Most Popular
10 days ago
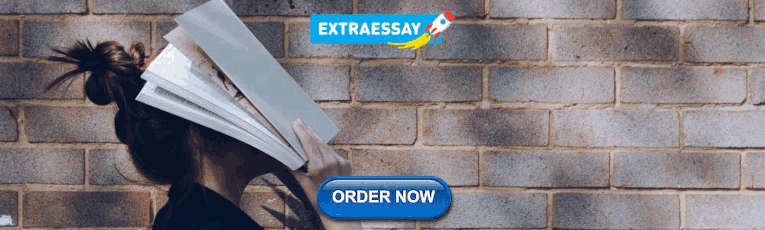
English and Social Studies Teachers Pioneer AI Usage in Schools, Study Finds
12 days ago
Best Summarising Strategies for Students
How to cite page numbers in apa, what is accidental plagiarism, is summarizing books a good way to retain knowledge redditors weigh in, climate change thesis statement examples.
freepik.com
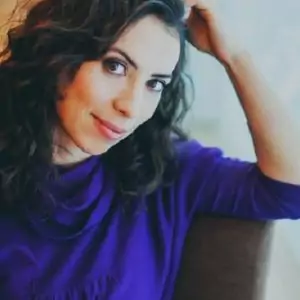
Climate change is an urgent global issue, characterized by rising temperatures, melting glaciers, and extreme weather events. Writing a thesis on this topic requires a clear and concise statement that guides the reader through the significance, focus, and scope of your study. In this piece, we will explore various examples of good and bad thesis statements related to climate change to guide students in crafting compelling research proposals.
Good Examples
Focused Approach: “This thesis will analyze the impact of climate change on the intensity and frequency of hurricanes, using data from the last three decades.” Lack of Focus: “Climate change affects weather patterns.”
The good statement is specific, indicating a focus on hurricanes and providing a time frame. In contrast, the bad statement is too vague, covering a broad topic without any specific angle.
Clear Stance: “Implementing carbon taxes is an effective strategy for governments to incentivize companies to reduce greenhouse gas emissions.” Not So Clear: “Carbon taxes might be good for the environment.”
The good statement takes a clear position in favor of carbon taxes, while the bad statement is indecisive, not providing a clear standpoint.
Researchable and Measurable: “The thesis explores the correlation between the rise in global temperatures and the increase in the extinction rates of North American mammal species.” Dull: “Global warming is harmful to animals.”
The good statement is researchable and measurable, with clear variables and a focused geographic location, while the bad statement is generic and lacks specificity.
Bad Examples
Overly Broad: “Climate change is a global problem that needs to be addressed.”
This statement, while true, is overly broad and doesn’t propose a specific area of focus, making it inadequate for guiding a research study.
Lack of Clear Argument: “Climate change has some negative and positive effects.”
This statement doesn’t take a clear stance or highlight specific effects, making it weak and uninformative.
Unoriginal and Unengaging: “Climate change is real.”
While the statement is factual, it doesn’t present an original argument or engage the reader with a specific area of climate change research.
Crafting a compelling thesis statement on climate change is crucial for directing your research and presenting a clear, focused, and arguable position. A good thesis statement should be specific, take a clear stance, and be researchable and measurable. Avoid overly broad, unclear, unoriginal, or unengaging statements that do not provide clear direction or focus for your research. Utilizing the examples provided, students can navigate the intricate process of developing thesis statements that are not only academically rigorous but also intriguing and relevant to the pressing issue of climate change.
Follow us on Reddit for more insights and updates.
Comments (0)
Welcome to A*Help comments!
We’re all about debate and discussion at A*Help.
We value the diverse opinions of users, so you may find points of view that you don’t agree with. And that’s cool. However, there are certain things we’re not OK with: attempts to manipulate our data in any way, for example, or the posting of discriminative, offensive, hateful, or disparaging material.
Cancel reply
Your email address will not be published. Required fields are marked *
Save my name, email, and website in this browser for the next time I comment.
More from Thesis Statement Examples and Samples
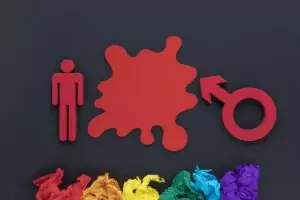
Sep 30 2023
Gender & Sexuality Studies Thesis Statement Examples
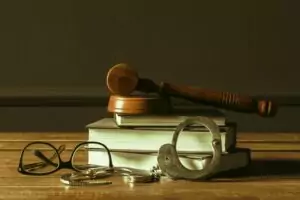
Criminal Justice Reform Thesis Statement Examples
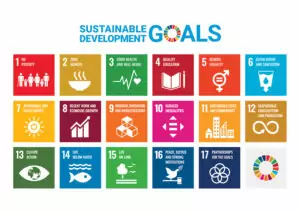
Sustainable Development Goals (SDGs) Thesis Statement Examples
Remember Me
What is your profession ? Student Teacher Writer Other
Forgotten Password?
Username or Email
- Clim. Change MSc
- About the MSc
- > ENV-7014A
- Dissertation
Click here for a listing in date order.
Previous dissertation topics
ESSAYS ON CLIMATE CHANGE MITIGATION, BUILDING ENERGY EFFICIENCY, AND URBAN FORM
Add to collection, downloadable content.
- March 21, 2019
- Affiliation: College of Arts and Sciences, Department of City and Regional Planning
- This dissertation includes three self-contained and interrelated papers on climate change mitigation, building energy efficiency, and urban form. Paper 1: Urban form and household electricity consumption: a multilevel study While urban form affects building energy consumption, the pathways, direction and magnitude of the effect are disputed in the literature. This paper uses a unique dataset to examine the effect of urban form on residential electricity consumption in Ningbo, China. Using survey and utility bill data of 534 households in 46 neighborhoods in the city, I model the electricity use of households using a multi-level regression model. I find that neighborhood street configuration and tree shade are important in controlling residential electricity consumption and, consequently, greenhouse gas emissions. The results suggest that seasonality and dwelling type condition the effect of neighborhood densities on electricity consumption. Neighborhood density is associated with household electricity consumption in summer months, while there is no such association in the winter months. As neighborhood density increases, households in slab and tower apartments in dense urban neighborhoods consume more electricity in summer months, which can be partly explained by exacerbated heat island effect. Interestingly, the neighborhood density is negatively associated with electricity consumption for single-family houses, suggesting that the effect of neighborhood density is different for different types of dwelling units. Paper 2: Explaining spatial variations in residential energy usage intensity in Chicago: the role of urban form and geomorphometry Understanding the spatial pattern of energy consumption within buildings is essential to urban energy planning and management. In this study, I explore the spatial complexity of residential energy usage intensity, with a focus on urban form and the geomorphometry attributes of urban ventilation, solar insolation, and vegetation. I use building energy use data in Chicago at a Census tract level and merge information from various datasets including parcel attributes, three-dimensional data geometry, aerial imagery, and Census. Using spatial regression models, I find that while vegetation has more local impact on energy intensity, urban porosity and roughness length have consistent spillover effects on building electricity usage intensity in Chicago. Additionally, these relationships are seasonally varied: while vegetation, ventilation, and insolation affect electricity usage in summer, they have no impact on the winter gas consumption. The results highlight the importance of spatially explicit policies and clear urban design and form frameworks for reducing urban energy consumption and mitigating climate change. Paper 3: Government response to climate change in China: a study of first-generation provincial and municipal plans In this paper, I provide an overview of the first-generation local and regional climate change plans in China by scrutinizing planning documents of 16 cities, four autonomous regions, and 22 provinces. I develop and apply an evaluation protocol to understand goals, process, and strategies in these plans. The results indicate that provincial and local plans include numerous policies and strategies, yet some important types of local policies, such as land use and urban form, are not well identified in the plans. The results also indicate that current climate change planning in China is characterized by the “top-down” approach, in which the central governmental incentives play a vital role in shaping provincial and municipal plans. In addition, most plans have the following issues: vague definition of what characterizes a low carbon city/region, deficiency in the quality of GHG inventory and reduction targets, inadequate stakeholder engagement, and weak horizontal coordination. Finally, I offer recommendations to improve climate change planning in China.
- Urban planning
- Urban sustainability
- Low carbon city
- Energy use intensity
- Plan evaluation
- Geomorphometry
- https://doi.org/10.17615/7kkx-f797
- Dissertation
- Doctor of Philosophy
- University of North Carolina at Chapel Hill Graduate School
- June 13, 2018
This work has no parents.
- UNC-Chapel Hill Climate Change Resources
Select type of work
Master's papers.
Deposit your masters paper, project or other capstone work. Theses will be sent to the CDR automatically via ProQuest and do not need to be deposited.
Scholarly Articles and Book Chapters
Deposit a peer-reviewed article or book chapter. If you would like to deposit a poster, presentation, conference paper or white paper, use the “Scholarly Works” deposit form.
Undergraduate Honors Theses
Deposit your senior honors thesis.
Scholarly Journal, Newsletter or Book
Deposit a complete issue of a scholarly journal, newsletter or book. If you would like to deposit an article or book chapter, use the “Scholarly Articles and Book Chapters” deposit option.
Deposit your dataset. Datasets may be associated with an article or deposited separately.
Deposit your 3D objects, audio, images or video.
Poster, Presentation, Protocol or Paper
Deposit scholarly works such as posters, presentations, research protocols, conference papers or white papers. If you would like to deposit a peer-reviewed article or book chapter, use the “Scholarly Articles and Book Chapters” deposit option.

Sustainable UCL
- What UCL does
- Take Action
- Search by Topic
- News and Events
- Case Studies

Sustainability Dissertation Topics
Students can use the campus to test solutions to real-world sustainability challenges as part of a Living Lab project. Here are some sustainability dissertation ideas.
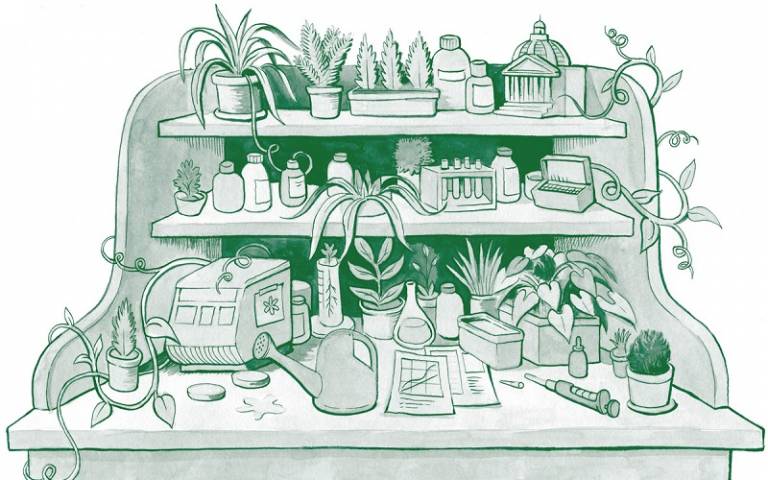
19 November 2022
Dissertation topics
Living lab for a positive climate .
Our goal is to have net zero-arbon buildings by 2024 and to be a net zero-carbon institution by 2030.
Research topics include:
- Review UCL buildings for climate change resilience and implement adaptation measures
- Research into enhancing video conferencing for large events
- Sourcing renewable energy providers for gas to enable a zero-carbon heating supply
- Research on academic travel reduction initiatives
- Research on UCL’s new Climate Accountability Scheme (combining a carbon price with behavioural nudges) to incentivise climate action by departments.
- Reducing the climate impact of UCL’s hospitality
Living lab on reducing waste and consumption
Our goal is to reduce waste per person by 20% and to become a single-use plastic-free campus by 2024.
- How to eliminate plastic across UCL
- How to increase recycling at UCL (campaign)
- Labels and messaging on food
Consumers are becoming more aware of sustainability considerations in food production and consumption. In addition to the traditional dietary and nutritional requirements, consumers are seeking labels and certifications to know where and how food is made, what it is made from, its carbon footprint and sustainability credentials. UCL would like to investigate what information we should supply, how this should be displayed, communicated and marketed, to allow our communities to make informed decisions and promote a flexitarian diet. It is anticipated this would require the following activity:
- Developing questionnaires for opinions on carbon footprinting, carbon pricing and other sustainability information they want to know about, to inform point-of-sale purchasing choices in outlets.
- Comparing marketing of food as “vegan” or “plant-based” and the impacts on consumer’s perceptions.
- Investigating the notion of “label fatigue “where consumers are overwhelmed by information on packaging, and the impact of the project on this.
- Baselining data on food choices.
- Developing a labelling system for packaging/ refectory display boards – using data and survey responses.
- Trialling the labelling system.
- Surveying responses.
- Recording food choices and comparing to baseline, to identify behavioural change.
Living lab on biodiversity
Our aim is to create 10,0000m2 of extra biodiverse space by 2024 – equivalent to more than one and a half football pitches as well as increasing health and wellbeing for the Bloomsbury community.
Research topics include:
- Research on different types of green infrastructure e.g. green walls, roofs, community gardens, and where UCL could implement them.
- Research on biodiversity and wildlife across UCL’s estate.
- Research on air pollution levels across UCL’s estate
Dissertation guide
Step 1: choose your topic.
- Take a look at the sustainability research topics list to see if they interest you.
- If you require data on energy, travel, procurement, UCL’s sustainability engagement programmes or water please email Sustainable UCL to request this.
- If you have alternative research topic ideas for a sustainable living lab dissertation or project please contact us.
Step 2: Discuss with your department
- Speak with your supervisor or someone in your department about undertaking a living lab dissertation and refining your topic.
- They can advise you on scales, time scales and marking criteria.
Step 3: Contact Sustainable UCL
- Arrange a meeting with Sustainable UCL to ensure your project can make a valuable contribution to UCL.
- Sustainable UCL can provide data and put you in touch with relevant operational staff such as catering members and plumbers to test out your ideas.
- You can invite your supervisor to this meeting, but it is not a requirement.
Step 4: Undertake your dissertation
- Sustainable UCL can meet a further 2 times or 2 hours maximum to support your dissertation or project.
Step 5: Sharing data and findings
- One of the principles of the Living Lab Project is to make available any additional data you generate for others to build on, so the work can keep ‘living’
- Once your dissertation is completed, we require a concise 2-3 page briefing or presentation on your findings so that we can implement your ideas onto campus or future students and staff can build on your research. Living Lab projects usually spark plenty of ideas for further research, including aspects you were not able to cover during the project.
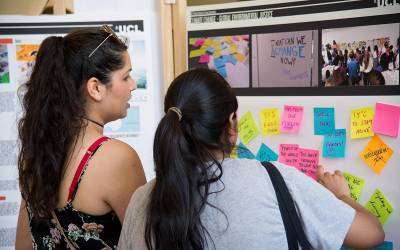
Sustainable Education
Climate Change
- UBC Resources & Guides
- Articles & Databases
- Archives & Historical Sources
UBC Theses & Dissertations
- Reports & Policies
- Data Sources & Visualizations
- News & Social Media
- Civic Engagement
- Indigenous Perspectives
- UBC Library Climate Action
Across UBC, faculty and students contribute to research on climate change. See below for recent theses on a few select topics, and search cIRcle , UBC's open access repository, for publications, theses/dissertation, and presentations to find more.
RSS feed searching the UBC Theses and Dissertations Collection for: "Global warming" OR "Climate change" OR "Greenhouse gas" OR "Renewable energy":
- Bielefeld Academic Search Engine
- EThOS UK e-theses online service (UK's national thesis service)
- NDLTD: Networked Digital Library of Theses and Dissertations A collaborative effort of the NDLTD, OCLC, VTLS, and Scirus, the NDLTD Union Catalog contains more than one million records of electronic theses and dissertations.
- Open Access Theses and Dissertations (OATD)
- OpenAire Explore

- SciELO Scientific Electronic Library Online
- UBC Library Guide: Theses and Dissertations A research guide for locating theses and dissertations from UBC, British Columbia, Canada, and International databases and repositories.
- UBC Theses and Dissertations UBC graduate theses and dissertations are available through the Open Collections portal dating back to 1919.
- << Previous: Archives & Historical Sources
- Next: Reports & Policies >>
- Last Updated: Apr 22, 2024 11:15 AM
- URL: https://guides.library.ubc.ca/climate
Thank you for visiting nature.com. You are using a browser version with limited support for CSS. To obtain the best experience, we recommend you use a more up to date browser (or turn off compatibility mode in Internet Explorer). In the meantime, to ensure continued support, we are displaying the site without styles and JavaScript.
- View all journals
- My Account Login
- Explore content
- About the journal
- Publish with us
- Sign up for alerts
- Open access
- Published: 17 April 2024
Climate damage projections beyond annual temperature
- Paul Waidelich ORCID: orcid.org/0000-0002-5081-776X 1 ,
- Fulden Batibeniz ORCID: orcid.org/0000-0002-1751-3385 2 , 3 , 4 ,
- James Rising ORCID: orcid.org/0000-0001-8514-4748 5 ,
- Jarmo S. Kikstra ORCID: orcid.org/0000-0001-9405-1228 6 , 7 , 8 &
- Sonia I. Seneviratne ORCID: orcid.org/0000-0001-9528-2917 2
Nature Climate Change ( 2024 ) Cite this article
9498 Accesses
294 Altmetric
Metrics details
- Climate-change impacts
- Environmental economics
- Projection and prediction
Estimates of global economic damage from climate change assess the effect of annual temperature changes. However, the roles of precipitation, temperature variability and extreme events are not yet known. Here, by combining projections of climate models with empirical dose–response functions translating shifts in temperature means and variability, rainfall patterns and extreme precipitation into economic damage, we show that at +3 ° C global average losses reach 10% of gross domestic product, with worst effects (up to 17%) in poorer, low-latitude countries. Relative to annual temperature damage, the additional impacts of projecting variability and extremes are smaller and dominated by interannual variability, especially at lower latitudes. However, accounting for variability and extremes when estimating the temperature dose–response function raises global economic losses by nearly two percentage points and exacerbates economic tail risks. These results call for region-specific risk assessments and the integration of other climate variables for a better understanding of climate change impacts.
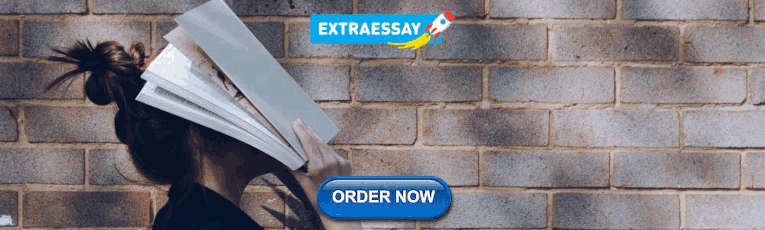
Similar content being viewed by others
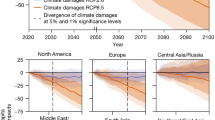
The economic commitment of climate change
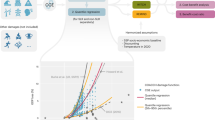
New damage curves and multimodel analysis suggest lower optimal temperature
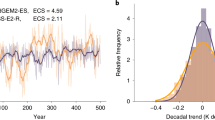
Decadal global temperature variability increases strongly with climate sensitivity
Projections of economic damage from climate change are key for evaluating climate mitigation benefits, identifying effects on vulnerable communities and informing discussions around adaptation needs, as well as loss and damage financing. On a global or country level, such assessments have focused on how projected changes in annual mean temperatures affect gross domestic product (GDP) 1 , 2 , 3 , 4 . However, the widespread losses in recent years driven by flooding and drought suggest that precipitation variability and extremes are similarly important 5 , 6 . Anthropogenic forcing is increasing the frequency and intensity of precipitation extremes and variability on multiple scales, altering daily temperature patterns and driving an overall increase in precipitation over land 7 , 8 . Continued global warming is expected to exacerbate these trends, potentially with uneven impacts across regions 5 , 9 , 10 . Therefore, including precipitation, variability and extremes can improve the precision, comprehensiveness and interpretability of climate change damage estimations 11 .
Economic damage from climate change can be assessed either bottom-up by quantifying, valuating and aggregating specific impacts (for example, crop failures or labour supply changes) or top-down by identifying the statistical relationship between observed climatic shifts and economic growth. While both approaches have advantages and limitations, top-down approaches usually neglect climatic shifts beyond annual temperature changes 12 . To address this shortcoming, recent studies have estimated the relationship between macrolevel income and a wider range of climatic indicators, such as total precipitation 13 , 14 , 15 , temperature variability 16 , 17 or temperature and precipitation extremes and anomalies 14 , 18 , 19 . However, these studies do not investigate how much the inclusion of these climate indicators alters previous economic assessments of climate change, which is highly relevant for policy-making and future adaptation. A notable exception is ref. 15 , which projects the effects of annual precipitation and temperature shifts on inequality. A comprehensive assessment of the projected economic impacts of intense periods of precipitation and temperature anomalies, however, is still missing.
In this study, we draw upon recent advances in estimating dose–response functions, which relate shifts in various climate indicators (total precipitation, temperature variability, precipitation anomalies and extremes) to GDP changes 14 . Combining these functions with projections from 33 models of Coupled Model Intercomparison Project Phase 6 (CMIP6), including two large ensembles, we investigate how including these indicators affects the understanding of future economic impacts at different global warming levels. Variability and extremes introduce substantial climatic and associated economic uncertainties and we conduct a variance decomposition to determine the main uncertainty drivers. Furthermore, we explore how including variability and extremes in empirical regressions alters the dose–response function for annual mean temperature, which the extant literature has estimated controlling only for annual precipitation 1 , 2 , 4 , 20 , 21 .
Projecting GDP impacts for six climate indicators
Compared to annual temperature, future changes in precipitation and temperature variability under climate change are subject to high uncertainties 8 , 22 , 23 . To capture these uncertainties, we use projections from various CMIP6 models 10 to analyse four climate indicators besides annual mean temperature and annual precipitation: (1) day-to-day temperature variability (how much daily temperatures deviate from monthly means); (2) extreme precipitation (the annual sum of precipitation on days with exceptionally high precipitation exceeding the historical 99.9th percentile); (3) monthly precipitation deviation (how much monthly precipitation deviates from historical means throughout the year); and (4) the number of ‘wet days’ with precipitation above 1 mm d −1 . These indicators have been linked to forcing from GHGs 24 , 25 as well as to income growth using a global sample 14 , 16 . Considering all indicators in one coherent estimation framework is crucial because variability and extremes correlate strongly with annual temperature, total precipitation and each other (Supplementary Fig. 3 ). Therefore, combining dose–response functions from different estimations risks double-counting impacts. Notably, our coherent estimation framework 14 does not model damage from droughts and heat waves. Therefore, we include heat in a complementary analysis, whereas we find no significant statistical link to economic growth for droughts, potentially due to limited spatial and temporal resolution and impact heterogeneity across regions (Supplementary Appendix F ).
We illustrate our approach for the example of extreme precipitation impacts on economic output for New York State at +3°C of global warming (Fig. 1 ). On the basis of how a given CMIP6 model and scenario project the respective climate indicator (Fig. 1a ), we compare the GDP impacts in a given year against the average impacts if the climate were to remain constant at levels of a recent baseline period (Fig. 1b ) 2 , 15 . For each model and scenario, the baseline period is the 41-year period during which global warming equals the historical warming between 1979 and 2019 (+0.84 °C), which is the climatic baseline used for estimating the dose–response functions used here ( Methods ) 14 . We then repeat this procedure for different CMIP6 models and potential damage parameter estimates based on statistical uncertainty and aggregate results to the national level. This yields a distribution of GDP impacts for each country featuring all years in each model and scenario associated with the same global warming level (Fig. 1c ). Therefore, the main sources of uncertainty in our GDP impact distribution for a given global warming level and territory are (1) internal variability for the same CMIP6 model because the magnitude of extremes can vary strongly from year to year and, for large ensembles, across model runs, (2) statistical uncertainty in the dose–response functions and (3) projection differences between CMIP6 models.
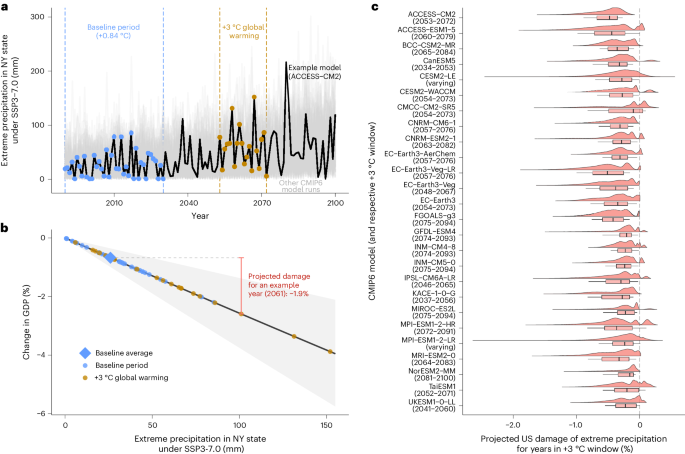
a , Projected extreme precipitation under SSP3-7.0 for an example model run (ACCESS-CM2, black) and other CMIP6 model runs (grey). Vertical lines denote the baseline period (blue) and the +3 °C global warming window (brown). b , Dose–response function for extreme precipitation (black line) and 95% confidence interval (grey area). Coloured dots and the blue diamond represent extreme precipitation levels from a and the baseline period average. The red error bar illustrates the difference between the dose–response function for an example year (2061) and the baseline average, which equals the projected damage for this year. Dose–response function values are transformed from the original logarithmic changes to percentage of GDP by exponentiating and subtracting one ( Methods ). c , Distribution of projected extreme precipitation damage at +3 °C by CMIP6 model. Boxplot centre, hinges and whiskers denote median, upper/lower quartiles and upper/lower deciles, respectively. For the CESM2-LE and MPI-ESM1-2-LR large ensembles, the +3 °C global warming-level window varies across ensemble members.
Source data
Global results.
We examine the impact on global GDP for all indicators combined, as well as the separate impacts from annual temperature, annual precipitation and the four variability and extremes indicators (Fig. 2a ). Global GDP is estimated to be 3.2% lower (lower/upper decile: 1.2–5.4%) at +1.5 °C of global warming, compared to a world with no further climate change beyond recent levels. At +3 °C, global GDP decreases by 10.0% (5.1–14.9%). When disaggregated by climate indicator, global impacts are strongly determined by the annual mean temperature changes, which account for a GDP reduction of 10.0% at +3 °C. This estimate is consistent with recent top-down studies focusing exclusively on damage from annual temperature changes and projecting impacts of 7–14% of GDP per capita loss by the end of the century at global warming levels of more than +4 °C (refs. 4 , 8 , 20 ); with other top-down studies estimating damage even higher 2 as a result of their assumption that temperature changes impact growth trajectories persistently 12 , 26 , 27 . For context, a 10% reduction exceeds the GDP loss of the COVID-19 pandemic when global growth plummeted from +2.6% in 2019 to −3.1% in 2020 or the effect of the global financial crisis in 2009 when global output shrunk by −1.3% (ref. 28 ). Importantly, recent research suggests that damage attributed to annual temperature covers heat wave impacts at least partially 18 . Indeed, when disentangling the two, we find that almost half of annual temperature damage can be attributed to heat extremes (Supplementary Appendix F ).
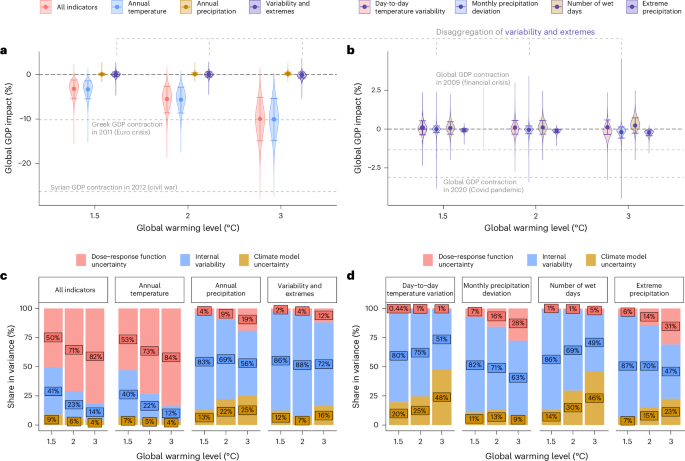
a , Points and the error bars centred around them denote the mean and upper-to-lower-decile range, respectively. ‘Variability and extremes’ comprises the four indicators in b . Labelled grey horizontal lines denote example growth rates in real GDP 28 . b , Same as a , with ‘variability and extremes’ impacts disaggregated by indicator. c , Variance decomposition for the combined GDP impacts of all climate indicators and for indicator-specific impacts. Indicator-specific decompositions are feasible because impacts in the underlying regression model are additive and hence can be projected separately. For absolute variances and coefficients of variation, see Supplementary Figs. 8 and 9 . d , Same as c , with ‘variability and extremes’ disaggregated by indicator.
By contrast, increases in annual precipitation in many areas have a small positive impact on global GDP (0.2% at +3 ° C warming) and the combined impact of the variability and extremes indicators remains centred around zero. While this suggests a lack of signal, disaggregating projections by individual indicators reveals otherwise (Fig. 2b ). At +3 °C, extreme precipitation reduces global GDP by 0.2% (0.1–0.4%), with 99% of our impact distribution indicating economic losses as extreme precipitation increases around the globe (Supplementary Fig. 5 ). Notably, these impacts are much lower than annual temperature damage. This is somewhat expected because extremes have a lower temporal and spatial correlation. Therefore, aggregation from daily, location-specific events to annual indicators and country-level projections reduces signals more strongly compared to annual mean temperature 13 , 14 . However, a 0.2% GDP loss due to extreme precipitation globally for an average year still represents a tenth of the damage caused by the catastrophic 2022 floods in Pakistan, estimated at 2.2% of GDP 29 . Global GDP losses from extreme precipitation are compensated, on average, by temperature variability reductions in higher latitudes (+0.1% of global GDP at +3 °C) 24 , 30 and fewer wet days (+0.2%). However, only 63% and 74% of the impact distribution imply global economic gains for these indicators, respectively. Monthly precipitation deviations, on average, add to global GDP losses (0.2% at +3 °C), but uncertainty ranges remain centred around zero.
To explore uncertainty drivers, we decompose the variance in GDP impacts from each climate indicator into statistical dose–response function uncertainty, climate model uncertainty and internal variability (Fig. 2c ). For annual temperature damage, uncertainty is primarily driven by the dose–response function, particularly at higher global warming levels. By contrast, impact uncertainty for annual precipitation and variability and extremes is dominated by internal variability. Additional analyses focusing on the two large ensembles in our sample suggest that this stems primarily from interannual variation within model runs rather than differences across ensemble members (Supplementary Figs. 15 and 16 ). Moreover, disagreement between CMIP6 models plays either a comparable or a more substantial role than dose–response function uncertainty for these additional indicators (except for monthly precipitation deviation) and is particularly pronounced for day-to-day temperature variability and the number of wet days (Fig. 2d ). Notably, the share of climate model uncertainty decreases in global warming for annual temperature impacts but not for variability and extremes because even for a stronger global warming signal, GDP impact projections do not converge between models.
Country-level results
Because global aggregates risk masking heterogeneities across regions, we further investigate the combined country-level GDP impacts from all six climate indicators at +3 ° C of warming (Fig. 3a ). All countries face GDP losses, in line with recent evidence that climate change might not benefit cooler countries economically 20 . Impacts are more severe in the Global South and highest in Africa and the Middle East, where higher initial temperatures make countries particularly vulnerable to additional warming. Considering the combined GDP impact of all four variability and extremes indicators reveals a clear North–South divide (Fig. 3b ). While for higher latitudes, the decrease in temperature variability and, for some countries, wet days (Supplementary Fig. 5) mitigates overall GDP damage, variability and extremes exacerbate GDP losses in most parts of the Global South. However, these effects vary substantially across the full distribution of projected impacts for each country.
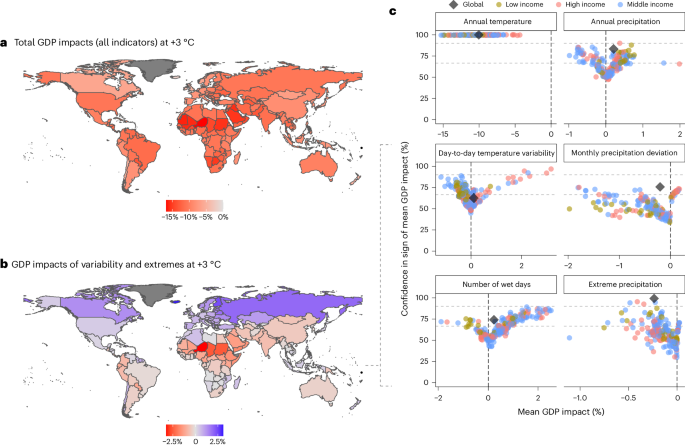
a , Mean GDP impact at +3 °C of global warming for sovereign countries (other territories in dark grey) considering all six indicators in c , using shapefiles from ref. 42 . b , Same as a but only considering the bottom four ‘variability and extremes’ indicators in c . c , Mean GDP impact ( x axis) and share of the impact distribution agreeing with the sign of the mean ( y axis) for sovereign countries by World Bank income group (colour) and the global economy (grey diamond) at +3 °C. Middle income comprises lower- and upper-middle-income countries for conciseness. Dashed horizontal lines denote thresholds for 66% and 90% likelihood following IPCC terminology 8 .
Annual temperature is the only indicator where negative impacts arise for at least 90% of our impact distribution for all countries (upper dashed line in Fig. 3c ), including substantial impacts in several colder countries partially because the temperature dose-response function deployed here implicitly features damages from higher inter-annual temperature variability as well (Supplementary Appendix C). Annual precipitation increases benefit most countries on average, but for many countries, less than two-thirds of the distribution supports the sign of expected impacts (lower dashed line). For day-to-day temperature variability, we find a clear divide between relatively certain gains for a few high-income countries and less certain, smaller losses for many lower-income countries as a result of variability increases in lower latitudes 24 . While extreme precipitation increases in most regions, projected damages are highest and least uncertain for middle- and high-income countries in higher latitudes such as China and the United States 31 . In contrast, low-income countries are more likely to face losses from shifts in precipitation deviation and wet days, but high uncertainties limit the conclusions that can be drawn.
Overall impact of including variability and extremes
The results in the previous sections seemingly suggest that including variability and extremes in GDP impact projections exacerbates disparities between higher- and lower-income countries (Fig. 3 ) but does not substantially alter the implications of climate change for global GDP (Fig. 2 ), particularly since annual temperature damages capture heat wave impacts to some extent already. However, providing an apples-to-apples comparison with the recent climate economics literature requires calculating damage on the basis of the current ‘status quo’ approach, which (1) projects only damage from annual temperature changes and (2) estimates the relationship between income growth and annual temperature controlling only for annual precipitation 1 , 2 , 4 , 20 , 21 . When comparing the resulting global GDP impacts following this status quo methodology to our approach, which (1) projects damage for all six indicators and (2) controls for all our climate indicators when estimating the temperature dose–response function (Fig. 4a ), we find that including variability and extremes increases global damage at +3 °C of global warming by 1.8 percentage points (10.0% instead of 8.2%).
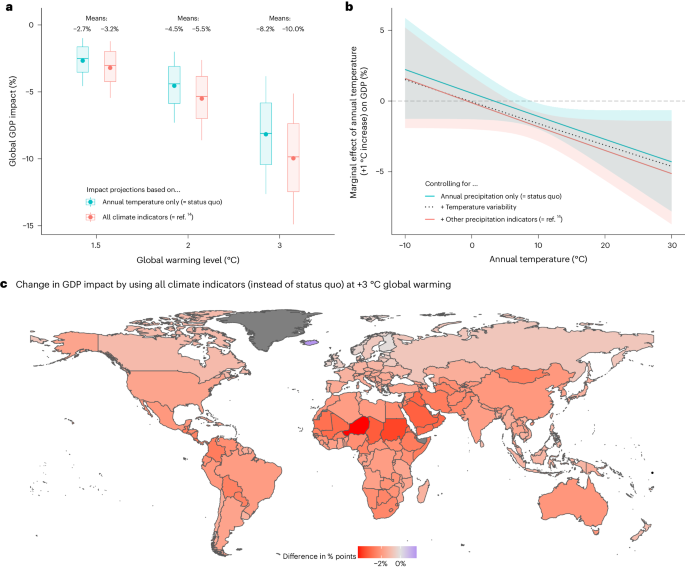
a , Dots represent mean values of the GDP impact distribution, while boxplot centre, hinges and whiskers denote median, upper/lower quartiles and upper/lower deciles, respectively. b , GDP impact of a +1 °C increase in the annual temperature of a territory for different initial temperature levels ( x axis) using mean marginal effects (lines) with 95% confidence intervals around them (shaded area). Estimated using Supplementary equation ( 22 ) and the regression models in Supplementary Table 7 (columns 1, 2 and 5). No confidence interval shown for ‘+ Temperature variability’ for visual conciseness. c , Difference in mean GDP impacts between our main approach and the status quo approach at +3 °C for sovereign countries (other territories marked in dark grey), using shapefiles from ref. 42 .
The main reason for this increase is that controlling for variability and extremes, instead of only for annual precipitation, increases the estimated effect of mean temperature changes (Fig. 4b ). The marginal GDP impact of a +1 °C rise in annual temperature increases by more than 0.5 percentage points irrespective of the initial temperature level when all climate indicators are included as control variables (red line). Most of this effect is driven by including temperature variability (dotted line), which leads to higher impacts, particularly for colder regions. Therefore, the positive impacts of temperature variability in Fig. 3 obscure that, in fact, including this parameter leads to higher global damage since it disentangles potential benefits of reduced variability from the negative effects of temperature increases. As a result, including all climate indicators exacerbates GDP impacts across the globe (Fig. 4c ).
Exposure to tail risks
Aside from average impacts and the uncertainty around them, prudent risk management by policy-makers also requires information about tail risks. Therefore, we examine the percentage of the present global population living in countries that have a non-negligible chance (at least 5%) of suffering from damage exceeding different thresholds at different global warming levels (Fig. 5a ), both for our main approach (solid line) and the status quo approach (dotted line). Even at +1.5 °C, tail risks are substantial, with 99% of the global population living in countries with a non-negligible risk of suffering GDP damage of 5% or higher if all climate indicators are included. Notably, including variability and extremes increases tail risks considerably (Fig. 5b ). While under the status quo, 54% of the global population is projected to face damage of at least 15% with a likelihood of at least 5% at +3 ° C of warming, this increases to 68% of the population when variability and extremes are included. The share of the global population facing catastrophic impacts of 20% or higher with a 5% chance rises from 4% to 17%. However, disaggregating these results by individual climate indicators (Supplementary Fig. 6 ) highlights that the increase in global exposure to catastrophic climate change damage is primarily driven by higher temperature damage if underlying regression models control for more climate indicators than just annual precipitation and less by the direct impacts of these indicators on global GDP.
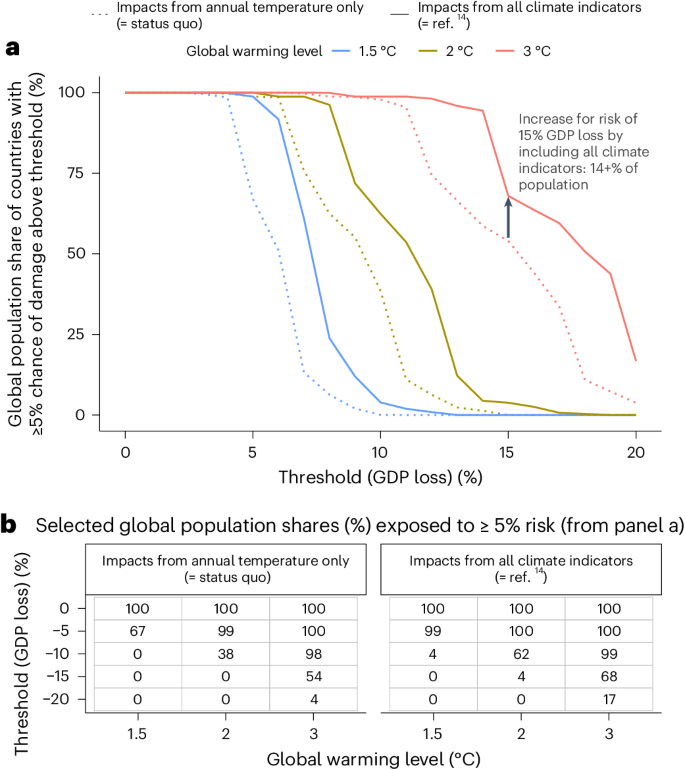
a , Share of the current global population living in countries whose projected GDP impacts for a given warming level (colour) exceeds the respective threshold ( x axis) for at least 5% of the GDP impact distribution, based on the status quo approach (dotted lines) and our main approach using all climate indicators (solid lines), respectively. The grey arrow and text annotation provide a reading example. b , Selected values from a .
Taken together, our results illustrate that projecting top-down damage of variability and extremes exacerbates global disparities further. Aggregate GDP loss projections, however, remain primarily driven by the impacts of mean temperature changes, which seem to cover economic losses due to heat waves at least partially 18 —an important finding for climate–economy modelling that complementary assessments of economic damage should corroborate to disentangle different impact channels better. As a result, overall uncertainty in GDP losses is dominated by the temperature dose–response function. However, substantial climatic uncertainties still limit the understanding of direct impacts by variability and extremes, particularly for low-income countries, which are expected to suffer the most but exhibit the largest uncertainties.
For scholars studying the economic effects of climate change, our results suggest a potential downward bias in temperature damage estimates by not disentangling the impacts of changes in temperature means and temperature variability. Future studies estimating temperature dose–response functions should test how including variability and extremes indicators linked to economic growth alters their findings. Notably, such biases could also be caused by other climate indicators not explicitly considered here and their direction and magnitude are likely to vary by location 32 . Furthermore, since the signal clarity is highest for extreme precipitation, this indicator seems most suitable to be included in climate–economy calculations, such as the social cost of carbon.
While our results rest on strong empirical foundations and a wide range of state-of-the-art climate models, there are several reasons why actual GDP impacts may exceed our projections. First, while the temperature dose–response function seems to include heat impacts at least partially, the dose–response functions used here do not explicitly cover important climate extremes, most notably droughts 33 . Second, to be conservative, we abstract from the possibility that climatic shifts do not only change GDP growth in a given year but alter a country’s long-run growth trajectory persistently. While such persistence in GDP losses remains empirically debated 1 , 2 , 14 , 21 , 34 , it would increase damage estimates substantially 26 , 27 . Third, aggregation across time and space is more likely to reduce signals in precipitation patterns because of their lower spatial and temporal correlation compared to annual mean temperature 13 , 14 . For these reasons, our results should be seen as an important first step, but they certainly do not exclude the possibility of larger GDP losses. Furthermore, econometric-based dose–response functions such as the ones used here have several limitations; for example, the risk of conflating weather impacts with climatic shifts or the extrapolation of impacts to warming levels that go far beyond historical observations, with the implicit assumption that adaptation remains at historically observed levels 35 , 36 . In addition, specification questions can further exacerbate socioeconomic uncertainties 21 and uniform dose–response functions for aggregate GDP can mask heterogeneities between countries, sectors and income segments 15 . Moreover, considering impacts in percentage of GDP implicitly assigns lower weights to poorer regions within countries that are disproportionately exposed to climate change risks 37 . Lastly, the distributions presented here might underestimate true climatic uncertainties for at least three reasons: (1) measurement imperfections in the reanalysis data underlying the dose–response functions, particularly for precipitation and lower-income regions 32 , 38 ; (2) using single runs for most CMIP6 models may underestimate tail risk events (Supplementary Appendix E ); and (3) not all CMIP6 models, despite representing the current frontier of global climatic projections, fully capture future changes in temperature variability and precipitation 24 , 25 , 39 , 40 , 41 .
Nevertheless, our study represents a key improvement in top-down damage projections, highlights the risks of omitting climate indicators beyond annual temperature, either as impact channels or control variables, and identifies the most promising fields for additional research. Building on our work, researchers could integrate further climate indicators, such as droughts, into a comprehensive assessment of climate change impacts. Aside from improvements in climate modelling, particularly for developing countries, this would also require more empirical studies to robustly identify the link between economic growth and different climatic extremes, ideally combined with an improved temporal or spatial resolution 17 . In addition, future research should assess the impact of controlling for these extremes on temperature dose–response functions and enhance the understanding of potential adaptation mechanisms and the persistence of GDP losses, ideally by consistently estimating and implementing persistence for each climate indicator under consideration.
Climatic data
Daily temperature and precipitation projections are taken from 33 CMIP6 models under two low-emission scenarios (Shared Socioeconomic Pathways SSP1-1.9 and SSP1-2.6) and one high-emission scenario (SSP3-7.0) to calculate bias-corrected, annual climate indicators for the 1850–2100 period. Owing to computational constraints, we use only one realization for most model–scenario pairs. However, to explore the role of intra-ensemble variation, we include 30 realizations of MPI-ESM1-2-LR and all 100 realizations of the CESM2-LE large ensemble under SSP3-7.0, which provides us with a total of 199 model-realization–scenario pairings (Supplementary Tables 1–3 ). Time series switch between historical scenarios and the respective Representative Concentration Pathway (RCP)–SSP pair in 2015 and are regridded onto a common 2.5° × 2.5° longitude–latitude grid using conservative remapping 43 .
Consistent with our source of empirically calibrated dose–response functions, which relies on ERA5 data 14 , we calculate annual average temperature T , annual total precipitation RA as well as four climate indicators using the equations listed below before downscaling and regridding the annual indicators from 2.5° to 0.25° (the grid resolution of ERA5). Notably, the indicators used here have been motivated and subjected to various robustness checks by previous studies 14 , 16 .
Day-to-day temperature variability:
where T x ,d,a,t is the temperature for grid cell x of day d of month a in year t and D a ∈ {28, 30, 31} is the number of days in the respective month a . \({\bar{T}}_{\rm{x,a,t}}\) denotes the mean temperature in month a of year t for the respective grid cell.
Extreme precipitation:
where R x,d,t is the precipitation of grid cell x on day d of year t , I () is an indicator function and R x,99p9,base denotes the 99.9th percentile of daily precipitation in grid cell x over a historical baseline period.
Number of wet days with precipitation exceeding 1 mm d −1 :
Grid-cell-level annual climate indicators are then aggregated to the subnational region level (ADM1) using the geospatial data from the Database of Global Administrative Areas (GADM, v.3.6) and area weighting.
Monthly precipitation deviation, which we calculate only at the ADM1 level and not at the grid-cell level, consistent with ref. 14 :
where R i, a ,t denotes precipitation totals in month a of year t for a given ADM1-level region i . Variables denoted by a bar represent averages across the baseline period, either for the full year or for a specific month, while σ i,a,base denotes the month-specific standard deviation across the baseline period for region i . As for all other climate indicators, region-level monthly precipitation R i,a,t is derived from grid-cell-level values based on area weighting.
For the baseline-dependent climate indicators \(\hat{\rm{RD}}\) and RM, our source of dose–response functions 14 uses 1979–2019 as the historical baseline period, during which global warming relative to pre-industrial levels over 1850–1900 averaged +0.84 °C according to Berkeley Earth data (the best estimate for the observed warming and, in a previous version, used in the IPCC AR6; ref. 8 ). However, the 1979–2019 time period can differ climatically across CMIP6 models, which warm at very different paces 44 . To maintain consistency and ensure that all climate indicators are based on the same baseline in terms of global warming, we, therefore, identify the corresponding 41-year window during which global warming relative to pre-industrial levels over 1850–1900 averages +0.84 °C for each climate model-realization and scenario. Then, we use the +0.84 °C window to calculate all values with a ‘base’ subscript in equations ( 2 ) and ( 4 ). Warming-level windows for each model-realization–scenario pairing are calculated following the approach by ref. 10 and shown in Supplementary Tables 1–3 . However, percentile-based indicators, such as our extreme precipitation measure, can exhibit artificial jumps at the end of the baseline period, causing potential frequency increases and discontinuities outside this period 10 , 45 , 46 . To correct this, we use the bootstrap resampling procedure developed by ref. 46 , estimating the percentile applied to each year in the baseline period on the basis of the remaining 40 years in the baseline period and then using the average across these percentiles for all years outside the baseline period. Mathematical expressions for the bootstrap resampling procedure and the calculation of global warming levels, as well as more information on the suitability of CMIP6 and ERA5 data to assess variability and extremes, are provided in Supplementary Appendix A .
Bias correction
We bias-correct annual climate indicators using the change factor method 47 , which adds model-projected changes compared to a reference period to the corresponding reference period average of an observational dataset. For any climate indicator C out of the six indicators considered here, bias-corrected values are obtained as follows:
where C x,t,m,r,s represents the raw climate indicator output of climate model m ’s realization r under scenario s in year t for grid cell x . \({\bar{C}}{\,\!}_{\rm{x},{\rm{ref}}}^{\rm{ERA5}}\) and \({\bar{C}}_{\rm{x},{\rm{ref}},m,r,s}\) represent the reference period average in ERA5 and for the climate model run, respectively. As a reference period, we use 1950–1990, during which global warming averaged +0.38 °C according to Berkeley Earth. Therefore, \({\bar{C}}_{\rm{x},{\rm{ref}},\rm{m,r,s}}\) is calculated for the 41-year global warming-level window corresponding to +0.38 °C (for the specific global warming-level windows, see Supplementary Tables 1–3 ). We bias-correct each annual indicator separately and, for the monthly precipitation deviation, apply the change factor method to the underlying monthly precipitation amounts. As a reference period, we use 1950–1990 because of its low influence of anthropogenic forcing and, to avoid impossible values, further impose zero lower bounds for all non-negative climate indicators and an upper bound of 365 for the number of wet days. In addition, the bias-corrected monthly precipitation deviation in some selected cases yields values that are one or two orders of magnitude above the maximum in our raw CMIP6 data. To address these outliers, we cap bias-corrected monthly precipitation deviation on the basis of the highest values observed for the raw CMIP6 data for up to +3 °C of global warming (~11.6; Supplementary Table 4 ), which affects only observations beyond the 99.993th percentile of our distribution.
Bias-correcting annual climate indicators ensures the highest consistency for each indicator with the ERA5 data used to estimate dose–response functions by ref. 14 (Supplementary Figs. 1–3 ). However, it can lead to inconsistencies between the different climate indicators derived from the same daily temperature or precipitation and, as outlined above, to outlier values in a few cases. As a robustness check, we apply the change factor method to the underlying daily temperature and precipitation values of an example model run instead, which increases the computational burden of bias correction considerably but leaves our conclusions unchanged (Supplementary Fig. 4 ).
GDP impacts
Dose–response functions for subnational economic growth are taken from ref. 14 , which jointly estimates the impact of all six indicators on income per capita growth. The resulting dose–response functions for each climate indicator are shown in Supplementary Fig. 7 and the underlying regression is reproduced in Supplementary Table 7 , column 5. Importantly, this regression model has been subjected to comprehensive robustness checks, such as using alternative datasets and variable definitions, controlling for region-specific time trends or assessing seasonal heterogeneities 14 . Mathematically, the specification of the regression can be summarized as
while the full model is written out in Supplementary equation ( 5 ). Here, g i,t denotes the economic growth of ADM1-level region i in year t , measured as the first difference of the log-transformed gross regional product per capita 48 . α i , δ t and ϵ i,t denote fixed effects and the error term and h C is the estimated dose–response function specific to climate indicator C i,t where \(C\in \{T,{\rm{RA}},\widetilde{T},\hat{{\rm{RD}}},{\rm{RD}},{\rm{RM}}\}\) . For instance, for annual precipitation RA, the relationship with economic growth is estimated as a quadratic relationship such that
where \({\beta }_{1}^{{\rm{RA}}}\) and \({\beta }_{2}^{{\rm{RA}}}\) are the respective regression coefficients.
To calculate the impacts of climate change, we compare annual economic impacts against the average impact during the historical baseline period for the same model–realization–scenario pairing, such that our impacts represent changes from a hypothetical scenario in which climate remains constant, following previous studies 2 , 15 . As a baseline period for GDP impacts, we again use the +0.84 ° C global warming-level window for a given realization r of climate model m and RCP–SSP pair s for consistency with the calculation of our climate indicators. Therefore, annual impacts, in percentage of GDP, of all climate indicators combined due to shifts relative to the baseline period are calculated as follows
where K is the 41-year model–realization–scenario-specific baseline period corresponding to +0.84 °C of global warming. Note that we exponentiate and subtract one to convert logarithmic changes to percentage of GDP, but impacts of different indicators and years are added and averaged in log scale. Individual GDP impacts of each climate indicator are obtained by using only the respective individual dose–response function in equation ( 8 ), instead of the sum across dose–response functions ∑ C h C ( C i,k ). Similarly, the combined GDP impacts of variability and extremes are calculated by summing only the dose–response functions for \(\widetilde{T}\) , \(\hat{{\rm{RD}}}\) , RD and RM in equation ( 8 ). More detailed mathematical expressions for all steps in equation ( 8 ) are provided in Supplementary Appendix C .
Importantly, the model specification by ref. 14 features annual temperature in first-differences compared to previous years and not in absolute levels:
To translate these regression coefficients into impact projections, we calculate cumulative impacts following ref. 4 , such that the dose–response function for annual temperature used in equation ( 8 ) reads as follows:
where k 0 denotes the first year in the baseline period K . As we discuss in Supplementary Appendix C , this procedure implicitly includes impacts of inter-annual temperature variability that persist over time.
For extreme precipitation \(\hat{{\rm{RD}}}\) , the dose–response function estimated by ref. 14 interacts extreme rainfall with the annual mean temperature T because the marginal impact of extreme precipitation is lower in warmer climates. Projecting this out under climate change, however, would make the strong assumption that global warming increases the resilience of countries to extreme precipitation worldwide. Because there is no evidence supporting such a positive feedback of warming and because the heterogeneity of extreme rainfall effects in ref. 14 is equally well-explained by the latitude of a country (see R2 and Adjusted R2 in Supplementary Table 4 of ref. 14 ), which is time-constant, we hold temperature in the interaction constant at the average level during the baseline period such that
When projecting damage of climate change, a core methodological choice is whether to assume that impacts affect GDP levels, such that the economy bounces back in the following year or whether to assume that a part of the damage persists and alters the long-run growth trajectory. Assuming persistence has a substantial impact on damage projections and the associated uncertainty 21 , 26 , 27 . Recent empirical analyses differ in methods and outcomes, with no consensus yet 1 , 2 , 12 , 21 , 34 . To be conservative, here we assume no persistence in implementing δ i,t , aside from any persistence implicit in the methodology by ref. 4 , and provide further mathematical expressions and discussions of damage persistence in Supplementary Appendix C . Furthermore, by holding historical dose–response functions and baseline periods for climate indicators constant, our approach rests on the common implicit assumption that future adaptation outcomes mirror historical ones 2 , 4 , 35 , in line with the mixed evidence on macro-economic adaptation so far 2 , 20 , 21 . In addition, we follow ref. 27 in equating relative GDP per capita impacts with relative GDP impacts (that is, assuming that any decrease in GDP per capita is caused by a climate change-induced reduction in economic output and not by an increase in population).
Spatial aggregation of GDP impacts
We aggregate the GDP impacts calculated via equation ( 8 ) from the subnational detail (ADM1) to the country level (ADM0) using GDP weighting. For GDP weights, we use 2010 GDP data downscaled to a 0.5° grid by ref. 49 . To deal with 105 outlier grid cells with raw GDP data exceeding US$10 20 , we apply a ceiling at $10 10 , which is the next highest grid-cell GDP value in the dataset. Note that we hold this intracountry income distribution constant across all years and SSPs. This simplification stems from the SSPs not directly informing spatial intracountry GDP per capita distributions and also prevents our results from being driven by changes in the weighting scheme over time rather than climatic changes, which is standard practice in the literature 2 . To calculate GDP impacts at the global level, we weigh each country i with its share in global GDP in year t as per the respective SSP. Since the SSP database does not provide GDP growth trajectories for a few sovereign countries, namely Andorra, Liechtenstein, Kosovo, Nauru, North Korea, San Marino, South Sudan and Tuvalu, these economies are not represented in our damage projections for the global economy.
GDP impact distribution
To capture dose–response function uncertainty, we draw 1,000 estimates for the dose–response function parameters \({\beta }_{1}^{{\rm{RA}}},{\beta }_{2}^{{\rm{RA}}},{\beta }_{1}^{\rm{T}},\ldots\) jointly from the multivariate Gaussian distribution estimated by ref. 14 (main specification, standard errors clustered at the country level). Applying each Monte Carlo draw to each of the 199 model–realization–scenario pairings provides us with 199,000 different impact projection pathways for each territory. For each model-realization–scenario pairing, we then identify the 20-year window corresponding to a global warming level of +1 °C, +1.5 °C, +2 °C, +3 °C and +4 °C, respectively, following the approach by ref. 10 (for the specific global warming-level windows, see Supplementary Tables 1–3 . This provides us with a conditional distribution of GDP impacts for a given territory and warming level. Aside from reducing the importance of individual RCP–SSP scenarios, conditioning results on global warming levels also reduces the need to omit or down-weight ‘hot models’ in CMIP6, which project too much warming 44 . Since not all models reach all warming levels and to prevent the two large ensembles from dominating our results, we weight models inversely such that each CMIP6 model has the same sampling probability for each warming level following ref. 18 . All summary statistics of the distribution (means, percentiles, variances and so on) are calculated using these CMIP6 model weights.
Variance decomposition
To decompose the observed variance in our conditional global GDP impact distribution for a given warming level, we adapt the approach by ref. 50 based on the partitioning method by ref. 51 . First, we carry out projections using point estimates for all dose–response function parameters (abstracting from dose–response function uncertainty) and calculate internal variability as the average across CMIP6 models of each model’s variance of global GDP impacts in a given global warming-level window. For CMIP6 models with only a single run in our analysis, this within-model variance stems from climatic differences between different scenario–years, whereas for the two large ensembles, it also includes differences between ensemble members. Correspondingly, we calculate climate model uncertainty as the variance between the mean global GDP impact of CMIP6 models for a given global warming level. Lastly, we calculate dose–response function uncertainty as the variance across dose–response function Monte Carlo draws of the mean GDP impact for each Monte Carlo draw (that is, an average across all CMIP6 models and scenario–years in the respective global warming-level window). Mathematical expressions for each variance component are provided in Supplementary Appendix D . Notably, this approach rests on the commonly made assumption that variance drivers are orthogonal, thus abstracting from interaction terms 52 . As a robustness check, we use an alternative approach by ref. 3 (Supplementary Appendix D ), which does not affect our conclusions.
Reporting summary
Further information on research design is available in the Nature Portfolio Reporting Summary linked to this article.
Data availability
CMIP6 temperature and precipitation indicators are available on the ETH Zurich CMIP6 repository 53 . CESM2 large ensemble outputs are available at https://www.earthsystemgrid.org/dataset/ucar.cgd.cesm2le.output.html . Tx5d and PDSI values from ref. 18 (used in Supplementary Appendix F ) are available at https://github.com/ccallahan45/CallahanMankin_ExtremeHeatEconomics_2022 (ref. 54 ). The historical climate data and the economic growth data to estimate the dose–response functions from ref. 14 are available from ref. 55 . ERA5 reanalysis data are available at https://www.ecmwf.int/en/forecasts/datasets/reanalysis-datasets/era5 . Source data are provided with this paper. All additional data are publicly available from ref. 56 .
Code availability
All scripts used to conduct the analysis and create the figures are publicly available from ref. 56 .
Dell, M., Jones, B. F. & Olken, B. A. Temperature shocks and economic growth: evidence from the last half century. Am. Econ. J. Macroecon. 4 , 66–95 (2012).
Article Google Scholar
Burke, M., Hsiang, S. M. & Miguel, E. Global non-linear effect of temperature on economic production. Nature 527 , 235–239 (2015).
Article CAS Google Scholar
Hsiang, S. et al. Estimating economic damage from climate change in the United States. Science 356 , 1362–1369 (2017).
Kalkuhl, M. & Wenz, L. The impact of climate conditions on economic production: evidence from a global panel of regions. J. Environ. Econ. Manag. 103 , 102360 (2020).
Seneviratne, S. I. et al. in Climate Change 2021: The Physical Science Basis (eds Masson-Delmotte, V. et al.) 1513–1766 (Cambridge Univ. Press, 2021).
Newman, R. & Noy, I. The global costs of extreme weather that are attributable to climate change. Nat. Commun. 14 , 6103 (2023).
Pendergrass, A. G., Knutti, R., Lehner, F., Deser, C. & Sanderson, B. M. Precipitation variability increases in a warmer climate. Sci. Rep. 7 , 17966 (2017).
Masson-Delmotte, V. et al. (eds) Climate Change 2021: The Physical Science Basis (Cambridge Univ. Press, 2021).
Bathiany, S., Dakos, V., Scheffer, M. & Lenton, T. M. Climate models predict increasing temperature variability in poor countries. Sci. Adv. 4 , eaar5809 (2018).
Batibeniz, F., Hauser, M. & Seneviratne, S. I. Countries most exposed to individual and concurrent extremes and near-permanent extreme conditions at different global warming levels. Earth Syst. Dynam. 14 , 485–505 (2023).
Burke, M. et al. Opportunities for advances in climate change economics. Science 352 , 292–293 (2016).
Piontek, F. et al. Integrated perspective on translating biophysical to economic impacts of climate change. Nat. Clim. Change 11 , 563–572 (2021).
Damania, R., Desbureaux, S. & Zaveri, E. Does rainfall matter for economic growth? Evidence from global sub-national data (1990–2014). J. Environ. Econ. Manag. 102 , 102335 (2020).
Kotz, M., Levermann, A. & Wenz, L. The effect of rainfall changes on economic production. Nature 601 , 223–227 (2022).
Palagi, E., Coronese, M., Lamperti, F. & Roventini, A. Climate change and the nonlinear impact of precipitation anomalies on income inequality. Proc. Natl Acad. Sci. USA 119 , e2203595119 (2022).
Kotz, M., Wenz, L., Stechemesser, A., Kalkuhl, M. & Levermann, A. Day-to-day temperature variability reduces economic growth. Nat. Clim. Change 11 , 319–325 (2021).
Linsenmeier, M. Temperature variability and long-run economic development. J. Environ. Econ. Manag. 121 , 102840 (2023).
Callahan, C. W. & Mankin, J. S. Globally unequal effect of extreme heat on economic growth. Sci. Adv. 8 , eadd3726 (2022).
Holtermann, L. Precipitation anomalies, economic production and the role of ‘first-nature’ and ‘second-nature’ geographies: a disaggregated analysis in high-income countries. Glob. Environ. Change 65 , 102167 (2020).
Kahn, M. E. et al. Long-term macroeconomic effects of climate change: a cross-country analysis. Energy Econ. 104 , 105624 (2021).
Newell, R. G., Prest, B. C. & Sexton, S. E. The GDP–temperature relationship: implications for climate change damages. J. Environ. Econ. Manag. 108 , 102445 (2021).
Orlowsky, B. & Seneviratne, S. I. Elusive drought: uncertainty in observed trends and short- and long-term CMIP5 projections. Hydrol. Earth Syst. Sci. 17 , 1765–1781 (2013).
Pfahl, S., O’Gorman, P. A. & Fischer, E. M. Understanding the regional pattern of projected future changes in extreme precipitation. Nat. Clim. Change 7 , 423–427 (2017).
Kotz, M., Wenz, L. & Levermann, A. Footprint of greenhouse forcing in daily temperature variability. Proc. Natl Acad. Sci. USA 118 , e2103294118 (2021).
Kotz, M., Lange, S., Wenz, L. & Levermann, A. Constraining the pattern and magnitude of projected extreme precipitation change in a multimodel ensemble. J. Clim. 37 , 97–111 (2024).
Moore, F. C. & Diaz, D. B. Temperature impacts on economic growth warrant stringent mitigation policy. Nat. Clim. Change 5 , 127–131 (2015).
Kikstra, J. S. et al. The social cost of carbon dioxide under climate–economy feedbacks and temperature variability. Environ. Res. Lett. 16 , 094037 (2021).
World Development Indicators: GDP (constant 2015 US$) (World Bank, accessed 3 May 2023); https://databank.worldbank.org/source/world-development-indicators
Pakistan Floods 2022: Post-Disaster Needs Assessment (Government of Pakistan, 2022); https://www.undp.org/pakistan/publications/pakistan-floods-2022-post-disaster-needs-assessment-pdna
Screen, J. A. Arctic amplification decreases temperature variance in northern mid- to high-latitudes. Nat. Clim. Change 4 , 577–582 (2014).
de Vries, I. E., Sippel, S., Pendergrass, A. G. & Knutti, R. Robust global detection of forced changes in mean and extreme precipitation despite observational disagreement on the magnitude of change. Earth Syst. Dynam. 14 , 81–100 (2023).
Auffhammer, M., Hsiang, S. M., Schlenker, W. & Sobel, A. Using weather data and climate model output in economic analyses of climate change. Rev. Environ. Econ. Policy 7 , 181–198 (2013).
Naumann, G., Cammalleri, C., Mentaschi, L. & Feyen, L. Increased economic drought impacts in Europe with anthropogenic warming. Nat. Clim. Change 11 , 485–491 (2021).
Bastien-Olvera, B. A., Granella, F. & Moore, F. C. Persistent effect of temperature on GDP identified from lower frequency temperature variability. Environ. Res. Lett. 17 , 084038 (2022).
Dell, M., Jones, B. F. & Olken, B. A. What do we learn from the weather? The new climate–economy literature. J. Econ. Lit. 52 , 740–798 (2014).
Auffhammer, M. Quantifying economic damages from climate change. J. Econ. Perspect. 32 , 33–52 (2018).
Byers, E. et al. Global exposure and vulnerability to multi-sector development and climate change hotspots. Environ. Res. Lett. 13 , 055012 (2018).
Dinku, T. in Extreme Hydrology and Climate Variability (eds Melesse, A. M. et al.) 71–80 (Elsevier, 2019).
Kornhuber, K. et al. Risks of synchronized low yields are underestimated in climate and crop model projections. Nat. Commun. 14 , 3528 (2023).
Schwarzwald, K., Goddard, L., Seager, R., Ting, M. & Marvel, K. Understanding CMIP6 biases in the representation of the Greater Horn of Africa long and short rains. Clim. Dynam. 61 , 1229–1255 (2023).
Ayugi, B. et al. Comparison of CMIP6 and CMIP5 models in simulating mean and extreme precipitation over East Africa. Int. J. Climatol. 41 , 6474–6496 (2021).
South, A. rnaturalearthdata: World vector map data from Natural Earth used in ’rnaturalearth’. R package version 0.1.0 https://github.com/ropenscilabs/rnaturalearthdata (2017).
Jones, P. First- and second-order conservative remapping schemes for grids in spherical coordinates. Mon. Weather Rev. 127 , 2204–2210 (1999).
Hausfather, Z., Marvel, K., Schmidt, G. A., Nielsen-Gammon, J. W. & Zelinka, M. Climate simulations: recognize the ‘hot model’ problem. Nature 605 , 26–29 (2022).
Zhang, X. et al. Indices for monitoring changes in extremes based on daily temperature and precipitation data. WIREs Clim. Change 2 , 851–870 (2011).
Zhang, X., Hegerl, G., Zwiers, F. W. & Kenyon, J. Avoiding inhomogeneity in percentile-based indices of temperature extremes. J. Clim. 18 , 1641–1651 (2005).
Tabor, K. & Williams, J. W. Globally downscaled climate projections for assessing the conservation impacts of climate change. Ecol. Appl. 20 , 554–565 (2010).
Wenz, L., Carr, R. D., Kögel, N., Kotz, M. & Kalkuhl, M. DOSE—global data set of reported sub-national economic output. Sci. Data 10 , 425 (2023).
Murakami, D., Yoshida, T. & Yamagata, Y. Gridded GDP projections compatible with the five SSPs (shared socioeconomic pathways). Front. Built Environ. 7 , 760306 (2021).
Schwarzwald, K. & Lenssen, N. The importance of internal climate variability in climate impact projections. Proc. Natl Acad. Sci. USA 119 , e2208095119 (2022).
Hawkins, E. & Sutton, R. The potential to narrow uncertainty in regional climate predictions. Bull. Am. Meteorol. Soc. 90 , 1095–1108 (2009).
Lehner, F. et al. Partitioning climate projection uncertainty with multiple large ensembles and CMIP5/6. Earth Syst. Dynam. 11 , 491–508 (2020).
Brunner, L., Hauser, M., Lorenz, R. & Beyerle, U. The ETH Zurich CMIP6 next generation archive: technical documentation. Zenodo https://zenodo.org/doi/10.5281/zenodo.3734127 (2020).
Callahan, C. W. & Mankin, J. S. Globally unequal effect of extreme heat on economic growth. GitHub https://github.com/ccallahan45/CallahanMankin_ExtremeHeatEconomics_2022 (2022).
Kotz, M., Levermann, A., & Wenz, L. Data and code for the publication ‘The effect of rainfall changes on economic production’. Zenodo https://zenodo.org/doi/10.5281/zenodo.5657456 (2021).
Waidelich, P., Batibeniz, F., Rising, J., Kikstra, J. S., & Seneviratne, S. Scripts and data for ‘Climate damage projections beyond annual temperature’. Zenodo https://zenodo.org/doi/10.5281/zenodo.10465253 (2024).
Download references
Acknowledgements
We thank M. Hauser, J. Krug and I. de Vries, as well as participants at the NAVIGATE-ENGAGE Summer School 2023 and the 16th IAMC Annual Meeting 2023 for valuable comments. This work was supported by the European Union’s Horizon 2020 research and innovation programme, European Research Council under grant agreement no. 948220, project no. GREENFIN (P.W.) and by the Natural Environment Research Council under grant agreement no. NE/S007415/1 (J.S.K.).
Open access funding provided by Swiss Federal Institute of Technology Zurich.
Author information
Authors and affiliations.
Climate Finance and Policy Group, ETH Zurich, Zurich, Switzerland
Paul Waidelich
Institute for Atmospheric and Climate Science, ETH Zurich, Zurich, Switzerland
Fulden Batibeniz & Sonia I. Seneviratne
Oeschger Center for Climate Change Research, University of Bern, Bern, Switzerland
Fulden Batibeniz
Climate and Environmental Physics, Physics Institute, University of Bern, Bern, Switzerland
School of Marine Science & Policy, University of Delaware, Newark, DE, US
James Rising
Centre for Environmental Policy, Imperial College London, London, UK
Jarmo S. Kikstra
Grantham Institute for Climate Change and the Environment, Imperial College London, London, UK
Energy, Climate and Environment (ECE) Program, International Institute for Applied Systems Analysis, Laxenburg, Austria
You can also search for this author in PubMed Google Scholar
Contributions
All authors conceived the study. F.B. prepared the underlying climate data and conducted the bias correction. P.W. and J.R. performed the data aggregation and developed the projection methodology. P.W. carried out the impact projections, analysed and visualized the results and wrote the manuscript. All authors reviewed the manuscript.
Corresponding author
Correspondence to Paul Waidelich .
Ethics declarations
Competing interests.
The authors declare no competing interests.
Peer review
Peer review information.
Nature Climate Change thanks Marshall Burke and the other, anonymous, reviewer(s) for their contribution to the peer review of this work.
Additional information
Publisher’s note Springer Nature remains neutral with regard to jurisdictional claims in published maps and institutional affiliations.
Supplementary information
Supplementary information.
Supplementary Discussion (Appendices A–F), Figs. 1–19 and Tables 1–10.
Reporting Summary
Source data fig. 1.
Statistical source data.
Source Data Fig. 2
Source data fig. 3, source data fig. 4, source data fig. 5, rights and permissions.
Open Access This article is licensed under a Creative Commons Attribution 4.0 International License, which permits use, sharing, adaptation, distribution and reproduction in any medium or format, as long as you give appropriate credit to the original author(s) and the source, provide a link to the Creative Commons licence, and indicate if changes were made. The images or other third party material in this article are included in the article’s Creative Commons licence, unless indicated otherwise in a credit line to the material. If material is not included in the article’s Creative Commons licence and your intended use is not permitted by statutory regulation or exceeds the permitted use, you will need to obtain permission directly from the copyright holder. To view a copy of this licence, visit http://creativecommons.org/licenses/by/4.0/ .
Reprints and permissions
About this article
Cite this article.
Waidelich, P., Batibeniz, F., Rising, J. et al. Climate damage projections beyond annual temperature. Nat. Clim. Chang. (2024). https://doi.org/10.1038/s41558-024-01990-8
Download citation
Received : 24 July 2023
Accepted : 20 March 2024
Published : 17 April 2024
DOI : https://doi.org/10.1038/s41558-024-01990-8
Share this article
Anyone you share the following link with will be able to read this content:
Sorry, a shareable link is not currently available for this article.
Provided by the Springer Nature SharedIt content-sharing initiative
Quick links
- Explore articles by subject
- Guide to authors
- Editorial policies
Sign up for the Nature Briefing newsletter — what matters in science, free to your inbox daily.

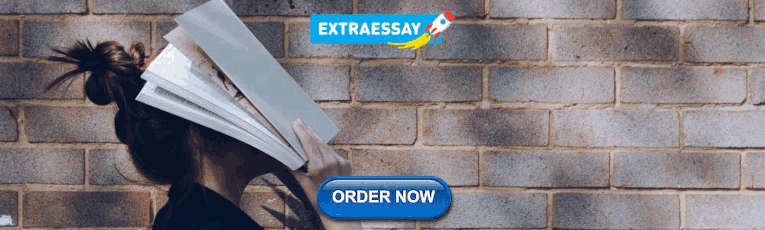
IMAGES
VIDEO
COMMENTS
the impacts of climate change and natural disasters. Overcrowded living conditions, inaccessibility to safe infrastructure and poor health conditions make the urban poor highly vulnerable to climate change impacts (Baker, 2011c). Climate change can change the pattern of diseases, mortality, human settlements, food, water, and sanitation.
ubiquitous. Therefore, this dissertation deals with the comprehensive topic of climate change and air pollution and their effects on public health. The first chapter examines the effect of temperature on mortality in 148 cities in the U.S. from 1973 through 2006. We focused on the timing of exposure to unseasonal temperature and
Good Examples. Focused Approach: "This thesis will analyze the impact of climate change on the intensity and frequency of hurricanes, using data from the last three decades.". Lack of Focus: "Climate change affects weather patterns.". The good statement is specific, indicating a focus on hurricanes and providing a time frame.
Thesis Advisor: Adam T. Thomas, Ph.D. ABSTRACT Increased emissions of carbon dioxide and greenhouse gases (GHG) have exacerbated the effects of climate change and have led to intensified weather events and a steady rise in the average global temperature. Countries sought to outline an aggressive agenda for combatting
Climate Change Adaptation Preparedness in Developing Countries: A Study of 21 Countries and Knowledge, Attitudes, and Practices Studies in Akwa Ibom and Lagos States in Nigeria ... This thesis presents two research studies on climate change adaptation preparedness in developing countries. In the first study, the policies and programs of 21 ...
A major challenge in understanding and implementing nature-based approaches to climate change adaptation and mitigation is that of scalability. Climate change is a global problem, requiring multi-jurisdictional and multinational governance, yet many of the examples of NbS concern proof of concept studies over relatively small spatial scales.
Community-based carbon reduction strategies are one example of action towards achieving sustainability and addressing climate change. Previous research into ... and attitudes towards mitigating climate change. My undergraduate dissertation (in 2008/09) scratched the surface of my curiosity with public perceptions and
And yet, action on climate change has been characterized by lack of progress and break downs in communication. It is widely assumed that the global response to climate change has so far been inadequate. Alarmed by this lack of progress, the thesis aims to explore exactly why we should consider current global climate change action as
institutions for climate change over time. Specifically, the thesis aims to identify which norms have gained centrality, how this has changed over time and which are the implications for climate governance. To do so the thesis employs an analytical framework which delineates different conceptualisations of justice, including cosmopolitanism ...
2013. Communication of environmental and climate change issues on social network sites, the scientists perspective: case study of digital practices of researchers engaged in environmental and climate change issues in the Environmental School, University of East Anglia. Marunye, Joalene. 2009.
This dissertation includes three self-contained and interrelated papers on climate change mitigation, building energy efficiency, and urban form. Paper 1: Urban form and household electricity consumption: a multilevel study While urban form affects building energy consumption, the pathways, direction and magnitude of the effect are disputed in ...
In this thesis, climate change activism is defined as taking concrete actions to advocate for a change in human behavior so that everyone can have a safe climate. These actions are mainly aimed toward directly reducing fossil fuel use and other . 2 . sources of atmospheric carbon, but also may be aimed at larger environmental and ...
This paper will discuss urban sustainability in the context of. climate change and address the following research objectives: (1) to examine how. climate change is affecting urban areas; (2) to assess how cities can enhance urban. sustainability by addressing climate change; (3) to discuss resources available for city.
Climate change is one of the defining issues of our time. In the past, it has been defined mainly in environmental and economic terms. For example, climate change was described as an 1 Jelmer Mommers, Thanks to this Landmark ourt Ruling, limate hange is Now Inseparable from Human Rights, _ The Correspondent, December 20, 2019, 1-2.
Whether it's increasing air pollution from prolonged wildfire seasons or the increasing number of heat events, climate change will impact everyone eventually. The overall goal of this study was to build on the literature elucidating the impacts of coexposures on human health that climate change is driving.
Climate change also presents challenges to decision makers who need new kinds of climate and water information, and will need the scientific research community to help provide improved means of knowledge transfer. ... This dissertation quantified the basin-wide distribution of snowpack across multiple decades in present and in projected climate ...
their main interests. For example, if you are interested in human-caused climate change the history becomes a "process by connecting the dots among more than a thousand of the most 1 C. E. P. Brooks, Climate through the Ages - A Study of the Climatic Factors and Their Variations, (London, UK: Ernest Benn Limited, 1926), 430-2.
Step 1: Choose your topic. Take a look at the sustainability research topics list to see if they interest you. If you require data on energy, travel, procurement, UCL's sustainability engagement programmes or water please email Sustainable UCL to request this. If you have alternative research topic ideas for a sustainable living lab ...
of climate change and to communicate this technical knowledge to policy makers and the general public. This dissertation contributes to both of these goals. Chapter 1 of the dissertation uses housing market data to estimate the welfare costs of shoreline loss along coastal beaches in Florida. In this chapter, I develop a forward-
Across UBC, faculty and students contribute to research on climate change. See below for recent theses on a few select topics, and search cIRcle, UBC's open access repository, for publications, theses/dissertation, and presentations to find more. RSS feed searching the UBC Theses and Dissertations Collection for: "Global warming" OR "Climate ...
climate change, adaptation and mitigation. Adaptation refers to adjusting to the expected level of climate change in order to reduce harm from inevitable consequences. Mitigation refers to reducing emissions to limit future climate change, for example, through renewable energy adoption, transition to a low carbon economy, and applying
Estimates of global economic damage from climate change assess the effect of annual temperature changes. However, the roles of precipitation, temperature variability and extreme events are not yet ...
dissertation on climate change and sustainable development: are local and international laws sufficient enough to help curb/reduce climate change moi university. Skip to document. ... Terrorism-Sample - Copy; Finance Anlysis for MBH Corp - Copy; Finance Anlysis for Morgan Sindall Group; Mba chuong 3 student 7437;
As climate change happens, people are devising new ways of survival. Humans adapt to their surroundings by modifying the natural environment to fulfill their requirements. They develop artificial ...