image mining Recently Published Documents
Total documents.
- Latest Documents
- Most Cited Documents
- Contributed Authors
- Related Sources
- Related Keywords
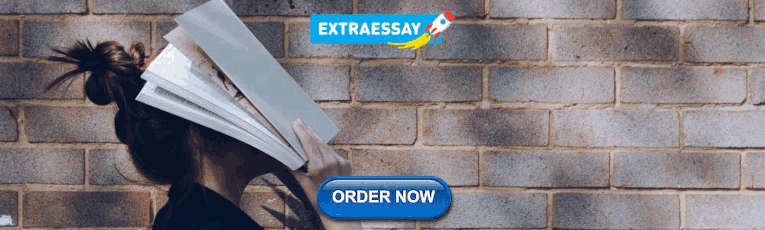
Extraction of fetal head section from ultrasound images using a soft-computing based image mining system—a study with Kapur’s thresholding and segmentation
Image mining applications for underwater environment management - a review and research agenda, application of improved boosting algorithm for art image classification.
In the field of computer science, data mining is a hot topic. It is a mathematical method for identifying patterns in enormous amounts of data. Image mining is an important data mining technique involving a variety of fields. In image mining, art image organization is an interesting research field worthy of attention. The classification of art images into several predetermined sets is referred to as art image categorization. Image preprocessing, feature extraction, object identification, object categorization, object segmentation, object classification, and a variety of other approaches are all part of it. The purpose of this paper is to suggest an improved boosting algorithm that employs a specific method of traditional and simple, yet weak classifiers to create a complex, accurate, and strong classifier image as well as a realistic image. This paper investigated the characteristics of cartoon images, realistic images, painting images, and photo images, created color variance histogram features, and used them for classification. To execute classification experiments, this paper uses an image database of 10471 images, which are randomly distributed into two portions that are used as training data and test data, respectively. The training dataset contains 6971 images, while the test dataset contains 3478 images. The investigational results show that the planned algorithm has a classification accuracy of approximately 97%. The method proposed in this paper can be used as the basis of automatic large-scale image classification and has strong practicability.
Image-mining: exploring the impact of video content on the success of crowdfunding
Massive picture retrieval system based on big data image mining, a security enhancement for the two way clustering algorithm for image mining, eye drowsiness tiredness detection based on driver experience using image mining.
These techniques introduce eye position state and it is parameter as a feasible means of sleepiness recognition. It has been recommended that an increase of eye sleepy state might indicates sleepiness. Thus this method can be used to caution the driver’s risk if driver drives the vehicle. These suggestion were derived from investigative an example of driver’s in attentive and sleepy situation. The gadget evaluate is base on tracking of the eye retina pupil (circular area) to calculate rate of eye sleepy condition. In this research study, individual change in the path of growing sleepiness from a drivers’ eye retina is examined. Data analysis study is interest on the improvement of a prepared display of sleepiness based on an arrangement of eye white and eye black measure values. This will use very accurate operational indicator of drowsiness. However, the main constraint of measure is that driver’s may not show this eye state until they are purely sleepy and/or weaken.
Chromatic-Luminance and True-Texture Analysis for Image Mining in the Social Sciences
This research note highlights the use of raw HSV (Hue, Saturation, Value) colorspace representation (capturing chromatic-luminance), and true-texture (matrix-based) representation of images for image mining applications in the social sciences. Specifically: we focus on the basics of teaching computers to ‘think like people’ in making decisions about what visual content is most interesting or important to human viewers. Our examples capture the facts that, (a) computers see ‘colors as numbers’, rather than as meaningful sections of an image, and (b) computers see texture as ‘numbers’, rather than as meaningful ‘hard’ or ‘soft’ sections of an image. Illustrations are provided using the R packages ‘colorfindr’, ‘glcm’, ‘imager’ and ‘plotly’.
A Survey Paper on Image Mining Techniques and Classification Brain Tumor
A scale and rotation invariant fast image mining for shapes, export citation format, share document.
Research about Image Mining Technique
- Conference paper
- Cite this conference paper
- Xianzhe Cao 3 &
- Shimin Wang 3
Part of the book series: Communications in Computer and Information Science ((CCIS,volume 288))
2140 Accesses
5 Citations
Image mining is a development potential technology for data mining which involves in multiple disciplines, it is also a challenging field which extends traditional data mining from structured data to unstructured data such as image data. The paper introduces the concepts, mining models and mining technology related with image mining. Although these image mining techniques have not yet developed mature, not long time later, image mining has its own distinctive theoretical system.
This is a preview of subscription content, log in via an institution to check access.
Access this chapter
- Available as PDF
- Read on any device
- Instant download
- Own it forever
- Compact, lightweight edition
- Dispatched in 3 to 5 business days
- Free shipping worldwide - see info
Tax calculation will be finalised at checkout
Purchases are for personal use only
Institutional subscriptions
Unable to display preview. Download preview PDF.
Liu, Z., Jiang, L.: The Research of Image Data Mining. Computer Engineering and Applications 39(33) (2003)
Google Scholar
Du, L., Chen, Y., Zhu, J.: Overview of Research on Image Data Mining. Computer Applications and Software 28(2) (2011)
Yu, X., Li, M.: Models and techniques of image data mining. Journal of Clinical Rehabilitative Tissue Engineering Research 14(35) (2010)
Zhang, J., Hsu, W., Lee, M.L.: An Information-Driven Framework for Image Mining. In: Mayr, H.C., Lazanský, J., Quirchmayr, G., Vogel, P. (eds.) DEXA 2001. LNCS, vol. 2113, p. 232. Springer, Heidelberg (2001)
Chapter Google Scholar
Zhang, J., Hsu, W., Lee, M.L.: Image Mining Issues. Frame Works, and Technique. In: Proceedings of the ACM SIGKDD International Conference on Knowledge Discovery&Data Mining, KDD 2001 (2001)
Datcu, M., Seidel, K.: Image Information Mining: Exploration of Image Content in Large Archives. In: IEEE Aerospace Conference Proceedings (March 2000)
Gardner, G.G., Keating, D.: Automatic detection of diabetic retinopathy using an artificial neural network: a screening tool. British Journal of Ophthalmology (1996)
Download references
Author information
Authors and affiliations.
Beijing Technology and Business University, China, 100048
Xianzhe Cao & Shimin Wang
You can also search for this author in PubMed Google Scholar
Editor information
Editors and affiliations.
Taiyuan University, Xueyuan Road 3, 030051, Taiyuan, Shanxi, China
Maotai Zhao
Xi’an University, Jinhua Road 4, 710032, Xi’an, Shanxi, China
Rights and permissions
Reprints and permissions
Copyright information
© 2012 Springer-Verlag Berlin Heidelberg
About this paper
Cite this paper.
Cao, X., Wang, S. (2012). Research about Image Mining Technique. In: Zhao, M., Sha, J. (eds) Communications and Information Processing. Communications in Computer and Information Science, vol 288. Springer, Berlin, Heidelberg. https://doi.org/10.1007/978-3-642-31965-5_15
Download citation
DOI : https://doi.org/10.1007/978-3-642-31965-5_15
Publisher Name : Springer, Berlin, Heidelberg
Print ISBN : 978-3-642-31964-8
Online ISBN : 978-3-642-31965-5
eBook Packages : Computer Science Computer Science (R0)
Share this paper
Anyone you share the following link with will be able to read this content:
Sorry, a shareable link is not currently available for this article.
Provided by the Springer Nature SharedIt content-sharing initiative
- Publish with us
Policies and ethics
- Find a journal
- Track your research
Academia.edu no longer supports Internet Explorer.
To browse Academia.edu and the wider internet faster and more securely, please take a few seconds to upgrade your browser .
Enter the email address you signed up with and we'll email you a reset link.
- We're Hiring!
- Help Center
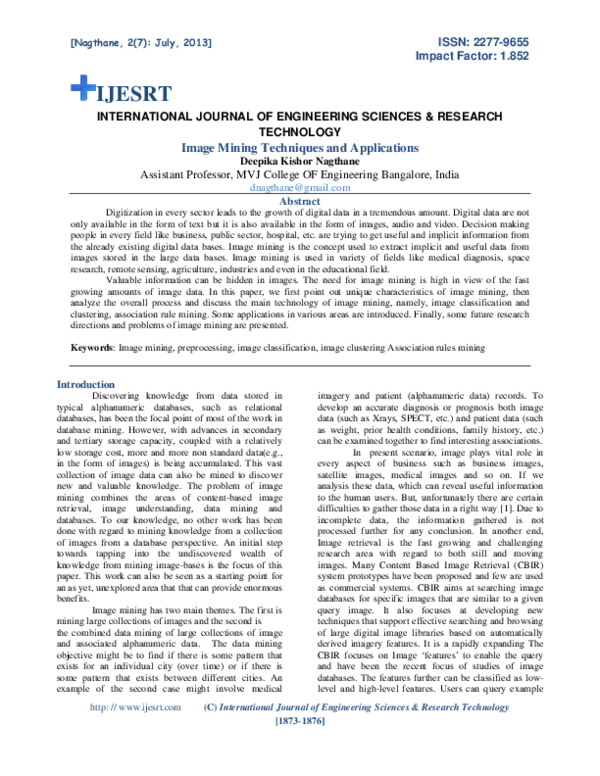
Image Mining Techniques and Applications.

2013, International Journal of Engineering Sciences & Research Technology
Related Papers
IJESRT Journal
Image mining refers to a data mining technique where images are used as data. It supports a large field of applications like medical diagnosis, agriculture, industrial work, space research and obviously the educational field. In this paper I would like to explain image mining-introduction, history, image mining process, image mining framework, application, techniques, various extraction mechanisms used in image mining and image retrieval based on semantics. Since image mining is now the most popular technique of retrieving information related to user query. These concepts are used to developing the research process and it gives the best image mining results.
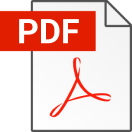
Mohammad Hadi Yousofi , Mahdi Esmaeili
Image mining is an interdisciplinary field that is based on specialties such as machine vision, image processing, image retrieval, data mining, machine learning, databases and artificial intelligence. Although many studies have been conducted in each of these areas, research on image mining and emerging issues is in its infancy. For instance, data mining techniques can not automatically extract useful information from the large amount of data set like images. In this paper, by presenting the unique features of image mining, we discussed about the general procedure of the analysis and the main techniques of image analysis. Finally we explored different image mining systems, and knowledge extraction from images to achieve progress and development in this area.
Advances in image acquisition and storage technology have led to tremendous growth in significantly large and detailed image databases. These images, if analyzed, can reveal useful information to the human users. Image mining deals with the extraction of implicit knowledge, image data relationship, or other patterns not explicitly stored in the images. Image mining is more than just an extension of data mining to image domain. It is an interdisciplinary endeavor that draws upon expertise in computer vision, image processing, image retrieval, data mining, machine learning, database, and artificial intelligence. Despite the development of many applications and algorithms in the individual research fields cited above, research in image mining is still in its infancy. In this paper, we will examine the research issues in image mining, current developments in image mining, particularly, image mining frameworks, state-of-the-art techniques and systems. We will also identify some future research directions for image mining at the end of this paper.
In this paper highlights a four-level information-driven framework for image mining systems, various methods available from literature, historical background, related work, algorithms and techniques that are frequently used in image mining, namely object recognition, image retrieval, image indexing, image classification and clustering, association rule mining and neural network.
Procedia Computer Science
udhav BHOSLE
International journal of computer and technology
Divakar Singh
Roshani Nage
This paper presents the analysis of existing literature which is relevant to mining and the mechanisms associated with weighted substructure. Though, the literature consists of a lot many research contributions, but, here, we have analysed around thirty-five research and review papers. The existing approaches are categorized based on the basic concepts involved in the mechanisms. The emphasis is on the concept used by the concerned authors, the database used for experimentations and the performance evaluation parameters. Their claims are also highlighted. Our findings from the exhaustive literature review are mentioned along with the identified problems. This paper is useful for comparative study of various approaches which is prerequisite for solving image mining problem.
IRJET Journal
Integrated Intelligent Reseach
Today data mining has become a vital role in all fields. This is because they discover interesting patterns and relationship in a data repository. Data mining is suitable for various fields such as image processing, artificial intelligence, machine learning, statistics and computation capabilities. Image segmentation is the fundamental step in various image processing tasks such as image analysis, visualization, and object representation and so on. The goal of image segmentation is to simplify, to partition image into meaningful regions and easier to analyze. This paper presents an overview of various data mining techniques associated with image segmentation. The data mining techniques such as clustering, classification and association are very easy to implement image segmentation that delivers valuable results. The above techniques are helpful to retrieve information from the images that are used to diagnose diseases, face detection, to improve the segmentation quality and so on. This paper provides crispy report about the data mining techniques and how they fetch the information from the images.
International Journal IJRITCC
digital image processing is one of the research areas for applicability of the data mining techniques. Clustering, k-nearest neighbor, decision tree classifier, neural networks are important techniques of mining that are useful for data analysis in most of the fields. Mining methods can works with the text, numbers, plus the multimedia like video and images also. The present paper target toward the analysis of the digital images stored in a system and this procedure includes many tasks say importing of the images for input, processing the images in that numeric values are computed for the given attributes of the input images, then performing mining operations to deduce the results. Due to advancement of mining tools this task is less time consuming and cost effective too moreover flexibility is provided to the user to select the desired method to get the different view of the outcomes.
RELATED PAPERS
Iranian Psychological Association
International Journal of Psychology (IPA)
Specijalna edukacija i rehabilitacija
Mile Vuković
Journal of Materials Chemistry
Nicoleta Cornei
Vagner Anabor
Control Engineering Practice
Ernesto Solanes
Photonics and Fiber Technology 2016 (ACOFT, BGPP, NP)
Haroldo Hattori
American Journal of Ophthalmology
Robert Feldman
Mathematics of Computation
eduardo godoy
Journal of Teaching and Learning in Higher Education
Marie Larneby
Asian Journal of Environment and Disaster Management
Nitin Srivastava
British Food Journal
Marco Tutino
As Metas Preconizadas para a Educação e a Pesquisa Integrada às Práticas Atuais 3
Pollyanna Ferreira
Future Generation Computer Systems
Colin A Low
John Jeyasekar
Journal of Health Economics
Maarten Lindeboom
Brill | Nijhoff eBooks
Symeon Symeonides
Bầu 12 tuần uống trà sữa có được hay không
Nguyễn Liệu
The American Surgeon
Cynthia Benz
FEBS Letters
Farhad Abdulkarim
Therya notes
Joel Saenz Mendez
Journal of Cardiac Failure
Samuel David Rocha Lazaro
World Journal of Emergency Surgery
Professor Dr. Kenneth Y Y Kok
Emergency Medicine Australasia
Theophilus I Emeto
The journal of physical chemistry. A
Victor Ryzhov
- We're Hiring!
- Help Center
- Find new research papers in:
- Health Sciences
- Earth Sciences
- Cognitive Science
- Mathematics
- Computer Science
- Academia ©2024
To read this content please select one of the options below:
Please note you do not have access to teaching notes, from words to pixels: text and image mining methods for service research.
Journal of Service Management
ISSN : 1757-5818
Article publication date: 9 October 2019
Issue publication date: 15 November 2019
The purpose of this paper is to describe and position the state-of-the-art of text and image mining methods in business research. By providing a detailed conceptual and technical review of both methods, it aims to increase their utilization in service research.
Design/methodology/approach
On a first stage, the authors review business literature in marketing, operations and management concerning the use of text and image mining methods. On a second stage, the authors identify and analyze empirical papers that used text and image mining methods in services journals and premier business. Finally, avenues for further research in services are provided.
The manuscript identifies seven text mining methods and describes their approaches, processes, techniques and algorithms, involved in their implementation. Four of these methods are positioned similarly for image mining. There are 39 papers using text mining in service research, with a focus on measuring consumer sentiment, experiences, and service quality. Due to the nonexistent use of image mining service journals, the authors review their application in marketing and management, and suggest ideas for further research in services.
Research limitations/implications
This manuscript focuses on the different methods and their implementation in service research, but it does not offer a complete review of business literature using text and image mining methods.
Practical implications
The results have a number of implications for the discipline that are presented and discussed. The authors provide research directions using text and image mining methods in service priority areas such as artificial intelligence, frontline employees, transformative consumer research and customer experience.
Originality/value
The manuscript provides an introduction to text and image mining methods to service researchers and practitioners interested in the analysis of unstructured data. This paper provides several suggestions concerning the use of new sources of data (e.g. customer reviews, social media images, employee reviews and emails), measurement of new constructs (beyond sentiment and valence) and the use of more recent methods (e.g. deep learning).
- Deep learning
- Computer vision
- Machine learning
- Text mining
- Service research
- Image mining
Acknowledgements
The authors appreciate the help of the doctoral candidate Alec Slepchuk in assisting the compilation of text mining papers.
Villarroel Ordenes, F. and Zhang, S. (2019), "From words to pixels: text and image mining methods for service research", Journal of Service Management , Vol. 30 No. 5, pp. 593-620. https://doi.org/10.1108/JOSM-08-2019-0254
Emerald Publishing Limited
Copyright © 2019, Emerald Publishing Limited
Related articles
We’re listening — tell us what you think, something didn’t work….
Report bugs here
All feedback is valuable
Please share your general feedback
Join us on our journey
Platform update page.
Visit emeraldpublishing.com/platformupdate to discover the latest news and updates
Questions & More Information
Answers to the most commonly asked questions here

An official website of the United States government
The .gov means it’s official. Federal government websites often end in .gov or .mil. Before sharing sensitive information, make sure you’re on a federal government site.
The site is secure. The https:// ensures that you are connecting to the official website and that any information you provide is encrypted and transmitted securely.
- Publications
- Account settings
Preview improvements coming to the PMC website in October 2024. Learn More or Try it out now .
- Advanced Search
- Journal List
- Comput Intell Neurosci
- v.2022; 2022

Building Image Feature Extraction Using Data Mining Technology
1 School of Architecture and Urban Planning, Guangzhou University, Guangzhou 510006, Guangdong, China
Chengyue Xing
2 Guangdong Provincial Institute of Cultural Relics and Archaeology, Guangzhou 510075, Guangdong, China
Associated Data
The data that support the findings of this study are available from the corresponding author upon reasonable request.
At present, data mining technology is continuously researched in science and application. With the rapid development of remote sensing satellite industry, especially the launch of remote sensing satellites with high-resolution sensors, the amount of information obtained from remote sensing images has increased dramatically, which has largely promoted the application of remote sensing data in various industries. This technique mines useable information from less complete and accurate data while ensuring low program complexity. In order to determine the impact of data mining techniques on feature extraction of graphic images, this paper explores the relevant steps in the image recognition process, especially the image preenhancement and image extraction processes. This paper develops a preliminary set of relevant data and investigates two different extraction methods based on the availability or absence of nursing information. Aiming at the advantages and disadvantages of the two house extraction methods, this work discusses how to effectively integrate remote sensing data. It uses different data sources to describe different characteristics of buildings, analyzes and extracts effective information, and finally derives building information. The research results show that, using the SVM algorithm in data mining for image feature extraction, in the verified filtering window, the accuracy can be effectively improved by about 20%. Buildings are important objects in high-resolution remote sensing images, and their feature extraction and recognition technology is of great significance in many fields such as digital city construction, urban planning, and military reconnaissance.
1. Introduction
With the rapid development of the current city, the remote technology of urban buildings has attracted more and more attention of professionals. Synthetic Aperture Radar (SAR) and Pol Synthetic Aperture Radar (PolSAR) are widely used due to their daily characteristics and ability to store general weather forecast and multimedia information. This work uses data mining technology to study internal SAR and PolSAR imaging data mining technology, focusing on four aspects, retrieval analysis, attribution search, attribution allocation, and customization, and provides a complete remote city radar image system. An efficient and robust information retrieval system provides strong support for political planning, national economic construction, national security, and military equipment.
Data mining is the process of extracting hidden, unknown but useful information and knowledge from large amounts of flawed, noisy, chaotic, and random application data. Data mining can perform functions such as identification grouping, customization, prediction, cultural analysis, isolation analysis, and parallel analysis. How to accurately extract buildings has always been a current research hotspot. This paper proposes introducing the invariant moment algorithm into the feature extraction of buildings from high-resolution remote sensing images and verifying it through experiments.
This paper investigates and analyzes image processing technology, feature extraction and segmentation methods, and existing problems and introduces the development and status of imaging technology in detail. Data mining techniques are studied in detail and applied to the image recognition process. This paper introduces many common computing algorithms, compares the performance of different computing algorithms, and uses different computing algorithms at different levels and uses. It then briefly introduces and analyzes the advantages and disadvantages of different feature extraction algorithms.
2. Related Work
For data mining and feature extraction, domestic and foreign experts have done a lot of research. Angeli C. introduced two examples of using data mining techniques, namely, association rule mining and fuzzy representation. He found association rule mining to be a useful way to obtain reliable data on learners' use of simulations and their performance. His research illustrates how data mining can be used to advance the practice of educational software evaluation in the field of educational technology, addressing issues related to data privacy [ 1 ]. Zhang M. studied the definition of data mining technology, summarized the steps and methods of data mining technology, and analyzed its application in the badminton court tactical analysis system [ 2 ]. Divya S. proposed a framework for retrieving similar building plans under the example query paradigm. He proposed a new algorithm for extracting high-level semantic features from building floor plans. Later, fine-grained retrieval using weighted sum of features was proposed, during which one feature can be prioritized over other features [ 3 ]. Kuroda Y.'s research focused on the big data formed by images accumulated on the social media “Instagram” dedicated to image posting and mainly focused on the two following points. (1) big data visualization of museum images and (2) exploring big data analysis methods to help art museum planning. He made these findings not to show the subjectivity reflected in each image but to show some of the characteristics and impressions of the museum from the image data accumulated by visitors [ 4 ]. Yongjun detected unripe green fruits in citrus trees under natural light conditions. He developed three supervised classifiers, logistic regression, random forests, and support vector machines (SVMs), using texture features. Logistic regression, random forest, and SVM models have detection accuracies of 79%, 75%, and 86%, respectively. The algorithm he developed shows great potential for identifying immature green citrus for early yield estimation [ 5 ]. Edavoor P. J. proposed a new method to obtain a complete binary (low complexity) 6-tap orthogonally symmetric wavelet filter bank (FB) with near-perfect reconstruction. This is achieved by changing the PR conditions slightly to make the quadrature filter symmetric and obtain the full binary filter coefficients. His proposed wavelet FB provides comparable performance in image compression and achieves excellent performance in iris recognition systems (feature extraction) and OFDM [ 6 ]. Matsukawa G. introduced a low-power object recognition processor VLSI. The processor processes HDTV resolution video at 60 frames per second (fps) using an object recognition algorithm with sparse FIND features. Using this architectural design, he achieved 60 fps HDTV resolution video object recognition performance at 130 MHz operating frequency [ 7 ].
3. Building Image Feature Extraction Methods
3.1. data mining.
Data mining is also used as a term for knowledge discovery (KDD), which is an important step in the knowledge discovery process [ 8 – 10 ]. The knowledge acquisition process follows these steps, as shown in Figure 1 .

Basic steps of data mining.
In the process of data mining, frequently used data systems have default information or wrong information. Data clearing is the process of clearing default data and erroneous data [ 11 ]. Data systems can identify extraneous data and process the extraneous data using mathematical calculations to arrive at the cause of the error. Data customization eliminates inconsistencies and default data in data and provides a more accurate data system for further data mining process to improve mining efficiency [ 12 ].
Data integration documents a more complete data system by transforming data in different formats into data in the same format. After cleaning the data and filling the default values, unnecessary features in the data system and features that are not relevant to the data mining step should be removed [ 13 ]. Data selection and data reduction are different from data systems. Data reduction is the removal of features, while data selection is the addition of feature data without affecting the results of cognitive research [ 14 , 15 ].
The z-score transformation is usually used to transform the data into a normal distribution, because the general statistical analysis method assumes that the data obeys a normal distribution, and all models require the input data to be normally distributed. A z-score transformation needs to be applied. Data exchange is routine data processing. During data mining, the collected data can have different sources and different formats. The data must therefore be balanced to obtain a simple data format for processing in the data mining step [ 16 ]. The data mining step allows intelligent analytical methods to extract data and manipulate procedures. According to the user's needs, it selects the appropriate algorithm, uses the selected algorithm to analyze the data, obtains the desired knowledge, and presents the results through visualization and other means [ 17 ].
Data mining is mainly based on aggregation algorithm, which can be subdivided into partition method, process state method, density source method, and grid source method. Among them, a feature of the hierarchical approach is the multirow customization of the database, which is suitable for high-resolution data [ 18 – 20 ]. In a cluster-based clustering system, the uncertainty of the weight parameters has a significant impact on the clustering effect. Although the weight of each cluster is uneven, the variation is large, and the clustering effect is poor. The grid-based loading method is fast loading, which is fast, but the quality and accuracy are not enough [ 21 ]. The partition-based loading method is simple and efficient and can handle large data systems without worrying about noise. In view of the large amount of data using household energy and the characteristics of some data silencing, the article chooses a group-based analysis method [ 22 ]. By analyzing the physical location of secret files and directories, all information is erased bit by bit, and random characters or custom characters can be used to fill multiple times to ensure that the storage medium data is overwritten multiple times to achieve the purpose of unrecoverable file data. This paper analyzes the current research progress on building extraction from remote sensing images. From the literature and research status, it can be seen that the invariant moment algorithm has a good identification function in the matching and retrieval of digital images and databases.
The grouping algorithm divides data objects into multiple parts according to the characteristics of the data to reduce the external similarity of each category and increase the internal similarity of each element. While both grouping and adaptation are data isolation, grouping is an unmanaged learning process, while isolation is a managed training process [ 23 ]. The isolation algorithm must first understand the structural characteristics of the data and classify according to the characteristics of the data structure and at the same time integrate the algorithm to find the characteristics of the data structure. Therefore, in many data mining processes, grouping is also used as part of data mining to record data structure elements. The study of loading algorithms involves several loading algorithms, including distribution method, location method, weight source method, grid source method, and model source method [ 24 ]. Image processing is the technique of analyzing images with a computer to achieve the desired results. Image processing generally refers to digital image processing. Digital image refers to a large two-dimensional array obtained by shooting with industrial cameras, video cameras, scanners, and other equipment. The elements of the array are called pixels, and their values are called gray values.
3.2. SVM Algorithm
The advantage of SVM is to solve small sample, nonlinear, and high-dimensional regression and binary classification problems. Small sample means that the number of samples required by SVM is relatively small compared to the complexity of the problem. Support vector machine was first proposed in 1995, which is based on statistical theory and was originally designed for binary classification problems. The algorithm not only introduces the concept of structural risk but also adopts the idea of kernel mapping. SVM algorithm shows many advantages in solving small samples, nonlinear data, and high-dimensional spatial pattern recognition.
The binary classification problem is also called binary classification. In the training data sample space, the sample data categories are divided into two categories. The purpose of the binary classification problem is to find a classifier that can successfully separate the two types of data.
Figure 2 shows the binary classification problem in a two-dimensional space, and the solid and hollow points represent two types of training data. In two-dimensional space, if a straight line can separate two types of data, such as straight lines L1, L2, and L3 in the figure, these data are said to be linearly separable; otherwise they are nonlinearly separable. The actual discriminant function can be expressed as
where f ( x ) is the value of the discriminant function. The distance from a point in space to the hyperplane can be written as
where δ is called the geometric interval and the geometric interval represents the Euclidean distance from the sample point in the space to the hyperplane.

Schematic diagram of binary classification in two-dimensional space.
Using y to represent the sample category, it is obvious that the sample points meet the following conditions:
In order to determine the optimal classification hyperplane, it is necessary to solve conditions w and b under the premise of the largest geometric interval; namely,
In order to facilitate the calculation of the deduction process such as derivation in the future, it can be rewritten as
The above formula is the basic type of SVM. Its objective function is a quadratic function of w , and the feasible region is a convex set, which is a convex quadratic programming problem. In order to solve efficiently, the Lagrange multiplier is introduced to obtain
where the vector is a =( a 1 , a 2 , ..., a n ) and the partial derivatives of w and b are equal to 0; there are
The following can be obtained by substituting the formula into its dual problem:
In the above formula, all are known quantities except a , and the discriminant function can be obtained by solving w and b:
With the development of remote sensing science and technology, the accuracy of remote sensing data is getting higher and higher, the acquisition speed is getting faster and faster, and the amount of data acquired is getting larger and larger, but the amount of information acquired by a single sensor is limited, and it is often difficult to meet the needs of applications. In practical applications, the training data is often nonlinearly separable. A linear hyperplane cannot completely separate the sample points correctly. At this time, in order to obtain a linear hyperplane, the points in the sample space can be mapped to a higher-dimensional space.
The classification function that maps to a higher-dimensional space can be rewritten as
The above formula can be rewritten as
There exists a function that accepts input values in a low-dimensional space. It calculates the inner product value of the high-dimensional space mapped to
This function K is called the kernel function.
For nonlinearly separable training vectors, it can be extended by modifying the formula
3.3. Remote Sensing Technology Feature Extraction
Airborne LiDAR systems acquire spatial information by acquiring high-precision 3D coordinates of ground targets. However, the airborne LiDAR cannot obtain the attribute information of the ground object surface from the point cloud information. This leads to the inability to construct the structure of the ground object based on the point cloud information, which increases the difficulty of extracting the topological information of the ground object. At present, airborne radar technology has accumulated rich experience in data acquisition and has relatively mature point cloud acquisition technology. However, the processing of airborne radar data is still in the research and development stage, and the postprocessing technology of the data is still relatively lagging behind. Most of the current point cloud data postprocessing algorithms have certain defects, and some algorithms with better performance have harsh conditions and poor applicability. The biggest problem that limits the development of point cloud data postprocessing technology is the filtering of point cloud data. Point data filtering is a fundamental and very important step in postprocessing of airborne LiDAR data. The pros and cons of point cloud filtering accuracy directly image the subsequent point cloud classification, object recognition, DEM generation, and 3D reconstruction of buildings. The realization process of the 3D reconstruction system is shown in Figure 3 .

System implementation process.
Morphological algorithms applied to image processing are based on nonlinear set theory. The biggest advantage of morphological theory is that it introduces the concept of “structural elements.” The basic idea is to use a set of known structural elements to perform correlation operations with images. According to the set judgment rules, it judges the attributes of the pixels in the coverage area of the structural elements and verifies the validity of the filled structural elements. Sequential structure is the simplest program structure and the most commonly used program structure, as long as the corresponding statements are written in the order in which the problem is solved, and its execution order is top-down and sequentially executed.
As shown in Figure 4 , according to the transformation relationship between the geodetic coordinates, camera coordinates, image physical coordinates, and image pixel coordinates during the imaging process of the optical sensor, the coordinates of the target point are obtained and the structural elements have various geometric characteristics.

Strict sensor model imaging principle.
The combination of elements of different structures will have different binding property characteristics. In practical applications, it is necessary to appropriately select structural elements to perform related operations according to the image characteristics to be processed. When processing images, we require a rectangular array of structuring elements, which is achieved by adding the smallest possible number of background elements to form a rectangular array. According to the geometrical characteristics of structural elements, structural elements can be divided into horizontal structural elements, vertical structural elements, square structural elements, diamond structural elements, and other types. The following are commonly used structural elements, as shown in Figure 5 .

3 ∗ 3 structural elements.
According to the transformation relationship between O geodetic coordinates, camera coordinates, image physical coordinates, and image pixel coordinates during the imaging process of the optical sensor, the coordinates of the target point are got, and the calculation method is
The camera coordinates are
There exists the following relationship between them:
It establishes the connection between the physical coordinates G of the image and the camera coordinates.
This is not the final strictly sensor imaging model yet. On this basis, the relationship between image pixel coordinates and image coordinates also needs to be considered, as shown in the following formula:
The strict sensor model defined by the collinear formula is rigorous in theory, and the relevant parameters are directly related to the physical quantities of the imaging sensor, which has distinct significance.
Compared with point cloud classification processing, the technology of digital image processing is quite mature. Image preprocessing technology, image segmentation, image compression, image restoration, and reconstruction technology have been very mature, and some new wavelet processing, morphological processing, and neural network technology have also achieved good results. Image processing technology has been successfully used in remote sensing, printing, medical imaging, and other fields.
The single-polarization SAR image can complete the urban scene classification, but, because of the limited information of the target scattering mechanism, it cannot effectively distinguish the buildings with different orientations. The advantage of PolSAR images is that the scattering mechanism of different types of objects can be characterized by using the polarization target decomposition method. It in turn can utilize the target scattering power to achieve urban classification of PolSAR images. Buildings with different radar orientations have different scattering mechanisms on PolSAR images. The multiphase polarization target path fault based on the scattering model proposed in this paper can illustrate the diffusion pattern of buildings under different radar azimuth conditions, which can be used to judge the particularity of cities. In view of the superpixels proposed in this paper which can help improve the classification accuracy of remote sensing images, the next step is to use superpixels to replace single pixels to achieve superpixel-level classification.
However, compared with the point cloud data, the image contains less information, and there is no coordinate information of the ground objects. At the same time, with the continuous advancement of technology, high-resolution image acquisition has become easier. High-resolution images bring not only more information but also more interference information, such as the green belts on both sides of the road, the interference of vehicles, and pedestrians on the road. Secondly, in most cases, the images obtained by photogrammetry are nonorthophoto images. This means that what we see is not what we get. That is, there is a certain deviation between the objects displayed on the image and their real geographic locations. This is mainly caused by the certain flight attitude of the aircraft and many other uncertain factors when collecting data. Therefore, image preprocessing needs to perform image correction, and there will inevitably be errors in the process of image correction, which will improve the accuracy of later feature extraction. The collection of point cloud data, due to the strict flight requirements and the use of GPS/inertial navigation system, can detect and record the flight attitude of the aircraft in real time. It can obtain high-precision coordinate information by using the supporting solution software. That is, “what you see is what you get,” and the point cloud reflects the real information of the ground objects.
3.4. Image Processing
In image recognition technology, the image quality directly affects the accuracy of the recognition algorithm. Therefore, imaging technology plays an important role in the entire image recognition process. Its main purpose is to remove trivial factors in images, add useful information, and make data as simple as possible to improve the reliability and accuracy of operations. Commonly used basic imaging algorithms include sampling algorithm, processing algorithm, and convergence algorithm. In order to achieve good results in practical applications, the above algorithms have their own characteristics in implementation methods. Imaging is the imaging technology of biological samples, which can be roughly divided into tissue imaging and cellular and molecular microscopy according to the size of the sample. These generally require the development of optical technology in conjunction with the characteristics of biological samples, and a few use wave properties other than light, such as nuclear magnetic resonance and ultrasound.
Due to various environmental factors, images can contain a lot of unwanted information. The filtering algorithm is to multiply the element parameter by the corresponding position, take the sliding window as the center, and take the corresponding value. Imaging algorithms have boundary issues such as performance and convergence. This means that when the limit cannot be improved, the further designed limit is treated separately. The general application scenarios of this algorithm are as follows. The size of the actual image cannot meet the constraints of the processing algorithm where the input panel is a multiple of the window size. The self-processing algorithm is computed by moving the sash backwards. Therefore, there will be some border elements without pixel values around them, which cannot be calculated by integration and require additional processing. By detecting the extreme point of the parameter in the parameter space, the curve corresponding to the parameter in the space domain is determined, so as to extract the regular curve in the image.
Among them, the sampling algorithm is divided into an upsampling algorithm and a downsampling algorithm. Bottom sampling refers to the process of compressing the original image to reduce the image to a certain factor, depending on the actual application scenario. The basic rule is to take a small image after calculation based on the local data in the image input panel. The upgrade process is to enlarge the image below to the original size. The whole process and parameters will be determined according to the actual application. However, the kernel process is implemented by computing the correlation of the local data of the original image with the parameters of the table. The goal of image preparation is to minimize the image when both the input and output are bright images. Currently, image processing matrices are commonly used to represent the value of each pixel in an image. The main purpose of preprocessing is to improve image data and provide technical support for subsequent image processing. The image processing material discussed in this article is part of image enhancement, where similar parallel processing algorithms are used to enhance visual effects and replace image features.
The experimental data must be preprocessed before image capability can be removed. In the process of identifying a building, it is important to highlight the structure and corners of the building. Therefore, grayscale and filtering functions are usually performed to weaken the junk information in the image and highlight the valuable information. Since this process basically computes the correlation between the pixel table and the input image, it has good parallelism.
4. Experimental Design and Result Analysis
Before the experiment, the parameters in the algorithm need to be set. When using the SVM algorithm for three classifications, the Libsvm toolbox is used for experiments, and the RBF kernel function is applied. It uses the svm_cross_validation function and the grid_search function to optimize parameters and obtain the optimal parameters. The cost matrix of the two-state decision problem is shown in Table 1 .
Two-state decision problem cost matrix.
It takes an ancient building as an example. First, the building is processed in grayscale, and then edge detection is performed with the Log operator to extract features. The result is shown in Figure 6 .

Building image edge detection: (a) original image, (b) image grayscale, and (c) edge detection image.
The extracted features are marked, and the result is shown in Figure 7 .

Feature extraction results.
It selects a corner of the building and processes its features, and the result is shown in Figure 8 .

Image segmentation optimization.
It can be seen that, after image segmentation and threshold segmentation optimization, the results of building removal are compared with the results obtained by the previous single method, the results of total building removal are significantly improved, and the basic layout and shape of buildings are kept well. However, the data also showed that there were still some components that were mistakenly removed and omitted. The main reason for the disappearance is that when the SVM algorithm performs image segmentation, the area distribution on the roofs of some buildings with opposite textures is different. Some of this is due to the angle of incidence of the light, and the roof is partially obscured, and some roofs have uneven and uniform levels of texture due to various objects like skylights. Drawing on the current research status of building image classification and the development of feature extraction technology, color features, texture features, and shape features are established as the indicators of building image classification.
As shown in Figure 9 , with the continuous increase of the filter window, the accuracy of the building exit shows a trend of first increasing and then decreasing. It can effectively improve the influence of small structural differences on the final output accuracy. The accuracy of suitable window extraction can be effectively improved by about 20%.

Building extraction accuracy.
For the cross-scattering components of the data and the polarization target decomposition results obtained by different methods. We compared the results of different mining methods in building image processing, and the energy consumption results of different methods are shown in Figure 10 .

Energy consumption comparison of different methods. (a) Unit energy consumption. (b) Overall energy consumption.
It can be seen from Figure 10 that, under different bowl fern methods, the energy consumption required for feature extraction of buildings is also different. The unit energy consumption of the traditional extraction method is about 6, and, with the increase of extraction times, the unit energy consumption will be too high. In the SVM algorithm used in this paper, the unit energy consumption is stable at about 3, which is much better than the traditional method.
We make statistics on the percentage of different scattering components when the buildings are located in different areas, and the results are shown in Tables Tables2 2 and and3 3 .
Scattering component ratios (overall) obtained by different methods.
Scattering component ratios (corners) obtained by different methods.
It discusses the influence of aperture decomposition and reflection asymmetry on building detection, sets the number of image subapertures to 2, 3, 4, 5, 6, 7, and 8, and repeats the traditional detection algorithm and SVM algorithm. The result is shown in Figure 11 .

Aperture decomposition and the effect of reflection asymmetry on building detection.
It can be seen that the forest and the two different types of buildings are always mixed together and indistinguishable, and the log-likelihood ratios of the three types of targets of road, small target, and bare ground are also very similar under different subapertures. This is because the nonstationarity of roads and small targets is weak under limited subapertures, and if the number of subapertures is increased, the image resolution will become lower, resulting in loss of target information.
5. Discussion
Although the morphological algorithm can filter out the noise on the image, the original image has too much noise. Its use of morphological filtering algorithm will destroy edge information to a certain extent. Such noise can be filtered out by performing multiple ring morphological algorithms. We used the circular complementary morphological filtering algorithm twice, using a 5 × 5 circular template and a 3 × 3 square template for the complementary template. The number of iterations used by the algorithm depends on the actual image noise. Larger images and more noisy images can increase the number of iterations. However, too many iterative calculations will destroy the edge information to a certain extent, so it is necessary to synthesize the whole process of processing to reasonably select the number of iterations.
Taking the actual survey area as an example, this paper elaborates the process of extracting buildings from LiDAR images using the improved morphological filtering algorithm described in this paper. Judging from the extraction effect of LiDAR point cloud image in the survey area in the actual project, the extraction framework is constructed reasonably.
The method described in this paper has high generality, and the effect of building edge detection is ideal. Based on the concept of multisource data processing, this paper applies imaging technology to the LiDAR cloud computing platform. In this work, traditional morphological processing is continued based on the properties of lidar point cloud data and the geometric features of lidar images. The usefulness and reliability of the method are proved by tests:
- ① According to scientific principles, taking into account the geometric characteristics of the building and the characteristics of the building space cloud, this work developed a custom automatic algorithm. It proposes a ring structure algorithm that enhances the smooth running of its surface. Experiments show that the algorithm has a good effect in removing the additive noise of the image and protecting the edge of the image.
- ② Due to the variability and inaccuracy of the distribution of airborne LiDAR lasers, as well as the influence of environmental factors during operation, the detected surface density is not uniform, resulting in inconsistent LiDAR images. Aiming at the complete hole problem, this work proposes a model algorithm and a two-dimensional object-based filling algorithm according to a scientific method, which can effectively protect the edge information of the image.
- ③ Compared with the traditional image processing algorithm, the SVM algorithm used in this paper has a great improvement in image clarity, edge output accuracy, and image quality. The image distortion is low, which confirms the reliability, applicability, and usability of its advanced algorithm.
The classification of urban buildings from radar remote sensing images is one of the important applications in the field of urban remote sensing. It is of great significance to urban planning, dynamic monitoring of buildings, and urban disaster monitoring. Since the radar is a side-view imaging, the azimuth of the building relative to the radar directly affects its scattering characteristics on the radar image. Buildings with different orientations are also of great significance in actual urban planning.
6. Conclusion
In this paper, a building feature extraction method based on SVM algorithm is proposed. First, it combines high-resolution spectral data with DSM data. Second, it extracts the underlying features of the merged data, including local neighborhood features and structural features. Next, the high-level semantic features of buildings are summarized through SVM, and the high-level semantic features and low-level initial features are combined, and the buildings are rederived using SVM. Finally, morphological filters are used to improve the extraction results. The results show that the building derivation algorithm studied in this paper has a strong application value for the reconstruction of ground objects. It can be considered that the key areas of the image are divided into blocks to extract key features, so that the acquisition of features is more accurate and complete, thereby providing guarantee for subsequent accurate classification.
Data Availability
Conflicts of interest.
The authors declare no potential conflicts of interest with respect to the research, authorship, and/or publication of this article.
Thank you for visiting nature.com. You are using a browser version with limited support for CSS. To obtain the best experience, we recommend you use a more up to date browser (or turn off compatibility mode in Internet Explorer). In the meantime, to ensure continued support, we are displaying the site without styles and JavaScript.
- View all journals
- My Account Login
- Explore content
- About the journal
- Publish with us
- Sign up for alerts
- Open access
- Published: 22 April 2023
Global mining footprint mapped from high-resolution satellite imagery
- Liang Tang ORCID: orcid.org/0000-0002-0967-6811 1 &
- Tim T. Werner ORCID: orcid.org/0000-0002-0565-4762 2
Communications Earth & Environment volume 4 , Article number: 134 ( 2023 ) Cite this article
25k Accesses
11 Citations
120 Altmetric
Metrics details
- Economic geology
- Environmental impact
- Sustainability
An Author Correction to this article was published on 11 May 2023
This article has been updated
Mining is of major economic, environmental and societal consequence, yet knowledge and understanding of its global footprint is still limited. Here, we produce a global mining land use dataset via remote sensing analysis of high-resolution, publicly available satellite imagery. The dataset comprises 74,548 polygons, covering ~66,000 km 2 of features like waste rock dumps, pits, water ponds, tailings dams, heap leach pads and processing/milling infrastructure. Our polygons finely contour the edges of mine features and do not include the space between them. This distinguishes our dataset from others that employ broader definitions of mining lands. Hence, despite our database being the largest to date by number of polygons, comparisons show relatively lower global land use. Our database is made freely available to support future studies of global mining impacts. A series of spatial analyses are also presented that highlight global mine distribution patterns and broader environmental risks.
Similar content being viewed by others
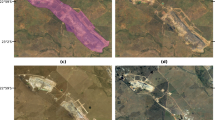
A global-scale data set of mining areas
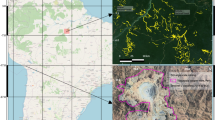
An update on global mining land use
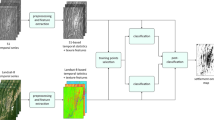
Outlining where humans live, the World Settlement Footprint 2015
Introduction.
Extractive industries can dramatically alter landscapes and cause irreversible damage to surrounding environments and communities. They simultaneously promise economic and social development and are essential to many key supply chains 1 , 2 . As mine areas continue their expansion across the globe, there is an increasing need for research that identifies impacts on surrounding landscapes. A subset of this research uses GIS and remote sensing methods to map the extent of land transformation due to mining activity 3 , 4 , 5 , 6 , 7 , 8 , 9 . These mapping exercises foster a more sophisticated understanding of the scale and location of risks posed by mining activity from local to global scales. They can also permit more nuanced planning of future developments in mining regions, for example, by informing the likely scale of future mine developments when mineral discoveries are made.
Research on the spatial patterns of mining can be mine- or region-specific 6 , 10 , or may examine mining as a global geographic phenomenon 11 , 12 . Global-scale studies have often relied on global corporate mining databases such as the Standard & Poor’s SNL metals and mining database 13 and review studies in economic geology (e.g., 14 , 15 ) that provide data on the coordinates of larger-scale mines. However, delineating the complete area occupied by mines at each coordinate is a comparatively more complex endeavor. Typically, it requires visual inspection or machine learning analysis of satellite imagery, followed by validation of mapped areas using alternative satellite imagery, corporate data, and/or field investigations. Studies like Yang et al. 16 and Yu et al. 17 have sought to use image processing algorithms to automatically identify and delineate mine areas, typically based on an already known dataset of mine areas. However, such studies typically focus on single regions, as the environments in which mines operate can be so variable that applying a singular visual approach or training dataset entails considerable uncertainty between different mining regions. On a global scale, studies like Tang et al. 8 , Werner et al. 3 , and Maus et al. 7 have recently employed manual visual inspection methods to delineate mines as polygons. These studies have employed slightly different approaches to classifying mine areas, yet collectively have improved the global coverage of sites mapped and sought to increase the precision of estimates for already mapped areas. The most recent of these efforts by Maus et al. 18 contributed around 44,929 polygons of global mining areas. Below, we outline an update that builds on these efforts to provide a mine area polygon dataset significantly larger (by the number of polygons) than any previous study. It therefore offers an advanced spatial dataset that progresses toward comprehensive and refined mapping of global mining land use. In the following sections, we explain our approach to updating global mine area data and provide a series of spatial analyses. We also compare to other global mining land use studies to illustrate differences in methodology and understandings of what comprises the global mining footprint. These comparisons allow for methodological refinement and replicability in future mapping endeavors. Our analyses indicate hot- and cold-spots of global mining activity, explain patterns occurring at large- and small-scales of mining, and show the enormous potential differences between areas occupied by mining itself, versus the broader areas impacted by mining. The complete polygon dataset is available for download via Zenodo, at https://doi.org/10.5281/zenodo.6806817 .
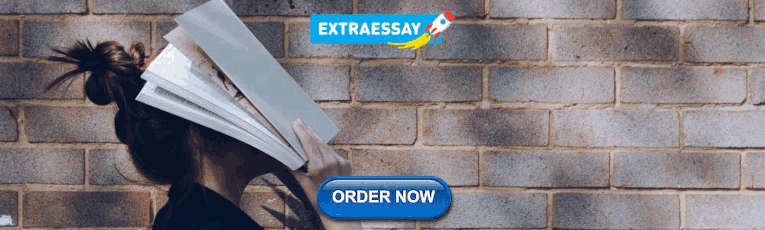
Results and discussion
Global mine areas and mine sites.
74,548 mine area polygons are reported in the present study, with global coverage and example delineated areas illustrated in Fig. 1 . A total estimated mine area of 65,585.4 km 2 is mapped, with an arithmetic mean of 0.88 km 2 (258,493.0 km total perimeter, 3.5 km/polygon). Figure 1 shows that while mines are composed of common features including (but not limited to) pits, waste rock dumps, water ponds, ore stockpiles, processing infrastructure and tailings dams, they show considerable variability in their spatial forms and scales. Table 1 summarizes areas from the most prominent mining nations. Our dataset comprises mine area polygons from 135 countries and regions, though these areas are highly clustered. Approx. 79% of the polygons are situated within 13 countries: China, USA, Russia, Australia, Indonesia, South Africa, Ukraine, Ghana, Canada, India, Brazil, Kazakhstan, and Chile. Correspondingly, 122 countries and regions have ~21% of the total mine area polygons, with fewer than 625 polygons each (<1%). Major mining nations can broadly be divided into high mineral demand countries (e.g., China, India, and USA) and high mineral export countries (e.g., Australia, Canada, South Africa, and Russia). Naturally, mine areas are situated in highly diverse geological, socio-political and environmental contexts. For example, mine areas in Ukraine arise from intensive coal exploration in the Soviet period 7 , and Ghana’s mine areas largely arise from placer gold resources in local riverine environments 19 .
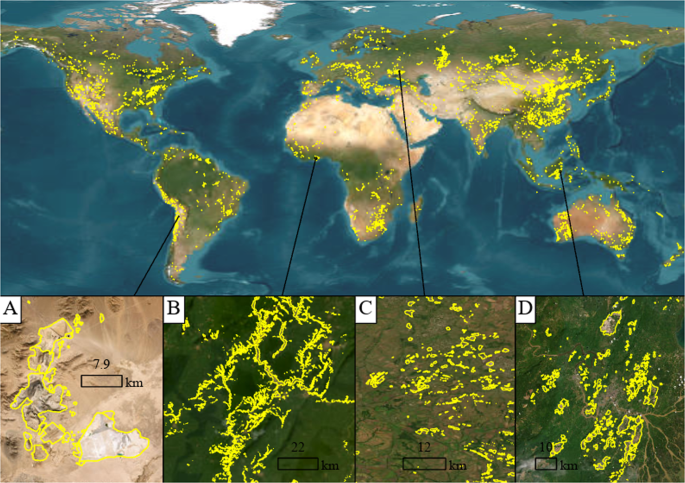
A Larger open pit operations (Escondida mine, copper porphyry deposit, Chile, 24°16’15 S, 69°4’14 W); B Formal/artisanal placer gold mining, Ankobra River, Ghana, where all mining sites are along the riversides or in the riverbed, forming a dendritic configuration (5°43’ N, 2°6’ W). C Coal mine sites and gangue heaps in Donetsk, Ukraine (48°12’ N, 38°39’ E); D Coal mine areas surrounding Samarinda in East Kalimantan, Indonesia (0°29’48 S 117°08’10 E). Imagery credit: Esri, Maxar, and Earthstar Geographics.
An overlay analysis of mine polygons versus SNL database coordinates showed that 58% of our polygons intersected with the 10 km buffers of 12,179 known mine operations. Of these, 9023 are “Active”, 1802 “Inactive”, and the remainder classified as “Care and Maintenance”, “Rehabilitation”, “Under Litigation” or “On Hold”. This compares to 6201 “Active” sites covered in Maus et al. 7 , which was subsequently updated with approx. double the count of polygons in Maus et al. 18 , but without updating the specific number of sites represented. The extent of overlap with SNL data on a per-commodity basis is presented in supplementary Table S1 .
Spatial distribution characteristics
To further explore global distribution patterns, we equally subdivided global land areas into 8,653 equal rectangular cells (fishnets 20 ), with an average area of 15,400 km 2 per cell. Mine area polygons were mapped to 2021 of these fishnets, revealing that mine area densities range from 0 to 16.1% per fishnet, at an average of 0.028%. Colder regions (e.g., northern Canada, Russia, and Greenland), high altitude areas (e.g., Qinghai-Tibet Plateau, China), and arid regions with limited resource demands (e.g., Afghanistan, Arabian Peninsula, Sahara Desert) exhibited lower mine area density. The former is potentially explained by hazardous climatic conditions that inhibit large-scale mining and exploration, and the latter is correlated with limited population. Reduced mine density in inland Africa is potentially an artefact of limited public reporting that inhibits the identification of small-scale mining. With rare exceptions, intensive mining is conducted ~4000 m above the sea level in Andes Belt, South America, which features a barbell vertical distribution 8 . By contrast, more mine areas are situated in mid-latitude regions, which corroborates increasing concerns linking mining to deforestation and environmental degradation 5 , 21 , 22 . Ultimately, the global distribution of mines is bound by the distribution of geological phenomena that concentrate minerals (e.g., geological fault zones, orogenic belts, great igneous provinces, and stable sedimentary basins) and the varying capacity for localities and companies to economically develop such sites, which is in turn influenced by a number of factors, including the depth of mineralization, and proximity to populations, roads and rail links 23 .
In statistical terms, the Moran’s I analysis of clustering behavior shows high index values both in global and local terms, indicating strong spatial autocorrelation behavior 24 . The Global Moran’s I index of 0.115 is notably higher than Tang et al. 8 (Global Moran’s I index = 0.081). This is expected, as our study has increased global coverage and our method involved identification of additional mine areas adjacent to previously known features.
A map of local spatial autocorrelation patterns (Fig. 2 ) shows that NS clustering behavior was observed for 67.8% of land areas. LL clusters occupied 23.8% of the global land surface, followed by LH outliers (6.1%), HH clusters (1.2%), and HL outlier areas (1.1%, Fig. 2 , see methodological descriptions for these cluster types below).
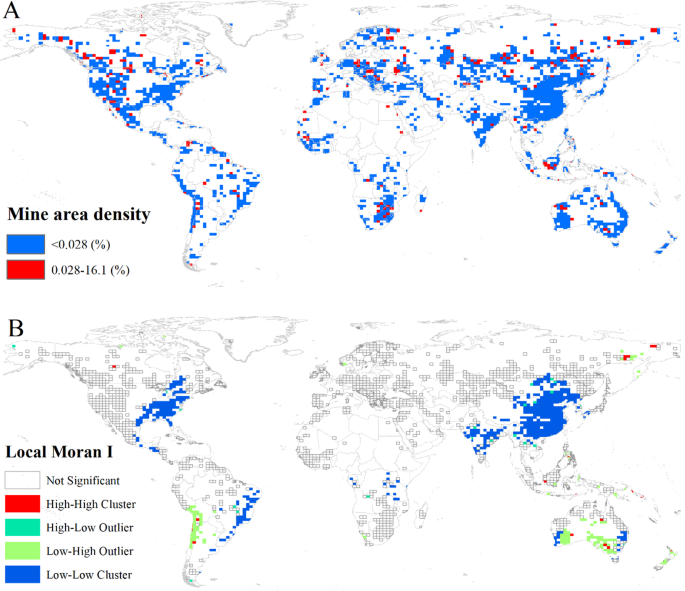
Global Moran’s I index = 0.115, z -score = 240, p value < 0.001. Mine areas are aggregated to 8653 fishnet cells (15,400 km 2 per cell, with some cells bisected due to irregular coastal borders) projected to Interrupted Goode Homolosine. A The global distribution density of mapped mining areas; B Anselin Local Moran’s I 55 .
Assessing scales of mining activity
There is high variability in mine area scales, with a global standard deviation of 7.64 km 2 . The sum of polygons presents a lognormal probability graph distribution and approximately straight lines 25 . This demonstrates that the data frequency distribution conforms to the lognormal distribution. The geometric mean (~0.123 km 2 ) may therefore best represent the average land occupied per mining feature (polygon).
Collectively, the quantity of mine sites varies substantially across scales (Fig. 3 ). An exponential decline is evident up to ~0.15 km 2 , such that areas <0.15 km 2 account for 54.6% of all polygons. Mine areas from 1350 m 2 to 7200 m 2 are the most frequent, and the mode mine polygon size of 1800 m 2 accounts for ~0.54% of all mapped mine areas. Beyond 0.15 km 2 , Fig. 3 shows a slight linear decrease. Mine areas also feature an S-curve accumulative tendency (logarithmic scale for the abscissa). The \(\frac{K}{2}\) index of abscissa is equal to ~6.35 km 2 , revealing those areas of around 6.35 km 2 account for the majority of total mine land use. Despite larger-scale mine sites remaining notably fewer in number, the scale of such mines still strongly influences total land occupation among the 74,548 mine polygons. By comparing with a smooth increasing curve in small-scale mine areas (e.g., <6.35 km 2 per mine site), the largest mine areas (>40 km 2 per mine site) show relatively jagged growth. In summary, while global mineral supply chains are dominated by the largest mines 3 , minor-scale mining still contributes comparable areas to global mine land use.
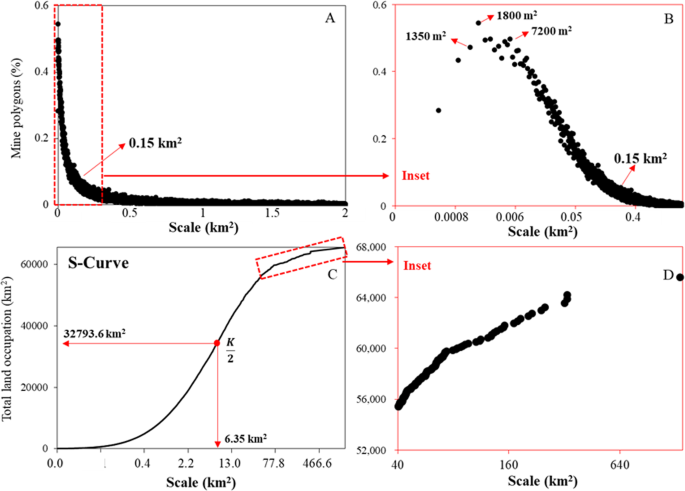
A Overall frequency distribution of mine area scales, noting that there may be under representation of polygons at the smallest scales due to limitations in ASM coverage; B Inset of small-scale mine areas; C S-curve of increasing total mine area versus the scale of mine polygons; D Inset of S-the curve of the largest-scale mine areas.
Larger-scale mine areas may be characterized as including more than ~12.3 km 2 per polygon (per Fig. 3 ), and such sites represent the dominant share of global metals production 3 . On this basis, ~761 of the mine polygons mapped may be considered larger scale, corresponding to a total land use of 23,334.3 km 2 (35.6% of the total database area, averaging ~30.7 km 2 per polygon). These larger-scale mine areas are situated in 61 of the 135 countries mapped. By number of large-scale mine polygons, the national-level sequence is Russia ( n = 114) > USA ( n = 97) > Australia ( n = 67) > China ( n = 58) > Indonesia ( n = 51) > Canada ( n = 50) > Chile ( n = 35) > South Africa ( n = 30) > Colombia ( n = 22) > Kazakhstan ( n = 22) > Brazil ( n = 18) > India (17) > other countries (180). Approx. 34 metals and nonmetals are produced from such sites (portions are polymetallic deposits). The largest mine area is the Salar de Atacama in Chile, as this site uniquely incorporates the broader salt flat from which lithium brines are pumped. In terms of the polygons by commodity, coal mining is the most prominent ( n = 304, 7282.0 km 2 ), followed by gold ( n = 152, 4623.1 km 2 ), copper ( n = 80, 1722.6 km 2 ), iron ( n = 68, 1339.0 km 2 ), phosphate ( n = 44, 883.1 km 2 ), salt ( n = 21, 2877.7 km 2 ), diamond ( n = 18, 431.7 km 2 ), oil sands ( n = 16, 738.1 km 2 ), bauxite ( n = 15, 473.0 km 2 ), sand ( n = 11, 249.6 km 2 ), uranium ( n = 7, 123.3 km 2 ), and others ( n = 18, 2591.1 km 2 ). As would be expected, the average polygon size (as opposed to total area) can also differ substantially between commodities. The largest mine type per polygon is a salt field (mean 137.03 km 2 ), which is typically situated in arid and inland regions. Other large types (on a per-polygon basis) include oil sands areas (46.13 km 2 ), which are mainly located in the Alberta province, Canada, and the large-scale bauxite mines (31.5 km 2 ), notably in northeast Australia, the Amazon Plain, and Kazakhstan.
ASM refers to mining by individuals, groups, families, or cooperatives, often in the market’s informal (potentially illegal) sector 26 . While characteristically small on a per mine site or per polygon basis, the global ASM footprint is thought to be substantial. As such sites often do not comply with environmental regulations, the broader risks of these sites can be magnified 27 . ASM production has been estimated to account for 15–20% of the world’s non-fuel minerals, 20% of gold, 40% of diamonds, and almost all colored gemstones 28 , 29 . However, the coverage of ASM in past global mapping endeavors has been limited. It is therefore recognized that developing an understanding of the global ASM footprint is an important advancement. Table 2 presents the results of known ASM areas that were mapped in this study on a case study basis. We note that there is incomplete global coverage of ASM in our database. Some ASM areas require particularly high-resolution imagery and occasionally access to paid satellite services to appropriately analyze. Such sites can be difficult to include in a global-scale study due to their high dispersion. It is noted that national- and regional-scale studies have also mapped specific ASM areas, which we examine in the following section.
In this study, on a case study basis, we mapped 4058 polygons of ASM to examine the spatial characteristics of known small-scale, informal, and artisanal mining activity worldwide. These areas totaled 1071.2 km 2 , constituting 1.63% of the total mine areas mapped (Table 3 ), yet are noted as representing only a portion of global ASM activity. For example, Asner et al. 10 estimate an additional 500 km 2 of small-scale gold mining in the Madre de Dios region of Peru alone.
ASM polygons in this study were highly variable in size, ranging from 0.0004 to 31.33 km 2 , with arithmetic and geometric means of 0.264 km 2 and 0.062 km 2 per polygon respectively (Table 3 ). As the polygons fall approximately on a straight line in a lognormal probability graph, the geometric mean can represent the average scale of ASM 25 . ASM is ubiquitous in some of the developing countries assessed, including China, Ghana, Burkina Faso, Malawi, Mali, Mozambique, South Africa, Tanzania, Zambia, Zimbabwe, India, Indonesia, Papua New Guinea, Philippines, Bolivia, Brazil, Ecuador, and Peru 26 . As large-scale mines are more likely to feature controlled mine waste site selection, comprehensive management, and post-mining rehabilitation works, elevated risks or environmental damage have emerged from ASM activities 30 . ASM areas are often associated with severe environmental, health, and safety risks 31 , 32 , 33 , 34 , and the mapped land and riverbed areas subject to ASM did not exhibit apparent ecological protection measures. Thus, while the global large-scale metal mines covered substantially more land per polygon (≥12.3 km 2 ), the proportional impacts of ASM cannot be disregarded at any scale 3 . In some countries, ASM activity centers on the production of gold, bauxite, gemstones, iron ore, marble, and limestone 26 , where related geological enrichment zones can lead to both formal and informal activity coexisting. Among the countries where ASM was prevalent, most activity was informal and considered illegal.
It should be noted, however, that it can be challenging to classify mine sites as ASM based on visual inspection alone, and indeed the proper definitions of such areas can also be unclear. As such, for the purposes of this study, we discuss areas based on a characteristic polygon size cutoff (~<0.062 km 2 ), visual appearance, and review of existing classifications of otherwise previously described ASM activity. Globally, a considerable portion of the formal mining areas appeared to host small-scale mining in surrounding areas. Yet some of these areas may incorporate the beginnings of new mineral excavation and construction of affiliated facilities. The land coverage of tailings ponds depends on the lifecycle of mining and may cover less land during early stages such that they appear as small-scale operations. Geographic restrictions that inhibit the expansion of mining, such as steep terrain and dense population 35 , as well as minor ore bodies that limit larger-scale operations can also have an influence on the appearance of mine areas. Small coal-waste dumps in Ukraine are evidence of this 36 . Applying a marker of 0.062 km 2 per mine area (as the geometric mean of smaller scales), 27,410 polygons (36.8%) may be considered small scale (but not necessarily ASM), with the mean value of 0.024 km 2 accumulating only 665.3 km 2 in total (~1.0% of global mapped mining land use). Collectively, except for Guinea-Bissau with a limited mine sample size, North Korea exhibited the greatest proportion of small-scale mining in the world (74.4% of mine polygons). The majority of the smallest polygons are distributed in developing nations, including Sierra Leone, Albania, Algeria, Pakistan, and Angola. However, this is not a constant trait of all such countries. China hosts substantial small-scale mining, with ~16,676 small-scale mining polygons (53.5%) identified (Fig. 4 ).
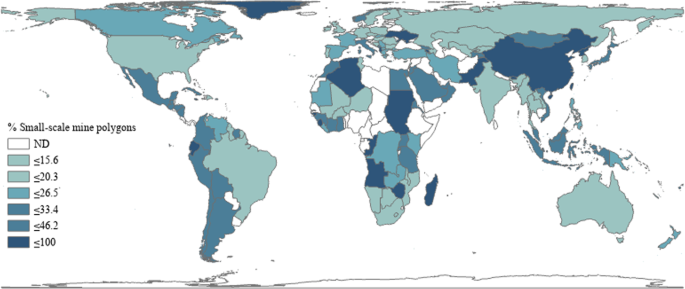
Percentage of mine polygons <0.062 km 2 per country, signaling and validating an increased prevalence of ASM.
Agricultural poverty, the potential to generate extensive distributional benefits, and customary land tenure practices may also be influential in encouraging informal mine operations 37 , 38 . Yet the average mine polygon size positively correlates with human development 8 . More developed countries with ample mineral resources exhibit lower percentages of small-scale mining, with an average percentage of 18.8% among countries like New Zealand, Canada, Australia, USA, and South Africa. This is likely attributable to planning and management practices that encourage larger scale operations, alongside access to technologies and equipment employed at larger scales 39 . The regulatory environment of developed countries can add to the cost of mining, creating conditions more favorable to larger scales 8 . In Russia, where only 13.4% of polygons may be considered small scale, larger reserves of mineral resources, or deposits otherwise classified as high grade are often prioritized for larger-scale developments (Fig. 4 ).
Comparisons, uncertainties and replicability
Comparing to previous studies mapping mine areas at national or global scales can help to identify what consistency or replicability has been achieved. A comparison of results from a sample of recent literature is presented in Table 3 . Our current dataset represents 165% of the polygons of Maus et al. 18 , while only indicating 65% of the total area. This is likely explained by Maus et al. 18 including spaces between adjacent mine features within an overall polygon shape, a difference in methodology explored in Tang et al. 8 . Tang et al. 8 employ the same delineation methodology as this study, yet our study produces a 303% comparative polygon count for a 208% increase in total area. Our higher count of polygons per unit area may be explained by our increased coverage of small-scale mining (which often exhibit more numerous, smaller and dispersed features). ASM areas mapped in this study are subject to different land-use change dynamics, and can cumulatively represent a considerable land area, albeit representing a relatively smaller proportion of global mineral production. Within this subset, we also observe some differences to other studies. For example in Ghana, ~470 km 2 of vegetation has been reported as lost to mining, along with the expansion of artisanal mining 19 . In our study, we mapped 1003.8 km 2 of mine polygons in Ghana, supported by past studies identifying the location of 394 artisanal mining and 104 industrial mining sites for gold extraction in Ghana’s southwestern forests 189 .
Other studies also exhibit apparent dissimilarities to each other, albeit with different stated scopes. For example, Werner et al. 3 , mapped 295 global metal mine sites (at ~28.2 km 2 /site), specifically focusing on delineating large-scale mines in a similar fashion to the present study. Sonter et al. 5 mapped 62,381 mine sites (as points) with 50 km buffers leading to 49.9 million km 2 of land potentially impacted (at ~799 km 2 per site). These comparisons show that variability in sampling and mapping methodology may lead to considerably different understandings of mine land use and impacts on landscapes. Each approach has benefits and drawbacks, e.g., it can be quite practical to use buffer tools to assign equal land area per site, as manual delineation efforts are considerably reduced 6 , 7 . However, this approach does not allow the complex boundaries of mines sites to be assessed.
The results of the MapBiomas initiative in Brazil 40 also highlight the effects of different mapping methodologies. Their inclusion of areas that might be termed mine “lands” (areas wherein mining activity is the primary land use) situated between and surrounding mine “features” (e.g., pits, waste rock disposal areas, tailings dams, etc) leads MapBiomas to report a total area of 2061 km 2 (lands) for Brazil (Table 3 ), versus 1395 km 2 (features) mapped in our study (Table 1 ). Additional visual comparisons between our data and that of MapBiomas are provided in supplementary Fig. S1 , highlighting these differences.
Collectively, these comparisons highlight that definitions of mining as mine features, or as broader areas wherein mining activity takes place, or yet even broader areas of impact can lead to considerable differences in total calculated area. These comparisons also highlight that our capacity to evaluate the accuracy of mapped mine areas will vary between countries, depending on the local availability of corroborating sources.
A further source of uncertainty is that mine areas are highly dynamic. A mine lifecycle may involve periods of expansion followed by periods of reclamation or revegetation. Considering the many challenges faced in restoring ecological function at former mine sites 41 , 42 , 43 , areas with vegetation cover may still be heavily impacted, raising questions as to whether such areas ought to still be classified as mining. Our visual inspection methods cannot detect such aspects. We are restricted to representing mine features to the extent that they are, or at least have recently been, visible in satellite imagery. Compounding these challenges, satellite imagery itself can have high temporal variability, such that the available imagery for a single mine can only be represented by a mosaic of multiple years. Depending on image availability at the time of analysis, this factor alone can lead to substantial differences between studies.
Under these caveats, our validation steps show that when a uniform mine area definition and delineation method are applied to the same image, 92% consistency in mapping of mine areas is achievable. This level of internal accuracy is consistent with past work 7 , 18 .
Broader environmental impact potential
Examining the intersection of mines with protected areas, we identified 2558 boundary violations, totaling ~6232 km 2 , or ~9.5% of all mine polygons. The potential impacts of these intersections is variable. For example, the largest intersection is a single 1355 km 2 area of the Atacama Desert designated as Ramsar wetland overlapping lithium brine operations. Such brine operations may encompass entire salt flats, but the direct influence of mining on wetlands can be unclear 44 . In other zones, such as in protected forests in Venezuela, visible traces of mine-associated deforestation indicate a much clearer attribution of impacts. Intersecting mine areas spanned 147 different types of protected area categories, including state and national parks, Ramsar wetlands, and World Heritage areas. A global map of these intersections is shown in supplementary Fig. S2 . These intersections raise concerns about the global environmental footprint of mining, yet they are compounded by the fact that mine areas can expand over time. Globally, about 58.1% of mine areas in our dataset are located in flat terrain areas 8 .
Mines also exert influence well beyond the boundaries delineated in this study. This distinction between direct land use, and broader areas of induced impact (particularly environmental impact) is an important one 45 , 46 . It has been found, for instance, that in the open-cut pit Grasberg gold/copper mine in West New Guinea, forest losses can be >42 times larger than the mine itself 47 . Artisanal gold mining in the Peruvian Amazon was identified to cause nearly 1000 km 2 of deforestation 46 . A recent study on mining-induced deforestation in tropical regions also finds 3264 km 2 of directly induced forest loss 22 .
To further illustrate the broader areas of impact arising from mining, we use the index R to express a ratio of an area of impact or influence to a mine area:
A Buffer zone refers to an extended region outside the mine area. By one measure, a 1 km buffer zone can broadly illustrate zones where the effects of a tailings pond collapse, the transportation of toxic elements, and/or immediate impacts on indigenous settlements, arable land, river quality, and transportation line are most notable 48 , 49 . Alternatively, a buffer zone of 50 km has also been argued as an appropriate measure of zones affected by mining activity 6 , 22 . These zones are by no means prescriptive, but can help to provide a sense of the scales of mine-associated impacts. To illustrate the effect of these assumptions in the context of our dataset, we adopted 1 and 50 km as the buffer distance to model the buffer zone variation from mine sites. The total (dissolved) buffer zone from 1 km is ~0.29 million km 2 , and ~24.5 million km 2 from a 50 km buffer distance (see supplementary Fig. S3 ). The 50 km buffer area in our database is approximately half that of the 49.9 million km 2 footprint estimated in Sonter et al. 6 . This is likely because our study does not consider pre-operational deposits without current visible footprints, and does not approximate results to 1 km 2 grid cells. This comparison highlights the considerable potential for footprint estimates to increase as new deposits are developed, and as existing sites expand.
Meanwhile, the R collectively decreases with the extent of the mine area (decreasing ratio of land use to perimeter), revealing that small-scale mining causes a comparatively larger zone of influence per unit of mine area. It follows that large-scale mining may achieve economies of scale that result in reduced overall impacts on land. For ASM, a 1 km buffer distance yields R = 7.80/km 2 mine area (8352.3 km 2 buffer area, 1071.2 km 2 mine area, 4058 mine polygons). Large-scale mining yields R = 1.09/km 2 mine area (25,491.7 km 2 buffer area, 23,334.3 km 2 mine area, 761 mine polygons).
Contributions and further work
This study has sought to consolidate our understanding of the global extent of mining activity through extensive mapping and analysis of recent satellite imagery. By incorporating additional areas and types of mining activity, we have substantially updated previous global mine land-use datasets that focus on the specific contour of mine features. However, despite these efforts, it is evident that additional mining activity still remains to be assessed. This is likely because (1) production is ongoing at many of the sites mapped, leading to an expected increase in the footprints of sites in our database, (2) exploration success will lead to the development of new sites, and (3) continued effort may yield further identification of unidentified sites. This is particularly so for mine areas operating under informal conditions, which we have primarily assessed on a case study basis, due to no complete global inventory of such sites available to guide our mapping. It was noted during validation that characteristically small aggregate quarry sites, particularly those operating in urban contexts, may also be underrepresented in our dataset. Given these challenges, a necessary future advancement will be to automate mapping processes through the development of advanced machine learning technology that can recognize mine features and appropriately classify them in satellite imagery, despite their visual and spectral heterogeneity. The database provided here may be an invaluable training dataset toward such an endeavor.
Materials and methods
This study demonstrates a replication of previously established methods for identifying and delineating mine areas in satellite imagery. These methods are described at length in refs. 3 , 7 , 8 , but are also expanded upon here.
Identification of mine sites
To identify the location of mine sites, we conducted an online literature review comprising published literature, government and industry publications, and corporate and national mining databases. In cases where coordinates of a mine or minefield were not provided (e.g., from sources such as mindat.org, or the SNL database), but qualitative descriptors were available (e.g., proximity to known landmarks), then visual inspection of satellite imagery was used to support site identification. As this study expands upon previous global mine area research, we also re-examined previously mapped mining regions to determine if global coverage could be improved or updated. Specifically, we identified additional sites by (1) using navigation tools in Google Earth Pro to scan imagery of likely locations adjacent to existing known features, and (2) expanding the analysis of countries previously underrepresented in past studies. This process was further aided by considering factors like potential adjacent coal mines for coke supply of iron smelters, or areas within surrounding zones of mineralization, such as faults (e.g., quartz-vein-type gold ore), orogenic belts (e.g., porphyry copper deposit), and sedimentary basins (e.g., coal mines), including a small proportion of unidentified waste disposal and dumping sites, deposits of surficial mines, cessation tailings ponds, and affiliated mineral processing facilities (see supplementary Fig. S4 for examples).
Prior studies have been limited in their spatial coverage by focusing primarily on regulated/formal mining activity, yet ~40–100 million people are estimated to depend on small-scale mining for their livelihoods 28 , 29 , indicating a potential for cumulatively large mine areas that require mapping. The recent update from Maus et al. 18 has also sought to address this. More ASM sites were identified in this study using high-resolution remote sensing images visible in Google Earth Pro and Sentinel II, particularly in Africa and Latin America (see ref. 50 ). A challenge of including such areas is that formal documentation of these sites is seldom available, adding uncertainty to the identification of such mine sites and limiting opportunities for verification/validation. We nonetheless identified sites where ASM features were highly recognizable, considering aspects like the structure, location, color, texture, composition, topography, mine type, local industry type, and evidence of pollutants. For example, for placer gold mining in Ofin River, Ghana, the turbid river water and the highly reflective areas along the river render mining activity in this region quite distinctive (see Fig. 1 ).
Delineation of mine feature polygons
Once mine site locations were known, polygons outlining mine features were drawn where recent and adequately well-defined Google Earth imagery was available, such that mine areas were possible to distinguish from their surrounds.
To delineate mine areas, the authors drew upon extensive experience in recognizing mine features in satellite imagery 3 , 4 , 8 , 9 . Although it is noted that a mine site can be composed of a diverse range of features, we focused primarily on delineating features that relate primarily to the core functioning of the sites, such as open cut pits, tunnel entrances, ore stockpiles, heap leach pads, waste rock disposal areas, infrastructure areas, tailings storage facilities and water ponds. This excludes downstream processing facilities such as smelters, refineries, unless they were also co-located with on-site milling/beneficiation infrastructure. We did not seek to delineate areas of impact arising from such features, for example gaseous of liquid wastes arising from such features, or secondary/tertiary land use changes emerging from the presence of a mine. Revegetated areas, backfilled pits, or pits subsequently employed for water storage were identified using historical imagery as well as public reports, such as news articles or company publications. We only delineated infrastructure areas that were clearly related to mineral production, therefore generally excluding features like roads and railways. In cases where mines operated close to built-up areas, this process entailed distinguishing milling infrastructure from surrounding buildings. Areas were delineated manually using polygon tools and publicly available imagery in Google Earth Pro. Depending on the location, this imagery varied in resolution. For example, some rural areas only receive coverage from SPOT satellites where resolution from 1.5 to 10 m is achieved. Ikonos imagery may cover other zones at 1 m resolution. Other services, including WorldView-1 to 3 and GeoEye-1 achieve a resolution of 0.5 m, and in some parts of Japan, North America and Europe, imagery is available from localized sources (e.g., aerial imagery) that achieve 0.15 m resolution. Assessing these images, we considered aspects like texture, color (e.g., reflectance of exposed rock versus surrounding vegetation), composition (positioning within the landscape), topography (determining whether the presence of such a feature makes sense given the surrounding terrain), light (e.g., identifying shadows cast from pits of rack of dumps) and overall form (recognizing familiar features as a whole) to manually draw boundaries. We aimed to maintain polygon boundaries that closely contoured the form of specific mine features, as seen in Fig. 1A . The location and global coverage of sites mapped in this study are shown in Fig. 1 . It includes both currently active mines and abandoned and historic mine sites 35 .
Historical satellite imagery available in Google Earth Pro was also assessed to cross-check areas, particularly if newer imagery was obscured by low resolution or cloud cover. This also enabled the identification and verification of some closed mine sites and closed tailings ponds in Japan, the United States, and Europe, where substantial mining activity is known to have taken place in the early- and mid-20th century. Many of these areas, notably some coal extraction areas in Germany, Poland, Belgium, and lead-zinc areas in Ireland, exhibited characteristics of processes of ecological restoration (Fig. 1 , supplementary Fig. S4 ). Some coal disposal areas in China are notable for their transition to agricultural land uses, presenting an increased risk of classification uncertainty (see ref. 3 ).
Where available, additional literature or imagery (e.g., company websites, aerial photographs, technical reports, or Landsat imagery) were examined to verify features that remained unclear to authors after initial cross-checking. Notwithstanding these efforts, the process of delineating mine areas is subject to inherent uncertainties such as incorrect interpretation/classification, temporal variation in the landscapes mapped, and precision of the satellite images (see ref. 3 ). The imagery assessed via Google Earth has an overall positional root mean squared error (RMSE) of 39.7 m related to the reality on the ground 51 , 52 , which has been considered for past global scale mine area analyses 8 . All polygons were converted to a projected coordinate system (WGS 1984 EASE Grid Global). Post-processing checks were conducted to identify invalid polygons (e.g., empty mine areas or distorted boundaries) and repair geometry to ensure polygons are accurate.
Additional data validation
To verify that mine areas were correctly delineated, we used ground photography and GPS coordinates obtained from recent and past field excursions in China (Panzhihua, and Ordos), Germany (Harz Mountain), and Australia (North-west Tasmania), and utilized an external reviewer to replicate and compare output maps.
The most recent available remote sensing imagery for mapping was used as a first preference, recognizing the potential for sites to grow over time (Fig. 5A ). The effects of a dynamically changing landscape, and hence issues of changing vegetation cover (either naturally or as part of concerted restoration efforts) obscuring operational boundaries are shown in (Fig. 5B ).
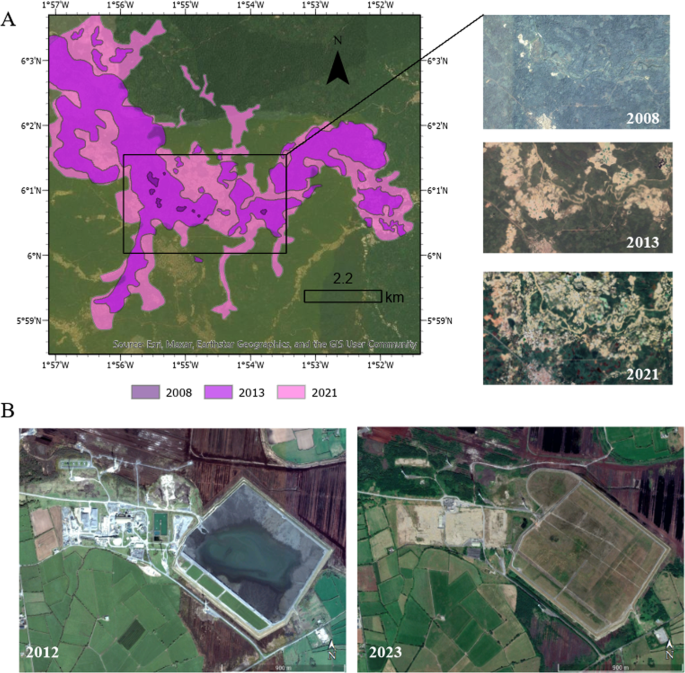
A Spatiotemporal variation of ASM areas (placer gold mining, Ofin River, Ghana 6°1’00” N, 1°52’27” W). Image © 2023 CNES/Airbus and Maxar Technologies, visualized in Google Earth Pro. B Cessation mine tailings in the Lisheen Mine, Ireland. This mine was operational in 2012 and increasing vegetation cover is evident in 2023 (lead-zinc-silver mine; 52°45’01” N, 7°40’35” W). Images © 2023 CNES/Airbus, visualized in Google Earth Pro.
We validated and updated mine area datasets of 24,605 polygons (33%) from Tang et al. 8 to match more recent imagery where available, and newly delineated 49,943 additional mine area polygons (66%) for the present study. The independent delineation of mine areas from an volunteer reviewer sought to represent different countries, climate divisions, and topography. The reviewer, Dr. Yang Jingsong, is familiar with the application of mine delineation methods as described here, and as used in the previous iteration of our database 8 . Their outputs are summarized in Table 4 and a visualization of their polygons in provided in supplementary Fig. S5 . We found that there was ~92% agreement between mapped areas, suggesting that our global estimates could vary in the order of ±8% through the propagation of human error. This also highlights that even the application of consistent delineation criteria cannot guarantee full replicability in total areas, however the overall location and magnitude of impacted areas remains broadly consistent.
Spatial analysis
Following delineation, ArcGIS 10.8 and ArcGIS Pro 2.4.0 programs were used to assess spatial attributes of the mine areas dataset and the concurrence of human and environmental overlays. Using the SNL database, we applied 10 km buffers (consistent with Maus et al. 7 , 18 ) to point locations of mine operations. We then performed a spatial overlay (intersection) analysis to identify the area of polygons that sit within these buffers, and hence determine the number and type of mine operations potentially represented by our dataset. The World Database on Protected Areas 53 , 54 was also downloaded to ArcGIS Pro 2.4.0, and a spatial overlay analysis was performed using a the spatial join and intersect tools to identify the extent of overlap between protected areas and mine polygons. This allowed us to determine the extent that mining may induce impacts on environmentally sensitive regions.
The Global and Local Moran’s I indices were employed to examine the spatial distribution characteristics of the mine areas. The Anselin Moran’s I is a commonly used indicator of spatial autocorrelation (clustering behavior) based on the Pearson correlation coefficient. The Global Moran’s I index is used to study the degree of autocorrelation for the dataset as a whole, while the local indicators of the spatial association are applied to identify specific locations that exhibit different clustering behaviors 55 . Such areas may then be visualized via GIS 56 . The test employed 9999 permutations in this study, with p < 0.05. A strong positive Local Moran’s I value indicates that the location under study has similarly high or low values to its neighbors. Conversely, a strong negative Local Moran’s I value implies that the sites under investigation are significantly different from the values of their surrounding locations, indicating areas of unusually high or low clustering of mine areas relative to their surrounds. The global spread of mining activity can be distinguished according to statistically significant clusters of: high density (HH), low density (LL), outliers of high density surrounded by low density (HL), and outliers of low density surrounded by high density (LH). Further explanations of these categories are provided by 55 and 57 . A ‘Not Significant’ (NS) classification represents highly isolated or completely absent mining, and is evident across many high altitude, alpine, and arid regions (e.g., Central Asian Orogenic Belt, Andes Belt, Northern Europe). LL regions are often associated with higher population density, hence minimizing land available for extraction. Agricultural land, transportation lines, and topographical restrictions may also artificially subdivide a minefield into multiple, lower-density mine sections. Such regions include parts of Eastern China (e.g., polymetallic metal mining), Western USA (e.g., with some coal mining in the Appalachian Mountains), India (e.g., iron ore mining), and the east coast of Brazil (e.g., iron ore mining). HL zones are situated within the LL pattern boundaries or NS areas. They represent high density mining activity with statistically low-density surrounds. Zones of significant mine area density, surrounded by yet more significant mining activity constitute a HH cluster. These regions were characteristically lower population density. Examples of such areas included Western Australia (e.g., Tropicana gold mine), Queensland, Australia (e.g., Mount Isa copper, lead, zinc, and silver mine), Magadanskaya Oblast, Russia (gold), Chukotskiy Avtonomnyy Okrug, Russia (gold, diamond) and Bolivia (San Cristóbal silver, lead and zinc mine). Within HH areas, we may identify some LH regions of notably low mine site density. Such areas may feature within highly mineralized belts, such as the Andes, where mine areas appear in relative abundance, yet geographical factors like steep mountain ridges prevent parts of these belts from being developed.
Data availability
The spatial data that support the findings of this study are available from https://doi.org/10.5281/zenodo.6806817 .
Change history
11 may 2023.
A Correction to this paper has been published: https://doi.org/10.1038/s43247-023-00831-4
Bebbington, A., Hinojosa, L., Bebbington, D. H., Burneo, M. L. & Warnaars, X. Contention and ambiguity: Mining and the possibilities of development. Dev. Change 39 , 887–914 (2008).
Article Google Scholar
Mudd G. M., et al. Critical Minerals in Australia: A Review of Opportunities and Research Needs. (Geoscience Australia, Canberra, 2019). https://doi.org/10.11636/Record.2018.051 .
Werner, T. T., Mudd, G. M., Schipper, A. M., Huijbregts, M. & Northey, S. A. Global-scale remote sensing of mine areas and analysis of factors explaining their extent. Global Environ. Change 60 https://doi.org/10.1016/j.gloenvcha.2019.102007 (2020).
Werner, T. T. A Geospatial Database for Effective Mine Rehabilitation in Australia. Minerals 10 , 745 (2020).
Article CAS Google Scholar
Sonter, L. J. et al. Mining drives extensive deforestation in the Brazilian Amazon. Nat. Commun. 8 , 1–7 (2017).
Sonter, L. J., Dade, M. C., Watson, J. E. M. & Valenta, R. K. Renewable energy production will exacerbate mining threats to biodiversity. Nat. Commun. 11 , 4174 (2020).
Maus, V. & Giljum, S. a global-scale data set of mining areas. Sci. Data https://doi.org/10.1038/s41597-020-00624-w (2020).
Tang, L., Tim, T. W., Xie, H., Yang, J. & Shi, Z. A global-scale spatial assessment and geodatabase of mine areas. Global Planetary Change 204 , 103578 (2021).
Tang, L., Liu, X., Wang, X., Liu, S. & Deng, H. Statistical analysis of tailings ponds in China. J. Geochem. Explor. 216 , 106579 (2020).
Asner, G. P., Llactayo, W., Tupayachi, R. & Luna, E. R. Elevated rates of gold mining in the Amazon revealed through high-resolution monitoring. Proc. Natl Acad. Sci. USA 110 , 18454–18459 (2013).
Northey, S. A. et al. The exposure of global base metal resources to water criticality, scarcity and climate change. Global Environ. Change 44 , 109–124 (2017).
Sonter, L. J., Ali, S. H. & Watson, J. E. M. Mining and biodiversity: key issues and research needs in conservation science. Proc. Biol. Sci. 285 , 20181926 (2018).
Google Scholar
S&P Global Market Intelligence. SNL Metals and Mining Database. https://www.spglobal.com/marketintelligence/en/campaigns/metals-mining . (2018).
Mudd, G. M. Assessing the Availability of Global Metals and Minerals for the Sustainable Century: From Aluminium to Zirconium. Sustainability 13 , 10855 (2021).
Mudd, G. M. & Jowitt, S. M. Growing Global Copper Resources, Reserves and Production: Discovery Is Not the Only Control on Supply. Econ. Geol. 113 , 1235–1267 (2018).
Yang, Y. et al. Detecting the dynamics of vegetation disturbance and recovery in surface mining area via Landsat imagery and LandTrendr algorithm. J. Cleaner Product. 178 , 353–362 (2018).
Yu, X., Zhang, K. & Zhang, Y. Land use classification of open-pit mine based on multi-scale segmentation and random forest model. PLOS ONE 17 , e0263870 (2022).
Maus, V. et al. An update on global mining land use. Sci. Data 9 , 433 (2022).
Barenblitt, A. et al. The large footprint of small-scale artisanal gold mining in Ghana. Sci. Total Environ. 781 , 146644 (2021).
ESRI. Create Fishnet (Data Management) , https://pro.arcgis.com/en/pro-app/latest/tool-reference/data-management/create-fishnet.htm (2022).
Butt, N. et al. Conservation. Biodiversity risks from fossil fuel extraction. Science 25 , 425–426 (2013).
Giljum, S. et al. A pantropical assessment of deforestation caused by industrial mining. Proc. Natl Acad. Sci. 119 , e2118273119 (2022).
Walsh, S. D., Northey, S. A., Huston, D., Yellishetty, M. & Czarnota, K. Bluecap: A geospatial model to assess regional economic-viability for mineral resource development. Resourc. Policy 66 , 101598 (2020).
Huo, X.-N. et al. Combining Geostatistics with Moran’s I Analysis for Mapping Soil Heavy Metals in Beijing, China. Int. J. Environ. Res. Public Health 9 https://doi.org/10.3390/ijerph9030995 (2012).
Wu, Y., Chen, J., Zheng, C., Wei, F. & Adriano, D. C. Background concentrations of elements in soils of China. Water Air Soil Pollut. 57-58 , 699–712 (1991).
Hentschel, T., Hruschka, F. & Priester, M. 2002. Global report on artisanal and small-scale mining. Report commissioned by the Mining, Minerals and Sustainable Development of the International Institute for Environment and Development. http://www.iied.org/mmsd/mmsd_pdfs/asm_global_report_draft_jan02.pdf , 20 (08), 2008.
Siqueira-Gay, J. & Sánchez, L. E. The outbreak of illegal gold mining in the Brazilian Amazon boosts deforestation. Region. Environ. Change 21 , 28 (2021).
Lottermoser, B. G. Mine Wastes. Characterization, Treatment and Environmental Impacts https://doi.org/10.1007/978-3-642-12419-8 (2010).
Kossoff, D. et al. Mine tailings dams: Characteristics, failure, environmental impacts, and remediation. Appl. Geochem. 51 , 229–245 (2014).
Kaninga, B. K. et al. Review: mine tailings in an African tropical environment—mechanisms for the bioavailability of heavy metals in soils. Environ. Geochem. Health 42 , 1069–1094 (2020).
Shandro, J. A., Veiga, M. M. & Chouinard, R. Reducing mercury pollution from artisanal gold mining in Munhena, Mozambique. J. Cleaner Product. 17 , 525–532 (2009).
Hilson, G. Abatement of mercury pollution in the small-scale gold mining industry: Restructuring the policy and research agendas. Sci. Total Environ. 362 , 1–14 (2006).
Drace, K. et al. Mercury-free, small-scale artisanal gold mining in Mozambique: utilization of magnets to isolate gold at clean tech mine. J. Cleaner Product. 32 , 88–95 (2012).
Malpeli, K. C. & Chirico, P. G. The influence of geomorphology on the role of women at artisanal and small-scale mine sites. Nat. Resourc. Forum 37 , 43–54 (2013).
Li, X., Yang, F., Peng, X., Li, H. & Tang, L. Risk assessment of small scale mining waste dump: a case study of Jialing River Basin (In Chinese). Resourc. Environ. Yangtze Basin 30 , 2755–2762 (2021).
Nádudvari, Á. et al. Self-Heating Coal Waste Fire Monitoring and Related Environmental Problems: Case Studies from Poland and Ukraine. J. Environ. Geogr. 14 , 26–38 (2021).
Ofosu, G., Dittmann, A., Sarpong, D. & Botchie, D. Socio-economic and environmental implications of Artisanal and Small-scale Mining (ASM) on agriculture and livelihoods. Environ. Sci. Policy 106 , 210–220 (2020).
Banchirigah, S. M. Challenges with eradicating illegal mining in Ghana: A perspective from the grassroots. Resourc. Policy 33 , 29–38 (2008).
Iwatsuki, Y., Nakajima, K., Yamano, H., Otsuki, A. & Murakami, S. Variation and changes in land-use intensities behind nickel mining: Coupling operational and satellite data. Resourc. Conser. Recycling 134 , 361–366 (2018).
Azevedo Sr, T., Souza Jr, C., Shimbo, J. & Alencar, A. MapBiomas initiative: Mapping annual land cover and land use changes in Brazil from 1985 to 2017. American Geophysical Union, Fall Meeting 2018, Washington D.C., USA, Dec 10-14, 2018. Abstract #B22A-04 AGU Fall Meeting Abstracts .
Rosa, J. C., Morrison‐Saunders, A., Hughes, M. & Sánchez, L. E. Planning mine restoration through ecosystem services to enhance community engagement and deliver social benefits. Restor. Ecol. 28 , 937–946 (2020).
Li, M. Ecological restoration of mineland with particular reference to the metalliferous mine wasteland in China: a review of research and practice. Sci. Total Environ. 357 , 38–53 (2006).
Chen, Z. et al. Ecological restoration in mining areas in the context of the Belt and Road initiative: Capability and challenges. Environ. Impact Assess. Rev. 95 , 106767 (2022).
Moran, B. J. et al. Relic groundwater and prolonged drought confound interpretations of water sustainability and lithium extraction in arid lands. Earth’s Future 10 , e2021EF002555 (2022).
Asner, G. P. & Tupayachi, R. Accelerated losses of protected forests from gold mining in the Peruvian Amazon. Environ. Res. Lett. 12 , 094004 (2017).
Caballero Espejo, J. et al. Deforestation and Forest Degradation Due to Gold Mining in the Peruvian Amazon: A 34-Year Perspective. Remote Sensing 10 , 1903 (2018).
Alonzo, M., Van Den Hoek, J. & Ahmed, N. Capturing coupled riparian and coastal disturbance from industrial mining using cloud-resilient satellite time series analysis. Sci. Rep. 6 , 35129 (2016).
Wang, K. et al. Integration of DSM and SPH to Model Tailings Dam Failure Run-Out Slurry Routing Across 3D Real Terrain. Water 10 https://doi.org/10.3390/w10081087 (2018).
Kun, W., Peng, Y., Hudson-Edwards, K., Wen-sheng, L. & Lei, B. Status and development for the prevention and management of tailings dam failure accidents. Chinese J. Eng. 40 , 526–539 (2018).
Djibril, K. N. G. et al. Artisanal gold mining in Batouri area, East Cameroon: Impacts on the mining population and their environment. J. Geol. Mining Res. 9 , 1–8 (2017).
Potere, D. Horizontal Positional Accuracy of Google Earth’s High-Resolution Imagery Archive. Sensors 8 , 7973–7981 (2008).
Olofsson, P. et al. Good practices for estimating area and assessing accuracy of land change. Remote Sensing Environ. 148 , 42–57 (2014).
Hanson, J. O. wdpar: Interface to the World Database on Protected Areas. J. Open Source Softw. 7 , 4594 (2022).
Bingham, H. C. et al. Sixty years of tracking conservation progress using the World Database on Protected Areas. Nat. Ecol. Evol. 3 , 737–743 (2019).
Anselin, L. Local Indicators of Spatial Association-LISA. Geogr. Analys. 27 , 93–115 (1995).
Harries, K. Extreme spatial variations in crime density in Baltimore County, MD. Geoforum 37 , 404–416 (2006).
Mitchel, A. The ESRI Guide to GIS analysis, Volume 2: Spartial measurements and statistics. ESRI Guide to GIS analysis (2005). ISBN:9781589486089, 1589486080
Li, X.-y., Yang, F., Xiu-hong, P., Li, H.-l. & Tang, L. Security Assessment of Mining Wastes in Jialing River Basin-Indicative Significance for Small-scale Storage Yards (in Chinese). Resour. Environ. Yangtze Basin 30 , 2755–2762 (2021).
Peel, M. C., Finlayson, B. L. & McMahon, T. A. Updated world map of the Köppen-Geiger climate classification. Hydrol. Earth Syst. Sci. 11 , 1633–1644 (2007).
Sayre, R. et al. A New High-Resolution Map of World Mountains and an Online Tool for Visualizing and Comparing Characterizations of Global Mountain Distributions. Mountain Res. Dev. 38 , 240–249 (2018).
Download references
Acknowledgements
Dr. Tim Werner was supported by an Australian Research Council Discovery Early Career Researcher Award: ‘Mapping resources, demands and constraints to critical metal supplies’ (Grant DE220101153).
Author information
Authors and affiliations.
College of Earth Sciences, Chengdu University of Technology, 610059, Chengdu, China
School of Geography, Earth and Atmospheric Sciences, Faculty of Science, The University of Melbourne, Carlton, VIC, Australia
- Tim T. Werner
You can also search for this author in PubMed Google Scholar
Contributions
L.T.: Conceptualization, Methodology, Investigation, Visualization, Writing—original draft. T.W.: Methodology, Investigation, Visualization, Supervision, Writing—original draft, Writing—review & editing.
Corresponding author
Correspondence to Tim T. Werner .
Ethics declarations
Competing interests.
The authors declare no competing interests.
Peer review
Peer review information.
Communications Earth & Environment thanks Luis Enrique Sanchez, Susmita Sharma and the other, anonymous, reviewer(s) for their contribution to the peer review of this work. Primary Handling Editors: Sadia Ilyas and Joe Aslin.
Additional information
Publisher’s note Springer Nature remains neutral with regard to jurisdictional claims in published maps and institutional affiliations.
Supplementary information
Supplementary information, rights and permissions.
Open Access This article is licensed under a Creative Commons Attribution 4.0 International License, which permits use, sharing, adaptation, distribution and reproduction in any medium or format, as long as you give appropriate credit to the original author(s) and the source, provide a link to the Creative Commons license, and indicate if changes were made. The images or other third party material in this article are included in the article’s Creative Commons license, unless indicated otherwise in a credit line to the material. If material is not included in the article’s Creative Commons license and your intended use is not permitted by statutory regulation or exceeds the permitted use, you will need to obtain permission directly from the copyright holder. To view a copy of this license, visit http://creativecommons.org/licenses/by/4.0/ .
Reprints and permissions
About this article
Cite this article.
Tang, L., Werner, T.T. Global mining footprint mapped from high-resolution satellite imagery. Commun Earth Environ 4 , 134 (2023). https://doi.org/10.1038/s43247-023-00805-6
Download citation
Received : 06 September 2022
Accepted : 14 April 2023
Published : 22 April 2023
DOI : https://doi.org/10.1038/s43247-023-00805-6
Share this article
Anyone you share the following link with will be able to read this content:
Sorry, a shareable link is not currently available for this article.
Provided by the Springer Nature SharedIt content-sharing initiative
This article is cited by
Impacts for half of the world’s mining areas are undocumented.
- Victor Maus
Nature (2024)
Chemical weathering profile in the V–Ti–Fe mine tailings pond: a basalt-weathering analog
- Xiaolin Zhang
- Yinger Deng
- Jinsong Yang
Acta Geochimica (2023)
By submitting a comment you agree to abide by our Terms and Community Guidelines . If you find something abusive or that does not comply with our terms or guidelines please flag it as inappropriate.
Quick links
- Explore articles by subject
- Guide to authors
- Editorial policies
Sign up for the Nature Briefing: Anthropocene newsletter — what matters in anthropocene research, free to your inbox weekly.

Our approach
- Responsibility
- Infrastructure
- Try Meta AI
COMPUTER VISION
Imagine flash: accelerating emu diffusion models with backward distillation.
April 18, 2024
Diffusion models are a powerful generative framework, but come with expensive inference. Existing acceleration methods often compromise image quality or fail under complex conditioning when operating in an extremely low-step regime. In this work, we propose a novel distillation framework tailored to enable high-fidelity, diverse sample generation using just one to three steps. Our approach comprises three key components: (i) Backward Distillation, which mitigates training-inference discrepancies by calibrating the student on its own backward trajectory; (ii) Shifted Reconstruction Loss that dynamically adapts knowledge transfer based on the current time step; and (iii) Noise Correction, an inference time technique that enhances sample quality by addressing singularities in noise prediction. Through extensive experiments, we demonstrate that our method outperforms existing competitors in quantitative metrics and human evaluations. Remarkably, it achieves performance comparable to the teacher model using only three denoising steps, enabling efficient high-quality generation.
Jonas Kohler
Albert Pumarola
Edgar Schoenfeld
Artsiom Sanakoyeu
Roshan Sumbaly
Peter Vajda
Research Topics
Computer Vision
Related Publications
March 20, 2024
SceneScript: Reconstructing Scenes With An Autoregressive Structured Language Model
Armen Avetisyan , Chris Xie , Henry Howard-Jenkins , Tsun-Yi Yang , Samir Aroudj , Suvam Patra , Fuyang Zhang , Duncan Frost , Luke Holland , Campbell Orme , Jakob Julian Engel , Edward Miller , Richard Newcombe , Vasileios Balntas

February 13, 2024
IM-3D: Iterative Multiview Diffusion and Reconstruction for High-Quality 3D Generation
Luke Melas-Kyriazi , Iro Laina , Christian Rupprecht , Natalia Neverova , Andrea Vedaldi , Oran Gafni , Filippos Kokkinos
January 25, 2024
LRR: Language-Driven Resamplable Continuous Representation against Adversarial Tracking Attacks
Felix Xu , Di Lin , Jianjun Zhao , Jianlang Chen , Lei Ma , Qing Guo , Wei Feng , Xuhong Ren
December 08, 2023
Learning Fine-grained View-Invariant Representations from Unpaired Ego-Exo Videos via Temporal Alignment
Sherry Xue , Kristen Grauman

Help Us Pioneer The Future of AI
We share our open source frameworks, tools, libraries, and models for everything from research exploration to large-scale production deployment..
Product experiences
Foundational models
Latest news
Meta © 2024
Content Based Image Retrieval Using Data Mining Techniques
Ieee account.
- Change Username/Password
- Update Address
Purchase Details
- Payment Options
- Order History
- View Purchased Documents
Profile Information
- Communications Preferences
- Profession and Education
- Technical Interests
- US & Canada: +1 800 678 4333
- Worldwide: +1 732 981 0060
- Contact & Support
- About IEEE Xplore
- Accessibility
- Terms of Use
- Nondiscrimination Policy
- Privacy & Opting Out of Cookies
A not-for-profit organization, IEEE is the world's largest technical professional organization dedicated to advancing technology for the benefit of humanity. © Copyright 2024 IEEE - All rights reserved. Use of this web site signifies your agreement to the terms and conditions.
- Computer Vision
- Federated Learning
- Reinforcement Learning
- Natural Language Processing
- New Releases
- AI Dev Tools
- Advisory Board Members
- 🐝 Partnership and Promotion
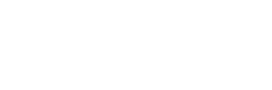
Researchers from Google DeepMind and Google Research have introduced the Gecko framework, designed to significantly refine the evaluation process of T2I models. Unique to Gecko is its use of a QA-based auto-evaluation metric, which correlates more accurately with human judgments than prior metrics. This approach allows for a nuanced assessment of how well images align with textual prompts, making it possible to identify specific areas where models excel or fail.
The methodology behind the comprehensive Gecko framework involves rigorous testing of T2I models using the extensive Gecko2K dataset, which includes the Gecko(R) and Gecko(S) subsets. Gecko(R) ensures broad evaluation coverage by sampling from well-established datasets like MSCOCO, Localized Narratives, and others. Conversely, Gecko(S) is meticulously designed to test specific sub-skills, enabling focused assessments of models’ abilities in nuanced areas such as text rendering and action understanding. Models such as SDXL, Muse, and Imagen are evaluated against these benchmarks using a set of over 100,000 human annotations, ensuring the evaluations reflect accurate image-text alignment.
The Gecko framework demonstrated its efficacy with quantitative improvements over previous models in rigorous testing. For example, Gecko achieved a correlation improvement of 12% compared to the next best metric when matched against human judgment ratings across multiple templates. Detailed analysis showed that specific model discrepancies were detected under Gecko with an 8% higher accuracy in image-text alignment. Additionally, in evaluations across a dataset of over 100,000 annotations, Gecko reliably enhanced model differentiation, reducing misalignments by 5% compared to standard benchmarks, confirming its robust capability in assessing T2I generation accuracy.
To conclude, the research introduces Gecko, an innovative QA-based evaluation metric and a comprehensive benchmarking system that significantly enhances the accuracy of T2I model evaluations. Gecko represents a substantial advancement in evaluating generative models by achieving a closer correlation with human judgments and providing detailed insights into model capabilities. This research is crucial for future developments in AI, ensuring that T2I technologies produce more accurate and contextually appropriate visual content, thus improving their applicability and effectiveness in real-world scenarios.
Check out the Paper . All credit for this research goes to the researchers of this project. Also, don’t forget to follow us on Twitter . Join our Telegram Channel , Discord Channel , and LinkedIn Gr oup .
If you like our work, you will love our newsletter..
Don’t Forget to join our 40k+ ML SubReddit
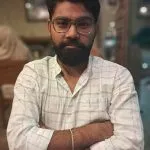
Nikhil is an intern consultant at Marktechpost. He is pursuing an integrated dual degree in Materials at the Indian Institute of Technology, Kharagpur. Nikhil is an AI/ML enthusiast who is always researching applications in fields like biomaterials and biomedical science. With a strong background in Material Science, he is exploring new advancements and creating opportunities to contribute.
- Nikhil https://www.marktechpost.com/author/nikhil0980/ This AI Paper from Google DeepMind Introduces Enhanced Learning Capabilities with Many-Shot In-Context Learning
- Nikhil https://www.marktechpost.com/author/nikhil0980/ How Scientific Machine Learning is Revolutionizing Research and Discovery
- Nikhil https://www.marktechpost.com/author/nikhil0980/ DeepMind Researchers Propose Naturalized Execution Tuning (NExT): A Self-Training Machine Learning Method that Drastically Improves the LLM's Ability to Reason about Code Execution
- Nikhil https://www.marktechpost.com/author/nikhil0980/ CATS (Contextually Aware Thresholding for Sparsity): A Novel Machine Learning Framework for Inducing and Exploiting Activation Sparsity in LLMs
RELATED ARTICLES MORE FROM AUTHOR
This ai paper from apple introduces a weakly-supervised pre-training method for vision models using publicly available web-scale image-text data, cleanlab introduces the trustworthy language model (tlm) that addresses the primary challenge to enterprise adoption of llms: unreliable outputs and hallucinations, mistral.rs: a lightning-fast llm inference platform with device support, quantization, and open-ai api compatible http server and python bindings, this machine learning paper from icmc-usp, nyu, and capital-one introduces t-explainer: a novel ai framework for consistent and reliable machine learning model explanations, from lost to found: information-intensive (in2) training revolutionizes long-context language understanding, this ai paper from google deepmind introduces enhanced learning capabilities with many-shot in-context learning, this ai paper from apple introduces a weakly-supervised pre-training method for vision models using..., cleanlab introduces the trustworthy language model (tlm) that addresses the primary challenge to enterprise..., mistral.rs: a lightning-fast llm inference platform with device support, quantization, and open-ai api compatible..., this machine learning paper from icmc-usp, nyu, and capital-one introduces t-explainer: a novel ai..., meet openlayer: an ai evaluation tool that fits into development and production pipelines to..., top artificial intelligence ai courses for beginners in 2024, lmsys org introduces arena-hard: a data pipeline to build high-quality benchmarks from live data..., this ai paper proposes flora: a novel machine learning approach that leverages federated learning....
- AI Magazine
- Privacy & TC
- Cookie Policy
🐝 FREE AI Webinar: Meet Llama 3: Run Locally with More Power, Less Cost, Full Privacy [April 30, 2024]
Thank You 🙌
Privacy Overview
Best Development Upgrades in Manor Lords
Prioritise these Development Upgrades for the best possible start in Manor Lords
What are the best Development Upgrades in Manor Lords? If you've progressed to the point in Manor Lords where you've reached a new Settlement Level, you'll likely already have seen the game's Development Upgrade tech tree, accessible by clicking on the name of your town at the top of the screen. Here you can spend Development Points to unlock key passive benefits for your entire settlement.
If you're unsure where to start within this tech tree, never fear. There are plenty of good options here, but the very best Development Upgrades are the ones we'll reveal to you below. So if you want the perfect start in Manor Lords, keep reading.
What are Development Upgrades in Manor Lords?
Development Upgrades are essentially your tech tree in Manor Lords. You can access your Development Upgrades at any time by clicking on the name of your settlement at the top of the screen. Your Development Upgrades are divided into four sectors:
- Top: Farming
- Right: Trade
- Bottom: Industry
- Left: Gathering
Each new Development Upgrade costs 1 Development Point. You earn a new Development Point each time you reach a new Settlement Level in one of your settlements. A single settlement making its way from a Small Village to a Large Town will net you a total of 5 Development Points. So if you want to unlock new Development Upgrades, you need to focus on building and upgrading your Burgage Plots.
As of the current early access version, there are 21 Development Upgrades in Manor Lords. All of them are useful in different ways, but there are a few absolute essential upgrades to prioritise. Here are the Development Upgrades we think are the best at the moment in Manor Lords:
- Trade Logistics (Trade)
- Better Deals (Trade)
- Deep Mining (Industry)
- Trapping (Gathering)
- Heavy Plow (Farming)
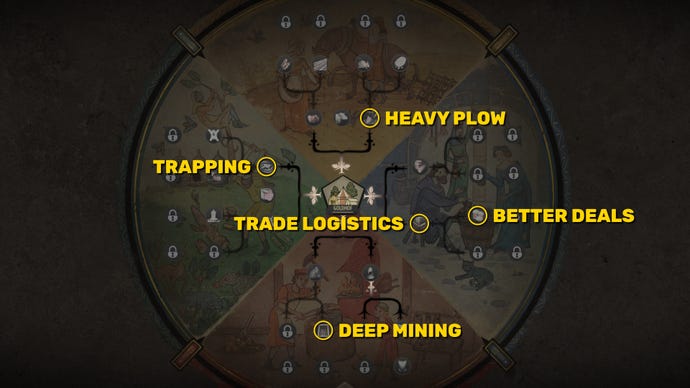
Trade Logistics
Description: Establishing a new Trade Route always costs a maximum of 25 Regional Wealth.
Trade Logistics is always my first Development Upgrade in any game, because establishing profitable trade routes is the most important thing that the tech tree can help with in the early-game. Setting up trade routes at a Trading Post can be astonishingly expensive (for example, 576 for Plate Armor), but this Development Upgrade will cap all those costs to a maximum of 25 Regional Wealth . Plus, it's a required tech for potentially an even more important Development Upgrade: Better Deals.
Better Deals
Description: Removes the tariff from foreign imports, effectively reducing all import prices by 10.
Better Deals has got to be the best Development Upgrade in Manor Lords right now. Suddenly, it only costs 2 Regional Wealth to import Eggs, whereas before it cost 12. This is perhaps most important if you need to quickly import equipment for your armies . It's very hard to furnish an army of 20 men with Polearms when each Polearm costs 18 Regional Wealth. At 8 Regional Wealth, it's a whole lot easier.
Deep Mining
Description: Enables the Deep Mine upgrade to Mining Pits to extract resources indefinitely if placed over a Rich Deposit.
The Deep Mining Development Upgrade is vital for mid-to-late-game settlements in Manor Lords, because it essentially turns finite resources into infinite resources. Upgrade a Mining Pit into a Deep Mine over your Rich Iron Deposit, and that mine can keep churning out Iron Ore forever . It's one of the Development Upgrades with the longest-lasting effects on your entire settlement.
Description: Enables hunters to skillfully lay traps in the forest, which give a passive income of Meat.
Trapping is a great early-game Development Upgrade, and usually the first thing I research after unlocking Better Deals. Just like Deep Mining, it basically turns a finite resource (Meat from Wild Animal deposits) into an infinite resource (Meat from traps anywhere in the forest). It's a great benefit especially for food in the early-game, because often Food is the bottleneck that will prevent you from growing your Population to massive levels.
Description: Enables employing Oxen at the Farmhouse for significantly faster plowing of large fields, as well as bringing crops back to storage more efficiently.
Heavy Plow is definitely the best Development Upgrade in the Farming sector at the moment. Farming is very important, but it's a slow process (which is why I'd recommend investing your first couple of Development Upgrades into Trade instead). The Plowing Station upgrade for your Farmhouse is a game-changer, allowing you to leverage your powerful Oxen to dramatically speed up the entire farming process. Essential for large farms.
That wraps up this guide on the best Development Upgrades in Manor Lords. If you're looking for more tips to make your settlement as strong as can be, check out our other guides on how to increase Influence , Regional Wealth , and Treasury . Or if you're after particular resources, we've got you covered with our guides on Leather , Sheep , Ale , Clothes , and Herbs .
Read this next
- Manor Lords publisher reassures players struggling to buy at launch that "we'll run those 25% discounts often"
- Manor Lords dev staves off early access disappointment by explaining his game isn't here to murder Total War
- Manor Lords is Total War, Crusader Kings and Age of Empires in a blender, and it's headed to Game Pass
Past Wordle answers
Wordle hint and answer today #1045 (April 29 2024)
Today's NYT Connections hint and answers (Mon, Apr 29)
NYT Connections hint and answers (Sun, Apr 28)
Wordle hint and answer #1044 (April 28 2024)
NYT Connections hint and answers (Sat, Apr 27)
Wordle hint and answer #1043 (April 27 2024)
How to make weapons and armor in Manor Lords
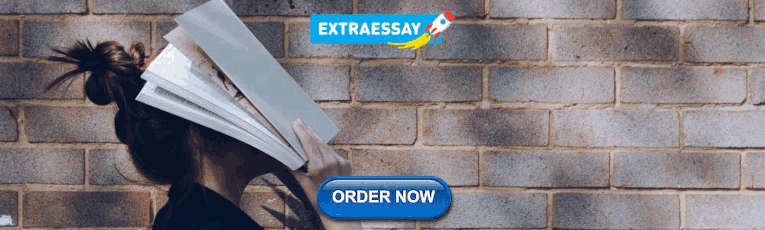
IMAGES
VIDEO
COMMENTS
fields cited above, research in image mining is still in its infancy. In this paper, we will examine the research issues in image mining, current developments in image mining, particularly, image mining frameworks, state-of-the-art techniques and systems. We will also identify some future research directions for image mining at the end of this ...
Efficient image searching, browsing and retrieval tools are required by users from various domains, including remote sensing, fashion, crime prevention, publishing, medicine, in architecture, etc. Current research in image mining is still in its infancy. In this paper, we tried to focus on current developments in image mining, particularly ...
It is a mathematical method for identifying patterns in enormous amounts of data. Image mining is an important data mining technique involving a variety of fields. In image mining, art image organization is an interesting research field worthy of attention. The classification of art images into several predetermined sets is referred to as art ...
Y et, image processing stays a. domain where humans still can outperform computer. This paper aims at re vie wing the steps of image mining, the most often utilised techniques for the individual ...
Image mining involves extracting patterns from extensive collections of images, whereas image processing techniques involve extracting features from a single image. In the view of increasing underwater image research papers, finding meaningful publications for researchers by normal search process is considered a big challenge and time-consuming ...
This paper will examine the research issues in image mining, current developments in imagemining, particularly, image mining frameworks, state-of-the-art techniques and systems, and identify some future research directions for image mining. Advances in image acquisition and storage technology have led to tremendous growth in very large and detailed image databases. These images, if analyzed ...
This paper examines the research issues in image mining, current developments in imagemining, particularly, image mining frameworks, state-of-the-art techniques and systems, and some future research directions for image mining. Advances in image acquisition and storage technology have led to tremendous growth in significantly large and detailed image databases. These images, if analyzed, can ...
In recent years, a striking increase in the number of papers published on image mining occurred. In fact, especially in 2017 and 2018, 66 (22%) and 131 (44%) articles, respectively, were published. ... The translation of image mining research in the clinical arena is limited by the huge variability of the methods used for image analysis ...
Image mining is more than just an extension of data mining to image domain. It is an interdisciplinary endeavor that draws upon expertise in computer vision, image processing, image retrieval, data mining, machine learning, database, and artificial intelligence. In this paper, we will examine the research issues in image mining, current ...
Valuable information can be hidden in images. The need for image mining is high in view of the fast growing amounts of image data. In this paper, we first point out unique characteristics of image mining, then analyze the overall process and discuss the main technology of image mining, namely, image classification and clustering, association rule mining. Some applications in various areas are ...
The use of multimedia data such as images, audio and video etc in daily life led to a huge amount of images in fairly large image databases. Nowadays, the image becomes a focal source of information because it hides a precious knowledge. In Image Mining, the aim is to discover this knowledge and gives the relevant information or patterns that are presented. This paper is dedicated to a review ...
The main technology of image mining is discussed, namely, image classification and clustering, association rule mining, some applications in various areas are introduced and some future research directions and problems of imagemining are presented. Valuable information can be hidden in images. The need for image mining is high in view of the fast growing amounts of image data. In this paper ...
Abstract: Image mining refers to a data mining technique where images are. used as data. It supports a large field of applications like medical diagnosis, agriculture, industrial work, space ...
gence (AI) era, we propose an image mining procedure that combines the usage of machine learning image recognition tools and network analysis frameworks to overcome this obstacle. This paper aims to exploit large-scale visual content publicly available online and investigate its inuence on the viewer's behavior. To do so, we collect and process a
This review paper provides a comprehensive overview of recent advancements, methodologies, challenges, and applications in image mining. It explores fundamental concepts such as preprocessing, feature extraction, representation, classification, and association rule mining. Furthermore, it discusses the diverse applications of image mining ...
The main intent of the image mining is to remove the data loss and obtain the meaningful information which is expected need of human. Bioinformatics Research, 2011 In this paper most of image mining techniques have been discussed. These all techniques have their own advantages and disadvantages. For particular International ,
Image mining is a development potential technology for data mining which involves in multiple disciplines, it is also a challenging field which extends traditional data mining from structured data to unstructured data such as image data. The paper introduces the concepts, mining models and mining technology related with image mining. Although ...
This paper aims at reviewing the steps of image mining, the most often utilised techniques for the individual sub-processes of IM and at identifying the major current issues and challenges in image mining. II. IMAGE ANALYSIS Image analysis is an inevitable step of image Mining. The analysis is often said to be a pre-processing stage of the ...
Image mining is the concept used to extract implicit and useful data from images stored in the large data bases. Image mining is used in variety of fields like medical diagnosis, space research, remote sensing, agriculture, industries and even in the educational field. Valuable information can be hidden in images.
The manuscript identifies seven text mining methods and describes their approaches, processes, techniques and algorithms, involved in their implementation. Four of these methods are positioned similarly for image mining. There are 39 papers using text mining in service research, with a focus on measuring consumer sentiment, experiences, and ...
This paper investigates and analyzes image processing technology, feature extraction and segmentation methods, and existing problems and introduces the development and status of imaging technology in detail. Data mining techniques are studied in detail and applied to the image recognition process. This paper introduces many common computing ...
A subset of this research uses GIS and remote sensing methods to map the extent of land transformation due to mining activity 3,4,5,6,7,8,9. These mapping exercises foster a more sophisticated ...
Existing acceleration methods often compromise image quality or fail under complex conditioning when operating in an extremely low-step regime. In this work, we propose a novel distillation framework tailored to enable high-fidelity, diverse sample generation using just one to three steps.
Image processing is a method of removing information from photographs. The agreement covers picture compression, noise removal, image recognition, thankfulness delineation, image retrieval, and image variation. Probing and obtaining images from a database of photos is used in a variety of industries, including medicine, research, engineering, and many others. The two most prevalent approaches ...
Gene editing has the potential to solve fundamental challenges in agriculture, biotechnology, and human health. CRISPR-based gene editors derived from microbes, while powerful, often show significant functional tradeoffs when ported into non-native environments, such as human cells. Artificial intelligence (AI) enabled design provides a powerful alternative with potential to bypass ...
Text-to-image (T2I) models are central to current advances in computer vision, enabling the synthesis of images from textual descriptions. These models strive to capture the essence of the input text, rendering visual content that mirrors the intricacies described. The core challenge in T2I technology lies in the model's ability to accurately reflect the detailed elements of textual prompts in ...
Deep Mining (Industry) Trapping (Gathering) Heavy Plow (Farming) These five Development Upgrades are the ones I would recommend prioritising at the beginning of every Manor Lords playthrough. | Image credit: Rock Paper Shotgun/Hooded Horse Trade Logistics. Description: Establishing a new Trade Route always costs a maximum of 25 Regional Wealth.