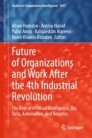
Future of Organizations and Work After the 4th Industrial Revolution pp 145–156 Cite as
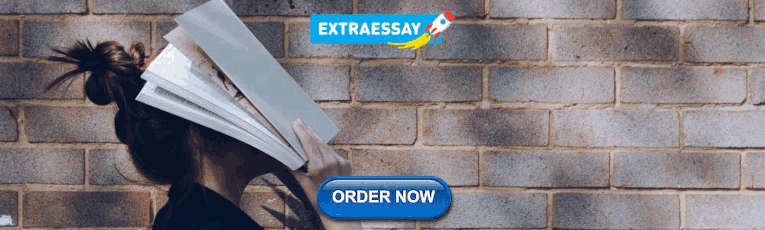
The Impact of Artificial Intelligence on the Banking Industry Performance
- Abdulrahman Rashed Abdulrahman Mohamed Husain 7 ,
- Allam Hamdan 8 &
- Sayed Mohamed Fadhul 8
- First Online: 14 June 2022
1478 Accesses
2 Citations
Part of the book series: Studies in Computational Intelligence ((SCI,volume 1037))
Artificial intelligence (AI) is an advance technology which has positioned itself as one of the revolutionary technology. As a result of increased adoption of new technical advancements, the artificial intelligence industry has grown at an unprecedented rate and is now being implemented in a wide variety of fields. Artificial intelligence systems have the potential to alter the banking industry's whole operations and to enhance the banks’ performance. They are referred to as (AI) and are met with excitement owing to their capacity to make human-like judgments and avoid human-like mistakes. While artificial intelligence has been implemented more broadly in certain sectors than others, the banking industry is one of the few that has shown a reasonable degree of acceptance and implementation of this technology. This literature review defines artificial intelligence, explains how it is being used in banks and specifies the impact of it on banks’ performance.
- Artificial Intelligence
- Robotic process automation
- Banking industry
- Performance
This is a preview of subscription content, log in via an institution .
Buying options
- Available as PDF
- Read on any device
- Instant download
- Own it forever
- Available as EPUB and PDF
- Compact, lightweight edition
- Dispatched in 3 to 5 business days
- Free shipping worldwide - see info
- Durable hardcover edition
Tax calculation will be finalised at checkout
Purchases are for personal use only
Abusalma, A. (2021). The effect of implementing artificial intelligence on job performance in commercial banks of Jordan. Management Science Letters , 2061–2070.
Google Scholar
Aguirre, S. (2017). Automation of a business process using robotic process automation (RPA): A case study . Retrieved October 18, 2019, from https://www.researchgate.net/publication/319343356_Automation_of_a_Business_Process_Using_Robotic_Process_Automation_RPA_A_Case_Study .
Alzaidi, A. A. (2018). Impact of artificial intelligence on performance of banking industry in middle east. International Journal of Computer Science and Network Security , 140–148.
Awwad, B., & Razia, B. (2021). Adapting Altman’s model to predict the performance of the Palestinian industrial sector. Journal of Business and Socio-economic Development, 1 (2), 149–164. https://doi.org/10.1108/JBSED-05-2021-0063
Castelli, M., Manzoni, L., & PopoviI, A. (2016). An artificial intelligence system to predict quality of service in banking organizations. Computational Intelligence and Neuroscience .
Copeland, B. (2021, December 14). Artificial intelligence . https://www.britannica.com/technology/artificial-intelligence .
Frederica, D., & Murwaningsari, E. (2019). The effect of the use of artificial intelligence and operational risk management on banking performance with the implementation of regulation as a moderation variable. A.S.B.L Degres , 146–158.
Hasheminejad, S., & Reisjafari, Z. (2017). ATM management prediction using artificial intelligence techniques: A survey. Intelligent Decision Technologies , 375–398.
IBM. (2021). Artificial intelligence (AI) . https://www.ibm.com/cloud/learn/what-is-artificial-intelligence .
Kaya, O., Schildbach, J., & Schneider, S. (2019). Artificial intelligence in banking. Artificial Intelligence .
Kumar, K. N. (2017). Robotic process automation—A study of the impact on customer experience in retail banking industry. Journal of Internet Banking and Commerce . https://www.icommercecentral.com/open-access/robotic-process-automation-a-study-of-the-impact-on-customer-experience-in-retail-banking-industry.php?aid=87176 .
Lee, H. (2020). Role of artificial intelligence and enterprise risk management to promote corporate entrepreneurship and business performance: evidence from Korean banking sector. Journal of Intelligent & Fuzzy Systems , 5369–5386.
MOVEN. (2020). AI driven PFM. https://moven.com/wp-content/uploads/2020/09/AI-Driven_PFM-The_Heart_of_Digital_Banking_Strands.pdf .
Ris, K., Stanković, Ž., & Avramović, Z. (2020). Implications of implementation of artificial intelligence in the banking business in relation to the human factor. Journal of Information Technology & Applications , 49–57.
Romão, M., Costa, J., & Costa, C. (2019). Robotic process automation: A case study in the banking industry. In Iberian conference on information systems & technologies (pp. 19–22).
Satheesh, M. K., & Nagaraj, S. (2021). Applications of artificial intelligence on customer experience and service. International Management Review, 17 , 1–17. http://www.americanscholarspress.us/journals/IMR/pdf/IMR-1-2021/V17n121-art2.pdf .
Srivastava, U., & Gopalkrishnan, S. (2015). Impact of big data analytics on banking sector: Learning for Indian banks. Procedia Computer Science , 643–652.
Thomas, M. (2021, April 1). 6 Dangerous risks of artificial intelligence. https://builtin.com/artificial-intelligence/risks-of-artificial-intelligence .
Tien, H. N., & Tang, S. M. (2020). Impact of artificial intelligence on vietnam commercial bank operations. International Journal of Social Science and Economics Invention , 296–303. https://doi.org/10.23958/ijssei/vol06-i07/216
Vives, X. (2017). The impact of FinTech on banking. European Economy , 97–105.
Download references
Author information
Authors and affiliations.
College of Business and Finance, MBA, Manama, Bahrain
Abdulrahman Rashed Abdulrahman Mohamed Husain
Ahlia University, Manama, Bahrain
Allam Hamdan & Sayed Mohamed Fadhul
You can also search for this author in PubMed Google Scholar
Corresponding author
Correspondence to Abdulrahman Rashed Abdulrahman Mohamed Husain .
Editor information
Editors and affiliations.
College of Business & Finance, Ahlia University, Manama, Bahrain
Allam Hamdan
Department of Business Studies, Box Hill College Kuwait, Kuwait City, Kuwait
Arezou Harraf
International Centre for Cross Cultural Research and Human Resource Management (ICccR and HRM), University of Jammu, Jammu, Jammu and Kashmir, India
Pallvi Arora
Middle East Technical University, Northern Cyprus Campus, Kalkanlı, Güzelyurt, KKTC, Mersin, Turkey
Bahaaeddin Alareeni
University College of Bahrain, Manama, Bahrain
Reem Khamis Hamdan
Rights and permissions
Reprints and permissions
Copyright information
© 2022 The Author(s), under exclusive license to Springer Nature Switzerland AG
About this chapter
Cite this chapter.
Husain, A.R.A.M., Hamdan, A., Fadhul, S.M. (2022). The Impact of Artificial Intelligence on the Banking Industry Performance. In: Hamdan, A., Harraf, A., Arora, P., Alareeni, B., Khamis Hamdan, R. (eds) Future of Organizations and Work After the 4th Industrial Revolution. Studies in Computational Intelligence, vol 1037. Springer, Cham. https://doi.org/10.1007/978-3-030-99000-8_8
Download citation
DOI : https://doi.org/10.1007/978-3-030-99000-8_8
Published : 14 June 2022
Publisher Name : Springer, Cham
Print ISBN : 978-3-030-98999-6
Online ISBN : 978-3-030-99000-8
eBook Packages : Intelligent Technologies and Robotics Intelligent Technologies and Robotics (R0)
Share this chapter
Anyone you share the following link with will be able to read this content:
Sorry, a shareable link is not currently available for this article.
Provided by the Springer Nature SharedIt content-sharing initiative
- Publish with us
Policies and ethics
- Find a journal
- Track your research
The impact of artificial intelligence in the banking sector & how AI is being used in 2022
- A majority of financial services firms have implemented AI in risk management or revenue generation.
- AI use cases aren't limited to retail banking—they could benefit back and middle offices as well.
- Do you work in the Banking industry? Get business insights on the latest tech innovations, market trends, and your competitors with data-driven research .
- The following is a preview of one Financial Services report, the AI in Banking report. You can purchase this report here.
AI in banking is maturing, bringing the potential for higher-complexity solutions that generate positive ROI across business segments. Adoption of AI solutions in banking has become more mainstream: A majority of financial services companies say they've implemented the technology in business domains like risk management (56%) and revenue generation through new products and processes (52%), per the Cambridge Centre for Alternative Finance and the World Economic Forum. As AI gains popularity in banking, financial institutions (FIs) are building on their existing solutions to solve increasingly complex challenges.
Most banks (80%) are highly aware of the potential benefits presented by AI and machine learing, per an OpenText survey of financial services professionals. In fact, many banks are planning to deploy solutions enabled by AI: 75% of respondents at banks with over $100 billion in assets say they're currently implementing AI strategies, compared with 46% at banks with less than $100 billion in assets, per a UBS Evidence Lab report seen by Insider Intelligence. Certain AI use cases have already gained prominence across banks' operations, with chatbots in the front office and anti-payments fraud in the middle office the most mature.
Banks can use AI to transform the customer experience by enabling frictionless, 24/7 customer service interactions — but AI in banking applications isn't just limited to retail banking services . The back and middle offices of investment banking and all other financial services for that matter could also benefit from AI.
Applications of AI in Banking
The three main channels where banks can use artificial intelligence to save on costs are front office (conversational banking), middle office (fraud detection and risk management) and back office (underwriting).
In this report, Business Insider Intelligence identifies the most meaningful AI and machine learning applications across banks' front and middle offices. We also discuss the winning AI strategies used by fintechs and legacy financial institutions so far, as well as provide recommendations for how banks can best approach an AI-enabled digital transformation.
Related stories
The companies mentioned in this report are: Capital One, Citi, HSBC, JPMorgan Chase, Personetics, Quantexa, and U.S. Bank
Here are some of the key takeaways from the report:
- Front- and middle-office AI applications offer the greatest cost savings opportunity across digital banking .
- Banks are leveraging algorithsm on the front end to smooth customer identification and authentication, mimic live employees through chatbots and voice assistants, deepen customer relationships, and provide personalized insights and recommendations.
- AI is also being implemented by banks within middle-office functions to assess risks, detect and prevent payments fraud, improve processes for anti-money laundering (AML) and perform know-your-customer (KYC) regulatory checks.
- The winning strategies employed by banks that are undergoing an AI-enabled transformation reveal how to best capture the opportunity. These strategies highlight the need for a holistic AI strategy that extends across banks' business lines, usable data, partnerships with external partners, and qualified employees.
In full, the report:
- Outlines the benefits of using AI in the banking industry .
- Details the key use cases for transforming the front and middle office using the technology.
- Highlights players that have successfully implemented AI solutions.
- Examines winning strategies used by financial institutions that are leveraging AI to transform their entire organizations.
- Discusses how banks can best capture the AI opportunity, including considerations on internal culture, staffing, operations, and data.
Interested in getting the full report? Here's how you can gain access:
- Join other Insider Intelligence clients who receive this report, along with thousands of other Financial Services forecasts, briefings, charts, and research reports to their inboxes. >> Become a Client
- Purchase the individual report from our store. >> Buy The Report Here
- Main content

An official website of the United States government
The .gov means it’s official. Federal government websites often end in .gov or .mil. Before sharing sensitive information, make sure you’re on a federal government site.
The site is secure. The https:// ensures that you are connecting to the official website and that any information you provide is encrypted and transmitted securely.
- Publications
- Account settings
Preview improvements coming to the PMC website in October 2024. Learn More or Try it out now .
- Advanced Search
- Journal List
- Springer Nature - PMC COVID-19 Collection

Utilization of artificial intelligence in the banking sector: a systematic literature review
Omar h. fares.
Ted Rogers School of Retail Management, Toronto Metropolitan University, 350 Victoria St, Toronto, ON M5B 2K3 Canada
Seung Hwan Mark Lee
This study provides a holistic and systematic review of the literature on the utilization of artificial intelligence (AI) in the banking sector since 2005. In this study, the authors examined 44 articles through a systematic literature review approach and conducted a thematic and content analysis on them. This review identifies research themes demonstrating the utilization of AI in banking, develops and classifies sub-themes of past research, and uses thematic findings coupled with prior research to propose an AI banking service framework that bridges the gap between academic research and industry knowledge. The findings demonstrate how the literature on AI and banking extends to three key areas of research: Strategy, Process, and Customer. These findings may benefit marketers and decision-makers in the banking sector to formulate strategic decisions regarding the utilization and optimization of value from AI technologies in the banking sector. This study also provides opportunities for future research.
Introduction
Digital innovations in the modern banking landscape are no longer discretionary for financial institutions; instead, they are becoming necessary for financial institutions to cope with an increasingly competitive market and changing customer expectations (De Oliveira Santini, 2018 ; Eren, 2021 ; Hua et al., 2019 ; Rajaobelina and Ricard, 2021 ; Valsamidis et al., 2020 ; Yang, 2009 ). In the era of modern banking, many new digital technologies have been driven by artificial intelligence (AI) as the key engine (Dobrescu and Dobrescu, 2018 ), leading to innovative disruptions of banking channels (e.g., automated teller machines, online banking, mobile banking), services (e.g., imaging of checks, voice recognition, chatbots), and solutions (e.g., AI investment advisors and AI credit selectors).
The application of AI in banking is across the board, with uses in the front office (voice assistants and biometrics), middle office (anti-fraud risk monitoring and complex legal and compliance workflows), and back office (credit underwriting with smart contracts infrastructure). Banks are expected to save $447 billion by 2023, by employing AI applications. Almost 80% of the banks in the USA are cognizant of the potential benefits offered by AI (Digalaki, 2022 ). Indeed, the emergence of AI has generated a wealth of opportunities and challenges (Malali and Gopalakrishnan, 2020 ). In the banking context, the use of AI has led to more seamless sales and has guided the development of effective customer relationship management systems (Tarafdar et al., 2019 ). While the focus in the past was on the automation of credit scoring, analyses, and the grants process (Mehrotra, 2019 ), capabilities evolved to support internal systems and processes as well (Caron, 2019 ).
The term AI was first used in 1956 by John McCarthy (McCarthy et al., 1956 ); it refers to systems that act and think like humans in a rational way (Kok et al., 2009 ). In the aftermath of the dot com bubble in 2000, the field of AI shifted toward Web 2.0. era in 2005, and the growth of data and availability of information encouraged more research in AI and its potential (Larson, 2021 ). More recently, technological advancements have opened the doors for AI to facilitate enterprise cognitive computing, which involves embedding algorithms into applications to support organizational processes (Tarafdar et al., 2019 ). This includes improving the speed of information analysis, obtaining more accurate and reliable data outputs, and allowing employees to perform high-level tasks. In recent years, AI-based technologies have been shown to be effective and practical. However, many corporate executives still lack knowledge regarding the strategic utilization of AI in their organizations. For instance, Ransbotham et al. ( 2017 ) found that 85% of business executives viewed AI as a key tool for providing businesses with a sustainable competitive advantage; however, only 39% had a strategic plan for the use of AI, due to the lack of knowledge regarding implementation of AI for their organizations.
Here, we systematically analyze the past and current state of AI and banking literature to understand how it has been utilized within the banking sector historically, propose a service framework, and provide clear future research opportunities. In the past, a limited number of systematic literature reviews have studied AI within the management discipline (e.g., Bavaresco et al., 2020 ; Borges et al., 2020 ; Loureiro et al., 2020 ; Verma et al., 2021 ). However, the current literature lacks either research scope and depth, and/or industry focus. In response, we seek to differentiate our study from prior reviews by providing a specific focus on the banking sector and a more comprehensive analysis involving multiple modes of analysis.
In light of this, we aim to address the following research questions:
- What are the themes and sub-themes that emerge from prior literature regarding the utilization of AI in the banking industry?
- How does AI impact the customer's journey process in the banking sector, from customer acquisition to service delivery?
- What are the current research deficits and future directions of research in this field?
Methodology
Selection of articles.
Adhering to the best practices for conducting a Systematic Literature Review (SLR) (see Khan et al., 2003 ; Tranfield et al, 2003 ; Xiao and Watson, 2019 ), we began by selecting the appropriate database and identifying keywords, based on an in-depth review of the literature. Research papers were extracted from Web of Science (WoS) and Scopus. These databases were selected to complement one another and provide access to scholarly articles (Mongeon and Paul-Hus, 2016 ); this was also the first step in ensuring the inclusion of high-quality articles (Harzing and Alakangas, 2016 ). The following query was used to search the title, abstract, and keywords: “Artificial intelligence OR machine learning OR deep learning OR neural networks OR Intelligent systems AND Bank AND consumer OR customer OR user.” The keywords were selected, based on prior literature review, with the goal of covering various business functions, especially focusing on the banking sector (Loureiro et al., 2020 ; Verma et al., 2021 ; Borges et al., 2020 ; Bavaresco et al., 2020 ). The initial search criteria yielded 11,684 papers. These papers were then filtered by “English,” “article only” publications, and using the subject area filter of “Management, Business Finance, accounting and Business,” which resulted in 626 papers.
In this study, we used the preferred reporting method for systematic reviews and meta-analyses (PRISMA) to ensure that we follow the systematic approach and track the flow of data across different stages of the SLR (Moher et al., 2009 ). After extracting the articles, each of the 626 papers was given a distinctive ID number to help differentiate the papers; the ID number was maintained throughout the analysis process. The data were then organized using the following columns: “ID number,” “database source,” “Author,” “title,” “Abstract,” “keywords,” “Year,” Australian Business Deans Council (ABDC) Journals, “and keyword validation columns.”
The exclusion of papers was done systematically in the following manner: a) All duplicate papers in the database were eliminated (105 duplicates); b) as a second quality check, papers not published in ABDC journals (163 papers) were omitted to ensure a quality standard for inclusion in the review,Query a practice consistent with other recent SLRs (Goyal and Kumar, 2021 ; Nusair et al., 2019 ; Pahlevan-Sharif et al., 2019 ); c) in order to ensure the relevance of articles included, and following our research objectives, we excluded non-consumer-related papers, searching for consumers (consumer, customer, user) in the title, abstract, and keywords; this resulted in the removal of 314 papers; d) for the remaining 48 papers, a relevance check was manually conducted to determine whether the papers were indeed related to AI and banking. Papers that specifically focused on the technical computational process of AI were removed (4 papers). This process resulted in the selection of 44 articles for subsequent analyses.
Thematic analysis
A thematic analysis classifies the topics and subtopics being researched. It is a method for identifying, analyzing, and reporting patterns within data (Boyatzis, 1998 ). We followed Chatha and Butt ( 2015 ) to classify the articles into themes and sub-themes using manual coding. Second, we employed the Leximancer software to supplement the manual classification process. The use of these two approaches provides additional validity and quality to the research findings.
Leximancer is a text-mining software that provides conceptual and relational information by identifying concept occurrences and co-occurrences (Leximancer, 2019 ). After uploading all the 44 papers onto Leximancer, we added “English” to the stoplist, which removed words such as “or/and/like” that are not relevant to developing themes. We manually removed irrelevant filler words, such as “pp.,” “Figure,” and “re.” Finally, our results consisted of two maps: a) a conceptual map wherein central themes and concepts are identified, and b) a relational cloud map where a network of connections and relationships are drawn among concepts.
RQ 1: What are the themes and sub-themes that emerge from prior literature regarding the utilization of AI in the banking industry?
We began with a deductive approach to categorize articles into predetermined themes for the theme identification process. We then employed an inductive approach to identify the sub-themes and provide context for the primary themes (See Fig. Fig.1). The 1 ). The procedure for determining the primary themes included, a) reviewing previous related systematic literature reviews (Bavaresco et al., 2020 ; Borges et al., 2020 ; Loureiro et al., 2020 ; Verma et al., 2021 ), b) identifying keywords and developing codes (themes) from selected papers; and c) reviewing titles, abstracts, and full papers, if needed, to identify appropriate allocation within these themes. Three primary themes were curated from the process: Strategy, Processes, and Customers (see Fig. 2 ).

Thematic map

Themes by timeline
In the Strategy theme (21 papers), early research shows the potential uses and adoption of AI from an organizational perspective (e.g., Akkoç, 2012 ; Olson et al., 2012 ; Smeureanu et al., 2013 ). Data mining (an essential part of AI) has been used to predict bankruptcy (Olson et al., 2012 ) and to optimize risk models (Akkoç, 2012 ). The increasing use of AI-driven tools to drive organizational effectiveness creates greater business efficiency opportunities for financial institutions, as compared to traditional modes of strategizing and risk model development. The sub-theme Organizational use of AI (14 papers) covers a range of current activities wherein banks use AI to drive organizational value. These organizational uses include the use of AI to drive business strategies and internal business activities. Medhi and Mondal ( 2016 ) highlighted the use of an AI-driven model to predict outsourcing success. Our findings indicate the effectiveness of AI tools in driving efficient organizational strategies; however, there remain several challenges in implementing AI technologies, including the human resources aspect and the organizational culture to allow for such efficiencies (Fountain et al., 2019 ). More recently, there has been a noticeable focus on discussing some of the challenges associated with AI implementation in banking institutions (e.g., Jakšič and Marinč, 2019 ; Mohapatra, 2020 ). The sub-theme Challenges with AI (three papers) covers a range of challenges that organizations face, including the integration of AI in their organizations. Mohapatra ( 2020 ) characterizes some of the key challenges related to human–machine interactions to allow for the sustainable implementation of AI in banking. While much of the current research has focused on technology, our findings indicate that one of the main areas of opportunity in the future is related to adoption and integration. The sub-theme AI and adoption in financial institutions (six papers) covered a range of topics regarding motivation, and barriers to the adoption of AI technology from an organizational standpoint. Fountain et al. ( 2019 ) conceptually highlighted some barriers to organizational adoption, including workers’ fear, company culture, and budget constraints. Overall, in the Strategy theme, organizational uses of AI seemed to be the most prominent, which highlights the consistent focus on technology development compared with technology implementation. However, the literature remains limited in terms of discussions related to the organizational challenges associated with AI implementation.
In the Processes theme (34 papers), after the dot com bubble and with the emergence of Web 2.0, research on AI in the banking sector started to emerge. This could have been triggered by the suggested use of AI to predict stock market movements and stock selection (Kim and Lee, 2004 ; Tseng, 2003 ). At this stage, the literature on AI in the banking sector was related to its use in credit and loan analysis (Baesens et al., 2005 ; Ince and Aktan, 2009 ; Kao et al., 2012 ; Khandani et al., 2010 ). In the early stages of AI implementation, it is essential to develop fast and reliable AI infrastructure (Larson, 2021 ). Baesens et al. ( 2005 ) utilized a neural network approach to better predict loan defaults and early repayments. Ince and Aktan ( 2009 ) used a data mining technique to analyze credit scores and found that the AI-driven data mining approach was more effective than traditional methods. Similarly, Khandani et al. ( 2010 ) found machine-learning-driven models to be effective in analyzing consumer credit risk. The sub-theme, AI and credit (15 papers), covers the use of AI technology, such as machine learning and data mining, to improve credit scoring, analysis, and granting processes. For instance, Alborzi and Khanbabaei ( 2016 ) examined the use of data mining neural network techniques to develop a customer credit scoring model. Post-2013, there has been a noticeable increase in investigating how AI improves processes that go beyond credit analysis. The sub-theme AI and services (20 papers) covers the uses of AI for process improvement and enhancement. These process-related uses of technology include institutional uses of technology to improve internal service processes. For example, Soltani et al. ( 2019 ) examined the use of machine learning to optimize appointment scheduling time, and reduce service time. Overall, regarding the process theme, our findings highlight the usefulness of AI in improving banking processes; however, there remains a gap in practical research regarding the applied integration of technology in the banking system. In addition, while there is an abundance of research on credit risk, the exploration of other financial products remains limited.
In the Customer theme (26 papers), we uncovered the increasing use of AI as a methodological tool to better understand customer adoption of digital banking services. The sub-theme AI and Customer adoption (11 papers) covers the use of AI as a methodological tool to investigate customers’ adoption of digital banking technologies, including both barriers and motivational factors. For example, Arif et al. ( 2020 ) used a neural network approach to investigate barriers to internet-banking adoption by customers. Belanche et al. ( 2019 ) investigate factors related to AI-driven technology adoption in the banking sector. Payne et al. ( 2018 ) examine the drivers of the usage of AI-enabled mobile banking services. In addition, bank marketers have found an opportunity to use AI to better segment, target, and position their banking products and services. The sub-theme, AI and marketing (nine papers), covers the use of AI for different marketing activities, including customer segmentation, development of marketing models, and delivery of more effective marketing campaigns. For example, Smeureanu et al. ( 2013 ) proposed a machine learning technique to segment banking customers. Schwartz et al. ( 2017 ) utilized an AI-based method to examine the resource allocation in targeted advertisements. In recent years, there has been a noticeable trend in investigating how AI shapes customer experience (Soltani et al., 2019 ; Trivedi, 2019 ). The sub-theme of AI and customer experience (Papers 11) covers the use of AI to enhance banking experience and services for customers. For example, Trivedi ( 2019 ) investigated the use of chatbots in banking and their impact on customer experience.
Table Table1 1 highlights the number of papers included in the themes and sub-themes. Overall, the papers related to Processes (77%) were the most frequently occurring, followed by Customer (59%) and Strategy-based (48%) papers. From 2013 onward, there was an increase in the inter-relation between all three areas of Strategy, Processes, and Customers. Since 2016, there has been a surge in research linking the themes of Processes and Customers. More recently, since 2017, papers combining Customers with Strategy have become more frequent.
Thematic count
Leximancer analysis
A Leximancer analysis was conducted on all the papers included in the study. This resulted in two major classifications and 56 distinct concepts. Here, a “concept” refers to a combination of closely related words. When referring to “concept co-occurrence,” we refer to the total number of times two concepts appear together. In comparison, the word association percentage refers to the conditional probability that two concepts will appear side-by-side.
Conceptual and relational analyses
Conceptual analysis refers to the analysis of data based on word frequency and word occurrence, whereas relational analysis refers to the analysis that draws connections between concepts and captures the co-occurrences between words (Leximancer, 2019 ). As Fig. 3 shows, the most prominent concept is “customer,” which provides additional credence to our customer theme. The concept “customer” appeared 2,231 times across all papers. For the concept “customer,” some of the key concept associations include satisfaction (324 co-occurrences and 64% word association), service (185 co-occurrences and 43% word association), and marketing (86 co-occurrences and 42% word association). This may imply the importance of utilizing AI in improving customer service and satisfaction, and in marketing to retain and grow the customer base. For instance, Trivedi ( 2019 ) examined the factors affecting chatbot satisfaction and found that information, system, and service quality, all have a significant positive association with it. Ekinci et al. ( 2014 ) proposed a customer lifetime value model, supported by a deep learning approach, to highlight key indicators in the banking sector. Xu et al. ( 2020 ) examined the effects of AI versus human customer service, and found that customers are more likely to use AI for low-complexity tasks, whereas a human agent is preferred for high-complexity tasks. It is worth noting that most of the research related to the customer theme has utilized a quantitative approach, with limited qualitative papers (i.e., four papers) in recent years.

Concept map of content of all papers included in the study
Not surprisingly, the second most prominent concept is “banking,” which is expected as it is the sector that we are examining. The concept “banking” appeared 1,033 times across all the papers. In the “banking” concept, some of the key concept associations include mobile (248 co-occurrences and 88% word association), internet (152 co-occurrences and 82% word association), adoption (220 co-occurrences and 50% word association), and acceptance (71 co-occurrences and 42% word association). This implies the importance of utilizing AI in mobile- and internet-banking research, along with inquiries related to the adoption and acceptance of AI for such uses. Belanche et al. ( 2019 ) proposed a research framework to provide a deeper understanding of the factors driving AI-driven technology adoption in the banking sector. Payne et al. ( 2018 ) examined digital natives' comfort and attitudes toward AI-enabled mobile banking activities and found that the need for services, attitude toward AI, relative advantage, and trust had a significant positive association with the usage of AI-enabled mobile banking services.
Figure 4 highlights the concept associations and draws connections between concepts. The identification and classification of themes and sub-themes using the deductive method in thematic analysis, and the automated approach using Leximancer, provide a reliable and detailed overview of the prior literature.

Cloud map of content of all papers included in the study
Customer credit solution application-service blueprint
RQ 2: How does AI impact the banking customer’s journey?
A service blueprint is a method that conceptualizes the customer journey while providing a framework for the front/back-end and support processes (Shostack, 1982 ). For a service blueprint to be effective, the core focus should be on the customer, and steps should be developed based on data and expertise (Bitner et al., 2008 ). As previously discussed, one of the key research areas, AI and banking, relates to credit applications and granting decisions; these are processes that directly impact customer accessibility and acquisition. Here, we develop and propose a Customer Credit Solution Application-Service Blueprint (CCSA) based on our earlier analyses.
Not only was the proposed design developed but the future research direction was also extracted from the articles included in this study. We also validated the framework through direct consultation with banking industry professionals. The CCSA model allows marketers, researchers, and banking professionals to gain a deeper understanding of the customer journey, understand the role of AI, provide an overview of future research directions, and highlight the potential for future growth in this field. As seen in Fig. 5 , we divided the service blueprint into four distinct segments: customer journey, front-stage, back-stage, and support processes. The customer journey is the first step in building a customer-centric blueprint, wherein we highlight the steps taken by customers to apply for a credit solution. The front-stage refers to how the customer interacts with a banking touchpoint (e.g., chatbots). Back-stage actions provide support to customer-facing front-stage actions. Support processes aid in internal organizational interactions and back-stage actions. This section lays out the steps for applying for credit solutions online and showcases the integration and use of AI in the process, with examples from the literature.

Customer credit solution application journey
Acquire customer
We begin from the initial step of customer acquisition, and proceed to credit decision, and post-decision (Broby, 2021 ). In the acquisition step, customers are targeted with the goal of landing them on the website and converting them to active customers. The front-stage includes targeted ads , where customers are exposed to ads that are tailored for them. For instance, Schwartz et al. ( 2017 ) utilized a multi-armed bandit approach for a large retail bank to improve customer acquisition, and proposed a method that allows bank marketers to maintain the balance between learning from advertisement data and optimizing advertisement investment. At this stage, the support processes focus on integrating AI as a methodological tool to better understand customers' banking adoption behaviors, in combination with utilizing machine learning to evaluate and update segmentation activities. The building block at this stage, is understanding the factors of online adoption. Sharma et al. ( 2017 ) used the neural network approach to investigate the factors influencing mobile banking adoption. Payne et al. ( 2018 ) examined digital natives' comfort and attitudes toward AI-enabled mobile banking activities. Markinos and Daskalaki ( 2017 ) used machine learning to classify bank customers based on their behavior toward advertisements.
Visit bank’s website & apply for a credit solution
At this stage, banking institutions aim to convert website traffic to credit solution applicants. The integration of robo-advisors will help customers select a credit solution that they can best qualify for, and which meets their banking needs. The availability of a robo-advisor can enhance the service offering, as it can help customers with the appropriate solution after gathering basic personal financial data and validating it instantly with credit reporting agencies. Trivedi ( 2019 ) found that information, system, and service quality are key to ensuring a seamless customer experience with the chatbot, with personalization moderating the constructs. Robo-advisors have task-oriented features (e.g., checking bank accounts) coupled with problem-solving features (e.g., processing credit applications). Following this, the data collected will be consistently examined through the use of machine learning to improve the offering and enhance customer experience. Jagtiani and Lemieux ( 2019 ) used machine learning to optimize data collected through different channels, which helps arrive at appropriate and inclusive credit recommendations. It is important to note that while the proposed process provides immense value to customers and banking institutions, many customers are hesitant to share their information; thus, trust in the banking institution is key to enhancing customer experience.
Receive a decision
After the data have been collected through the online channel, data mining and machine learning will aid in the analysis and provide optimal credit decisions. At this stage, the customer receives a credit decision through the robo-advisor. The traditional approaches for credit decisions usually take up to two weeks, as the application goes to the advisory network, then to the underwriting stage, and finally back to the customer. However, with the integration of AI, the customer can save time and be better informed by receiving an instant credit decision, allowing an increased sense of empowerment and control. The process of arriving at such decisions should provide a balance between managing organizational risk, maximizing profit, and increasing financial inclusion. For instance, Khandani et al. ( 2010 ) utilized machine learning techniques to build a model predicting customers' credit risk. Koutanaei et al. ( 2015 ) proposed a data mining model to provide more confidence in credit scoring systems. From an organizational risk standpoint, Mall ( 2018 ) used a neural network approach to examine the behavior of defaulting customers, so as to minimize credit risk, and increase profitability for credit-providing institutions.
Customer contact call center
At this stage, we outline the relationship between humans and AI. As Xu et al. ( 2020 ) found that customers prefer humans for high-complexity tasks, the integration of human employees for cases that require manual review is vital, as AI can make errors or misevaluate one of the C's of credit (Baiden, 2011 ). While AI provides a wealth of benefits for customers and organizations, we refer to Jakšič and Marinč's ( 2019 ) discussion that relationship banking still plays a key role in providing a competitive advantage for financial institutions. The integration of AI at this stage can be achieved by optimizing banking channels. For instance, banking institutions can optimize appointment scheduling time and reduce service time through the use of machine learning, as proposed by Soltani et al. ( 2019 ).
General discussion
Researchers have recognized the viable use of AI to provide enhanced customer service. As discussed in the CCSA service advice, facilities, such as robo-advisors, can aid in product selection, application for banking solutions, and time-saving in low-complexity tasks. As AI has been shown to be an effective tool for automating banking processes, improving customer satisfaction, and increasing profitability, the field has further evolved to examine issues pertaining to strategic insights. Recent research has been focused on investigating the use of AI to drive business strategies. For instance, researchers have examined the use of AI to simplify internal audit reports and evaluate strategic initiatives (Jindal, 2020 ; Muñoz-Izquierdo et al., 2019 ). The latest research also highlights the challenges associated with AI, whether from the perspective of implementation, culture, or organizational resistance (Fountain et al., 2019 ). Moreover, one of the key challenges uncovered in the CCSA is privacy and security concerns of customers in sharing their information. As AI technologies continue to grow in the banking sector, the privacy-personalization paradox has become a key research area that needs to be examined.
In addition, the COVID-19 pandemic has brought on a plethora of challenges in the implementation of AI in the banking sector. Although banks' interest in AI technologies remains high, the reduction in revenue has resulted in a decrease in short-term investment in AI technologies (Anderson et al., 2021 ). Wu and Olson ( 2020 ) highlight the need for banking institutions to continue investing in AI technologies to reduce future risks and enhance the integration between online and offline channels. From a customer perspective, COVID-19 has led to an uptick in the adoption of AI-driven services such as chatbots, E-KYC (Know your client), and robo-advisors (Agarwal et al., 2022 ).
Future research directions
RQ 3: What are the current research deficits and the future directions of research in this field?
Tables Tables2, 2 , ,3, 3 , and and4 4 provide a complete list of recommendations for future research. These recommendations were developed by reviewing all the future research directions included in the 44 papers. We followed Watkins' ( 2017 ) rigorous and accelerated data reduction (RADaR) technique, which allows for an effective and systematic way to analyze and synthesize calls for future research (Watkins, 2017 ).
Detailed future research directions—Theme: Strategy
Detailed future research recommendations—Theme: Processes
Detailed future research recommendations—Theme: Customer
Regarding strategy, as AI continues to grow in the banking industry, financial institutions need to examine how internal stakeholders perceive the value of embracing AI, the role of leadership, and multiple other variables that impact the organizational adoption of AI. Therefore, we recommend that future research investigate the different factors (e.g., leadership role) that impact the organizational adoption of AI technologies. In addition, as more organizations use and accept AI, internal challenges emerge (Jöhnk et al., 2021 ). Thus, we recommend examining the different organizational challenges (e.g., organizational culture) associated with AI adoption.
Regarding processes, AI and credit is one of the areas that has been extensively explored since 2005 (Bhatore et al., 2020 ). We recommend expanding beyond the currently proposed models and challenging the underlying assumptions by exploring new aspects of risks presented with the introduction of AI technologies. In addition, we recommend the use of more practical case studies to validate new and existing models. Additionally, the growth of AI has evoked further exploration of how internal processes can be improved (Akerkar, 2019 ). For instance, we suggest investigating AI-driven models with other financial products/solutions (e.g., investments, deposit accounts, etc.).
Regarding customers, the key theories mentioned in the research papers included in the study are the Technology Acceptance Model (TAM) and diffusion of innovation theories (Anouze and Alamro, 2019 ; Azad, 2016 ; Belanche et al., 2019 ; Payne et al., 2018 ; Sharma et al., 2015 , 2017 ). However, as customers continue to become accustomed to AI, it may be imperative to develop theories that go beyond its acceptance and adoption. Thus, we recommend investigating different variables (e.g., social influence and user trends) and methods (e.g., cross-cultural studies) that impact customers' relationship with AI. The gradual shift toward its customer-centric utilization has prompted the exploration of new dimensions of AI that influence customer experience. Going forward, it is important to understand the impact of AI on customers and how it can be used to improve customer experience.
Limitations and implications
This study had several limitations. During our inclusion/exclusion criteria, it is plausible that some AI/banking papers may have been missed because of the specific keywords used to curate our dataset. In addition, articles may have been missed due to the time when the data were collected, such as Manrai and Gupta ( 2022 ), who examined investors' perceptions of robo-advisors. Second, regarding theme identification, there may be a potential bias toward selecting themes, which may lead to misclassification. In addition, we acknowledge that the papers were extracted only from the WoS and Scopus databases, which may limit our access to certain peer-reviewed outlets.
This research provides insights for practitioners and marketers in the North American banking sector. To assist in the implementation of AI-based decision-making, we encourage banking professionals to consider further refining their use of AI in the credit scoring, analysis, and granting processes to minimize risk, reduce costs, and improve customer experience. However, in doing so, we recommend using AI not only to improve internal processes but also as a tool (e.g., chatbots) to improve customer service for low-complexity tasks, thereby directing employees' efforts to other business-impacting activities. Moreover, we recommend using AI as a marketing segmentation tool to target customers for optimal solutions.
This study systematically reviewed the literature (44 papers) on AI and banking from 2005 to 2020. We believe that our findings may benefit industry professionals and decision-makers in formulating strategic decisions regarding the different uses of AI in the banking sector, and optimizing the value derived from AI technologies. We advance the field by providing a more comprehensive outlook specific to the area of AI and banking, reflecting the history and future opportunities for AI in shaping business strategies, improving logistics processes, and enhancing customer value.
Biographies
has a Master of Science in Management from Toronto Metropolitan University.
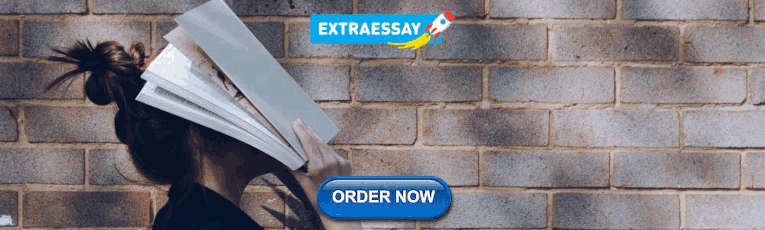
Dr. Irfan Butt
is Assistant Professor of Marketing at Toronto Metropolitan University.
Dr. Seung Hwan Mark Lee
is a Professor of Retail Management at Toronto Metropolitan University.
See Tables Tables2, 2 , ,3 3 and and4 4 .
Declarations
On behalf of all authors, the corresponding author states that there is no conflict of interest.
Publisher's Note
Springer Nature remains neutral with regard to jurisdictional claims in published maps and institutional affiliations.
Contributor Information
Omar H. Fares, Email: [email protected] .
Irfan Butt, Email: [email protected] .
Seung Hwan Mark Lee, Email: [email protected] .
- Agarwal, P., Swami, S., & Malhotra, S. K. 2022. Artificial intelligence adoption in the post COVID-19 new-normal and role of smart technologies in transforming business: a review. Journal of Science and Technology Policy Management .
- Akerkar R. Employing AI in business. In artificial intelligence for business. Cham: Springer; 2019. pp. 63–74. [ Google Scholar ]
- Akkoç S. An empirical comparison of conventional techniques, neural networks and the three-stage hybrid adaptive neuro fuzzy inference system (ANFIS) model for credit scoring analysis: the case of Turkish credit card data. European Journal of Operational Research. 2012; 222 (1):168–178. doi: 10.1016/j.ejor.2012.04.009. [ CrossRef ] [ Google Scholar ]
- Ala'raj M, Abbod MF. Classifiers consensus system approach for credit scoring. Knowledge-Based Systems. 2016; 104 :89–105. doi: 10.1016/j.knosys.2016.04.013. [ CrossRef ] [ Google Scholar ]
- Alborzi M, Khanbabaei M. Using data mining and neural networks techniques to propose a new hybrid customer behavior analysis and credit scoring model in banking services based on a developed RFM analysis method. International Journal of Business Information Systems. 2016; 23 (1):1–22. doi: 10.1504/IJBIS.2016.078020. [ CrossRef ] [ Google Scholar ]
- Anagnostopoulos I, Rizeq A. Confining value from neural networks: a sectoral study prediction of takeover targets in the US technology sector. Managerial Finance. 2019; 45 (10–11):1433–1457. doi: 10.1108/MF-12-2017-0523. [ CrossRef ] [ Google Scholar ]
- Anderson, J., Bholat, D., Gharbawi, M., & Thew, O. 2021. The impact of COVID-19 on artificial intelligence in banking. Bruegel-Blogs , NA-NA.
- Anouze ALM, Alamro AS. Factors affecting intention to use e-banking in Jordan. International Journal of Bank Marketing. 2019; 38 :86–112. doi: 10.1108/IJBM-10-2018-0271. [ CrossRef ] [ Google Scholar ]
- Arif I, Aslam W, Hwang Y. Barriers in adoption of internet banking: a structural equation modeling-neural network approach. Technology in Society. 2020; 61 :101231. doi: 10.1016/j.techsoc.2020.101231. [ CrossRef ] [ Google Scholar ]
- Azad MAK. Predicting mobile banking adoption in Bangladesh: a neural network approach. Transnational Corporations Review. 2016; 8 (3):207–214. doi: 10.1080/19186444.2016.1233726. [ CrossRef ] [ Google Scholar ]
- Baesens B, Van Gestel T, Stepanova M, Van den Poel D, Vanthienen J. Neural network survival analysis for personal loan data. Journal of the Operational Research Society. 2005; 56 (9):1089–1098. doi: 10.1057/palgrave.jors.2601990. [ CrossRef ] [ Google Scholar ]
- Baiden, J.E. 2011. The 5 C's of Credit in the Lending Industry. Available at SSRN 1872804.
- Bavaresco R, Silveira D, Reis E, Barbosa J, Righi R, Costa C, Moreira C. Conversational agents in business: a systematic literature review and future research directions. Computer Science Review. 2020; 36 :100239. doi: 10.1016/j.cosrev.2020.100239. [ CrossRef ] [ Google Scholar ]
- Belanche D, Casaló LV, Flavián C. Artificial intelligence in FinTech: understanding robo-advisors adoption among customers. Industrial Management Data Systems. 2019; 119 :1411–1430. doi: 10.1108/IMDS-08-2018-0368. [ CrossRef ] [ Google Scholar ]
- Bhatore S, Mohan L, Reddy YR. Machine learning techniques for credit risk evaluation: A systematic literature review. Journal of Banking and Financial Technology. 2020; 4 (1):111–138. doi: 10.1007/s42786-020-00020-3. [ CrossRef ] [ Google Scholar ]
- Bitner MJ, Ostrom AL, Morgan FN. Service blueprinting: A practical technique for service innovation. California Management Review. 2008; 50 (3):66–94. doi: 10.2307/41166446. [ CrossRef ] [ Google Scholar ]
- Borges AF, Laurindo FJ, Spínola MM, Gonçalves RF, Mattos CA. The strategic use of artificial intelligence in the digital era: Systematic literature review and future research directions. International Journal of Information Management. 2020; 57 :102225. doi: 10.1016/j.ijinfomgt.2020.102225. [ CrossRef ] [ Google Scholar ]
- Boyatzis RE. Transforming qualitative information: Thematic analysis and code development. Thousand Oaks, CA: Sage; 1998. [ Google Scholar ]
- Broby D. Financial technology and the future of banking. Financial Innovation. 2021; 7 (1):1–19. doi: 10.1186/s40854-021-00264-y. [ CrossRef ] [ Google Scholar ]
- Caron MS. The transformative effect of AI on the banking industry. Banking & Finance Law Review. 2019; 34 (2):169–214. [ Google Scholar ]
- Chatha KA, Butt I. Themes of study in manufacturing strategy literature. International Journal of Operations & Production Management. 2015; 35 (4):604–698. doi: 10.1108/IJOPM-07-2013-0328. [ CrossRef ] [ Google Scholar ]
- Chopra A, Bhilare P. Application of ensemble models in credit scoring models. Business Perspectives and Research. 2018; 6 (2):129–141. doi: 10.1177/2278533718765531. [ CrossRef ] [ Google Scholar ]
- Daqar MAA, Arqawi S. Fintech in the eyes of millennials and generation Z (the financial behavior and fintech perception) Banks and Bank Systems. 2020; 15 (3):20. doi: 10.21511/bbs.15(3).2020.03. [ CrossRef ] [ Google Scholar ]
- De Oliveira Santini F, Ladeira WJ, Sampaio CH, Perin MG. Online banking services: A meta-analytic review and assessment of the impact of antecedents and consequents on satisfaction. Journal of Financial Services Marketing. 2018; 23 (3):168–178. doi: 10.1057/s41264-018-0059-4. [ CrossRef ] [ Google Scholar ]
- Digalaki, E. 2022. The impact of artificial intelligence in the banking sector & how AI is being used in 2022. https://www.businessinsider.com/ai-in-banking-report?r=US&IR=T
- Dobrescu EM, Dobrescu EM. Artificial intelligence (Ai)-the technology that shapes the world. Global Economic Observer. 2018; 6 (2):71–81. [ Google Scholar ]
- Dushimimana Bernard, Wambui Yvonne, Lubega Timothy, McSharry Patrick E. Use of Machine Learning Techniques to Create a Credit Score Model for Airtime Loans. Journal of Risk and Financial Management. 2020; 13 (8):180. doi: 10.3390/jrfm13080180. [ CrossRef ] [ Google Scholar ]
- Ekinci Y, Uray N, Ülengin F. A customer lifetime value model for the banking industry: A guide to marketing actions. European Journal of Marketing. 2014; 48 (3–4):761–784. doi: 10.1108/EJM-12-2011-0714. [ CrossRef ] [ Google Scholar ]
- Eren BA. Determinants of customer satisfaction in chatbot use: Evidence from a banking application in Turkey. International Journal of Bank Marketing. 2021; 39 (2):294–331. doi: 10.1108/IJBM-02-2020-0056. [ CrossRef ] [ Google Scholar ]
- Fountain T, McCarthy B, Saleh T. Building the AI-powered organization technology isn't the biggest challenge, culture is. Harvard Business Review. 2019; 97 (4):62. [ Google Scholar ]
- Frączek B. A system to support the transparency of consumer credit offers. Journal of Risk and Financial Management. 2020; 13 (12):317. doi: 10.3390/jrfm13120317. [ CrossRef ] [ Google Scholar ]
- Gallego-Gomez C, De-Pablos-Heredero C. Artificial intelligence as an enabling tool for the development of dynamic capabilities in the banking industry. International Journal of Enterprise Information Systems (IJEIS) 2020; 16 (3):20–33. doi: 10.4018/IJEIS.2020070102. [ CrossRef ] [ Google Scholar ]
- Goyal K, Kumar S. Financial literacy: A systematic review and bibliometric analysis. International Journal of Consumer Studies. 2021; 45 (1):80–105. doi: 10.1111/ijcs.12605. [ CrossRef ] [ Google Scholar ]
- Guotai C, Abedin MZ, Moula FE. Modeling credit approval data with neural networks: An experimental investigation and optimization. Journal of Business Economics and Management. 2017; 18 (2):224–240. doi: 10.3846/16111699.2017.1280844. [ CrossRef ] [ Google Scholar ]
- Harzing AW, Alakangas S. Google scholar, scopus and the web of science: A longitudinal and cross-disciplinary comparison. Scientometrics. 2016; 106 (2):787–804. doi: 10.1007/s11192-015-1798-9. [ CrossRef ] [ Google Scholar ]
- Hua X, Huang Y, Zheng Y. Current practices, new insights, and emerging trends of financial technologies. Industrial Management & Data Systems. 2019; 119 (7):1401–1410. doi: 10.1108/IMDS-08-2019-0431. [ CrossRef ] [ Google Scholar ]
- Ince H, Aktan B. A comparison of data mining techniques for credit scoring in banking: A managerial perspective. Journal of Business Economics and Management. 2009; 10 (3):233–240. doi: 10.3846/1611-1699.2009.10.233-240. [ CrossRef ] [ Google Scholar ]
- Jagtiani J, Lemieux C. The roles of alternative data and machine learning in fintech lending: Evidence from the LendingClub consumer platform. Financial Management. 2019; 48 (4):1009–1029. doi: 10.1111/fima.12295. [ CrossRef ] [ Google Scholar ]
- Jakšič M, Marinč M. Relationship banking and information technology: The role of artificial intelligence and FinTech. Risk Management. 2019; 21 (1):1–18. doi: 10.1057/s41283-018-0039-y. [ CrossRef ] [ Google Scholar ]
- Jindal N. The impact of advertising and R&D on bankruptcy survival: A double-edged sword. Journal of Marketing. 2020; 84 (5):22–40. doi: 10.1177/0022242920936205. [ CrossRef ] [ Google Scholar ]
- Jöhnk J, Weißert M, Wyrtki K. Ready or not, AI comes—an interview study of organizational AI readiness factors. Business & Information Systems Engineering. 2021; 63 (1):5–20. doi: 10.1007/s12599-020-00676-7. [ CrossRef ] [ Google Scholar ]
- Kao LJ, Chiu CC, Chiu FY. A Bayesian latent variable model with classification and regression tree approach for behavior and credit scoring. Knowledge-Based Systems. 2012; 36 :245–252. doi: 10.1016/j.knosys.2012.07.004. [ CrossRef ] [ Google Scholar ]
- Khan KS, Kunz R, Kleijnen J, Antes G. Five steps to conducting a systematic review. Journal of the Royal Society of Medicine. 2003; 96 (3):118–121. doi: 10.1177/014107680309600304. [ PMC free article ] [ PubMed ] [ CrossRef ] [ Google Scholar ]
- Khandani AE, Kim AJ, Lo AW. Consumer credit-risk models via machine-learning algorithms. Journal of Banking & Finance. 2010; 34 (11):2767–2787. doi: 10.1016/j.jbankfin.2010.06.001. [ CrossRef ] [ Google Scholar ]
- Kim KJ, Lee WB. Stock market prediction using artificial neural networks with optimal feature transformation. Neural Computing & Applications. 2004; 13 (3):255–260. doi: 10.1007/s00521-004-0428-x. [ CrossRef ] [ Google Scholar ]
- Kok JN, Boers EJ, Kosters WA, Van der Putten P, Poel M. Artificial intelligence: Definition, trends, techniques, and cases. Artificial Intelligence. 2009; 1 :270–299. [ Google Scholar ]
- Königstorfer F, Thalmann S. Applications of artificial intelligence in commercial banks–a research agenda for behavioral finance. Journal of Behavioral and Experimental Finance. 2020; 27 :100352. doi: 10.1016/j.jbef.2020.100352. [ CrossRef ] [ Google Scholar ]
- Koutanaei FN, Sajedi H, Khanbabaei M. A hybrid data mining model of feature selection algorithms and ensemble learning classifiers for credit scoring. Journal of Retailing and Consumer Services. 2015; 27 :11–23. doi: 10.1016/j.jretconser.2015.07.003. [ CrossRef ] [ Google Scholar ]
- Larson EJ. The myth of artificial intelligence. In The Myth of Artificial Intelligence: Harvard University Press; 2021. [ Google Scholar ]
- Leximancer. (2019, November 25). Leximancer User Guide Release 5.0. Leximancer. https://static1.squarespace.com/static/539bebd7e4b045b6dc97e4f7/t/5e58d901137e3077d4409092/1582881372656/LeximancerUserGuide5.pdf .
- Loureiro SMC, Guerreiro J, Tussyadiah I. Artificial intelligence in business: State of the art and future research agenda. Journal of Business Research. 2020 doi: 10.1016/j.jbusres.2020.11.001. [ CrossRef ] [ Google Scholar ]
- Malali AB, Gopalakrishnan S. Application of artificial intelligence and its powered technologies in the indian banking and financial industry: An overview. IOSR Journal of Humanities and Social Science. 2020; 25 (4):55–60. [ Google Scholar ]
- Mall S. An empirical study on credit risk management: The case of nonbanking financial companies. Journal of Credit Risk. 2018; 14 (3):49–66. doi: 10.21314/JCR.2017.239. [ CrossRef ] [ Google Scholar ]
- Manrai R, Gupta KP. Investor's perceptions on artificial intelligence (AI) technology adoption in investment services in India. Journal of Financial Services Marketing. 2022 doi: 10.1057/s41264-021-00134-9. [ CrossRef ] [ Google Scholar ]
- Marinakos G, Daskalaki S. Imbalanced customer classification for bank direct marketing. Journal of Marketing Analytics. 2017; 5 (1):14–30. doi: 10.1057/s41270-017-0013-7. [ CrossRef ] [ Google Scholar ]
- McCarthy, J., Minsky, M.L., & Rochester, N. 1956. The Dartmouth summer research project on artificial intelligence. Artificial intelligence: past, present, and future. Available at: http://www.dartmouth.edu/*vox/0607/ 0724/ai50.html
- Medhi PK, Mondal S. A neural feature extraction model for classification of firms and prediction of outsourcing success: Advantage of using relational sources of information for new suppliers. International Journal of Production Research. 2016; 54 (20):6071–6081. doi: 10.1080/00207543.2016.1174342. [ CrossRef ] [ Google Scholar ]
- Mehrotra, A. (2019, April). Artificial Intelligence in Financial Services–Need to Blend Automation with Human Touch. In 2019 International Conference on Automation, Computational and Technology Management (ICACTM) (pp. 342–347). IEEE.
- Mohapatra S. Human and computer interaction in information system design for managing business. Information Systems and e-Business Management. 2020 doi: 10.1007/s10257-020-00475-3. [ CrossRef ] [ Google Scholar ]
- Moher, D., Liberati, A., Tetzlaff, J., Altman, D.G., & Prisma Group. 2009. Preferred reporting items for systematic reviews and meta-analyses: the PRISMA statement. PLoS medicine , 6(7), e1000097 [ PMC free article ] [ PubMed ]
- Mongeon P, Paul-Hus A. The journal coverage of Web of Science and Scopus: A comparative analysis. Scientometrics. 2016; 106 (1):213–228. doi: 10.1007/s11192-015-1765-5. [ CrossRef ] [ Google Scholar ]
- Muñoz-Izquierdo N, Camacho-Miñano MDM, Segovia-Vargas MJ, Pascual-Ezama D. Is the external audit report useful for bankruptcy prediction? Evidence using artificial intelligence. International Journal of Financial Studies. 2019; 7 (2):20. doi: 10.3390/ijfs7020020. [ CrossRef ] [ Google Scholar ]
- Nusair K, Butt I, Nikhashemi SR. A bibliometric analysis of social media in hospitality and tourism research. International Journal of Contemporary Hospitality Management. 2019; 31 :2691–2719. doi: 10.1108/IJCHM-06-2018-0489. [ CrossRef ] [ Google Scholar ]
- Olson DL, Delen D, Meng Y. Comparative analysis of data mining methods for bankruptcy prediction. Decision Support Systems. 2012; 52 (2):464–473. doi: 10.1016/j.dss.2011.10.007. [ CrossRef ] [ Google Scholar ]
- Pahlevan-Sharif S, Mura P, Wijesinghe SN. A systematic review of systematic reviews in tourism. Journal of Hospitality and Tourism Management. 2019; 39 :158–165. doi: 10.1016/j.jhtm.2019.04.001. [ CrossRef ] [ Google Scholar ]
- Payne EM, Peltier JW, Barger VA. Mobile banking and AI-enabled mobile banking: The differential effects of technological and non-technological factors on digital natives' perceptions and behavior. Journal of Research in Interactive Marketing. 2018; 12 (3):328–346. doi: 10.1108/JRIM-07-2018-0087. [ CrossRef ] [ Google Scholar ]
- Payne EH, Peltier J, Barger VA. Enhancing the value co-creation process: Artificial intelligence and mobile banking service platforms. Journal of Research in Interactive Marketing. 2021; 15 :68–85. doi: 10.1108/JRIM-10-2020-0214. [ CrossRef ] [ Google Scholar ]
- Rajaobelina L, Ricard L. Classifying potential users of live chat services and chatbots. Journal of Financial Services Marketing. 2021; 26 (2):81–94. doi: 10.1057/s41264-021-00086-0. [ CrossRef ] [ Google Scholar ]
- Ransbotham, S., Kiron, D., Gerbert, P., & Reeves, M. 2017. Reshaping business with artificial intelligence closing the gap between ambition and action. MIT Sloan Management Review , 59(1).
- Rantanen A, Salminen J, Ginter F, Jansen BJ. Classifying online corporate reputation with machine learning: A study in the banking domain. Internet Research. 2019; 30 :45–66. doi: 10.1108/INTR-07-2018-0318. [ CrossRef ] [ Google Scholar ]
- Schwartz EM, Bradlow ET, Fader PS. Customer acquisition via display advertising using multi-armed bandit experiments. Marketing Science. 2017; 36 (4):500–522. doi: 10.1287/mksc.2016.1023. [ CrossRef ] [ Google Scholar ]
- Sharma SK, Govindaluri SM, Al Balushi SM. Predicting determinants of Internet banking adoption. Management Research Review. 2015; 38 (7):750–766. doi: 10.1108/MRR-06-2014-0139. [ CrossRef ] [ Google Scholar ]
- Sharma, S.K., Govindaluri, S.M., Al-Muharrami, S., & Tarhini, A. 2017. A multi-analytical model for mobile banking adoption: a developing country perspective. Review of International Business and Strategy .
- Shostack GL. How to design a service. European Journal of Marketing. 1982; 16 (1):49–63. doi: 10.1108/EUM0000000004799. [ CrossRef ] [ Google Scholar ]
- Smeureanu I, Ruxanda G, Badea LM. Customer segmentation in private banking sector using machine learning techniques. Journal of Business Economics and Management. 2013; 14 (5):923–939. doi: 10.3846/16111699.2012.749807. [ CrossRef ] [ Google Scholar ]
- Soltani M, Samorani M, Kolfal B. Appointment scheduling with multiple providers and stochastic service times. European Journal of Operational Research. 2019; 277 (2):667–683. doi: 10.1016/j.ejor.2019.02.051. [ CrossRef ] [ Google Scholar ]
- Tarafdar M, Beath CM, Ross JW. Using AI to enhance business operations. MIT Sloan Management Review. 2019; 60 (4):37–44. [ Google Scholar ]
- Tian Z, Zhong RY, Vatankhah Barenji A, Wang YT, Li Z, Rong Y. A blockchain-based evaluation approach for customer delivery satisfaction in sustainable urban logistics. International Journal of Production Research. 2020; 59 :1–21. [ Google Scholar ]
- Tranfield D, Denyer D, Smart P. Towards a methodology for developing evidence-informed management knowledge by means of systematic review. British Journal of Management. 2003; 14 (3):207–222. doi: 10.1111/1467-8551.00375. [ CrossRef ] [ Google Scholar ]
- Trivedi J. Examining the customer experience of using banking Chatbots and its impact on brand love: The moderating role of perceived risk. Journal of Internet Commerce. 2019; 18 (1):91–111. doi: 10.1080/15332861.2019.1567188. [ CrossRef ] [ Google Scholar ]
- Tseng, C.C. (2003, July). Comparing artificial intelligence systems for stock portfolio selection. In The 9th international conference of computing in economics and finance (pp. 1–7).
- Vahid PR, Ahmadi A. Modeling corporate customers' credit risk considering the ensemble approaches in multiclass classification: Evidence from Iranian corporate credits. Journal of Credit Risk. 2016; 12 (3):71–95. doi: 10.21314/JCR.2016.213. [ CrossRef ] [ Google Scholar ]
- Valsamidis, S., Tsourgiannis, L., Pappas, D., & Mosxou, E. 2020. Digital banking in the new Era: Exploring customers' attitudes. In Business performance and financial institutions in Europe (pp. 91–104). Springer, Cham.
- Verma S, Sharma R, Deb S, Maitra D. Artificial intelligence in marketing: Systematic review and future research direction. International Journal of Information Management Data Insights. 2021; 1 :100002. doi: 10.1016/j.jjimei.2020.100002. [ CrossRef ] [ Google Scholar ]
- Watkins DC. Rapid and rigorous qualitative data analysis: The "RADaR" technique for applied research. International Journal of Qualitative Methods. 2017; 16 (1):1609406917712131. doi: 10.1177/1609406917712131. [ CrossRef ] [ Google Scholar ]
- Wu, D.D., & Olson, D.L. 2020. The effect of COVID-19 on the banking sector. In Pandemic risk management in operations and finance (pp. 89–99). Springer, Cham.
- Xiao Y, Watson M. Guidance on conducting a systematic literature review. Journal of Planning Education and Research. 2019; 39 (1):93–112. doi: 10.1177/0739456X17723971. [ CrossRef ] [ Google Scholar ]
- Xu Y, Shieh CH, van Esch P, Ling IL. AI customer service: Task complexity, problem-solving ability, and usage intention. Australasian Marketing Journal. 2020; 28 (4):189–199. doi: 10.1016/j.ausmj.2020.03.005. [ CrossRef ] [ Google Scholar ]
- Yang AS. Exploring adoption difficulties in mobile banking services. Canadian Journal of Administrative Sciences/revue Canadienne Des Sciences De L'administration. 2009; 26 (2):136–149. doi: 10.1002/cjas.102. [ CrossRef ] [ Google Scholar ]
- Zeinalizadeh N, Shojaie AA, Shariatmadari M. Modeling and analysis of bank customer satisfaction using neural networks approach. International Journal of Bank Marketing. 2015; 33 (6):717–732. doi: 10.1108/IJBM-06-2014-0070. [ CrossRef ] [ Google Scholar ]

Artificial Intelligence in Banking Systems: Trends and Possible Consequences of Implementation
79 citations
52 citations
33 citations
3 citations
Related Papers (5)
Trending questions (1).
The paper does not provide information about adapting AI with a theoretical framework in banks.
Ask Copilot
Related papers
Related topics
Artificial Intelligence in Banking sector: Evidence from Bahrain
Ieee account.
- Change Username/Password
- Update Address
Purchase Details
- Payment Options
- Order History
- View Purchased Documents
Profile Information
- Communications Preferences
- Profession and Education
- Technical Interests
- US & Canada: +1 800 678 4333
- Worldwide: +1 732 981 0060
- Contact & Support
- About IEEE Xplore
- Accessibility
- Terms of Use
- Nondiscrimination Policy
- Privacy & Opting Out of Cookies
A not-for-profit organization, IEEE is the world's largest technical professional organization dedicated to advancing technology for the benefit of humanity. © Copyright 2024 IEEE - All rights reserved. Use of this web site signifies your agreement to the terms and conditions.
Artificial Intelligence Act: MEPs adopt landmark law
Share this page: .
- Safeguards on general purpose artificial intelligence
- Limits on the use of biometric identification systems by law enforcement
- Bans on social scoring and AI used to manipulate or exploit user vulnerabilities
- Right of consumers to launch complaints and receive meaningful explanations

On Wednesday, Parliament approved the Artificial Intelligence Act that ensures safety and compliance with fundamental rights, while boosting innovation.
The regulation, agreed in negotiations with member states in December 2023, was endorsed by MEPs with 523 votes in favour, 46 against and 49 abstentions.
It aims to protect fundamental rights, democracy, the rule of law and environmental sustainability from high-risk AI, while boosting innovation and establishing Europe as a leader in the field. The regulation establishes obligations for AI based on its potential risks and level of impact.
Banned applications
The new rules ban certain AI applications that threaten citizens’ rights, including biometric categorisation systems based on sensitive characteristics and untargeted scraping of facial images from the internet or CCTV footage to create facial recognition databases. Emotion recognition in the workplace and schools, social scoring, predictive policing (when it is based solely on profiling a person or assessing their characteristics), and AI that manipulates human behaviour or exploits people’s vulnerabilities will also be forbidden.
Law enforcement exemptions
The use of biometric identification systems (RBI) by law enforcement is prohibited in principle, except in exhaustively listed and narrowly defined situations. “Real-time” RBI can only be deployed if strict safeguards are met, e.g. its use is limited in time and geographic scope and subject to specific prior judicial or administrative authorisation. Such uses may include, for example, a targeted search of a missing person or preventing a terrorist attack. Using such systems post-facto (“post-remote RBI”) is considered a high-risk use case, requiring judicial authorisation being linked to a criminal offence.
Obligations for high-risk systems
Clear obligations are also foreseen for other high-risk AI systems (due to their significant potential harm to health, safety, fundamental rights, environment, democracy and the rule of law). Examples of high-risk AI uses include critical infrastructure, education and vocational training, employment, essential private and public services (e.g. healthcare, banking), certain systems in law enforcement, migration and border management, justice and democratic processes (e.g. influencing elections). Such systems must assess and reduce risks, maintain use logs, be transparent and accurate, and ensure human oversight. Citizens will have a right to submit complaints about AI systems and receive explanations about decisions based on high-risk AI systems that affect their rights.
Transparency requirements
General-purpose AI (GPAI) systems, and the GPAI models they are based on, must meet certain transparency requirements, including compliance with EU copyright law and publishing detailed summaries of the content used for training. The more powerful GPAI models that could pose systemic risks will face additional requirements, including performing model evaluations, assessing and mitigating systemic risks, and reporting on incidents.
Additionally, artificial or manipulated images, audio or video content (“deepfakes”) need to be clearly labelled as such.
Measures to support innovation and SMEs
Regulatory sandboxes and real-world testing will have to be established at the national level, and made accessible to SMEs and start-ups, to develop and train innovative AI before its placement on the market.
During the plenary debate on Tuesday, the Internal Market Committee co-rapporteur Brando Benifei (S&D, Italy) said: “We finally have the world’s first binding law on artificial intelligence, to reduce risks, create opportunities, combat discrimination, and bring transparency. Thanks to Parliament, unacceptable AI practices will be banned in Europe and the rights of workers and citizens will be protected. The AI Office will now be set up to support companies to start complying with the rules before they enter into force. We ensured that human beings and European values are at the very centre of AI’s development”.
Civil Liberties Committee co-rapporteur Dragos Tudorache (Renew, Romania) said: “The EU has delivered. We have linked the concept of artificial intelligence to the fundamental values that form the basis of our societies. However, much work lies ahead that goes beyond the AI Act itself. AI will push us to rethink the social contract at the heart of our democracies, our education models, labour markets, and the way we conduct warfare. The AI Act is a starting point for a new model of governance built around technology. We must now focus on putting this law into practice”.
The regulation is still subject to a final lawyer-linguist check and is expected to be finally adopted before the end of the legislature (through the so-called corrigendum procedure). The law also needs to be formally endorsed by the Council.
It will enter into force twenty days after its publication in the official Journal, and be fully applicable 24 months after its entry into force, except for: bans on prohibited practises, which will apply six months after the entry into force date; codes of practise (nine months after entry into force); general-purpose AI rules including governance (12 months after entry into force); and obligations for high-risk systems (36 months).
The Artificial Intelligence Act responds directly to citizens’ proposals from the Conference on the Future of Europe (COFE), most concretely to proposal 12(10) on enhancing EU’s competitiveness in strategic sectors, proposal 33(5) on a safe and trustworthy society, including countering disinformation and ensuring humans are ultimately in control, proposal 35 on promoting digital innovation, (3) while ensuring human oversight and (8) trustworthy and responsible use of AI, setting safeguards and ensuring transparency, and proposal 37 (3) on using AI and digital tools to improve citizens’ access to information, including persons with disabilities.
Contacts:
Yasmina yakimova .
- Phone number: (+32) 2 28 42626 (BXL)
- Mobile number: (+32) 470 88 10 60
- E-mail: [email protected]
- Twitter account: @EP_SingleMarket
Janne OJAMO
- Phone number: (+32) 2 284 12 50 (BXL)
- Mobile number: (+32) 470 89 21 92
- E-mail: [email protected]
- Twitter account: @EP_Justice
Further information
- Link to adopted text (13.03.2024)
- Plenary debate (12.03.2024)
- Procedure file
- EP Research Service: compilation of studies on Artificial Intelligence
- Result of roll-call votes (13.03.2024)
- Committee on the Internal Market and Consumer Protection
- Committee on Civil Liberties, Justice and Home Affairs
Product information
More From Forbes
Artificial general intelligence or agi: a very short history.
- Share to Facebook
- Share to Twitter
- Share to Linkedin
Artificial General Intelligence, AGI concept. AI can learn and solve any human's intellectual tasks.
AGI is the new AI, promoted by tech leaders and AI experts , all promising its imminent arrival, for better or for worse. Anyone frightened by Elon Musk’s warning that “AGI poses a grave threat to humanity, perhaps the greatest existential threat we face today,” should first study the evolution of AGI from science-fiction to real-world fiction.
The term AGI was coined in 2007 when a collection of essays on the subject was published. The book, titled Artificial General intelligence , was co-edited by Ben Goertzel and Cassio Pennachin. In their introduction, they provided a definition:
“AGI is, loosely speaking, AI systems that possess a reasonable degree of self-understanding and autonomous self-control, and have the ability to solve a variety of complex problems in a variety of contexts, and to learn to solve new problems that they didn’t know about at the time of their creation.” The rationale for “christening” AGI for Goertzel and Pennachin was to distinguish it from “run-of-the-mill ‘artificial intelligence’ research,” as AGI is “explicitly focused on engineering general intelligence in the short term.”
In 2007, “run-of-the-mill” research focused on narrow challenges and AI programs of the time could only “generalize within their limited context.” While “work on AGI has gotten a bit of a bad reputation,” according to Goertzel and Pennachin, “AGI appears by all known science to be quite possible. Like nanotechnology, it is ‘merely an engineering problem’, though certainly a very difficult one.”
AGI is considered by Goertzel and Pennachin as only an engineering challenge because “we know that general intelligence is possible, in the sense that humans – particular configurations of atoms – display it. We just need to analyze these atom configurations in detail and replicate them in the computer.”
Best High-Yield Savings Accounts Of 2024
Best 5% interest savings accounts of 2024.
Goertzel and Pennachin seem to contradict themselves when they also assert that the Japanese 5 th generation Computer System project “was doomed by its pure engineering approach, by its lack of an underlying theory of mind.” But maybe there’s no contradiction here because they assume that the mind is also a collection of atoms that can be emulated in a computer by the right engineering approach: “We have several contributions in this book that are heavily based on cognitive psychology and its ideas about how the mind works. These contributions pay greater than zero attention to neuroscience, but they are clearly more mind-focused than brain-focused.”
The brain-focused approach presented in the book is “a neural net based approach, trying to model the behavior of nerve cells in the brain and the emergence of intelligence therefrom. Or one can proceed at a higher level, looking at the general ways that information processing is carried out in the brain, and seeking to emulate these in software.”
This was written, of course, when the real fringe of the AI community—ignored in this 2007 book—were the handful of people (e.g., 2018 Turing Award winners Geoffrey Hinton, Yann LeCun and Joshua Bengio) who in 2007 coined the term “deep learning” to describe their machine learning approach to finding patterns in lots of data using statistical analysis algorithms. These have been called since the 1950s “artificial neural networks,” algorithms that have been presented throughout the years with no empirical evidence as “mimicking the brain.”
In 2007, the people that were the first to discuss various approaches to achieving the newly-termed “AGI” completely ignored the fringe approach to “AI” that in 2012 became the mainstream approach to AI with the successful marriage of GPUs, lots of data, and artificial neural networks. Still, the researchers previously on the fringe of AI and now the kings of the data mountain understood well the branding and marketing power of “AGI” and continued in the exalted tradition of promising the imminent arrival of machines with human-like intelligence (or superintelligence) and the possible extinction of humanity by these possibly malevolent machines.
The key person in the importation of this tradition to the new successful approach to AI was apparently Shane Legg, a co-founder of DeepMind. Legg suggested to Goertzel the term “Artificial General Intelligence” and described to Cade Metz (who quoted Legg in his book Genius Makers ) the general attitude to the subject in the AI community around 2007: “If you talked to anybody about general AI, you would be considered at best eccentric, at worst some kind of delusional, nonscientific character.”
Aspiring to build superintelligence while worrying about what it could do to humanity, Legg joined his colleague Demis Hassabis (they were exploring the connections between the brain and machine learning at UCL) to establish DeepMind. Hassabis told Legg that “they could raise far kore money from venture capitalists than they ever could writing grant proposals as professors,” Metz reports. With AGI as the stated aim of DeepMind, mentioned in the first line of their business plan, “they told anyone who would listen, including potential investors, that this research could be dangerous.”
To get to Peter Thiel, their first investor, Hassabis gave a presentation at the 2010 Singularity Conference, arguing that the best way to build artificial intelligence was to mimic the way the brain worked: “We should be focusing on the algorithmic level of the brain, extracting the kind of representations and algorithms the brain uses to solve the kind of problems we want to solve with AGI.”
There you have it. Using the term “AGI”—with its exciting connotations of both saving and destroying humanity—to get the attention and deep pockets of investors, claiming to replicate the human brain in the computer while pursuing a statistical analysis method that has nothing to do empirically speaking with how the human brain works .
Whether insisting that their approach to AI resembles the biological processes of the human brain (“connectionism”) or that they can replicate the process of human thinking in the computer (“symbolic AI”), the two key approaches to AI since the term was coined in 1955 have banked on the widely accepted notion that “ we are as gods .” This belief in modern man’s ability to conquer all frontiers, even replicate man in the machine, has been based on the centuries-old idea that humans are a “particular configurations of atoms.”
Next in my AGI Washing Series, I will offer a short pre-history of AGI.
- Editorial Standards
- Reprints & Permissions

- Commercial Lending
- Community Banking
- Compliance and Risk
- Cybersecurity
- Human Resources
- Mutual Funds
- Retail and Marketing
- Tax and Accounting
- Wealth Management
- Magazine Archive
- Newsletter Archive
- Sponsored Archive
- Podcast Archive

Two investment advisers agree to pay $400,000 to resolve SEC AI washing allegations
Issue: Delphia (USA) Inc. and Global Predictions Inc.’s consent orders with the Securities and Exchange Commission (SEC) related to artificial intelligence (AI) washing allegations.
Case Summary: Delphia (USA) Inc. and Global Predictions Inc. agreed to pay a combined $400,000 to resolve SEC allegations that it participated in artificial intelligence washing. AI washing is the use of deceptive and inaccurate claims about a company’s use of AI or machine-learning capabilities to capitalize on the publicity surrounding the technology.
The SEC alleged Delphia made false and misleading statements in its regulatory filings, advertisements, and social media related to its purported use of AI and machine-learning from August 2019 to August 2023. Delphia is an investment adviser formerly registered with the SEC. According to the SEC, Delphia represented it used AI and machine-learning in its Form ADV Part 2A brochures, in a press release, and on its website. Delphia allegedly articulated its advice was “powered by the insights it makes when individuals … connect their social media, banking, and other accounts … or respond to Delphia’s questionnaires,” which make its investment decisions “more robust and accurate.” Additionally, Delphia allegedly declared that client data was used in “predictive algorithmic model” for the selection of investments. The SEC alleged that no such data was used in its investment process.
The SEC’s Division of Examination conducted an examination and found that Delphia did not create an algorithm to use client data, nor did it otherwise use the client data. Additionally, the SEC alleged Delphia failed to adopt and implement written policies and procedures reasonably designed to prevent violations of the Advisers Act and corresponding rules. As a result, the SEC claimed Delphia willfully violated Sections 206(2) and 206(4) of the Advisers Act and Rules 206(4)-1 and 206(4)-7.
The SEC also alleged Global Predictions Inc. made false and misleading claims about its use of AI, its status as the “first regulated AI financial advisor,” and the services it offered. Global Predictions registered with the SEC as an internet investment adviser on Aug. 14, 2023. The SEC claimed Global Predictions: was unable to substantiate performance claims upon demand by the commission; failed to disclose material conflicts of interest resulting from its relationships with certain individuals giving testimonial; and advertised hypothetical performance on its public website without adopting and implementing the policies and procedures required by Advisers Act Rule 206(4)-1.
To comply with the SEC’s order, Delphia and Global Predictions agreed to pay $225,000 and $175,000 respectively in civil money penalties. The companies also agreed to cease and desist from committing or causing any violation and any future violations of Sections 206(2) and 206(4) of the Advisers Act and Rules 206(4)-1 and 206(4)-7.
Bottom Line: Neither Delphia nor Global Predictions admitted to or denied the SEC’s findings.
Documents: Delphia Consent Order , Global Predictions Consent Order
Related Posts
Lender trade groups sue challenging colorado’s ‘rate opt-out law’.
The National Association of Industrial Bankers, American Financial Services Association and American Fintech Council sued Colorado Attorney General Philip Weiser...
New York federal court determines reasonableness of investigation into alleged identity theft is a factual question under FCRA
A New York federal court denied a motion for summary judgment filed by HSBC in an FCRA lawsuit because the...
Third Circuit rules student loan trusts subject to CFPB enforcement authority
In a 3-0 decision, a Third Circuit panel concluded student loan trusts are subject to CFPB enforcement under the Consumer...
Virginia district court dismisses foreign currency swap overcharge lawsuit against Capital One
A Virginia federal district court dismissed a lawsuit alleging Capital One overcharged credit and debit card customers on transactions made...
SEC climate disclosure final rule lawsuit to be heard in 8th Circuit
The Eighth Circuit will determine whether the Securities and Exchange Commission’s climate disclosure final rule violates the Administrative Procedure Act.
Alabama district court rules Corporate Transparency Act is unconstitutional
An Alabama federal district court held that the Corporate Transparency Act is unconstitutional because it exceeds Congress’ enumerated powers.
Fed’s Bowman: Proposed bank merger reforms step in wrong direction
Bank survey: middle-income americans feeling more financially secure, union state in iowa to purchase exchange state; capital bancorp to acquire ifh, factory orders increase in february, new orders for manufactured goods increased in february, strategy, not serendipity: three steps to better creative, sponsored content.

ERM Model Risk and AI

The Federal Reserve’s Nick Stanescu shares what’s next for the FedNow® Service

AI Compliance and Regulation: What Financial Institutions Need to Know

Gain Efficiencies and Other Timely Benefits with Data Analytics
American Bankers Association 1333 New Hampshire Ave NW Washington, DC 20036 1-800-BANKERS (800-226-5377) www.aba.com About ABA Privacy Policy Contact ABA

© 2024 American Bankers Association. All rights reserved.
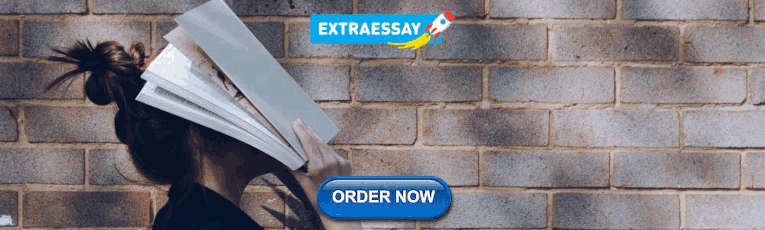
IMAGES
VIDEO
COMMENTS
As a result of increased adoption of new technical advancements, the artificial intelligence industry has grown at an unprecedented rate and is now being implemented in a wide variety of fields. Artificial intelligence systems have the potential to alter the banking industry's whole operations and to enhance the banks' performance.
This essay addresses the possible advantages of artificial intelligence in the banking sector. The study utilized a Systematic Literature Review (SLR) to evaluate the current literature on AI in ...
Applications of AI in Banking. The three main channels where banks can use artificial intelligence to save on costs are front office (conversational banking), middle office (fraud detection and ...
While the banking sector has long been technology-dependent and data-intensive, new data-enabled AI technology has the capability to drive innovation further and faster than ever before. AI can help improve efficiency, enable a growth agenda, boost differentiation, manage risk and regulatory needs, and positively influence customer experience.
In 2016, AlphaGo, a machine, defeated 18-time world champion Lee Sedol at the game of Go, a complex board game requiring intuition, imagination, and strategic thinking—abilities long considered distinctly human. Since then, artificial intelligence (AI) technologies have advanced even further, 1 AI can be defined as the ability of a machine to perform cognitive functions associated with human ...
The primary aim of this systematic literature review (SLR) was to identify, assess and synthesize the extant evidence about the opportunities and challenges concerning the use of Artificial Intelligence (AI) in the banking sector. From the SLR, it is evident that AI has several opportunities for the sector.
Artificial Intelligence has numerous advantages to offer for the financial segment. Based on the findings, it can be concluded that Artificial Intelligence in Banking and Financial Services satisfying their clients or consumer's needs. Banking and Financial Services consumers have good awareness about Artificial Intelligence applications.
Artificial intelligence has come a long way since English mathematician, logician, and cryptographer Alan Turing's seminal 1950 essay, "Computing Machinery and Intelligence," which explored the idea of building computers capable of imitating human thought.In 1997, almost 50 years after Turing's essay, AI posted a historic breakthrough when the IBM supercomputer Deep Blue won a chess match ...
The McKinsey Global Institute (MGI) estimates that across the global banking sector, gen AI could add between $200 billion and $340 billion in value annually, or 2.8 to 4.7 percent of total industry revenues, largely through increased productivity. 1 "The economic potential of generative AI: The next productivity frontier," McKinsey, June 14, 2023.
Central bank collaboration, for instance through knowledge-sharing and pooling of expertise, holds great promise in keeping central banks at the vanguard of developments in artificial intelligence. Long before artificial intelligence (AI) became a focal point of popular commentary and widespread fascination, central banks were early adopters of ...
The old traditional manual banking has been now transformed into the new technology-based automatic banking with easy access to all the services 24*7 and 365 days. Almost all the banks are using their AI-based chatbots such as Sia [5], Baroda Brainy, Digital Lab, EVA, and emPower.
The simulation of human intelligence in machines, called Artificial intelligence, has risen, and plays an important role in the new banking era. The present study aims to discuss the consumer's perspective on artificial intelligence's adoption in Asian countries. The questionnaire was developed and distributed to collect data from five Asian countries (Pakistan, China, Iran, Saudi Arabia ...
This study provides a holistic and systematic review of the literature on the utilization of artificial intelligence (AI) in the banking sector since 2005. In this study, the authors examined 44 articles through a systematic literature review approach and conducted a thematic and content analysis on them. This review identifies research themes ...
(DOI: 10.1007/978-981-19-7411-3_37) The paper aims to form a pattern for assessing the use of artificial intelligence (AI) in banking systems, which allows consistently analyzing the application of AI at all levels of these systems and identifying the possible consequences of the introduction of AI in banking systems. The methodological basis of the study includes a systemic approach, methods ...
Abstract: Recently, there has been a strong wave of digital transformation to the banking sector worldwide. In this paper, we explore using one of the emerging technologies, being Artificial intelligence, in banks in Bahrain. We find that only a few banks have utilized Artificial intelligence applications and that the robotic process automation and chatbots are the most employed Artificial ...
This essay aims to discuss some aspects and reasons for using Artificial Intelligence as an advanced issue in the banking sector. Introduction and Overview Basically, defining artificial ...
Abstract. Artificial Intelligence (AI) is a fast developing technology across the world. The banking sector is becoming one of the first adopters of Artificial Intelligence. Banks are exploring ...
of implementation in financial sector including SME banking services. This research seeks to map the AI implementation and its present state in the banking and financial sectors in India. Keywords: Artificial Intelligence, AI adaptation, Machine Learning, Diffusion, S-curve, Cyber Attack. 1. Introduction . As India moves towards the new economy ...
Cost Reduction. Banks could save a humongous $447 billion by 2023 by deploying artificial intelligence (AI), as stated by AI IN BANKING research report from Business Insider Intelligence. Employment of AI allows banks the scope of cutting down on 3 main areas: Reduces cycle time.
Although artificial intelligence is studied in e-health Mahdi, Battineni [8] and education setting however little research is found that talked about artificial intelligence enabled digital banking. Therefore, current study has established an amalgamated model with the determinants of artificial intelligence and expectation confirmation model ...
The term "artificial general intelligence" (AGI) has become ubiquitous in current discourse around AI. OpenAI states that its mission is "to ensure that artificial general intelligence benefits all of humanity." DeepMind's company vision statement notes that "artificial general intelligence…has the potential to drive one of the greatest transformations in history."
The research report, which is reportedly the end result of an executive order issued by President Joe Biden last year, had interviewed 42 banking industry executives regarding their cybersecurity ...
beyond banking. Exhibit 3 illustrates how such a bank could engage a retail customer throughout the day. Exhibit 4 shows an example of the banking experience of a small-business owner or the treasurer of a medium-size enterprise. Exhibit 3 How AI transforms banking for a retail customer.How AI transforms banking for a retail customer. Name: Anya
The Artificial Intelligence Act responds directly to citizens' proposals from the Conference on the Future of Europe (COFE), most concretely to proposal 12(10) on enhancing EU's competitiveness in strategic sectors, proposal 33(5) on a safe and trustworthy society, including countering disinformation and ensuring humans are ultimately in ...
Essay; Drone Swarms Are About to Change the Balance of Military Power On today's battlefields, drones are a manageable threat. When hundreds of them can be harnessed to AI technology, they will ...
The term AGI was coined in 2007 when a collection of essays on the subject was published. The book, titled Artificial General intelligence, was co-edited by Ben Goertzel and Cassio Pennachin. In ...
Delphia (USA) Inc. and Global Predictions Inc. agreed to pay a combined $400,000 to resolve SEC allegations that it participated in artificial intelligence washing. AI washing is the use of deceptive and inaccurate claims about a company's use of AI or machine-learning capabilities to capitalize on the publicity surrounding the technology.