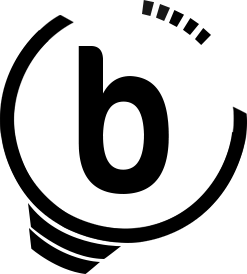
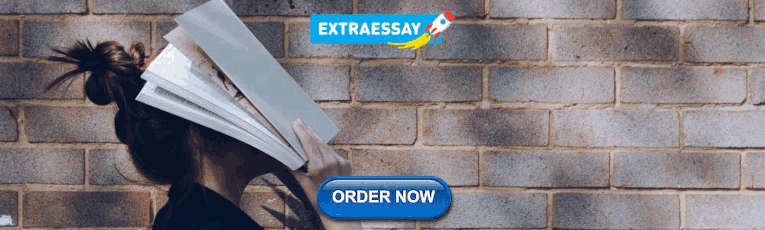
Sample Personal Statement Finance (MIT Sloan)
by Talha Omer, MBA, M.Eng., Harvard & Cornell Grad
In personal statement samples by field | personal statements samples by university.
Here is the personal statement of an applicant who got admitted to MIT Sloan’s Masters in Finance program . For personal statement, Sloan poses several questions to applicants, which the admissions committee expects to be answered in an essay form. MIT provides these personal statement prompts to encourage students to self-reflect and then to share their insights with the program.
The following essays are an example of a compelling story and reflect the original voice and personality of the applicant. Get inspiration from them and try to incorporate their strengths into your own personal statement.
In this Article
Personal Statement Prompt 1
Personal statement prompt 2, personal statement prompt 3.
Please discuss past academic and professional experiences and accomplishments that will help you succeed in the Master of Finance program. Include achievements in finance, math, statistics, and computer sciences, as applicable.
As an ardent finance student, I have always sought opportunities to develop a solid grounding in the subject.
During my undergraduate, I successfully co-founded a philanthropic long/short Impact Investment Hedge Fund, “Australian Students Asset Management” (ASAM). The fund secured firms like Goldman Sachs to provide pro-bono services and mentorship. In addition, I developed a proprietary ESG algorithm to identify investment opportunities per our social development mandate. As a result, our investments have consistently outperformed our benchmark. Furthermore, I attended two courses at LSE on Alternative Investments and Financial Risk with “A” grades. This involved in-depth exploration of topics like Monte Carlo, Bootstrapping, Financial Modelling and Stratification.
As an Associate at PwC, I created PwC’s first fully automated valuation process. This consisted of using industry-specific valuation templates using Python and VBA for data scraping. This solution saw significant savings for the firm and is now used by PwC throughout the world.
As an analyst with Barclays Capital, a Global Quantitative Hedge Fund, I gained exposure to arbitrage trading strategies by implementing statistical principles like mean reversion, volatility trends and co-integration in Python. This helped me develop successful trading algorithms yielding a highly desirable annualized return of 33%.
Describe your short-term and long-term professional goals. How will our MFin degree help you achieve these goals?
My short-term goal is to return to Australia and establish my own quantitative “for-profit” hedge fund. I will utilize my previous experience co-founding the philanthropic hedge fund, ASAM, to that effect. My long-term goal is to expand this hedge fund’s operations overseas and invest in the North American markets, as they present significant growth opportunities.
At MIT, I am eager to research the implications of current trends in institutional capital flows. This detailed exploration will enhance my understanding of the potential risks of passive investing and provide an edge in building trust with future investors.
As an Analyst at Barclays Capital, I developed event-driven and relative-value trading algorithms using Python. With the MFin, I will create an advanced, intuitive approach to data science problems, enabling the manipulation of alternative data sets to automate trading decisions and executions. The Advanced Analytics and Data Science courses and Analytics Certificate are especially exciting as they will allow me to understand shallow models and train deep neural networks in an economic context. I also hope to improve my technical skills around asset pricing and general dimension reduction techniques and ensemble methods which are critical to forming accurately priced derivatives.
Please share personal qualities that will enable you to contribute to the advancement of our mission.
THE MISSION
The mission of the MIT Sloan School of Management is to develop principled, innovative leaders who improve the world and generate ideas that advance management practice.
I believe success as a Leader requires one to have a passion for taking the initiative, thinking outside the box and persevering in adversity.
These traits have always been integral to my personal and professional pursuits, including my recent experience as a special education teacher in San Pedro, a rural village in Costa Rica. I was required to develop creative methods to overcome the language barrier and constructed visual aids from cardboard pieces to make block numbers. In addition, the school had a dire need for wheelchairs, so I devised a cost-effective design to repurpose old bicycles into wheelchairs that we implemented as a team. My commitment to improving the world through innovation will allow me to contribute actively to MIT’s mission.
Furthermore, my extensive involvement in boxing has enabled me to develop perseverance and increased focus in facing challenges. My first loss was devastating, and I considered giving up the sport, as my goal had been to maintain a perfect record. Ultimately, increasing training intensity, dance classes and the strong support from my team allowed me to win my next match and achieve the Most Improved Fighter award by the Sydney Boxing League.
WANT MORE AMAZING ARTICLES ON GRAD SCHOOL PERSONAL STATEMENTS?
- 100+ Outstanding Examples of Personal Statements
- The Ultimate Guide to Writing a Winning Personal Statement
- Common Pitfalls to Avoid in Your Personal Statement
- Writing a Killer Opening Paragraph for Your Personal Statement
- Ideal Length for a Graduate School Personal Statement
- 100 Inspiring Quotes to Jumpstart Your Personal Statement
Sample Personal Statement for Masters in International Business
Sample Personal Statement for Masters in International Business My journey began amidst the kaleidoscope of Qatar's landscapes, setting the stage for a life attuned to cultural nuances. Transitioning to Riyadh in my teens, I absorbed a mosaic of traditions, sparking a...
Sample Personal Statement for Family Medicine Residency
Personal Statement Prompt: A personal letter is required. We are looking for mature, enthusiastic physicians who bring with them a broad range of life experiences, are committed to providing excellent patient care, and can embrace the depth and breadth of experiences...
Harvard Personal Statement Example
In this article, I will be providing a sample grad school personal statement for Harvard University. This example aims to show how prospective applicants like you can seamlessly weave your passion, skills, and relevant experiences into a compelling narrative. In...
[2024] 4 Law School Personal Statement Examples from Top Programs
In this article, I will discuss 4 law school personal statement samples. These statements have been written by successful applicants who gained admission to prestigious US Law schools like Yale, Harvard, and Stanford. The purpose of these examples is to demonstrate...
Sample Personal Statement Cybersecurity
In this article, I will be providing a sample grad school personal statement in the field of cybersecurity. This sample was written by an applicant who got admitted into George Mason, Northeastern and Arizona State University. This example aims to show how prospective...
WANT AMAZING ARTICLES ON GRAD SCHOOL PERSONAL STATEMENTS?
- 100+ Personal Statement Templates
Clearing Universities & Courses
Clearing advice.
Recommended Clearing Universities
Popular Course Categories

Course Search & Discover
Start the search for your uni. Filter from hundreds of universities based on your preferences.
Search by Type
Search by region.
Recommended Universities

Ravensbourne University London
London (Greater) · 88% Recommended

University of Chester
North West England · 97% Recommended

University of Leicester
East Midlands Region · 93% Recommended
Search Open Days
What's new at Uni Compare

University of Bedfordshire
Bedfordshire has a 95% graduate employment rate for career prospects, find out more!

Ravensbourne University
90% of graduates going in to work or further study (Graduate Outcomes Survey, 2020)
Ranking Categories
Regional rankings.
More Rankings

Top 100 Universities
Taken from 65,000+ data points from students attending university to help future generations

About our Rankings
Discover university rankings devised from data collected from current students.
Guide Categories
Advice categories, recommended articles, popular statement examples, statement advice.

What to include in a Personal Statement

Personal Statement Tips
Personal statement examples finance personal statements.
Discover personal statement examples written by students accepted onto finance and related courses. Read through the examples to help shape your own personal statement.

Get the skills needed for an engaging and successful career
Pick Roehampton's Accounting degree for hands-on learning, networking opportunities, and paid placements setting your future up for success.

Are you a problem solver?
Delve into the world of banking at Bangor, Top 10 for Accounting & Finance (Guardian University Guide 2023).
Finance Personal Statements
Submitted by Samuel
Finance and Investment Personal Statement
Studying finance has always been a goal for me as it consists of elem...
Recommended Course

undergraduate Universities
Undergraduate uni's.

Ravensbourne

Uni of Chester
398 courses

Uni of Leicester
267 courses

Middlesex Uni
470 courses

Uni of Winchester
154 courses

Heriot-Watt Uni
208 courses

528 courses

Uni of Kent
413 courses

Uni of Sunderland
200 courses

238 courses

Uni of Roehampton
268 courses

Kingston Uni
376 courses

ARU Writtle
104 courses
.jpg)
Cardiff Met Uni
305 courses

Swansea Uni
771 courses

Uni of East London
317 courses

Northeastern Uni

444 courses

West London IoT

Coventry Uni
445 courses

Goldsmiths, UOL
273 courses

Leeds Beckett Uni
325 courses

Uni of Portsmouth
545 courses

Queen's Uni
411 courses

Uni of Surrey
435 courses

Uni of Suffolk
111 courses

Uni of Hertfordshire
419 courses

Uni for Creative Arts
323 courses

Uni of Bradford
197 courses

Uni of Bedfordshire
327 courses

Staffordshire Uni
272 courses

Uni of Westminster
336 courses

Anglia Ruskin Uni
464 courses
,-Bristol.jpg)
UWE, Bristol
252 courses

Leeds Arts University

Uni of Essex
797 courses

Escape Studios

Uni of Huddersfield
458 courses

353 courses

Wrexham Uni
168 courses

Uni of Brighton
257 courses

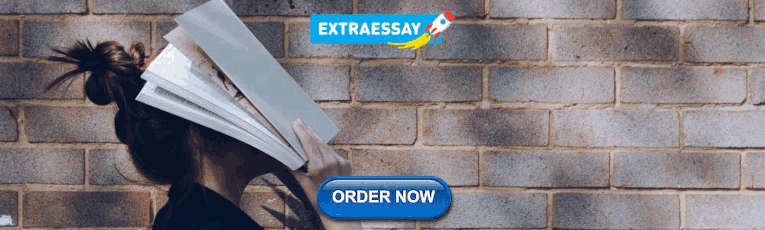
Uni of C.Lancashire
512 courses

Bath Spa Uni
292 courses

Edge Hill Uni
243 courses

Uni of Hull
274 courses

Nottingham Trent
539 courses

Edinburgh Napier
184 courses

Uni of Reading
391 courses

246 courses
Find the latest from Uni Compare

University of Kent
Awarded Silver in the TEF Rankings 2023, click here to learn more!

University of Surrey
Surrey has been shortlisted for the University of the Year 2023 - find out more here!
Delivered By
- Our Philosophy
- Global Partners
- New Candidates
- Positions Alumni Hold
- Alumni Testimonials
- Alumni Listing
- Our Faculty
- Who Is It For?
- How Do I Start?
- CQF Careers Service
- Three Phases
- Preparation
- CQF Qualification
- Lifelong Learning
- How to Study the CQF
- Learning Resources

Quant Finance 101: Top 50 Quantitative Finance Questions Answered
What is natural language processing? What is modern portfolio theory? What is the Black-Scholes formula? For answers to questions like these, explore the top 50 most searched questions in quantitative finance below to get all the information you need.
Accordion Heading
Accordion content.
Algorithmic trading, or algo trading, is frequently used in quantitative finance as way to execute financial transactions using computer algorithms. It involves the use of automated systems to make decisions, monitor markets, and execute trades. In algo trading, mathematical models and predefined rules are used to analyze market data, identify trading opportunities, and automatically generate buy or sell orders. These algorithms can be designed to consider various factors, including price movements, volume, timing, and other market indicators. Quantitative finance professionals develop and refine these algorithms by using statistical analysis, mathematical models, and historical data. Algo trading can be applied to various financial instruments, such as stocks, bonds, commodities, currencies, and derivatives. Benefits of algo trading include increased speed and accuracy in trade execution, reduced transaction costs, and the ability to process large volumes of data in real-time. Algo trading systems can monitor multiple markets simultaneously and execute trades based on pre-determined conditions, eliminating the need for manual intervention. However, it's important to note that algo trading also carries risks. The performance of algorithms depends on the quality of the underlying models and assumptions, and unforeseen market conditions or technical issues can lead to significant financial losses. Therefore, thorough testing, risk management , and continuous monitoring are essential in algo trading strategies. Overall, algo trading is a key component of modern quant finance, allowing market participants to leverage advanced technologies and mathematical models to make informed and efficient trading decisions. Algo trading and AI strategies for this are covered more in module 5 of the CQF program.
Arbitrage, in the context of quantitative finance, refers to the practice of profiting from price discrepancies in financial markets by simultaneously buying and selling related assets or securities. The goal of arbitrage is to take advantage of temporary market inefficiencies to generate risk-free profits. The basic principle is to exploit situations where the price of a financial instrument is mispriced or deviates from its intrinsic value. Quants use sophisticated mathematical models and algorithms to identify these pricing discrepancies and execute trades to capitalize on them. There are different types of arbitrage strategies employed in quant finance, including: Spatial Arbitrage: This involves taking advantage of price differences between the same asset traded in different markets or locations. For example, if a stock is priced lower on one exchange than another, an arbitrageur can buy it on the lower-priced exchange and simultaneously sell it on the higher-priced exchange to capture the price difference. Statistical Arbitrage: This strategy involves identifying and exploiting pricing discrepancies between related securities based on statistical patterns and historical relationships. Quants use quantitative models to identify pairs or groups of assets that are expected to move together in a predictable manner. When the relationship deviates from its expected behavior, trades are executed to profit from the convergence or divergence of prices. Merger Arbitrage: In the context of corporate events such as mergers and acquisitions, quants can engage in merger arbitrage. This strategy involves taking positions in the stocks of companies involved in a merger or acquisition deal. By buying the stock of the target company and simultaneously selling the stock of the acquirer, an arbitrageur aims to profit from the price discrepancy that arises until the deal is completed. It's important to note that while arbitrage opportunities offer the potential for risk-free profits, they are often difficult to find and execute due to intense competition and market efficiency. They are typically short-lived, as the market quickly adjusts to eliminate pricing discrepancies. Quants employ sophisticated mathematical models, data analysis techniques, and high-performance computing to identify and capitalize on these fleeting opportunities.
Arbitrage Pricing Theory (APT) is a financial model used to determine the expected return of an asset based on its exposure to various risk factors. It is an alternative to the Capital Asset Pricing Model (CAPM) and provides a framework for pricing assets by considering multiple sources of systematic risk. The main idea behind APT is that the expected return of an asset can be explained by its sensitivity to different risk factors rather than just the overall market risk. APT assumes that the returns of assets are driven by several common factors, such as interest rates, inflation, GDP growth, industry-specific variables, or other macroeconomic indicators. Below are the key principles involved in Arbitrage Pricing Theory: Factor Identification: The first step in APT is to identify the relevant risk factors that influence the returns of assets. These factors can be determined through statistical analysis or economic intuition. For example, in a stock market context, factors such as interest rate changes, market volatility, or sector-specific variables may be considered. Factor Sensitivity Estimation: Once the factors are identified, the next step is to estimate the sensitivity or exposure of an asset to each factor. This is typically done through regression analysis, where historical data is used to analyze the relationship between the asset's returns and the factors' returns. Factor Pricing: APT assumes that the expected return of an asset is a linear function of its factor sensitivities. The coefficients or factor loadings obtained from the regression analysis are used to price the asset. The asset's expected return is calculated as the sum of the risk-free rate and the product of the factor sensitivities and their corresponding risk premiums. Arbitrage Opportunities: A key assumption in APT is the absence of arbitrage opportunities. If an asset is mispriced, arbitrageurs would take advantage of the discrepancy by buying or selling the asset until its price adjusts to its fair value. The absence of such opportunities ensures that the pricing model is consistent and free of arbitrage. APT is a multifactor model that allows for a more nuanced assessment of asset pricing compared to the CAPM, which considers only the market risk. By considering multiple risk factors, APT can better capture the sources of risk that affect asset returns in specific contexts or industries. However, whilst APT offers a more flexible and comprehensive approach to asset pricing, its success relies on the identification and accurate estimation of the relevant risk factors. As with any financial model, APT has its limitations and assumptions that may not always hold in real-world scenarios.
ARCH (Autoregressive Conditional Heteroscedasticity) is a statistical model commonly used to analyze and forecast the volatility of financial time series data. It was introduced by Robert F. Engle in the early 1980s and has since become a widely used tool in econometrics and quantitative finance. The ARCH model is designed to capture the time-varying volatility or heteroscedasticity observed in many financial series, where the volatility of returns tends to cluster in periods of high volatility and dissipate during periods of low volatility. It assumes that the conditional variance of the series is a function of past squared residuals or error terms. Here are the key components and characteristics of an ARCH model: Conditional Variance Equation: The ARCH model specifies a conditional variance equation that models the dynamic behavior of the variance of the time series. The conditional variance, denoted as σ^2(t), is expressed as a function of lagged squared residuals or error terms. The model assumes that the variance at each time point is a weighted sum of the squared past residuals. Autoregressive Component: The ARCH model typically includes an autoregressive (AR) component to capture the dependence of the conditional variance on its past values. The AR component accounts for the persistence of volatility shocks. Residuals: The ARCH model assumes that the series follows a conditional mean equation (e.g., an ARMA or ARIMA model) to account for the mean behavior. The residuals obtained from the mean equation are then used to model the conditional variance in the ARCH equation. ARCH Order (p): The ARCH order, denoted as p, represents the number of past squared residuals used in the conditional variance equation. It determines the memory or persistence of volatility shocks. A higher order indicates a longer-lasting impact of past shocks on the current volatility. Estimation: The parameters of the ARCH model, including the autoregressive coefficients and the weights assigned to the past squared residuals, are estimated using methods such as maximum likelihood estimation or generalized method of moments. The ARCH model has been extended to include variations such as GARCH (Generalized Autoregressive Conditional Heteroscedasticity), which incorporates additional lagged terms to capture the time-varying volatility patterns more accurately. ARCH models are particularly useful in risk management, options pricing, and volatility forecasting. They provide insights into the clustering of volatility and allow for more accurate estimation of conditional volatility, which is crucial for assessing risk and making informed financial decisions. ARCH models are covered in more detail in module 2 of the CQF program.
Basel III is a set of global regulatory standards established by the Basel Committee on Banking Supervision (BCBS) to strengthen the regulation, supervision, and risk management practices of banks worldwide. The key objectives of Basel III are to enhance the resilience of the banking sector, improve risk management and transparency, and promote financial stability. It represents a significant reform of the previous Basel II framework and was developed in response to lessons learned from the 2008 financial crisis. Some of the main features and requirements of Basel III include: Capital Adequacy: Basel III introduces more stringent capital requirements for banks. It increases the minimum common equity Tier 1 (CET1) capital requirement and strengthens the quality and composition of capital. It also introduces a capital conservation buffer and a countercyclical buffer to help banks withstand periods of stress. Liquidity Standards: Basel III establishes liquidity requirements to ensure that banks maintain sufficient liquidity to meet their obligations in times of stress. It introduces two liquidity ratios: the Liquidity Coverage Ratio (LCR) and the Net Stable Funding Ratio (NSFR). The LCR focuses on short-term liquidity risk, while the NSFR addresses longer-term funding stability. Leverage Ratio: Basel III introduces a leverage ratio as a non-risk-based measure to limit excessive leverage in the banking system. It sets a minimum requirement for Tier 1 capital in relation to a bank's total exposure. Systemically Important Banks: Basel III addresses the systemic risks posed by globally systemically important banks (G-SIBs). It introduces additional capital requirements, loss absorbency mechanisms, and enhanced supervisory frameworks for G-SIBs to reduce the likelihood of their failure and minimize the impact on the financial system in case of failure. Counterparty Credit Risk: Basel III strengthens the regulation and supervision of counterparty credit risk in derivative transactions. It introduces higher capital requirements for counterparty credit risk exposures and promotes the use of central clearing and collateralization. Disclosure and Reporting: Basel III enhances the disclosure and reporting requirements for banks, aiming to improve transparency and enable market participants and regulators to assess banks' risk profiles and capital adequacy. The implementation of Basel III is carried out by national regulators and central banks in each jurisdiction, which may tailor certain aspects of the standards to their specific circumstances. The phased implementation of Basel III started in 2013 and continued over several years, with different components gradually coming into effect. Basel III is covered in more detail in module 2 of the CQF program.
The binomial model is a mathematical model used to value financial derivatives, particularly options, by modeling the movement of the underlying asset price over discrete time periods. It is a simple, yet powerful tool commonly employed in option pricing and risk analysis. Key features include: Discrete Time: The binomial model divides time into a series of discrete intervals or periods. Typically, these intervals are equal in length, such as days, months, or years. The number of periods chosen depends on the desired level of accuracy and the characteristics of the underlying asset. Two Possible Price Movements: The model assumes that the price of the underlying asset can move in only two directions at each time step: up or down. The magnitudes of these price movements are determined by the model parameters, such as an up factor and a down factor. Probabilistic Movement: At each time step, the model assigns probabilities to the up and down movements based on assumptions or historical data. These probabilities, often denoted as p and 1-p, represent the likelihood of each price movement occurring. Asset Price Calculation: Starting from the initial asset price, the binomial model calculates the potential asset prices at each subsequent time step based on the up and down movements. These calculations are typically performed using multiplication or division by the up and down factors. Option Valuation: The binomial model values options by calculating the expected present value of future cash flows associated with the option. At each time step, the model determines the option value based on the underlying asset prices and the option's payoff function. The option value is then discounted back to the present using a risk-free interest rate. Recursive Backward Calculation: The binomial model utilizes a backward calculation process, starting from the final time step and moving back to the initial time step. This process involves determining the option values at each step based on the expected values and probabilities of the subsequent steps. The binomial model is flexible and can handle a variety of option types, including European options, American options, and options with different exercise styles or features. It can accommodate various assumptions and market conditions, such as constant or time-varying volatility. While the binomial model is computationally intensive compared to other option pricing models like the Black-Scholes model, it is widely used due to its intuitive structure and ability to capture discrete price movements accurately. It's important to note that the binomial model is a simplification of the actual market dynamics and assumes certain assumptions about the underlying asset's behavior. Nonetheless, it serves as a valuable tool for option pricing and understanding the basic concepts of derivative valuation. The binomial model is covered in more detail in module 1 of the CQF program.
The Black-Scholes equation is a mathematical formula used to price European-style options, which are options that can only be exercised at expiration. It was developed by economists Fischer Black and Myron Scholes in 1973 and has become a cornerstone of option pricing theory. The equation is derived under certain assumptions, including efficient markets, no transaction costs, and constant volatility. It has practical applications in derivatives trading, risk management, and portfolio optimization. The Black-Scholes equation is as follows: C = S * N(d1) - X * e^(-r * T) * N(d2) Where: C represents the theoretical price of the call option. S is the current price of the underlying asset. N(d1) and N(d2) are the cumulative distribution functions of standard normal distribution, evaluated at d1 and d2, respectively. X is the strike price of the option. e is the base of the natural logarithm (approximately 2.71828). r is the risk-free interest rate. T is the time to expiration of the option, expressed in years. The d1 and d2 terms are calculated as follows: d1 = (ln(S / X) + (r + (σ^2) / 2) * T) / (σ * sqrt(T)) d2 = d1 - σ * sqrt(T) Where: ln is the natural logarithm. σ is the volatility of the underlying asset. The equation provides a way to estimate the fair price of a European call option based on the underlying asset's price, the strike price, the time to expiration, the risk-free interest rate, and the asset's volatility. It's important to note that the Black-Scholes equation assumes a range of assumptions, including constant volatility and no dividends. Various extensions and modifications, such as the Black-Scholes-Merton model, have been developed to account for additional factors or relax some assumptions. The Black-Scholes model is covered in more detail in module 3 of the CQF program.
The Capital Asset Pricing Model (CAPM) provides a framework for estimating the expected return of an investment based on its systematic risk. The model was developed by William Sharpe, John Lintner, and Jan Mossin in the 1960s and has since become a fundamental tool in finance and investment analysis. Key components of the Capital Asset Pricing Model include: Expected Return: The CAPM is concerned with the expected return of an investment, which represents the compensation an investor demands for taking on the investment's risk. Systematic Risk: The CAPM focuses on systematic risk, which is the risk that cannot be eliminated through diversification. It is measured by the beta (β) of an investment, which reflects its sensitivity to overall market movements. Risk-Free Rate: The model assumes the existence of a risk-free asset, such as a government bond, that offers a known return with no risk. The risk-free rate is typically represented by the yield on this asset. Market Risk Premium: The CAPM incorporates the market risk premium, which represents the excess return that investors expect to receive for holding a risky asset compared to a risk-free asset. It is determined by the overall level of market risk and the risk aversion of investors. CAPM Formula: The expected return of an investment according to the CAPM can be calculated using the following formula: Expected Return = Risk-Free Rate + β * (Market Risk Premium) This formula indicates that an investment's expected return is equal to the risk-free rate plus a risk premium determined by the investment's beta and the market risk premium. Efficient Frontier: The CAPM implies that in an efficient market, where all investors have access to the same information and hold diversified portfolios, the optimal portfolio lies on the efficient frontier. The efficient frontier represents the set of portfolios that offer the highest expected return for a given level of risk. The CAPM has practical applications in various areas, including investment valuation, portfolio management , and determining the required rate of return for capital budgeting decisions. However, it does rely on several simplifying assumptions, such as perfect markets, linear relationships, and homogenous expectations, which may limit its accuracy in real-world situations. The CAPM has also faced criticism and challenges, with alternative models and factors emerging to account for additional sources of risk and market anomalies. CAPM is covered in more detail in module 2 of the CQF program.
The Central Limit Theorem (CLT) is a fundamental concept in probability theory and statistics that has important implications in quantitative finance. It states that, under certain conditions, the distribution of the sum or average of many independent and identically distributed (i.i.d.) random variables approaches a normal distribution, regardless of the shape of the original distribution. In the context of quantitative finance, the Central Limit Theorem has several key implications: Market Returns: The Central Limit Theorem suggests that the distribution of market returns, which can be seen as the sum or average of numerous individual price changes, tends to be approximately normal. This assumption of normality is often made in quantitative models and statistical analysis. Portfolio Returns: The CLT has important implications for portfolio returns. If the returns of individual assets in a portfolio are i.i.d., the portfolio's overall return distribution will tend to become more normal as the number of assets in the portfolio increases. This allows for the application of various statistical techniques and portfolio optimization methods that assume normality. Hypothesis Testing: The Central Limit Theorem is frequently used in hypothesis testing in quantitative finance. Many statistical tests, such as t-tests and z-tests, rely on the assumption of normality. The CLT provides a justification for using these tests when sample sizes are sufficiently large. Option Pricing: The Central Limit Theorem is relevant in option pricing models. For example, in the Black-Scholes model, which assumes that stock price changes follow a log-normal distribution, the CLT helps establish the assumption of continuous trading and the normality of log returns. It's important to note that the Central Limit Theorem relies on specific assumptions, including the i.i.d. nature of the random variables and the existence of finite moments. Additionally, while the CLT provides a useful approximation in many situations, it may not hold precisely for all financial data, especially when dealing with extreme events or heavy-tailed distributions. CLT is covered in more detail in module 1 of the CQF program.
In quantitative finance, a coherent risk measure is a concept used to assess and quantify risk in a consistent and mathematically sound manner. It provides a framework for measuring and managing risk in a way that aligns with certain desirable properties and principles. Examples of coherent risk measures commonly used in quantitative finance include Value at Risk (VaR), Conditional Value at Risk (CVaR), and Tail Value at Risk (TVaR). These risk measures are widely employed in risk management , portfolio optimization, and regulatory frameworks. By utilizing coherent risk measures, financial institutions and investors can better quantify and manage risk in a consistent and robust manner, enabling more informed decision-making and risk mitigation strategies.
In quantitative finance, copulas are statistical tools used to model and analyze the dependence structure between multiple random variables. They offer a flexible framework to capture and quantify the dependence patterns, regardless of the marginal distributions of the variables. Copulas have gained popularity in finance for modeling the joint distribution of asset returns, assessing portfolio risk, pricing complex derivatives, and simulating correlated scenarios. There are several types of copulas commonly used in quantitative finance. Each type possesses specific characteristics that make it suitable for different types of dependence patterns. Common types include: Gaussian Copula: The Gaussian copula assumes that the joint distribution follows a multivariate normal distribution after transforming the marginals to standard normal distributions. It is widely used due to its simplicity and tractability, but it has limitations in capturing extreme tail dependencies observed in financial markets. t-Copula: The t-copula is an extension of the Gaussian copula that incorporates heavier tails to capture extreme dependencies. It introduces a parameter, called degrees of freedom, that controls the tail behavior. The t-copula is more flexible than the Gaussian copula and can better capture tail dependence, which is important for modeling extreme events. Archimedean Copulas: Archimedean copulas are a family of copulas that use specific generating functions to model dependence. Examples of Archimedean copulas include Clayton copula, Gumbel copula, and Frank copula. Each Archimedean copula has its own parameter that determines the strength of dependence and tail behavior. These copulas are often used when the dependence structure displays asymmetric or non-linear relationships. Vine Copulas: Vine copulas provide a more flexible and powerful way to model multivariate dependencies by using a combination of bivariate copulas. Vine copulas construct a tree-like structure to capture complex dependence patterns. They can better capture asymmetric and non-linear dependencies, and they offer more flexibility in modeling high-dimensional dependence structures. Copula Mixture Models: Copula mixture models combine multiple copulas to capture various types of dependence within the same model. They allow for capturing different tail behaviors and capturing different types of dependence patterns simultaneously. Copula mixture models provide greater flexibility but require more parameters to be estimated. It's important to note that the choice of copula depends on the specific characteristics of the data and the nature of the dependence being modeled. Selecting an appropriate copula requires careful analysis and consideration of the data's properties and the desired modeling objectives. They have proven to be valuable tools in quantitative finance by allowing practitioners to model and understand complex dependencies among financial variables, facilitating risk assessment, portfolio optimization, and derivative pricing. Copulas are covered in more detail in module 6 of the CQF program.
Credit Default Swaps (CDS) are financial derivatives that allow investors to protect against the risk of default or credit events on a specific debt instrument or credit entity. CDS contracts provide a form of insurance or hedging against credit risk. Here are some key points about credit default swaps: Structure: A credit default swap involves two parties - the protection buyer and the protection seller. The protection buyer pays periodic premiums to the protection seller in exchange for a promise to compensate for losses in the event of a credit event, such as a default or bankruptcy of a reference entity (e.g., a company, government, or financial institution). Reference Entity and Obligation: CDS contracts are linked to a specific reference entity or a basket of entities. The reference entity can be a corporate bond, a loan, or any debt instrument. The contract specifies the obligations that trigger a credit event, such as a missed payment, bankruptcy, or restructuring. Credit Event and Payout: If a defined credit event occurs, the protection seller is obligated to make a payout to the protection buyer. The payout amount is typically determined by the difference between the face value of the debt instrument and the recovery value of the defaulted debt. The recovery value is often estimated using auction processes or market prices. Trading and Market Liquidity: CDS contracts are traded over the counter (OTC) between market participants, rather than on centralized exchanges. The market for credit default swaps is substantial and serves as a key component of the broader credit derivatives market. However, trading volumes can vary depending on market conditions and the availability of market participants. Hedging and Speculation: CDS contracts can be used for both hedging and speculation purposes. Investors or institutions that hold credit risk exposure can use CDS to hedge against potential losses. Speculators, on the other hand, may trade CDS to take positions on the creditworthiness of specific entities or to express views on market conditions. Counterparty Risk: CDS contracts involve counterparty risk, as the protection seller may not be able to fulfill its payment obligations in the event of a credit event. This risk became prominent during the 2008 financial crisis when concerns arose about the ability of some protection sellers to honor their obligations, leading to systemic risks. It is important to note that credit default swaps have been the subject of regulatory scrutiny and debate due to their potential to amplify systemic risks and the need for transparency in the market. As a result, regulatory reforms have been implemented to enhance transparency and oversight of the CDS market. Credit Default Swaps are covered in more detail in module 6 of the CQF program.
Decision trees are a popular machine learning algorithm and a visual representation of decision-making processes. They are used for both classification and regression tasks and offer a simple yet powerful way to analyze and interpret data. Decision trees have broad applications across quantitative finance as they can be used in credit risk assessment, trading strategies, portfolio management , fraud detection, credit scoring and loan approval, and option pricing. How does a decision tree work? A decision tree is composed of nodes and branches. The nodes represent decision points or features, while the branches represent the possible outcomes or choices based on those features. The tree structure starts from the root node and branches out to subsequent nodes until reaching the leaf nodes, which represent the final predictions or outcomes. At each node, a decision tree algorithm selects the best splitting criterion based on certain measures, such as Gini impurity or information gain. These criteria evaluate the similarity or purity of the target variable within each potential branch. The goal is to split the data in a way that maximizes the separation between different classes or minimizes the variance in regression tasks. The algorithm then determines the most informative features for decision-making. It selects the feature that provides the greatest discriminatory power or predictive value. The selection process aims to create branches that result in the most accurate predictions or classifications. Something to be aware of is that decision trees can be prone to overfitting, where they become overly complex and tailor-made for the training data. To avoid overfitting, pruning techniques are often applied. This involves removing branches that do not significantly contribute to the predictive performance on unseen data. Decision trees can also be combined using ensemble methods such as Random Forests or Gradient Boosting. These methods create an ensemble of decision trees, each trained on a different subset of the data or with different parameter settings. The ensemble aggregates the predictions of individual trees, resulting in improved accuracy and robustness. However, decision trees also have limitations. They can be sensitive to small changes in the data, and they may struggle to capture relationships that require multiple levels of decision-making. Nonetheless, decision trees remain a popular and valuable tool in machine learning and data analysis. Decision trees are covered in more detail in module 4 of the CQF program.
The deterministic modeling approach in quantitative finance refers to a modeling framework that assumes all input variables and parameters are known with certainty and do not involve randomness or uncertainty. In this approach, the future values of variables and financial instruments are predicted based on specific assumptions and deterministic relationships. The deterministic approach assumes that all input variables, such as interest rates, asset prices, and market conditions, are known with complete certainty. There is no consideration for uncertainty or randomness in these variables. It’s the same for the relationships among the variables and financial instruments, these relationships are assumed to be known and fixed, allowing for precise calculations and predictions. The approach does not incorporate probabilistic analysis or attempt to estimate the likelihood or range of possible outcomes. Instead, it aims to provide precise predictions and outcomes based on known variables and assumptions. It assumes that the future behavior of the financial system or market can be accurately predicted based on the deterministic relationships in the model. However, as the deterministic modeling approach often relies on simplified assumptions and linear relationships to facilitate calculations and analysis, it may overlook complex dynamics and nonlinear interactions that exist in real-world financial markets. Financial markets are inherently uncertain and subject to various factors that cannot be accurately predicted with certainty. Ignoring uncertainty and randomness in modeling can lead to biased predictions and inadequate risk management. To address the limitations of the deterministic approach, stochastic modeling approaches are commonly used in quantitative finance. Stochastic models incorporate randomness and uncertainty, allowing for probabilistic analysis and a more realistic representation of financial markets. These models, such as Monte Carlo simulations or stochastic differential equations, consider the probabilistic nature of input variables and provide a range of possible outcomes, enabling better risk assessment and decision-making.
Dispersion trading is an options trading strategy that seeks to profit from the relative pricing differences or dispersion among the individual components of an underlying index or sector. It involves simultaneously buying and selling options on the constituent stocks or securities within the index. The key idea behind dispersion trading is to take advantage of the expected convergence or divergence of the individual stock or security prices within the index. The strategy assumes that while the overall index or sector may remain relatively stable, the prices of the individual components may exhibit greater variability or dispersion. Usually, the trader will select an underlying index or sector that comprises multiple individual stocks or securities. Common choices include broad market indices or sector-specific indices. The trader will then take both long and short positions in options contracts on the individual stocks or securities within the index. Typically, the strategy involves buying options on stocks that are expected to outperform and selling options on stocks that are expected to underperform or remain stable. Dispersion trading considers the expected volatility and correlation among the individual components, so the trader needs to assess the historical and implied volatilities of the stocks and consider their correlation dynamics. The strategy may involve adjusting the options positions based on changes in volatility or correlations. The profit in dispersion trading arises from the difference between the premiums collected from selling options and the premiums paid for buying options. If the dispersion among the individual stocks widens or narrows as anticipated, the trader can profit from the resulting changes in option prices. To ensure profit, risk management is crucial in dispersion trading. The trader needs to carefully monitor and control the overall risk exposure of the portfolio. Proper position sizing, diversification, and risk hedging techniques are employed to manage potential losses in case of adverse movements in the individual stock prices or overall market conditions. Additionally, liquidity and transaction costs should be carefully considered, especially when trading options on individual stocks with varying levels of liquidity. Dispersion trading is often implemented by sophisticated investors, hedge funds, or proprietary trading desks that have expertise in options trading and quantitative analysis. It requires a deep understanding of options pricing, market dynamics, and the relationships among the individual securities within the index.
The Efficient Markets Hypothesis (EMH) is an influential theory in finance that suggests that financial markets are highly efficient in reflecting all available information. The hypothesis asserts that market prices accurately and immediately reflect all relevant information, making it impossible to consistently achieve above-average returns by exploiting mispriced securities. Key aspects of the Efficient Markets Hypothesis include: Information Efficiency: The EMH posits that financial markets are informationally efficient, meaning that prices quickly and accurately adjust to new information. This includes both public information (such as financial statements, news, and economic data) and private information (such as insider information). According to the EMH, it is difficult to consistently outperform the market by trading on information since market prices already incorporate all available information. Three Forms of Market Efficiency: The EMH recognizes three forms of market efficiency: weak-form efficiency, semi-strong form efficiency, and strong-form efficiency. Weak-form efficiency suggests that current prices already reflect all past price and volume data, implying that technical analysis and trading rules based on historical patterns are unlikely to consistently generate excess returns. Semi-strong form efficiency argues that prices reflect all publicly available information, making it difficult to gain an edge by analyzing public information such as news or financial statements. Strong-form efficiency implies that prices incorporate all information, including public and private information, making it impossible for any market participant to consistently outperform the market. Implications for Active Management: The EMH has implications for active portfolio management and the ability to consistently beat the market. If markets are efficient, then active management strategies aiming to identify mispriced securities or time market movements may not consistently generate superior returns. Instead, the EMH suggests that passive investing, such as index funds or exchange-traded funds (ETFs), is a more suitable approach for most investors. The Efficient Markets Hypothesis has faced criticism and challenges. Some argue that markets are not perfectly efficient due to factors like behavioral biases, market frictions, and information asymmetry. Supporters of behavioral finance suggest that investor psychology and irrational behavior can lead to persistent market inefficiencies. However, the Efficient Markets Hypothesis remains a widely discussed and influential concept that has shaped modern finance and investment theory.
Exotic options are a class of complex and non-standard financial options that possess unique or customized features beyond those found in standard options, such as plain vanilla options. These options are often tailored to specific needs and investment strategies, offering investors more flexibility and customization in their risk and return profiles. Key characteristics of exotic options include: Payoff Structures: Exotic options can have unconventional payoff structures that differ from the simple call or put options of standard options. They may include features such as barrier options, binary options, Asian options, lookback options, or digital options. These structures allow investors to gain exposure to specific market scenarios or create customized risk-reward profiles. Path-Dependent Features: Exotic options often have path-dependent characteristics, meaning that the option's value depends on the historical path of the underlying asset price. For example, lookback options consider the highest or lowest price of the underlying asset during a specific period. Path-dependent features introduce complexities and considerations beyond standard options. Expiration and Exercise Features: Exotic options may have non-standard expiration dates or exercise rules. They can have Bermudan-style exercise, allowing exercise at specific intervals before expiration, or can be European-style with specific conditions for early exercise. These features provide additional flexibility for investors but require careful evaluation and analysis. Complex Pricing Models: Pricing exotic options can be more complex than standard options due to their unique features and path-dependency. Advanced mathematical models, such as Monte Carlo simulations, finite difference methods, or numerical techniques, are often employed to value exotic options accurately. Tailored Risk Management: Exotic options are often used for customized risk management and hedging purposes. Their specific features allow investors to mitigate risks associated with specific market conditions, volatility patterns, or asset price movements. Exotic options can provide tailored solutions to address specific risk exposures or market views. Liquidity and Market Accessibility: Exotic options are generally less liquid and traded less frequently than standard options. They are often traded over the counter (OTC) or on specialized derivatives exchanges. Due to their customization and complexity, pricing and executing exotic options may require the involvement of specialized market participants or financial institutions. Exotic options can be beneficial for sophisticated investors or institutions seeking tailored risk management, specific market exposures, or investment strategies beyond the scope of standard options. However, they require a deep understanding of their unique features, pricing models, and associated risks. It is essential to conduct thorough analysis, consult with experts, and fully comprehend the complexities involved before engaging in exotic option transactions. Exotic options are covered in more detail in module 3 of the CQF program.
Extreme Value Theory (EVT) is a statistical approach that focuses on analyzing extreme events or values in data, rather than assuming a normal or symmetric distribution. It provides a framework for understanding and modeling the behavior of extreme values that exceed a certain threshold. Unlike traditional statistical methods that focus on central tendencies, EVT concentrates on the tail behavior of distributions. In quantitative finance, EVT is used for modeling extreme stock market returns, assessing tail risks through measures like Value at Risk (VaR) and Expected Shortfall (ES), analyzing extreme losses in portfolio management, and calculating risk measures for extreme events. EVT typically employs three fundamental extreme value distributions: the Gumbel distribution, the Fréchet distribution, and the Weibull distribution. These distributions are used to model extreme events and capture the tail behavior of data. To analyze extreme values, EVT uses two main approaches: the block-maxima method and the peak-over-threshold method. The block-maxima approach selects the maximum value within each block of data, while the peak-over-threshold approach focuses on values exceeding a predefined threshold. When thinking about EVT, it is important to know about the Extreme Value Index (EVI). The EVI is an important parameter estimated in EVT. It quantifies the tail behavior of the distribution and helps measure the probability of extreme events occurring. It provides insights into the severity and frequency of extreme values. EVT does have some limitations as it assumes certain conditions, such as independence and stationarity of extreme events, which may not always hold in real-world financial data. Careful validation and consideration of data quality and model assumptions are necessary when applying EVT in practice. EVT is a valuable tool for understanding and managing tail risks in finance. It allows for a deeper analysis of extreme events that traditional statistical techniques might overlook, providing insights into the behavior of rare and extreme values.
Girsanov's Theorem is a fundamental result in stochastic calculus and mathematical finance. It provides a mathematical framework for transforming a stochastic process under one probability measure into an equivalent process under a different probability measure, particularly in the context of continuous-time financial models. Girsanov's Theorem is particularly relevant in the context of financial modeling, where it is used to derive the risk-neutral measure. The risk-neutral measure is an artificial probability measure under which the expected returns of risky assets are adjusted to be risk-free. It simplifies the pricing and valuation of derivatives by assuming a risk-free investment opportunity. The Theorem is closely related to the Martingale Representation Theorem which provides a way to express a stochastic process as a stochastic integral with respect to a certain martingale under the changed probability measure. This representation is essential for pricing derivatives and constructing optimal trading strategies. The Theorem also involves the construction of a Radon-Nikodym derivative, also known as the Girsanov density or Girsanov kernel. This derivative specifies the transformation between the original and the new probability measure. It quantifies the adjustments needed to account for the change in probability measure and the associated drift term. Girsanov's Theorem is widely used in option pricing and risk management. It enables the derivation of risk-neutral pricing formulas, such as the Black-Scholes-Merton formula, by changing the measure to the risk-neutral measure and integrating the derivative payoff under the transformed measure. However, it assumes certain conditions, such as the absence of arbitrage opportunities and the completeness of the financial market. It may not hold in the presence of transaction costs, market frictions, or other complexities. Careful considerations of market assumptions and limitations are necessary when applying Girsanov's Theorem in practice. Girsanov's Theorem plays a crucial role in quantitative finance, allowing for the modeling and pricing of derivative securities under the risk-neutral measure. It facilitates the analysis of financial market dynamics and the construction of optimal trading strategies in continuous-time models. Girsanov's Theorem is covered in more detail in module 3 of the CQF program.
In quantitative finance, "the Greeks" refers to a set of measures used to assess the sensitivity of options and other derivatives to various factors. These factors are typically related to the underlying asset, time, volatility, and interest rates. The Greeks are named after letters from the Greek alphabet, such as Delta, Gamma, Theta, Vega, and Rho. They play a crucial role in understanding and managing the risks associated with options and other derivative positions. Below are the key Greeks and their interpretations: Delta (Δ): Delta measures the sensitivity of an option's price to changes in the price of the underlying asset. It represents the change in the option price for a one-unit change in the underlying asset price. Delta ranges between 0 and 1 for call options (0 to -1 for put options), indicating the percentage change in the option price relative to a change in the underlying asset price. Gamma (Γ): Gamma measures the rate of change of an option's delta in response to changes in the price of the underlying asset. It quantifies the convexity of an option's delta and indicates how much the delta itself will change given a one-unit change in the underlying asset price. Theta (Θ): Theta measures the rate of change of an option's price with respect to the passage of time. It quantifies the erosion of the option's value due to the diminishing time to expiration. Theta is typically negative, indicating that options lose value over time. Vega (V): Vega measures the sensitivity of an option's price to changes in the implied volatility of the underlying asset. It quantifies the impact of changes in market expectations of future volatility on the option price. Vega is typically positive, suggesting that an increase in implied volatility will lead to a higher option price and vice versa. Rho (ρ): Rho measures the sensitivity of an option's price to changes in the risk-free interest rate. It quantifies the impact of interest rate changes on the option price. Rho is typically positive for call options (negative for put options), indicating that higher interest rates generally increase the value of call options and decrease the value of put options. These Greeks provide valuable insights into the risk exposures and behavior of options and other derivatives. They help traders, portfolio managers, and risk analysts assess and manage risks related to changes in asset prices, time decay, volatility fluctuations, and interest rate movements. It's important to note that the Greeks are not standalone measures but are interconnected and should be considered collectively to fully understand the risk profile of a derivative position. Additionally, the Greeks are based on various assumptions and simplifications, so their accuracy may be influenced by market conditions and modeling assumptions. The Greeks are covered in more detail in module 3 of the CQF program.
The finite difference method is a widely used numerical technique in quantitative finance for solving partial differential equations (PDEs) that arise in various financial models. It plays a significant role in option pricing, risk management, and other quantitative finance applications. In quantitative finance, the finite difference method involves discretizing the financial domain into a grid or mesh of points, approximating the derivatives using finite difference approximations, constructing a system of difference equations, solving the equations numerically, and incorporating boundary and initial conditions specific to the financial problem. By employing the finite difference method, quantitative finance professionals can numerically solve PDEs that appear in option pricing models, interest rate models, credit risk models, and other financial models. This enables the calculation of option prices, hedging strategies, risk measures, and other crucial financial quantities. The finite difference method provides a versatile and powerful approach for analyzing and valuing complex financial instruments and portfolios. However, it is important to carefully consider the choice of grid size, discretization, and numerical techniques to ensure accurate and reliable results. Overall, the finite difference method serves as a valuable tool in quantitative finance, aiding in the numerical analysis, pricing, and risk management of a wide range of financial derivatives and instruments. The finite difference method is covered in more detail in module 3 of the CQF program.
In quantitative finance, fixed income products refer to financial instruments that provide investors with a fixed stream of income over a specified period. These products are typically debt securities issued by governments, corporations, or other entities to raise capital. They are called "fixed income" because they offer a predetermined interest or coupon payment to the investor. Common examples of fixed income products include: Bonds: Bonds are debt securities where the issuer (government or corporation) borrows money from investors for a fixed period at a specified interest rate. The interest, known as the coupon, is paid to the bondholder periodically (e.g., annually or semi-annually) until maturity when the principal is repaid. Treasury Securities: These are bonds issued by governments, such as U.S. Treasury bonds, notes, and bills. They are considered low-risk investments and serve as benchmarks for other fixed income products. Corporate Bonds: These are bonds issued by corporations to raise capital. Corporate bonds typically offer higher yields compared to government bonds to compensate for the additional credit risk associated with the issuer. Municipal Bonds: Municipal bonds, or munis, are issued by state or local governments to fund public infrastructure projects. The interest earned on municipal bonds is often tax-exempt at the federal level and sometimes at the state and local levels. Mortgage-Backed Securities (MBS): MBS represent a pool of underlying residential or commercial mortgages. These securities are created by bundling individual mortgages and selling them to investors. The cash flows from the mortgage payments form the basis for the interest and principal payments to the MBS holders. Asset-Backed Securities (ABS): ABS are securities backed by pools of various types of assets, such as auto loans, credit card receivables, or student loans. These securities offer investors exposure to the cash flows generated by the underlying assets. Collateralized Debt Obligations (CDOs): CDOs are structured financial products that pool together various fixed income assets, including bonds, ABS, or MBS. They are divided into tranches with different levels of credit risk and return profiles. In quantitative finance, various models and techniques are used to analyze and value fixed income products, including yield curve analysis, duration and convexity measures, pricing models like the Black-Scholes model for options on fixed income securities, and risk management tools such as Value at Risk (VaR) calculations. Fixed income products are covered in more detail in module 6 of the CQF program.
Funding Value Adjustment (FVA) is an adjustment made to the valuation of derivatives or other financial instruments to account for the cost of funding the associated trades. It is a concept used in quantitative finance and risk management, particularly in the context of over-the-counter (OTC) derivative transactions. The Funding Value Adjustment takes into consideration the fact that when trading derivatives, a party is required to fund the necessary capital to support the position. This funding cost can be attributed to several factors, including the cost of borrowing funds, the collateral requirements imposed by counterparties, and the capital charges imposed by regulatory frameworks like Basel III. FVA also recognizes that when a trading desk enters into a derivative contract, it needs to allocate funds to finance the position. This capital requirement incurs costs, such as the cost of borrowing money or the opportunity cost of using capital for the trade. FVA also accounts for the credit risk associated with the derivative transaction. If the counterparty defaults, the funding costs and potential losses need to be considered in the valuation. In many OTC derivative transactions, collateral is exchanged between counterparties to mitigate credit risk. The FVA incorporates the cost of posting collateral or the benefit of receiving collateral in the valuation. Additionally, regulatory frameworks like Basel III impose capital charges on banks and financial institutions. These charges depend on the riskiness of the derivative positions, and FVA incorporates these capital costs in the valuation. FVA is typically calculated by modeling the cost of funding and taking into account the various factors mentioned above. The adjustment is applied to the fair value of the derivative contract to reflect the funding cost associated with the position. However, FVA is a complex and debated topic in quantitative finance, and different institutions may have varying methodologies and approaches for calculating and incorporating FVA into their valuations. FVA is covered in more detail in module 6 of the CQF program.
Itô's lemma, also known as the Itô-Doeblin Formula, is a fundamental result in stochastic calculus. It provides a rule for differentiating stochastic processes involving Brownian motion. Named after the Japanese mathematician Kiyoshi Itô, this lemma plays a central role in the development of stochastic calculus and its applications in quantitative finance. It is extensively used in mathematical finance for pricing and risk management of derivative securities. It allows for the derivation of stochastic differential equations and facilitates the calculation of various quantities, such as option prices, implied volatilities, and hedging strategies, through the application of stochastic calculus. Key aspects of Itô's lemma to consider are: Stochastic Differential Equation (SDE): Itô's lemma is used to differentiate stochastic processes described by stochastic differential equations (SDEs). SDEs incorporate the effects of random fluctuations, such as Brownian motion, into the evolution of a variable over time. The lemma helps compute the differential of a function involving these stochastic processes. Chain Rule for Stochastic Calculus: Itô's lemma is a stochastic analog of the chain rule in ordinary calculus. It provides a way to compute the derivative of a function that involves a stochastic process by considering the contributions from both the drift (deterministic) and diffusion (stochastic) components. Expansion of Stochastic Processes: Itô's lemma expands a function of a stochastic process into a Taylor series expansion, considering the impact of the stochastic term (Brownian motion) on the higher-order differentials. The lemma takes into account the quadratic variation of the Brownian motion, which captures its random and non-linear behavior. Limitations and Assumptions: Itô's lemma assumes that the stochastic process follows a continuous-time, continuous-sample path. It is based on the assumption of Itô diffusion, which represents a specific type of stochastic process. Deviations from these assumptions may require modifications to the application of Itô's lemma. Itô's lemma is covered in more detail in module 1 of the CQF program.
Jensen's Inequality is a fundamental result in mathematical analysis that relates the convexity of a function to its expected value. It provides a mathematical relationship that holds for convex or concave functions and is widely used in various fields, including economics, finance, and probability theory. In economics and finance, it is used to establish bounds on risk and return relationships, pricing models, portfolio optimization, and utility theory. In probability theory, it helps derive bounds on expected values and moments of random variables. Jensen's Inequality applies to convex functions, but what is a convex function? A function is considered convex if the line segment connecting any two points on the graph lies above or on the graph. Intuitively, it means the function curves upward or is "bowed" upward. Geometrically, Jensen's Inequality can be visualized as the fact that the graph of a convex function lies below the secant line connecting two points on the graph. The inequality implies that the average value of a convex function is greater than or equal to the function evaluated at the average value. Jensen's Inequality states that for a convex function, the expected value of the function evaluated at a random variable is greater than or equal to the function of the expected value of the random variable. Symbolically, for a convex function f(x) and a random variable X, it can be expressed as E[f(X)] ≥ f(E[X]). The expected value E[X] of a random variable X represents the average value it would take over a large number of trials. In the context of Jensen's Inequality, the expected value is calculated by summing or integrating the product of the random variable's values and their respective probabilities or densities. Jensen's Inequality also holds for concave functions, but with the direction of the inequality reversed. For a concave function, the expected value of the function evaluated at a random variable is less than or equal to the function of the expected value. Symbolically, for a concave function f(x) and a random variable X, it can be expressed as E[f(X)] ≤ f(E[X]).
A jump diffusion model is a mathematical framework used to describe the dynamics of an asset's price or other financial variables that exhibit both continuous changes (diffusion) and sudden, discontinuous changes (jumps). It is a popular model in quantitative finance for capturing the presence of unpredictable, significant price movements or events in financial markets. Jump diffusion models have various applications in quantitative finance, particularly in option pricing, risk management, and the analysis of market dynamics. They allow for a more accurate representation of market behavior by incorporating sudden, unpredictable price movements. However, it's worth noting that jump diffusion models are just one way to capture jumps in asset prices, and other models, such as stochastic volatility models, can be used depending on the specific requirements and characteristics of the financial data. Explore the key aspects of a jump diffusion model below: Diffusion Component: The diffusion component in a jump diffusion model represents the continuous, smooth changes in the asset's price or other financial variables. It is typically modeled using a stochastic differential equation (SDE) driven by Brownian motion. The diffusion component accounts for the gradual, random fluctuations observed in financial markets. Jump Component: The jump component in a jump diffusion model captures the sudden, discontinuous changes or "jumps" in the asset's price or other financial variables. Jumps occur due to unforeseen events, news releases, or significant market movements. The jump component is usually modeled as a Poisson process or a compound Poisson process, where the jump sizes and jump arrival times are stochastic. Stochastic Process: A jump diffusion model combines both the diffusion component and the jump component into a single stochastic process. The stochastic process incorporates both continuous and discontinuous dynamics, allowing for a more realistic representation of asset price movements. Jump Intensity and Jump Sizes: In a jump diffusion model, the jump intensity refers to the rate at which jumps occur, while the jump sizes represent the magnitude of the jumps. The jump intensity is typically modeled as a function of time or asset price volatility, and the jump sizes follow certain distributions, such as a normal or exponential distribution. Calibration and Estimation: Estimating the parameters of a jump diffusion model typically involves calibrating the model to historical data or using option prices. The calibration process involves finding the values of model parameters that best fit the observed market data, such as the asset's historical prices or option prices.
The Kelly criterion, named after the mathematician John L. Kelly Jr., is a formula used to determine the optimal allocation of capital in order to maximize long-term growth in a sequence of investments or bets. It provides a guideline for determining the size of each bet or investment based on the expected return and risk. The Kelly criterion is widely used in various fields, including gambling, trading, and investment management. It is expressed as a mathematical formula: f* = (bp - q) / b where: f* is the fraction of capital to be wagered or invested, b is the net odds received on the bet (the profit if the bet wins divided by the initial stake), p is the probability of winning, q is the probability of losing (1 - p). The key aspects of the Kelly criterion are as follows: The Kelly criterion is based on the expected value, which is the average return that can be anticipated from each bet or investment. It considers both the probability of winning and the magnitude of the potential gain or loss. The goal is to maximize the long-term growth rate of wealth. It aims to strike a balance between risk and reward, allowing for aggressive growth while managing the risk of ruin. The Kelly criterion suggests investing a fraction of capital that is proportional to the expected edge or advantage of the bet. It indicates that the optimal bet size is directly related to the perceived value and probability of success. While the Kelly criterion maximizes long-term growth, it is important to consider the risk of ruin or substantial losses. Aggressive use of the Kelly fraction can result in significant drawdowns or potential loss of capital, especially in situations with imperfect information or volatile markets. Variations and modifications have also been proposed to handle multiple investment choices, constraints, and practical considerations, such as transaction costs and portfolio diversification. It is essential to carefully consider the assumptions, limitations, and practical considerations when applying the Kelly criterion in real-world scenarios. Risk management, diversification, and individual risk preferences should also be taken into account alongside the Kelly criterion.
The LIBOR Market Model (LMM) is a mathematical model used to simulate and price interest rate derivatives, particularly those based on the London Interbank Offered Rate (LIBOR). It is a forward-rate-based model that aims to capture the dynamics of the yield curve and the evolution of forward interest rates over time. Key aspects of the LIBOR Market Model include: Forward Rates and LIBOR: The LMM represents the interest rate market using a set of forward rates, which are implied rates for future periods. LIBOR rates, which are widely used in the financial industry, are based on these forward rates. The model focuses on simulating and forecasting the future evolution of these forward rates. Monte Carlo Simulation: The LMM typically employs a Monte Carlo simulation method to generate multiple possible interest rate paths. Each path represents a possible realization of the future interest rate movements. The simulation incorporates random variables, such as normally distributed shocks or factors, to capture the uncertainty and randomness in interest rate movements. Stochastic Volatility: The LMM recognizes that interest rate volatility can vary over time. It introduces stochastic volatility components in the model to capture the fluctuations and changes in the volatility of forward rates. This allows for more realistic modeling of interest rate behavior and volatility smile effects observed in the market. Calibration: To use the LMM for pricing interest rate derivatives, the model needs to be calibrated to market data. Calibration involves adjusting the model's parameters to fit observed market prices of liquid interest rate instruments, such as interest rate swaps or bond options. The calibration process aims to minimize the difference between the model-generated prices and market prices. Pricing and Risk Management: Once the LMM is calibrated, it can be used to price a wide range of interest rate derivatives, including interest rate swaps, caps, floors, swaptions, and other complex structured products. The model provides the ability to value these instruments, calculate risk measures, and perform risk management activities associated with interest rate exposures. The LIBOR Market Model is widely used in quantitative finance for pricing and risk management of interest rate derivatives. Its ability to capture the dynamics of forward rates and incorporate stochastic volatility allows for more accurate modeling of interest rate behavior. However, it's important to note that the LMM is just one of several models used in interest rate modeling, and alternative models, such as the Heath-Jarrow-Morton (HJM) framework or the Hull-White model, may be more appropriate depending on the specific requirements and characteristics of the interest rate market.
Linear regression is a statistical modeling technique used in quantitative finance for various purposes, including asset pricing, risk management, portfolio management, and factor modeling to analyze and predict the relationship between two variables. It provides a way to understand the linear association between a dependent variable and one or more independent variables and allows for the estimation of a linear equation that best fits the data. The dependent variable (also called the response variable) is the variable being predicted or explained, while the independent variables (also known as explanatory variables or predictors) are used to explain or predict the dependent variable. In finance, the dependent variable can be, for example, a stock price, an asset return, or a financial indicator, and the independent variables can be factors like interest rates, economic indicators, or company-specific variables. Linear regression assumes a linear relationship between the dependent and independent variables. It assumes that changes in the dependent variable are directly proportional to changes in the independent variables, with a constant slope. The goal is to estimate the slope and intercept of the linear equation that best fits the observed data. Linear regression estimates the parameters of the linear equation using a method called ordinary least squares (OLS). OLS minimizes the sum of the squared differences between the observed values and the predicted values based on the linear equation. The estimated parameters represent the coefficients that determine the impact of the independent variables on the dependent variable. The coefficients in linear regression provide insights into the relationship between the independent variables and the dependent variable. They indicate the direction and magnitude of the impact of the independent variables on the dependent variable. Positive coefficients suggest a positive relationship, while negative coefficients suggest a negative relationship. Linear regression can be used for prediction by plugging in values for the independent variables into the estimated linear equation to estimate the corresponding value of the dependent variable. Additionally, various statistical measures, such as R-squared, adjusted R-squared, and significance tests, are used to evaluate the model's goodness of fit and the statistical significance of the estimated coefficients. Linear regression allows for the identification of relationships between financial variables, the prediction of future values, and the analysis of the impact of independent variables on the dependent variable. Linear regression is covered in more detail in module 4 of the CQF program.
Mathematical modeling in quantitative finance refers to the use of mathematical techniques and models to describe, analyze, and predict financial markets, instruments, and phenomena. It involves constructing mathematical representations of financial variables, relationships, and processes to gain insights, make predictions, and facilitate decision-making in the field of finance. It helps in pricing derivatives, developing trading strategies, risk management, and asset allocation. However, it's important to acknowledge that models are simplifications of complex reality and rely on assumptions, data quality, and ongoing validation and refinement to maintain their usefulness. Here are the key aspects of mathematical modeling in quantitative finance: Representation of Financial Variables: Mathematical modeling involves representing financial variables, such as asset prices, interest rates, volatility, and other market factors, using mathematical symbols and equations. These variables are often modeled as random processes or stochastic differential equations to capture their inherent uncertainty and randomness. Building Models: Mathematical models in quantitative finance can take various forms, including stochastic models, time series models, optimization models, and simulation models. These models are constructed based on the underlying assumptions and characteristics of the financial phenomena being studied. They may incorporate statistical techniques, differential equations, probability theory, optimization methods, and other mathematical tools. Understanding Relationships: Mathematical models enable the exploration and understanding of relationships between financial variables. They help identify dependencies, correlations, and causal relationships between market factors, asset prices, and other relevant financial quantities. Models provide a structured framework to analyze the impact of different factors on financial outcomes. Prediction and Forecasting: Mathematical models are utilized for prediction and forecasting in quantitative finance. By incorporating historical data, statistical techniques, and underlying market dynamics, models can generate forecasts of future market behavior, asset prices, or other financial quantities. These predictions help inform investment decisions, risk management strategies, and trading strategies. Risk Analysis and Management: Mathematical models are instrumental in assessing and managing financial risk. Models, such as value-at-risk (VaR) models, stress testing models, or credit risk models, quantify the potential losses and risks associated with various financial positions or portfolios. These models aid in the measurement, analysis, and mitigation of risk exposures. Model Calibration and Validation: Mathematical models require calibration to real-world data to ensure accuracy and relevance. Calibration involves estimating model parameters based on historical market data or observed market prices. Models are also subject to validation to assess their performance and evaluate their ability to capture the behavior of financial markets and instruments. Mathematical modeling is covered in more detail in module 4 of the CQF program.
Maximum Likelihood Estimation (MLE) is a statistical method used to estimate the parameters of a statistical model by maximizing the likelihood function. It is a widely used approach in quantitative finance and other fields of statistics, including parameter estimation in asset pricing models, option pricing models, risk management models, and econometric models. It allows for data-driven estimation of model parameters, enabling statistical inference, hypothesis testing, and predictive analysis. Within Maximum Likelihood Estimation, the likelihood function represents the probability of observing the given data as a function of the model parameters. It quantifies how well the model, with specific parameter values, explains the observed data. The goal of MLE is to find the parameter values that maximize the likelihood function. MLE aims to estimate the values of unknown parameters in a statistical model. By maximizing the likelihood function, MLE selects the parameter values that make the observed data most probable. The estimated parameter values are referred to as the maximum likelihood estimates. In practice, it is common to work with the log-likelihood function instead of the likelihood function. Taking the logarithm of the likelihood function simplifies the mathematical calculations, as the log transforms the product of probabilities into a sum of logarithms. Maximizing the likelihood (or log-likelihood) function typically involves solving an optimization problem. Various optimization techniques, such as gradient descent, Newton's method, or the Expectation-Maximization algorithm, can be used to find the maximum likelihood estimates. Under certain regularity conditions, the maximum likelihood estimates possess desirable statistical properties, such as consistency, asymptotic normality, and efficiency. Consistency means that as the sample size increases, the estimates converge to the true parameter values. Asymptotic normality implies that the estimates follow an approximately normal distribution as the sample size increases. It's important to note that the success of MLE relies on several assumptions, such as the correct specification of the statistical model, independence of observations, and absence of measurement errors. Careful consideration and diagnostic checks are necessary to ensure the validity of the MLE results. MLE is covered in more detail in module 4 of the CQF program.
The Merton model, also known as the Merton structural credit model, is a financial model developed by economist Robert C. Merton in 1974. It is used to assess and quantify the default risk of a company's debt or the probability of a company defaulting on its obligations. The model provides a framework for understanding the relationship between a company's asset value, its debt structure, and the likelihood of default. The Merton model is based on the assumption that a company's asset value follows a continuous stochastic process, typically modeled as a geometric Brownian motion. The company's liabilities are typically represented by its debt, which is assumed to be in the form of a zero-coupon bond. The key idea behind the model is that a company defaults when the value of its assets falls below the value of its debt. The model calculates the probability of default by comparing the distribution of the company's asset value at a specified future time (typically the maturity of the debt) with the face value of the debt. If the asset value is below the debt value, default is assumed to occur. The probability of default can be estimated using option pricing techniques, specifically the Black-Scholes formula, by treating default as analogous to the exercise of a put option. The Merton model has been influential in the field of credit risk modeling and has provided a foundation for subsequent developments in quantitative credit risk analysis. It is widely used by financial institutions and credit rating agencies to estimate the creditworthiness of corporations and the pricing of credit derivatives such as credit default swaps. The Merton model does make several assumptions, such as constant asset volatility, no transaction costs, and a single risk-free interest rate. Additionally, the model assumes that the company's capital structure and asset value process are known and can be modeled accurately. While the model has been influential, it is a simplification of real-world dynamics, and its assumptions may not hold in all circumstances. The Merton model is covered in more detail in module 6 of the CQF program.
Modern Portfolio Theory (MPT), developed by Nobel Laureate, Harry Markowitz, in the 1950s, is a framework for constructing optimal investment portfolios. MPT provides a mathematical approach to portfolio selection by considering the trade-off between risk and return. The key principles of Modern Portfolio Theory are as follows: Diversification: MPT emphasizes the importance of diversifying investments across different asset classes, such as stocks, bonds, and other financial instruments. Diversification helps reduce the overall risk of the portfolio by spreading investments across various assets with different risk and return characteristics. By combining assets that are not perfectly correlated, MPT seeks to achieve a more efficient risk-return trade-off. Efficient Frontier: MPT introduces the concept of the efficient frontier, which represents the set of portfolios that offer the highest expected return for a given level of risk or the lowest risk for a given level of expected return. The efficient frontier is obtained by combining assets in different proportions, considering their expected returns, volatilities, and correlations. Risk and Return Trade-off: MPT recognizes that investors are generally risk-averse and seek to maximize returns for a given level of risk or minimize risk for a given level of returns. MPT quantifies risk as the volatility or standard deviation of returns. It assumes that investors make decisions based on the expected returns and risk of the assets and aim to construct portfolios that maximize expected return while minimizing risk. Capital Asset Pricing Model (CAPM): MPT incorporates the Capital Asset Pricing Model, which estimates the expected return of an asset based on its systematic risk (beta) and the expected market return. CAPM helps determine the optimal allocation of assets in the portfolio based on their expected returns and risk contributions. Mean-Variance Optimization: MPT utilizes mean-variance optimization to find the optimal portfolio allocation. It aims to maximize the portfolio's expected return for a given level of risk or minimize the portfolio's risk for a given level of expected return. Mean-variance optimization involves considering the expected returns, volatilities, and correlations of the assets in the portfolio. MPT has significantly influenced portfolio management practices and the field of finance. It provides a framework for constructing diversified portfolios based on quantitative analysis and the consideration of risk and return characteristics. However, it's important to note that MPT has certain assumptions and limitations, such as the reliance on historical data, the assumption of normal distribution of asset returns, and the neglect of non-financial factors. As a result, some variations and extensions of MPT have been developed to address these limitations and incorporate additional factors. Modern Portfolio Theory is covered in more detail in module 2 of the CQF program.
Monte Carlo Simulation is a computational technique used to model and analyze complex systems or processes by generating random samples of input variables and observing their impact on the system's output. It is named after the Monte Carlo casino in Monaco, known for its games of chance. Monte Carlo Simulation is particularly useful in situations where analytical or closed-form solutions are not feasible, or when the system is influenced by multiple uncertain factors. It is applied in various fields, including finance, engineering, physics, economics, and risk analysis. Some specific applications include portfolio optimization, option pricing, project management, reliability analysis, and decision-making under uncertainty. The process of Monte Carlo Simulation involves the following steps: Define the Model: The first step is to establish a mathematical or computational model that represents the system under study. This model should include input variables (parameters) that affect the system's behavior and an output variable of interest. Define Probability Distributions: For each input variable, probability distributions are assigned to capture the uncertainty or variability associated with the variable. These distributions can be based on historical data, expert opinions, or assumptions. Generate Random Samples: Random samples are drawn from the probability distributions assigned to the input variables. The number of samples generated depends on the desired accuracy and precision of the simulation. Run the Model: For each set of randomly generated input values, the model is executed to compute the corresponding output. The model may involve mathematical equations, simulations, or other computational methods. Analyze the Results: The generated output values from multiple simulations are collected and analyzed to understand the system's behavior, assess risk, or make predictions. Statistical analysis techniques are often employed to summarize and interpret the results. Monte Carlo Simulation allows decision-makers to gain insights into the range of possible outcomes, quantify risks, and evaluate different strategies or scenarios. It provides a powerful tool for decision-making under uncertainty and aids in understanding the behavior and potential outcomes of complex systems. Monte Carlo Simulation is covered in more detail in module 3 of the CQF program.
A Naïve Bayes classifier is a probabilistic machine learning algorithm that is based on Bayes' theorem and assumes independence between the features (hence the term "naïve"). It is widely used for classification tasks, particularly in natural language processing, text classification, and spam filtering. The Naïve Bayes classifier calculates the probability of a given instance belonging to a particular class based on the probabilities of its features. It assumes that the presence or absence of each feature is independent of the presence or absence of other features, which simplifies the calculations. On a top-level, a Naïve Bayes classifier works in the following way: Training Phase: During the training phase, the classifier learns the probabilities of different features given each class from a labeled dataset. It calculates the prior probability of each class and the conditional probability of each feature given the class. Feature Independence Assumption: The Naïve Bayes classifier assumes that the features are conditionally independent given the class label. This assumption allows the classifier to calculate the probability of an instance belonging to a particular class by multiplying the conditional probabilities of its features given that class. Classification Phase: In the classification phase, the classifier uses the learned probabilities to assign a class label to a new, unseen instance. It calculates the posterior probability of each class given the instance's features using Bayes' theorem. The class with the highest posterior probability is selected as the predicted class. The Naïve Bayes classifier is efficient, easy to implement, and performs well on large datasets. However, its independence assumption may not hold in all cases, leading to suboptimal results when the features are highly correlated. Despite this limitation, Naïve Bayes classifiers are still widely used due to their simplicity and effectiveness, particularly in text-based classification tasks. There are different variants of Naïve Bayes classifiers, such as Gaussian Naïve Bayes (for continuous numerical features), Multinomial Naïve Bayes (for discrete features), and Bernoulli Naïve Bayes (for binary features). These variants handle different types of data and are adapted to specific application domains. Naïve Bayes classifiers are covered in more detail in module 4 of the CQF program.
Natural Language Processing (NLP) is a field of artificial intelligence (AI) that focuses on the interaction between computers and human language. It involves the development of algorithms and models to enable computers to understand, interpret, and generate human language in a way that is meaningful and useful. In quantitative finance, NLP is used to extract insights and information from textual data sources such as news articles, financial reports, social media posts, and regulatory filings. It relies on various techniques such as text preprocessing, tokenization, part-of-speech tagging, named entity recognition, sentiment analysis, topic modeling, and machine learning algorithms for classification or regression tasks. Below are just some ways NLP is applied in quantitative finance: Sentiment Analysis: NLP techniques are used to analyze the sentiment expressed in financial news and social media data. By assessing the sentiment of market participants, sentiment analysis can provide insights into market sentiment and help predict market movements. Positive or negative sentiment can be used as a feature in quantitative models for trading strategies or risk management. News Analysis and Event Detection: NLP enables the extraction of key information from news articles and other textual sources. It can help identify important events such as mergers and acquisitions, earnings announcements, or regulatory changes. By processing news data, NLP algorithms can generate signals or triggers for trading strategies and help understand the impact of news on financial markets. Textual Data Mining: NLP techniques facilitate the mining of large volumes of textual data for patterns, relationships, and insights. By analyzing financial reports, research articles, and market commentaries, NLP algorithms can uncover hidden information, detect anomalies, or identify patterns that may impact investment decisions or risk assessment. Information Extraction: NLP algorithms are used to extract structured information from unstructured textual data. This includes extracting key entities such as company names, financial figures, dates, or other relevant information from financial reports, SEC filings, or news articles. Extracted information can be used for quantitative analysis, building financial models, or risk assessment. Question-Answering Systems: NLP techniques are employed to develop question-answering systems that can understand and respond to natural language queries about financial data, market trends, or specific investments. These systems provide users with quick access to relevant information and facilitate decision-making processes. By leveraging NLP, quantitative finance practitioners can gain insights from unstructured textual data, augment quantitative models with textual information, and make more informed investment decisions. NLP complements traditional quantitative techniques and helps bridge the gap between unstructured data and quantitative analysis. NLP is covered in more detail in module 4 of the CQF program.
Option-Adjusted Spread (OAS) is a financial metric used to assess the additional yield investors demand for taking on the risk associated with an option-embedded security, typically bonds or mortgage-backed securities (MBS). It quantifies the spread over a risk-free interest rate that compensates investors for the uncertainty and potential cash flow variations resulting from embedded options. To calculate the OAS, the expected cash flows of the security are estimated, considering both scheduled payments and potential cash flows from option exercise. These cash flows are then discounted using a risk-free interest rate, and the security's present value is determined. The option-free value is derived by removing the value associated with the embedded options, and the OAS is obtained by subtracting the risk-free yield from the yield of the option-free value. The OAS provides a means to compare securities with different option characteristics and assess their relative value and risk-return trade-offs. A positive OAS implies a higher yield than a risk-free security, compensating investors for the additional risk, while a negative OAS indicates a lower yield. However, it is crucial to consider other factors such as credit risk and market conditions when evaluating securities. While OAS aids in evaluating option-embedded securities, it relies on certain assumptions and models that may not capture all market dynamics or risks. Therefore, it should be used alongside other measures and considered in the context of a comprehensive analysis of the security and its associated risks.
Put-Call Parity is a fundamental concept in options pricing theory that establishes a relationship between the prices of call options and put options with the same underlying asset, strike price, and expiration date. It is based on the principle that the value of a call option plus the present value of the strike price equals the value of a put option plus the current price of the underlying asset. The put-call parity equation can be expressed as follows: C + PV(K) = P + S Where: C is the price of the call option PV(K) is the present value of the strike price (K) discounted to the present time P is the price of the put option S is the current price of the underlying asset The put-call parity equation holds under the assumptions of no arbitrage and efficient markets. It implies that the combination of a long call option and a short put option, both with the same strike price and expiration date, should have the same value as holding the underlying asset. Put-call parity has several implications and applications in options trading and risk management. It allows for the determination of the theoretical prices of options based on the prices of other options and the underlying asset. Any deviation from put-call parity could create arbitrage opportunities, where traders can exploit mispricing by executing a combination of trades to make risk-free profits. Put-call parity is also used for options pricing models, such as the Black-Scholes model, as it provides a consistency check on the model's assumptions and output. It is a fundamental concept for options traders and analysts, helping them assess the fair value of options and construct hedging or trading strategies. However, it's important to note that put-call parity assumes ideal market conditions, including no transaction costs, no restrictions on short selling, no dividend payments, and no market frictions. Deviations from these assumptions or the presence of market imperfections can lead to temporary violations of put-call parity. Traders closely monitor such deviations and take advantage of potential arbitrage opportunities to restore equilibrium.
Statistical arbitrage is a popular quantitative trading strategy used in quant finance. It involves exploiting pricing discrepancies or deviations from expected statistical relationships between related securities or financial instruments. The basic premise of statistical arbitrage is that certain relationships between securities tend to revert to their mean or exhibit predictable patterns over time. Quants develop mathematical models and algorithms to identify these relationships and estimate the expected behavior of the securities involved. Here's a quick overview of how statistical arbitrage works: Pair Selection: Quants select a pair (or sometimes a group) of related securities that they believe exhibit a statistical relationship. This relationship can be based on factors such as historical price patterns, correlation analysis, or fundamental characteristics. Model Development: A quantitative model is developed to estimate the expected behavior of the selected pair of securities. This model can be based on statistical techniques, time series analysis, machine learning algorithms, or other quantitative methods. Deviation Detection: The model continuously monitors the prices or other relevant indicators of the selected securities. When a deviation from the expected relationship is detected, a trading signal is generated. The deviation can be measured by statistical metrics such as z-scores, moving averages, or other quantitative indicators. Trade Execution: When a trading signal is generated, the strategy triggers trades to profit from the expected convergence or divergence of prices. The strategy can involve buying one security and simultaneously selling the other in the pair, aiming to capture the price discrepancy. Risk Management: As with any trading strategy, risk management is crucial. Statistical arbitrage strategies often employ risk controls, such as stop-loss orders, position sizing rules, and portfolio diversification, to manage the potential downside risks. It's important to note that statistical arbitrage strategies are typically executed with high-speed trading systems and rely on the ability to process large volumes of data in real-time. Quants continuously refine their models and strategies to adapt to changing market conditions and to stay ahead of the competition. These strategies can be applied to various financial instruments, such as stocks, futures, options, or currencies. Statistical arbitrage is covered in more detail in module 6 of the CQF program.
Supervised learning techniques are a class of machine learning algorithms that learn from labeled training data to make predictions or classify new, unseen data points. In supervised learning, the algorithm is provided with input-output pairs, where the input data is accompanied by corresponding labels or target values. Below are some commonly used supervised learning techniques: Regression: Regression algorithms are used for predicting continuous numeric values. The algorithm learns the relationship between the input variables and the continuous target variable to make predictions. Examples include Linear Regression, Decision Trees, Random Forests, and Support Vector Regression (SVR). Classification: Classification algorithms are used to assign categorical labels or class memberships to new instances based on training data. The algorithm learns the patterns and relationships in the training data to classify new data points. Popular classification algorithms include Logistic Regression, Decision Trees, Random Forests, Naïve Bayes, and Support Vector Machines (SVM). Ensemble Methods: Ensemble methods combine multiple base models to make more accurate predictions. They leverage the wisdom of crowds by aggregating the predictions of individual models. Examples of ensemble methods include Bagging (e.g., Random Forests), Boosting (e.g., AdaBoost, Gradient Boosting Machines), and Stacking. Neural Networks: Neural networks are a class of models inspired by the structure and functioning of the human brain. They consist of interconnected nodes (neurons) organized into layers. Neural networks are powerful for modeling complex relationships and are used in applications such as image recognition, natural language processing, and time series analysis. Instance-based Learning: Instance-based learning, also known as lazy learning, focuses on storing and retrieving training instances to make predictions. Algorithms like k-Nearest Neighbors (k-NN) classify new instances by finding the k nearest neighbors in the training data. Supervised learning techniques are used for tasks such as predicting stock prices and sentiment analysis. To apply supervised learning effectively, it is important to have high-quality labeled training data, properly preprocess the data, select appropriate features, and tune the algorithm's hyperparameters. Additionally, regular model evaluation and validation are crucial to ensure the model's accuracy and generalization performance. Supervised learning techniques are covered in more detail in module 4 of the CQF program.
Support Vector Machines (SVMs) are supervised machine learning models that are widely used for classification and regression tasks. SVMs are particularly effective in dealing with high-dimensional and complex datasets. The key idea behind SVMs is to find an optimal hyperplane that separates the data points of different classes with the maximum margin. The hyperplane is defined as the decision boundary that maximizes the distance between the closest data points of different classes, called support vectors. SVMs aim to achieve both good classification performance and robustness to new data. Key aspects of Support Vector Machines are: Linear and Nonlinear Classification: SVMs can perform linear classification by finding a hyperplane that separates the data points. They can also handle nonlinear classification by using kernel functions that map the data into a higher-dimensional feature space, where a linear decision boundary can be found. Margin Maximization: SVMs seek to maximize the margin, which is the distance between the decision boundary and the support vectors. By maximizing the margin, SVMs promote generalization and help avoid overfitting, leading to better classification performance on new, unseen data. Kernel Functions: Kernel functions allow SVMs to efficiently operate in high-dimensional feature spaces. They implicitly map the data to a higher-dimensional space, avoiding the need to explicitly compute the transformations. Popular kernel functions include linear, polynomial, radial basis function (RBF), and sigmoid kernels. C-parameter and Soft Margin: SVMs introduce a regularization parameter, C, which controls the trade-off between the margin width and the training errors. A smaller C allows more errors but wider margins, while a larger C reduces the margin but allows fewer errors. This parameter helps balance model complexity and generalization. Support Vector Regression: In addition to classification, SVMs can also be used for regression tasks. Support Vector Regression (SVR) aims to find a regression function that lies within a specified margin of the training data points. It seeks to fit the data while limiting the deviation from the true function. Support Vector Machines have several advantages, including their ability to handle high-dimensional data, resilience to overfitting, and effective handling of nonlinear relationships. However, SVMs can be sensitive to the choice of parameters and can be computationally expensive for large datasets. To use SVMs effectively, it is important to carefully select the appropriate kernel function and tune the hyperparameters, such as the C-parameter and kernel parameters. Additionally, preprocessing the data and addressing class imbalances can also impact the performance of SVM models. Support Vector Machines are covered in more detail in module 4 of the CQF program.
The Taylor series is a mathematical representation of a function as an infinite sum of terms. It allows us to approximate a function using its derivatives at a particular point. The series is named after the mathematician Brook Taylor, who introduced it in the 18th century. The Taylor series expansion of a function f(x) around a point a is given by: f(x) = f(a) + f'(a)(x-a)/1! + f''(a)(x-a)²/2! + f'''(a)(x-a)³/3! + ... Each term in the series corresponds to a derivative of the function evaluated at the point a, multiplied by powers of the difference between x and a, divided by the factorial of the order of the derivative. The terms capture the local behavior of the function at the point a. The series is often truncated after a certain number of terms to create an approximation of the function. The more terms included, the closer the approximation is to the original function. The Taylor series expansion is valuable in calculus and mathematical analysis. It provides a way to represent functions that may be difficult to work with directly. It allows for the estimation of function values beyond the range of known values and aids in the understanding of the behavior of functions near a particular point. In quantitative finance, the Taylor series is used in various ways to approximate and analyze financial functions and models, such as: Option Pricing Models: The Taylor series expansion is employed to approximate option pricing models, such as the Black-Scholes model. By expanding the model's equations using the Taylor series, it is possible to derive simpler approximations or closed-form solutions for pricing options. These approximations can help in quickly estimating option prices and Greeks (sensitivity measures) without relying on complex numerical methods. Numerical Methods: The Taylor series is utilized in numerical methods to approximate financial derivatives, such as option sensitivities (e.g., delta, gamma, vega). By approximating the derivative using the Taylor series expansion, numerical techniques like finite difference methods can be employed to calculate sensitivities accurately and efficiently. Risk Management Models: The Taylor series is incorporated into risk management models, such as risk factor models or stress testing frameworks. By expanding the models using the Taylor series, the impact of changes in risk factors on portfolio risk can be analyzed. This enables the assessment of potential losses under different scenarios or shocks. It's important to note that the Taylor series approximations are most effective for small deviations from the expansion point and may introduce errors as the deviation increases. Careful consideration and validation are necessary when employing Taylor series approximations in quantitative finance to ensure their accuracy and reliability. The Taylor series is covered in more detail in module 1 of the CQF program.
A time series model is a statistical model used to analyze and predict the behavior of a sequence of data points collected over time. It assumes that the values of the data points are dependent on previous values, and the goal is to capture the underlying patterns, trends, and relationships in the time series data. In quantitative finance, time series models are extensively used for various applications: Forecasting: Time series models enable the prediction of future values based on historical data. By analyzing the patterns and trends in the time series, models like Autoregressive Integrated Moving Average (ARIMA), Exponential Smoothing (ETS), or Seasonal ARIMA (SARIMA) can generate forecasts. These forecasts aid in market analysis, asset pricing, portfolio optimization, and risk management. Risk Management: Time series models play a crucial role in risk management. Techniques such as GARCH (Generalized Autoregressive Conditional Heteroskedasticity) models help estimate and forecast the volatility of financial assets, which is vital for measuring market risk, pricing derivatives, and constructing risk management strategies. Market Analysis: Time series models assist in analyzing financial market dynamics. They help identify patterns, trends, and cycles in market data. Models like the Random Walk or Brownian Motion are used to test the efficiency of financial markets and evaluate the predictability of asset prices. Trading Strategies: Time series models are employed to develop quantitative trading strategies. These strategies involve analyzing historical price and volume data to generate signals for buying or selling assets. Technical analysis indicators, such as moving averages, oscillators, or momentum indicators, are often incorporated into time series models for trading decision-making. Event Studies: Time series models are utilized in event studies to analyze the impact of specific events or news on financial markets. By comparing the behavior of the time series around an event, it is possible to assess the event's effect on asset prices, trading volume, or other market variables. It's important to note that time series models rely on assumptions about the underlying data and often require careful consideration of factors such as stationarity, seasonality, autocorrelation, and the appropriate choice of model parameters. Model selection, estimation, and validation are crucial steps in time series analysis to ensure the reliability and accuracy of the results. Overall, time series models provide a quantitative framework for understanding and predicting the behavior of financial markets, asset prices, and other time-dependent variables in quantitative finance. They help uncover patterns, estimate future values, and support decision-making processes in various financial applications. Time series models are covered in more detail in module 1 of the CQF program.
Uniform Manifold Approximation and Projection (UMAP) is a dimensionality reduction technique used in machine learning and data analysis. It aims to preserve the global structure and relationships of the data by mapping high-dimensional data points onto a lower-dimensional space. UMAP is particularly effective at capturing complex patterns and non-linear relationships in the data. UMAP is based on the concept of preserving local and global distances. It starts by constructing a weighted graph representation of the data, where each data point is connected to its nearest neighbors. The weights of the edges represent the similarities between the data points. The algorithm then optimizes the embedding of the data points in the lower-dimensional space, seeking to preserve the topological structure of the original data. It achieves this by minimizing the discrepancy between pairwise distances in the high-dimensional and low-dimensional spaces. By iteratively optimizing the embedding, UMAP uncovers the underlying geometric relationships among the data points. UMAP is known for its flexibility, scalability, and ability to capture both global and local structures. It can handle large datasets efficiently and is robust to various types of data, including numerical, categorical, or mixed data. UMAP is also computationally efficient, making it suitable for exploratory data analysis and visualization tasks. The resulting low-dimensional representation obtained by UMAP can be used for data visualization, clustering, anomaly detection, and other downstream tasks. UMAP has gained popularity as a powerful alternative to other dimensionality reduction techniques, such as t-SNE and PCA, due to its ability to preserve more global structures while maintaining local relationships. It's important to note that UMAP, like other dimensionality reduction techniques, relies on parameter settings and data characteristics. Proper parameter tuning and interpretation of the results are necessary to ensure meaningful and reliable insights from the reduced-dimensional representation. UMAP is covered in more detail in module 5 of the CQF program.
Unsupervised learning techniques are a subset of machine learning algorithms used to discover patterns, structures, or relationships in unlabeled data without explicit guidance or predefined outcomes. Unlike supervised learning, where labeled examples are provided for training, unsupervised learning aims to find inherent structures or patterns within the data itself. Commonly used unsupervised learning techniques include: Clustering: Clustering algorithms group similar data points together based on their intrinsic characteristics. They aim to identify clusters or subgroups within the data. Popular clustering algorithms include k-means, hierarchical clustering, and density-based clustering (e.g., DBSCAN). Dimensionality Reduction: These techniques reduce the number of input variables while retaining the essential information. Dimensionality reduction methods, such as Principal Component Analysis (PCA) and t-SNE (t-distributed Stochastic Neighbor Embedding), transform high-dimensional data into a lower-dimensional space, simplifying the representation of the data. Anomaly Detection: Anomaly detection algorithms identify unusual or abnormal data points that deviate significantly from the majority of the data. These techniques are useful for detecting outliers, fraud, or rare events. Examples include Gaussian Mixture Models (GMMs), Isolation Forest, and Local Outlier Factor (LOF). Association Rule Mining: This technique discovers interesting relationships or associations between variables in the data. It identifies frequently occurring patterns or item sets in transactional data. The Apriori algorithm is a well-known approach for mining association rules. Generative Models: Generative models learn the underlying probability distribution of the data and can generate new samples similar to the training data. Examples include Gaussian Mixture Models (GMMs), Hidden Markov Models (HMMs), and Generative Adversarial Networks (GANs). Unsupervised learning techniques have various applications, including customer segmentation, anomaly detection, recommender systems, data preprocessing, and exploratory data analysis. They enable insights and discoveries in large and complex datasets where the underlying patterns or structures are not explicitly known. However, the interpretation and evaluation of unsupervised learning results can be more challenging than in supervised learning, as there are no ground truth labels to compare against. Unsupervised learning techniques are covered in more detail in module 5 of the CQF program.
Value at Risk (VaR) - not to be confused with VAR - is a widely used risk measurement and management tool in quantitative finance. It quantifies the potential loss that an investment or portfolio may face within a given confidence level and time horizon. VaR provides a single number that represents the maximum expected loss under normal market conditions. VaR is typically defined as the maximum loss that an investment or portfolio may experience with a specified probability over a specific time period. For example, a 1-day 95% VaR of $1 million implies that there is a 5% chance that the portfolio will lose more than $1 million within a single trading day. VaR can be calculated using different statistical methods, including historical simulation, variance-covariance (parametric) approach, or Monte Carlo simulation. Each method has its own assumptions and limitations. Historical simulation uses past data to estimate the potential losses, while the parametric approach assumes a specific distribution for asset returns. Monte Carlo simulation generates random scenarios based on specified assumptions to estimate potential losses. VaR allows risk managers and traders to understand and quantify the downside risk of their portfolios, aiding in decision-making, risk control, and setting risk limits. It provides a concise measure of risk that can be compared across different assets, portfolios, or trading strategies. VaR is also a key component in regulatory frameworks, such as Basel III, which require financial institutions to hold capital reserves based on their VaR estimates. It's important to note that VaR has some limitations. It assumes that historical relationships and market conditions will persist in the future, and it may not capture extreme events or tail risk adequately. Additionally, VaR does not provide information about the magnitude of losses beyond the specified level, and it does not consider potential gains. VaR should be used in conjunction with other risk measures and stress testing techniques to obtain a more comprehensive view of risk. VaR is covered in more detail in module 2 of the CQF program.
The Vector Autoregression (VAR) model is a statistical model used to analyze the dynamic relationships among multiple time series variables. It allows for the examination of interdependencies and feedback effects between the variables. In a VAR model, each variable in the system is regressed on its own lagged values as well as the lagged values of the other variables. This captures the autoregressive nature of the variables and allows for the exploration of their mutual interactions. The model assumes that each variable is influenced by its own past values, the past values of other variables, and potentially exogenous shocks. VAR models enable the analysis of Granger causality, which assesses the causal relationships between variables. By examining the significance of lagged values of one variable in explaining the current value of another variable, Granger causality helps identify the direction and strength of causality. The determination of the appropriate lag order is essential in VAR modeling. Various criteria, such as AIC or BIC, assist in selecting the optimal number of lagged terms to include in the model. Impulse response functions are employed to understand the dynamic response of each variable to a one-time shock in another variable. They provide insights into the transmission and persistence of shocks among the variables. Estimating a VAR model involves estimating the coefficients using techniques like OLS or MLE. Inference and hypothesis testing are conducted to assess the significance of coefficients and evaluate the model's goodness of fit. VAR models find applications in economic forecasting, macroeconomic analysis, policy evaluation, financial risk management, and more. They provide a flexible framework for analyzing the interactions and dynamic behavior of multiple time series variables, helping to understand the complex relationships in a system and make informed decisions. VAR models are covered in more detail in module 6 of the CQF program.
The Vector Error Correction Model (VECM) is an econometric model used to analyze the long-term equilibrium relationship and short-term dynamics between multiple time series variables. It extends the Autoregressive Moving Average (ARMA) model to account for both the short-run deviations from equilibrium and the long-run equilibrium relationship among the variables. VECM has various applications in economics, finance, and time series analysis. It is widely used to analyze relationships among economic variables, such as exchange rates, interest rates, GDP components, and asset prices. Here are the key aspects of the Vector Error Correction Model: Cointegration: VECM is employed when the time series variables under consideration are cointegrated. Cointegration occurs when there is a long-term equilibrium relationship among the variables, even though they may exhibit short-term deviations from this equilibrium. It is a useful concept for analyzing non-stationary variables that have a stable long-term relationship. Error Correction Term: VECM includes an error correction term that adjusts for short-term deviations from the long-run equilibrium relationship. This term captures the speed at which the variables adjust back to equilibrium after a shock or disturbance. It reflects the idea that any deviation from equilibrium will be corrected over time. Stationarity and Differencing: VECM typically operates on differenced variables to ensure stationarity. By differencing the variables, non-stationary components are removed, allowing for the analysis of the stationary residual series. Differencing also facilitates the interpretation of the error correction term, which represents the adjustment process towards equilibrium. Estimation and Inference: Estimating a VECM involves estimating the parameters through maximum likelihood or least squares methods. Hypothesis testing and inference are conducted based on standard statistical tests, such as t-tests, F-tests, or likelihood ratio tests, to assess the significance of coefficients, test for cointegration, and examine the stability of the model. Granger Causality and Forecasting: VECM enables the analysis of Granger causality, which examines the causal relationships among the variables. It helps determine the direction and significance of the relationships between variables in both the short and long run. VECM can also be utilized for forecasting future values of the variables based on the estimated model parameters. VECM is covered in more detail in module 6 of the CQF program.
Volatility is a fundamental concept in finance that captures the degree of variation or fluctuation in the price or value of a financial instrument. It measures the speed and magnitude of price changes over a specific period of time. High volatility implies larger price swings and greater uncertainty, while low volatility suggests more stable and predictable price behavior. Volatility plays a crucial role in several aspects of finance. Investors and traders closely monitor volatility to assess the potential risks and returns associated with investments. It helps in determining appropriate strategies, managing portfolio risk, and setting expectations for future price movements. Volatility can be quantified using various statistical measures. One commonly used measure is standard deviation, which calculates the dispersion of returns around the mean. Implied volatility, derived from options prices, reflects the market's expectations of future volatility. Historical volatility is calculated based on past price movements. Volatility can be influenced by various factors, including economic indicators, news events, market sentiment, and investor behavior. Certain events, such as economic crises or major geopolitical developments, can lead to significant increases in volatility. Volatility is not inherently good or bad; it simply represents the level of uncertainty and risk in the market. Some traders and investors actively seek high-volatility environments as they present opportunities for profit. However, higher volatility also entails greater potential for losses. Understanding and managing volatility is essential for effective risk management. Diversification, hedging strategies, and the use of derivatives are common techniques employed to mitigate the impact of volatility on portfolios. Volatility is covered in more detail in module 2 of the CQF program.
Volatility arbitrage is a trading strategy that aims to exploit discrepancies in implied or realized volatility across different financial instruments. It involves taking positions that profit from expected changes in volatility levels rather than directional movements in the underlying asset price. Volatility refers to the degree of fluctuation or variability in the price of an asset or market. It can be measured using various techniques, such as implied volatility derived from options prices or realized volatility calculated from historical price movements. Volatility arbitrage seeks to identify situations where the implied or realized volatility of an asset differs from its expected or historical levels. This discrepancy can arise due to market expectations, supply and demand imbalances, or mispricing. Volatility arbitrage strategies can take different forms, including: Volatility Spreads: This strategy involves taking offsetting positions in options or other derivatives to capture the difference in implied volatility between different strike prices or expiration dates. For example, a trader may sell overpriced options with high implied volatility and buy underpriced options with low implied volatility. Dispersion Trading: Dispersion trading involves trading the spread between the implied volatilities of different assets within the same market or sector. The strategy takes advantage of the expected convergence or divergence of volatilities among related assets, such as index components or correlated stocks. Variance Swaps: Variance swaps are derivative contracts that allow investors to trade the volatility of an underlying asset. Volatility arbitrageurs may take positions in variance swaps to profit from expected differences between realized volatility and implied volatility. Volatility arbitrage strategies often involve complex positions and exposure to various sources of risk, including directional risk, volatility risk, and correlation risk. Effective risk management techniques, such as proper hedging, position sizing, and portfolio diversification, are crucial to mitigate potential losses. It also relies on the assumption that market participants do not accurately price volatility or that the market will correct pricing discrepancies over time. However, due to competition and market efficiency, opportunities for pure volatility arbitrage may be limited and short-lived. Traders need to continuously monitor and adapt their strategies to changing market conditions. Volatility arbitrage is covered in more detail in module 3 of the CQF program.
Ready to learn the latest quant finance and machine learning techniques? Download a brochure today to discover how the CQF could help you gain the skills you need to go further in your career.
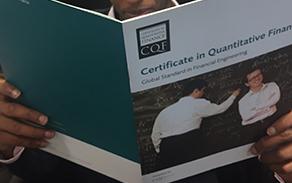
Contact our team
- Download a Brochure >
- Watch a Sample Lecture >
- Join an Information Session >
- Explore the Careers Guide >
- Learning Portal
Which program are you applying to?
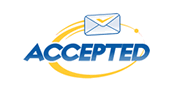
Accepted Admissions Blog
Everything you need to know to get Accepted
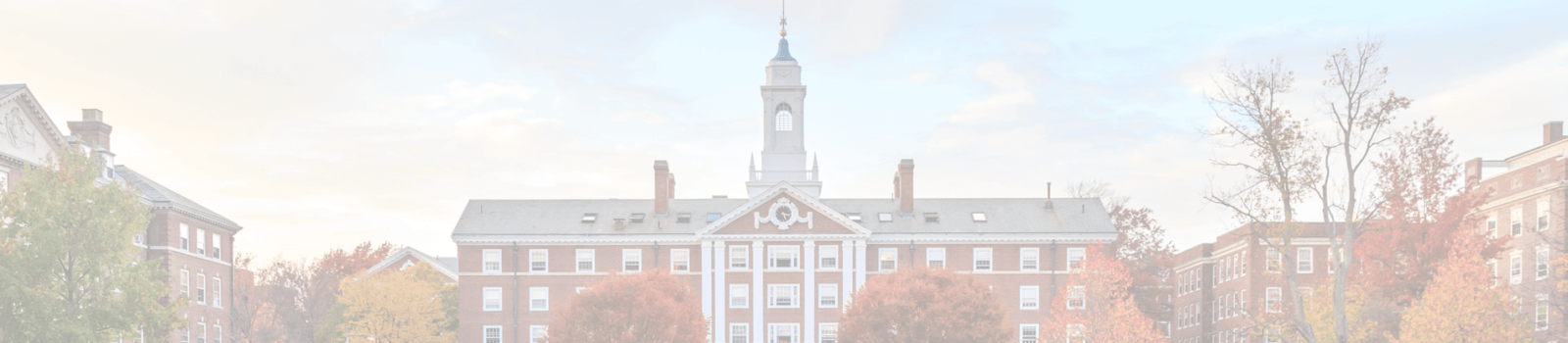
October 1, 2020
MIT Sloan Master of Finance Application Essay Questions, Tips & Deadlines [2020 – 2021]
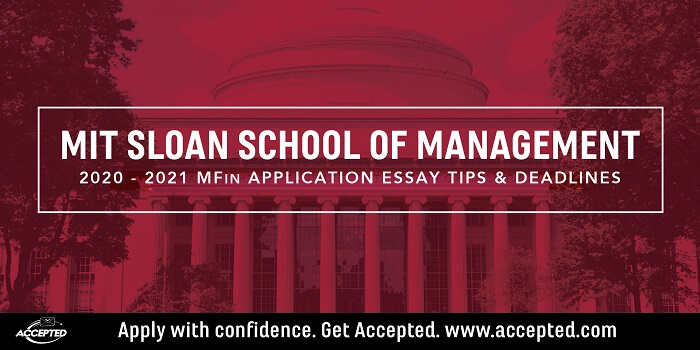
The MIT Sloan Master o f Finance program is significantly influenced by being part of both the Sloan School of Management and MIT more broadly. The culture and values of those two entities infuse the MFin program. Yes, being a quant geek helps, but the program’s adcom wants more: people who have a distinctive perspective, a sense of purpose, curiosity about and interest in using their quant chops to make a difference and build a better future, and the capacity become a leader within their area of finance. The written essays and video essays together will draw out those qualities and help the adcom identify the applicants who best fit the program.
MIT Sloan MFin 2020-21 application essays and video questions
Using relevant, verifiable details, such as awards, rankings, media references, etc., please respond to the three short answer questions below. ( Each response should be 200 words or less. )
MIT Sloan MFin essay #1
Please discuss past academic and professional experiences and accomplishments that will help you succeed in the Master of Finance program. Include achievements in finance, math, statistics, and computer sciences, as applicable.
The challenge here is to avoid being merely redundant of your resume and transcript. And the solution is to identify accomplishments and achievements that are meaningful to you in some way AND will be relevant to your future path. Rather than listing, say 5 or 6 items, present 3-4 and (for at least 1-2) succinctly explain their importance to you – the quality of your insight is what will set this essay apart and show you to be an engaged, appealing candidate.
MIT Sloan MFin essay #2
Describe your short-term and long-term professional goals. How will our MFin degree help you achieve these goals?
Be specific for your short-term goals : type of position, industry, what special experiences you’ll bring to the role (beyond an MIT MFin), possibly geography – and perhaps most important, WHY you want to do that role. Longer-term goals can be less specific and detailed, but motivation should be clear. Of course, link specific elements and qualities of the MFin program to your goals – it may seem obvious how it will help you, but you need a thoughtful discussion. This essay is core to portraying your fit with the program in terms of making productive use of its content and resources.
MIT Sloan MFin essay #3
Please share personal qualities that will enable you to contribute to the advancement of our mission.
This essay is the other part of “fit ” – where you will show that you understand and align with MIT and the program in terms of culture and values. Again, avoid lists of qualities. Select 2-3 qualities that enable you to contribute to the mission as indicated and present those qualities via anecdote – MIT is always interested in what you’ve actually done ; just “talk” doesn’t convey credibility. An anecdote can be as short as a sentence sometimes (“When ABC happened, I did DEF, and realized GHI, which gave me the courage to XYZ…”). And, please, avoid using qualities that don’t really add to the picture, like strong analytic skills, hard worker – the adcom knows that already!
MIT Sloan MFin video question #1
Please upload a one-minute (60 second) video introducing yourself to your future classmates and letting us know why you are interested in the finance industry.
The video should be a single take (no editing) and lasting no more than one minute, consisting of you speaking directly to the camera. You should not use background music or subtitles.
Don’t feel the need to be clever or funny or dramatic . Start with a brief intro – where are you from? What are you doing now? Then tell a story – the story of how you became interested in the finance industry. Include a setting – when, where – and share that first spark of interest. Also, the question says “finance industry” but that is too broad for the video purposes; be more specific, i.e. what aspect of finance or finance industry. Are you interested in Investment Banking, Quantitative Finance, Corporate Finance, PE, VC, something else? Public Investment? Research? Delineate your interest in the specific area in the video. That will be both more credible and more interesting than “finance” broadly.
For process: write a script, practice a few times to feel natural , and test out the visuals. Then go for it!
MIT Sloan MFin video question #2
All MFin applicants must submit a brief video statement in response to a simple, open-ended general interest question. The question is designed to help us get to know you better; to see how you express yourself and to assess fit with the MIT Sloan culture. It does not require prior preparation and will not be a technical question. The Video Question 2 is a part of your required application materials and will appear as a page within the application, once the other parts of your application are completed.
Each applicant receives a randomly generated question and has one minute to prepare a 60-second response .
This is more like an interview, and the best way to prepare is simply to practice random questions in front of a video camera with the same specs, i.e., 1-minute prep time. The aim is to practice enough so that generating a strong, engaging reply is second nature by the time you do it for real .
Get professional guidance with your MIT MiF application! Check out Accepted’s MiF Application Packages , which include advising, editing, interview coaching, and a resume edit for the MIT MiF application.
MIT Sloan Master of Finance 2020 – 2021 application deadline
Source: MIT Sloan Master’s in Finance website
***Disclaimer: Information is subject to change. Please check with individual programs to verify the essay questions, instructions and deadlines.***
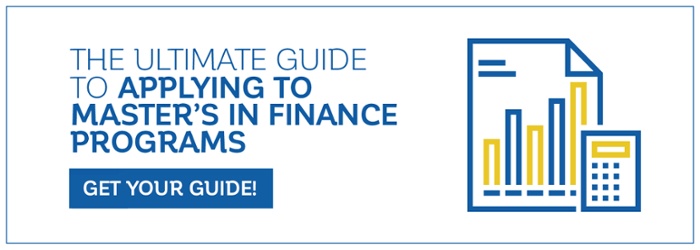
Related Resources:
- 5 Fatal Flaws to Avoid in Your Application Essays , a free guide
- How to Get Accepted to Master’s in Finance Programs
- A Glimpse into the MIT MFin Experience of an International Student
About Us Press Room Contact Us Podcast Accepted Blog Privacy Policy Website Terms of Use Disclaimer Client Terms of Service
Accepted 1171 S. Robertson Blvd. #140 Los Angeles CA 90035 +1 (310) 815-9553 © 2022 Accepted

404 Not found
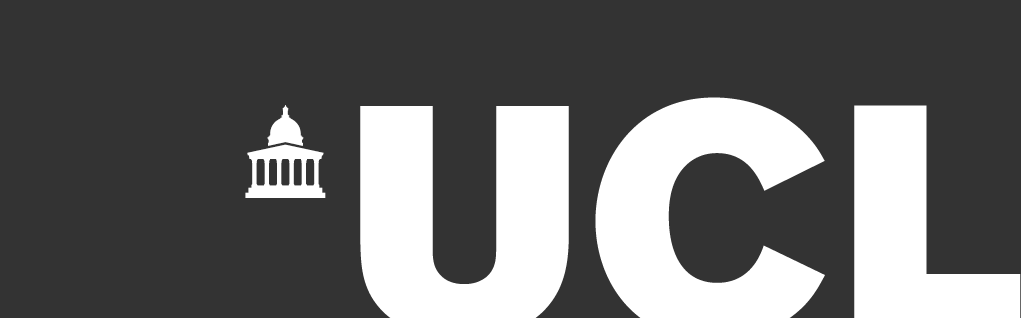
Computational Finance MSc
London, Bloomsbury
The rising sophistication of the financial sector is bringing a great demand for experts with skills across mathematics, finance, statistics and computer science. The Computational Finance MSc produces talented quantitative analysts or ‘quants’ in these areas, in just one year. This fast-paced and innovative programme is taught at UCL Computer Science, a renowned centre of academic excellence, with world-class credentials in computational statistics and machine learning.
UK tuition fees (2024/25)
Overseas tuition fees (2024/25), programme starts, applications accepted.
Applications closed
Applications open
- Entry requirements
A minimum of an upper second-class UK Bachelor's degree (or an international qualification of an equivalent standard) in a relevant discipline with a strong quantitative component evidenced by good performance in mathematics and statistics examinations. Good performance is defined as scores in these subjects not falling below a UK upper second-class or international equivalent level. There is not an exhaustive list of relevant disciplines, but individuals with a background in mathematics, statistics, physics, computer science, engineering, economics, or finance are encouraged to apply.
The English language level for this programme is: Level 2
UCL Pre-Master's and Pre-sessional English courses are for international students who are aiming to study for a postgraduate degree at UCL. The courses will develop your academic English and academic skills required to succeed at postgraduate level.
Further information can be found on our English language requirements page.
This programme is suitable for international students on a Student visa – study must be full-time, face-to-face, starting September.
Equivalent qualifications
Country-specific information, including details of when UCL representatives are visiting your part of the world, can be obtained from the International Students website .
International applicants can find out the equivalent qualification for their country by selecting from the list below. Please note that the equivalency will correspond to the broad UK degree classification stated on this page (e.g. upper second-class). Where a specific overall percentage is required in the UK qualification, the international equivalency will be higher than that stated below. Please contact Graduate Admissions should you require further advice.
About this degree
As a student on the Computational Finance MSc, you will learn advanced quantitative, modelling and programming skills, which are essential for quant roles in trading, research, regulation, and risk. This applied taught postgraduate programme is distinctive in that it provides a combination of finance, mathematics, statistics, and computer science.
Computational finance is at the intersection of mathematics, statistics, computer science, finance and economics. Elevating your skills in these disciplines is essential if you want to make your mark as a quant in a large bank, hedge fund or financial regulator, or in the world of fintech and innovative start-ups.
You will learn from our world-leading experts in this field. The syllabus is constantly reviewed to stay ahead of the trends; core modules span financial engineering and numerical methods for finance, to data science and machine learning for finance.
Optional modules allow you to dive deeper into specific areas of interest, such as algorithmic trading, market microstructure, blockchain technologies, the management of markets, systemic and operational risk, numerical optimisation, and financial market modelling and analysis. You will conclude your experience with a project that brings an opportunity to work with an industry partner on a real-world problem, or to delve into a research project supervised by one of our leading academics.
This programme gives you key skills that will enable you to pursue a career as a ‘quant’, while you immerse yourself in London life and the benefits of living in a global financial centre.
Who this course is for
What this course will give you.
UCL is ranked 9th globally in the latest QS World University Rankings (2024) , giving you an exciting opportunity to study at one of the world’s best universities.
UCL Computer Science is recognised as a world leader in teaching and research. The department was ranked 1st in England and 2nd in the UK for research power in Computer Science and Informatics in the UK's most recent Research Excellence Framework (REF) You will learn from leading experts at the forefront of computer science innovation.
Additionally, the UCL Computational Finance MSc has been ranked 2nd in the UK, 6th in Europe and 24th in the world in the 2023 Risk.net Quant Finance Master’s Guide , which assesses the quality of quantitative finance programmes around the world.
The location in the global financial centre of London coupled with the Department’s extensive links with industry in the City give you ample opportunities for contact with potential future employers, as well as opportunities for practical, hands-on experience with these companies through the UCL Industry Exchange Network (IXN) .
The foundation of your career
Graduates of this programme go on to work as quantitative analysts, quantitative traders or quantitative developers, plus a range of other jobs in asset allocation, risk management, fintech, regulation, or research for a large spectrum of employers.
They work for large banks such as Credit Suisse and JP Morgan, regulators such as the Bank of England and the Financial Conduct Authority, hedge funds and fintech companies, or they stay in academia to pursue a PhD. Altogether, the options for graduates of the Computational Finance MSc are exciting and varied.
Employability
In a programme that embraces mathematics, statistics, finance and computer science in equal measure, you can expect to acquire a combination of highly sought-after skills currently needed in the financial industry. You will graduate with advanced quantitative, modelling and programming skills, which are essential for ‘quant’ roles in trading, asset allocation, risk management, fintech, regulation and research.
UCL is proud to support innovation and link our students and research directly to real-world business applications. From internships to solving complex problems with commercial partners, UCL Engineering has a collaborative, innovative spirit at its core.
As a student and later as a graduate, you will have access to a UCL Engineering careers events programme, connecting you with employers and alumni. This programme provides invaluable insight into the reality of different roles, sectors, and current application processes.
Entrepreneurial minds thrive at UCL. For example, UCL’s IDEALondon was the first innovation centre led by a university in London, and incubates companies post-seed to reach technical and business milestones. Our academic and industrial networks provide a safe and supportive environment to grow a company.
Teaching and learning
The programme’s core curriculum is typically delivered through a combination of lectures, tutorials, and lab classes, as well as directed and self-directed learning supported by teaching materials and resources, published through each module’s online virtual learning environment. Each module employs a teaching strategy that aligns with and supports its intended learning outcomes.
You will be assessed through a range of methods across the programme, which will vary depending on any optional or elective module choices. The programme’s core curriculum is typically assessed by methods including coursework, programming tests, individual and group projects, class tests, written exams, oral assessments, and a final research project/dissertation.
Contact time takes various forms, including lectures, seminars, tutorials, project supervisions, demonstrations, practical classes and workshops, visits, placements, office hours (where staff are available for consultation), email, videoconferencing, or other media, and situations where feedback on assessed work is given (one-to-one or in a group).
Each module has a credit value that indicates the total notional learning hours a learner will spend to achieve its learning outcomes. One credit is considered equal to 10 hours of notional learning, which includes all contact time, self-directed study, and assessment.
The contact time for each of your 15 credit taught modules will typically include 22-30 hours of teaching activity over the term of its delivery, with the balance then comprised of self-directed learning and working on your assessments. You will have ongoing contact with teaching staff via each module’s online discussion forum, which is typically used for discussing and clarifying concepts or assessment matters and will have the opportunity to access additional support via regular office hours with module leaders and programme directors.
Your research project/dissertation module is 60 credits and will include regular contact with your project supervisor(s), who will guide and support you throughout your project. You will dedicate most of your time on this module to carrying out research in connection with your project and writing up your final report.
The Computational Finance MSc is a one-year programme.
In term 1, you will be introduced to financial engineering and to numerical methods in finance. You will choose from optional topics which include probability theory and stochastic processes, market risk and portfolio theory, market microstructure, operational risk management for financial institutions, financial institutions and markets, and blockchain technologies.
In term 2, you will study data science and machine learning with applications in finance. You will choose from optional topics that include algorithmic trading, networks and systemic risk, applied computational finance, financial market modelling and analysis, numerical optimisation, and advanced machine learning in finance. You will also begin preparation for your final research project/dissertation.
In term 3, you will focus on any examinations that take place in the main examination period and undertake your final research project/dissertation; the project is usually done within a placement organised by UCL at a bank, hedge fund, fintech, or other financial services firm located in London, or sometimes at a regulatory authority.
Compulsory modules
Optional modules.
Please note that the list of modules given here is indicative. This information is published a long time in advance of enrolment and module content and availability are subject to change. Modules that are in use for the current academic year are linked for further information. Where no link is present, further information is not yet available.
Students undertake modules to the value of 180 credits. Upon successful completion of 180 credits, you will be awarded an MSc in Computational Finance.
Accessibility
Details of the accessibility of UCL buildings can be obtained from AccessAble accessable.co.uk . Further information can also be obtained from the UCL Student Support and Wellbeing team .
Online - Open day
Graduate Open Events: Department of Computer Science
Join us for a live online information session to hear from Computer Science staff. We will cover areas such as the general admission process, careers support, and industry links/placements. There will also be an opportunity for you to ask staff and current students any questions you may have. Two sessions will run for this event. These sessions are the same and are repeated to cater to people in different time zones.
Fees and funding
Fees for this course.
The tuition fees shown are for the year indicated above. Fees for subsequent years may increase or otherwise vary. Where the programme is offered on a flexible/modular basis, fees are charged pro-rata to the appropriate full-time Master's fee taken in an academic session. Further information on fee status, fee increases and the fee schedule can be viewed on the UCL Students website: ucl.ac.uk/students/fees .
Additional costs
All full time students are required to pay a fee deposit of £2,000 for this programme. All part-time students are required to pay a fee deposit of £1,000.
Students will require a modern computer (PC or Mac) with minimum specifications 8GB RAM and 500GB SSD storage. A computer with the stated specifications is estimated to cost £500 or greater.
For more information on additional costs for prospective students please go to our estimated cost of essential expenditure at Accommodation and living costs .
Funding your studies
For a comprehensive list of the funding opportunities available at UCL, including funding relevant to your nationality, please visit the Scholarships and Funding website .
UCL East London Scholarship
Deadline: 20 June 2024 Value: Tuition fees plus £15,700 stipend () Criteria Based on financial need Eligibility: UK
UCL Friends & Alumni Association scholarship for Machine Learning
Deadline: 3 June 2024 Value: $20,000 (1 year) Criteria Based on both academic merit and financial need Eligibility: EU, Overseas
Students are advised to apply as early as possible due to competition for places. Those applying for scholarship funding (particularly overseas applicants) should take note of application deadlines.
There is an application processing fee for this programme of £90 for online applications and £115 for paper applications. Further information can be found at Application fees .
When we assess your application we would like to learn:
- why you want to study Computational Finance at graduate level
- why you want to study Computational Finance at UCL
- what particularly attracts you to the chosen programme
- how do your academic and professional background and skills meet the demands of this challenging programme
- where would you like to go professionally with your degree
Together with essential academic requirements, the personal statement is your opportunity to illustrate whether your reasons for applying to this programme match what the programme will deliver.
Due to competition for places on this programme, no late applications will be considered. Students with visa requirements or applying for scholarships are advised to apply early.
Please note that you may submit applications for a maximum of two graduate programmes (or one application for the Law LLM) in any application cycle.
Choose your programme
Please read the Application Guidance before proceeding with your application.
Year of entry: 2024-2025
Got questions get in touch.
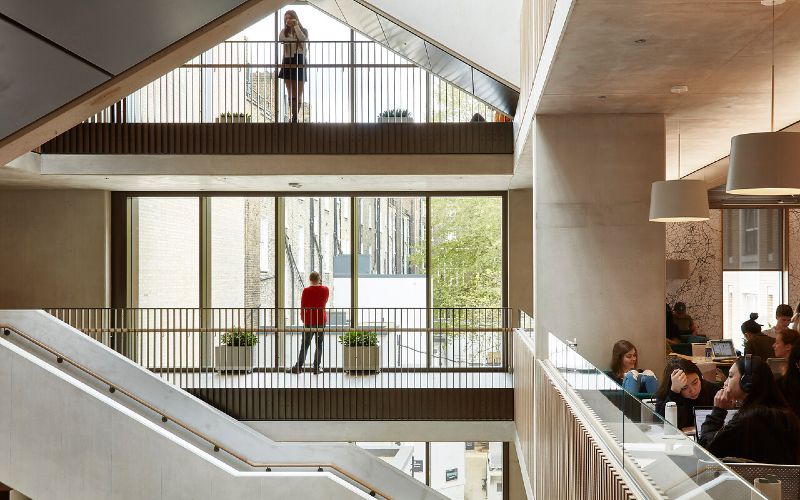
Computer Science
UCL is regulated by the Office for Students .
Prospective Students Graduate
- Graduate degrees
- Taught degrees
- Taught Degrees
- Applying for Graduate Taught Study at UCL
- Research degrees
- Research Degrees
- Funded Research Opportunities
- Doctoral School
- Funded Doctoral Training Programmes
- Applying for Graduate Research Study at UCL
- Teacher training
- Teacher Training
- Early Years PGCE programmes
- Primary PGCE programmes
- Secondary PGCE programmes
- Further Education PGCE programme
- How to apply
- The IOE approach
- Teacher training in the heart of London
- Why choose UCL?
- Entrepreneurship
- Inspiring facilities and resources
- Careers and employability
- Your global alumni community
- Your wellbeing
- Postgraduate Students' Association
- Your life in London
- Accommodation
- Funding your Master's
- Free Samples
- Premium Essays
- Editing Services Editing Proofreading Rewriting
- Extra Tools Essay Topic Generator Thesis Generator Citation Generator GPA Calculator Study Guides Donate Paper
- Essay Writing Help
- About Us About Us Testimonials FAQ
- Studentshare
- Finance & Accounting
- Master of Science in Quantitative Finance
Master of Science in Quantitative Finance - Personal Statement Example
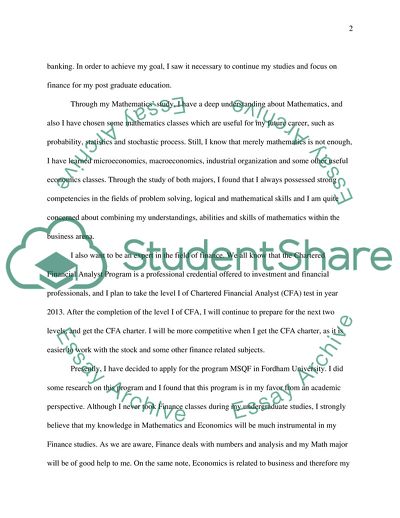
- Subject: Finance & Accounting
- Type: Personal Statement
- Level: Undergraduate
- Pages: 2 (500 words)
- Downloads: 30
- Author: hbarrows
Extract of sample "Master of Science in Quantitative Finance"
The paper "Concerning Career Prospects in Finance" is a perfect example of a personal statement on finance and accounting. I still remember what my feeling was for the first time when my parents asked me to take the Olympiad mathematics class in primary school. I could not understand why I needed to learn the things which were beyond my means. At first, I tried my best to get a higher grade in the class in order to avoid the blame from my parents. However, something interesting happened to me after several months’ study in Olympiad mathematics.
I found that mathematics is a very interesting subject as it was not only about problem-solving but also related to thought spreading. Almost all the mathematics problems could be solved in a number of different ways, and I tried to find the easiest way for each one, therefore, I spent a lot of time in reading mathematics books, and solving mathematics problems. Since my primary school days, I was regarded as one of the best students in the area of mathematics. My passion for mathematics remains to date and this is the most important reason that I selected mathematics as my major for undergraduate education.
Also, finance as an academic area has always captivated me since my school days. I am of the view that my interest in this area comes from the fact that business and finance runs in my family. Therefore, I have continued interacting with business as well as finance-related activities right from a very young age. This is no doubt an important factor that made me choose Economics as my second major.Because of my interest in Mathematics and Business, my career goal is to engage in an industry related to both subjects; such as Investment Company, Securities Company, and banking.
In order to achieve my goal, I saw it necessary to continue my studies and focus on finance for my post-graduate education. Through my Mathematics study, I have a deep understanding of Mathematics, and also I have chosen some mathematics classes which are useful for my future careers, such as probability, statistics, and stochastic process. Still, I know that merely mathematics is not enough, I have learned microeconomics, macroeconomics, industrial organization, and some other useful economics classes.
Through the study of both majors, I found that I always possessed strong competencies in the fields of problem-solving, logical and mathematical skills and I am quite concerned about combining my understandings, abilities, and skills of mathematics within the business arena. I also want to be an expert in the field of finance. We all know that the Chartered Financial Analyst Program is a professional credential offered to investment and financial professionals, and I plan to take the level I of Chartered Financial Analyst (CFA) test in the year 2013.
After the completion of the level I of CFA, I will continue to prepare for the next two levels, and get the CFA charter. I will be more competitive when I get the CFA charter, as it is easier to work with the stock and some other finance-related subjects. Presently, I have decided to apply for the program MSQF at Fordham University. I did some research on this program and I found that this program is in my favor from an academic perspective. Although I never took Finance classes during my undergraduate studies, I strongly believe that my knowledge of Mathematics and Economics will be much instrumental in my Finance studies.
As we are aware, Finance deals with numbers and analysis and my Math major will be of good help to me. On the same note, Economics is related to business and therefore my bachelor’s degree will act as a good foundation. Because I am an international student, I will also be able to interact with individuals from diverse cultures of the world in the university. This will boost my understanding regarding the world that we live in, as well as share knowledge and culture that I have. I am highly concerned about my career prospects in finance.
In this regard, the kind of prospects and contentment provided at American University is quite encouraging for me. This has stimulated me to dream about obtaining higher education from the American University. If I am able to obtain a chance of studying in the American University, I will certainly make special contributions to the institution as well as gain invaluable academic and practical knowledge, since I am highly passionate about studying finance and mathematics.
- Cited: 1 times
- Copy Citation Citation is copied Copy Citation Citation is copied Copy Citation Citation is copied
CHECK THESE SAMPLES OF Master of Science in Quantitative Finance
Marketing strategies in the uk retail car business, personal statements, my personal statement, uk personal statement(4000 characters), teacher reference, personal statement for masters degree of computational finance, personal statement, personal investment in the uk is it science or matter of good fortune, statement of purpose.
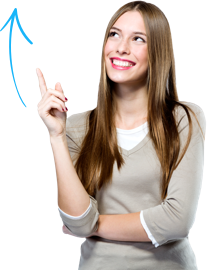
- TERMS & CONDITIONS
- PRIVACY POLICY
- COOKIES POLICY
Master of Science in Computational Finance
Quant research.
The goal of a sell-side research organization is to provide its cash sales desk, derivatives sales desk, hedge fund sales desk with trading ideas to present to the firm’s clients. As a quant research analyst, you will develop and back-test systematic strategies across many asset classes, often including a broader analysis of the economic and political issues driving the markets. Your research may serve many areas of the firm including portfolio management and risk management. On the buy-side, many hedge funds hire quant research analysts to create investment strategies for their clients.
Job titles in Quant Research:
Employers for Quant Research:
Alumni in the Industry
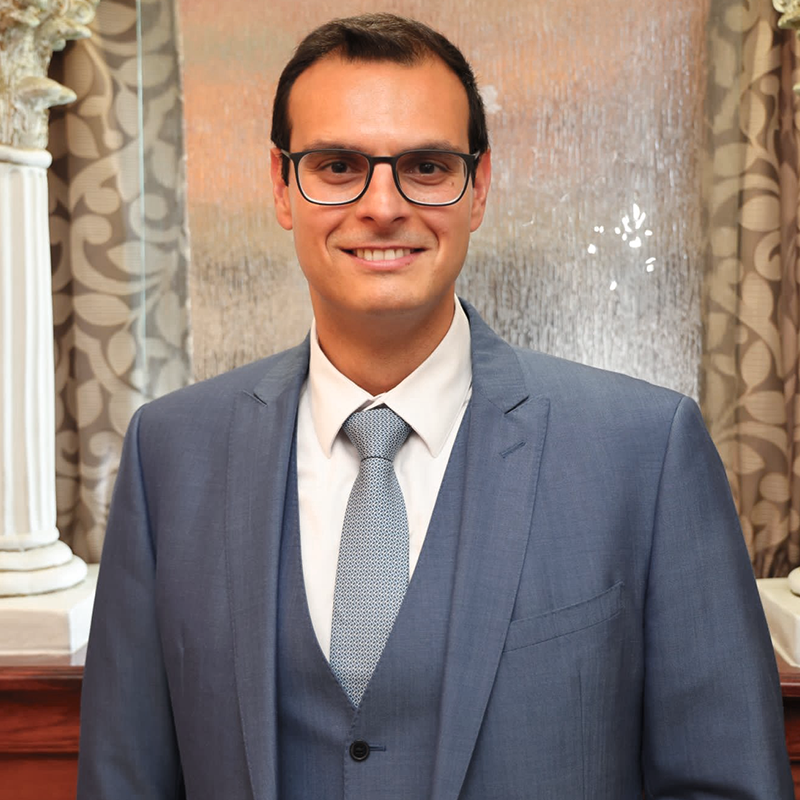
Akram Ayyash, MSCF '14 Credit Portfolio Finance Trader - Citadel
"I enjoy the opportunity to work with a variety of clients from private banks and hedge funds to corporations and asset managers. From my seat, I get to see their different perspectives on trades and prices and how each of them view risk – it’s a really unique position."
Read the full interview with Akram
Industry Article
- The future is quant [top1000funds]
Pittsburgh Location Carnegie Mellon University, TCS Hall 5000 Forbes Avenue Pittsburgh, PA 15213 Office: (412) 268- 3629
New York City Location Carnegie Mellon University 88 Pine Street, 12th Floor New York, NY 10005 Office: (412) 268-8446
MSCF Admissions Carnegie Mellon University Pittsburgh, PA 15213 Phone: (412) 268-3679 [email protected]
The Quantitative Finance and Risk Management Program
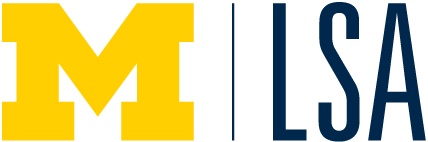
HOW TO APPLY
Admissions timeline.
The Quant Program accepts applications for Fall term only.
September 2024: Rackham Graduate Application opens. Applicants can begin working on and submitting their applications, though no applications will be reviewed until after the submission deadline.
February 1, 2025: Deadline to submit the Rackham Graduate School Application.
February 8, 2025: Recommended deadline for test scores and letters of recommendations to reach our office in order to guarantee consideration in the review process.
Mid-March 2025: First round of decisions released.
April 15, 2025: Enrollment deposit ($3,000 domestic/ $5,000 international) deadline for first-round admits.
April through May 1, 2025: Additional decisions may be issued on a rolling basis. Enrollment deposits are due by the date indicated in the applicant’s admissions letter.
August 25, 2025: Fall classes begin.
FIONA YAN ZHUANG, M.S. ’16, SENIOR QUANTITATIVE ANALYST AT CAPITAL ONE
My experience in the Quant Program was wonderful! The curriculum was designed to provide students with opportunities to develop problem-solving skills in mathematics, statistics, and finance. Since graduating, I’ve been able to apply the expertise I gained in my master’s degree to working on real banking risk management projects. I truly appreciate the enthusiasm that all of the faculty and staff demonstrate toward helping students in their coursework and job placement.
APPLICATION MATERIALS
To be considered complete and receive full consideration, your application should consist of the following:
1. Rackham Online Application. More information is available on the Rackham Graduate School website . Please be sure to select the admissions application for the option you are applying to, either the Quantitative Finance and Risk Management or the Accelerated Master’s Degree Program. The fee for international students is $90. The application fee for U.S. citizens and permanent residents is waived (please email [email protected] for details before you submit your application). You can learn more about application waiver here .
The following materials comprise the Rackham Application:
- Statement of Purpose: The Statement of Purpose should be a concise, well-written essay about your mathematical background, your career goals, and how the Quantitative Finance and Risk Management program will help you meet your career and educational objectives.
- Personal Statement: This is different from the Statement of Purpose in that we ask that you write about things not captured by the application or the Statement of Purpose. Tell us about where you grew up, when you first decided you wanted to study quantitative finance, what challenges you faced and how you overcame them in your pursuit of your education and career.
- Resume: Upload your resume that describes your education, work experience, research experience, professional affiliations, volunteer activity and community engagement, honors and awards. Please limit your resume to 1 page.
- Three letters of recommendation: Register your recommenders for the electronic Letters of Recommendation system in the online application. AMDP applicants need to only submit 2 letters of recommendation, though we recommend that both come from U-M faculty in mathematics or statistics.
2. Official GRE General Test scores: Request ETS to send official scores directly to the Rackham Graduate School at the University of Michigan. Applicants may submit multiple scores, but at least one score must be received by the designated deadline in order for an applicant to be considered. Our ETS school code is 1839. You do not need a department code. AMDP applicants are encouraged, but not required, to submit GRE scores.
3. Official Transcripts: Submit official transcripts from all post secondary institutions are submitted to the University of Michigan Rackham Graduate School. Directions on what qualifies as an official transcript and how to submit official transcripts can be found here. We will consider your application complete and proceed with reviewing it using the unofficial transcripts uploaded with your application, but you cannot be admitted without official transcripts on file.
4. TOEFL or IELTS Scores (for non-native English speakers only) : This requirement may be waived for some applicants depending on their educational background. Visit the Rackham Graduate School’s website to determine whether you qualify for a waiver. For Quant Program applicants, your overall TOEFL score must be 95 or higher. Your overall IELTS score must be 7 or higher. Current U-M students are automatically exempt from this requirement.
Frequently Asked Questions
The Department of Labor-sponsored occupation website provides some information at https://www.onetonline.org/link/summary/13-2099.01
Yes, the Quant Program is designated as a STEM program, so our students are eligible to apply for the 24-month STEM OPT extension.
Yes, it is possible to extend your degree program to four semesters if you postpone taking some courses to the fourth semester.
Please email [email protected] for a waiver (for U.S. domestic students and permanent residents only). There are other application fee waivers offered by the Rackham Graduate School, found at https://rackham.umich.edu/admissions/applying/application-fee-and-waivers/
No, AMDP students must be enrolled for a minimum of two full terms of full-time (9+ credits) in Rackham with no other U-M registration.
GRE general test scores are required for all (domestic and international) applicants except for U-M Math major students.
Yes, we do accept the GRE General Test at home results.
You can learn about the variety of the scholarships and fellowships offered at https://sites.lsa.umich.edu/quant/cost/
There are no mandatory prerequisite courses needed to apply for the master’s program. We have admitted students from a variety of backgrounds (Mathematics, Statistics, Computer Science, Economics, Finance etc.) However, if you are accepted into the Quant program, it’s important to familiarize yourself prior to starting the program with advanced-level undergraduate Mathematics topics such as advanced calculus, linear algebra, analysis, ordinary/ partial differential equations. Otherwise, one may find the program to be challenging.
Internship experience is not a mandatory requirement to apply for the Quant program, but having internship experiences (whether they are in Finance) is certainly helpful. Please highlight your achievements and skill set gained, other (academic or professional) project experiences, and why you’re interested in a career in quantitative finance in your application materials.
- Applying to Uni
- Apprenticeships
- Health & Relationships
- Money & Finance
Personal Statements
- Postgraduate
- U.S Universities
University Interviews
- Vocational Qualifications
- Accommodation
- Budgeting, Money & Finance
- Health & Relationships
- Jobs & Careers
- Socialising
Studying Abroad
- Studying & Revision
- Technology
- University & College Admissions
Guide to GCSE Results Day
Finding a job after school or college
Retaking GCSEs
In this section
Choosing GCSE Subjects
Post-GCSE Options
GCSE Work Experience
GCSE Revision Tips
Why take an Apprenticeship?
Applying for an Apprenticeship
Apprenticeships Interviews
Apprenticeship Wage
Engineering Apprenticeships
What is an Apprenticeship?
Choosing an Apprenticeship
Real Life Apprentices
Degree Apprenticeships
Higher Apprenticeships
A Level Results Day 2024
AS Levels 2024
Clearing Guide 2024
Applying to University
SQA Results Day Guide 2024
BTEC Results Day Guide
Vocational Qualifications Guide
Sixth Form or College
International Baccalaureate
Post 18 options
Finding a Job
Should I take a Gap Year?
Travel Planning
Volunteering
Gap Year Guide
Gap Year Blogs
Applying to Oxbridge
Applying to US Universities
Choosing a Degree
Choosing a University or College
Personal Statement Editing and Review Service
Guide to Freshers' Week
Student Guides
Student Cooking
Student Blogs
Top Rated Personal Statements
Personal Statement Examples
Writing Your Personal Statement
Postgraduate Personal Statements
International Student Personal Statements
Gap Year Personal Statements
Personal Statement Length Checker
Personal Statement Examples By University
Personal Statement Changes 2025
Personal Statement Template
Job Interviews
Types of Postgraduate Course
Writing a Postgraduate Personal Statement
Postgraduate Funding
Postgraduate Study
Internships
Choosing A College
Ivy League Universities
Common App Essay Examples
Universal College Application Guide
How To Write A College Admissions Essay
College Rankings
Admissions Tests
Fees & Funding
Scholarships
Budgeting For College
Online Degree
Platinum Express Editing and Review Service
Gold Editing and Review Service
Silver Express Editing and Review Service
UCAS Personal Statement Editing and Review Service
Oxbridge Personal Statement Editing and Review Service
Postgraduate Personal Statement Editing and Review Service
You are here
Finance personal statement example 1.
Since my early childhood I was the one playing banker in the Czech equivalent of Monopoly, as my parents (both graduates of economic study) mentioned that I had special smile when I touch, count and distribute money. While my dad saw the heights of the international market and my mum worked trough the paperwork that is hidden under the annual reports of every company, I became aware of the world of business rather early. Thanks to my older friend I came across a book called ‘Rich Dad, Poor Dad’ from Mr. Kiyosaki, which gave my early experiences a bit of sense and structure and when I was twelve I was able to discuss assets and liabilities with my older peers and mainly with my dad. Around that time I was given the opportunity to join a new type of school project called Open Gate, which is a bilingual boarding school which specializes in a western style education. As I successfully jumped into it, I’d learned during the study of an optional subject called Globalization, about the interdependence among countries, about wealth and poverty and about injustice in the world. It captured my attention as once Monopoly had done. Therefore I’ve chosen to do HL Economics and Geography in the IB Diploma Program. Step-by-step I realized the connection of Kiyosaki’s world of finance with the injustice of the poor ones. I knew that cement which holds our global economies together is money. Through my extended research into demand for oil I can appreciate the dependence of our society on the oil supply and how those who set oil prices affect literally everybody in the developed world. Meanwhile I found myself enjoying the maths around it and therefore I extended my knowledge by taking higher-level maths in the Czech State Diploma. During my studies I’ve been able to work in a small accounting firm where I could get a taste for what my mum was doing – the accounting. It fascinated me how much work is being done with every single invoice and using the skills I picked up as part of my mums’ work I manage the school cafeteria finance. I was able to contribute with a new, simple, excel system that makes the accounting more comprehensible and transparent. Studying isn’t the only interest of mine; I leave the silent world of letters and numbers to engage in physical activity. I’ve played football since my early childhood and I liked the way our team was collaborating. As I entered Open Gate a few friends and me established an independent football club to satisfy our needs and on the basis of interest we are meeting up for more than 5 years already. My position is usually goalkeeper; therefore I’ve learned how to take the pressure as the last line of defense for the team. It strengthened my state of mind, which helped me sustain more than one difficult situation. My resolution to try at least one new sport every year has meant I’ve experienced paragliding, canyoning, racket ball and windsurfing. But something that stands out from it all is down hill biking, which I have been doing for four years now and it’s become a priority to spend holidays riding down the hills. I think that this combination of sport and studies is making me abnormally happy, as my friends don’t remember seeing me in a bad mood. I’ve chosen England for my future as I prefer to study in English and I want the opportunity to meet with an international environment. University study offers me a great deal of practical education and the option of integrated placement to gain real life experience, which I simply can’t refuse. I seek great education and after graduate studies I want to assert myself in well-situated work places. I want to return back to the Czech Republic with a better sense of what I can achieve to improve our environment here. After all it is my home and if the world is based on finance, I want to understand and contribute to the enhancement of it.
Profile info
This personal statement was written by FunTomator for application in 2011.
FunTomator's university choices Aston University The University of Manchester Bournemouth University London Metropolitan University University of Plymouth
Green : offer made Red : no offer made
Degree FInance at Aston University
FunTomator's Comments
First I made notes for few months. Keep adding and adding new ideas what could i write into the statement about my self. I was even asking my family, girlfriend and all of my friends about my preferences. Then I sit down for one afternoon and wrote the first draft. I asked two schoolmates that has the best english essays and also my English teacher and university adviser. All gave me some new interesting ideas and suggestions. Afterwards I was procrastinating for a couple weeks and then it took me one afternoon again to complete it.
Absolutely DO give it to your english teacher to check grammer after making the last last last final draft even if you think about yourself that you have to annoy them already, because we speak about your future here!!!
Related Personal Statements
Add new comment.
- Search forums
Follow along with the video below to see how to install our site as a web app on your home screen.
Note: This feature may not be available in some browsers.
- Education Advice
MMF/MFE/MAFM Personal Statement Essay Advice
- Thread starter CNS
- Start date 9/12/12
Hi guys, I am applying for upcoming Fall 2013. I realize each essay has to be unique obviously, but I just wanted some general guidelines it. Let me tell you a bit about my background. I graduated from an Australian (Top 5) university double majoring in Economics and Finance. I have a solid GPA and decent internship experience. I realized my strong interest in FM after graduating and hence currently improving on my math skills (discussed with a couple of admission officers and they appreciate the courses I am taking) by completing a Math Diploma and have learned C++ as well. Now, about the personal statement. First, I was wondering whether I should introduce a lot of technical terms about fin mathematics and/mfe as to why that interests me? Or is it better to write in more simple language why I want to do the MFE/Fin Math program. Also, some of the personal statements require you to write about your immediate career plans. I was talking to some individuals in the finance industry and who have already done their masters from top schools itself. They were telling me that its good to be confident and have a plan but not to show to the admofficers that I am too focused on one thing? Is it true? And do I really need to portray that I know a lot about quant finance in my essay or can I just write why I am moving towards a more technical area of finance and what i can expect to gain out of it? Would be pleased if anyone could help.

Andy Nguyen
The best approach in just answer the essay questions straight to the point. Keep in mind that applicants such as yourself have no real world experience in quant finance so the more you talk about it, the more chance it will show your lack of understanding about the field, the job requirement, etc. If you decide to talk specific, make sure you express and convince people that you have done enough research/talking to practitioners. It's a tight rope you have to walk. You don't want to sound clueless by talking nothing or too way out of your head. It's a pretty sure bet that when you graduate you won't be doing something you say you plan to do in your essay.
A lot of time, it comes down to your essay and how your resume looks on screen. Lot of people have similar profile, scores. If you want to have empirical data on your chance, look at the Tracker .
I understand. But am I at a disadvantage because I come from an Australian degree and not a US undergrad degree? Yeah, I was going through the tracker sometime back. I pretty much come to this site often for advice/ information.
CNS said: I understand. But am I at a disadvantage because I come from an Australian degree and not a US undergrad degree? Click to expand...
I agree with Andy. I come from Australia too but I think if you are still worried about people not being familiar with an Australian degree, you could perhaps find out if there is any alumni/current student from the programs that you are interested in.
Andy Nguyen said: How so? I know more programs are trying to diversify their income student profile so it is not made up of 90% of Chinese/Indian applicants. You see where you can play up your different education background now? Click to expand...
It is to gauge if you are genuinely interested in the field and have done homework. Imagine putting on your essay "I'd like to work on a prop trading desk at a BB after the MFE". You can say you want to do interest rate derivatives or whatever but be prepared to show you've done your HW. If you have talked to a lot of people and read a lot of books and keep up with the news, you should have no problem with this essay. Otherwise, it's like blowing up smokes and people can see this easily.
- This site uses cookies to help personalise content, tailor your experience and to keep you logged in if you register. By continuing to use this site, you are consenting to our use of cookies. Accept Learn more…
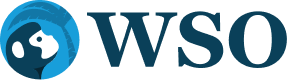
- Recently Active
- Top Discussions
- Best Content
By Industry
- Investment Banking
- Private Equity
- Hedge Funds
- Real Estate
- Venture Capital
- Asset Management
- Equity Research
- Investing, Markets Forum
- Business School
- Fashion Advice
- Business School and GMAT Forum BSCH
Personal Statement for Msc Finance

- Share on Facebook
- Share on Twitter
- Share on LinkedIn
- Share via Email
Hi guys, can anyone give me some advice on how to write a good personal statement and what do they look for?
Masters In Finance Personal Statement
Your personal statement for your Masters in Finance should tell a story. People relate to stories and remember them. It needs to be a story that shows you in a good light and displays your strengths. It must not be dull. You want to find a way to make it stand out while keeping it simple.
User @IlliniProgrammer suggests:
How did you get where you are now? Why do you want to study finance? What do you bring to the table at XYZ University? What do you need at XYZ University? Now that you've answered the questions honestly, remember that your personal statement is a marketing document. You need to the tell the truth and nothing but the truth, but you don't need to tell the whole truth, and you should present things in the best light possible while still being mostly honest.
Watch the video below to learn more about personal statements from Wordvice
Remember that being honest can sometimes feel like you’re being arrogant and while you don’t want to self-aggrandize or go over the top, remember that making yourself small is not the point of this exercise.
Considering A Career On Wall Street? Read More On WSO
- Advice From An Ex-Ib Md On How To Make It To Wall Street
- MBA? Go To Wall Street Not A Start Up!
- Average Age To Apply To Business School
Want Your Resume Reviewed by a Pro?
The WSO Resume Review Service has hand-picked the best professionals from thousands of currently practicing finance professionals… people who live and breathe their industry - day in and day out… who can tell you what’s changing firsthand…who LOVE giving back and will keep you up-to-date on everything you need to polish your resume and land more interviews.
Resume Review Service

This is going to sound jack ass, but make it personal. This is where you write about how a MSc will benefit you, why such a specialized degree, why finance, etc. Simple as that.

making it personal is a good idea. It was a winning bet in my case.

You've gotta tell a story. A story that makes you look strong and shows you in a good light.
How did you get where you are now? Why do you want to study finance? What do you bring to the table at XYZ University? What do you need at XYZ University? Now that you've answered the questions honestly, remember that your personal statement is a marketing document. You need to the tell the truth and nothing but the truth, but you don't need to tell the whole truth, and you should present things in the best light possible while still being mostly honest.
Bear in mind that honest doesn't mean humble, modest, or whatever. If you write a good essay, it might feel arrogant or self-aggrandizing. But if your parents and friends like it, it might be a good essay to submit.

It doesn't have to be all great and insightful. Make it personal, keep it simple, and outline your goals clearly and ways the program will help you towards your goals. I don't think European schools care too much about you're innate desires. But just make sure it's not a deal breaker.

MSF Personal Statement Tips ( Originally Posted: 12/10/2014 )
Getting ready to put together my apps and I was wondering what schools are looking for in the personal statement. Do they want you to prove you're motivated? Prove you're smart? Just tell them why you want to go there?
Also, is it advisable to use the optional essay to explain a bad grade or two? I had a loss of a loved one the day before two finals last semester, and bombed an economics exam. I got a C in the class -- would it be too personal to explain why I got such a bad grade in the optional essay?

I would say the best thing you could do is think about the perspective of the admission committee (they mostly care about employability). @"Esuric" has an excellent post on this.
The Stranger: I would say the best thing you could do is think about the perspective of the admission committee (they mostly care about employability). @Esuric has an excellent post on this.
Any idea where the post is?

I feel that most statements ask for the same thing: Why MSF , why our program, why now? Long/short term goals and how you think this program will help you achieve them.
I'm sure they also look at how you write -- your thought process, ability to convey thoughts effectively, grammar, etc.
http://www.wallstreetoasis.com/forums/how-to-nail-your-msf-interview
You want to articulate why you are seeking a masters in finance and how it will help you achieve your career goals. I've seen and done countless of these and they are all essentially the same.

Grad school personal statement ( Originally Posted: 01/15/2010 )
I'm thinking of grad school and im in some serious need of examples of "personal statement of education objectives". I've been doing some researching and everything is totally different. If anyone has some examples - please let me know! Thank you all!

I don't know how to sell myself on the letter of intent /purpose of study/personal statement ( Originally Posted: 11/14/2015 )
Hey guys I have trouble coming up with a good purpose of study letter. To be honest I have no idea how I should approach this letter. I wrote about the school and program and why I wish to study there but at the same time I also sound kind of repetitive since I will be applying to multiple programs that belong to the same University. My problem is I don't know how to sell myself. I don't know how to paint myself as a confident person because usually I am pretty humble( or that's what I think). I want them to know is what they read is what they get.
I'm talking about graduate programs by the way.
+SB for genuine advice.
Think along these lines: SOP = Movie Initial Paragraph = Your story = Stuff before interval So what happens when you watch a boring/dull/story-less movie? You walk out. (auto-ding) Keep re-writing it till you get your story in order. It should be something related to your program of choice.
After you get this, also focus on your strengths (skills) and how they could be utilized to their full potential while studying for this program.
P.S. - 'since childhood' kind of stuff = auto-ding

yea it's fundamentally wrong to write about the school too much - they know themselves and need not to have you tell them.
You want to project yourself like someone they want to have, someone that fits in, and someone who does great jobs in the past and have big goals for future.
I want to mention the programs I am applying to are pre-experience master programs since I don't really have any work experience.
Focus: How did you get interested in this field/program? (The First para aka Story) What are your skills? (subsequent paras) Have you participated in competitions/research/projects? (subsequent paras) Why do you want to join this university/program? (Last para. Keep it very brief and focus on main points only)
Overall: Keep it crisp and concise Cheers
I would like some more advice please. This is really important for me since I have graduated this year, and I would really like to have a really stellar letter of intent/purpose of study/personal statement that I can use now and for my future applications.

Explain what kind of programs you're applying to first. The "multiple programs by the same university" thing throws me off a little. MSF and Masters in Management or...?
Well I am applying programs related to finance or economics such MSF , MA Economics or MS financial economics .

Dolore ipsa quia qui non. Recusandae commodi et veniam minus sit iste.
Debitis consequuntur officia aliquid non velit. Est sit quos quas ut corrupti ipsa qui autem. Natus nihil perferendis quo. Veniam iste perferendis dolorum voluptas quia.
Aliquam odit perspiciatis eos. Ea iusto eligendi quo ut. Id aut voluptas quo eos magnam. Facilis eveniet dolor est magni delectus. Aliquam qui quos neque sit aliquam nobis. Dicta ab sed ut commodi porro pariatur provident ea.
Qui eos rerum earum officia. Maxime molestiae praesentium molestiae doloremque labore eligendi. Aut enim quaerat nobis neque et quidem. Inventore minus facilis sed. At est quasi laboriosam blanditiis.
See All Comments - 100% Free
WSO depends on everyone being able to pitch in when they know something. Unlock with your email and get bonus: 6 financial modeling lessons free ($199 value)
or Unlock with your social account...

Omnis incidunt minima explicabo qui in. Officiis ut nesciunt placeat voluptate. Autem rerum esse quia cum. Facilis id quia voluptas quae fugiat nemo adipisci. Sapiente praesentium reprehenderit et dolores optio. Voluptatum alias praesentium odit asperiores esse totam. Aspernatur eum qui molestias voluptatem.
Beatae consequatur facilis sed nesciunt et aut et. Veniam officia minima et blanditiis est fugiat. Dolorem eum reiciendis dolore quo et autem distinctio.
Libero magni sit beatae praesentium. Ut velit tenetur aut natus doloremque fugiat quaerat. Natus exercitationem consequatur rerum ut veniam.
Want to Vote on this Content?! No WSO Credits?
Already a member? Login
Trending Content
Career Resources
- Financial Modeling Resources
- Excel Resources
- Download Templates Library
- Salaries by Industry
- Investment Banking Interview Prep
- Private Equity Interview Prep
- Hedge Fund Interview Prep
- Consulting Case Interview Prep
- Resume Reviews by Professionals
- Mock Interviews with Pros
- WSO Company Database
WSO Virtual Bootcamps
- Apr 27 Foundations Bootcamp 10:00AM EDT
- May 04 Venture Capital Bootcamp 10:00AM EDT
- May 11 Financial Modeling & Valuation Bootcamp May 11 - 12 10:00AM EDT
- May 18 Investment Banking Interview Bootcamp 10:00AM EDT
- Jun 01 Private Equity Interview Bootcamp 10:00AM EDT
Career Advancement Opportunities
April 2024 Investment Banking
Overall Employee Satisfaction
Professional Growth Opportunities
Total Avg Compensation
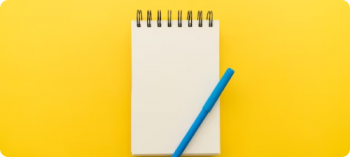
“... there’s no excuse to not take advantage of the resources out there available to you. Best value for your $ are the...”
Leaderboard
- Silver Banana
- Banana Points
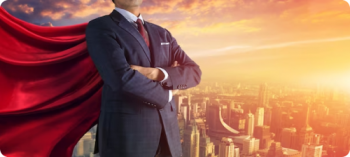
“... I believe it was the single biggest reason why I ended up with an offer...”
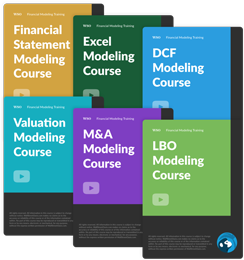
Get instant access to lessons taught by experienced private equity pros and bulge bracket investment bankers including financial statement modeling, DCF, M&A, LBO, Comps and Excel Modeling.
or Want to Sign up with your social account?
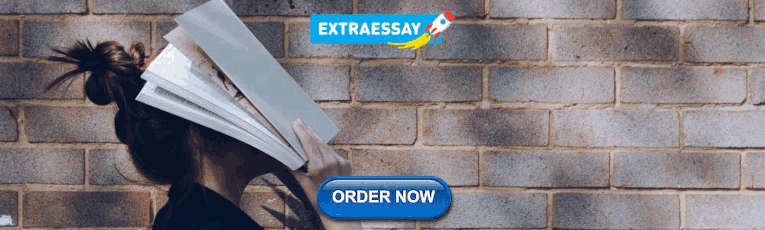
IMAGES
VIDEO
COMMENTS
Personal statements/statements of purpose don't really mean anything. First, non technical staff (like secretaries) filter applications based on GPA and test scores. Second, the technical admissions committee looks at your transcript/courses and publications (if any). At this point they pretty much accept or reject you.
Economics and Finance Personal Statement Example 1. The crucial importance and relevance of economics related disciplines to the modern world have led me to want to pursue the study of these social sciences at a higher level. My experiences of A-Level Economics has shown me the fundamental part it plays in our lives and I would like to approach ...
I am a highly motivated, organised and committed student that possesses the passion to become a successful mathematics and finance graduate at your university. This personal statement was written by Samiksha for application in 2013. Samiksha's university choices. Queen Mary, University of London. Royal Holloway.
11/24/13. #1. Hello, I have read lots of advices on personal statement. By following these advices, I drafted my own one. I'm not confident about its quality. Please feel free to leave your comments or correct my draft. Any advice is welcomed. I'm really appreciated for your time and effort.
3/12/20. #1. Hi everyone, I would really appreciate some comments on my personal statement. Here are the requirements: Address your mathematical qualifications, any relevant experience and eventual career ambitions. (4,000 characters maximum) My passion for quantitative finance stems from my desire to model risks into the valuation of financial ...
Personal Statement Prompt 1. Please discuss past academic and professional experiences and accomplishments that will help you succeed in the Master of Finance program. Include achievements in finance, math, statistics, and computer sciences, as applicable. As an ardent finance student, I have always sought opportunities to develop a solid ...
2 Personal Statements (approximately 500-600 words each) on the following topics: Explain your career and personal aspirations, and your motivation for pursuing the MSc in Quantitative Finance (MQF) programme. Discuss one of the following topics: Describe your greatest weakness and how you have tried to overcome it.
Hi everyone, I am targeting Oxford (msc mathematical and computational finance) , Imperial (msc mathematical finance) , UCL, Kings and possibly Warwick (sorry can't remember the specific course name) for a September 2021 start. I will be sending apps off this September. As MSc course...
Uni of Reading. 391 courses. Visit Website. Browse our range of Finance personal statement examples. Gain inspiration & make sure you're on the right track when writing your own personal statement.
Finance personal statements. On this page you'll find a collection of real personal statements written by students applying to study finance courses at university. These personal statements are written by real students - don't expect them all to be perfect! But by reading through a few of these samples, you'll be able to get some ideas and ...
It has practical applications in derivatives trading, risk management, and portfolio optimization. The Black-Scholes equation is as follows: C = S * N (d1) - X * e^ (-r * T) * N (d2) Where: C represents the theoretical price of the call option. S is the current price of the underlying asset.
The MIT Sloan Master o f Finance program is significantly influenced by being part of both the Sloan School of Management and MIT more broadly. The culture and values of those two entities infuse the MFin program. Yes, being a quant geek helps, but the program's adcom wants more: people who have a distinctive perspective, a sense of purpose, curiosity about and interest in using their quant ...
Accounting and Finance Personal Statement (Anonymous 3) ... Business public: Academy away Bath selectors what expecting scholars to have an interest and aptitude for 'quantitative analysis a economic issues.' Demonstrate get with detection that shows your facilities for applying mathematical skills to the analysis of business problems.
The rising sophistication of the financial sector is bringing a great demand for experts with skills across mathematics, finance, statistics and computer science. The Computational Finance MSc produces talented quantitative analysts or 'quants' in these areas, in just one year. This fast-paced and innovative programme is taught at UCL Computer Science, a renowned centre of
Extract of sample "Master of Science in Quantitative Finance". The paper "Concerning Career Prospects in Finance" is a perfect example of a personal statement on finance and accounting. I still remember what my feeling was for the first time when my parents asked me to take the Olympiad mathematics class in primary school.
Sofiah Sidqi is currently studying BSc Financial Mathematics and Statistics at the London School of Economics and Political Science. She completed her A-Levels in Oxford International College and will be graduating in 2022. This personal statement was part of her successful application to LSE, UCL and King's College London for Financial Maths and Stats.
Quant Research. The goal of a sell-side research organization is to provide its cash sales desk, derivatives sales desk, hedge fund sales desk with trading ideas to present to the firm's clients. As a quant research analyst, you will develop and back-test systematic strategies across many asset classes, often including a broader analysis of ...
Personal Statement: This is different from the Statement of Purpose in that we ask that you write about things not captured by the application or the Statement of Purpose. Tell us about where you grew up, when you first decided you wanted to study quantitative finance, what challenges you faced and how you overcame them in your pursuit of your ...
Office Hours: 9am - 6pm, Monday to Friday UK Address Personal Statement Service. The Old Dairy 12 Stephen Road Headington, Oxford, OX3 9AY United Kingdom. VAT Number 425 5446 95. 24/7 0800 334 5952 London 020 364 076 91 [email protected]
Having majored in mathematics as an undergraduate at the University College of London and in statistics as a master's student at the University of Chicago, I would now like to concentrate my future study in quantitative finance, especially in risk management. As a result of the 2008 financial crisis, regulators around the world have realized the importance of financial regulatory reforms.
Finance Personal Statement Example 1. Since my early childhood I was the one playing banker in the Czech equivalent of Monopoly, as my parents (both graduates of economic study) mentioned that I had special smile when I touch, count and distribute money. While my dad saw the heights of the international market and my mum worked trough the ...
273. 9/13/12. #2. The best approach in just answer the essay questions straight to the point. Keep in mind that applicants such as yourself have no real world experience in quant finance so the more you talk about it, the more chance it will show your lack of understanding about the field, the job requirement, etc.
Masters In Finance Personal Statement. Your personal statement for your Masters in Finance should tell a story. People relate to stories and remember them. ... Quant trading best MSc option: BSCH: 3: 3d +12: International Student : Break into USA IB : BSCH: 2: 4d +12: Worth extending my undergrad another year in order to transfer to target school: