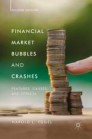
Financial Market Bubbles and Crashes, Second Edition pp 189–218 Cite as
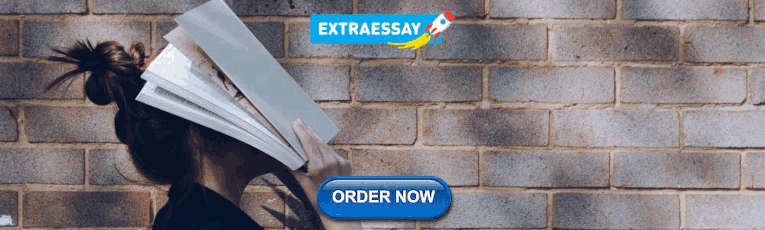
Random Walks
- Harold L. Vogel 2
- First Online: 17 August 2018
832 Accesses
Random walk was an early descriptive phrase and plank in what has come to be called modern portfolio theory. It includes the notions of efficient markets and equilibrium economics. This chapter reviews the subject in detail, not because the theory is necessarily correct and useful, but because it provides a benchmark against which newer and better approaches can be compared. Volatility is a key factor in all bubbles and crashes and an extreme events line based on option volatility is introduced.
This is a preview of subscription content, log in via an institution .
Buying options
- Available as EPUB and PDF
- Read on any device
- Instant download
- Own it forever
Tax calculation will be finalised at checkout
Purchases are for personal use only
According to Fama ( 1970 ), “[A] market in which prices always ‘fully reflect’ available information is called ‘efficient’.” A key insight by Fama ( 1991 ) is that “market efficiency per se is not testable. It must be tested jointly with some model of equilibrium, an asset-pricing model.” Without such a model, the degree of the market’s efficiency is indeterminate. In Clement ( 2007 ) Fama elaborates: “You can’t test models of market equilibrium without market efficiency because most models of market equilibrium start with the presumption that markets are efficient….Tests of market efficiency are tests of some model of market equilibrium and vice versa. The two are joined at the hip…prices could be random and still be inefficient…what market efficiency says is that the deviation of the realized price from the equilibrium expected value is unpredictable based on any past information.” However, as is expressed throughout the current text, the core problem with this approach is that the nineteenth-century mechanistic notion that markets are ever in “equilibrium,” always moving toward “equilibrium,” or even that “fundamental value ” can be adequately estimated is totally inapplicable to financial markets. See also Radner ( 1968 ) and Fama and Litterman ( 2012 ).
Fox ( 2009 , p. 193) quotes Clive Granger and Oskar Morgenstern, writing in their 1970 book, Predictability of Stock Prices : “The random walk hypothesis does not say that price changes are unpredictable: it says they are not predictable using (linear) combinations of previous price changes.” See also Shleifer ( 2000 ) and Lo ( 2004 ) for historical perspective.
Campbell et al. ( 1997 , p. 34) .
Lo and MacKinlay ( 1999 , p. 5) suggest that , at least early on, many economists implicitly and erroneously linked the random-walk hypothesis and the EMH. It is easy to see how the two ideas might be confused, even though many researchers “have shown in many ways and in many contexts that the random walk hypothesis is neither a necessary nor a sufficient condition for rationally determined security prices.”
On the EMH, as Ross ( 2003 , p. 213) explained, “critics of this hypothesis argue that new information is absorbed by different investors at different rates; thus, past price movements are a reflection of information that has not yet been universally recognized but will affect future prices.” Evidence from statistical analysis of a large base (110,000) of actual discount brokerage trading records by Coval et al. ( 2002 ) suggests further that there are some individual investors who are consistently able on a risk-adjusted basis to beat the market—which is a violation of even a weak form of the EMH. It appears that such traders are better able to exploit market inefficiencies than are large professional money managers.
In my own experience as a professional fundamental analyst, I can point to several instances in which deep company and industry knowledge and research provided significantly large market-beating results, particularly when going against the grain of normally bullish consensus opinion and taking a longer-term view. However, such opportunities do not arise frequently. I also can point to others such as James Chanos, founder of Kynikos Associates, who, for example, brought early attention to the problems at Enron (which lost all value), or William Ackman, head of Pershing Square Capital Management, who identified important weaknesses at mortgage bond insurer MBIA (which fell from around $70–$10, April 2007 to January 2008). Similarly, see Lewis ( 2010 ) The Big Short , on Steven Eisman’s correct predictions on subprime mortgage defaults.
As indicated in Dugan ( 2005 ), Sharpe believes that the ratio is misused. “Past average experience may be a terrible predictor of future performance,” he says.
An offshoot of this approach (known as the Treynor ratio) then for any specified period of time enables the estimation and ranking of the expected excess return for portfolio, p , above the risk-free rate, r f , per unit of the portfolio’s beta , b p , as E (r p − r f )/ b p . The Treynor Ratio is similar to the Sharpe ratio, which instead measures expected excess return per unit of standard deviation.
By the late 1970s, when Wall Street came to embrace the CAPM approach, a new beta - and alpha - measuring industry had been formed in the brokerage community. Importantly, in applications of the CAPM and decisions concerning asset allocations, Markowitz is quoted in Bernstein ( 2007 , p. 159) as saying, “It isn’t the variance you have to worry about, it’s the covariance.”
In a review of the October 2013 Nobel Laureate prize to Robert Shiller , Eugene Fama , and Lars Hansen, Asness and Liew ( 2014 ) explain that the joint hypothesis (see note 1) is that the EMH holds and that the capital asset pricing model (CAPM) is how prices are set. “CAPM says the expected return on any security is proportional to the risk of the security as measured by its market beta . Nothing else should matter…Say you…find evidence against this pairing (as has been found). The problem is, you don’t know which of the two (or both) ideas you are rejecting. EMH may be true, but CAPM may be a poor model of how investors set prices…You can have an efficient market that is hard to beat because of the limits of arbitrage.” See also Montier ( 2002 , pp. 31–6) on instances of failed arbitrage.
The inherent contradiction between different approaches is noted by Fox ( 2009 , p. 149), who observes that in the CAPM “of Treynor , Sharpe , Lintner, and Black , risk could be manipulated and controlled and reduced, but never entirely eliminated – not even in theory.” But in “the no-arbitrage version pioneered by Merton , the right combination of securities could eliminate risk entirely.” The Sharpe ratio’s measurement of returns to risk using price variances is also potentially misleading as the calculation includes upside deviation, which many or most investors consider to be favorable. For most people, risk is actually reflected in the downside deviations. The Sortino ratio, developed by Frank A. Sortino, reflects this bias by calculating the denominator only using downside deviations (i.e., those of negative asset returns).
Quote from Malkiel ( 1999 , p. 235). Chua et al. ( 2009 ) suggest that diversification is a grossly oversimplified concept, showing that “[C]orrelations, as typically measured over the full sample of returns, often belie an asset’s diversification properties in market environments when diversification is most needed.” This is supported in a Barron’s interview in Willoughby ( 2010 ) with Lou Harvey, founder of Dalbar, an investment ratings firm in Boston. Dalbar’s study found “that all of the methods based on modern portfolio theory worked within a certain range. Outside of that range, they all failed.”
From Fabozzi et al. ( 2014 , p. 34): “…although diversification is considered to be a sound probabilistic concept, the benefits of diversification may change as market states change…as correlations and expected returns change. The naïve belief that by simply diversifying a portfolio the investor is protected against major losses is just that – naïve.”
This paraphrases the words of stochastic modeling expert Dr. Peter Carr, currently affiliated with New York University, but it is an offshoot of the important Heston ( 1993 ) and Bates ( 1996 ) model approaches. See also Mandelbrot and Hudson ( 2004 , p. 125).
Bailey ( 2005 , p. 160), for example, says, “the CAPM implies that each asset’s beta -coefficient, β j is a more appropriate measure of risk than its standard deviation of return, σ j .” Greenwald et al. ( 2001 , p. xvii) say that permanent loss of capital is “the more common-sense understanding of risk.” In Baker-Said ( 2008 ), Taleb notes that people mistake low volatility for low risk. Hassett ( 2002 , p. 17) also argues that the traditional way of evaluating risk by equating it with volatility is inherently flawed and incomplete as it makes buying a stock like wagering on a coin flip. If a stock fluctuates a lot in price it is regarded as risky. If the price is stable, then it is not. However, this simplistic notion doesn’t recognize that the shares of new companies trying something completely new may fundamentally alter the economic landscape. Shares of such companies may thus be less risky despite the volatility that derives from the shares being relatively unseasoned. That’s because unlike the coin-flip situation, where there is a 50% probability of heads or tails, “when a firm is trying something completely new…there are no probabilities. Nobody knows for sure what might happen.” The inappropriateness of measuring risk via volatility of share prices would therefore, by extension, be applicable to the market as a whole. In Wallace ( 2010 ), behavioral finance authority Meir Statman says, “risk is not what underlies outperformance…[I]t’s emotion; it is sentiment.” Taylor ( 2010 , p. 3) writes that “Keynes explicitly and emphatically rejected the notion that risk is quantifiable.”
Black et al. ( 1972 ) provided additional evidence that the specifications of the CAPM are faulty. Using four subperiods of 105 months each, the authors found that the slope of the SML was flat for the third-period sample and negative for the fourth-period sample. These results say that returns in the third period were the same regardless of risk and that in the fourth period higher risk provided lower returns , not higher, as the CAPM theory would have predicted (indeed, demanded). With this evidence of instability in the risk/return tradeoff, it becomes more difficult to support the notion that collective investor behavior is always rational (i.e., valuing and pricing securities on the basis of all available information). From the behavioralist’s standpoint that is discussed in the next chapter, Statman ( 1995 ) adds:
The assumptions about human behavior that underlie the CAPM are not simplified versions of observed behavior. Rather, they contradict observed behavior…Standard finance does not do well…as a descriptive theory of finance. Investors regularly overlook arbitrage opportunities, fail to use Markowitz’s principles in constructing their portfolios, and fail to drive stock returns to levels commensurate with the CAPM.
Burton Malkiel , a pioneer in the development of the random-walk/EMH approach, has continued in Malkiel ( 1999 [2003, 2007], pp. 13–15) to endorse the concept that “Investors would be far better off buying and holding an index fund than attempting to buy and sell individual securities or actively managed mutual funds…I conclude that reports of the death of the efficient market theory are vastly exaggerated.” In the eighth edition (2003, p. 258), he says, “I believe the stock market is fundamentally logical.” However, he then explains that “a number of studies by academics and practitioners, completed during the 1980s and 1990s have cast doubts on the validity of the theory.” In the eighth edition (2003) he writes of the weaknesses:
The first is that perfect pricing exists…Another fragile assumption is that news travels instantaneously… Finally, there is the enormous difficulty of translating known information about a stock into an estimate of true value. (pp. 194–5)
Although we still have much to learn about the market’s evaluation of risk, I believe it is fair to conclude that risk is unlikely to be captured adequately by a single beta statistic (the risk measure of the CAPM). It appears that several other systematic risk measures affect the valuation of securities. Unfortunately, beta also has its warts. The actual relationship between beta and rate of return has not corresponded to the relationships predicted in theory during the last third of the twentieth century. Moreover, betas are not stable from period to period, and they are very sensitive to the particular market proxy against which they are measured. (pp. 237–8)
See also eleventh edition (2015).
In Malkiel ( 1999 , pp. 194–5). Gulko ( 2005 ) writes: “Investors do not need to be rational for markets to be efficient…price efficiency and individual irrationality are not necessarily mutually exclusive.”
Malkiel ( 1999 [2003, 2007], pp. 235, 244) says: “Markets can be efficient even if many market participants are quite irrational. Markets can be efficient even if stock prices exhibit greater volatility than can apparently be explained by fundamentals such as earnings and dividends.”
Malkiel (2015, pp. 226–7).
A detailed defense of the random walk/EMH against more recent challenges (e.g., indications of the possibility of partial long-term predictability of returns as based on negative serial correlation studies) is offered in Malkiel ( 2003 ). Rockel ( 2010 ) provides a concise description of the EMH and writes of Markowitz , explaining why MPT is still correct. Kaizoji and Sornette ( 2008 ) provide a review of bubbles and crashes as related to the EMH.
Bailey ( 2005 , p. 144) further condenses these assumptions into three sets of conditions: (1) asset markets are in equilibrium, (2) all investors behave according to a mean -variance criterion, and (3) investors base their decisions on the same values of means, variances, and covariances. And Montier ( 2002 , p. 86) concisely states the CAPM assumptions, among which are no transaction costs, no taxes, size of trading positions acquired not affecting market prices, and investors sharing a common time horizon. Jovanovic and Schinckus ( 2017 , p. 11) note that use of logarithmic returns of stocks rather than of prices provides assurance that the stochastic process is stationary.
Peters ( 1994 , pp. 30, 43–4) also writes that “the term structure of volatility shows that the stock market is not a random walk. At best, it is a stochastic ‘bounded’ set…This means that there are limits to how far the random walker will wander before he or she heads back…This is not unexpected because the EMH and the CAPM are equilibrium models.”
From the perspective of the adaptive market hypothesis of Lo ( 2017 , p. 3), “the EMH isn’t wrong, it’s just incomplete.” Lo’s approach is interesting but flawed and unconvincing, for as Prechter ( 2016 , p. 400) writes: “[W]hile nature produces many successful species, no ‘species’ of speculator learns anything reliable or achieves long-run financial success, so there must be no process of adaptation, natural selection or evolution going on….” This view is supported by the ever-recurring patterns of speculation and bubbles that date back centuries and as discussed in Chaps. 1 , 2 , and 3 . That is, there is absolutely no evidence that via a natural selection process, a new species of asset speculator has evolved. People make the same mistakes and misjudgments again and again.
Wilmott and Orrell ( 2017 , p. 25).
Peters ( 1994 , p. 42).
Peters ( 1989 , 1991 , 1996 ) makes a further case against the EMH through application of what is known as rescaled range analysis, or “range over standard deviation” (R/S), which was originated by H. E. Hurst in the early 1900s. Hurst took the range of the data in a time series, that is, the maximum minus the minimum, and standardized the number by dividing by the standard deviation of the observations, which provides a dimensionless ratio. He found that many natural phenomena, including temperatures, rainfall, and sunspots, follow a “biased” random walk. The simple relationship was
where a is a constant, N is the number of observations, and H is the Hurst exponent.
Peters conducted R/S analyses on many different time series, including those for economic indicators, for Treasury bill yields, for S&P 500 returns over many different intervals, and for individual shares. In almost all situations, the R/S analysis indicated persistence (i.e., autocorrelation and fractal features), which is not consistent with the underlying independence and random-walk assumptions of the EMH. The Hurst exponent was developed for range changes in the water level of the Nile River. A river level is somewhat analogous to a stock price level in that it rises in some years and falls in others, but once in a great while there is a major event, say a 100-year flood, that surprises everyone with its intensity and size and goes far beyond the usual ranges of water-level expectations. This is precisely the type of action seen in financial asset price bubbles and crashes, in which the usual expected price ranges and growth trends are far exceeded . Buchanan ( 2013 , pp. 162–165) provides a clear discussion of the Hurst method.
Some reservations against use of R/S analysis are to be found in Lo and MacKinlay ( 1999 , p. 148) and also in the earlier work of Mandelbrot ( 1972 ). See Greene and Fielitz ( 1977 ), Campbell et al. ( 1997 , p. 63), Lobato and Savin ( 1998 ), and Lo and MacKinlay ( 1999 , p. 166) for criticisms of R/S analysis. Related material also includes Poterba and Summers ( 1988 ), Lo ( 1991 ), Mills ( 1993 , p. 8), and Mantegna and Stanley ( 2000 , p. 45).
For example , Fama and French ( 1996 ) posited a three-factor (FF3 ) model as an extension of the original CAPM approach. In addition to the excess market return of the standard CAPM, the FF3 multifactor approach adds the return on small stocks minus the return on big stocks (SMB), and the return on value stocks minus the return on growth stocks (HML, high minus low book/market). “Value” stocks have relatively high ratios of book value to market cap (>70th percentile); “growth” stocks are in the lowest (<30th percentile). Although Fama and French develop factor portfolios using firm characteristics, Chen et al. ( 1986 ) specify macroeconomic variables as factors. Estrada ( 2005 , Ch. 8) provides an overview of the three-factor model approach. In the April 2010 GMO Quarterly Letter ( www.GMO.com ), Jeremy Grantham takes issue with the Fama and French as well as the Graham and Dodd approach to securities analysis. See also Zwieg (2018).
Turner and Weigel ( 1990 ) also cast doubt upon the assumption that returns follow a normal distribution, N (0, σ 2 ), with mean zero and variance σ 2 . In fact, more observations than in the normal were observed by these studies to be on the negative left-hand side of the distribution, and the peak around the mean was higher than would be predicted by a normal distribution, a condition called leptokurtosis. Such a condition may be what it seems, but it may also be caused by changes in the distribution over time . Friedman and Laibson ( 1989 ), who studied quarterly S&P 500 returns from 1946 through 1988, however, concluded that significant leptokurtosis “appears regardless of the period chosen.” And Hall ( 2001 , p. 2) says, “Most suggestions of irrationality appear to deal with mistakes in probability rather than mistakes in marginal utility…rational beliefs about probabilities are only loosely constrained in a nonstationary world.”
Arbitrage pricing theory (APT), which was developed by Ross ( 1976 ), takes a somewhat different approach. As Campbell et al. ( 1997 , p. 156) explain, the CAPM “is an equilibrium theory where the expected return of a given asset is a linear function of its covariance with the return of the market portfolio…APT …is an asset pricing theory where in the absence of asymptotic arbitrage the expected return of a given asset is determined by its covariances with multiple factors.” Ross is quoted in Dunbar ( 2000 , p. 71) as saying, “Whatever the systematic risks in the market, arbitrage will force excess expected returns to be proportional to the exposure to those risks.”
Cuthbertson and Nitzsche ( 2004 , p. 181) indicate that “APT implies that the return on a security can be broken down into an expected return and an unexpected, ‘surprise’ or ‘news’ component .” Armitage ( 2005 , p. 53) adds that APT shows “relations between expected returns that result from an absence of arbitrage opportunities, rather than from the maximisation of investor utility.” This makes the APT approach more general than, but still consistent with that of the CAPM.
Fama and French ( 1992 ) marked a turning point in the theoretical and practical viability of the CAPM in suggesting that there is no link between risk as measured by volatility and return. Subsequently , Fama and French ( 2015 ) introduced a five-factor model that tries to explain stock returns by adding factors of profitability and investment to the original three factors. But the new model still doesn’t fully account for the better performance of the small caps. Thaler ( 2015 , p. 229) observes that “it is difficult to tell a plausible story in which highly profitable firms are riskier than firms losing money.”
For instance, mortgage-backed securities indices had not been notably volatile just prior to the collapse that began in late 2006, but that didn’t prevent them from soon thereafter falling by more than half. And in the cases of Bear Stearns and Lehman , balance sheet leverage at greater than 30 to 1 meant that only a 3% or so decrease in valuation of the securities on the balance sheet was sufficient to totally wipe out equity.
Follows Bookstaber ( 2017 , p. 16).
Campbell et al. ( 1997 , p. 283) similarly say “that the stock market is too volatile to be consistent with the view that stock prices are optimal forecasts of future dividends discounted at a constant rate.”
Such “excess volatility” was also found in British stock prices from 1870 to 1990 and in the German stock market, 1876–1990. See De Long and Grossman ( 1993 ) who say that for the British market, “the strongest evidence against the simple constant required rate of return dividend-discount model is found in the pre-World War I experience.” See De Long and Becht ( 1992 ) on the German market.
Bernstein ( 2007 , p. 70). In Christensen and Prabhala ( 1998 ) the relation between implied and realized volatility is analyzed and it is found that “implied volatility outperforms past volatility in forecasting….”
LeRoy and Parke ( 1992 ) make a correction to Shiller’s 1981 paper. They note that some data transformation that ensures that the appropriate variables are stationary must be found, but that Shiller’s fitted log-linear trend method will still be nonstationary . A critique is also provided by Smant ( 2003 ), who cites the work on variance-bound tests done by Kleidon ( 1986 ). Smant suggests that Shiller’s measurements of variance are not significant to the behavior of stock market prices because variance-bound tests must be applied to dividends and prices in a cross-sectional framework, not a time-series variance framework, as Shiller has done. That is because the expected probability distribution of future dividends changes over time and is conditional on the information set available at each subsequent time, t . But even if Smant’s view is accepted, Shiller’s concern that bubbles create social welfare, savings and investment, and policy distortions nevertheless remains valid. A review of policy implications appears in Hunter et al. ( 2003 [2005]).
All of this is consistent with the Gopikrishnan et al. ( 1998 ) study of 40 million stock trading data points in which an asymptotic power-law behavior for the cumulative distribution found. See also Mantegna ( 1991 ), Cont et al. ( 1997 ), Scalas and Kim ( 2006 ), and Gerow and Keane ( 2012 ).
Mantegna and Stanley ( 2000 , p. 33) have observed that “[S]table non-Gaussian random processes have infinite variance , whereas the Gaussian process is the only stable process with finite variance.” Taylor ( 2005 , pp. 73–6) notes that the infinite -variance stable distribution advocated by Mandelbrot is used by few researchers, with most studies based on variations of the normal that sometimes include gamma and lognormal versions. The reason is that such variations have the high kurtosis and approximate symmetry that appears to characterize the distribution of daily returns . Hagerman ( 1978 ), for example, argues that monthly returns distributions are nearly normal.
However, the underlying data cover all types of market conditions and are not specific as to periods that might contain “bubbles” and “crashes.” And near-normal distributions are not likely to reveal the power-law fractal nature of stock price patterns that are evident from visual inspection. That is, the characteristics of price charts taken over a period of five minutes are not readily distinguishable from those of charts taken over five months if time-scale information, as shown in Fig. 6.3 , is not separately revealed.
Also, see Ané and Geman ( 2000 ), in which it is shown that normality of asset returns can be recovered through a stochastic time change. An example of “tail risk” given by Mandelbrot is that if the DJIA followed a normal distribution, it should have moved by more than 7% only once in every 300,000 years, yet it did so 48 times in the twentieth century. See also Orlowski ( 2012 ) and “In Plato’s Cave,” The Economist , January 22, 2009.
Critics such as in Campbell et al. ( 1997 , p. 19) have for example, argued that such stable distributions
imply that sample estimates of the variance and higher moments of returns will tend to increase as the sample size increases, whereas in practice these estimates seem to converge. Second, they imply that long -horizon returns will be just as non-normal as short -horizon returns…In practice the evidence for non-normality is much weaker for long -horizon returns than for short -horizon returns.
See also Weatherall ( 2013 , p. 124).
Mantegna and Stanley ( 2000 , p. 60) and Jovanovic and Schinckus ( 2017 , p. 1) write : “Financial economists’ insistence on maintaining the Gaussian distribution hypothesis meets with incomprehension among econophysicists.”
These are generally based on the autoregressive conditional heteroskedastic (ARCH) techniques that were originated by Engle ( 1982 ). Such models imply unevenly spread regression error terms, ε τ , , and see market volatility as being conditional upon previous levels. Note that Conrad and Kaul ( 1988 ) found “variation through time in expected returns is well characterized by a stationary first-order autoregressive process…the degree of variation in expected returns also changes systematically over time.”
From Mauboussin ( 2006 , p. 39): “extreme days (up and down) come in bunches.” Also, Vandewalle et al. ( 1999 ) find a tendency for volatility to cluster around crashes. This clustering tendency is implemented in the WABSI and WACSI measures described in Chap. 10 . Tsay ( 2005 , p. 99) writes that “volatility evolves over time in a continuous manner – that is, volatility jumps are rare…volatility does not diverge to infinity.” However, especially for 2008–2009, Figs. 8.5 and 10.4 visually contradict this view.
Studies such as by Rahman and Saadi ( 2008 ) and Cunningham ( 1993 ) indicate that the random-walk hypothesis cannot be confirmed through use of unit root tests for efficient markets because such tests (e.g., Samuelson-Fama and augmented Dickey-Fuller) are unable to “discriminate between a fully deterministic times series , generated from a nonlinear (chaotic) process, and a random walk.”
Mills ( 1993 , pp. 139–41) explains that although generalized ARCH (GARCH ) models have proven to be a popular method of modeling volatility in financial markets, they have limitations: GARCH models assume that only the magnitude and not the sign of unanticipated returns determines volatility and suggest different statistical properties for different time horizons, therefore failing, as Mantegna and Stanley ( 2000 , p. 87) note , “to describe properly the scaling properties of pdfs at different time horizons.” Ridley ( 1993 ) has further observed about GARCH, “All it means is that volatility is clustered. Prices tend to be volatile when they have just been volatile and not when they have not.”
Poterba and Summers ( 1986 ) suggested that shocks to stock market volatility do not persist for long periods, having half-lives of less than six months. French et al. ( 1987 ) thereafter indicated that the expected market risk premium is positively related to the predictable volatility of stock returns. Investigation by Kim et al. ( 2004 ) also found a positive relationship between volatility and the equity premium.
Adrian and Rosenberg ( 2005 ) developed a two-component (ICAPM framework) process for asset pricing purposes that allow short-lived volatility shocks (e.g., transitory liquidity events) to have different risk premiums than long-lived shocks to volatility (e.g., changes in economic outlook). They demonstrated that within an ICAPM framework there is strong cross-sectional evidence for the pricing of volatility of risk. And Schwert ( 1989a ) observed that “[E]stimates of the standard deviation of monthly stock returns vary from two to twenty percent a month during the 1857-1987 period. Tests for whether differences this large could be attributable to estimation error strongly reject the hypothesis of constant variance.”
Todorov and Tauchen ( 2010 ) found evidence for this. Todorov ( 2010 ) examines temporal variation in the variance risk premium and looks at jumps in stock prices and in volatility. See also Cartea and Karyampas ( 2009 ).
Advanced work on understanding volatility is centered at New York University’s V-Lab ( vlab.stern.nyu.edu ), headed by Nobel Laureate Robert F. Engle . Cuthbertson and Nitzsche ( 2004 ) summarize : “{E}mpirically, the evidence for a link between stock market volatility and expected returns is not overly strong (p. 252)…Stock prices appear to be excessively volatile even when we allow time-varying discount rates and dividend forecasts (p. 295).” Munk ( 2013 , pp. 14–6) reviews findings that stock volatility tends to be negatively correlated with returns, so that high volatility appears in periods of low returns and vice versa. In addition, while daily returns on market indexes tend to be negatively skewed, daily returns on individual stocks are roughly symmetric with zero skewness, stock dividends have different statistical properties than those of stock returns, and portfolio returns over short horizons exhibit positive autocorrelation (i.e., momentum). In the long run, however, particularly for individual stocks more than for broad indices, there is mean reversion, with past returns tending to negatively predict returns over longer periods . Adrian and Rosenberg ( 2005 ) explain that “[M]ost studies…do not find a consistent significant relationship between risk and return…it is puzzling why the risk-return tradeoff is so difficult to detect empirically.”
The average volatility for the S&P 500 for 2012 to 2016 was under 13% as compared to the annualized daily returns volatility of around 19% from 1930 to 2016. Table 5.1 shows that poor performance and high volatility tend to be followed by strong performance and low volatility. This, however, does not contradict the presentation of Fig. P 1 which displays six-month rolling data, and not the long-term cyclical data shown here. See also Mackintosh (2018).
The Merrill Lynch Option Volatility Estimate (MOVE) Index does for bonds what the VIX does for stocks.
See “In the Shadows of Black Monday” by Christopher Cole of Artemis Capital Management in Zero Hedge , October 21, 2017.
This was seen when the DJIA lost 7% in two days, one of which (5 February 2018) saw the largest-ever one-day point drop of 1175.21 points. The S&P 500 fell 7.79% from 2872.87 on 26 January to 2648.94 on 5 February. Meanwhile, the VIX streaked from around 11 to 50 as the heavily-traded XIV inverse ETF collapsed to near zero. Grant’s Interest Rate Observer, May 5, 2017, called such instruments portfolio insurance of the twenty-first century.
Cootner ( 1962 ) first linked the random-walk model to information and economic equilibrium, thereby setting the stage for the EMH.
Wilmott and Orrell ( 2017 , p. 12).
Skidelsky ( 2009 , p. 85). For insurance-type markets, risks can be probabilistically reflected from historical data. But the irreducible uncertainty of financial markets can never allow for such calculations.
Redleaf and Vigilante ( 2010 , p. 73) say “[I]nvestors are paid for being right, not for the possibility of being wrong.” See also Redleaf and Vigilante ( 2010 , p. 55). Cooper ( 2008 ) emphasizes the weakness of the assumption that markets move toward an optimal state of equilibrium. Keynes and Minsky , who espoused a no-equilibrium theory, are cited as predecessors. It is noted that use of Gaussian returns distributions and the EMH approach leads to a situation in which assets will appear to be riskier after a steep decline, whereas they are, of course, riskier prior to the decline’s occurrence. Lo ( 2012 ) writes , “During periods of market dislocation – when fear rules the day – investors will reduce their holdings of risky assets and move into safer investments. This will have the effect of reducing the average return on risky assets and increasing the average return on safer ones, exactly the opposite of what rational finance predicts.”
Malkiel in a Bloomberg’s Masters in Business interview with Barry Ritholtz, May 28, 2016, said that markets are efficient in the sense that new information is quickly reflected in stock prices. In Malkiel (2015, p. 108) he writes, “Markets can be highly efficient even if they make errors…Markets are not always or even usually correct.”
Paraphrase of Bernstein ( 2004 , p. 50).
See also Jensen ( 1968 ) and Chan and Lakonishok ( 2004 ), in which it was shown that US mutual fund managers consistently underperformed the S&P 500 (i.e., they were unable to systematically generate abnormal returns). The coefficient has come to be known as “Jensen’s alpha .” Strasburg and Pulliam ( 2011 ) discuss hedge fund herding. The increasing scarcity of alpha is described in Segal ( 2013 ).
Perhaps most damning, however, is the view expressed in Mandelbrot and Hudson ( 2004 , p. 237):
Continuity is a fundamental assumption of conventional finance. The mathematics of Bachelier, Markowitz , Sharpe , and Black-Scholes all assume continuous change from one price to the next. Without that their formulae simply do not work…Alas, the assumption is false and so the math is wrong. Financial prices certainly jump, skip, and leap – up and down.
Although Bernstein ( 2005 ) views the EMH/CAPM framework and the Black -Scholes option pricing model as remarkable advances in the way we now understand capital risks and allocation strategies, Triana ( 2009 ) and Haug and Taleb ( 2009 ) have much frostier views.
Thaler ( 2016 ) writes that “[I]t is an error because…the path is also not likely to be the result of maximizing some well-formed objective function…” Lowenstein ( 2000 , p. 74) attributes the Shiller quote to Summers , but a check of original source Donnelly ( 1987 ) provides the proper attribution as shown . Shiller ( 1992 , p. 2) contains the same thought. And Buiter ( 2009 ) follows similarly, writing with regard to the crash episode that began in 2007, “The EMH is surely the most notable empirical fatality of the financial crisis. By implication, the complete markets macroeconomics of Lucas, Woodford et al. is the most prominent theoretical fatality. The future surely belongs to behavioural approaches….Confusing the equilibrium of a decentralized market economy, competitive or otherwise, with the outcome of a mathematical programming exercise should no longer be acceptable.” See also The Economist , July 16, 2009, in which “What Went Wrong with Economics” is surveyed in three articles.
In Gray and Vogel ( 2016 , p. 65). (No relation.)
This was later followed by the suggestion in Fama and French ( 2008 ) that momentum investing is an embarrassment to the EMH and that momentum is the “premier anomaly .”
Mehrling ( 2005 , p. 91) writes , “…Fama’s allegiance was always more to efficient markets than to any particular theory of expected return. In time, he would give up CAPM to save efficient markets even as his erstwhile colleague Fischer Black would do the opposite.” Fox ( 2009 ) reviews the history of efficient markets and the rational expectations approach. See also Clement ( 2007 ) in which Fama says “The word ‘bubble’ drives me nuts” and explains that the fatal problem in the CAPM is its reliance on beta (volatility of an individual stock relative to overall market volatility). In Silver ( 2012 , p. 347) Fama says with regard to bubbles that “the term has lost its meaning…A bubble is something that has a predictable ending. If you can’t tell you’re in a bubble, it’s not a bubble.” After sharing the 2013 Nobel award with Robert J. Shiller and Lars Peter Hansen, Fama again in an interview (Sommer 2013 ) says that he doesn’t know what a bubble is. And in Appelbaum ( 2013 ), he says that it is impossible to consistently identify asset bubbles before a collapse.
Fabozzi et al. ( 2014 , pp. 7–10).
On probabilistic predictions, Fischer Black in Black ( 1986 ) wrote “Noise makes it very difficult to test either practical or academic theories about the way that financial or economic markets work. We are forced to act largely in the dark.”
See also Lo and Merton ( 2009 ) and Fabozzi et al. ( 2014 ).
Adrian, T., & Rosenberg, J. (2005, 2008). Stock Returns and Volatility: Pricing the Long-Run and Short-Run Components of Market Risk. Federal Reserve Bank of New York and Journal of Finance, 63 (6), 2997–3030.
Google Scholar
Ané, T., & Geman, H. (2000). Order Flow, Transaction Clock, and Normality of Asset Returns. Journal of Finance, 55 (5), 2259–2284.
Appelbaum, B. (2013, October 15). Economists Clash on Theory, but Will Still Share the Nobel. New York Times .
Armitage, S. (2005). The Cost of Capital: Intermediate Theory . New York: Cambridge University Press.
Asness, C., & Liew, J. (2014, March). The Great Divide . Institutional Investor .
Bailey, R. E. (2005). The Economics of Financial Markets . Cambridge: Cambridge University Press.
Bak, P. (1996). How Nature Works: The Science of Self-Organized Criticality . New York: Springer-Verlag.
Baker-Said, S. (2008, May). Flight of the Black Swan. Bloomberg Markets .
Bates, D. S. (1996). Jumps and Stochastic Volatility: Exchange Rate Processes Implicit in Deutsche Mark Options. Review of Financial Studies, 9 (1), 69–108.
Beechey, M., Gruen, D., & Vickery, J. (2000). The Efficient Market Hypothesis: A Survey (Research Discussion Paper, 2000-01). Federal Reserve Bank of Australia.
Bernstein, P. L. (2004). What’s It All About, Alpha? Institutional Investor .
Bernstein, P. L. (2005a). Capital Ideas: From the Past to the Future. Financial Analysts Journal, 61 (6), 55–59.
Bernstein, P. L. (2007). Capital Ideas Evolving . Hoboken: Wiley.
Black, F. (1986). Noise. Journal of Finance, 41 (3), 529–534.
Black, F., Jensen, M. C., & Scholes, M. (1972). The Capital Asset Pricing Model: Some Empirical Tests. In M. C. Jensen (Ed.), Studies in the Theory of Capital Markets . New York: Praeger.
Bookstaber, R. (2017). The End of Theory: Financial Crises, the Failure of Economics, and the Sweep of Human Interaction . Princeton: Princeton University Press.
Buchanan, M. (2013). Forecast: What Physics, Meteorology, and the Natural Sciences Can Teach Us About Economics . New York: Bloomsbury.
Buiter, W. H. (2009, March 3). The Unfortunate Uselessness of Most ‘State of the Art’ Academic Monetary Economics. Financial Times .
Burton, J. (1998). Revisiting the Capital Asset Pricing Model. Dow Jones Asset Manager . http://www.stanford.edu/~wfsharpe/art/djam/djam.htm
Campbell, J. Y., Lo, A. W., & MacKinlay, A. C. (1997). The Econometrics of Financial Markets . Princeton: Princeton University Press.
Cartea, A., & Karyampas, D. (2009). The Relationship Between the Volatility of Returns and the Number of Jumps in Financial Markets. Birkbeck Working Paper 0914, Birkbeck, University of London. Available at http://www.ems.bbk.ac.uk/research/wp/PDF/BWPEF0914.pdf
Chan, L., & Lakonishok, J. (2004). Value and Growth Investing: Review and Update. Financial Analysts Journal, 60 (1), 71–86.
Chen, N., Roll, R., & Ross, S. (1986). Economic Forces and the Stock Market. Journal of Business, 59 (3), 383–403.
Christensen, B. J., & Prabhala, N. R. (1998). The Relation Between Implied and Realized Volatility. Journal of Financial Economics, 50 , 125.
Chua, D. B., Kritzman, M., & Page, S. (2009). The Myth of Diversification. Journal of Portfolio Management, 36 (1), 26–35.
Clement, D. (2007). Interview with Eugene Fama. The Region , Federal Reserve Bank of Minneapolis. Available at www.minneapolisfed.org/publications_papers/pub_display.cfm?id=1134
Conrad, J., & Kaul, G. (1988, October). Time-Variation in Expected Returns. Journal of Business, 61 (4), 409–425.
Cont, R., Potters, M., & Bouchaud, J-P. (1997). Scaling in Stock Market Data: Stable Laws and Beyond. Available at http://arxiv.org/pdf/cond-mat/9705087.pdf
Cooper, G. (2008). The Origin of Financial Crises: Central Banks, Credit Bubbles, and the Efficient Market Fallacy . New York/Petersfield: Vintage Paperback/Harriman House.
Cootner, P. H. (1962). Stock Prices: Random vs Systematic Changes. Industrial Management Review, 3 (2), 24–45.
Coval, J. D., Hirshleifer, D. A., & Shumway, T. G. (2002). Can Individual Investors Beat the Market? Harvard NOM Working Paper, No. 02-45. Cambridge, MA: Harvard University.
Cunningham, S. R. (1993, Fall). Unit Root Testing: A Critique from Chaos Theory. Review of Financial Economics, 3 (1), 1–18.
Cuthbertson, K., & Nitzsche, D. (2004). Quantitative Financial Economics (2nd ed.). Chichester: Wiley.
De Long, J. B., & Becht, M. (1992). ‘Excess Volatility’ and the German Stock Market, 1876–1990 . NBER Working Paper No. 4054. Available at www.j-bradford-delong.net/pdf_files/German_Volatility.pdf
De Long, J. B., & Grossman, R. S. (1993). ‘Excess Volatility’ on the London Stock Market, 1870–1990. Working Paper, Department of Economics, Harvard University. Available at www.j-bradford-delong.net/pdf_files/London_Volatility.pdf
Donnelly, B. (1987, October 23). Efficient-Market Theorists Are Puzzled by Recent Gyrations in Stock Market. Wall Street Journal .
Dugan, I. J. (2005, August 31). Sharpe Point: Risk Gauge Is Misused. Wall Street Journal .
Dunbar, N. (2000). Inventing Money: The Story of Long-Term Capital Management and the Legends Behind It . Chichester: Wiley.
Engle, R. F. (1982). Autoregressive Conditional Heteroscedasticity with Estimates of the Variance of U.K. Inflation. Econometrica, 50 , 987–1008.
Estrada, J. (2005). Finance in a Nutshell . London: Pearson/FT Prentice Hall.
Fabozzi, F. J., Focardi, S. M., & Jonas, C. (2014). Investment Management: A Science to Teach or an Art to Learn? Research Foundation Review 2015 . CFA Institute Research Foundation at www.CFApubs.org/doi/pdf/10.2470
Fama, E. F. (1970, May). Efficient Capital Markets: A Review of Theory and Empirical Work. Journal of Finance, 25 (2), 383–417.
Fama, E. F. (1991). Efficient Capital Markets: II. Journal of Finance, XLVI (5), 1575–1617.
Fama, E. F., & French, K. (1992, June). The Cross-Section of Expected Stock Returns. Journal of Finance, 47 (2), 427–465.
Fama, E. F., & French, K. (1996). Multifactor Explanations of Asset Pricing Anomalies. Journal of Finance, 51 (1), 55.
Fama, E. F., & French, K. (2008). Dissecting Anomalies. Journal of Finance, 63 (4), 1653–1678.
Fama, E. F., & French, K. (2015). A Five-Factor Asset Pricing Model. Journal of Financial Economics, 116 (1), 1–22.
Fama, E. F., & Litterman, R. (2012, November/December). An Experienced View on Markets and Investing. Financial Analysts Journal, 68 (6), 1–5.
Fox, J. (2009). The Myth of the Rational Market: A History of Risk, Reward, and Delusion on Wall Street . New York: HarperCollins.
French, K. R., Schwert, G. W., & Stambaugh, R. F. (1987). Expected Stock Returns and Volatility. Journal of Financial Economics, 19 , 3–29.
Friedman, B. M., & Laibson, D. I. (1989). Economic Implications of Extraordinary Movements in Stock Prices. Brookings Papers on Economic Activity. Washington, DC: Brookings Institute.
Gerow, A., & Keane, M. T. (2012). Mining the Web for the ‘Voice of the Herd’ to Track Stock Market Bubbles. Available at http://arxiv.org/abs/1212.2676v1
Gopikrishnan, P., Meyer, M., Amaral, L. A. N., & Stanley, H. E. (1998). Inverse Cubic Law for the Distribution of Stock Price Variations. The European Physical Journal, 3 , 139–140.
Gray, W. R., & Vogel, J. R. (2016). Quantitative Momentum . Hoboken: Wiley.
Greene, M., & Fielitz, B. (1977). Long-Term Dependence in Common Stock Returns. Journal of Financial Economics, 4 , 339–349.
Greenwald, B. C. N., Kahn, J., Sonkin, P. D., & van Biema, M. (2001). Value Investing: From Graham to Buffett and Beyond . New York: Wiley (Paperback edition).
Gulko, L. (2005). Efficient Irrational Markets. Journal of Portfolio Management, 31 (2), 64–72.
Hagerman, R. L. (1978). More Evidence on the Distribution of Security Returns. Journal of Finance, 33 (4), 1213–1221.
Hall, R. E. (2001). Struggling to Understand the Stock Market. American Economic Review, 91 (2), 1–11.
Hassett, K. A. (2002). Bubbleology: The New Science of Stock Market Winners and Losers . New York: Crown.
Haug, E. S., & Taleb, N. N. (2009, February 26). Why We Have Never Used the Black-Scholes-Merton Option Pricing Formula (Fifth Version). Available at SSRN: http://ssrn.com/abstract=1012075
Heston, S. L. (1993). A Closed-Form Solution for Options with Stochastic Volatility with Applications to Bond and Currency Options. Review of Financial Studies, 6 (2), 327–343.
Hunter, W. C., Kaufman, G. G., & Pomerleano, M. (Eds.). (2003). Asset Price Bubbles: The Implications for Monetary, Regulatory, and International Policies . Cambridge, MA: MIT Press. Paperback edition, 2005.
Jensen, M. C. (1968). The Performance of Mutual Funds in the Period 1945–1964. Journal of Finance, 23 (2), 389–416.
Jensen, M. C. (1972). Studies in the Theory of Capital Markets . New York: Praeger.
Jensen, M. C. (1978). Some Anomalous Evidence Regarding Market Efficiency. Journal of Financial Economics, 6 (2–3), 95–101.
Jovanovic, F., & Schinckus, C. (2017). Econophysics and Financial Economics: An Emerging Dialog . New York: Oxford University Press.
Kaizoji, T., & Sornette, D. (2008). Market Bubbles and Crashes. MPRA Paper 15204, University Library Munich, Germany and Available at http://arxiv.org/pdf/0812.2449
Kim, C.-J., Morley, J. C., & Nelson, C. R. (2004). Is There a Positive Relationship Between Stock Market Volatility and the Equity Premium? Journal of Money, Credit and Banking, 36 (3), 339–360.
Kleidon, A. W. (1986, October). Variance Bounds Tests and Stock Price Valuation Models. Journal of Political Economy, 94 , 953–1001.
LeRoy, S. F., & Parke, W. R. (1992, September). Stock Price Volatility: Tests Based on the Geometric Random Walk. American Economic Review, 82 , 981–992.
Lewis, M. (2010). The Big Short: Inside the Doomsday Machine . New York: W. W. Norton.
Lintner, J. (1965). The Valuation of Risk Assets and the Selection of Risk Investments in Stock Portfolios and Capital Budgets. Review of Economic Statistics, 47 , 13–37.
Lo, A. W. (1991). Long-Term Memory in Stock Market Prices. Econometrica, 59 , 1279–1313.
Lo, A. W. (2004). The Adaptive Markets Hypothesis. Journal of Portfolio Management, 30 (5), 15–29.
Lo, A. W. (2012). Adaptive Markets and the New World Order. Financial Analysts Journal, 68 (2), 18–29.
Lo, A. W. (2017). Adaptive Markets: Financial Evolution at the Speed of Thought . Princeton: Princeton University Press.
Lo, A. W., & MacKinlay, A. C. (1999). A Non-random Walk Down Wall Street . Princeton: Princeton University Press.
Lo, A. W., & Merton, R. C. (2009). Preface to the Annual Review of Financial Economics. Annual Review of Financial Economics, 1 , 01–17. Palo Alto: Annual Reviews.
Lobato, I. N., & Savin, N. E. (1998, July). Real and Spurious Long Memory Properties of Stock Market Data. The Journal of Business and Economic Statistics, 16 , 261–268.
Lorie, J. H., & Hamilton, M. T. (1973). The Stock Market: Theories and Evidence . Homewood: Richard D. Irwin.
Lowenstein, R. (2000). When Genius Failed: The Rise and Fall of Long-Term Capital Management . New York: Random House.
Malkiel, B. G. (1999). A Random Walk Down Wall Street (7th ed., 8th ed. (2003), 9th ed. (2007), 11th ed. (2015)). New York: W.W. Norton.
Malkiel, B. G. (2003). The Efficient Market Hypothesis and Its Critics. Journal of Economic Perspectives, 17 (1), 59–82.
Mandelbrot, B. (1972). Statistical Methodology for Non-periodic Cycles: From Covariance to R/S Analysis. Annals of Economic and Social Measurement, 1 , 259–290.
Mandelbrot, B., & Hudson, R. L. (2004). The (Mis)Behavior of Markets: A Fractal View of Risk, Ruin, and Reward . New York: Basic Books.
Mantegna, R. N. (1991). Lévy Walks and Enhanced Diffusion in Milan Stock Exchange. Physica A, 179 (2), 232–242.
Mantegna, R. N., & Stanley, H. E. (2000). An Introduction to Econophysics: Correlations and Complexity in Finance . Cambridge: Cambridge University Press.
Markowitz, H. M. (1952). Portfolio Selection. Journal of Finance, 7 , 77–91.
Mauboussin, M. J. (2006). More Than You Know: Finding Financial Wisdom in Unconventional Places. New York: Columbia University Press.
Mehrling, P. (2005). Fischer Black and the Revolutionary Idea of Finance . Hoboken: Wiley.
Merton, R. C. (1992). Continuous-Time Finance (Rev. ed.). Oxford: Blackwell.
Mills, T. C. (1993). The Econometric Modelling of Financial Time Series . Cambridge: Cambridge University Press.
Modigliani, F., & Pogue, G. A. (1974, March/April). An Introduction to Risk and Return. Financial Analysts Journal , 30 (2), 68–80.
Montier, J. (2002). Behavioural Finance: Insights into Irrational Minds and Markets . Chichester/Hoboken: Wiley.
Mood, A. (1940). The Distribution Theory of Runs. Annals of Mathematical Statistics, 11 , 367–392.
Mossin, J. (1966). Equilibrium in a Capital Asset Market. Econometrica, 34 , 768–783.
Munk, C. (2013). Financial Asset Pricing Theory . Oxford: Oxford University Press.
Orlowski, L. T. (2012). Financial Crisis and Extreme Market Risks: Evidence from Europe. Review of Financial Economics, 21 (3), 120–130.
Peters, E. E. (1989, July/August). Fractal Structure in the Capital Markets. Financial Analysts Journal, 45 (4), 32–37.
Peters, E. E. (1991). A Chaotic Attractor for the S&P 500. Financial Analysts Journal, 47 (2), 55–81.
Peters, E. E. (1994). Fractal Market Analysis: Applying Chaos Theory to Investment and Economics . New York: Wiley.
Peters, E. E. (1996). Chaos and Order in the Capital Markets: A New View of Cycles, Prices, and Market Volatility (2nd ed.). New York: Wiley.
Poterba, J., & Summers, L. H. (1986). The Persistence of Volatility and Stock Market Fluctuations. American Economic Review, 76 .
Poterba, J., & Summers, L. H. (1988). Mean Reversion in Stock Returns: Evidence and Implications. Journal of Financial Economics, 22 (1), 27–59.
Prechter, R. R., Jr. (2016). The Socionomic Theory of Finance . Gainesville: Socionomics Institute Press.
Radner, R. (1968, January). Competitive Equilibrium Under Uncertainty. Econometrica, 36 (1), 31–58.
Rahman, A., & Saadi, S. (2008). Random Walk and Breaking Trend in Financial Series: An Econometric Critique of Unit Root Tests. Review of Financial Economics, 17 (3), 204–212.
Redleaf, A., & Vigilante, R. (2010). Panic: The Betrayal of Capitalism by Wall Street and Washington . Minneapolis: Richard Vigilante Books.
Ridley, M. (1993, October 9). Frontiers of Finance: On the Edge. The Economist .
Rockel, N. (2010, May). Evolutionary Road: The Enduring Popularity of MPT Hasn’t Stopped Practitioners from Trying to Improve It. Institutional Investor .
Ross, S. M. (1976). The Arbitrage Theory of Capital Asset Pricing. Journal of Economic Theory, 13 , 341–360.
Ross, S. M. (2003). An Elementary Introduction to Mathematical Finance, Options and Other Topics (2nd ed.). New York/Cambridge: Cambridge University Press.
Scalas, E., & Kim, K. (2006). The Art of Fitting financial Time Series with Lévy Stable Distributions. MPRA Paper No. 336 Available at http://mpra.ub.uni-muenchen.de/336/
Schwert, G. W. (1989a, May). Business Cycles, Financial Crises, and Stock Volatility. Working Paper No. 2957, NBER.
Segal, J. (2013, September). Is Alpha Dead? Institutional Investor .
Sharpe, W. F. (1964). Capital Asset Prices: A Theory of Market Equilibrium Under Conditions of Risk. Journal of Finance, 19 , 425–442.
Shiller, R. J. (1992). Market Volatility . Cambridge, MA: MIT Press.
Shiller, R. J. (2000, 2005, 2015). Irrational Exuberance . Princeton: Princeton University Press (3rd ed, 2015).
Shleifer, A. (2000). Inefficient Markets: An Introduction to Behavioral Finance . Oxford: Oxford University Press.
Silver, N. (2012). The Signal and the Noise: Why So Many Predictions Fail – But Some Don’t . New York: Penguin.
Skidelsky, R. (2009). Keynes: The Return of the Master . New York: Public Affairs (Perseus).
Smant, D. J. C. (2003). The Variance-Bound Fallacy. Rotterdam: Erasmus University, Rotterdam School of Economics. Available at www.few.eur.nl/few/people/smant
Sommer, J. (2013, October 27). Eugene Fama, King of Predictable Markets. New York Times .
Statman, M. (1995). Behavioral Finance Versus Standard Finance. In Behavioral Finance and Decision Theory in Investment Management . Charlottesville: Association for Investment Management and Research.
Strasburg, J., & Pulliam, S. (2011, January 14). Hedge Funds’ Pack Behavior Magnifies Swings in Market. Wall Street Journal .
Summers, L. H. (1986). Does the Stock Market Rationally Reflect Fundamental Values? Journal of Finance, 41 (3), 591–602.
Taylor, S. (2005). Asset Price Dynamics, Volatility, and Prediction . Princeton: Princeton University Press.
Taylor, L. (2010). Maynard’s Revenge: The Collapse of Free Market Macroeconomics . Cambridge, MA: Harvard University Press.
Thaler, R. H. (2015). Misbehaving: The Making of Behavioral Economics . New York: W.W. Norton.
Thaler, R. H. (2016, July). Behavioral Economics: Past, Present, and Future. American Economic Review, 106 (7), 1577–1600.
Tobin, J. (1958, February). Liquidity Preference as Behavior Toward Risk. Review of Economic Studies, 25 (67), 65–86.
Todorov, V. (2010). Variance Risk Premium Dynamics: The Role of Jumps. Review of Financial Studies, 23 (1), 345–383.
Todorov, V., & Tauchen, G. (2010). Volatility Jumps. Working Paper, Northwestern University, Kellogg School of Management, Evanston.
Treynor, J. L. (1965, January/February). How to Rate Management of Investment Funds. Harvard Business Review, 43 .
Triana, P. (2009). Lecturing Birds on Flying: Can Mathematical Theories Destroy the Financial Markets? Hoboken: Wiley.
Tsay, R. (2005). Analysis of Financial Time Series (2nd ed.). Hoboken: Wiley.
Turner, A. L., & Weigel, E. J. (1990). “An Analysis of Stock Market Volatility,” Russell Research Commentaries. Tacoma: Frank Russell Company.
Vandewalle, N., Ausloos, M., Boveroux, P., & Minguet, A. (1999). Visualizing the Log-Periodic Pattern Before Crashes. European Physical Journal B, 9 (2), 355–359.
Vogel, H. L., & Werner, R. A. (2015, March). An Analytical Review of Volatility Metrics for Bubbles and Crashes. International Review of Financial Analysis, 38 , 15–28.
Wallace, C. (2010, May). Mind Over Markets . Institutional Investor .
Weatherall, J. O. (2013). The Physics of Wall Street: A Brief History of Predicting the Unpredictable . Boston: Houghton Mifflin Harcourt.
Willoughby, J. (2010, July 26). Did Investors Learn Anything from 2008’s Crash? Barron’s .
Wilmott, P., & Orrell, D. (2017). The Money Formula: Dodgy Finance, Psuedo Science, and How Mathematicians Took Over the Markets . Hoboken: Wiley.
Download references
Author information
Authors and affiliations.
New York, NY, USA
Harold L. Vogel
You can also search for this author in PubMed Google Scholar
Rights and permissions
Reprints and permissions
Copyright information
© 2018 The Author(s)
About this chapter
Cite this chapter.
Vogel, H.L. (2018). Random Walks. In: Financial Market Bubbles and Crashes, Second Edition. Palgrave Macmillan, Cham. https://doi.org/10.1007/978-3-319-71528-5_5
Download citation
DOI : https://doi.org/10.1007/978-3-319-71528-5_5
Published : 17 August 2018
Publisher Name : Palgrave Macmillan, Cham
Print ISBN : 978-3-319-71527-8
Online ISBN : 978-3-319-71528-5
eBook Packages : Economics and Finance Economics and Finance (R0)
Share this chapter
Anyone you share the following link with will be able to read this content:
Sorry, a shareable link is not currently available for this article.
Provided by the Springer Nature SharedIt content-sharing initiative
- Publish with us
Policies and ethics
- Find a journal
- Track your research

- Previous Issue
- Previous Article
- Next Article
- The Evolution of Hall's Random-Walk Hypothesis
- The Evolution of Hall's Original Object through the Lenses of Co-citation Networks and Semantic Analysis
- Habit Formation and DSGE Modeling
- Conclusions
The Drifting Influence of Hall's Random-Walk Hypothesis on Consumption Modeling
- Article contents
- Figures & tables
- Supplementary Data
- Peer Review
- Open the PDF for in another window
- Permissions
- Cite Icon Cite
- Search Site
Carlos García , Daniel Otero , Boris Salazar; The Drifting Influence of Hall's Random-Walk Hypothesis on Consumption Modeling. History of Political Economy 1 February 2023; 55 (1): 103–143. doi: https://doi.org/10.1215/00182702-10213653
Download citation file:
- Reference Manager
Hall's 1978 article titled “Stochastic Implications of the Life Cycle–Permanent Income Hypothesis” was meant to revolutionize consumption modeling. It indeed delivered, but not by becoming the dominant modeling strategy, but by unleashing a complex process of interaction between researchers in consumption and interconnected fields that transformed Hall's original object, from a local theory of consumption into a growing research area spanning the fields of consumption, finance, monetary policy and econometric modeling, and becoming, at the turn of the century, a building block of the DSGE modeling framework. Researchers reacting to innovative work produced new models that acted modified the context of research inducing a feedback system responsible for a connected set of innovations in the fields mentioned above. Along that process the practice of consumption modeling drifted away from Hall's original proposal, suggesting the necessity of a more nuanced view of the notion of influence in contemporary macroeconomics. In this particular case, we found that drifting occurred within the bounds set by the Euler equation and the assumptions of intertemporal optimization and rational expectations.
Advertisement
Citing articles via
Email alerts, related topics, affiliations.
- About History of Political Economy
- Editorial Board
- For Authors
- Rights and Permissions Inquiry
- Online ISSN 1527-1919
- Print ISSN 0018-2702
- Copyright © 2024
- Duke University Press
- 905 W. Main St. Ste. 18-B
- Durham, NC 27701
- (888) 651-0122
- International
- +1 (919) 688-5134
- Information For
- Advertisers
- Book Authors
- Booksellers/Media
- Journal Authors/Editors
- Journal Subscribers
- Prospective Journals
- Licensing and Subsidiary Rights
- View Open Positions
- email Join our Mailing List
- catalog Current Catalog
- Accessibility
- Get Adobe Reader
This Feature Is Available To Subscribers Only
Sign In or Create an Account

An official website of the United States government
The .gov means it’s official. Federal government websites often end in .gov or .mil. Before sharing sensitive information, make sure you’re on a federal government site.
The site is secure. The https:// ensures that you are connecting to the official website and that any information you provide is encrypted and transmitted securely.
- Publications
- Account settings
Preview improvements coming to the PMC website in October 2024. Learn More or Try it out now .
- Advanced Search
- Journal List
- v.7(4); 2021 Apr

On the (in)efficiency of cryptocurrencies: have they taken daily or weekly random walks?
Associated data.
The authors do not have permission to share data.
The legitimacy of virtual currencies as an alternative form of monetary exchange has been the centre of an ongoing heated debated since the catastrophic global financial meltdown of 2007–2008. Our study tests the informational market efficiency of cryptomarkets by investigating the weak-form efficiency of the top-five cryptocurrencies using random walk testing procedures which are robust to asymmetries and unobserved smooth structural breaks. Moreover, our study employs two frequencies of cryptocurrency returns, one corresponding to daily returns and the other to weekly returns. Our findings validate the random walk hypothesis for daily series hence validating the weak-form efficiency for daily returns. On the other hand, weekly returns are observed to be stationary processes which is evidence against weak-form efficiency for weekly returns. Overall, our study has important implications for market participants within cryptocurrency markets.
Efficient market hypothesis (EMH); Cryptocurrencies; Random walk model (RWM); Flexible fourier form (FFF) unit root tests; Smooth structural breaks.
1. Introduction
The role of cryptocurrencies as an alternative decentralized monetary system has gained a lot of traction following the disastrous sub-prime financial crisis of 2007–2008 which retarded the global financial system at levels not experienced since the infamous ‘Great Recession’ period of the 1930's. The digital currency market, though relatively new, is gaining increasing popularity as a ‘decentralized’ monetary system which could replace traditional centralized monetary systems and is attracting capital in lieu of the innovative technology underpinning cryptocurrencies. Proponents of digital currencies argue that the development of virtual currency markets will result in more inclusive financial markets for the future whereas opponents propose that the cryptocurrency market can only be characterised as a speculative bubble ( Sovbetov, 2018 ). Nevertheless, the economic background of competitive private currencies such as digital currencies, though theoretically compelling, is still limited in its practical implementation. The widespread minting of virtual currencies has caused a substantial debate concerning the need for their existence, where their value is derived and if adopted, how sustainable a decentralised monetary system would look like. In any case, the sustainability of either centralised or decentralised monetary regimes, would depend on the efficiency of these monetary systems in directing the currency in circulation to its most productive uses. Owing to the fact that the technical feasibility studies of decentralised monetary systems are still at nascent stages of development, the primary focus of our study is centred on the informational efficiency of digital currency asset markets.
The role of blockchain technology in reshaping traditional financial markets as well as the innovative potential of this technology in solving some of the recurrent socio-economic problems, particularly in developing countries characterized by unstable monetary and fiscal policies, financial exclusion, extreme poverty and high corruption levels, cannot be understated ( Weber, 2016 ). However, this innovation can only be enhanced by mitigating the risks that come with it since digital currency markets are rife with speculation. Despite the volatile nature of the cryptocurrency market as well as the risks involved in the digital currency trade, the market is growing at a rapid rate and investors as well as digital currency miners are making huge profits. It is due to these abnormally high profits, particularly those experienced in 2017, that market efficiency became a vital point of investigation. The efficiency of capital markets, of which virtual currencies have been adopted into as speculative investments, pre-empts the allocation of national resources to their most productive uses as perceived by investors. At the outset of this study, the credence of analysing the efficiency of this relatively unfettered sub-set capital markets is put forward not only because efficiency affects investment policies adopted by firms but also because it affects investing decisions taken by individual market participants. Our main concern is that speculative asset prices tend to exhibit bubble-like dynamics that eventually lead to catastrophic crashes. Therefore, evidence of market inefficiency in cryptocurrency markets would create a need for regulatory intervention in these markets and would discredit it's immediate acceptance as an alternative monetary system.
Our analytical approach is centred on informational efficiency, particularly, the weak form of the efficient markets theory in finance which is grounded in the random walk hypothesis of Nobel laureates Paul Samuelson and Eugene Fama. Our study contributes to the existing literature, Kristoufek, 2015a , Kristoufek, 2015b , Dyhberg (2016) , Urquhart (2016) , Bariviera et al. (2017) , Kurihara and Fukushima (2017) , Nadarajah and Chu (2017) , Chu et al. (2017) , Latif et al. (2017) , Katsiampa (2017) , Peng et al. (2018) , Ardia (2018) , Tiwari et al. (2018 , 2019) , Caporale and Plastun (2018) , Caporale et al. (2018) , Mensi (2018) , Caporale and Zekokh (2019) , Aggarwal (2019) , Hu et al. (2019) , Jana et al., 2019 , Bundi and Wildi (2019) , in three ways. Firstly, unlike a majority if previous studies which tend to focus on singular cryptocurrencies such as Bitcoin (see Bouri et al. (2019) ; Bouoiyour and Selmi (2016) ; Bariviera et al. (2017) ; Nadarajah and Chu (2017) ; Troster (2018) ; Tiwari et al. (2018) ; Caporale and Plastun (2018) ; Alvarez-Ramirez et al. (2018) ; Aggarwal (2019) ), our study examines market efficiency in 5 cryptocurrency markets (i.e. Bitcoin (BTC), Ethereum (ETH), Litecoin, Bitcoin Cash (BTCC) and Ripple (RIP)). To the best of our knowledge, only the recent studies of Caporale et al. (2018) , Brauneis and Mestel (2018) , Vidal-Tomas et al. (2019) , Hu et al. (2019) , Kristoufek and Vosvrda (2019) , Tran and Leirvik (2019) , Palamalai et al. (2020) have analysed efficiency on multiple cryptocurrency. Our study contributes this relatively small but growing strand of literature. Secondly, our study employs flexible Fourier form (FFF) unit root testing procedure described in Enders and Lee (2012) which is robust to asymmetries and unobserved structural breaks which are likely to exist in high frequency financial series such cryptocurrency prices and returns. The FFF-based unit root tests are considered superior testing procedures since the econometrician does not need to know a prior the form of nonlinearity underlying the time series or the number of structural breaks which exist in the data. Thirdly, instead of focusing on one frequency of time series, for instance on daily series as is the case in an overwhelming majority of existing empirical works (see Bouoiyour and Selmi (2016) , Nadarajah and Chu (2017) , Latif et al. (2017) , Caporale et al. (2018) , Hu et al. (2019) and Aggarwal (2019) ), our study employs both weekly and daily series to increase the robustness of our empirical analysis. As earlier argued by Martikainen et al. (1994) and Huang and Hoje (1995) , if the underlying statistical properties for alternative return intervals for asset prices significantly differs from each other, then the efficiency of stock markets is contingent on the frequency (of) trading strategies employed by market participants. Overall, our empirical analysis presents a robust analysis of weak-form informational efficiency for the cryptocurrency market which has important implications for policymakers and investors alike.
The remainder of our study is organized as follows. The following section of the paper provides an overview of cryptocurrencies as a decentralized monetary system. The third section presents a review of the associated literature. The fourth section outlines the methodology of the study, starting with the underlying random walk model of asset returns and building onto our specific nonlinear and Fourier based unit root testing procedures. The empirical results are presented in the fifth section of the paper whilst the study is concluded in the sixth section.
2. Cryptocurrencies as a decentralized monetary system
The legitimacy of centralized monetary systems has been long called into question and the most recent financial crisis of 2007–2009 has further weakened the public's trust in the ability of Central Banks to manage fiat currency particularly under fractional reserve systems ( Weber, 2016 ). The rise of Blockchain technology is envisioned as a libertarian response to the global centralized monetary systems and blockchain-based currencies employ crypto-technology to directly eliminate the need for an intermediary, such as a Central Bank, in transferring value amongst market participants. These blockchain networks facilitate inexpensive and expedient cross-border value exchanges that virtually eliminates arbitrage costs in international transactions. Digital currencies particularly offer an alternative way of managing payments in economies where Central Banks have either dismal or insufficient capital controls. And even beyond their role of currency creation, an important facet of cryptocurrencies is their decentralised public ledger network which offers more utility to market participants and is a driving factor for virtual currency valuation ( Wang and Vergne, 2017 ).
Despite their purported benefits, the adoption of synthetic currencies as money is not without its concerns. The problems associated with the practical use of cryptocurrencies within monetary economies can be described as two-fold. Firstly, high levels of volatility in prices and returns of cryptocurrencies creates much uncertainty in valuing cryptomarkets and causes cash flow problems for businesses transactions based on digital currencies, which altogether renders cryptocurrencies ill-suited as a store of value and a unit of account ( Weber, 2016 ). Secondly, the regulation of cryptomarkets is difficult because of the unresolved money versus speculative asset debate surrounding cryptocurrencies ( Kubat, 2015 ). Regulatory policies required to sustain cryptocurrency technologies need to be put into place to cater for future anticipated shifts attributable to the mass adoption of novel technologies ( Tasca, 2016 ). Consequentially, if regulation structures in decentralized currency systems are able to secure price stability and market efficiency, then the direct intervention of central authorities or private monopolies in financial markets can be kept at a minimal. However, the extent to which cryptocurrencies have been found to market efficient is subject to a growing debate as will be demonstrated in the literature review presented in next section of our study.
3. Review of the associated literature
Notably the empirical literature on cryptocurrencies is still in its embryonic stages of development which is not surprising when considering that virtual currencies only came into existence as a post-global financial crisis phenomenon. A systematic review of the existing literature on cryptocurrencies recently provided by Corbert et al. (2019) segregates the areas of academic focus into five categories, namely i) studies on bubble dynamics ii) studies on regulation iii) studies on cybercriminality iv) studies on diversification v) studies on market efficiency. As shown in the review, most of the existing studies are focused on the price efficiency of cryptocurrencies, with a bulk majority centred on examining the weak-form market efficiency i.e. investors cannot earn abnormal profits by analysing past data. More distinct categorizations of these studies can be made based on the differing econometric techniques used to verify or invalidate weak-form efficiency in cryptocurrencies.
Urquhart (2016) was amongst the first to examine weak-form market efficiency in cryptocurrencies by employing a battery of tests (Ljung-Box, runs test, variance ratio test, wild-bootstrap AVR test, BDS test, Hurst component) to Bitcoin returns and lent support to the inefficiency of the Bitcoin market although the author found evidence of market efficiency towards the latter periods of 2016. Using similar techniques to those employed in Urquhart (2016) , Nadarajah and Chu (2017) test the weak-form efficient market hypothesis using an odd integer power of the Bitcoin returns and find evidence in favour of weak-form market efficiency. Moreover, Bariviera et al. (2017) extended upon Urquhart (2016) by computing a Hurst component via detrended fluctuation analysis and found Bitcoin to be inefficient until 2013 when the returns turn efficient. Alvarez-Ramirez et al. (2018) also made use of detrended functional analysis to reveal switching efficiency with anti-persistent behaviour being found during increasing ‘bears’ markets. Jiang et al. (2018) employ an efficiency index to examine the time-varying long-memory in Bitocin and find strong persistence in the returns series implying weak-form inefficiency. Tiwari et al. (2018) test for market efficiency by constructing a market efficiency index based on time-varying Hurst exponent and find that Bitcoin has been informationally efficient since its inception with the exception of the mid-2013 and late-2016 periods. Sensoy (2019) examine time-varying permutation entropy for high frequency returns in two Bitcoin currencies and find that whilst the whilst intra-day returns have been more efficient since 2016, higher frequency returns are more informational inefficient. Selmi et al. (2018) make use of a multifractal detrend fluctuation analysis as well as the generalized Hurst component to examine weak-form efficiency in Bitocin and found that the efficiency of Bitcoin is more prominent during ‘bear’ periods and the returns series also has a more persistent long memory in short-term components.
There also exists a separate group of studies extended the which has examined for weak-form efficiency on more than one cryptocurrency. For instance, Caporale et al. (2018) investigates long-memory behaviour in 4 cryptocurrencies returns (Bitocin, Litecoin, Ripple and Dash) using fractional integration and the Hurst component and find evidence of market inefficiency in these cryptocurrencies although they more efficiency with the passing of time. Brauneis and Mestel (2018) applied the methods used in Urquhart (2016) to examine market efficiency 73 cryptocurrencies and find evidence of Bitcoin been most market efficient cryptocurrency. Moreover, for all observed cryptocurrencies the authors compute the Garman/Klass volatility, Amihud's illiquidity measure, the Corwin/Schultz spread estimator, turnover ratio and market capitalization and find liquidity and market capitalization to positively affect efficiency whilst the spread negatively affects market efficiency. Vidal-Tomas et al. (2019) adopted a portfolio approach to measuring weak-form efficiency for 118 cryptocurrencies. Extending upon the methodologies early used by Urquhart (2016) the authors find inefficiency of cryptocurrencies and the creation of new altcoins does not improve the efficiency of the market. Noda (2021) use the generalized least squares (GLS)-based time-varying autoregressive (TV-AR) model of Noda (2016) to show that the efficiency of Bitcoin and Ethereum evolves in over time, with Bitcoin being more market efficient compared to Ethereum. Tran and Leirvik (2020) investigate weak form efficiency for 5 cryptocurrencies using the adjusted market inefficiency magnitude (AMIM) estimator of Tran and Leirvik (2019) which is a robust measure of the market inefficiency magnitude (MIM) estimator of Noda (2016) . The authors find improving market efficiency in cryptocurrencies since 2013.
Besides the use of the Hurst exponent and long memory analysis in determining the informational efficiency for cryptocurrencies, there exists an even smaller scope of studies, which have more recently investigated weak-form efficiency in cryptomarkets using unit root testing procedures. For example, Latif et al. (2017) employ traditional ADF, PP and KPSS as well as the de-trend based DF-GLS and Ng-Perron tests to investigate the weak-form efficiency of Bitcoin and Litecoin returns and find that both cryptocurrency returns are stationary hence providing evidence against weak-form market efficiency. Similarly, Aggarwal (2019) applies traditional linear ADF, PP, KPSS tests as well as the structural break point tests of Perron (1989) and Zivot and Andrews (1992) and find Bitcoin returns to violate the random walk hypothesis as the series are found to be significantly stationary. Recently, Hu et al. (2019) adopt a panel approach to testing for unit roots is a panel of 31 of the top market-cap cryptocurrencies using the cross-sectional dependency tests of Chang (2002) , Moon and Perron (2004) , Breitung and Das (2005) and Costantini and Lupi (2013) . The authors find strong evidence against weak-form market efficiency for the cryptocurrencies. And even more recently, Palamalai et al. (2020) apply parametric ADF and PP unit root tests alongside other non-parametric methods tests to examine weak-form efficiency in 10 cryptocurrencies and find evidence against the random walk hypothesis for the returns series. Nonetheless, it is well known that conventional unit root tests, such as the ADF and PP tests, used in most previous studies can be misleading if one does not account for possible nonlinearities and structural breaks in the data. Moreover, the proper modelling of structural breaks poses as a serious problem for econometricians since the number, duration, location and form of structural breaks are not known aprior ( Pascalau, 2010 ). Our study circumvents these issues and contributes to the empirical literature by employing Fourier-based unit root testing procedures which are robust to both nonlinearities and unobserved structural breaks existing in the data.
4. Methodology
4.1. the random walk model of asset returns.
Samuelson (1965) and Fama (1965) initially proposed the random walk theory as a means of testing for weak-form efficiency within a series of asset returns. The authors describe the statistical independence of asset returns (R t ) using a general stochastic probability function defined as:
Where the first half represents the conditional probability that changes in price will take the value of R conditional on the knowledge that previous price changes took the values R t-1 , R t-2 and so on whereas the second half is the unconditional probability that the price changes during time t will take on the value R ( Fama, 1965 ). Malkiel and Fama (1970) re-specified the random walk model using one period percentage returns R j,t+1 i.e.
Where Ω t is the full historical information set from which the price at time t is derived and f is the density function for all t. In making use of the expected returns theory outlined in Mandelbrot (1966) , Malkiel and Fama (1970) obtained the following statistical representation of weak form efficiency:
Where E is an expectations operator. Eq. (3) illustrates that the expected return does not vary over time nor is it dependent on the historical information set, therefore analysing past information on asset values would not result in above-market profits. An alternative way of expressing Eq. (3) , would be to define it as the following autoregressive (AR) random walk model of stock returns 1 :
From Eq. (4) , the stock returns series, R t , is consider a random walk which confirms to the weak-form EMH only if ρ < 1 whereas if ρ = 1, then the series evolves as a stationary, predictable process which violates the weak-form EMH. Conventional random walk testing procedure such as the ADF, PP and KPSS tests commonly used amongst econometricians suffer from low power properties in distinguishing between nonlinear stationarity and unit root process as well as between random walk processes with breaks and unit root processes. To circumvent such issues, our study employs two ‘non-conventional’ unit root testing procedures, the first being the Kapetanois et al. (2003) ESTAR unit root testing procedure which is robust to asymmetries, and second the flexible Fourier form (FFF) testing procedure described in Enders and Lee (2012) which is robust to asymmetries and smooth structural breaks.
4.2. Testing the random walk using the KSS nonlinear unit root
In applying the KSS test to our returns series, we specify following globally stationary STAR regression:
Where e t ~ iid N (0, σ 2 ), γ is a smoothing parameter, c is the location parameter, d is the delay parameter and the transition function, Θ(θ; R t-d ), used is of exponential form such that:
Where Θ(θ; R t-d ):ℜ→[0,1] and is symmetrically U-shaped around zero. Kapetanois et al. (2003) consider the random walk null hypothesis a special case where β = 0 and θ = 0 in Eq. (5) whereas under the alternative hypothesis R t follows an asymmetric, globally stationary process. By substitution Eq. (6) into (5) and setting β = 0 and d = 1 results in the empirically exponential smooth transition autoregressive (ESTAR) model:
Since γ is unidentified in Eq. (7) , Kapetanois et al. (2003) propose the application of a first-order Taylor expansion to Eq. (7) around γ = 0 and obtain the following nonlinear random walk testing model:
In considering a more generalized form of regression (8) where the errors are serially correlated, Eq. (9) can be extended to included lags on the differenced time series as follows:
From Eqs. (8) and (9) , the null hypothesis of a random walk is tested as:
Against the alternative of a stationary process i.e.
And the test statistic evaluating these hypotheses is defined as:
Where δ ˆ is the OLS estimator of δ and s e ( δ ˆ ) is the standard error of δ ˆ . Since the t KSS statistic does not follow an asymptotic standard normal distribution, Kapetanios et al. (2003) derive critical values for the test statistics for the test performed on raw time series, de-meaned data (i.e. z t = x t – x ¯ t ) and de-trended data (i.e. z t = x t – μ ˆ – δ ˆ t ) where x ¯ t is the sample mean and μ ˆ and δ ˆ t are the OLS estimates of μ and δ, respectively.
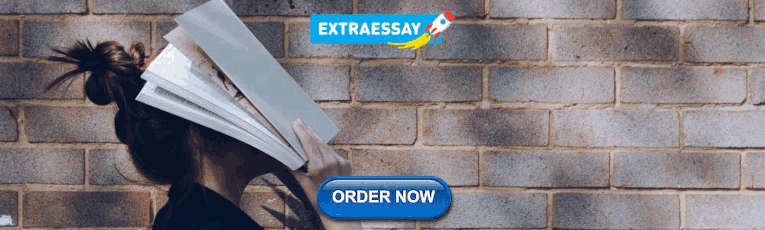
4.3. Testing the random walk via flexible fourier functions
Enders and Lee (2012) consider a simple modification of the Dickey-Fuller test in which a time-dependent deterministic term, d(t), is added to the test regression i.e.
Assuming that the functional form of d(t) is known, the unit root null hypothesis of p = 1 can be tested by approximating d(t) with the following single frequency Fourier function/equation:
Where k is the single frequency component and measure the amplitude and displacement of the sinusoidal component of d(t). Using our cryptocurrency return series (R t ), we model the underlying data generating process as the following FFF function:
To test the random walk null hypothesis of ρ = 1 against the stationary alternative ρ < 1, we follow Enders and Lee (2012) by employing a langrage Multiplier (LM) procedure to the following first differences econometric regression i.e.
And by using the estimated coefficients δ ˜ 0, δ ˜ 1 and δ ˜ 2 from Eq. (17) we construct a de-trended series as follows:
Where ψ ˜ = R 1 - δ ˜ 1 - δ ˜ 1 Δsin (2πkt/T) - δ ˜ 2 Δcos (2πkt/T). In subtracting ψ ˜ from R t we then obtain S ˜ 1 = 0. Using the de-trended series, S ˜ t , we formulate our econometric random walk test regression as:
And in adding lags to the first differences of S ˜ t regression (19) to remove possible serial correlation, produces the following augment FFF-based unit testing regression:
From Eqs. (19) and (20) the random walk null hypothesis is tested as the t-statistic (τ DF_t ) for the following null hypothesis, H 0 : φ = 0 and the empirical procedure is then practically carried out in the following four-steps:
Perform a two-dimensional grid search for combinations of all integers of k bounded between 1 ≤ k ≤ 5 and lags lengths bounded between 0 < j < 20. The optimal values of [k∗, j∗] are those associated with the regression that yields the lowest sum of squared residuals (SSR).
Perform a test of linearity using the F-statistics to test the null hypothesis of d 1 = d 2 = 0. Since the distribution of the F-statistic is non-standard, we rely on the critical values tabulated in Enders and Lee (2012) .
Evaluate the estimated regression for serial correlation using the traditional Durbin Watson (DW) statistic.
Compare the τ LM statistic against the critical values of reported in Enders and Lee (2012) for different sample sizes.
5. Data and results
5.1. empirical data and descriptive statistics.
The sample data was sourced from Coingecko ( https://www.coingecko.com/en ), a cryptocurrency exchange platform, and we specifically collect daily and weekly trading values for the five cryptocurrencies, namely Bitcoin (BTC), Ethereum (ETH), Litecoin, Bitcoin Cash (BTCC) and Ripple (RIP). Table 1 provides a summary of these cryptocurrencies in terms of founder, date found, market capitalization in US dollars in 2018, our data source as well as the sampled time period collected for each cryptocurrency examined in our empirical analysis:
Table 1
Data sources and cryptocurrency overview.
The daily and weekly returns, R t , are computed using the following continuously compounded returns formula:
Figure 1 below presents the time series plots of the log-returns form of our cryptocurrencies for daily and weekly frequencies. Table 2 presents summary statistics for the returns series which are difficult to dissect solely through visual appreciation of the time series plots. In terms of financial performance of the individual asset returns, ETH outperforms BTC attracting higher risk investors as its high yields are commensurate with the highly volatile nature of its returns. LTC and RIP are moderate-return assets with lower risk than BTC and ETH but higher returns than BTX. The relatively new BTX, which joined the cryptocurrency market in 2017, has more strides to make to establish itself in the virtual currency market as it attracts a lot of risk which is associated with suboptimal returns compared to the more established digital currencies. A noteworthy statistic which was manually computed for the purpose of making comparisons across the different series was the coefficient of variation (CV), which is indicative of the risk-return trade-off that an investor is offered when evaluating an asset for their portfolio. The computed CV supports this analysis as it shows the most profitable cryptocurrencies with higher units of risk being commensurate with the anticipated return.

Daily and weekly returns for all cryptocurrencies
Table 2
Summary statistics.
Notes: “∗∗∗”, ‘∗∗’, ‘∗’ denote 1%, 5%, 10% critical levels, respectively.
Besides the initial moments of mean and standard deviation (St.Dev), it is essential to also describe the higher moments of skewness and kurtosis. The former describes lack of symmetry about the mean akin to the normal distribution whereas the latter focuses on fatness of the tails and peakedness at the mean, a significant characteristic of financial asset returns. All observed time series appear to be positively skewed exhibiting longer upper tails than lower tails pointing to the result a substantial portion of the returns tend to be more positive. The kurtosis of the of the asset returns generally appears to be in excess of 3, meaning their distribution is mostly characterised as leptokurtic which is to be expected with financial time series. The reported Jarque-Bera (JB) statistics for all the daily and weekly cryptocurrencies returns series reject normality at all levels of significance with sole exception of the BTX weekly series which only rejects the normality hypothesis at a 10% critical level. More of the results of non-parametric test of Broock et al. (1996) for independence and identical distribution in the cryptocurrency returns, as reported in Table 3 , fails to confirm that any of the series is i.i.d., which is a violation of the weak-form market efficiency. Nonetheless, the absence of normality in the observed cryptocurrencies returns series is not atypical to characteristics of finical time series and strengthens our case for possible existing asymmetries and structural breaks existing within the series.
Table 3
BDS test for linear dependence (p-values).
5.2. Preliminary unit root tests
For comparison sake, we begin our empirical analysis by providing estimates from the first generation unit root tests, that is, unit root testing procedures which neither take into consideration asymmetries or structural breaks in the data generating process. Table 4 presents the results from the conventional ADF, PP and KPSS integration tests performed on the cryptocurrency returns. The results obtained from both ADF and PP unit root tests reject the random walk null hypothesis at critical levels of at least 5 percent for all cryptocurrencies regardless of whether the tests are performed with a drift only or inclusive of a trend, or whether the tests are performed on daily or weekly series. Note that this evidence against weak form market efficiency in the cryptomarkets has been previously established in the works of Latif et al. (2017) and Aggarwal (2019) who use similar ADF and PP unit root testing procedures albeit on weekly series. Moreover, these results are also consistent with findings obtained from the cross-sectional dependent, panel unit root tests employed by Hu et al. (2019) . Conversely, the outcomes from the KPSS test, which examines the null hypothesis of a stationary returns process, were not significantly different from those of the ADF and PP tests, with the exception of ETH and BTX daily (D) returns, which rejected the null of stationarity, at critical values of 10 percent and 5 percent respectively, in favour of a random walk in the cryptocurrency returns.
Table 4
ADF, PP and KPSS test results.
Notes: The modified SC criterion is used to determine optimal lag length of the ADF tests.
W – Weekly series, D – Daily series, I – intercept, IT – trend and intercept.
“∗∗∗”, ‘∗∗’, ‘∗’ denote 1%, 5%, 10% critical levels, respectively.
However, it is well-known that conventional unit root tests suffer from low power properties and size distortions problems in distinguishing between unit root and close-to-unit root processes. Therefore, we further supplement our preliminary unit root tests by estimating the modified Dickey-Fuller generalised least squares (DF-GLS) statistic of Elliot, Rothenberg and Stock (1996) and Ng and Perron (2001) which are considered more powerful in the estimation of parameters in a deterministic autoregressive process. Findings from these tests are presented in Table 5 , and as can be easily observed, the findings are mixed. For the DF-GLS tests, BTC, ETH and BTX daily (D) returns series favoured non-stationarity whereas the LTC and RIP series rejected non-stationarity. For the weekly (W) series, BTC, BTX and RIP rejected the null of a unit root whereas ETH and LTC failed to reject the unit root null hypothesis. The Ng-Perron results also comprised mixed results with most findings coinciding with the DF-GLS test results. For the Ng-Perron tests, RIP daily returns series as well as BTX weekly returns series both failed to reject the null when the inclusion of a trend. Notably the inconclusiveness of our DF-GLS and Ng-Perron tests in evaluating random walk behaviour for the Bitcoin returns has been previously reported in an earlier study by Latif et al. (2017) and Aggarwal (2019) and Palamalai et al. (2020) albeit strictly for daily Bitcoin returns.
Table 5
DF-GLS and Ng-Perron test results.
Notes: The modified SC criterion is used to determine optimal lag length of the tests.
5.3. Second generation unit root tests
The next phase in our empirical process is to determine whether accounting for nonlinearities would change the outlook on the inefficiency of cryptocurrencies generally assumed by the results of our conventional unit root tests. As argued by Kapetanois et al. (2003) , transaction costs and other frictions in financial assets markets are likely to lead to nonlinear equilibrium adjustments which linear unit root tests would exert low power in differentiating from unit root processes. Table 6 presents the empirical results for the KSS nonlinear test performed on our cryptocurrency returns. Note that these tests have been performed on raw, de-meaned and de-trended transformations of the returns series for daily and weekly data. The KSS tests statistics obtained from the raw data manage to reject the random walk hypothesis at all critical levels for 9 out of 15 cases with the random walk hypothesis failing to be rejected for the raw, de-meaned and de-trended BTC daily series as well as for the raw, de-meaned and de-trended RIP weekly series. Note that these findings are comparable to the findings obtained from the conventional ADF, PP and KPSS testing procedures but are in stark contrast to the outcomes of the DF-GLS and Ng-Perron tests.
Table 6
KSS test results.
R – Raw series, DM – De-meaned series, DT – De-tended series.
5.4. FFF-unit root test results
In the final stage of our empirical analysis, we carry out the LM-type Fourier unit root testing procedure described in Enders and Lee (2012) on the cryptocurrency returns series and record our findings in Table 7 . Note that we carry out this testing procedure in four phases. Firstly, we performed a two-dimensional grid search for the optimal combinations of the length of Fourier function frequencies, k∗, and the lag length of the first series of the ‘FFF de-trended series, j∗. The optimal combinations of k∗ and j∗ are obtained as those which simultaneously minimizes the RSS and these estimates are reported in columns (1) and (2) of Table 7 , respectively. Note that the optimal frequencies, k∗, were found to be either one or two, which is in line with the findings of other authors including the Enders and Lee (2012) , Becker et al. (2006) , Pascalau (2010) and Rodrigues and Taylor (2012) . Secondly, to ensure that the optimal lag length, j∗, removes all possible serial correlation we estimate the DW statistic and obtained statistics reported in column (3) of Table 6 indicate that our FFF-based unit root regression estimates are devoid of an autocorrelation. Note that for the BTX, LTC and RIP weekly series, we did not include lags as there was no evidence of serial correlation present (indicated by the Durbin Watson statistic). In fact, including lags resulted in a less than optimal Durbin Watson (DW) statistic, therefore the test regression that was estimated for the BTX, LTC and RIP weekly series was estimated with no lags.
Table 7
LM test results with the flexible Fourier form.
W – Weekly series, D – Daily series.
Thirdly, we test our regressions for linearity using the LM test described in Enders and Lee (2012) and report the obtained F-statistics in column (4) of Table 6 . Critical values reported in Enders and Lee (2012) were adopted at T = 500 for all daily returns with the exception of BTX which had fewer observations hence utilising critical values at T = 100. Similarly, with respect to the weekly data, the critical values utilised were at T = 500 with the exception of BTX which we relied on utilising critical values at T = 100. In performing our linearity tests, we find all cryptocurrencies rejected the null hypothesis in favour of nonlinearity, regardless of whether daily or weekly series are used. Lastly, we provide estimates of our τ LM statistic for all cryptocurrency series with the results reported in column (5) of Table 7 . Interestingly enough, before adding lags to the regressions we found that all currencies rejected the null in favour of stationarity and yet the test statistics are significant reduced in absolute value for most of the daily series subsequent to the addition of lags except for the LTC daily series as shown in Table 7 . Note that most cryptocurrencies in the daily series failed to reject the null of a unit root except for the LTC returns series. This notwithstanding, all cryptocurrency returns in the weekly series rejected the null of non-stationarity in favour of stationarity. These latter findings bear close similarity to those obtained from the conventional linear unit root test results as well as the outcomes of the KSS test where most series failed to provide formal evidence of weak-form market efficiency.
6. Conclusions
The exponential growth of the peer-to-peer digital currency trading in the aftermath of 2007–2008 sub-prime crisis has attracted increasing interest over whether these cryptocurrency markets are informational efficient. In our study, we rely on the random walk model of stock returns to investigate the weak-form market efficiency hypothesis for five of the most dominant cryptocurrencies (Bitcoin, Ethereum, Litecoin, Bitcoin Cash and Ripple) by employing a battery of random walk tests ranging from conventional unit root tests to integration tests which account for nonlinearities and unobserved structural breaks. Moreover, we examine the impact of return frequency intervals on the market efficiency for the case of daily and weekly return series. The outcomes from our empirical endeavours can be summarized in two main findings.
Firstly, in applying traditional unit root tests such as the ADF, PP and KPSS tests as well as the KSS nonlinear test provided strong evidence against weak-form informational efficiency for all observed cryptocurrencies regardless of whether daily or weekly returns are employed. Secondly, in relying on the more powerful FFF-based unit root testing procedure which is robust to both asymmetries and unobserved structural breaks, our findings show discrepancies with respect to the frequency intervals of the cryptocurrency returns. In particular, we find that, with the exception of Litecoin, daily series are generally market efficient whilst all weekly returns are informationally inefficient. In other words, ‘less-noisy’ weekly trading systems, as opposed to daily trading systems, can be used to generate abnormal profits in cryptocurrency markets. Therefore, high frequency traders who use algorithmic trading programmes to make multiple high-speed trades on an intra-day basis are unlikely to beat the market whilst those using weekly-based strategies face a better chance of beating the market. Our findings hence imply that the cryptocurrencies are not market efficient enough to be considered as a more formal exchange system and more regulatory intervention is required in cryptocurrency markets.
Declarations
Author contribution statement.
Andrew Phiri and Natalya Apopo: Conceived and designed the experiments; Performed the experiments; Analyzed and interpreted the data; Contributed reagents, materials, analysis tools or data; Wrote the paper.
Funding statement
This research did not receive any specific grant from funding agencies in the public, commercial, or not-for-profit sectors.
Data availability statement
Declaration of interests statement.
The authors declare no conflict of interest.
Additional information
No additional information is available for this paper.
1 It is important to note that whilst there is no strict connection between efficient market hypothesis and the random walks (see LeRoy (1973) and Lucas (1978) ) most empirical studies are focused on the weak-form efficiency to examine whether future prices are purely unpredictable based on the asset's price history.
- Aggarwal D. Do Bitcoins follow a random walk model? Res. Econ. 2019; 73 (1):15–22. [ Google Scholar ]
- Alvarez-Ramirez J., Rodriguez E., Ibarra-Valdez C. Long-range correlations an asymmetry in the Bitcoin market. Phys. Stat. Mech. Appl. 2018; 492 :498–955. [ Google Scholar ]
- Ardia D., Bluteau K., Ruede M. Finance Research Letters; 2018. “Regime Changes in Bitcoin GARCH Volatility Dynamics” (forthcoming) [ Google Scholar ]
- Bariviera A., Basgall M., Hasperue W., Naiouf M. Some stylized facts of the Bitcoin market. Phys. Stat. Mech. Appl. 2017; 484 (15):82–90. [ Google Scholar ]
- Becker R., Enders W., Lee J. A stationary test with an unknown number of smooth breaks. J. Time Anal. 2006; 27 (3):381–409. [ Google Scholar ]
- Bouoiyour J., Selmi R. Bitcoin: a beginning of a new phase? Econ. Bull. 2016; 36 (3):1430–1440. [ Google Scholar ]
- Bouri E., Gil-Alana L., Gupta R., Roubaud D. Modelling long memory volatility in the Bitcoin market: evidence of persistence and structural breaks. Int. J. Finance Econ. 2019; 24 (1):412–426. [ Google Scholar ]
- Broock W., Schieinkman J., Dechert W., LeBaron B. A test for independence based on the correlation dimension. Econom. Rev. 1996; 15 (3):197–235. [ Google Scholar ]
- Breitung J., Das S. Panel unit root tests under cross-sectional dependence. Stat. Neerl. 2005; 59 (4):414–433. [ Google Scholar ]
- Bundi N., Wildi M. Bitcoin and market-(in)efficiency: a systematic time series approach. Digital Finance. 2019; 1 (1):47–65. [ Google Scholar ]
- Brauneis A., Mestel R. Price discovery of cryptocurrecies: bitcoin and beyond. Econ. Lett. 2018; 165 :58–61. [ Google Scholar ]
- Caporale G., Plastun A. Finance Research Letters; 2018. “The Day of the Week Effect in the Cryptocurrency Market” (forthcoming) [ Google Scholar ]
- Caporale G., Gil-Alana L., Plastun A. Persistence in the cryptocurrency market. Res. Int. Bus. Finance. 2018; 46 :141–148. [ Google Scholar ]
- Caporale G., Zekokh T. The day of the week effect in the cryptocurrency market. Res. Int. Bus. Finance. 2019; 48 :143–155. [ Google Scholar ]
- Chang Y. Nonlinear IV unit root tests in panels with cross-sectional dependency. J. Econom. 2002; 110 (2):261–292. [ Google Scholar ]
- Chu J., Chan S., Nadarajah S., Osterrieder J. GARCH modelling of cryptocurrencies. J. Risk Financ. Manag. 2017; 10 (4):1–15. [ Google Scholar ]
- Corbet S., Lucey B., Urquhart A., Yarovaya L. Cryptocurrencies as a financial asset: a systematic analysis. Int. Rev. Financ. Anal. 2019; 62 :182–199. [ Google Scholar ]
- Costantini M., Lupi C. A simple panel-CADF test for unit roots. Oxf. Bull. Econ. Stat. 2013; 75 (2):276–296. [ Google Scholar ]
- Dyhberg A. “Bitcoin, gold and the dollar – a GARCH volatility analysis” Finance Res. Lett. 2016; 16 :85–92. [ Google Scholar ]
- Elliot Rothenberg, Stock Efficient test for an autoregressive unit root. Econometrica. 1996; 64 (4):813–836. [ Google Scholar ]
- Enders W., Lee J. A unit root test using Fourier series to approximate smooth breaks. Oxf. Bull. Econ. Stat. 2012; 74 (4):574–599. [ Google Scholar ]
- Fama E. The behaviour of stock market prices. J. Bus. 1965; 38 (1):34–105. [ Google Scholar ]
- Hu Y., Valera H., Oxley L. Market efficiency of the top market-cap cryptocurrencies: further evidence from a panel framework. Finance Res. Lett. 2019; 31 :138–145. [ Google Scholar ]
- Huang R., Hoje J. Data frequency and the number of factors in stock returns. J. Bank. Finance. 1995; 19 (6):987–1003. [ Google Scholar ]
- Jana R., Tiwari A., Hammoudeh S. The inefficiency of Litecoin: a dynamic analysis. J. Quant. Econ. 2019; 17 :447–457. [ Google Scholar ]
- Jiang Y., Nie H., Ruan W. Time-varying long-term memory in bitcoin market. Finance Res. Lett. 2018; 25 :280–284. [ Google Scholar ]
- Kapetanois G., Shin Y., Snell A. Testing for a unit root in the nonlinear STAR framework. J. Econom. 2003; 112 (2):359–379. [ Google Scholar ]
- Katsiampa P. Volatility estimation for Bitcoin: a comparison of GARCH models. Econ. Lett. 2017; 158 (C):3–6. [ Google Scholar ]
- Kristoufek L. What are the main drivers of the Bitcoin price? Evidence from wavelet coherence analysis. PloS One. 2015; 10 (4):1–5. [ PMC free article ] [ PubMed ] [ Google Scholar ]
- Kristoufek L. On bitcoin markets (in) efficiency and its evolution. Phys. Stat. Mech. Appl. 2015; 503 :257–262. [ Google Scholar ]
- Kristoufek L., Vosvrda M. Cryptocurrencies market efficiency ranking: not so straightforward. Phys. Stat. Mech. Appl. 2019; 531 [ Google Scholar ]
- Kubat M. Virtual currency Bitcoin in the scope of money definition and store of value. Proc. Econom. Finance. 2015; 30 :409–416. [ Google Scholar ]
- Kurihara Y., Fukushima A. The market efficiency of Bitcoin: a weekly anomaly perspective. J. Appl. Finance Bank. 2017; 7 (3-4):57–64. [ Google Scholar ]
- Latif S., Mohd M., Amin M., Mohamad A. Testing the weak-form of efficient market in cryptocurrency. J. Eng. Appl. Sci. 2017; 12 (9):2285–2288. [ Google Scholar ]
- LeRoy S. Risk aversion and the martingale property of stock prices” Int. Econ. Rev. 1973; 14 (2):436–446. [ Google Scholar ]
- Lucas R. Asset prices in an exchange economy. Econometrica. 1978; 46 (6):1429–1445. [ Google Scholar ]
- Malkiel B., Fama E. Efficient capital markets: a review of theory and empirical work. J. Finance. 1970; 25 (2):383–417. [ Google Scholar ]
- Mandelbrot B. “Forecasts of future prices, unbiased markets, and the ‘martingale models” J. Bus. 1966; 39 (1):242–255. [ Google Scholar ]
- Martikainen T., Perttunen J., Yli-Olli P., Gunasekaran A. The impact of return interval on common factors in stock returns: evidence from thin security markets. J. Bank. Finance. 1994; 18 (4):659–672. [ Google Scholar ]
- Mensi W., Al-Yahyaee K., Kang S. Finance Research Letters; 2018. “Structural Breaks and Double Long Memory of Cryptocurrency Prices: A Comparative Analysis from Bitcoin and Ethereum” (forthcoming) [ Google Scholar ]
- Moon H., Perron B. Testing for a unit root in panels with dynamic factors. J. Econom. 2004; 122 (1):81–126. [ Google Scholar ]
- Nadarajah S., Chu J. On the inefficiency of Bitcoin. Econ. Lett. 2017; 150 :6–9. [ Google Scholar ]
- Ng S., Perron P. Lag selection and the construction of unit root tests with good size and power. Econometrica. 2001; 69 (6):1519–1554. [ Google Scholar ]
- Noda A. A test of the adaptive market hypothesis using a time-varying AER model in Japan. Finance Res. Lett. 2016; 69 (6):1519–1554. [ Google Scholar ]
- Noda A. On the efficiency of cryptocurrency market efficiency. Appl. Econ. Lett. 2021; 69 (6):1519–1554. [ Google Scholar ]
- Palamalai S., Kumar K., Maity B. Borsa Instanbul Review; 2020. Testing the Random Walk Hypothesis for Leading Cryptocurrencies. (forthcoming) [ Google Scholar ]
- Pascalau R. Unit root tests with smooth breaks: an application to the Nelson-Plosser data set. Appl. Econ. Lett. 2010; 17 (6):565–570. [ Google Scholar ]
- Peng Y., Albuquerque P., de Sa J., Padula A., Montenegro M. The best of two worlds: forecasting high frequency volatility for cryptocurrencies and traditional currencies with support vector regressions. Expert Syst. Appl. 2018; 17 :177–192. [ Google Scholar ]
- Perron P. The Great Crash, the oil price shock, and the unit root hypothesis. Econometrica. 1989; 57 (6):1361–1401. [ Google Scholar ]
- Rodrigues P., Taylor R. The Flexible Fourier Form and local generalized least squares de-trended unit root tests. Oxf. Bull. Econ. Stat. 2012; 74 (5):736–759. [ Google Scholar ]
- Samuelson P. Proof that properly anticipated prices fluctuate randomly. Ind. Manag. Rev. IMR. 1965; 6 (2):41–49. [ Google Scholar ]
- Selmi R., Tiwari A., Hammoudeh S. Efficiency or speculation? A dynamic analysis of the Bitcoin market. Econ. Bull. 2018; 38 (4):2037–2046. [ Google Scholar ]
- Sensoy A. The inefficiency of Bitcoin revisited: a high-frequency analysis will alternative currencies. Finance Res. Lett. 2019; 28 :68–73. [ Google Scholar ]
- Sovbetov Y. Factors influencing cryptocurrency prices: evidence from bitcoin, Ethereum, Dash, litcoin, and monero. Journal of Economics and Financial Analysis. 2018; 2 (2):1–27. [ Google Scholar ]
- Tasca P. The dual nature of bitcoin as a payment network and money. VI Chapter SUERF Conference Proceedings. 2016 [ Google Scholar ]
- Tiwari A., Jana K., Das D., Roubaud D. “Informational efficiency of Bitcoin – an extension” Economic Letters. 2018; 163 :106–109. [ Google Scholar ]
- Tiwari A., Kumar S., Pathak R. Modelling the dynamics of Bitcoin and Litecoin: GARCH versus stochastic volatility models. Applied Economics. 2019; 51 (37):4073–4082. [ Google Scholar ]
- Tran V., Leirvik T. A simple but powerful measure of market efficiency. Finance Research Letters. 2019; 15 :s101382. [ Google Scholar ]
- Tran V., Leirvik T. Efficiency in the markets of cryptocurrencies. Finance Research Letters. 2020; 15 :s101382. [ Google Scholar ]
- Troster V., Tiwari A., Shahbaz M., Macedo D. Finance Research Letters; 2018. “Bitcoin Returns and Risk: A General GARCH and GAS Analysis” (forthcoming) [ Google Scholar ]
- Urquhart A. The inefficiency of Bitcoin. Economics Letters. 2016; 148 (C):80–82. [ Google Scholar ]
- Vidal-Tomas D., Ibanez A., Farinos J. Weak efficiency of the cryptocurrency market. A market portfolio approach. Applied Economic Letters. 2019; 26 (19):1627–1633. [ Google Scholar ]
- Wang S., Vergne J. “Buzz factor or innovation potential: what explains cryptocurrencies’ returns?” PLOS ONE. 2017; 12 (5) [ PMC free article ] [ PubMed ] [ Google Scholar ]
- Weber W. Bitcoin and legitimacy crisis of money. Cambridge Journal of Economics. 2016; 40 (1):17–41. [ Google Scholar ]
- Zivot E., Andrews D. Further evidence on the Great Crash, the oil-price shock, and the unit-root hypothesis. Journal of Business and Economic Statistics. 1992; 10 (3):251–270. [ Google Scholar ]
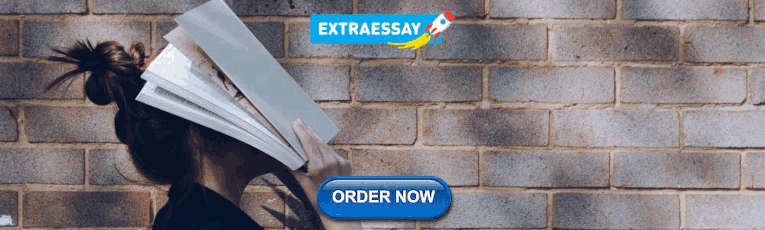
IMAGES
VIDEO
COMMENTS
Abstract. In this paper, we test the Johannesburg Stock Exchange market for the existence of the random walk hypothesis using monthly time series of the All Share Index (ALSI) covering the period ...
The basic idea behind the random walk hypothesis is that in a free competitive market the price currently quoted for a particular good or service should reflect all of the information available to participants in the market that influence its present price. ... PORTFOLIO RETURNS AND THE RANDOM WALK THEORY: COMMENT. The Journal of Finance, Vol ...
The purpose of this paper is to test empirically the random-walk theory relative to certain decision rules based upon past stock-price movements. A random. sample of thirty stocks on the New York Stock Exchange was selected. Daily closing prices of these stocks were studied over the January, 1960 to June, 1966 period.
Random walk was an early descriptive phrase and plank in what has come to be called modern portfolio theory. It includes the notions of efficient markets and equilibrium economics. This chapter reviews the subject in detail, not because the theory is necessarily correct and useful, but because it provides a benchmark against which newer and ...
The author's own empirical investigations of the random walk hypothesis are not summarized in this article. ... 1967); and "Relative Strength As a Criterion for Investment Selection," scheduled for March 1968 publication in The Journal of Finance. The latter two articles, in particular, offer evidence intended to refute the theory of random ...
Submit an article Journal homepage. 215 Views 5 CrossRef citations to date 0. Altmetric ... When we use single break tests, the random-walk hypothesis is rejected. However, when we use tests of double level shifts in the mean and make due allowance for multiple structural breaks, the results are consistent with the random-walk hypothesis in the ...
The basic idea behind the random walk hypothesis is that in a free competitive market the price currently quoted for a particular good or service should reflect all of the information available to participants in the market that influence its present price. To the extent that future conditions of the demand or supply are currently known, their effect on the current price should be properly ...
This article discusses the random walk hypothesis, examines some of the evidence that has been put forward to support it, and draws some implications for the theory of investment analysis. There seem to be only three references to the random walk hypothesis in U.K. actuarial literature—Weaver and Hall (1967), Hemstead (1969) and Moore (1972 ...
It is not generally understood that the random walk hypothesis requires, among other things, that the volatility of successive price changes be constant. ... Footnote 8 Indeed, since about 2000, the Journal has published many articles documenting the ways—both great and small—that financial market prices diverge from what might be perceived ...
A market where successive price changes in indi- vidual securities are independent is, by definition, a random walk market. Most simply the theory of random walks implies that a series of stock price changes has. no memory-the past history of the series cannot be. used to predict the future in any meaningful way.
The nature of risk and long-term returns is not fully understood. There is need for a measure of long-term risk at multiple horizons. Frequency domain digital signal processing, with an additive noise model, tests the random walk hypothesis for individual firm total and idiosyncratic risk at 2-month to 4-year periods.
This conclusion has been summarized by two hypotheses. First, the random walk hypothesis, states that daily returns are uncorrelated. Few people believe that this is exactly correct; indeed, the hypothesis has been refuted both for small stock markets [13] and for American commodity futures markets [3].
Abstract. Hall's 1978 article titled "Stochastic Implications of the Life Cycle-Permanent Income Hypothesis" was meant to revolutionize consumption modeling. It indeed delivered, but not by becoming the dominant modeling strategy, but by unleashing a complex process of interaction between researchers in consumption and interconnected fields that transformed Hall's original object, from a ...
The author's own empirical investigations of the random walk hypothesis are not summarized in this article. ... 1967); and "Relative Strength As a Criterion for Investment Selection," scheduled for March 1968 publication in The Journal of Finance. The latter two articles, in particular, offer evidence intended to refute the theory of random ...
The random walk estimation furnishes: AC~ = 9.34 + et. (3) The intercept has a positive value as required by theory. Further- more, the hypothesis that the errors are white noise cannot be re- jected: Q(12) = 19.7. Hall's original errors are serially correlated. 12 The evidence found with the filter is consistent with the random walk model.
The Efficient Market Hypothesis and Its Critics. Burton G. Malkiel. A. generation ago, the efficient market hypothesis was widely accepted by academic financial economists; for example, see Eugene Fama's (1970) influential survey article, "Efficient Capital Markets.". It was generally be- lieved that securities markets were extremely ...
The statistical results illustrated strong evidence refuting the presence of the random walk hypothesis in this emerging market, thus implying inefficiency in the cryptocurrency market. Furthermore, the absence of random walk in the cryptocurrency makes this financial asset predictable, giving investors an arbitrage edge to earn abnormal gains ...
The data, however, do not reject the hypothesis that disposable personal income is a random walk with drift. If income is indeed a random walk, then the standard testing procedure is greatly biased toward finding excess sensitivity. ... The stochastic implications of the life cycle-permanent income hypothesis: Theory and evidence, Journal of ...
The KSS tests statistics obtained from the raw data manage to reject the random walk hypothesis at all critical levels for 9 out of 15 cases with the random walk hypothesis failing to be rejected for the raw, de-meaned and de-trended BTC daily series as well as for the raw, de-meaned and de-trended RIP weekly series. ... Journal of Economics ...
Enter journal, DOI, article type, keywords, authors, etc. Quick search in Citations. ... The random-walk hypothesis is not rejected for any window ending before 75 mya and is rejected for all windows ending after 60 mya, which is similar to Fig. 2 A. The complexity increases and the statistical significance of the analysis decreases with series ...
The random walk hypothesis is a financial theory stating that stock market prices evolve according to a random walk (so price changes are random) and thus cannot be predicted. ... In 1993 in the Journal of Econometrics, K. Victor Chow and Karen C. Denning published a statistical tool ...
International Journal of Economics and Financial Issues (Sep 2016) ... Neo-Keynesian and Monetarist. Among these approaches, Permanent Income Hypothesis (PIH) and Random Walk Hypothesis (RWH) have been searching/analyzing the alternative consumption functions with related possible significant parameters since 1930s. This paper, first observes ...