- Statistical Analysis Plan: What is it & How to Write One

- Data Collection
Statistics give meaning to data collected during research and make it simple to extract actionable insights from the data. As a result, it’s important to have a guide for analyzing data, which is where a statistical analysis plan (SAP) comes in.
A statistical analysis plan provides a framework for collecting data , simplifying and interpreting it , and assessing its reliability and validity.
Here’s a guide on what a statistical analysis plan is and how to write one.
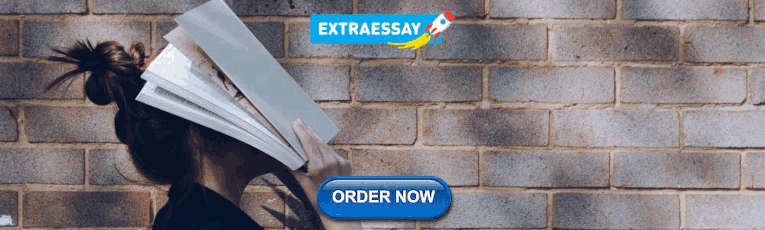
What Is a Statistical Analysis Plan?
A statistical analysis plan (SAP) is a document that specifies the statistical analysis that will be performed on a given dataset. It serves as a comprehensive guide for the analysis, presenting a clear and organized approach to data analysis that ensures the reliability and validity of the results.
SAPs are most widely used in research, data science, and statistics. They are a necessary tool for clearly communicating the goals and methods of analysis, as well as documenting the decisions made during the analysis process.
SAPs typically outline the steps needed to prepare data for analysis, the methods to use, and how details such as sample size, data sources, and any assumptions or limitations of the analysis.
The first step in creating a statistical analysis plan is to identify the research question or hypothesis you’re testing.
Next, choose the appropriate statistical techniques for analyzing the data and specify the analysis details, such as sample size and data sources. It should also include the strategy for presenting and interpreting the results.
How to Develop a Statistical Analysis Plan
Here are the steps for creating a successful statistical analysis plan (SAP):
Identify the Research Question or Hypothesis
This is the main goal of the analysis, and it will guide the rest of the SAP. Here are the steps to identifying research questions or hypotheses:
Define the Analysis’s Goal
The research question or hypothesis should be related to the analysis’s main goal or purpose. If the goal is to evaluate the effectiveness of a content strategy, the research question could be “Is the new strategy more effective than the previous or standard strategy?”
Determine the Variables of Interest
Determine which variables are important to the research question or hypothesis. In the preceding example, the variables could include the effectiveness of the content strategy and its drawbacks.
Formulate the Question or Hypothesis
After identifying the variables, use them to research the question in a clear and precise way. For example, “is the new content strategy more effective than the current one in terms of user acquisition?
Check for Clarity and Specificity
Review the research question or hypothesis for precision and clarity. If a question isn’t well-structured enough to be tested with the data and resources at hand, revise it.
Determine the Sample Size
The main factors that influence the sample size are the type of data being analyzed and the resources available. For example, if the data is continuous, you’ll probably need a large sample size.
Also, your sample size should be tailored to your available resources, time, and budget. You could also calculate the sample size using a sample size formula or software.
Select the Appropriate Statistical Techniques
Choose the most appropriate statistical techniques for the analysis based on the research question, data type, and sample size.
Specify the Details of the Analysis
This includes the data sources, any analysis assumptions or limitations, and any variables that need modifications.
Plan For Presenting and Interpreting the Results
Plan how the results will be interpreted and communicated to your audience. Choose how you want to present the information, such as a report or a presentation.
Identifying the Need for a Statistical Analysis Plan
Here are some real-world examples of where a statistical analysis plan is needed:
Research Studies
Health researchers need SAP to determine the effectiveness of a new drug in treating a specific medical condition. It also outlines the methods and procedures for analyzing the study’s data, including sample size, data sources, and statistical techniques to be used.
Clinic Trials
Clinical trials help to test the safety and efficacy of new medical treatments, which would necessitate gathering a large amount of data on how patients respond to treatment, side effects, and comparisons to existing treatments.
A clinic trial SAP should emphasize the statistical analysis that will be performed on the trial data, such as sample size, data sources, and statistical techniques to be used.
Data-Driven Projects
SAP is used by marketing research firms to outline the statistical analysis that will be performed on market research data. It specifies the sample size, data sources, and statistical techniques that will be used to analyze data and provide insights into consumer behavior.
Government Agencies
When government agencies collect data for new policies such as new tax laws or population censuses, they require a statistical analysis plan outlining how the data will be collected, interpreted, and used. The SAP would specify the sample size, data sources, and statistical techniques that will be used to analyze the data and assess the effectiveness of the policy or program.
Nonprofit Organizations
Nonprofits could also use SAPs to analyze data collected as part of a research study or program evaluation. A non-profit, for example, could gather information about who is likely to donate to their cause and how to contact them to solicit donations.
How Do You Write a Statistical Analysis Plan?
Here are the steps to writing a simple and effective Statistical analysis plan:
Introduction
A statistical analysis plan (SAP) introduction should provide an overview of the research question or hypothesis being tested as well as the goals and objectives of the analysis. It should also provide some context for the topic and the context in which the analysis is being conducted.
This section should describe how the data was collected and prepared for analysis, including sample size, data sources, and any analysis assumptions or limitations.
For example, a clinical trial involving 100 patients with a specific medical condition. The sample will be assigned at random to either the new or current standard treatment.
The SAP will include data on the treatment’s effectiveness in reducing symptoms, which will be collected at the start of the trial and at regular intervals throughout and after it. To avoid common survey bias, data is collected using standardized questionnaires created by researchers.
Next, the data will be cleaned and prepared for analysis by removing any missing or invalid values and ensuring that it is in the correct format. Also, any data collected outside of the specified time frame will be excluded from the analysis.
The small sample size and brief duration of the clinical trial are two of the study’s limitations. These constraints should be considered when interpreting the results of this analysis.
Statistical Techniques
This section should describe the statistical techniques that will be used in the analysis, including any specific software or tools.
Using the preceding example, you can use software such as SPSS or R. They use t-tests and regression analysis to determine the effectiveness of the two treatments.
You can make further investigations using additional statistical techniques such as ANOVA. It enables you to investigate the effects of various variables on treatment efficacy and identify any significant inter-variable interactions.
This section describes how the results will be presented and interpreted, including any plans for visualizing the data or using statistical tests to determine their significance.
Using the clinical trial example, you can visualize the data and find patterns in the data by using graphical representations. Next, interpret the result in light of the research question or hypothesis, as well as any limitations or assumptions of the analysis.
Assess the implications of the clinical trial results and future research on the medical condition’s treatment. Then, develop a summary of the results including any recommendations or conclusions drawn from the research.
The “Conclusion” section should provide a concise summary of the main findings of the analysis as well as any recommendations or implications. It should also highlight any limitations or assumptions of the analysis and discuss the implications of the results for clinical practice and future research.
Information in the Statistical Analysis Plan
1. Statistics on who wrote the SAP, when it was approved, and who signed it.
2. Expected number of participants, and sample size calculation.
3. A detailed explanation of the main and short-term analysis techniques used for analyzing the data. This includes:
- Study goals
- Specify the primary and secondary hypotheses, as well as the parameters you’ll use to assess how well you met the study objectives.
- A detailed description of the study’s sample size.
- A summary of the primary and secondary outcomes of each study. Typically, there should be just one primary outcome.
4. The SAP should also specify how each outcome metric will be assessed. Statistical tests are typically used to examine outcome measures and the method for accounting for missing data.
5. The SAP should also explain the procedures used to analyze and display the study results in detail. This includes:
- The level of statistical significance that will be used, and if one-tailed or two-tailed tests will be used.
- How to deal with missing data.
- Outlier management techniques.
- Protocol variations, noncompliance, and withdrawal procedures.
- Estimation methods for points and intervals.
- How to calculate composite or derived variables, including data-driven definitions and any additional details needed to reduce uncertainties.
- Baseline and covariate data
- Add randomization factors
- Methods for dealing with data from multiple sources
- How to deal with participant interactions
- Multiple comparisons and subgroup analysis methods
- Interim or sequential analyses
- Step-by-step procedure to terminate research and its implications
- Statistical software for analyzing the data
- Validate critical analysis assumptions and sensitivity analyses.
- Visual representation of the research data
- Define the safe population
6. Alternative models for data analysis if the data does not fit the chosen statistical model
Making Modifications to Statistical Analysis Plan
It is not unusual for a statistical analysis plan (SAP) to undergo adjustments during the project’s life cycle. Here’s why you may need to modify your SAP:
- Research question or hypothesis change : As the project progresses, the research question or hypothesis may evolve or change, requiring changes to the SAP.
- New data : As new data is collected or becomes available, it may be necessary to modify the SAP to include the new information.
- Unpredicted challenges : Unexpected challenges may arise during the project, requiring SAP alteration. For example, the data may not be of the expected quality, or the sample size may need to be adjusted.
- Improved Data Understanding : The researcher may gain a better understanding of the data as the analysis progresses and may need to modify the SAP to reflect this enhanced understanding.
Make sure to document the changes made to the SAP, as well as the reasons for them. This ensures the analysis’s reliability and accuracy.
You could also work with a statistician or research expert to ensure that the SAP changes are appropriate and do not jeopardize the results’ reliability and validity.
A statistical analysis plan (SAP) is a step-by-step plan that highlights the methods and techniques to be used in data analysis for a research project. SAPs ensure the reliability and validity of the results and provide a clear roadmap for the analysis.
You have to include the research question or hypothesis, sample size, data sources, statistical techniques, variables, and guidelines for interpreting and presenting the results to have an effective SAP.
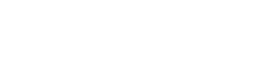
Connect to Formplus, Get Started Now - It's Free!
- data analysis
- statistical analysis
- statistical analysis plan
- Moradeke Owa
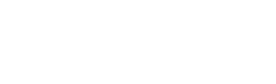
You may also like:
What is Field Research: Meaning, Examples, Pros & Cons
Introduction Field research is a method of research that deals with understanding and interpreting the social interactions of groups of...

Unit of Analysis: Definition, Types & Examples
Introduction A unit of analysis is the smallest level of analysis for a research project. It’s important to choose the right unit of...
What Are Research Repositories?
A research repository is a database that helps organizations to manage, share, and gain access to research data to make product and...
Statistical Analysis Software: A Guide For Social Researchers
Introduction Social research is a complex endeavor. It takes a lot of time, energy, and resources to gather data, analyze and present...
Formplus - For Seamless Data Collection
Collect data the right way with a versatile data collection tool. try formplus and transform your work productivity today..
educational research techniques
Research techniques and education.

Developing a Data Analysis Plan
It is extremely common for beginners and perhaps even experience researchers to lose track of what they are trying to achieve or do when trying to complete a research project. The open nature of research allows for a multitude of equally acceptable ways to complete a project. This leads to an inability to make a decision and or stay on course when doing research.
Data Analysis Plan
A data analysis plan includes many features of a research project in it with a particular emphasis on mapping out how research questions will be answered and what is necessary to answer the question. Below is a sample template of the analysis plan.

The majority of this diagram should be familiar to someone who has ever done research. At the top, you state the problem , this is the overall focus of the paper. Next, comes the purpose , the purpose is the over-arching goal of a research project.
After purpose comes the research questions . The research questions are questions about the problem that are answerable. People struggle with developing clear and answerable research questions. It is critical that research questions are written in a way that they can be answered and that the questions are clearly derived from the problem. Poor questions means poor or even no answers.
After the research questions, it is important to know what variables are available for the entire study and specifically what variables can be used to answer each research question. Lastly, you must indicate what analysis or visual you will develop in order to answer your research questions about your problem. This requires you to know how you will answer your research questions
Below is an example of a completed analysis plan for simple undergraduate level research paper

In the example above, the student wants to understand the perceptions of university students about the cafeteria food quality and their satisfaction with the university. There were four research questions, a demographic descriptive question, a descriptive question about the two main variables, a comparison question, and lastly a relationship question.
The variables available for answering the questions are listed off to the left side. Under that, the student indicates the variables needed to answer each question. For example, the demographic variables of sex, class level, and major are needed to answer the question about the demographic profile.
The last section is the analysis. For the demographic profile, the student found the percentage of the population in each sub group of the demographic variables.
A data analysis plan provides an excellent way to determine what needs to be done to complete a study. It also helps a researcher to clearly understand what they are trying to do and provides a visual for those who the research wants to communicate with about the progress of a study.
Share this:
Leave a reply cancel reply, discover more from educational research techniques.
Subscribe now to keep reading and get access to the full archive.
Type your email…
Continue reading
Customize Your Path
Filters Applied
Customize Your Experience.
Utilize the "Customize Your Path" feature to refine the information displayed in myRESEARCHpath based on your role, project inclusions, sponsor or funding, and management center.
Design the analysis plan
Need assistance with analysis planning?
Get help with analysis planning.
Contact the Biostatistics, Epidemiology, and Research Design (BERD) Methods Core:
- Submit a help request
- 1-icon/ui/arrow_right Amsterdam Public Health
- 1-icon/ui/arrow_right Home
- 1-icon/ui/arrow_right Research Lifecycle
More APH...
- 1-icon/ui/arrow_right About
- 1-icon/ui/arrow_right News
- 1-icon/ui/arrow_right Events
- 1-icon/ui/arrow_right Research information
- 1-icon/ui/arrow_right Our strenghts
- Amsterdam Public Health
- Research Lifecycle
- Research information
- Our strenghts
- Proposal Writing
- Study Preparation
- Methods & Data Collection
- Process & Analyze Data
- Writing & Publication
- Archiving & Open Data
- Knowledge Utilization
- Supervision
- Analysis plan
- Set-up & Conduct
- Quantitative research
Data analysis
- Initial data analysis
- Post-hoc & sensitivity analyses
- Data analysis documentation
- Handling missing data
To promote structured targeted data analysis.
Requirements
An analysis plan should be created and finalized prior to the data analyses.
Documentation
The analysis plan (Guidelines per study type are provided below)
Responsibilities
- Executing researcher: To create the analysis plan prior to the data analyses, containing a description of the research question and what the various steps in the analysis are going to be. This should also be signed and dated by the PI.
- Project leaders: To inform the executing researcher about setting up the analysis plan before analyses are undertaken.
- Research assistant: N.a.
An analysis plan should be created and finalized (signed and dated by PI) prior to the data analyses. The analysis plan contains a description of the research question and what the various steps in the analysis are going to be. It also contains an exploration of literature (what is already know? What will this study add?) to make sure your research question is relevant (see Glasziou et al. Lancet 2014 on avoiding research waste).The analysis plan is intended as a starting point for the analysis. It ensures that the analysis can be undertaken in a targeted manner, and promotes research integrity.
If you will perform an exploratory study you can adjust your analysis based on the data you find; this may be useful if not much is known about the research subject, but it is considered as relatively low level evidence and it should be clearly mentioned in your report that the presented study is exploratory. If you want to perform an hypothesis-testing study (be it interventional or using observational data) you need to pre-specify the analyses you intend to do prior to performing the analysis, including the population, subgroups, stratifications and statistical tests. If deviations from the analysis plan are made during the study this should be documented in the analysis plan and stated in the report (i.e. post-hoc tests). If you intend to do hypothesis-free research with multiple testing you should pre-specify your threshold for statistical significance according to the number of analyses you will perform. Lastly, if you intend to perform an RCT, the analysis plan is practically set in stone. (Also see ICH E9 - statistical principles for clinical trials )
If needed, an exploratory analysis may be part of the analysis plan, to inform the setting up of the final analysis (see initial data analysis ). For instance, you may want to know distributions of values in order to create meaningful categories, or determine whether data are normally distributed. The findings and decisions made during these preliminary exploratory analyses should be clearly documented, preferably in a version two of the analysis plan, and made reproducible by providing the data analysis syntax (in SPSS, SAS, STATA, R) (see guideline Documentation of data analysis ).
The concrete research question needs to be formulated firstly within the analysis plan following the literature review; this is the question intended to be answered by the analyses. Concrete research questions may be defined using the acronym PICO: Population, Intervention, Comparison, Outcomes. An example of a concrete question could be: “Does frequent bending at work lead to an elevated risk of lower back pain occurring in employees?” (Population = Employees; Intervention = Frequent bending; Comparison = Infrequent bending; Outcome = Occurrence of back pain). Concrete research questions are essential for determining the analyses required.
The analysis plan should then describe the primary and secondary outcomes, the determinants and data needed, and which statistical techniques are to be used to analyse the data. The following issues need to be considered in this process and described where applicable:
- In case of a trial: is the trial a superiority, non-inferiority or equivalence trial.
- Superiority: treatment A is better than the control.
- Non-inferiority: treatment A is not worse than treatment B.
- Equivalence: testing similarity using a tolerance range.
In other studies: what is the study design (case control, longitudinal cohort etc).
- Which (subgroup of the) population is to be included in the analyses? Which groups will you compare?;
- What are the primary and secondary endpoints? Which data from which endpoint (T1, T2, etc.) will be used?;
- Which (dependent and independent) variables are to be used in the analyses and how are the variables to be analysed (e.g. continuous or in categories)?;
- Which variables are to be investigated as potential confounders or effect modifiers (and why) and how are these variables to be analysed? There are different ways of dealing with confounders. We distinguish the following: 1) correct for all potential confounders (and do not concern about the question whether or not a variable is a ‘real’ confounder). Mostly, confounders are split up in little groups (demographic factors, clinical parameters, etc.). As a result you get corrected model 1, corrected model 2, etc. However, pay attention to collinearity and overcorrection if confounders coincide too much with primary determinants. 2) if the sample size is not big enough relative to the number of potential confounders, you may consider to only correct for those confounders that are relevant for the association between determinant and outcome. To select the relevant confounders, mostly a forward selection procedure is performed. In this case the confounders are added to the model one by one (the confounder that is associated strongest first). Subsequently, consider to what extent the effect of the variable of interest is changed. Then first choose the strongest confounder in the model. Subsequently, repeat this procedure untill no confounder has a relevant effect (<10% change in regression coefficient). Alternatively, you can select the confounders that univariately change the point estimate of the association with >10%. 3) Another option is to set up a Directed Acyclic Graph (DAG), to determine which confounders should be added to the model. Please see http://www.dagitty.net/ for more information.
- How to deal with missing values? (see chapter on handeling missing data );
- Which analyses are to be carried out in which order (e.g. univariable analyses, multivariable analyses, analysis of confounders, analysis of interaction effects, analysis of sub-populations, etc.)?; Which sensitivity analyses will be performed?
- Do the data meet the criteria for the specific statistical technique?
A statistician may need to be consulted regarding the choice of statistical techniques (also see this intanetpage on statistical analysis plan ).
It is recommended to already design the empty tables to be included in the article prior to the start of data analysis. This is often very helpful in deciding which analyses are exactly required in order to analyse the data in a targeted manner.
You may consider to make your study protocol including the (statistical) analysis plan public, either by placing in on a publicly accessible website (Concept Paper/Design paper) or by uploading it in an appropriate studies register (for human trials: NTR / EUDRACT / ClinicalTrials.gov , for non-/preclinicaltrials: preclinicaltrials.eu ).
Check the reporting guidelines when writing an analysis plan . These will help increase the quality of your research and guide you.
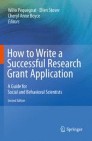
How to Write a Successful Research Grant Application pp 283–298 Cite as
Writing the Data Analysis Plan
- A. T. Panter 4
- First Online: 01 January 2010
5745 Accesses
3 Altmetric
You and your project statistician have one major goal for your data analysis plan: You need to convince all the reviewers reading your proposal that you would know what to do with your data once your project is funded and your data are in hand. The data analytic plan is a signal to the reviewers about your ability to score, describe, and thoughtfully synthesize a large number of variables into appropriately-selected quantitative models once the data are collected. Reviewers respond very well to plans with a clear elucidation of the data analysis steps – in an appropriate order, with an appropriate level of detail and reference to relevant literatures, and with statistical models and methods for that map well into your proposed aims. A successful data analysis plan produces reviews that either include no comments about the data analysis plan or better yet, compliments it for being comprehensive and logical given your aims. This chapter offers practical advice about developing and writing a compelling, “bullet-proof” data analytic plan for your grant application.
- Latent Class Analysis
- Grant Application
- Grant Proposal
- Data Analysis Plan
- Latent Transition Analysis
These keywords were added by machine and not by the authors. This process is experimental and the keywords may be updated as the learning algorithm improves.
This is a preview of subscription content, log in via an institution .
Buying options
- Available as PDF
- Read on any device
- Instant download
- Own it forever
- Available as EPUB and PDF
- Compact, lightweight edition
- Dispatched in 3 to 5 business days
- Free shipping worldwide - see info
Tax calculation will be finalised at checkout
Purchases are for personal use only
Aiken, L. S. & West, S. G. (1991). Multiple regression: testing and interpreting interactions . Newbury Park, CA: Sage.
Google Scholar
Aiken, L. S., West, S. G., & Millsap, R. E. (2008). Doctoral training in statistics, measurement, and methodology in psychology: Replication and extension of Aiken, West, Sechrest and Reno’s (1990) survey of PhD programs in North America. American Psychologist , 63 , 32–50.
Article PubMed Google Scholar
Allison, P. D. (2003). Missing data techniques for structural equation modeling. Journal of Abnormal Psychology , 112 , 545–557.
American Psychological Association (APA) Task Force to Increase the Quantitative Pipeline (2009). Report of the task force to increase the quantitative pipeline . Washington, DC: American Psychological Association.
Bauer, D. & Curran, P. J. (2004). The integration of continuous and discrete latent variables: Potential problems and promising opportunities. Psychological Methods , 9 , 3–29.
Bollen, K. A. (1989). Structural equations with latent variables . New York: Wiley.
Bollen, K. A. & Curran, P. J. (2007). Latent curve models: A structural equation modeling approach . New York: Wiley.
Cohen, J., Cohen, P., West, S. G., & Aiken, L. S. (2003). Multiple correlation/regression for the behavioral sciences (3rd ed.). Mahwah, NJ: Erlbaum.
Curran, P. J., Bauer, D. J., & Willoughby, M. T. (2004). Testing main effects and interactions in hierarchical linear growth models. Psychological Methods , 9 , 220–237.
Embretson, S. E. & Reise, S. P. (2000). Item response theory for psychologists . Mahwah, NJ: Erlbaum.
Enders, C. K. (2006). Analyzing structural equation models with missing data. In G. R. Hancock & R. O. Mueller (Eds.), Structural equation modeling: A second course (pp. 313–342). Greenwich, CT: Information Age.
Hosmer, D. & Lemeshow, S. (1989). Applied logistic regression . New York: Wiley.
Hoyle, R. H. & Panter, A. T. (1995). Writing about structural equation models. In R. H. Hoyle (Ed.), Structural equation modeling: Concepts, issues, and applications (pp. 158–176). Thousand Oaks: Sage.
Kaplan, D. & Elliott, P. R. (1997). A didactic example of multilevel structural equation modeling applicable to the study of organizations. Structural Equation Modeling , 4 , 1–23.
Article Google Scholar
Lanza, S. T., Collins, L. M., Schafer, J. L., & Flaherty, B. P. (2005). Using data augmentation to obtain standard errors and conduct hypothesis tests in latent class and latent transition analysis. Psychological Methods , 10 , 84–100.
MacKinnon, D. P. (2008). Introduction to statistical mediation analysis . Mahwah, NJ: Erlbaum.
Maxwell, S. E. (2004). The persistence of underpowered studies in psychological research: Causes, consequences, and remedies. Psychological Methods , 9 , 147–163.
McCullagh, P. & Nelder, J. (1989). Generalized linear models . London: Chapman and Hall.
McDonald, R. P. & Ho, M. R. (2002). Principles and practices in reporting structural equation modeling analyses. Psychological Methods , 7 , 64–82.
Messick, S. (1989). Validity. In R. L. Linn (Ed.), Educational measurement (3rd ed., pp. 13–103). New York: Macmillan.
Muthén, B. O. (1994). Multilevel covariance structure analysis. Sociological Methods & Research , 22 , 376–398.
Muthén, B. (2008). Latent variable hybrids: overview of old and new models. In G. R. Hancock & K. M. Samuelsen (Eds.), Advances in latent variable mixture models (pp. 1–24). Charlotte, NC: Information Age.
Muthén, B. & Masyn, K. (2004). Discrete-time survival mixture analysis. Journal of Educational and Behavioral Statistics , 30 , 27–58.
Muthén, L. K. & Muthén, B. O. (2004). Mplus, statistical analysis with latent variables: User’s guide . Los Angeles, CA: Muthén &Muthén.
Peugh, J. L. & Enders, C. K. (2004). Missing data in educational research: a review of reporting practices and suggestions for improvement. Review of Educational Research , 74 , 525–556.
Preacher, K. J., Curran, P. J., & Bauer, D. J. (2006). Computational tools for probing interaction effects in multiple linear regression, multilevel modeling, and latent curve analysis. Journal of Educational and Behavioral Statistics , 31 , 437–448.
Preacher, K. J., Curran, P. J., & Bauer, D. J. (2003, September). Probing interactions in multiple linear regression, latent curve analysis, and hierarchical linear modeling: Interactive calculation tools for establishing simple intercepts, simple slopes, and regions of significance [Computer software]. Available from http://www.quantpsy.org .
Preacher, K. J., Rucker, D. D., & Hayes, A. F. (2007). Addressing moderated mediation hypotheses: Theory, methods, and prescriptions. Multivariate Behavioral Research , 42 , 185–227.
Raudenbush, S. W. & Bryk, A. S. (2002). Hierarchical linear models: Applications and data analysis methods (2nd ed.). Thousand Oaks, CA: Sage.
Radloff, L. (1977). The CES-D scale: A self-report depression scale for research in the general population. Applied Psychological Measurement , 1 , 385–401.
Rosenberg, M. (1965). Society and the adolescent self-image . Princeton, NJ: Princeton University Press.
Schafer. J. L. & Graham, J. W. (2002). Missing data: Our view of the state of the art. Psychological Methods , 7 , 147–177.
Schumacker, R. E. (2002). Latent variable interaction modeling. Structural Equation Modeling , 9 , 40–54.
Schumacker, R. E. & Lomax, R. G. (2004). A beginner’s guide to structural equation modeling . Mahwah, NJ: Erlbaum.
Selig, J. P. & Preacher, K. J. (2008, June). Monte Carlo method for assessing mediation: An interactive tool for creating confidence intervals for indirect effects [Computer software]. Available from http://www.quantpsy.org .
Singer, J. D. & Willett, J. B. (1991). Modeling the days of our lives: Using survival analysis when designing and analyzing longitudinal studies of duration and the timing of events. Psychological Bulletin , 110 , 268–290.
Singer, J. D. & Willett, J. B. (1993). It’s about time: Using discrete-time survival analysis to study duration and the timing of events. Journal of Educational Statistics , 18 , 155–195.
Singer, J. D. & Willett, J. B. (2003). Applied longitudinal data analysis: Modeling change and event occurrence . New York: Oxford University.
Book Google Scholar
Vandenberg, R. J. & Lance, C. E. (2000). A review and synthesis of the measurement invariance literature: Suggestions, practices, and recommendations for organizational research. Organizational Research Methods , 3 , 4–69.
Wirth, R. J. & Edwards, M. C. (2007). Item factor analysis: Current approaches and future directions. Psychological Methods , 12 , 58–79.
Article PubMed CAS Google Scholar
Download references
Author information
Authors and affiliations.
L. L. Thurstone Psychometric Laboratory, Department of Psychology, University of North Carolina, Chapel Hill, NC, USA
A. T. Panter
You can also search for this author in PubMed Google Scholar
Corresponding author
Correspondence to A. T. Panter .
Editor information
Editors and affiliations.
National Institute of Mental Health, Executive Blvd. 6001, Bethesda, 20892-9641, Maryland, USA
Willo Pequegnat
Ellen Stover
Delafield Place, N.W. 1413, Washington, 20011, District of Columbia, USA
Cheryl Anne Boyce
Rights and permissions
Reprints and permissions
Copyright information
© 2010 Springer Science+Business Media, LLC
About this chapter
Cite this chapter.
Panter, A.T. (2010). Writing the Data Analysis Plan. In: Pequegnat, W., Stover, E., Boyce, C. (eds) How to Write a Successful Research Grant Application. Springer, Boston, MA. https://doi.org/10.1007/978-1-4419-1454-5_22
Download citation
DOI : https://doi.org/10.1007/978-1-4419-1454-5_22
Published : 20 August 2010
Publisher Name : Springer, Boston, MA
Print ISBN : 978-1-4419-1453-8
Online ISBN : 978-1-4419-1454-5
eBook Packages : Medicine Medicine (R0)
Share this chapter
Anyone you share the following link with will be able to read this content:
Sorry, a shareable link is not currently available for this article.
Provided by the Springer Nature SharedIt content-sharing initiative
- Publish with us
Policies and ethics
- Find a journal
- Track your research
Data Analysis Plan: Ultimate Guide and Examples
Learn the post survey questions you need to ask attendees for valuable feedback.
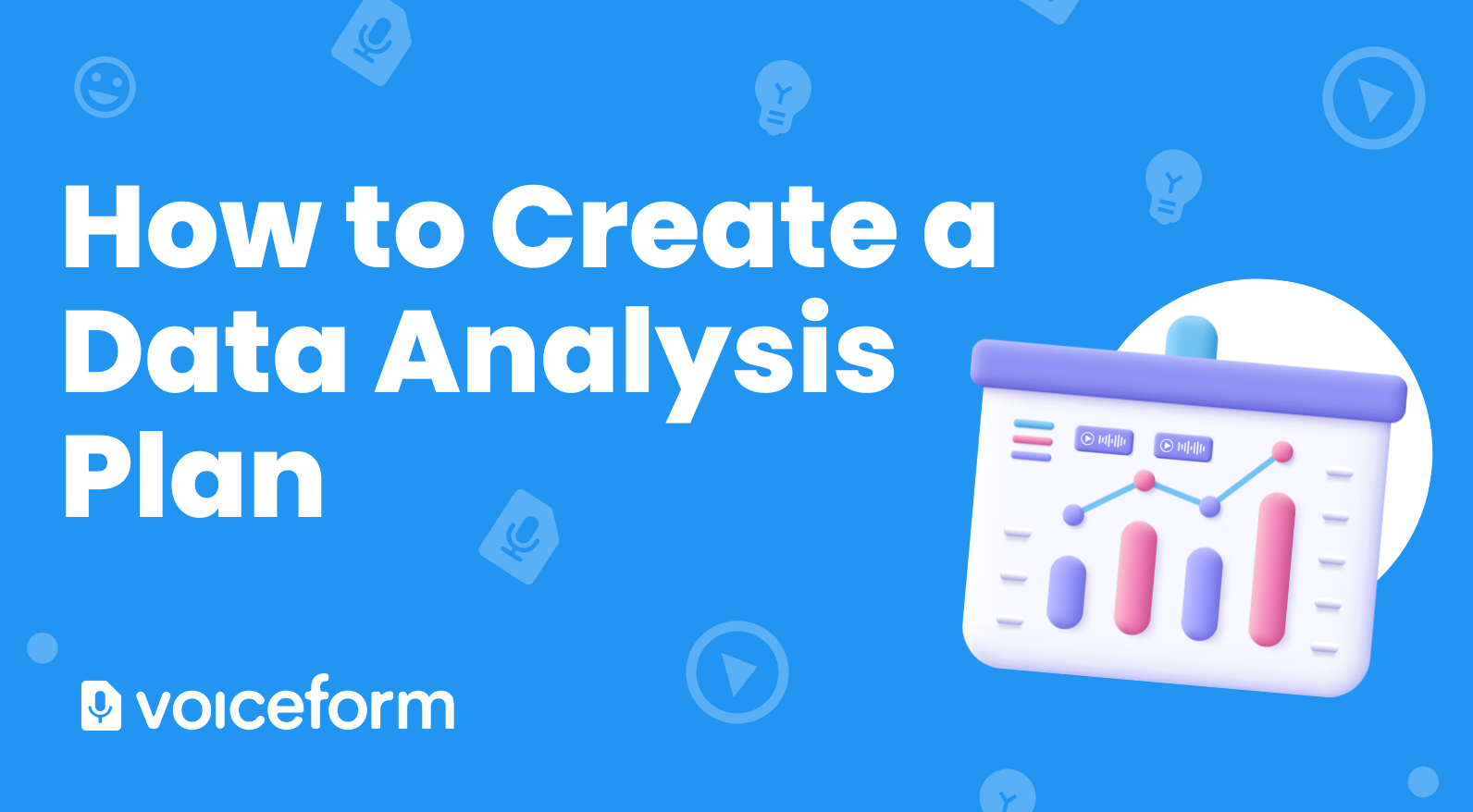
Once you get survey feedback , you might think that the job is done. The next step, however, is to analyze those results. Creating a data analysis plan will help guide you through how to analyze the data and come to logical conclusions.
So, how do you create a data analysis plan? It starts with the goals you set for your survey in the first place. This guide will help you create a data analysis plan that will effectively utilize the data your respondents provided.
What can a data analysis plan do?
Think of data analysis plans as a guide to your organization and analysis, which will help you accomplish your ultimate survey goals. A good plan will make sure that you get answers to your top questions, such as “how do customers feel about this new product?” through specific survey questions. It will also separate respondents to see how opinions among various demographics may differ.
Creating a data analysis plan
Follow these steps to create your own data analysis plan.
Review your goals
When you plan a survey, you typically have specific goals in mind. That might be measuring customer sentiment, answering an academic question, or achieving another purpose.
If you’re beta testing a new product, your survey goal might be “find out how potential customers feel about the new product.” You probably came up with several topics you wanted to address, such as:
- What is the typical experience with the product?
- Which demographics are responding most positively? How well does this match with our idea of the target market?
- Are there any specific pain points that need to be corrected before the product launches?
- Are there any features that should be added before the product launches?
Use these objectives to organize your survey data.
Evaluate the results for your top questions
Your survey questions probably included at least one or two questions that directly relate to your primary goals. For example, in the beta testing example above, your top two questions might be:
- How would you rate your overall satisfaction with the product?
- Would you consider purchasing this product?
Those questions offer a general overview of how your customers feel. Whether their sentiments are generally positive, negative, or neutral, this is the main data your company needs. The next goal is to determine why the beta testers feel the way they do.
Assign questions to specific goals
Next, you’ll organize your survey questions and responses by which research question they answer. For example, you might assign questions to the “overall satisfaction” section, like:
- How would you describe your experience with the product?
- Did you encounter any problems while using the product?
- What were your favorite/least favorite features?
- How useful was the product in achieving your goals?
Under demographics, you’d include responses to questions like:
- Education level
This helps you determine which questions and answers will answer larger questions, such as “which demographics are most likely to have had a positive experience?”
Pay special attention to demographics
Demographics are particularly important to a data analysis plan. Of course you’ll want to know what kind of experience your product testers are having with the product—but you also want to know who your target market should be. Separating responses based on demographics can be especially illuminating.
For example, you might find that users aged 25 to 45 find the product easier to use, but people over 65 find it too difficult. If you want to target the over-65 demographic, you can use that group’s survey data to refine the product before it launches.
Other demographic segregation can be helpful, too. You might find that your product is popular with people from the tech industry, who have an easier time with a user interface, while those from other industries, like education, struggle to use the tool effectively. If you’re targeting the tech industry, you may not need to make adjustments—but if it’s a technological tool designed primarily for educators, you’ll want to make appropriate changes.
Similarly, factors like location, education level, income bracket, and other demographics can help you compare experiences between the groups. Depending on your ultimate survey goals, you may want to compare multiple demographic types to get accurate insight into your results.
Consider correlation vs. causation
When creating your data analysis plan, remember to consider the difference between correlation and causation. For instance, being over 65 might correlate with a difficult user experience, but the cause of the experience might be something else entirely. You may find that your respondents over 65 are primarily from a specific educational background, or have issues reading the text in your user interface. It’s important to consider all the different data points, and how they might have an effect on the overall results.
Moving on to analysis
Once you’ve assigned survey questions to the overall research questions they’re designed to answer, you can move on to the actual data analysis. Depending on your survey tool, you may already have software that can perform quantitative and/or qualitative analysis. Choose the analysis types that suit your questions and goals, then use your analytic software to evaluate the data and create graphs or reports with your survey results.
At the end of the process, you should be able to answer your major research questions.
Power your data analysis with Voiceform
Once you have established your survey goals, Voiceform can power your data collection and analysis. Our feature-rich survey platform offers an easy-to-use interface, multi-channel survey tools, multimedia question types, and powerful analytics. We can help you create and work through a data analysis plan. Find out more about the product, and book a free demo today !
We make collecting, sharing and analyzing data a breeze
Get started for free. Get instant access to Voiceform features that get you amazing data in minutes.


An official website of the United States government
The .gov means it’s official. Federal government websites often end in .gov or .mil. Before sharing sensitive information, make sure you’re on a federal government site.
The site is secure. The https:// ensures that you are connecting to the official website and that any information you provide is encrypted and transmitted securely.
- Publications
- Account settings
Preview improvements coming to the PMC website in October 2024. Learn More or Try it out now .
- Advanced Search
- Journal List
- Contemp Clin Trials Commun
- v.34; 2023 Aug
- PMC10300078
A template for the authoring of statistical analysis plans
Gary stevens.
a DynaStat Consulting, Inc., 119 Fairway Court, Bastrop, TX, 78602, USA
Shawn Dolley
b Open Global Health, 710 12th St. South, Suite 2523, Arlington, VA, 22202, USA
c Takeda Pharmaceuticals USA Inc., 95 Hayden Avenue, Lexington, MA, 02421, USA
Jason T. Connor
d ConfluenceStat, 3102 NW 82nd Way, Cooper City, Florida, 33024, USA
e University of Central Florida College of Medicine, 6850 Lake Nona Blvd, Orlando, FL, 32827, USA
Associated Data
All data is contained within the manuscript.
No data was used for the research described in the article.
A number of principal investigators may have limited access to biostatisticians, a lack of biostatistical training, or no requirement to complete a timely statistical analysis plan (SAP). SAPs completed early will identify design or implementation weak points, improve protocols, remove the temptation for p-hacking, and enable proper peer review by stakeholders considering funding the trial. An SAP completed at the same time as the study protocol might be the only comprehensive method for at once optimizing sample size, identifying bias, and applying rigor to study design. This ordered corpus of SAP sections with detailed definitions and a variety of examples represents an omnibus of best practice methods offered by biostatistical practitioners inside and outside of industry. The article presents a protocol template for clinical research design, enabling statisticians, from beginners to advanced.
- • Comprehensive template for biostatisticians: best practices, annotations, code samples, and more. Perfect for both early and mature statisticians.
- • Promotes equity by bridging the gap for biostatisticians in low-resource settings, ensuring access to tools available in the Global North.
- • Benefits of earlier SAP completion alongside the protocol are described.
1. Introduction
1.1. the statistical analysis plan.
The Statistical Analysis Plan (SAP) is a key document that complements the study protocol in randomized controlled trials (RCT). SAPs are a vital component of transparent, objective, rigorous, reproducible research. The SAP “describes the planned analysis of study objectives … it describes what variables and outcomes will be collected and which statistical methods will be used to analyze them [ 1 ]”. National regulatory agencies around the world require SAPs to be submitted when considering drugs, biologics, and devices for approval. The SAP is meant to supplement the protocol and provide richer detail for all prospectively planned statistical analyses. In addition, it defines the population(s) and time point(s) used for each analysis. It defines details such as multiplicity control, sensitivity analyses, methods used to handle missing data, subsets analyses prospectively identified, and the specific analyses performed on each subset of interest. For those populations defined, the SAP should provide clear rules for who is included in each analysis population.
The SAP functions as contract between the study team and the potential consumers of their research [ 2 ]. It identifies analyses described in the protocol and ensures there is sufficient detail so prospectively defined methods can be precisely executed. While there may always be post-hoc/exploratory analysis after data is collected, the SAP is used to identify all primary, secondary, and pre-specified exploratory analysis and the precise methods to be used for those. The situation where further detail is required after unblinding of the data is one to be avoided as it may allow for the introduction of bias if the investigators, who have now seen the unblinded trial data, must be approached to provide clarity.
One key goal of a well-written SAP is reproducibility. The standard for this reproducibility can be measured by this idealistic exercise: if multiple statisticians have access to (1) the analysis dataset and (2) the SAP, they would all conduct very similar to the same analyses, and ideally produce the same results. While the trial protocol may describe all primary and secondary endpoints and analyses, the SAP is the place where greater technical detail can be provided to the target audience of statisticians and statistical programmers, in order to achieve this high level of reproducibility.
As the vehicle for key findings and solid science, the SAP is a foundational document to reproducible research. Given the data and the SAP, there should be a very limited number of subjective decisions necessary at the time of the analysis. This ought to help the resulting analyses have the highest possible integrity. Any analysis not prospectively defined in the SAP should be clearly noted as post hoc. Likewise, any analysis that differed in any way from the prospectively planned analysis described within the SAP should be noted with the difference and its rationale.
1.2. Create the SAP when creating the trial protocol
The SAP cannot be completed before the protocol is completed. The SAP must be completed before the study is unblinded (in a blinded trial) or the PI or statistical team has access to the accumulating data (in an unblinded trial). For years, conventional wisdom maintained an SAP ought to be finished “after the protocol is finalized and before the blind is broken” [ 2 ]. In recent years, many have adopted a best practice that an SAP ought to be finalized before the first patient is enrolled. In some cases, however, protocols that are finished, funded and frozen without an SAP are not clear and detailed enough to carry out a true prospective analysis. One approach to avoid this is to prepare and complete the SAP in parallel with the protocol. Rather than delay, “a statistical analysis plan should be prepared at the time of protocol development [ 3 ]”.
There are significant benefits to completing the SAP while the protocol is being completed. The protocol clarity needed by the statistician for the SAP can act as a catalyst to unearth design flaws in a protocol that is ‘in development’. This identification of design flaws is secondary to the primary goal of completing an SAP. This secondary effect is a significant benefit to creating the SAP at the time of the protocol. Evans and Ting confirm that secondary uses of an SAP are valid, including describing the SAP as: “a pseudo-contract between the statisticians and other members of the project team” and “… a communication mechanism with regulatory agencies [ 2 ]”. Those who do not complete the SAP concomitantly with the protocol lose a unique opportunity to find study design flaws. Otherwise, those flaws can live in the design until found by the trial implementation team on site or during the data analysis phase. If uncovered during the data analysis phase, it may suddenly dawn on the PI team that the research question cannot be answered with the trial that was implemented. If a trial is implemented to answer a statistically well-defined research question, then “consideration of the statistical methods should underpin all aspects of an RCT, including development of the specific aims and design of the protocol [ 3 ]”.
An additional benefit of co-developing the protocol and SAP is the resulting increase in likelihood the study will end informatively. Deborah Zarin and colleagues created the term “uninformative clinical trials” in 2019 [ 4 ]. Uninformativeness is a type of research inefficiency. “An uninformative trial is one that provides results that are not of meaningful use for a patient, clinician, researcher, or policy maker [ 4 ]”. They describe one potential driver of uninformativeness as when a “study design is pre-specified in a trial protocol, but [the] trial is not conducted, analyzed or reported in manner consistent with [the] protocol [ 4 ]”. There is established evidence of SAPs being at odds with their protocol pair at study publication [ 5 , 6 ]. An obvious method to ensure against this possible disconnect is to finalize the SAP during the development of the study protocol.
1.3. Engage biostatisticians with domain experience
There are unique characteristics and statistics that tend to repeat within specific types of trials. Examples of these specific types of trials include those with particular pathologies (e.g., cancer, malnutrition), interventions (e.g., vaccines, digital health applications), or study designs (e.g., enrichment, cluster randomized, or challenge trials). RCTs touching different domains will need to apply statistical techniques specific to those domains. For example, in the case of human challenge trials, the SAP would need to include a model for estimating infection fatality risk. For example, recently one team created a Bayesian meta-analysis model to estimate infection fatality in COVID-19 human challenge trials including young participants [ 7 ]. In cluster randomized trials, handling the similarities in outcomes amongst participants inside and outside of clusters—referred to as intracluster correlation—adds particular complexities to estimating proper sample sizes [ 8 ]. Employing biostatisticians with experience in creating statistical analysis plans for particular trial varietals increases the likelihood of success of those trials.
1.4. An excellent SAP rewards the principal investigator (PI) and team
There are a number of secondary positive effects of having a solidly constructed and thorough SAP. These include, but are not limited to:
- 1. Regulatory Review. If the PI knows there will be a path to engage regulators for approval, or pivots to that decision later, the quality of the SAP will be paramount. Since one cannot re-write or create the SAP post hoc, the commitment to SAP excellence must happen up front.
- 2. Ethics Committee/Institutional Review Board. Although the SAP is not a core document that an Ethics Committee or Institutional Review Board might use to make key decisions about a study, it might be useful to the members of such groups. In this case, a high-quality SAP will speed approval, while raising the credibility of the PI team.
- 3. Unanticipated Review. If there is some heavy scrutiny of the RCT, either for positive or negative reasons, requests may emerge for the SAP. If that SAP is reviewed more widely and the stakes are high, the PI team and SAP authors would want a complete, high-quality document.
- 4. Future Re-Use. With a high-quality SAP, it might be a useful template for future studies. Because it includes a number of endpoints or populations you may use in future studies, regular updates will keep it fresh. This will save time in writing new SAPs from scratch each time.
- 5. Funding Asset. Showing a thorough and professional SAP enables funders, donors, and sponsors to give grant funding with confidence. As they compare research teams, it is in places like the SAP where the ground truth about capabilities shines through.
- 6. Future Readiness. More funders, donors, and sponsors are realizing the value of early SAPs for informativeness. It is likely that SAPs will increasingly be requested or be requested pre-funding. Excellent SAPs and the discipline it takes to maintain their creation will make it easier when those shifts occur. Readiness for publication will be higher, as more and more high impact journals are requiring Protocols and SAPs as online appendices during publication.
To realize these benefits as well as the primary goal of ensuring transparent, objective, rigorous, reproducible research, one or more biostatisticians must be engaged to create an SAP. While some biostatisticians write and edit SAPs frequently and are ‘living’ in the world of trial design, more of them are not. Those who are new to SAPs or are irregularly writing such documents could benefit from current best practices and refreshers of fundamentals. Fortunately, a number of contemporary peer-reviewed publications have included SAP checklists. These checklists are designed to ensure SAP authors include all necessary and best practice items [ 1 , 2 , 9 ]. In addition to these SAP creation checklists, a recent checklist includes items that a biostatistician might look for when reviewing an SAP on behalf of donors, funders, or sponsors [ 10 ].
2. Materials and methods
The template that follows is organized as an example SAP, with guidance included. While all clinical trials and prospective studies are different, this document describes sections that should or may be desirable to produce a document that will guide objective analyses at the project's conclusion. Not all sections mentioned here will be necessary for all trials or studies and some may be omitted. Furthermore, there may be unique aspects of a study not contained herein that may be required for studies using novel methodology or having uncommon characteristics. The key is that the SAP must contain detailed descriptions of all study populations, endpoints, and preplanned analyses to maximize study integrity and eliminate or limit the subjectivity required in the study's analysis phase. The SAP template herein includes examples from a number of different trials and trial types. This was necessary to provide a wider breadth of examples, and for examples with richer content.
No human participants were included nor involved in this work. As no humans nor animals were involved, there was no opportunity for informed consent, and no ethical approval was sought.
While all clinical trials and prospective studies are different, this document describes sections that should or may be desirable to produce a document that will guide objective analyses at the project's conclusion. Too often, an SAP contains large chunks of text copied and pasted from the protocol. In some places, e.g., inclusion/exclusion criteria, this may be appropriate. Otherwise the SAP should be viewed as a place to add detail. Therefore, when copying from the protocol, consider whether technical details can or should be added to guide the statistical team at the time of analysis.
The following document is organized as an example Statistical Analysis Plan. Not all sections mentioned here will be necessary for all trials/studies and some may be omitted. Furthermore, there may be unique aspects of a study not contained herein that may be required for studies using novel methodology or having uncommon characteristics. The key is that the SAP contain detailed descriptions of all study populations, endpoints, and preplanned analyses to maximize study integrity and eliminate or limit the subjectivity required in the study's analysis phase.
Descriptive text defining the aims or providing descriptive detail for each section is found in italics and is meant to guide the SAP authors and it not meant for inclusion in an SAP. Bold underlined text are examples meant to be replaced by authors with their protocol-specific text . Other black text is meant as example description and may be kept entirely, edited as necessary, or replaced in its entirety.
4. Statistical analysis plan
5. statistical analysis plan approval signature page, 6. sap revision history.
Each time the SAP is given a new version number/the version is incremented, add here a date of the new version, the name of the primary author of the changes, a summary list of changes made, the reasons for those revisions, and any other information that seems suitable to record. Add to each row or entry the estimated number of weeks prior to the first interim analysis the revision was made.
SAP revisions may be aligned with specific protocol revisions as well. Each version of the SAP should reference the latest version of the protocol to which it is aligned.
7. SAP roles
SAP Primary Author: ____________________ (This is the name of the person directly writing most of the document.)
Senior Statistician: _______________________ (This is the name of the person who is the most organizationally senior who would actually read and sign off on and be accountable for the correct approaches being included.)
SAP Contributor ([Role]): _________________ (This is someone on the team who contributed to the SAP but did not do most of the writing nor are they the senior statistician.)
List of abbreviations
This list typically follows the terms from the protocol that are used in the SAP plus additional terms that are specific to the SAP that are statistical in nature, i.e., MMRM = Mixed Model Repeated Measures. The list below is offered as an example; if you want to use it, please remove terms not used in the protocol, and replace it with your terms.
Example wording to explain the purpose of this SAP. This should lay out the background, rationale, hypotheses, and objectives of the study and may be similar to the intro to the protocol.
The primary objective of this study is to assess the efficacy and safety of Product Name or Healthcare intervention strategy in the treatment of disease name in target population .
This document outlines the statistical methods to be implemented during the analyses of data collected within the scope of Trial Group 's Protocol Number titled “ Protocol Title ”.
This Statistical Analysis Plan (SAP) was prepared in accordance with the Protocol, Protocol Number , dated add date . (Original protocol version & date from which first SAP was created) .
This SAP was modified to be in accordance with the protocol revision(s) Protocol Number dated Date modified . (Protocol revisions which necessitated updates to SAP) .
The purpose of this Statistical Analysis Plan (SAP) is to provide a framework in which answers to the protocol objectives may be achieved in a statistically rigorous fashion, without bias or analytical deficiencies, following methods identified prior to database lock. Specifically, this plan has the following purposes:
- • To prospectively outline the specific types of analyses and presentations of data that will form the basis for conclusions.
- • To explain in detail how the data will be handled and analyzed, adhering to commonly accepted standards and practices of biostatistical analysis. Any deviations from these guidelines must be substantiated by sound statistical reasoning and documented in writing in the final clinical study report (CSR).
Because the SAP is easier to update than the protocol, there may be situations where the SAP is updated later and deviates from the statistical methods described in the protocol. A summary statement should be included to cover this situation indicating the SAP takes precedent when the protocol and SAP deviate:
The analyses described in this analysis plan are consistent with the analyses described in the study protocol. The order may be changed for clarity. If there are discrepancies between the protocol and SAP, the SAP will serve as the definitive analysis plan.
Any analysis performed not prospectively defined in this document will be labeled as post hoc & exploratory.
If a substantive change occurs after the final protocol version it may be included such as:
During the course of data collection, while randomization assignment was blinded, it became evident the primary outcome was heavily skewed right. Therefore, while the protocol cites a regression model for the primary outcome with unity link, the SAP is updated to include a log transformation followed by the same regression model.
2. Overview & Objectives of Study Design
This is used to give a brief synopsis of the study design. Typically, this can be from the synopsis in the protocol. Should include study design, dose, phase, and patient population. Authors should confirm that each objective is aligned with one or more study endpoints.
This example is for an oncology product.
This is a multicenter, double-blind, randomized study with a phase 2 portion and a phase 3 portion. Approximately X patients will be enrolled in this study. The phase 2 portion will be open label with all patients receiving study drug at one of two doses.
In Phase 2, patients only with advanced or metastatic NSCLC after failing standard therapy will be enrolled.
In Phase 3, patients with one of the following conditions will be enrolled:
- 1) advanced or metastatic breast cancer, who have failed ≥1 but <5 prior lines of chemotherapy; advanced or metastatic NSCLC after failing drug xxx-based therapy; or
- 2) hormone refractory (androgen independent) metastatic prostate cancer.
The eligibility of all patients will be determined during a 28-day screening period.
Approximately X patients with advanced and metastatic NSCLC will be enrolled. Patients are randomly assigned, with xx patients enrolled in each arm, with the arm designation and planned intervention as follows:
- Arm 1: Arm 1 Description, Dosing strategy
- Arm 2: Arm 2 Description, Dosing strategy
The study will be temporarily closed to enrollment when Z patients have been enrolled and completed at least 1 treatment cycle in each arm in phase 2. The Sponsor will notify the study sites when this occurs.
Once the study is temporarily closed to enrollment in phase 2, a PK/PD analysis will be performed to determine the RP3D. The PK/PD analysis will be done by an independent party (may define 3 rd party here) at the time 40 patients in Phase 2 have completed at least Cycle 1. This analysis will be blinded to the study team.
Remember all newly introduced abbreviations used above (e.g., RP3D and PK/PD) need to be in the list of abbreviations.
Phase 3 will not begin until RP3D has been determined based on the phase 2 PK/PD analysis as mentioned above. The dose chosen as the RP3D will constitute one arm and active control the other.
Approximately YYY patients are planned to be enrolled in the Phase 3 with one of the following diagnosis: Put in conditions for enrollment– this is in the protocol as inclusion/exclusion criteria and should match.
Patients will be randomly assigned with equal probability (1:1 ratio), with the arm designation and planned intervention as follows:
- Arm 1: Describe Arm 1, e.g., the RP3D identified in Phase 2.
- Arm 2: Describe Arm 2, e.g., the standard of care/control
For multi-stage seamless trials, it is necessary to define whether data from the initial stage will be combined with data from the follow stages, and if so how.
Data from all patients receiving the RP3D DRUG A dose in Phase 2 and Phase 3 will not be pooled for assessing the primary and secondary study endpoints. Phase 2 is for dose selection. Phase 3 will serve as independent validation and comparison of the chosen dose to active control. Therefore Phase 3 data will be analyzed separately. The primary results will be calculated only from patients enrolled in Phase 3 that have concurrent controls.
Rescue Treatment or other treatments or procedures:
This is usually detailed in the protocol and those details should be presented here if appropriate.
Section 14 of this SAP has further details regarding the schedule of events.
2.1. Phase 2 objectives
Objectives should be stated here and should match the objectives in the protocol.
Primary objective:
- • To establish the Recommended Phase 3 Dose (RP3D) based on PK/PD analysis.
Primary efficacy pharmacodynamic objective :
- • To assess DSN in treatment Cycle 1 in patients treated with Dose 1or with Dose 2. Neutrophils count will be assessed at baseline; Pre-dose during Cycle 1, Day 1, 2, 5, 6, 7, 8, 9, 10, 15.
Primary Safety Pharmacodynamic objective:
- • To assess blood pressure semi-continuously with 15-min intervals, starting 15 min pre-dose and lasting 6 h after start of infusion with drug xxx or drug yyy.
Secondary objectives:
- • To characterize the pharmacokinetic profile of Dose 1and Dose 2
- • To characterize the exposure-response relationships between measures of drug xxx exposure and pharmacodynamic endpoints of interest (e.g., duration of severe neutropenia [DSN]).
- • To characterize the exposure-safety relationships between measures of drug xxx exposure and safety events of interest.
Exploratory objectives:
- • To assess CD34 + at baseline, Days 2, 5, and 8 in Cycle 1 and Day 1 in Cycle 2
- • Quality of Life as assessed by EORTC QLQ-C30 and EQ-5D-5L
- • Disease Progression
Safety objectives:
- • Incidence, occurrence, and severity of AEs/SAEs
- • Incidence, occurrence, and severity of bone pain
- • Systemic tolerance (physical examination and safety laboratory assessments)
2.2. Phase 3 objectives
- • To assess DSN in treatment Cycle 1 in patients with advanced or metastatic breast cancer, who have failed ≥1 but <5 prior lines of chemotherapy; advanced or metastatic non-small cell lung cancer (NSCLC) after failing DRUG-based therapy. Neutrophils count will be assessed at baseline; Pre-dose during Cycle 1, Day 1, 2, 5, 6, 7, 8, 9, 10, 15.
- − Incidence of Grade 4 neutropenia (ANC <0.5 × 10 9 /L) on Days 8 and 15 in Cycles 1 to 4
- − Incidence of FN (ANC<0.5 × 10 9 /L and body temperature ≥38.3 °C) in Cycles 1 to 4
- − Neutrophil nadir during Cycle 1
- − Incidence of documented infections in Cycles 1 to 4
- − Incidence and duration of hospitalizations due to FN in Cycles 1 to 4
- − Health-related Quality of Life (QoL) questionnaire evaluated with European Organization for Research and Treatment of Cancer (EORTC) QLQ-C30 and EQ-5D-5L
- − Use of pegfilgrastim or filgrastim as treatment with neutropenia
- − Incidence of antibiotic use
- − Incidence of docetaxel dose delay, dose reduction, and/or dose discontinuation
Safety objectives .
3. Sample Size Justification
This section should contain the complete details for the justification of the sample size. This should include all details and assumptions necessary so the sample size could be independently replicated based upon provided information.
Many organizations, agencies and funders are increasingly encouraging simulation to be used for power calculations, even for designs that may have closed for sample size calculations. Simulation enables various sensitivity analyses such a sensitivity to violated assumptions, to missing data, etc. If simulation was used to calculate the sample size/power, then a reference should be made to archived simulation code. If manageable, the code could be included in a later portion of the SAP or provided elsewhere, e.g. reference to GitHub.
Note here also the phase 2 is a convenience sample and that is stated along with no hypotheses are being tested.
Phase 2 Sample Size Justification.
In the Phase 2 portion of this study, 30 patients with advanced or metastatic NSCLC will be enrolled. It is not powered for testing any statistical hypotheses but a standard sample size for this type of study to support Pk/Pd analysis. No formal hypotheses are being tested in the Phase 2 portion of this study.
Phase 3 Sample Size Justification.
Approximately 150 patients are planned to be enrolled with 1 of the following diagnoses: advanced or metastatic breast cancer, NSCLC, or HRPC. A sample size of 75 patients in each of the treatment arms DRUG A versus Standard of Care, with matching placebos achieve at least a 90% power to reject the null hypothesis of 0.65 day of inferiority in DSN between the treatment means with standard deviations of 0.75, at a significance level (alpha) of 0.05 two-sided two-sample zero-inflated Poisson model. Simulation code to confirm the power for N = 150 patients is contained in a later section.
Another example that uses simulation.
Negative binomial regression is used to test whether the intervention decreases the need for medical services in the following six months. Assuming medical service utilization will decrease from an average of 3 to 1.5, with SD = 1.25 x the mean for each group, 67 patients per group, 134 total, offers 90% power at the two-sided a = 0.05 level.

4. Randomization, stratification, blinding, and Replacement of Patients
Here, a description of the randomization procedures for the study are needed. If open label, state the same. If randomized, stratified, or any other type of grouping, that needs to be made clear. Also, address if this is a fixed randomization or dynamic as from a central IVRS/IWRS system.
This section should also contain specific information if patients are stratified, and if so how, and details on whether block randomization was performed, along with block size.
Also, if patients withdraw after consent and confirmed eligibility but before randomization, this section may contain information on whether such patients may be replaced.
Patients will be identified by a patient number assigned at the time of informed consent.
4.1. Treatment assignment
Patients will be stratified based on his or her diagnosis. Strata 1 and 2 are:
Patients will be randomized using IVRS/IWRS to 1 of the following treatment groups:
Phase 2 (10 patients in each arm):
Arm 1 : Describe treatment arm.
Arm 2: Describe treatment arm.
No blocking is used in Phase 2.
Phase 3 (75 patients enrolled in each arm):
Arm 1: Describe Arm.
Arm 2: Describe Arm.
Random blocks of 4 or 6 are used within each strata in Phase 3.
4.2. Rescue treatment/other treatments/dose escalation/other procedures are related
Describe here any other treatments/procedures that may be administered to the patient that result from efficacy/lack of efficacy or safety issues .
For example, for a pain trial.
A patient will be considered censored if they require opioids for rescue treatment. The patient will continue to be followed for safety outcomes, but efficacy assessments will terminate and will be counted as missing from that point forward. Such patients will not be replaced in the trial.
5. Definitions of Patients Populations to be analyzed
This section should clearly define all potential populations to be used in the data analyses. Each data analysis should then reference the population(s) to be used.
5.1. Analysis Sets of Phase 2
5.1.1. intent-to-treat analysis set (itt).
The intent-to-treat analysis set for Phase 2 is comprised of all Phase 2 patients that have been randomized.
The analysis of all endpoints, unless noted otherwise, will be conducted on the intent-to-treat analysis set.
5.1.2. Safety analysis set
The safety analysis set will include all patients who receive one or more doses of study drug.
5.1.3. Per Protocol Analysis Set (PP)
Patients who qualify for the ITT population who complete the study period without a major protocol deviation will be included in the PP analysis. Expected major protocol deviations include:
Also include any other circumstances which would preclude a study subject from inclusion in the per protocol population. Examples include:
- 1. Rescue therapy requiring opioids or other protocol prohibited medications
- 2. Primary outcome measures out of window
- 3. Subjects who did not meet all inclusion exclusion criteria
5.1.4. Interim Analysis Set
If the design calls for an interim analysis, define which population will be used. Usually this can denote the ITT or PP analysis sets described above. Sometimes it may include a unique set.
Should also include how it is capped, e.g., ‘first 30 patients enrolled who reach their 3-month endpoint’, or ‘first 30 patients to reach their 3-month endpoint.’
The interim analysis will use the intent-to-treat population but include only patients who have complete primary endpoint data at the time of the interim analysis.
A sensitivity analysis will be performed using the per protocol population, including only subjects with complete primary endpoint data, at the time of the analysis, and presented to the DMC.
5.1.5. Pharmacokinetic Analysis Set
Describe the conditions of the patient population utilized the PK Set.
All subjects who received at least 1 dose of any study and had at least 1 PK sample collected will be included in the PK analysis set. These subjects will be evaluated for PK unless significant protocol deviations affect the data analysis or if key dosing, dosing interruption, or sampling information is missing. For phase 3, PK samples may be collected with a schedule of collection based on the emerging data from Phase 2. Population pharmacokinetic modeling will be utilized to analyze the PK data, and optimal sampling approaches will be used to determine the PK time points for Phase 3.
5.1.6. Pharmacodynamic Analysis Set
Describe the conditions of the patient population utilized in any PD Set.
All patients who had blood pressure and DSN collected at any time during the study will be included in the PD analysis set. For phase 3, PD data may be collected with a schedule of collection to be confirmed based on the emerging data to be determined. Exploratory PK/PD and exposure-response analyses will be conducted to evaluate the effects of DRUG A on safety and efficacy endpoints. Details of these analyses will be summarized in the statistical analysis plan and may be reported outside of the main clinical study report.
5.2. Analysis Sets of Phase 3
5.2.1. intent-to-treat analysis set.
The intent-to-treat analysis set for Phase 3 is comprised of all Phase 3 patients that have been randomized.
5.2.2. Safety analysis set
The safety analysis set will be the same as the intent-to-treat analysis set for Phase 3.
5.2.3. Per Protocol Set
Also include any other circumstances which would preclude a study subject from inclusion in the per protocol population. Again, there should be sufficient detail in the SAP so that multiple people reviewing the data and the SAP would make the same judgement on who is and is not eligible for the PP set.
5.2.4. Modified intent-to-treat analysis set
Some studies include a modified intent-to-treat analysis which is a minor modification to the ITT analysis.
The intent-to-treat analysis set for Phase 3 is comprised of all Phase 3 patients that have been randomized in the study and have received at least one dose of study medication.
5.2.5. Interim Analysis Set
6. endpoints.
This section describes the primary, key secondary (if any), secondary, and exploratory endpoints and any safety endpoints that are tracked.
This section can be used to provide greater detail, description, or references for endpoints. For example, if the primary endpoint is a composite endpoint (e.g., MACE events), the set of conditions defined the presence of absence of the endpoint should be included. The primary endpoint section below provides an example.
Furthermore, this is the section where you would provide detail on how to calculate derived endpoints, e.g., if a set of survey questions were used to provide a composite score (e.g. HAQ-DI). The key secondary endpoint section provides an example.
Again here, authors should confirm that each objective is aligned with one or more study endpoints.
6.1. Primary endpoint
The primary endpoint is the time to first Major Adverse Cardiac Event (MACE). MACE events include the presence of any one or more of the following:
- 1) Non-fatal stroke
- 2) Non-fatal myocardial infarction
- 3) Heart failure leading to hospital admission
- 4) Ischemic cardiac events
- 5) Peripheral vascular disease leading to hospital admission
- 6) Cardiovascular death
Patients experiencing multiple events will have their first, not most severe, MACE event count toward the primary endpoint. All potential MACE events will be reported and sent to the blinded Clinical Events Committee (CEC). The CEC will have access to all clinical information except treatment assignment. If a patient experiences no event their days from randomization to last known office visit will be considered their censored time without an event.
6.2. Key Secondary Endpoints
The following is an example of a derived endpoint. A 20-question survey is used to produce a single score for Rheumatoid Arthritis. The endpoint is defined, described, and the analytical method detailed for its calculation. Survey instruments may have missing data for select questions. Ideally this section details how missing data is internally handled with in the calculation of the summary score or cites the paper that describes how piecewise missing data is handled.
The functional status of the subject will be assessed by means of the Disability Index of the Health Assessment Questionnaire (HAQ-DI). This 20-question instrument assesses the degree of difficulty a person has in accomplishing tasks in 8 functional areas: (1) Dressing and grooming, (2) Arising, (3) Eating, (4) Walking, (5) Hygiene (6) Reach, (7) Grip, and (8) Common Daily Activities.
Each functional area contains at least 2 questions. For each question, there is a 4-level response set that is scored from 0 (without any difficulty) to 3 (unable to do). If aids or devices or physical assistance are used for a specific functional area the maximum response of this functional area is 0 or 1 the according value is increased to a score of 2.
If “other” is marked as an aid or equipment, then this can be assigned to a group of four functional areas and will be handled as an aid or equipment for each of the four functional areas. Therefore, if the maximum score of a functional area is 0 or 1 that value is increased to a score of 2 for each of the four functional areas.
Regarding these corrections, the highest response within each functional area determines the score of that specific functional area. If no questions within a given functional area were answered, no score will be provided for that category (even if answers on aids or equipment are available).
HAQ-DI score is only calculated if there are at least 6 functional area scores available. The average of these non-missing functional area scores defines the continuous HAQ-DI score ranging from 0 to 3. If there are less than 6 functional area scores available, no imputation will be done, and the HAQ-DI will be set to missing for the corresponding assessment.
6.3. Secondary endpoints
Include additional secondary endpoints.
The following six secondary endpoints will be reported and compared. Because these are secondary endpoints, no formal multiplicity adjustments are made.
- 1. Time to first stroke within 1-year
- 2. Time to first myocardial infarction within 1-year
- 3. Time to first Heart failure leading to hospital admission within 1-year
- 4. Time to first Ischemic cardiac events within 1-year
- 5. Time to first Peripheral vascular disease leading to hospital admission within 1-year
- 6. Time to Cardiovascular death within 1-year
Each secondary endpoint listed here is tracked independently, e.g., if a patient has a peripheral artery event at 4 months and a fatal stroke at 8 months, the first event would contribute to (5) and the second event would contribute to (1) and (6). The patient would be considered censored for all other analyses after month 8 due to his death.
6.4. Exploratory endpoints
Include additional exploratory endpoints with descriptions and derivation instructions if necessary.
6.5. Safety endpoints
Detail key safety endpoints. Potentially all AEs will be tracked and reported, and all need not be listed here. But key safety concerns that will be specifically monitored should listed.
7. Statistical analyses
7.1. general principles.
This is a general section to present the planned descriptive statistics, and what software is going to be utilized to analyze the data, and, if appropriate, what quality control (QC) checks will be utilized to ensure QC on tabulations and analyses. The section should also include the validation process, if present.
Statistical analyses will be reported using summary tables, figures, and data listings. Continuous variables will be summarized with counts, means, standard deviations, medians, confidence intervals, minimums, and maximums. Categorical variables will be summarized by counts and by percentage of patients.
Formal inferential statistical analyses techniques will be discussed in subsequent sections of this SAP.
Individual patient data obtained from the case report forms (CRFs), electrocardiogram (ECG), core laboratory, PK data and any derived data will be presented in by-patient listings sorted by study phase (2 or 3), study center, and patient number.
All analyses and tabulations will be performed using SAS Version 9.3 or higher on a PC platform. Table, listings, and figures will be presented in RTF format. Upon completion, all SAS programs will be validated by an independent programmer. In addition, all program output will undergo a senior level statistical review. The validation process will be used to confirm that statistically valid methods have been implemented and that all data manipulations and calculations are accurate. Checks will be made to ensure accuracy, consistency with this plan, consistency within tables, and consistency between tables and corresponding data listings. Upon completion of validation and quality review procedures, all documentation will be collected and filed by the project statistician or designee.
Missing or invalid data will be generally treated as missing, not imputed, unless otherwise stated.
7.2. Major protocol violations
This section is generally from the protocol but is presented here and if any updates and/or special analyses are to be done, they are described here. Such as analyzing patients who had major deviations where data verification was not possible . This may also provide additional detail describing how individual cases are adjudicated to decide if they meet the level of a protocol deviation.
Major protocol violations will be identified by the clinical study team and provided to Biostatistics prior to database lock. A protocol deviation is any noncompliance with the clinical trial protocol or Good Clinical Practice (GCP). The noncompliance may be either on the part of the patient, the investigator, or the study site staff. All patients with major protocol violations will be listed by study center and patient numbers. A protocol deviation committee consisting of the lead study coordinator, medical monitor and lead statistician will review all cases prior to unblinded. Deviations will be determined without knowledge of randomization assignment prior to database lock.
7.3. Patient Enrollment and Disposition
Describe how enrollment data is to be analyzed and summarized.
Patient enrollment by site will be tabulated by treatment arm and overall.
Patient disposition will be summarized by treatment arm and overall. This will include number of patients screened, number of patients who were screen failures with reason, number of patients who consented, and number of patients who were randomized.
The summary will include the number and percentage of patients in each of the defined analysis populations in Section 5 above. In addition, frequency counts and percentages of patients’ reported reasons for ending the study will be summarized.
A listing will be presented to describe patient study arm, date of first and last dose, date of last visit or contact, total number of completed cycles, and the reason for ending the study for each patient.
Listings of inclusion/exclusion criteria responses will also be provided.
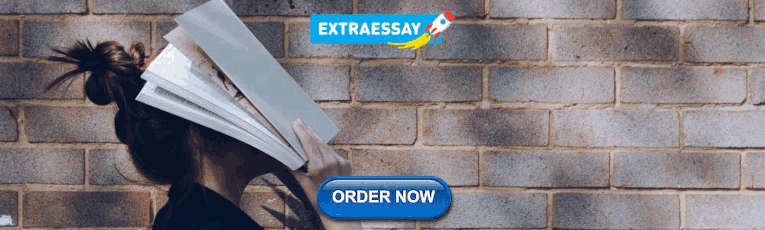
7.4. Description of Demographic and Baseline Characteristics
Describe the summarization or analysis of this data. Note the study populations used for each analysis are detailed.
A summary of age, gender, race, ethnicity, vital signs, ECOG status, tumor staging, tumor type, and prior: medical surgery, radiotherapy, disease surgery, and chemotherapy (Yes/No); along with the number of prior chemotherapy regimens will be presented.
The categorical (discrete) variables will be summarized using counts and percentages. The continuous variables will be summarized using mean, median, standard deviation, and range (maximum, minimum).
All demographic and baseline characteristics will be listed by study center, and subject number.
These summaries will include patients in the ITT population and PP population. Summary statistics described here will be presented for each study arm and overall.
7.5. Medical history
This section may be applicable primarily for regulatory trials. Patient medical histories and pre-existing conditions are typically recorded at baseline and included by randomization assignment for the Safety Analysis Set or ITT set.
Medical history data will be coded by system organ class and preferred term, using the MedDRA dictionary.
Medical history will be summarized by body system for each study arm in the Safety Population. The table will be sorted in alphabetic order by system organ class, as well as by incidence and preferred term, and the statistics n and % will be presented by study arm where: n is the number of subjects who present at least one occurrence of the medical history and % is the percentage of subjects. The denominator used for calculating the percentages will be the total number of subjects included in the Safety Analysis set for each study arm.
7.6. Specific Relevant Medical History
This section may be more applicable to registration trials and would be used to summarize analysis of medical history or medical condition that may be germane and specific to protocol and/or analysis of efficacy or safety data. For example, demographics are relevant across all trials, but specific characteristics, attributions of disease, or disease duration are specific to the topic being studies.
Histology of NSCLC, disease status, prior treatment, and best response will be summarized in the Phase 2 Study Population using frequency counts and percentage.
For the Phase 3 study, History of advanced or metastatic breast cancer, who have failed ≥1 but <5 prior lines of chemotherapy; advanced or metastatic NSCLC after failing platinum-based therapy; or hormone refractory (androgen independent) metastatic prostate cancer will be summarized using counts and percentages.
7.7. Concomitant medications
Concomitant medications relevant to the study that a patient is taking at trial entry or initiated during trial participation may be summarized here.
All medication data will be coded by drug class and indication, using the WHODrug dictionary. All medication taken prior to the first dose of study drug will be classified as prior medication. All medication taken on or after the first dose of study drug will be classified as concomitant medication. Medications with start and stop date that bracket the date of first dose will be summarized as both prior and concomitant medication.
For the purpose of inclusion concomitant medication tables, incomplete medication start and stop date will be imputed as detailed in Section11. Based on imputed start and stop dates, medications that started on or after date of first dose will be included in the concomitant medications table.
Concomitant medications will be summarized in the Safety Population by giving the number and percentage of subjects by preferred term within each therapeutic class, with therapeutic class and medications in each class sorted in alphabetical order. The total number of drugs in each selected therapeutic class will also be presented, where, for example two drugs each belonging to the same class will only contribute once to the presented count.
All prior and concomitant medications, as well as medical procedures will be listed by study center, and subject number.
For the Phase 3 study, the number and percentage of patients who use antibiotics will be summarized and tested for differences between the two arms using Fisher's exact test. Also, the number and percentage of patients who use DRUG D as treatment for neutropenia will be summarized and tested for differences between the two arms using Fisher's exact test.
7.8. Physical examination
Summary methodologies and analyses if appropriate in detail.
7.9. Study Drug Exposure
This section explains in detail how the Drug Exposure data will be summarized and, if appropriate, analyzed (i.e., missing doses between dose groups, or dose adjustments within subgroups).
For each study phase, study treatment exposure will be summarized in the Safety Population.
For each treatment arm for each product, the following will be summarized using descriptive statistics by study arm and overall:
- • Duration of exposure, calculated as (date of last dose – date of first dose+1).
- • Number of cycles received per patient.
- • Number of cycles with dose modification and (or) dose delay.
- • Reasons for dose deviations from planned therapy.
All study drug administration data will be listed by study center and patient number.
For the Phase 3 study, the number and percentage of patients who have dose delayed, dose delayed, and/or dose discontinued will be summarized by treatment arm and tested for differences between the two arms using Fisher's exact test.
7.10. Efficacy analysis
Perhaps the most important section of the SAP. This section contains all necessary details for the primary and secondary analyses. The section should contain the explicit hypothesis tests for all primary and secondary analyses, the completely specified model(s) to be used, the alpha-level or Type 1 error strategies to be incorporated through the hierarchy of statistical tests.
In some cases, it may be beneficial to include example statistical code for the primary and secondary analyses, especially if methodologies are not standard.
The primary efficacy endpoint is the Phase 3 analysis of the Duration of Severe Neutropenia (DSN) in Cycle 1 of treatment. However, to define efficacy analyses in chronological order, Phase 2 analyses are described first.
7.10.1. Phase 2
As shown, the null and alternative statistical hypothesis are described. Furthermore, this section illustrates there may be cases where a separate statistical analysis plan, e.g., for PK/PD analyses, are referenced. This is sometimes the case for specialized analyses which may have been authored by a subcontractor or consultant.
All of the pharmacokinetic and pharmacodynamics efficacy and safety endpoints will be analyzed according to the separate PK SAP.
7.10.1.1. Primary efficacy exploratory analysis
The ANC will be summarized by treatment arm and day. The nadir, the day of the nadir, and the percentage of patients in each treatment arm who have Severe Neutropenia will also be summarized.
In addition, an exploratory analysis to assess DSN in treatment Cycle 1 in patients treated with DRUG A with DRUG B at two doses and DRUG A with DRUG C at two does each at, using the Jonckheere-Terpstra Test for Ordered Alternatives. With this statistical procedure, the null hypothesis of equality among treatment group means will be tested (μ j 's, j = 1, 2, 3, 4)
against the alternative in which order is specified
where at least one of the inequalities is strict. The mean indices have the following interpretation: 1 = DRUG A DRUG B dose1; 2 = DRUG A DRUG B dose2, 3 = DRUG A DRUG C dose1 and 4 = DRUG A DRUG C dose2. The statistically significant rejection of the null hypothesis will be interpreted, that there is an ordered alternative of responses as indicated by the alternative hypothesis H 1 .
If rejection of a primary hypothesis leads to secondary hypotheses being tested, this condition should be clearly detailed and the subsequent tests be described. For example:
If the null hypothesis is rejected, then pair wise Wilcoxon tests will be performed to aid in the assessment of which treatment(s) contributed to the rejection of the null hypothesis.
Since the Jonckheere-Terpstra Test for Ordered Alternatives is a non-parametric test, this will be performed in SAS using PROC FREQ with JT option. The DSN for this analysis is calculated as in a further Section and since this is an exploratory, non-parametric analysis, no adjustment will be made for the potential number of 0 values of the DSN. The non-parametric nature of the test factors these into consideration.
The primary efficacy analysis will be conducted for the ITT and PP populations.
7.10.1.2. Exploratory analyses
A second exploratory analysis is to assess CD34 + at baseline, Days 2, 5, and 8 in Cycle 1 and Day 1 in Cycle 2. This data will be summarized by Cycle and Day and the differences between the treatment arms will be assessed by day using an Analysis of Variance (ANOVA) with a term for ARM. Pairwise differences will be assessed using pre-defined contrasts.
The primary efficacy analysis will be conducted for the ITT population.
7.10.2. Phase 3
7.10.2.1. primary analysis.
While the protocol is likely to contain near full details for the primary analysis, the SAP offers additional space and should include all details so that the primary analysis is completely replicable and requires no interpretation or additional assumptions. For example, with a simple chi-square test, this will include the precise test used, whether it will include a continuity correction, when (or if) a Fisher's exact test might be used in lieu of the chi-square test, and the conditions that would necessitate the change. It should specifically include the population on which the primary analysis will be conducted.
This section should contain the precise hypothesis to be tested with null (H 0 ) and alternative (H 1 ) hypotheses, including whether it is a superiority, equivalence, or non-inferiority testing framework. Because it uses a slightly non-standard model, the SAP also provides illustrative code that defines some of the finer statistical aspects of the predefined model. It should also be clear whether tests are one or two-tailed and the alpha-level used for all statistical tests.
The primary hypothesis of the Phase 3 study is to establish the non-inferiority of DRUG A to DRUG B with respect to DSN in Cycle 1. This non-inferiority trial design will utilize a difference (arm 2 minus arm 1) of 0.65 days (non-inferiority margin) in DSN in Cycle 1 as the largest acceptable difference between DRUG A and DRUG B. The non-inferiority test will evaluate the null hypothesis that the difference in means is greater than or equal to 0.65
against the alternative hypothesis that the difference in means is less than 0.65
DRUG A will be considered non-inferior to DRUG B if in Cycle 1, the upper limit of the 2-sided 95% confidence interval for the true difference in mean duration of Grade 4 neutropenia was <0.65 days.
The analysis of this data will assume a Zero Inflated Poisson Model and will conducted using PROC GENMOD as:

Confidence intervals for the difference will be calculated from the estimate statement and those confidence intervals will be utilized in the assessment of non-inferiority. Also, if the null hypothesis is rejected and non-inferiority is established, then superiority will be tested using the p-value from the above analysis.
Here again when Ho is rejected leading to the conclusion of non-inferiority, superiority is subsequently tested. All potential pre-defied analyses resulting from the result of pre-defined analysis should be clearly stated along with the condition that would lead to their testing.
Another circumstance which may result in the model not being entirely prespecified a priori is when it involves a complicated covariance structure. In such a case the method used to select the covariance structure can be pre-specified. For example, below the primary model is prespecified but the correlation structure of the MMRM model is chosen according to the option that provides the optimal AIC:
The primary endpoint, change from baseline, will be analyzed using Mixed-effect Model Repeated Measure (MMRM) statistics. The repeated-measures analysis will be based on the restricted maximum likelihood method assuming an unstructured covariance structure to model the within-subject errors. The model will include treatment group (Placebo = 0, Treatment = 1), location (US = 0, OUS = 1), visit (Week 0, 2, 4, 6, 8, 10, and 12), and treatment-by-visit interaction as fixed effects and baseline as a covariate. Treatment effects will be calculated via LSMEANS for each timepoint but the treatment effect at Week 12 will be considered the Primary Efficacy Endpoint. Patient will be considered a random effect.
The data collected after receiving rescue therapy will be set to missing. Therefore, the MMRM analysis assumes a missing-at-random (MAR) mechanism for missing data due to dropout and post-rescue data.
In addition to using an (1) unstructured covariance matrix, the same model will be fit using a (2) compound symmetric covariance matrix and (3) and AR(1) covariance matrix to model the covariance in repeated measures within patient.
Akaike Information Criteria (AIC) will be calculated for each of the three models. The AIC form used will be AIC = 2 K–2 log(L) where K is the number of parameters and L is the log-likelihood. The model with the lowest AIC will be chosen as the primary model and the estimated treatment effect will be reported based upon this model.
The primary efficacy analysis will be reported for the ITT and PP populations.
7.10.2.2. Secondary analyses
The ANC will be summarized for each day within Cycle 1. The neutrophil nadir will be summarized for both treatment arms and tested for difference using a two-sample t -test at the 2-sided 0.05 level.
For all four Cycles and all days, the number and percentage of patients who have:
- 1) Grade 4 Neutropenia
- 2) Incidence of FN
Will be summarized and tested for differences between the treatment arms using Fisher's exact test.
Occasionally the chosen statistical test may depend on the final form of the data. This should be detailed in the SAP. For example, if the data is predicted to be normally distributed, but there is concern, a priori , regarding this assumption, it can be prospectively defined that a non-parametric test could be used. In such a case the conditions that would lead to the change should be clearly defined and the primary analysis method for if the conditions are or are not met. For example:
Normality of the primary endpoint will be tested using the Kolmogorov–Smirnov test. If the null hypothesis of normality cannot be rejected, p > 0.05, then a two-sample t -test will be used to test the null hypothesis of a difference in means. If p ≤ 0.05 for the K–S test, then normality will be rejected and a Wilcoxon rank sum test will be used to test for a difference in central tendency of the two groups.
7.10.2.3. Exploratory analyses
The statistical methods should be detailed for each exploratory analysis separately. This is also the place to prospectively describe statistical graphics (e.g., histograms, boxplots, barplots, etc.) that will be created. Just like with primary analysis, it is beneficial to define the parameters for the statistical graphics, e.g., bin size for histograms, what categories CDFs may be split by for subsets, etc.
This may oftentimes be a very long section because lots of exploratory analyses may be planned.
During Cycle 1, the nadir for Absolute Neutrophil Count (ANC) will be calculated:
- 1) If that nadir is not grade 4 in nature (ANC <0.5 × 10 9 /L), then that patient will have a DSN equal to 0 days. That is, the duration is 0 days since the patient did not have severe neutropenia.
- 2) If the nadir is grade 4 then a regression of ANC vs days will conducted with the first day utilized in the regression being the day the nadir occurred. From this regression, the time (in days) at which the predicted ANC from the regression is ≥ 0.5 × 10 9 /L will be the DSN for that patient.
The regression analysis for each patient will be performed using PROC MIXED in SAS with both a random slope and intercept and, when possible, an unstructured covariance model. If the parameters are not estimable (lack of model convergence) using an unstructured covariance model, then ordinary least squares estimates of the slope and intercept will be performed. The difference will be tested using a Wilcoxon test and confidence intervals will be established using a boot strap methodology.
The results of the Quality of Life assessments will be summarized via descriptive statistics for each treatment group and differences tested using a t -test. Since this is exploratory no adjustment for multiple tests will be utilized.
All exploratory analysis will be conducted for the ITT population.
7.10.2.4. Subset analyses
This section should contain all prespecified subset analyses. For example:
The primary, key secondary and secondary analysis will be repeated by.
- a. Diabetes Yes vs. No
- b. Prior MI Yes vs. No
- 3. Age <40 vs. Age ≥ 40
- If ever a subset has <5% of the total data, analysis of that subset will be omitted.
All analyses will be performed on the ITT populations and reported with p-values and 95% confidence intervals. Results, however, will be viewed as exploratory/hypothesis generating.
In addition to tables for each analysis, a forest plot will be produced for each outcome (primary, key secondary and all secondaries) illustrating treatment effects and 95% CIs by each subset.
7.10.2.5. Sensitivity analyses & missing data
This section should contain all specified sensitivity analyses including sensitivity analyses to evaluate the impact of missing data. For example, to be used with the MMRM methods from above:
A number of sensitivity analyses will be performed to study the effect of missing data on the primary efficacy analysis.
Below are the descriptions for the imputation methods that will be used throughout the efficacy analyses. For example:
The primary efficacy analysis will be performed with observed cases (OC). Missing values remain missing. The primary efficacy analysis will be repeated with the following methods:
- (1) For the primary effectiveness analysis at Week 12, multiple imputation will be used to assign a value to those cases with missing data. The full conditional specification method with predictive means matching as described in Berglund & Heeringa (2014) will be used. This method uses all of an individual's known primary outcome measures at Baseline, 2, 4, 6, 8, 10, and/or 12 weeks to impute any missing values.
- (2) Last observation carried forward (LOCF). Baseline measurements will not be carried forward to post-baseline. Only post-baseline measurements will be LOCF. For the composite endpoints, the last non-missing post-baseline observation will be carried forward to subsequent visits for each individual component first, and then the composite endpoints using individual components imputed by LOCF will be calculated as described above. If a subject does not have a non-missing observed record for a post-baseline visit, the last post-baseline record prior to the missed visit will be used for this post-baseline visit. If the last non-missing observation prior to the missing visits cannot be determined due to multiple measurements occurring at the same time or the time not available within the same day, the worst outcome will be used for LOCF. If missing components still exist after LOCF, the composite endpoints will be calculated using the same rules as described in OC.
- (3) Tipping point analysis. If the primary analysis is statistically significant, a Tipping Point analysis will be used. For patients randomized to treatment who fail to complete the 12-week study period, 0.1 will be subtracted to each of their 12-week primary outcome scores (made worse), while for patients randomized to control who fail to complete the 12-week study period, 0.1 will be added to each of their average last 14 available days (made better), and the analysis repeated. This will be repeated, in increments of 0.1, until the primary analysis fails to be statistically significant at the α = 0.025 level. The increment value at which statistical significance ceases will be reported as the Tipping point.
7.10.2.6. Multiplicity control
Type 1 error control is an important part of a clinical trial. Tight Type 1 error control requires the order of testing and methods for error control to be pre-defined. This increases the integrity and validity of inferences found to be statistically significant.
If multiple primary hypotheses are to be tested, or formal claims are desired for secondary endpoints, then multiplicity (Type 1 error) control should be strictly controlled.
Methods to test multiple hypotheses at once such a Bonferroni method, Hochberg method or Holm's method, should be detailed. Or if a hierarchical testing procedure or hierarchical family of tests is used, it should be clearly defined along with the order of testing.
The primary efficacy analysis will be tested at the one-sided 0.025 level. If it is statistically significance, then the four secondary endpoints will be tested using Hochberg's method.
Hochberg's method is used to control the familywise Type 1 error rate (FWER) among these 4 secondary endpoints. If and only if the primary efficacy objective is met, the Hochberg's step-up procedure (Hochberg 1988) will be used to control the FWER at a 1-sided significance level of 0.025 for the following 4 secondary endpoints:
- Endpoint 1 at Week 12 from Day 0
Test: H 0 : pt - pc = 0 H A : pt - pc > 0, using stratified CMH test, as detailed in the secondary analysis section.
- Endpoint 2 Week 12 from baseline where baseline is the day of informed consent
Test: H 0 : pt - pc = 0 H A : pt - pc > 0, using stratified CMH test.
- Endpoint 3 at Week 12 from baseline on the day of informed consent
- Endpoint 4 at Week 12 from baseline on the day of informed consent
The procedure ranks the p-values from the above 4 tests from the least significant (largest p-value, p [ 4 ]) to the most significant (smallest p-value, p [ 1 ]) and examines the other p-values in a sequential manner until it reaches the most significant one, i.e., p [ 4 ] > p [ 3 ] > p [ 2 ] > p [ 1 ].
The decision rule for the Hochberg procedure is defined as follows:
- Step 1. If [ 4 ] > 0.025, retain H [ 4 ] and go to the next step, here H [ 4 ] is the hypothesis corresponding to p [ 4 ], i.e., the hypothesis with the largest p-value. Otherwise reject all hypotheses and stop.
- Step 2. If p [ 3 ] > 0.025/2, retain H [ 3 ] and go to the next step. Otherwise reject all hypotheses and stop.
- Steps 3. If p [ 2 ] > 0.025/3, retain H [ 2 ] and go to the next step. Otherwise reject all remaining hypotheses and stop.
- Steps 4. If p [ 1 ] > 0.025/4, retain H [ 1 ] otherwise reject it.
The adjusted p-values are calculated as detailed below:
Adjusted p[i] = p(4) for i = 4 other adjusted p[i] = minimum of [adjusted p[i+1], (5-i)*p[i]] for i = 3, 2, 1.
If any adjusted p-value exceeds 1, it is set to 1. Using this procedure, any adjusted one-sided p-value that is < 0.025 is statistically significant and supports a claim for the corresponding endpoint, while any adjusted p-value ≥0.025 is not statistically significant. Both adjusted and unadjusted p-values will be reported.
7.11. Safety analysis
Frequently no specific hypotheses are tested for safety. Rather adverse events and their severity (e.g., mild, moderate, severe) are recorded and presented in tabular form. These may be grouped by body system or type (e.g., neurological, GI, etc.).
The Safety analysis set will be used for all safety analysis. Patients will be evaluable for safety analysis if they receive at least one dose of study drug. All subjects receiving a dose of study drug will be included in all safety summaries. The safety data will be presented by study arm in individual listings and summary tables, including frequency tables for adverse events and frequency and shift tables for laboratory variables. All adverse events and abnormal laboratory variables will be assessed according to the NCI CTCAE (v 4.0) grading system. Descriptive statistics will be used to summarize ECOG performance status. Vital signs will be reported in listings. AEs and SAEs will be reported in combined tables. However, SAEs will be tabulated in their own table as well.
All safety information will be listed by study center and subject number.
7.11.1. Adverse events (AEs)
For the final analyses of the safety and tolerance of study drug, all treatment - emergent and overall incidences of adverse events will be summarized by system organ class and by preferred terms (MedDRA). AEs will be considered as treatment - emergent adverse events (TEAE) if onset is on or after the initiation of study treatment. Adverse events with missing onset dates will be summarized as TEAE regardless of severity and relationship to study medication.
The incidence of adverse events by severity and/or CTCAE adverse events grade (mild, moderate, severe, life threatening or death) and by relationship to study drug will be tabulated similarly. Each adverse event will be reported by greatest known severity and by strongest relationship to the study drug.
Each patient will be counted only once within a system organ class or a preferred term by using the AEs with the highest severity grade.
All information pertaining to AEs noted during the study will be listed per patient, detailing verbatim, preferred term, system organ class, start date, stop date, severity, and relationship to study treatment. AE onset will be shown relative (in number of days) to the day of the first study treatment.
For the Phase 3 study, the percentage of patients with an adverse event and the distribution of adverse events across CTCAE grades will be tested for differences between the two treatment arms using Fisher's exact test.
For the Phase 3 study, the number and percentage of patients who have infections will be summarized for each treatment group and tested for differences using Fisher's exact test. Also, the number and percentage of patients who have hospitalizations due to FN will be summarized by cycle and overall for each treatment group and tested for differences using Fisher's exact test.
7.11.2. Serious adverse events
All serious adverse events will be listed by study arm.
7.11.3. Adverse events leading to discontinuation from study
All adverse events leading to discontinuation from study will be listed by study arm.
7.11.4. Deaths
All deaths within 30 days of last study treatment will be listed by study arm. Treatment emergent deaths are those deaths within 30 days of last dose of any study therapy. Early deaths are those deaths within 60 days of the first dose of study therapy.
Treatment emergent and/or early deaths will be tabulated and summarized by treatment groups.
For Phase 3, The difference in the percentage of patients in the percentage of patients of who had treatment emergent deaths and who had early deaths will be tested using Fisher's exact test.
7.11.5. Clinical Laboratory Tests
Safety laboratory data will include clinical chemistries, hematology, and urinalysis. Safety summaries in the form of shift tables for key laboratory parameters showing the number and percentage of patients who experience changes in laboratory parameters during the course of the study (e.g., change from normal to high, based on the laboratory reference ranges) will be displayed. Also shift tables for changes in CTCAE grades will be summarized by counts and percents. If appropriate for specific time points, differences in distributions in shifts between the two treatment arms in Phase 3 will be evaluated using Fisher's exact test.
Descriptive summary statistics (mean, standard deviation, median, minimum, maximum, frequencies, and percentages, as appropriate) for laboratory values will be presented at baseline, the follow-up time points, and change from baseline for each study treatment arm.
All laboratory data, values, units, normal reference range, and out-of-range flags collected in the clinical database will be included in by-patient listings for further medical review.
7.11.6. Vital signs
Vital signs (including temperature, respiratory rate, blood pressure, heart rate, and weight) will be presented descriptively at baseline and for each follow-up time point for each study treatment arm. The number (n), mean, standard deviation, median, range will be presented. Changes from baseline to each time point will also be summarized as well as shifts from normal to abnormal results.
All vital sign parameters will be included in by-patient listings for further medical review.
7.11.7. ECGs
For patients in the Phase 3 study, will be analyzed for QTc prolongation by Fridericia's adjustment to QTc. The average of the three replicates will be used and compared to the average of the three replicates done just prior to the start of the infusion. The incidence of QTC prolongation by either calculation of >30 and > 60 ms will be presented. The incidence of QTc prolongation by either calculation of >480 ms post-infusion will be presented.
All ECGs will be summarized descriptively using N, mean, standard deviation, median, and range for each treatment arm at each visit ECGs are collected (see Section 13 ).
All ECG parameters will be included in by-patient listings for further medical review.
7.11.8. Other Safety Parameters
The health related QOL EORTC QLQ-C30 and the EQ-5D-dL questionnaires will be summarized for each visit performance status was assessed and as changes from the baseline assessment. Differences between treatment arms will be tested using a t -test for each time point where the data is collected.
7.11.9. Population Pharmacokinetic Analysis
This is the spot for details of this analysis if any. If the analysis is detailed using specialized Population PK software a separate PK analysis plan may be needed.
Population pharmacokinetic analyses will be conducted to evaluate the effect of intrinsic and extrinsic factors on the PK of DRUG A and its active metabolite(s), if identified, in humans. Intrinsic factors such as gender, age, hepatic or renal impairment, and race and/or ethnicity and extrinsic factors such as concomitant drugs, herbal products will be assessed in relationship to drug exposure according to FDA Guidance for Industry, “Population Pharmacokinetics”.
Further details of PK analysis will be presented in the PK analysis plan.
8. Interim analysis
If an interim analysis(es) is going to be performed describe it here. Include number of patients, alpha level adjustments, statistical methodologies, etc. This may include a choice to reference the methodology described above or provide full detail of the analytical methods at the interim analysis. For example:
The study will incorporate one interim analysis after 100 patients, 2/3 of the total sample size, have reached their primary 12-week outcome. An O'Brien-Fleming stopping rule will be used. The independent statistician will report the results of the interim analysis only to the Data Monitoring Committee.
The primary analysis method described above will be used after 100 patients meeting the ITT criteria have been enrolled and reached their 12-week primary efficacy outcome.
The one-sided p-value from the primary analysis will be compared to a critical value of 0.0071. If the p-value ≤0.0071, the DMC may recommend the trial stop early for overwhelming success. Otherwise, provided no safety concerns, the DMC will recommend the trial continue. If the study continues to the maximum sample size, a critical value of 0.0226 will be used to account for the 0.0071 error spend at the interim analysis.
Alternatively, some adaptive trials have a separate, smaller Interim Statistical Analysis Plan (iSAP) that may be referenced. This may be done for more complex adaptive trials where a small report is preferred to a section of the SAP. Alternatively, sometimes this is done to blind investigators to details of the interim analysis that could lead investigators to reverse engineer an effect size and become partially unblinded. For example, see Hager et al. in the references. Additional file #2 ( https://static-content.springer.com/esm/art%3A10.1186%2Fs13063-019-3254-2/MediaObjects/13063_2019_3254_MOESM2_ESM.pdf )
is an adaptive design report specifically detailing the calculations that take place only at the interim analyses.
The study will include a futility analysis and potential sample size re-estimation after ½ of enrolled patients have achieved their primary 12-week outcomes. Full details of the futility analysis and sample size re-estimated are included in the Interim Statistical Analysis Plan.
The futility is a non-binding futility analysis and Type 1 error is conserved even if the futility rule is achieved but not invoked. The independent statistical will provide results to the Data Monitoring Committee (DMC) at the interim analysis.
19. Statistical Analysis Changes From the Protocol
Describe changes in methodology that deviate from protocol. There should almost always be none, but if some new methodology is appropriate that was not known at protocol time, that may be described here. For example, if the blinded data was analyzed after the protocol was complete but before SAP completion and database lock. At that time, perhaps it became apparent that the protocol-defined method may not be statistically appropriate for the primary analysis. Two examples:
No changes were made from the original protocol.
Also include a statement to cover how necessary, and ideally rare, deviations from the SAP will be communicated.
Any necessary deviations from these guidelines will be.
- • documented in the final clinical study report (CSR)
- • include the reason the predefined methods here were not appropriate
- • detail why the updated methods represent sound statistical reasoning.
10. Conventions
The example here is a standard convention that is almost universally accepted. The section should, however, be tailored to each protocol's requirements.
The precision of original measurements will be maintained in summaries, when possible. Means, medians and standard deviations will be presented with an increased level of precision; means and medians will be presented to one more decimal place than the raw data, and the standard deviations will be presented to two more decimal places than the raw data.
Summaries of continuous variables that have some values recorded using approximate values (e.g., lab values < 0.001 or >200) will use imputed values. The approximate values will be imputed using the closest exact value for that measurement. For tables where rounding is required, rounding will be done to the nearest round-off unit. For example, if the round-off unit is the ones place (i.e., integers), values ≥ XX.5 will be rounded up to XX+1 while values < XX.5 will be rounded down to XX.
Percentages will be based on available data and denominators will generally exclude missing values. For frequency counts of categorical variables, categories whose counts are zero will be displayed for the sake of completeness. For example, if none of the patients discontinue due to “lost to follow-up,” this reason will be included in the table with a count of 0. Categories with zero counts will not have zero percentages displayed.
For adverse event incidence tables:
- • The order of SOCs presented in tables will be according to the internationally agreed order of SOCs according to MedDRA. Within each SOC, the preferred terms will be shown in alphabetic order.
- • Patients who have multiple events in the same SOC and/or preferred term will be counted only once at each level of summation (overall, by SOC, and by preferred term) in the tables. For summaries of AEs by severity, only the highest severity of AE will be counted at each level of summation (overall, by SOC, and by preferred term) in the tables. For summaries of related AEs, patients with more than one related AE will be counted only once at each level of summation (overall, by SOC, and by preferred term) in the tables.
11. Standard Calculations
The example here is a standard conventions that is almost universally accepted. The section should, however, be tailored to each protocol's requirements.
Variables requiring calculation will be derived using the following formulas:
Study day – For a given date (date), study day is calculated as days since the date of first dose of study drug (firstdose):
- • Study day = date – firstdose + 1, where date ≥ firstdose
- • Study day = date – firstdose, where date < firstdose
Days – Durations, expressed in days between one date (date1) and another later date (date2), are calculated using the following formula: duration in days = (date2-date1).
Weeks – Durations, expressed in weeks between one date (date1) and another later date (date2), are calculated using the following formula: duration in weeks = (date2-date1)/7.
Months – Durations, expressed in months between one date (date1) and another later date (date2), are calculated using the following formula: duration in months = (date2-date1)/30.4.
Years – Durations, expressed in years between one date (date1) and another later date (date2), are calculated using the following formula: duration in years = (date2-date1)/365.25.
Minutes – Durations, expressed in minutes between one timepoint (time1) and another later timepoint (time2), are calculated using the following formula: duration in minutes = (time2-time1)/60.
Age – The patient's age is calculated as the number of years from the subject's date of birth to the date of randomization into the study:
- • Age = ([Randomization Date - Date of Birth]/365.25).
12. Imputation of Dates
Add specific cases and examples if germane to study i.e., disease diagnosis dates.
12.1. Incomplete cancer diagnosis
If day is missing, day will be set to 15th of the month, or date of first dose, whichever is earlier. If month and day are missing, month and day will be set to July 1st, or date of first dose, whichever is earlier.
12.2. Adverse event
If onset date is completely missing, onset date is set to date of first dose unless end date is before date of first dose, in which case the onset date is set to 28 days prior to end date.
If (year is present and month and day are missing) or (year and day are present and month is missing):
- • If year = year of first dose, then set month and day to month and day of first dose unless end date is before date of first dose, in which case the onset date is set to 28 days prior to end date.
- • If year < year of first dose, then set month and day to December 31st.
- • If year > year of first dose, then set month and day to January 1st.
If month and year are present and day is missing:
- • If year = year of first dose and if month = month of first dose then set day to day of first dose date unless end date is before date of first dose, in which case the onset date is set to 28 days prior to end date.
- • if month < month of first dose then set day to last day of month
- • if month > month of first dose then set day to 1st day of month
- • if year < year of first dose then set day to last day of month
- • if year > year of first dose then set day to 1st day of month
For all other cases, set onset date to date of first dose unless end date is before date of first dose, in which case the onset date is set to 28 days prior to end date.
12.3. Concomitant Medications
If start date is completely missing: start date will not be imputed.
If (year is present and month and day are missing) or (year and day are present and month is missing): set month and day to January 1.
If year and month are present and day is missing: set day to 1st day of month.
If end date is completely missing: end date will not be imputed.
If (year is present and month and day are missing) or (year and day are present and month is missing): set month and day to December 31.
If year and month are present and day is missing: set day to last day of the month.
Any partial dates will be displayed in data listings without imputation of missing days and/or months (e.g., MAR2011, 2009). No other imputation of missing data will be performed.
13. Statistical packages
Include here all software products/statistical packages used in creation of simulations for calculating sample sizes, used for other statistical needs in this SAP, as well as the expected statistical package(s) expected to be used in the final analyses or interim analyses.
All analyses and tabulations will be performed using SAS Version 9.3 or higher on a PC platform. R Version 3.1 or higher may be used for statistical graphics.
13.1. SAP sample references
References should be provided for unique methodology and/or for subject-specific endpoints, e.g., papers that described a validated endpoint. References may also be provided for software and specific software packages. The following references are not necessary to cite but are frequently cited within SAPs and/or protocols.
- 1. International Conference on Harmonisation of Technical Requirements for Registration of Pharmaceuticals for Human Use, ICH Harmonised Tripartite Guideline, Statistical Principles for Clinical Trials (E9), 5 February 1998.
- 2. International Conference on Harmonisation of Technical Requirements for Registration of Pharmaceuticals for Human Use, ICH Harmonised Tripartite Guideline, Structure and Content of Clinical Study Reports (E3), 30 November 1995.
- 3. International Federation of Pharmaceutical Manufacturers and Associations. Medical Dictionary for Regulatory Activities (MedDRA). Version 14.0 Reston, Virginia, USA; 2008.
- 4. WHO Collaborating Center for International Drug Monitoring. WHO Drug Dictionary. June 2012 B Format edition. Uppsala, Sweden; 2008.
- 5. SAS Institute Inc. SAS Version 9.1. Cary, NC, USA; 2002–2003.
- 6. Hager, D.N., Hooper, M.H., Bernard, G.R. et al. The Vitamin C, Thiamine and Steroids in Sepsis (VICTAS) Protocol: a prospective, multi-center, double-blind, adaptive sample size, randomized, placebo-controlled, clinical trial. Trials 20, 197 (2019). https://doi.org/10.1186/s13063-019-3254-2
- 7. Berry Consultants. Adaptive Design Report for a Trial of the Virginia Cocktail. Supplementary materials to Hager et al. (above). https://static-content.springer.com/esm/art%3A10.1186%2Fs13063-019-3254-2/MediaObjects/13063_2019_3254_MOESM2_ESM.pdf
14. Schedule of events
To make following the sequence of events and timing of patient assessments, the schedule of events from the protocol should be reproduced here. It may be necessary to insert other statistical events into the schedule i.e., interim analyses, second randomization schemes, timing of final analysis, or analysis patient responder status.
15. List of Tables, listings, and figures
This section may contain a numbered list of Tables, Listing and Figures to be presented. It is recommended that the primary statistician use partial data without randomization assignments, or dummy randomization assignments, and execute the SAP. This will elucidate if there are uncertainties or ambiguities in the SAP that need to be clarified prior to database lock and breaking of the blind.
Some SAPs, though admittedly rare, may also include pseudocode and table shells or example figures [ 11 ].
16. Discussion
One weakness of this paper is that it leaves as out of scope the presentation of a complete “exemplar” SAP for a single trial. Fortunately, examples of complete single-trial SAPs can increasingly be found as supplemental materials attached to trial records on clinicaltrials.gov or in supplemental online-only materials for published trials [ [11] , [12] , [13] , [14] , [15] ]. For example, a recent search on clinicaltrials.gov for completed interventional trials with the keyword “malaria” that included an SAP returned 51 records. Alternately, SAPs can sometimes be found as public or global ‘goods’ on open repositories, or via basic internet searches. One consequence of the multiple-trial approach for examples used herein is that it could create conceptual disconnects if table shells, listing shells, or other shells were added to the example SAP. Beyond single complete SAPs, it may be helpful to review at depth both examples of poorly constructed or executed analysis plans, and global standards for analysis publication [ 16 ].
Another weakness is the lack of detail on the updating and amendment processes that may occur during the trial. Every time the protocol is updated, the investigators and statistician(s) should evaluate whether SAP changes are also necessary. When the SAP is updated, it should indicate the revision number and ideally contain an easy-to-follow revision history. Oftentimes the original and final SAP are published in supplementary materials, for example Kalil et al.'s recent COVID-19 trial presented both online [ 17 , 18 ].
Further, the paper does not list general keys, tips, and best practices for writing SAPs, nor does it enumerate evidence-based or anecdotal ‘common mistakes’, pitfalls, or risks. Fortunately, a number of contemporary publications identify both positive suggestions and risks, and ought to be consulted to augment this or other practical guides [ 1 , 3 , 5 , 6 , 9 , 16 , 19 , 20 ].
This paper is a novel contribution to scientific literature in part because it is the first time a peer-reviewed, full-length statistical analysis plan (SAP) template, with instructions, is published in full. Previously, guides or sections have been listed and described, but not including the full template needed by new practitioners. This is significant as global health human clinical trial research is increasingly desired by both Global North and Global South stakeholders to be originated and planned within the Global South. Aspiring principal investigators in low-resource settings lack full-length tools that are prevalent amongst Global North contract research organizations, industry, and academic researchers who originate current SAPs. This is compounded by the relative lack of advanced biostatistical education in locations like Africa—where such SAP templates might be expected to exist.
Ethics approval and consent to participate
Not applicable.
Consent for publication
Availability of data and materials.
Partial funding of this work was provided by The Bill & Melinda Gates Foundation. That funder had no role in if any, in study design; in generation of content; in the collection, analysis and interpretation of data; in the writing of the report; and in the decision to submit the article for publication.
Author's contributions
GS: Conceptualization, Formal Analysis, Investigation, Methodology, Project Administration, Software, Visualization, Writing-Original Draft Preparation.
SD: Conceptualization, Funding Acquisition, Visualization, Writing-Original Draft Preparation.
RM: Validation, Writing-Review & Editing.
JC: Conceptualization, Formal Analysis, Funding Acquisition, Supervision, Validation, Visualization, Writing-Review & Editing.
Declaration of competing interest
The authors declare that they have no known competing financial interests or personal relationships that could have appeared to influence the work reported in this paper.
Acknowledgements
Research Data For This Article.
No research data was generated by this article. As such, there is no data to be shared.
Data availability

Qualitative Data Analysis Methods 101:
The “big 6” methods + examples.
By: Kerryn Warren (PhD) | Reviewed By: Eunice Rautenbach (D.Tech) | May 2020 (Updated April 2023)
Qualitative data analysis methods. Wow, that’s a mouthful.
If you’re new to the world of research, qualitative data analysis can look rather intimidating. So much bulky terminology and so many abstract, fluffy concepts. It certainly can be a minefield!
Don’t worry – in this post, we’ll unpack the most popular analysis methods , one at a time, so that you can approach your analysis with confidence and competence – whether that’s for a dissertation, thesis or really any kind of research project.
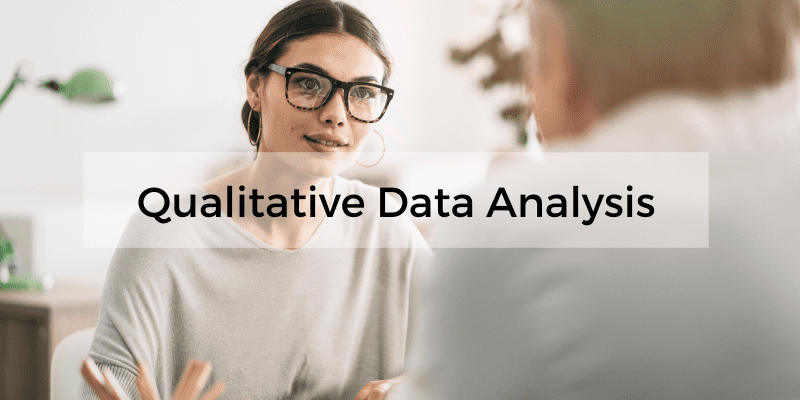
What (exactly) is qualitative data analysis?
To understand qualitative data analysis, we need to first understand qualitative data – so let’s step back and ask the question, “what exactly is qualitative data?”.
Qualitative data refers to pretty much any data that’s “not numbers” . In other words, it’s not the stuff you measure using a fixed scale or complex equipment, nor do you analyse it using complex statistics or mathematics.
So, if it’s not numbers, what is it?
Words, you guessed? Well… sometimes , yes. Qualitative data can, and often does, take the form of interview transcripts, documents and open-ended survey responses – but it can also involve the interpretation of images and videos. In other words, qualitative isn’t just limited to text-based data.
So, how’s that different from quantitative data, you ask?
Simply put, qualitative research focuses on words, descriptions, concepts or ideas – while quantitative research focuses on numbers and statistics . Qualitative research investigates the “softer side” of things to explore and describe , while quantitative research focuses on the “hard numbers”, to measure differences between variables and the relationships between them. If you’re keen to learn more about the differences between qual and quant, we’ve got a detailed post over here .
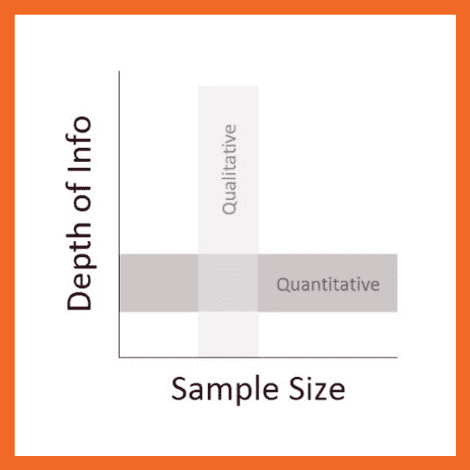
So, qualitative analysis is easier than quantitative, right?
Not quite. In many ways, qualitative data can be challenging and time-consuming to analyse and interpret. At the end of your data collection phase (which itself takes a lot of time), you’ll likely have many pages of text-based data or hours upon hours of audio to work through. You might also have subtle nuances of interactions or discussions that have danced around in your mind, or that you scribbled down in messy field notes. All of this needs to work its way into your analysis.
Making sense of all of this is no small task and you shouldn’t underestimate it. Long story short – qualitative analysis can be a lot of work! Of course, quantitative analysis is no piece of cake either, but it’s important to recognise that qualitative analysis still requires a significant investment in terms of time and effort.
Need a helping hand?
In this post, we’ll explore qualitative data analysis by looking at some of the most common analysis methods we encounter. We’re not going to cover every possible qualitative method and we’re not going to go into heavy detail – we’re just going to give you the big picture. That said, we will of course includes links to loads of extra resources so that you can learn more about whichever analysis method interests you.
Without further delay, let’s get into it.
The “Big 6” Qualitative Analysis Methods
There are many different types of qualitative data analysis, all of which serve different purposes and have unique strengths and weaknesses . We’ll start by outlining the analysis methods and then we’ll dive into the details for each.
The 6 most popular methods (or at least the ones we see at Grad Coach) are:
- Content analysis
- Narrative analysis
- Discourse analysis
- Thematic analysis
- Grounded theory (GT)
- Interpretive phenomenological analysis (IPA)
Let’s take a look at each of them…
QDA Method #1: Qualitative Content Analysis
Content analysis is possibly the most common and straightforward QDA method. At the simplest level, content analysis is used to evaluate patterns within a piece of content (for example, words, phrases or images) or across multiple pieces of content or sources of communication. For example, a collection of newspaper articles or political speeches.
With content analysis, you could, for instance, identify the frequency with which an idea is shared or spoken about – like the number of times a Kardashian is mentioned on Twitter. Or you could identify patterns of deeper underlying interpretations – for instance, by identifying phrases or words in tourist pamphlets that highlight India as an ancient country.
Because content analysis can be used in such a wide variety of ways, it’s important to go into your analysis with a very specific question and goal, or you’ll get lost in the fog. With content analysis, you’ll group large amounts of text into codes , summarise these into categories, and possibly even tabulate the data to calculate the frequency of certain concepts or variables. Because of this, content analysis provides a small splash of quantitative thinking within a qualitative method.
Naturally, while content analysis is widely useful, it’s not without its drawbacks . One of the main issues with content analysis is that it can be very time-consuming , as it requires lots of reading and re-reading of the texts. Also, because of its multidimensional focus on both qualitative and quantitative aspects, it is sometimes accused of losing important nuances in communication.
Content analysis also tends to concentrate on a very specific timeline and doesn’t take into account what happened before or after that timeline. This isn’t necessarily a bad thing though – just something to be aware of. So, keep these factors in mind if you’re considering content analysis. Every analysis method has its limitations , so don’t be put off by these – just be aware of them ! If you’re interested in learning more about content analysis, the video below provides a good starting point.
QDA Method #2: Narrative Analysis
As the name suggests, narrative analysis is all about listening to people telling stories and analysing what that means . Since stories serve a functional purpose of helping us make sense of the world, we can gain insights into the ways that people deal with and make sense of reality by analysing their stories and the ways they’re told.
You could, for example, use narrative analysis to explore whether how something is being said is important. For instance, the narrative of a prisoner trying to justify their crime could provide insight into their view of the world and the justice system. Similarly, analysing the ways entrepreneurs talk about the struggles in their careers or cancer patients telling stories of hope could provide powerful insights into their mindsets and perspectives . Simply put, narrative analysis is about paying attention to the stories that people tell – and more importantly, the way they tell them.
Of course, the narrative approach has its weaknesses , too. Sample sizes are generally quite small due to the time-consuming process of capturing narratives. Because of this, along with the multitude of social and lifestyle factors which can influence a subject, narrative analysis can be quite difficult to reproduce in subsequent research. This means that it’s difficult to test the findings of some of this research.
Similarly, researcher bias can have a strong influence on the results here, so you need to be particularly careful about the potential biases you can bring into your analysis when using this method. Nevertheless, narrative analysis is still a very useful qualitative analysis method – just keep these limitations in mind and be careful not to draw broad conclusions . If you’re keen to learn more about narrative analysis, the video below provides a great introduction to this qualitative analysis method.
QDA Method #3: Discourse Analysis
Discourse is simply a fancy word for written or spoken language or debate . So, discourse analysis is all about analysing language within its social context. In other words, analysing language – such as a conversation, a speech, etc – within the culture and society it takes place. For example, you could analyse how a janitor speaks to a CEO, or how politicians speak about terrorism.
To truly understand these conversations or speeches, the culture and history of those involved in the communication are important factors to consider. For example, a janitor might speak more casually with a CEO in a company that emphasises equality among workers. Similarly, a politician might speak more about terrorism if there was a recent terrorist incident in the country.
So, as you can see, by using discourse analysis, you can identify how culture , history or power dynamics (to name a few) have an effect on the way concepts are spoken about. So, if your research aims and objectives involve understanding culture or power dynamics, discourse analysis can be a powerful method.
Because there are many social influences in terms of how we speak to each other, the potential use of discourse analysis is vast . Of course, this also means it’s important to have a very specific research question (or questions) in mind when analysing your data and looking for patterns and themes, or you might land up going down a winding rabbit hole.
Discourse analysis can also be very time-consuming as you need to sample the data to the point of saturation – in other words, until no new information and insights emerge. But this is, of course, part of what makes discourse analysis such a powerful technique. So, keep these factors in mind when considering this QDA method. Again, if you’re keen to learn more, the video below presents a good starting point.
QDA Method #4: Thematic Analysis
Thematic analysis looks at patterns of meaning in a data set – for example, a set of interviews or focus group transcripts. But what exactly does that… mean? Well, a thematic analysis takes bodies of data (which are often quite large) and groups them according to similarities – in other words, themes . These themes help us make sense of the content and derive meaning from it.
Let’s take a look at an example.
With thematic analysis, you could analyse 100 online reviews of a popular sushi restaurant to find out what patrons think about the place. By reviewing the data, you would then identify the themes that crop up repeatedly within the data – for example, “fresh ingredients” or “friendly wait staff”.
So, as you can see, thematic analysis can be pretty useful for finding out about people’s experiences , views, and opinions . Therefore, if your research aims and objectives involve understanding people’s experience or view of something, thematic analysis can be a great choice.
Since thematic analysis is a bit of an exploratory process, it’s not unusual for your research questions to develop , or even change as you progress through the analysis. While this is somewhat natural in exploratory research, it can also be seen as a disadvantage as it means that data needs to be re-reviewed each time a research question is adjusted. In other words, thematic analysis can be quite time-consuming – but for a good reason. So, keep this in mind if you choose to use thematic analysis for your project and budget extra time for unexpected adjustments.
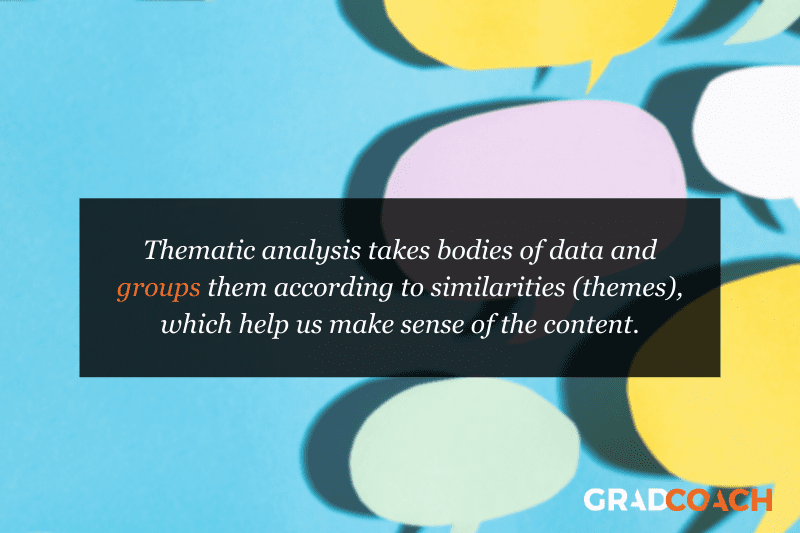
QDA Method #5: Grounded theory (GT)
Grounded theory is a powerful qualitative analysis method where the intention is to create a new theory (or theories) using the data at hand, through a series of “ tests ” and “ revisions ”. Strictly speaking, GT is more a research design type than an analysis method, but we’ve included it here as it’s often referred to as a method.
What’s most important with grounded theory is that you go into the analysis with an open mind and let the data speak for itself – rather than dragging existing hypotheses or theories into your analysis. In other words, your analysis must develop from the ground up (hence the name).
Let’s look at an example of GT in action.
Assume you’re interested in developing a theory about what factors influence students to watch a YouTube video about qualitative analysis. Using Grounded theory , you’d start with this general overarching question about the given population (i.e., graduate students). First, you’d approach a small sample – for example, five graduate students in a department at a university. Ideally, this sample would be reasonably representative of the broader population. You’d interview these students to identify what factors lead them to watch the video.
After analysing the interview data, a general pattern could emerge. For example, you might notice that graduate students are more likely to read a post about qualitative methods if they are just starting on their dissertation journey, or if they have an upcoming test about research methods.
From here, you’ll look for another small sample – for example, five more graduate students in a different department – and see whether this pattern holds true for them. If not, you’ll look for commonalities and adapt your theory accordingly. As this process continues, the theory would develop . As we mentioned earlier, what’s important with grounded theory is that the theory develops from the data – not from some preconceived idea.
So, what are the drawbacks of grounded theory? Well, some argue that there’s a tricky circularity to grounded theory. For it to work, in principle, you should know as little as possible regarding the research question and population, so that you reduce the bias in your interpretation. However, in many circumstances, it’s also thought to be unwise to approach a research question without knowledge of the current literature . In other words, it’s a bit of a “chicken or the egg” situation.
Regardless, grounded theory remains a popular (and powerful) option. Naturally, it’s a very useful method when you’re researching a topic that is completely new or has very little existing research about it, as it allows you to start from scratch and work your way from the ground up .
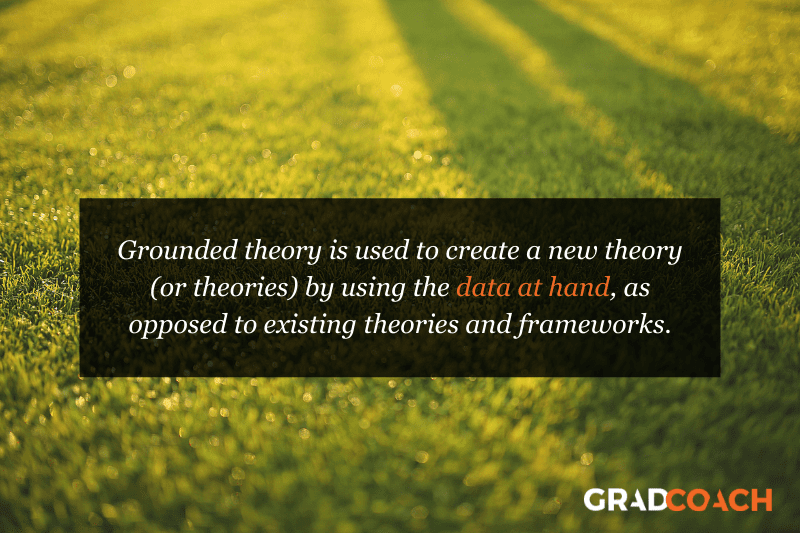
QDA Method #6: Interpretive Phenomenological Analysis (IPA)
Interpretive. Phenomenological. Analysis. IPA . Try saying that three times fast…
Let’s just stick with IPA, okay?
IPA is designed to help you understand the personal experiences of a subject (for example, a person or group of people) concerning a major life event, an experience or a situation . This event or experience is the “phenomenon” that makes up the “P” in IPA. Such phenomena may range from relatively common events – such as motherhood, or being involved in a car accident – to those which are extremely rare – for example, someone’s personal experience in a refugee camp. So, IPA is a great choice if your research involves analysing people’s personal experiences of something that happened to them.
It’s important to remember that IPA is subject – centred . In other words, it’s focused on the experiencer . This means that, while you’ll likely use a coding system to identify commonalities, it’s important not to lose the depth of experience or meaning by trying to reduce everything to codes. Also, keep in mind that since your sample size will generally be very small with IPA, you often won’t be able to draw broad conclusions about the generalisability of your findings. But that’s okay as long as it aligns with your research aims and objectives.
Another thing to be aware of with IPA is personal bias . While researcher bias can creep into all forms of research, self-awareness is critically important with IPA, as it can have a major impact on the results. For example, a researcher who was a victim of a crime himself could insert his own feelings of frustration and anger into the way he interprets the experience of someone who was kidnapped. So, if you’re going to undertake IPA, you need to be very self-aware or you could muddy the analysis.
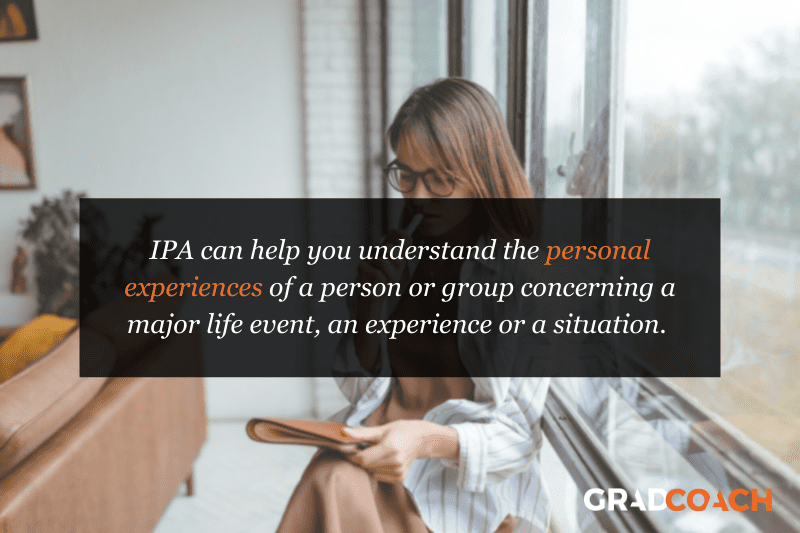
How to choose the right analysis method
In light of all of the qualitative analysis methods we’ve covered so far, you’re probably asking yourself the question, “ How do I choose the right one? ”
Much like all the other methodological decisions you’ll need to make, selecting the right qualitative analysis method largely depends on your research aims, objectives and questions . In other words, the best tool for the job depends on what you’re trying to build. For example:
- Perhaps your research aims to analyse the use of words and what they reveal about the intention of the storyteller and the cultural context of the time.
- Perhaps your research aims to develop an understanding of the unique personal experiences of people that have experienced a certain event, or
- Perhaps your research aims to develop insight regarding the influence of a certain culture on its members.
As you can probably see, each of these research aims are distinctly different , and therefore different analysis methods would be suitable for each one. For example, narrative analysis would likely be a good option for the first aim, while grounded theory wouldn’t be as relevant.
It’s also important to remember that each method has its own set of strengths, weaknesses and general limitations. No single analysis method is perfect . So, depending on the nature of your research, it may make sense to adopt more than one method (this is called triangulation ). Keep in mind though that this will of course be quite time-consuming.
As we’ve seen, all of the qualitative analysis methods we’ve discussed make use of coding and theme-generating techniques, but the intent and approach of each analysis method differ quite substantially. So, it’s very important to come into your research with a clear intention before you decide which analysis method (or methods) to use.
Start by reviewing your research aims , objectives and research questions to assess what exactly you’re trying to find out – then select a qualitative analysis method that fits. Never pick a method just because you like it or have experience using it – your analysis method (or methods) must align with your broader research aims and objectives.
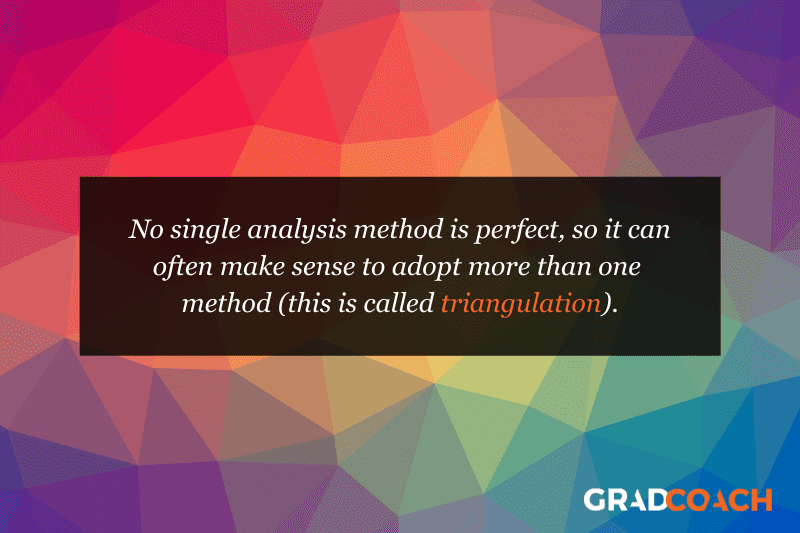
Let’s recap on QDA methods…
In this post, we looked at six popular qualitative data analysis methods:
- First, we looked at content analysis , a straightforward method that blends a little bit of quant into a primarily qualitative analysis.
- Then we looked at narrative analysis , which is about analysing how stories are told.
- Next up was discourse analysis – which is about analysing conversations and interactions.
- Then we moved on to thematic analysis – which is about identifying themes and patterns.
- From there, we went south with grounded theory – which is about starting from scratch with a specific question and using the data alone to build a theory in response to that question.
- And finally, we looked at IPA – which is about understanding people’s unique experiences of a phenomenon.
Of course, these aren’t the only options when it comes to qualitative data analysis, but they’re a great starting point if you’re dipping your toes into qualitative research for the first time.
If you’re still feeling a bit confused, consider our private coaching service , where we hold your hand through the research process to help you develop your best work.
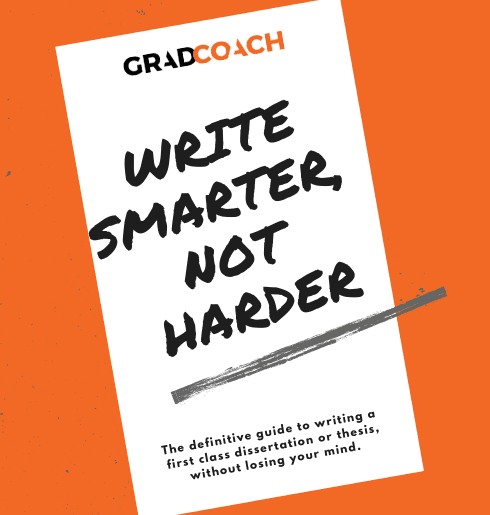
Psst… there’s more (for free)
This post is part of our dissertation mini-course, which covers everything you need to get started with your dissertation, thesis or research project.
You Might Also Like:
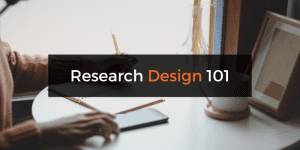
84 Comments
This has been very helpful. Thank you.
Thank you madam,
Thank you so much for this information
I wonder it so clear for understand and good for me. can I ask additional query?
Very insightful and useful
Good work done with clear explanations. Thank you.
Thanks so much for the write-up, it’s really good.
Thanks madam . It is very important .
thank you very good
This has been very well explained in simple language . It is useful even for a new researcher.
Great to hear that. Good luck with your qualitative data analysis, Pramod!
This is very useful information. And it was very a clear language structured presentation. Thanks a lot.
Thank you so much.
very informative sequential presentation
Precise explanation of method.
Hi, may we use 2 data analysis methods in our qualitative research?
Thanks for your comment. Most commonly, one would use one type of analysis method, but it depends on your research aims and objectives.
You explained it in very simple language, everyone can understand it. Thanks so much.
Thank you very much, this is very helpful. It has been explained in a very simple manner that even a layman understands
Thank nicely explained can I ask is Qualitative content analysis the same as thematic analysis?
Thanks for your comment. No, QCA and thematic are two different types of analysis. This article might help clarify – https://onlinelibrary.wiley.com/doi/10.1111/nhs.12048
This is my first time to come across a well explained data analysis. so helpful.
I have thoroughly enjoyed your explanation of the six qualitative analysis methods. This is very helpful. Thank you!
Thank you very much, this is well explained and useful
i need a citation of your book.
Thanks a lot , remarkable indeed, enlighting to the best
Hi Derek, What other theories/methods would you recommend when the data is a whole speech?
Keep writing useful artikel.
It is important concept about QDA and also the way to express is easily understandable, so thanks for all.
Thank you, this is well explained and very useful.
Very helpful .Thanks.
Hi there! Very well explained. Simple but very useful style of writing. Please provide the citation of the text. warm regards
The session was very helpful and insightful. Thank you
This was very helpful and insightful. Easy to read and understand
As a professional academic writer, this has been so informative and educative. Keep up the good work Grad Coach you are unmatched with quality content for sure.
Keep up the good work Grad Coach you are unmatched with quality content for sure.
Its Great and help me the most. A Million Thanks you Dr.
It is a very nice work
Very insightful. Please, which of this approach could be used for a research that one is trying to elicit students’ misconceptions in a particular concept ?
This is Amazing and well explained, thanks
great overview
What do we call a research data analysis method that one use to advise or determining the best accounting tool or techniques that should be adopted in a company.
Informative video, explained in a clear and simple way. Kudos
Waoo! I have chosen method wrong for my data analysis. But I can revise my work according to this guide. Thank you so much for this helpful lecture.
This has been very helpful. It gave me a good view of my research objectives and how to choose the best method. Thematic analysis it is.
Very helpful indeed. Thanku so much for the insight.
This was incredibly helpful.
Very helpful.
very educative
Nicely written especially for novice academic researchers like me! Thank you.
choosing a right method for a paper is always a hard job for a student, this is a useful information, but it would be more useful personally for me, if the author provide me with a little bit more information about the data analysis techniques in type of explanatory research. Can we use qualitative content analysis technique for explanatory research ? or what is the suitable data analysis method for explanatory research in social studies?
that was very helpful for me. because these details are so important to my research. thank you very much
I learnt a lot. Thank you
Relevant and Informative, thanks !
Well-planned and organized, thanks much! 🙂
I have reviewed qualitative data analysis in a simplest way possible. The content will highly be useful for developing my book on qualitative data analysis methods. Cheers!
Clear explanation on qualitative and how about Case study
This was helpful. Thank you
This was really of great assistance, it was just the right information needed. Explanation very clear and follow.
Wow, Thanks for making my life easy
This was helpful thanks .
Very helpful…. clear and written in an easily understandable manner. Thank you.
This was so helpful as it was easy to understand. I’m a new to research thank you so much.
so educative…. but Ijust want to know which method is coding of the qualitative or tallying done?
Thank you for the great content, I have learnt a lot. So helpful
precise and clear presentation with simple language and thank you for that.
very informative content, thank you.
You guys are amazing on YouTube on this platform. Your teachings are great, educative, and informative. kudos!
Brilliant Delivery. You made a complex subject seem so easy. Well done.
Beautifully explained.
Thanks a lot
Is there a video the captures the practical process of coding using automated applications?
Thanks for the comment. We don’t recommend using automated applications for coding, as they are not sufficiently accurate in our experience.
content analysis can be qualitative research?
THANK YOU VERY MUCH.
Thank you very much for such a wonderful content
do you have any material on Data collection
What a powerful explanation of the QDA methods. Thank you.
Great explanation both written and Video. i have been using of it on a day to day working of my thesis project in accounting and finance. Thank you very much for your support.
very helpful, thank you so much
Submit a Comment Cancel reply
Your email address will not be published. Required fields are marked *
Save my name, email, and website in this browser for the next time I comment.
- Print Friendly
- Skip to main content
- Skip to primary sidebar
- Skip to footer
- QuestionPro

- Solutions Industries Gaming Automotive Sports and events Education Government Travel & Hospitality Financial Services Healthcare Cannabis Technology Use Case NPS+ Communities Audience Contactless surveys Mobile LivePolls Member Experience GDPR Positive People Science 360 Feedback Surveys
- Resources Blog eBooks Survey Templates Case Studies Training Help center

Home Market Research
Data Analysis in Research: Types & Methods
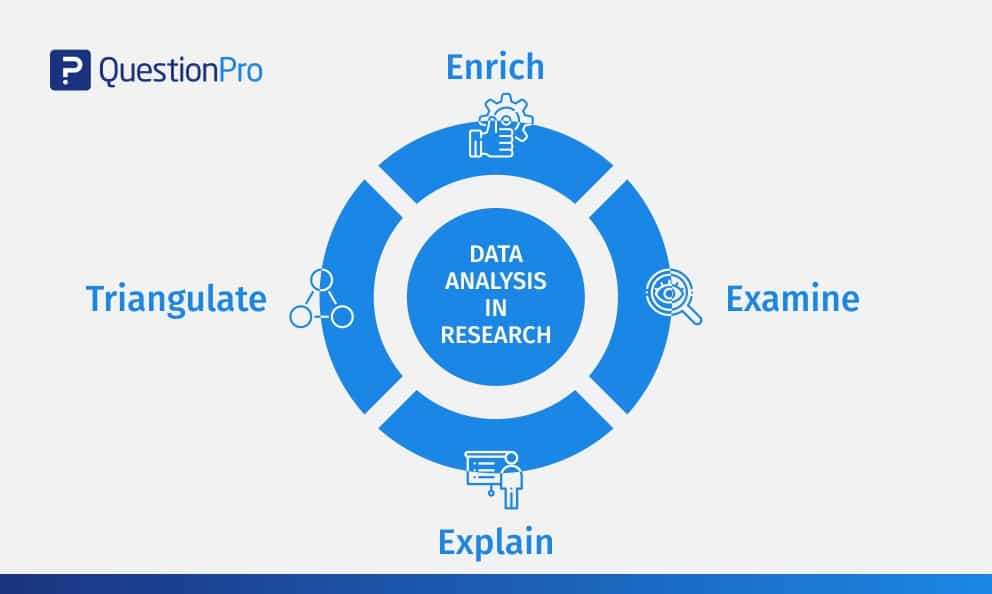
Content Index
Why analyze data in research?
Types of data in research, finding patterns in the qualitative data, methods used for data analysis in qualitative research, preparing data for analysis, methods used for data analysis in quantitative research, considerations in research data analysis, what is data analysis in research.
Definition of research in data analysis: According to LeCompte and Schensul, research data analysis is a process used by researchers to reduce data to a story and interpret it to derive insights. The data analysis process helps reduce a large chunk of data into smaller fragments, which makes sense.
Three essential things occur during the data analysis process — the first is data organization . Summarization and categorization together contribute to becoming the second known method used for data reduction. It helps find patterns and themes in the data for easy identification and linking. The third and last way is data analysis – researchers do it in both top-down and bottom-up fashion.
LEARN ABOUT: Research Process Steps
On the other hand, Marshall and Rossman describe data analysis as a messy, ambiguous, and time-consuming but creative and fascinating process through which a mass of collected data is brought to order, structure and meaning.
We can say that “the data analysis and data interpretation is a process representing the application of deductive and inductive logic to the research and data analysis.”
Researchers rely heavily on data as they have a story to tell or research problems to solve. It starts with a question, and data is nothing but an answer to that question. But, what if there is no question to ask? Well! It is possible to explore data even without a problem – we call it ‘Data Mining’, which often reveals some interesting patterns within the data that are worth exploring.
Irrelevant to the type of data researchers explore, their mission and audiences’ vision guide them to find the patterns to shape the story they want to tell. One of the essential things expected from researchers while analyzing data is to stay open and remain unbiased toward unexpected patterns, expressions, and results. Remember, sometimes, data analysis tells the most unforeseen yet exciting stories that were not expected when initiating data analysis. Therefore, rely on the data you have at hand and enjoy the journey of exploratory research.
Create a Free Account
Every kind of data has a rare quality of describing things after assigning a specific value to it. For analysis, you need to organize these values, processed and presented in a given context, to make it useful. Data can be in different forms; here are the primary data types.
- Qualitative data: When the data presented has words and descriptions, then we call it qualitative data . Although you can observe this data, it is subjective and harder to analyze data in research, especially for comparison. Example: Quality data represents everything describing taste, experience, texture, or an opinion that is considered quality data. This type of data is usually collected through focus groups, personal qualitative interviews , qualitative observation or using open-ended questions in surveys.
- Quantitative data: Any data expressed in numbers of numerical figures are called quantitative data . This type of data can be distinguished into categories, grouped, measured, calculated, or ranked. Example: questions such as age, rank, cost, length, weight, scores, etc. everything comes under this type of data. You can present such data in graphical format, charts, or apply statistical analysis methods to this data. The (Outcomes Measurement Systems) OMS questionnaires in surveys are a significant source of collecting numeric data.
- Categorical data: It is data presented in groups. However, an item included in the categorical data cannot belong to more than one group. Example: A person responding to a survey by telling his living style, marital status, smoking habit, or drinking habit comes under the categorical data. A chi-square test is a standard method used to analyze this data.
Learn More : Examples of Qualitative Data in Education
Data analysis in qualitative research
Data analysis and qualitative data research work a little differently from the numerical data as the quality data is made up of words, descriptions, images, objects, and sometimes symbols. Getting insight from such complicated information is a complicated process. Hence it is typically used for exploratory research and data analysis .
Although there are several ways to find patterns in the textual information, a word-based method is the most relied and widely used global technique for research and data analysis. Notably, the data analysis process in qualitative research is manual. Here the researchers usually read the available data and find repetitive or commonly used words.
For example, while studying data collected from African countries to understand the most pressing issues people face, researchers might find “food” and “hunger” are the most commonly used words and will highlight them for further analysis.
LEARN ABOUT: Level of Analysis
The keyword context is another widely used word-based technique. In this method, the researcher tries to understand the concept by analyzing the context in which the participants use a particular keyword.
For example , researchers conducting research and data analysis for studying the concept of ‘diabetes’ amongst respondents might analyze the context of when and how the respondent has used or referred to the word ‘diabetes.’
The scrutiny-based technique is also one of the highly recommended text analysis methods used to identify a quality data pattern. Compare and contrast is the widely used method under this technique to differentiate how a specific text is similar or different from each other.
For example: To find out the “importance of resident doctor in a company,” the collected data is divided into people who think it is necessary to hire a resident doctor and those who think it is unnecessary. Compare and contrast is the best method that can be used to analyze the polls having single-answer questions types .
Metaphors can be used to reduce the data pile and find patterns in it so that it becomes easier to connect data with theory.
Variable Partitioning is another technique used to split variables so that researchers can find more coherent descriptions and explanations from the enormous data.
LEARN ABOUT: Qualitative Research Questions and Questionnaires
There are several techniques to analyze the data in qualitative research, but here are some commonly used methods,
- Content Analysis: It is widely accepted and the most frequently employed technique for data analysis in research methodology. It can be used to analyze the documented information from text, images, and sometimes from the physical items. It depends on the research questions to predict when and where to use this method.
- Narrative Analysis: This method is used to analyze content gathered from various sources such as personal interviews, field observation, and surveys . The majority of times, stories, or opinions shared by people are focused on finding answers to the research questions.
- Discourse Analysis: Similar to narrative analysis, discourse analysis is used to analyze the interactions with people. Nevertheless, this particular method considers the social context under which or within which the communication between the researcher and respondent takes place. In addition to that, discourse analysis also focuses on the lifestyle and day-to-day environment while deriving any conclusion.
- Grounded Theory: When you want to explain why a particular phenomenon happened, then using grounded theory for analyzing quality data is the best resort. Grounded theory is applied to study data about the host of similar cases occurring in different settings. When researchers are using this method, they might alter explanations or produce new ones until they arrive at some conclusion.
LEARN ABOUT: 12 Best Tools for Researchers
Data analysis in quantitative research
The first stage in research and data analysis is to make it for the analysis so that the nominal data can be converted into something meaningful. Data preparation consists of the below phases.
Phase I: Data Validation
Data validation is done to understand if the collected data sample is per the pre-set standards, or it is a biased data sample again divided into four different stages
- Fraud: To ensure an actual human being records each response to the survey or the questionnaire
- Screening: To make sure each participant or respondent is selected or chosen in compliance with the research criteria
- Procedure: To ensure ethical standards were maintained while collecting the data sample
- Completeness: To ensure that the respondent has answered all the questions in an online survey. Else, the interviewer had asked all the questions devised in the questionnaire.
Phase II: Data Editing
More often, an extensive research data sample comes loaded with errors. Respondents sometimes fill in some fields incorrectly or sometimes skip them accidentally. Data editing is a process wherein the researchers have to confirm that the provided data is free of such errors. They need to conduct necessary checks and outlier checks to edit the raw edit and make it ready for analysis.
Phase III: Data Coding
Out of all three, this is the most critical phase of data preparation associated with grouping and assigning values to the survey responses . If a survey is completed with a 1000 sample size, the researcher will create an age bracket to distinguish the respondents based on their age. Thus, it becomes easier to analyze small data buckets rather than deal with the massive data pile.
LEARN ABOUT: Steps in Qualitative Research
After the data is prepared for analysis, researchers are open to using different research and data analysis methods to derive meaningful insights. For sure, statistical analysis plans are the most favored to analyze numerical data. In statistical analysis, distinguishing between categorical data and numerical data is essential, as categorical data involves distinct categories or labels, while numerical data consists of measurable quantities. The method is again classified into two groups. First, ‘Descriptive Statistics’ used to describe data. Second, ‘Inferential statistics’ that helps in comparing the data .
Descriptive statistics
This method is used to describe the basic features of versatile types of data in research. It presents the data in such a meaningful way that pattern in the data starts making sense. Nevertheless, the descriptive analysis does not go beyond making conclusions. The conclusions are again based on the hypothesis researchers have formulated so far. Here are a few major types of descriptive analysis methods.
Measures of Frequency
- Count, Percent, Frequency
- It is used to denote home often a particular event occurs.
- Researchers use it when they want to showcase how often a response is given.
Measures of Central Tendency
- Mean, Median, Mode
- The method is widely used to demonstrate distribution by various points.
- Researchers use this method when they want to showcase the most commonly or averagely indicated response.
Measures of Dispersion or Variation
- Range, Variance, Standard deviation
- Here the field equals high/low points.
- Variance standard deviation = difference between the observed score and mean
- It is used to identify the spread of scores by stating intervals.
- Researchers use this method to showcase data spread out. It helps them identify the depth until which the data is spread out that it directly affects the mean.
Measures of Position
- Percentile ranks, Quartile ranks
- It relies on standardized scores helping researchers to identify the relationship between different scores.
- It is often used when researchers want to compare scores with the average count.
For quantitative research use of descriptive analysis often give absolute numbers, but the in-depth analysis is never sufficient to demonstrate the rationale behind those numbers. Nevertheless, it is necessary to think of the best method for research and data analysis suiting your survey questionnaire and what story researchers want to tell. For example, the mean is the best way to demonstrate the students’ average scores in schools. It is better to rely on the descriptive statistics when the researchers intend to keep the research or outcome limited to the provided sample without generalizing it. For example, when you want to compare average voting done in two different cities, differential statistics are enough.
Descriptive analysis is also called a ‘univariate analysis’ since it is commonly used to analyze a single variable.
Inferential statistics
Inferential statistics are used to make predictions about a larger population after research and data analysis of the representing population’s collected sample. For example, you can ask some odd 100 audiences at a movie theater if they like the movie they are watching. Researchers then use inferential statistics on the collected sample to reason that about 80-90% of people like the movie.
Here are two significant areas of inferential statistics.
- Estimating parameters: It takes statistics from the sample research data and demonstrates something about the population parameter.
- Hypothesis test: I t’s about sampling research data to answer the survey research questions. For example, researchers might be interested to understand if the new shade of lipstick recently launched is good or not, or if the multivitamin capsules help children to perform better at games.
These are sophisticated analysis methods used to showcase the relationship between different variables instead of describing a single variable. It is often used when researchers want something beyond absolute numbers to understand the relationship between variables.
Here are some of the commonly used methods for data analysis in research.
- Correlation: When researchers are not conducting experimental research or quasi-experimental research wherein the researchers are interested to understand the relationship between two or more variables, they opt for correlational research methods.
- Cross-tabulation: Also called contingency tables, cross-tabulation is used to analyze the relationship between multiple variables. Suppose provided data has age and gender categories presented in rows and columns. A two-dimensional cross-tabulation helps for seamless data analysis and research by showing the number of males and females in each age category.
- Regression analysis: For understanding the strong relationship between two variables, researchers do not look beyond the primary and commonly used regression analysis method, which is also a type of predictive analysis used. In this method, you have an essential factor called the dependent variable. You also have multiple independent variables in regression analysis. You undertake efforts to find out the impact of independent variables on the dependent variable. The values of both independent and dependent variables are assumed as being ascertained in an error-free random manner.
- Frequency tables: The statistical procedure is used for testing the degree to which two or more vary or differ in an experiment. A considerable degree of variation means research findings were significant. In many contexts, ANOVA testing and variance analysis are similar.
- Analysis of variance: The statistical procedure is used for testing the degree to which two or more vary or differ in an experiment. A considerable degree of variation means research findings were significant. In many contexts, ANOVA testing and variance analysis are similar.
- Researchers must have the necessary research skills to analyze and manipulation the data , Getting trained to demonstrate a high standard of research practice. Ideally, researchers must possess more than a basic understanding of the rationale of selecting one statistical method over the other to obtain better data insights.
- Usually, research and data analytics projects differ by scientific discipline; therefore, getting statistical advice at the beginning of analysis helps design a survey questionnaire, select data collection methods, and choose samples.
LEARN ABOUT: Best Data Collection Tools
- The primary aim of data research and analysis is to derive ultimate insights that are unbiased. Any mistake in or keeping a biased mind to collect data, selecting an analysis method, or choosing audience sample il to draw a biased inference.
- Irrelevant to the sophistication used in research data and analysis is enough to rectify the poorly defined objective outcome measurements. It does not matter if the design is at fault or intentions are not clear, but lack of clarity might mislead readers, so avoid the practice.
- The motive behind data analysis in research is to present accurate and reliable data. As far as possible, avoid statistical errors, and find a way to deal with everyday challenges like outliers, missing data, data altering, data mining , or developing graphical representation.
LEARN MORE: Descriptive Research vs Correlational Research The sheer amount of data generated daily is frightening. Especially when data analysis has taken center stage. in 2018. In last year, the total data supply amounted to 2.8 trillion gigabytes. Hence, it is clear that the enterprises willing to survive in the hypercompetitive world must possess an excellent capability to analyze complex research data, derive actionable insights, and adapt to the new market needs.
LEARN ABOUT: Average Order Value
QuestionPro is an online survey platform that empowers organizations in data analysis and research and provides them a medium to collect data by creating appealing surveys.
MORE LIKE THIS
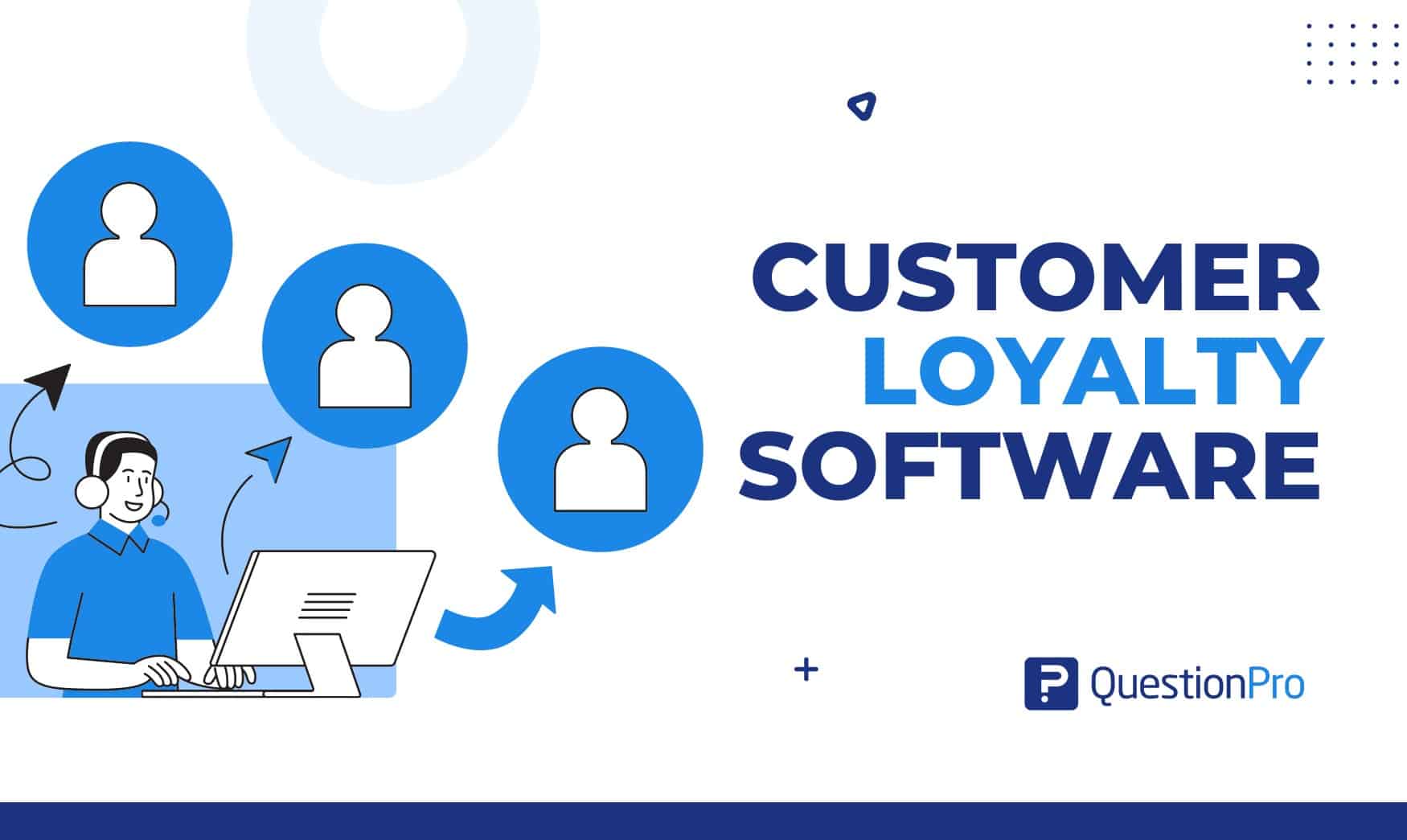
10 Top Customer Loyalty Software to Boost Your Business
Mar 25, 2024
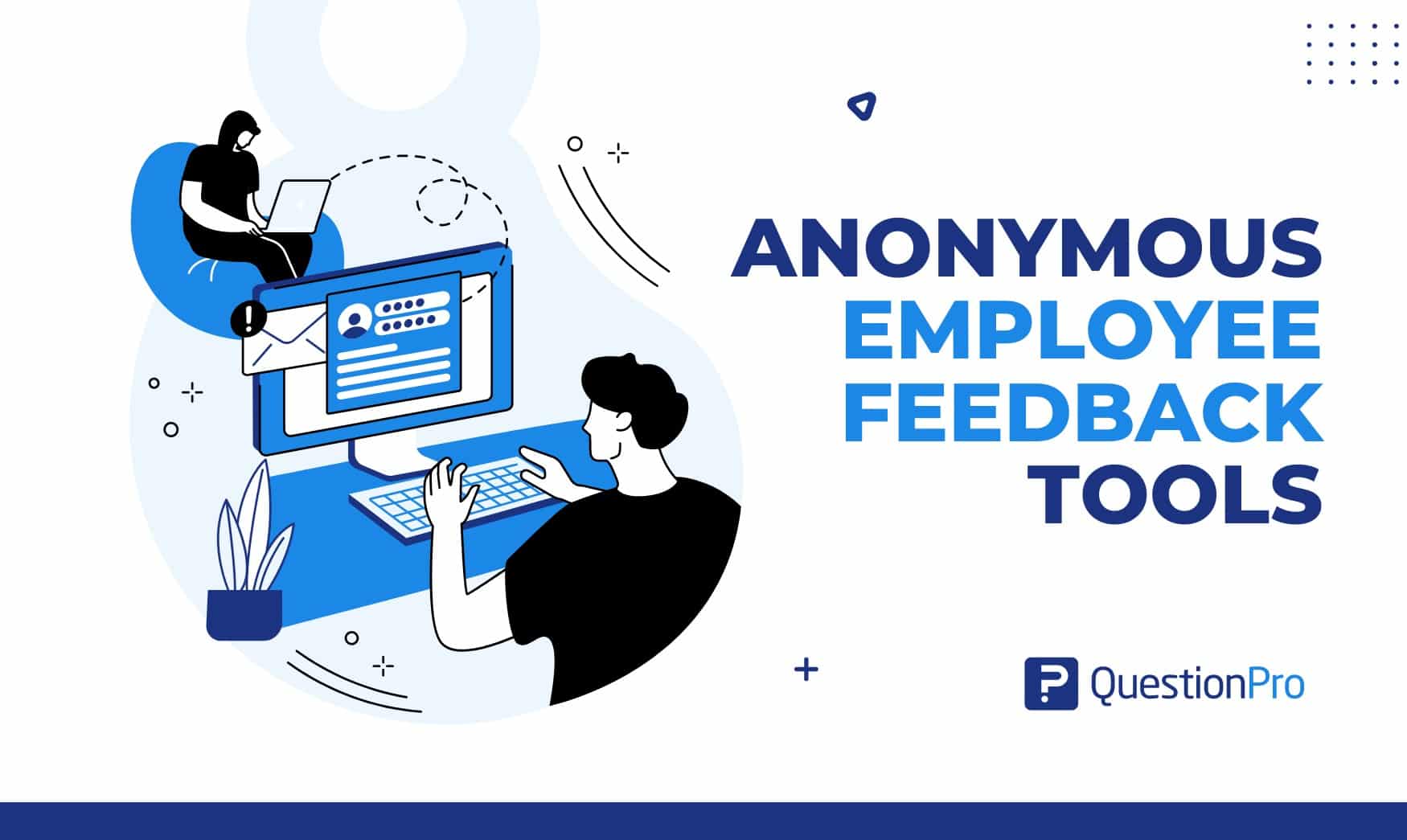
Top 13 Anonymous Employee Feedback Tools for 2024
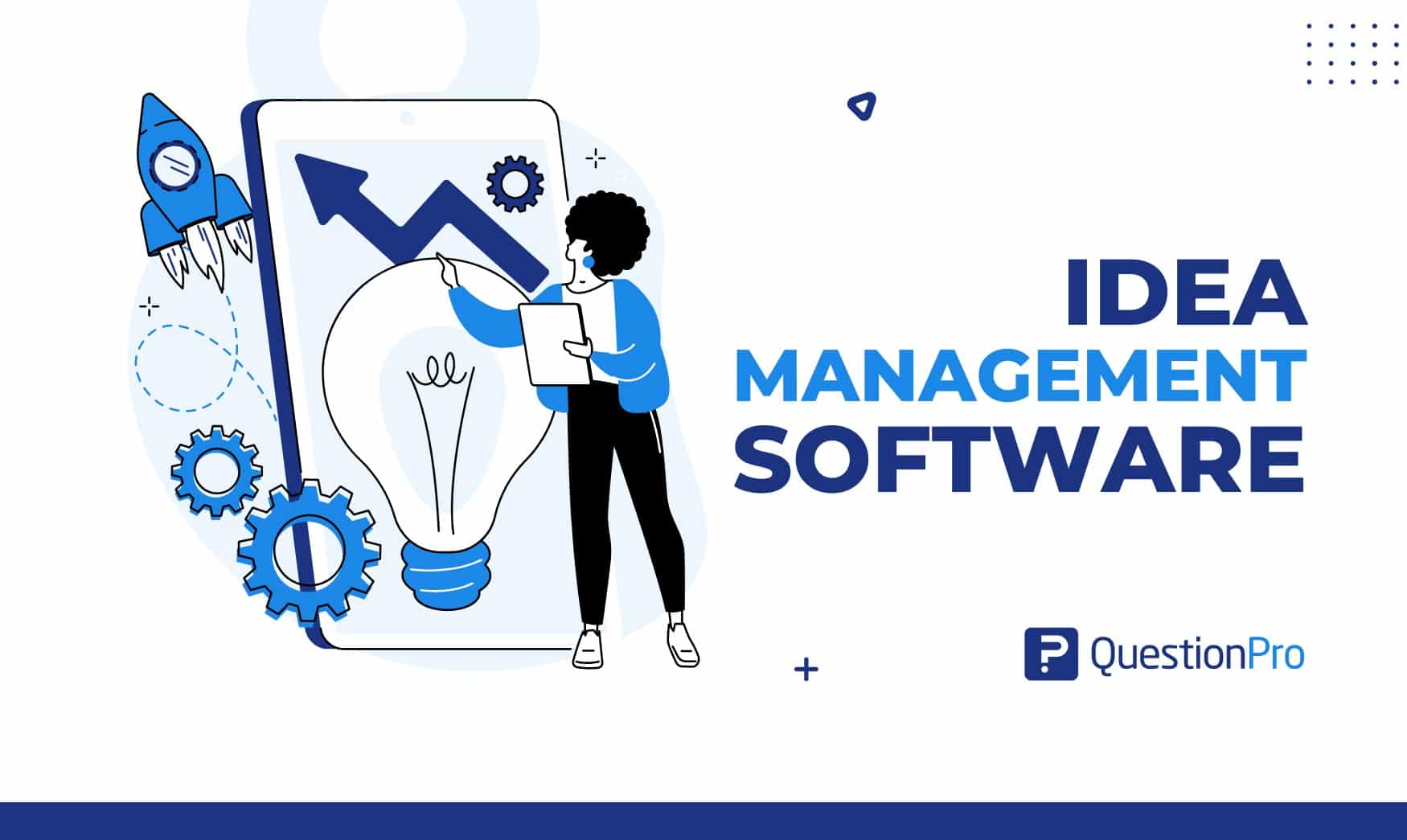
Unlocking Creativity With 10 Top Idea Management Software
Mar 23, 2024
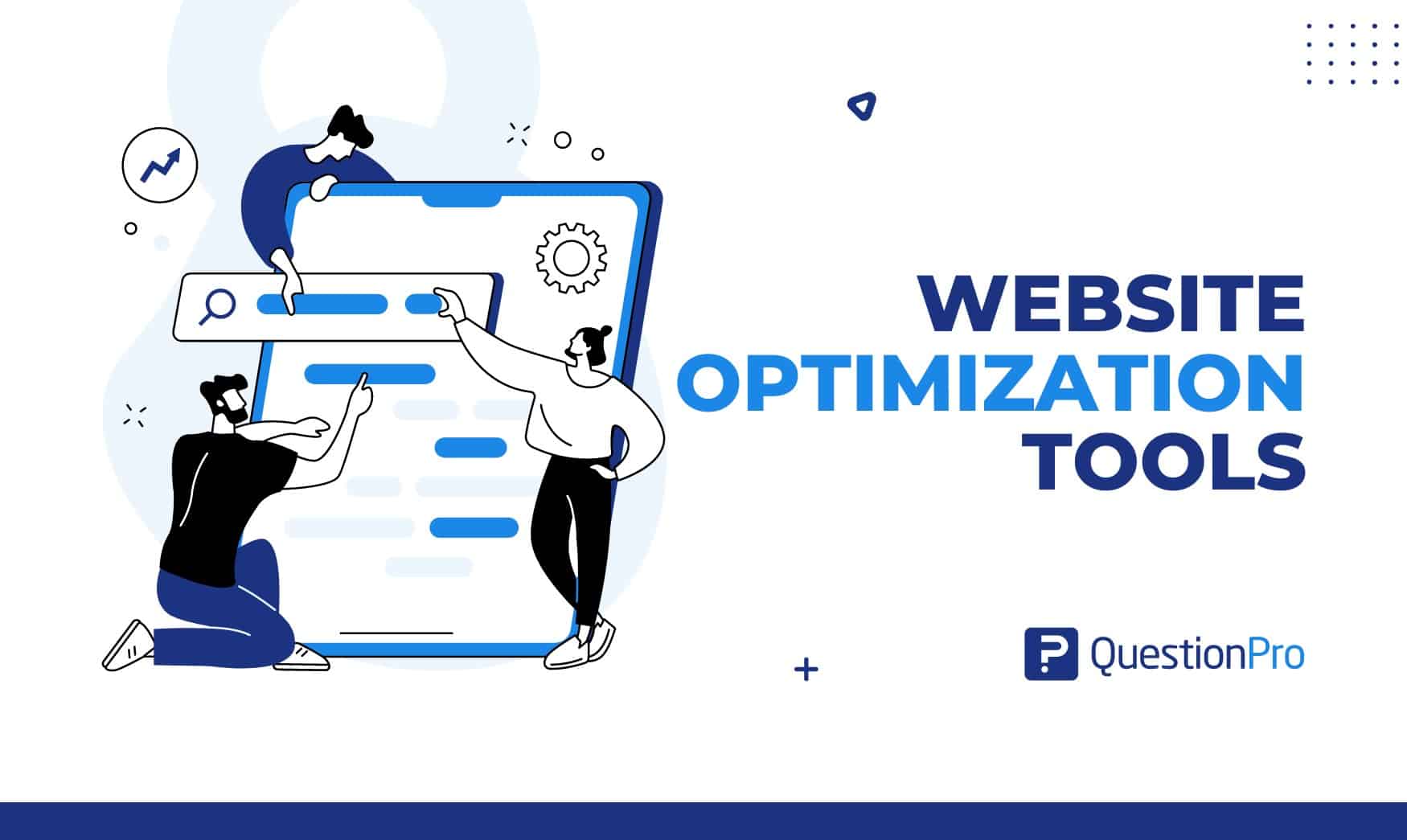
20 Best Website Optimization Tools to Improve Your Website
Mar 22, 2024
Other categories
- Academic Research
- Artificial Intelligence
- Assessments
- Brand Awareness
- Case Studies
- Communities
- Consumer Insights
- Customer effort score
- Customer Engagement
- Customer Experience
- Customer Loyalty
- Customer Research
- Customer Satisfaction
- Employee Benefits
- Employee Engagement
- Employee Retention
- Friday Five
- General Data Protection Regulation
- Insights Hub
- Life@QuestionPro
- Market Research
- Mobile diaries
- Mobile Surveys
- New Features
- Online Communities
- Question Types
- Questionnaire
- QuestionPro Products
- Release Notes
- Research Tools and Apps
- Revenue at Risk
- Survey Templates
- Training Tips
- Uncategorized
- Video Learning Series
- What’s Coming Up
- Workforce Intelligence
Have a language expert improve your writing
Run a free plagiarism check in 10 minutes, generate accurate citations for free.
- Knowledge Base
Methodology
- How to Do Thematic Analysis | Step-by-Step Guide & Examples
How to Do Thematic Analysis | Step-by-Step Guide & Examples
Published on September 6, 2019 by Jack Caulfield . Revised on June 22, 2023.
Thematic analysis is a method of analyzing qualitative data . It is usually applied to a set of texts, such as an interview or transcripts . The researcher closely examines the data to identify common themes – topics, ideas and patterns of meaning that come up repeatedly.
There are various approaches to conducting thematic analysis, but the most common form follows a six-step process: familiarization, coding, generating themes, reviewing themes, defining and naming themes, and writing up. Following this process can also help you avoid confirmation bias when formulating your analysis.
This process was originally developed for psychology research by Virginia Braun and Victoria Clarke . However, thematic analysis is a flexible method that can be adapted to many different kinds of research.
Table of contents
When to use thematic analysis, different approaches to thematic analysis, step 1: familiarization, step 2: coding, step 3: generating themes, step 4: reviewing themes, step 5: defining and naming themes, step 6: writing up, other interesting articles.
Thematic analysis is a good approach to research where you’re trying to find out something about people’s views, opinions, knowledge, experiences or values from a set of qualitative data – for example, interview transcripts , social media profiles, or survey responses .
Some types of research questions you might use thematic analysis to answer:
- How do patients perceive doctors in a hospital setting?
- What are young women’s experiences on dating sites?
- What are non-experts’ ideas and opinions about climate change?
- How is gender constructed in high school history teaching?
To answer any of these questions, you would collect data from a group of relevant participants and then analyze it. Thematic analysis allows you a lot of flexibility in interpreting the data, and allows you to approach large data sets more easily by sorting them into broad themes.
However, it also involves the risk of missing nuances in the data. Thematic analysis is often quite subjective and relies on the researcher’s judgement, so you have to reflect carefully on your own choices and interpretations.
Pay close attention to the data to ensure that you’re not picking up on things that are not there – or obscuring things that are.
Here's why students love Scribbr's proofreading services
Discover proofreading & editing
Once you’ve decided to use thematic analysis, there are different approaches to consider.
There’s the distinction between inductive and deductive approaches:
- An inductive approach involves allowing the data to determine your themes.
- A deductive approach involves coming to the data with some preconceived themes you expect to find reflected there, based on theory or existing knowledge.
Ask yourself: Does my theoretical framework give me a strong idea of what kind of themes I expect to find in the data (deductive), or am I planning to develop my own framework based on what I find (inductive)?
There’s also the distinction between a semantic and a latent approach:
- A semantic approach involves analyzing the explicit content of the data.
- A latent approach involves reading into the subtext and assumptions underlying the data.
Ask yourself: Am I interested in people’s stated opinions (semantic) or in what their statements reveal about their assumptions and social context (latent)?
After you’ve decided thematic analysis is the right method for analyzing your data, and you’ve thought about the approach you’re going to take, you can follow the six steps developed by Braun and Clarke .
The first step is to get to know our data. It’s important to get a thorough overview of all the data we collected before we start analyzing individual items.
This might involve transcribing audio , reading through the text and taking initial notes, and generally looking through the data to get familiar with it.
Next up, we need to code the data. Coding means highlighting sections of our text – usually phrases or sentences – and coming up with shorthand labels or “codes” to describe their content.
Let’s take a short example text. Say we’re researching perceptions of climate change among conservative voters aged 50 and up, and we have collected data through a series of interviews. An extract from one interview looks like this:
In this extract, we’ve highlighted various phrases in different colors corresponding to different codes. Each code describes the idea or feeling expressed in that part of the text.
At this stage, we want to be thorough: we go through the transcript of every interview and highlight everything that jumps out as relevant or potentially interesting. As well as highlighting all the phrases and sentences that match these codes, we can keep adding new codes as we go through the text.
After we’ve been through the text, we collate together all the data into groups identified by code. These codes allow us to gain a a condensed overview of the main points and common meanings that recur throughout the data.
Next, we look over the codes we’ve created, identify patterns among them, and start coming up with themes.
Themes are generally broader than codes. Most of the time, you’ll combine several codes into a single theme. In our example, we might start combining codes into themes like this:
At this stage, we might decide that some of our codes are too vague or not relevant enough (for example, because they don’t appear very often in the data), so they can be discarded.
Other codes might become themes in their own right. In our example, we decided that the code “uncertainty” made sense as a theme, with some other codes incorporated into it.
Again, what we decide will vary according to what we’re trying to find out. We want to create potential themes that tell us something helpful about the data for our purposes.
Now we have to make sure that our themes are useful and accurate representations of the data. Here, we return to the data set and compare our themes against it. Are we missing anything? Are these themes really present in the data? What can we change to make our themes work better?
If we encounter problems with our themes, we might split them up, combine them, discard them or create new ones: whatever makes them more useful and accurate.
For example, we might decide upon looking through the data that “changing terminology” fits better under the “uncertainty” theme than under “distrust of experts,” since the data labelled with this code involves confusion, not necessarily distrust.
Now that you have a final list of themes, it’s time to name and define each of them.
Defining themes involves formulating exactly what we mean by each theme and figuring out how it helps us understand the data.
Naming themes involves coming up with a succinct and easily understandable name for each theme.
For example, we might look at “distrust of experts” and determine exactly who we mean by “experts” in this theme. We might decide that a better name for the theme is “distrust of authority” or “conspiracy thinking”.
Finally, we’ll write up our analysis of the data. Like all academic texts, writing up a thematic analysis requires an introduction to establish our research question, aims and approach.
We should also include a methodology section, describing how we collected the data (e.g. through semi-structured interviews or open-ended survey questions ) and explaining how we conducted the thematic analysis itself.
The results or findings section usually addresses each theme in turn. We describe how often the themes come up and what they mean, including examples from the data as evidence. Finally, our conclusion explains the main takeaways and shows how the analysis has answered our research question.
In our example, we might argue that conspiracy thinking about climate change is widespread among older conservative voters, point out the uncertainty with which many voters view the issue, and discuss the role of misinformation in respondents’ perceptions.
If you want to know more about statistics , methodology , or research bias , make sure to check out some of our other articles with explanations and examples.
- Normal distribution
- Measures of central tendency
- Chi square tests
- Confidence interval
- Quartiles & Quantiles
- Cluster sampling
- Stratified sampling
- Discourse analysis
- Cohort study
- Peer review
- Ethnography
Research bias
- Implicit bias
- Cognitive bias
- Conformity bias
- Hawthorne effect
- Availability heuristic
- Attrition bias
- Social desirability bias
Cite this Scribbr article
If you want to cite this source, you can copy and paste the citation or click the “Cite this Scribbr article” button to automatically add the citation to our free Citation Generator.
Caulfield, J. (2023, June 22). How to Do Thematic Analysis | Step-by-Step Guide & Examples. Scribbr. Retrieved March 26, 2024, from https://www.scribbr.com/methodology/thematic-analysis/
Is this article helpful?
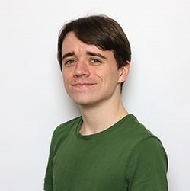
Jack Caulfield
Other students also liked, what is qualitative research | methods & examples, inductive vs. deductive research approach | steps & examples, critical discourse analysis | definition, guide & examples, "i thought ai proofreading was useless but..".
I've been using Scribbr for years now and I know it's a service that won't disappoint. It does a good job spotting mistakes”
Listen to your gut: Research discusses using microbiota analysis for precision health care
T he human body harbors approximately 30 trillion microbes, known collectively as the microbiota. These microorganisms influence various bodily functions, including digestion and metabolism to immune response, according to Pak Kin Wong, Penn State professor of biomedical engineering and of mechanical engineering. Analysis of microbiota holds potential for informing disease diagnosis, prognosis predictions and treatment, Wong said, but has yet to be adopted into clinical decision-making.
Penn State News spoke to Wong about his recent paper , published in Nature Reviews Bioengineering , that discusses the methods available for incorporating microbiota analysis into clinical decision-making, the challenges of doing so and the need for new technologies to capitalize on the potential of microbiota's role in medicine.
What is microbiota analysis? Why is it important? What medically useful information does it provide?
Microbiota analysis involves examining the composition, diversity, abundance, distribution, evolution and functions of microorganisms within specific environments, employing advanced genomic and bioinformatic tools. In the human body, these microbes are pivotal to health and disease management.
For instance, the gut microbiota is essential for intestinal health through the production of vital fermentation products and metabolites. This microbial community is also linked to various bodily functions and diseases, highlighting its significance beyond just the gut.
By analyzing the microbiota, we can gain invaluable insights for disease diagnosis, predict disease progression and tailor treatments to individual needs, paving the way for personalized medicine. For example, microbiota analysis has been crucial in understanding conditions like inflammatory bowel disease and obesity, offering new avenues for intervention.
Why isn't microbiota analysis currently incorporated into medical decision-making? What needs to be done in order to change that?
High-throughput sequencing, which allows rapid evaluation of the DNA content in a sample, has been a game-changer for studying human microbiomes, helping us dive deep into research and discovery. Yet, turning these insights into something we can use in clinics isn't straightforward. These methods can be expensive, slow, complex and labor intensive.
For microbiota analysis to fit into medical settings, it needs to be affordable, quick and user-friendly. And there's more—there are standard sequencing struggles with things like telling apart living from dead microbes, analyzing different kinds of biological molecules together and mapping out where microbes are located. In addition, since the mix of microbes varies so much from person to person, and because there's no one-size-fits-all for what a "healthy" microbiome looks like, it's tricky to adopt microbiota analysis into medical decision-making.
To really get how diseases progress, we need to keep an eye on how someone's microbiota changes over time. This tracking is key not just for understanding diseases but also for making sure treatments that affect the microbiome are working as they should. All these challenges are significant hurdles for making the most of our knowledge about the microbiome in real-world health care.
What are some examples of medical conditions that could benefit from microbiota analysis? How would it work?
Analyzing the differences in microbial composition between healthy individuals and those with specific diseases offers medical professionals crucial insights into patient health, disease risk and potential treatment outcomes. The diversity and types of microbes in our intestines, for example, are linked to a range of health issues, such as C. difficile infections, inflammatory bowel diseases and neurodegenerative conditions.
Beyond diagnosis and prognosis, there are groundbreaking approaches to altering these microbial communities to fight diseases more effectively. Strategies like the use of prebiotics, probiotics and fecal microbiota transplants are being employed to tackle persistent infections and improve the efficacy of treatments. Specifically, fecal transplants have emerged as a pivotal therapy for refractory C. difficile infections and show promise in enhancing cancer treatment outcomes.
Moreover, the direct bladder administration of the BCG, a form of bacterial immunotherapy approved by FDA, has been an effective bladder cancer treatment for decades. These developments highlight the critical importance of microbiota analysis and the innovative potential of leveraging our microbiota to combat diseases.
What next steps do you plan on taking in your research related to this topic?
We have been collaborating with experts from academia, industry and the clinical field, each bringing extensive knowledge in technology, biology and health care. Currently, we are developing innovative microbiota analysis platforms that employ single-cell analysis and artificial intelligence.
A significant challenge we face is determining the most effective methods to unlock the medical potential of the human body's microbiota. Our primary focus is on identifying a viable application that not only underscores the intrinsic value of microbiota analysis but also accelerates its integration into clinical practice.
As the health care landscape evolves, the full utilization of microbiota analysis stands to revolutionize the field. This journey we're on is all about innovation and collaboration. We believe it's going to take us to a future where precision medicine makes better health and well-being achievable for a wider array of people.
More information: Jyong-Huei Lee et al, Translating microbiota analysis for clinical applications, Nature Reviews Bioengineering (2024). DOI: 10.1038/s44222-024-00168-3
Provided by Pennsylvania State University
- Find a Branch
- Schwab Brokerage 800-435-4000
- Schwab Password Reset 800-780-2755
- Schwab Bank 888-403-9000
- Schwab Intelligent Portfolios® 855-694-5208
- Schwab Trading Services 888-245-6864
- Workplace Retirement Plans 800-724-7526
... More ways to contact Schwab
Chat
- Schwab International
- Schwab Advisor Services™
- Schwab Intelligent Portfolios®
- Schwab Alliance
- Schwab Charitable™
- Retirement Plan Center
- Equity Awards Center®
- Learning Quest® 529
- Mortgage & HELOC
- Charles Schwab Investment Management (CSIM)
- Portfolio Management Services
- Open an Account
Bull Flags: Sample Plan
Throughout this course we've broken down what an investing plan is and talked about its different components in great detail. In this lesson, we brought concepts together to demonstrate the construction of an investing plan. Next, we'll examine a sample investing plan, which you can find on the course dashboard, based on swing trading bull flag patterns. We'll walk through the reasoning behind the plan to help you develop your own plan.
Bull flags as short-term price patterns
The bull flag plan is a very short-term investing plan with trades that often last just a couple of days. It's also fairly discretionary, which means it leaves a lot of interpretation up to the trader. In this plan, we're simply considering trading almost any pullback or consolidation in an uptrend as a bull flag pattern.
Therefore, you'll be applying the breakout and bounce entries you learned about in a previous lesson within the context of bull flag patterns.
As a quick refresher, bull flags are short-term price patterns used by swing traders to potentially capitalize on quick market moves. They're usually three days to three months in length. Their construction starts with a strong initial move known as a flagpole, which is followed by a short-term sideways trend, or pullback, with roughly parallel support and resistance. There are different types of flag patterns, and we'll cover those as we examine sample entry rules.

Bull flag objective
A sample objective for this pattern could be: "To learn how to trade short-term rallies in an intermediate-term uptrend using bull flag price patterns." Remember an uptrend doesn't guarantee the trend will continue and the stock price may move in the opposite direction.
The objective defines the trend as short term, within the context of the larger trend. It then states flag patterns as the trading technique. Finally, it points out that it's a bullish plan so you know in which market conditions to use it.
Now let's discuss sample watchlist criteria for potential bull flag stocks. One way to identify stocks with potential bull flag patterns is to use charts and a scanner to find stocks exhibiting upward momentum and liquidity.
- Upward momentum can be identified with an intermediate uptrend using a one-year daily chart where the stock's price is creating higher highs and higher lows.
- Stocks that have a minimum average daily volume of more than 500,000 per day and trade at no less than $10 per share tend to have enough liquidity to reduce the risk of slippage.
Let's look at an example. Below is a historical chart of Skyworks Solutions (SWKS). As you can see, the stock was uptrending denoted by its higher highs and higher lows. The volume ranged between 3 million and 15 million shares per day. And it traded well above $10 per share.

For illustrative purposes only. Not a recommendation of any security or strategy.
These broad watchlist criteria allow you to find bull flag candidates in many places. However, it can still be difficult to search efficiently. The paperMoney ® Scan tab can make searching easier. In the following video, you'll learn you how to find and use the Scan tab to build a watchlist of potential candidates.
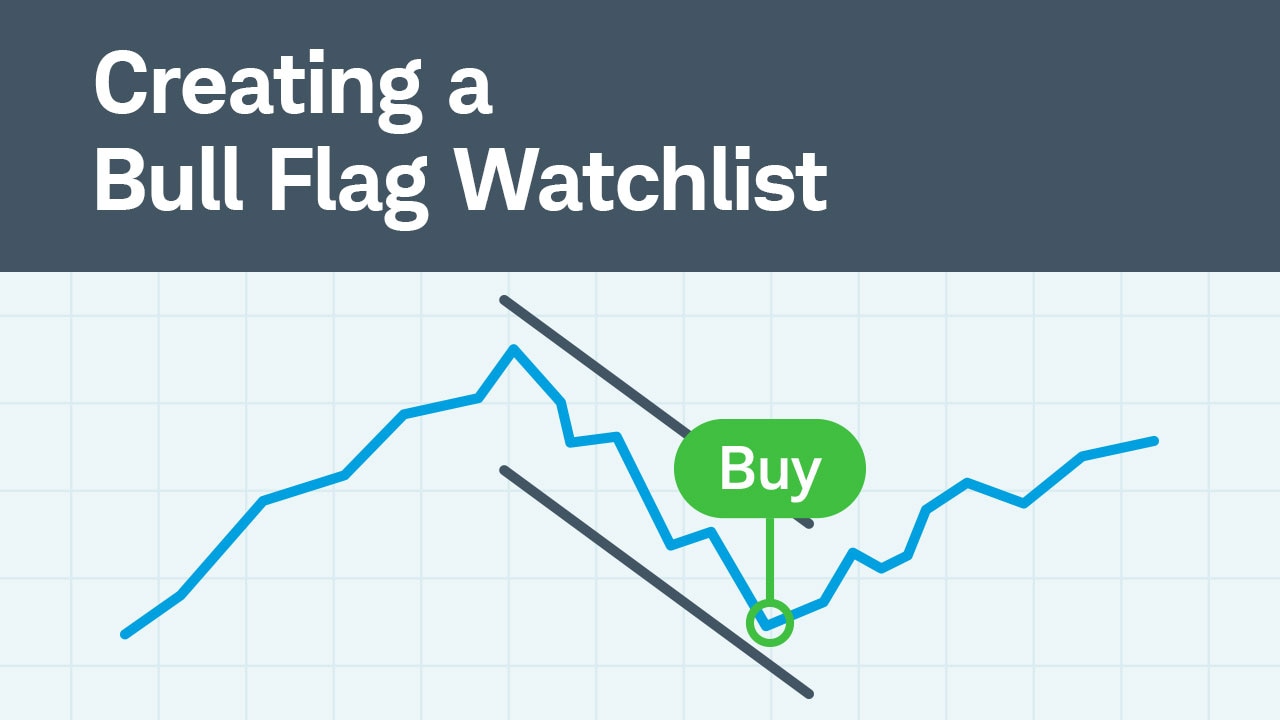
Watch video: Creating a Bull Flag Watchlist
Creating a Bull Flag Watchlist
Upbeat music plays throughout.
Narrator: The paperMoney ® platform's Scan tab allows you to search for stocks that meet certain criteria. In this demo, I'll show you how to use the scanner to create a watchlist based on the criteria from the bull flag sample investing plan.
On-screen text: Disclosure: The paperMoney® software application is for educational purposes only. Successful virtual trading during one time period does not guarantee successful investing of actual funds during a later time period as market conditions change continuously.
Narrator: To get to the scanner, first I'll click the Scan tab.
The scanner works by creating filters to weed through the thousands of publicly traded stocks. There are a few types of filters to choose from. For this example, we use stock filters and study filters.
We'll add filters for each of the rules we established in our sample watchlist criteria. The first rule states, "Upward momentum can be identified with an intermediate uptrend using a one-year daily chart where the stock's price is creating higher highs and higher lows."
While there isn't a filter for that exact criteria, we can assume that a stock that has positive price performance is more likely to be uptrending, so we'll start there.
To create the filter, I'll click the Add study filter . Now I need to set the criteria. First, I'll click the Study list. Next, I'll point to Price Performance and then select Price Change .
Notice how the filter almost reads like a sentence. By default the study would filter for stocks whose most recent closing price is at least 2% greater than it was 10 bars ago (or 10 days ago in this case). For this example, we'll set a minimum of 10%, which will filter out common fluctuations and help make sure the stock price is actually moving up.
Now we need to determine the number of days. A 10% increase over 10 days is pretty big, so for this example we'll use 100 days, which is more likely to reflect an extended uptrend.
In addition to finding stocks that are simply uptrending, I also want to find stocks that have pulled back a little. A pullback usually increases the likelihood of finding a flag pattern. In order to do this, I'll add two more filters--the Near High Lows study and the Price Direction study.
To understand what the Near_High_Lows does, let's read it like a sentence again: "The study will filter for stocks whose current price is within 3% of the 252-period high."
On average there are 252 trading days in a year. So, this will help us first identify stocks that are likely near their 52-week highs, which usually increases the chances the stock is uptrending.
Second, the range of 3% allows the stocks to pull back slightly from this high, which gives the potential for a flag pattern. Let's keep this default and move on.
The Price Direction filter finds stocks that have moved in a certain direction for a certain period of time. Since, as I mentioned, we want stocks that have pulled back a bit, I'll select decreased .
The next watchlist criteria deals with liquidity. It states, "Stocks that have a minimum average daily volume of 250,000 per day and trade at no less than $10 per share tend to have enough liquidity to help reduce the risk of slippage."
These liquidity measures deal directly with the stock's characteristics. So, I'll add two stock filters: one for volume and one for price.
With the first filter I'll search for stocks with a minimum volume of 250,000 shares. This helps to reduce the chance that we'll get stocks that are easily manipulated by a few large orders. Notice that this volume filter reduced the potential number of candidates to 1,719.
Next, I'll search for stocks with a closing price of at least $10. A $10 minimum avoids penny stocks and stocks that are easily manipulated by a few large orders. Notice the potential matches dropped to 6,320. Let's run the scan and see what happens when these are filtered through the studies. I'll click Scan to run the search.
It appears three stocks meet our criteria today. I'll save these results as a watchlist by clicking the action menu and selecting Save as Watchlist .
In this new window I'll name the watchlist Bull Flags and click Save .
Next, we can examine these stocks for potential bull flags by going to the Charts tab.
The watchlist is available in the account pane. To see the list, I'll select Bull Flags .
Now, here's a tip. To speed up your analysis, you can link the watchlist with the chart. First, set a color for the watchlist. I'll select the red box with the number one.
Now, I'll do the same with the chart.
As we move through the watchlist, stock charts show up immediately. The last step is to look through the charts to find a potential bull flag.
Remember, the goal of running a scan is to build a watchlist of potential candidates. You can experiment with the filters until you find the results you're looking for. Then you can keep an eye on the watchlist until entry signals appear.
On-screen text: [Schwab logo] Own your tomorrow ®
Schwab traders get in-depth research tools
More from charles schwab.

How to Use Momentum Indicators

How to Calculate Stock Profit

What Are Options Collars?
Related topics.
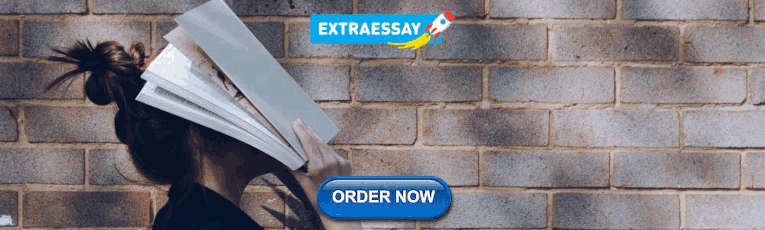
IMAGES
VIDEO
COMMENTS
A good data analysis plan should summarize the variables as demonstrated in Figure 1 below. Figure 1. Presentation of variables in a data analysis plan. 5. Statistical software. There are tons of software packages for data analysis, some common examples are SPSS, Epi Info, SAS, STATA, Microsoft Excel.
A Data Analysis Plan (DAP) is about putting thoughts into a plan of action. Research questions are often framed broadly and need to be clarified and funnelled down into testable hypotheses and action steps. The DAP provides an opportunity for input from collaborators and provides a platform for training. Having a clear plan of action is also ...
analysis plan: example. • The primary endpoint is free testosterone level, measured at baseline and after the diet intervention (6 mo). • We expect the distribution of free T levels to be skewed and will log-transform the data for analysis. Values below the detectable limit for the assay will be imputed with one-half the limit.
The first step in a data analysis plan is to describe the data collected in the study. This can be done using figures to give a visual presentation of the data and statistics to generate numeric descriptions of the data. Selection of an appropriate figure to represent a particular set of data depends on the measurement level of the variable.
Analysis Plan and Manage Data. The main tasks are as follows: 1. Create an analysis plan • Identify research questions and/or hypotheses. • Select and access a dataset. • List inclusion/exclusion criteria. • Review the data to determine the variables to be used in the main analysis. • Select the appropriate statistical methods and ...
A data analysis plan is a roadmap for how you're going to organize and analyze your survey data—and it should help you achieve three objectives that relate to the goal you set before you started your survey: Answer your top research questions. Use more specific survey questions to understand those answers. Segment survey respondents to ...
A statistical analysis plan (SAP) is a document that specifies the statistical analysis that will be performed on a given dataset. It serves as a comprehensive guide for the analysis, presenting a clear and organized approach to data analysis that ensures the reliability and validity of the results. SAPs are most widely used in research, data ...
A data analysis plan includes many features of a research project in it with a particular emphasis on mapping out how research questions will be answered and what is necessary to answer the question. Below is a sample template of the analysis plan. The majority of this diagram should be familiar to someone who has ever done research.
Get Help. Designing an analysis plan ensures data collection methods meet the needs of the research question, and that the study is accurately powered to produce meaningful results. Based on investigator affiliations and the type of analysis, consultative services are available to discuss statistical methods, analysis software, or potential ...
A data analysis plan is a roadmap for how you can organise and analyse your survey data. Learn how to write an effective survey data analysis plan today. ... Doing this will help you know which survey questions to refer to for specific research topics. For example, to find out which parts of the conference attendees liked the best, look at the ...
22.1 Writing the Data Analysis Plan. Congratulations! You have now arrived at one of the most creative and straightforward, sections of your grant proposal. You and your project statistician have one major goal for your data analysis plan: You need to convince all the reviewers reading your proposal that you would know what to do with your data ...
Concrete research questions are essential for determining the analyses required. The analysis plan should then describe the primary and secondary outcomes, the determinants and data needed, and which statistical techniques are to be used to analyse the data. The following issues need to be considered in this process and described where ...
for your research project (e.g., a dated measure, a measure with poor reliability, a mea-sure that is too long, too short, too difficult, or not in the format that you would prefer for your particular sample). You may be proposing to use a standardized instrument in an untested sample (e.g., a community sample, a sample with representation from a
Data Analysis Plan: Ultimate Guide and Examples. Learn the post survey questions you need to ask attendees for valuable feedback. Once you get survey feedback, you might think that the job is done. The next step, however, is to analyze those results. Creating a data analysis plan will help guide you through how to analyze the data and come to ...
Step 1: Write your hypotheses and plan your research design. To collect valid data for statistical analysis, you first need to specify your hypotheses and plan out your research design. Writing statistical hypotheses. The goal of research is often to investigate a relationship between variables within a population. You start with a prediction ...
It is driven by the analytic objectives and is a key part of the analysis plan. In its essence, it is a process of data selection by source (e.g., individual, group, observation event), domain of inquiry, and/or question asked. Here we illustrate this process with two examples from our research.
Research proposal examples. Writing a research proposal can be quite challenging, but a good starting point could be to look at some examples. We've included a few for you below. Example research proposal #1: "A Conceptual Framework for Scheduling Constraint Management".
1.1. The statistical analysis plan. The Statistical Analysis Plan (SAP) is a key document that complements the study protocol in randomized controlled trials (RCT). SAPs are a vital component of transparent, objective, rigorous, reproducible research.
Creating an analysis plan is an important way to ensure you collect all the data you need and that you use all the data you collect. 2. An FETP resident created an analysis plan by including the following: Research question(s) and/or hypotheses. Dataset to be used. Table shells for univariable analysis.
QDA Method #1: Qualitative Content Analysis. Content analysis is possibly the most common and straightforward QDA method. At the simplest level, content analysis is used to evaluate patterns within a piece of content (for example, words, phrases or images) or across multiple pieces of content or sources of communication. For example, a collection of newspaper articles or political speeches.
Definition of research in data analysis: According to LeCompte and Schensul, research data analysis is a process used by researchers to reduce data to a story and interpret it to derive insights. The data analysis process helps reduce a large chunk of data into smaller fragments, which makes sense. Three essential things occur during the data ...
When to use thematic analysis. Thematic analysis is a good approach to research where you're trying to find out something about people's views, opinions, knowledge, experiences or values from a set of qualitative data - for example, interview transcripts, social media profiles, or survey responses. Some types of research questions you might use thematic analysis to answer:
If you want to learn how to write your own plan for your research project, consider the following seven steps: 1. Define the project purpose. The first step to creating a research plan for your project is to define why and what you're researching. Regardless of whether you're working with a team or alone, understanding the project's purpose can ...
Penn State News spoke to Wong about his recent paper, published in Nature Reviews Bioengineering, that discusses the methods available for incorporating microbiota analysis into clinical decision ...
A sample objective for this pattern could be: "To learn how to trade short-term rallies in an intermediate-term uptrend using bull flag price patterns." Remember an uptrend doesn't guarantee the trend will continue and the stock price may move in the opposite direction. The objective defines the trend as short term, within the context of the larger trend.