHLBI NIH HHS (1)
Grant ID: R01 HL142302
49 publication s
NIDDK NIH HHS (3)
Grant ID: R01 DK124097
20 publication s
Grant ID: R01 DK107786
46 publication s
Grant ID: R01 DK110113
54 publication s
NNF Center for Basic Metabolic Research (1)
Grant ID: CBMR - 008
Novo Nordisk Fonden (2)
Grant ID: NNF20OC0059313
25 publication s
Grant ID: NNF21SA0072102
122 publication s
Europe PMC is part of the ELIXIR infrastructure
- Visit the Gateway
- Visit the Alliance
- Visit HDR UK Futures
Understanding the genetics involved in obesity
27 June 2019
Professor Cecilia Lindgren’s research at the Big Data Institute (BDI), University of Oxford, seeks to advance understanding of the underlying mechanisms involved in obesity. By applying a range of genetic approaches, her team looks to identify genetic variants influencing fat distribution around the body, and to investigate the biological pathways involved.
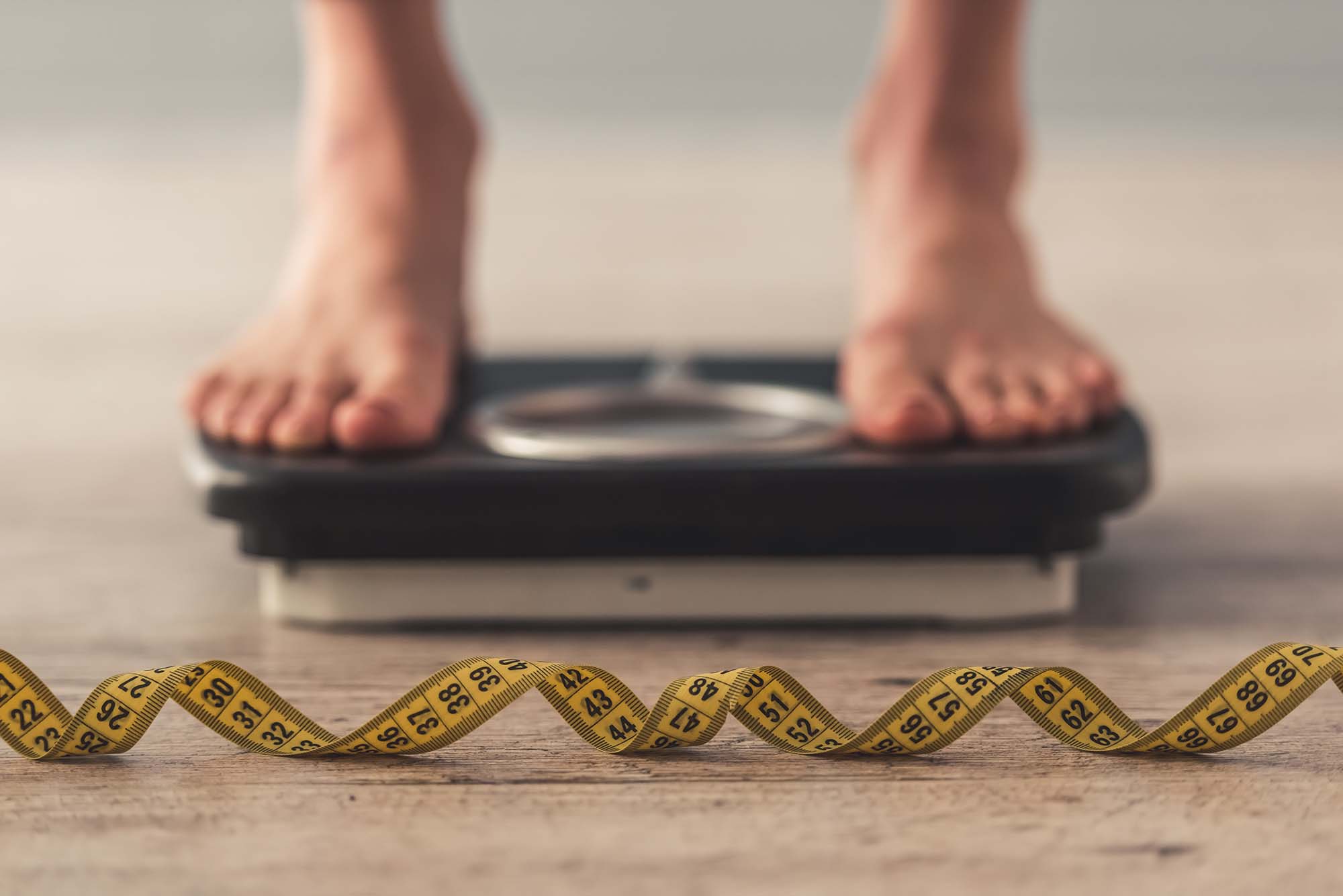
Share this page
Professor Lindgren recently led one of the largest ever studies to explore the influence of rare, low frequency genetic variants on body-fat distribution. It was found that although these variants are rare in the population, their effects on individuals are usually large.
“My science wouldn’t happen without collaboration – I work with many organisations, institutes and consortia, accessing, linking up and analysing different data types. This is why I am so excited about HDR UK and what we stand for – we are connecting the right people and ensuring we stay at the forefront of scientific research,” says Professor Lindgren.
The obesity epidemic
According to the World Health Organisation, 62% of adults in the UK are classified as overweight with 28% of adults clinically obese. Obesity often starts in early life, doesn’t have a specific treatment and can lead to a range of illnesses including heart disease, diabetes, a range of cancers and fertility issues. Professor Lindgren’s career started in diabetes research. She became interested in obesity as a key co-morbidity, which led her to join international efforts to unravel the underlying mechanisms of obesity.
“There is a huge stigma around obesity. Whilst people connect obesity with over-indulging, not exercising and a lack of willpower, there is strong evidence that a significant proportion of the disease is explained by genetic predisposition,” says Professor Lindgren.
Genetics and obesity
Professor Lindgren studies the genetic component of obesity and how different people carry different risks. In particular, a recent study looked at fat distribution and where in the body people aggregate fat. Obese men traditionally have an ‘apple shape’ whilst women can develop a ‘pear shape’. The accumulation of fat in specific areas proves to be connected to risk of cardiovascular disease or diabetes. Her research looked at specific genetic variants that determine where the fat is stored and therefore its links to health. As fat distribution is genetically regulated (and not directly related to diet), if you have a specific genetic predisposition to lay down fat in dangerous areas, you could possibly require early therapeutic intervention to help prevent or manage this.
Access to health data
Professor Lindgren’s work is dependent on UK Biobank where she analyses large scale patient data and processes it with high-performance computing infrastructure at the BDI, part-funded by HDR UK.
Impact of the research
Professor Lindgren’s research is providing additional understanding of the causes of obesity and fat distribution. Her overall aim is to reduce the stigma attached to obesity and to accelerate therapeutic development. She is collaborating with many colleagues who are tackling related issues. She works to influence government public health policy as well as influencing the Medtech industry, so that new therapeutic targets might be exploited to help people prevent or manage their conditions.
Science abstract : Obesity and its consequences are major and growing challenges for health care worldwide. Many common genetic variants have been identified, which influence the severity of and predisposition to obesity at the population level: these findings should lead to improved understanding of the mechanisms involved in the regulation of overall energy balance. However, not all obese individuals are equally vulnerable to diabetes, cardiovascular disease and other adverse consequences of obesity and it has long been appreciated that the distribution of fat (particularly the degree of visceral fat) is an additional and independent determinant of individual risk of metabolic and cardiovascular disease.
Read the full paper here . Find out more about Prof Lindgren’s research here .
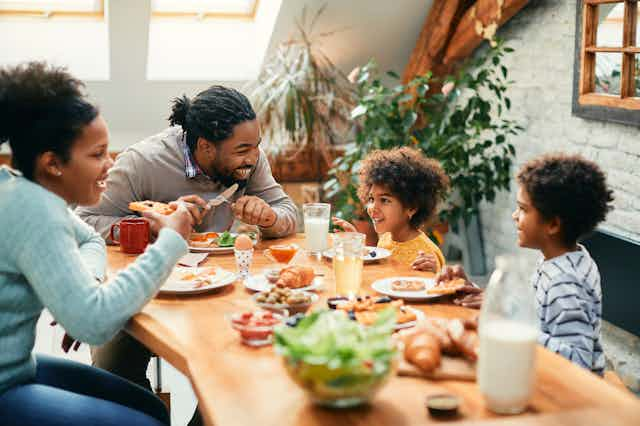
What you eat could alter your unborn children and grandchildren’s genes and health outcomes
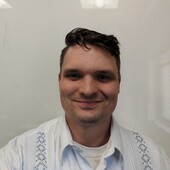
Assistant Professor of Nutrition and Dietetics, University of North Dakota
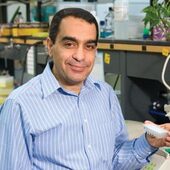
Associate Chair and Professor of Genetics and Epigenetics, University of Wisconsin-Madison
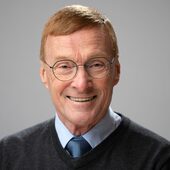
Professor of Animal and Dairy Sciences, University of Wisconsin-Madison
Disclosure statement
Nathaniel Johnson receives funding from the United States Department of Agriculture and the National Institutes of Health. He has previously received funding through the National Science Foundation, the National Cattlemen's Beef Association, and the North Dakota Beef Checkoff.
Hasan Khatib receives funding no. 2023-67015-39527 from the USDA National Institute of Food and Agriculture
Thomas D Crenshaw receives funding from Hatch Multi-State Research Formula Funds; USDA/Natl. Institute of Food and Agriculture; DHHS, PHS, National Institutes of Health.
University of North Dakota and University of Wisconsin–Madison provide funding as members of The Conversation US.
View all partners
Within the last century, researchers’ understanding of genetics has undergone a profound transformation.
Genes, regions of DNA that are largely responsible for our physical characteristics, were considered unchanging under the original model of genetics pioneered by biologist Gregor Mendel in 1865. That is, genes were thought to be largely unaffected by a person’s environment.
The emergence of the field of epigenetics in 1942 shattered this notion .
Epigenetics refers to shifts in gene expression that occur without changes to the DNA sequence. Some epigenetic changes are an aspect of cell function, such as those associated with aging .
However, environmental factors also affect the functions of genes, meaning people’s behaviors affect their genetics. For instance, identical twins develop from a single fertilized egg, and as a result, they share the same genetic makeup. However, as the twins age, their appearances may differ due to distinct environmental exposures. One twin may eat a healthy balanced diet, whereas the other may eat an unhealthy diet, resulting in differences in the expression of their genes that play a role in obesity, helping the former twin have lower body fat percentage.
People don’t have much control over some of these factors, such as air quality . Other factors, though, are more in a person’s control: physical activity , smoking , stress, drug use and exposure to pollution , such as that coming from plastics, pesticides and burning fossil fuels, including car exhaust.
Another factor is nutrition, which has given rise to the subfield of nutritional epigenetics . This discipline is concerned with the notions that “you are what you eat” – and “you are what your grandmother ate.” In short, nutritional epigenetics is the study of how your diet, and the diet of your parents and grandparents, affects your genes . As the dietary choices a person makes today affects the genetics of their future children, epigenetics may provide motivation for making better dietary choices.
Two of us work in the epigenetics field . The other studies how diet and lifestyle choices can help keep people healthy . Our research team is comprised of fathers, so our work in this field only enhances our already intimate familiarity with the transformative power of parenthood.
A story of famine
The roots of nutritional epigenetics research can be traced back to a poignant chapter in history – the Dutch Hunger Winter in the final stages of World War II.
During the Nazi occupation of the Netherlands, the population was forced to live on rations of 400 to 800 kilocalories per day, a far cry from the typical 2,000-kilocalorie diet used as a standard by the Food and Drug Administration . As a result, some 20,000 people died and 4.5 million were malnourished.
Studies found that the famine caused epigentic changes to a gene called IGF2 that is related to growth and development. Those changes suppressed muscle growth in both the children and grandchildren of pregnant women who endured the famine. For these subsequent generations, that suppression led to an increased risk of obesity, heart disease, diabetes and low birth weight .
These findings marked a pivotal moment in epigenetics research – and clearly demonstrated that environmental factors, such as famine, can lead to epigenetic changes in offspring that may have serious implications for their health.
The role of the mother’s diet
Until this groundbreaking work, most researchers believed epigenetic changes couldn’t be passed down from one generation to the next. Rather, researchers thought epigenetic changes could occur with early-life exposures, such as during gestation – a highly vulnerable period of development. So initial nutritional epigenetic research focused on dietary intake during pregnancy.
The findings from the Dutch Hunger Winter were later supported by animal studies, which allow researchers to control how animals are bred, which can help control for background variables. Another advantage for researchers is that the rats and sheep used in these studies reproduce more quickly than people, allowing for faster results. In addition, researchers can fully control animals’ diets throughout their entire lifespan, allowing for specific aspects of diet to be manipulated and examined. Together, these factors allow researchers to better investigate epigenetic changes in animals than in people.
In one study, researchers exposed pregnant female rats to a commonly used fungicide called vinclozolin. In response to this exposure, the first generation born showed decreased ability to produce sperm, leading to increased male infertility . Critically, these effects, like those of the famine, were passed to subsequent generations.
As monumental as these works are for shaping nutritional epigenetics, they neglected other periods of development and completely ignored the role of fathers in the epigenetic legacy of their offspring. However, a more recent study in sheep showed that a paternal diet supplemented with the amino acid methionine given from birth to weaning affected the growth and reproductive traits of the next three generations. Methionine is an essential amino acid involved in DNA methylation , an example of an epigenetic change.
Healthy choices for generations to come
These studies underscore the enduring impact parents’ diets have on their children and grandchildren. They also serve as a powerful motivator for would-be parents and current parents to make more healthy dietary choices , as the dietary choices parents make affect their children’s diets .
Meeting with a nutrition professional, such as a registered dietitian, can provide evidence-based recommendations for making practical dietary changes for individuals and families .
There are still many unknowns about how diet affects and influences our genes. What research is starting to show about nutritional epigenetics is a powerful and compelling reason to consider making lifestyle changes.
There are many things researchers already know about the Western Diet, which is what many Americans eat . A Western Diet is high in saturated fats, sodium and added sugar, but low in fiber ; not surprisingly, Western diets are associated with negative health outcomes, such as obesity, type 2 diabetes, cardiovascular disease and some cancers .
A good place to start is to eat more whole, unprocessed foods, particularly fruits, vegetables and whole grains, and fewer processed or convenience foods – that includes fast food, chips, cookies and candy, ready-to-cook meals, frozen pizzas, canned soups and sweetened beverages.
These dietary changes are well known for their health benefits and are described in the 2020-2025 Dietary Guidelines for Americans and by the American Heart Association .
Many people find it difficult to embrace a lifestyle change , particularly when it involves food. Motivation is a key factor for making these changes . Luckily, this is where family and friends can help – they exert a profound influence on lifestyle decisions .
However, on a broader, societal level, food security – meaning people’s ability to access and afford healthy food – should be a critical priority for governments, food producers and distributors, and nonprofit groups. Lack of food security is associated with epigenetic changes that have been linked to negative health outcomes such as diabetes , obesity and depression .
Through relatively simple lifestyle modifications , people can significantly and measurably influence the genes of their children and grandchildren. So when you pass up a bag a chips – and choose fruit or a veggie instead – keep in mind: It’s not just for you, but for the generations to come.
- Epigenetics
- Dietary supplements
- Healthy diet

Project Offier - Diversity & Inclusion

Senior Lecturer - Earth System Science

Sydney Horizon Educators (Identified)

Deputy Social Media Producer

Associate Professor, Occupational Therapy

- Meet The Team
- Where We Work
- What Have We Discovered?
- What Are We Doing Now?
- Professionals
- Research Programme
- Gene Discovery
- Molecular Mechanisms
- Eating Behaviour
- Useful Resources
- Clinical Trials and Treatments
- Genetic Testing
- Patients And Families
- What Genes Have We Found?
- What Happens When I Come To Cambridge?
- Personal Experiences
- Useful Links
- When is a Genetic Test Needed?
- Patient Information
- Resource Centre
- Newsletters
Latest News
- Media Centre
- New Treatments
- We analyse chromosomes to identify key genes whose disruption causes obesity. Genetic variants increase or decrease the risk of weight gain
- Our vision is to deliver new treatments for people with severe obesity by understanding the fundamental mechanisms controlling body weight. Neurons in the hypothalamus regulate appetite and weight
- We conduct trials of new treatments in people with obesity caused by changes in their genes. We are developing precision medicine approaches to treat people with obesity
- We are discovering how fat cells work to burn calories. Fat cells expand to store extra calories as lipids

New Weight Loss Treatments
Find out about new Anti-Obesity Medications

Professor Sadaf Farooqi receives Outstanding Clinical Investigator Award
Professor Farooqi who has been announced as the recipient of the 2024 Outstanding Clinical Investigator Award from the Endocrine Society.

Deletion on Chromosome 16 leads to early-onset obesity and diabetes
Adults with a deletion involving SH2B1 develop early type 2 diabetes which is difficult to treat.

Welcome to GOOS
Genetics of Obesity Study
Within a given environment, some people put on weight very easily, whilst others remain thin. We aim to understand the key mechanisms that control body weight to inform the development of safe, effective weight loss interventions. We use genetic, molecular and clinical approaches to study cohorts of individuals with severe obesity and thinness.

Latest News See all news>>

We would like to thank colleagues who refer patients to GOOS, research collaborators, funding bodies, former members of the GOOS team and the patients, families and volunteers who support our research.
Featured Clinical Reviews
- Screening for Atrial Fibrillation: US Preventive Services Task Force Recommendation Statement JAMA Recommendation Statement January 25, 2022
- Evaluating the Patient With a Pulmonary Nodule: A Review JAMA Review January 18, 2022
Select Your Interests
Customize your JAMA Network experience by selecting one or more topics from the list below.
- Academic Medicine
- Acid Base, Electrolytes, Fluids
- Allergy and Clinical Immunology
- American Indian or Alaska Natives
- Anesthesiology
- Anticoagulation
- Art and Images in Psychiatry
- Artificial Intelligence
- Assisted Reproduction
- Bleeding and Transfusion
- Caring for the Critically Ill Patient
- Challenges in Clinical Electrocardiography
- Climate and Health
- Climate Change
- Clinical Challenge
- Clinical Decision Support
- Clinical Implications of Basic Neuroscience
- Clinical Pharmacy and Pharmacology
- Complementary and Alternative Medicine
- Consensus Statements
- Coronavirus (COVID-19)
- Critical Care Medicine
- Cultural Competency
- Dental Medicine
- Dermatology
- Diabetes and Endocrinology
- Diagnostic Test Interpretation
- Drug Development
- Electronic Health Records
- Emergency Medicine
- End of Life, Hospice, Palliative Care
- Environmental Health
- Equity, Diversity, and Inclusion
- Facial Plastic Surgery
- Gastroenterology and Hepatology
- Genetics and Genomics
- Genomics and Precision Health
- Global Health
- Guide to Statistics and Methods
- Hair Disorders
- Health Care Delivery Models
- Health Care Economics, Insurance, Payment
- Health Care Quality
- Health Care Reform
- Health Care Safety
- Health Care Workforce
- Health Disparities
- Health Inequities
- Health Policy
- Health Systems Science
- History of Medicine
- Hypertension
- Images in Neurology
- Implementation Science
- Infectious Diseases
- Innovations in Health Care Delivery
- JAMA Infographic
- Law and Medicine
- Leading Change
- Less is More
- LGBTQIA Medicine
- Lifestyle Behaviors
- Medical Coding
- Medical Devices and Equipment
- Medical Education
- Medical Education and Training
- Medical Journals and Publishing
- Mobile Health and Telemedicine
- Narrative Medicine
- Neuroscience and Psychiatry
- Notable Notes
- Nutrition, Obesity, Exercise
- Obstetrics and Gynecology
- Occupational Health
- Ophthalmology
- Orthopedics
- Otolaryngology
- Pain Medicine
- Palliative Care
- Pathology and Laboratory Medicine
- Patient Care
- Patient Information
- Performance Improvement
- Performance Measures
- Perioperative Care and Consultation
- Pharmacoeconomics
- Pharmacoepidemiology
- Pharmacogenetics
- Pharmacy and Clinical Pharmacology
- Physical Medicine and Rehabilitation
- Physical Therapy
- Physician Leadership
- Population Health
- Primary Care
- Professional Well-being
- Professionalism
- Psychiatry and Behavioral Health
- Public Health
- Pulmonary Medicine
- Regulatory Agencies
- Reproductive Health
- Research, Methods, Statistics
- Resuscitation
- Rheumatology
- Risk Management
- Scientific Discovery and the Future of Medicine
- Shared Decision Making and Communication
- Sleep Medicine
- Sports Medicine
- Stem Cell Transplantation
- Substance Use and Addiction Medicine
- Surgical Innovation
- Surgical Pearls
- Teachable Moment
- Technology and Finance
- The Art of JAMA
- The Arts and Medicine
- The Rational Clinical Examination
- Tobacco and e-Cigarettes
- Translational Medicine
- Trauma and Injury
- Treatment Adherence
- Ultrasonography
- Users' Guide to the Medical Literature
- Vaccination
- Venous Thromboembolism
- Veterans Health
- Women's Health
- Workflow and Process
- Wound Care, Infection, Healing
- Download PDF
- Share X Facebook Email LinkedIn
- Permissions
People With Genetic Risk of Obesity Need More Exercise to Mitigate It
- JAMA Insights What Are Polygenic Scores and Why Are They Important? Leo P. Sugrue, MD, PhD; Rahul S. Desikan, MD, PhD JAMA
- Original Investigation Physical Activity and Incident Obesity Across the Spectrum of Genetic Risk Evan L. Brittain, MD, MSc; Lide Han, PhD; Jeffrey Annis, PhD; Hiral Master, PhD; Andrew Hughes, MD; Dan M. Roden, MD; Paul A. Harris, PhD; Douglas M. Ruderfer, PhD JAMA Network Open
People with increased polygenic risk scores for higher body mass index (BMI) would need to walk about 2300 more steps each day to have the same risk of obesity as those with lower scores, a recent retrospective study in JAMA Network Open found. Polygenic risk scores reflect the risk of disease determined by many variants in a person’s DNA . The results suggest that exercise recommendations that don’t take genetic predispositions for obesity into account might underestimate the amount of activity individuals might need to reduce their risk, the researchers noted.
The findings were based on data from about 3100 participants in the US with European ancestry included in the All of Us Research Program who did not have obesity during the first 6 months of the 5-year study.
In addition, the researchers found that participants who had higher genetic risk scores and started the study with BMIs of 22 or greater would need to walk even more—up to about 6400 additional daily steps for those who began with a BMI of 28—to lower their risk of obesity so that it was equal to those with less genetic susceptibility.
Published Online: April 19, 2024. doi:10.1001/jama.2024.5921
See More About
Harris E. People With Genetic Risk of Obesity Need More Exercise to Mitigate It. JAMA. Published online April 19, 2024. doi:10.1001/jama.2024.5921
Manage citations:
© 2024
Artificial Intelligence Resource Center
Cardiology in JAMA : Read the Latest
Browse and subscribe to JAMA Network podcasts!
Others Also Liked
- Register for email alerts with links to free full-text articles
- Access PDFs of free articles
- Manage your interests
- Save searches and receive search alerts
- Open access
- Published: 22 April 2024
The influence of maternal prepregnancy weight and gestational weight gain on the umbilical cord blood metabolome: a case–control study
- Xianxian Yuan ORCID: orcid.org/0000-0001-8762-8471 1 ,
- Yuru Ma 1 ,
- Jia Wang 2 ,
- Yan Zhao 1 ,
- Wei Zheng 1 ,
- Ruihua Yang 1 ,
- Lirui Zhang 1 ,
- Xin Yan 1 &
- Guanghui Li ORCID: orcid.org/0000-0003-2290-1515 1
BMC Pregnancy and Childbirth volume 24 , Article number: 297 ( 2024 ) Cite this article
22 Accesses
Metrics details
Maternal overweight/obesity and excessive gestational weight gain (GWG) are frequently reported to be risk factors for obesity and other metabolic disorders in offspring. Cord blood metabolites provide information on fetal nutritional and metabolic health and could provide an early window of detection of potential health issues among newborns. The aim of the study was to explore the impact of maternal prepregnancy overweight/obesity and excessive GWG on cord blood metabolic profiles.
A case control study including 33 pairs of mothers with prepregnancy overweight/obesity and their neonates, 30 pairs of mothers with excessive GWG and their neonates, and 32 control mother-neonate pairs. Untargeted metabolomic profiling of umbilical cord blood samples were performed using UHPLC‒MS/MS.
Forty-six metabolites exhibited a significant increase and 60 metabolites exhibited a significant reduction in umbilical cord blood from overweight and obese mothers compared with mothers with normal body weight. Steroid hormone biosynthesis and neuroactive ligand‒receptor interactions were the two top-ranking pathways enriched with these metabolites ( P = 0.01 and 0.03, respectively). Compared with mothers with normal GWG, in mothers with excessive GWG, the levels of 63 metabolites were increased and those of 46 metabolites were decreased in umbilical cord blood. Biosynthesis of unsaturated fatty acids was the most altered pathway enriched with these metabolites ( P < 0.01).
Conclusions
Prepregnancy overweight and obesity affected the fetal steroid hormone biosynthesis pathway, while excessive GWG affected fetal fatty acid metabolism. This emphasizes the importance of preconception weight loss and maintaining an appropriate GWG, which are beneficial for the long-term metabolic health of offspring.
Peer Review reports
The obesity epidemic is an important public health problem in developed and developing countries [ 1 ] and is associated with the emergence of chronic noncommunicable diseases, including type 2 diabetes mellitus (T2DM), hypertension, cardiovascular disease, nonalcoholic fatty liver disease (NAFLD), and cancer [ 2 , 3 , 4 ]. Maternal obesity is the most common metabolic disturbance in pregnancy, and the prevalence of obesity among women of childbearing age is 7.1% ~ 31.9% in some countries [ 5 ]. In China, the prevalence of overweight and obesity has also increased rapidly in the past four decades. Based on Chinese criteria, the latest national prevalence estimates for 2015–2019 were 34.3% for overweight and 16.4% for obesity in adults (≥ 18 years of age) [ 6 ].
Increasing evidence implicates overnutrition in utero as a major determinant of the health of offspring during childhood and adulthood, which is compatible with the developmental origins of health and disease (DOHaD) framework [ 7 ]. Maternal obesity and excessive gestational weight gain (GWG) are important risk factors for several adverse maternal outcomes, including gestational diabetes and hypertensive disorders, fetal death, and preterm birth [ 8 , 9 , 10 ]. More importantly, they have negative implications for offspring, both perinatally and later in life. Evidence from cohort studies focusing on offspring development confirms the relationship between maternal obesity/excessive GWG and offspring obesity programming [ 11 , 12 , 13 ]. Currently, there is no unified mechanism to explain the adverse outcomes associated with maternal obesity and excessive GWG, which may be the independent and interactive effects of the obese maternal phenotype itself and the diet associated with this phenotype. In addition to genetic and environmental factors, metabolic programming may also lead to the intergenerational transmission of obesity through epigenetic mechanisms.
Metabolomics, which reflects the metabolic phenotype of human subjects and animals, is the profiling of metabolites in biofluids, cells and tissues using high-throughput platforms, such as mass spectrometry. It has unique potential in identifying biomarkers for predicting occurrence, severity, and progression of diseases, as well as exploring underlying mechanistic abnormalities [ 14 , 15 ]. Umbilical cord metabolites can provide information about fetal nutritional and metabolic health, and may provide an early window for detection of potential health issues in newborns [ 16 ]. Previous studies have reported differences in umbilical cord metabolite profiles associated with maternal obesity [ 17 , 18 ]. However, the results were inconsistent due to differences in sample sizes, ethnicity and region, and mass spectrometry. In addition, most studies have not considered the difference in the effects of prepregnancy body mass index (BMI) and GWG on cord blood metabolites.
To investigate the relationship between early metabolic programming and the increased incidence of metabolic diseases in offspring, we studied the associations between elevated prepregnancy BMI/excessive GWG and umbilical cord metabolic profiles. Another purpose of this study was to explore whether there were differences in the effects of prepregnancy overweight/obesity and excessive GWG on cord blood metabolites.
Study population
This was a hospital-based, case control study that included singleton pregnant women who received prenatal care and delivered vaginally at Beijing Obstetrics and Gynecology Hospital, Capital Medical University, from January 2022 to March 2022. We selected 33 pregnant women with a prepregnancy BMI ≥ 24.0 kg/m 2 regardless of their gestational weight gain as the overweight/obese group, 30 pregnant women with a prepregnancy BMI of 18.5–23.9 kg/m 2 and a GWG > 14.0 kg as the excessive GWG group, and 32 pregnant women with a BMI of 18.5–23.9 kg/m 2 and a GWG of 8.0–14.0 kg as the control group. The ages of the three groups were matched (± 1.0 years), and the prepregnancy BMIs of the excessive GWG and control groups were matched (± 1.0 kg/m 2 ).
The inclusion criteria were women with singleton pregnancies, those aged between 20 and 45 years, those with full-term delivery (gestational age ≥ 37 weeks), those with a prepregnancy BMI ≥ 18.5 kg/m 2 , those without prepregnancy diabetes mellitus (DM) or hypertension, and those without gestational diabetes mellitus (GDM). The exclusion criteria were women with multiple pregnancies, those less than 20 years or more than 45 years old, those with a prepregnancy BMI < 18.5 kg/m 2 , those with prepregnancy DM, hypertension or GDM, and those without cord blood samples.
We classified pregnant women into BMI categories based on Chinese guidelines [ 19 ]: normal weight (prepregnancy BMI 18.5–23.9 kg/m 2 ), overweight (prepregnancy BMI 24.0–27.9 kg/m 2 ), and obese (prepregnancy BMI ≥ 28.0 kg/m 2 ). GWG guideline concordance was defined by the 2021 Chinese Nutrition Society recommendations according to prepregnancy BMI. The upper limits of GWG for normal weight, overweight, and obesity were 14.0 kg, 11.0 kg, and 9.0 kg, respectively.
Ethical approval and written informed consent were obtained from all participants. The study has been performed according to the Declaration of Helsinki, and the procedures have been approved by the ethics committees of Beijing Obstetrics and Gynecology Hospital, Capital Medical University (2021-KY-037).
Sample and data collection
Maternal and neonatal clinical data were collected from the electronic medical records system of Beijing Obstetrics and Gynecology Hospital. Maternal clinical characteristics included age, height, prepregnancy and predelivery weight, education level, smoking and drinking status during pregnancy, parity, conception method, comorbidities and complications of pregnancy, family history of DM and hypertension, gestational age, mode of delivery, and biochemical results during pregnancy. Prepregnancy BMI was calculated as prepregnancy weight in kilograms divided by the square of height in meters. GWG was determined by subtracting the prepregnancy weight in kilograms from the predelivery weight in kilograms. GDM was defined using the IAPDSG’s diagnostic criteria at 24 to 28 +6 weeks gestation and the fasting glucose and 1- and 2-h glucose concentrations at the time of the oral glucose tolerance test (OGTT). Neonatal clinical characteristics included sex, birth weight and length. Macrosomia was defined as a birth weight of 4,000 g or more [ 20 ]. Low birth weight (LBW) was defined as a birth weight less than 2,500 g [ 21 ].
Umbilical cord blood samples were obtained by trained midwives after clamping the cord at delivery. Whole blood samples were collected in EDTA tubes, refrigerated for < 24 h, and centrifuged at 2,000 r.p.m. at 4 ℃ for 10 min. Plasma aliquots were stored at -80 ℃ until shipment on dry ice to Novogene, Inc. (Beijing, China) for untargeted metabolomic analysis.
Untargeted metabolomic analyses
Ultrahigh-performance liquid chromatography tandem mass spectrometry (UHPLC‒MS/MS) analyses were performed using a Vanquish UHPLC system (Thermo Fisher, Germany) coupled with an Orbitrap Q Exactive™ HF mass spectrometer (Thermo Fisher, Germany) at Novogene Co., Ltd. (Beijing, China). Detailed descriptions of the sample preparation, mass spectrometry and automated metabolite identification procedures are described in the Supplementary materials .
Statistical analysis
Clinical data statistical analysis.
Quantitative data are shown as the mean ± standard deviation (SD) or median (interquartile range), and categorical data are presented as percentages. The Mann‒Whitney U test, chi-square test, and general linear repeated-measures model were used to assess the differences between the control and study groups when appropriate. A P value < 0.05 was considered statistically significant. All analyses were performed using Statistical Package of Social Sciences version 25.0 (SPSS 25.0) for Windows (SPSS Inc).
Umbilical cord metabolome statistical analysis
These metabolites were annotated using the Human Metabolome Database (HMDB) ( https://hmdb.ca/metabolites ), LIPIDMaps database ( http://www.lipidmaps.org/ ), and Kyoto Encylopaedia of Genes and Genomes (KEGG) database ( https://www.genome.jp/kegg/pathway.html ). Principal component analysis (PCA) and partial least-squares discriminant analysis (PLS-DA) were performed at metaX. We applied univariate analysis ( T test) to calculate the statistical significance ( P value). Metabolites with a variable importance for the projection (VIP) > 1, a P value < 0.05 and a fold change (FC) ≥ 2 or FC ≤ 0.5 were considered to be differential metabolites. A false discovery rate (FDR) control was implemented to correct for multiple comparisons. The q -value in the FDR control was defined as the FDR analog of the P -value. In this study, the q -value was set at 0.2. For clustering heatmaps, the data were normalized using z scores of the intensity areas of differential metabolites and were plotted by the Pheatmap package in R language.
The correlations among differential metabolites were analyzed by cor () in R language (method = Pearson). Statistically significant correlations among differential metabolites were calculated by cor.mtest () in R language. A P value < 0.05 was considered statistically significant, and correlation plots were plotted by the corrplot package in R language. The functions of these metabolites and metabolic pathways were studied using the KEGG database. The metabolic pathway enrichment analysis of differential metabolites was performed when the ratio was satisfied by x/n > y/N, and the metabolic pathway was considered significantly enriched when P < 0.05.
Demographic characteristics of study participants
The demographic and clinical characteristics of the three population groups enrolled in the study are summarized in Table 1 . Mothers had no significant difference regarding their ages or gestational ages. Compared to the mothers in the excessive GWG and control groups, those in the prepregnancy overweight/obesity group had a significantly higher prepregnancy BMI (25.6 (24.5, 27.2) kg/m 2 ). However, there was no significant difference in prepregnancy BMI between mothers in the excessive GWG group (20.3 ± 1.2 kg/m 2 ) and mothers in the control group (20.6 ± 1.5 kg/m 2 ). Mothers in the excessive GWG group had the highest GWG (17.0 (15.5, 19.1) kg) among the three groups. The mean GWG of the mothers in the prepregnancy overweight/obesity group was 12.9 ± 3.8 kg, which was similar to that of the control group (11.8 ± 1.5 kg). It was noteworthy that among the 33 prepregnancy overweight/obese pregnant women, 20 of them had appropriate GWG, 1 had insufficient GWG, and 12 had excessive GWG. The proportion of mothers who underwent invitro fertilization and embryo transfer (IVF-ET) in the prepregnancy overweight/obesity group (15.2%) was significantly higher than that in the excessive GWG and control groups. There were no statistically significant differences in the proportions of pregnancy outcomes among the three groups, including preeclampsia, premature rupture of membranes, postpartum hemorrhage, macrosomia, and LBW. The babies in the three groups showed no significant difference regarding their birth weights or lengths.
The biochemical parameters of the mothers during pregnancy are shown in Table 2 . The levels of triglyceride (TG) and uric acid (UA) of mothers in the prepregnancy overweight/obesity group were significantly higher than those of the mothers in the excessive GWG and control groups in the first trimester. However, there was no significant difference in the blood glucose and lipid levels in the second and third trimesters of pregnancy among the three groups.
PCA and PLS-DA analysis of cord blood metabolites
Functional and taxonomic annotations of the identified metabolites included the HMDB classification annotations, LIPID MAPS classification annotations, and KEGG pathway annotations. Those cord blood metabolites included lipids and lipid-like molecules, organic acids and their derivatives, and organoheterocyclic compounds, which were mainly involved in metabolism. To better understand the structure of the cord blood metabolome in cases versus controls, we used unsupervised PCA to identify metabolites contributing the most to observed differences in the dataset. PCA did not clearly separate the three groups. We next used PLS-DA to identify metabolites that were predictive of case versus control status. PLS-DA clearly distinguished the cases from the controls (Fig. 1 ), the prepregnancy overweight/obesity group vs. the control group (R2Y = 0.82, Q2Y = 0.37; R2Y = 0.77, Q2Y = 0.13, respectively) (Fig. 1 A), and the excessive GWG group vs. the control group (R2Y = 0.76, Q2Y = 0.16; R2Y = 0.81, Q2Y = 0.41) (Fig. 1 B).
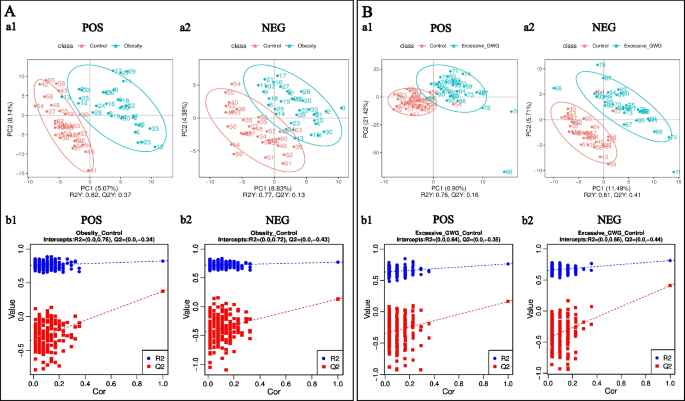
PLS-DA of identified cord blood metabolites. A the prepregnancy overweight/obesity group vs. the control group; B the excessive GWG group vs. the control group. (a) PLS-DA score. The horizontal coordinates are the score of the sample on the first principal component; the longitudinal coordinates are the score of the sample on the second principal component; R2Y represents the interpretation rate of the model, and Q2Y is used to evaluate the predictive ability of the PLS-DA model, and when R2Y is greater than Q2Y, it means that the model is well established. (b) PLS-DA valid. Horizontal coordinates represent the correlation between randomly grouped Y and the original group Y, and vertical coordinates represent the scores of R2 and Q2. (1) POS, positive metabolites; (2) NEG, negative metabolites
Maternal prepregnancy overweight/obesity
Screening differential metabolites according to a PLS-DA VIP > 1.0, a FC > 1.2 or < 0.833 and a P value < 0.05, a total of 106 cord blood metabolites (77 positive metabolites and 29 negative metabolites) differed between the prepregnancy overweight/obesity group and the control group. Compared with those in the control group, the levels of 46 metabolites (19 positive metabolites and 27 negative metabolites) were increased in the prepregnancy overweight/obesity group, among which octopamine was the metabolite with the largest increase, followed by (2S)-4-Oxo-2-phenyl-3,4-dihydro-2H-chromen-7-yl beta-D-glucopyranoside, N-tetradecanamide, stearamide, and methanandamide (Fig. 2 A). Compared with the control group, in the prepregnancy overweight/obesity group, there were 60 metabolites (58 positive metabolites and 2 negative metabolites) with reduced concentrations, among which senecionine was the metabolite with the largest decrease, followed by 3-(methylsulfonyl)-2H-chromen-2-one, methyl EudesMate, cuminaldehyde, and 2-(tert-butyl)-1,3-thiazolane-4-carboxylic acid (Fig. 2 A).
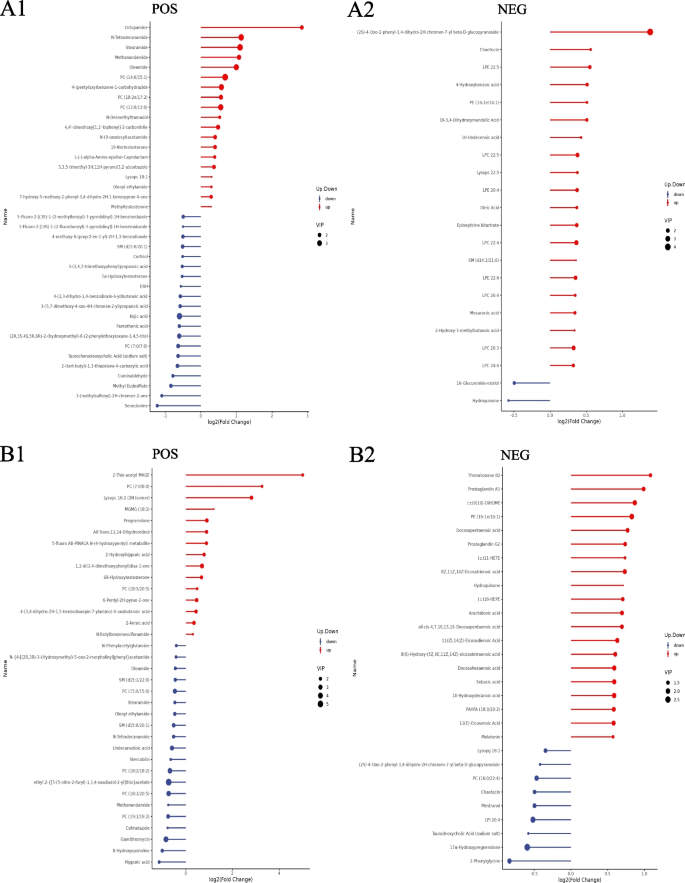
Stem plots of differential cord blood metabolites. A the prepregnancy overweight/obesity group vs. the control group; B the excessive GWG group vs. the control group. (1) positive metabolites; (2) negative metabolites. Notes: The color of the dot in the stem plots represents the upward and lower adjustment, the blue represents downward, and the red represents upward. The length of the rod represents the size of log2 (FC), and the size of the dot represents the size of the VIP value
A hierarchical analysis of the two groups of differential metabolites obtained was carried out, and the difference in metabolic expression patterns between the two groups and within the same comparison was obtained, which is shown in Fig. 3 . KEGG pathway analysis of differential cord blood metabolites associated with the prepregnancy overweight/obesity group versus the control group is shown in Table 3 and Fig. 4 A. The metabolite enrichment analysis revealed that steroid hormone biosynthesis ( P value = 0.01) and neuroactive ligand‒receptor interactions ( P value = 0.03) were the two pathways that were most altered between the prepregnancy overweight/obesity group and the control group. 19 metabolites were distributed in the pathway of steroid hormone biosynthesis, and 4 metabolites were distributed in the pathway of neuroactive ligand‒receptor interactions. In the steroid hormone biosynthesis pathway, the levels of corticosterone, 11-deoxycortisol, cortisol, testosterone, and 7α-hydroxytestosterone were decreased in the prepregnancy overweight/obesity group relative to those in the control group. In the neuroactive ligand‒receptor interaction pathway, the level of cortisol was decreased and the levels of trace amines were increased in the prepregnancy overweight/obesity group relative to the control group.
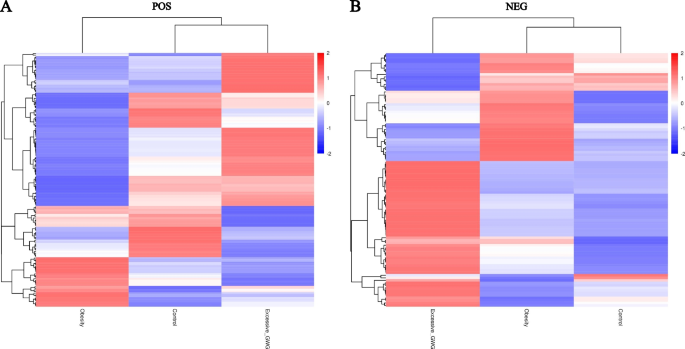
Clustering heat maps of differential cord blood metabolites of the three groups. A positive metabolites; B negative metabolites. Notes: Longitudinal clustering of samples and trans-verse clustering of metabolites. The shorter the clustering branches, the higher the similarity. Through horizontal comparison, we can see the relationship between groups of metabolite content clustering
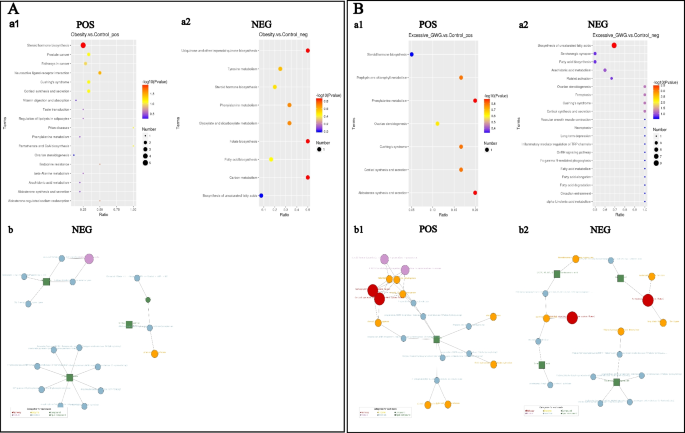
KEGG enrichment scatterplots (a) and net (b) of differential cord blood metabolites. A the prepregnancy overweight/obesity group vs. the control group; B the excessive GWG group vs. the control group. (1) positive metabolites; (2) negative metabolites. Notes: (a) The horizontal co-ordinates in the figure are x/y (the number of differential metabolites in the corresponding metabolic pathway/the total number of total metabolites identified in this pathway). The value represents the enrichment degree of differential metabolites in the pathway. The color of the point rep-resents the P -value of the hypergeometric test, and the size of the point represents the number of differential metabolites in the corresponding pathway. (b) The red dot represents a metabolic pathway, the yellow dot represents a substance-related regulatory enzyme information, the green dot represents the background substance of a metabolic pathway, the purple dot represents the molecular module information of a class of substances, the blue dot represents a substance chemical reaction, and the green square represents the differential substance obtained by this comparison
Maternal excessive GWG
A total of 109 cord blood metabolites (52 positive metabolites and 57 negative metabolites) differed between the excessive GWG group and the control group. Compared with the control group, in the excessive GWG group, there were 63 metabolites (15 positive metabolites and 48 negative metabolites) with increased concentrations, among which 2-thio-acetyl MAGE was the metabolite with the largest increase, followed by PC (7:0/8:0), lysopc 16:2 (2 N isomer), MGMG (18:2), and thromboxane B2 (Fig. 2 B). Compared with the levels in the control group, the levels of 46 metabolites (37 positive metabolites and 9 negative metabolites) in the excessive GWG group were reduced, among which hippuric acid had the largest decrease, followed by 8-hydroxyquinoline, gamithromycin, 2-phenylglycine, and cefmetazole (Fig. 2 B).
A hierarchical analysis of differential metabolites obtained in the two groups was carried out, and the difference in metabolic expression patterns between the two groups and within the same comparison was obtained, which is shown in Fig. 3 . KEGG pathway analysis of the cord blood metabolites associated with the excessive GWG group versus the control group is shown in Table 4 and Fig. 4 B. The metabolite enrichment analysis revealed that biosynthesis of unsaturated fatty acids was the most altered pathway between the excessive GWG and control groups ( P value < 0.01). There were 13 metabolites distributed in the enriched pathway. The levels of docosapentaenoic acid (DPA), docosahexaenoic acid (DHA), arachidonic acid, adrenic acid, palmitic acid, stearic acid, behenic acid, lignoceric acid, and erucic acid were increased in the excessive GWG group relative to those in the control group.
Our present study found that both maternal prepregnancy overweight/obesity and excessive GWG could affect umbilical cord blood metabolites, and they had different effects on these metabolites. Regardless of their gestational weight gain, the umbilical cord blood of prepregnancy overweight and obese mothers had 46 metabolites increased and 60 metabolites decreased compared with the umbilical cord blood of mothers with normal body weight and appropriate GWG. Steroid hormone biosynthesis and neuroactive ligand‒receptor interactions were the two top-ranking pathways enriched with these metabolites. Compared with mothers with normal prepregnancy BMI and appropriate GWG, in mothers with normal prepregnancy BMI but excessive GWG, the levels of 63 metabolites were increased and those of 46 metabolites were decreased in umbilical cord blood. Biosynthesis of unsaturated fatty acids was the most altered pathway enriched with these metabolites.
There were many differential metabolites in the cord blood between the prepregnancy overweight/obesity group and the control group and between the excessive GWG group and the control group. However, the roles of most of these differential metabolites are unknown. The levels of stearamide and methanandamide were increased in the prepregnancy overweight/obesity group. Stearamide, also known as octadecanamide or kemamide S, belongs to the class of organic compounds known as carboximidic acids. Stearamide, which is increased in the serum of patients with hepatic cirrhosis and sepsis, may be associated with the systemic inflammatory state [ 22 , 23 ]. Methanandamide is a stable analog of anandamide that participates in energy balance mainly by activating cannabinoid receptors. Methanandamide dose-dependently inhibits and excites tension-sensitive gastric vagal afferents (GVAs), which play a role in appetite regulation [ 24 ]. In mice fed a high-fat diet, only an inhibitory effect of methanandamide was observed, and GVA responses to tension were dampened [ 24 , 25 ]. These changes may contribute to the development and/or maintenance of obesity. Moreover, methanandamide can produce dose-related hypothermia and attenuate cocaine-induced hyperthermia by a cannabinoid 1-dopamine D2 receptor mechanism [ 26 ].
Metabolomic pathway analysis of the cord blood metabolite features in the prepregnancy overweight and obesity group identified two filtered significant pathways: steroid hormone biosynthesis and neuroactive ligand‒receptor interaction pathways. In the steroid hormone biosynthesis pathway, the levels of several glucocorticoids (including corticosterone, 11-deoxycortisol, cortisol, testosterone, and 7α-hydroxytestosterone) were decreased in the prepregnancy overweight/obesity group. In addition to the physiological role of glucocorticoids in the healthy neuroendocrine development and maturation of fetuses and babies, glucocorticoids are essential to human health by regulating different physiological events in mature organs and tissues, such as glucose metabolism, lipid biosynthesis and distribution, food intake, thermogenesis, and mood and learning patterns [ 27 ]. Glucocorticoids have been considered as a link between adverse early-life conditions and the development of metabolic disorders in later life [ 28 , 29 , 30 ]. However, there is still much controversy regarding the role of maternal obesity in the fetal–steroid hormone biosynthesis pathway. Studies of maternal obesity animal models showed that corticosterone and cortisol levels were increased in the offspring of obese mothers [ 31 , 32 ]. A study reported by Satu M Kumpulainen et al. showed that young adults born to mothers with higher early pregnancy BMIs show lower average levels of diurnal cortisol, especially in the morning [ 33 ]. Laura I. Stirrat et al. found that increased maternal BMI was associated with lower maternal cortisol, corticosterone, and 11-dehydrocorticosterone levels. However, there were no associations between maternal BMI and glucocorticoid levels in the cord blood [ 34 ]. The differences in the study protocols of these previous studies may explain the mixed findings, such as cortisol measured from peripheral blood, cord blood or saliva; variation in measurement time points; the number of samples. Although the effect of maternal obesity on fetal steroid hormone levels is controversial, dysregulation of glucocorticoids may be a plausible mechanism by which maternal obesity can increase the risk of metabolic disorders and mental health disorders in offspring.
The effect of excessive GWG on umbilical cord blood metabolites is different from that of maternal overweight and obesity. Compared with the control group, in the excessive GWG group, the level of thromboxane B2 was increased and the level of hippuric acid was decreased. Thromboxane B2, which is important in the platelet release reaction, is a stable, physiologically active compound formed in vivo from prostaglandin endoperoxides. Hippuric acid is an acyl glycine formed from the conjugation of benzoic acid with glycine. Several studies have confirmed that both thromboxane B2 and hippuric acid levels are associated with diet. Dietary fatty acids affect platelet thromboxane production [ 35 , 36 , 37 ]. In our study, several fatty acids (e.g., palmitic acid, stearic acid, behenic acid, and lignoceric acid) in the excessive GWG group were also increased, which may have led to the increase in thromboxane B2 levels. Hippuric acid can be detected after the consumption of whole grains and anthocyanin-rich bilberries [ 38 , 39 ]. A healthy diet intervention increased the signals for hippuric acid to incorporate polyunsaturated fatty acids [ 38 ], and the low level of hippuric acid was associated with lower fruit-vegetable intakes [ 39 ]. Maternal overnutrition and unhealthy dietary patterns are the main reasons for excessive GWG [ 40 , 41 ]. Therefore, we speculated that the differences in thromboxane B2 and hippuric acid between the excessive GWG and control groups were associated with maternal diet during pregnancy. The effect of these differential metabolites on the long-term metabolic health of offspring after birth needs further study.
Metabolomic pathway analysis of the cord blood metabolite features in the excessive GWG group identified that biosynthesis of unsaturated fatty acids was the filtered significant pathway. The levels of several fatty acids in this pathway were increased in the excessive GWG group, including long-chain saturated fatty acids (e.g., palmitic acid (C 16:0), stearic acid (C 18:0), behenic acid (C 22:0), and lignoceric acid (C 23:0)), monounsaturated fatty acids (erucic acid), and polyunsaturated fatty acids (e.g., DPA, DHA, arachidonic acid, and adrenic acid). Because perinatal fatty acid status can be influenced by maternal dietary modifications or supplementation [ 42 ], we speculated that maternal diet during pregnancy caused the difference in umbilical cord blood fatty acids between the excessive GWG and control groups. A large body of evidence from mechanistic studies supports the potential of fatty acids to influence later obesity. However, the possible mechanisms and observed relationships are complex and related to the types and patterns of fatty acids [ 43 , 44 ]. Maternal dietary fatty acids have been found to induce hypothalamic inflammation, cause epigenetic changes, and alter the mechanisms of energy control in offspring [ 43 ]. Evidence from cell culture and rodent studies showed that polyunsaturated fatty acids might serve several complex roles in fetuses, including the stimulation and/or inhibition regulation of adipocyte differentiation [ 44 ]. The questions of whether lower n-6 long-chain polyunsaturated fatty acid levels or higher n-3 long-chain polyunsaturated fatty acid levels are of more relevance and whether the long-term effects differ with different offspring ages remain [ 44 ]. Although there is a biologically plausible case for the relevance of perinatal fatty acid status in later obesity risk, available data in humans suggest that the influence of achievable modification of perinatal n-3/n-6 status is not sufficient to influence offspring obesity risk in the general population [ 45 ]. Further studies seem justified to clarify the reasons.
The advantage of our present study is that we simultaneously analyzed the effects of prepregnancy overweight/obesity and excessive GWG on cord blood metabolites and explored their differences. In addition, to exclude the effect of hyperglycemia on cord blood metabolites, both women with prepregnancy diabetes mellitus and gestational diabetes mellitus were excluded from our study. The limitation of our study is that it was a single-center study with a small sample, especially in the prepregnancy overweight/obesity group. In the future, we can expand the sample size and conduct a subgroup analysis of the prepregnancy overweight/obesity group and analyze the differences in the effects of different degrees of obesity on cord blood metabolites. The prepregnancy overweight/obesity group can be further divided into an appropriate GWG group and an excessive GWG group, and the differences in the effects of these two groups on umbilical cord blood metabolites can be analyzed. Moreover, the dietary pattern of the pregnant woman could affect the production of cord blood metabolites. We did not investigate the dietary patterns of the mothers in this study, which is another limitation of this study. In future studies, we should investigate maternal dietary patterns as a very important confounding variable.
In conclusion, our present study confirmed that both prepregnancy overweight/obesity and excessive GWG could affect umbilical cord blood metabolites, and they had different effects on these metabolites. Prepregnancy overweight and obesity affected the fetal steroid hormone biosynthesis pathway, while normal prepregnancy body weight but excessive GWG affected fetal fatty acid metabolism. This emphasizes the importance of preconception weight loss and maintaining an appropriate GWG, which are beneficial for the long-term metabolic health of offspring.
Availability of data and materials
Data sets generated during the current study are not publicly available but will be available from the corresponding author at a reasonable request. Responses to the request for the raw data will be judged by a committee including XXY and GHL.
Abbreviations
Excessive gestational weight gain
Ultrahigh-performance liquid chromatography tandem mass spectrometry
Type 2 diabetes mellitus
Nonalcoholic fatty liver disease
The developmental origins of health and disease
Body mass index
Diabetes mellitus
Gestational diabetes mellitus
Oral glucose tolerance test
Low birth weight
Standard deviation
The Human Metabolome Database
Kyoto Encylopaedia of Genes and Genomes
Principal component analysis
Partial least-squares discriminant analysis
Importance for the projection
Fold change
Invitro fertilization and embryo transfer
Triglyceride
Docosapentaenoic acid
Docosahexaenoic acid
Gastric vagal afferents
Collaborators GBDO, Afshin A, Forouzanfar MH, Reitsma MB, Sur P, Estep K, Lee A, Marczak L, Mokdad AH, Moradi-Lakeh M, et al. Health effects of overweight and obesity in 195 countries over 25 years. N Engl J Med. 2017;377(1):13–27.
Article Google Scholar
Bjerregaard LG, Jensen BW, Angquist L, Osler M, Sorensen TIA, Baker JL. Change in overweight from childhood to early adulthood and risk of type 2 diabetes. N Engl J Med. 2018;378(14):1302–12.
Article PubMed Google Scholar
Sharma V, Coleman S, Nixon J, Sharples L, Hamilton-Shield J, Rutter H, Bryant M. A systematic review and meta-analysis estimating the population prevalence of comorbidities in children and adolescents aged 5 to 18 years. Obes Rev. 2019;20(10):1341–9.
Article PubMed PubMed Central Google Scholar
Llewellyn A, Simmonds M, Owen CG, Woolacott N. Childhood obesity as a predictor of morbidity in adulthood: a systematic review and meta-analysis. Obes Rev. 2016;17(1):56–67.
Article CAS PubMed Google Scholar
Poston L, Caleyachetty R, Cnattingius S, Corvalan C, Uauy R, Herring S, Gillman MW. Preconceptional and maternal obesity: epidemiology and health consequences. Lancet Diabetes Endocrinol. 2016;4(12):1025–36.
Pan XF, Wang L, Pan A. Epidemiology and determinants of obesity in China. Lancet Diabetes Endocrinol. 2021;9(6):373–92.
Barker DJ. The developmental origins of adult disease. J Am Coll Nutr. 2004;23(6 Suppl):588S-595S.
LifeCycle Project-Maternal O, Childhood Outcomes Study G, Voerman E, Santos S, Inskip H, Amiano P, Barros H, Charles MA, Chatzi L, Chrousos GP, et al. Association of gestational weight gain with adverse maternal and infant outcomes. JAMA. 2019;321(17):1702–15.
Aune D, Saugstad OD, Henriksen T, Tonstad S. Maternal body mass index and the risk of fetal death, stillbirth, and infant death: a systematic review and meta-analysis. JAMA. 2014;311(15):1536–46.
Ukah UV, Bayrampour H, Sabr Y, Razaz N, Chan WS, Lim KI, Lisonkova S. Association between gestational weight gain and severe adverse birth outcomes in Washington State, US: a population-based retrospective cohort study, 2004–2013. PLoS Med. 2019;16(12):e1003009.
Starling AP, Brinton JT, Glueck DH, Shapiro AL, Harrod CS, Lynch AM, Siega-Riz AM, Dabelea D. Associations of maternal BMI and gestational weight gain with neonatal adiposity in the Healthy Start study. Am J Clin Nutr. 2015;101(2):302–9.
Voerman E, Santos S, Patro Golab B, Amiano P, Ballester F, Barros H, Bergstrom A, Charles MA, Chatzi L, Chevrier C, et al. Maternal body mass index, gestational weight gain, and the risk of overweight and obesity across childhood: an individual participant data meta-analysis. PLoS Med. 2019;16(2):e1002744.
Heslehurst N, Vieira R, Akhter Z, Bailey H, Slack E, Ngongalah L, Pemu A, Rankin J. The association between maternal body mass index and child obesity: a systematic review and meta-analysis. PLoS Med. 2019;16(6):e1002817.
Newgard CB. Metabolomics and metabolic diseases: where do we stand? Cell Metab. 2017;25(1):43–56.
Johnson CH, Ivanisevic J, Siuzdak G. Metabolomics: beyond biomarkers and towards mechanisms. Nat Rev Mol Cell Biol. 2016;17(7):451–9.
Article CAS PubMed PubMed Central Google Scholar
Hivert MF, Perng W, Watkins SM, Newgard CS, Kenny LC, Kristal BS, Patti ME, Isganaitis E, DeMeo DL, Oken E, et al. Metabolomics in the developmental origins of obesity and its cardiometabolic consequences. J Dev Orig Health Dis. 2015;6(2):65–78.
Schlueter RJ, Al-Akwaa FM, Benny PA, Gurary A, Xie G, Jia W, Chun SJ, Chern I, Garmire LX. Prepregnant obesity of mothers in a multiethnic cohort is associated with cord blood metabolomic changes in offspring. J Proteome Res. 2020;19(4):1361–74.
Shokry E, Marchioro L, Uhl O, Bermudez MG, Garcia-Santos JA, Segura MT, Campoy C, Koletzko B. Impact of maternal BMI and gestational diabetes mellitus on maternal and cord blood metabolome: results from the PREOBE cohort study. Acta Diabetol. 2019;56(4):421–30.
Chen C, Lu FC, Department of Disease Control Ministry of Health PRC. The guidelines for prevention and control of overweight and obesity in Chinese adults. Biomed Environ Sci. 2004;17(Suppl):1–36.
PubMed Google Scholar
The American College of Obstetricians and Gynecologists. Macrosomia: ACOG practice bulletin, number 216. Obstet Gynecol. 2020;135(1):e18–e35.
Goldenberg RL, Culhane JF. Low birth weight in the United States. Am J Clin Nutr. 2007;85(2):584S-590S.
Lian JS, Liu W, Hao SR, Guo YZ, Huang HJ, Chen DY, Xie Q, Pan XP, Xu W, Yuan WX, et al. A serum metabonomic study on the difference between alcohol- and HBV-induced liver cirrhosis by ultraperformance liquid chromatography coupled to mass spectrometry plus quadrupole time-of-flight mass spectrometry. Chin Med J (Engl). 2011;124(9):1367–73.
CAS PubMed Google Scholar
Ding W, Xu S, Zhou B, Zhou R, Liu P, Hui X, Long Y, Su L. Dynamic plasma lipidomic analysis revealed cholesterol ester and amides associated with sepsis development in critically Ill patients after cardiovascular surgery with cardiopulmonary bypass. J Pers Med. 2022;12(11):1838.
Christie S, O’Rielly R, Li H, Nunez-Salces M, Wittert GA, Page AJ. Modulatory effect of methanandamide on gastric vagal afferent satiety signals depends on nutritional status. J Physiol. 2020;598(11):2169–82.
Christie S, O’Rielly R, Li H, Wittert GA, Page AJ. High fat diet induced obesity alters endocannabinoid and ghrelin mediated regulation of components of the endocannabinoid system in nodose ganglia. Peptides. 2020;131:170371.
Rasmussen BA, Kim E, Unterwald EM, Rawls SM. Methanandamide attenuates cocaine-induced hyperthermia in rats by a cannabinoid CB1-dopamine D2 receptor mechanism. Brain Res. 2009;1260:7–14.
Facchi JC, Lima TAL, Oliveira LR, Costermani HO, Miranda GDS, de Oliveira JC. Perinatal programming of metabolic diseases: the role of glucocorticoids. Metabolism. 2020;104:154047.
Reynolds RM, Walker BR, Syddall HE, Andrew R, Wood PJ, Whorwood CB, Phillips DI. Altered control of cortisol secretion in adult men with low birth weight and cardiovascular risk factors. J Clin Endocrinol Metab. 2001;86(1):245–50.
Valtat B, Dupuis C, Zenaty D, Singh-Estivalet A, Tronche F, Breant B, Blondeau B. Genetic evidence of the programming of beta cell mass and function by glucocorticoids in mice. Diabetologia. 2011;54(2):350–9.
Jia Y, Li R, Cong R, Yang X, Sun Q, Parvizi N, Zhao R. Maternal low-protein diet affects epigenetic regulation of hepatic mitochondrial DNA transcription in a sex-specific manner in newborn piglets associated with GR binding to its promoter. PLoS ONE. 2013;8(5):e63855.
Rodriguez JS, Rodriguez-Gonzalez GL, Reyes-Castro LA, Ibanez C, Ramirez A, Chavira R, Larrea F, Nathanielsz PW, Zambrano E. Maternal obesity in the rat programs male offspring exploratory, learning and motivation behavior: prevention by dietary intervention pre-gestation or in gestation. Int J Dev Neurosci. 2012;30(2):75–81.
Tuersunjiang N, Odhiambo JF, Long NM, Shasa DR, Nathanielsz PW, Ford SP. Diet reduction to requirements in obese/overfed ewes from early gestation prevents glucose/insulin dysregulation and returns fetal adiposity and organ development to control levels. Am J Physiol Endocrinol Metab. 2013;305(7):E868-878.
Kumpulainen SM, Heinonen K, Kaseva N, Andersson S, Lano A, Reynolds RM, Wolke D, Kajantie E, Eriksson JG, Raikkonen K. Maternal early pregnancy body mass index and diurnal salivary cortisol in young adult offspring. Psychoneuroendocrinology. 2019;104:89–99.
Stirrat LI, Just G, Homer NZM, Andrew R, Norman JE, Reynolds RM. Glucocorticoids are lower at delivery in maternal, but not cord blood of obese pregnancies. Sci Rep. 2017;7(1):10263.
Prisco D, Filippini M, Francalanci I, Paniccia R, Gensini GF, Serneri GG. Effect of n-3 fatty acid ethyl ester supplementation on fatty acid composition of the single platelet phospholipids and on platelet functions. Metabolism. 1995;44(5):562–9.
Kaapa P, Uhari M, Nikkari T, Viinikka L, Ylikorkala O. Dietary fatty acids and platelet thromboxane production in puerperal women and their offspring. Am J Obstet Gynecol. 1986;155(1):146–9.
Teng KT, Chang CY, Kanthimathi MS, Tan AT, Nesaretnam K. Effects of amount and type of dietary fats on postprandial lipemia and thrombogenic markers in individuals with metabolic syndrome. Atherosclerosis. 2015;242(1):281–7.
Hanhineva K, Lankinen MA, Pedret A, Schwab U, Kolehmainen M, Paananen J, de Mello V, Sola R, Lehtonen M, Poutanen K, et al. Nontargeted metabolite profiling discriminates diet-specific biomarkers for consumption of whole grains, fatty fish, and bilberries in a randomized controlled trial. J Nutr. 2015;145(1):7–17.
Brunelli L, Davin A, Sestito G, Mimmi MC, De Simone G, Balducci C, Pansarasa O, Forloni G, Cereda C, Pastorelli R, et al. Plasmatic hippuric acid as a hallmark of frailty in an Italian cohort: the mediation effect of fruit-vegetable intake. J Gerontol A Biol Sci Med Sci. 2021;76(12):2081–9.
Ferreira LB, Lobo CV, Miranda A, Carvalho BDC, Santos LCD. Dietary patterns during pregnancy and gestational weight gain: a systematic review. Rev Bras Ginecol Obstet. 2022;44(5):540–7.
Tielemans MJ, Garcia AH, Peralta Santos A, Bramer WM, Luksa N, Luvizotto MJ, Moreira E, Topi G, de Jonge EA, Visser TL, et al. Macronutrient composition and gestational weight gain: a systematic review. Am J Clin Nutr. 2016;103(1):83–99.
Lewis RM, Wadsack C, Desoye G. Placental fatty acid transfer. Curr Opin Clin Nutr Metab Care. 2018;21(2):78–82.
Cesar HC, Pisani LP. Fatty-acid-mediated hypothalamic inflammation and epigenetic programming. J Nutr Biochem. 2017;42:1–6.
Demmelmair H, Koletzko B. Perinatal polyunsaturated fatty acid status and obesity risk. Nutrients. 2021;13(11):3882.
Hauner H, Brunner S. Early fatty acid exposure and later obesity risk. Curr Opin Clin Nutr Metab Care. 2015;18(2):113–7.
Download references
Acknowledgements
The authors thank the study participants for their involvement and research assistants for their help conducting the study.
This research was funded by the Beijing Natural Science Foundation, grant number 7214231.
Author information
Authors and affiliations.
Division of Endocrinology and Metabolism, Department of Obstetrics, Beijing Obstetrics and Gynecology Hospital, Capital Medical University, Beijing Maternal and Child Health Care Hospital, No. 251, Yaojiayuan Road, Chaoyang District, Beijing, 100026, China
Xianxian Yuan, Yuru Ma, Yan Zhao, Wei Zheng, Ruihua Yang, Lirui Zhang, Xin Yan & Guanghui Li
Department of Obstetrics and Gynecology, The Second Hospital of Jilin University, Changchun, 130041, Jilin, China
You can also search for this author in PubMed Google Scholar
Contributions
XXY designed the study. XXY, WZ, LRZ and XY analyzed the data. YRM, JW, YZ and RHY took part in data collection and management. XXY wrote the manuscript. XXY and GHL reviewed the manuscript and contributed to manuscript revision. All authors contributed to the article and approved the submitted version. All authors reviewed the manuscript.
Corresponding author
Correspondence to Guanghui Li .
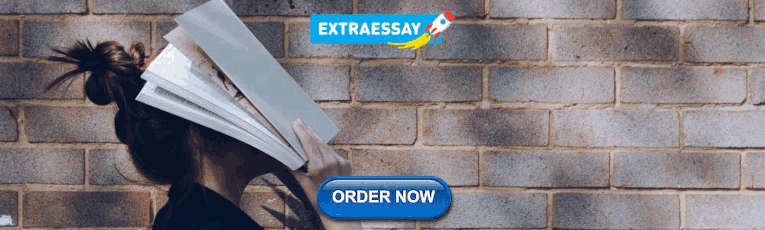
Ethics declarations
Ethics approval and consent to participate.
This study has been performed in accordance with the Declaration of Helsinki and has been approved by the ethics committee of Beijing Obstetrics and Gynecology Hospital, Capital Medical University (2021-KY-037). Informed consent was obtained from all subjects involved in the study to publish this paper. All methods were carried out in accordance with relevant guidelines and regulations in the declaration.
Consent for publication
Not applicable.
Competing interests
The authors declare no competing interests.
Additional information
Publisher’s note.
Springer Nature remains neutral with regard to jurisdictional claims in published maps and institutional affiliations.
Supplementary Information
Supplementary material 1., rights and permissions.
Open Access This article is licensed under a Creative Commons Attribution 4.0 International License, which permits use, sharing, adaptation, distribution and reproduction in any medium or format, as long as you give appropriate credit to the original author(s) and the source, provide a link to the Creative Commons licence, and indicate if changes were made. The images or other third party material in this article are included in the article's Creative Commons licence, unless indicated otherwise in a credit line to the material. If material is not included in the article's Creative Commons licence and your intended use is not permitted by statutory regulation or exceeds the permitted use, you will need to obtain permission directly from the copyright holder. To view a copy of this licence, visit http://creativecommons.org/licenses/by/4.0/ . The Creative Commons Public Domain Dedication waiver ( http://creativecommons.org/publicdomain/zero/1.0/ ) applies to the data made available in this article, unless otherwise stated in a credit line to the data.
Reprints and permissions
About this article
Cite this article.
Yuan, X., Ma, Y., Wang, J. et al. The influence of maternal prepregnancy weight and gestational weight gain on the umbilical cord blood metabolome: a case–control study. BMC Pregnancy Childbirth 24 , 297 (2024). https://doi.org/10.1186/s12884-024-06507-x
Download citation
Received : 30 September 2023
Accepted : 11 April 2024
Published : 22 April 2024
DOI : https://doi.org/10.1186/s12884-024-06507-x
Share this article
Anyone you share the following link with will be able to read this content:
Sorry, a shareable link is not currently available for this article.
Provided by the Springer Nature SharedIt content-sharing initiative
- Maternal obesity
- Gestational weight gain
- Offspring health
- Metabolites
- Umbilical cord blood
BMC Pregnancy and Childbirth
ISSN: 1471-2393
- Submission enquiries: [email protected]
- General enquiries: [email protected]
- Open access
- Published: 19 April 2024
GbyE: an integrated tool for genome widely association study and genome selection based on genetic by environmental interaction
- Xinrui Liu 1 , 2 ,
- Mingxiu Wang 1 ,
- Jie Qin 1 ,
- Yaxin Liu 1 ,
- Shikai Wang 1 ,
- Shiyu Wu 1 ,
- Ming Zhang 1 ,
- Jincheng Zhong 1 &
- Jiabo Wang 1
BMC Genomics volume 25 , Article number: 386 ( 2024 ) Cite this article
257 Accesses
Metrics details
The growth and development of organism were dependent on the effect of genetic, environment, and their interaction. In recent decades, lots of candidate additive genetic markers and genes had been detected by using genome-widely association study (GWAS). However, restricted to computing power and practical tool, the interactive effect of markers and genes were not revealed clearly. And utilization of these interactive markers is difficult in the breeding and prediction, such as genome selection (GS).
Through the Power-FDR curve, the GbyE algorithm can detect more significant genetic loci at different levels of genetic correlation and heritability, especially at low heritability levels. The additive effect of GbyE exhibits high significance on certain chromosomes, while the interactive effect detects more significant sites on other chromosomes, which were not detected in the first two parts. In prediction accuracy testing, in most cases of heritability and genetic correlation, the majority of prediction accuracy of GbyE is significantly higher than that of the mean method, regardless of whether the rrBLUP model or BGLR model is used for statistics. The GbyE algorithm improves the prediction accuracy of the three Bayesian models BRR, BayesA, and BayesLASSO using information from genetic by environmental interaction (G × E) and increases the prediction accuracy by 9.4%, 9.1%, and 11%, respectively, relative to the Mean value method. The GbyE algorithm is significantly superior to the mean method in the absence of a single environment, regardless of the combination of heritability and genetic correlation, especially in the case of high genetic correlation and heritability.
Conclusions
Therefore, this study constructed a new genotype design model program (GbyE) for GWAS and GS using Kronecker product. which was able to clearly estimate the additive and interactive effects separately. The results showed that GbyE can provide higher statistical power for the GWAS and more prediction accuracy of the GS models. In addition, GbyE gives varying degrees of improvement of prediction accuracy in three Bayesian models (BRR, BayesA, and BayesCpi). Whatever the phenotype were missed in the single environment or multiple environments, the GbyE also makes better prediction for inference population set. This study helps us understand the interactive relationship between genomic and environment in the complex traits. The GbyE source code is available at the GitHub website ( https://github.com/liu-xinrui/GbyE ).
Peer Review reports
Genetic by environmental interaction (G × E) is crucial of explaining individual traits and has gained increasing attention in research. It refers to the influence of genetic factors on susceptibility to environmental factors. In-depth study of G × E contributes to a deeper understanding of the relationship between individual growth, living environment and phenotypes. Genetic factors play a role in most human diseases at the molecular or cellular level, but environmental factors also contribute significantly. Researchers aim to uncover the mechanisms behind complex diseases and quantitative traits by investigating the interactions between organisms and their environment. Common, complex, or rare human diseases are often considered as outcomes resulting from the interplay of genes, environmental factors, and their interactions. Analyzing the joint effects of genes and the environment can provide valuable insights into the underlying pathway mechanisms of diseases. For instance, researchers have successfully identified potential loci associated with asthma risk through G × E interactions [ 1 ], and have explored predisposing factors for challenging-to-treat diseases like cancer [ 2 , 3 ], rhinitis [ 4 ], and depression [ 5 ].
However, two main methods are currently being used by breeders in agricultural production to increase crop yields and livestock productivity [ 6 ]. The first is to develop varieties with relatively low G × E effect to ensure stable production performance in different environments. The second is to use information from different environments to improve the statistical power of genome-wide association study (GWAS) to reveal potential loci of complex traits. The first method requires long-term commitment, while the second method clearly has faster returns. In GWAS, the use of multiple environments or phenotypes for association studies has become increasingly important. This not only improves the statistical power of environmental susceptibility traits[ 7 ], but also allows to detect signaling loci for G × E. There are significant challenges when using multiple environments or phenotypes for GWAS, mainly because most diseases and quantitative traits have numerous associated loci with minimal impact [ 8 ], and thus it is impossible to determine the effect size regulated by environment in these loci. The current detection strategy for G × E is based on complex statistical model, often requiring the use of a large number of samples to detect important signals [ 9 , 10 ]. In GS, breeders can use whole genome marker data to identify and select target strains in the early stages of animal and plant production [ 11 , 12 , 13 ]. Initially, GS models, similar to GWAS models, could only analyze a single environment or phenotype [ 14 ]. To improve the predictive accuracy of the models, higher marker densities are often required, allowing the proportion of genetic variation explained by these markers to be increased, indirectly obtaining higher predictive accuracy. It is worth mentioning that the consideration of G × E and multiple phenotypes in GS models [ 15 ] has been widely studied in different plant and animal breeding [ 16 ]. GS models that allow G × E have been developed [ 17 ] and most of them have modeled and interpreted G × E using structured covariates [ 18 ]. In these studies, most of the GS models provided more predictive accuracy when combined with G × E compared to single environment (or phenotype) analysis. Hence, there is need to develop models that leverage G × E information for GWAS and GS studies.
This study developed a novel genotype-by-environment method based on R, termed GbyE, which leverages the interaction among multiple environments or phenotypes to enhance the association study and prediction performance of environmental susceptibility traits. The method enables the identification of mutation sites that exhibit G × E interactions in specific environments. To evaluate the performance of the method, simulation experiments were conducted using a dataset comprising 282 corn samples. Importantly, this method can be seamlessly integrated into any GWAS and GS analysis.
Materials and methods
Support packages.
The development purpose of GbyE is to apply it to GWAS and GS research, therefore it uses the genome association and prediction integrated tool (GAPIT) [ 19 ], Bayesian Generalized Linear Regression (BGLR) [ 20 ], and Ridge Regression Best Linear Unbiased Prediction (rrBLUP) [ 21 ]package as support packages, where GbyE only provides conversion of interactive formats and file generation. In order to simplify the operation of the GbyE function package, the basic calculation package is attached to this package to support the operation of GbyE, including four function packages GbyE.Simulation.R (Dual environment phenotype simulation based on heritability, genetic correlation, and QTL quantity), GbyE.Calculate.R (For numerical genotype and phenotype data, this package can be used to process interactive genotype files of GbyE), GbyE.Power.FDR.R (Calculate the statistical power and false discovery rate (FDR) of GWAS), and GbyE.Comparison.Pvalue.R (GbyE generates redundant calculations in GWAS calculations, and SNP effect loci with minimal p -values can be filtered by this package).
Samples and sequencing data
In this study, a small volume of data was used for software simulation analysis, which is widely used in testing tasks of software such as GAPIT, TASSEL, and rMPV. The demonstration data comes from 282 inbred lines of maize, including 4 phenotypic data. In any case, there are no missing phenotypes in these data, and this dataset can be obtained from the website of GAPIT ( https://zzlab.net/GAPIT/index.html , accessed on May 1, 2022). Among them, our phenotype data was simulated using a self-made R simulation function, and the Mean and GbyE phenotype files were calculated. Convert this format to HapMap format using PLINK v1.09 and scripts written by oneself.
Simulated traits
Phenotype simulation was performed by modifying the GAPIT.Phenotype.Simulation function in the GAPIT. Based on the input parameter NQTN, the random selected markers’ genotype from whole genome were used to simulate genetic effect in the simulated trait. The genotype effects of these selected QTNs were randomly sampled from a multivariate normal distribution, the correlation value between these normal distribution was used to define the genetic relationship between each environments. The additive heritability ( \({{\text{h}}}_{{\text{g}}}^{2}\) ) was used to scale the relationship between additive genetic variance and phenotype variance. The simulated phenotype conditions in this paper are set as follows: 1) The three levels of \({{\text{h}}}_{{\text{g}}}^{2}\) were set at 0.8, 0.5, and 0.2, representing high ( \({{\text{h}}}_{{\text{h}}}^{2}\) ), median ( \({{\text{h}}}_{{\text{m}}}^{2}\) ) and low ( \({{\text{h}}}_{{\text{l}}}^{2}\) ) heritability; 2) Genetic correlation were set three levels 0.8, 0.5, 0.2 representing high ( \({{\text{R}}}_{{\text{h}}}\) ), medium ( \({{\text{R}}}_{{\text{m}}}\) ) and low ( \({{\text{R}}}_{{\text{l}}}\) ) genetic correlation; 3) 20 pre-set effect loci of QTL. The phenotype values in each environment were simulated together following above parameters.
Genetic by environment interaction model
The pipeline analysis process of GbyE includes three steps: data preprocessing, production converted, Association analysis. Normalize the phenotype data matrix Y of the dual environment and perform GbyE conversion to generate phenotype data in GbyE.Y format. The genotype data format, such as hapmap, vcf, bed and other formats firstly need to be converted into numerical genotype format (homozygotes were coded as 0 or 2, heterozygotes were coded as 1) using software or scripts such as GAPIT, PLINK, etc. The environment (E) matrix is environment index matrix. The G (n × m) originally of genotype matrix was converted as GbyE.GD(2n × 2 m) \(\left[\begin{array}{cc}G& 0\\ G& G\end{array}\right]\) during the Kronecker product, and the Y vector (n × 1) was also converted as the GbyE.Y vector (2n × 1) after normalization. The duplicated data format indicated different environments, genetic effect, and populations. The genomic data we used in the analysis was still retained the whole genome information. The first column of E is the additive effect, which was the average genetic effect among environments. The others columns of E are the interactive effect, which should be less one column than the number of environments. Because it need to avoid the linear dependent in the model. In the GbyE algorithm, we coded the first environment as background as default, that means the genotype in the first environment are 0, the others are 1. Then the Kronecker product of G and environment index matrix was named as GbyE.GD. The interactive effect part of the GbyE.GD matrix in the GWAS and GS were the relative values based on the first environment (Fig. 1 ). The GbyE environmental interaction matrix can be easily obtained by constructing the interaction matrix E (e.g., Eq. 1 ) such that the genotype matrix G is Kronecker-product with the design interaction matrix E (e.g., Eq. 2 ), in which \(\left[\begin{array}{c}G\\ G\end{array}\right]\) matrix is defined as additive effect and \(\left[\begin{array}{c}0\\ G\end{array}\right]\) matrix is defined as interactive effect. \(\left[\begin{array}{cc}G& 0\\ G& G\end{array}\right]\) matrix is called gene by environment interaction matrix, hereinafter referred to as the GbyE matrix. The phenotype file (GbyE.Y) and genotype file (GbyE.GD) after transformation by GbyE will be inputted into the GWAS and GS models and computed as standard phenotype and genotype files.
where G is the matrix of whole genotype and E is the design matrix for exploring interactive effects. GbyE mainly uses the Kronecker product of the genetic matrix (G) and the environmental matrix (E) as the genotype for subsequent GWAS as a way to distinguish between additive and interactive effects.
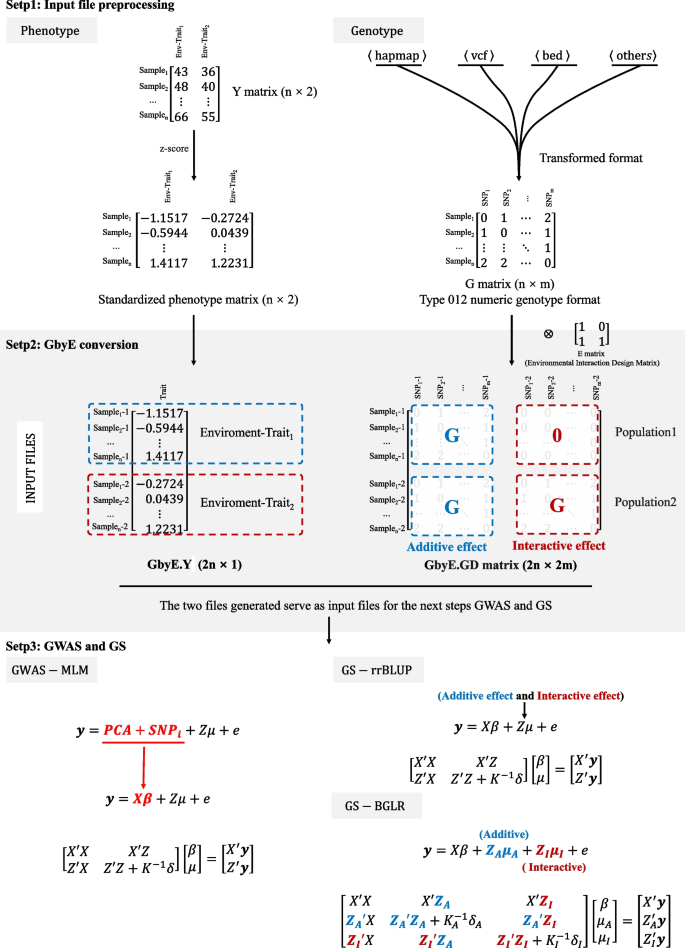
The workflow pipeline of GbyE. The GbyE contains three main steps. (Step 1) Preprocessing of phenotype and genotype data,. The phenotype values in each environment was normalized respectively. Meanwhile, all genotype from HapMap, VCF, BED, and other types were converted to numeric genotype; (Step 2) Generate GbyE phenotype and interactive genotype matrix through the transformation of GbyE. In GbyE.GD matrix, the blue characters indicate additive effect, and red ones indicate interactive effect; (Step 3) The MLM and rrBLUP and BGLR were used to perform GWAS and GS
Association analysis model
The mixed linear model (MLM) of GAPIT is used as the basic model for GWAS analysis, and the principal component analysis (PCA) parameter is set to 3. Then the p -values of detection results are sorted and their power and FDR values are calculated. General expression of MLM (Fig. 1 ):
where Y is the vector of phenotypic measures (2n × 1); PCA and SNP i were defined as fixed effects, with a size of (2n × 2 m); Z is the incidence matrix of random effects; μ is the random effect vector, which follows the normal distribution μ ~ N(0, \({\delta }_{G}^{2}\) K) with mean vector of 0 and variance covariance matrix of \({\delta }_{G}^{2}\) K, where the \({\delta }_{G}^{2}\) is the total genetic variance including additive variance and interactive variance, the K is the kinship matrix built with all genotype including additive genotype and interactive genotype; e is a random error vector, and its elements need not be independent and identically distributed, e ~ N(0, \({\delta }_{e}^{2}\) I), where the \({\delta }_{e}^{2}\) is the residual and environment variance, the I is the design matrix.
Detectivity of GWAS
In the GWAS results, the list of markers following the order of P-values was used to evaluate detectivity of GWAS methods. When all simulated QTNs were detected, the power of the GWAS method was considered as 1 (100%). From the list of markers, following increasing of the criterion of real QTN, the power values will be increasing. The FDR indicates the rate between the wrong criterion of real QTNs and the number of all un-QTNs. The mean of 100 cycles was used to consider as the reference value for statistical power comparison. Here, we used a commonly used method in GWAS research with multiple traits or environmental phenotypes as a comparison[ 22 ]. This method obtains the mean of phenotypic values under different conditions as the phenotypic values for GWAS analysis, called the Mean value method, Compare the calculation results of GbyE with the additive and interactive effects of the mean method to evaluate the detection power of the GbyE strategy. Through the comprehensive analysis of these evaluation indicators, we aim to comprehensively evaluate the statistical power of the GbyE strategy in GWAS and provide a reference for future optimization research.
Among them, the formulae for calculating Power and FDR are as follows:
where \({{\text{n}}}_{{\text{i}}}\) indicates whether the i-th detection is true, true is 1, false is 0; \({{\text{m}}}_{{\text{r}}}\) is the total number of all true QTLs in the sample size; the maximum value of Power is 1.
where \({{\text{N}}}_{{\text{i}}}\) represents the i-th true value detected in the pseudogene, true is 1, false is 0. and cumulative calculation; \({{\text{M}}}_{{\text{f}}}\) is the number of all labeled un-QTNs in the total samples; the maximum value of FDR is 1.
Genomic prediction
To comparison the prediction accuracy of different GS models using GbyE, we performed rrBLUP, Bayesian methods using R packages. All phenotype of reference population and genotype of all population were used to train the model and predict genomic estimated breeding value (gEBV) of all individuals. The correlation between real phenotypes and gEBV of inference population was considered as prediction accuracy. fivefold cross-validation and 100 times repeats was performed to avoid over prediction and reduce bias. In order to distinguish the additive and interactive effects in GbyE, we designed two lists of additive and interactive effects in the "ETA" of BGLR, and put the additive and interactive effects into the model as two kinships for random objects. However, it was not possible to load the gene effects of the two lists in rrBLUP, so the additive and interactive genotypes together were used to calculate whole genetic kinship in rrBLUP (Fig. 1 ). Relevant parameters in BGLR are set as follows: 1) model set to "RRB"; 2) nIter is set to "12000"; 3) burnIn is set to "10000". The results of the above operations are averaged over 100 cycles. We also validated the GbyE method using four other Bayesian methods (BayesA, BayesB, BayesCpi, and Bayesian LASSO) in addition to RRB in BGLR.
Partial missing phentoype in the prediction
In this study, we artificially missed phenotype values in the single and double environments in the whole population from 281 inbred maize datasets. In the missing single environment case, the inference set in the cross-validation was selected from whole population, and each individual in the inference were only missed phenotypes in the one environment. The phenotype in the other environment was kept. The genotypes were always kept. In the case of missing double environments, both phenotypes and genotypes of environment 1 and environment 2 are missing, and the model can only predict phenotypic values in the two missing environments through the effects of other markers. In addition, the data were standardized and unstandardized to assess whether standardization had an effect on the estimation of the model. This experiment was tested using the "ML" method in rrBLUP to ensure the efficiency of the model.
GWAS statistical power of models at different heritabilities and genetic correlations
Power-FDR plots were used to demonstrate the detection efficiency of GbyE at three genetic correlation and three genetic power levels, with a total of nine different scenarios simulated (from left to right for high and low genetic correlation and from top to bottom for high and low genetic power). In order to distinguish whether the effect of improving the detection ability of genome-wide association analysis in GbyE is an additive effect or an effect of environmental interactions, we plotted their Power-FDR curves separately and added the traditional Mean method for comparative analysis. As shown in Fig. 2 , GbyE algorithm can detect more statistically significant genetic loci with lower FDR under any genetic background. However, in the combination with low heritability (Fig. 2 A, B, C), the interactive effect detected more real loci than GbyE under low FDR, but with the continued increase of FDR, GbyE detected more real loci than other groups. Under the combination with high heritability, all groups have high statistical power at low FDR, but with the increase of FDR, the statistical effect of GbyE gradually highlights. From the analysis of heritability combinations at all levels, the effect of heritability on interactive effect is not obvious, but GbyE always maintains the highest statistical power. The average detection power of GWAS in GbyE can be increased by about 20%, and with the decrease of genetic correlation, the effect of GbyE gradually highlights, indicating that the G × E plays a role.
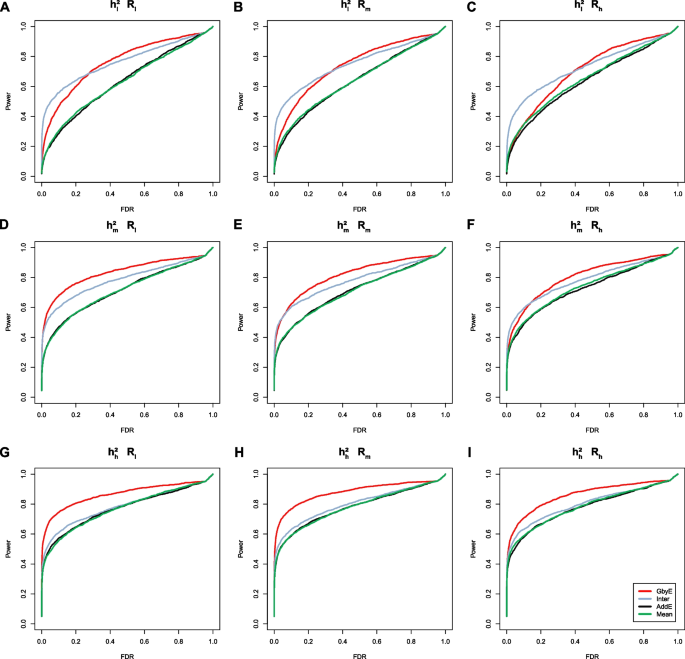
The power-FDR testing in simulated traits. Comparing the efficacy of the GbyE algorithm with the conventional mean method in terms of detection power and FDR. From left to right, the three levels of genetic correlation are indicated in order of low, medium and high. From top to bottom, the three levels of heritability, low, medium and high, are indicated in order. (1) Inter: Interactive section extracted from GbyE; (2) AddE: Additive section extracted from GbyE; (3) \({{\text{h}}}_{{\text{l}}}^{2}\) , \({{\text{h}}}_{{\text{m}}}^{2}\) , \({{\text{h}}}_{{\text{g}}}^{2}\) : Low, medium, high heritability; (4) \({{\text{R}}}_{{\text{l}}}\) , \({{\text{R}}}_{{\text{m}}}\) , \({{\text{R}}}_{{\text{l}}}\) : where R stands for genetic correlation, represents three levels of low, medium and high
Resolution of additive and interactive effect
The output results of GbyE could be understood as resolution of additive and interactive genetic effect. Hence, we created a combined Manhattan plots with Mean result from MLM, additive, and interactive results from GbyE. As shown in Fig. 3 , true marker loci were detected on chromosomes 1, 6 and 9 in Mean, and the same loci were detected on chromosomes 1 and 6 for the additive result in GbyE (the common loci detected jointly by the two results were marked as solid gray lines in the figure). All known pseudo QTNs were labeled with gray dots in the circle. Total 20 pseudo QTNs were simulated in such trait (The heritability is set to 0.9, and the genetic correlation is set to 0.1). Although the additive section in GbyE did not catch the locus on chromosome 9 yet (those p-values of markers did not show above the significance threshold (p-value < 3.23 × 10 –6 )), it has shown high significance relative to other markers of the same chromosome. In the reciprocal effect of GbyE, we detected more significant loci on chromosomes 1, 2, 3 and 10, and these loci were not detected in either of the two previous sections. An integrate QQ plot (Fig. 3 D) shows that the overall statistical power of the additive section in Mean and GbyE are close, nevertheless, the interactive section in the GbyE provided a bit of inflation.
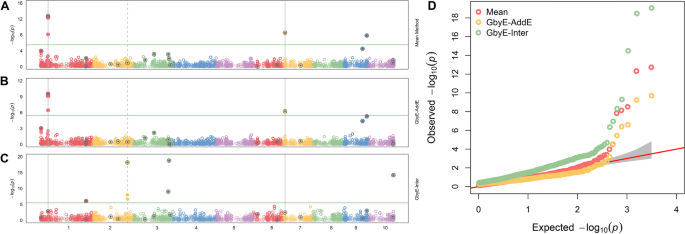
Manhattan statistical comparison plot. Manhattan comparison plots of mean ( A ), additive ( B ) and gene-environment interactive sections ( C ) at a heritability of 0.9 and genetic correlation of 0.1. Different colors are used in the diagram to distinguish between different chromosomes (X-axis). Loci with reinforcing circles and centroids are set up as real QTN loci. Consecutive loci found in both parts are shown as id lines, and loci found separately in the reciprocal effect only are shown as dashed lines. Parallel horizontal lines indicate significance thresholds ( p -value < 3.23 × 10 –6 ). D Quantile–quantile plots of simulated phenotypes for demo data from genome-wide association studies. x-axis indicates expected values of log p -values and y-axis is observed values of log p -values. The diagonal coefficients in red are 1. GbyE-inter is the interactive section in GbyE; GbyE-AddE is the additive section in GbyE
Genomic selection in assumption codistribution
The prediction accuracy of GbyE was significantly higher than the Mean value method by model statistics of rrBLUP in most cases of heritability and genetic correlation (Fig. 4 ). The prediction accuracy of the additive effect was close to that of Mean value method, which was consistent with the situation under the low hereditary. The prediction accuracy of interactive sections in GbyE remains at the same level as in GbyE, and interactive section plays an important role in the model. We observed that in \({{\text{h}}}_{{\text{l}}}^{2}{{\text{R}}}_{{\text{h}}}\) (Fig. 4 C), \({{\text{h}}}_{{\text{m}}}^{2}{{\text{R}}}_{{\text{h}}}\) (Fig. 4 F), \({{\text{h}}}_{{\text{h}}}^{2}{{\text{R}}}_{{\text{l}}}\) (Fig. 4 G), the prediction accuracy of GbyE was slightly higher than the Mean value method, but there was no significant difference overall. In addition, we only observed that the prediction accuracy of GbyE was slightly lower than the Mean value method in \({{\text{h}}}_{{\text{h}}}^{2}{{\text{R}}}_{{\text{l}}}\) (Fig. 4 H), but there was still no significant difference between GbyE and Mean value methods. Under the combination of low heritability and genetic correlation, the prediction accuracy of Mean value method and additive effect model remained at a similar level. However, with the continuous increase of heritability and genetic correlation, the difference in prediction accuracy between the two gradually increases. In summary, the GbyE algorithm can improve the accuracy of GS by capturing information on multiple environment or trait effects under the rrBLUP model.
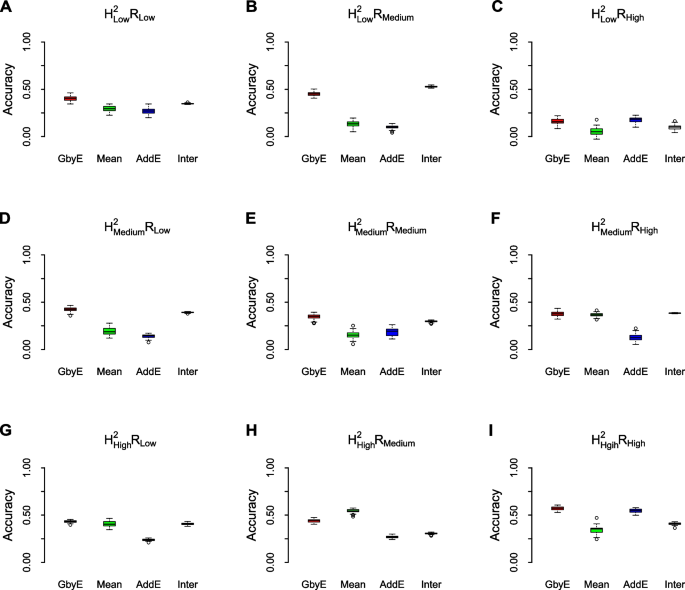
Box-plot of model prediction accuracy. The prediction accuracy (pearson's correlation coefficient) of the GbyE algorithm was compared with the tradition al Mean value method in a simulation experiment of genomic selection under the rrBLUP operating environment. The effect of different levels of heritability and genetic correlation on the prediction accuracy of genomic selection was simulated in this experiment. Each row from top to bottom represents low heritability ( \({{\text{h}}}_{{\text{l}}}^{2}\) ), medium heritability ( \({{\text{h}}}_{{\text{m}}}^{2}\) ) and high heritability ( \({{\text{h}}}_{{\text{h}}}^{2}\) ), respectively; each column from left to right represents low genetic correlation ( \({{\text{R}}}_{{\text{l}}}\) ), medium genetic correlation ( \({{\text{R}}}_{{\text{m}}}\) ) and high genetic correlation ( \({{\text{R}}}_{{\text{h}}}\) ), respectively; The X-axis shows the different test methods and effects, and the Y-axis shows the prediction accuracy
Genomic selection in assumption un-codistribution
The overall performance of GbyE under the 'BRR' statistical model based on the BGLR package remained consistent with rrBLUP, maintaining high predictive accuracy in most cases of heritability and genetic relatedness (Fig. S1 ). However, when the heritability is set to low and medium, the difference between the prediction accuracy of GbyE algorithm and Mean value method gradually decreases with the continuous increase of genetic correlation, and there is no statistically significant difference between the two. The prediction accuracy of the model by GbyE in \({{\text{h}}}_{{\text{h}}}^{2}{{\text{R}}}_{{\text{l}}}\) (Fig. S1 G) and \({{\text{h}}}_{{\text{h}}}^{2}{{\text{R}}}_{{\text{h}}}\) (Fig. S1 I) is significantly higher than that by Mean value method when the heritability is set to be high. On the contrary, when the genetic correlation is set to medium, there is no significant difference between GbyE and Mean value method in improving the prediction accuracy of the model, and the overall mean of GbyE is lower than Mean. When GbyE has relatively high heritability and low genetic correlation, its prediction accuracy is significantly higher than the mean method, such as \({{\text{h}}}_{{\text{m}}}^{2}{{\text{R}}}_{{\text{l}}}\) (Fig. S1 D), \({{\text{h}}}_{{\text{h}}}^{2}{{\text{R}}}_{{\text{l}}}\) (Fig. S1 G), and \({{\text{h}}}_{{\text{h}}}^{2}{{\text{R}}}_{{\text{m}}}\) (Fig. S1 H). Therefore, GbyE is more suitable for situations with high heritability and low genetic correlation.
Adaptability of Bayesian models
Next, we tested a more complex Bayesian model. The GbyE algorithm and Mean value method were combined with five Bayesian algorithms in BGLR for GS analysis, and the computing R script was used for phenotypic simulation test, where heritability and genetic correlation were both set to 0.5. The results indicate that among the three Bayesian models of RRB, BayesA, and BayesLASSO, the predictive accuracy of GbyE is significantly higher than that of Mean value method (Fig. 5 ). In contrast, under the Bayesian models of BayesB and BayesCpi, the prediction accuracy of GbyE is lower than that of the Mean value method. The GbyE algorithm improves the prediction accuracy of the three Bayesian models BRR, BayesA, and BayesLASSO using information from G × E and increases the prediction accuracy by 9.4%, 9.1%, and 11%, respectively, relative to the Mean value method. However, the predictive accuracy of the BayesB model decreased by 11.3%, while the BayescCpi model decreased by 6%.
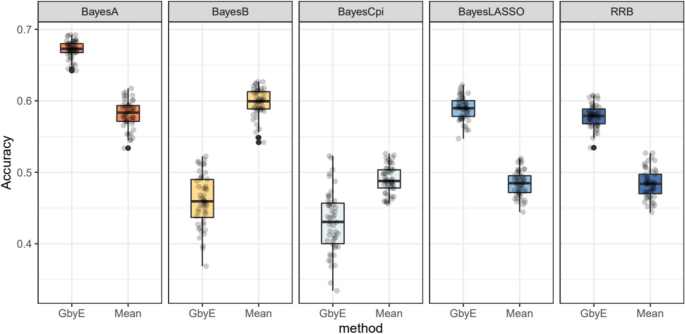
Relative prediction accuracy histogram for different Bayesian models. The X-axis is the Bayesian approach based on BGLR, and the Y-axis is the relative prediction accuracy. Where we normalize the prediction accuracy of Mean (the prediction accuracy is all adjusted to 1); the prediction accuracy of GbyE is the increase or decrease value relative to Mean in the same group of models
Impact of all and partial environmental missing
We tested missing the environmental by using simulated data. In the case of the simulated data, we simulated a total of nine situations with different heritability and genetic correlations (Fig. 6 ) and conducted tests on single and dual environment missing. The improvement in prediction accuracy by the GbyE algorithm was found to be significantly higher than the Mean value method in single environment deletion, regardless of the combination of heritability and genetic correlation. In the case of \({{\text{h}}}_{{\text{h}}}^{2}{{\text{R}}}_{{\text{h}}}\) , the prediction accuracy of GbyE is higher than 0.5, which is the highest value among all simulated combinations. When GbyE estimates the phenotypic values of Environment 1 and Environment 2 separately, its predictive accuracy seems too accurate. On the other hand, when the phenotypic values of both environments are missing on the same genotype, the predictive accuracy of GbyE does not show a significant decrease, and even maintains accuracy comparable to that of a single environment missing. However, when GbyE estimates Environment 1 and Environment 2 separately, the prediction accuracy significantly decreases compared to when a single environment is missing, and the prediction accuracy of Environment 1 and Environment 2 in \({{\text{h}}}_{{\text{l}}}^{2}{{\text{R}}}_{{\text{m}}}\) is extremely low (Fig. 6 B). In addition, the prediction accuracy of GbyE is lower than Mean values only in \({{\text{h}}}_{{\text{l}}}^{2}{{\text{R}}}_{{\text{h}}}\) , whether it is missing in a single or dual environment.
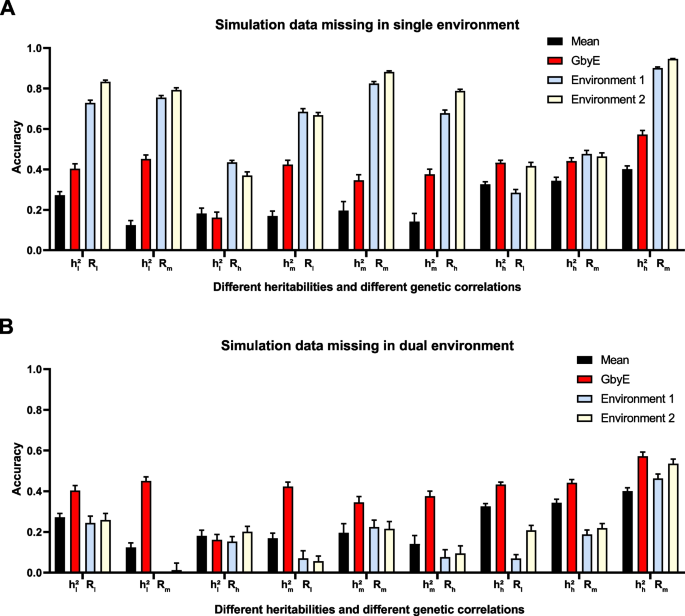
Prediction accuracy of simulated data in single and dual environment absence. The prediction effect of GbyE was divided into two parts, environment 1 and environment 2, to compare the prediction accuracy of GbyE when predicting these two parts separately. This includes simulations with missing phenotypes and genotypes in environment 1 only ( A ) and simulations with missing in both environments ( B ). The horizontal coordinates of the graph indicate the different combinations of heritabilities and genetic correlations of the simulations
The phenotype of organisms is usually controlled by multiple factors, mainly genetic [ 23 ] and environmental factors [ 24 ], and their interactive factors. The phenotype of quantitative traits is often influenced by these three factors [ 25 , 26 ]. However, based on the computing limitation and lack of special tool, the interactive effect always was ignored in most GWAS and GS research, and it is difficult to distinguish additive and interactive effects. The rate between all additive genetic variance and phenotype variance was named as narrow sense heritability. The accuracy square of prediction of additive GS model is considered that can not surpass narrow sense heritability. In this study, the additive effects in GbyE are essentially equivalent to the detectability of traditional models, the key advantage of GbyE is the interactive section. More significant markers with interactive effects were detected. Detecting two genetic effects (additive and interactive sections) in GWAS and GS is a boost to computational complexity, while obtaining genotypes for genetic interactions by Kronecker product is an efficient means. This allows the estimation of additive and interactive genetic effects separately during the analysis, and ultimately the estimated genetic effects for each GbyE genotype (including additive and interactive genetic effect markers) are placed in a t-distribution for p -value calculation, and the significance of each genotype is considered by multiple testing. The GbyE also expanded the estimated heritability as generalized heritability which could be explained as the rate between total genetics variance and phenotype variance.
The genetic correlation among traits in multiple environments is the major immanent cause of GbyE. When the genetic correlation level is high, then additive genetic effects will play primary impact in the total genetic effect, and interactive genetic effects with different traits or environments are often at lower levels [ 27 ]. Therefore, the statistical power of the GbyE algorithm did not improve significantly compared with the traditional method (Mean value) when simulating high levels of genetic correlation. On the contrary, in the case of low levels of genetic correlation, the genetic variance of additive effects is relatively low and the genetic variance of interactive effects is major. At this time, GbyE utilizes multiple environments or traits to highlight the statistical power. Since the GbyE algorithm obtains additive, environmental, and interactive information by encoding numerical genotypes, it only increases the volume of SNP data and can be applied to any traditional GWAS association statistical model. However, this may slightly increase the correlation operation time of the GWAS model, but compared to other multi environment or trait models [ 28 , 29 ], GbyE only needs to perform a complete traditional GWAS once to obtain the results.
In GS, rrBLUP algorithm is a linear mixed model-based prediction method that assumes all markers provide genetic effects and their values following a normal distribution [ 30 ]. In contrast, the BGLR model is a linear mixed model, which assumes that gene effects are randomly drawn from a multivariate normal distribution and genotype effects are randomly drawn from a multivariate Gaussian process, which takes into account potential pleiotropy and polygenic effects and allows inferring the effects of single gene while estimating genomic values [ 31 ]. The algorithm typically uses Markov Chain Monte Carlo methods for estimation of the ratio between genetic variances and residual variances [ 32 , 33 ]. The model has been able to take into account more biological features and complexity, and therefore the overall improvement of the GbyE algorithm under BGLR is smaller than Mean method. In addition, the length of the Markov chain set on the BGLR package is often above 20,000 to obtain stable parameters and to undergo longer iterations to make the chain stable [ 34 ]. GbyE is effective in improving the statistical power of the model under most Bayesian statistical models. In the case of the phenotypes we simulated, more iterations cannot be provided for the BayesB and BayesCpi models because of the limitation of computation time, which causes low prediction accuracy. It is worth noting that the prediction accuracy of BayesCpi may also be influenced by the number of QTLs [ 35 ], and the prediction accuracy of BayesB is often related to the distribution of different allele frequencies (from rare to common variants) at random loci [ 36 ].
The overall statistical power of GbyE was significantly higher in missing single environment than in missing double environment, because in the case of missing single environment, GbyE can take full advantage of the information from the phenotype in the second environment. And the correlation between two environments can also affect the detectability of the GbyE algorithm in different ways. On the one hand, a high correlation between two environments can improve the predictive accuracy of the GbyE algorithm by using the information from one environment to predict the breeding values in the other environment, even if there is only few relationship with that environment [ 37 , 38 ]. On the other hand, when two environments are extremely uncorrelated, GbyE algorithm trained in one environment may not export well to another environment, which may lead to a decrease in prediction accuracy [ 39 ]. In the testing, we found that when the GbyE algorithm uses a GS model trained in one environment and tested in another environment, the high correlation between environments may result to the model capturing similarities between environments unrelated to G × E information [ 40 ]. However, when estimating the breeding values for each environment separately, GbyE still made effective predictions using the genotypes in that environment and maintained high prediction accuracy. As expected, the additive effect calculates the average genetic effect between environments, and its predictive effect does not differ much from the mean method. The interactive effect, however, has one less column than the number of environments, and it calculates the relative values between environments, a component that has a direct impact on the predictive effect. The correlation between the two environments may have both positive and negative effects on the detectability of the GbyE, so it is important to carefully consider the relationship between the two environments in subsequent in development and testing.
A key advantage of the GbyE algorithm is that it can be applied to almost all current genome-wide association and prediction. However, the focus of GbyE is still on estimating additive and interactive effects separately, so that it is easy to determine which portion of the is playing a role in the computational estimation.. The GbyE algorithm may have implications for the design of future GS studies. For example, the model could be used to identify the best environments or traits to include in GS studies in order to maximize prediction accuracy. It is particularly important to test the model on large datasets with different genetic backgrounds and environmental conditions to ensure that it can accurately predict genome-wide effects in a variety of contexts.
GbyE can simulate the effects of gene-environment interactions by building genotype files for multiple environments or multiple traits, normalizing the effects of multiple environments and multiple traits on marker effects. It also enables higher statistical power and prediction accuracy for GWAS and GS. The additive and interactive effects of genes under genetic roles could be revealed clearly, which makes it possible to utilize environmental information to improve the statistical power and prediction accuracy of traditional models, thus helping us to better understand the interactions between genes and the environment.
Availability of data and materials
The GbyE source code, demo script, and demo data are freely available on the GitHub website ( https://github.com/liu-xinrui/GbyE ).
Abbreviations
- Genome-widely association study
Genome selection
Genetic by environmental interaction
Genome association and prediction integrated tool
Mixed linear model
Bayesian generalized linear regression
Ridge regression best linear unbiased prediction
False discovery rate
Principal component analysis
Genomic estimated breeding value
Maazi H, Hartiala JA, Suzuki Y, Crow AL, Shafiei Jahani P, Lam J, Patel N, Rigas D, Han Y, Huang P. A GWAS approach identifies Dapp1 as a determinant of air pollution-induced airway hyperreactivity. PLoS Genet. 2019;15(12):e1008528.
Article PubMed PubMed Central Google Scholar
Simonds NI, Ghazarian AA, Pimentel CB, Schully SD, Ellison GL, Gillanders EM, Mechanic LE. Review of the gene-environment interaction literature in cancer: what do we know? Genet Epidemiol. 2016;40(5):356–65.
Wang X, Chen H, Kapoor PM, Su Y-R, Bolla MK, Dennis J, Dunning AM, Lush M, Wang Q, Michailidou K. A Genome-Wide Gene-Based Gene-Environment Interaction Study of Breast Cancer in More than 90,000 Women. Cancer research communications. 2022;2(4):211–9.
Article CAS PubMed PubMed Central Google Scholar
Chen R-X, Dai M-D, Zhang Q-Z, Lu M-P, Wang M-L, Yin M, Zhu X-J, Wu Z-F, Zhang Z-D, Cheng L. TLR Signaling Pathway Gene Polymorphisms, Gene-Gene and Gene-Environment Interactions in Allergic Rhinitis. Journal of Inflammation Research. 2022;15:3613–30.
Zhao M-Z, Song X-S, Ma J-S. Gene× environment interaction in major depressive disorder. World Journal of Clinical Cases. 2021;9(31):9368.
Falconer DS. The problem of environment and selection. Am Nat. 1952;86(830):293–8.
Article Google Scholar
Kim J, Zhang Y, Pan W. Powerful and adaptive testing for multi-trait and multi-SNP associations with GWAS and sequencing data. Genetics. 2016;203(2):715–31.
Visscher PM, Wray NR, Zhang Q, Sklar P, McCarthy MI, Brown MA, Yang J. 10 years of GWAS discovery: biology, function, and translation. The American Journal of Human Genetics. 2017;101(1):5–22.
Article CAS PubMed Google Scholar
van Os J, Rutten BP. Gene-environment-wide interaction studies in psychiatry. Am J Psychiatry. 2009;166(9):964–6.
Article PubMed Google Scholar
Winham SJ, Biernacka JM. Gene–environment interactions in genome-wide association studies: current approaches and new directions. Journal of Child Psychology Psychiatry. 2013;54(10):1120–34.
Windhausen VS, Atlin GN, Hickey JM, Crossa J, Jannink J-L, Sorrells ME, Raman B, Cairns JE, Tarekegne A, Semagn K. Effectiveness of genomic prediction of maize hybrid performance in different breeding populations and environments. G3: Genes|Genomes|Genetics. 2012;2(11):1427–36.
Xu S, Zhu D, Zhang Q. Predicting hybrid performance in rice using genomic best linear unbiased prediction. Proc Natl Acad Sci. 2014;111(34):12456–61.
Zhao Y, Mette M, Gowda M, Longin C, Reif J. Bridging the gap between marker-assisted and genomic selection of heading time and plant height in hybrid wheat. Heredity. 2014;112(6):638–45.
Crossa J, Perez P, Hickey J, Burgueno J, Ornella L, Cerón-Rojas J, Zhang X, Dreisigacker S, Babu R, Li Y. Genomic prediction in CIMMYT maize and wheat breeding programs. Heredity. 2014;112(1):48–60.
Crossa J, Pérez-Rodríguez P, Cuevas J, Montesinos-López O, Jarquín D, De Los CG, Burgueño J, González-Camacho JM, Pérez-Elizalde S, Beyene Y. Genomic selection in plant breeding: methods, models, and perspectives. Trends Plant Sci. 2017;22(11):961–75.
Roorkiwal M, Jarquin D, Singh MK, Gaur PM, Bharadwaj C, Rathore A, Howard R, Srinivasan S, Jain A, Garg V. Genomic-enabled prediction models using multi-environment trials to estimate the effect of genotype× environment interaction on prediction accuracy in chickpea. Sci Rep. 2018;8(1):11701.
Burgueño J, de los Campos G, Weigel K, Crossa J. Genomic prediction of breeding values when modeling genotype× environment interaction using pedigree and dense molecular markers. Crop Science. 2012;52(2):707–19.
Jarquín D, Crossa J, Lacaze X, Du Cheyron P, Daucourt J, Lorgeou J, Piraux F, Guerreiro L, Pérez P, Calus M. A reaction norm model for genomic selection using high-dimensional genomic and environmental data. Theoretical applied genetics. 2014;127:595–607.
Wang JB, Zhang ZW. GAPIT Version 3: boosting power and accuracy for genomic association and prediction. Genomics Proteomics Bioinformatics. 2021;19(4):629–40.
Pérez P, de Los CG. Genome-wide regression and prediction with the BGLR statistical package. Genetics. 2014;198(2):483–95.
Endelman JB. Ridge Regression and other kernels for genomic selection with R package rrBLUP. Plant Genome J. 2011;4:250–5.
Turley P, Walters RK, Maghzian O, Okbay A, Lee JJ, Fontana MA, Nguyen-Viet TA, Wedow R, Zacher M. Furlotte NAJNg. Multi-trait analysis of genome-wide association summary statistics using MTAG. 2018;50(2):229–37.
CAS Google Scholar
Falconer DS. Introduction to quantitative genetics. Pearson Education India; 1996.
Google Scholar
Lynch M, Walsh B. Genetics and analysis of quantitative traits, vol. 1: Sinauer Sunderland, MA. 1998.
Mackay TF. The genetic architecture of quantitative traits. Annu Rev Genet. 2001;35(1):303–39.
Visscher PM, Hill WG, Wray NR. Heritability in the genomics era—concepts and misconceptions. Nat Rev Genet. 2008;9(4):255–66.
Van der Sluis S, Posthuma D, Dolan CV. TATES: efficient multivariate genotype-phenotype analysis for genome-wide association studies. PLoS Genet. 2013;9(1):e1003235.
O’Reilly PF, Hoggart CJ, Pomyen Y, Calboli FC, Elliott P, Jarvelin M-R, Coin LJ. MultiPhen: joint model of multiple phenotypes can increase discovery in GWAS. PLoS ONE. 2012;7(5):e34861.
Chung J, Jun GR, Dupuis J, Farrer LA. Comparison of methods for multivariate gene-based association tests for complex diseases using common variants. Eur J Hum Genet. 2019;27(5):811–23.
Pérez-Rodríguez P, Gianola D, González-Camacho JM, Crossa J, Manès Y, Dreisigacker S. Comparison between linear and non-parametric regression models for genome-enabled prediction in wheat. G3: Genes|Genomes|Genetics. 2012;2(12):1595–16605.
VanRaden PM. Efficient methods to compute genomic predictions. J Dairy Sci. 2008;91(11):4414–23.
Meuwissen TH, Hayes BJ, Goddard M. Prediction of total genetic value using genome-wide dense marker maps. Genetics. 2001;157(4):1819–29.
de Los CG, Hickey JM, Pong-Wong R, Daetwyler HD, Calus MP. Whole-genome regression and prediction methods applied to plant and animal breeding. Genetics. 2013;193(2):327–45.
Andrieu C, De Freitas N, Doucet A, Jordan MI. An introduction to MCMC for machine learning. Mach Learn. 2003;50:5–43.
Daetwyler HD, Pong-Wong R, Villanueva B, Woolliams JA. The impact of genetic architecture on genome-wide evaluation methods. Genetics. 2010;185(3):1021–31.
Clark SA, Hickey JM, Van der Werf JH. Different models of genetic variation and their effect on genomic evaluation. Genet Sel Evol. 2011;43(1):1–9.
Yang J, Benyamin B, McEvoy BP, Gordon S, Henders AK, Nyholt DR, Madden PA, Heath AC, Martin NG, Montgomery GW. Common SNPs explain a large proportion of the heritability for human height. Nat Genet. 2010;42(7):565–9.
González-Recio O, Forni S. Genome-wide prediction of discrete traits using Bayesian regressions and machine learning. Genet Sel Evol. 2011;43:1–12.
Korte A, Farlow A. The advantages and limitations of trait analysis with GWAS: a review. Plant Methods. 2013;9(1):1–9.
Gauderman WJ. Sample size requirements for matched case-control studies of gene–environment interaction. Stat Med. 2002;21(1):35–50.
Download references
Acknowledgements
Thank you to all colleagues in the laboratory for their continuous help.
This project was partially funded by the National Key Research and Development Project of China, China (2022YFD1601601), the Heilongjiang Province Key Research and Development Project, China (2022ZX02B09), the Qinghai Science and Technology Program, China (2022-NK-110), Sichuan Science and Technology Program, China (Award #s 2021YJ0269 and 2021YJ0266), the Program of Chinese National Beef Cattle and Yak Industrial Technology System, China (Award #s CARS-37), and Fundamental Research Funds for the Central Universities, China (Southwest Minzu University, Award #s ZYN2023097).
Author information
Authors and affiliations.
Key Laboratory of Qinghai-Tibetan Plateau Animal Genetic Resource Reservation and Utilization, Sichuan Province and Ministry of Education, Southwest Minzu University, Chengdu, 6110041, China
Xinrui Liu, Mingxiu Wang, Jie Qin, Yaxin Liu, Shikai Wang, Shiyu Wu, Ming Zhang, Jincheng Zhong & Jiabo Wang
Nanchong Academy of Agricultural Sciences, Nanchong, 637000, China
You can also search for this author in PubMed Google Scholar
Contributions
JW and XL conceived and designed the project. XL managed the entire trial, conducted software code development, software testing, and visualization. MW, JQ, YL, SW, MZ and SW helped with data collection and analysis. JQ, and YL assisted with laboratory analyses. JW, and XL had primary responsibility for the content in the final manuscript. JZ supervised the research. JW designed software and project methodology. All authors approved the final manuscript. All authors have reviewed the manuscript.
Corresponding author
Correspondence to Jiabo Wang .
Ethics declarations
Ethics approval and consent to participate.
Not applicable.
Consent for publication
Competing interests.
The authors have declared no competing interests.
Additional information
Publisher’s note.
Springer Nature remains neutral with regard to jurisdictional claims in published maps and institutional affiliations.
Supplementary Information
Supplementary material 1., rights and permissions.
Open Access This article is licensed under a Creative Commons Attribution 4.0 International License, which permits use, sharing, adaptation, distribution and reproduction in any medium or format, as long as you give appropriate credit to the original author(s) and the source, provide a link to the Creative Commons licence, and indicate if changes were made. The images or other third party material in this article are included in the article's Creative Commons licence, unless indicated otherwise in a credit line to the material. If material is not included in the article's Creative Commons licence and your intended use is not permitted by statutory regulation or exceeds the permitted use, you will need to obtain permission directly from the copyright holder. To view a copy of this licence, visit http://creativecommons.org/licenses/by/4.0/ . The Creative Commons Public Domain Dedication waiver ( http://creativecommons.org/publicdomain/zero/1.0/ ) applies to the data made available in this article, unless otherwise stated in a credit line to the data.
Reprints and permissions
About this article
Cite this article.
Liu, X., Wang, M., Qin, J. et al. GbyE: an integrated tool for genome widely association study and genome selection based on genetic by environmental interaction. BMC Genomics 25 , 386 (2024). https://doi.org/10.1186/s12864-024-10310-5
Download citation
Received : 27 December 2023
Accepted : 15 April 2024
Published : 19 April 2024
DOI : https://doi.org/10.1186/s12864-024-10310-5
Share this article
Anyone you share the following link with will be able to read this content:
Sorry, a shareable link is not currently available for this article.
Provided by the Springer Nature SharedIt content-sharing initiative
- Genomic selection
BMC Genomics
ISSN: 1471-2164
- Submission enquiries: [email protected]
- General enquiries: [email protected]
Thank you for visiting nature.com. You are using a browser version with limited support for CSS. To obtain the best experience, we recommend you use a more up to date browser (or turn off compatibility mode in Internet Explorer). In the meantime, to ensure continued support, we are displaying the site without styles and JavaScript.
- View all journals
- Explore content
- About the journal
- Publish with us
- Sign up for alerts
- Population Study Article
- Published: 22 April 2024
Association of obesity and menarche SNPs and interaction with environmental factors on precocious puberty
- Peng Xue 1 , 2 ,
- Jianfei Lin 3 ,
- Jingyi Tang 1 ,
- Yao Chen 4 ,
- Tingting Yu 1 , 5 ,
- Chang Chen 1 ,
- Huijun Kong 6 ,
- Cuilan Lin 7 &
- Shijian Liu ORCID: orcid.org/0000-0002-7050-463X 1 , 2
Pediatric Research ( 2024 ) Cite this article
Metrics details
Obesity is an important cause for the precocious or early puberty. However, the association between obesity-related loci and the risk of precocious puberty as well as the effect of gene-environment interaction are unclear, especially in the Chinese children population.
This was a case-control study using baseline data from two cohorts and hospital cases in China. 15 SNPs loci and several environmental factors were included in the analysis of 1201 participants. Chi-square test and logistic regression were used to analyze the association between SNPs and precocious puberty. Additionally, exploratory factor analysis was conducted on 13 environmental variables, and then to explore their interaction with genes on precocious puberty.
The effect allele C of rs571312, and G of rs12970134 MC4R were associated with precocious puberty in girls with obesity. Regarding the gene-environment interaction, we found that when girls were in the high socioeconomic status, the rs571312 (OR: 3.996; 95% CI: 1.694–9.423) and rs12970134 (OR: 3.529; 95% CI: 1.452–8.573) risk genotypes had a greater effect on precocious puberty.
Conclusions
The obesity risk gene polymorphisms MC4R rs571312 and rs12970134 were associated with precocious puberty in Chinese girls with obesity, and girls with risk genotypes and high socioeconomic status should be given extra attention.
This is the first study that identified the association between rs571312 and rs12970134 of MC4R gene and precocious puberty in Chinese children.
We found that when girls were in the high socioeconomic status, the risk genotypes of rs571312 and rs12970134 had a greater effect on precocious puberty.
The results of this study have great public health implications. It is recommended that girls who are in high socioeconomic status and have a high genetic risk for early sexual maturity should closely monitor their pubertal development and consider early intervention strategies.
This is a preview of subscription content, access via your institution
Access options
Subscribe to this journal
Receive 14 print issues and online access
251,40 € per year
only 17,96 € per issue
Buy this article
- Purchase on Springer Link
- Instant access to full article PDF
Prices may be subject to local taxes which are calculated during checkout
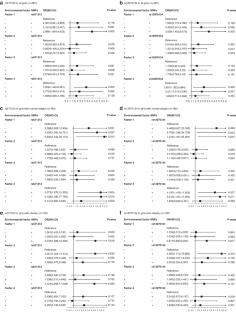
Similar content being viewed by others
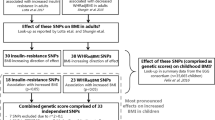
Genetic predisposition to higher body fat yet lower cardiometabolic risk in children and adolescents
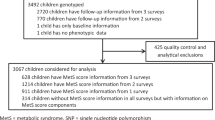
Common genetic variation in obesity, lipid transfer genes and risk of Metabolic Syndrome: Results from IDEFICS/I.Family study and meta-analysis
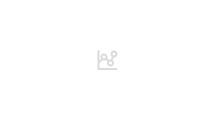
Predicting anthropometric and metabolic traits with a genetic risk score for obesity in a sample of Pakistanis
Data availability.
The raw data reported in this paper has been deposited in the Genome Variation Map in National Genomics Data Center, China National Center for Bioinformation / Beijing Institute of Genomics, Chinese Academy of Sciences, under accession number GVM000377 that can be publicly accessible at http://bigd.big.ac.cn/gvm/getProjectDetail?project= GVM000377 .
Abreu, A. P. & Kaiser, U. B. Pubertal development and regulation. Lancet Diabetes Endocrinol. 4 , 254–264 (2016).
Article PubMed PubMed Central Google Scholar
Brix, N. et al. Timing of puberty in boys and girls: a population-based study. Paediatr. Perinat. Epidemiol. 33 , 70–78 (2019).
Article PubMed Google Scholar
Lee, M. H., Kim, S. H., Oh, M., Lee, K. W. & Park, M. J. Age at menarche in Korean adolescents: trends and influencing factors. Reprod. Health 13 , 121 (2016).
Meng, X., Li, S., Duan, W., Sun, Y. & Jia, C. Secular trend of age at menarche in Chinese adolescents born from 1973 to 2004. Pediatrics 140 , e20170085 (2017).
Liu, Y. et al. Prevalence of precocious puberty among Chinese children: a school population-based study. Endocrine 72 , 573–581 (2021).
Article CAS PubMed Google Scholar
Zhang, Y. et al. The prevalence of precocious puberty among children in Qufu City, Shandong Province, China, a population-based study. Front. Endocrinol. 13 , 910119 (2022).
Article Google Scholar
Berkey, C. S., Frazier, A. L., Gardner, J. D. & Colditz, G. A. Adolescence and breast carcinoma risk. Cancer 85 , 2400–2409 (1999).
Day, F. R., Elks, C. E., Murray, A., Ong, K. K. & Perry, J. R. B. Puberty timing associated with diabetes, cardiovascular disease and also diverse health outcomes in men and women: the UK Biobank study. Sci. Rep. 5 , 11208 (2015).
Graber, J. A. Pubertal timing and the development of psychopathology in adolescence and beyond. Horm. Behav. 64 , 262–269 (2013).
Elks, C. E. et al. Thirty new loci for age at menarche identified by a meta-analysis of genome-wide association studies. Nat. Genet. 42 , 1077–1085 (2010).
Article CAS PubMed PubMed Central Google Scholar
Day, F. R. et al. Shared genetic aetiology of puberty timing between sexes and with health-related outcomes. Nat. Commun. 6 , 8842 (2015).
Ong, K. K. et al. Genetic variation in LIN28B is associated with the timing of puberty. Nat. Genet. 41 , 729–733 (2009).
Karapanou, O. & Papadimitriou, A. Determinants of menarche. Reprod. Biol. Endocrin. 8 , 115 (2010).
Fujimura, Y., Sekine, M. & Yamada, M. The relationship between quality of life and pubertal timing in adolescence: the Toyama birth cohort study, Japan. J. Adolesc. Health 65 , 790–798 (2019).
Sun, Y., Fang, J., Wan, Y., Su, P. & Tao, F. Role of polygenic risk in susceptibility to accelerated pubertal onset following chronic stress exposure. Eur. J. Endocrinol. 181 , 129–137 (2019).
Chen, C. et al. Investigating the relationship between precocious puberty and obesity: a cross-sectional study in Shanghai, China. BMJ Open 7 , e014004 (2017).
Chen, Y.-C. et al. Assessing causality between childhood adiposity and early puberty: a bidirectional Mendelian randomization and longitudinal study. Metabolism 100 , 153961 (2019).
Gavela-Pérez, T., Garcés, C., Navarro-Sánchez, P., López Villanueva, L. & Soriano-Guillén, L. Earlier menarcheal age in Spanish girls is related with an increase in body mass index between pre-pubertal school age and adolescence. Pediatr. Obes. 10 , 410–415 (2015).
Busch, A. S., Højgaard, B., Hagen, C. P. & Teilmann, G. Obesity is associated with earlier pubertal onset in boys. J. Clin. Endocrinol. Metab. 105 , dgz222 (2020).
Lee, J. M. et al. Timing of puberty in overweight versus obese boys. Pediatrics 137 , e20150164 (2016).
Li, D. et al. Obesity-related genetic polymorphisms are associated with the risk of early puberty in Han Chinese girls. Clin. Endocrinol. 96 , 319–327 (2022).
Article CAS Google Scholar
Jiang, Y. et al. Interaction effects of FTO rs9939609 polymorphism and lifestyle factors on obesity indices in early adolescence. Obes. Res. Clin. Pract. 13 , 352–357 (2019).
Wei, B. L. et al. The MC4R SNPs, their haplotypes and gene-environment interactions on the risk of obesity. Mol. Med. 26 , 77 (2020).
Hu, Y. et al. Exposure to antibiotics and precocious puberty in children: a school-based cross-sectional study in China. Environ. Res. 212 , 113365 (2022).
Yu, T. et al. Effects of childhood obesity and related genetic factors on precocious puberty: protocol for a multi-center prospective cohort study. BMC Pediatr. 22 , 310 (2022).
Cole, T. J. & Lobstein, T. Extended international (IOTF) body mass index cut-offs for thinness, overweight and obesity. Pediatr. Obes. 7 , 284–294 (2012).
Marshall, W. A. & Tanner, J. M. Variations in pattern of pubertal changes in girls. Arch. Dis. Child. 44 , 291–303 (1969).
Marshall, W. A. & Tanner, J. M. Variations in the pattern of pubertal changes in boys. Arch. Dis. Child. 45 , 13–23 (1970).
Cheuiche, A. V., da Silveira, L. G., de Paula, L. C. P., Lucena, I. R. S. & Silveiro, S. P. Diagnosis and management of precocious sexual maturation: an updated review. Eur. J. Pediatr. 180 , 3073–3087 (2021).
Chen, C. et al. Association between dietary patterns and precocious puberty in children: a population-based study. Int. J. Endocrinol. 2018 , 4528704 (2018).
Akiyama, M. et al. Genome-wide association study identifies 112 new loci for body mass index in the Japanese population. Nat. Genet. 49 , 1458–1467 (2017).
Locke, A. E. et al. Genetic studies of body mass index yield new insights for obesity biology. Nature 518 , 197–206 (2015).
Perry, J. R. et al. Parent-of-origin-specific allelic associations among 106 genomic loci for age at menarche. Nature 514 , 92–97 (2014).
Purcell, S. et al. Plink: PLINK: a tool set for whole-genome association and population-based linkage analyses. Am. J. Hum. Genet. 81 , 559–575 (2007).
Gong, W. et al. Effects of gene-environment interaction on obesity among Chinese adults born in the early 1960s. Genes 12 , 270 (2021).
Farooqi, I. S. et al. Clinical spectrum of obesity and mutations in the melanocortin 4 receptor gene. N. Engl. J. Med. 348 , 1085–1095 (2003).
Lotta, L. A. et al. Human gain-of-function MC4R variants show signaling bias and protect against obesity. Cell 177 , 597–607.e9 (2019).
Speliotes, E. K. et al. Association analyses of 249,796 individuals reveal 18 new loci associated with body mass index. Nat. Genet. 42 , 937–948 (2010).
Wen, W. et al. Meta-analysis identifies common variants associated with body mass index in East Asians. Nat. Genet. 44 , 307–311 (2012).
Cousminer, D. L. et al. Genome-wide association study of sexual maturation in males and females highlights a role for body mass and menarche loci in male puberty. Hum. Mol. Genet. 23 , 4452–4464 (2014).
Karim, A., Qaisar, R. & Hussain, M. A. Growth and socio-economic status, influence on the age at menarche in school going girls. J. Adolesc. 86 , 40–53 (2021).
Hiatt, R. A. et al. Childhood socioeconomic status and menarche: a prospective study. J. Adolesc. Health 69 , 33–40 (2021).
Oelkers, L. et al. Socioeconomic status is related to pubertal development in a German cohort. Horm. Res. Paediatr. 93 , 548–557 (2020).
Cheng, M. et al. The influence of socioeconomic status on menarcheal age among Chinese school-age girls in Tianjin, China. Eur. J. Pediatr. 180 , 825–832 (2021).
Cerutti, J., Lussier, A. A., Zhu, Y., Liu, J. & Dunn, E. C. Associations between indicators of socioeconomic position and DNA methylation: a scoping review. Clin. Epigenetics 13 , 221 (2021).
Gao, L. et al. MC4R single nucleotide polymorphisms were associated with metabolically healthy and unhealthy obesity in Chinese northern Han populations. Int. J. Endocrinol. 2019 , 4328909 (2019).
Rasmussen, L. et al. The impact of lifestyle, diet and physical activity on epigenetic changes in the offspring-a systematic review. Nutrients 13 , 2821 (2021).
Download references
Acknowledgements
We would like to express our sincere gratitude to all the staff, as well as all the study participants, their teachers and parents who participated in this study.
This study was supported by National Natural Science Foundation of China [82173534, 81872637].
Author information
Authors and affiliations.
School of Public Health, Shanghai Jiao Tong University, Shanghai, China
Peng Xue, Jingyi Tang, Tingting Yu, Chang Chen & Shijian Liu
Pediatric Translational Medicine Institute, Shanghai Children’s Medical Center, School of Medicine, Shanghai Jiao Tong University, Shanghai, China
Peng Xue & Shijian Liu
Department of Developmental and Behavioral Pediatrics, Shanghai Children’s Medical Center, School of Medicine, Shanghai Jiao Tong University, Shanghai, China
Jianfei Lin
Department of Endocrinology and Genetic Metabolism, Shanghai Children’s Medical Center, School of Medicine, Shanghai Jiao Tong University, Shanghai, China
Office of Hospital Infection Management, The Fourth Affiliated Hospital of Anhui Medical University, Hefei, Anhui, China
Tingting Yu
Department of Pediatrics, Qufu People’s Hospital, Qufu, Shandong, China
Huijun Kong
Boai Hospital of Zhongshan, Southern Medical University, Zhongshan, Guangdong, China
You can also search for this author in PubMed Google Scholar
Contributions
S.L., C.L. and H.K. performed study design and supervision. P.X., T.Y., J.L., J.T. and C.C. collected the data. P.X., J.L., J.T. and Y.C. carried out the data analyses and interpretation. P.X., J.L. and Y.C. drafted the manuscript. S.L. revised the manuscript. All authors have read and approved the final manuscript.
Corresponding author
Correspondence to Shijian Liu .
Ethics declarations
Competing interests.
The authors declare that there is no conflict of interest that could be perceived as prejudicing the impartiality of this study.
Ethics approval and consent to participate
Informed consent was obtained by the parents or guardians of the participating children.
Additional information
Publisher’s note Springer Nature remains neutral with regard to jurisdictional claims in published maps and institutional affiliations.
Supplementary information
Supplement tables, rights and permissions.
Springer Nature or its licensor (e.g. a society or other partner) holds exclusive rights to this article under a publishing agreement with the author(s) or other rightsholder(s); author self-archiving of the accepted manuscript version of this article is solely governed by the terms of such publishing agreement and applicable law.
Reprints and permissions
About this article
Cite this article.
Xue, P., Lin, J., Tang, J. et al. Association of obesity and menarche SNPs and interaction with environmental factors on precocious puberty. Pediatr Res (2024). https://doi.org/10.1038/s41390-024-03168-6
Download citation
Received : 19 June 2023
Revised : 21 February 2024
Accepted : 10 March 2024
Published : 22 April 2024
DOI : https://doi.org/10.1038/s41390-024-03168-6
Share this article
Anyone you share the following link with will be able to read this content:
Sorry, a shareable link is not currently available for this article.
Provided by the Springer Nature SharedIt content-sharing initiative
Quick links
- Explore articles by subject
- Guide to authors
- Editorial policies


An official website of the United States government
The .gov means it’s official. Federal government websites often end in .gov or .mil. Before sharing sensitive information, make sure you’re on a federal government site.
The site is secure. The https:// ensures that you are connecting to the official website and that any information you provide is encrypted and transmitted securely.
- Publications
- Account settings
Preview improvements coming to the PMC website in October 2024. Learn More or Try it out now .
- Advanced Search
- Journal List
- PMC10058966

The Genetic Basis of Childhood Obesity: A Systematic Review
Aikaterini vourdoumpa.
1 Division of Endocrinology, Metabolism and Diabetes, First Department of Pediatrics, National and Kapodistrian University of Athens Medical School, ‘Aghia Sophia’ Children’s Hospital, 11527 Athens, Greece
George Paltoglou
Evangelia charmandari.
2 Division of Endocrinology and Metabolism, Center for Clinical, Experimental Surgery and Translational Research, Biomedical Research Foundation of the Academy of Athens, 11527 Athens, Greece
Associated Data
Not applicable.
Overweight and obesity in childhood and adolescence represents one of the most challenging public health problems of our century owing to its epidemic proportions and the associated significant morbidity, mortality, and increase in public health costs. The pathogenesis of polygenic obesity is multifactorial and is due to the interaction among genetic, epigenetic, and environmental factors. More than 1100 independent genetic loci associated with obesity traits have been currently identified, and there is great interest in the decoding of their biological functions and the gene–environment interaction. The present study aimed to systematically review the scientific evidence and to explore the relation of single-nucleotide polymorphisms (SNPs) and copy number variants (CNVs) with changes in body mass index (BMI) and other measures of body composition in children and adolescents with obesity, as well as their response to lifestyle interventions. Twenty-seven studies were included in the qualitative synthesis, which consisted of 7928 overweight/obese children and adolescents at different stages of pubertal development who underwent multidisciplinary management. The effect of polymorphisms in 92 different genes was assessed and revealed SNPs in 24 genetic loci significantly associated with BMI and/or body composition change, which contribute to the complex metabolic imbalance of obesity, including the regulation of appetite and energy balance, the homeostasis of glucose, lipid, and adipose tissue, as well as their interactions. The decoding of the genetic and molecular/cellular pathophysiology of obesity and the gene–environment interactions, alongside with the individual genotype, will enable us to design targeted and personalized preventive and management interventions for obesity early in life.
1. Introduction
Overweight and obesity have reached epidemic proportions in contemporary societies, not only in adulthood but also in childhood and adolescence [ 1 ]. The prevalence of overweight and obesity in the pediatric population has more than quadrupled over the last 40 years, ranging from 4% in 1975 to >18% in 2016 [ 1 ]. Children with obesity demonstrate a 5-fold increased risk to remain obese in adulthood [ 2 ], especially in cases of severe obesity and/or obesity in one or both parents [ 3 ]. In addition, data from the World Health Organization (WHO) European Region estimate that over 60% of prepubertal children with overweight will be overweight as young adults [ 4 ]. According to the International Classification of Diseases 11 (ICD-11), obesity is a chronic complex disease, characterized by excess body fat accumulation, which adversely affects human health [ 5 ]. Increased adiposity in childhood results in numerous comorbidities, including obstructive sleep apnea, hypertension, left ventricular hypertrophy, acanthosis nigricans, insulin resistance and diabetes mellitus type 2, nonalcoholic fatty liver disease (NAFLD), polycystic ovary syndrome (PCOS), orthopedic problems, and impaired psychological health, which may present as early as childhood or adolescence [ 3 , 6 ]. Moreover, childhood obesity has been independently correlated with cardiovascular and metabolic diseases, cancer, and early mortality later in adult life [ 7 , 8 , 9 , 10 , 11 , 12 ].
The pathogenesis of polygenic obesity, the most common form of obesity, is multifactorial with genetic, epigenetic, and environmental factors all interacting among them and contributing to its development [ 13 , 14 , 15 , 16 ]. The term “obesogenic environment” refers to the role that environmental factors may play in determining both nutrition and physical activity and how they result in obesity [ 17 ]. In principle, weight gain occurs when energy intake (calories consumed) exceeds total daily energy expenditure for a prolonged period of time [ 15 ]. A current pathogenetic model states that the obesogenic environment, on one hand, triggers obesity-predisposing genes, and on the other hand, results in epigenetic changes, which both consequently contribute to the development of obesity [ 14 , 18 , 19 ]. Studies in twins show that the genetic heritability of obesity is as high as 47–90% [ 20 ], and equally high rates of inheritance have been associated with robust measures of adiposity, such as increased waist-to-hip ratio and others [ 21 , 22 ]. The inheritance pattern of polygenic obesity does not follow the principles of Mendelian inheritance but is the result of common genetic variations following the inheritance model of other complex diseases [ 23 ].
Genetic variations include single-nucleotide polymorphisms (SNPs), copy number variants (CNVs), and small insertions and deletions [ 15 , 23 ], each contributing to the development of obesity according to either the common-disease–common-variant hypothesis (common complex traits are largely due to common variants with small-to-modest effect) or the opposing, rare-variant hypothesis (common complex traits are the summation of low-frequency, high-penetrance variants) [ 23 , 24 ]. The upper percentiles of body mass index (BMI; the weight in kilograms divided by the square of the height in meters) distribution have been associated with rarer variants of high penetrance, explaining a proportion of the heritability [ 15 ]. In addition, the influence of the genotype in the pathogenesis of obesity follows a biphasic model, increasing with increasing age in childhood and adolescence, and attenuating with increasing age in adulthood [ 20 ]. More than 1100 independent loci associated with obesity have been identified to date through genome-wide association studies (GWAS) [ 23 ]. Although there is an overlap between variants related to obesity in childhood, adolescence, and adulthood, unique variants have also been associated with obesity in childhood [ 25 , 26 , 27 ]. Some of the genetic loci associated with the pathogenesis of obesity are MC4R , BDNF , SH2B1 , POMC , LEP , LEPR , NPY , SIM1 , NTRK2 , and PCSK1 [ 15 , 23 ], as well as CNVs in 11q11 ( OR4P4 , OR4S2 , OR4C6 ), 1p21.1 ( AΜΥ1 ), 10q11.22 ( NPY4R ), 10q26.3 ( CYP2E1 ), 16q12.2 ( FTO ), 16p12.3 ( GPRC5b ), and 4q25 loci [ 28 ]. The most recent Genetic Investigation of ANthropometric Traits (GIANT) meta-analysis of 700,000 people featured 941 nearly independent SNPs associated with BMI, with the lowest minor allele frequency (MAF) being 1.6% and the minimum per allele effect being 0.04 kg/m 2 per allele [ 23 ]. However, the variation of BMI currently explained by GWAS is limited to 6% [ 29 ]. Therefore, it is of great interest to decode the biological functions of the obesity-associated genetic loci identified through GWAS in order to enrich our understanding of the pathogenesis of obesity [ 23 ].
The obese state is characterized by a complex metabolic imbalance, associated with major changes in metabolic processes, including the central and peripheral nervous system regulation of energy balance, glucose, lipid, and adipose tissue homeostasis, as well as their interactions [ 30 ]. It is well-established that the central nervous system (CNS) plays a fundamental role in the control of food intake and energy homeostasis through genes expressed in the hypothalamus and pituitary gland, as well as in other brain regions involved in learning, cognition, addiction, reward, and emotional responses, including the hippocampus, the limbic system, the insula, and substantia nigra [ 23 ]. Among those, the leptin–melanocortin pathway and neuronal circuits involved in structural organization and plasticity, as well as their downstream signaling pathways, such as the dopaminergic and serotonergic pathways, mediate appetite and energy balance [ 23 , 31 , 32 ].
Furthermore, in the obese state, multiple changes in adipocyte-related gene expression modulate both biological pathways and the hormonal milieu of the organism by altering the expression of adipokines [ 33 ]. In addition, adipogenesis is characterized by adipose tissue hyperplasia (increased cell number), which appears mainly in the early stages of adipose tissue development, and hypertrophy (increased cell size), which occurs prior to hyperplasia to meet the need for additional fat storage capacity in the progression of obesity [ 34 ]. However, it has proven difficult to understand how energy balance and genetics specifically and differentially affect hyperplasia or hypertrophy of adipocytes that contribute to the metabolic state of the organism in obesity [ 35 ]. In metabolically healthy obesity, new, small adipocytes are formed (hyperplasia), while in impaired adipogenesis, the existing adipocytes become hypertrophic, which is a state associated with metabolic disorders of obesity favoring stress-induced hypoxia, low-grade inflammation, and insulin resistance [ 35 ]. Further complexity is added when considering the different histological forms of adipocytes (white, brown, and beige) [ 36 , 37 ].
A cross-talk between adipose tissue and the immune system takes place, with multiple genes associated with obesity encoding immune-cell-related proteins [ 38 ]. An excess in macronutrients intake leads to obesity and creates a chronic low-grade aseptic systemic inflammation [ 39 ]. Indeed, enlargement of adipocytes creates hypoxic conditions, enhancing inflammatory signaling, adipose tissue fibrosis, cellular death, and macrophage infiltration, with a shift from an anti-inflammatory M2-like to a pro-inflammatory M1-like phenotype, thereby increasing inflammatory mediators, such as IL-6 and TNF-α [ 39 ]. The proinflammatory state of obesity is also characterized by an anti-inflammatory adaptive response, characterized by changes in T-cell subpopulations in the adipose tissue, such as a CD8+ T cell activation, a decrease in Tregs and Th2 cells, and an increase in Th1 and Th17 cells, as well as disruption of the expression of many adipokines, including adiponectin, resistin, and visfatin [ 39 ]. Finally, microbial agents, such as viruses and viral-like agents, also play an important role in the pathogenesis of obesity, a phenomenon referred to as “infectobesity” [ 40 ].
To the best of our knowledge, very few studies have reviewed the gene–environment interactions in relation to BMI and changes in body composition after the implementation of lifestyle interventions to combat obesity, and they either focused on adult populations [ 41 , 42 ] or examined variations in single genes [ 43 , 44 , 45 ]. The aim of the present study was to systematically review the literature in order to explore the relation of SNPs and CNVs with changes in BMI and other measures of body composition in children and adolescents with overweight or obesity who undertook a lifestyle intervention program.
2. Materials and Methods
2.1. study design.
The present study was designed and conducted in accordance with the Preferred Reporting Items for Systematic Reviews and Meta-Analyses (PRISMA) protocol [ 46 ]. The review was registered in the International Prospective Register of Ongoing Systematic Reviews (PROSPERO Registration Number: CRD42022313595). To formulate the objectives of our review, the PICO (P—Populations/People/Patient/Problem, I—Intervention(s), C—Comparison, O—Outcome) model was implemented ( Table 1 ).
PICO Model.
PICO: P—Populations/People/Patient/Problem, I—Intervention(s), C—Comparison, O—Outcome, SNPs: single-nucleotide polymorphisms, CNVs: copy number variants, BMI: body mass index.
2.2. Eligibility Criteria
The present review included interventional cohorts and control trials, which examined the change in ΒΜΙ and/or body composition in relation to the genotype (SNVs and/or CNVs) in children and adolescents with overweight or obesity who followed a lifestyle intervention management program. Reviews, editorials, books and book chapters, notes, letters, conference papers, and surveys were not eligible for inclusion. Moreover, there were no restrictions on language, year of publication, or geographic location in order to ensure a broad and comprehensive literature search and minimize bias. Inclusion and exclusion criteria are presented in Table 2 .
Inclusion and Exclusion Criteria.
SNPs: single-nucleotide polymorphisms, CNVs: copy number variants, BMI: body mass index.
2.3. Literature Search
We conducted a literature search in three databases, Medline (PubMed), Scopus, and Cochrane Central Register of Controlled Trials (CENTRAL), prior to February 2022. Designing the search strategy, five main term categories were identified and combined using “AND”: (1) SNVs or CNVs, (2) BMI and body composition measures, (3) children and adolescents, (4) obesity, and (5) lifestyle interventions. Synonyms and representative key words of the terms within each of the five categories were defined by the Medical Subject Headings (MeSH) database and by a literature review [ 47 ], and they were combined using “OR”.
The final search in Medline was formulated as follows: ((((((((allel*) OR (variant*)) OR (polymorphi*)) OR (CNV)) OR (copy number variation*)) AND (((((((((((((((((((((BMI) OR (“mass”)) OR (fat)) OR (adipos*)) OR (circumference*)) OR (skinfold*)) OR (waist*)) OR (body* composition*)) OR (plethysmograph*)) OR (hydrodensitometr*)) OR (hydrometry)) OR (isotope* dilution*)) OR (bioimpedance)) OR (impedance)) OR (Dual-energy X-ray*)) OR (DXA)) OR (DEXA)) OR (MRI)) OR (Magnetic resonance)) OR (Compute* tomograph*)) OR (“CT”))) AND (((child*) OR (pediatric*)) OR (adolescen*))) AND ((obes*) OR (overweight))) AND (((((((intervention*) OR (diet*)) OR (nutrition*)) OR (exercis*)) OR (“physical activit*”)) OR (fitness*)) OR (weight loss)). This search strategy was appropriately adjusted to match the rest of the databases.
Furthermore, an additional backward-and-forward search for identification of eligible studies was performed in the reference articles of the included studies.
2.4. Study Selection
The identification, screening, eligibility, and inclusion process were performed by two independent reviewers (A.V. and G.P.), who remained blinded to each other’s work. Duplicates were removed using the EndNote X7 software. The eligibility assessment of the studies for meeting inclusion and exclusion criteria was made in two stages. The first eligibility check was based on the title and abstract, while the second eligibility check evaluated the full articles’ text. Discussion between the authors was used to resolve any data conflicts that occurred.
2.5. Data Extraction, Outcomes and Data Synthesis
Two authors (A.V. and G.P.), working independently, extracted the data on standardized Excel work templates, and any disagreements were solved through discussion, achieving a consensus. The extracted data items concerned the following fields: general study information (study type, outcomes examined, sample size, country, age, sex, pubertal status, obesity definition, study parameters examined, main findings) ( Table 3 ), obesity intervention characteristics (type of intervention, dietary, exercise and behavioral intervention description, compliance measurement, dropout rate) (available in Supplementary Table S1 ). and genotype-related information (genes, SNPs, risk alleles, alleles combinations and GRS examined, effect of genotype after intervention on weight, ΒΜΙ/ΒΜΙ-SDS, body composition, genetic inheritance model) (available in Supplementary Table S4 ).
General characteristics of the interventional studies included in the systematic review.
Abbreviations: NW: normal weight, OW: overweight, OB: obese, N/A: not available, BMI: body mass index, SDS: standard deviation score, SNP: single-nucleotide polymorphism, GRS: genetic risk score, WHO: World Health Organization, CDC: Centers for Disease Control and Prevention, IOTF: International Obesity Task Force.
The main outcomes examined in the present systematic review were genotype-related changes of BMI/BMI-SDS and/or body composition after lifestyle interventions implemented as part of the management of overweight and obesity. Secondary, we evaluated the specific components of the interventions, leading to significant changes, as well as the effect of the rest of the extracted data mentioned above. A qualitive synthesis of the data took place based on these outcomes.
2.6. Validity Assessment
Further to the above, the same two authors (A.V. and G.P.) separately assessed quality using the Newcastle–Ottawa Scale (NOS) [ 75 ] for cohort studies and randomized control trials. Any mismatches between the reviewers were discussed to reach a consensus.
The three main domains of NOS and the individual subgroups within them were evaluated, as shown in Supplementary Tables S2 and S3 , through a star-awarding system attributed to each subsection. Studies were categorized as being of good, fair, or poor quality. To assess the adequate duration of the follow-up period for the outcome to occur in the respective subsection of the Exposure category in NOS for cohort studies, a threshold of 6 months for the lifestyle interventions was applied [ 76 ].
Figure 1 summarizes the identification, screening, eligibility, and inclusion process of articles for review. Our initial database search identified 1986 articles, while one additional article was discovered through backward-and-forward reference searches. The articles were uploaded in EndNote X7, and after the removal of duplicates, 1420 studies remained for further evaluation. The screening of titles and abstracts resulted in the removal of 1369 studies due to their not meeting the inclusion criteria. Ultimately, 51 full-text articles were assessed for eligibility, leading to the exclusion of 24 articles and the inclusion of 27 studies in the qualitative synthesis of this systematic review.

Flow diagram describing the identification, screening, eligibility, and inclusion process.
A total of 27 studies [ 46 , 47 , 48 , 49 , 50 , 51 , 52 , 53 , 54 , 55 , 56 , 57 , 58 , 59 , 60 , 61 , 62 , 63 , 64 , 65 , 66 , 67 , 68 , 69 , 70 , 71 , 72 , 73 , 74 ], 26 non-randomized control studies and 1 randomized control trial were analyzed, consisting of 7928 overweight/obese children and adolescents within the age range of 4.5–20 years who were at different stages of pubertal development. The interventions took place in ten countries (Brazil, Poland, China, USA, Germany, Denmark, Spain, Italy, Portugal, and Czechia), representing a variety of populations. Table 3 summarizes the general data extracted from each study. The interventions were multidisciplinary, having dietary, exercise, behavioral, and/or medical evaluation components, and their duration ranged from 4 weeks to 10 years. The specific elements of each lifestyle intervention are analytically presented in Supplementary Table S1 . These studies examined the gene–environment interaction in obesity lifestyle interventions, considering the effect of SNPs in 92 different genetic loci on BMI or body composition improvement. Polymorphisms in 24 genes were significantly associated with BMI and/or body composition amelioration ( Table 4 , Supplementary Table S4 ), and the full set of genetic loci examined and the identified genetic associations are available in Supplementary Table S4 . However, no studies were found through our literature search, testing the relation of CNVs with BMI/body composition alteration during childhood obesity management programs.
Summary of the main associations identified between SNPs and BMI and/or body composition change after multidisciplinary lifestyle interventions in children and adolescents with excess adiposity.
Abbreviations: ΒΜΙ: body mass index, SNP: single-nucleotide polymorphism, GRS: genetic risk score.
The assessment of validity showed that the included studies in the present systematic review are of high quality, since they were rated as good in the NOS scale ( Supplementary Tables S2 and S3 ).
4. Discussion
In the present study, we systematically reviewed the literature to decode the gene–environment interactions with BMI and body composition alteration in obesity lifestyle interventions in children and adolescents, identifying significant associations with SNPs in 24 genetic loci. The main significant findings of the present study with respect to the effect of the genotype in the alteration of BMI and body composition after the implementation of pediatric lifestyle interventions are summarized in Table 4 , while all of the examined genotypic associations are analytically presented in Supplementary Table S4 .
4.1. Central Nervous System and Obesity
Among the assessed genes, the cell adhesion molecule 2 ( CADM2 ; OMIM * 609938) gene encodes a synaptic cell adhesion molecule that is a member of the immunoglobulin superfamily, which is widely expressed in multiple areas of the central nervous system [ 77 , 78 ]. During a 4- to 6-week standardized in-hospital lifestyle intervention program in 1198 children and adolescents with excess adiposity, Heitkamp et al., showed that the G allele in rs13078960 SNP of CADM2 was significantly associated with reduced BMI-SDS change [ 55 ]. The CADM2 gene mediates synaptic organization and function and coordinates signaling networks in several brain areas, thereby contributing to the control of adiposity and energy balance [ 78 , 79 , 80 ]. Genome-wide association studies have identified SNPs in CADM2 (rs13078960, rs1307880) as obesity susceptibility loci, similarly with its related family member CADM1 [ 81 , 82 ]. Interestingly, the obesity risk allele in rs13078960 SNP leads to increased expression of CADM2 in multiple areas of the human brain (caudate basal ganglia, putamen basal ganglia, cerebellum, and hypothalamus), providing further support of the association of CADM2 with increased adiposity and its role in energy homeostasis [ 79 , 83 ]. Studies in rodent models have additionally shown that CADM2 is expressed in brown adipose tissue and skeletal muscles of high-fat-fed mice [ 79 , 83 ], while deficiency of CADM2 in mice is associated with a leaner phenotype. Moreover, research data highlight a leptin-mediated control of CADM2 expression, indicating an involvement of this protein in the leptin signaling pathway [ 79 ]. Exogenous leptin administration in obese and insulin-resistant mice counterbalanced the overexpression of CADM2 in the obese state in the brain, while loss of CADM2 resulted in increased leptin sensitivity [ 79 ]. The introduction of a low-carbon ketogenic diet in obese mice produced the same effects on CADM1 overexpression, with CADM1 being involved in the functions of CADM2 [ 83 ]. A treatment effect of CADM2, independent of leptin, on the signaling cascade was also observed, given that CADM2 knockout mice show increased levels of phosphoryliated-STAT3 in the hypothalamus [ 79 ]. Overall, the risk allele in rs13078960 SNP for CADM2 predisposes to obesity [ 82 ] and incommodes the weight loss process in the pediatric population with overweight and obesity [ 55 ]. Further research into the role of CADM2 during obesity lifestyle interventions is necessary, while the ketogenic diet approach seems to be a promising topic for research [ 83 ].
Furthermore, when assessing the dopamine receptor D2 ( DRD2 ; OMIM * 126450) gene, Roth et al. showed that homozygous carriers of Τ allele in rs18000497 SNP near the DRD2 gene exhibited decreased BMI-SDS reduction after a one-year outpatient obesity lifestyle intervention program [ 67 ]. The rs18000497 SNP or TaqIA, a restriction fragment length polymorphism, is located within exon 8 of the ankyrin repeat and kinase domain containing protein 1 gene ( ANKK1 ; OMIM * 608774), possibly altering the substrate-binding specificity of ANKK1 protein [ 84 ]. This SNP is also in linkage disequilibrium with the DRD2 gene, which is positioned more than 10 kb downstream and encodes the post synaptic dopamine DRD2 receptor [ 84 ]. In accordance to the findings by Roth et al., other research groups have identified an association of the minor T allele with a difficulty to improve BMI following interventions [ 85 , 86 ]. The rs18000497 plays a central role in the dopaminergic-associated mechanistic models [ 84 ]. To begin with, feeding behavior and energy expenditure are regulated by a complex interplay between overlapping homeostatic and reward neurocircuits, with the latter being mediated by multiple neurotransmitter systems, such as the dopaminergic, endocannabinoid, opioid, GABAergic, cholinergic, and serotonergic [ 87 ]. Dopamine (DA), a metabolite of tyrosine, acts as a hormone and a major neurotransmitter, mediating cognition, locomotor activity, emotional responses, endocrine functions, metabolic sensing, and the “gut-brain” axis interaction, thereby determining feeding behavior [ 32 ]. Dopamine deficiency in the dorsal striatum can result in starvation due to lack of motivational eating [ 88 , 89 ]. Overall, unhealthy dietary patterns and excess adiposity have been associated with disruption in dopaminergic signaling, impacting compulsive-like feeding, food preferences, nutrient sensing, glucose metabolism, and neurocognitive impairment, thus reflecting an association of alterations in the dopaminergic pathways with diet-induced obesity [ 84 ].
Other studies have revealed similar neuroadaptations in the DA pathway between food-seeking behavior and substance abuse, introducing a model of addiction to highly palatable food consumption, leading to obesity, in an underlying hypodopaminergic environment [ 32 ]. Interestingly, the presence of the minor T allele (TaqIA1) relates to diminished DRD2 receptor density in the brain, causing alterations in reinforcement and cognition learning [ 90 ]. Overall, an inverted U-shaped model of dopaminergic effect on cognition learning has been proposed, which varies based on the allele in the rs18000497 SNP [ 84 ]. In addition, a diet rich in fat was associated with blunted DA response in the striatum and reduced DRD2 levels [ 91 ], indicating a nutrient-specific control of the dopaminergic system [ 84 ]. TaqIA1 carriers, who have reduced DRD2 function, displayed significant weight gain following a highly palatable diet [ 92 , 93 ]. A relation of the downregulation of DR2D, such as in TaqIA1 carriers, with enhanced food intake is also evident given the role of DRD2 antagonists in the establishment of food-seeking behavior and the inhibition of the anorexic effects of leptin, further supporting the model of food addiction [ 32 ]. Moreover, a reduction in DRD2 levels is thought to reduce physical activity and the related energy expenditure, thus increasing adiposity, which further downregulates DR2D function, creating a futile cycle [ 94 ]. Regarding the underlying molecular mechanisms, chronic exposure to saturated lipids is recognized as a crucial mediator of DRD2, possibly through inflammatory pathways connecting astrocytes with DRD2 [ 84 ]. Chronic consumption of dietary fat generates inflammatory-like changes in the brain, partially mediated by NF-κΒ, resulting in transcriptional adaptations in DRD2 levels and signaling [ 84 ]. Carriers of the TaqIA1 may be more susceptible to these inflammatory responses [ 84 ]. Finally, TaqIA seems to interact with the FTO gene, one of the most strongly associated genetic loci with obesity, influencing dopaminergic pathways connected to obesity-related impaired learning functions, adiposity, and insulin resistance [ 84 ].
Another gene linking dopamine and serotoninergic models with the control of energy homeostasis is the LIM homeobox transcription factor 1-beta ( LMX1B ; OMIM * 602575), which encodes the LMX1B protein, a member of the LIM-homeodomain family of proteins [ 95 ]. In a multidisciplinary lifestyle intervention program conducted by Heitkamp et al. to address childhood obesity in more than one thousand children and adolescents, the homozygous state for the A allele in rs10733682 of LMX1B gene was shown to promote greater weight loss [ 55 ]. Conversely, Hollensted et al. associated the T allele in rs3829849 SNP of LMX1B with decreased BMI-SDS change after a family-centered multidisciplinary behavioral lifestyle intervention in 754 children and adolescents with excess adiposity [ 56 ]. These two studies examined different SNPs of the LMX1B gene and used different methodology designs [ 55 , 56 ]. Another study conducted in adults associated females homozygous for the AA genotype in LMX1B rs10733682 polymorphism with increased adiposity and revealed an interaction of this genotype with macronutrient intake and dietary patterns, resulting in increased triglycerides with fat and carbohydrate consumption and increased BMI and waist circumference with protein intake [ 96 ]. In addition, the obesity risk allele in rs10733682 SNP has been associated with reduced satiety responsiveness in the pediatric population [ 97 ]. The association of the rs10733682 and rs3829849 polymorphisms in LMX1B loci with obesity has been established by GWAS both in adult and pediatric populations [ 27 , 82 ] and may be possibly explained by the role of LMX1B in serotonergic and dopaminergic pathways [ 98 , 99 ]. LMX1B acts as a continuously expressed transcription factor at successive stages, regulating serotonin (5-HT) genesis and reuptake, as well as 5-HT neuronal axon primary formation, targeted routing, and terminal arborization [ 98 ]. Serotonergic signaling in the central and peripheral nervous system is a major mediator of food intake and energy homeostasis, taking part in the homeostatic and hedonic/reward pathways of feeding behavior [ 31 ]. Serotonergic pathways in the central nervous system are anorexigenic and promote energy expenditure by increasing thermogenesis in the brown adipose tissue [ 31 ], while 5-HT pathways in the peripheral nervous system promote energy storage by enhancing lipid anabolism [ 100 ]. Data from rodent models and humans reveal impaired serotonergic circuits in the obese state, with reduced 5-HT levels and attenuated signaling [ 31 ]. These changes occur early in overfeeding conditions with high-fat and -sugar diets [ 101 , 102 ]. Moreover, meal timing plays a crucial role in 5-HT regulation: during a 4-week hypocaloric diet, the striatal dopamine transporter and thalamic serotonin signaling increased when most caloric daily intake was consumed in breakfast and decreased when it was consumed in the evening [ 103 ].
The pleiotropic functions of leptin in energy homeostasis and metabolism are mediated through the leptin receptor, which is encoded by the leptin receptor gene ( LEPR ; OMIM * 601007) [ 104 ]. Two of the included studies examined SNPs in LEPR [ 49 , 52 ]. Firstly, polymorphisms of LEPR were studied by Gajewska et al. in prepubertal children undertaking a weight management program, in which carrying at least one minor G allele in Q223R together with the wild-type K665N led to the greatest weight loss, fat mass reduction, and increase in soluble leptin receptor levels [ 52 ]. Secondly, in a research study conducted by Corgosinho et al., adolescents carrying the C allele of rs2767485 in LEPR presented increased leptin concentrations and increased expression of orexigenic peptides NPY, AgRP, and MCH, a metabolic state constituting an obstacle to weight loss, with a concomitant increase of AgRP concentration after a one-year obesity lifestyle intervention [ 49 ]. On the other hand, TT homozygotes showed greater BMI change [ 49 ]. Adult female carriers of the T allele in LEPR Ser (T) 343 Ser (C) polymorphism showed a stronger predisposition to obesity, while carriers of the C allele demonstrated greater weight loss in response to a low-caloric diet intervention compared to non-carriers [ 105 ]. In addition, the Lys656Asn polymorphism in LEPR has been associated with variations in the response to lifestyle modifications in adults [ 106 ]. LEPR is a type I cytokine transmembrane receptor, signaling through a tyrosine kinase, the Janus kinase 2 (JAK2), and thus activating multiple molecular pathways [ 104 ]. Moreover, LEP–LEPR binding can lead to the control of energy homeostasis independently of tyrosine phosphorylation, which is possible through pathways involving phosphoinositide 3-kinase (PI3K) [ 104 ]. Leptin acts in the central nervous system through the long isoform of LEPR, being expressed in neuronal cells in multiple regions of the brain, and is responsible for feeding and energy balance [ 107 ]. The soluble leptin receptor, a short form of leptin receptor, binds the circulating leptin, preventing clearance [ 108 ]. Leptin is secreted by adipose tissue in relation to its stores of triglycerides and has pleiotropic actions in energy balance and adiposity regulation, in growth, thermogenesis, and glycemic control, the production of adrenal corticosteroids, and the function of the reproductive and immune system [ 104 , 108 ]. Leptin is a key component of the leptin–melanocortin pathway, exercising anorexic and lipolytic functions [ 104 ]. Interestingly, excess adiposity is characterized by hyperleptinemia [ 108 ], while weight loss is associated with decreased leptin concentrations [ 52 ].
Associated with the above-mentioned leptin pathway, the melanocortin 4 receptor gene ( MC4R ; OMIM * 155541) encodes a G-protein-coupled receptor with unique structure [ 109 ]. Homozygosity for the C allele in s17782313 of MC4R has been associated with greater BMI and body composition outcomes in an inpatient weight-reduction program by Zlatohlavek et al. in pediatric subjects with excess adiposity [ 73 ]. The C allele in s17782313 has also been associated with better BMI and body composition change as a part of genetic risk score (GRS) during a three-month multidisciplinary lifestyle intervention by Moleres et al. [ 61 ]. Finally, Vogel et al. showed that female carriers of at least one risk allele for the MC4R rs17782313 and rs12970134 polymorphisms demonstrated more efficient BMI-SDS reduction than males during a one-year multicomponent lifestyle intervention to manage obesity during childhood [ 71 ]. Exploring the nutritional interaction with rs17782313 SNP, regular consumption of meals (5 meals including breakfast versus ≤ 4 meals) in adolescence seem to attenuate the obesity risk from the risk alleles in MC4R rs17782313 in both genders and from FTO rs1421085 only in males, possibly because of more favorable postprandial thermogenic and glucose metabolism responses [ 110 , 111 ]. Moreover, an increased consumption of salt in MC4R rs17782313, SEC16B rs543874, and KCTD15 rs11084753 risk allele carriers increases the risk of obesity by both increasing the intake of sugar-sweetened soft drink consumption and by extension the intake of calories and causing water retention and disruption of fat metabolism [ 112 ]. The MC4R is embedded in the leptin–melanocortin signaling pathway and is expressed in key brain areas [ 113 ], contributing significantly to energy homeostasis, appetite regulation, thermogenesis, and peripheral glucose metabolism [ 114 ]. The MC4R has important anorexigenic effects. The entire spectrum of MC4R genetic variations has been related to BMI heterogeneity [ 114 ]. More than 200 mutations have been identified so far in obese patients, ranging from rare loss of function mutations leading to extreme monogenic obesity to common variations connected with the pathogenesis of polygenic obesity [ 114 ]. The MC4R receptor is activated in the satiety state by the adrenocorticotropic hormone (ACTH) and the post-translational derivatives of POMC and the a-MSH, β-MSH, and γ-MSH neuropeptides; it is inhibited by orexigenic AgRP-mediated signaling, expressed in AgRP/NPY neurons in the arcuate nucleus [ 114 ]. The rs17782313 SNP is located 188 kb downstream of MC4R and has been strongly associated with the development of obesity in populations of different ethnicity and age, including children and adolescents [ 115 , 116 , 117 , 118 ]. Ligand-receptor changes could possibly contribute to the positive effect of the minor allele of rs17782313 SNP in BMI reduction in childhood obesity lifestyle interventions [ 71 ]. On the other hand, gain-of-function mutations in MC4R protect from obesity and its cardiometabolic comorbidities, possibly through a signaling bias toward β-arrestin recruitment [ 119 ], causing an increased MC4R expression in the cell surfaces [ 119 ]. Overall, the obesity risk allele in the MC4R rs17782313 may beneficially affect the decrease of adiposity in short-term pediatric therapeutic interventions, either individually or as part of a GRS, possibly through a sex-specific interaction.
The melanocortin 3 receptor gene ( MC3R ; OMIM * 155540) encodes the MC3R, a G-protein-coupled receptor, which is a part of the melanocortin system and a key component of energy homeostasis, adiposity, and food-intake regulation, participating in appetite control and eating-induced motivational responses in situations of nutrient restriction and negative energy equilibrium, as well as glucose metabolism [ 120 , 121 ]. Santoro et al. examined the role of C17A (Thr6Lys) and G241A (Val81Ile) polymorphisms of MC3R gene in a multicomponent weight reduction intervention, associating carriers 6Lys and 81Ile alleles with reduced BMI change [ 68 ], in accordance with studies in adults [ 122 ]. The MC3R signaling leads to inhibition of POMC in the central nervous system, while it is also expressed in peripheral organs, such as heart, kidneys, liver, gut, and adipose tissue [ 68 ]. In mice, MC3R deficiency has been associated with the obese phenotype with Cushing-like characteristics, such as increased cortisol levels and visceral fat, and to an unbalanced metabolic rate and profile with reduced lipolysis [ 121 ]. In MC3R dysfunction, obesity presents in milder levels compared with MC4R deletion, while a coexistence of these two deficiencies has a synergic effect on increased adiposity [ 121 ]. In addition, increased anthropometric measures of adiposity, such as BMI and fat mass, have been observed in the homozygous state for T6K + V81I in pediatric population studies [ 123 , 124 , 125 ]. The T6K +V81I haplotype is by far the most well-studied; its phenotypic effects are most prominent in the homozygous state, with milder clinical effects seen in heterozygous carriers [ 121 ]. The haplotype’s effects may be more pronounced in infancy and childhood [ 121 ]. In addition, both human and rodent models suggest that T6K + V81I carriers have lower rates of lipid oxidation, combined with increased oxidation of glucose [ 121 ], possibly explaining the difficulty to lose weight during interventions [ 68 ].
4.2. Adipose Tissue and Obesity
Among the genes associated with adipose tissue formation, metabolism, and obesity during childhood, Heitkamp et al. showed that children/adolescents homozygous for the G allele in the rs12940622 polymorphism of the regulatory-associated protein of MTOR ( RPTOR ; OMIM * 607130) gene are resistant to weight loss compared to homozygous non-carriers in a 4–6-week obesity intervention program [ 55 ]. The RPTOR gene encodes an evolutionarily conserved protein, which forms a stoichiometric complex with the mechanistic target of rapamycin (mTOR) [ 126 ]. The mTOR signaling pathway modulates fundamental cellular processes, and its dysregulation contributes to the pathogenesis of tumors, metabolic diseases, neurodegeneration, and aging [ 126 ]. The relation of the rs12940622 SNP of the RPTOR gene with excess adiposity has been identified in large GWAS [ 82 ] and is possibly explained by the crucial role of RPTOR in the functions of the mTORC1 pathway [ 55 ]. The mTORC1 pathway integrates nutritional and hormonal signals, orchestrating anabolic and catabolic processes and enabling the transition between them [ 126 ]. Indeed, growth factors, insulin, amino acids adequacy, such as in feeding conditions, oxygen, and ATP availability promote mTORC1 anabolic signaling [ 126 ]. Protein synthesis, lipogenesis, and nucleotide biosynthesis are enhanced, and energy homeostasis is regulated [ 126 ]. Simultaneously, mTORC1 suppresses catabolism and autophagy [ 126 ].
In contrast, in a nutrient-deprived environment, under energetic stress, hypoxia, DNA damage, or rapamycin therapy, mTORC1 is inhibited, thus promoting catabolic pathways towards autophagy and lysosome biogenesis [ 126 ]. In this way, cellular amino acids can be replenished, reactivating mTORC1 and establishing an important feedback mechanism for survival between the lysosomes and mTORC1 [ 126 ]. Interestingly, hepatic RPTOR deficiency in mice attenuates lipogenesis in the liver [ 126 ]. In rodent models, RPTOR deletion in adipocytes diminishes white adipose tissue (WAT) and promotes lipolysis and gradual lipodystophy with a parallel lipid accumulation in the liver, developing hepatic steatosis, hepatomegaly, and insulin resistance [ 127 ]. Interestingly, these RTOR knockout mice have a lean phenotype and present hyperphagia with a resistance to diet-induced obesity [ 127 , 128 ]. Nevertheless, RPTOR depletion, inhibiting the mTORC1 pathway, has been associated with an expansion of lifespan in multiple organisms [ 126 ]. Overall, the association of RPTOR/mTORC1 with adiposity regulation becomes evident [ 126 ]. In overfeeding conditions with a surplus of nutrient and hormonal signals, the mTOR pathway is overactivated, contributing to the pathogenesis and maintenance of many diseases, such as obesity, diabetes mellitus type 2, and metabolic syndrome [ 126 ]. Research towards developing mTORC1 specific inhibitors could be promising in the treatment of obesity [ 126 ]. Some researchers have suggested a U-shaped inverted model in the association of mTORC1 function with adiposity, where optimal mTORC1 signaling is necessary for pro-adipogenic and lipogenic effects [ 129 ].
The FTO alpha-ketoglutarate-dependent dioxygenase gene ( FTO ; OMIM * 610966) encodes the namesake nuclear protein, which belongs to the AlkB-related non-heme iron and 2-oxoglutarate-dependent oxygenase protein superfamily and is ubiquitously expressed in the body, with the highest concentrations found in the brain and the pancreatic islets [ 130 , 131 ]. Barbian et al. [ 48 ], do Nascimento et al. [ 51 ], Moraes et al. [ 63 ], and Müller et al. [ 64 ] studied the rs9939609 SNP of FTO , Hollensted et al. [ 56 ] studied the rs1421085 SNP of FTO , Scherag et al. [ 69 ] studied the rs1558902 SNP of FTO , and Schum et al. [ 70 ] studied the rs1421085, rs17817449, and rs9939609 SNPs of FTO and showed that these variants did not modify BMI or body composition following lifestyle interventions for management of childhood obesity. Conversely, Moleres et al. [ 61 ] found a greater BMI reduction after a 3-month multidisciplinary lifestyle intervention in A-allele carriers of rs9939609 SNP both individually and as a part of a GRS. Moreover, Reinehr et al. [ 66 ] described a synergistic effect in the homozygous state of the C allele in rs7566605 SNP of INSIG2 together with the A allele in rs9939609 SNP of FTO , leading to reduced BMI change after a one-year outpatient lifestyle intervention. In addition, Zlatohlavek et al. [ 73 ] associated the homozygosity for the G allele in rs17817449 SNP of FTO genetic loci with greater BMI change both individually and in synergy with the CC homozygosity in rs17782313 of MC4R during a 4-week inpatient weight reduction program. Finally, Hagman et al. [ 54 ] associated the A-allele homozygosity in rs8050136 SNP of FTO with greater BMI reduction after a 12-month to 10-year clinic-based behavioral modification therapy aimed at children and parents. The FTO gene mediates oxidative demethylation of various RNA forms and takes part in fat mass regulation, adipocyte differentiation, and energy homeostasis [ 131 ]. The genetic loci of FTO emits one of the strongest signals reported in GWAS related to obesity and energy balance, implicating FTO in the pathogenesis of excess adiposity, while mutations of the gene cause growth retardation, developmental delay, and facial dysmorphism [ 130 ]. Claussnitzer et al. in 2015 elucidated the underlying pathophysiological mechanisms associating FTO with obesity development by identifying its effect on the IRX3 gene promoter, located 0.5 MB downstream of FTO [ 132 ]. The T-to-C allele substitution in the rs1421085 SNP of FTO impairs the binding of the ARID5B transcriptional suppressor, lifting its inhibitory effect on a major preadipocyte enhancer [ 132 ]. In this way, the expression of IRX3 and IRX5 is doubled in the early stages of adipocyte differentiation, and a cell-cycle-independent turnover of beige adipocytes to white takes place, leading to a reduction of mitochondrial thermogenesis, a process regulated by UCP1, PGC1α, and PRDM16, and to an increase in lipid storage [ 132 ]. Therefore, a conversion of the biological processes from energy expenditure to energy storage is reflected [ 132 ].
Another gene associated with adipogenesis and possibly the low-grade inflammation of obesity is the ETS protooncogene 2 transcriptional factor gene ( ETS2 ; OMIM * 164740), which encodes the ETS2 proto-oncogene and transcription factor, which specifically recognizes and binds to the core motif DNA sequence GGAA/T of target genes [ 133 ]. In their study, Heitkamp et al. showed a significantly greater reduction in body weight and BMI in children/adolescents homozygous for the effect C allele in rs2836754 SNP of the ETS2 gene compared to non-carriers during a 4–6-week in-hospital lifestyle intervention [ 55 ]. The ETS2 plays a fundamental role in a broad spectrum of cellular processes, regulating development, proliferation, differentiation, migration, transformation, and apoptosis [ 133 ]. The rs2836754 SNP association with susceptibility to the obese phenotype has been recognized in GWAS [ 82 ]. ETS2 is a transcriptional regulator of early adipocyte differentiation both in vivo and in vitro [ 134 ]. Furthermore, ETS2 has a diverse role in inflammatory processes [ 135 ], potential affecting the inflammatory state of obesity. ETS2 has been identified as a transcriptional regulator of many cytokines, such as IL-5 and the p-40 subunit of IL-12 [ 136 ]. Animal models show that in acute inflammation, ETS2 has anti-inflammatory effects, acting as a negative regulator of lipopolysaccharides and VSV-induced inflammation by directly binding to the IL-6 promoter, inhibiting transcription and inhibiting MAPK/NF-κB signaling [ 135 ]. Moreover, ETS2 regulates miR-155 inflammatory production both positively and negatively by an IL-10 mediated inhibition [ 137 ]. On chronic inflammation, ETS2 is essential to persistent TNF-a production [ 138 ].
Another gene associated with adipogenesis is the lysine acetyltransferase 8 ( ΚAΤ8 ; OMIM * 609912) gene. The A allele in rs9925964 SNP of the ΚAΤ8 gene was associated with greater BMI-SDS reduction in a 4–6-week in-hospital lifestyle intervention for the management of obesity in childhood conducted by Heitkamp et al. [ 55 ]. The KAT8 gene encodes the catalytic subunit of two multiprotein complexes (male-specific lethal-MLS and non-specific lethal-NSL) [ 139 ]. The produced protein is a member of the MYST histone acetylase family, also known as MYST1, which was first identified as males-absent-on-the-first (MOF) in Drosophila melanogaster [ 139 ]. Overall, KAT8 plays a crucial role in multiple physiological functions, such as gene transcription control, chromatin architecture maintenance, cell cycle regulation, autophagy, early embryogenesis, mitochondrial transcription, and DNA damage repairment, while its dysfunction has been associated with tumorigenesis and defects in these fundamental processes [ 139 , 140 , 141 , 142 ]. Findings from GWAS have highlighted rs9925964 SNP in KAT8 gene as a risk locus for obesity [ 82 ], an association possibly explained by data associating KAT8 with adipose tissue development and function [ 143 , 144 , 145 ], central control of metabolism, and diet-induced obesity [ 146 ]. Furthermore, studies in KAT8 haploinsufficient mice have shown that KAT8 is an important epigenetic regulator of carbohydrate, amino acid, and adipose tissue homeostasis [ 146 ]. In addition, other pathways except glucose metabolism are potentially affected, such as brain and heart vitamin B6 metabolism [ 146 ]. Another study by Brenachot and colleagues introduced KAT8 as a mediator of energy balance in the brain through the regulation of polysialylation, a necessary component of synaptic plasticity in feeding pathways [ 147 ]. Using mice with KAT8 total depletion in the mediobasal hypothalamus, St8sia4 gene transcription was inhibited and polysialic acid levels were reduced, leading to increased feeding behaviors and an obese phenotype [ 147 ]. Small molecule inhibitors of acetylation in a cell-specific manner could constitute a future research field in obesity treatment [ 146 ].
The transmembrane protein 18 gene ( TMEM1 ; OMIM * 613220) is an evolutionarily highly conserved gene, encoding the nuclear transmembrane protein 18 [ 148 ], which mediates the migration process of neural precursors to glioblastoma cells [ 149 ]. In a 3-month multidisciplinary lifestyle intervention by Moleres et al., the risk G allele in rs7561317 SNP of TMEM18 was associated with greater BMI-SDS and fat mass reduction both on its own and as a part of a high GRS score [ 61 ]. Conversely, the rs4854349 SNP studied by Hollensted et al. in a 6–24-month family-centered multidisciplinary behavioral lifestyle intervention [ 56 ], the rs4854344 SNP studied by Zlatohlavek et al. in a 4-week in-patient weight reduction program [ 74 ], and the rs11127485 SNP studied by Scherag et al. in a one-year out-patient lifestyle intervention program [ 69 ] did not have significant associations with BMI or body composition change. The TMEM18 is a preserved gene and has a well-established role among genes involved in obesity pathogenesis [ 150 ]. Various polymorphisms in the TMEM18 loci have been implicated in obesity through GWAS and observational studies, an association more pronounced in the pediatric population [ 81 , 82 , 150 , 151 , 152 , 153 , 154 , 155 , 156 ]. Transmembrane protein 18 is ubiquitously expressed in most cells in the body, including the adipose tissue, the hypothalamus, and the brainstem, which are major modulators of energy homeostasis [ 150 , 155 , 157 ]. Interestingly, Rask-Andersen et al. associated body weight with the expression of TMEM18 in mice prefrontal cortex, which regulates executive functions and behavior characteristics [ 156 ]. Trying further to understand the pathophysiological mechanisms of TMEM18-mediated obesity, Landgraf and colleagues in 2020 turned their research towards adipose tissue metabolism instead of the central nervous system [ 155 ]. They showed that the expression of TMEM18 varies in adipose tissue of children without excess adiposity, depending on the presence of the obesity risk allele in rs7561317 and rs17729501 polymorphisms [ 155 ]. Moreover, TMEM18 expression in adipocytes is attenuated in children [ 155 ] and adults [ 158 ] with obesity, as well as with obesity-induced adipose tissue and metabolic dysregulation, such as in insulin resistance. However, an association with eating behavior traits was nοt confirmed [ 155 ], in accordance with data from a cohort in Greek pediatric population [ 156 ]. In addition, TMEM18 was identified as an enhancer of PPARG1 promoter-induced transcription, exercising a major role in PPARG1-dependent adipogenesis [ 155 ]. In the inflammatory state of obesity, TMEM18 downregulation reduces PPARG1 levels, contributing to the metabolic dysregulation, adipocyte hypertrophy, adiponectin reduction, impaired glucose, and lipid metabolism and obesity aggravation [ 155 ].
Another significant finding from the study by Moleres et al. (2012) was the evidence that children with a higher obesity risk GRS score, constituting the obesity risk G allele of rs1801282 SNP in the peroxisome proliferator activated receptor gamma gene ( PPARG / PPARγ ; OMIM * 601487) alongside SNPs in FTO , MC4R , TMEM18 , IL6 , and ADIPQ had greater BMI and fat mass at the beginning together with a greater BMI and fat mass reduction after a 3-month lifestyle intervention, whereas children with lower GRS presented a greater improvement in their metabolic profile after intervention [ 61 ]. The PPARγ gene encodes a member of the nuclear receptor superfamily PPARs, the PPARγ or PPARG, and is mainly expressed in adipose tissue (white and brown), as well as in other organs such as macrophages, endothelium, liver, colon, muscles, and kidneys [ 159 ]. It has multiple different isoforms, created through alternative transcription and splicing, including PPARγ1 and PPARγ2, with the latter being mainly expressed in the adipose tissue [ 160 , 161 ]. PPAR gamma presents pleiotropic functions, taking part in a variety of biological processes, including adipogenesis, glucose metabolism, lipid oxidation and storage, control of autophagy, and inflammatory responses, and has therefore been implicated in the pathological state of obesity, type 2 diabetes mellitus, atherosclerosis, cancer, and Familial Partial Lipodystrophy Type 3 [ 159 , 162 ]. Among the natural agonists of PPARγ are the polyunsaturated fatty acids, glucocorticoids, and insulin [ 35 ], with thiazolidinedione also having a high affinity for PPARγ, which exercise their therapeutic effects in type 2 diabetes mellitus [ 159 , 163 ]. Data show that caloric restriction antagonizes PPARγ, whereas dietary fat activates it [ 164 ]. Genetic variations in PPARγ have been associated with BMI distribution from birth to adulthood in Caucasians, possibly in a sex-specific manner, including the rs1801282 SNP, also known as Pro12Ala [ 161 , 164 , 165 ]. PPARγ is characterized as the master regulator of adipogenesis [ 35 ]. PPARγ deficiency in rodents leads to hepatic steatosis and lipodystrophy, since there is no other component to replace the role of PPARγ in adipogenesis [ 166 ]. A high PPARγ activity induces the process of hyperplasia during adipogenesis, while under a low PPARγ drive, the adipogenesis dynamic is impaired, with the existing adipocytes becoming hypertrophic [ 35 ]. This expansion in size impairs the function of PPARγ and limits the adipogenetic turnover of mesenchymal cells to adipocytes, while it favors mechanical-stress-induced hypoxia, the low-grade inflammation of obesity, and insulin resistance [ 35 ]. Acute inflammation may promote tissue remodeling through the enhancement of adipogenesis [ 35 ]. However, in chronic inflammation like that in obesity, PPARγ expression can be downregulated, and the protein is degraded [ 167 ]. The rs1801282 SNP, located in exon B, creates an amino acid change of proline to alanine (Pro12Ala) in the N-terminus of the PPARγ2 isoform [ 161 ]. This substitution has been associated with reduced binding affinity to the receptor of target genes [ 161 ], attenuating the transcriptional functions of PPARγ, and with impaired recruitment of tissue-specific cofactors, which dysregulate its role in adipogenesis and the different expression patterns in different cell types and increase insulin sensitivity [ 168 ]. Indeed, carriers of the Pro allele have an increased risk of developing diabetes mellitus type 2 [ 169 ]. Considering the functions of PPARγ in metabolism, this amino acid change may explain the findings in Moleres et al. study [ 61 ], but further research is needed on its effect on obesity lifestyle interventions. Interestingly, a gene–environment interaction has been described between rs1801282 SNP and nutrition, where BMI and insulin concentration are inversely associated with the polyunsaturated to saturated fatty acids ratio of the diet in carriers of the obesity G risk allele [ 170 ].
The sonic hedgehog (SHH) signaling and ciliogenesis regulator SDCCAG8 ( SDCCAG8 ; OMIM * 613524) gene encodes a protein associated with the centrosome, which may regulate centrosome during interphase and mitosis [ 171 ]. Scherag et al. studied variations in five ( FTO , MC4R , TMEM18 , SDCCAG8 , TNKS / MSRA ) genetic loci and identified an association of the homozygosity for three intronic SNPs (rs10926984, rs12145833, rs2783963) in SDCCAG8 with reduced BMI change in children/adolescents undergoing a one-year multidisciplinary obesity lifestyle intervention, although similar correlations were nοt identified in an adult sample undergoing an hypocaloric diet intervention [ 69 ]. The SDCCAG8 protein is essential for ciliogenesis through its modulatory role on RABEP2-dependent centrosomal localization, as well as for the optimum activation of multiple signaling pathways, such as the Hedgehog pathway, which require fully functional cilia [ 172 ]. It also contributes to the creation of cell polarity and the epithelial lumen [ 172 ]. Mutations of SDCCAG8 are implicated in the pathogenesis of retinal–renal ciliopathies [ 171 ]. Bardet–Biedl Syndrome, one of the major causes of syndromic obesity, and Senior–Loken Syndrome 7 are associated with mutations in SDCCAG8 [ 171 ]. Moreover, polymorphisms of SDCCAG8 gene, such as the ones mentioned above, have been implicated in the development of obesity through GWAS [ 173 ]. Indeed, both deleterious mutations and common polymorphisms in genes regulating ciliary related proteins have been associated with all obesity phenotypes, from monogenic and syndromic to multifactorial polygenic obesity [ 174 ].
Children/adolescents carrying the A allele in rs11170468 SNP of copine 8 ( CPNE8 ) gene were resistant to BMI-SDS reduction in a 4–6 week obesity lifestyle intervention program conducted by Heitkamp et al. [ 55 ]. The CPNE8 gene encodes the copine 8 calcium-dependent membrane-binding protein, which takes part in calcium-mediated molecular events and interactions in cell membranes and the cytoplasm [ 175 ]. Calcium is an important molecule in various cellular processes [ 175 ]. Obesity has been associated with dysregulation of cytoplasm and organelle Ca 2+ homeostasis and the related signaling transmission [ 176 ]. Excess adiposity and overfeeding leads to Ca 2+- mediated disruption of hepatic glucose metabolism, mitochondrial dysfunction, endoplasmic reticulum stress, and autophagy in liver and immune-cell dysfunction [ 176 ]. Moreover, in adipose tissue, Ca 2+ contributes to the regulation of adipogenesis, lipid synthesis, and thermogenesis in brown adipocytes [ 176 ].
4.3. Adipose Tissue Metabolism
Moleres et al. in 2014 studied genes implicated in lipid and energy metabolism and associated the minor allele in rs670 (−75 G/A) apolipoprotein A1 gene ( APOA1 ; OMIM * 107680) with increased BMI and other measures of body composition, as well as with greater weight and BMI reduction after a 10-week intervention, while a combined analyses with the rs1800777 SNP in CETP explained up to 24% of BMI-SDS amelioration [ 62 ]. The APOA1 gene encodes apolipoprotein A-I, the most abundant component of high-density lipoprotein cholesterol (HDL), and has antiatherogenic and anti-inflammatory actions [ 177 , 178 ]. Apolipoprotein A-I also promotes energy expenditure and modulates body fat content, lipolysis, and glucose metabolism, exercising an anti-obesity effect [ 177 , 178 ]. Indeed, APOA1 knockout mice significantly increase their body weight and body fat accumulation despite a restriction in caloric intake because of an attenuation of lipolytic activity [ 179 ]. Conversely, APOA1 transgenic mice or mice treated with the ApoA-I mimetic peptide D-4F, when fed a highly palatable diet, showed a reduction in adipose tissue, increased insulin sensitivity, and enhanced energy expenditure [ 177 , 178 ]. In addition, APOA1 promotes the β-adrenergic stimulated lipolysis and is speculated to participate in the differentiation of pre-adipocytes [ 177 ]. The common polymorphism rs670 is located 75 bp upstream from the transcriptional site of APOA1 gene and may contribute to the variable gene environment interactions affecting metabolism [ 180 ]. Indeed, the A allele has been associated with an improvement of anthropometric measures, lipid profile, and insulin sensitivity following a hypocaloric diet in patients with obesity [ 181 , 182 ]. Homozygotes for the G allele have a higher risk of metabolic syndrome, possibly explained by their increased adiposity and insulin resistance [ 183 ].
Another genetic variant associated with lipid metabolism assessed in the study by Moleres et al. is the minor allele in rs1800777 (R451Q) cholesteryl ester transfer protein gene ( CETP ; OMIM * 118470), which was correlated with increased BMI and other measures of body composition and greater weight and BMI reduction after a 10-week multidisciplinary lifestyle intervention, while a combined analyses with the rs670 SNP in APOA1 explained up to 24% of BMI-SDS reduction [ 62 ]. The CETP encodes a glycoprotein responsible for the exchange of lipids, such as cholesteryl ester and triglycerides, among lipoprotein particles, as well as the cholesterol efflux [ 184 ]. CETP regulates the transfer of esters from HDL to Apolipoprotein B (ApoB)–containing lipoproteins (VLDL, LDL) in exchange for triglycerides, thus affecting HDL particle size [ 184 ]. CETP deficiency leads to increased HDL concentrations and has an antiatherogenic effect [ 185 ]. Therefore, CETP inhibitors may be promising for the prevention of cardiovascular disease, since they increase HDL and decrease LDL and ApoB [ 185 ]. Polymorphisms in CETP affect the lipid profile and influence the risk of developing coronary heart disease [ 185 , 186 ]. Moreover, variations in this gene are associated with an alteration of the lipid profile in response to dietary fat intake, revealing a gene–nutrient interaction [ 187 ]. The SNP in the CETP (rs1800777) gene may affect lipid metabolism [ 188 ]. The presence of the minor allele has been associated with increased risk of developing central obesity, increased fat mass, waist circumference, and waist-to-hip ratio [ 189 ], as well as reduced HDL concentrations [ 189 , 190 , 191 , 192 ], enhanced CETP activity [ 191 , 193 ], and increased intimal wall thickness of the carotid arteries [ 191 , 194 ].
The lipoprotein lipase gene ( LPL ; OMIM * 609708) [ 195 ] encodes a lipoprotein lipase, a member of the lipase gene family, which catalyzes the hydrolysis of triglycerides in chylomicrons and very low-density lipoproteins (VLDLs), contributing to lipoprotein uptake in the tissues, the exchange of lipids between lipoproteins, and the lipoprotein-independent uptake of lipoprotein-derived lipids and lipophilic vitamins [ 196 ]. In the study by Gao et al., the rs283 SNP in LPL gene was associated in the homozygous GG allele carriers with greater body fat reduction and triglyceride concentrations and improvement in HOMA-IR following a 4-week aerobic training [ 53 ]. The authors attributed their findings to metabolic changes occurring during exercise, in which fatty acids are translocated from adipose tissue to muscles, where the rate of fat oxidation is increased [ 53 ]. A catecholamine-mediated increase of cAMP and an increase in intracellular calcium through muscle contraction were observed during exercise in skeletal muscles, upregulating LPL gene expression and increasing the hydrolyses of triglycerides to fatty acids [ 53 ]. Conversely, the exercise-mediated reduction of insulin downregulated LPL expression and activity in adipose tissue, attenuating the triglyceride absorption in adipose tissue [ 53 ]. The rs283 polymorphism is located in intron 6, and the authors speculated that the G allele can enhance LPL gene expression, increase LPL activity, and positively affect exercise-induced metabolic and adiposity changes [ 53 ].
In the studies performed by Reinehr et al. in 2008 [ 65 ] and 2009 [ 66 ], a statistically significant lower BMI and BMI-SDS reduction was noted in homozygous CC carriers in rs7566605 SNP of insulin-induced gene 2 ( INSIG2 ; OMIM * 608660) gene during obesity lifestyle management interventions, while the 2009 study highlighted a synergistic effect in reduced BMI change after intervention in combined homozygous carriers of the CC genotype in rs7566605 SNP of INSIG2 and the AA genotype in rs9939609 SNP of FTO . The INSIG2 gene encodes the INSIG2 oxysterol-binding protein, which binds to the SREBP cleavage-activating protein (SCAP), retaining the SREBP/SCAP to the endoplasmic reticulum and inhibiting its transportation to the Golgi, while it also promotes the ubiquitination and degradation of HMGCR, negatively regulating lipid biosynthesis [ 197 , 198 ]. The association of the rs7566605 SNP of INSIG2 [ 199 ] with predisposition to obesity is controversial [ 200 ], with data supporting this correlation in adults with severe obesity [ 201 ]. In the pediatric population, some studies have identified an association of the rs7566605 SNP with predisposition to obesity [ 153 ], while others have found such an association only when diet combined with physical exercise are taken into account [ 202 ]. Interestingly, in a pediatric cohort, homozygosity for the obesity risk C allele was associated with higher glucose concentrations, possibly introducing an indirect role of INSIG2 in promoting adiposity, through its effect on glucose metabolism [ 203 ]. Indeed, INSIG2 gene has been implicated in diabetes pathogenesis [ 204 , 205 ].
Deram and colleagues identified two different patterns that link pediatric obesity, insulin resistance, and lipid metabolism for the perilipin 1 gene ( PLIN1 ; OMIM * 170290) variations in children and adolescents [ 50 ]. Firstly, the T allele in rs1052700 was associated with improved BMI and body composition outcomes and lower HOMA-IR levels [ 50 ]. Secondly, the minor A allele in rs894160 was related to a high-risk metabolic profile in the pediatric population, observing an impaired glucose metabolism both at baseline and after intervention, and a higher prevalence of metabolic syndrome, without correlating this genotype with BMI and its alteration [ 50 ]. PLIN1 is located on chromosome 15q26, a chromosomal location connected with obesity, impaired glucose metabolism, and hypertriglyceridemia [ 206 , 207 ]. Genetic variations in PLIN1 gene have been associated with human disease [ 206 , 207 ]. The encoded perilipins are a family of phosphorylated proteins, highly conserved through species, encircling intracellular lipid storage droplets in adipocytes, steroid producing cells, liver, heart, and muscle cells [ 206 , 207 , 208 ]. They play a primary role in the regulation of lipid, glucose, and energy homeostasis through the formation and mobilization of adipocyte stores [ 206 , 207 , 208 ]. The PLIN1 (rs1052700) is an obesity risk factor [ 209 , 210 , 211 ], which is also associated with better weight loss outcomes [ 50 , 212 ]. Indeed, the minor alleles in rs2304795 and rs1052700 are associated with body fat, waist circumference, and obesity risk in Caucasian adult women [ 209 ]. A gender-specific interaction and an ethnicity-dependent intragenic linkage disequilibrium (LD) structure (in Asians, rs1052700 and rs894160 are in LD, while rs2289487 and rs894160 are in LD in Whites) in the PLIN1 locus may affect the different associations in different populations [ 213 ].
In addition, the T allele in rs2304795 predisposes to the development of obesity in adolescence [ 211 ]. Examining the effect of rs1052700 polymorphism in response to diet modification, Jang et al. showed that carriers of the minor allele in rs894160 or rs1052700 of Korean origin showed a more significant decrease in waist circumference, fat mass, and free fatty acids, indicating increased lipolysis [ 206 ], during an energy-restricted weight loss intervention [ 212 ], similar to findings in Deram et al. [ 50 ]. Moreover, the rs894160 and rs1052700 were associated with a gene–diet interaction between increased saturated fatty acids and carbohydrate dietary intake and insulin resistance in Asian adult women [ 214 ]. In contrast, homozygotes for the minor T allele in rs1052700 had lower body weight and fat mass both at baseline and after a 6-week dietary weight loss intervention program [ 215 ], as shown by Deram et al. [ 50 ]. Interestingly, the minor A allele of rs894160 has been associated with prevention of obesity [ 215 , 216 , 217 ] but with resistance to weight loss following intervention [ 216 , 218 ]. In Deram et al., children were introduced to a balanced but not restrictive dietary education program, consisting of 1800 kcal/day [ 50 ]. The authors concluded that despite the association of the minor A allele in rs894160 with lower BMI in the literature [ 215 , 216 , 217 ], this protective effect is removed in the obese state, resulting in a metabolic unbalance and increased circulating non-esterified fatty acids, predisposing to a worse metabolic profile, insulin resistance, and type 2 diabetes [ 50 ]. Other research findings support the association of perilipin variations with diabetes risk, modified by central obesity in females [ 219 ]. Moreover, in the adipocytes of women with obesity homozygous for the A allele in rs894160, increased basal and noradrenaline-induced lipolysis combined with significantly lower levels of perilipin content have been identified, constituting a risk for type 2 diabetes [ 220 ].
The adipocyte-, C1q-, and collagen-domain-containing gene ( ADIPOQ ; OMIM * 605441) encodes the major adipokine, adiponectin [ 221 ]. The rs266729, rs16861194, rs822395, rs2241766, and rs1501299 SNPs were not associated with BMI change when examined individually in a 3-month outpatient multidisciplinary intervention program by Gajewska et al., nor in a 3-month multidisciplinary lifestyle intervention by Moleres et al. [ 52 , 61 ]. However, Moleres et al. associated a higher GRS, including the obesity risk alleles in the rs822395, rs2241766, and rs1501299 polymorphisms of ADIPOQ , with greater BMI and fat mass reduction [ 61 ]. Decreased concentrations of adiponectin were observed in overweight and obesity, dysregulating glucose, and lipid metabolism, contributing to the pathogenesis of type 2 diabetes and cardiovascular disease [ 222 ]. Interestingly, the rs266729 polymorphism of the adiponectin gene promoter variant has been correlated with decreased concentrations of adiponectin, predisposing to obesity and coronary atherosclerosis [ 223 ]. Considering the crucial roles of adiponectin in energy homeostasis and the pathophysiology of obesity, even in childhood and early puberty [ 104 , 224 ], interventions of longer duration and in larger population samples of children and adolescents are necessary to further investigate its associations with BMI improvement.
4.4. Adipose Tissue Inflammation and Obesity
Recent studies have highlighted the importance of low-grade aseptic inflammation in the pathogenesis of obesity [ 39 ]. In the study by Heitkamp and colleagues, homozygous carriers of the G allele of the rs13201877 polymorphism of the interferon-gamma receptor 1 ( IFNGR1 ; OMIM * 107470) gene, when compared to homozygous non-carriers, showed significantly greater reduction in weight and BMI during a 4–6-week pediatric obesity intervention program [ 55 ]. The IFNGR1 gene, located in chromosome 6q23.3, encodes the ligand-binding chain (alpha) of the gamma interferon receptor [ 225 , 226 ]. The gamma interferon receptor constitutes a heterodimer of IFNGR1 and IFNGR2 that is expressed in almost every cell type and exerts important antimicrobial, antiviral, and antitumor functions through its role in immune cell activation and the enhancement of antigen presentation [ 225 , 226 ]. The association of the rs13201877 polymorphism of IFNGR1 gene with the risk of obesity development has been well-established in large GWAS [ 82 ]. Interferons are major cytokines, regulating anti-viral and autoimmune responses through cellular adaptations in energy homeostasis, protein and lipid metabolism, and cell cycle, structure, and metabolism [ 40 ]. In the acute phase of viral infections, interferons have an antiviral and immune stimulatory function, possibly by suppressing protein and lipid metabolism and reducing energy flow and lipogenesis [ 40 ]. On the other hand, chronic viral infection induces a prolonged but attenuated IFN activation, promoting immune dysfunction, obesity-related immune suppression, low-grade inflammation, and adipogenesis [ 40 ]. Furthermore, under chronic stimulation, IFNs can reprogram cellular lipid synthesis and transport, thus enhancing adipogenesis [ 40 ]. IFN-γ also contributes to the metabolism of ceramides (sphingolipid family), a biomarker of insulin resistance and adiposity [ 40 ]. The association of IFN-γ signaling with excess adiposity is further supported by the fact that treatment with IFN-γ results in decreased insulin sensitivity and inhibition of differentiation of pre-adipocytes to mature cells, thereby exerting an antiadipogenic effect, possibly through JAK-STAT1-mediated cascades [ 38 , 227 ].
Heitkamp et al., in their 4–6-week multidisciplinary lifestyle intervention, also demonstrated a lesser BMI-SDS reduction in carriers of the risk allele in rs13107325 SNP of the solute carrier family 39, member 8 ( SLC39A8 ; OMIM * 608732) gene [ 55 ]. The SLC39A8 gene is a highly evolutionarily conserved gene in vertebrates and a member of the solute-carrier gene superfamily, encoding the transmembrane transporter of cation ZIP8 [ 228 ]. ZIP8 is ubiquitously distributed throughout the body, mainly expressed in cell-surface membranes, but also in lysosomal, endoplasmic reticulum, and mitochondrial membranes [ 229 ]. ZIP8 plays a crucial role in cell processes, such as cell morphology, cytoskeleton formation, adhesion, migration, and proliferation, and exerts multiple pleiotropic functions both during embryogenesis and later in life. [ 229 ]. GWAS have associated SLC39A8 gene variations with pathologies in multiple systems, such as dysmorphogenesis and immune, cardiovascular, gastrointestinal, coagulation, musculoskeletal, central nervous system, eye, kidney, and lung disorders [ 229 ]. ZIP8 is regulated by lipopolysaccharides, cytokines (such as TNF-α, IL-1β and IL-5), glucose, estrogen, and the concentrations of Zn 2+ and Fe 2+ [ 230 ]. The rs13107325 polymorphism is a nonsynonymous SNP located in exon 8 of SLC39A8 gene and results in an amino acid change of hydrophobic alanine (major allele) to hydrophilic and polar threonine (minor allele) [ 230 , 231 ]. The carrier state of T allele has been associated with more than 20 pathological traits, including increased BMI, reduced HDL concentrations, coronary artery disease, hypotension, smoking-induced atherosclerotic plaques, higher risk of cardiovascular death, liver inflammation and fibrosis, inflammatory bowel disease, allergy, schizophrenia, low stature, and adolescent idiopathic scoliosis [ 229 ]. ZIP8 exerts a zinc-mediated protection from the toxic effects of TNF-α in lung epithelial cells early during inflammation [ 229 ]. Obesity is characterized by decreased plasma zinc concentrations [ 232 ], while weight loss results in an increase in plasma zinc concentrations [ 233 ]. The role of zinc in body weight regulation is further supported by the results of a recent meta-analysis in which zinc supplementation was associated with a decrease in body weight in individuals with overweight/obesity but who were otherwise healthy [ 234 ]. Overall, disruption of zinc homeostasis has been associated with oxidative stress, inflammatory processes, dyslipidemia, and diabetes mellitus type 2, mostly through mechanisms mediated by the rs13266634 polymorphism in the SLC30A8 gene encoding zinc transporter ZnT8 [ 232 ].
The interleukin 6 gene ( IL6 ; OMIM * 147620) encodes the major pro- and anti- inflammatory cytokine IL6, which binds to interleukin 6 receptor alpha (IL6R) and signals through the receptor protein gp130 [ 235 ], while an alternative “trans signaling” pathway also coexists [ 236 ]. The study by Moleres et al. in 2012 correlated a high GRS score, including the obesity risk allele G in the rs1800795 (−174 G > C) SNP of the IL6 gene, with greater BMI and fat mass at the beginning, as well as with greater BMI and fat mass reduction following the introduction of lifestyle interventions [ 61 ]. Interleukin 6 (IL6) is expressed in multiple tissues, including the hypothalamus, and serves a dual role as a proinflammatory cytokine and anti-inflammatory myokine, explaining its pleiotropic functions [ 38 ]. Beyond its crucial role in immunology, infection, and inflammation, IL6 is also a homeostatic regulator implicated in energy, glucose, protein, and lipid metabolism [ 237 ]. In humans, variations in the IL6 gene have been associated with BMI and other measures of adiposity, such as waist circumference [ 38 , 238 , 239 ]. The beneficial effect of IL6 on metabolism regulation is supported by animal models in which exogenous IL6 administration led to weight reduction and by treatment of patients with Castleman disease with anti-IL6 antibody receptor, which reversed disease-associated cachexia [ 237 , 240 ]. In patients with obesity, IL6 is overproduced by white adipose tissue [ 240 ]. Increased IL6 levels found in obesity possibly serve as an adaptive mechanism in order to limit inflammation and balance metabolic comorbidities [ 236 ]. In obesity, stress, hormones (insulin, catecholamines, glucocorticoids), hypoxia, and inflammation interact and affect IL6 production [ 240 ]. As mentioned above, obesity results in a low-grade chronic systemic inflammation associated with increases in two inflammatory mediators, IL-6 and TNF-α [ 39 ]. Regarding the IL6 (rs1800795) polymorphism, some studies suggest that the obesity risk allele is the G, while others suggest that it is the C [ 241 , 242 ]. Moreover, carriers of the C allele in rs1800795 show increased postprandial fat oxidation [ 243 ], and this allele protects from weight regain after weight loss alongside the G allele in rs1801282 of PPARγ2 [ 244 ]. During physical exercise, IL6 produced from skeletal muscles is upregulated up to 100-fold, independently of TNF-α and without a significant increase in other inflammatory molecules, leading to insulin sensitivity, lipolysis, and fat oxidation [ 38 , 245 ]. The increase in IL6 expression during exercise also suppresses hyperphagia and reduces obesity-induced hypothalamic inflammation, thereby promoting insulin and leptin sensitivity and re-establishing balances in the control of appetite and energy homeostasis [ 246 ].
In summary, in the present study, we systematically reviewed the literature, aiming to decode the interaction of the genotype with diet, physical activity, and behavior interventions in children and adolescents with overweight and obesity. We found statistically significant associations with variants in 24 genetic loci, exercising an important effect on BMI and/or body composition change through lifestyle interventions in pediatric subjects. Such knowledge will enable us to overcome the present limitations of obesity lifestyle interventions and design targeted and personalized interventions to prevent and manage obesity during childhood based on individual genotype–nutrition and genotype–exercise interactions early in life. In the future, a knowledge of the individual genotype alongside a thorough understanding of the pathophysiological mechanisms mediating obesity and gene–environment interactions may contribute significantly to the prevention or management of excess adiposity in individuals that are genetically predisposed to and/or with overweight and obesity. Considering the comorbidities of obesity early in life, from the age of childhood and adolescence, as well as the independent risk of developing such obesity-induced diseases later in life, it becomes evident that such interventions should be implemented from a young age.
Our review has several important strengths. The methodology was structured on a strict application of the PRISMA guidelines. To the best of our knowledge, this is the first review to systematically examine the gene–environment interplay in childhood obesity lifestyle interventions, and it also examined the potential underlying pathophysiological mechanisms. As far as the limitations are concerned, most of the included studies focused on genetic variations on a limited number of genes, from one to three, with few of them having examined a greater number [ 62 , 69 ], reaching a maximum in studied variants of 56 obesity susceptibility loci in the study by Heitkamp et al. [ 55 ]. The cumulative effect of the genetic loci was examined only by Moleres et al. in 2012 [ 61 ] and Hollensted et al. [ 56 ], who used a genetic risk score approach. In addition, a great heterogeneity in the duration of the interventions was noted, ranging from 4 weeks to 10 years. This subject has long been debated in the literature, with the necessity for continuous weight loss and maintenance interventions [ 247 , 248 ]. A recent systematic review concerning the pediatric population suggested a minimum intervention of 6 months to reach a reduction in BMI/BMI z-score [ 76 ]. Other limitations to consider are the small sample size in many of the studies, the heterogeneity in the components of the interventions, and the lack of studies examining the effect of CNVs.
5. Conclusions
Preventing and treating obesity early in life, in childhood, and in adolescence should be a priority for public healthcare systems. Despite advances in pharmacotherapy such as the approval of liraglutide, a glucagon-like peptide (GLP-1) analogue, and setmelanotide, an MC4R agonist, for use in the pediatric population and the improvement in the techniques of bariatric surgery towards the management of obesity during adolescence, multidisciplinary lifestyle intervention programs remain the first-line treatment. Behavioral management programs have been proven successful in the improvement of BMI, body composition, and/or cardiometabolic profile in children and adolescents with overweight and obesity. Nevertheless, an inter-individual variability in the response to such interventions is observed, and a remission often occurs after the end of the active phase of interventions, leading to moderate results in the long term. This variability may be partially explained by genetic variations, genetic susceptibility to obesity, and gene–environment interactions. Therefore, interest has been focused on precision medicine and the design and implementation of multidisciplinary, personalized obesity lifestyle interventions through the decoding of the genetic and molecular/cellular pathophysiology of obesity and the gene–environment interactions.
Overall, it is of paramount importance to design robust GWAS, using either genome-wide SNP micro-arrays or whole-genome sequencing (WGS) techniques, in which a large sample of pediatric patients with overweight and obesity undergo structured obesity management lifestyle interventions of adequate duration. Such studies would enable researchers to study a vast number of genetic loci throughout the genome, providing an integrated view, further elucidating the gene–environment interplay, and facilitating translational research.
Supplementary Materials
The following supporting information can be downloaded at https://www.mdpi.com/article/10.3390/nu15061416/s1 : Table S1: Lifestyle intervention characteristics; Table S2: Newcastle—Ottawa Quality Assessment Scale for Non-Randomized Control Studies—Cohort Studies; Table S3: Newcastle—Ottawa Quality Assessment Scale for Randomized Controlled Trials; Table S4: BMI and body composition outcomes in relation to the genotype.
Funding Statement
This research received no external funding.
Author Contributions
Conceptualization, A.V.; methodology, investigation, and resources, A.V. and G.P.; writing—original draft preparation, A.V.; writing—review and editing, E.C., G.P. and A.V.; supervision, E.C. All authors have read and agreed to the published version of the manuscript.
Institutional Review Board Statement
Informed consent statement, data availability statement, conflicts of interest.
The authors declare no conflict of interest.
Disclaimer/Publisher’s Note: The statements, opinions and data contained in all publications are solely those of the individual author(s) and contributor(s) and not of MDPI and/or the editor(s). MDPI and/or the editor(s) disclaim responsibility for any injury to people or property resulting from any ideas, methods, instructions or products referred to in the content.
- Case report
- Open access
- Published: 23 April 2024
Genetic exploration of Dravet syndrome: two case report
- Agung Triono 1 ,
- Elisabeth Siti Herini ORCID: orcid.org/0000-0003-2571-8310 1 &
Journal of Medical Case Reports volume 18 , Article number: 215 ( 2024 ) Cite this article
Metrics details
Dravet syndrome is an infantile-onset developmental and epileptic encephalopathy (DEE) characterized by drug resistance, intractable seizures, and developmental comorbidities. This article focuses on manifestations in two Indonesian children with Javanese ethnicity who experienced Dravet syndrome with an SCN1A gene mutation, presenting genetic analysis findings using next-generation sequencing.
Case presentation
We present a case series involving two Indonesian children with Javanese ethnicity whom had their first febrile seizure at the age of 3 months, triggered after immunization. Both patients had global developmental delay and intractable seizures. We observed distinct genetic findings in both our cases. The first patient revealed heterozygous deletion mutation in three genes ( TTC21B , SCN1A , and SCN9A ). In our second patient, previously unreported mutation was discovered at canonical splice site upstream of exon 24 of the SCN1A gene. Our patient’s outcomes improved after therapeutic evaluation based on mutation findings When comparing clinical manifestations in our first and second patients, we found that the more severe the genetic mutation discovered, the more severe the patient’s clinical manifestations.
These findings emphasize the importance of comprehensive genetic testing beyond SCN1A , providing valuable insights for personalized management and tailored therapeutic interventions in patients with Dravet syndrome. Our study underscores the potential of next-generation sequencing in advancing genotype–phenotype correlations and enhancing diagnostic precision for effective disease management.
Peer Review reports
Dravet syndrome (DS), previously known as severe myoclonic epilepsy of infancy (SMEI), is an infantile-onset developmental and epileptic encephalopathy (DEE) characterized by drug resistance, intractable seizures, and comorbidities including intellectual disability, behavioral problems, sleep disturbances, gait disturbances, and an increased risk of sudden unexpected death in epilepsy [ 1 , 2 ]. The incidence of DS is approximately 1 in every 15,700 births [ 3 ]. The first symptom of DS is seizures in the first year of life, followed by developmental delay [ 1 ]. This first seizure is either generalized tonic–clonic or focal (occasionally hemiclonic) clonic, and in more than half of the cases, it is a febrile seizure, making it difficult to distinguish from a self-limiting febrile seizure. Infection, hot environment, exhaust, sunlight, or exercise can initiate an attack of DS [ 4 , 5 ]. Approximately 80% of patients with DS carry a pathogenic variant of the sodium channel alpha 1 subunit ( SCN1A ) gene resulting in haploinsufficiency Nav1.1, the alpha-1 subunit of the sodium channel. PCDH19, SCN2A, SCN8A, SCN1B, GABRA1, GABRB3, GABRG2, KCNA2, CHD2, CPLX1, HCN1A, and STXBP1 variants may also be involved in DS or DS-like phenotypes. Accordingly, genetic testing is required to identify other genes that play a role in the DS phenotype and to expand genotype-DS phenotype correlations to enhance the future management of this disease [ 6 ]. In the last decade, next-generation sequencing (NGS) technology has been able to analyze a set of genes (targeted panel sequencing), exome [(whole exome sequencing (WES)], or genome [whole genome sequencing (WGS)] in a single sequencing process, making it possible to diagnose rare diseases such as early childhood epilepsy [ 7 ]. Identification of the genetic basis of DS can provide additional information regarding pathophysiology, prognosis, and individual drug therapy options according to the patient’s condition.
We present a case series involving two children, one aged 11 years and 2 months, and the other aged 1 year and 4 months. Both children were diagnosed with DS, exhibiting symptoms of intractable seizures, global developmental delay, and seizures triggered by postimmunization fever. Despite displaying similar symptoms, the two individuals possess different genetic variants of the SCN1A gene and also possible novel mutation in DS. We also discuss the main clinical characteristics, treatment course, and management of DS at tertiary referral hospitals in Indonesia.
A boy with Javanese ethnicity aged 11 years and 2 months with uncontrollable seizures regularly visits our hospital. The patient had his first seizure at the age of 3 months with a duration of 15 min, and it was triggered after receiving diphtheria–pertussis–tetanus (DPT) immunization, which was accompanied by fever. The patient has about six to seven seizures per day for 1 min in the form of generalized tonic–clonic and absence seizures. He was the first child of nonconsanguineous healthy parents with normal prenatal and birth history. He has a younger sister with normal development. There is no history of family members with febrile seizure. The patient was born at 40 weeks of gestation, with a birth weight of 4000 g, length of 52 cm, and head circumference of 33 cm. The patient is currently experiencing global developmental delay and is still in kindergarten. He had learning difficulties and was unable to speak words at an age-appropriate level. He had delayed motor development and was unable to perform age-appropriate motor activities. Head circumference was 46.5 cm (microcephaly). There were no signs of meningeal irritation nor Babinski response. The motor examination revealed no increased tone in the upper and lower limb. Other systemic examinations revealed no abnormalities. Interictal electroencephalography (EEG) showed diffuse epileptiform irritative abnormality on a normal background (Fig. 1 ). Magnetic resonance imaging (MRI) of the brain showed cerebral atrophy, bilateral frontal subarachnoid enlargement, bilateral occipital lobe and polymicrogyria, and a neuroglial cyst in the right temporal lobe (Fig. 2 ). He was recommended to get genetic testing done since he was suspected of having DS.
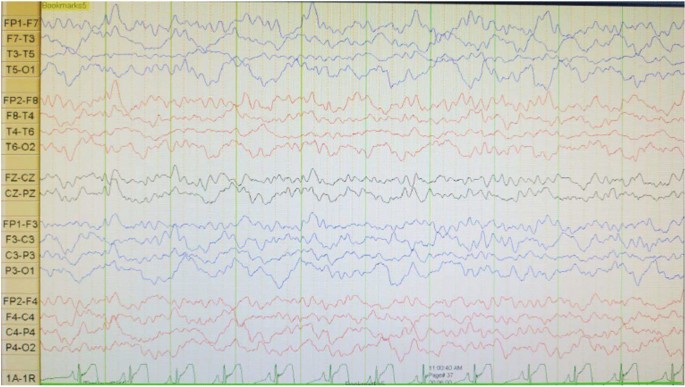
Electroencephalography (EEG) shows diffuse epileptiform irritative abnormality on a normal background
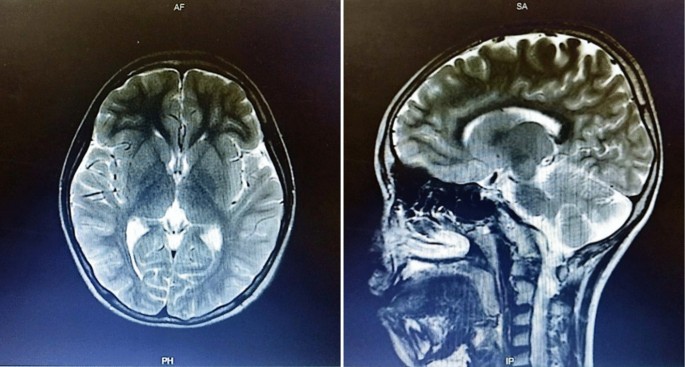
Axial brain magnetic resonance imagery shows cerebral atrophy, bilateral frontal subarachnoid enlargement, bilateral occipital lobe polymicrogyria, and a neuroglial cyst in the right temporal lobe
Whole genome sequencing (WGS), whole exome sequencing (WES), and Sanger sequencing were performed at 3Billion (Seoul, Korea). The WGS and WES procedures were conducted according to the protocols of Richards et al . [ 8 ] and Seo et al . [ 9 ], respectively. Both WES and WGS are comprised of four main parts: (1) high-quality sequencing; (2) sequencing data analysis including alignment to the genome reference consortium human 37 (GRCh37)/hg19 for WES, also alignment to the genome reference consortium human 38 (GRCh38) and revised Cambridge reference sequence (rCRS) of the mitochondrial genome for WGS; (3) variant annotation and prioritization by EVIDENCE [a software that was developed in house to prioritize variants based on the American College of Medical Genetics and Genomics (ACMG) guidelines [ 10 ]]; and (4) variant interpretation in the context of the patient’s symptoms and reporting of disease-causing variants. Once EVIDENCE prioritizes the top candidate variants/genes, 3Billion’s highly-trained clinical/medical geneticists manually curate each variant to identify the disease-causing variant for reporting.
In our initial examination, we performed WES on patient 1 and subsequently identified a copy number variant (CNV), prompting us to proceed with WGS. The WGS analysis revealed a heterozygous pathogenic 552.9 Kb deletion variant in 2q24.3. The heterozygous deletion NC_000002.12:g.165811316_166364199delinsTGTACACTA at 2q24.3 spans across three genes ( TTC21B , SCN1A , and SCN9A ). The variant is not observed in the gnomAD SVs v2.1.1 dataset. SCN1A is subject to haploinsufficiency. Other pathogenic variants have been reported in this region. There are multiple similarly affected individuals reported with similar likely pathogenic copy–number–loss overlapping this region [ 11 , 12 ]. Therefore, this variant was classified as pathogenic. Due to region-spanning mutation in SCN1A, which suitable with clinical manifestation, the patient was diagnosed with DS (OMIM 607208: since we were unable to perform a Sanger sequencing study on both of the parents, the pattern of inheritance is still uncertain.
The arents were counseled about their child’s condition and agreed to undergo multipronged therapy. Before the patient was diagnosed with DS, he had received valproic acid (30 mg/kg per day), phenobarbital (2.5 mg/kg per day), and oxcarbazepine (5 mg/kg per day), also physio, occupation, and speech therapy but had not shown significant improvement. He was seizure-free for 3 months after oxcarbazepine was changed to levetiracetam (27 mg/kg per day). However, the patient then had another episodes of less than 5 minutes general tonic–clonic seizure (GTCS)-induced by fever. Interictal EEG was performed to evaluate his condition, and we found that the diffuse epileptiform irritative abnormality persisted.
A 1 year and 4 month-old-girl with Javanese ethnicity was referred to our hospital due experiencing myoclonic seizure followed by 20 minute GTCS at 3 months, after fever following DPT immunization. She then continued to experience generalized tonic–clonic seizures one to two times per day for 10–15 seconds. At 9 months of age, the patient received a second DPT immunization, and on the same day, she had another generalized tonic–clonic seizure that lasted > 30 minutes, resulting in her admission to the pediatric intensive care unit. Before the first seizure, the patient could lift her head, grasp a toy and make eye contact, but after that, she could neither lift her head nor grasp an object. The patient has no previous history of trauma.
She had a normal head circumference increased physiological reflexes in all extremities. Other systemic examinations revealed no abnormalities. Computed tomography (CT) scan examination of the head showed a subdural hygroma in the right and left frontoparietal region, without any other abnormalities (Fig. 3 ). Electroencephalography (EEG) at the beginning of the seizure did not show any abnormalities, but the EEG follow-up 7 months after the onset of the seizure showed an abnormal epileptiform (spike wave) with a normal background (Fig. 4 ). Thus, she was suspected of having DS and was recommended to undergo genetic examination.
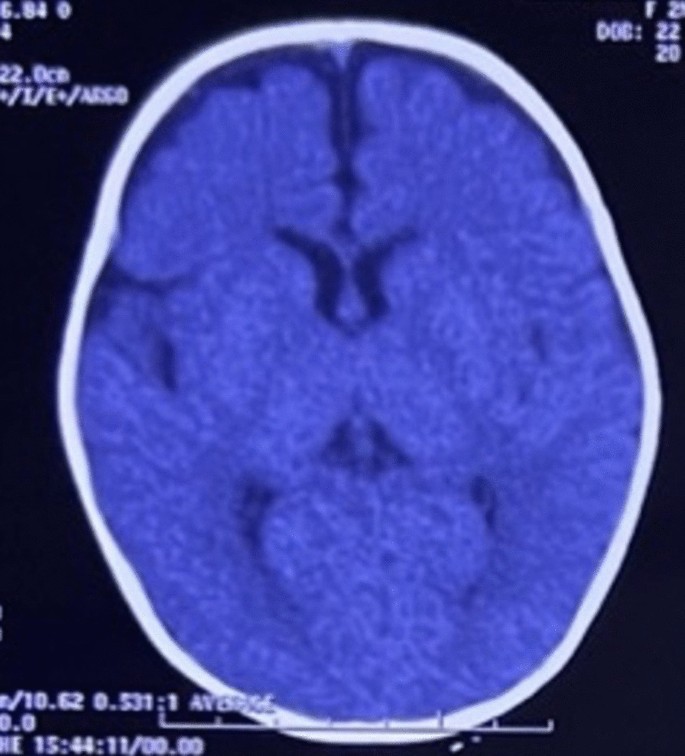
Axial brain computed tomography scan shows a subdural hygroma in the right and left frontoparietal region, without any other abnormalities
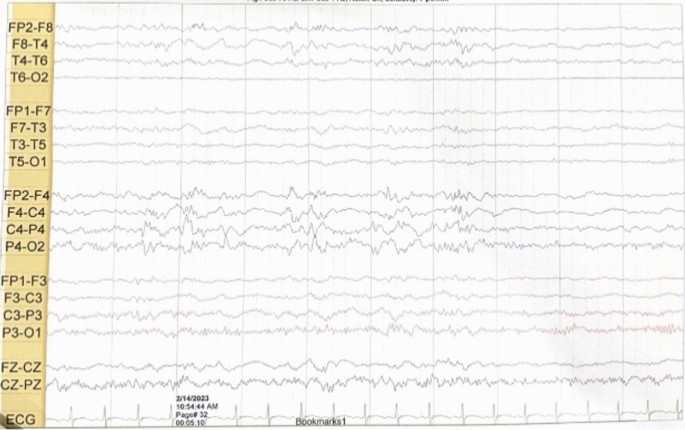
Electroencephalography shows abnormal irritative epileptiform with a normal background
Whole exome sequencing (WES) showed a likely pathogenic variant identified as a heterozygous mutation of the SCN1A gene with genomic position 2-166859265-T-C (GRCh37), [NM_001165963.4:C.4003-2A > G [NP_001159435.1:p.?]. The variant is located in the canonical splice site upstream of exon 24 of SCN1A gene (NM_001165963.4 transcript). Since this variant is an essential splicing variant, the protein consequence is uncertain and therefore represented as (p.?). In this patient’s genetic mutation, the canonical junction site occurs which is expected to alter the junction and result in loss or disruption of normal protein function. However, using an in silico predictor, spliceAI ( https://spliceailookup.broadinstitute.org/ ), the variant is predicted to result in a loss of 22 base pairs at end of exon 24. This loss is expected to create a frameshift at the Gly1342 position. Sanger sequencing confirmed the patient’s genotype (Fig. 5 A), but the mother’s Sanger analysis was negative (Fig. 5 B). Due to familial issues, Sanger sequencing was not performed on the father, leaving the inheritance pattern unresolved.
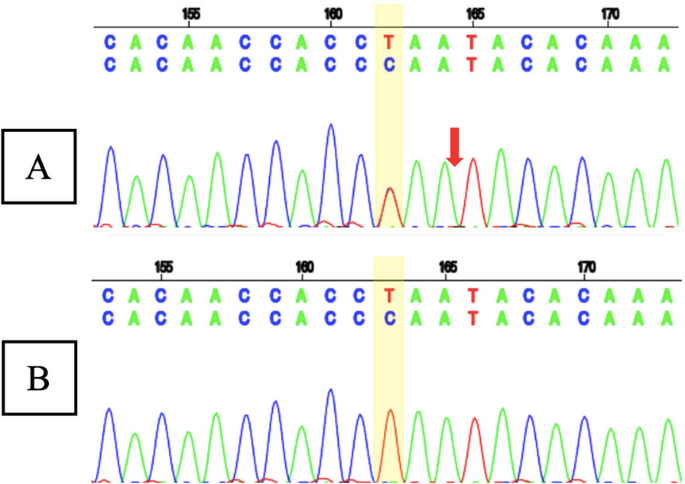
A Sanger sequencing result of patient 2 showed a heterozygous mutation of the SCN1A gene with the genomic position 2-166859265-T-C (GRCh37), [NM_001165963.4:C.4003-2A >G [NP_001159435.1:p.?] (red arrow); and B Sanger sequencing result of patient 2’s mother showed normal sequence
The parents were counseled about their child’s condition and agreed to undergo multipronged therapy. Before patient was diagnosed with DS, she received clonazepam (0.01 mg/kg per day), valproic acid (29 mg/kg per day), and phenytoin (5 mg/kg per day), but seizure persisted. When phenytoin was stopped, with valproic acid (30 mg/kg per day) and clonazepam (0.04 mg/kg per day) adjusted, seizures were greatly decreased. Later, patient only experienced one seizure per year. The patient routinely received physio, speech, and occupational therapy.
When comparing the clinical features and outcomes of the two patients (Table 1 ), we found that our first patient, who had three medications, was still having a generalized seizure induced by fever with duration less than 5 minutes after they had been seizure-free for 3 months (at the age 11 years and 8 months. Our second patient, however, only experienced one seizure annually after receiving two medications (at the age 1 year and 10 months). This difference implies that the clinical state of the first patient was worse than that of the second.
Research on the identification of DS genetic mutations using NGS has never been done in Indonesia. In 2010, we conducted a study to identify pathogenic variants of the SCN1A gene using the Sanger sequencing method and successfully reported cases of novel SCN1A mutations in Indonesia in patients with severe myoclonic epilepsy in infancy (SMEI) and borderline SMEI (SMEB). The first boy identified with SMEI experienced a variety of seizures, including his first febrile seizure and general tonic–clonic seizure at 7 months of age, and later suffered from myoclonic seizures, left-sided hemiconvulsions, also focal convulsions without fever, along with delayed speech development. The second patient with SMEB had his first febrile seizures with GTCS after immunization at 3 months old, then later on experienced status epilepticus, GTCS, and atonic convulsions without fever [ 13 ]. We also conducted another research on the spectrum of generalized epilepsy with febrile seizure plus (GEFS+) focusing on clinical manifestations and SCN1A gene mutations. That study analyzed a total of 34 patients who suffered from SMEI (7 patients), SMEB (7 patients), febrile seizure plus (FS+) and absence/myoclonic/atonic/partial seizures (11 patients), and FS+ (9 patients) [ 14 ].
However, the research that we have done uses the Sanger sequencing genetic examination, which is expensive and takes considerable time. Additionally, it is unable to find any other gene besides SCN1A in patients with DS. A study by Djémié et al . in Belgium reported the discovery of 28 pathogenic variants of the SCN1A gene using the NGS method which were previously missed or undiagnosed using Sanger sequencing [ 7 ]. To link DS cases more effectively, we are attempting to conduct NGS genetic tests, specifically WES and WGS.
Dravet syndrome (DS) was infrequently reported in Indonesia due to its difficulty in diagnosis, misdiagnosis as febrile seizures or other epilepsy syndromes, or lack of follow-up and genetic testing in our country. According to the to the International League Against Epilepsy (ILAE) [ 15 ], the diagnostic criteria for this condition should consist of a number of the following symptoms: (1) a family history of epilepsy or febrile seizures; (2) normal development before seizures onset; (3) seizure before 1 year of age; (4) EEG with generalized spike and polyspike waves; (5) pleomorphic epilepsy (myoclonic, focal, clonic, absence, and generalized seizures); (6) focal abnormalities or early photosensitivity; (7) psychomotor retardation after 24 months; (8) exacerbation of seizures with increased body temperature; and (9) the appearance of subsequent ataxia, pyramidal signs or interictal myoclonus after the beginning of psychomotor slowing. Both of our patients had seizures beginning with increased body temperature and regression of development after seizure onset, which were resistant to the majority of anticonvulsant medications. The seizures began as generalized tonic–clonic seizures, followed by absence seizures. Both of our patients also experienced subsequent ataxia and pyramidal signs. Thus, they were suspected of having DS and were advised to undergo genetic testing.
Infants with DS have normal physical and psychomotor development at the time of their first seizure, which typically occurs between the ages of 5 and 8 months. In our case series, both of our patients experienced their first seizure at the age of 3 months [ 16 , 17 ]. In the first year of life, the most common form of seizure is febrile tonic–clonic. Some patients may experience myoclonic and dyscognitive seizures infrequently. Frequently, protracted seizures result in status epilepticus. In the first year of life, seizures are precipitated by fever/illness, immunization, and cleansing [ 16 ]. As the infant develops, he or she will experience a variety of seizure types, as well as fever and emotional stress, flashes of light, and overexertion being seizure triggers. The child with DS will develop hypotonia, ataxia, incoordination, and pyramidal signs, dysautonomia events, cognitive impairment, and behavioral disturbances such as attention deficit, hyperactivity, or autistic characteristics [ 15 ]. Some of the conditions above are very consistent with what happened to our patients.
The EEG performed during the early phases of the disease is normal. However, as the child grows, generalized spike waves with isolated or brief discharges of fast polyspike waves may be present [ 15 , 18 ]. In the first case, we found diffuse epileptiform irritative abnormality with a normal background, whereas in the second case, initially it was found normal, then a few months later it became abnormal irritative epileptiform with a normal background.
Genetic testing is developing rapidly and playing a significant role in the specific diagnosis and management of epilepsy [ 19 , 20 ]. Several genes with pathogenic mutations produce DS or DS-like phenotypes, which inevitably require different drug therapy approaches. Genes that cause DS can be grouped based on how they work: specifically, three sodium channel-related genes ( SCN2A, SCN8A , and SCN1B ), one potassium channel-related gene ( KCNA2 ), three gamma-aminobutyric acid receptors ( GABAR ) genes ( GABRA2, GABRB3 , and GABRG2 ), a cyclic nucleotide gated cation channel gene ( HCN1 ), and other functional genes including CHD2, CPLX1 , and STXBP1 . Approximately 80% of patients with DS have a pathogenic variant of the SCN1A gene, from which the majority of SCN1A variants are de novo, but 10% of people inherit the SCNA1 mutation from one or both parents [ 6 ]. Both of our patients had a mutation in the SCN1A gene, which is the most common mutation seen in DS.
Furthermore, TTC21B and SCN9A mutations were also found in our first patient. A study conducted by Suls et al . also reported a four generation Bulgarian family with epilepsy, revealing a heterozygous 400 kb deletion on chromosome 2q24 that included the SCN1A and TTC21B genes [ 21 ]. The patients exhibited variable phenotypes, but all experienced generalized tonic–clonic seizures around the first year of life, with some presenting myoclonic or absence seizures. Febrile seizures occurred in three of the four patients during infancy. Notably, one patient had mild mental retardation, another had psychomotor slowing, and a third had mental retardation from early infancy; all showed reduced seizures on medication. The findings in that study parallel the situation observed in our initial patient case. Meanwhile, a study by Singh et al . identified a heterozygous mutation in the SCN9A gene in two patients diagnosed with DS [ 22 ]. One of these patients also exhibited a mutation in the SCN1A gene. The study provided evidence suggesting that the SCN9A gene on chromosome 2q24 could potentially serve as a modifier for DS. Among 109 patients with DS, 8% were found to have an SCN9A mutation. This included six patients with double heterozygosity for SCN9A and SCN1A mutations and three patients with only heterozygous SCN9A mutations, supporting the notion of a multifactorial inheritance pattern [ 22 ]. The previous research confirmed the severity of clinical symptoms in our first patient, whom we identified mutations in the SCN1A, SCN9A , and TTC21B genes.
In the last decade, there has been a very rapid development of neurogenetic science and diagnostic technology. NGS is the latest method of genetic examination that allows for the discovery of causal mutations, including de novo, novel, and familial mutations related to epilepsy syndromes that have variable phenotypic features [ 23 ]. The first generation of DNA sequencing using the Sanger method could only examine one gene at a time and had limitations especially when examining large genomic regions, so the NGS method is more widely used today [ 7 , 23 ]. A study conducted by Kim et al . in Seoul reported an increase in diagnostic yield using WES after targeted panel sequencing with negative results in infantile onset epilepsy by 8%. This result suggests that WES assays increase the opportunity to search for new epilepsy genes and uncover less well-known epileptic phenotypes from known neurological diseases [ 24 ]. The WES examination also allows for the discovery of de novo or inherited mutations if the patient and both parents are examined [ 25 ].
According to the recommendations of the North American consensus panel, clobazam and valproic acid are the first-line therapies for antiepileptic drugs, followed by stiripentol, topiramate and levetiracetam. Patients with a suboptimal response to clobazam and valproic acid have been advised to consider the ketogenic diet as a second-line treatment [ 17 ]. SCN1A is a gene that codes for sodium channel channels, so drugs that work as sodium channel blockers, such as lamotrigine, phenytoin, carbamazepine, oxcarbazepine, lacosamide, and rufinamide, are contraindicated in patients with DS because they can increase the frequency of seizures [ 4 ]. After the failure of first- and second-line therapy, surgical therapies, such as vagus nerve stimulation (VNS), were moderately agreed upon and should be considered [ 17 ]. Besides medication, controlling infections and body temperature variations also showed to decrease the frequency of seizures and severity of the disease [ 18 ]. Initially, the first patient received oxcarbazepine and the second patient got phenytoin, which had been contraindicated to patients with DS. Futhermore, after eliminating medications that were contraindicated, both patients’ outcome improved.
In this study, we discovered unique mutations that have never been documented before, particularly in Indonesia, where NGS analysis of DS genetic variants has never been done. However, the limitation of this study, is that the information comes from two cases only. Further research is needed to explore more cases from Indonesia population.
In summary, our case series utilizing next-generation sequencing (NGS) unveils the intricate genetic landscape of Dravet syndrome (DS) in two Indonesian pediatric cases. By using WGS and WES, we identified distinct mutations in the SCN1A gene, as well as contributions from genes, such as TTC21B and SCN9A . The power of WGS lies in its ability to uncover rare pathogenic variants, including a 552.9 Kb deletion in the 2q24.3 region. These findings emphasize the importance of comprehensive genetic testing beyond SCN1A , providing valuable insights for personalized management and tailored therapeutic interventions in patients with DS. Our study underscores the potential of NGS in advancing genotype–phenotype correlations and enhancing diagnostic precision for effective disease management. Furthermore, we found that the clinical condition of the first patient was worse than that experienced by the second patient. This difference suggests that the more severe the genetic mutation detected, the more severe the clinical manifestations of the patient.
Availability of data and materials
The dataset used and/or analyzed during the current study are available from the corresponding author on reasonable request.
Abbreviations
American College Of Medical Genetics
Copy number variant
Computed tomography
- Dravet syndrome
Developmental and epileptic encephalopathy
Electroencephalography
Febrile seizure plus
Generalized epilepsy with febrile seizure plus
Genome reference consortium human 37
Genome reference consortium human 38
General tonic clonic seizure
International league against epilepsy
Magnetic resonance imaging
- Next-generation sequencing
Revised Cambridge reference sequence
Sodium channel alpha 1 subunit
Severe myoclonic epilepsy of infancy-borderline
Severe myoclonic epilepsy in infancy
Vagus nerve stimulation
Whole-exome sequencing
Whole-genome sequencing
Zuberi SM, Wirrell E, Yozawitz E, Wilmshurst JM, Specchio N, Riney K, et al . ILAE classification and definition of epilepsy syndromes with onset in neonates and infants: position statement by the ILAE Task Force on Nosology and Definitions. Epilepsia. 2022;63(6):1349–97.
Article PubMed Google Scholar
Wirrell EC, Hood V, Knupp KG, Meskis MA, Nabbout R, Scheffer IE, et al . International consensus on diagnosis and management of Dravet syndrome. Epilepsia. 2022;63(7):1761–77.
Article CAS PubMed PubMed Central Google Scholar
Isom LL, Knupp KG. Dravet syndrome: novel approaches for the most common genetic epilepsy. Neurotherapeutics. 2021;18(3):1524–34.
Cardenal-Muñoz E, Auvin S, Villanueva V, Cross JH, Zuberi SM, Lagae L, et al . Guidance on Dravet syndrome from infant to adult care: road map for treatment planning in Europe. Epilepsia Open. 2022;7(1):11–26.
Chen C, Fang F, Wang X, Lv J, Wang X, Jin H. Phenotypic and genotypic characteristics of SCN1A associated seizure diseases. Front Mol Neurosci. 2022;28(15): 821012.
Article Google Scholar
Ding J, Wang L, Jin Z, Qiang Y, Li W, Wang Y, et al . Do all roads lead to Rome? Genes causing Dravet syndrome and Dravet syndrome-like phenotypes. Front Neurol. 2022;11(13): 832380.
Djémié T, Weckhuysen S, Von Spiczak S, Carvill GL, Jaehn J, Anttonen A, et al . Pitfalls in genetic testing: the story of missed SCN1A mutations. Mol Genet Genomic Med. 2016;4(4):457–64.
Article PubMed PubMed Central Google Scholar
Richards S, Aziz N, Bale S, Bick D, Das S, Gastier-Foster J, et al . Standards and guidelines for the interpretation of sequence variants: a joint consensus recommendation of the American College of Medical Genetics and Genomics and the Association for Molecular Pathology. Genet Med. 2015;17(5):405–24.
Seo GH, Kim T, Choi IH, Park J, Lee J, Kim S, et al . Diagnostic yield and clinical utility of whole exome sequencing using an automated variant prioritization system, EVIDENCE . Clin Genet. 2020;98(6):562–70.
Riggs ER, Andersen EF, Cherry AM, Kantarci S, Kearney H, Patel A, et al . Technical standards for the interpretation and reporting of constitutional copy-number variants: a joint consensus recommendation of the American College of Medical Genetics and Genomics (ACMG) and the Clinical Genome Resource (ClinGen). Genet Med. 2020;22(2):245–57.
Lim BC, Hwang H, Kim H, Chae JH, Choi J, Kim KJ, et al . Epilepsy phenotype associated with a chromosome 2q243 deletion involving SCN1A: migrating partial seizures of infancy or atypical Dravet syndrome? Epilepsy Res. 2015;109:34–9.
Article CAS PubMed Google Scholar
Fry AE, Rees E, Thompson R, Mantripragada K, Blake P, Jones G, et al . Pathogenic copy number variants and SCN1A mutations in patients with intellectual disability and childhood-onset epilepsy. BMC Med Genet. 2016;17(1):34.
Herini ES, Gunadi, Van Kempen MJA, Yusoff S, Sutaryo, Sunartini, et al. Novel SCN1A mutations in Indonesian patients with severe myoclonic epilepsy in infancy. Pediatr Int. 2010;52(2):234–9.
Herini ES, Gunadi, Harahap ISK, Yusoff S, Morikawa S, Patria SY, et al. Generalized epilepsy with febrile seizures plus (GEFS+) spectrum: clinical manifestations and SCN1A mutations in Indonesian patients. Epilepsy Res. 2010;90(1–2):132–9.
Anwar A, Saleem S, Patel UK, Arumaithurai K, Malik P. Dravet syndrome: an overview. Cureus. 2019. https://www.cureus.com/articles/20900-dravet-syndrome-an-overview . Accessed 20 Nov 2023.
Brunklaus A, Dorris L, Ellis R, Reavey E, Lee E, Forbes G, et al . The clinical utility of an SCN1A genetic diagnosis in infantile-onset epilepsy. Dev Med Child Neurol. 2013;55(2):154–61.
Wirrell EC, Laux L, Donner E, Jette N, Knupp K, Meskis MA, et al . Optimizing the diagnosis and management of Dravet syndrome: recommendations from a North American Consensus Panel. Pediatr Neurol. 2017;68:18-34.e3.
Yadav R, Shah S, Bhandari B, Marasini K, Mandal P, Murarka H, et al . Patient with Dravet syndrome: a case report. Clin Case Rep. 2022;10(5): e05840.
Møller RS, Dahl HA, Helbig I. The contribution of next generation sequencing to epilepsy genetics. Expert Rev Mol Diagn. 2015;15(12):1531–8.
Yozawitz E, Moshé SL. The influence of genetics on epilepsy syndromes in infancy and childhood. Acta Epileptol. 2022;4(1):41.
Suls A, Velizarova R, Yordanova I, Deprez L, Van Dyck T, Wauters J, et al . Four generations of epilepsy caused by an inherited microdeletion of the SCN1A gene. Am Acad Neurol. 2010;75(72):72–6.
CAS Google Scholar
Singh NA, Pappas C, Dahle EJ, Claes LRF, Pruess TH, De Jonghe P, et al . A role of SCN9A in human epilepsies, as a cause of febrile seizures and as a potential modifier of Dravet syndrome. PLoS Genet. 2009;5(9): e1000649.
Dunn P, Albury CL, Maksemous N, Benton MC, Sutherland HG, Smith RA, et al . Next generation sequencing methods for diagnosis of epilepsy syndromes. Front Genet. 2018;7(9):20.
Kim SY, Jang SS, Kim H, Hwang H, Choi JE, Chae J, et al . Genetic diagnosis of infantile-onset epilepsy in the clinic: application of whole-exome sequencing following epilepsy gene panel testing. Clin Genet. 2021;99(3):418–24.
Poduri A, Sheidley BR, Shostak S, Ottman R. Genetic testing in the epilepsies—developments and dilemmas. Nat Rev Neurol. 2014;10(5):293–9.
Download references
Acknowledgements
The authors express their gratitude to the patient and their families for their cooperation, as well as to all the staff and nurses who provided care for the patient. We are also thankful for the Faculty of Medicine, Public Health, and Nursing, Universitas Gadjah Mada for funding this research and providing English editing services for assistance in the editing and proofreading process. Additionally, we appreciate the assistance of Kristy Iskandar, Marissa Leviani Hadiyanto and Khansadhia Hasmaradana Mooiindie during the data collection and editing phases.
This study was supported by the Faculty of Medicine, Public Health and Nursing, Universitas Gadjah Mada, (Dana Masyarakat to ESH). The funding body did not influence the study design, data analysis, data interpretation, nor manuscript writing.
Author information
Authors and affiliations.
Department of Child Health, Faculty of Medicine, Public Health and Nursing, Universitas Gadjah Mada, Dr. Sardjito Hospital, Jl. Kesehatan No. 1, Yogyakarta, 55281, Indonesia
Agung Triono & Elisabeth Siti Herini
Pediatric Surgery Division, Department of Surgery/Genetics Working Group/Translational Research Unit, Faculty of Medicine, Public Health and Nursing, Universitas Gadjah Mada, Dr. Sardjito Hospital, Yogyakarta, 55281, Indonesia
You can also search for this author in PubMed Google Scholar
Contributions
ESH, AG, and G made substantial contributions to the conception and design of the work. AG contributed to data acquisition. ESH, AG, and G performed the data analyses and the interpretation of the data. ESH and AG drafted the text and prepared the figures. ESH, AG, and G revised, read, and approved the final manuscript. All authors approve the present version for publication, and are accountable for all aspects related to the study.
Corresponding author
Correspondence to Elisabeth Siti Herini .
Ethics declarations
Ethics approval and consent to participate.
The Medical and Health Research Ethics Committee of the Faculty of Medicine, Public Health and Nursing, Universitas Gadjah Mada, Yogyakarta, Indonesia approved all recruitment and research protocols. Eligible patients signed an informed consent form (ethical clearance number KE-FK-0455-EC-2023; dated March 2023). Patients older than 12 years old and/or their parents or guardian (for patients < 12 years old) signed a written informed consent form to be included in this study.
Consent for publication
Written informed consent was obtained from the patients’ legal guardians for publication of this case report and any accompanying images.
Competing interests
The authors declare that they have no competing interests.
Additional information
Publisher’s note.
Springer Nature remains neutral with regard to jurisdictional claims in published maps and institutional affiliations.
Rights and permissions
Open Access This article is licensed under a Creative Commons Attribution 4.0 International License, which permits use, sharing, adaptation, distribution and reproduction in any medium or format, as long as you give appropriate credit to the original author(s) and the source, provide a link to the Creative Commons licence, and indicate if changes were made. The images or other third party material in this article are included in the article's Creative Commons licence, unless indicated otherwise in a credit line to the material. If material is not included in the article's Creative Commons licence and your intended use is not permitted by statutory regulation or exceeds the permitted use, you will need to obtain permission directly from the copyright holder. To view a copy of this licence, visit http://creativecommons.org/licenses/by/4.0/ . The Creative Commons Public Domain Dedication waiver ( http://creativecommons.org/publicdomain/zero/1.0/ ) applies to the data made available in this article, unless otherwise stated in a credit line to the data.
Reprints and permissions
About this article
Cite this article.
Triono, A., Herini, E.S. & Gunadi Genetic exploration of Dravet syndrome: two case report. J Med Case Reports 18 , 215 (2024). https://doi.org/10.1186/s13256-024-04514-2
Download citation
Received : 31 January 2024
Accepted : 18 March 2024
Published : 23 April 2024
DOI : https://doi.org/10.1186/s13256-024-04514-2
Share this article
Anyone you share the following link with will be able to read this content:
Sorry, a shareable link is not currently available for this article.
Provided by the Springer Nature SharedIt content-sharing initiative
- Case series
Journal of Medical Case Reports
ISSN: 1752-1947
- Submission enquiries: Access here and click Contact Us
- General enquiries: [email protected]
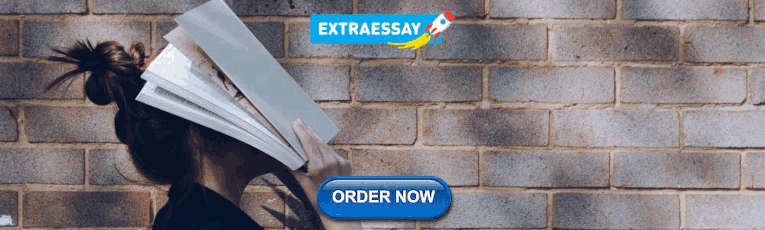
IMAGES
VIDEO
COMMENTS
By contrast, genetic variation associated with common forms of obesity have been identified in large-scale population studies, either using a case-control design or continuous traits such as BMI.
By contrast, genetic variation associated with common forms of obesity have been identified in large-scale population studies, either using a case-control design or continuous traits such as BMI. Gene discovery for both forms of obesity was initially hypothesis driven; that is, restricted to a set of candidate genes that evidence suggests ...
Recent progress in genetics of polygenic traits, particularly represented by genome-wide association studies, led to the discovery of hundreds of genetic variants associated with obesity, which allows constructing polygenic risk scores (PGS). In addition, epigenome-wide association studies helped identifying novel targets and methylation sites ...
The genetics of obesity could be classified into syndromic and non-syndromic obesity with or without congenital defects and developmental delay. For example, ... MC4R) had a combined effect on overweight and obesity at an odds ratio of 1.65 (p = 0.008) in a large case-control study in the Brazilian population . The same MC4R variant ...
The Biology and Genetics of Obesity — A Century of Inquiries. Author: Chin Jou, Ph.D. Author Info & Affiliations. Published May 15, 2014. N Engl J Med 2014;370: 1874 - 1877.
In the early phase of obesity genetic research, the emphasis was on candidate genes of obesity (See Rankinen et al. for a review) (). A literature search identified 547 candidate genes derived from multiple types of studies, and the contribution of SNPs located in ±10 kb flanking sequences around these genes was investigated (). It was ...
Genome-wide association studies (GWAS) have successfully revealed numerous susceptibility loci for obesity. However, identifying the causal genes, pathways, and tissues/cell types responsible for ...
Genetic causes associated with obesity account for 7% of cases of extreme obesity in children [ 16 ]. Inheritability ranges from 40% to 70%, with cultural transmission playing a minor role [ 17 ]. There are several gaps in the study of the genetic causes of obesity, such as lack of awareness, insufficient information on its pathophysiology, and ...
The genetics of obesity could be classified into syndromic and non-syndromic obesity with or without congenital defects and developmental delay. For example, Prader-Willi, fragile X, Bardet-Biedl, Cohen, and Albright Hereditary Os- ... in a large case-control study in the Brazilian population [28]. The same MC4R variant (rs17782313) and an ...
Obesity is a global challenge that greatly impacts human health and behavior. Many studies have shown that obesity, generally defined as high body mass index (BMI), can lead to a range of physical ...
The β3-adrenergic receptor is also expressed in adipose tissue and influences fat metabolism and heat production. An interaction between the Pro12Ala and the Trp64Arg polymorphisms of the PPARγ and β3-AR genes has been observed in a family study of Mexican Americans and a case control study of obesity in Spanish children (47, 48). In the ...
Obesity / epidemiology*. Obesity / etiology. Obesity / genetics*. Obesity (OMIM #601665) is a disease where excessive stores of body fat impact negatively on health. The first law of thermodynamics dictates that energy cannot be created or destroyed so if energy is taken into the body, but not transformed to ATP for metabolic work or dissipated ...
Some genetic and lifestyle factors affect an individual's likelihood of adult obesity; thus, the significant clusters of obesity observed in specific geographical regions and contexts also signal the impact of socioeconomic and environmental factors in "obesogenic" environments [13].Understanding the causes and determinants of obesity is a critical step toward creating effective policy and ...
For the obesity-related polymorphism rs17817449 of the FTO gene, statistically significant associations were found with regard to weight loss during the weight loss intervention in a study by Zlatohlavek et al. 17 However, 7 studies reported no statistically significant associations 9,11,12,14,15,18 or only a trend regarding a genetic ...
Abstract. Obesity (OMIM #601665) is a disease where excessive stores of body fat impact negatively on health. The first law of thermodynamics dictates that energy cannot be created or destroyed so ...
By contrast, genetic variation associated with common forms of obesity have been identified in large-scale population studies, either using a case-control design or continuous traits such as BMI. Gene discovery for both forms of obesity was initially hypothesis driven; that is, restricted to a set of candidate genes that evidence suggests ...
Obesity is closely linked to genetics and environmental factors. The newest studies in the field of epigenetics further our understanding of the effect of the environment on genetics. This article describes the genetic causes of obesity, including syndromic, monogenic, and polygenic causes, and cites specific examples of epigenetic ...
Case study Understanding the genetics involved in obesity . 27 June 2019 . Professor Cecilia Lindgren's research at the Big Data Institute (BDI), University of Oxford, seeks to advance understanding of the underlying mechanisms involved in obesity. By applying a range of genetic approaches, her team looks to identify genetic variants ...
Obesity is a public health crisis, and its prevalence disproportionately affects African Americans in the United States. Dysregulation of organelle calcium homeostasis is associated with obesity. The mitochondrial calcium uniporter (MCU) complex is primarily responsible for mitochondrial calcium homeostasis. Obesity is a multifactorial disease in which genetic underpinnings such as single ...
Studies show a parent's poor diet could affect the genes of generations to come - and set up children and grandchildren for obesity and cardiovascular issues.
Similar to the main case-control study, the influence of genes is greater in men than in women ... Our findings clearly showed that the role played by the studied factors (obesity, PH, and genes ...
Within a given environment, some people put on weight very easily, whilst others remain thin. We aim to understand the key mechanisms that control body weight to inform the development of safe, effective weight loss interventions. We use genetic, molecular and clinical approaches to study cohorts of individuals with severe obesity and thinness ...
People with increased polygenic risk scores for higher body mass index (BMI) would need to walk about 2300 more steps each day to have the same risk of obesity as those with lower scores, a recent retrospective study in JAMA Network Open found. Polygenic risk scores reflect the risk of disease determined by many variants in a person's DNA.The results suggest that exercise recommendations ...
A preclinical study by Weill Cornell Medicine investigators shows that a specific human genetic variant of a receptor that stimulates insulin release may help individuals resist obesity. The ...
The FTO story constitutes a textbook case: the FTO gene has been cloned in mice in 1999 but the "buzz" around this gene started after the publication of two seminal genetic studies demonstrating a link between FTO common gene variation and human obesity in 2007 (at this time, FTO was a gene of unknown function in an unknown pathway) [18, 19 ...
The aim of the study was to explore the impact of maternal prepregnancy overweight/obesity and excessive GWG on cord blood metabolic profiles. A case control study including 33 pairs of mothers with prepregnancy overweight/obesity and their neonates, 30 pairs of mothers with excessive GWG and their neonates, and 32 control mother-neonate pairs.
The growth and development of organism were dependent on the effect of genetic, environment, and their interaction. In recent decades, lots of candidate additive genetic markers and genes had been detected by using genome-widely association study (GWAS). However, restricted to computing power and practical tool, the interactive effect of markers and genes were not revealed clearly.
Numerous studies have revealed that obesity is an important cause for the precocious or early puberty in children, 16,17 particularly noting a higher likelihood in girls with obesity. 18 However ...
As far as the limitations are concerned, most of the included studies focused on genetic variations on a limited number of genes, from one to three, with few of them having examined a greater number [62,69], reaching a maximum in studied variants of 56 obesity susceptibility loci in the study by Heitkamp et al. .
A study conducted by Suls et al. also reported a four generation Bulgarian family with epilepsy, revealing a heterozygous 400 kb deletion on chromosome 2q24 that included the SCN1A and TTC21B genes . The patients exhibited variable phenotypes, but all experienced generalized tonic-clonic seizures around the first year of life, with some ...