- How It Works
- PhD thesis writing
- Master thesis writing
- Bachelor thesis writing
- Dissertation writing service
- Dissertation abstract writing
- Thesis proposal writing
- Thesis editing service
- Thesis proofreading service
- Thesis formatting service
- Coursework writing service
- Research paper writing service
- Architecture thesis writing
- Computer science thesis writing
- Engineering thesis writing
- History thesis writing
- MBA thesis writing
- Nursing dissertation writing
- Psychology dissertation writing
- Sociology thesis writing
- Statistics dissertation writing
- Buy dissertation online
- Write my dissertation
- Cheap thesis
- Cheap dissertation
- Custom dissertation
- Dissertation help
- Pay for thesis
- Pay for dissertation
- Senior thesis
- Write my thesis
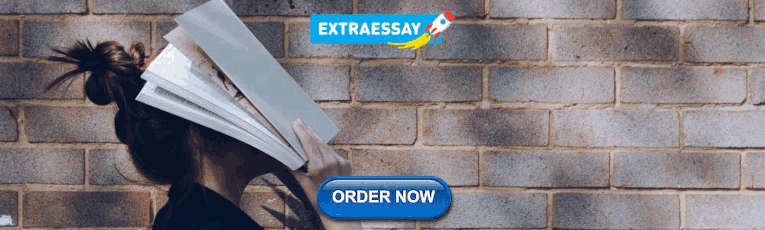
214 Best Big Data Research Topics for Your Thesis Paper
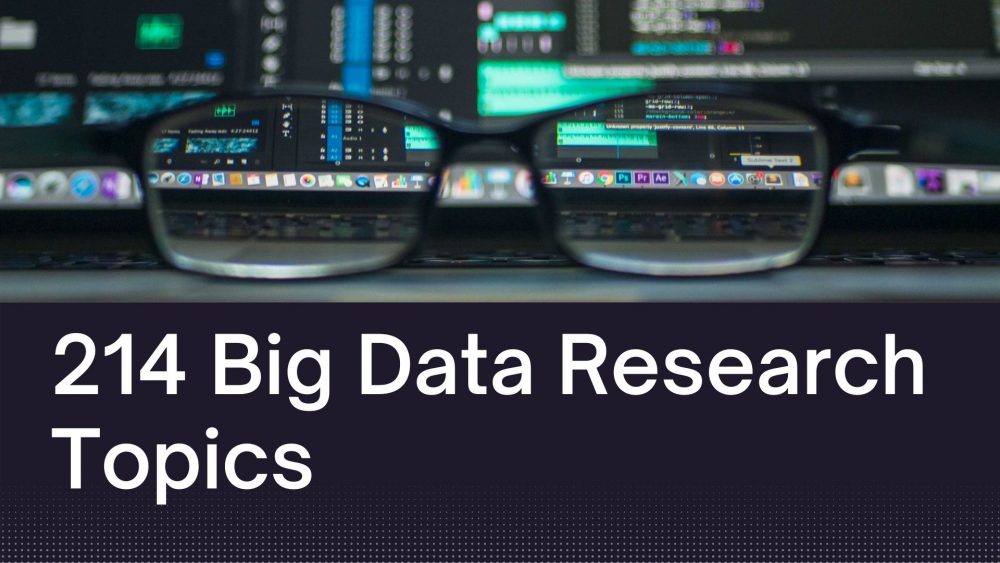
Finding an ideal big data research topic can take you a long time. Big data, IoT, and robotics have evolved. The future generations will be immersed in major technologies that will make work easier. Work that was done by 10 people will now be done by one person or a machine. This is amazing because, in as much as there will be job loss, more jobs will be created. It is a win-win for everyone.
Big data is a major topic that is being embraced globally. Data science and analytics are helping institutions, governments, and the private sector. We will share with you the best big data research topics.
On top of that, we can offer you the best writing tips to ensure you prosper well in your academics. As students in the university, you need to do proper research to get top grades. Hence, you can consult us if in need of research paper writing services.
Big Data Analytics Research Topics for your Research Project
Are you looking for an ideal big data analytics research topic? Once you choose a topic, consult your professor to evaluate whether it is a great topic. This will help you to get good grades.
- Which are the best tools and software for big data processing?
- Evaluate the security issues that face big data.
- An analysis of large-scale data for social networks globally.
- The influence of big data storage systems.
- The best platforms for big data computing.
- The relation between business intelligence and big data analytics.
- The importance of semantics and visualization of big data.
- Analysis of big data technologies for businesses.
- The common methods used for machine learning in big data.
- The difference between self-turning and symmetrical spectral clustering.
- The importance of information-based clustering.
- Evaluate the hierarchical clustering and density-based clustering application.
- How is data mining used to analyze transaction data?
- The major importance of dependency modeling.
- The influence of probabilistic classification in data mining.
Interesting Big Data Analytics Topics
Who said big data had to be boring? Here are some interesting big data analytics topics that you can try. They are based on how some phenomena are done to make the world a better place.
- Discuss the privacy issues in big data.
- Evaluate the storage systems of scalable in big data.
- The best big data processing software and tools.
- Data mining tools and techniques are popularly used.
- Evaluate the scalable architectures for parallel data processing.
- The major natural language processing methods.
- Which are the best big data tools and deployment platforms?
- The best algorithms for data visualization.
- Analyze the anomaly detection in cloud servers
- The scrutiny normally done for the recruitment of big data job profiles.
- The malicious user detection in big data collection.
- Learning long-term dependencies via the Fourier recurrent units.
- Nomadic computing for big data analytics.
- The elementary estimators for graphical models.
- The memory-efficient kernel approximation.
Big Data Latest Research Topics
Do you know the latest research topics at the moment? These 15 topics will help you to dive into interesting research. You may even build on research done by other scholars.
- Evaluate the data mining process.
- The influence of the various dimension reduction methods and techniques.
- The best data classification methods.
- The simple linear regression modeling methods.
- Evaluate the logistic regression modeling.
- What are the commonly used theorems?
- The influence of cluster analysis methods in big data.
- The importance of smoothing methods analysis in big data.
- How is fraud detection done through AI?
- Analyze the use of GIS and spatial data.
- How important is artificial intelligence in the modern world?
- What is agile data science?
- Analyze the behavioral analytics process.
- Semantic analytics distribution.
- How is domain knowledge important in data analysis?
Big Data Debate Topics
If you want to prosper in the field of big data, you need to try even hard topics. These big data debate topics are interesting and will help you to get a better understanding.
- The difference between big data analytics and traditional data analytics methods.
- Why do you think the organization should think beyond the Hadoop hype?
- Does the size of the data matter more than how recent the data is?
- Is it true that bigger data are not always better?
- The debate of privacy and personalization in maintaining ethics in big data.
- The relation between data science and privacy.
- Do you think data science is a rebranding of statistics?
- Who delivers better results between data scientists and domain experts?
- According to your view, is data science dead?
- Do you think analytics teams need to be centralized or decentralized?
- The best methods to resource an analytics team.
- The best business case for investing in analytics.
- The societal implications of the use of predictive analytics within Education.
- Is there a need for greater control to prevent experimentation on social media users without their consent?
- How is the government using big data; for the improvement of public statistics or to control the population?
University Dissertation Topics on Big Data
Are you doing your Masters or Ph.D. and wondering the best dissertation topic or thesis to do? Why not try any of these? They are interesting and based on various phenomena. While doing the research ensure you relate the phenomenon with the current modern society.
- The machine learning algorithms are used for fall recognition.
- The divergence and convergence of the internet of things.
- The reliable data movements using bandwidth provision strategies.
- How is big data analytics using artificial neural networks in cloud gaming?
- How is Twitter accounts classification done using network-based features?
- How is online anomaly detection done in the cloud collaborative environment?
- Evaluate the public transportation insights provided by big data.
- Evaluate the paradigm for cancer patients using the nursing EHR to predict the outcome.
- Discuss the current data lossless compression in the smart grid.
- How does online advertising traffic prediction helps in boosting businesses?
- How is the hyperspectral classification done using the multiple kernel learning paradigm?
- The analysis of large data sets downloaded from websites.
- How does social media data help advertising companies globally?
- Which are the systems recognizing and enforcing ownership of data records?
- The alternate possibilities emerging for edge computing.
The Best Big Data Analysis Research Topics and Essays
There are a lot of issues that are associated with big data. Here are some of the research topics that you can use in your essays. These topics are ideal whether in high school or college.
- The various errors and uncertainty in making data decisions.
- The application of big data on tourism.
- The automation innovation with big data or related technology
- The business models of big data ecosystems.
- Privacy awareness in the era of big data and machine learning.
- The data privacy for big automotive data.
- How is traffic managed in defined data center networks?
- Big data analytics for fault detection.
- The need for machine learning with big data.
- The innovative big data processing used in health care institutions.
- The money normalization and extraction from texts.
- How is text categorization done in AI?
- The opportunistic development of data-driven interactive applications.
- The use of data science and big data towards personalized medicine.
- The programming and optimization of big data applications.
The Latest Big Data Research Topics for your Research Proposal
Doing a research proposal can be hard at first unless you choose an ideal topic. If you are just diving into the big data field, you can use any of these topics to get a deeper understanding.
- The data-centric network of things.
- Big data management using artificial intelligence supply chain.
- The big data analytics for maintenance.
- The high confidence network predictions for big biological data.
- The performance optimization techniques and tools for data-intensive computation platforms.
- The predictive modeling in the legal context.
- Analysis of large data sets in life sciences.
- How to understand the mobility and transport modal disparities sing emerging data sources?
- How do you think data analytics can support asset management decisions?
- An analysis of travel patterns for cellular network data.
- The data-driven strategic planning for citywide building retrofitting.
- How is money normalization done in data analytics?
- Major techniques used in data mining.
- The big data adaptation and analytics of cloud computing.
- The predictive data maintenance for fault diagnosis.
Interesting Research Topics on A/B Testing In Big Data
A/B testing topics are different from the normal big data topics. However, you use an almost similar methodology to find the reasons behind the issues. These topics are interesting and will help you to get a deeper understanding.
- How is ultra-targeted marketing done?
- The transition of A/B testing from digital to offline.
- How can big data and A/B testing be done to win an election?
- Evaluate the use of A/B testing on big data
- Evaluate A/B testing as a randomized control experiment.
- How does A/B testing work?
- The mistakes to avoid while conducting the A/B testing.
- The most ideal time to use A/B testing.
- The best way to interpret results for an A/B test.
- The major principles of A/B tests.
- Evaluate the cluster randomization in big data
- The best way to analyze A/B test results and the statistical significance.
- How is A/B testing used in boosting businesses?
- The importance of data analysis in conversion research
- The importance of A/B testing in data science.
Amazing Research Topics on Big Data and Local Governments
Governments are now using big data to make the lives of the citizens better. This is in the government and the various institutions. They are based on real-life experiences and making the world better.
- Assess the benefits and barriers of big data in the public sector.
- The best approach to smart city data ecosystems.
- The big analytics used for policymaking.
- Evaluate the smart technology and emergence algorithm bureaucracy.
- Evaluate the use of citizen scoring in public services.
- An analysis of the government administrative data globally.
- The public values are found in the era of big data.
- Public engagement on local government data use.
- Data analytics use in policymaking.
- How are algorithms used in public sector decision-making?
- The democratic governance in the big data era.
- The best business model innovation to be used in sustainable organizations.
- How does the government use the collected data from various sources?
- The role of big data for smart cities.
- How does big data play a role in policymaking?
Easy Research Topics on Big Data
Who said big data topics had to be hard? Here are some of the easiest research topics. They are based on data management, research, and data retention. Pick one and try it!
- Who uses big data analytics?
- Evaluate structure machine learning.
- Explain the whole deep learning process.
- Which are the best ways to manage platforms for enterprise analytics?
- Which are the new technologies used in data management?
- What is the importance of data retention?
- The best way to work with images is when doing research.
- The best way to promote research outreach is through data management.
- The best way to source and manage external data.
- Does machine learning improve the quality of data?
- Describe the security technologies that can be used in data protection.
- Evaluate token-based authentication and its importance.
- How can poor data security lead to the loss of information?
- How to determine secure data.
- What is the importance of centralized key management?
Unique IoT and Big Data Research Topics
Internet of Things has evolved and many devices are now using it. There are smart devices, smart cities, smart locks, and much more. Things can now be controlled by the touch of a button.
- Evaluate the 5G networks and IoT.
- Analyze the use of Artificial intelligence in the modern world.
- How do ultra-power IoT technologies work?
- Evaluate the adaptive systems and models at runtime.
- How have smart cities and smart environments improved the living space?
- The importance of the IoT-based supply chains.
- How does smart agriculture influence water management?
- The internet applications naming and identifiers.
- How does the smart grid influence energy management?
- Which are the best design principles for IoT application development?
- The best human-device interactions for the Internet of Things.
- The relation between urban dynamics and crowdsourcing services.
- The best wireless sensor network for IoT security.
- The best intrusion detection in IoT.
- The importance of big data on the Internet of Things.
Big Data Database Research Topics You Should Try
Big data is broad and interesting. These big data database research topics will put you in a better place in your research. You also get to evaluate the roles of various phenomena.
- The best cloud computing platforms for big data analytics.
- The parallel programming techniques for big data processing.
- The importance of big data models and algorithms in research.
- Evaluate the role of big data analytics for smart healthcare.
- How is big data analytics used in business intelligence?
- The best machine learning methods for big data.
- Evaluate the Hadoop programming in big data analytics.
- What is privacy-preserving to big data analytics?
- The best tools for massive big data processing
- IoT deployment in Governments and Internet service providers.
- How will IoT be used for future internet architectures?
- How does big data close the gap between research and implementation?
- What are the cross-layer attacks in IoT?
- The influence of big data and smart city planning in society.
- Why do you think user access control is important?
Big Data Scala Research Topics
Scala is a programming language that is used in data management. It is closely related to other data programming languages. Here are some of the best scala questions that you can research.
- Which are the most used languages in big data?
- How is scala used in big data research?
- Is scala better than Java in big data?
- How is scala a concise programming language?
- How does the scala language stream process in real-time?
- Which are the various libraries for data science and data analysis?
- How does scala allow imperative programming in data collection?
- Evaluate how scala includes a useful REPL for interaction.
- Evaluate scala’s IDE support.
- The data catalog reference model.
- Evaluate the basics of data management and its influence on research.
- Discuss the behavioral analytics process.
- What can you term as the experience economy?
- The difference between agile data science and scala language.
- Explain the graph analytics process.
Independent Research Topics for Big Data
These independent research topics for big data are based on the various technologies and how they are related. Big data will greatly be important for modern society.
- The biggest investment is in big data analysis.
- How are multi-cloud and hybrid settings deep roots?
- Why do you think machine learning will be in focus for a long while?
- Discuss in-memory computing.
- What is the difference between edge computing and in-memory computing?
- The relation between the Internet of things and big data.
- How will digital transformation make the world a better place?
- How does data analysis help in social network optimization?
- How will complex big data be essential for future enterprises?
- Compare the various big data frameworks.
- The best way to gather and monitor traffic information using the CCTV images
- Evaluate the hierarchical structure of groups and clusters in the decision tree.
- Which are the 3D mapping techniques for live streaming data.
- How does machine learning help to improve data analysis?
- Evaluate DataStream management in task allocation.
- How is big data provisioned through edge computing?
- The model-based clustering of texts.
- The best ways to manage big data.
- The use of machine learning in big data.
Is Your Big Data Thesis Giving You Problems?
These are some of the best topics that you can use to prosper in your studies. Not only are they easy to research but also reflect on real-time issues. Whether in University or college, you need to put enough effort into your studies to prosper. However, if you have time constraints, we can provide professional writing help. Are you looking for online expert writers? Look no further, we will provide quality work at a cheap price.
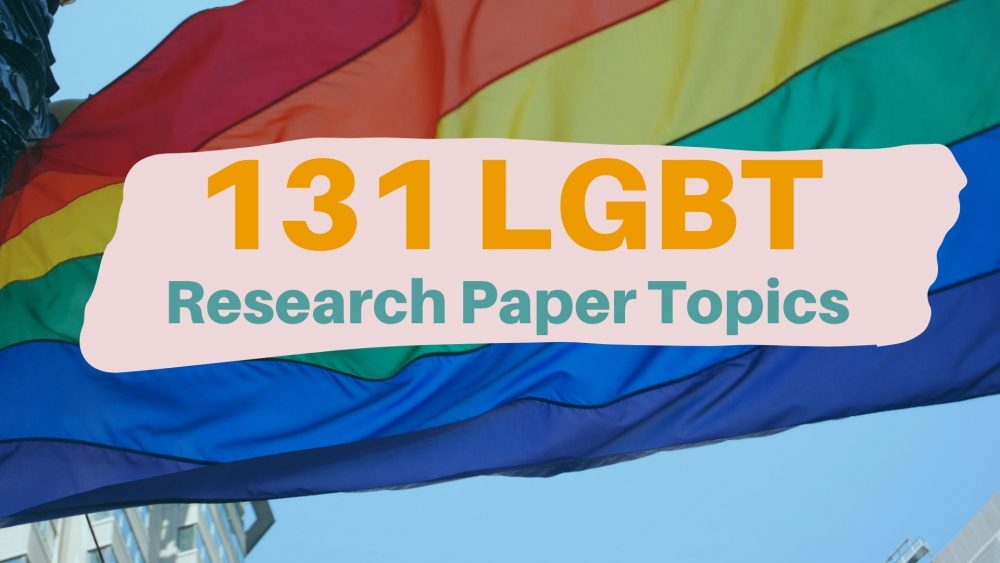
Leave a Reply Cancel reply
Your email address will not be published. Required fields are marked *
Comment * Error message
Name * Error message
Email * Error message
Save my name, email, and website in this browser for the next time I comment.
As Putin continues killing civilians, bombing kindergartens, and threatening WWIII, Ukraine fights for the world's peaceful future.
Ukraine Live Updates
Thesis Helpers

Find the best tips and advice to improve your writing. Or, have a top expert write your paper.
Top 100 Big Data Research Topics For Students
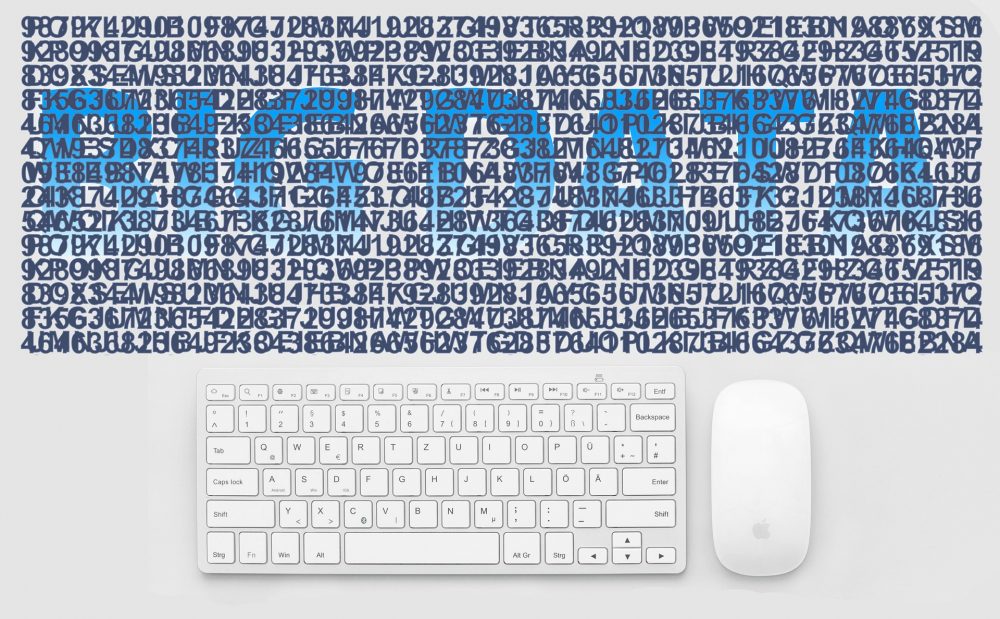
Selecting the right big data research topics is the first and most important step in the process of writing academic papers or essays. Big data is becoming a popular phenomenon among scholars and practitioners. The multidisciplinary background of big data research encompasses a wide spectrum that covers scientific publications in different study areas.
Nevertheless, some students have difficulties choosing big data topics for their computer science thesis or research paper. That’s because finding information to write about some topics is not easy. To solve this problem, we list the top 100 topics in data science that learners can choose from.
Trendy Big Data Research Topics
Students that want to focus on emerging issues when writing academic papers and essays should choose trendy data science topics. Big data covers the initiatives and technologies that tackle massive and diverse data when it comes to addressing traditional skills, technologies, and infrastructure efficiently. Here are some of the latest data topics to consider when writing a research paper or essay.
- Tools and software for processing big data
- Privacy and security issues that face big data
- Scalable architectures for processing massively parallel data
- Analyzing large scale data for social networks
- Scalable big data storage systems
- Platforms for big data computing- Big data analytics and adoption
- How to analyze big data
- How to effectively manage big data
- Parallel big data programming and processing techniques
- Semantics in big data
- Visualization of big data
- Business intelligence and big data analytics
- Map-reduce architecture and Hadoop programming
- Methods for machine learning in big data
- Big data analytics and privacy preservation
- How to process stream data in big data
- Uncertainty in big data management
- Anomaly detection in large scale data systems
- Analytics for big data in the Smart Healthcare systems
- The importance of big data technologies for modern businesses
These are great data research topics that learners at different study levels should consider when asked to write academic papers or essays. However, extensive research is required to come up with great write-ups on these topics.
Data Mining Research Topics for Students
Data mining refers to the extraction of useful information from raw data. It’s a technique that companies apply to accomplish tasks like prediction analysis, generation of the association rule, and clustering. Data mining topics can explain this technique or address issues that are associated with it. Here are some of the best data mining project topics that learners can consider.
- Big data mining techniques and tools
- Model-based clustering of texts
- Describe the concept of data spectroscopic clustering
- Parallel spectral clustering within a distributed system
- Describe asymmetrical spectral clustering
- What is information-based clustering?
- Self-turning spectral clustering
- Symmetrical spectral clustering
- Discuss the K-Means algorithms in data clustering
- Discuss the package of MATLAB spectral clustering
- Discuss the K-Means clustering from an online spherical perspective
- Discuss the hierarchical clustering application
- Explain the importance of probabilistic classification in data mining
- How can the effectiveness of nonlinear and linear regression analysis be improved?
- Explain the Association Rule Learning regarding data mining
- Explain the performance of dependency modeling
- Discuss the performance of representative-based clustering
- Explain the need for density-based clustering
- Discuss the importance of subject-based data mining when it comes to reducing terrorism
- How can data mining be used to analyze transaction data in a supermarket?
Most data mining current research topics focus on finding or establishing patterns. Students can even find some of the best data mining case study topics in this category. Nevertheless, every idea requires detailed and extensive research to come up with facts that make a great paper or essay.
Big Data Analysis Topics
The moderns IT industry depends on data analytics as its lifeline. Big data is one of the techniques and technologies that are used to analyze vast data volumes. The industry is using data analytics as a strategy for gaining insights into system performance and customer behavior. Here are some of the best data analytics research topics that students can consider when writing academic papers.
- Internet of Things
- Describe the importance of augmented reality
- How important is artificial intelligence?
- Explain the graph analytics process
- What is agile data science?
- Why is machine intelligence for modern businesses?
- What is hyper-personalization?
- Explain the behavioral analytics process
- What is the experience economy?
- Discuss journey sciences
- Discuss knowledge validation and extraction
- What is semantic data management?
- Explain the deep learning process
- Explain software engineering for big data science
- What is structured machine learning?
- Explain semantic question answering
- What is distributed semantic analytics?
- Why is domain knowledge important in data analysis?
- Why is data exploration important in data analysis?
- Who uses big data analytics?
Writing about data analytics topics requires background knowledge of the issues being discussed. That’s because the analysis entails harnessing data and extracting its value.
Data Management Project Topics
This category has some of the best data science research topics. The enormous amount of data that modern organizations have to deal with every day is not easy to handle. As such, its effective management is required to ensure its effective use. Here are some of the best topics that students can write about in this aspect.
- Describe some of the most innovative bid data management concepts
- Data catalogs: Describe approaches and their implementation, as well as, adoption
- How to manage platforms for enterprise analytics
- Discuss the impact of data quality on a business
- Explain the best data management strategies for modern enterprises
- New technologies and AI in data management
- What is data retention and why is it important?
- Describe the basics of data management
- Explain the application of data management basics
- Data publishing and access by modern companies
- Explain the process of analyzing and managing data for reproducible research
- Explain how to work with images during research
- How can an organization ensure secure and confidential handling and management of data?
- How to promote research and scientific outreach through data management
- How to source and manage external data
- How to ensure effective data protection through proper management
- Data catalog reference model and market study
- What is data valuation and why does it matter in data management?
- How can machine learning improve the data quality?
- How can a company implement data governance?
This category also has some of the best big data seminar topics. That’s because some of the ideas featured in this section are about issues that affect almost every organization.
Resent Data Security Topics for Research
Big data that comes from different computers and devices require security. That’s because such data is vulnerable to different cyber threats. Some of the best research topics in this category include the following.
- How changing data from Terabytes to Petabytes affects its security
- What are the major vulnerabilities for big data?
- Why big data owners should update security measures regularly
- How can poor data security lead to loss of important information
- Describe security technologies that can be used to protect big data
- Explain how Hadoop integrates with modern security tools
- Which are the best encryption tools for protecting transit data?
- Explain how data encryption tools work
- What is token-based authentication?
- Explain how intrusion prevention and detection systems work
- What are the most effective physical systems for securing data?
- Which is the best intrusion detection system?
- Describe the most suitable key management system when it comes to processing massive data
- Which tool or algorithm can be used for data owner and user’s authentication?
- Explain how you can determine the amount of secure data
- How to identify a legit data user
- How to prevent illegitimate data access
- How to implement attribute-access or role-based access control
- Explain the importance of centralized key management
- Why is user-access control important?
Any topic in this category can be used to write a brilliant paper or essay that will earn the learner the top grade. However, time and efforts are required to work on these ideas.
Whether students opt to write about data visualization topics or data structure research topics, the most important thing is to choose ideas they like and find interesting. What’s more, learners should pick topics they can find adequate information for online. That way, they will find the research and writing process enjoyable. They can also buy dissertations or any other academic papers that will impress educators to award them the top grades.
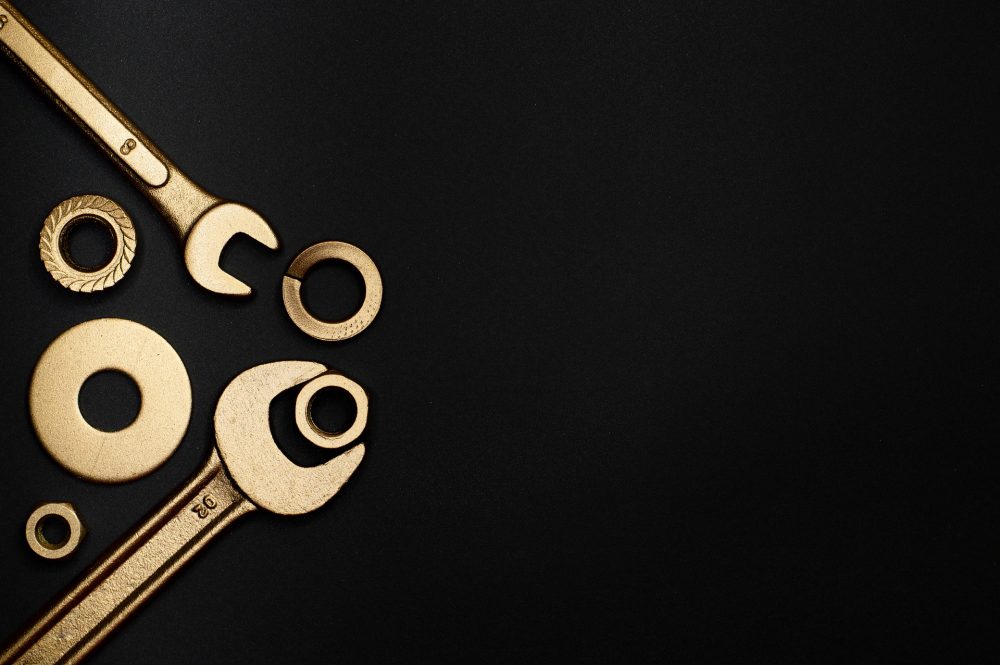
Make PhD experience your own
Leave a Reply Cancel reply
Your email address will not be published. Required fields are marked *

Research Topics & Ideas: Data Science
50 Topic Ideas To Kickstart Your Research Project
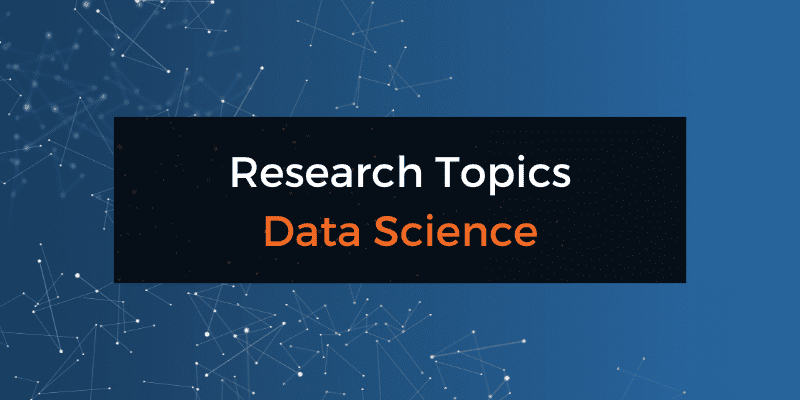
If you’re just starting out exploring data science-related topics for your dissertation, thesis or research project, you’ve come to the right place. In this post, we’ll help kickstart your research by providing a hearty list of data science and analytics-related research ideas , including examples from recent studies.
PS – This is just the start…
We know it’s exciting to run through a list of research topics, but please keep in mind that this list is just a starting point . These topic ideas provided here are intentionally broad and generic , so keep in mind that you will need to develop them further. Nevertheless, they should inspire some ideas for your project.
To develop a suitable research topic, you’ll need to identify a clear and convincing research gap , and a viable plan to fill that gap. If this sounds foreign to you, check out our free research topic webinar that explores how to find and refine a high-quality research topic, from scratch. Alternatively, consider our 1-on-1 coaching service .
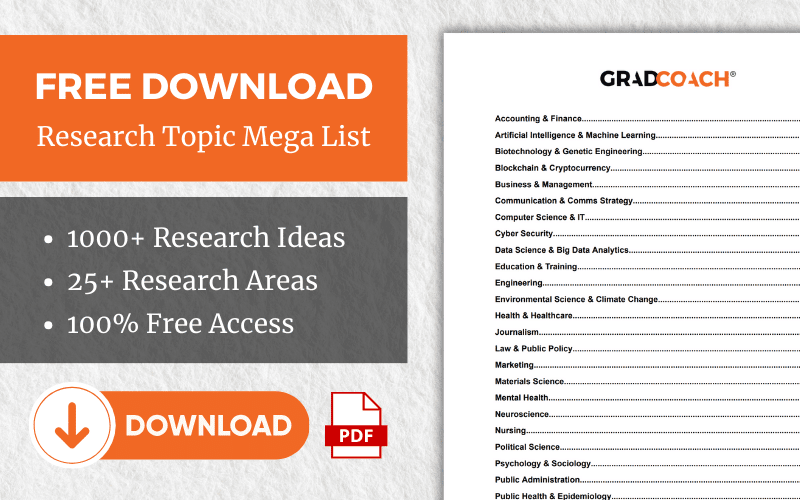
Data Science-Related Research Topics
- Developing machine learning models for real-time fraud detection in online transactions.
- The use of big data analytics in predicting and managing urban traffic flow.
- Investigating the effectiveness of data mining techniques in identifying early signs of mental health issues from social media usage.
- The application of predictive analytics in personalizing cancer treatment plans.
- Analyzing consumer behavior through big data to enhance retail marketing strategies.
- The role of data science in optimizing renewable energy generation from wind farms.
- Developing natural language processing algorithms for real-time news aggregation and summarization.
- The application of big data in monitoring and predicting epidemic outbreaks.
- Investigating the use of machine learning in automating credit scoring for microfinance.
- The role of data analytics in improving patient care in telemedicine.
- Developing AI-driven models for predictive maintenance in the manufacturing industry.
- The use of big data analytics in enhancing cybersecurity threat intelligence.
- Investigating the impact of sentiment analysis on brand reputation management.
- The application of data science in optimizing logistics and supply chain operations.
- Developing deep learning techniques for image recognition in medical diagnostics.
- The role of big data in analyzing climate change impacts on agricultural productivity.
- Investigating the use of data analytics in optimizing energy consumption in smart buildings.
- The application of machine learning in detecting plagiarism in academic works.
- Analyzing social media data for trends in political opinion and electoral predictions.
- The role of big data in enhancing sports performance analytics.
- Developing data-driven strategies for effective water resource management.
- The use of big data in improving customer experience in the banking sector.
- Investigating the application of data science in fraud detection in insurance claims.
- The role of predictive analytics in financial market risk assessment.
- Developing AI models for early detection of network vulnerabilities.
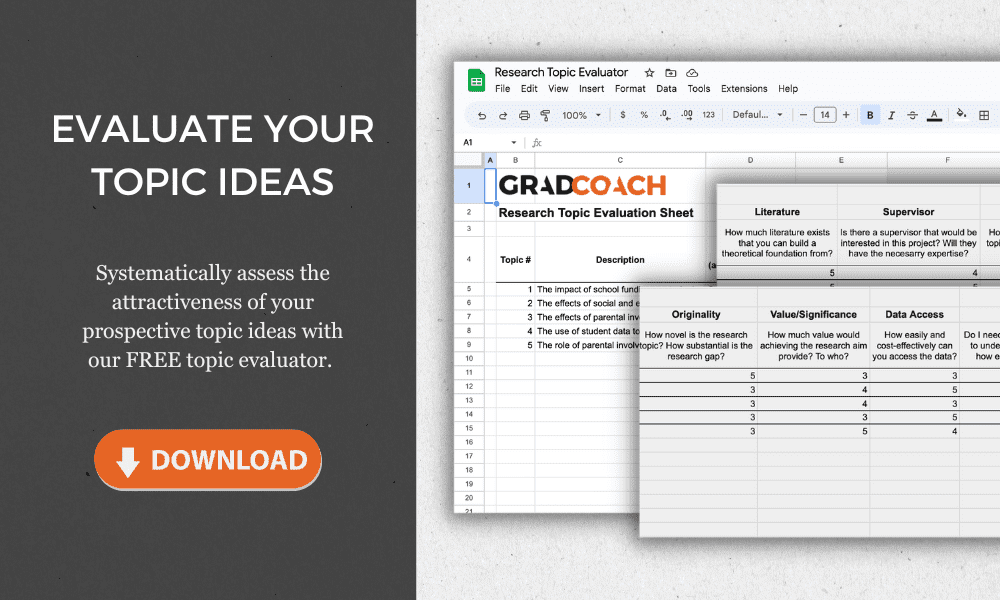
Data Science Research Ideas (Continued)
- The application of big data in public transportation systems for route optimization.
- Investigating the impact of big data analytics on e-commerce recommendation systems.
- The use of data mining techniques in understanding consumer preferences in the entertainment industry.
- Developing predictive models for real estate pricing and market trends.
- The role of big data in tracking and managing environmental pollution.
- Investigating the use of data analytics in improving airline operational efficiency.
- The application of machine learning in optimizing pharmaceutical drug discovery.
- Analyzing online customer reviews to inform product development in the tech industry.
- The role of data science in crime prediction and prevention strategies.
- Developing models for analyzing financial time series data for investment strategies.
- The use of big data in assessing the impact of educational policies on student performance.
- Investigating the effectiveness of data visualization techniques in business reporting.
- The application of data analytics in human resource management and talent acquisition.
- Developing algorithms for anomaly detection in network traffic data.
- The role of machine learning in enhancing personalized online learning experiences.
- Investigating the use of big data in urban planning and smart city development.
- The application of predictive analytics in weather forecasting and disaster management.
- Analyzing consumer data to drive innovations in the automotive industry.
- The role of data science in optimizing content delivery networks for streaming services.
- Developing machine learning models for automated text classification in legal documents.
- The use of big data in tracking global supply chain disruptions.
- Investigating the application of data analytics in personalized nutrition and fitness.
- The role of big data in enhancing the accuracy of geological surveying for natural resource exploration.
- Developing predictive models for customer churn in the telecommunications industry.
- The application of data science in optimizing advertisement placement and reach.
Recent Data Science-Related Studies
While the ideas we’ve presented above are a decent starting point for finding a research topic, they are fairly generic and non-specific. So, it helps to look at actual studies in the data science and analytics space to see how this all comes together in practice.
Below, we’ve included a selection of recent studies to help refine your thinking. These are actual studies, so they can provide some useful insight as to what a research topic looks like in practice.
- Data Science in Healthcare: COVID-19 and Beyond (Hulsen, 2022)
- Auto-ML Web-application for Automated Machine Learning Algorithm Training and evaluation (Mukherjee & Rao, 2022)
- Survey on Statistics and ML in Data Science and Effect in Businesses (Reddy et al., 2022)
- Visualization in Data Science VDS @ KDD 2022 (Plant et al., 2022)
- An Essay on How Data Science Can Strengthen Business (Santos, 2023)
- A Deep study of Data science related problems, application and machine learning algorithms utilized in Data science (Ranjani et al., 2022)
- You Teach WHAT in Your Data Science Course?!? (Posner & Kerby-Helm, 2022)
- Statistical Analysis for the Traffic Police Activity: Nashville, Tennessee, USA (Tufail & Gul, 2022)
- Data Management and Visual Information Processing in Financial Organization using Machine Learning (Balamurugan et al., 2022)
- A Proposal of an Interactive Web Application Tool QuickViz: To Automate Exploratory Data Analysis (Pitroda, 2022)
- Applications of Data Science in Respective Engineering Domains (Rasool & Chaudhary, 2022)
- Jupyter Notebooks for Introducing Data Science to Novice Users (Fruchart et al., 2022)
- Towards a Systematic Review of Data Science Programs: Themes, Courses, and Ethics (Nellore & Zimmer, 2022)
- Application of data science and bioinformatics in healthcare technologies (Veeranki & Varshney, 2022)
- TAPS Responsibility Matrix: A tool for responsible data science by design (Urovi et al., 2023)
- Data Detectives: A Data Science Program for Middle Grade Learners (Thompson & Irgens, 2022)
- MACHINE LEARNING FOR NON-MAJORS: A WHITE BOX APPROACH (Mike & Hazzan, 2022)
- COMPONENTS OF DATA SCIENCE AND ITS APPLICATIONS (Paul et al., 2022)
- Analysis on the Application of Data Science in Business Analytics (Wang, 2022)
As you can see, these research topics are a lot more focused than the generic topic ideas we presented earlier. So, for you to develop a high-quality research topic, you’ll need to get specific and laser-focused on a specific context with specific variables of interest. In the video below, we explore some other important things you’ll need to consider when crafting your research topic.
Get 1-On-1 Help
If you’re still unsure about how to find a quality research topic, check out our Research Topic Kickstarter service, which is the perfect starting point for developing a unique, well-justified research topic.
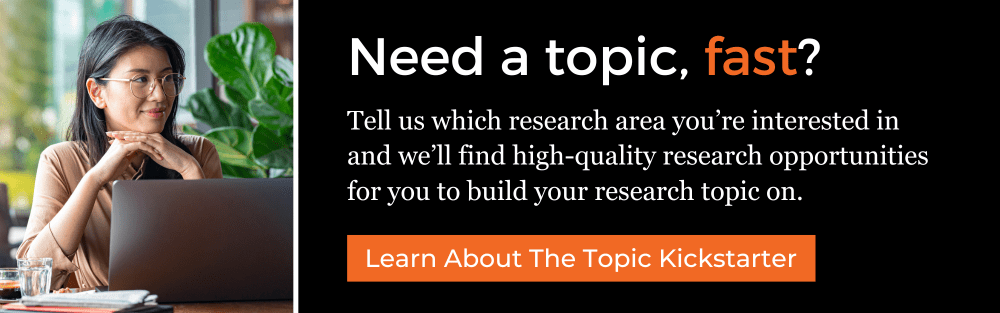
You Might Also Like:
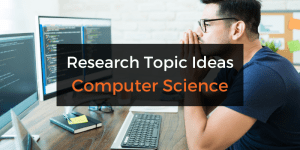
Submit a Comment Cancel reply
Your email address will not be published. Required fields are marked *
Save my name, email, and website in this browser for the next time I comment.
- Print Friendly
Mon - Sat 9:00am - 12:00am
- Get a quote
List of Best Research and Thesis Topic Ideas for Data Science in 2022
In an era driven by digital and technological transformation, businesses actively seek skilled and talented data science potentials capable of leveraging data insights to enhance business productivity and achieve organizational objectives. In keeping with an increasing demand for data science professionals, universities offer various data science and big data courses to prepare students for the tech industry. Research projects are a crucial part of these programs and a well- executed data science project can make your CV appear more robust and compelling. A broad range of data science topics exist that offer exciting possibilities for research but choosing data science research topics can be a real challenge for students . After all, a good research project relies first and foremost on data analytics research topics that draw upon both mono-disciplinary and multi-disciplinary research to explore endless possibilities for real –world applications.
As one of the top-most masters and PhD online dissertation writing services , we are geared to assist students in the entire research process right from the initial conception to the final execution to ensure that you have a truly fulfilling and enriching research experience. These resources are also helpful for those students who are taking online classes .
By taking advantage of our best digital marketing research topics in data science you can be assured of producing an innovative research project that will impress your research professors and make a huge difference in attracting the right employers.
Get an Immediate Response
Discuss your requirments with our writers
Get 3 Customize Research Topic within 24 Hours
Undergraduate Masters PhD Others
Data science thesis topics
We have compiled a list of data science research topics for students studying data science that can be utilized in data science projects in 2022. our team of professional data experts have brought together master or MBA thesis topics in data science that cater to core areas driving the field of data science and big data that will relieve all your research anxieties and provide a solid grounding for an interesting research projects . The article will feature data science thesis ideas that can be immensely beneficial for students as they cover a broad research agenda for future data science . These ideas have been drawn from the 8 v’s of big data namely Volume, Value, Veracity, Visualization, Variety, Velocity, Viscosity, and Virility that provide interesting and challenging research areas for prospective researches in their masters or PhD thesis . Overall, the general big data research topics can be divided into distinct categories to facilitate the research topic selection process.
- Security and privacy issues
- Cloud Computing Platforms for Big Data Adoption and Analytics
- Real-time data analytics for processing of image , video and text
- Modeling uncertainty
How “The Research Guardian” Can Help You A lot!
Our top thesis writing experts are available 24/7 to assist you the right university projects. Whether its critical literature reviews to complete your PhD. or Master Levels thesis.
DATA SCIENCE PHD RESEARCH TOPICS
The article will also guide students engaged in doctoral research by introducing them to an outstanding list of data science thesis topics that can lead to major real-time applications of big data analytics in your research projects.
- Intelligent traffic control ; Gathering and monitoring traffic information using CCTV images.
- Asymmetric protected storage methodology over multi-cloud service providers in Big data.
- Leveraging disseminated data over big data analytics environment.
- Internet of Things.
- Large-scale data system and anomaly detection.
What makes us a unique research service for your research needs?
We offer all –round and superb research services that have a distinguished track record in helping students secure their desired grades in research projects in big data analytics and hence pave the way for a promising career ahead. These are the features that set us apart in the market for research services that effectively deal with all significant issues in your research for.
- Plagiarism –free ; We strictly adhere to a non-plagiarism policy in all our research work to provide you with well-written, original content with low similarity index to maximize chances of acceptance of your research submissions.
- Publication; We don’t just suggest PhD data science research topics but our PhD consultancy services take your research to the next level by ensuring its publication in well-reputed journals. A PhD thesis is indispensable for a PhD degree and with our premier best PhD thesis services that tackle all aspects of research writing and cater to essential requirements of journals , we will bring you closer to your dream of being a PhD in the field of data analytics.
- Research ethics: Solid research ethics lie at the core of our services where we actively seek to protect the privacy and confidentiality of the technical and personal information of our valued customers.
- Research experience: We take pride in our world –class team of computing industry professionals equipped with the expertise and experience to assist in choosing data science research topics and subsequent phases in research including findings solutions, code development and final manuscript writing.
- Business ethics: We are driven by a business philosophy that‘s wholly committed to achieving total customer satisfaction by providing constant online and offline support and timely submissions so that you can keep track of the progress of your research.
Now, we’ll proceed to cover specific research problems encompassing both data analytics research topics and big data thesis topics that have applications across multiple domains.
Get Help from Expert Thesis Writers!
TheresearchGuardian.com providing expert thesis assistance for university students at any sort of level. Our thesis writing service has been serving students since 2011.
Multi-modal Transfer Learning for Cross-Modal Information Retrieval
Aim and objectives.
The research aims to examine and explore the use of CMR approach in bringing about a flexible retrieval experience by combining data across different modalities to ensure abundant multimedia data.
- Develop methods to enable learning across different modalities in shared cross modal spaces comprising texts and images as well as consider the limitations of existing cross –modal retrieval algorithms.
- Investigate the presence and effects of bias in cross modal transfer learning and suggesting strategies for bias detection and mitigation.
- Develop a tool with query expansion and relevance feedback capabilities to facilitate search and retrieval of multi-modal data.
- Investigate the methods of multi modal learning and elaborate on the importance of multi-modal deep learning to provide a comprehensive learning experience.
The Role of Machine Learning in Facilitating the Implication of the Scientific Computing and Software Engineering
- Evaluate how machine learning leads to improvements in computational APA reference generator tools and thus aids in the implementation of scientific computing
- Evaluating the effectiveness of machine learning in solving complex problems and improving the efficiency of scientific computing and software engineering processes.
- Assessing the potential benefits and challenges of using machine learning in these fields, including factors such as cost, accuracy, and scalability.
- Examining the ethical and social implications of using machine learning in scientific computing and software engineering, such as issues related to bias, transparency, and accountability.
Trustworthy AI
The research aims to explore the crucial role of data science in advancing scientific goals and solving problems as well as the implications involved in use of AI systems especially with respect to ethical concerns.
- Investigate the value of digital infrastructures available through open data in aiding sharing and inter linking of data for enhanced global collaborative research efforts
- Provide explanations of the outcomes of a machine learning model for a meaningful interpretation to build trust among users about the reliability and authenticity of data
- Investigate how formal models can be used to verify and establish the efficacy of the results derived from probabilistic model.
- Review the concept of Trustworthy computing as a relevant framework for addressing the ethical concerns associated with AI systems.
The Implementation of Data Science and their impact on the management environment and sustainability
The aim of the research is to demonstrate how data science and analytics can be leveraged in achieving sustainable development.
- To examine the implementation of data science using data-driven decision-making tools
- To evaluate the impact of modern information technology on management environment and sustainability.
- To examine the use of data science in achieving more effective and efficient environment management
- Explore how data science and analytics can be used to achieve sustainability goals across three dimensions of economic, social and environmental.
Big data analytics in healthcare systems
The aim of the research is to examine the application of creating smart healthcare systems and how it can lead to more efficient, accessible and cost –effective health care.
- Identify the potential Areas or opportunities in big data to transform the healthcare system such as for diagnosis, treatment planning, or drug development.
- Assessing the potential benefits and challenges of using AI and deep learning in healthcare, including factors such as cost, efficiency, and accessibility
- Evaluating the effectiveness of AI and deep learning in improving patient outcomes, such as reducing morbidity and mortality rates, improving accuracy and speed of diagnoses, or reducing medical errors
- Examining the ethical and social implications of using AI and deep learning in healthcare, such as issues related to bias, privacy, and autonomy.
Large-Scale Data-Driven Financial Risk Assessment
The research aims to explore the possibility offered by big data in a consistent and real time assessment of financial risks.
- Investigate how the use of big data can help to identify and forecast risks that can harm a business.
- Categories the types of financial risks faced by companies.
- Describe the importance of financial risk management for companies in business terms.
- Train a machine learning model to classify transactions as fraudulent or genuine.
Scalable Architectures for Parallel Data Processing
Big data has exposed us to an ever –growing volume of data which cannot be handled through traditional data management and analysis systems. This has given rise to the use of scalable system architectures to efficiently process big data and exploit its true value. The research aims to analyses the current state of practice in scalable architectures and identify common patterns and techniques to design scalable architectures for parallel data processing.
- To design and implement a prototype scalable architecture for parallel data processing
- To evaluate the performance and scalability of the prototype architecture using benchmarks and real-world datasets
- To compare the prototype architecture with existing solutions and identify its strengths and weaknesses
- To evaluate the trade-offs and limitations of different scalable architectures for parallel data processing
- To provide recommendations for the use of the prototype architecture in different scenarios, such as batch processing, stream processing, and interactive querying
Robotic manipulation modelling
The aim of this research is to develop and validate a model-based control approach for robotic manipulation of small, precise objects.
- Develop a mathematical model of the robotic system that captures the dynamics of the manipulator and the grasped object.
- Design a control algorithm that uses the developed model to achieve stable and accurate grasping of the object.
- Test the proposed approach in simulation and validate the results through experiments with a physical robotic system.
- Evaluate the performance of the proposed approach in terms of stability, accuracy, and robustness to uncertainties and perturbations.
- Identify potential applications and areas for future work in the field of robotic manipulation for precision tasks.
Big data analytics and its impacts on marketing strategy
The aim of this research is to investigate the impact of big data analytics on marketing strategy and to identify best practices for leveraging this technology to inform decision-making.
- Review the literature on big data analytics and marketing strategy to identify key trends and challenges
- Conduct a case study analysis of companies that have successfully integrated big data analytics into their marketing strategies
- Identify the key factors that contribute to the effectiveness of big data analytics in marketing decision-making
- Develop a framework for integrating big data analytics into marketing strategy.
- Investigate the ethical implications of big data analytics in marketing and suggest best practices for responsible use of this technology.
Looking For Customize Thesis Topics?
Take a review of different varieties of thesis topics and samples from our website TheResearchGuardian.com on multiple subjects for every educational level.
Platforms for large scale data computing: big data analysis and acceptance
To investigate the performance and scalability of different large-scale data computing platforms.
- To compare the features and capabilities of different platforms and determine which is most suitable for a given use case.
- To identify best practices for using these platforms, including considerations for data management, security, and cost.
- To explore the potential for integrating these platforms with other technologies and tools for data analysis and visualization.
- To develop case studies or practical examples of how these platforms have been used to solve real-world data analysis challenges.
Distributed data clustering
Distributed data clustering can be a useful approach for analyzing and understanding complex datasets, as it allows for the identification of patterns and relationships that may not be immediately apparent.
To develop and evaluate new algorithms for distributed data clustering that is efficient and scalable.
- To compare the performance and accuracy of different distributed data clustering algorithms on a variety of datasets.
- To investigate the impact of different parameters and settings on the performance of distributed data clustering algorithms.
- To explore the potential for integrating distributed data clustering with other machine learning and data analysis techniques.
- To apply distributed data clustering to real-world problems and evaluate its effectiveness.
Analyzing and predicting urbanization patterns using GIS and data mining techniques".
The aim of this project is to use GIS and data mining techniques to analyze and predict urbanization patterns in a specific region.
- To collect and process relevant data on urbanization patterns, including population density, land use, and infrastructure development, using GIS tools.
- To apply data mining techniques, such as clustering and regression analysis, to identify trends and patterns in the data.
- To use the results of the data analysis to develop a predictive model for urbanization patterns in the region.
- To present the results of the analysis and the predictive model in a clear and visually appealing way, using GIS maps and other visualization techniques.
Use of big data and IOT in the media industry
Big data and the Internet of Things (IoT) are emerging technologies that are transforming the way that information is collected, analyzed, and disseminated in the media sector. The aim of the research is to understand how big data and IoT re used to dictate information flow in the media industry
- Identifying the key ways in which big data and IoT are being used in the media sector, such as for content creation, audience engagement, or advertising.
- Analyzing the benefits and challenges of using big data and IoT in the media industry, including factors such as cost, efficiency, and effectiveness.
- Examining the ethical and social implications of using big data and IoT in the media sector, including issues such as privacy, security, and bias.
- Determining the potential impact of big data and IoT on the media landscape and the role of traditional media in an increasingly digital world.
Exigency computer systems for meteorology and disaster prevention
The research aims to explore the role of exigency computer systems to detect weather and other hazards for disaster prevention and response
- Identifying the key components and features of exigency computer systems for meteorology and disaster prevention, such as data sources, analytics tools, and communication channels.
- Evaluating the effectiveness of exigency computer systems in providing accurate and timely information about weather and other hazards.
- Assessing the impact of exigency computer systems on the ability of decision makers to prepare for and respond to disasters.
- Examining the challenges and limitations of using exigency computer systems, such as the need for reliable data sources, the complexity of the systems, or the potential for human error.
Network security and cryptography
Overall, the goal of research is to improve our understanding of how to protect communication and information in the digital age, and to develop practical solutions for addressing the complex and evolving security challenges faced by individuals, organizations, and societies.
- Developing new algorithms and protocols for securing communication over networks, such as for data confidentiality, data integrity, and authentication
- Investigating the security of existing cryptographic primitives, such as encryption and hashing algorithms, and identifying vulnerabilities that could be exploited by attackers.
- Evaluating the effectiveness of different network security technologies and protocols, such as firewalls, intrusion detection systems, and virtual private networks (VPNs), in protecting against different types of attacks.
- Exploring the use of cryptography in emerging areas, such as cloud computing, the Internet of Things (IoT), and blockchain, and identifying the unique security challenges and opportunities presented by these domains.
- Investigating the trade-offs between security and other factors, such as performance, usability, and cost, and developing strategies for balancing these conflicting priorities.
Meet Our Professionals Ranging From Renowned Universities
Related topics.
- Sports Management Research Topics
- Special Education Research Topics
- Software Engineering Research Topics
- Primary Education Research Topics
- Microbiology Research Topics
- Luxury Brand Research Topics
- Cyber Security Research Topics
- Commercial Law Research Topics
- Change Management Research Topics
- Artificial intelligence Research Topics
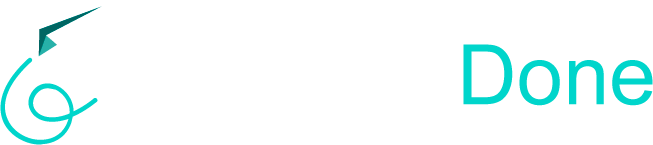
- Services Paper editing services Paper proofreading Business papers Philosophy papers Write my paper Term papers for sale Term paper help Academic term papers Buy research papers College writing services Paper writing help Student papers Original term papers Research paper help Nursing papers for sale Psychology papers Economics papers Medical papers Blog
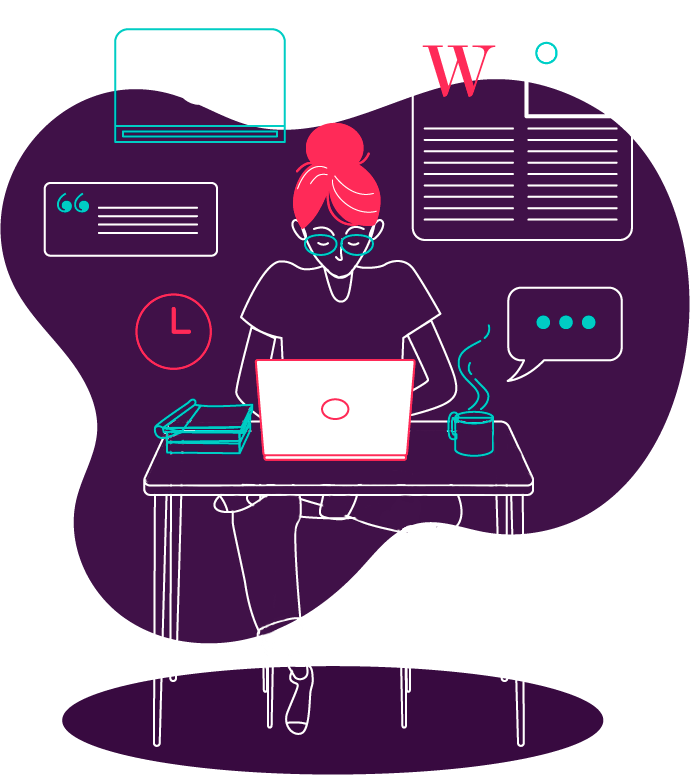
166 Latest Big Data Research Topics And Fascinating Ideas
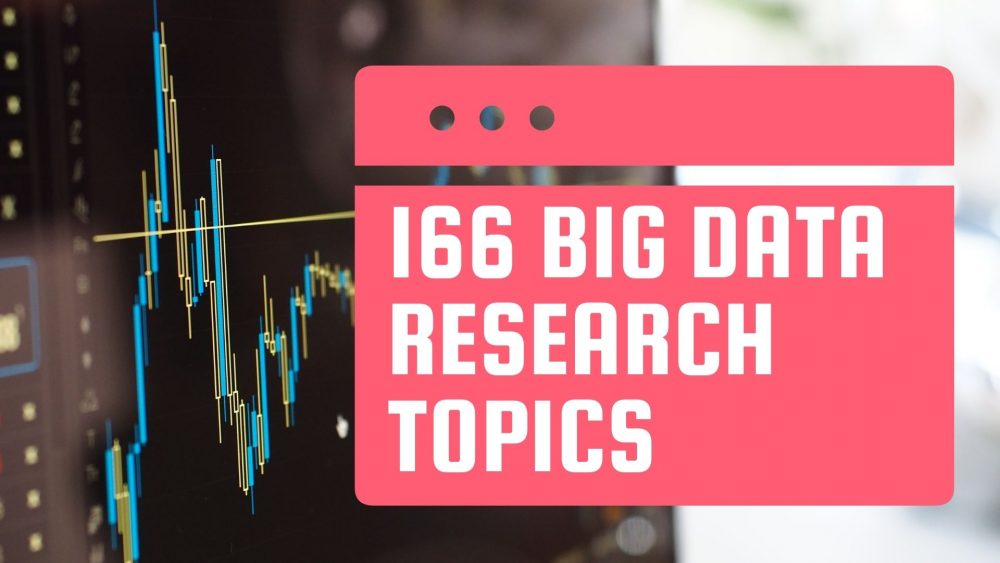
Big data refers to a huge volume of data, whether organized or unorganized, whose analysis shapes technologies and methodologies. Big data is so massive and complicated that it cannot be handled using ordinary application software. For instance, some frameworks, such as Hadoop, are built to process large amounts of data. Big data has gained much attention, hence it’s a trendy topic and essay for students and researchers who want to write thesis, projects, and dissertations. Based on this, there are several searchable and interesting topics to explore for undergraduate and master’s theses in big data, same as doctoral degrees. In this article, we have provided every topic you need on big data. Our topics stretch from big data analytics, big data research questions, to IoT and database essays. If you’ve been looking for the latest big data research topics, your search stops here. Read on to see some of the most interesting topics for your thesis.
Interesting Big Data Analytics Research Topics
Data analytics is the lifeblood of the modern IT sector. Big data is one of the strategies and technologies for analyzing large amounts of data. Data analytics is being used by the industry to acquire knowledge of system performance and customer behavior. Here are some of the best big data analytics topics and ideas for academic papers.
- The surge of Internet of Things (IoT)
- Explain the significance of augmented reality.
- What is the significance of artificial intelligence?
- Describe the graph analytics procedure.
- What is agile data science, and how does it differ from traditional data science?
- What role does machine intelligence play in today’s businesses?
- What is hyper-personalization, and how does it work?
- Describe how behavioral analytics works.
- What is the experience economy, and how does it work?
- Talk about the science of travel.
- Discuss the validation and extraction of knowledge.
- What is semantic data management, and how does it work?
- Describe the process of deep learning.
- Describe software engineering in the context of big data science.
- What is structured machine learning, and how does it work?
- Describe how to answer a semantic question
- What is distributed semantic analytics, and how does it work?
- What role does domain knowledge play in data analysis?
- Why is data exploration important in data analysis?
- Who uses big data analytics?
So, it’s not an easy task to write a paper for a high grade. Sometimes every student need a professional help with research paper writing. Therefore, don’t be afraid to hire a writer to complete your assignment. Just contact us and get your paper done soon.
Trending Big Data Research Topics
Students and researchers who want to write about big data latest research topics on appearing issues and topics should pick current topics in data science. Below are some current big data analysis research topics and essays to look into if writing a research essay or paper.
- Analyze the digital tools and programs for processing large data.
- Discuss the effect of the sophistication of big data on human privacy.
- Evaluate how scalable architectures can be used for processing parallel data.
- List the different growth oriented big data storage mechanics.
- Visualizing big data.
- Business acumen in combination with big data analytics.
- Map-reductionist architecture.
- Methods of machine learning in big data.
- Big data analytics and impact on privacy preservation.
- The processing of big data and impact on climate change.
- Risks and uncertainties in big data management.
- Detecting anomalies in large-scale data systems.
- Analyze the big data for social networks.
- Platforms for large scale data computing: big data analysis and acceptance.
- Discuss the procedures of analyzing big data.
- Discuss the many effective ways of managing big data.
- Big data programming and process methods.
- Big data semantics.
- How big data influences biomedical information and strategies.
- The significance of big data strategies on small and medium-sized businesses.
Most Debatable Big Data Research Topics and Essays
The rapid rise of big data in our current time is not without controversy. There is a myriad of ongoing debates in the discipline that have gone unresolved for quite some time. The list below contains the most common big data debate topics.
- Big data and its major vulnerabilities.
- What measures are in place to recognize a legit user of big data?
- Explain the significance of user-access control.
- Investigate the importance of centralized key management.
- Identify ways to prevent illegal access of data.
- Intrusion-detection system: Which is the best?
- Does machine learning enhance data quality?
- Which security technology has proven to be the best for big data protection?
- What strategies should be used for data governance and who should implement data policies?
- Should tech giants regularly update security measures and be transparent about them?
- How has poor data security contributed to the loss of historical evidence?
- What are the most important big data management tools and strategies?
- What is data retention and explain its relevance?
- Artificial intelligence will lead to the loss of employment and human interaction.
- Enterprise analytics: How to manage platforms?
- Can data management foster the promotion of peace and freedom?
- Who should be in control of data security: Tech giants or the government?
- What are the functions of the government in big data management and security?
- Discuss how big data is leading to the end of morals and ethics.
- How is big data contributing to the rise of global climate and why tech should pay carbon taxes.
Interesting Dissertation Topics on Big Data
Many research theses and big data topics can be found online for undergraduates, Masters, and Ph.D. students. The list below comprises some dissertation topics on big data.
- Privacy and security issues in big data and how to curtail them.
- Impacts of storage systems of scalable big data.
- The significance of big data processing and data management to industrial development.
- Techniques and data mining tools for big data.
- The benefits of data analytics and cloud computing to the future of work.
- Parallel data processing: effective data architecture and how to go about it.
- Impacts of machine learning algorithms on the fashion industry.
- Using bandwidth provision, how the world of streaming is changing.
- What are the benefits and threats of dedicated networks to governance?
- Cloud gaming and impacts on Millennials and Generation Z.
- Ways to enhance and maximize spread efficiency using flow authority model.
- How divergent and convergent is the Internet of Things (IoT) on manufacturing?
- Data mining and environmental impact: The way forward.
- Geopolitics and the surge of demographic mapping in big data.
- Impacts of travel patterns on big data analytics and data management.
- The rise of deep learning in the automotive industry.
- The sophistication of big data and its implications on cybersecurity.
- Discuss how the big data manufacturing process indicates positive globalization.
- Evaluate the future of data mining and the adaptation of humans to big data.
- Human and material wastes in big data management.
Interesting Research Topics on A/B Testing in Big Data
The A/B testing is also known as controlled experiments and is used widely by companies and firms to make decisions in product launches. Tech companies use the test to know the acceptability of a certain product by the users. However, below are some key research topics on A/B testing in Big Data
- Evaluate the common A/B pitfalls in the automotive industry.
- Discuss the benefits of improving library user experience with A/B Testing.
- How to design A/B tests in a collaboration network.
- Analyze how the future of social network advertising can be improved by A/B testing.
- Effectiveness of A/B experiments in MOOCs for better instructional methods.
- Strategies of Bayesian A/B testing for business decisions.
- A/B testing challenges in large-scale social networks and online controlled experiments.
- Illustrate how consumer behaviors and trends are shaped by A/B testing.
List of Research Topics on Big Data and Local Governments
Big data offers tremendous value to grassroots governments with the ability to optimize cost through data-induced decisions that reduce the crime rate, traffic congestion and improve the environment. Below are interesting topics on big data and local governments.
- How local governments can measure crime using big data testing.
- Big data and algorithmic policy in local government policies.
- Application of data science technologies to civil service in the local government.
- Combating grassroots crime and corruption through algorithmic government.
- Big data in the public sector: how local governments can benefit from the algorithmic policy.
Innovative Research Topics on Big Data and IoT
Big data has a lot in common with the Internet of Things (IoT). Indeed, IoT is an integral part of big data. Below are researchable IoT and big data research topics.
- The impacts of big data and the Internet of Things (IoT) on the fourth industrial revolution.
- The importance of big data and the Internet of Things (IoT) on public health systems.
- Explain how big data and the Internet of Things (IoT) dictate the flow of information in the media sector.
- Challenges of big data and the Internet of Things (IoT) on governance and sustainability.
- The disruption of big data and its attendant effects on the Internet of Things (IoT).
- Illustrate the surge in household smart devices and the role of big data analytics.
- An analysis of the disruption of the supply chain of traditional goods through the Internet of Things (IoT).
- A comprehensive evaluation of machine and deep learning for IoT-enabled healthcare systems.
- The future evaluation of the internet of things and big data analytics in the public infrastructure systems.
- Discuss how AI-induced security can guarantee effective data protection.
- IoT privacy: what data protection means to households and the impacts of security infringement.
- Discuss the role of big data and the integrity of the Internet of Things (IoT).
- How do dedicated networks work through the Internet of Things (IoT)?
- The threats and benefits of the Internet of Things (IoT) forensic science.
- Big data distributed storage and impacts on IoT-enabled industries.
Most Engaging Database Big Data Research Topics
The database category of big data has some interesting data science research topics. Due to the large data, modern companies have to analyze every day, which are difficult to handle, strict managing is essential to make sure of the effective use of data. Check out some intriguing big data database research topics students and researchers can write about.
- Explain the most inventive big data information concepts and strategies.
- Clarify the most ideal data management strategies and techniques for present-day businesses.
- New advancements and AI in information management.
- What is information maintenance and for what reason is it significant?
- Depict the essentials of information management.
- Clarify the use of information management in e-learning.
- Information distribution and access by present-day organizations.
- Clarify the most common way of investigating and overseeing information for biomedical exploration.
- Disclose how to function with 3D pictures during research.
- How could an association guarantee secure and classified information management and security?
- Information indexes: Describe approaches and their execution as well as their reception.
- Talk about the effect of information quality on a business.
- Instructions on how to advance medical examination and reach logical effort through information management.
- The most effective method to source and oversee external data.
- Evaluate the procedures available to organizations in ensuring information security through appropriate administration.
- Information catalog reference model and global market study.
- What is information valuation and what difference does it make in information management?
- How could AI further develop database security?
- How might an organization carry out effective data administration?
- Database management and the cost of disruptive cybersecurity.
If you are ready to boost your grades with a little effort, j ust write a message “Please, write a custom research paper for me” to hire one of our professional writers. Contact us today to get a 100% original paper.
Compelling Big Data Scala Research Topics
Big Data Scala is the product of algorithmic frameworks in deep and machine learning. Below are listed topics on big data Scala for students and young researchers.
- Large information versatility dependent on Scala and Spark Machine Learning Libraries
- Analyze versatile large information stockpiling frameworks in deep learning.
- Dealing with Data and Model drift for practical applications.
- Building generative systems based on conversational frameworks (Chatbot systems).
- Adaptable designs for parallel data building.
- Dealing with continuous video analytics in cloud computing.
- Proficient graph processing at a machine learning scale.
- Dimensional reduction approaches for information management.
- Compelling anonymization of sensitive fields in computer vision.
- Versatile security safeguarding on big data.
List of Independent Research Topics for Big Data
Independent researches are pieces of research that may be considered unorthodox in big data testing and management. These are research studies generated by individual researchers. Here is a list of the most fascinating independent research topics on big data.
- Significance of effective data mining tools and procedures.
- What is data-driven clustering in deep and machine learning?
- How impactful is the graph analytics process to the Internet of Things?
- Explain the significance of AI for present-day businesses.
- Significance of information investigation in information examination on deep learning.
- Evaluate the usefulness of coding in Artificial Intelligence.
- Clarify the AI strategies in big data management.
- Data security: what it means to computer vision.
- Impact of open-source deep learning libraries on developers.
- The significance of token-based authentication to data security.
- Using big data to identify disinformation and misinformation.
- Data management and the fundamental principles of Artificial Intelligence.
- Big data analytics and why it should be more user-friendly.
- Why business intelligence should focus more on privacy preservation.
- Social networks and impact on privacy infringement.
Is Your Big Data Paper Not Coming Along?
Although we have provided you with a list of big data essays to choose from, we dare say university research topics go beyond mere writing tips. As a student, you may need quality college paper writing services and professional assistance to writing an A-graded and top-notch thesis or dissertations. Here is where we come in. You can consult our reliable and professional writing experts to ease your degree courses at a pocket-friendly price. Aside, you can also refer your colleagues online to enjoy our discounted services that will make your research experience less tacky and frustrating.
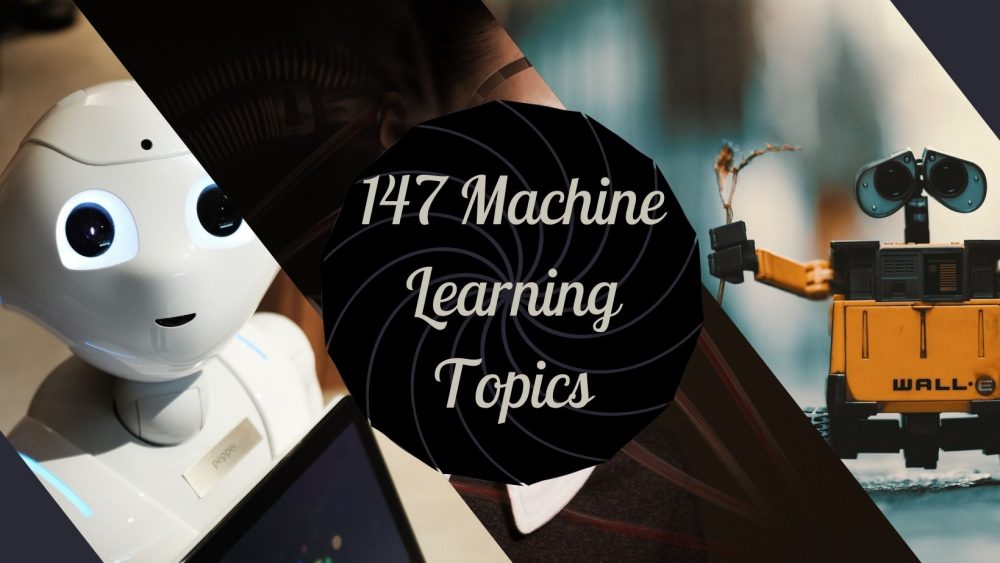
Leave a Reply Cancel reply
Your email address will not be published. Required fields are marked *
Save my name, email, and website in this browser for the next time I comment.
Terms & Conditions Loyalty Program Privacy Policy Money-Back Policy
Copyright © 2013-2024 MyPaperDone.com
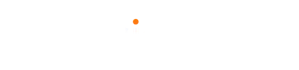
140 Excellent Big Data Research Topics to Consider
Table of Contents
Are you a computer science student searching for recent big data research topics for your final year project? Do you want to write a top-quality big data research paper but are confused about what topic to choose? If yes, then this blog post is for you.
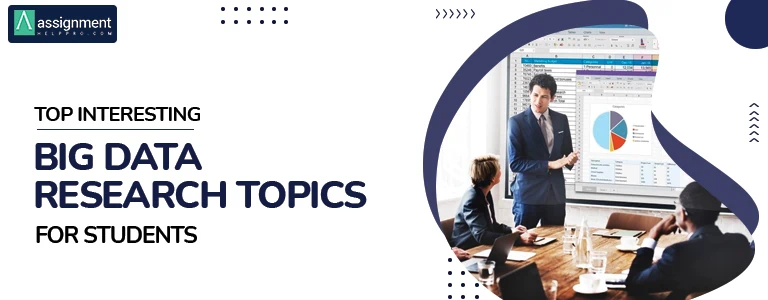
Big Data is one of the recently emerging technologies that have gained a lot of attraction among professionals, especially computer science engineers and information technologists. In the latest internet world, we are surrounded by data and information. Particularly, after the advent of digital systems, data is considered to be precious. In order to process, store, and analyze a large volume of data, the concept of Big Data came into existence.
To write an excellent computer science thesis on big data, you must have a valid research topic. As big data is a broad subject, choosing a new trending research topic is a challenging task. So, to help you, here, in this blog post, we have listed the top interesting big data topics for you to consider for research or academic writing.
List of Outstanding Big Data Research Topics
When it comes to writing research papers and essays, it is necessary to choose trendy research topics to get an A+ grade. As far as big data is concerned, you can conduct research on any interesting data science topics, data mining topics, data analysis topics, or data security topics.
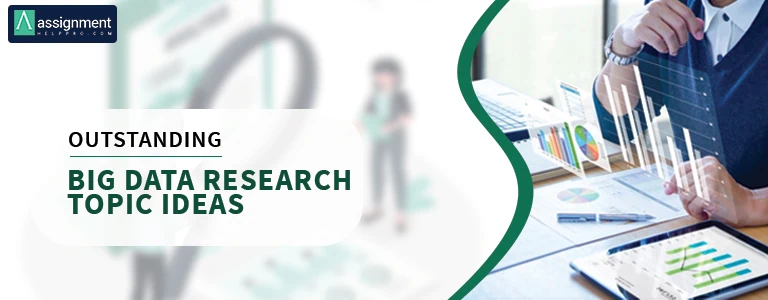
Listed below are a few top-notch big data research topic ideas. You can go through the complete list and identify the best big data research topic of your desire.
Popular Big Data Research Topics
- How to analyze big data?
- Visualization of big data
- How to manage big data?
- Scalable big data storage systems
- Scalable architectures for processing massively parallel data
- Tools and software for processing big data
- Privacy and security issues that face big data
- Platforms for big data computing- Big data analytics and adoption
- Parallel big data programming and processing techniques
- Semantics in big data
- Machine learning in big data
- The basics of data management
- The importance of big data technologies for modern businesses
- How to process stream data in big data?
- Map-reduce architecture and Hadoop programming
- Business intelligence and big data analytics
- Uncertainty in big data management
- How to source and manage external data?
- How does the smart grid influence energy management?
- How can an organization ensure secure and confidential handling and management of data?
Simple Big Data Research Ideas
- Maturity model of big data.
- How far is data science relevant as a master’s thesis and research in today’s date?
- How can big data develop organizational operations and enhance its competitive advantage in the current competitive market?
- Briefly describe the Hadoop Ecosystem
- Describe the use of NoSQL Database and R Programming
- Evaluation of SQL-based Technologies
- Describe the application of Predictive Analytics
- Comparative analysis of the application of Apache Spark and Elasticsearch
- Describe the difference between Tensor Flow, Beam, and Apache Airflow
- Compare and contrast Docker and Kubernetes
- How does the use of data analytics bring positive social impact?
- Discuss the use of Big Data in therapies and genomics
- Describe the three major components of big data
- What are the major challenges of big data?
- Discuss the impact of Big Data on bioinformatics
Big Data Analysis Research Topics
- Who uses big data analytics?
- Why is domain knowledge important in data analysis?
- What is distributed semantic analytics?
- Why is data exploration important in data analysis?
- Define semantic questions answering
- What is structured machine learning?
- What is semantic data management ?
- The Internet of Things
- How important is artificial intelligence?
- Describe the importance of augmented reality.
- What is agile data science?
- Explain the knowledge validation and extraction.
- Explain the deep learning process.
- Significance of machine learning for modern business.
- What is hyper-personalization?
- Experience economy and its relevance.
- Analyzing large-scale data for social networks
- Discuss the behavioral analytics process.
- Explain journey sciences.
- Discuss the graph analytics process.
- Explore the problems associated with big data.
- Analyze the use of GIS and spatial data.
- How far is big data for storage and transfer
- How can big data be used for efficiently modeling uncertainty?
- Explore the use of Quantum computing for big data Analytics
- Describe the five latest Big Data trends in 2022
- Discuss DataOps and data stewardship
- What are the essential practices related to big data analytics for manufacturing businesses?
- Discuss the best way to preserve and Assess Big Data, Video Integrity, and Images using AI
- Evaluate the Use of Big Data in Healthcare
- Evaluation of the effectiveness of healthcare diagnoses and using deep learning
- Synergies of machine learning and data management: methods, problems, and future directions
- Describe the usefulness of Big Data analysis
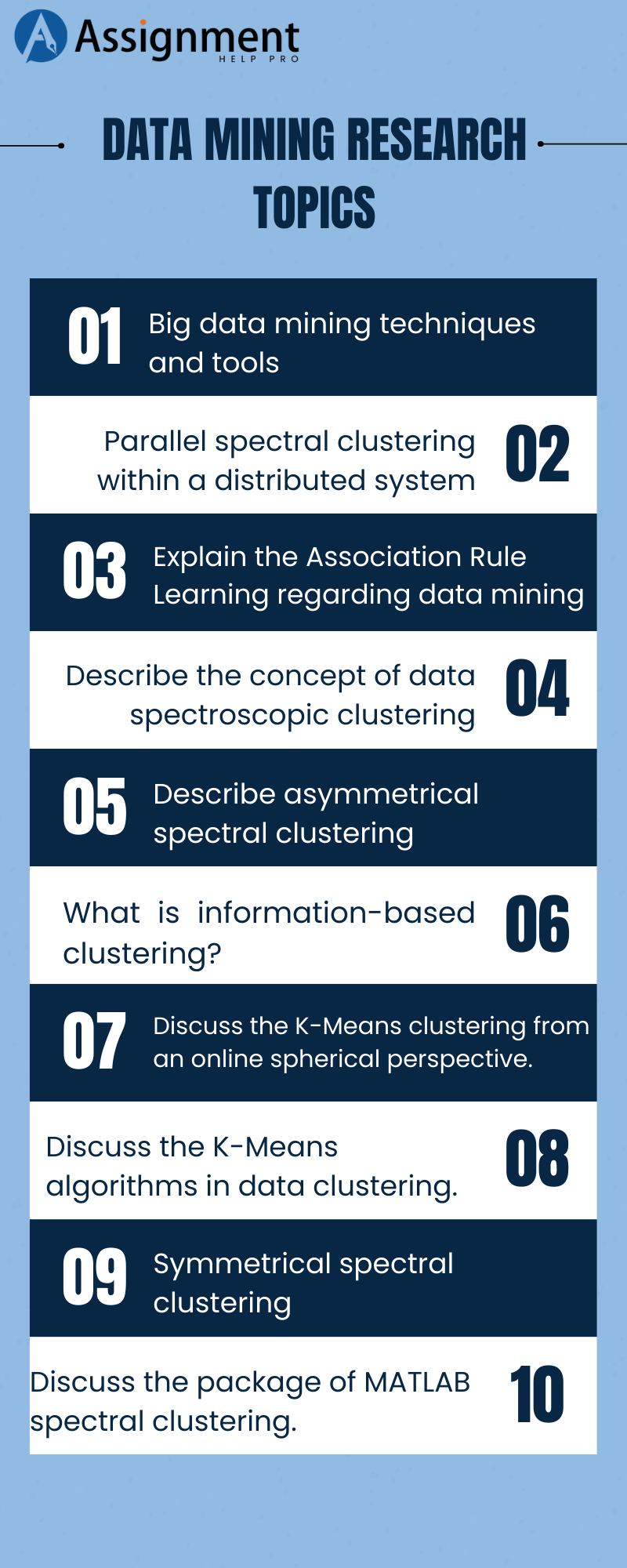
Data Mining Research Topics
- Big data mining techniques and tools
- The role of data mining in analyzing transaction data in a supermarket.
- Parallel spectral clustering within a distributed system
- Explain the Association Rule Learning regarding data mining
- Describe the concept of data spectroscopic clustering
- Describe asymmetrical spectral clustering
- What is information-based clustering?
- Self-turning spectral clustering
- Discuss the K-Means clustering from an online spherical perspective.
- Discuss the K-Means algorithms in data clustering.
- Symmetrical spectral clustering
- Discuss the performance of representative-based clustering.
- Discuss the package of MATLAB spectral clustering.
- How can the effectiveness of nonlinear and linear regression analysis be improved?
- Discuss the hierarchical clustering application.
- Explain the performance of dependency modeling.
- Explain the importance of probabilistic classification in data mining.
- Model-based clustering of texts
- Explain the need for density-based clustering.
- Discuss the importance of subject-based data mining in minimizing terrorism.
- Explore how data mining can be used in automatic content generation.
- The use of data mining in evaluating employee performance.
- Discuss about Parallel Spectral Clustering in Distributed System
- What are K-Means Algorithms for Data Clustering and how it gets applied in Data Mining?
- Why Data mining is called an iterative process?
- How does Data mining go through the phases laid down by the Cross Industry Standard Process for Data Mining (CRISP-DM) process model?
- Compare and contrast Data Mining and Web Mining
- Discuss the differences between Oracle Data Mining and Test Mining
- Analyze Data Mining as a Service(DMaaS)
- What is called Domain Driven Data Mining and Opinion Mining?
- How Predictive Analytics is Used in Data Mining?
- Discuss the benefits and drawbacks of using Web mining for businesses that depend on the web
Read more: Innovative Technology Research Topics To Explore and Write About
Data Security Research Topics
- Why should big data owners update security measures regularly?
- How does changing the data from Terabytes to Petabytes affect its security?
- What are the major vulnerabilities of big data?
- The security technologies that can be used to protect big data
- How does Hadoop integrate with modern security tools?
- Token-based authentication
- How do data encryption tools work?
- How can poor data security lead to the loss of important information?
- Why is user access control important?
- How to prevent illegitimate data access?
- How to identify a legit data user?
- The importance of centralized key management
- How to implement attribute-access or role-based access control?
- How do intrusion prevention and detection systems work?
- The best intrusion detection system
- Which tool or algorithm can be used for data owner and user authentication?
- What are the most effective physical systems for securing data?
- The implementation of attribute-access or role-based access control.
- Explain how you can determine the amount of secure data.
- The best encryption tools for protecting transit data.
Recent Trending Big Data Research Topics
- Data retention and its importance.
- Describe data catalog approaches, implementations, and adoption.
- Describe some of the most innovative bid data management concepts.
- Analytics for Big Data in the Smart Healthcare Systems
- New technologies and AI in data management
- Explain the best data management strategies for modern enterprises.
- How to manage platforms for enterprise analytics
- The impact of data quality on business
- How can a company implement data governance?
- How can machine learning improve the data quality?
- Anomaly detection in large-scale data systems
- The process of analyzing and managing data for reproducible research.
- Data catalog reference model and market study
- The role of data valuation in data management.
- Explain software engineering for big data science.
- How to ensure effective data protection through proper management
- Big data analytics and privacy preservation
- Data publishing and access by modern companies
- How to work with images during research?
- How to promote research and scientific outreach through data management?
Read more: Interesting Cybercrime Research Topics To Deal With
Unique Big Data Research Topics
- Evaluate the logistic regression modeling.
- Explain the malicious user detection in big data collection.
- Evaluate data stream management in task allocation.
- Explain how to gather and monitor traffic information using CCTV images
- What is the difference between edge computing and in-memory computing?
- Explain the difference between agile data science and Scala language.
- Evaluate how Scala includes a useful REPL for interaction.
- Discuss the influence of big data and smart city planning in society.
- Evaluate the adaptive systems and models at runtime.
- Explain the relation between urban dynamics and crowdsourcing services.
The Bottom Line
From the list of 100+ ideas suggested above, choose any topic that matches your university requirements and compose a brilliant big data research paper . In case, you are not satisfied with the topics recommended here, contact us immediately. We have plenty of subject professionals on our platform to offer premium-quality Big data assignment help . Especially, starting from research paper topic selection to writing and editing, our assignment helpers who are experts in big data would provide the best assistance as per your needs at an affordable cost. Moreover, by availing of our big data research paper writing service, you can also submit plagiarism-free academic papers on time and secure the grades you desire to score.
Without any dilemma, take our academic writing service and enjoy all the scholastic benefits it offers.
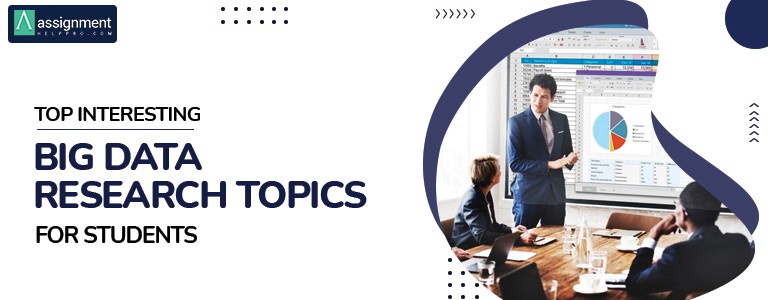
Related Post

220 Amazing Religious Research Paper Topics and Ideas
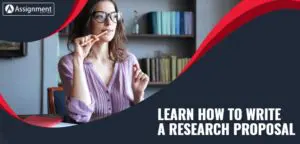
Read and Understand How to Write a Research Proposal
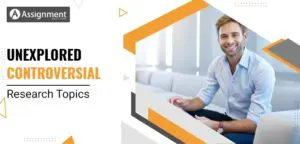
100+ Controversial Research Topics and Ideas to Focus On
About author.
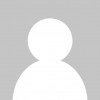
Jacob Smith
I am an Academic Writer and have affection to share my knowledge through posts’. I do not feel tiredness while research and analyzing the things. Sometime, I write down hundred of research topics as per the students requirements. I want to share solution oriented content to the students.
Leave a Reply Cancel reply
You must be logged in to post a comment.
- Featured Posts
140 Unique Geology Research Topics to Focus On
200+ outstanding world history topics and ideas 2023, 190 excellent ap research topics and ideas, 150+ trending group discussion topics and ideas, 170 funny speech topics to blow the minds of audience, who invented exams learn the history of examination, how to focus on reading 15 effective tips for better concentration, what is a rhetorical analysis essay and how to write it, primary school teacher in australia- eligibility, job role, career options, and salary, 4 steps to build a flawless business letter format, get help instantly.
Raise Your Grades with Assignment Help Pro
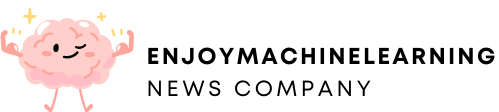
37 Research Topics In Data Science To Stay On Top Of
- February 22, 2024
As a data scientist, staying on top of the latest research in your field is essential.
The data science landscape changes rapidly, and new techniques and tools are constantly being developed.
To keep up with the competition, you need to be aware of the latest trends and topics in data science research.
In this article, we will provide an overview of 37 hot research topics in data science.
We will discuss each topic in detail, including its significance and potential applications.
These topics could be an idea for a thesis or simply topics you can research independently.
Stay tuned – this is one blog post you don’t want to miss!
37 Research Topics in Data Science
1.) predictive modeling.
Predictive modeling is a significant portion of data science and a topic you must be aware of.
Simply put, it is the process of using historical data to build models that can predict future outcomes.
Predictive modeling has many applications, from marketing and sales to financial forecasting and risk management.
As businesses increasingly rely on data to make decisions, predictive modeling is becoming more and more important.
While it can be complex, predictive modeling is a powerful tool that gives businesses a competitive advantage.
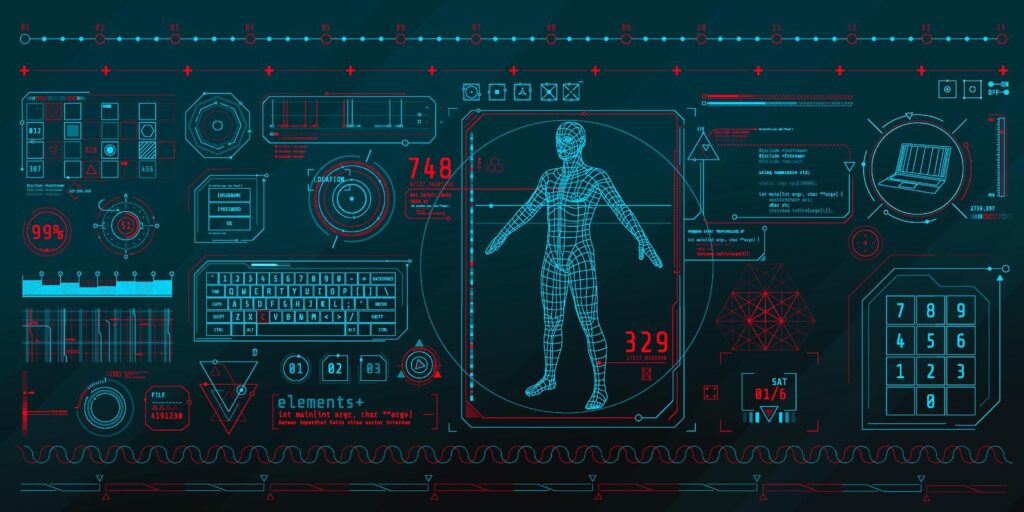
2.) Big Data Analytics
These days, it seems like everyone is talking about big data.
And with good reason – organizations of all sizes are sitting on mountains of data, and they’re increasingly turning to data scientists to help them make sense of it all.
But what exactly is big data? And what does it mean for data science?
Simply put, big data is a term used to describe datasets that are too large and complex for traditional data processing techniques.
Big data typically refers to datasets of a few terabytes or more.
But size isn’t the only defining characteristic – big data is also characterized by its high Velocity (the speed at which data is generated), Variety (the different types of data), and Volume (the amount of the information).
Given the enormity of big data, it’s not surprising that organizations are struggling to make sense of it all.
That’s where data science comes in.
Data scientists use various methods to wrangle big data, including distributed computing and other decentralized technologies.
With the help of data science, organizations are beginning to unlock the hidden value in their big data.
By harnessing the power of big data analytics, they can improve their decision-making, better understand their customers, and develop new products and services.
3.) Auto Machine Learning
Auto machine learning is a research topic in data science concerned with developing algorithms that can automatically learn from data without intervention.
This area of research is vital because it allows data scientists to automate the process of writing code for every dataset.
This allows us to focus on other tasks, such as model selection and validation.
Auto machine learning algorithms can learn from data in a hands-off way for the data scientist – while still providing incredible insights.
This makes them a valuable tool for data scientists who either don’t have the skills to do their own analysis or are struggling.
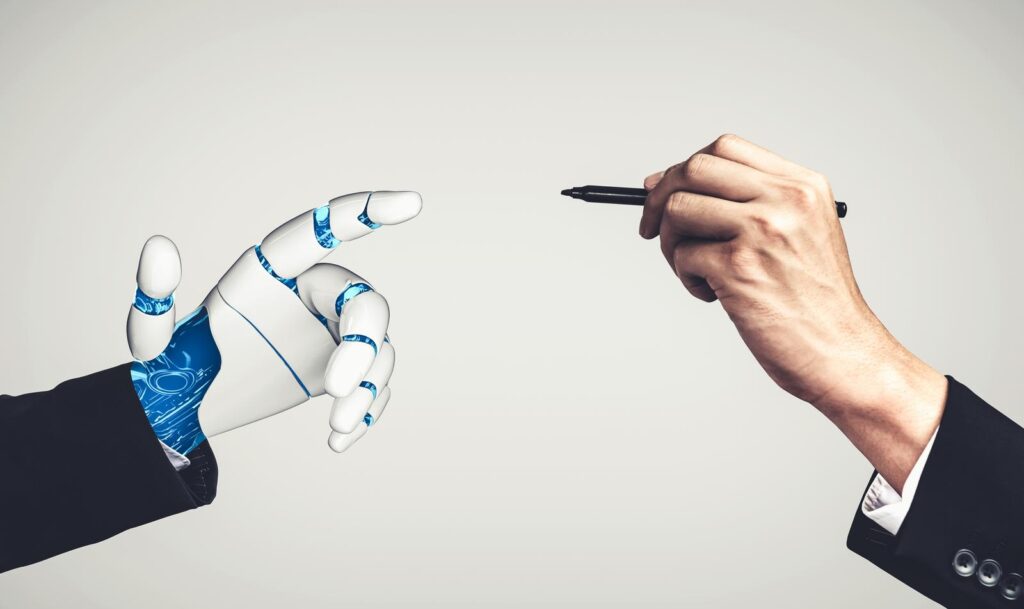
4.) Text Mining
Text mining is a research topic in data science that deals with text data extraction.
This area of research is important because it allows us to get as much information as possible from the vast amount of text data available today.
Text mining techniques can extract information from text data, such as keywords, sentiments, and relationships.
This information can be used for various purposes, such as model building and predictive analytics.
5.) Natural Language Processing
Natural language processing is a data science research topic that analyzes human language data.
This area of research is important because it allows us to understand and make sense of the vast amount of text data available today.
Natural language processing techniques can build predictive and interactive models from any language data.
Natural Language processing is pretty broad, and recent advances like GPT-3 have pushed this topic to the forefront.
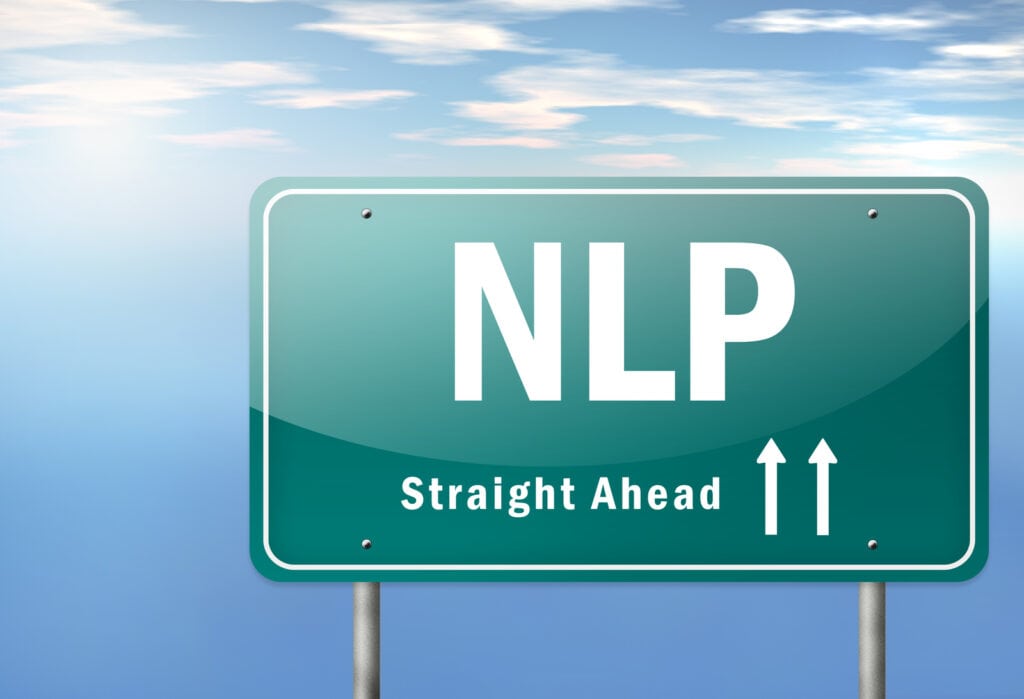
6.) Recommender Systems
Recommender systems are an exciting topic in data science because they allow us to make better products, services, and content recommendations.
Businesses can better understand their customers and their needs by using recommender systems.
This, in turn, allows them to develop better products and services that meet the needs of their customers.
Recommender systems are also used to recommend content to users.
This can be done on an individual level or at a group level.
Think about Netflix, for example, always knowing what you want to watch!
Recommender systems are a valuable tool for businesses and users alike.
7.) Deep Learning
Deep learning is a research topic in data science that deals with artificial neural networks.
These networks are composed of multiple layers, and each layer is formed from various nodes.
Deep learning networks can learn from data similarly to how humans learn, irrespective of the data distribution.
This makes them a valuable tool for data scientists looking to build models that can learn from data independently.
The deep learning network has become very popular in recent years because of its ability to achieve state-of-the-art results on various tasks.
There seems to be a new SOTA deep learning algorithm research paper on https://arxiv.org/ every single day!
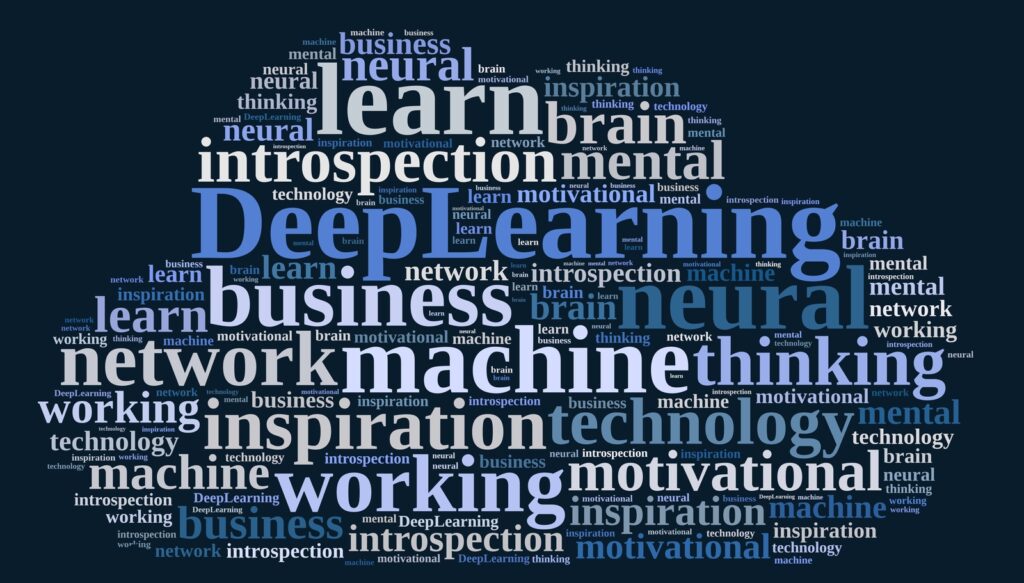
8.) Reinforcement Learning
Reinforcement learning is a research topic in data science that deals with algorithms that can learn on multiple levels from interactions with their environment.
This area of research is essential because it allows us to develop algorithms that can learn non-greedy approaches to decision-making, allowing businesses and companies to win in the long term compared to the short.
9.) Data Visualization
Data visualization is an excellent research topic in data science because it allows us to see our data in a way that is easy to understand.
Data visualization techniques can be used to create charts, graphs, and other visual representations of data.
This allows us to see the patterns and trends hidden in our data.
Data visualization is also used to communicate results to others.
This allows us to share our findings with others in a way that is easy to understand.
There are many ways to contribute to and learn about data visualization.
Some ways include attending conferences, reading papers, and contributing to open-source projects.
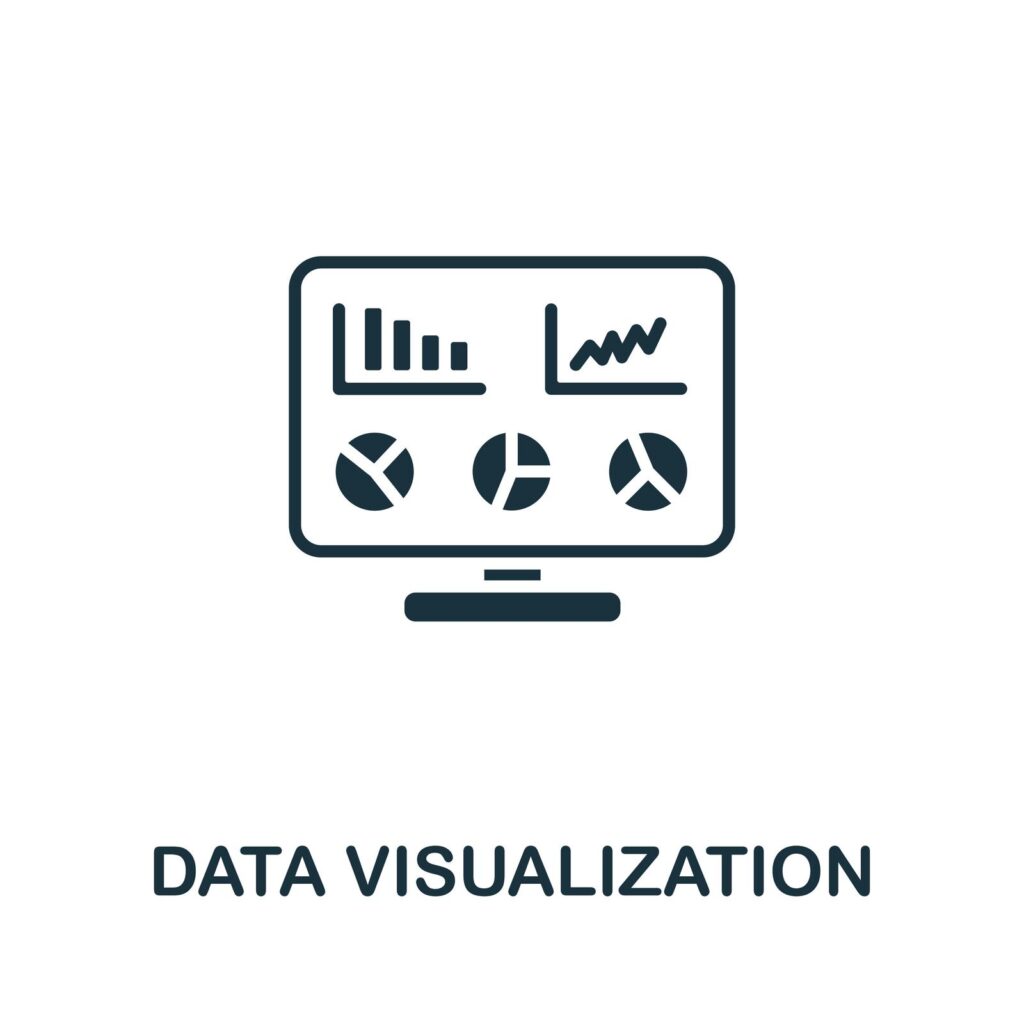
10.) Predictive Maintenance
Predictive maintenance is a hot topic in data science because it allows us to prevent failures before they happen.
This is done using data analytics to predict when a failure will occur.
This allows us to take corrective action before the failure actually happens.
While this sounds simple, avoiding false positives while keeping recall is challenging and an area wide open for advancement.
11.) Financial Analysis
Financial analysis is an older topic that has been around for a while but is still a great field where contributions can be felt.
Current researchers are focused on analyzing macroeconomic data to make better financial decisions.
This is done by analyzing the data to identify trends and patterns.
Financial analysts can use this information to make informed decisions about where to invest their money.
Financial analysis is also used to predict future economic trends.
This allows businesses and individuals to prepare for potential financial hardships and enable companies to be cash-heavy during good economic conditions.
Overall, financial analysis is a valuable tool for anyone looking to make better financial decisions.
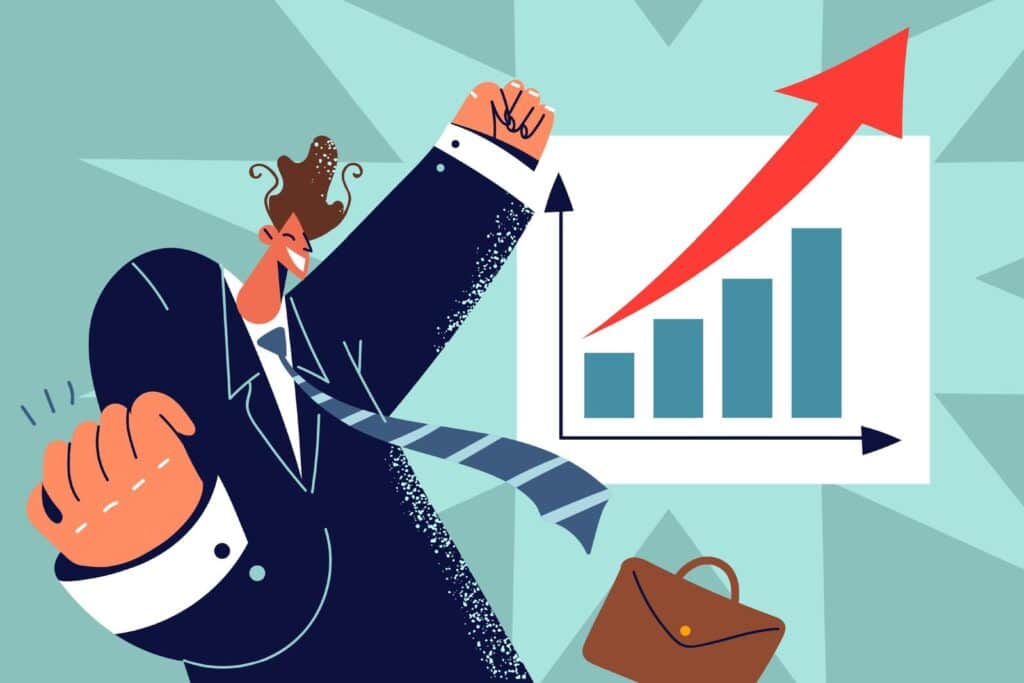
12.) Image Recognition
Image recognition is one of the hottest topics in data science because it allows us to identify objects in images.
This is done using artificial intelligence algorithms that can learn from data and understand what objects you’re looking for.
This allows us to build models that can accurately recognize objects in images and video.
This is a valuable tool for businesses and individuals who want to be able to identify objects in images.
Think about security, identification, routing, traffic, etc.
Image Recognition has gained a ton of momentum recently – for a good reason.
13.) Fraud Detection
Fraud detection is a great topic in data science because it allows us to identify fraudulent activity before it happens.
This is done by analyzing data to look for patterns and trends that may be associated with the fraud.
Once our machine learning model recognizes some of these patterns in real time, it immediately detects fraud.
This allows us to take corrective action before the fraud actually happens.
Fraud detection is a valuable tool for anyone who wants to protect themselves from potential fraudulent activity.
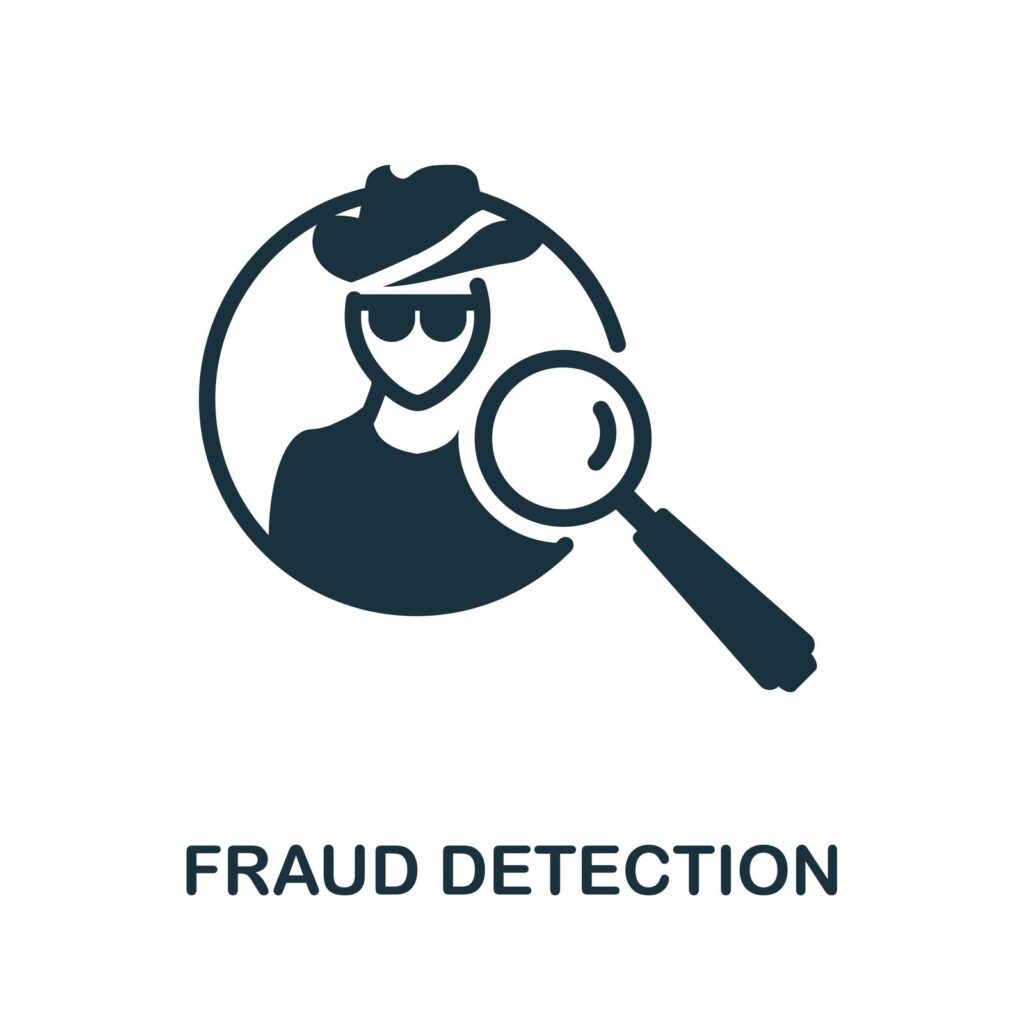
14.) Web Scraping
Web scraping is a controversial topic in data science because it allows us to collect data from the web, which is usually data you do not own.
This is done by extracting data from websites using scraping tools that are usually custom-programmed.
This allows us to collect data that would otherwise be inaccessible.
For obvious reasons, web scraping is a unique tool – giving you data your competitors would have no chance of getting.
I think there is an excellent opportunity to create new and innovative ways to make scraping accessible for everyone, not just those who understand Selenium and Beautiful Soup.
15.) Social Media Analysis
Social media analysis is not new; many people have already created exciting and innovative algorithms to study this.
However, it is still a great data science research topic because it allows us to understand how people interact on social media.
This is done by analyzing data from social media platforms to look for insights, bots, and recent societal trends.
Once we understand these practices, we can use this information to improve our marketing efforts.
For example, if we know that a particular demographic prefers a specific type of content, we can create more content that appeals to them.
Social media analysis is also used to understand how people interact with brands on social media.
This allows businesses to understand better what their customers want and need.
Overall, social media analysis is valuable for anyone who wants to improve their marketing efforts or understand how customers interact with brands.
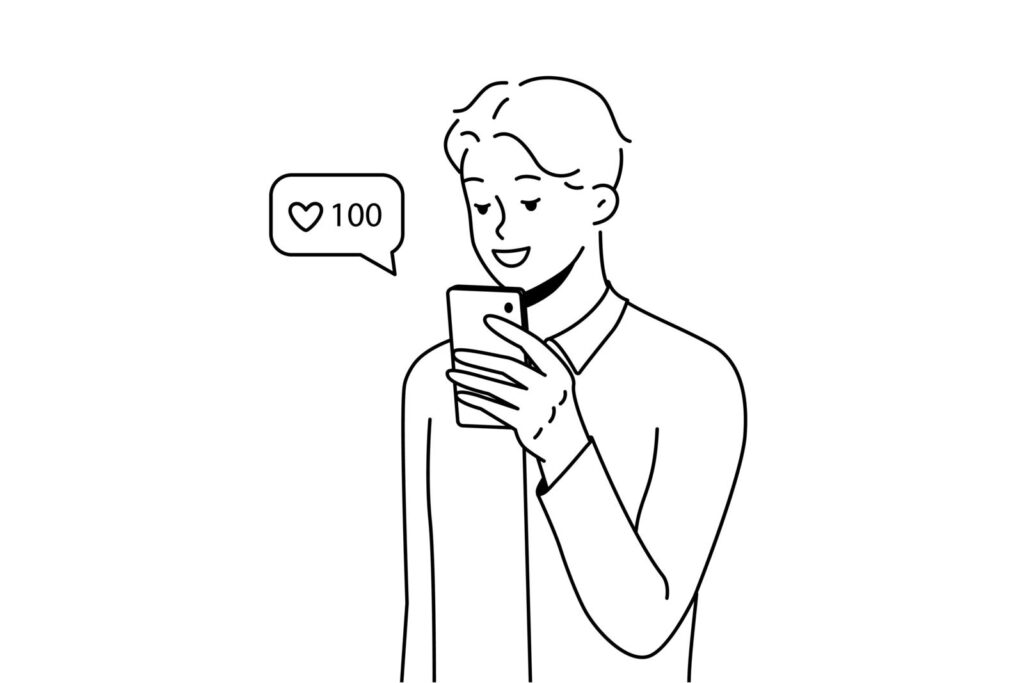
16.) GPU Computing
GPU computing is a fun new research topic in data science because it allows us to process data much faster than traditional CPUs .
Due to how GPUs are made, they’re incredibly proficient at intense matrix operations, outperforming traditional CPUs by very high margins.
While the computation is fast, the coding is still tricky.
There is an excellent research opportunity to bring these innovations to non-traditional modules, allowing data science to take advantage of GPU computing outside of deep learning.
17.) Quantum Computing
Quantum computing is a new research topic in data science and physics because it allows us to process data much faster than traditional computers.
It also opens the door to new types of data.
There are just some problems that can’t be solved utilizing outside of the classical computer.
For example, if you wanted to understand how a single atom moved around, a classical computer couldn’t handle this problem.
You’ll need to utilize a quantum computer to handle quantum mechanics problems.
This may be the “hottest” research topic on the planet right now, with some of the top researchers in computer science and physics worldwide working on it.
You could be too.
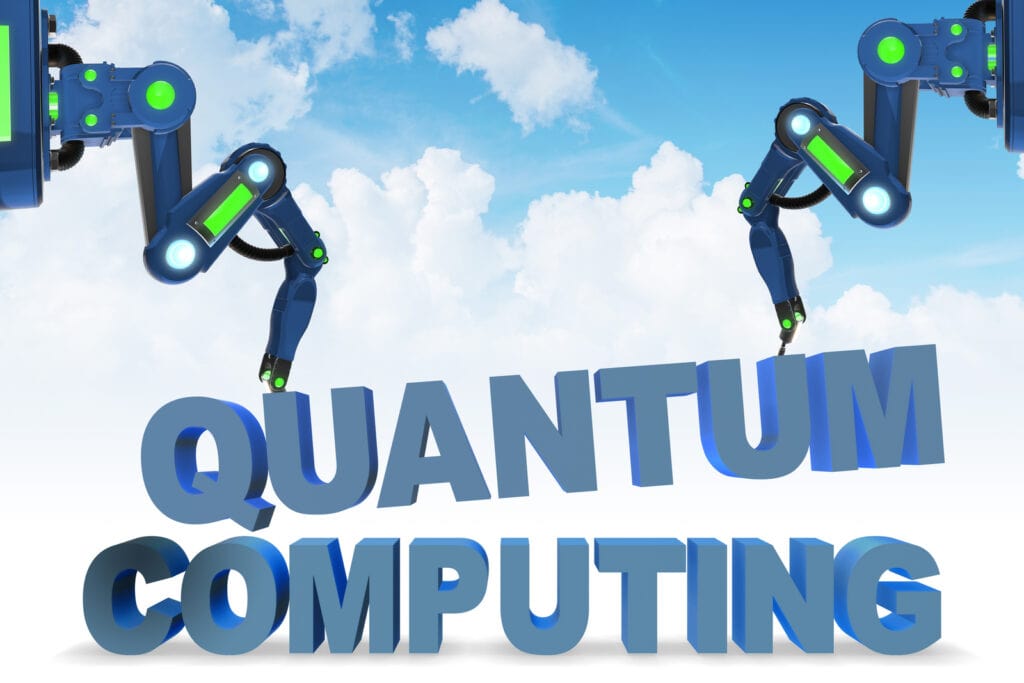
18.) Genomics
Genomics may be the only research topic that can compete with quantum computing regarding the “number of top researchers working on it.”
Genomics is a fantastic intersection of data science because it allows us to understand how genes work.
This is done by sequencing the DNA of different organisms to look for insights into our and other species.
Once we understand these patterns, we can use this information to improve our understanding of diseases and create new and innovative treatments for them.
Genomics is also used to study the evolution of different species.
Genomics is the future and a field begging for new and exciting research professionals to take it to the next step.
19.) Location-based services
Location-based services are an old and time-tested research topic in data science.
Since GPS and 4g cell phone reception became a thing, we’ve been trying to stay informed about how humans interact with their environment.
This is done by analyzing data from GPS tracking devices, cell phone towers, and Wi-Fi routers to look for insights into how humans interact.
Once we understand these practices, we can use this information to improve our geotargeting efforts, improve maps, find faster routes, and improve cohesion throughout a community.
Location-based services are used to understand the user, something every business could always use a little bit more of.
While a seemingly “stale” field, location-based services have seen a revival period with self-driving cars.
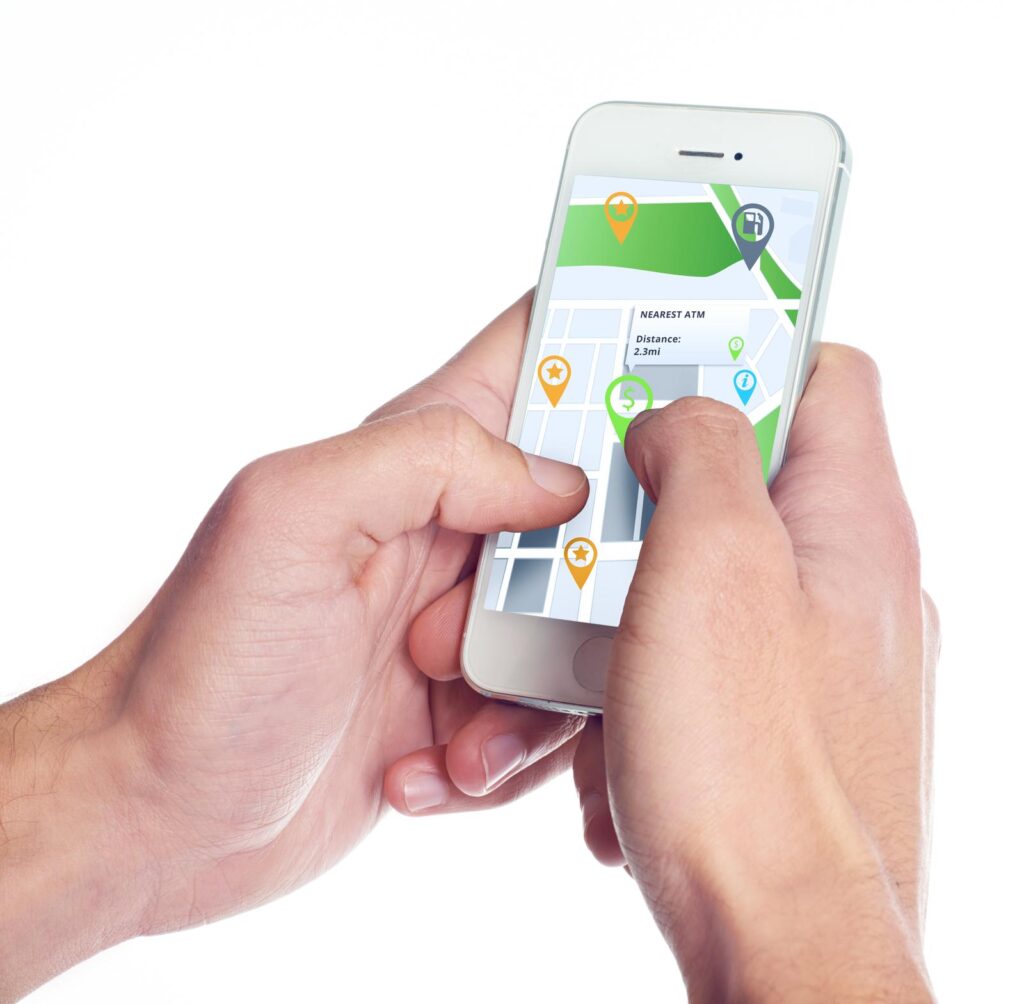
20.) Smart City Applications
Smart city applications are all the rage in data science research right now.
By harnessing the power of data, cities can become more efficient and sustainable.
But what exactly are smart city applications?
In short, they are systems that use data to improve city infrastructure and services.
This can include anything from traffic management and energy use to waste management and public safety.
Data is collected from various sources, including sensors, cameras, and social media.
It is then analyzed to identify tendencies and habits.
This information can make predictions about future needs and optimize city resources.
As more and more cities strive to become “smart,” the demand for data scientists with expertise in smart city applications is only growing.
21.) Internet Of Things (IoT)
The Internet of Things, or IoT, is exciting and new data science and sustainability research topic.
IoT is a network of physical objects embedded with sensors and connected to the internet.
These objects can include everything from alarm clocks to refrigerators; they’re all connected to the internet.
That means that they can share data with computers.
And that’s where data science comes in.
Data scientists are using IoT data to learn everything from how people use energy to how traffic flows through a city.
They’re also using IoT data to predict when an appliance will break down or when a road will be congested.
Really, the possibilities are endless.
With such a wide-open field, it’s easy to see why IoT is being researched by some of the top professionals in the world.
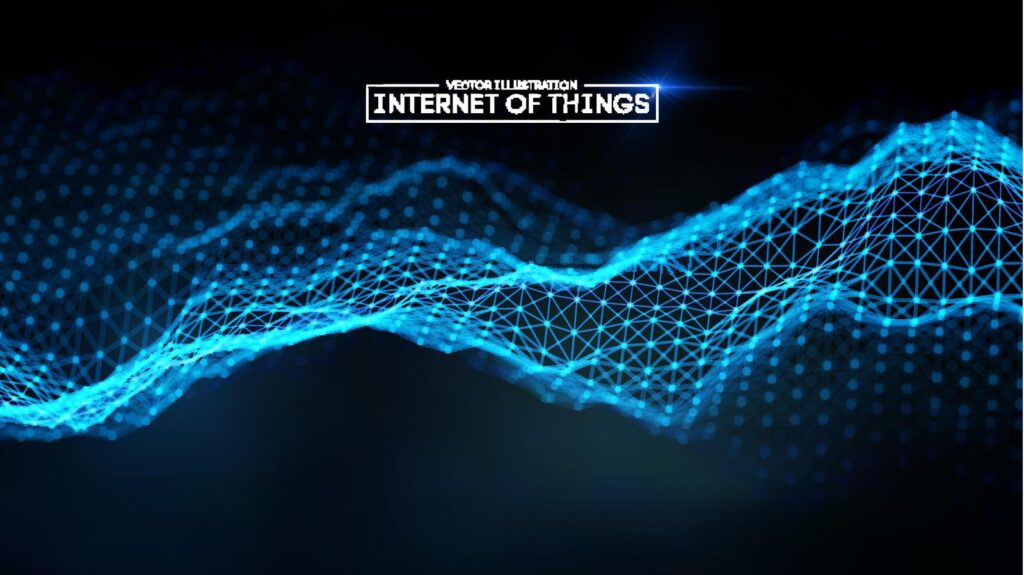
22.) Cybersecurity
Cybersecurity is a relatively new research topic in data science and in general, but it’s already garnering a lot of attention from businesses and organizations.
After all, with the increasing number of cyber attacks in recent years, it’s clear that we need to find better ways to protect our data.
While most of cybersecurity focuses on infrastructure, data scientists can leverage historical events to find potential exploits to protect their companies.
Sometimes, looking at a problem from a different angle helps, and that’s what data science brings to cybersecurity.
Also, data science can help to develop new security technologies and protocols.
As a result, cybersecurity is a crucial data science research area and one that will only become more important in the years to come.
23.) Blockchain
Blockchain is an incredible new research topic in data science for several reasons.
First, it is a distributed database technology that enables secure, transparent, and tamper-proof transactions.
Did someone say transmitting data?
This makes it an ideal platform for tracking data and transactions in various industries.
Second, blockchain is powered by cryptography, which not only makes it highly secure – but is a familiar foe for data scientists.
Finally, blockchain is still in its early stages of development, so there is much room for research and innovation.
As a result, blockchain is a great new research topic in data science that vows to revolutionize how we store, transmit and manage data.
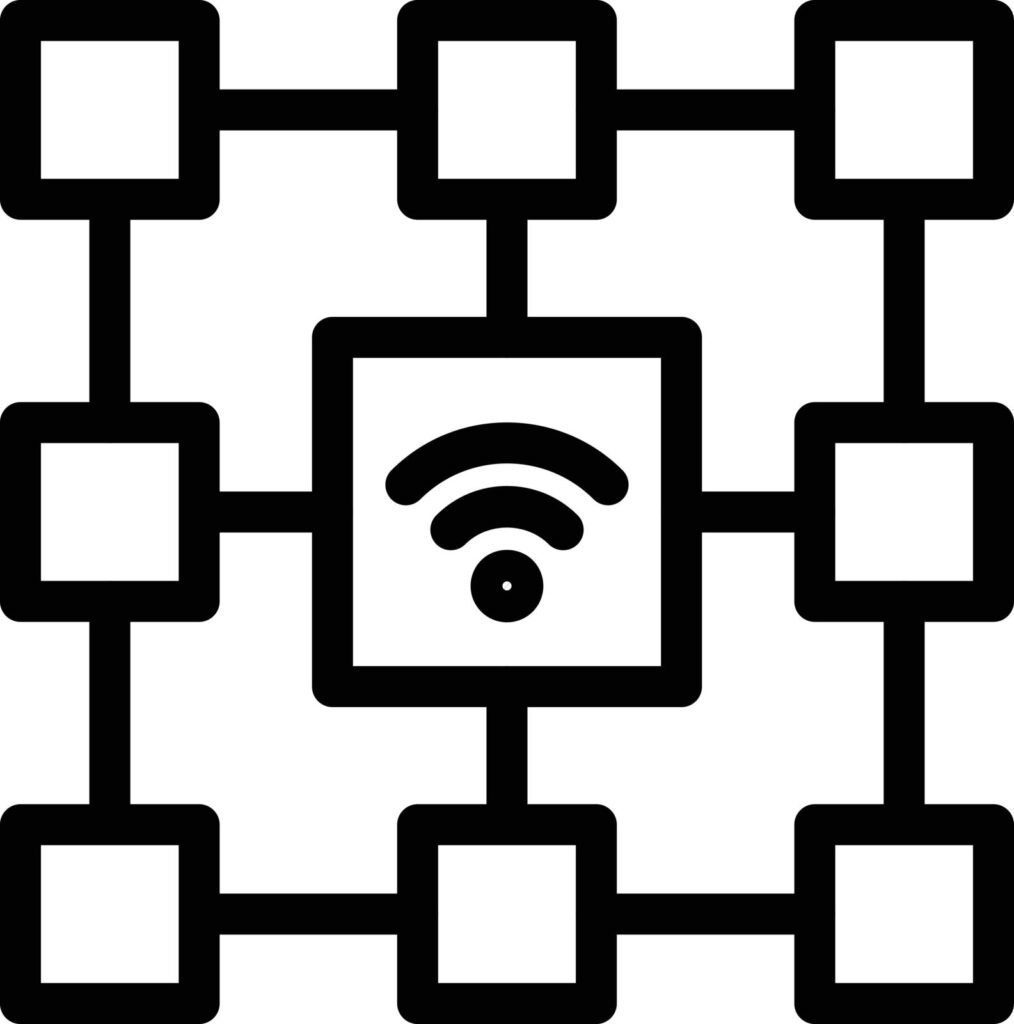
24.) Sustainability
Sustainability is a relatively new research topic in data science, but it is gaining traction quickly.
To keep up with this demand, The Wharton School of the University of Pennsylvania has started to offer an MBA in Sustainability .
This demand isn’t shocking, and some of the reasons include the following:
Sustainability is an important issue that is relevant to everyone.
Datasets on sustainability are constantly growing and changing, making it an exciting challenge for data scientists.
There hasn’t been a “set way” to approach sustainability from a data perspective, making it an excellent opportunity for interdisciplinary research.
As data science grows, sustainability will likely become an increasingly important research topic.
25.) Educational Data
Education has always been a great topic for research, and with the advent of big data, educational data has become an even richer source of information.
By studying educational data, researchers can gain insights into how students learn, what motivates them, and what barriers these students may face.
Besides, data science can be used to develop educational interventions tailored to individual students’ needs.
Imagine being the researcher that helps that high schooler pass mathematics; what an incredible feeling.
With the increasing availability of educational data, data science has enormous potential to improve the quality of education.
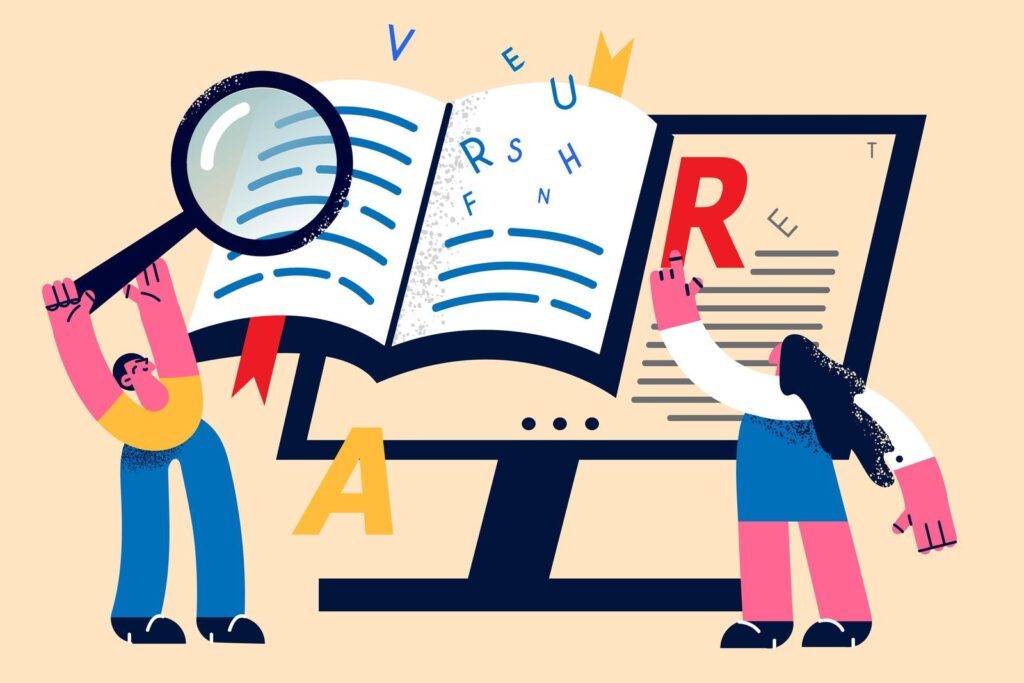
26.) Politics
As data science continues to evolve, so does the scope of its applications.
Originally used primarily for business intelligence and marketing, data science is now applied to various fields, including politics.
By analyzing large data sets, political scientists (data scientists with a cooler name) can gain valuable insights into voting patterns, campaign strategies, and more.
Further, data science can be used to forecast election results and understand the effects of political events on public opinion.
With the wealth of data available, there is no shortage of research opportunities in this field.
As data science evolves, so does our understanding of politics and its role in our world.
27.) Cloud Technologies
Cloud technologies are a great research topic.
It allows for the outsourcing and sharing of computer resources and applications all over the internet.
This lets organizations save money on hardware and maintenance costs while providing employees access to the latest and greatest software and applications.
I believe there is an argument that AWS could be the greatest and most technologically advanced business ever built (Yes, I know it’s only part of the company).
Besides, cloud technologies can help improve team members’ collaboration by allowing them to share files and work on projects together in real-time.
As more businesses adopt cloud technologies, data scientists must stay up-to-date on the latest trends in this area.
By researching cloud technologies, data scientists can help organizations to make the most of this new and exciting technology.
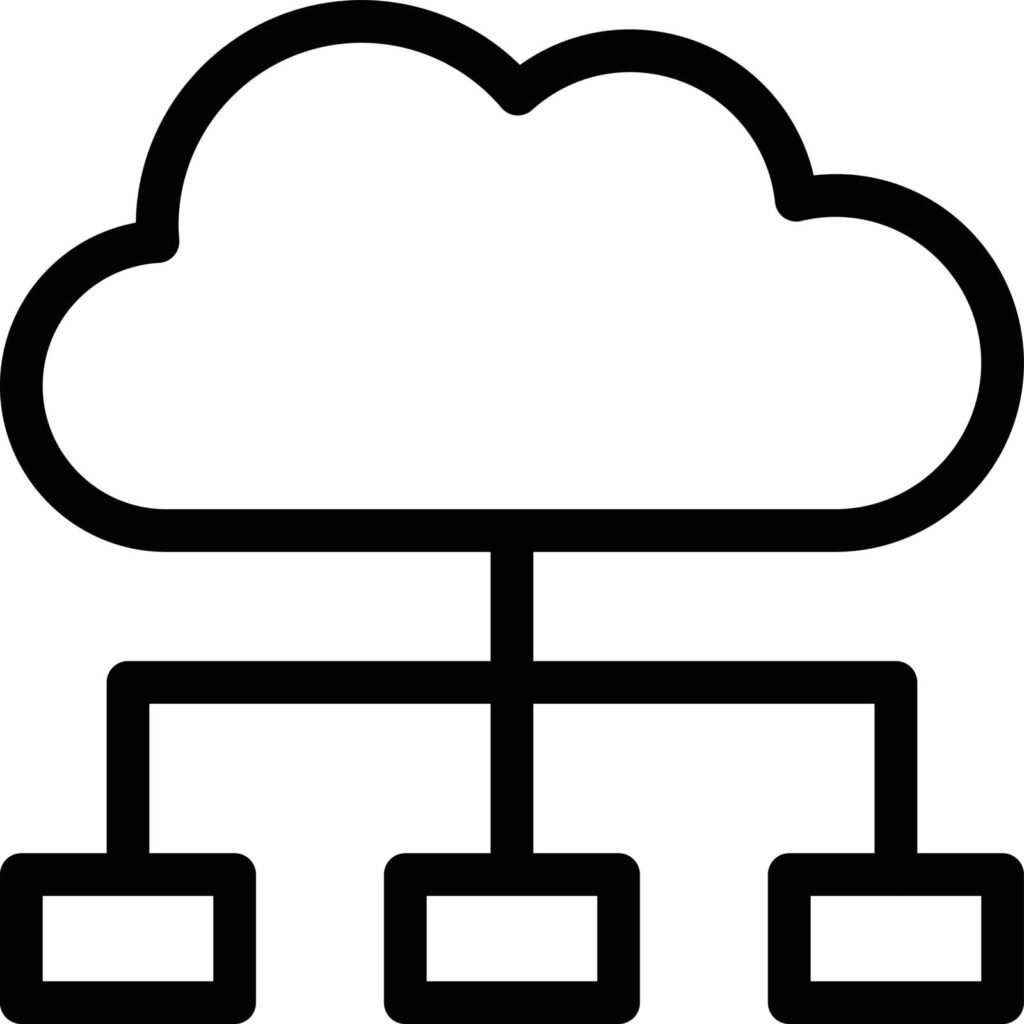
28.) Robotics
Robotics has recently become a household name, and it’s for a good reason.
First, robotics deals with controlling and planning physical systems, an inherently complex problem.
Second, robotics requires various sensors and actuators to interact with the world, making it an ideal application for machine learning techniques.
Finally, robotics is an interdisciplinary field that draws on various disciplines, such as computer science, mechanical engineering, and electrical engineering.
As a result, robotics is a rich source of research problems for data scientists.
29.) HealthCare
Healthcare is an industry that is ripe for data-driven innovation.
Hospitals, clinics, and health insurance companies generate a tremendous amount of data daily.
This data can be used to improve the quality of care and outcomes for patients.
This is perfect timing, as the healthcare industry is undergoing a significant shift towards value-based care, which means there is a greater need than ever for data-driven decision-making.
As a result, healthcare is an exciting new research topic for data scientists.
There are many different ways in which data can be used to improve healthcare, and there is a ton of room for newcomers to make discoveries.
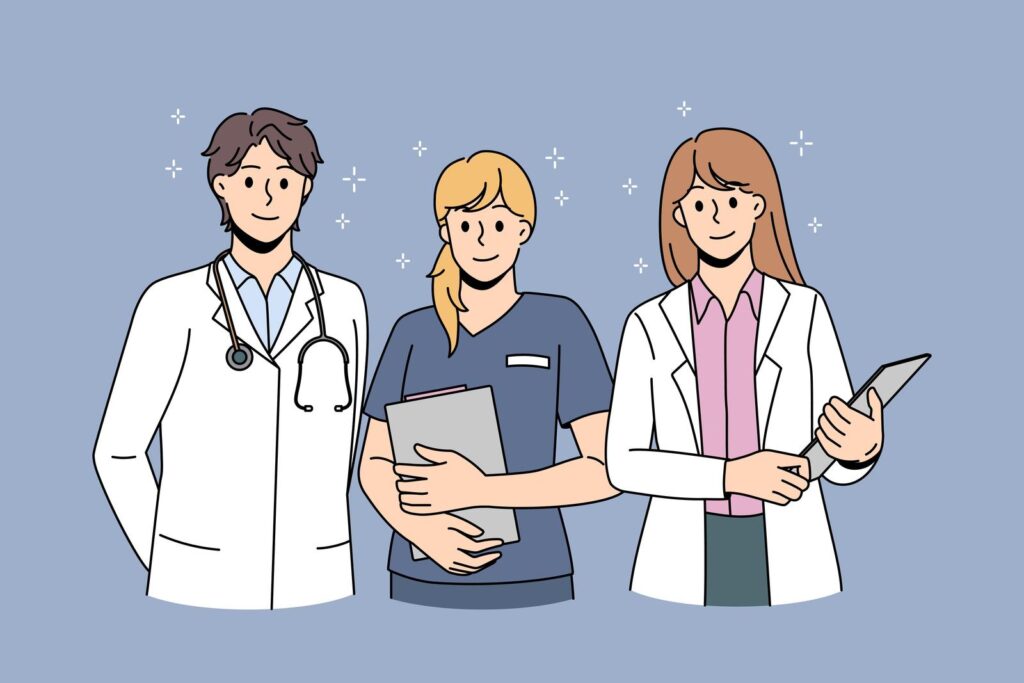
30.) Remote Work
There’s no doubt that remote work is on the rise.
In today’s global economy, more and more businesses are allowing their employees to work from home or anywhere else they can get a stable internet connection.
But what does this mean for data science? Well, for one thing, it opens up a whole new field of research.
For example, how does remote work impact employee productivity?
What are the best ways to manage and collaborate on data science projects when team members are spread across the globe?
And what are the cybersecurity risks associated with working remotely?
These are just a few of the questions that data scientists will be able to answer with further research.
So if you’re looking for a new topic to sink your teeth into, remote work in data science is a great option.
31.) Data-Driven Journalism
Data-driven journalism is an exciting new field of research that combines the best of both worlds: the rigor of data science with the creativity of journalism.
By applying data analytics to large datasets, journalists can uncover stories that would otherwise be hidden.
And telling these stories compellingly can help people better understand the world around them.
Data-driven journalism is still in its infancy, but it has already had a major impact on how news is reported.
In the future, it will only become more important as data becomes increasingly fluid among journalists.
It is an exciting new topic and research field for data scientists to explore.
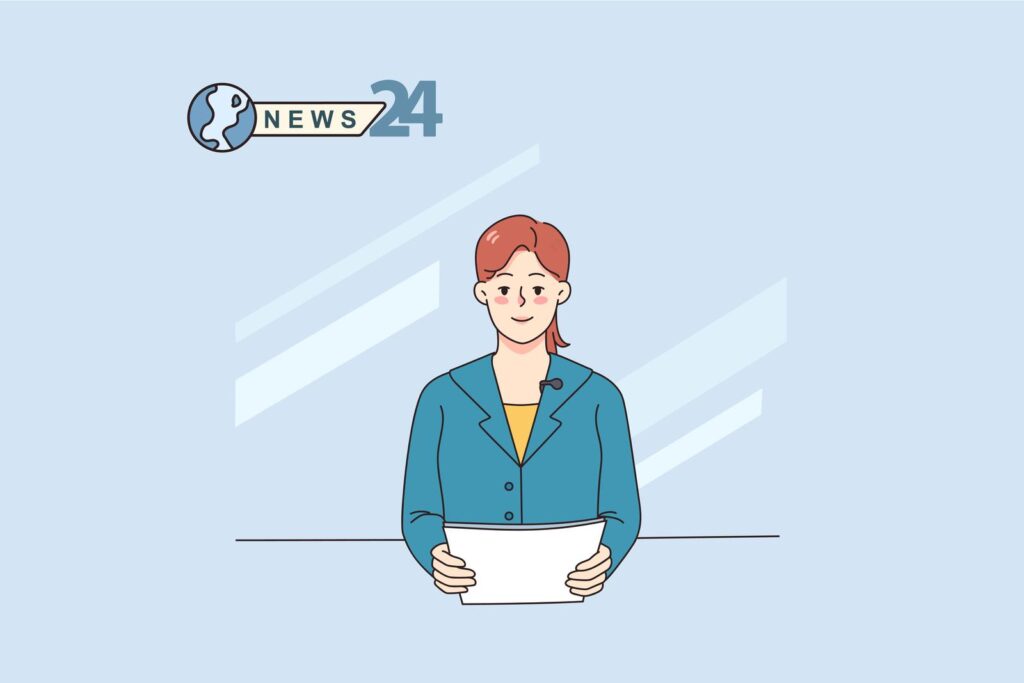
32.) Data Engineering
Data engineering is a staple in data science, focusing on efficiently managing data.
Data engineers are responsible for developing and maintaining the systems that collect, process, and store data.
In recent years, there has been an increasing demand for data engineers as the volume of data generated by businesses and organizations has grown exponentially.
Data engineers must be able to design and implement efficient data-processing pipelines and have the skills to optimize and troubleshoot existing systems.
If you are looking for a challenging research topic that would immediately impact you worldwide, then improving or innovating a new approach in data engineering would be a good start.
33.) Data Curation
Data curation has been a hot topic in the data science community for some time now.
Curating data involves organizing, managing, and preserving data so researchers can use it.
Data curation can help to ensure that data is accurate, reliable, and accessible.
It can also help to prevent research duplication and to facilitate the sharing of data between researchers.
Data curation is a vital part of data science. In recent years, there has been an increasing focus on data curation, as it has become clear that it is essential for ensuring data quality.
As a result, data curation is now a major research topic in data science.
There are numerous books and articles on the subject, and many universities offer courses on data curation.
Data curation is an integral part of data science and will only become more important in the future.
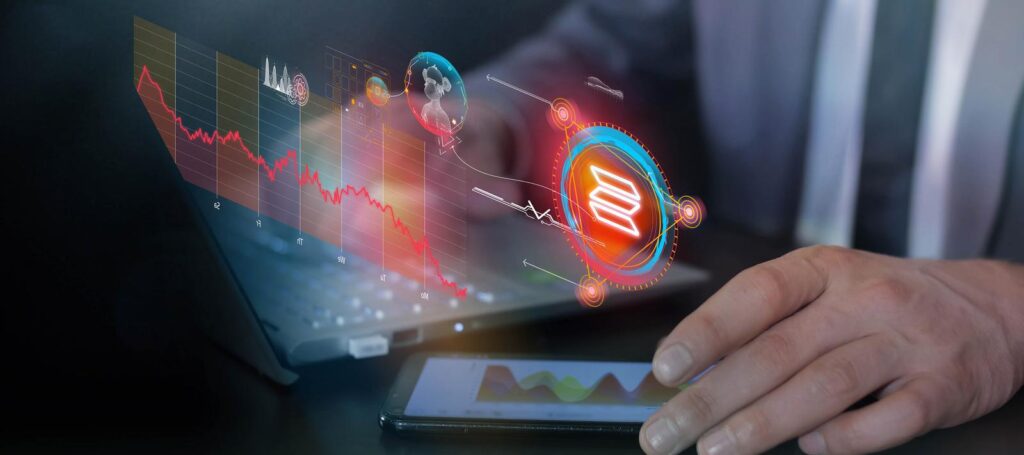
34.) Meta-Learning
Meta-learning is gaining a ton of steam in data science. It’s learning how to learn.
So, if you can learn how to learn, you can learn anything much faster.
Meta-learning is mainly used in deep learning, as applications outside of this are generally pretty hard.
In deep learning, many parameters need to be tuned for a good model, and there’s usually a lot of data.
You can save time and effort if you can automatically and quickly do this tuning.
In machine learning, meta-learning can improve models’ performance by sharing knowledge between different models.
For example, if you have a bunch of different models that all solve the same problem, then you can use meta-learning to share the knowledge between them to improve the cluster (groups) overall performance.
I don’t know how anyone looking for a research topic could stay away from this field; it’s what the Terminator warned us about!
35.) Data Warehousing
A data warehouse is a system used for data analysis and reporting.
It is a central data repository created by combining data from multiple sources.
Data warehouses are often used to store historical data, such as sales data, financial data, and customer data.
This data type can be used to create reports and perform statistical analysis.
Data warehouses also store data that the organization is not currently using.
This type of data can be used for future research projects.
Data warehousing is an incredible research topic in data science because it offers a variety of benefits.
Data warehouses help organizations to save time and money by reducing the need for manual data entry.
They also help to improve the accuracy of reports and provide a complete picture of the organization’s performance.
Data warehousing feels like one of the weakest parts of the Data Science Technology Stack; if you want a research topic that could have a monumental impact – data warehousing is an excellent place to look.
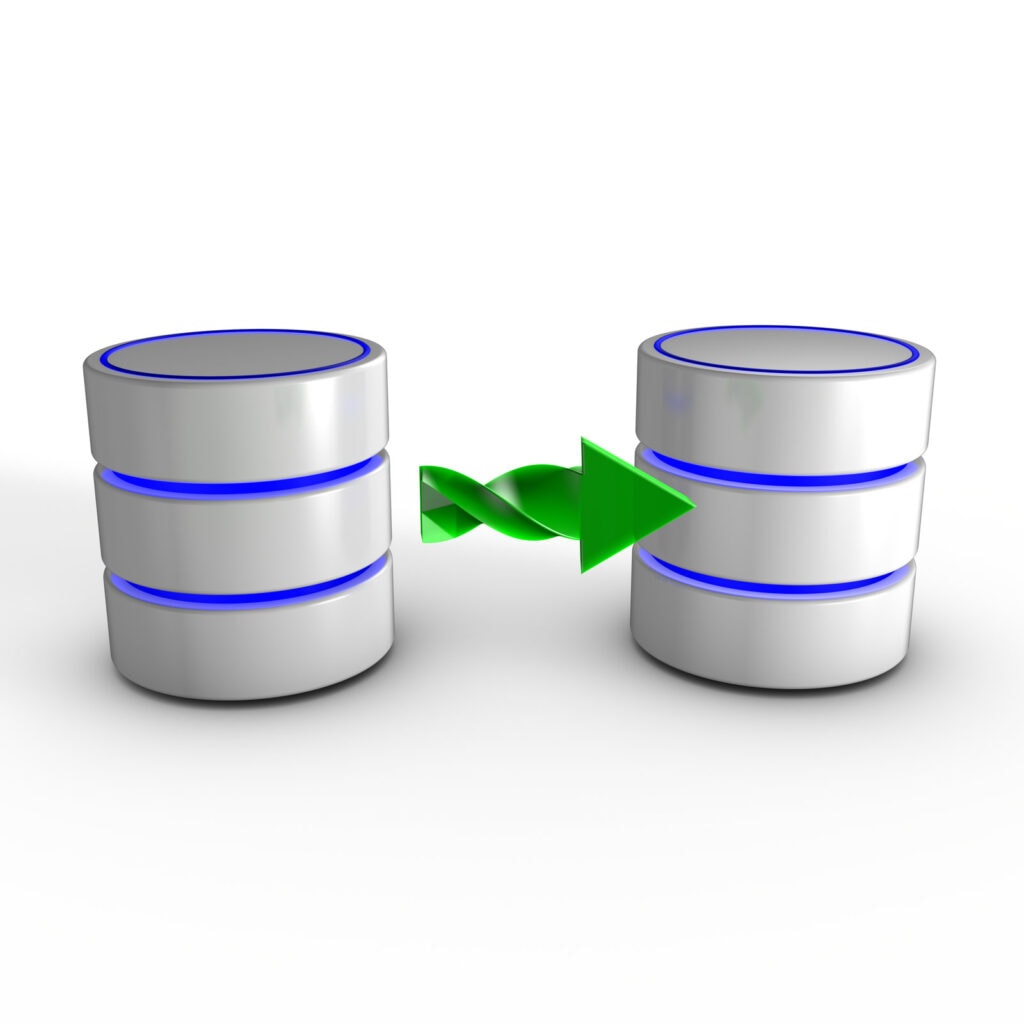
36.) Business Intelligence
Business intelligence aims to collect, process, and analyze data to help businesses make better decisions.
Business intelligence can improve marketing, sales, customer service, and operations.
It can also be used to identify new business opportunities and track competition.
BI is business and another tool in your company’s toolbox to continue dominating your area.
Data science is the perfect tool for business intelligence because it combines statistics, computer science, and machine learning.
Data scientists can use business intelligence to answer questions like, “What are our customers buying?” or “What are our competitors doing?” or “How can we increase sales?”
Business intelligence is a great way to improve your business’s bottom line and an excellent opportunity to dive deep into a well-respected research topic.
37.) Crowdsourcing
One of the newest areas of research in data science is crowdsourcing.
Crowdsourcing is a process of sourcing tasks or projects to a large group of people, typically via the internet.
This can be done for various purposes, such as gathering data, developing new algorithms, or even just for fun (think: online quizzes and surveys).
But what makes crowdsourcing so powerful is that it allows businesses and organizations to tap into a vast pool of talent and resources they wouldn’t otherwise have access to.
And with the rise of social media, it’s easier than ever to connect with potential crowdsource workers worldwide.
Imagine if you could effect that, finding innovative ways to improve how people work together.
That would have a huge effect.
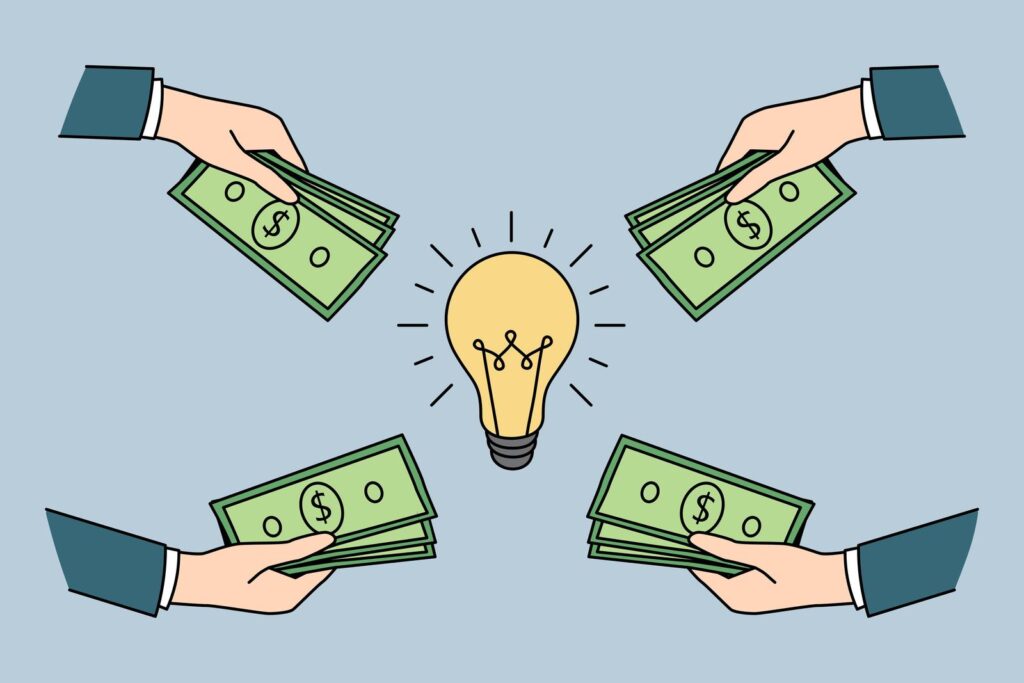
Final Thoughts, Are These Research Topics In Data Science For You?
Thirty-seven different research topics in data science are a lot to take in, but we hope you found a research topic that interests you.
If not, don’t worry – there are plenty of other great topics to explore.
The important thing is to get started with your research and find ways to apply what you learn to real-world problems.
We wish you the best of luck as you begin your data science journey!
Other Data Science Articles
We love talking about data science; here are a couple of our favorite articles:
- Why Are You Interested In Data Science?
- Recent Posts
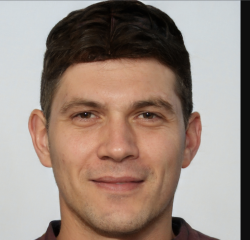
- How YubiKey Works for Enhanced Data Security [Unlock the Mystery] - April 25, 2024
- Is DevOps better than software development? [Find out the surprising truth!] - April 24, 2024
- Crack Puzzle Questions for Machine Learning Interview [Ace Your Interview Now] - April 24, 2024
Trending now
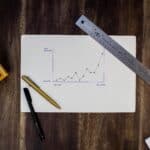
- Warning : Invalid argument supplied for foreach() in /home/customer/www/opendatascience.com/public_html/wp-includes/nav-menu.php on line 95 Warning : array_merge(): Expected parameter 2 to be an array, null given in /home/customer/www/opendatascience.com/public_html/wp-includes/nav-menu.php on line 102
- ODSC EUROPE
- AI+ Training
- Speak at ODSC

- Data Analytics
- Data Engineering
- Data Visualization
- Deep Learning
- Generative AI
- Machine Learning
- NLP and LLMs
- Business & Use Cases
- Career Advice
- Write for us
- ODSC Community Slack Channel
- Upcoming Webinars

10 Compelling Machine Learning Ph.D. Dissertations for 2020
Machine Learning Modeling Research posted by Daniel Gutierrez, ODSC August 19, 2020 Daniel Gutierrez, ODSC
As a data scientist, an integral part of my work in the field revolves around keeping current with research coming out of academia. I frequently scour arXiv.org for late-breaking papers that show trends and reveal fertile areas of research. Other sources of valuable research developments are in the form of Ph.D. dissertations, the culmination of a doctoral candidate’s work to confer his/her degree. Ph.D. candidates are highly motivated to choose research topics that establish new and creative paths toward discovery in their field of study. Their dissertations are highly focused on a specific problem. If you can find a dissertation that aligns with your areas of interest, consuming the research is an excellent way to do a deep dive into the technology. After reviewing hundreds of recent theses from universities all over the country, I present 10 machine learning dissertations that I found compelling in terms of my own areas of interest.
[Related article: Introduction to Bayesian Deep Learning ]
I hope you’ll find several that match your own fields of inquiry. Each thesis may take a while to consume but will result in hours of satisfying summer reading. Enjoy!
1. Bayesian Modeling and Variable Selection for Complex Data
As we routinely encounter high-throughput data sets in complex biological and environmental research, developing novel models and methods for variable selection has received widespread attention. This dissertation addresses a few key challenges in Bayesian modeling and variable selection for high-dimensional data with complex spatial structures.
2. Topics in Statistical Learning with a Focus on Large Scale Data
Big data vary in shape and call for different approaches. One type of big data is the tall data, i.e., a very large number of samples but not too many features. This dissertation describes a general communication-efficient algorithm for distributed statistical learning on this type of big data. The algorithm distributes the samples uniformly to multiple machines, and uses a common reference data to improve the performance of local estimates. The algorithm enables potentially much faster analysis, at a small cost to statistical performance.
Another type of big data is the wide data, i.e., too many features but a limited number of samples. It is also called high-dimensional data, to which many classical statistical methods are not applicable.
This dissertation discusses a method of dimensionality reduction for high-dimensional classification. The method partitions features into independent communities and splits the original classification problem into separate smaller ones. It enables parallel computing and produces more interpretable results.
3. Sets as Measures: Optimization and Machine Learning
The purpose of this machine learning dissertation is to address the following simple question:
How do we design efficient algorithms to solve optimization or machine learning problems where the decision variable (or target label) is a set of unknown cardinality?
Optimization and machine learning have proved remarkably successful in applications requiring the choice of single vectors. Some tasks, in particular many inverse problems, call for the design, or estimation, of sets of objects. When the size of these sets is a priori unknown, directly applying optimization or machine learning techniques designed for single vectors appears difficult. The work in this dissertation shows that a very old idea for transforming sets into elements of a vector space (namely, a space of measures), a common trick in theoretical analysis, generates effective practical algorithms.
4. A Geometric Perspective on Some Topics in Statistical Learning
Modern science and engineering often generate data sets with a large sample size and a comparably large dimension which puts classic asymptotic theory into question in many ways. Therefore, the main focus of this dissertation is to develop a fundamental understanding of statistical procedures for estimation and hypothesis testing from a non-asymptotic point of view, where both the sample size and problem dimension grow hand in hand. A range of different problems are explored in this thesis, including work on the geometry of hypothesis testing, adaptivity to local structure in estimation, effective methods for shape-constrained problems, and early stopping with boosting algorithms. The treatment of these different problems shares the common theme of emphasizing the underlying geometric structure.
5. Essays on Random Forest Ensembles
A random forest is a popular machine learning ensemble method that has proven successful in solving a wide range of classification problems. While other successful classifiers, such as boosting algorithms or neural networks, admit natural interpretations as maximum likelihood, a suitable statistical interpretation is much more elusive for a random forest. The first part of this dissertation demonstrates that a random forest is a fruitful framework in which to study AdaBoost and deep neural networks. The work explores the concept and utility of interpolation, the ability of a classifier to perfectly fit its training data. The second part of this dissertation places a random forest on more sound statistical footing by framing it as kernel regression with the proximity kernel. The work then analyzes the parameters that control the bandwidth of this kernel and discuss useful generalizations.
6. Marginally Interpretable Generalized Linear Mixed Models
A popular approach for relating correlated measurements of a non-Gaussian response variable to a set of predictors is to introduce latent random variables and fit a generalized linear mixed model. The conventional strategy for specifying such a model leads to parameter estimates that must be interpreted conditional on the latent variables. In many cases, interest lies not in these conditional parameters, but rather in marginal parameters that summarize the average effect of the predictors across the entire population. Due to the structure of the generalized linear mixed model, the average effect across all individuals in a population is generally not the same as the effect for an average individual. Further complicating matters, obtaining marginal summaries from a generalized linear mixed model often requires evaluation of an analytically intractable integral or use of an approximation. Another popular approach in this setting is to fit a marginal model using generalized estimating equations. This strategy is effective for estimating marginal parameters, but leaves one without a formal model for the data with which to assess quality of fit or make predictions for future observations. Thus, there exists a need for a better approach.
This dissertation defines a class of marginally interpretable generalized linear mixed models that leads to parameter estimates with a marginal interpretation while maintaining the desirable statistical properties of a conditionally specified model. The distinguishing feature of these models is an additive adjustment that accounts for the curvature of the link function and thereby preserves a specific form for the marginal mean after integrating out the latent random variables.
7. On the Detection of Hate Speech, Hate Speakers and Polarized Groups in Online Social Media
The objective of this dissertation is to explore the use of machine learning algorithms in understanding and detecting hate speech, hate speakers and polarized groups in online social media. Beginning with a unique typology for detecting abusive language, the work outlines the distinctions and similarities of different abusive language subtasks (offensive language, hate speech, cyberbullying and trolling) and how we might benefit from the progress made in each area. Specifically, the work suggests that each subtask can be categorized based on whether or not the abusive language being studied 1) is directed at a specific individual, or targets a generalized “Other” and 2) the extent to which the language is explicit versus implicit. The work then uses knowledge gained from this typology to tackle the “problem of offensive language” in hate speech detection.
8. Lasso Guarantees for Dependent Data
Serially correlated high dimensional data are prevalent in the big data era. In order to predict and learn the complex relationship among the multiple time series, high dimensional modeling has gained importance in various fields such as control theory, statistics, economics, finance, genetics and neuroscience. This dissertation studies a number of high dimensional statistical problems involving different classes of mixing processes.
9. Random forest robustness, variable importance, and tree aggregation
Random forest methodology is a nonparametric, machine learning approach capable of strong performance in regression and classification problems involving complex data sets. In addition to making predictions, random forests can be used to assess the relative importance of feature variables. This dissertation explores three topics related to random forests: tree aggregation, variable importance, and robustness.
10. Climate Data Computing: Optimal Interpolation, Averaging, Visualization and Delivery
This dissertation solves two important problems in the modern analysis of big climate data. The first is the efficient visualization and fast delivery of big climate data, and the second is a computationally extensive principal component analysis (PCA) using spherical harmonics on the Earth’s surface. The second problem creates a way to supply the data for the technology developed in the first. These two problems are computationally difficult, such as the representation of higher order spherical harmonics Y400, which is critical for upscaling weather data to almost infinitely fine spatial resolution.
I hope you enjoyed learning about these compelling machine learning dissertations.
Editor’s note: Interested in more data science research? Check out the Research Frontiers track at ODSC Europe this September 17-19 or the ODSC West Research Frontiers track this October 27-30.

Daniel Gutierrez, ODSC
Daniel D. Gutierrez is a practicing data scientist who’s been working with data long before the field came in vogue. As a technology journalist, he enjoys keeping a pulse on this fast-paced industry. Daniel is also an educator having taught data science, machine learning and R classes at the university level. He has authored four computer industry books on database and data science technology, including his most recent title, “Machine Learning and Data Science: An Introduction to Statistical Learning Methods with R.” Daniel holds a BS in Mathematics and Computer Science from UCLA.

University of Maryland Introduces AI Interdisciplinary Institute
AI and Data Science News posted by ODSC Team Apr 24, 2024 In the latest move that sees institutions of higher learning integrating AI, The University of Maryland...

AI is Revolutionizing Cardiovascular Risk Assessments
AI and Data Science News posted by ODSC Team Apr 24, 2024 In a new study conducted by Cedars-Sinai, researchers have leveraged artificial intelligence to significantly advance how...

Microsoft Unveils New Cost Effective AI Model – Phi-3
AI and Data Science News posted by ODSC Team Apr 24, 2024 Microsoft announced on Tuesday the launch of Phi-3-mini, a new lightweight AI model aimed at providing...


Analytics Insight
Compelling Thesis Topics in the Field of Data Science 2024
Dynamic Thesis Topics Propelling Data Science into 2024’s Technological Frontier
As the realm of data science continues to evolve, students seeking to make their mark in this dynamic field are confronted with the challenge of selecting thesis topics that are not only relevant but also hold the promise of contributing significantly to the discipline. In 2024, the landscape of data science is marked by a fusion of emerging technologies, ethical considerations, and real-world applications. In this article, we explore ten compelling thesis topics that encapsulate the essence of contemporary data science.
Deep Learning: Unraveling the Depths of Neural Networks:
Deep learning remains at the forefront of data science, driving advancements in image recognition, natural language processing, and more. A thesis in this domain could delve into optimizing deep learning architectures, exploring transfer learning applications, or investigating the interpretability of complex neural networks.
Exploratory Data Analysis (EDA): Navigating the Data Wilderness:
EDA is the compass that guides data scientists through uncharted territories. A thesis on exploratory data analysis could focus on developing innovative EDA techniques, integrating visualizations for deeper insights, or applying EDA methodologies to specific industries such as healthcare or finance.
Fake News Detection: The Battle Against Information Manipulation:
In an era dominated by information, combating fake news is paramount. A thesis in fake news detection could explore novel machine learning algorithms , examine the role of social media in spreading misinformation, or propose frameworks for automated verification and fact-checking.
Chatbot Revolution: Bridging the Human-Machine Communication Gap:
Chatbots have become ubiquitous, transforming customer service and user engagement. A thesis on chatbots could investigate natural language processing algorithms, assess user experience in chatbot interactions, or explore ethical considerations in the deployment of conversational agents.
Credit Card Fraud Detection: Safeguarding Financial Transactions:
As digital transactions surge, the need for robust fraud detection systems intensifies. A thesis in credit card fraud detection could explore anomaly detection methods, leverage machine learning for real-time monitoring, or investigate the impact of imbalanced datasets on fraud prediction models.
Data Visualization: Painting Insights with Data:
Data visualization is the art of storytelling in the data science realm. A thesis on data visualization could delve into the design principles for effective visualizations, explore the impact of storytelling in conveying data insights, or assess the accessibility of visualizations for diverse audiences.
Natural Language Processing (NLP): Decoding the Language of Machines:
Natural Language Processing (NLP) constitutes the core of language-centric applications, ranging from sentiment analysis to language translation. A thesis in NLP could explore advanced language models, sentiment analysis techniques, or the ethical implications of language processing in applications like virtual assistants.
Quantum Computing for Big Data Analytics: Bridging Classical and Quantum Realms:
The integration of quantum computing and big data analytics presents transformative potential with profound implications for various industries. A thesis in this domain could explore quantum algorithms for data analysis, assess the scalability of quantum computing in handling massive datasets, or investigate hybrid models that leverage both classical and quantum computing resources.
Scalable Architectures for Parallel Data Processing: Navigating the Data Deluge:
As data volumes grow exponentially, scalable architectures are essential for efficient data processing. A thesis in scalable architectures could explore distributed computing frameworks, assess the performance of parallel processing in handling diverse data types, or propose innovative solutions for real-time data processing.
Sentiment Analysis: Deciphering Emotions in the Digital Era:
Understanding public sentiment is vital in various domains, from marketing to politics. A thesis in sentiment analysis could delve into advanced sentiment classification models, explore cross-cultural sentiment variations, or investigate the impact of sentiment analysis on decision-making processes.
Conclusion:
The field of data science in 2024 is characterized by a convergence of cutting-edge technologies and the imperative to address real-world challenges. The ten compelling thesis topics outlined above offer students the opportunity to embark on a journey of exploration and innovation. Whether unravelling the intricacies of deep learning, combating misinformation, or navigating the vast landscape of data visualization, each topic represents a gateway to making a meaningful contribution to the ever-evolving field of data science. As students embark on their thesis endeavors, these topics provide a roadmap to the pinnacle of data science in 2024.

Disclaimer: Any financial and crypto market information given on Analytics Insight are sponsored articles, written for informational purpose only and is not an investment advice. The readers are further advised that Crypto products and NFTs are unregulated and can be highly risky. There may be no regulatory recourse for any loss from such transactions. Conduct your own research by contacting financial experts before making any investment decisions. The decision to read hereinafter is purely a matter of choice and shall be construed as an express undertaking/guarantee in favour of Analytics Insight of being absolved from any/ all potential legal action, or enforceable claims. We do not represent nor own any cryptocurrency, any complaints, abuse or concerns with regards to the information provided shall be immediately informed here .
You May Also Like

Ready For The Second Leg Of Atletico Mineiro vs. Millonarios? Get The Best Odds With ONWIN, Bet365, and Sportingbet

10 Database Admin Jobs to Apply for In Sep 2023

Litecoin (LTC) and Avalanche (AVAX) investors Are Eyeing Yachtify (YCHT) As The Next High-Growth Project

Top 10 Front-Running AI Consulting Firms to Know in 2023

Analytics Insight® is an influential platform dedicated to insights, trends, and opinion from the world of data-driven technologies. It monitors developments, recognition, and achievements made by Artificial Intelligence, Big Data and Analytics companies across the globe.

- Select Language:
- Privacy Policy
- Content Licensing
- Terms & Conditions
- Submit an Interview
Special Editions
- Dec – Crypto Weekly Vol-1
- 40 Under 40 Innovators
- Women In Technology
- Market Reports
- AI Glossary
- Infographics
Latest Issue

Disclaimer: Any financial and crypto market information given on Analytics Insight is written for informational purpose only and is not an investment advice. Conduct your own research by contacting financial experts before making any investment decisions, more information here .
Second Menu


A List of 20 Data Science Dissertation Topics for 2023
Looking for intriguing data science dissertation topics for 2023? Look no further! This comprehensive article presents a list of 20 trending data science dissertation topics that will spark your interest and set you on the path of cutting-edge research.
Data Science Dissertation Topics for 2023
The handout provides a curated list of 20 data science dissertation topics for students in 2023. It emphasizes the importance of selecting a research area aligned with personal interests and addressing current trends and challenges in the field. The topics cover machine learning, data mining, artificial intelligence, and big data analytics. Each topic is accompanied by a brief description, offering insights into potential research directions. The handout advises students to conduct thorough background research, consult with advisors, and consider feasibility, relevance, and novelty when choosing a topic. It serves as a valuable resource, enabling students to contribute to advancements in the field. By choosing one of these trending topics, students can embark on a meaningful research journey in data science, exploring the fascinating possibilities and making significant contributions. Covering a wide range of areas such as machine learning, data mining, and predictive analytics, these topics offer abundant opportunities for groundbreaking research. Discover the exciting possibilities and make your mark in the world of data science with these trending dissertation topics for 2023.
Are you ready to dive into the world of data science and explore its vast potential? If you’re pursuing a dissertation in data science, you’re in luck! In this handout, we’ve compiled a list of 20 trending data science dissertation topics for 2023. These topics cover a wide range of fascinating areas within data science, offering you ample opportunities to explore and contribute to this rapidly evolving field. Whether you’re interested in machine learning, big data analytics, or natural language processing, you’ll find exciting and innovative topics to ignite your research journey. So, let’s delve into the list and discover the trending data science topics that can shape the future of the field in 2023.
Trending Data Science Dissertation Topics for 2023:
- Deep Learning for Image Recognition: Exploiting advanced deep learning techniques to enhance image recognition accuracy and efficiency.
- Natural Language Processing for Sentiment Analysis: Leveraging NLP algorithms to extract sentiment and emotions from textual data.
- Predictive Analytics for Customer Segmentation: Using predictive models to identify distinct customer segments for targeted marketing strategies.
- Anomaly Detection in Time Series Data: Developing novel algorithms to detect anomalies and outliers in time-dependent data.
- Explainable Artificial Intelligence: Investigating interpretable models to provide transparent explanations for AI-based decisions.
- Data Privacy and Ethics in Machine Learning: Examining ethical implications and privacy concerns associated with machine learning algorithms and data collection.
- Intelligent Fraud Detection Systems: Designing intelligent systems to detect and prevent fraudulent activities in financial transactions.
- Social Network Analysis for Influence Detection: Analyzing social networks to identify influential individuals and their impact on information diffusion.
- Machine Learning for Healthcare Diagnostics: Applying machine learning techniques to enhance disease diagnosis and prediction accuracy.
- Data Visualization for Exploratory Data Analysis: Developing interactive visualizations to aid in the exploration and understanding of complex datasets.
- Automated Machine Learning: Exploring techniques that automate the process of feature engineering, algorithm selection, and hyperparameter tuning.
- Cybersecurity Analytics: Investigating data-driven approaches for identifying and mitigating cyber threats in real-time.
- Recommender Systems for Personalized Recommendations: Developing intelligent recommendation algorithms to provide personalized suggestions to users.
- Blockchain Technology for Secure Data Sharing: Examining the use of blockchain technology to ensure secure and tamper-proof data sharing.
- Machine Learning for Fraud Detection in E-commerce: Developing machine learning models to detect fraudulent activities in online transactions.
- Fairness in Machine Learning: Addressing bias and fairness issues in machine learning algorithms to ensure equitable decision-making.
- Optimization Techniques in Data Science: Exploring optimization methods to improve the efficiency and performance of data science algorithms.
- Data Mining for Social Media Analysis: Extracting valuable insights from social media data to understand user behavior and trends.
- Deep Reinforcement Learning for Autonomous Systems: Applying deep reinforcement learning techniques to enable autonomous decision-making in complex environments.
- Machine Learning for Climate Change Prediction: Investigating machine learning models to predict and analyze the impact of climate change.
With the ever-increasing importance of data science in various domains, the demand for groundbreaking research in this field continues to grow. These 20 trending data science dissertation topics for 2023 offer an exciting opportunity to contribute to the advancement of data science and make a significant impact. Whether you’re interested in developing innovative algorithms, addressing ethical concerns, or applying data science in specific domains, these topics provide a solid foundation for your research journey. So, choose a topic that aligns with your interests and embark on an exciting exploration of data science’s endless possibilities in 2023.
Short On Time? Get Help With Your Dissertation Today!
Let's talk on whatsapp.
+1 (919) 904-4950
Send Us An Email
All Over Social Media
@dissertationmasterclass
Get To The Finish Line!
Are you working on your dissertation, thesis or research project? Our consultants will be delighted to find out more about your project and objectives and to talk about how we can help you succeed.
M.Tech/Ph.D Thesis Help in Chandigarh | Thesis Guidance in Chandigarh
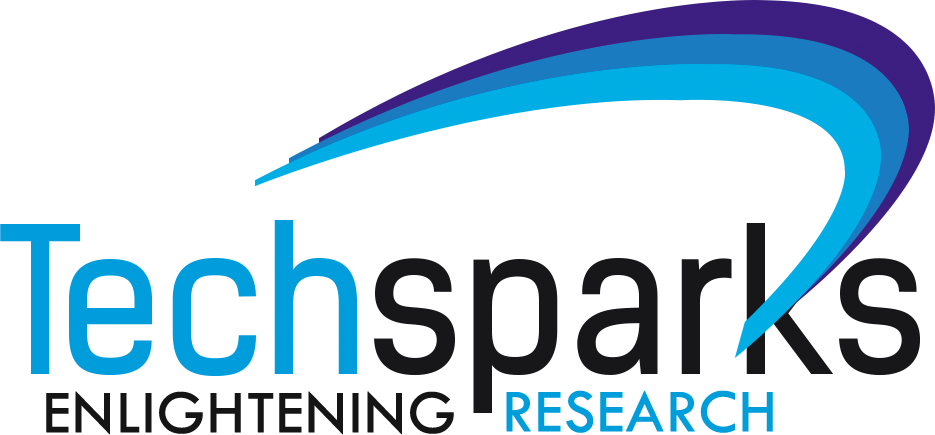

+91-9465330425

These days the internet is being widely used than it was used a few years back. It has become a core part of our life. Billions of people are using social media and social networking every day all across the globe. Such a huge number of people generate a flood of data which have become quite complex to manage. Considering this enormous data, a term has been coined to represent it. So, what is this term called? Yes, Big Data Big Data is the term coined to refer to this huge amount of data. The concept of big data is fast spreading its arms all over the world. It is a trending topic for thesis, project, research, and dissertation. There are various good topics for the master’s thesis and research in Big Data and Hadoop as well as for Ph.D. First of all know, what is big data and Hadoop?
Find the link at the end to download the latest thesis and research topics in Big Data
What is Big Data?
Big Data refers to the large volume of data which may be structured or unstructured and which make use of certain new technologies and techniques to handle it. An organized form of data is known as structured data while an unorganized form of data is known as unstructured data. The data sets in big data are so large and complex that we cannot handle them using traditional application software. There are certain frameworks like Hadoop designed for processing big data. These techniques are also used to extract useful insights from data using predictive analysis, user behavior, and analytics. You can explore more on big data introduction while working on the thesis in Big Data. Big Data is defined by three Vs:
Volume – It refers to the amount of data that is generated. The data can be low-density, high volume, structured/unstructured or data with unknown value. This unknown data is converted into useful one using technologies like Hadoop. The data can range from terabytes to petabytes. Velocity – It refers to the rate at which the data is generated. The data is received at an unprecedented speed and is acted upon in a timely manner. It also requires real-time evaluation and action in case of the Internet of Things(IoT) applications. Variety – Variety refers to different formats of data. It may be structured, unstructured or semi-structured. The data can be audio, video, text or email. In this additional processing is required to derive the meaning of data and also to support the metadata. In addition to these three Vs of data, following Vs are also defined in big data. Value – Each form of data has some value which needs to be discovered. There are certain qualitative and quantitative techniques to derive meaning from data. For deriving value from data, certain new discoveries and techniques are required. Variability – Another dimension for big data is the variability of data i.e the flow of data can be high or low. There are challenges in managing this flow of data.
Thesis Research Topics in Big Data
- Privacy, Security Issues in Big Data .
- Storage Systems of Scalable for Big Data .
- Massive Big Data Processing of Software and Tools.
- Techniques and Data Mining Tools for Big Data .
- Big Data Adoptation and Analytics of Cloud Computing Platforms.
- Scalable Architectures for Parallel Data Processing.
Can you imagine how big is big data? Of course, you can’t. The amount of big data that is generated and stored on a global scale is unbelievable and is growing day by day. But do you know, only a small portion of this data is actually analyzed mainly for getting useful insights and information?
Big Data Hadoop
Hadoop is an open-source framework provided to process and store big data. Hadoop makes use of simple programming models to process big data in a distributed environment across clusters of computers. Hadoop provides storage for a large volume of data along with advanced processing power. It also gives the ability to handle multiple tasks and jobs.
Big Data Hadoop Architecture
HDFS is the main component of Hadoop architecture. It stands for Hadoop Distributed File Systems. It is used to store a large amount of data and multiple machines are used for this storage. MapReduce Overview is another component of big data architecture. The data is processed here in a distributed manner across multiple machines. YARN component is used for data processing resources like CPU, RAM, and memory. Resource Manager and Node Manager are the elements of YARN. These two elements work as master and slave. Resource Manager is the master and assigns resources to the slave i.e. Node Manager. Node Manager sends the signal to the master when it is going to start the work. Big Data Hadoop for the thesis will be plus point for you.

Importance of Hadoop in big data
Hadoop is essential especially in terms of big data . The importance of Hadoop is highlighted in the following points: Processing of huge chunks of data – With Hadoop, we can process and store huge amount of data mainly the data from social media and IoT(Internet of Things) applications. Computation power – The computation power of Hadoop is high as it can process big data pretty fast. Hadoop makes use of distributed models for processing of data. Fault tolerance – Hadoop provide protection against any form of malware as well as from hardware failure. If a node in the distributed model goes down, then other nodes continue to function. Copies of data are also stored. Flexibility – As much data as you require can be stored using Hadoop. There is no requirement of preprocessing the data. Low Cost – Hadoop is an open-source framework and free to use. It provides additional hardware to store the large quantities of data. Scalability – The system can be grown easily just by adding nodes in the system according to the requirements. Minimal administration is required.
Challenges of Hadoop
No doubt Hadoop is a very good platform for big data solution, still, there are certain challenges in this.
These challenges are:
- All problems cannot be solved – It is not suitable for iteration and interaction tasks. Instead, it is efficient for simple problems for which division into independent units can be made.
- Talent Gap – There is a lack of talented and skilled programmers in the field of MapReduce in big data especially at entry level.
- Security of data – Another challenge is the security of data. Kerberos authentication protocol has been developed to provide a solution to data security issues.
- Lack of tools – There is a lack of tools for data cleaning, management, and governance. Tools for data quality and standardization are also lacking.
Fields under Big Data
Big Data is a vast field and there are a number of topics and fields under it on which you can work for your thesis, dissertation as well as for research. Big Data is just an umbrella term for these fields.
Search Engine Data – It refers to the data stored in the search engines like Google, Bing and is retrieved from different databases. Social Media Data – It is a collection of data from social media platforms like Facebook, Twitter. Stock Exchange Data – It is a data from companies indulged into shares business in the stock market. Black box Data – Black Box is a component of airplanes, helicopters for voice recording of fight crew and for other metrics.
Big Data Technologies
Big Data technologies are required for more detailed analysis, accuracy and concrete decision making. It will lead to more efficiency, less cost, and less risk. For this, a powerful infrastructure is required to manage and process huge volumes of data.
The data can be analyzed with techniques like A/B Testing, Machine Learning, and Natural Language Processing.
The big data technologies include business intelligence, cloud computing, and databases.
The visualization of data can be done through the medium of charts and graphs.
Multi-dimensional big data can be handled through tensor-based computation. Tensor-based computation makes use of linear relations in the form of scalars and vectors. Other technologies that can be applied to big data are:
Massively Parallel Processing Search based applications Data Mining Distributed databases Cloud Computing
These technologies are provided by vendors like Amazon, Microsoft, IBM etc to manage the big data.
MapReduce Algorithm for Big Data
A large amount of data cannot be processed using traditional data processing approaches. This problem has been solved by Google using an algorithm known as the MapReduce algorithm. Using this algorithm, the task can be divided into small parts and these parts are assigned to distributed computers connected on the network. The data is then collected from individual computers to form a final dataset.
The MapReduce algorithm is used by Hadoop to run applications in which parallel processing of data is done on different nodes. Hadoop framework can develop applications that can run on clusters of computers to perform statistical analysis of a large amount of data.
The MapReduce algorithm consist of two tasks: Map Reduce
A set is of data is taken by Map which is converted into another set of data in which individual elements are broken into pairs known as tuples. Reduce takes the output of Map task as input. It combines data tuples into smaller tuples set.
The MapReduce algorithm is executed in three stages: Map Shuffle Reduce
In the map stage, the input data is processed and stored in the Hadoop file system(HDFS). After this a mapper performs the processing of data to create small chunks of data. Shuffle stage and Reduce stage occur in combination. The Reducer takes the input from the mapper for processing to create a new set of output which will later be stored in the HDFS. The Map and Reduce tasks are assigned to appropriate servers in the cluster by the Hadoop. The Hadoop framework manages all the details like issuing of tasks, verification, and copying. After completion, the data is collected at the Hadoop server. You can get thesis and dissertation guidance for the thesis in Big Data Hadoop from data analyst.
Applications of Big Data
Big Data find its application in various areas including retail, finance, digital media, healthcare, customer services etc.
Big Data is used within governmental services with efficiency in cost, productivity, and innovation. The common example of this is the Indian Elections of 2014 in which BJP tried this to win the elections. The data analysis, in this case, can be done by the collaboration between the local and the central government. Big Data was the major factor behind Barack Obama’s win in the 2012 election campaign.
Big Data is used in finance for market prediction. It is used for compliance and regulatory reporting, risk analysis, fraud detection, high-speed trading and for analytics. The data which is used for market prediction is known as alternate data.
Big Data is used in health care services for clinical data analysis, disease pattern analysis, medical devices and medicines supply, drug discovery and various other such analytics. Big Data analytics have helped in a major way in improving the healthcare systems. Using these certain technologies have been developed in healthcare systems like eHealth, mHealth, and wearable health gadgets.
Media uses Big Data for various mechanisms like ad targeting, forecasting, clickstream analytics, campaign management and loyalty programs. It is mainly focused on following three points:
Targeting consumers Capturing of data Data journalism
Big Data is a core of IoT(Internet of Things) . They both work together. Data can be extracted from IoT devices for mapping which helps in interconnectivity. This mapping can be used to target customers and for media efficiency by the media industry.
Information Technology
Big Data has helped employees working in Information Technology to work efficiently and for widespread distribution of Information Technology. Certain issues in Information Technology can also be resolved using Big Data. Big Data principles can be applied to machine learning and artificial intelligence for providing better solutions to the problems.
Advantages of Big Data
Big Data has certain advantages and benefits, particularly for big organizations.
- Time Management – Big data saves valuable time as rather than spending hours on managing the different amount of data, big data can be managed efficiently and at a faster pace.
- Accessibility – Big Data is easily accessible through authorization and data access rights and privileges.
- Trustworthy – Big Data is trustworthy in the sense that we can get valuable insights from the data.
- Relevant – The data is relevant whereas irrelevant data require filtering which can lead to complexity.
- Secure – The data is secured using data hosting and through various advanced technologies and techniques.
Challenges of Big Data
Although Big Data has come in a big way in improving the way we store data, there are certain challenges which need to be resolved.
- Data Storage and quality of Data – The data is growing at a fast pace as the number of companies and organizations are growing. Proper storage of this data has become a challenge. This data can be stored in data warehouses but this data is inconsistent. There are issues of errors, duplicacy, conflicts while storing this data in their native format. Moreover, this changes the quality of data.
- Lack of big data analysts – There is a huge demand for data scientists and analysts who can understand and analyze this data. But there are very few people who can work in this field considering the fact that huge amount of data is produced every day. Those who are there don’t have proper skills.
- Quality Analysis – Big companies and organizations use big for getting useful insights to make proper decisions for future plans. The data should also be accurate as inaccurate data can lead to wrong decisions that will affect the company business. Therefore quality analysis of the data should be there. For this testing is required which is a time-consuming process and also make use of expensive tools.
- Security and Privacy of Data – Security, and privacy are the biggest risks in big data. The tools that are used for analyzing, storing, managing use data from different sources. This makes data vulnerable to exposure. It increases security and privacy concerns.
Thus Big Data is providing a great help to companies and organizations to make better decisions. This will ultimately lead to more profit. The main thesis topics in Big Data and Hadoop include applications, architecture, Big Data in IoT, MapReduce, Big Data Maturity Model etc.
Latest Thesis and Research Topics in Big Data
There are a various thesis and research topics in big data for M.Tech and Ph.D. Following is the list of good topics for big data for masters thesis and research:
Big Data Virtualization
Internet of Things(IoT)
Big Data Maturity Model
Data Science
Data Federation
Big Data Analytics
SQL-on-Hadoop
Predictive Analytics
Big Data Virtualization is the process of creating virtual structures rather than actual for Big Data systems. It is very beneficial for big enterprises and organizations to use their data assets to achieve their goals and objectives. Virtualization tools are available to handle big data analytics.
Big Data and IoT work in coexistence with each other. IoT devices capture data which is extracted for connectivity of devices. IoT devices have sensors to sense data from its surroundings and can act according to its surrounding environment.
Big Data Maturity Models are used to measure the maturity of big data. These models help organizations to measure big data capabilities and also assist them to create a structure around that data. The main goal of these models is to guide organizations to set their development goals.
Data Science is more or less related to Data Mining in which valuable insights and information are extracted from data both structured and unstructured. Data Science employs techniques and methods from the fields of mathematics, statistics, and computer science for processing.
Data Federation is the process of collecting data from different databases without copying and without transferring the original data. Rather than whole information, data federation collects metadata which is the description of the structure of original data and keep them in a single database.
Sampling is a technique of statistics to find and locate patterns in Big Data. Sampling makes it possible for the data scientists to work efficiently with a manageable amount of data. Sampled data can be used for predictive analytics. Data can be represented accurately when a large sample of data is used.
It is the process of exploring large datasets for the sake of finding hidden patterns and underlying relations for valuable customer insights and other useful information. It finds its application in various areas like finance, customer services etc. It is a good choice for Ph.D. research in big data analytics.
Clustering is a technique to analyze big data. In clustering, a group of similar objects is grouped together according to their similarities and characteristics. In other words, this technique partitions the data into different sets. The partitioning can be hard partitioning and soft partitioning. There are various algorithms designed for big data and data mining. It is a good area for thesis and researh in big data.
SQL-on-Hadoop is a methodology for implementing SQL on Hadoop platform by combining together the SQL-style querying system to the new components of the Hadoop framework. There are various ways to execute SQL in Hadoop environment which include – connectors for translating the SQL into a MapReduce format, push down systems to execute SQL in Hadoop clusters, systems that distribute the SQL work between MapReduce – HDFS clusters and raw HDFS clusters. It is a very good topic for thesis and research in Big Data.
It is a technique of extracting information from the datasets that already exist in order to find out the patterns and estimate future trends. Predictive Analytics is the practical outcome of Big Data and Business Intelligence(BI). There are predictive analytics models which are used to get future insights. For this future insight, predictive analytics take into consideration both current and historical data. It is also an interesting topic for thesis and research in Big Data.
These were some of the good topics for big data for M.Tech and masters thesis and research work. For any help on thesis topics in Big Data, contact Techsparks . Call us on this number 91-9465330425 or email us at [email protected] for M.Tech and Ph.D. help in big data thesis topics.
Click on the following link to download the latest thesis and research topics in Big Data
Latest Thesis and Research Topics in Big Data(pdf)
Leave a Reply Cancel reply
Your email address will not be published. Required fields are marked *
Save my name, email, and website in this browser for the next time I comment.
Quick Enquiry
Get a quote, share your details to get free.
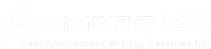
- Why Choose Us
- Vision and Mission
- Hire Writers
- How it Works
Latest Data Science Dissertation Topics to Grab Reader's Attention
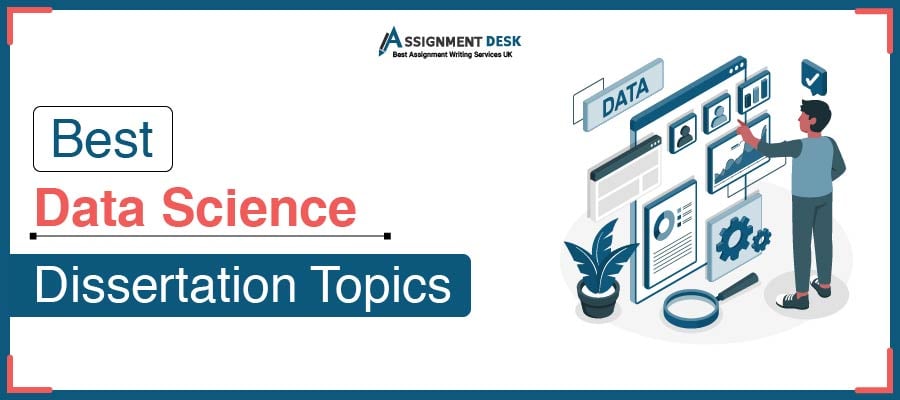
Table of Contents
What Is Data Science and Its Importance?
A list of latest data science dissertation topics, how to choose a data science dissertation topic, stuck with data science dissertation topics we can help.
Data science is one of the fastest growing fields in present times, which makes it one of the befitting subjects among students. But, getting a degree in the field is not a piece of cake, as you have to overcome several hurdles. One such problem is to draft an ideal dissertation. Although, creating a paper can be easier when you have a precise topic. Thus, this blog will help you to explore data science dissertation topics to ease your workload. So, to begin with, have an insight into what the data science field is and why it is necessary.
Need Personalised Assistance from Our Experts?
Share Your Requirements via Whatsapp!
Data science is a field that studies data to extract valuable insights for a business. In other words, it is a means to use scientific techniques to evaluate and extract meaningful information from the ocean of data. Moreover, as per professional data science dissertation writers, it is an interdisciplinary approach that combines practices and principles of several fields. These subjects are mathematics, statistics, computer engineering, and artificial intelligence. However, you can learn all these in the dissertation writing process, which is a crucial thing in academics. Furthermore, its importance and use is increasing day by day in the field to:
Explore Transformative Patterns
Data science enables a business to explore new relationships and patterns that have the ability to transform the organisation and take it to new heights. Moreover, it can evaluate the low cost of resource management to get higher profits.
Innovation of New Products
Data science has the capability to reveal the gaps and the problems present the existing information that might go unnoticed otherwise. You can do it by evaluating the purchase decisions, consumer preferences, business process and more.
After gaining insight into data science and perceiving its importance, it is time to move ahead. Constructing a dissertation while you are pursuing your academic journey is necessary. Although it is a challenging task, but referring to online dissertation help can guide you on the right track. To move forward, explore the topics you can use to frame your dissertation and impress your professor.
In this section of the blog, you will explore dissertation topics in data science that you can use to build your paper on. These are shortlisted by the experts that will help you leave an impression on your professor and grab your readers' attention. Thus, begin to perceive them all listed by the professional dissertation writers in UK :
Best Data Science Dissertation Topics
Here are the hand-picked dissertation topics for data science that can help you grab the reader's attention quickly and without too much effort.
1. Compare the implementation of data science in various investigations concerning wildfires.
2. Explain the K-means clustering from the perspective of online spherical.
3. Explore how linear and nonlinear regression analyses' efficacy can be increased.
4. Evaluate the platforms for big data computing: Big data analytics and the adoption.
5. Discuss the best data management strategies for modern enterprises to use.
Trending Data Science Dissertation Topics
As you know, trends are changing rapidly in every field, and you have to cope with them to grow. Thus, in this section, you will find some of the most trending data science dissertation ideas to adjust to the changing things.
6. Explain massive data processing and the appropriate key management system.
7. Discuss the deep learning process and its relevance in the field.
8. What is the application of big data in improving supply chain management of an institution?
9. Analyse the implementation of data science in economic theory.
10. What is the use of big data analytics to power AI and ML?
Need Help with Dissertation?
Get a 100% Original Dissertation Written by EXPERTS
Compelling Data Science Dissertation Topics
Attracting the readers and making them stick to the end of the document is the most challenging task. But, if you have chosen ideal MSC data science dissertation topics, you can ace it easily. Thus, here are some of them:
11. Explain the Hadoop programming and the map-reduce architecture.
12. What is hyper-personalisation and its importance in the field?
13. Explore the value big data provides to innovation management.
14. Perform a comparative study on the implementation of data science in the teaching profession.
15. Overview of data valuation and why it matters in data management.
Data Science Dissertation Topics to Score Well
The motive behind constructing a dissertation is to score well apart from studying the subject. Thus, to make the paper effective, you can either buy dissertation service or select a topic which has the potential to fetch you good grades. So, here are some of the appropriate data science dissertation ideas:
16. Have a discussion about the MATLAB code for decision trees along with semantic data governance.
17. What is the necessity of big data technologies for modern businesses?
18. State the societal implications of using predictive analytics within education.
19. Mention the association rule learning regarding data mining.
20. Give an overview of the relevance of Artificial Intelligence.
Struggling to Find Best Dissertation Topic?
Get a Unique Title & Dissertation Proposal Outline for FREE!
Unique Data Science Dissertation Topics
You must know that uniqueness is the key towards an ideal dissertation. Thus, in this section, you will explore the unique data science dissertation topics that will help you achieve your goal.
21. What is the implementation of data science, and how does it impact the management environment and sustainability?
22. How to apply attribute-access or role-based access control in an organisation?
These are some of the dissertation topics for data science that will help you ease the process of selecting the topic. So, move ahead to know the technique that you can implement and find the perfect data science research topics for your paper.
This section of the blow will help you plan your dissertation topic selection process to smoothen the path. So, read further to perceive the procedure that you should follow while selecting data science dissertation topics:
Pick the Meaningful Topic
As you know, there is a never-ending list of data science dissertation topics you can choose from and build your paper on. But you are opting for the appropriate one within your interest and trending simultaneously. However, if it is challenging for you, check examples of dissertation that can rescue you.
Work with Consistent Data
Due to the variety of data science dissertation topics available, you must choose the one with consistent data. It means some topics do not have an accurate amount of information available to research. So, to ensure that you do not get stuck in the middle, you must ensure that the theme you are opting for has a consistent flow of information.
Ease the Complexity of the Model
While finalising the data science dissertation topics, you need to ensure that it does not have a complex model to work with. It is so because, sometimes, for the sake of uniqueness, students go for the topic with complicated theories. Thus, it makes them struggle and confuses them while creating the paper. So, to ensure a smooth process, you must work on something with lower complexity.
Acknowledge the Day to Day Problems
While selecting a theme, you must keep yourself updated with the daily problems faced by the targeted audience. You can refer to the data science dissertation examples available to understand this better. It is crucial as it will grab the attention of the audience faster, and they can connect with it easily. To do this, enhance your knowledge in the field you are working in.
These were some easy steps that you should adhere to while selecting ideal dissertation topics for data science. So, if you are still struggling with the topics, you can seek professional help.
The data science dissertation topics listed in the blog are more than enough, and you must have found the one that perfectly fits your interest area. However, if you are still stuck with dissertation topic and want to explore more, our team of experts is there. Moreover, we can guide you with other challenging areas that might become a hurdle in your way. So, when you seek our data science dissertation help, you will get the following:
- 100% Unique Data
- On-Time Delivery
- 24/7 Assistance
- Affordable Prices
- Six Free Tools
- Flawless Content
It is not the end, as you can avail of several other benefits when you hire us to assist you. Moreover, seeking help from the experts at the Assignment Desk will not burn a hole in your pocket as the prices will fit into your budget.
Let Us Help With Dissertation
Share Your Requirements Now for Customized Solutions.
Delivered on-time or your money back
Our Services
- Assignment Writing Service
- Essay Writing Help
- Dissertation Writing Service
- Coursework Writing Service
- Proofreading & Editing Service
- Online Exam Help
- Term paper writing service
- Ghost Writing Service
- Case Study Writing Service
- Research Paper Writing Service
- Personal Statement Writing Service
- Resume Writing Service
- Report Writing Service
To Make Your Work Original
Check your work against paraphrasing & get a free Plagiarism report!
Check your work against plagiarism & get a free Plagiarism report!
Quick and Simple Tool to Generate Dissertation Outline Instantly
Get citations & references in your document in the desired style!
Make your content free of errors in just a few clicks for free!
Generate plagiarism-free essays as per your topic’s requirement!
Generate a Compelling Thesis Statement and Impress Your Professor
FREE Features
- Topic Creation USD 3.87 FREE
- Outline USD 9.33 FREE
- Unlimited Revisions USD 20.67 FREE
- Editing/Proofreading USD 28 FREE
- Formatting USD 8 FREE
- Bibliography USD 7.33 FREE
Get all these features for
USD 80.67 FREE
RELATED BLOGS
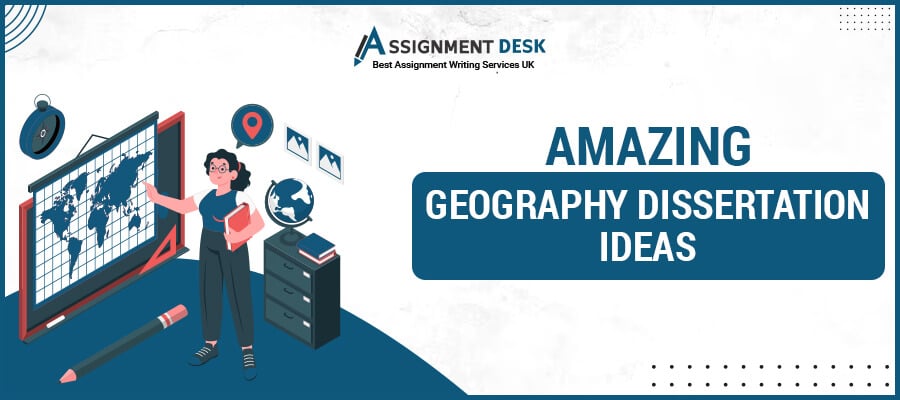
50+ In- Depth Geography Dissertation Ideas & Topics [2024]
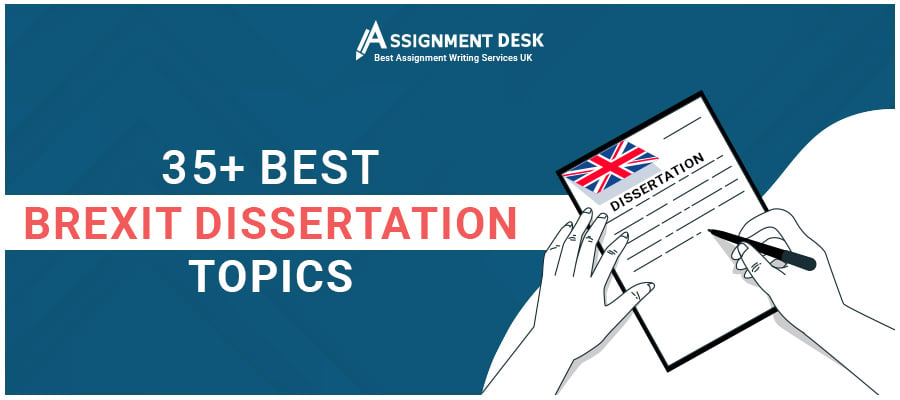
A Comprehensive List of 35+ Trending Brexit Dissertation Topics
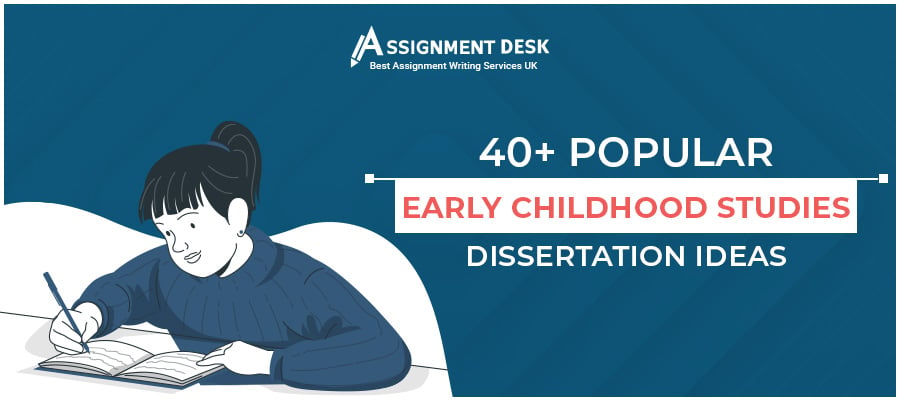
Interesting 40+ Early Childhood Studies Dissertation Ideas
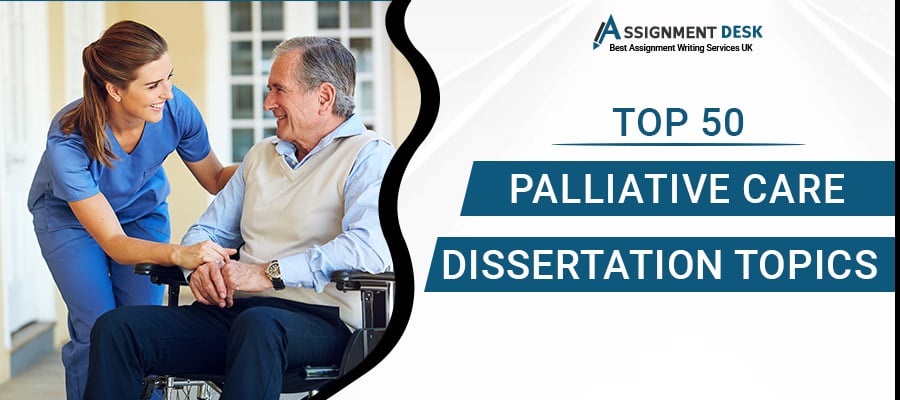
Top 50 Unique Topics for Writing Palliative Care Dissertation
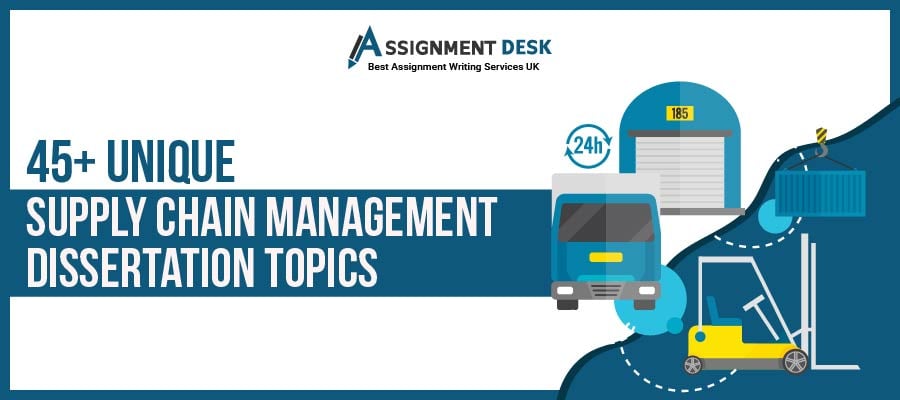
45+ Best Supply Chain Management Dissertation Topics 2024
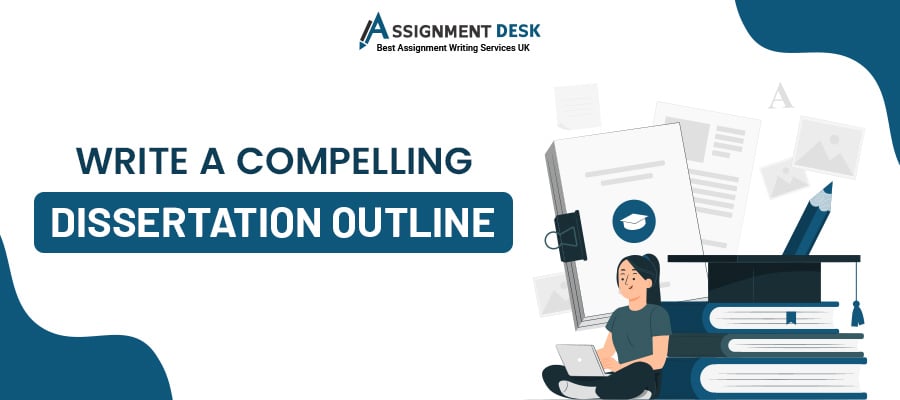
How to Write a Compelling Dissertation Outline?
Professional assignment writers.
Choose a writer for your task among hundreds of professionals
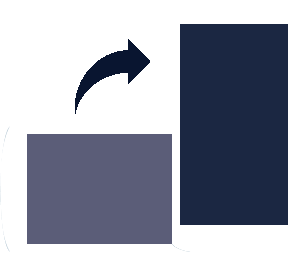
Please rotate your device
We don't support landscape mode yet. Please go back to portrait mode for the best experience
We use cookies to ensure that we give you the best experience on our website. If you continue to use this site we will assume that you are happy with it. Know more
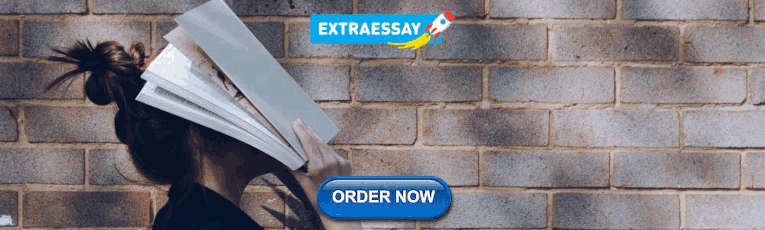
Calculate the Price
Professional Academic Help at Pocket-Friendly Prices!
Estimated Price
Limited Time Offer
Exclusive Library Membership + FREE Wallet Balance
1 Month Access !
5000 Student Samples
10,000 Answers by Experts
Get $300 Now
PhD Research Topics in Big Data Analytics
PhD research topics in Big Data Analytics is our superb research service for PhD/MS pupils. To be sure, it aids to “prove the creativity” in their engrossed field.
The only way to earn good things in research is “FACE CHALLENGES”…We guide you on overcoming hurdles…
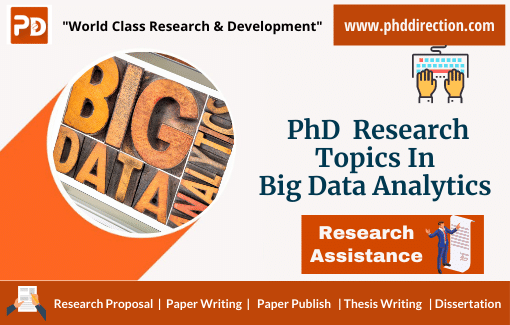
By all means, in recent studies, Big Data Analytics is gaining more fame. In fact, this happens due to its growing demand in today’s digital world. Reach us for exciting PhD research topics in big data analytics .
On this one hand, it is the best field to “manage” as well as “manipulate” data in all forms. The other service also covers vital challenges in big data under many aspects such as scientific work, sensors, and more .
Join us right now…Come with your dream and Go with your success…
Based, on the whole, we are elite in “42V’s” of big data as Value, Volume, Variety, and so on.
Major Real-Time Applications of Big Data Analytics
- Intelligent Traffic Control
- Smart City Surveillance
- Healthcare Monitoring
- Risk and also fraud Management
- Predictive Product Grading
In this, PhD research topics in Big Data Analytics are very much familiar to access also a various “online repositories of datasets”.
Few Online sources for Datasets
- FiveThirtyEight
- Socrata OpenData
- And aslo Github
As a matter of support for that, our experts will provide you a wide range of services to bring out the “top class” research. Last, of all, we are also good to give complete aid in all the phases of your R&D.
Main Phases of Your Research
- Domain Identification
- Research and also Issue Selection
- Finding Possible also Solutions
- Code Development
- And also Manuscript Writing
Don’t stop until you get succeed in your journey….
Here, we also suggest you brainy discoveries from our PhD research topics in Big Data Analytics,
Pedigree-ing: Distributed Data-Driven Big Data Privacy in Big Data
Using Big Data Assessing Data Provenance Theory-Based Quality of Data for on-line Checking and Maesuring Power Quality.
A Co-operative Semantic Services using an OWL Ontology in Big Data Environments
A Selection Method for Taking commercial hotspot investigationwith Weibo check-in data as an example in social media based big data analysis
A Effective Big Data Methodology for Large-Scale Data-Driven Financial Risk Developing
A Scalable Data System in Automotive Big Data Marketplaces for AutoMat CVIM
A Spark-Based Internet-of-Vehicles in Big Data Analytics System
A Hadoop-Based Runtimes of Mathematical Systems using Big Data
A Review on user behavior-based information recommendation of scientific and technological achievements in big data mining
Using Big Data propose DCT with its Application based algorithm for Adaptive Blind Watermarking
Developing and Evaluating of Material Supply Network based on Traffic-Big Data Pack
An Effects of fare reduction policy based on urban public transport sharing rate in for public transportation using big data analysis
Developing a Predictive System for Joint Strike Fighter in Big Data Analytics
A Spatio-Temporal–Based College Students` Deep Entrepreneurship Patterns Analysis in Flow of Big Data
An Open one-side uncertain probability simulation for Fusion System of uncertain data and uncertain data relation
An Effective Unstructured Data using Quality Assessment System of Big Data
An Analysis of Big Data Oriented Application in Urban Intelligent Transportation Model
A Combination of Semantic Data for Intelligent, Value-Driven Big Data Analytics
A Multi-Domain Optical Networks using an Optical Network Management Information Combination and Fusion
An Analysis of Land-Use Degree and Spatial Autocorrelation using Kunming City
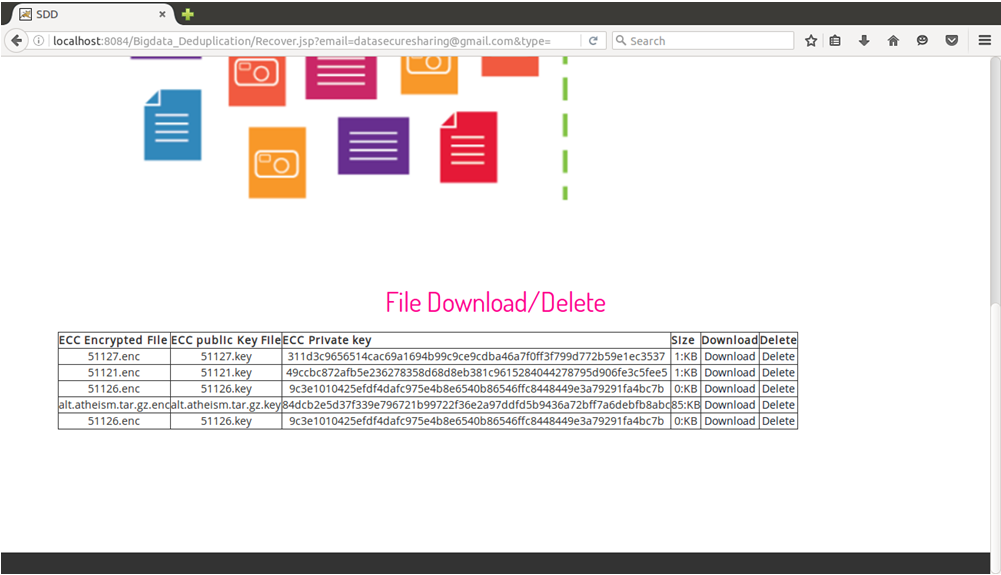
Why Work With Us ?
Senior research member, research experience, journal member, book publisher, research ethics, business ethics, valid references, explanations, paper publication, 9 big reasons to select us.
Our Editor-in-Chief has Website Ownership who control and deliver all aspects of PhD Direction to scholars and students and also keep the look to fully manage all our clients.
Our world-class certified experts have 18+years of experience in Research & Development programs (Industrial Research) who absolutely immersed as many scholars as possible in developing strong PhD research projects.
We associated with 200+reputed SCI and SCOPUS indexed journals (SJR ranking) for getting research work to be published in standard journals (Your first-choice journal).
PhDdirection.com is world’s largest book publishing platform that predominantly work subject-wise categories for scholars/students to assist their books writing and takes out into the University Library.
Our researchers provide required research ethics such as Confidentiality & Privacy, Novelty (valuable research), Plagiarism-Free, and Timely Delivery. Our customers have freedom to examine their current specific research activities.
Our organization take into consideration of customer satisfaction, online, offline support and professional works deliver since these are the actual inspiring business factors.
Solid works delivering by young qualified global research team. "References" is the key to evaluating works easier because we carefully assess scholars findings.
Detailed Videos, Readme files, Screenshots are provided for all research projects. We provide Teamviewer support and other online channels for project explanation.
Worthy journal publication is our main thing like IEEE, ACM, Springer, IET, Elsevier, etc. We substantially reduces scholars burden in publication side. We carry scholars from initial submission to final acceptance.
Related Pages
Phd Research Topics In Cse
Phd Research Topics In Contiki Os
Phd Research Topics In Contiki Coap
Phd Research Topics In Contiki Mqtt
Phd Research Topics In Contikios
Phd Research Topics In Cryptography
Phd Research Topics In Contiki 6lowpan
Phd Research Topics In Cybersecurity
Phd Research Topics In Cooja Simulator
Phd Research Topics In Context Aware Computing
Phd Research Topics In Controller Area Network
Phd Research Topics In Constant Bitrate Network
Phd Research Topics In Dependable Secure Computing
Phd Research Topics In Contiki Cooja Simulator
Phd Research Topics In Dependable And Secure Computing
Our Benefits
Throughout reference, confidential agreement, research no way resale, plagiarism-free, publication guarantee, customize support, fair revisions, business professionalism, domains & tools, we generally use, wireless communication (4g lte, and 5g), ad hoc networks (vanet, manet, etc.), wireless sensor networks, software defined networks, network security, internet of things (mqtt, coap), internet of vehicles, cloud computing, fog computing, edge computing, mobile computing, mobile cloud computing, ubiquitous computing, digital image processing, medical image processing, pattern analysis and machine intelligence, geoscience and remote sensing, big data analytics, data mining, power electronics, web of things, digital forensics, natural language processing, automation systems, artificial intelligence, mininet 2.1.0, matlab (r2018b/r2019a), matlab and simulink, apache hadoop, apache spark mlib, apache mahout, apache flink, apache storm, apache cassandra, pig and hive, rapid miner, support 24/7, call us @ any time, +91 9444829042, [email protected].
Questions ?
Click here to chat with us
25+ Solved End-to-End Big Data Projects with Source Code
Solved End-to-End Real World Mini Big Data Projects Ideas with Source Code For Beginners and Students to master big data tools like Hadoop and Spark.
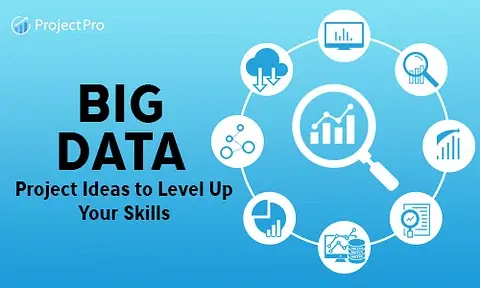
Ace your big data analytics interview by adding some unique and exciting Big Data projects to your portfolio. This blog lists over 20 big data analytics projects you can work on to showcase your big data skills and gain hands-on experience in big data tools and technologies. You will find several big data projects depending on your level of expertise- big data projects for students, big data projects for beginners, etc.
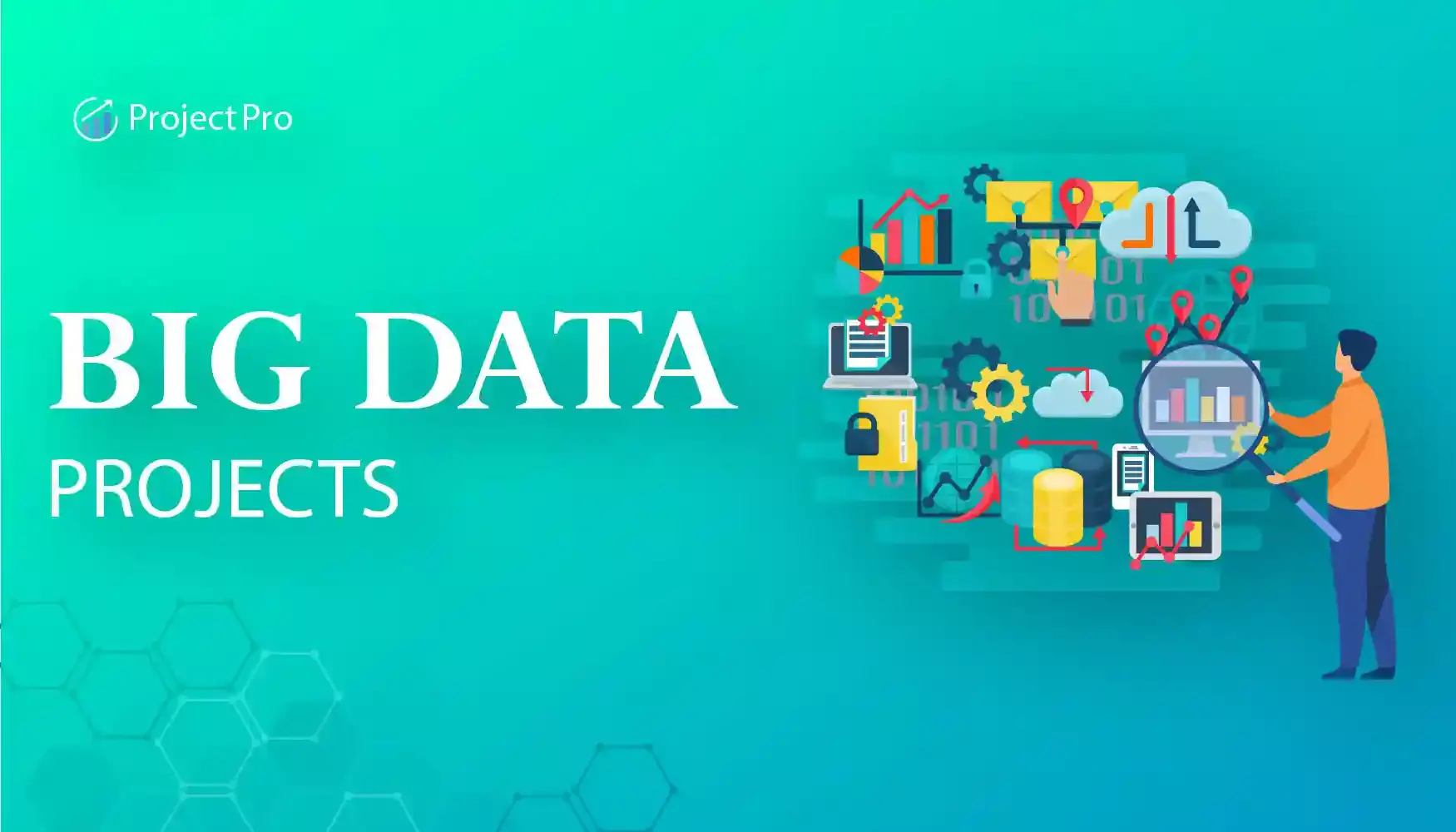
Build a big data pipeline with AWS Quicksight, Druid, and Hive
Downloadable solution code | Explanatory videos | Tech Support
Have you ever looked for sneakers on Amazon and seen advertisements for similar sneakers while searching the internet for the perfect cake recipe? Maybe you started using Instagram to search for some fitness videos, and now, Instagram keeps recommending videos from fitness influencers to you. And even if you’re not very active on social media, I’m sure you now and then check your phone before leaving the house to see what the traffic is like on your route to know how long it could take you to reach your destination. None of this would have been possible without the application of big data analysis process on by the modern data driven companies. We bring the top big data projects for 2023 that are specially curated for students, beginners, and anybody looking to get started with mastering data skills.
Table of Contents
What is a big data project, how do you create a good big data project, 25+ big data project ideas to help boost your resume , big data project ideas for beginners, intermediate projects on data analytics, advanced level examples of big data projects, real-time big data projects with source code, sample big data project ideas for final year students, big data project ideas using hadoop , big data projects using spark, gcp and aws big data projects, best big data project ideas for masters students, fun big data project ideas, top 5 apache big data projects, top big data projects on github with source code, level-up your big data expertise with projectpro's big data projects, faqs on big data projects.
A big data project is a data analysis project that uses machine learning algorithms and different data analytics techniques on structured and unstructured data for several purposes, including predictive modeling and other advanced analytics applications. Before actually working on any big data projects, data engineers must acquire proficient knowledge in the relevant areas, such as deep learning, machine learning, data visualization , data analytics, data science, etc.
Many platforms, like GitHub and ProjectPro, offer various big data projects for professionals at all skill levels- beginner, intermediate, and advanced. However, before moving on to a list of big data project ideas worth exploring and adding to your portfolio, let us first get a clear picture of what big data is and why everyone is interested in it.
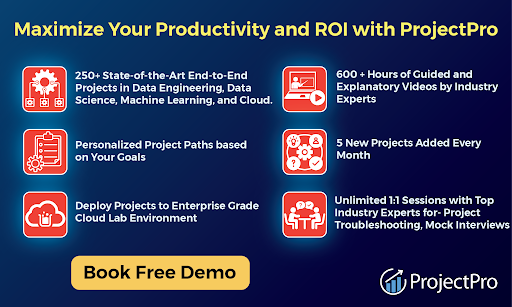
Kicking off a big data analytics project is always the most challenging part. You always encounter questions like what are the project goals, how can you become familiar with the dataset, what challenges are you trying to address, what are the necessary skills for this project, what metrics will you use to evaluate your model, etc.
Well! The first crucial step to launching your project initiative is to have a solid project plan. To build a big data project, you should always adhere to a clearly defined workflow. Before starting any big data project, it is essential to become familiar with the fundamental processes and steps involved, from gathering raw data to creating a machine learning model to its effective implementation.
Understand the Business Goals of the Big Data Project
The first step of any good big data analytics project is understanding the business or industry that you are working on. Go out and speak with the individuals whose processes you aim to transform with data before you even consider analyzing the data. Establish a timeline and specific key performance indicators afterward. Although planning and procedures can appear tedious, they are a crucial step to launching your data initiative! A definite purpose of what you want to do with data must be identified, such as a specific question to be answered, a data product to be built, etc., to provide motivation, direction, and purpose.
Here's what valued users are saying about ProjectPro
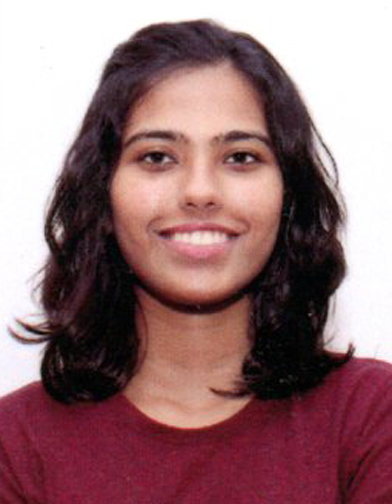
Savvy Sahai
Data Science Intern, Capgemini
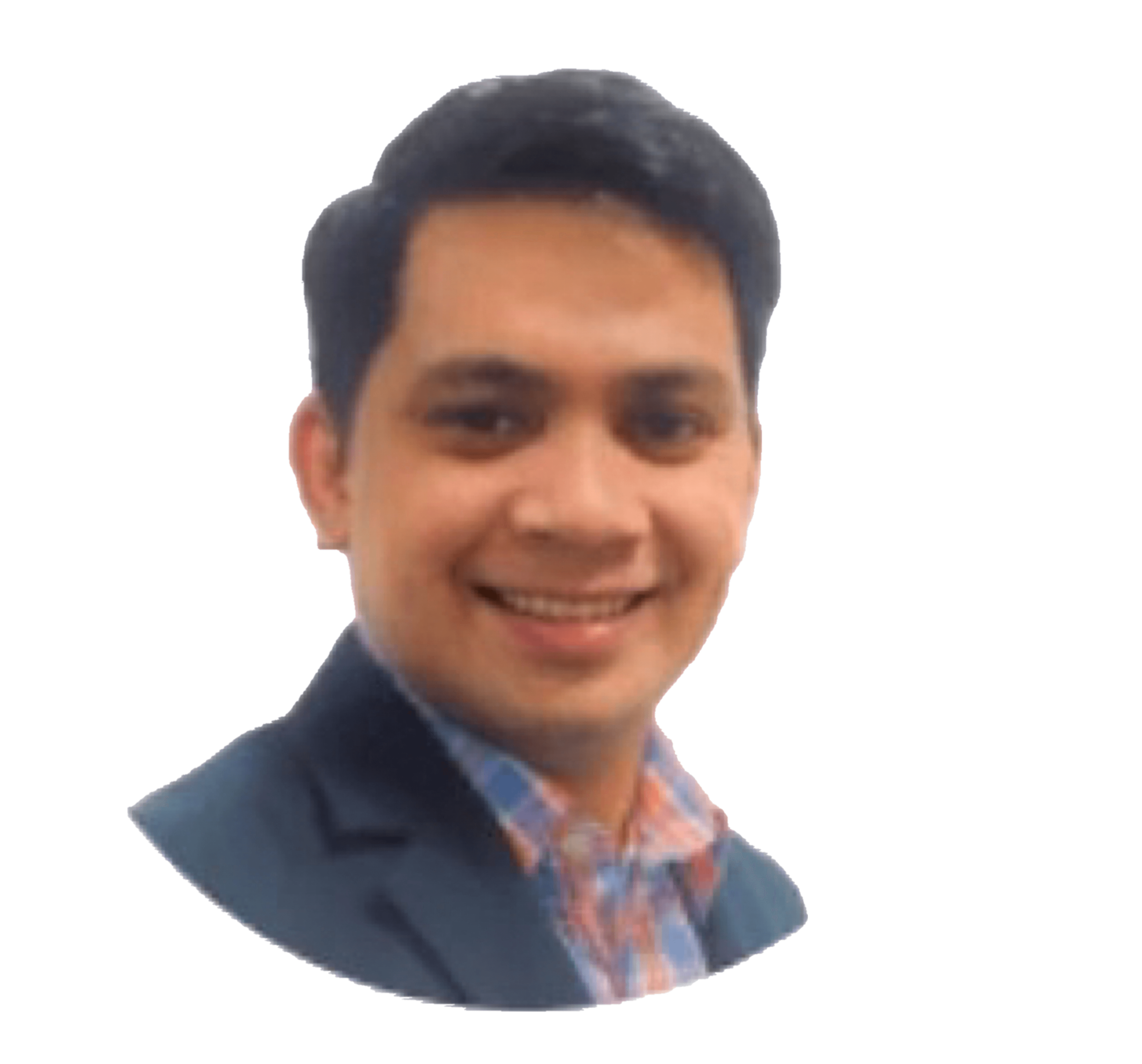
Director Data Analytics at EY / EY Tech
Not sure what you are looking for?
Collect Data for the Big Data Project
The next step in a big data project is looking for data once you've established your goal. To create a successful data project, collect and integrate data from as many different sources as possible.
Here are some options for collecting data that you can utilize:
Connect to an existing database that is already public or access your private database.
Consider the APIs for all the tools your organization has been utilizing and the data they have gathered. You must put in some effort to set up those APIs so that you can use the email open and click statistics, the support request someone sent, etc.
There are plenty of datasets on the Internet that can provide more information than what you already have. There are open data platforms in several regions (like data.gov in the U.S.). These open data sets are a fantastic resource if you're working on a personal project for fun.
Data Preparation and Cleaning
The data preparation step, which may consume up to 80% of the time allocated to any big data or data engineering project, comes next. Once you have the data, it's time to start using it. Start exploring what you have and how you can combine everything to meet the primary goal. To understand the relevance of all your data, start making notes on your initial analyses and ask significant questions to businesspeople, the IT team, or other groups. Data Cleaning is the next step. To ensure that data is consistent and accurate, you must review each column and check for errors, missing data values, etc.
Making sure that your project and your data are compatible with data privacy standards is a key aspect of data preparation that should not be overlooked. Personal data privacy and protection are becoming increasingly crucial, and you should prioritize them immediately as you embark on your big data journey. You must consolidate all your data initiatives, sources, and datasets into one location or platform to facilitate governance and carry out privacy-compliant projects.
New Projects
Data Transformation and Manipulation
Now that the data is clean, it's time to modify it so you can extract useful information. Starting with combining all of your various sources and group logs will help you focus your data on the most significant aspects. You can do this, for instance, by adding time-based attributes to your data, like:
Acquiring date-related elements (month, hour, day of the week, week of the year, etc.)
Calculating the variations between date-column values, etc.
Joining datasets is another way to improve data, which entails extracting columns from one dataset or tab and adding them to a reference dataset. This is a crucial component of any analysis, but it can become a challenge when you have many data sources.
Visualize Your Data
Now that you have a decent dataset (or perhaps several), it would be wise to begin analyzing it by creating beautiful dashboards, charts, or graphs. The next stage of any data analytics project should focus on visualization because it is the most excellent approach to analyzing and showcasing insights when working with massive amounts of data.
Another method for enhancing your dataset and creating more intriguing features is to use graphs. For instance, by plotting your data points on a map, you can discover that some geographic regions are more informative than some other nations or cities.
Build Predictive Models Using Machine Learning Algorithms
Machine learning algorithms can help you take your big data project to the next level by providing you with more details and making predictions about future trends. You can create models to find trends in the data that were not visible in graphs by working with clustering techniques (also known as unsupervised learning). These organize relevant outcomes into clusters and more or less explicitly state the characteristic that determines these outcomes.
Advanced data scientists can use supervised algorithms to predict future trends. They discover features that have influenced previous data patterns by reviewing historical data and can then generate predictions using these features.
Lastly, your predictive model needs to be operationalized for the project to be truly valuable. Deploying a machine learning model for adoption by all individuals within an organization is referred to as operationalization.
Repeat The Process
This is the last step in completing your big data project, and it's crucial to the whole data life cycle. One of the biggest mistakes individuals make when it comes to machine learning is assuming that once a model is created and implemented, it will always function normally. On the contrary, if models aren't updated with the latest data and regularly modified, their quality will deteriorate with time.
You need to accept that your model will never indeed be "complete" to accomplish your first data project effectively. You need to continually reevaluate, retrain it, and create new features for it to stay accurate and valuable.
If you are a newbie to Big Data, keep in mind that it is not an easy field, but at the same time, remember that nothing good in life comes easy; you have to work for it. The most helpful way of learning a skill is with some hands-on experience. Below is a list of Big Data analytics project ideas and an idea of the approach you could take to develop them; hoping that this could help you learn more about Big Data and even kick-start a career in Big Data.
Yelp Data Processing Using Spark And Hive Part 1
Yelp Data Processing using Spark and Hive Part 2
Hadoop Project for Beginners-SQL Analytics with Hive
Tough engineering choices with large datasets in Hive Part - 1
Finding Unique URL's using Hadoop Hive
AWS Project - Build an ETL Data Pipeline on AWS EMR Cluster
Orchestrate Redshift ETL using AWS Glue and Step Functions
Analyze Yelp Dataset with Spark & Parquet Format on Azure Databricks
Data Warehouse Design for E-commerce Environments
Analyzing Big Data with Twitter Sentiments using Spark Streaming
PySpark Tutorial - Learn to use Apache Spark with Python
Tough engineering choices with large datasets in Hive Part - 2
Event Data Analysis using AWS ELK Stack
Web Server Log Processing using Hadoop
Data processing with Spark SQL
Build a Time Series Analysis Dashboard with Spark and Grafana
GCP Data Ingestion with SQL using Google Cloud Dataflow
Deploying auto-reply Twitter handle with Kafka, Spark, and LSTM
Dealing with Slowly Changing Dimensions using Snowflake
Spark Project -Real-Time data collection and Spark Streaming Aggregation
Snowflake Real-Time Data Warehouse Project for Beginners-1
Real-Time Log Processing using Spark Streaming Architecture
Real-Time Auto Tracking with Spark-Redis
Building Real-Time AWS Log Analytics Solution
Explore real-world Apache Hadoop projects by ProjectPro and land your Big Data dream job today!
In this section, you will find a list of good big data project ideas for masters students.
Hadoop Project-Analysis of Yelp Dataset using Hadoop Hive
Online Hadoop Projects -Solving small file problem in Hadoop
Airline Dataset Analysis using Hadoop, Hive, Pig, and Impala
AWS Project-Website Monitoring using AWS Lambda and Aurora
Explore features of Spark SQL in practice on Spark 2.0
MovieLens Dataset Exploratory Analysis
Bitcoin Data Mining on AWS
Create A Data Pipeline Based On Messaging Using PySpark And Hive - Covid-19 Analysis
Spark Project-Analysis and Visualization on Yelp Dataset
Project Ideas on Big Data Analytics
Let us now begin with a more detailed list of good big data project ideas that you can easily implement.
This section will introduce you to a list of project ideas on big data that use Hadoop along with descriptions of how to implement them.
1. Visualizing Wikipedia Trends
Human brains tend to process visual data better than data in any other format. 90% of the information transmitted to the brain is visual, and the human brain can process an image in just 13 milliseconds. Wikipedia is a page that is accessed by people all around the world for research purposes, general information, and just to satisfy their occasional curiosity.
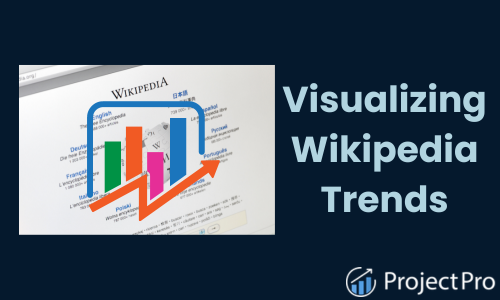
Raw page data counts from Wikipedia can be collected and processed via Hadoop. The processed data can then be visualized using Zeppelin notebooks to analyze trends that can be supported based on demographics or parameters. This is a good pick for someone looking to understand how big data analysis and visualization can be achieved through Big Data and also an excellent pick for an Apache Big Data project idea.
Visualizing Wikipedia Trends Big Data Project with Source Code .
2. Visualizing Website Clickstream Data
Clickstream data analysis refers to collecting, processing, and understanding all the web pages a particular user visits. This analysis benefits web page marketing, product management, and targeted advertisement. Since users tend to visit sites based on their requirements and interests, clickstream analysis can help to get an idea of what a user is looking for.
Visualization of the same helps in identifying these trends. In such a manner, advertisements can be generated specific to individuals. Ads on webpages provide a source of income for the webpage, and help the business publishing the ad reach the customer and at the same time, other internet users. This can be classified as a Big Data Apache project by using Hadoop to build it.
Big Data Analytics Projects Solution for Visualization of Clickstream Data on a Website
3. Web Server Log Processing
A web server log maintains a list of page requests and activities it has performed. Storing, processing, and mining the data on web servers can be done to analyze the data further. In this manner, webpage ads can be determined, and SEO (Search engine optimization) can also be done. A general overall user experience can be achieved through web-server log analysis. This kind of processing benefits any business that heavily relies on its website for revenue generation or to reach out to its customers. The Apache Hadoop open source big data project ecosystem with tools such as Pig, Impala, Hive, Spark, Kafka Oozie, and HDFS can be used for storage and processing.
Big Data Project using Hadoop with Source Code for Web Server Log Processing
This section will provide you with a list of projects that utilize Apache Spark for their implementation.
4. Analysis of Twitter Sentiments Using Spark Streaming
Sentimental analysis is another interesting big data project topic that deals with the process of determining whether a given opinion is positive, negative, or neutral. For a business, knowing the sentiments or the reaction of a group of people to a new product launch or a new event can help determine the profitability of the product and can help the business to have a more extensive reach by getting an idea of the feel of the customers. From a political standpoint, the sentiments of the crowd toward a candidate or some decision taken by a party can help determine what keeps a specific group of people happy and satisfied. You can use Twitter sentiments to predict election results as well.
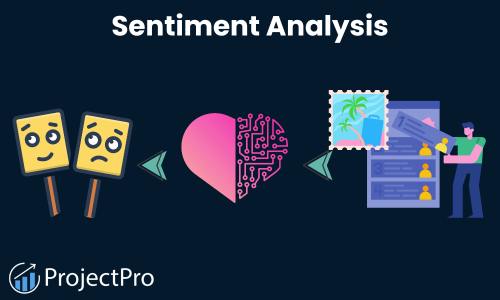
Sentiment analysis has to be done for a large dataset since there are over 180 million monetizable daily active users ( https://www.businessofapps.com/data/twitter-statistics/) on Twitter. The analysis also has to be done in real-time. Spark Streaming can be used to gather data from Twitter in real time. NLP (Natural Language Processing) models will have to be used for sentimental analysis, and the models will have to be trained with some prior datasets. Sentiment analysis is one of the more advanced projects that showcase the use of Big Data due to its involvement in NLP.
Access Big Data Project Solution to Twitter Sentiment Analysis
5. Real-time Analysis of Log-entries from Applications Using Streaming Architectures
If you are looking to practice and get your hands dirty with a real-time big data project, then this big data project title must be on your list. Where web server log processing would require data to be processed in batches, applications that stream data will have log files that would have to be processed in real-time for better analysis. Real-time streaming behavior analysis gives more insight into customer behavior and can help find more content to keep the users engaged. Real-time analysis can also help to detect a security breach and take necessary action immediately. Many social media networks work using the concept of real-time analysis of the content streamed by users on their applications. Spark has a Streaming tool that can process real-time streaming data.
Access Big Data Spark Project Solution to Real-time Analysis of log-entries from applications using Streaming Architecture
6. Analysis of Crime Datasets
Analysis of crimes such as shootings, robberies, and murders can result in finding trends that can be used to keep the police alert for the likelihood of crimes that can happen in a given area. These trends can help to come up with a more strategized and optimal planning approach to selecting police stations and stationing personnel.
With access to CCTV surveillance in real-time, behavior detection can help identify suspicious activities. Similarly, facial recognition software can play a bigger role in identifying criminals. A basic analysis of a crime dataset is one of the ideal Big Data projects for students. However, it can be made more complex by adding in the prediction of crime and facial recognition in places where it is required.
Big Data Analytics Projects for Students on Chicago Crime Data Analysis with Source Code
Explore Categories
In this section, you will find big data projects that rely on cloud service providers such as AWS and GCP.
7. Build a Scalable Event-Based GCP Data Pipeline using DataFlow
Suppose you are running an eCommerce website, and a customer places an order. In that case, you must inform the warehouse team to check the stock availability and commit to fulfilling the order. After that, the parcel has to be assigned to a delivery firm so it can be shipped to the customer. For such scenarios, data-driven integration becomes less comfortable, so you must prefer event-based data integration.
This project will teach you how to design and implement an event-based data integration pipeline on the Google Cloud Platform by processing data using DataFlow .
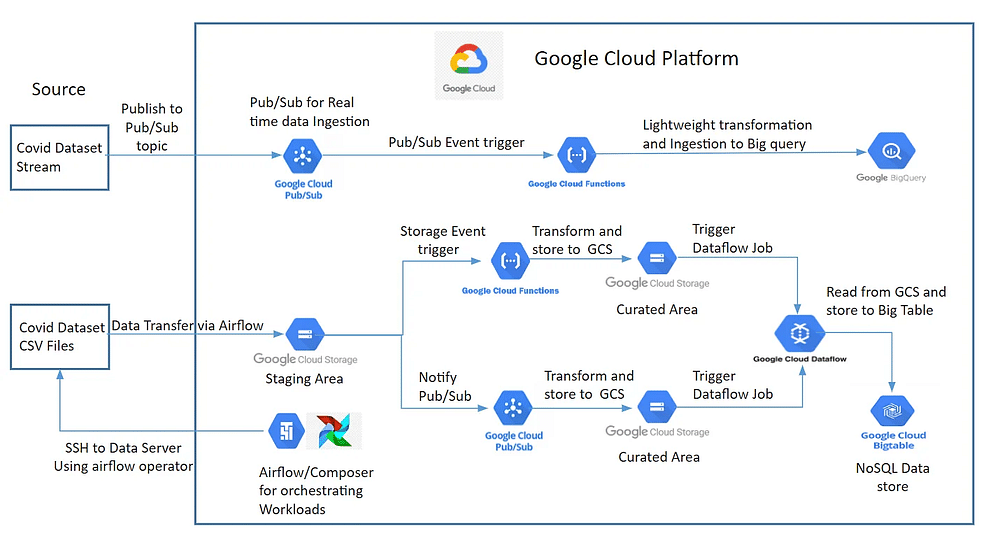
Data Description: You will use the Covid-19 dataset(COVID-19 Cases.csv) from data.world , for this project, which contains a few of the following attributes:
people_positive_cases_count
county_name
data_source
Language Used: Python 3.7
Services: Cloud Composer , Google Cloud Storage (GCS), Pub-Sub , Cloud Functions, BigQuery, BigTable
Big Data Project with Source Code: Build a Scalable Event-Based GCP Data Pipeline using DataFlow
8. Topic Modeling
The future is AI! You must have come across similar quotes about artificial intelligence (AI). Initially, most people found it difficult to believe that could be true. Still, we are witnessing top multinational companies drift towards automating tasks using machine learning tools.
Understand the reason behind this drift by working on one of our repository's most practical data engineering project examples .
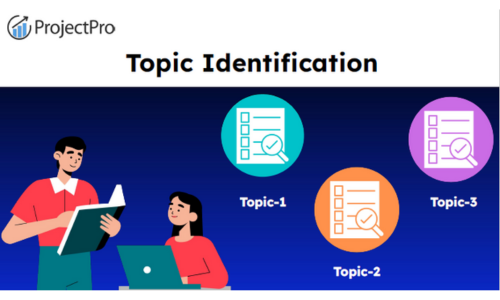
Project Objective: Understand the end-to-end implementation of Machine learning operations (MLOps) by using cloud computing .
Learnings from the Project: This project will introduce you to various applications of AWS services . You will learn how to convert an ML application to a Flask Application and its deployment using Gunicord webserver. You will be implementing this project solution in Code Build. This project will help you understand ECS Cluster Task Definition.
Tech Stack:
Language: Python
Libraries: Flask, gunicorn, scipy , nltk , tqdm, numpy, joblib, pandas, scikit_learn, boto3
Services: Flask, Docker, AWS, Gunicorn
Source Code: MLOps AWS Project on Topic Modeling using Gunicorn Flask
9. MLOps on GCP Project for Autoregression using uWSGI Flask
Here is a project that combines Machine Learning Operations (MLOps) and Google Cloud Platform (GCP). As companies are switching to automation using machine learning algorithms, they have realized hardware plays a crucial role. Thus, many cloud service providers have come up to help such companies overcome their hardware limitations. Therefore, we have added this project to our repository to assist you with the end-to-end deployment of a machine learning project .
Project Objective: Deploying the moving average time-series machine-learning model on the cloud using GCP and Flask.
Learnings from the Project: You will work with Flask and uWSGI model files in this project. You will learn about creating Docker Images and Kubernetes architecture. You will also get to explore different components of GCP and their significance. You will understand how to clone the git repository with the source repository. Flask and Kubernetes deployment will also be discussed in this project.
Tech Stack: Language - Python
Services - GCP, uWSGI, Flask, Kubernetes, Docker
Build Professional SQL Projects for Data Analysis with ProjectPro
Unlock the ProjectPro Learning Experience for FREE
This section has good big data project ideas for graduate students who have enrolled in a master course.
10. Real-time Traffic Analysis
Traffic is an issue in many major cities, especially during some busier hours of the day. If traffic is monitored in real-time over popular and alternate routes, steps could be taken to reduce congestion on some roads. Real-time traffic analysis can also program traffic lights at junctions – stay green for a longer time on higher movement roads and less time for roads showing less vehicular movement at a given time. Real-time traffic analysis can help businesses manage their logistics and plan their commute accordingly for working-class individuals. Concepts of deep learning can be used to analyze this dataset properly.
11. Health Status Prediction
“Health is wealth” is a prevalent saying. And rightly so, there cannot be wealth unless one is healthy enough to enjoy worldly pleasures. Many diseases have risk factors that can be genetic, environmental, dietary, and more common for a specific age group or sex and more commonly seen in some races or areas. By gathering datasets of this information relevant for particular diseases, e.g., breast cancer, Parkinson’s disease, and diabetes, the presence of more risk factors can be used to measure the probability of the onset of one of these issues.
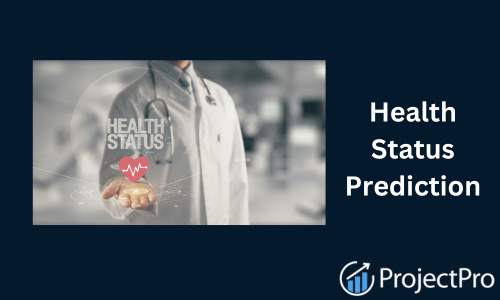
In cases where the risk factors are not already known, analysis of the datasets can be used to identify patterns of risk factors and hence predict the likelihood of onset accordingly. The level of complexity could vary depending on the type of analysis that has to be done for different diseases. Nevertheless, since prediction tools have to be applied, this is not a beginner-level big data project idea.
12. Analysis of Tourist Behavior
Tourism is a large sector that provides a livelihood for several people and can adversely impact a country's economy.. Not all tourists behave similarly simply because individuals have different preferences. Analyzing this behavior based on decision-making, perception, choice of destination, and level of satisfaction can be used to help travelers and locals have a more wholesome experience. Behavior analysis, like sentiment analysis, is one of the more advanced project ideas in the Big Data field.
13. Detection of Fake News on Social Media
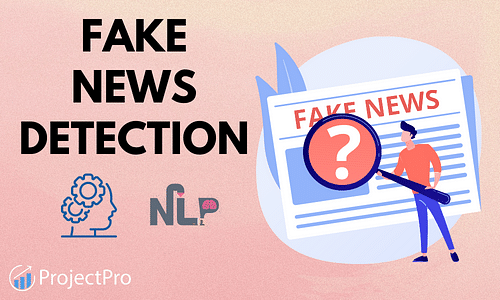
With the popularity of social media, a major concern is the spread of fake news on various sites. Even worse, this misinformation tends to spread even faster than factual information. According to Wikipedia, fake news can be visual-based, which refers to images, videos, and even graphical representations of data, or linguistics-based, which refers to fake news in the form of text or a string of characters. Different cues are used based on the type of news to differentiate fake news from real. A site like Twitter has 330 million users , while Facebook has 2.8 billion users. A large amount of data will make rounds on these sites, which must be processed to determine the post's validity. Various data models based on machine learning techniques and computational methods based on NLP will have to be used to build an algorithm that can be used to detect fake news on social media.
Access Solution to Interesting Big Data Project on Detection of Fake News
14. Prediction of Calamities in a Given Area
Certain calamities, such as landslides and wildfires, occur more frequently during a particular season and in certain areas. Using certain geospatial technologies such as remote sensing and GIS (Geographic Information System) models makes it possible to monitor areas prone to these calamities and identify triggers that lead to such issues.
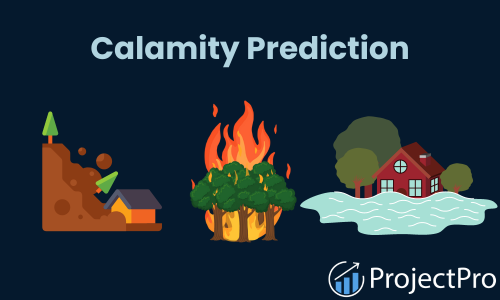
If calamities can be predicted more accurately, steps can be taken to protect the residents from them, contain the disasters, and maybe even prevent them in the first place. Past data of landslides has to be analyzed, while at the same time, in-site ground monitoring of data has to be done using remote sensing. The sooner the calamity can be identified, the easier it is to contain the harm. The need for knowledge and application of GIS adds to the complexity of this Big Data project.
15. Generating Image Captions
With the emergence of social media and the importance of digital marketing, it has become essential for businesses to upload engaging content. Catchy images are a requirement, but captions for images have to be added to describe them. The additional use of hashtags and attention-drawing captions can help a little more to reach the correct target audience. Large datasets have to be handled which correlate images and captions.
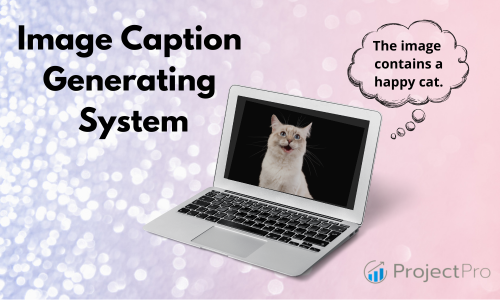
This involves image processing and deep learning to understand the image and artificial intelligence to generate relevant but appealing captions. Python can be used as the Big Data source code. Image caption generation cannot exactly be considered a beginner-level Big Data project idea. It is probably better to get some exposure to one of the projects before proceeding with this.
Get confident to build end-to-end projects
Access to a curated library of 250+ end-to-end industry projects with solution code, videos and tech support.
16. Credit Card Fraud Detection
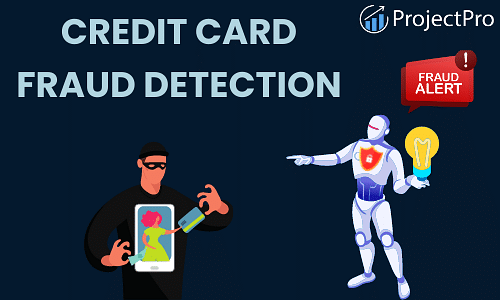
The goal is to identify fraudulent credit card transactions, so a customer is not billed for an item that the customer did not purchase. This can tend to be challenging since there are huge datasets, and detection has to be done as soon as possible so that the fraudsters do not continue to purchase more items. Another challenge here is the data availability since the data is supposed to be primarily private. Since this project involves machine learning, the results will be more accurate with a larger dataset. Data availability can pose a challenge in this manner. Credit card fraud detection is helpful for a business since customers are likely to trust companies with better fraud detection applications, as they will not be billed for purchases made by someone else. Fraud detection can be considered one of the most common Big Data project ideas for beginners and students.
If you are looking for big data project examples that are fun to implement then do not miss out on this section.
17. GIS Analytics for Better Waste Management
Due to urbanization and population growth, large amounts of waste are being generated globally. Improper waste management is a hazard not only to the environment but also to us. Waste management involves the process of handling, transporting, storing, collecting, recycling, and disposing of the waste generated. Optimal routing of solid waste collection trucks can be done using GIS modeling to ensure that waste is picked up, transferred to a transfer site, and reaches the landfills or recycling plants most efficiently. GIS modeling can also be used to select the best sites for landfills. The location and placement of garbage bins within city localities must also be analyzed.
18. Customized Programs for Students
We all tend to have different strengths and paces of learning. There are different kinds of intelligence, and the curriculum only focuses on a few things. Data analytics can help modify academic programs to nurture students better. Programs can be designed based on a student’s attention span and can be modified according to an individual’s pace, which can be different for different subjects. E.g., one student may find it easier to grasp language subjects but struggle with mathematical concepts.
In contrast, another might find it easier to work with math but not be able to breeze through language subjects. Customized programs can boost students’ morale, which could also reduce the number of dropouts. Analysis of a student’s strong subjects, monitoring their attention span, and their responses to specific topics in a subject can help build the dataset to create these customized programs.
19. Real-time Tracking of Vehicles
Transportation plays a significant role in many activities. Every day, goods have to be shipped across cities and countries; kids commute to school, and employees have to get to work. Some of these modes might have to be closely monitored for safety and tracking purposes. I’m sure parents would love to know if their children’s school buses were delayed while coming back from school for some reason.
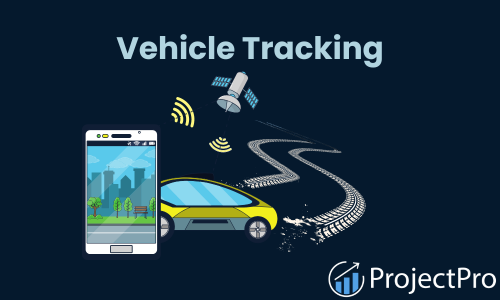
Taxi applications have to keep track of their users to ensure the safety of the drivers and the users. Tracking has to be done in real-time, as the vehicles will be continuously on the move. Hence, there will be a continuous stream of data flowing in. This data has to be processed, so there is data available on how the vehicles move so that improvements in routes can be made if required but also just for information on the general whereabouts of the vehicle movement.
20. Analysis of Network Traffic and Call Data Records
There are large chunks of data-making rounds in the telecommunications industry. However, very little of this data is currently being used to improve the business. According to a MindCommerce study: “An average telecom operator generates billions of records per day, and data should be analyzed in real or near real-time to gain maximum benefit.”
The main challenge here is that these large amounts of data must be processed in real-time. With big data analysis, telecom industries can make decisions that can improve the customer experience by monitoring the network traffic. Issues such as call drops and network interruptions must be closely monitored to be addressed accordingly. By evaluating the usage patterns of customers, better service plans can be designed to meet these required usage needs. The complexity and tools used could vary based on the usage requirements of this project.
This section contains project ideas in big data that are primarily open-source and have been developed by Apache.
Apache Hadoop is an open-source big data processing framework that allows distributed storage and processing of large datasets across clusters of commodity hardware. It provides a scalable, reliable, and cost-effective solution for processing and analyzing big data.
22. Apache Spark
Apache Spark is an open-source big data processing engine that provides high-speed data processing capabilities for large-scale data processing tasks. It offers a unified analytics platform for batch processing, real-time processing, machine learning, and graph processing.
23. Apache Nifi
Apache NiFi is an open-source data integration tool that enables users to easily and securely transfer data between systems, databases, and applications. It provides a web-based user interface for creating, scheduling, and monitoring data flows, making it easy to manage and automate data integration tasks.
24. Apache Flink
Apache Flink is an open-source big data processing framework that provides scalable, high-throughput, and fault-tolerant data stream processing capabilities. It offers low-latency data processing and provides APIs for batch processing, stream processing, and graph processing.
25. Apache Storm
Apache Storm is an open-source distributed real-time processing system that provides scalable and fault-tolerant stream processing capabilities. It allows users to process large amounts of data in real-time and provides APIs for creating data pipelines and processing data streams.
Does Big Data sound difficult to work with? Work on end-to-end solved Big Data Projects using Spark , and you will know how easy it is!
This section has projects on big data along with links of their source code on GitHub.
26. Fruit Image Classification
This project aims to make a mobile application to enable users to take pictures of fruits and get details about them for fruit harvesting. The project develops a data processing chain in a big data environment using Amazon Web Services (AWS) cloud tools, including steps like dimensionality reduction and data preprocessing and implements a fruit image classification engine.
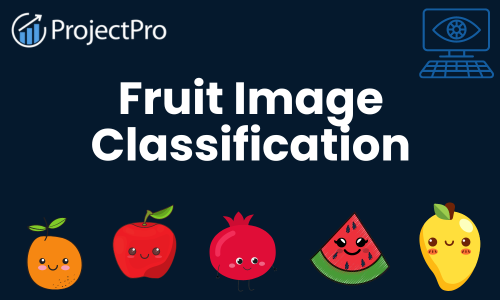
The project involves generating PySpark scripts and utilizing the AWS cloud to benefit from a Big Data architecture (EC2, S3, IAM) built on an EC2 Linux server. This project also uses DataBricks since it is compatible with AWS.
Source Code: Fruit Image Classification
27. Airline Customer Service App
In this project, you will build a web application that uses machine learning and Azure data bricks to forecast travel delays using weather data and airline delay statistics. Planning a bulk data import operation is the first step in the project. Next comes preparation, which includes cleaning and preparing the data for testing and building your machine learning model.
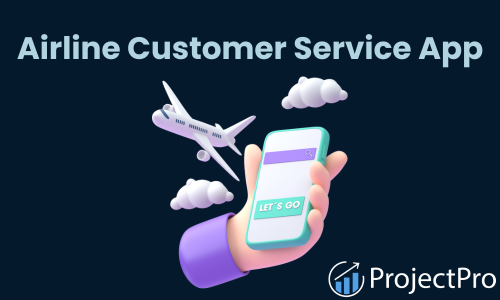
This project will teach you how to deploy the trained model to Docker containers for on-demand predictions after storing it in Azure Machine Learning Model Management. It transfers data using Azure Data Factory (ADF) and summarises data using Azure Databricks and Spark SQL . The project uses Power BI to visualize batch forecasts.
Source Code: Airline Customer Service App
28. Criminal Network Analysis
This fascinating big data project seeks to find patterns to predict and detect links in a dynamic criminal network. This project uses a stream processing technique to extract relevant information as soon as data is generated since the criminal network is a dynamic social graph. It also suggests three brand-new social network similarity metrics for criminal link discovery and prediction. The next step is to develop a flexible data stream processing application using the Apache Flink framework, which enables the deployment and evaluation of the newly proposed and existing metrics.
Source Code- Criminal Network Analysis
Trying out these big data project ideas mentioned above in this blog will help you get used to the popular tools in the industry. But these projects are not enough if you are planning to land a job in the big data industry. And if you are curious about what else will get you closer to landing your dream job, then we highly recommend you check out ProjectPro . ProjectPro hosts a repository of solved projects in Data Science and Big Data prepared by experts in the industry. It offers a subscription to that repository that contains solutions in the form of guided videos along with supporting documentation to help you understand the projects end-to-end. So, don’t wait more to get your hands dirty with ProjectPro projects and subscribe to the repository today!
Get FREE Access to Data Analytics Example Codes for Data Cleaning, Data Munging, and Data Visualization
1. Why are big data projects important?
Big data projects are important as they will help you to master the necessary big data skills for any job role in the relevant field. These days, most businesses use big data to understand what their customers want, their best customers, and why individuals select specific items. This indicates a huge demand for big data experts in every industry, and you must add some good big data projects to your portfolio to stay ahead of your competitors.
2. What are some good big data projects?
Design a Network Crawler by Mining Github Social Profiles. In this big data project, you'll work on a Spark GraphX Algorithm and a Network Crawler to mine the people relationships around various Github projects.
Visualize Daily Wikipedia Trends using Hadoop - You'll build a Spark GraphX Algorithm and a Network Crawler to mine the people relationships around various Github projects.
Modeling & Thinking in Graphs(Neo4J) using Movielens Dataset - You will reconstruct the movielens dataset in a graph structure and use that structure to answer queries in various ways in this Neo4j big data project.
3. How long does it take to complete a big data project?
A big data project might take a few hours to hundreds of days to complete. It depends on various factors such as the type of data you are using, its size, where it's stored, whether it is easily accessible, whether you need to perform any considerable amount of ETL processing on the data, etc.
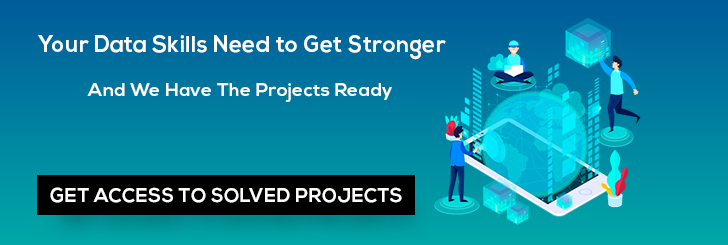
About the Author

ProjectPro is the only online platform designed to help professionals gain practical, hands-on experience in big data, data engineering, data science, and machine learning related technologies. Having over 270+ reusable project templates in data science and big data with step-by-step walkthroughs,

© 2024
© 2024 Iconiq Inc.
Privacy policy
User policy
Write for ProjectPro
- How it works
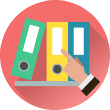
Sample Masters Big Data Full Dissertation
Here is a sample that showcases why we are one of the world’s leading academic writing firms. This assignment was created by one of our expert academic writers and demonstrated the highest academic quality. Place your order today to achieve academic greatness.
View a different grade
Investigating the Impact of Big Data on Automobile Industry Operations
The current study uses a quantitative research approach to analyze how Big Data initiatives impact the operation functions of automobile companies in the UK. The research used a survey as the research instrument to gather data from 132 participants working in automobile companies in the UK. The survey looked to examine the opinions that executives had held about Big Data and how it impacted the company. The survey was distributed online to individuals that worked for automobile companies in the UK using Survey Monkey. The data obtained were then analyzed using descriptive statistics to find factors that may be influencing the use of Big Data in automobile companies. Based on these results, it is concluded that more significant investments in Big Data bring about positive impacts. The results presented conclude that investing more than 1 billion GBP on Big Data initiatives would provide greater tangible benefits for a business and positively impact the company. The results also found that companies with greater analytical abilities on the adequate and above adequate range could see measurable results. In the end, Big Data did have a positive and large impact on the operations business function of automobile companies.
Chapter 1: Introduction to the Research Topic
Introduction.
Big Data is recently on the rise as imperative information and tools need to be incorporated into businesses and daily life. Pflugfelder (2013) defines Big Data as large in volume, high in velocity, extensive in its variety, unable to be handled using conventional systems like relational databases, and needs unique and advanced technology for storage management analysis and visualization. However, the actual definition of Big Data varies from industry to industry and business to business. Schroeck et al. (2012) found in their research that 18 percent of businesses defined Big Data as a vast source of information, 15 percent of companies named it as real-time information, and seven percent of these businesses considered Big Data as a source of information from social media. By combining these demarcations, the resultant is a definition that portrays Big Data as a source of information that can be structured, unstructured, and semi-structured, which needs new technology, tools, and techniques for its storage, processing, analysis, and visualization for a large volume of data that is emitted at high speed and variety.
Significance of the Research Area
The automobile industry is increasingly becoming competitive in sustaining economies, especially with fierce competition between Western and Eastern manufacturers (Wallner and Kriglstein 2013). The industry has had a significant impact on regional and world economies and societies (Lee et al., 2014). To capture a large chunk of the market and consumers’ interest in an increasingly competitive market, it is crucial to make decisions based on real-time data. For this reason, many automobile companies around the world have begun to integrate Big Data into their decision-making process that ranges from manufacturing to marketing. Walker (2015) has found that integrating Big Data into business-related tasks in the automobile industry can be accomplished through the following; • Recalculating entire risk assortments within minutes • Identifying fraudulent behaviour quickly, which might affect the automobile industry. • Regulating root causes of problems, issues, failures, and defects could affect the longer term and shorter term. • Generating sales based on market research of consumer behaviour. With automobiles being an intricate part of developed society, it becomes mandatory for companies to ensure that they are providing quality products for the masses. Big Data can play a significant role in the business activities of automobile companies. With changing consumer behaviour and more informed consumers, it has become essential for companies to integrate real-time information into business decisions.
Problem Statement
Automobile manufacturing in the UK has become a vital part of its economy. According to the Society of Motor Manufacturers and Traders (2016), there were 2.63 million cars registered in 2015, which increased six percent from 2014. Due to the rapid changes and developments in the automobile industry, Big Data analysis has become vital to ensure new success levels in this revolutionary period. For this reason, the current study looks to understand the significance that Big Data plays in the Automobile industry. To compete in an already competitive environment, it has become necessary for businesses to understand the value that Big Data can bring to them. This makes it imperative for Big Data users to make decisions that can bring a competitive edge to the business, or else integrating Big Data becomes of use now. Schroeck et al. (2012) find that a vast deal of available data to companies is commonly unrelated. It comes from various data sources such as sensors, mobiles, transactions, social medial, log files, audio, video, images, and emails. The processing of such large amounts of data to produce meaningful decisions has become critical for businesses to thrive and succeed in markets where consumer trends cannot change rapidly (Shah et al., 2014). The automobile industry in the UK needs to improve its decision-making process to advance critical operations to compete in a highly competitive regional and international market. Monaghan (2016) notes that the British car industry has been enjoying prolonged periods of growth, as witnessed with a car production increase in June 2016 that rose by 10.4% to 159,000 cars, the highest since June 1998. According to the Society of Motor Manufacturers and Traders (SMMT), by 2017, the UK has the possibility of building a record number of vehicles per year that may overtake France and Spain to become Europe’s second-largest producer after Germany (Foy 2014). However, Foy (2014) points that such success may be hindered due to the eroding supply chain and operations of the British car industry, primarily smaller companies that provide parts and electronic components that go into cars, making it the biggest concern of the industry. To overcome these concerns, many are looking towards including more efficient data that may help industry leaders make better decisions for more prosperous businesses (Shooter 2013).
Research Aim and Objectives
Big Data is now widely being used in the automobile industry to take quick actions, saving time and cost prices. Understanding how the automobile industry can integrate the analysis of Big Data into its daily operations has become imperative to improve its integration and ensure that Big Data is being used correctly to obtain the maximum benefit from it. Therefore, the following research question has been formulated.
How has Big Data Impacted the UK Automobile Industry’s Operations?
Based on the research question, the research’s main aim is to investigate the impact of Big Data on the automobile industry, specifically in the UK, operations such as sales, customer retention, the manufacturing process, performance, marketing, logistics, and supply chain management. To achieve the research aim and answer the research question, the following objectives have been developed. • Assess the impact of Big Data on sales of automobiles and their marketing. • Assess the impact Big Data has on ensuring customer retention. • Examine how Big Data has revolutionized the automobile industry in the Uk and increased the potential use for business analytics. • Assess the impact that Big Data can have on improving the performance and efficiency of an automobile company.
Research Approach
The current study will be conducted using a qualitative research approach. Based on the sections above, the study’s aim and objectives have been developed to pursue the study’s research question using the proposed research approach. To build a research approach, a literature review was conducted to understand previous studies that have attempted to analyze Big Data’s influence in various industries. The results of the literature review (i.e., chapter two) aided in building the research approach. Under this approach, primary research is conducted using semi-structured interviews as the research instrument for data collection. The justification of this approach will be discussed in detail in chapter three of the study.
Project Outline
The current study is divided into six chapters. Below is the outline of the study;
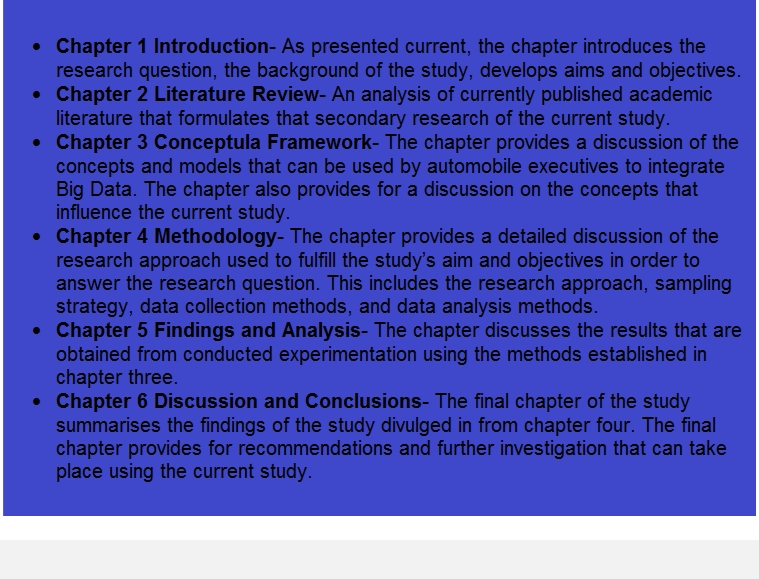
Big Data has been influential in the 21st century by providing industries and companies with detailed information to make more intelligent business decisions. Very little research has been conducted on how Big Data impacts the automobile industry. Therefore, the current study aims to analyze and comprehend how Big Data impacts the UK automobile industry in influencing operations, sales, marketing, and other business aspects. For this purpose, the study developed a set of objectives that will be used to fulfil the study’s aim and the primary research question. The study is structured according to a qualitative research approach. Building the research approach designated the need for a literature review presented in the next chapter (i.e., Chapter two).
Chapter 2: Literature Review
The literature review chapter is constructed based on systematic research principles to provide an in-depth analysis of previously published literature on topics related to the current research. The literature review will provide critical insight into various definitions relevant to developing the current research and its primary focus throughout the dissertation. To conduct this literature review, it was essential to search for relevant papers through various databases such as Wiley Online Library, Science Direct, IEEE Xplore Digital Library, and Google Scholar. For the current literature review, the chapter is divided into sections that answer the literature reviews research questions, which are as follows;
- How to have various other fields and domains, other than the automobile industry, used Big Data analytics and visual analytics?
- What are the types of data sources that have been reported in the literature?
- What are types of Big Data visualization techniques and tools for Big Data visual analytics?
Previous literature that can provide understanding based on these questions was included in the literature review. Based on the analysis of the literature included, the methodology of the current research will be constructed.
The 7V’s of Big Data
Big Data is defined using the 7V’s known as volume, velocity, variety, variability, veracity, visualization, and value.
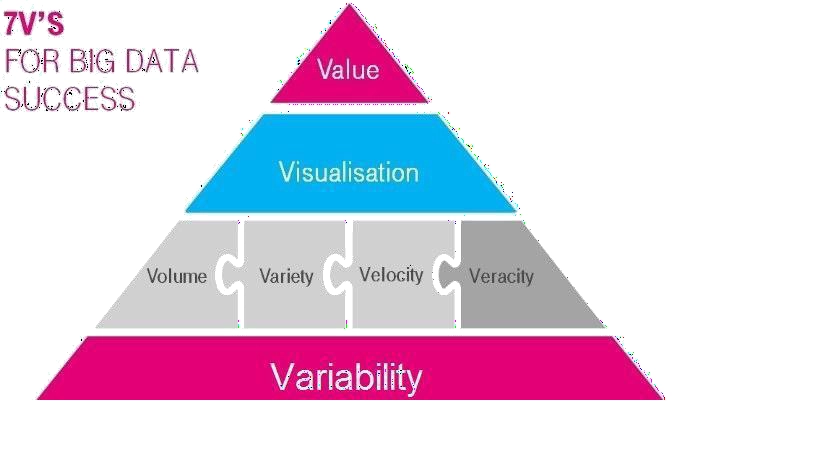
Figure 2.2-1: 7V’s of Big Data
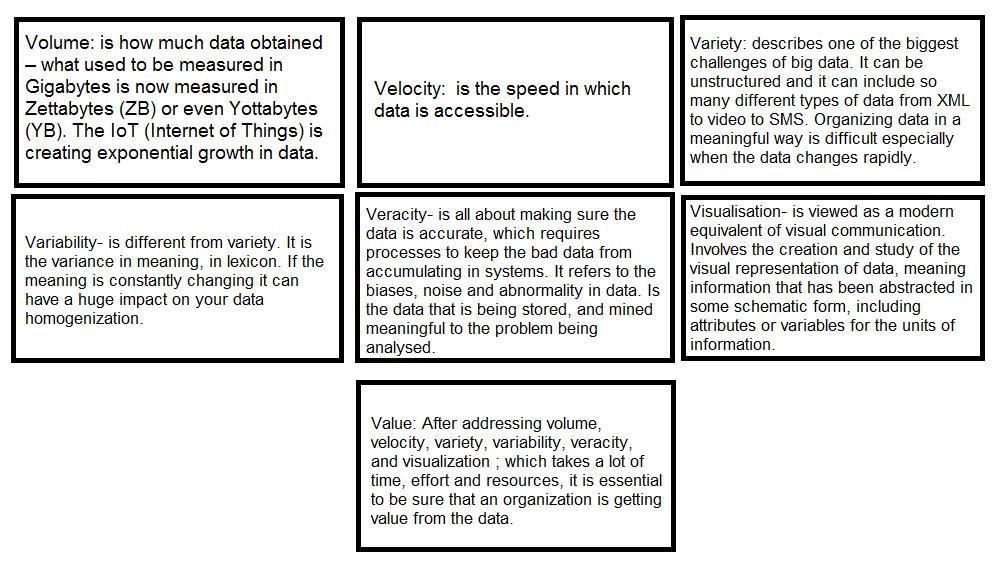
Fields and Domains using Big Data and Visual Analytics
Based on the literature review, there are practically little to no publications available that portray the extent or detailed use of Big Data analytics in the automobile industry. However, many vital publications have noted that Big Data analytics is becoming a trend impacting businesses globally. Wozaniak et al. (2015) examined and determined to comprehend the type of data available to Volvo and how it was extracting such data. Based on the study, it is found that Volvo used data from its production planes and service centres to obtain data about their vehicles to assess information such as customer satisfaction, mileage coverage, and other vital factors that would improve decision making. Wozniak et al. (2015) found that Volvo uses data sources from logged production information; product specifications, client information, dealer information, product session information, telematics data, service history, repair history, warranties, and service contracts, which are then dispersed throughout the organization to specific departments, software teams, and engineers to use the data for production or operations improvements. Many other industries are also using Big Data analytics for their services and products.
Big Data analytics can have a profound impact on the future of banking industries. Collecting data at a massive scale can allow banks to comprehend the needs and expectations of their customers. However, banks lack the skills to execute and deploy significant data initiatives as they leverage more familiar technologies and software-development lifecycle (SDLC) methodologies. To develop analytic tools that experts in the banking industry comprehend, it is essential to meld together accurate data interpretation on a user-friendly interface. Commotion is an example of a Big Data analytics tool that keeps the user in mind. Commotion allows for a comfortable and easy experience for bank data exploration (Laberge, Anderson, et al., 2012). The analytics tool will enable analysts to drag and drop data collections that produce variable chart visualizations. The process is formally known as the “think loop process,” allowing analysts to dig and separate larger data collections to explore particular hypotheses based on smaller groupings to understand banks’ network anomalies (Laberge, Anderson, et al., 2012).
Implementing Big Data into the transportation industry has allowed it to become resilient in extreme scenarios. A large portion of the world’s population has shifted to urban living areas requiring cities to deliver sustainable, effective, and efficient services. Big data analytic research projects are currently commenced in the transportation industry to deal with massive data coming from roads & vehicle sensors, GPS devices, customer apps, and other websites. Ben Ayed et al. (2015) have reported using Big Data analytics in Dublin to improve the city’s public bus transportation network and reduce issues with increased traffic congestion. Using advanced analytics on the collected data, specific traffic problems were identified. The optimal time needed to start bus lanes was answered, and recommendations were made to add bus lanes (Ayed et al., 2015).
Ferreira, Poco et al.’s 2013 study provides insight into taxi trips to visually query taxi trips allowing taxi companies to make better decisions to schedule driver shifts and increase revenue. The use of Big Data analytics in transportation has also allowed policymakers to develop improved preparation plans and disaster management plans for high-risk events such as accidents, public gatherings, and natural disasters. Using smart card data and social media data, the resilience of transportation systems can be increased by analyzing changes in passenger behaviour, replaying historical events within the specific area to discover anomalous situations, and customer service (Itoh, Yokoyama, et al. 2014).
Types of Big Data Sources
Unlike typical data, Big Data contains videos, text, audio, images, and other forms of data collected from numerous datasets making it difficult to process with traditional database management tools giving rise to a new generation of tools specifically designed to analyze and visualization Big Data.
Santourian et al. (2014) observe that Big Data is often generated from transactions (i.e., invoices, payment orders, delivery records, and storage records) or unstructured data such as text extracts from websites, social media, or images.
However, Santourian et al. (2104) note that Big Data can also be collected in “real-time” from sensors such as those found in smartphones or from logs extracted from behaviour found online.
Big Data’s rawness due to the velocity by which it is being received oftentimes is unable to serve a statistical purpose as they have been collected by third parties who don’t emphasize data collection.
Big Data sources vary across industries as data collection needs to fit the purpose for they are to be used in the analysis. For example, Fiore et al. (2015) use data sources that have been made available by project partners or made available through national and international agencies developing a more static setup for Big Data analysis.
This included sources of data coming from satellite imagery, remote sensing data, hyperspectral imagery, and climate data used to formulate a use case infrastructure to analyze climate change trends in Manaus, Brazil (Fiore et al., 2015).
A study conducted by Baciu et al. (2015) has reported that use of sources that vary across fields, such as extracting data from a website known as a Bright kite that collects data from 4.5 million mobile users locations, such as their latitude and longitudes of each of the mobile users over specific intervals of time.
Studies that are less scientifically complex in theme use other sources of data; such as text sources which include words, phrases, and even entire documents extracted from social media platforms (i.e., Facebook) is used to analyze and predict events such as market trends, analyses product defects, and management of calamities (Fan and Gordon 2014; Mahmud et al. 2014).
Large companies also use various data sources to collect raw data to turn it into meaningful knowledge that can then be used to improve customer service, examine product defects, analyze organizational changes, and comprehend changing consumer trends (Heer and Kandel 2012; Kateja et al. 2014).
Volvo, an automobile manufacturer, uses data sources from logged product information; product specifications, client information, dealer information, product session information, telematics data, service history, repair history, warranties, and service contracts, which is then dispersed throughout the organization to specific departments/divisions, software teams, and engineers to use the data for improvements (Wozniak et al. 2015).
Big Data Visualization Techniques and Tools for Big Data Visual Analytics
Vatrapu et al. (2015) define data visualization as a method to communicate and transfer information clearly and effectively through graphical means. Given the rise of Big Data, analysts have begun to use data visualization methods to visualize, recognize, differentiate, interpret, and communicate configured data patterns based on the new visualization techniques specifically for massive datasets.
With new techniques, data scientists, analysts, and industry leaders benefit from comprehending massive amounts of data, recognizing emerging properties within the data, data quality control, feature detection on a small and large scale, and evidence for formulating hypotheses.
Generally, all visualization techniques and tools follow a similar pattern which includes the use of processing steps of data acquisition data transforming, mapping data onto visual models, and lastly, rendering or viewing the data (Zhang et al. 2013; Goonetilleke et al. Liu et al. 2015; Fu et al. 2014). Following is a brief discussion of visualization tools and techniques that have been used across diverse industries and studies to Big Data.
Popular domains that highly demand Big Data are healthcare, automobile, transport/Urban infrastructure, banking, and retail. The chapter also found sources through which the domains discussed retrieve vital information/data to use as meaningful knowledge. It is evident from the literature review that sources for retrieval of data diverge significantly from normal sources.
Firstly, the Big Data sources will contain massive data from sensors such as those on a phone that monitor health. With such massive data, it is necessary to follow specific steps laid out for Big Data analytics. Data analysis is done to the most microscopic level that a researcher can go with such tremendous amounts of data.
Finally, data visualization becomes necessary for producing information that can be used to help in decision-making.
A systematic literature review has also revealed the numerous different sources from which Big Data is extracted. Sources vary depending on the domain, which is the source to extract specific kinds of data.
Literature reveals that typical Big Data contains videos, texts, audio, and images at massive levels of datasets. The datasets’ complexity produces a challenge for traditional database management tools to handle the volume of the data that is being analyzed.
Familiar sources for Big Data generation are payment orders, delivery records, invoices, and storage records. However, sources can be “real-time” if it is collected by sensors such as those present in smartphones.
Unstructured data is also commonly seen in Big Data ranging from social media posts, images, text extracts from websites, or even whole websites. Regardless of what type of data it is, the sources from which it is obtained will vary from industry to industry.
Data can come from social media data such as Facebook wall posts, comments, likes, and Twitter tweets, to name a few. Simultaneously, more experimental and scientific sources also provide specific data such as temperature, humidity, and wind speeds data in “real-time” to analyze and make predictions towards climate change.
Hire an Expert Dissertation Writer
Orders completed by our expert writers are
- Formally drafted in the academic style
- 100% Plagiarism-free & 100% Confidential
- Never resold
- Include unlimited free revisions
- Completed to match exact client requirements
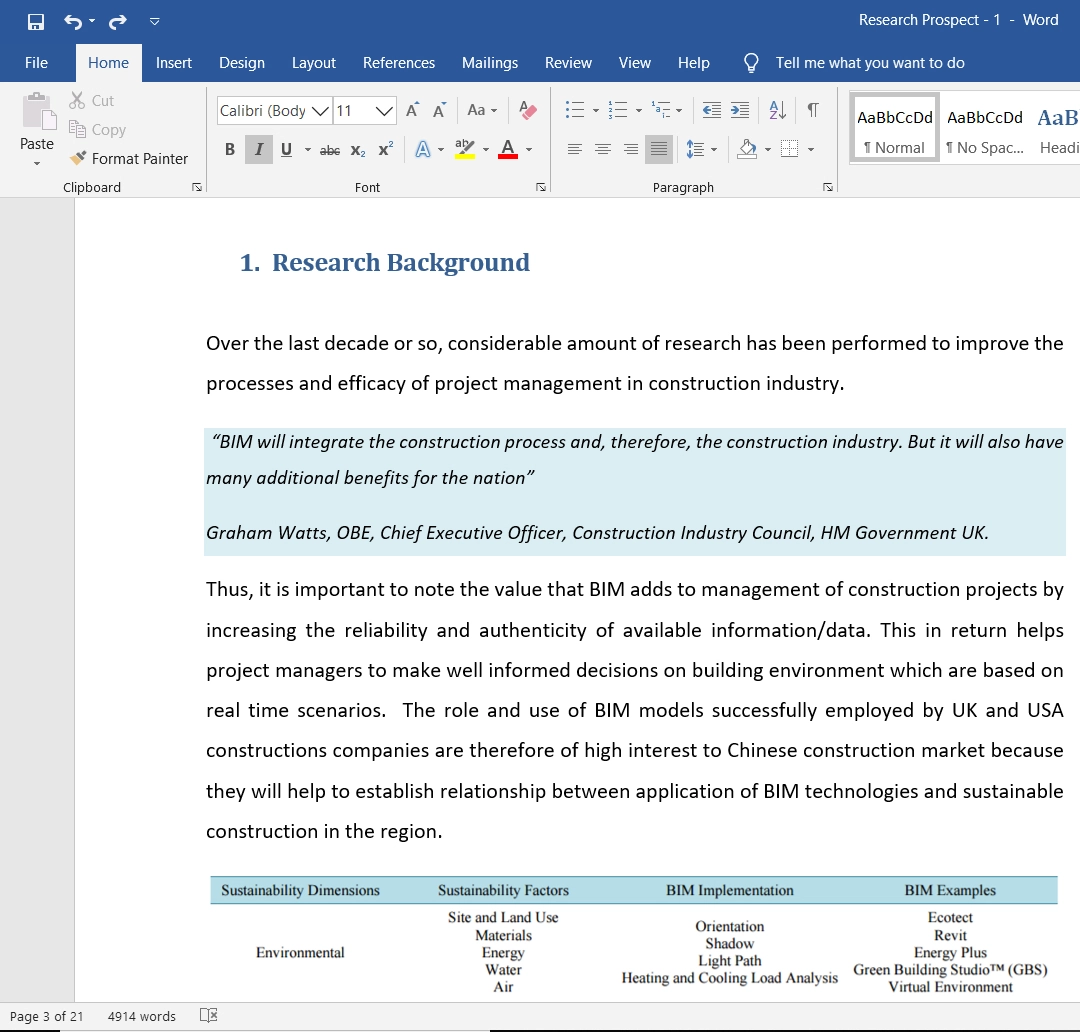
Chapter 3: Conceptual Framework
The chapter presents the conceptual framework for automobile company executives to adopt. This is achieved using the adopters’ category under the diffusion of innovations theory proposed by Rogers (2003).
Theoretical Development
The diffusion of innovations theory was heavily relied upon to develop the conceptual framework, as Rogers (2003) proposed. Based on the idea, diffusion is the process by which innovation is communicated over some time among those participating in a social system. According to Rogers (2003), four main elements influence the spread of a new idea; innovation, communication channels, time, and a social system. Currently, automobile companies are slowly creeping into Big Data to handle operations, as evident from the literature review. The process of diffusion relies extremely on human capital. This means that innovation needs to be widely adopted within a setting to self-sustain itself.
There are various strategies available to help an innovation reach the stage of critical mass. This includes the strategy of when an innovation is adopted by a highly respective person in an organisation and develops an instinctive desire for a specific innovation. Rogers (2003) argues that one of the best strategies is to place innovation into a group of individuals who can readily use the technology and provide positive reactions resulting in benefits to early adopters. By using the adoption process under innovation theory diffusion, automobile companies can target respected high-level executives to shift their support towards big data initiatives.
The proposed conceptual framework provides automobile companies with strategies that adopt big data initiatives to promote innovation. The best way to do so is to present innovation to highly respectable executives in the company to promote innovation to self-sustain it.
Chapter 4: Methodology
The current chapter presents developing the research methods needed to complete the experimentation portion of the current study. The chapter will discuss in detail the various stages of developing the methodology of the current study. This includes a detailed discussion of the philosophical background of the research method chosen. In addition to this, the chapter describes the data collection strategy, including a selection of research instrumentation and sampling. The chapter closes with a discussion on the analysis tools used to analyse the data collected.
Selecting an Appropriate Research Approach
Creswall (2013) stated that research approaches are plans and procedures that range from making broad assumptions to detailed methods of data collection, analysis, and interpretation.
The several decisions involved in the process are used to decide which approach should be used in a specific study that is informed using philosophical assumptions brought to the study (Creswall 2013).
These are procedures of inquiry or research designs and specific research methods used for data collection, its analysis, and finally, its interpretation. However, Guetterman (2015); Lewis (2015); and Creswall (2013) argue that the selection of the specific research approach is based on the nature of the research problem, or the issue that is being addressed by any study, personal experiences of the researchers’, and even the audience for which the study is being developed for.
The main three categories with which research approaches are organised include; qualitative, quantitative, and mixed research methods. Creswall (2013) comments that all three approaches are not considered discrete or distinct.
Creswall (2013) states, “qualitative and quantitative approaches should not be viewed as rigid, distinct categories, polar opposite, or dichotomies” (p.32).
Guetterman (2015) points out that a clearer way of viewing gradations of differences between the approaches is to examine the basic philosophical assumptions brought to the study, the kinds of research strategies used, and the particular methods implemented in conducting the strategy.
Underlying Philosophical Assumptions
An important component of defining the research approach involves philosophical assumptions that contribute to the broad research approach of planning or proposing to conduct research. It involves the intersection of philosophy, research designs, and specific methods, as illustrated in Fig. 1 below.
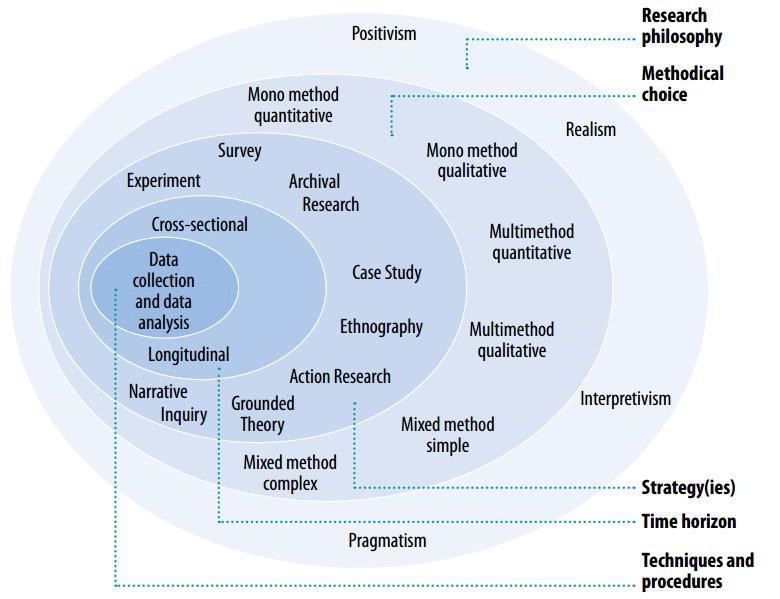
Figure 4.2-1- Research Onion (Source; Saunders and Tosey 2013)
Saunders et al. (2009) define research philosophy as a belief about how data about a phenomenon should be gathered, analyzed, and used. Positivism reflects the acceptance in adopting the philosophical stance of natural scientists (Saunders, 2003).
According to Remenyi et al. (1998), there is a greater preference in working with an “observable social reality” and that the outcome of such research can be “law-like” generalisations that are the same as those which physical and natural scientists produce.
Gill and Johnson (1997) add that it will also emphasise a highly structured methodology to replicate other studies. Dumke (2002) agrees and explains that a positivist philosophical assumption produces highly structured methods and allows for generalisation and quantification of objectives that statistical methods can evaluate.
For this philosophical approach, the researcher is considered an objective observer who should not be impacted by or impact the research subject.
The current study chooses positivist assumptions due to the literature review’s discussion of the importance of Big Data in industrial domains and the need to measure its success in business operations.
To identify a positive relationship between Big Data usage and beneficial business outcomes, the theory needs to be used to generate hypotheses that can later be tested of the relationship, which would allow for explanations of laws that can thereafter be assessed (Bryman and Bell, 2015).
Selecting Interpretive Research Approach
Interpretive research approaches are derived from the research philosophy that is adopted. According to Dumke (2002), the two main research approaches are deductive and inductive.
The inductive approach is commonly referred to when theory is derived from observations. Thus, the research begins with specific observations and measures. It is then from detecting some pattern that a hypothesis is developed.
Dumke (2002) argues that researchers who use an inductive approach usually work with qualitative data and apply various methods to gather specific information that places different views.
From the philosophical assumptions discussed in the previous section, it is reasonable to use the deductive approach for the current study. It is also considered the most commonly used theory to establish a relationship between theory and research. The figure below illustrates the steps used for the process of deduction.
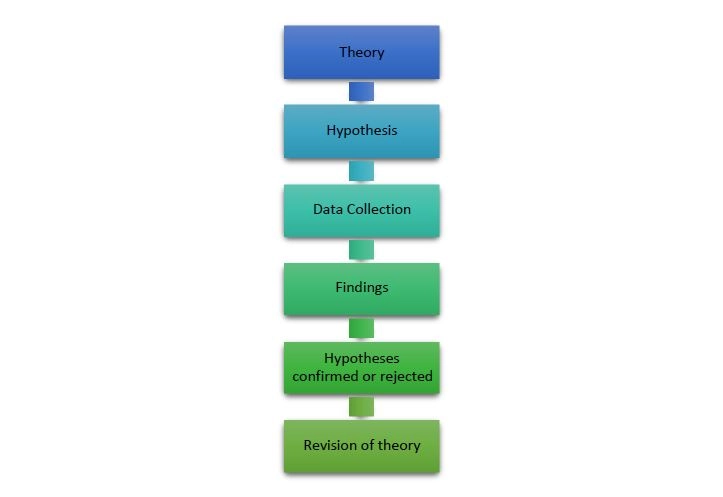
Figure 4.2-2- The process of deduction (Source, Bryman and Bell 2015)
Based on what is known about a specific domain, the theoretical considerations encompassing it a hypothesis or hypotheses are deduced that will later be subjected to empirical inquiry (Daum, 2013). Through these hypotheses, concepts of the subject of interest will be translated into rational entities for a study. Researchers are then able to deduce their hypotheses and convert them into operational terms.
Justifying the Use of Quantitative Research Method
Saunders (2003) notes that almost all research will involve numerical data or even contain data quantified to help a researcher answer their research questions and meet the study’s objectives.
However, quantitative data refers to all data that can be a product of all research strategies (Bryman and Bell, 2015; Guetterman, 2015; Lewis, 2015; Saunders, 2003).
Based on the philosophical assumptions and interpretive research approach, a quantitative research method is the best suited for the current study. Mujis (2010) defends the use of quantitative research because, unlike qualitative research, which argues that there is no pre-existing reality, quantitative assumes that there is only a single reality about a social condition that researchers cannot influence way.
Selecting an Appropriate Research Strategy
There are many strategies available to implement in a study, as evidence by Fig. 1. There are many mono-quantitative methods, such as telephone interviews, web-based surveys, postal surveys, and structured questionnaires (Haq 2014).
Each instrument has its own pros and cons in terms of quality, time, and data cost. Brymand (2006); Driscoll et al. (2007); Edwards et al. (2002); and Newby et al. (2003) note that most researchers use structured questionnaires for data collection they are unable to control or influence respondents, which leads to low response rates but more accurate data obtained.
Saunders and Tosey (2015) have argued that quantitative data is simpler to obtain and more concise to present. Therefore, the current study uses a survey-based questionnaire (See Appendix A).
Justifying the use of Survey Based Questionnaire
Surveys are considered the most traditional forms of research and use in non-experimental descriptive designs that describe some reality. Survey-based questionnaires are often restricted to a representative sample of a potential group of the study’s interest.
In this case, it is the executives currently working for automobile companies in the UK. The survey instrument is then chosen for its effectiveness at being practical and inexpensive (Kelley et al., 2003).
The philosophical assumptions, interpretive approach, and methodological approach are chosen. The current study’s survey design is considered the best instrument in line with these premises and cost-effectively.
Empirical Research Methodology
Research design.
This section describes how research is designed to use the techniques used for data collection, sampling strategy, and data analysis for a quantitative method. Before going into the strategies of data collection and analysis, a set of hypotheses were developed.
Hypotheses Development
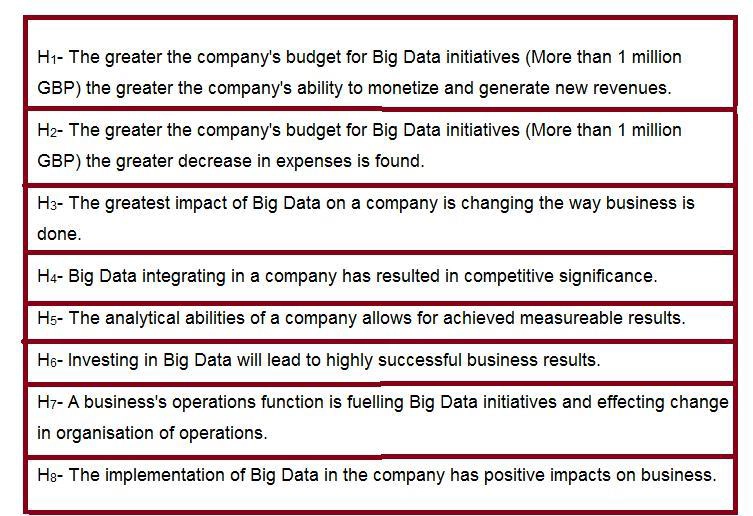
Data Collection
This section includes the sampling method used to collect the number of respondents needed to provide information then analysed after collection.
Sampling Method
Collis (2009) explains that there are many kinds of sampling methods that can be used for creating a specific target sample from a population. This current study uses simple random sampling to acquire respondents with which the survey will be conducted.
Simple random sampling is considered the most basic form of probability sampling. Under the method, elements taken from the population are random, with all elements having an equal chance of being selected.
According to the Office of National Statistics (ONS), as of 2014, there are about thirty-five active British car manufacturers in the UK, each having an employee population of 150 or more.
This is why the total population of employees in car manufacturers is estimated to be 5,250 employees. The sample therefore developed, used the following equation;
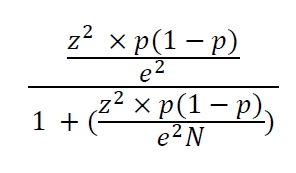
Where; N is the population size, e is a margin of error (as a decimal), z is confidence level (as a z-score), and p is percentage value (as a decimal). Thus, the sample size is with a normal distribution of 50%. With the above equation, a population of 5,250, with a 95% confidence level and 5% margin of error, the total sample size needed for the current equals 300. Therefore, N=300 is the sample size of the current study.
The survey development (see Appendix A) has a total of three sections, A, B, and C, with a total of 39 questions. Each section has its own set of questions to accomplish.
The survey is a mix of closed-ended questions that comprehend the respondent’s demographic make us, the Big Data initiatives of the company, and the impact that Big Data was having in their company. The survey is designed to take no longer than twenty minutes. The survey was constructed on Survey Monkey.com, an online survey-provided website.
The survey was left on the website for a duration of 3.5 weeks to ensure that a maximum number of respondents answered the survey. The only way the survey was allowed for a respondent is to pass a security question that asks if they are working for an automobile company in the UK to take the survey.
Gupta et al. (2004) believe that web surveys are visual stimuli, and the respondent has complete control concerning whether or how each question is read and understood. That is why Dillman (2000) argued that web questionnaires are expected to resemble those taken through the mail/postal services closely.
Data Analysis
The collected data is then analysed using the Statistical Package for Social Science (SPSS) version 24 for descriptive analysis. The demographic section of the survey will be analysed using descriptive statistics. Further analysis of the data also includes the use of descriptive statistics.
Conclusions
The chapter provides a descriptive and in-depth discussion of the methods involved in the current study’s research. The current study looks towards a quantitative approach that considers positivism as its philosophical undertaking, using deductive reasoning for its interpretive approach, a mono-quantitative method that involves using a survey instrument for data collection.
The methodology chapter also provided the data analysis technique, which is descriptive statistics through frequency analysis and regression analysis.
Chapter 5 Results and Analysis
The chapter provides the findings of the current study based on the survey results obtained. It provides a straightforward statement of the results using descriptive statistics, which would later be further analysed using SPSS v.24 software. The need for SPSS is to conduct a regression analysis to provide a detailed examination of the data.
Section A- Demographic Results
The study had called for 300 respondents to answer the survey using Survey Monkey, left online for 3.5 weeks. However, the total completed surveys obtained was 132, making the survey’s response rate only forty-four percent (44%). It was not the best response rate, but it still provided a broad range of participants to analyse.
The first question of the survey’s section A called for respondents to identify their job title for the current automobile company that they were working for. Fig. 5.2-1-1 shows that operations managers and supervisors made up the greatest number of respondents in the study.
Operations managers had composed 14 percent of the respondents, followed by the foreperson, supervisor, lead person of 13 percent, and the project managers that made 12 percent of the respondents.
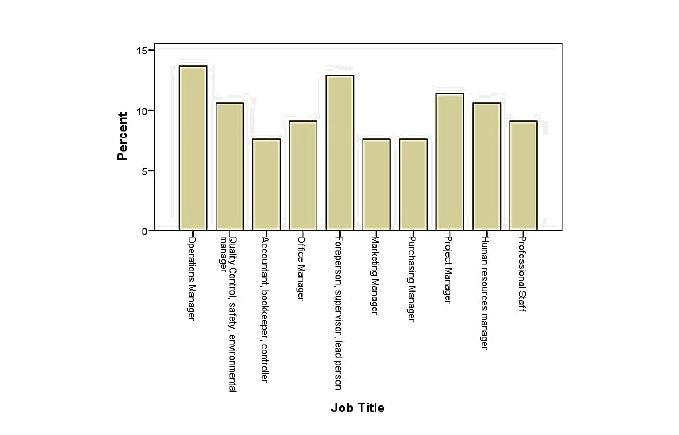
Respondents were also asked to indicate the number of years they have been employed in a specific organisation. Allowing for such insight would provide a sense of experience that the participant may have had while working in the company.
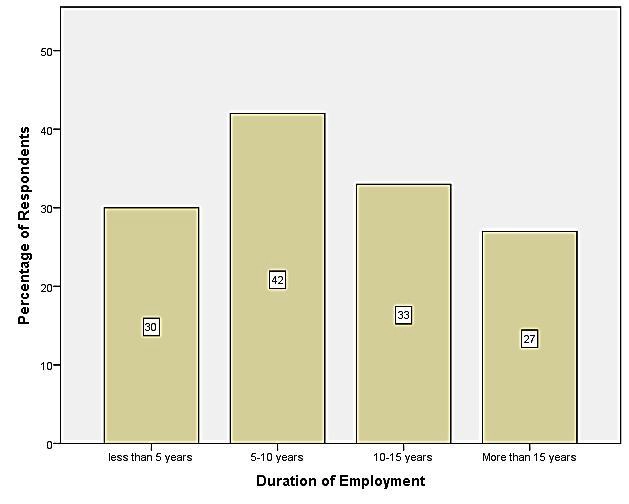
Figure 5.2-2.- How long have you worked for the organization?
This is illustrated in Fig. 5.2-2, in which 42 percent of respondents have indicated that they have worked for the company for five to ten years. Of the respondents, thirty-three percent have indicated that they have worked for their company for 10-15 years, while thirty percent indicated less than five years. The remaining 27 percent indicated employment for over fifteen years.
The survey also asked respondents to indicate the number of employees who worked for the firm ty was employed in. Having such knowledge would allow the researcher to understand the extent of operations conducted in the automobile company. Having such an understanding provides insight into the scope of use in Big Data being implemented in the company (see Fig.5.2-3).
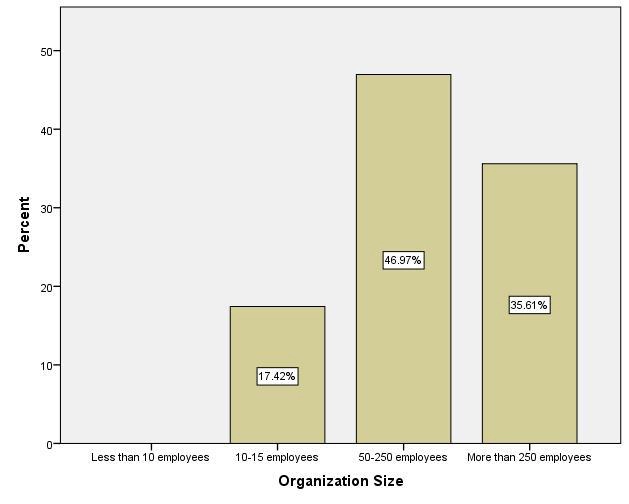
Figure 5.2-3- How many people are employed in the organization you work for?
A total of 46.97 percent of respondents indicated that they worked for companies that employed 50 to 250 employees. Also, 35.61 percent of employees indicated that they were employed by companies with more than 250 employees working for them.
Lastly, 17.42 percent of respondents indicated that the companies they worked for had 10-15 employees. Many respondents indicated that they worked for companies with more than 50 employees, indicating that the companies included in the study are small-to-medium businesses and large enterprises.
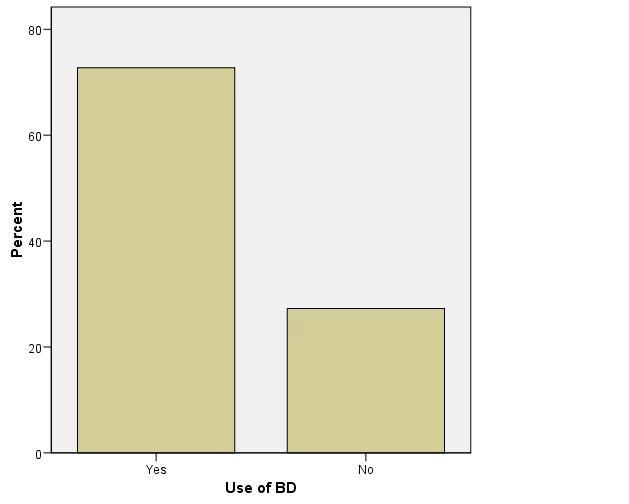
Figure 5.2-4 – Does your company use Big Data analytics?
Of the respondents participating, 72.73 percent indicated that their company was using Big Data analytics. This was crucial as it provided insight into the number of automobile companies with Big Data analytic systems.
As seen in Fig. 5.2.-5, only eighty-one (81) respondents from 132 had direct exposure to Big Data to either analyse, visualise, or make business decisions based on it. The pool of respondents was considerably smaller than anticipated.
Still, these slight details will provide greater insight into automobile companies’ workings regarding their use or integration of Big Data into the company. Based on the demographic analysis, participants who completed their survey had some access to Big Data analysis. But there is still a large group of people in these companies that do not have any exposure or access to Big Data.
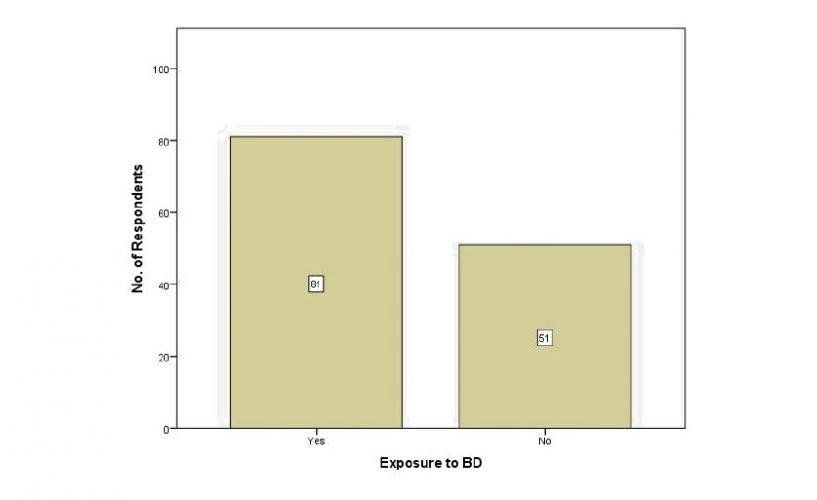
Figure 5.2-5 – Have you ever been exposed to using any form of Big Data in terms of analyzing it, visualizing it, or making decisions based on it?
Section B & C- Company Big Data Initiative and Impacts Results
The next section of the questionnaire, section B, aimed to analyse the respondent’s answers to identify the extent of integration or implementation of Big Data initiatives in the automobile company they worked for.
This section aims to understand the extent to which Big Data is present in automobile companies. This information can compare with the next team, which looks to understand and examine the effects of big data initiatives in the company.
Fig. 5.3-1 illustrates the main issues that may have caused the automobile company to implement Big Data initiatives. Based on the graph, it is concluded that analysing streaming data and data sets greater than 1 Terabyte (TB) were the greatest cause of initiating Big Data into the company, as per the response of 19.70 percent of respondents, respectively.
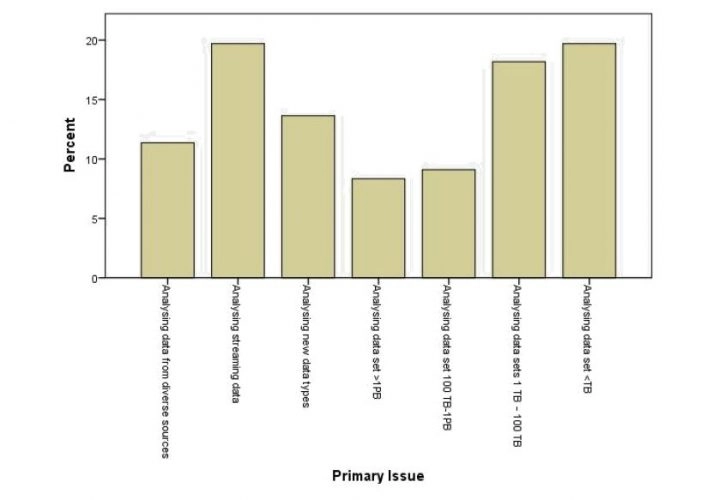
Figure 5.3-1- What were the organization’s primary data issues that led it to consider Big Data?
Another issue that instigated Big Data analytics in companies was analysing data sets from 1 TB to 100 TB, as indicated by 18.18 percent of respondents. Next in the rank was analysing new data types, which led to using Big Data analytics as indicated by 13.64 percent of respondents.
Fig. 5.3-2 illustrates the reaction of respondents to two questions.
- Questions 2. How would you rate the analytical abilities of the company you are employed in?
- Question 5. How would you rate the access to relevant, accurate, and timely data in your company today?
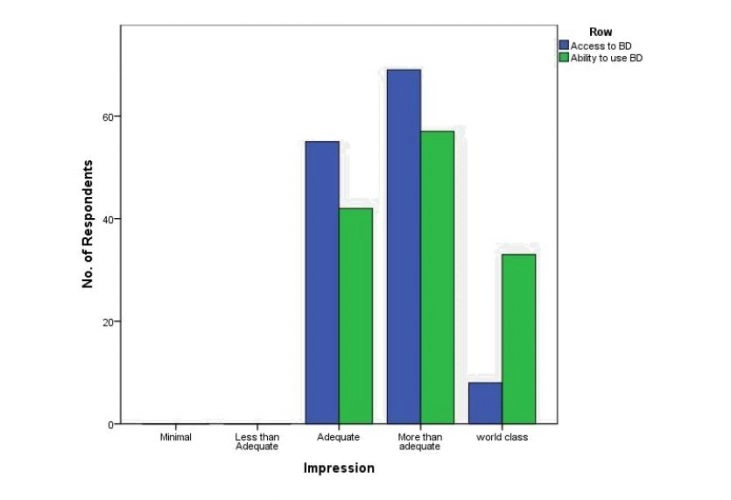
Figure 5.3-2 – How would you rate the analytical abilities of the company you are employed in? How would you rate the access to relevant, accurate, and timely data in your company today?
There is a strong correlation between access to Big Data and the analytical abilities of the company. Based on the illustration, 55 people who had access to Big Data thought the access was adequate, with 42 of them believing that its analytical ability was adequate. Furthermore, 69 participants indicated that access to Big Data was more than adequate, with 57 participants believing that the firm’s analytical ability was more than adequate. It can be concluded that the greater the access to Big Data, the adequate or more adequate the analytical abilities of the firm.
The next graph indicates the amount of spending that is placed on a Big Data initiative’s budget. Oftentimes, it was seen, as from the literature review, that funding Big Data analytics in a company allowed for greater business gains. Therefore, it was essential to understand the budget amount that was invested in Big Data initiatives.
A majority of respondents, about 47 percent, indicated that their company had a Big Data initiative budget of £1 million to GBP 10 million. Another 40 percent of respondents have indicated that their company spent £100,000 to GBP 1 million on their Big Data systems.
The amount of staff dedicated to Big Data analytics is also thought to play a part in advancing the goals that may be set for an automobile company regarding Big Data. The figure below takes two questions;
Questions 7- Approximately how many staff in your company are dedicated to analytics, modelling, data mining (not including routine reporting)? Question 8- Of these staff, are you mostly working in or for your consumer-facing (B2C) businesses, your commercial or wholesale (B2B) businesses, or both?
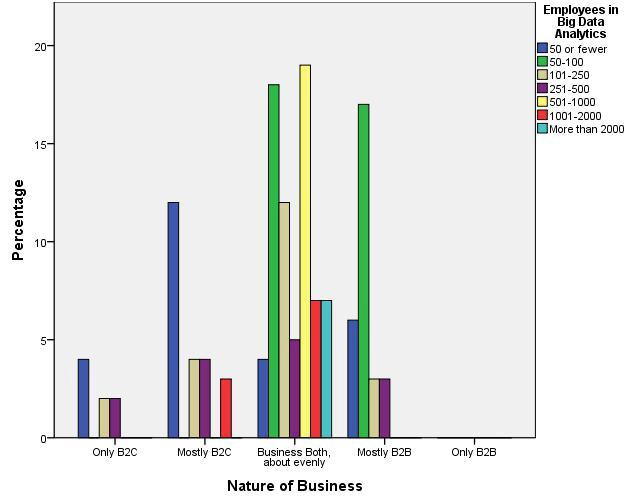
Figure 5.3-4 Approximately how many staff in your company are dedicated to analytics, modeling, data mining (not including routine reporting)? Of these staff, are you mostly working in or for your consumer-facing (B2C) businesses, your commercial or wholesale (B2B) businesses, or both?
Based on the illustration, nineteen (19) respondents indicated that 501-1000 employees are dedicated to B2B and B2C analytics. Using Big Data analytics for both B2B and B2C comprises the most agreement of respondents, with 72 of 132 indicated so.
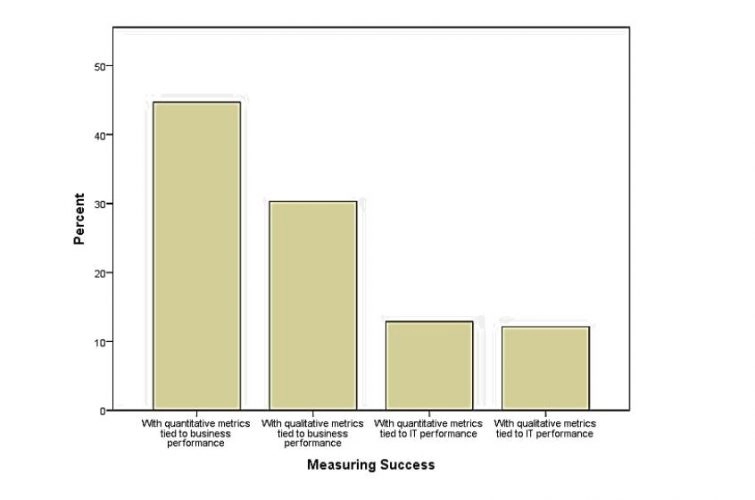
Figure 5.3-5 How does the company plan to measure the success of your Big Data initiatives?
The figure above represents the respondent’s answers to their automobile company’s plan for measuring Big Data’s success. Of the 132 participants, 44.70 percent responded that the company is planning on using quantitative metrics associated with business performance to analyse if Big Data is actually successful.
Another 30.30 percent indicated that their company was planning on using qualitative metrics tied to business performance. Using business performance to analyse Big Data’s success is coherent to the results of the literature review that indicated previous studies of doing such.
As an automobile company, they need to know the results of using Big Data analytics, and that is only by using business performance indicators regardless of being qualitative or quantitative.
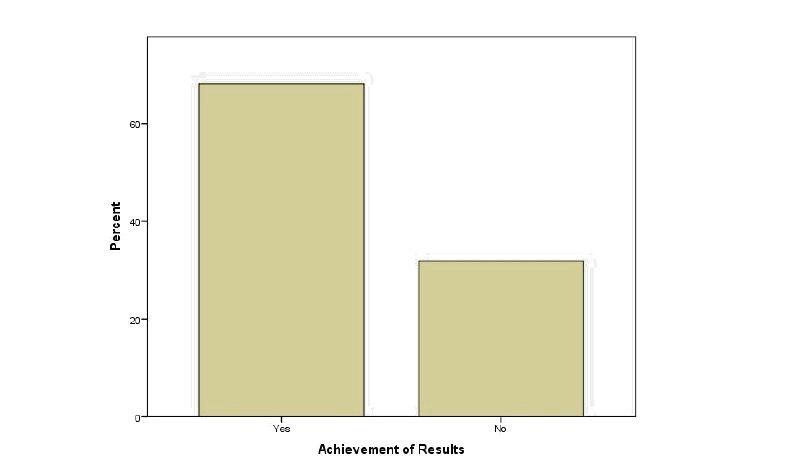
Figure 5.3-6 Has the company achieved measurable results from its investments in Big Data?
Fig. 5.3-6 portrays the response of participants regarding achieving measurable results from Big Data. According to 68.18percentt of the respondents,s the company they worked for did indeed show measurable results from their Big Data investments.
However, 31.82 percent indicated that there was indeed no measurable result in investing in Big Data. Based on these results and those presented in 5.3-2, the results support H5, which states that a company’s analytical abilities allow for measurable results.
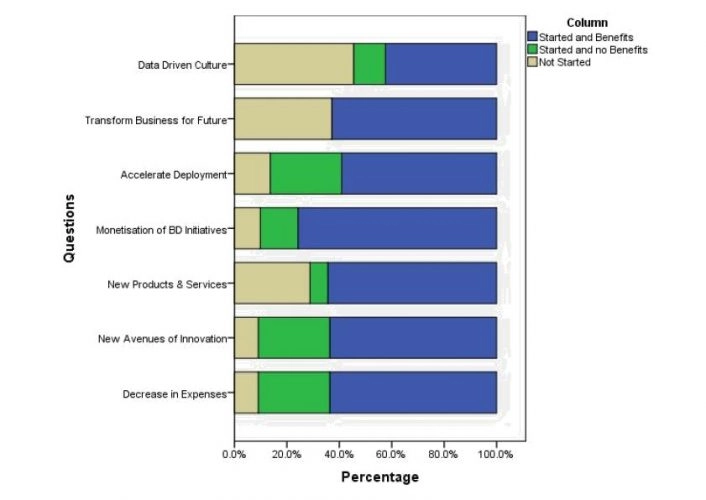
Figure 5.3-7: Impact of Big Data on Company
Fig. 5.3-7 presents the answers of respondents of the impact of Big Data on automobile companies. An estimate of 60% of participants indicated that Big Data initiatives had been started, and the company has benefited from a decrease in expenses.
This response, coupled with the responses seen in Fig. 5.3-3, supports hypothesis H2 that the greater company’s budget (>1million GBP) would decrease expenses.
Also, over 70% of respondents indicate that their companies had started and benefited from Big Data initiatives by monetising from the initiatives. These results, coupled with those presented in Fig. 5.3-3, supports H1, which suggested that larger investments (<1million GBP) would result in the company’s ability to monetise and generate new revenues.
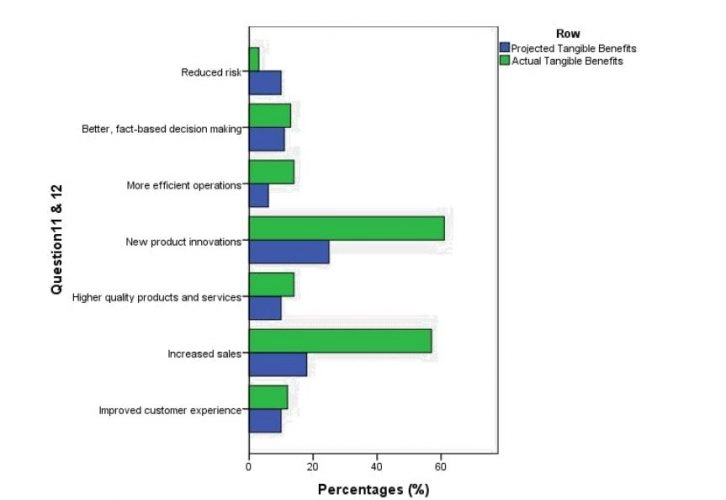
Figure 5.3-8: Questions 11 & 12 11. Since the Big Data initiatives implemented, what tangible benefits have been achieved in the company? What are the tangible benefits the company is aiming to achieve using Big Data initiatives?
Fig. 5.3-8 presents the actual and projected benefits of Big Data initiatives. Over 60% of respondents indicated that their automobile company had witnessed actual benefits in increasing sales and product innovations since their Big Data initiatives.
Other benefits that overcame project benefits include improved customer experience, higher quality products/services, efficient operations, and improved decision making. Coupled with the results from 5.3-3, the data support hypotheses H6 and H8.
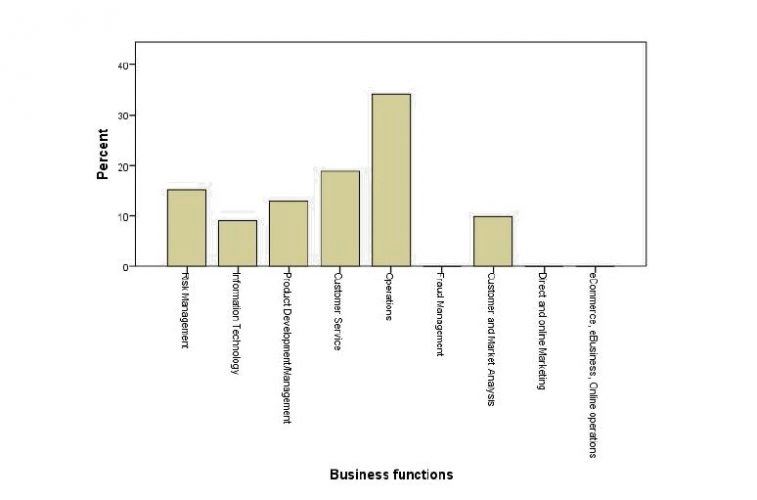
Figure 5.3-9: What business functions in the company are fueling Big Data initiatives?
Fig. 5.3-9 presents the results of question 13 in section C of the questionnaire. Respondents were asked which business function may be fueling the drive for Big Data initiatives.
The sample, 34.09%, indicated that operations were the main business function fueling Big Data in the company. After operations, the second-highest function is customer service, with 18.94% of respondents indicating this.
The business function thought to be the least influential in driving Big Data in automobile companies was Information Technology, with 9.09% indicating it.
The results of this partially support H7 because according to question 15 section C, 25% of respondents indicated that in the next five years, Big Data would impact and fundamentally change the way business is done in the organisation as opposed to 15.91% of respondents that indicated it would change the way the business will organise operations.
Based on the study results, hypotheses H1, H2, H5, H6, H8, and part of H7 have been supported. This leads to the conclusion that Big Data initiatives in automobile companies have had a significant impact on the company’s operations.
The companies have significantly benefited from increased sales, greater product innovations, improved customer care, and efficient decision-making. Greater investment of more than 1 billion GBP has led to better results obtained from Big Data initiatives.
If you need assistance with writing your dissertation, our professional dissertation writers are here to help!
Chapter 6: conclusion and discussion, research overview.
The current study aimed to analyze the impact that Big Data initiatives had on automobile companies in the UK, especially its operations. The current study was developed using a quantitative approach, which meant using philosophical assumptions from the positivist school of thought and producing a methodology that would follow deductive reasoning.
Under these assumptions, the quantitative approach was selected and used the survey instrument to gather data. This data was then analyzed using descriptive statistics to examine the results and link it to a set of proposed hypotheses.
The results presented conclude that investing more than 1 billion GBP on Big Data initiatives would provide greater tangible benefits for a business and positively impact the company.
The results also found that companies with greater analytical abilities on the adequate and above adequate range could see measurable results. In the end, Big Data did have a positive and large impact on the operations business function of automobile companies.
Meeting the Aim and Objectives of the Project
The research’s main aim was to investigate the impact of Big Data on the automobile industry, specifically in the UK, operations such as sales, customer retention, the manufacturing process, performance, marketing, logistics, and supply chain management.
The current study was able to accomplish this using the objectives. The study’s aims and objectives were supported by the revelation that the following hypotheses are supported by the results and analysis in Chapter 5.
H1- The greater the company’s budget for Big Data initiatives (More than 1 million GBP), the greater its ability to monetize and generate new revenues.
H2- The greater the company’s budget for Big Data initiatives (More than 1 million GBP), the greater decrease in expenses is found.
H5- The analytical abilities of a company allow for achieved measurable results.
H6- Investing in Big Data will lead to highly successful business results.
H7- A business’s operations function is fueling Big Data initiatives.
H8- The implementation of Big Data in the company has positive impacts on business.
Statement of Contributions and Research Novelty
Based on the literature review conducted in chapter 2, there is little to no academic research on Big Data’s impact on automobile companies. Due to this significant gap in research, the current study can contribute to literature using the insight provided by this study’s results.
The study analyzed how executives in automobile companies in the UK perceive the contributions made by Big Data in their companies. This insight can then be used to attract other researchers to study the phenomena. Big Data and its emergence in the current markets is fairly new, making the idea behind the current a novel idea.
Research Limitations
The research was severely limited due to the number of respondents being a lot less than those proposed; 300 respondents were needed; however, only 132 had completed the survey.
This may be because the survey was distributed online. This makes it difficult to tell how many people had seen the survey link but had not participated. The idea that the survey may have been too long, making respondents weary of answering the questions due to the great length of time it took to answer.
Due to the sample constraint, the results obtained from the current study cannot be generalized to the population sampled. It is recommended that other forms of distributing surveys be used to garner the maximum number of respondents.
There is also the inability of automobile companies to speak to researchers on the phone, which led to the drop in using interviews in the study. With interviews, a greater deal of insight can be brought to the results obtained from the survey. Complementing these would have made the results of the study more accurate and reliable.
Recommendations for Future Research
It is recommended that future studies take into account the loopholes of the current study. From the literature review, very little literature is available on the impact of Big Data on automobile companies.
Due to this lack, future researchers are encouraged to research this industry because drastic changes may result in increased use of Big Data. Future researchers are recommended to use a mixed-methods approach to obtaining and analyzing data.
With a mixed-methods approach, qualitative and quantitative data can complement each other to make assumptions stronger and test hypotheses in a highly effective manner.
Abusharekh, A., Stewart, S. A., Hashemian, N., Abidi, S. S. R. 2015. H-Drive: A big health data analytics platform for evidence-informed decision making. IEEE International Congress on Big Data, p. 416- 432.
Aihara, K., Imura, H., Takasu, A., Tanaka, Y., Adachi, J. 2014. Crowdsourced mobile sensing in smarter city life. IEEE 7th International Conference on Service-Oriented Computing and Applications, p. 334- 337.
Amelia, A., and Saptawati, G. A. P. 2014. Detection of potential traffic jams based on traffic characteristic data analysis. IEEE, p. 1- 5.
Bryman, A., Bell, E., 2015. Business Research Methods. Oxford University Press.
Cook, K., Grinstein, G., Whiting, M., Cooper, M., Having, P., Ligget, K., Nebesh, B., and Paul, C. L. 2012. VAST Challenge 2012: Visual Analytics for Big Data. IEEE Symposium on Visual Analytics Science and Technology 2012, p. 251- 257. Seattle, WA: Print.
Daum, P., 2013. International Synergy Management: A Strategic Approach for Raising Efficiencies in the Cross-border Interaction Process. Anchor Academic Publishing (aap_verlag).
Dümke, R., 2002. Corporate Reputation and its Importance for Business Success: A European Perspective and its Implication for Public Relations Consultancies. diplom.de.
Foy, H. 2014. UK’s resurgent car industry still faces challenges. Financial Times.
Guetterman, T.C., 2015. Descriptions of Sampling Practices Within Five Approaches to Qualitative Research in Education and the Health Sciences. Forum Qualitative Sozialforschung / Forum: Qualitative Social Research 16.
Haq, M., 2014. A Comparative Analysis of Qualitative and Quantitative Research Methods and a Justification for Adopting Mixed Methods in Social Research (PDF Download Available). ResearchGate 1–22. doi:http://dx.doi.org/10.13140/RG.2.1.1945.8640
Itoh, M., Yokoyama, D., Toyoda, M., and Tomita, Y. 2014. Visual fusion of Mega-City Big Data: An application to traffic and tweets data analysis of Metro passengers. IEEE International Conference on Big Data, p. 431-442.
Kerr, K., Hausman, B. L., Gad, S., and Javen, W. 2013. Visualization and rhetoric: key concerns for utilizing bid data in humanities research- A case study of vaccination discourse 1918-1919. IEEE International Conference on Big Data, p.25- 32.
Kelley, K., Clark, B., Brown, V., Sitzia, J., 2003. Good practice in the conduct and reporting of survey research. Int J Qual Health Care 15, 261–266. doi:10.1093/intqhc/mzg031.
Lee, J., Noh, G., Kim, C. K. 2014. Analysis & visualization of movie’s popularity and reviews. IEEE International Conference on Big Data, pp. 189-190.
Lewis, S., 2015. Qualitative Inquiry and Research Design: Choosing Among Five Approaches. Health Promotion Practice 16, 473–475. doi:10.1177/1524839915580941
Liu, D., Kitamura, Y., Zeng, X. 2015. Analysis and visualization of traffic conditions of the road network by route bus probe data. IEEE International Conference on Multimedia Big Data, p. 248-251.
Lorenzo, G. D., Sbodio, M. L., Calabrese, F., Berlongerio, M., Nair, R., and Pinelli, F. 2014. All aboard: Visual exploration of cellphone mobility data to optimize public transport. IUI Haifa, Israel, p. 335- 340. Print.
Monaghan, A. 2016. UK car manufacturing hits high but industry warns of Brexit effect. The Guardian. [online] < https://www.theguardian.com/business/2016/jul/28/uk-car-manufacturing-hits-high-industry-warns-brexit-effect >. [Accessed : 2017 March 2].
Pu, J., Liu, S., Qu, H., Ni, L. 2013. T-watcher: A new visual analytic system for effective traffic surveillance. IEEE 14th International Conference on Mobile Data Management, p. 127- 136.
Rysavy, S. J., Bromley, D., and Daggett, V. 2014. DIVE: A graph-based visual analytics framework for Big Data. Visual Analytics for Biological Data, IEEE Computer Graphics and Applications, p. 26-37.
Saunders, M., 2003. Research Methods for Business Students. Pearson Education India.
Saunders, M.N.K., Tosey, P., 2015. Handbook of Research Methods on Human Resource Development. Edward Elgar Publishing.
Shah, A. H., Gopalakrishnan, G., Rajendran, A., and Liebel, U. 2014. Data mining and sharing tool for high content screening large scale biological image data. IEEE International Conference on Big Data, p. 1068- 1076.
Steiger, E., Ellersiek, T., and Sipf, A. 2014. Explorative public transport flow analysis from uncertain social media data. SIGSPATAL, p. 1-7. Print.
Walker, R. 2015. From Big Data to Big Profits (1st ed.). Print.
Wallner, G., and Kriglstein, S. 2013. Visualization-based analysis of gameplay data- A review of the literature. Entertainment Computing, 4, pp. 143-155.
Wozniak, P., Valton, R., Fjeld, M. 2015. Volvo single view of vehicle: Building a Big Data service from scratch in the automotive industry. CHI: Crossings, Seoul, Korea, p. 671-678.
Xiao, S., Liu, C. X., Wang, Y. 2015. Data driven geospatial-enabled transportation platform for freeway performance. IEEE Intelligent Transportation Systems Magazine, p. 10-21.
Zhang, Z., Wang, S., Cao, G., Padmanabhan, A., and Wu, K. 2014. A scalable approach to extracting mobility patterns from social media data. National Science Foundation, p. 1-6. Print.
9 Appendix A- Survey
Please contact us to get access to the full Appendix Survey.
10 Appendix B- Raw Data
11 appendix c- responses job title x organization size.
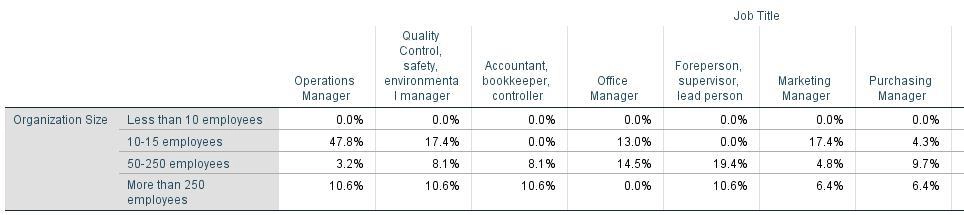
12 Appendix D- Responses Question 2, 5, & 6
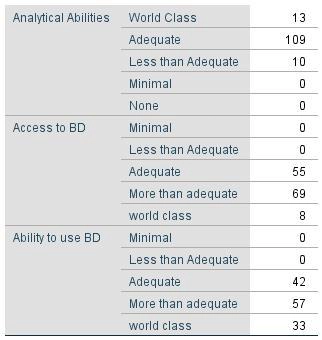
Frequently Asked Questions
How much time it takes to write a masters level full dissertation.
The time required to write a master’s level full dissertation varies, but it typically takes 6-12 months, depending on research complexity and individual pace.
USEFUL LINKS
LEARNING RESOURCES
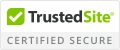
COMPANY DETAILS
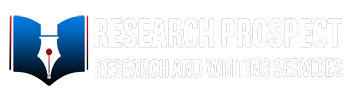
- How It Works
977 Dissertation Topics & Good Thesis Ideas
18 January 2024
last updated
Dissertation topics encapsulate the individual’s interests and passion while simultaneously making a noteworthy contribution to the respective field of study. Potential topics span a wide range of disciplines and interests, from an exploration of recent advancements in artificial intelligence to a comprehensive investigation into the ramifications of climate change on agricultural practices. Some subjects may entail a thorough examination of contemporary socio-political dynamics, an in-depth analysis of the psychological implications of social media usage, or a detailed study of the economic consequences of global trade policies. Literature scholars may choose to critique unconventional interpretations of literary works, while science-oriented individuals may prefer to investigate uncharted aspects of human genomics. In turn, the careful selection of a good dissertation topic can demonstrate an individual’s expertise, ignite intellectually stimulating dialogues, and pave the pathway for future academic and professional pursuits.
Best Topics for Thesis & Dissertation
- Cybersecurity Measures: Protecting Personal Data in the Digital Age
- Quantum Computing: Breakthroughs and Potential Applications
- Sustainable Agriculture: Innovations for Food Security
- Artificial Intelligence: Ethical Considerations in Decision-Making
- Mental Health Stigma: Strategies for Awareness and Acceptance
- Urban Planning: Revitalizing Spaces for Green Living
- Microplastics: Tracing Their Journey in Marine Ecosystems
- Climate Change: Mitigation Strategies in the 21st Century
- Cryptocurrency Regulation: Balancing Innovation and Security
- Alternative Energy: Harnessing the Power of Tidal Waves
- Women in STEM: Encouraging Participation and Leadership
- Blockchain Technology: Disrupting the Supply Chain Industry
- Dark Matter: Unveiling Cosmic Mysteries
- Virtual Reality: Enhancing Remote Education Experiences
- Gene Editing: Exploring the Ethics of CRISPR Technologies
- Space Tourism: Legal and Ethical Implications
- Autonomous Vehicles: Navigating Safety and Regulation Challenges
- Personalized Medicine: Tailoring Treatment Through Genomics
- Plastic Alternatives: Innovations in Biodegradable Materials
- Language Revitalization: Strategies for Preserving Endangered Languages

Easy Thesis Topics
- Influences of Social Media on Teenage Behavior
- Veganism and Its Effects on Health and Environment
- Digital Marketing Trends in the E-Commerce Industry
- Climate Change and Its Effects on Seasonal Migration of Birds
- Effectiveness of Online Learning During the Pandemic
- Artificial Sweeteners: A Study on Health Implications
- Cyberbullying: Strategies for Prevention and Education
- Immigration Policies: A Comparative Analysis Between Nations
- Recycling Programs: Assessing Effectiveness in Major Cities
- Animal-Assisted Therapy and Its Mental Health Benefits
- Genetically Modified Foods: Pros and Cons
- Music Therapy: The Impact on Stress Management
- Exploring the Psychological Impact of Unemployment
- Television’s Influence on Body Image Perception Among Adolescents
- Virtual Reality’s Role in Modern Physical Therapy
- Green Buildings: A Study on Energy Efficiency
- Video Games: Analyzing Their Effect on Cognitive Development
- Public Transportation Systems: A Case Study of Urban Development
- Solar Power: Assessing Viability for Residential Use
Interesting Thesis Topics
- Cryptocurrency: Future of Financial Transactions
- Dark Tourism: Motivations and Ethical Implications
- Autonomous Vehicles: An Exploration Into Safety Concerns
- Robotic Surgery: Advancements and Challenges
- Quantum Computing: Potential Effects on Cybersecurity
- Space Tourism: Feasibility and Future Prospects
- Neuroplasticity: The Effects of Mindfulness and Meditation
- Food Security in Climate Change Scenario
- Alternative Learning Systems in Special Education
- Mental Health Benefits of Urban Green Spaces
- Integration of AI in Customer Service
- Fusion Energy: Potential and Challenges
- Underwater Archaeology: Discoveries and Controversies
- Exoplanets and the Possibility of Life
- Microplastics in Aquatic Ecosystems
- Language Acquisition in Bilingual Children
- Fashion Industry and Sustainability Practices
- Music’s Influence on Exercise Performance
- Tiny Homes: A Solution for Housing Crisis?
- Biohacking: Ethical Implications and Health Risks
Dissertation Topics & Ideas
- Gene Editing: Ethical Boundaries in Modern Science
- Metamaterials: An In-Depth Study on Invisibility Cloaking
- Climate Refugees: Assessing Global Preparedness
- Cybernetics in Prosthetics: A Study of User Experience
- Epigenetics and Aging: Potential Interventions
- Dark Matter: An Examination of Detection Techniques
- Artificial Intelligence in Precision Medicine
- Virtual Reality in Post-Traumatic Stress Disorder Therapy
- Biodegradable Plastics: A Solution to Pollution?
- Cryptocurrency Regulations and Economic Impact
- Quantum Cryptography: Future of Secure Communication
- Blockchain Applications Beyond Finance
- Advanced Study on Carbon Capture Technologies
- Mars Colonization: Ethical and Logistical Challenges
- Nano-Pharmaceuticals: Potential in Targeted Drug Delivery
- Study on Renewable Energy Storage Technologies
- Advanced Wireless Communication: 6G and Beyond
- Examining Food Waste Reduction Strategies
- Circular Economy: A Sustainable Approach for Industries
- Machine Learning Algorithms in Weather Forecasting
Education Dissertation Topics
- Fostering Emotional Intelligence in Primary Education
- Gamification: An Effective Approach in Higher Learning?
- Teacher Retention: Unraveling the Causes and Solutions
- Assessing the Outcomes of Blended Learning Approaches
- Critical Thinking Skills: Integration Strategies in Curriculum
- Standardized Testing: An Evaluation of Benefits and Drawbacks
- Mindfulness in Education: Potential for Enhancing Student Focus
- Language Learning Strategies for Bilingual Students
- Challenges and Opportunities in Remote Learning
- Early Childhood Education: Innovative Approaches and Outcomes
- Special Education Inclusion: A Study on Best Practices
- Student Motivation: Unravelling the Role of Parental Engagement
- Cultural Sensitivity: Inclusion Strategies in Diverse Classrooms
- STEM Education: Addressing Gender Disparity
- Exploring the Impact of Art Education on Cognitive Development
- Holistic Development: Role of Sports in Education
- Student Stress: The Role of Academic Pressure
- Coping Strategies for Students With Learning Disabilities
- Unpacking the Effect of Social Media on Academic Performance
Business Dissertation Topics
- Consumer Decision Making: The Power of Branding
- Sustainable Business Practices: An Evaluation of Success Factors
- E-Commerce Trends: A Forecast for the Post-Pandemic World
- Business Ethics in the Tech Industry: A Study on Data Privacy
- Leadership Styles: Their Influence on Employee Retention
- Artificial Intelligence in Customer Service: Opportunities and Challenges
- Small Businesses and Local Economies: Interplay and Outcomes
- Diversity in Corporate Boards: A Study on Performance Outcomes
- Cryptocurrency: Disrupting Traditional Business Operations
- Green Marketing: Consumer Perception and Behavior
- Workplace Culture: Its Influence on Employee Satisfaction
- Strategic Alliances: Risks and Rewards in Global Business
- Corporate Social Responsibility: Perception and Influence on Consumers
- Economic Recession: Survival Strategies for Small Businesses
- Supply Chain Management: Modern Challenges and Solutions
- Employee Training Programs: Effectiveness and Outcomes
- Crowdfunding: Its Influence on Entrepreneurship
- Organizational Change: Leadership Tactics for Smooth Transition
- Business Innovation: Strategies for Staying Ahead in a Competitive Market
- Startups: Examining the Success Factors and Pitfalls
Law Dissertation Topics for Ph.D. Students
- Digital Privacy Laws: Global Comparisons and Contrasts
- Hate Speech Regulations: Balancing Free Speech and Public Safety
- Intellectual Property Rights: Challenges in the Digital Age
- Environmental Laws: Evaluating Enforcement Mechanisms
- Immigration Policies: Human Rights Perspective
- Cybercrime Legislation: Addressing Modern Challenges
- Child Custody Laws: Analyzing Best Interests Standards
- International Law: Effectiveness in Preventing Armed Conflicts
- Patent Law: Relevance in Technological Advancements
- Juvenile Justice System: Evaluating Rehabilitation Efforts
- Healthcare Laws: Disparities in Access and Quality
- Bankruptcy Laws: Protection for Small Businesses
- Family Law: The Dynamics of Same-Sex Marriage Legislation
- Human Trafficking: International Laws and Their Implementation
- Gun Control Laws: Analyzing Effectiveness in Crime Prevention
- Tort Law: The Question of Medical Malpractice
- Labor Laws: Protection for Gig Economy Workers
- Whistleblower Protections: Assessing Laws and Outcomes
- Animal Rights: Legal Perspectives and Implications
Psychology Dissertation Topics
- Cognitive Therapy: Dealing With Childhood Trauma
- Emotional Intelligence: Its Influence on Workplace Success
- Behavioral Psychology: Exploring Aggression Triggers
- Human Perception: The Effects of Virtual Reality on the Mind
- Clinical Psychology: Efficacy of Mindfulness-Based Treatments
- Cultural Factors: Their Contribution to Depression
- Psychology of Language: Cognitive Processes Behind Bilingualism
- Stress Management: Investigating the Power of Music Therapy
- Social Psychology: Conformity and Rebellion in Adolescents
- Psychoanalysis: Unraveling Dreams and Their Meanings
- Mental Health: Exploring Resilience in Trauma Survivors
- Eating Disorders: Investigating Body Image Perception
- Neurological Psychology: Understanding Memory Loss Mechanisms
- Positive Psychology: Happiness and Its Determinants
- Child Development: Analyzing Effects of Parenting Styles
- Forensic Psychology: Studying Criminal Minds and Behaviors
- Educational Psychology: Learning Difficulties and Strategies for Overcoming Them
- Personality Psychology: Impact of Social Media on Self-Image
- Health Psychology: Assessing Lifestyle Changes on Mental Health
- Counseling Psychology: Effectiveness of Online Therapy Sessions
Nursing Dissertation Topics
- Patient Safety: Measures to Minimize Medical Errors
- Palliative Care: Strategies for Effective Pain Management
- Mental Health Nursing: Approaches to Dealing With Suicidal Patients
- Nursing Leadership: Exploring Nurse-Led Clinical Decision Making
- Geriatric Nursing: Challenges in Caring for the Aging Population
- Child Health: Improving Pediatric Care in Emergency Departments
- Public Health Nursing: Tackling Health Inequalities in Urban Areas
- Oncology Nursing: Emotional Support Strategies for Cancer Patients
- Maternity Care: Best Practices in Prenatal Nursing
- Community Health: Examining Home Visit Programs for New Mothers
- Pediatric Nursing: Strategies for Managing Childhood Obesity
- Critical Care Nursing: Handling Moral Distress Among Nurses
- Neonatal Care: Technological Advancements in Premature Baby Nursing
- Nursing Ethics: Balancing Patient Autonomy and Care Obligations
- Holistic Nursing: Evaluating the Effectiveness of Complementary Therapies
- Cardiac Nursing: Prevention Strategies for Heart Disease
- Diabetic Care: Innovative Nursing Approaches to Patient Education
- Nursing Education: Exploring Simulation in Training for Complex Procedures
- Hospice Care: Investigating the Role of Nurses in End-of-Life Decisions
Marketing Dissertation Topics
- Digital Marketing Trends: Implications for Consumer Behavior
- Green Marketing: Investigating Its Influence on Sustainable Consumption
- Neuromarketing: The Science Behind Consumer Decision-Making
- Social Media Marketing: Examining the Power of Influencer Endorsements
- Emotional Branding: How Companies Foster Consumer Connections
- Content Marketing: Strategies for Boosting Online Engagement
- Ethical Marketing: Exploring Its Effect on Corporate Reputation
- Mobile Marketing: Enhancing User Experience for Higher Conversion Rates
- Celebrity Endorsements: Unpacking Their Effect on Brand Loyalty
- Customer Relationship Management: Nurturing Long-Term Consumer Bonds
- Brand Storytelling: A Narrative Approach to Marketing Communication
- B2B Marketing: Understanding Decision-Making in Corporate Purchasing
- Viral Marketing: Techniques for Maximum Social Media Exposure
- Affiliate Marketing: Analyzing Its Profitability in the E-Commerce Sphere
- Product Placement: Its Persuasiveness in Film and Television Media
- Experiential Marketing: Designing Memorable Brand Encounters
- Retail Marketing: Personalization Techniques in Brick-and-Mortar Stores
- Data-Driven Marketing: Leveraging Big Data for Personalized Marketing
- Fashion Marketing: Successful Strategies for Luxury Brands
- Sustainable Marketing: Balancing Profitability With Ecological Responsibility
History Dissertation Topics
- Colonial Narratives: Reinterpreting Spanish Conquests in Latin America
- Silent Heroes: Unveiling Women Warriors in Ancient Civilizations
- Architectural Wonders: Decoding the Construction Techniques of the Egyptian Pyramids
- Power Symbols: Analyzing Iconography in Byzantine Art
- Political Rhetoric: Dissecting Oratory Techniques of Roman Emperors
- Silk Road: Unraveling the Complex Trade Networks of Ancient Eurasia
- War Tactics: Examining Strategies Used in the Hundred Years’ War
- Cultural Exchange: Exploring Islamic Influence on Medieval European Architecture
- Diplomatic Maneuvers: Investigating the Treaty of Tordesillas
- Religious Reform: Understanding the Causes and Consequences of the Great Schism
- Plague Narratives: Chronicling the Black Death and Its Societal Aftermath
- Maritime Innovation: Assessing Technological Advancements During the Age of Discovery
- Indigenous Perspectives: Re-Evaluating European Colonization From Native American Viewpoints
- Feudal Dynamics: Evaluating the Power Structures in Medieval Japan
- Globalization Pioneers: Assessing the Influence of Dutch Trade Empires
- Chivalry Codes: Deconstructing Knighthood Rituals and Ideals in the Middle Ages
- Renaissance Art: Tracing the Shift From Religious to Humanist Themes
- Industrial Revolution: Investigating the Technological Progress in the 18th Century
- Historic Epidemics: Comparing the Spanish Flu and the Bubonic Plague
- Protestant Reformation: Assessing Its Impact on European Political Landscape
Dissertation Topics in Management
- Remote Work: Navigating the Challenges of Virtual Team Leadership
- Organizational Resilience: Strategies for Thriving in a VUCA World
- Business Ethics: Managing Corporate Responsibility in the Era of Globalization
- Innovation Management: Unlocking Creativity in Traditional Organizations
- Knowledge Management: Optimizing Intellectual Capital in Tech Industries
- Workplace Culture: Influencing Employee Satisfaction and Retention
- Sustainable Business: Implementing Green Practices in Manufacturing Sectors
- Crisis Leadership: Devising Effective Response Plans to Unexpected Events
- Diversity and Inclusion: Cultivating a Multicultural Work Environment
- Artificial Intelligence: Integrating AI Into Human-Centric Business Models
- Conflict Resolution: Mediating Interpersonal Disputes in Corporate Settings
- Agile Methodologies: Adapting to Rapid Change in Project Management
- Digital Transformation: Steering Organizational Change in the Information Age
- Employee Wellness: Investigating the Link Between Well-Being and Productivity
- Supply Chain Management: Mitigating Risks in International Logistics
- Strategic Planning: Aligning Long-Term Goals With Operational Objectives
- Change Management: Overcoming Resistance to Organizational Reforms
- Human Resource Management: Exploring the Effects of Remote Hiring Practices
- Data-Driven Decision Making: Incorporating Big Data in Management Strategies
Qualitative Dissertation: Ideas for Proposals
- Interpretive Phenomenology: Understanding Patients’ Experience With Chronic Pain
- Digital Ethnography: Exploring Social Media Behaviors Among Teenagers
- Narrative Inquiry: War Veterans and Their Battle With PTSD
- Grounded Theory: Examining Resilience Among Single Parents
- Action Research: Implementing Anti-Bullying Programs in Elementary Schools
- Case Study: A Closer Look at Successful Women Entrepreneurs
- Discourse Analysis: Examining Political Rhetoric in Recent Election Campaigns
- Feminist Methodology: Perceptions and Experiences of Women in STEM Fields
- Phenomenography: Exploring Different Ways People Understand Climate Change
- Longitudinal Study: Tracking Career Progression in the Gig Economy
- Ethnomethodology: Everyday Practices Among a Religious Community
- Symbolic Interactionism: Identity Construction in Online Gaming Communities
- Autoethnography: A Personal Narrative on Migration and Cultural Identity
- Hermeneutics: Interpreting Ancient Texts in a Modern Context
- Historical Analysis: Re-Evaluating Major Revolutions From a Social Perspective
- Ethnography: Assessing Cultural Practices of Remote Indigenous Tribes
- Participant Observation: A Deep Dive Into College Student Life
- Field Research: Insights Into Behavioral Patterns of Endangered Species
- Content Analysis: Investigating Gender Stereotypes in Children’s Literature
- Conversation Analysis: Studying Communication Patterns in Virtual Team Meetings
Quantitative Dissertation Proposal Topics
- Statistical Correlation: Cybersecurity Breaches and Business Performance
- Factor Analysis: Key Elements Influencing Consumer Buying Behavior
- Regression Analysis: Predicting Property Prices in Metropolitan Areas
- Logistic Regression: Determining Factors Affecting Voter Turnout
- Analysis of Variance (ANOVA): Studying Teacher Effectiveness Across Different Education Systems
- Time Series Analysis: Examining Fluctuations in Cryptocurrency Values
- Path Analysis: Assessing the Mediating Factors of Workplace Stress
- Chi-Square Test: Investigating Race and Employment Opportunities
- Data Envelopment Analysis: Evaluating Efficiency in Healthcare Delivery
- T-Test Analysis: Comparing Mental Health Outcomes of Different Therapeutic Interventions
- Hierarchical Linear Modeling: Understanding Student Academic Performance in Multi-Level Education Systems
- Discriminant Analysis: Predicting Corporate Bankruptcy
- Survival Analysis: Identifying Key Factors Impacting Patient Survival Rates in Oncology
- Cluster Analysis: Unveiling Customer Segmentation in the E-Commerce Industry
- Canonical Correlation: Understanding Interrelationships Between Sets of Multiple Economic Indicators
- Structural Equation Modeling: Testing the Validity of Theoretical Models in Social Psychology
- Multivariate Analysis: Profiling Smartphone User Behavior
- Non-Parametric Test: Measuring the Effectiveness of Non-Traditional Teaching Methods
- Multiple Regression: Evaluating the Impact of Socioeconomic Status on Student Success
- Analysis of Covariance (ANCOVA): Comparing Weight Loss Programs While Controlling for Age and Gender
Dissertation Topics in Educational Leadership
- Transformational Leadership: Effects on Student Achievement
- Charismatic Educational Leadership and Its Influence on Teacher Morale
- Distributed Leadership in Schools: An Analysis of Effectiveness
- Principal Leadership Styles and Their Effect on School Climate
- School Leadership: Its Influence on Parental Engagement
- Ethical Leadership in Education: Ensuring Equity and Inclusion
- Instructional Leadership: Its Effect on Curriculum Implementation
- Efficacy of Servant Leadership in Promoting Teacher Retention
- School Leaders: Their Influence on Students’ Career Aspirations
- Succession Planning in School Leadership: Strategies and Implications
- Leadership Development Programs: Their Impact on Educational Leaders
- Emotional Intelligence in Educational Leadership: A Crucial Factor?
- Female Leadership in Education: Challenges and Opportunities
- Culturally Responsive Leadership: Improving Multicultural Education
- Leadership in Special Education: Navigating Unique Challenges
- Transformation of School Culture Through Effective Leadership
- Application of Adaptive Leadership in Higher Education
- Leadership and School Safety: An Uncharted Territory
- Principal Mentoring Programs: An Examination of Their Impact
Environmental Science Dissertation Topics
- Climate Change: Evaluating the Effectiveness of Mitigation Strategies
- Marine Biodiversity: Exploring Conservation Approaches
- Sustainable Agriculture: Innovations for Lowering Carbon Footprints
- Green Energy Transition: Policies and Their Efficacy
- Biomimicry: Unraveling Nature’s Sustainable Design Principles
- Urban Ecology: Delving Into City-Based Ecosystems
- Deforestation: Analyzing Long-Term Effects on Local Climates
- Environmental Toxicology: Assessing Chemical Impact on Wildlife
- Ecosystem Services: Valuation and Its Socioeconomic Influence
- Coral Reef Resilience: Strategies for Adaptation to Climate Change
- Plastic Pollution: Solutions for Oceanic Microplastics Issue
- Geoengineering: Assessing Potential Climate Change Solutions
- Permaculture Design: Evaluating Its Role in Sustainable Living
- Endangered Species: Genetic Conservation Approaches
- Wetland Conservation: Assessing the Impact on Water Quality
- Air Quality: Analyzing the Effect of Urban Green Spaces
- Soil Health: Impact of Organic Farming Practices
- Water Resource Management: Strategies for Drought Prone Areas
- Ecological Footprint: Measures to Reduce Resource Consumption
- Invasive Species: Implications for Biodiversity Loss
Health and Social Care Dissertation Topics
- Patient Experience: Understanding Perception and Satisfaction in Healthcare
- Obesity Prevention: Evaluating Community-Based Initiatives
- Elder Care: Innovations in Dementia Support Strategies
- Mental Health: Assessing the Effectiveness of Mindfulness Interventions
- Social Determinants: Exploring Their Influence on Health Disparities
- Telemedicine: Unraveling Challenges and Opportunities in Rural Healthcare
- Health Literacy: Measuring Its Effect on Patient Outcomes
- End-of-Life Care: Ethical Considerations in Assisted Dying
- Childhood Immunization: Assessing Parental Resistance
- Substance Abuse: Effectiveness of Community Support Programs
- Postnatal Depression: Interventions for Better Maternal Health
- Healthcare Inequity: Socioeconomic Factors and Policy Recommendations
- Digital Health: Patient Data Privacy and Security Challenges
- Adolescent Mental Health: Early Intervention Strategies
- Physical Disabilities: Accessibility Challenges in Healthcare Facilities
- Health Promotion: Evaluating School-Based Nutrition Programs
- HIV/AIDS Prevention: Addressing Stigma and Discrimination
- Healthcare Workforce: Exploring Burnout and Its Consequences
- Public Health: The Effect of Climate Change on Infectious Diseases
Engineering Dissertation Topics
- Biomedical Engineering: Tissue Engineering Techniques for Organ Replication
- Renewable Energy: Designing Efficient Wind Turbine Blades
- Software Engineering: Agile Methodology and Rapid Application Development
- Chemical Engineering: Sustainable Methods for Plastic Degradation
- Aerospace Engineering: Exploring Lightweight Materials for Aircraft Construction
- Structural Engineering: Seismic Resistant Design of Buildings
- Civil Engineering: Advancements in Smart Road Technology
- Materials Science: Graphene and Its Potential Applications
- Mechanical Engineering: Robotics in Automated Manufacturing
- Electrical Engineering: Quantum Computing and Its Future Implications
- Environmental Engineering: Technologies for Wastewater Treatment
- Computer Science: Cybersecurity Measures in Cloud Computing
- Robotics: Ethical Considerations in Autonomous Systems
- Nanotechnology: Developments in Drug Delivery Systems
- Automotive Engineering: Electric Vehicle Battery Efficiency
- Telecommunication: 5G and Potential Health Concerns
- Geotechnical Engineering: Soil Liquefaction during Earthquakes
- Bioengineering: Wearable Devices for Monitoring Vital Signs
- Nuclear Engineering: Safety Measures in Nuclear Reactor Design
- Industrial Engineering: Optimization Techniques in Supply Chain Management
International Relations Dissertation Topics
- Understanding Trade Wars: A Case Study on U.S. and China Relations
- Cyber Diplomacy: Analyzing Its Influence in Modern International Politics
- Rise of Soft Power: Bollywood’s Effect on India’s Global Image
- Climate Change Agreements: An Assessment of Compliance and Enforcement
- Global Human Trafficking: Unraveling the Geopolitical Underpinnings
- Peacekeeping Missions: United Nations Interventions in African Conflicts
- Brexit’s Aftershock: Disentangling European Union’s Future Prospects
- Rethinking Terrorism: Case Study on the Islamic State’s Ideology
- China’s Belt and Road Initiative: Implications for Global Trade Dynamics
- Digital Divides: Internet Access Disparities in Developing Nations
- Crisis Management: Nuclear Proliferation in North Korea
- The Resurgence of Populism: Effects on Transatlantic Relations
- Foreign Aid Effectiveness: Evaluation in Sub-Saharan Africa
- Global Health Governance: Deciphering the Response to the COVID-19 Pandemic
- Petrodollar System: Its Influence on Middle East-US Relations
- Post-Conflict Reconstruction: Challenges in Iraq and Afghanistan
- Transnational Corporations: Assessing Influence on Host Country Policies
- Rise of Non-State Actors: Effect on Global Security Landscape
- Post-Soviet Transition: Studying the Transformation in Ukraine
Finance Dissertation Topics
- Cryptocurrency Boom: Analyzing Market Volatility
- Mobile Banking Revolution: Case Study of Developing Economies
- Sustainable Investment Strategies: Exploring Green Bonds
- Behavioral Finance: Cognitive Biases in Investment Decision Making
- Microfinance Effectiveness: An Analysis of Poverty Alleviation in Sub-Saharan Africa
- Deconstructing Financial Crises: The 2008 Global Meltdown
- Venture Capital Influence: A Study on Startup Ecosystem
- Digital Payment Systems: Security Issues and Challenges
- Financial Derivatives: Risk Management Strategies in the Banking Sector
- Financial Inclusion: Investigating the Role of FinTech
- AI in Banking: Efficiency Evaluation in Credit Scoring
- Corporate Social Responsibility: Its Effect on Shareholder Value
- Economic Downturn: Assessing Resilience of Small and Medium Enterprises
- Debt Restructuring: Case Study on Greek Financial Crisis
- Regulatory Sandbox: Unpacking Financial Innovation and Regulation
- Economic Value Added: Relevance in Corporate Financial Management
- Bitcoin Adoption: Unveiling Motives and Barriers
- Corporate Governance: Impact on Financial Performance in Emerging Markets
- Green Financing: Unlocking Private Sector Participation
- Crowdfunding Success: Determinants in the Technology Sector
Media and Communication Dissertation Topics
- Digital Diplomacy: Social Media Influence on International Relations
- Post-Truth Politics: Media’s Influence on Democracy
- Evaluating Internet Censorship in Authoritarian Regimes
- Feminism in Advertising: Deconstructing Stereotypes
- Decoding Media Framing: Case Study of Climate Change
- Instagram Culture: A Study on Body Image Perception
- Artificial Intelligence in Journalism: Risks and Opportunities
- Augmented Reality in Advertising: Consumer Engagement Analysis
- Critical Discourse Analysis of LGBTQ+ Representation in Media
- YouTube Vlogging Phenomenon: A Cultural Shift in Media Consumption
- Children’s Interaction With Digital Media: Parental Mediation Practices
- Investigating Crisis Communication in Social Media Era
- Internet Memes and Political Satire: An Analytical Approach
- Media Literacy Education: Approaches and Effectiveness
- Social Media and Citizen Journalism: A New Era of Reporting
- Media’s Role in Shaping Immigration Narratives
- Digital Activism: Case Study of the #MeToo Movement
- Impact of Streaming Services on Traditional Television Broadcasting
- Analyzing Fake News Spread on Social Media Platforms
- Mobile Gaming and Its Cultural Implications
Information Technology Dissertation Topics
- Blockchain Technology: An Exploration of Cryptocurrency Security
- Artificial Intelligence in Healthcare: Potential and Challenges
- Big Data Analytics: Addressing Privacy Concerns
- Cybersecurity in Cloud Computing: Risk Mitigation Strategies
- Machine Learning Algorithms for Predictive Maintenance
- Green IT: Strategies for Energy-Efficient Data Centers
- Internet of Things (IoT) in Smart Cities: A Study on Security
- Quantum Computing: Implications for Cryptography
- Virtual Reality Applications in Education: Efficacy and User Experience
- Investigating Ethical Challenges in AI and Machine Learning
- Digital Forensics: Modern Techniques in Cybercrime Investigation
- Social Networking Sites: An Analysis of User Privacy Awareness
- Adaptive User Interfaces: Improving User Experience With AI
- Internet Governance: Balancing Regulation and Freedom
- Edge Computing: An Approach to Improve IoT Performance
- Study of Advanced Algorithms for Real-Time Data Processing
- User Behavior Analysis for Cybersecurity in E-Commerce
- Assessing the Vulnerabilities in the Internet of Medical Things (IoMT)
- Application of Machine Learning in Cyber Threat Detection
Sports Science Dissertation Topics
- Biomechanics and Energy Efficiency in Competitive Swimming
- Strength Training Regimens for Endurance Athletes: A Comparative Study
- Youth Football Training and Injury Prevention Strategies
- Influence of Mindfulness Training on Athletes’ Performance
- Effects of Altitude Training on Endurance Sport Performance
- Analyzing Nutritional Strategies for Recovery in Elite Athletes
- Influence of Sleep Quality on Athletic Performance and Recovery
- Assessment of Hydration Strategies in High-Performance Athletics
- Exercise and Mental Health: A Comparative Analysis in Different Age Groups
- Sport and Society: Exploring the Social Impact of Major Sporting Events
- Investigation Into the Use of Technology in Enhancing Athletic Performance
- Analysis of Female Representation in Sports Leadership Positions
- Socio-Psychological Factors Affecting Team Cohesion in Professional Sports
- Comparative Study on Training Regimes for Different Climbing Disciplines
- Doping in Professional Sports: Ethical, Legal, and Medical Perspectives
- Concussion Management in Contact Sports: An Evaluative Study
- Effects of Different Yoga Practices on Flexibility and Balance in Athletes
- Use of Virtual Reality for Training in Precision Sports
- Investigation Into Injury Rates in CrossFit Participants
- Effect of Cold Exposure on Muscle Recovery and Performance
Music Dissertation Topics
- Sonic Exploration: Understanding Ambient Music in the Digital Age
- Analysis of Western Influence on Japanese Popular Music
- Indigenous Music Traditions and Their Preservation in Modern Context
- Influence of Music on Cognitive Development: A Neuroscientific Perspective
- Digitization and Its Effects on the Preservation of Classical Music
- Harmonic Complexity in Late Twentieth-Century Jazz
- Psychoacoustic Effects of Dissonance in Contemporary Music
- Historical Analysis of Protest Songs and Their Cultural Significance
- Music Therapy for Stress Reduction: An Evidence-Based Study
- Influence of Music Streaming Platforms on Independent Musicians
- Exploration of Synesthesia and Its Implications for Music Composition
- Gender Representation in Opera: A Critical Analysis
- Comparative Analysis of Baroque and Classical Orchestration Techniques
- Investigation Into the Adaptation of Folk Tunes in Modern Composition
- Music and Spirituality: A Study of Sacred Music in Different Cultures
- Exploring the Impact of AI on Music Composition
- Music and Identity: The Role of Hip Hop in Social Movements
- Technological Advancements and Their Influence on Electronic Music Production
- Music Education and Its Effect on Mathematical Proficiency
- Use of Music in Healthcare Settings: An Interdisciplinary Approach
Philosophy Dissertation Topics
- Unearthing the Metaphysics of Time: A Qualitative Analysis
- Understanding Human Morality: Quantitative Approaches
- Platonic Ethics: A Qualitative Investigation
- Quantitative Aspects of Aesthetic Judgement
- Analyzing Freedom of Will: A Qualitative Examination
- Kantian Philosophy and Moral Responsibility: A Quantitative Study
- Qualitative Study of Personal Identity and Consciousness
- The Problem of Induction: Quantitative Insights
- Emotions in Stoic Philosophy: A Qualitative Analysis
- Materialism Versus Dualism: A Quantitative Study
- Qualitative Examination of Neoplatonism and Its Influence
- Quantitative Study on Ethical Dilemmas in Virtue Ethics
- Analyzing Eastern and Western Philosophy: A Qualitative Approach
- Understanding Determinism and Free Will: A Quantitative Study
- Existentialist Thoughts on the Meaning of Life: A Qualitative Analysis
- Analyzing Solipsism: Quantitative Perspectives
- Feminist Philosophy: A Qualitative Study
- Quantitative Analysis of Logic and Rationality in Philosophy
- Exploring Philosophical Themes in Science Fiction: A Qualitative Approach
Public Administration Dissertation Topics
- Accountability Measures in Government Agencies
- Optimizing Public Sector Efficiency: A Quantitative Analysis
- Exploring Participatory Governance: A Qualitative Study
- Decentralization and Its Effects on Public Services
- Analyzing Gender Equality in the Public Sector
- Qualitative Examination of Leadership Styles in Public Administration
- Quantitative Metrics for Evaluating Public Procurement Processes
- Public Policy and Environmental Sustainability: A Qualitative Approach
- Assessing E-Government Initiatives: A Quantitative Study
- Public Health Policy: Qualitative Case Studies
- Quantitative Analysis of Human Resource Management in the Public Sector
- Ethics and Transparency in Government: A Qualitative Investigation
- Organizational Culture in Public Sector: An In-Depth Qualitative Analysis
- Public Education Policies: A Quantitative Evaluation
- Crisis Management in Public Sector: A Qualitative Study
- Studying Innovation in Public Service Delivery Using Quantitative Data
- The Efficiency of Inter-Organizational Collaboration in Public Administration
- Public Sector Reforms: A Qualitative Comparative Analysis
- Challenges in Implementing Change: Quantitative Insights From Public Administration
- Public Service Motivation: A Qualitative Approach
Economics Dissertation Topics
- Game Theory Insights: A Quantitative Analysis of Market Behavior
- Qualitative Approach to Behavioral Economics: Exploring Irrationality in Consumer Choices
- Assessing Inflation Targets: A Quantitative Study on Central Bank Policies
- Investigating Income Inequality: Qualitative Case Studies From Developing Countries
- Cryptocurrency Market Dynamics: Quantitative Research on Price Fluctuations
- Exploring Corporate Social Responsibility: A Qualitative Perspective
- Quantitative Approach to Labor Market Flexibility and Unemployment Rates
- Sustainable Economics: A Qualitative Examination of Green Policies
- Statistical Analysis of Economic Bubbles: A Quantitative Study
- Interpreting Welfare Economics: A Qualitative Research on Social Fairness
- Gender Pay Gap: Quantitative Insights Across Industries
- Understanding Economic Resilience: A Qualitative Study of Post-Crisis Recovery
- Quantitative Study on the Determinants of Foreign Direct Investment
- Examination of Post-Keynesian Economic Theory: A Qualitative Approach
- Demographic Changes and Economic Growth: A Quantitative Analysis
- Circular Economy Principles: A Qualitative Research on Waste Reduction Strategies
- Quantitative Modeling of Monetary Policy Effectiveness
- Exploring Sustainable Development Goals: A Qualitative Assessment
- Digital Economies and Big Data: Quantitative Analysis of Economic Impacts
- Deconstructing Neoliberal Economic Policies: A Qualitative Approach
Public Health Dissertation Topics
- Exploring Health Literacy: A Qualitative Inquiry Into Patient Comprehension
- Statistical Analysis of Smoking Cessation Programs: A Quantitative Study
- Qualitative Insights Into the Barriers to Physical Activity in Urban Areas
- Examination of Vaccine Hesitancy: A Quantitative Research on Public Perception
- Investigating Mental Health Stigma: A Qualitative Perspective
- Quantitative Approach to the Efficacy of Telemedicine in Chronic Disease Management
- Food Insecurity and Public Health: A Qualitative Study in Low-Income Communities
- Analyzing Childhood Obesity Rates: A Quantitative Research on Dietary Habits
- Qualitative Examination of the Experience of Aging in Long-Term Care Facilities
- Statistical Investigation of Air Pollution Effects on Respiratory Health
- Qualitative Analysis of Postpartum Depression: Personal Narratives and Support Systems
- Disparities in Health Care Access: A Quantitative Study Based on Socioeconomic Status
- Qualitative Research Into the Influence of Community Gardens on Public Health
- Quantitative Analysis of the Correlation Between Work Stress and Cardiovascular Health
- In-Depth Qualitative Investigation Into Experiences of Health Care Workers During a Pandemic
- Examining Health Outcomes in Urban Vs. Rural Areas: A Quantitative Study
- Exploring Palliative Care Services: A Qualitative Study on Patient Satisfaction
- Analysis of Physical Activity Programs in Schools: A Quantitative Approach
- Understanding the Socio-Cultural Determinants of Health: A Qualitative Inquiry
Linguistics Dissertation Topics
- Multimodal Discourse Analysis in Advertising: A Comprehensive Study
- Syntax and Semantics Interface: A Deep-Dive Into Universal Grammar
- Corpus-Based Approach to Machine Translation: Challenges and Opportunities
- Discourse Analysis of Political Speeches: An Examination of Rhetorical Strategies
- Applying Phonetics to Speech Recognition Systems: Technological Developments
- Exploration of Pragmatics in Social Media Communication: A Case Study
- Critical Discourse Analysis of Media Coverage on Migration Issues
- Conceptual Metaphor Theory in Contemporary Poetry: An In-Depth Analysis
- Sociolinguistics in Multilingual Societies: A Case Study of Language Shift
- Semantic Processing in Artificial Intelligence: An Analytical Study
- Investigating Gender Differences in Speech: A Phonological Analysis
- Comparative Study of Dialect Variation Across Regions
- Acoustic Phonetics in Voice Recognition Systems: Technological Innovations
- Investigation of Code Switching in Bilingual Education: A Sociolinguistic Perspective
- Understanding Neurolinguistics: An Examination of Language Acquisition in the Brain
- Examining Language Change and Evolution: Historical Linguistics Approach
- Comparative Study of Lexical Borrowing in Language Contact Situations
- Exploring Cognitive Linguistics: A Study of Metaphor and Thought
- Applied Linguistics in Second Language Acquisition: An Empirical Study
- Functional Syntax in Natural Language Processing: A Computational Perspective
Theology Dissertation Topics
- Interfaith Dialogue in the Modern World: A Qualitative Inquiry
- Biblical Hermeneutics and Postmodernism: A Comparative Analysis
- Epistemology of Divine Omnipotence: A Quantitative Approach
- Moral Theology in the Context of Climate Change: A Case Study
- Comparative Analysis of Liberation Theology in Different Cultural Contexts
- Application of Phenomenology in Understanding Religious Experiences
- Investigating Theodicy in Islamic Thought: A Historical Research
- Eschatology in Medieval Christianity: An Archival Study
- Interpretative Phenomenological Analysis of Faith and Doubt
- Examination of Sacramental Theology in Orthodox Christianity: A Mixed-Methods Study
- Application of Grounded Theory in Understanding Religion and Morality
- Exploring Soteriology in Modern Christian Thought: A Qualitative Study
- Pneumatology in Pentecostalism: A Quantitative Research
- Process Theology and the Problem of Evil: An Analytical Study
- Ethnographic Study on the Influence of Charismatic Movement in Latin America
- Interdisciplinary Study of Theology and Literature in the Works of C.S. Lewis
- Comparative Analysis of Christian and Buddhist Views on Suffering
- Theistic Evolution: An Inquiry Into Its Acceptance and Rejection
- Feminist Interpretation of the Bible: A Hermeneutical Approach
Gender Studies Dissertation Topics
- Queer Theory in Modern Literature: An Analytical Study
- Depiction of Femininity in Children’s Literature: A Qualitative Study
- Influence of Social Media on Gender Identity Formation: A Mixed-Methods Research
- Transgender Experiences in the Workplace: An Ethnographic Study
- Understanding the Influence of Pop Culture on Feminism: A Discourse Analysis
- Examining Gender Representation in Video Games: A Content Analysis
- Performative Aspects of Masculinity in Professional Sports: A Case Study
- Queer Representation in Modern Cinema: A Qualitative Analysis
- Exploring Gender Fluidity in Young Adults: A Longitudinal Study
- Transgender Rights in Different Legal Systems: A Comparative Study
- Non-Binary Identities and Social Acceptance: An Empirical Study
- Cultural Perception of Gender Roles in Scandinavia: An Ethnographic Approach
- Feminist Analysis of Patriarchy in Classical Literature
- Experiences of Gender Dysphoria in Adolescents: A Phenomenological Approach
- Intersectionality in Women’s Health Care Access: A Quantitative Study
- Depiction of Queer Relationships in Young Adult Fiction: A Narrative Analysis
- Gendered Language in Job Advertisements: A Content Analysis
- Understanding Misogyny in Online Communities: A Netnographic Study
- Examining Gender Bias in Artificial Intelligence: A Qualitative Research
Anthropology Dissertation Topics
- Culture and Mental Health: An Ethnographic Exploration
- Archaeological Analysis of Prehistoric Settlement Patterns in Northern Europe
- Decoding Human Migration Patterns Through Genetic Anthropology
- Religion and Social Cohesion: A Qualitative Study in Indigenous Societies
- Food Rituals in Eastern Societies: A Comparative Study
- Linguistic Anthropology of Endangered Languages: A Case Study
- Exploring Kinship Systems in Matrilineal Societies: A Quantitative Analysis
- Material Culture and Economic Practices in Ancient Civilizations: An Archaeological Perspective
- Understanding Collective Memory in Post-Conflict Societies: A Phenomenological Approach
- Cultural Beliefs and Medical Practices: An Ethnographic Study in Remote Communities
- Exploring Body Modifications in Tribal Societies: A Comparative Anthropological Perspective
- Navigating Transnational Identities: A Longitudinal Study of Migrant Communities
- Shamanism and Healing Practices in Indigenous Cultures: An Ethnographic Study
- Anthropology of Sports: A Quantitative Analysis of Cultural Traditions in Sports
- Unraveling Human Evolutionary Biology Through Paleoanthropology
- Social Media and the Construction of Cultural Identity: A Netnographic Study
- Rites of Passage in Different Cultures: A Comparative Study
- Cultural Practices and Sustainable Agriculture in Rural Societies: An Ethnobotanical Study
- Cyborg Anthropology: Interactions of Humans and Technology in Modern Society
Thesis Topics & Ideas
Computer science thesis topics.
- Quantum Computing: A Mathematical Modelling Approach
- Algorithmic Game Theory: An Analytical Study of Multi-Player Games
- Natural Language Processing and Sentiment Analysis: An Empirical Investigation
- Human-Computer Interaction: A Phenomenological Analysis of User Experience
- Advanced Cryptography: A Case Study of Blockchain Security
- Machine Learning for Predictive Analysis in Healthcare: A Quantitative Study
- Data Mining in Social Media: A Netnographic Approach
- Artificial Intelligence in Robotics: A Longitudinal Study of Progress Over the Decade
- Mobile Computing and IoT Integration: A Qualitative Exploration
- Cybersecurity Measures in Banking: A Comparative Analysis
- Decentralized Networks in Web 3.0: A Grounded Theory Study
- Computer Vision for Autonomous Vehicles: An Empirical Research
- Bioinformatics and Genomic Data Analysis: A Quantitative Exploration
- Exploring Augmented Reality and Virtual Reality for Education: A Mixed-Methods Study
- Deep Learning for Natural Disaster Prediction: A Case Study
- Scalability Challenges in Cloud Computing: A Qualitative Study
- Neural Networks and Brain-Computer Interfaces: An Interdisciplinary Study
- Data Structures and Algorithms for Large Scale Databases: An Analytical Approach
- Computational Complexity in Quantum Algorithms: A Mathematical Study
- Software Development Practices in Agile Teams: A Phenomenological Study
Humanities and Art History Thesis Topics
- Understanding Medieval Iconography: A Visual Analysis
- Digital Humanities and Archival Practices: A Case Study
- Postmodernism in Contemporary Sculpture: A Qualitative Review
- Film as Cultural Text: A Semiotic Analysis
- Interpreting Graffiti as Street Art: An Ethnographic Study
- Neoclassicism and the French Revolution: A Historical Analysis
- Expressionism in Music: A Quantitative Study of Schoenberg’s Compositions
- Urban Spaces in Modern Literature: A Thematic Exploration
- Feminist Perspectives in Contemporary Theater: A Phenomenological Study
- Virtual Reality in Art Galleries: An Empirical Study
- Art During the Renaissance: A Comparative Analysis
- Narrative Strategies in Graphic Novels: A Structuralist Approach
- Cultural Significance of Folk Art: A Qualitative Inquiry in Rural Communities
- Pop Art and Consumer Culture: A Discourse Analysis
- Religious Symbolism in Byzantine Mosaics: A Visual Analysis
- Experiencing Performance Art: An Ethnographic Study
- Depictions of the Industrial Revolution in 19th Century Art: A Historical Review
- Dadaism as a Reaction to World War I: A Thematic Exploration
- Digital Art and Traditional Aesthetics: A Comparative Study
List of Science Topics for Your Thesis
- Exploring Dark Matter: A Quantitative Analysis of Galactic Rotation Curves
- Chemistry of Superconductors: A Spectroscopic Study
- Computational Modelling of Protein Folding: A Monte Carlo Approach
- Influence of Microbiota on Human Health: An Empirical Study
- Biodiversity in Urban Ecosystems: A Taxonomic Investigation
- Nanotechnology in Medicine: A Literature Review
- Climate Change Adaptation Strategies in Marine Ecosystems: A Qualitative Analysis
- Genetic Algorithms in Machine Learning: A Case Study
- Cosmic Microwave Background Radiation: A Statistical Analysis
- Ecological Dynamics of Coral Reefs: A Longitudinal Study
- Neural Networks in Artificial Intelligence: A Simulation-Based Investigation
- Tectonic Shifts and Earthquake Patterns: A Geostatistical Analysis
- Bioinformatics Approach to Predict Protein Structure: An Empirical Study
- Probing Quantum Entanglement: A Theoretical Framework
- Carbon Sequestration in Forest Ecosystems: An Empirical Study
- Effect of GMO Crops on Biodiversity: A Qualitative Review
- Virology and Vaccine Development: A Quantitative Study on COVID-19
- Radioactive Decay Chains: A Lab-Based Investigation
- Astrobiology and Search for Extraterrestrial Life: A Literature Review
- Nuclear Fusion as a Sustainable Energy Source: A Feasibility Study
Architecture Thesis Topics
- Biophilic Design in Modern Urban Structures: A Case Study Approach
- Psychoanalysis of Spatial Configurations: An Interpretive Phenomenological Analysis
- Sustainable Material Choices in Contemporary Architecture: A Comparative Study
- Digital Fabrication Techniques in Modern Construction: A Quantitative Review
- Historic Building Conservation Techniques: An Empirical Investigation
- Parametric Design Strategies: A Meta-Analysis
- Societal Influences on Architectural Styles: A Cross-Cultural Study
- Transitional Spaces in Urban Landscapes: A Qualitative Analysis
- Adaptive Reuse of Industrial Buildings: A Case Study Approach
- Urban Design Principles for Pedestrian-Friendly Cities: A Comparative Study
- Influence of Climatic Factors on Architectural Design: A Thematic Analysis
- The Interplay of Light and Space in Sacred Architecture: A Phenomenological Study
- Performance-Based Design of Seismic-Resistant Buildings: An Empirical Study
- Architectural Solutions for Affordable Housing: A Quantitative Review
- Innovative Techniques for Architectural Acoustic Optimization: An Experimental Study
- Techniques of Incorporating Green Spaces in High-Rise Buildings: A Case Study Approach
- Mixed-Use Developments in Urban Planning: A Meta-Analysis
- Analysis of Architectural Strategies for Aging Populations: A Quantitative Review
- Investigating Building Lifecycles: A Qualitative Study
Thesis Topics in English Literature & World Literature
- Symbolism in Kafka’s “Metamorphosis”: A Semiotic Analysis
- Investigating Gender Dynamics in Woolf’s Novels: A Feminist Reading
- Postmodern Elements in Pynchon’s “Gravity’s Rainbow”: A Thematic Review
- Exploring Myth and Folklore in Gabriel Garcia Marquez’s Works: A Comparative Study
- Depictions of War in Hemingway’s Novels: A Thematic Analysis
- Metafictional Techniques in Nabokov’s “Pale Fire”: A Close Reading
- Postcolonial Identity Construction in Achebe’s “Things Fall Apart”: A Narrative Analysis
- Eco-Critical Interpretation of Wordsworth’s Poetry: An Analytical Review
- Utopian and Dystopian Themes in Huxley’s “Brave New World”: A Comparative Analysis
- Imagery in the Sonnets of Shakespeare: A Stylistic Study
- Magical Realism in Salman Rushdie’s “Midnight’s Children”: A Narrative Inquiry
- Gothic Motifs in Poe’s Short Stories: A Qualitative Analysis
- Victorian Societal Norms in Austen’s “Pride and Prejudice”: A Sociological Reading
- Modernism in James Joyce’s “Ulysses”: An Interpretive Study
- Existential Themes in Camus’ “The Stranger”: A Philosophical Investigation
- Eastern Philosophical Elements in Hesse’s “Siddhartha”: An Intertextual Analysis
- Challenging Gender Norms in Bronte’s “Jane Eyre”: A Queer Theory Reading
- Religion and Morality in Dostoevsky’s “Crime and Punishment”: An Analytical Study
- Manifestations of Madness in Charlotte Perkins Gilman’s “The Yellow Wallpaper”: A Psychoanalytical Reading
- American Dream Critique in Fitzgerald’s “The Great Gatsby”: A Socioeconomic Analysis
Criminal Justice Thesis Topics for Dissertation Papers
- Analyzing Community Policing Strategies: A Comparative Case Study
- Ethnographic Exploration of Prison Life: Understanding Inmate Culture
- Judicial Discretion in Sentencing: A Quantitative Review
- Effects of Mandatory Minimum Sentences: A Longitudinal Study
- Racial Disparities in Policing Tactics: An Empirical Investigation
- Restorative Justice Programs and Recidivism: A Meta-Analysis
- Forensic Science in Crime Scene Investigation: A Qualitative Inquiry
- Juvenile Delinquency and Rehabilitation Programs: An Analytical Evaluation
- Psychology of Crime: A Grounded Theory Approach
- Efficacy of Drug Courts: A Quantitative Assessment
- Sexual Assault on College Campuses: A Phenomenological Study
- Death Penalty and Its Deterrent Effect: An Econometric Analysis
- White Collar Crime: An Exploratory Study on Corporate Fraud
- Domestic Violence: Narrative Inquiry of Survivor Experiences
- Police Brutality and Accountability: An Action Research Approach
- Correctional Facilities and Mental Health: A Mixed-Methods Study
- Human Trafficking: Uncovering Its Global Networks Through Content Analysis
- Digital Forensics and Cybercrime: A Systematic Review
- False Confessions in Interrogation: An Ethnographic Study
- Criminology and Public Policy: A Delphi Study on Effective Reforms
Geography Thesis Topics
- Urban Land Use Patterns: An Econometric Analysis
- Migration and Settlement Dynamics: A Demographic Study
- Climate Change Perception and Adaptation: An Ethnographic Exploration
- Implications of Deforestation: A Longitudinal Satellite Imagery Analysis
- Water Resource Management: A Delphi Study on Policy Making
- Landform Changes and Erosion: A GIS-Based Study
- Urban Heat Islands: A Comparative Case Study
- Natural Disasters and Community Resilience: A Grounded Theory Approach
- Food Deserts in Urban Environments: An Empirical Investigation
- Population Aging and Geographic Dispersion: A Quantitative Review
- Impact of Tourism on Coastal Erosion: A Phenomenological Study
- Geospatial Technologies in Disaster Management: A Systematic Review
- Sustainable Agriculture and Land Use: A Mixed-Methods Study
- Mountain Geographies and Climate Change: An Ethnographic Study
- Exploring the Geopolitics of Energy: An Analytical Evaluation
- Historical Geography of Trade Routes: A Content Analysis
- Biodiversity Conservation in Urban Parks: An Action Research Approach
- Geographies of Social Inequality: A Narrative Inquiry
- Evolving Geopolitical Landscapes: A Discourse Analysis
Sociology Thesis Ideas
- Social Media Influence on Self-Identity: An Ethnographic Exploration
- Gender and Entrepreneurship: A Quantitative Analysis of the Glass Ceiling
- Patterns of Gentrification: A GIS-Based Investigation
- Cultural Adaptation in Immigrant Families: An Empirical Investigation
- Analyzing Socioeconomic Determinants of Health: A Longitudinal Study
- Religion and Social Cohesion: A Mixed-Methods Examination
- Intersectionality in Feminist Movements: A Discourse Analysis
- Globalization and Its Effects on Traditional Societies: A Phenomenological Inquiry
- Understanding Social Inequalities in Education: An Analytical Review
- Exploring Cyberbullying Phenomena: A Grounded Theory Approach
- Consumer Culture and Its Environmental Implications: An Action Research
- Mental Health Stigma in the Workplace: A Narrative Inquiry
- Youth Participation in Politics: A Delphi Study on Youth Activism
- Digital Divide and Social Inequality: A Comparative Case Study
- Residential Segregation and Racial Disparities: A Demographic Analysis
- Urban Poverty and Crime Rates: An Econometric Evaluation
- Elderly Care and Societal Perceptions: A Longitudinal Study
- LGBTQ+ Representation in Media: A Content Analysis
- Dynamics of Social Networks and Friendships: An Empirical Review
- Trends in Global Migration: A Systematic Review
Business and Marketing Thesis Topics
- Consumer Perceptions of Green Marketing: A Case Study Approach
- Digital Transformation in Small Businesses: An Action Research Study
- Understanding Customer Loyalty in E-Commerce: An Analytical Review
- Corporate Social Responsibility in Fast Fashion: A Discourse Analysis
- Effectiveness of Influencer Marketing: A Quantitative Assessment
- Blockchain Technologies in Supply Chain Management: An Empirical Investigation
- Cultural Differences in Consumer Behavior: A Comparative Study
- Artificial Intelligence in Customer Service: An Exploratory Study
- Branding Strategies in the Digital Age: A Phenomenological Inquiry
- Entrepreneurial Leadership Styles: A Mixed-Methods Examination
- Sustainable Practices in Hospitality Industry: An Ethnographic Exploration
- Organizational Culture and Employee Satisfaction: A Longitudinal Study
- Data Privacy Concerns in Online Marketing: A Grounded Theory Approach
- Machine Learning in Business Forecasting: An Analytical Review
- Remote Work Trends and Productivity: A Delphi Study
- Diversity in Corporate Boards and Financial Performance: An Econometric Evaluation
- Neuromarketing and Consumer Decision Making: A Systematic Review
- Ethics in AI-Based Marketing Practices: A Narrative Inquiry
- Gamification as a Marketing Tool: An Empirical Review
- Corporate Mergers and Brand Identity: A Case Study
Education Thesis Topics
- Digital Literacy in Secondary Education: An Empirical Investigation
- Bilingual Education and Student Achievement: A Quantitative Analysis
- Effectiveness of STEM Education in Rural Schools: A Longitudinal Study
- Social Emotional Learning in Early Childhood Education: An Ethnographic Exploration
- Inclusion of Special Needs Students in Mainstream Classes: A Case Study
- Distance Learning in Higher Education: A Mixed-Methods Examination
- Teacher Perceptions of School Leadership: An Analytical Review
- Active Learning Strategies in University Teaching: A Grounded Theory Approach
- Exploring Cyberbullying in High Schools: A Phenomenological Inquiry
- Mental Health Support in Schools: A Systematic Review
- Comparative Study of Differentiated Instruction in Elementary Schools
- Impact of Classroom Environment on Student Engagement: An Action Research Study
- Pedagogical Strategies in Multicultural Classrooms: A Discourse Analysis
- Student Motivation in Online Learning Environments: A Delphi Study
- Embracing Diversity in Early Childhood Education: An Ethnographic Study
- Curriculum Design in Vocational Education: An Analytical Review
- Understanding Teacher Burnout: A Mixed-Methods Study
- Home Schooling During the Pandemic: A Narrative Inquiry
- Academic Performance and Socioeconomic Status: An Econometric Evaluation
Environmental Science Thesis Topics
- Assessing Deforestation Rates: A Geospatial Analysis
- Microplastic Pollution in Coastal Waters: An Empirical Study
- Conservation Strategies for Endangered Species: A Meta-Analysis
- Climate Change Perception in Different Demographics: A Cross-Sectional Study
- Urban Green Spaces and Mental Health: An Observational Study
- Exploring E-Waste Management Practices: A Comparative Case Study
- Marine Biodiversity and Ocean Acidification: An Experimental Approach
- Green Energy Adoption in Developing Countries: A Longitudinal Analysis
- Hydrological Impact of Climate Change: A Simulation Study
- Assessing the Success of Wildlife Corridors: A Grounded Theory Approach
- Invasive Species and Ecosystem Disruption: A Quantitative Examination
- Soil Quality in Organic Farming: An Analytical Review
- Comparing Sustainable Farming Practices: A Mixed-Methods Inquiry
- Air Quality Indices and Public Health: An Econometric Analysis
- Climate Change Adaptation Strategies: A Narrative Inquiry
- Assessment of Global Climate Models: An Evaluation Review
- Understanding Sustainability in Urban Planning: A Phenomenological Study
- Natural Disaster Preparedness in Coastal Communities: A Case Study
- Geochemical Analysis of Groundwater Pollution
- Biodiversity in Urban Ecosystems: A Longitudinal Study
History Thesis Topics for Dissertation Papers
- Decolonization in Africa: A Comparative Analysis
- Women’s Suffrage Movements: A Historical Review
- Understanding Ancient Greek Democracy: An Archaeological Study
- Decoding the Indus Valley Script: A Linguistic Approach
- Civil Rights Movement Tactics: A Case Study
- Medieval Feudalism in Europe: A Quantitative Examination
- Industrial Revolution Effects on British Society: An Econometric Analysis
- Renaissance Artistic Expression: An Aesthetic Review
- Confucianism Influence on Chinese History: A Phenomenological Study
- European Migration Patterns in the 20th Century: A Longitudinal Study
- Slave Narratives From the Antebellum South: A Narrative Inquiry
- WWII Propaganda in the Axis and Allied Powers: A Comparative Analysis
- Cultural Impact of the British Raj in India: An Ethnographic Study
- Mesoamerican Pyramids: An Archaeological Investigation
- Cold War Espionage Tactics: A Grounded Theory Approach
- The Emergence of Modern Science in the Islamic Golden Age: A Historical Analysis
- Origins of the Black Death in Medieval Europe: A Microbiological Inquiry
- Comparing Samurai and Knight Codes of Honor: A Cross-Cultural Study
- Origins of Christianity in the Roman Empire: A Historical Review
- Reconstruction Era Policies in the Southern United States: An Archival Research
Medical Thesis Topics
- Integrative Approach to Chronic Pain Management: A Systematic Review
- Influence of Gut Microbiota on Obesity: A Metagenomic Study
- Understanding Autism Spectrum Disorders: A Neuroimaging Investigation
- Gene Therapy Applications in Hemophilia: A Literature Review
- Precision Medicine in Oncology: A Longitudinal Study
- Advanced Wound Healing Technologies: A Randomized Controlled Trial
- Cardiovascular Risk in Psoriasis Patients: A Cohort Study
- Emerging Techniques in Organ Transplantation: An Experimental Study
- Artificial Intelligence in Radiology: A Grounded Theory Approach
- Pediatric Leukemia Genomic Landscapes: A Bioinformatics Analysis
- Nanotechnology-Based Drug Delivery Systems: A Literature Review
- Diabetes Self-Management Education Strategies: A Meta-Analysis
- Understanding Cystic Fibrosis Pathophysiology: A Case Study
- Preventing Surgical Site Infections: An Interventional Study
- Genomic Insights Into Alzheimer’s Disease: A Genome-Wide Association Study
- Comparative Effectiveness of Rheumatoid Arthritis Treatments: A Systematic Review
- Improving Outcomes in Trauma Care: A Quality Improvement Project
- Resilience in Childhood Cancer Survivors: A Qualitative Study
Philosophy Thesis Topics
- Understanding Kantian Ethics Through Textual Analysis
- Moral Dilemmas in Artificial Intelligence: A Case Study Approach
- Platonic Forms: A Comparative Study in Ancient Greek Literature
- Free Will and Determinism Debate: A Historical Review
- Phenomenological Investigation of Sartre’s Existentialism
- Virtue Ethics in Contemporary Business Practices: An Empirical Study
- Deconstruction of Foucault’s Power Theory: A Critical Discourse Analysis
- Application of Buddhist Philosophy in Mindfulness Therapies: A Meta-Analysis
- Schopenhauer’s Pessimism and Its Influence: A Bibliometric Study
- Redefining Stoic Practices in Modern Psychotherapy: A Qualitative Inquiry
- Comparing Eastern and Western Approaches to Consciousness: A Thematic Analysis
- Ethics of Genetic Engineering: A Delphi Study on Expert Opinions
- Heidegger’s Concept of Being: A Hermeneutic Analysis
- Hume’s Empiricism and Its Relevance Today: A Literature Review
- Comparative Study of Confucianism and Taoism in Chinese Social Norms
- Bioethics in Clinical Trials: An Interpretative Phenomenological Analysis
- Postmodern Perspectives on Language: A Deconstructive Approach
- Applying Rawls’s Theory of Justice to Modern Politics: A Case Study
- The Philosophy of Happiness in Epicureanism: A Historical Analysis
- Understanding Transhumanism: A Grounded Theory Approach
Political Science Thesis Topics
- Post-Brexit UK Politics: A Qualitative Content Analysis of Parliamentary Debates
- Social Media Influence on Political Campaigns: A Quantitative Study
- Foreign Policy Shifts under the Trump Administration: A Comparative Analysis
- Political Discourse in Post-Apartheid South Africa: A Discourse Network Analysis
- Internet Censorship in Authoritarian Regimes: An Empirical Study
- Democratic Transitions in Post-Communist Eastern Europe: A Longitudinal Analysis
- Power Transition in the Middle East: A Predictive Modelling Study
- Gender Representation in U.S. Congress: A Descriptive Analysis
- Comparative Analysis of Health Policies in Developed Countries
- Climate Change Policies and International Relations: A Case Study of the Paris Agreement
- Public Opinion on Immigration Policies in EU Countries: A Survey Study
- Trade Agreements and Their Influence on Developing Economies: A Meta-Analysis
- Terrorism and Counterterrorism Strategies: A Case Study on the Middle East
- Interpreting Political Ideology in Mainstream Media: A Critical Discourse Analysis
- Identity Politics in Multicultural Societies: An Ethnographic Study
- Investigating Voter Behavior in Swing States: A Quantitative Study
- Decolonization Process and Its Effect on African Politics: A Historical Analysis
- Civil Society and Democratization in Latin America: An Interpretative Phenomenological Analysis
- Influence of Political Elites on Policy Making: A Network Analysis
Psychology Thesis Topics for Dissertation Papers
- Influence of Childhood Trauma on Adult Relationships: A Longitudinal Study
- Neuropsychological Aspects of Alzheimer’s Disease: A Case-Control Study
- Assessing Coping Mechanisms in Adolescents With Anxiety Disorders: A Qualitative Study
- Stigma Associated With Mental Health in College Students: A Survey Analysis
- Effectiveness of Cognitive Behavioral Therapy in Treating Insomnia: A Systematic Review
- Cyberbullying and Its Emotional Consequences on Adolescents: A Cross-Sectional Study
- Perceived Emotional Intelligence and Job Satisfaction: A Correlational Study
- Psycho-Social Impact of Climate Change: An Ethnographic Study
- Impact of Mindfulness Training on Stress Levels in High School Teachers: A Quasi-experimental Study
- Parenting Styles and Their Effects on Childhood Self-Esteem: A Meta-Analysis
- Exploring the Psychodynamic Factors in Eating Disorders: A Phenomenological Study
- Neural Correlates of Depression: An fMRI Study
- Understanding Resilience in Refugees: A Grounded Theory Approach
- How Grief Counseling Influences Bereavement Outcomes: A Randomized Controlled Trial
- Socio-Cultural Factors and Body Image Perception Among Adolescents: A Cross-Cultural Study
- Impact of Sleep Deprivation on Cognitive Functions: An Experimental Study
- Personality Traits and Online Dating Behavior: A Quantitative Study
- Gender Differences in Coping With Chronic Illness: A Mixed Methods Study
- Applied Behavior Analysis in Children With Autism: An Observational Study
- Perception of Self in Social Media Age: A Thematic Analysis
Technology and Engineering Thesis Topics
- Enhanced Energy Storage Using Graphene-Based Supercapacitors: A Comparative Study
- Cybersecurity Vulnerabilities in Blockchain Technology: An Exploratory Investigation
- Developing Efficient Algorithms for Real-Time Traffic Management: A Simulation-Based Research
- Advancements in Biodegradable Materials for 3D Printing: An Experimental Study
- Nanotechnology Applications in Wastewater Treatment: A Literature Review
- AI in Healthcare: Developing Predictive Models for Disease Diagnosis
- Smart Grids and Renewable Energy Integration: A Case Study Approach
- Investigating Quantum Computing Applications in Cryptography
- Efficient Antenna Design for 5G Wireless Communication: An Experimental Research
- Assessment of Carbon Capture Technologies and Their Potential Impact on Climate Change: A Delphi Study
- Harnessing Solar Energy for Desalination: A Comparative Study
- Integration of AI and IoT for Smart City Development: A Meta-Analysis
- Improvement of Seismic Resistance in Infrastructure Through Biomimicry: An Applied Research
- Exploring Machine Learning Algorithms for Predicting Stock Market Trends
- Assessing the Safety of Autonomous Vehicles: A Simulation Study
- Development and Optimization of Biofuel Production Processes: A Case Study
- Wearable Technology for Health Monitoring: An Experimental Validation Study
- Implementation of Virtual Reality in Architectural Design: A Qualitative Research
- Exploring Green Manufacturing Processes in the Automobile Industry: An Ethnographic Study
Women’s and Gender Studies Thesis Topics
- Perceptions of Gender Stereotypes in Children’s Literature: A Content Analysis
- The Intersection of Gender and Class in Microfinance Institutions: A Case Study Approach
- Decoding the Representation of Transgender Characters in Media: A Qualitative Analysis
- Exploring Gender Bias in Artificial Intelligence: An Empirical Study
- Body Image and Self-Esteem Among Adolescent Girls: A Cross-Sectional Study
- Analyzing Gendered Language in Corporate Communication: A Computational Linguistics Approach
- Feminist Movements and Social Media: An Ethnographic Study
- Women’s Health and Environmental Toxins: A Cohort Study
- Matriarchal Societies and Sustainable Development: An Analytical Investigation
- Gender Discrimination in Sports Sponsorship: A Mixed Methods Approach
- Gender Disparity in Academic Publishing: A Bibliometric Analysis
- Culture’s Influence on Gender Expression: A Cross-Cultural Analysis
- Sexual Harassment and University Campus Culture: A Case Study
- The Portrayal of Female Heroes in Graphic Novels: A Semiotic Analysis
- Masculinities in Contemporary Television Series: A Textual Analysis
- Intersectionality of Gender and Disability in Employment: A Quantitative Study
- Gender Inequality in Entrepreneurship: A Longitudinal Study
- Subversion of Gender Norms in Fantasy Literature: A Discourse Analysis
- Assessing Female Representation in Tech Startups: An Exploratory Study
To Learn More, Read Relevant Articles
472 popular culture essay topics & good ideas, 372 commemorative speech topics & good ideas.
- Our Promise
- Our Achievements
- Our Mission
- Proposal Writing
- System Development
- Paper Writing
- Paper Publish
- Synopsis Writing
- Thesis Writing
- Assignments
- Survey Paper
- Conference Paper
- Journal Paper
- Empirical Paper
- Journal Support
- Big Data Dissertation Ideas
Big Data is shortly referred to as large-scale data which is collected from different sources with mixed data types . The collection and arrangement of vast data is quite a complex task in conventional methods . It may create more technical issues while processing large data. However it is a challenging task to perform numerous technologies , and presently it’s available to make the task easier. This page presents you with new updates of Big Data Dissertation Ideas with other research fundamentals!!!
Before moving on to research fundamentals, we like to share the basics of Big Data for scholars / final year students who are new to this field. All these are necessary to know for p erforming big data research . Our resource teams have a strong foundation in both fundamentals and advanced big data theories. Also, we have more than enough practical experience in handling numerous big data projects . So, we are here to make you robust in the big data fundamentals in your desired areas.
Fundamentals of Big Data
- Empirical Data Analysis
- Statistical Analysis
- Descriptive Analysis
- RHadoop Integration
- R-based Mapreduce Programming
- Hive, HBase, HDFS, Mapreduce
- Java, Sqoop, Flume, etc.
- Clickstream Analytics
- Twitter-based Sentiment Analytics
- Monetary Analysis over Share Market Data
- Airline Data for Analyzing and Optimizing Flight Delay
Now, we can see that the requirements of big data models . In recent days, big data is recognized everywhere around us in the technical world. The main reasons behind big data’s vast developments are a digital transformation and big data capabilities. As well, some of the main capabilities are given below for your reference. All these are primary operations involved in big data projects . And also, this field is developed with huge reliable techniques and algorithms for processing these operations.
What are the uses of big data models?
- Data Collection from Sources
- Data Generation and Feature Extraction
- Data Storage and Analysis
- Data Distribution and Classification
In the above list, we have seen about capabilities/usages of the big data model . Now, we can see that the significant tasks present in the big data models. Currently, these tasks gain more attention among the research community of big data . We guided numerous research scholars in developing big data dissertation ideas . Our researchers and developers have long-term experience in working with a big data field. So, we are capable to work effectively for every operation/task of big data model through advanced technologies . Our ultimate goal is to process the large data to acquire useful information for further investigation.
What are the major tasks of big data models?
- Copying and transferring data between different locations
- Integrating different sourced data in one place called consolidated datastore
- Scale-up –Utilize minimum resources through architecture-aware techniques
- Scale-out – Utilize maximum resources through parallel approach for workload distribution and also cause more delay in data accessibility
- Presentingdata from the current location and storing the data independently
- Matching different sourced data through a simulated database
Why Big Data is Required?
To take effective decisions, it is essential to examine the data thoroughly. When someone is processing millions of data, it is complex from multiple aspects . As well, some of the launch points of challenges are data storage, data quality, data fusion , etc. Our developers are intelligent to find smart solutions for simplex / complex problems. So, our suggested solutions are always reliable to achieve the expected results.
Big Data Analytics Research Topics
- Data need to protect against malicious attacks and threats
- Safely store the data in databases
- Perform data encryption and frequent data backups to manage data privacy
- Processing and analyzing of the huge-scale dataset are difficult jobs
- Concentrates on 3 main big data “V”s – Variety, Volume, and Velocity
- Variety denotes a different type of data like audio, video, text, etc.
- Volume denotes large-scale data size
- Velocity denotes the speed of new data creation
- Continuous data management need more attention since the data vary constantly
- Sometimes, the infrastructure may also change in an unpredictable manner
- For instance: customer interest and orders vary in every purchase
- Data that is lost from the database.
- For instance: Missing email ID from the personal contacts database
- Data that take more duration to correct which occur on same attributes. For instance: “U.S.” Vs US
- Data that repeats more than once unknowingly. For instance: double-counted
- Data that is modified to change the meaning of original content. For instance: incorrect private information
As mentioned earlier, big data is extensively spreading fast in many fields due to its vast developments. Here, we have given the main demands of big data technology . To cope with technological advancements, we regularly update our knowledge in recent findings. Therefore, we are always familiarized with recent Hadoop demands and developments . On knowing the importance of this field, our developers have developed several novel big data dissertation ideas and project topics for our handhold scholars and final year students’ benefits.
Which big data technology is in demand?
- As a matter of fact, both Big data and Hadoop seem to be similar. Our professionals have the strong groundwork in almost every core area of Hadoop technology. For instance: Yarn, Pig, Flume, HDFS, Oozie, HBase, Mapreduce, Hive, etc.
- Similar to Hadoop, Spark gradually gained attention among the research community. By the by, it replaces the place of disk-based brute force by memory computation. Mainly, Spark is widely used for analytical operations and machine learning techniques.
- Similar to HDFS, Spark is also used for data storage purposes. As well as, cloud service providers provide object storage works for cloud users. In comparison with HDFS, it is cost-effective to use . As a result, usage of cloud storage increases than HDFS. For instance: Azure Blob Storage and Amazon’s S3.
For your information, here we have given you the core technologies of big data along with their characteristics. Here, we classified the technologies based on certain data important operations of big data. Our developers have practiced all these technologies through different levels of complex big data projects. So, we can work on any sort of big data application and services. Moreover, we also support you in other emerging big data technologies.
Research Ideas in Big Data Analytics
- Apache Hadoop
- Scalable Parallel Batch Processing
- Robust and Custom-based Infrastructure
- Hadoop MapReduce
- Workload Distribution among Nodes
- Divide whole problems into multiple small-problems
- R & Oracle R Enterprise
- Introduced for Statistical Analysis of Data
- Apache Hive
- Use MapReduce to execute query
- Provide HiveQL for SQL Querying
- Flexible to work with ETL process either Apache HBase and HDFS
- Oracle NoSQL
- Scalable and Dynamic Schema Modeling
- Robust Multi-node and Multi-Data center
- Efficient Key-value pair Data Storage System
- Enable ACID Operations
- Hadoop Distributed File System
- The open-source system used for storing distributed files
- Executes on highly efficient hardware
- Highly scalable and enable automated data duplication
- Oracle Data Integrators and Oracle Data Connectors
- Provide Graphical User Interface (GUI)
- The export outcome of MapReduce into Hadoop, RDMS, etc.
Now, we can see about the important big data databases which are non-commercial. Majorly, big data is used for processing and effectively storing large data. The following databases have sufficient memory space for storing massive data from different sources insecure way . Our developers have designed and developed so many real-time projects in all these databases. And also, we are currently working on different advance-level of projects in these technologies.
Big Data Project Database
- Facilitate massive NoSQL databases
- Support high accessibility, duplication, report-based storage, full index support
- Operating System – Linux, Solaris, OS X, Windows, etc.
- NoSQL database which designed by Facebook but not now handled by Apache foundation
- Commercially, it provides many services via third-party vendors
- For instance: Twitter, Reddit, Netflix, Digg, Cisco, Urban Airship, etc.
- Operating System: Independent to OS
- Type of NoSQL database
- Includes 150,000 files per second and Read graphs in ms
- Integrates both graph and document databases
- Enable characteristics like Quick indexes and ACID transactions
- Specially used for Web-related applications / services
- Safely stores data in JSON documents
- Access the Web through search query which is written in Javascript
- Provide distributed scaling along with robust storage
- Operating system: OS X, Windows, Android, and Linux
- Popularly referred to as Twitter’s database for saving social graphs
- For instance: who is following/blocking whom
- Provide horizontal scaling for fast record access
- Operating System – Independent to OS
- Specifically used for storing graphs
- High performance over relational databases
- Operating System: Linux and Windows
- Extension of Apache project
- Store non-relational data in Hadoop
- Support automated failover service, modular scalability, linearity, reliable reads and writes, etc.
- Operating System: Independent to OS
Are you looking for the Best Big Data Dissertation Ideas?
More than research and development, we also support you in dissertation writing. Our writer team is merely doing this service soulfully in all research areas of the big data field . When you complete your code development phase for your handpicked research topic , the next important phase is the dissertation phase. We are great at transforming your research work and thought into a well-organized dissertation through a chain of treasured words.
To create the best dissertation work for your project, handpick your interesting project topic from our list of big data dissertation ideas. We have different subject areas of big data to assist you in all advancing big data areas. Let us know your interesting research areas in big data to give you more insight information. Then, we provide a complete development service for your handpicked topic. Next, we provide support in preparing dissertation writing for your achieved research work.
In this dissertation preparation, we reveal your research aim, objective, importance, need, research problems, proposed solutions, experimental result, pieces of evidence, arguable point, etc. Further, we give unlimited revisions to accurately meet your research expectation. In addition, we also support other academic writings like assignments, reports, thesis, documents, proposals, literature reviews, etc. Overall, we provide all sorts of writings services. Here, we have given you some interesting big data dissertation ideas from current research areas.
Latest Big Data Dissertation Ideas
- Integration of Big Data with Scalable Computing
- Blockchain System for Big Data Privacy and Security
- Performance Enhancement for Massive Data Computing Resources
- Collection, Analysis, and Visualization of Big Data
- Big Data Analytics for Geographically Distributed Environs
- Large-scale Patient Health Monitoring from Remote Areas
- Pattern Analysis of Huge-scale Data using Deep-Learning Algorithms
Further, we have also given you the list of our overall writing services. In this, we have mentioned the specifications of each service for your information . So, connect with us to know more about research services in the big data field.
Our Dissertation Writing Services
- Here, we help you to identify innovative Big data dissertation topics with proposal plans
- Here, we help you with initial Big data dissertation proposal writing for PhD / MS scholars and other academic students
- Here, we help you from Big data dissertation topic selection to dissertation final submission with revision support
- Here, we help you in any chapter / whole chapters of Big data dissertation writing
- Here, we help you with statistical analysis of your Big data dissertation through appropriate software
- Here, we help you to fine-tune and upgrade Big data dissertation content quality in a clear and organized format
On the whole, we are here to support you in interesting research areas identification, dissertation topic selection, big data dissertation ideas, code development, and dissertation writing service in the big data field. For research scholars, we also elongate our support in proposal writing, literature study writing, big data master thesis topics , paper writing (with publication), and thesis writing . For final year students, we also extend our support in assignment writing and report writing. Overall, we provide end-to-end research services in all possible research areas of big data fields.
MILESTONE 1: Research Proposal
Finalize journal (indexing).
Before sit down to research proposal writing, we need to decide exact journals. For e.g. SCI, SCI-E, ISI, SCOPUS.
Research Subject Selection
As a doctoral student, subject selection is a big problem. Phdservices.org has the team of world class experts who experience in assisting all subjects. When you decide to work in networking, we assign our experts in your specific area for assistance.
Research Topic Selection
We helping you with right and perfect topic selection, which sound interesting to the other fellows of your committee. For e.g. if your interest in networking, the research topic is VANET / MANET / any other
Literature Survey Writing
To ensure the novelty of research, we find research gaps in 50+ latest benchmark papers (IEEE, Springer, Elsevier, MDPI, Hindawi, etc.)
Case Study Writing
After literature survey, we get the main issue/problem that your research topic will aim to resolve and elegant writing support to identify relevance of the issue.
Problem Statement
Based on the research gaps finding and importance of your research, we conclude the appropriate and specific problem statement.
Writing Research Proposal
Writing a good research proposal has need of lot of time. We only span a few to cover all major aspects (reference papers collection, deficiency finding, drawing system architecture, highlights novelty)
MILESTONE 2: System Development
Fix implementation plan.
We prepare a clear project implementation plan that narrates your proposal in step-by step and it contains Software and OS specification. We recommend you very suitable tools/software that fit for your concept.
Tools/Plan Approval
We get the approval for implementation tool, software, programing language and finally implementation plan to start development process.
Pseudocode Description
Our source code is original since we write the code after pseudocodes, algorithm writing and mathematical equation derivations.
Develop Proposal Idea
We implement our novel idea in step-by-step process that given in implementation plan. We can help scholars in implementation.
Comparison/Experiments
We perform the comparison between proposed and existing schemes in both quantitative and qualitative manner since it is most crucial part of any journal paper.
Graphs, Results, Analysis Table
We evaluate and analyze the project results by plotting graphs, numerical results computation, and broader discussion of quantitative results in table.
Project Deliverables
For every project order, we deliver the following: reference papers, source codes screenshots, project video, installation and running procedures.
MILESTONE 3: Paper Writing
Choosing right format.
We intend to write a paper in customized layout. If you are interesting in any specific journal, we ready to support you. Otherwise we prepare in IEEE transaction level.
Collecting Reliable Resources
Before paper writing, we collect reliable resources such as 50+ journal papers, magazines, news, encyclopedia (books), benchmark datasets, and online resources.
Writing Rough Draft
We create an outline of a paper at first and then writing under each heading and sub-headings. It consists of novel idea and resources
Proofreading & Formatting
We must proofread and formatting a paper to fix typesetting errors, and avoiding misspelled words, misplaced punctuation marks, and so on
Native English Writing
We check the communication of a paper by rewriting with native English writers who accomplish their English literature in University of Oxford.
Scrutinizing Paper Quality
We examine the paper quality by top-experts who can easily fix the issues in journal paper writing and also confirm the level of journal paper (SCI, Scopus or Normal).
Plagiarism Checking
We at phdservices.org is 100% guarantee for original journal paper writing. We never use previously published works.
MILESTONE 4: Paper Publication
Finding apt journal.
We play crucial role in this step since this is very important for scholar’s future. Our experts will help you in choosing high Impact Factor (SJR) journals for publishing.
Lay Paper to Submit
We organize your paper for journal submission, which covers the preparation of Authors Biography, Cover Letter, Highlights of Novelty, and Suggested Reviewers.
Paper Submission
We upload paper with submit all prerequisites that are required in journal. We completely remove frustration in paper publishing.
Paper Status Tracking
We track your paper status and answering the questions raise before review process and also we giving you frequent updates for your paper received from journal.
Revising Paper Precisely
When we receive decision for revising paper, we get ready to prepare the point-point response to address all reviewers query and resubmit it to catch final acceptance.
Get Accept & e-Proofing
We receive final mail for acceptance confirmation letter and editors send e-proofing and licensing to ensure the originality.
Publishing Paper
Paper published in online and we inform you with paper title, authors information, journal name volume, issue number, page number, and DOI link
MILESTONE 5: Thesis Writing
Identifying university format.
We pay special attention for your thesis writing and our 100+ thesis writers are proficient and clear in writing thesis for all university formats.
Gathering Adequate Resources
We collect primary and adequate resources for writing well-structured thesis using published research articles, 150+ reputed reference papers, writing plan, and so on.
Writing Thesis (Preliminary)
We write thesis in chapter-by-chapter without any empirical mistakes and we completely provide plagiarism-free thesis.
Skimming & Reading
Skimming involve reading the thesis and looking abstract, conclusions, sections, & sub-sections, paragraphs, sentences & words and writing thesis chorological order of papers.
Fixing Crosscutting Issues
This step is tricky when write thesis by amateurs. Proofreading and formatting is made by our world class thesis writers who avoid verbose, and brainstorming for significant writing.
Organize Thesis Chapters
We organize thesis chapters by completing the following: elaborate chapter, structuring chapters, flow of writing, citations correction, etc.
Writing Thesis (Final Version)
We attention to details of importance of thesis contribution, well-illustrated literature review, sharp and broad results and discussion and relevant applications study.
How PhDservices.org deal with significant issues ?
1. novel ideas.
Novelty is essential for a PhD degree. Our experts are bringing quality of being novel ideas in the particular research area. It can be only determined by after thorough literature search (state-of-the-art works published in IEEE, Springer, Elsevier, ACM, ScienceDirect, Inderscience, and so on). SCI and SCOPUS journals reviewers and editors will always demand “Novelty” for each publishing work. Our experts have in-depth knowledge in all major and sub-research fields to introduce New Methods and Ideas. MAKING NOVEL IDEAS IS THE ONLY WAY OF WINNING PHD.
2. Plagiarism-Free
To improve the quality and originality of works, we are strictly avoiding plagiarism since plagiarism is not allowed and acceptable for any type journals (SCI, SCI-E, or Scopus) in editorial and reviewer point of view. We have software named as “Anti-Plagiarism Software” that examines the similarity score for documents with good accuracy. We consist of various plagiarism tools like Viper, Turnitin, Students and scholars can get your work in Zero Tolerance to Plagiarism. DONT WORRY ABOUT PHD, WE WILL TAKE CARE OF EVERYTHING.
3. Confidential Info
We intended to keep your personal and technical information in secret and it is a basic worry for all scholars.
- Technical Info: We never share your technical details to any other scholar since we know the importance of time and resources that are giving us by scholars.
- Personal Info: We restricted to access scholars personal details by our experts. Our organization leading team will have your basic and necessary info for scholars.
CONFIDENTIALITY AND PRIVACY OF INFORMATION HELD IS OF VITAL IMPORTANCE AT PHDSERVICES.ORG. WE HONEST FOR ALL CUSTOMERS.
4. Publication
Most of the PhD consultancy services will end their services in Paper Writing, but our PhDservices.org is different from others by giving guarantee for both paper writing and publication in reputed journals. With our 18+ year of experience in delivering PhD services, we meet all requirements of journals (reviewers, editors, and editor-in-chief) for rapid publications. From the beginning of paper writing, we lay our smart works. PUBLICATION IS A ROOT FOR PHD DEGREE. WE LIKE A FRUIT FOR GIVING SWEET FEELING FOR ALL SCHOLARS.
5. No Duplication
After completion of your work, it does not available in our library i.e. we erased after completion of your PhD work so we avoid of giving duplicate contents for scholars. This step makes our experts to bringing new ideas, applications, methodologies and algorithms. Our work is more standard, quality and universal. Everything we make it as a new for all scholars. INNOVATION IS THE ABILITY TO SEE THE ORIGINALITY. EXPLORATION IS OUR ENGINE THAT DRIVES INNOVATION SO LET’S ALL GO EXPLORING.
Client Reviews
I ordered a research proposal in the research area of Wireless Communications and it was as very good as I can catch it.
I had wishes to complete implementation using latest software/tools and I had no idea of where to order it. My friend suggested this place and it delivers what I expect.
It really good platform to get all PhD services and I have used it many times because of reasonable price, best customer services, and high quality.
My colleague recommended this service to me and I’m delighted their services. They guide me a lot and given worthy contents for my research paper.
I’m never disappointed at any kind of service. Till I’m work with professional writers and getting lot of opportunities.
- Christopher
Once I am entered this organization I was just felt relax because lots of my colleagues and family relations were suggested to use this service and I received best thesis writing.
I recommend phdservices.org. They have professional writers for all type of writing (proposal, paper, thesis, assignment) support at affordable price.
You guys did a great job saved more money and time. I will keep working with you and I recommend to others also.
These experts are fast, knowledgeable, and dedicated to work under a short deadline. I had get good conference paper in short span.
Guys! You are the great and real experts for paper writing since it exactly matches with my demand. I will approach again.
I am fully satisfied with thesis writing. Thank you for your faultless service and soon I come back again.
Trusted customer service that you offer for me. I don’t have any cons to say.
I was at the edge of my doctorate graduation since my thesis is totally unconnected chapters. You people did a magic and I get my complete thesis!!!
- Abdul Mohammed
Good family environment with collaboration, and lot of hardworking team who actually share their knowledge by offering PhD Services.
I enjoyed huge when working with PhD services. I was asked several questions about my system development and I had wondered of smooth, dedication and caring.
I had not provided any specific requirements for my proposal work, but you guys are very awesome because I’m received proper proposal. Thank you!
- Bhanuprasad
I was read my entire research proposal and I liked concept suits for my research issues. Thank you so much for your efforts.
- Ghulam Nabi
I am extremely happy with your project development support and source codes are easily understanding and executed.
Hi!!! You guys supported me a lot. Thank you and I am 100% satisfied with publication service.
- Abhimanyu
I had found this as a wonderful platform for scholars so I highly recommend this service to all. I ordered thesis proposal and they covered everything. Thank you so much!!!
Related Pages
Best Hadoop Projects
- [email protected]
- +91-97 91 62 64 69
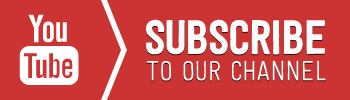
Dissertation Topics on Big Data
Dissertation Topics on Big Data is the battle ground to combat against your discourage to get world’s praise worthy achievements. Our professionals are organized our institution by their hard work with the only main vision of serve students. We provide highly confidential and in-death research topics for students and research intellectuals from various department of information technology, computer science, electrical & electronic engineering, electrical & communication engineering. Our universal celebrated experts are maintaining our confidentiality what you expert from us. We never disclose your research and your identity to third person. If you felt about your dissertation, you can contact our organization by dint of our 24/12 experts service.
Dissertation Topics on Big Data grant our momentous research guidance for students and research intellectuals in each and every point of their research. On these days, we prepared thousands of big data projects by uptrend research concepts. In project development phase, we are using wide numerous data sets such as data matrix, graphs & networks (Social, web and molecular structures), ordered data (Temporal data, genetic sequence data, multimedia & image data, spatial data, video data, sequential data), and relational record. Prepare for the Dissertation topic is not going to be easy, but we will make it for you….. Don’t miss an opportunity to contacting us……….
How do you choose Dissertation topics on Big Data ? There are many dissertation topics and the possibilities while choosing topic is almost endless. This is why choosing dissertation topic is a difficult task for researchers. Here’s we provides some steps for selecting a topics for a dissertation, you can follow these steps and make a good dissertation topic.
Steps for Topic Selection:
- Generate new ideas or find a new ideas rather than pick just one
- Test your each idea through some reputed journal papers.
- Refine your ideas, once you have knowledge about your choices
The following are some Dissertation topics on Big Data which we currently working on:
- Learning Platform for Primary school pupils
- Data Centre Consolidation based on open source cloud platforms
- Benchmarking the clouds
- Building a secure distributed environment for information sharing across organizations
- Building a scalable application based on Hibernate/Spring/J2EE
- Building a Grid or Web Service(RESTful/SOAP)
- Mining the Big Data
For your every Dissertation Topics on Big Data , you need to concentrate on the following suggestions:
Data Origins
- Sensors, Internet, Machines, etc.
Data Collection
- Web log, Images/audio, RFID, Videos, Sensor Data, etc.
Data Storage
- Technologies, Support data storage, etc.
Data Processing
- Programming framework, Processing framework, etc.
Data Analytics
- Patterns in data, Decision making, Predictive Analytics, etc.
Data Consumers
- Humans, Business processes, Applications etc.
Today’s Top Dissertation Topics on Big Data :
- Opening Up Digital Archives to Identify Sensitive Content Over the Usage of Analytics
- Convolutional Networks for Aerial Images Based Large Scale Solar Panel Mapping
- Trolls and Control Terror Awareness Level Identification Using Scalable Paradigm in Social Networks
- Scaling Morphological Tagging Based Character to Fourteen Languages
- Dynamic Feature Selection and Generation for Music Recommendation on Heterogeneous Graph
- Research on Large Scale Water Monitoring Application in Spatial-Temporal Data for Identify Dynamic Changes based on Noisy Labels
- Evaluate Code Level Performance Tuning Impacts on Power Efficiency
- Efficient Data Access Schemes on HPC Clusters with Heterogeneous Storage for Spark and Hadoop
- Hierarchical and Hybrid Outlier Detection Strategy for Protect Large Scale Data
- Rule Based Diagnosis and Hierarchical Correlation Based Performance Analysis for Big Data Paradigms
- Analyze Data Partitioning and Data Replication Performance using BEOWULF Approach in Cloud
- Parallel Clustering Method for Spark Paradigm Based Non-Disjoint Large Scale Data Partitioning
- Rare Failure Events Prediction on Large Scale manufacturing and Complex Interaction Using Classification Trees
- Labeling Actors by Integrated Information within and Through Multiple Views in Multi-View Social Networks
- Dynamic Distributed Data Structure Using Spatial Data Mining Algorithms for Efficient Data Distribution between Cluster Nodes
Recent Posts
- Hadoop related projects
- Hadoop based projects
- Hadoop Research Projects
- Sample Hadoop Projects
- big data hadoop projects
- hadoop big data projects
- hadoop open source projects
- projects on big data hadoop
- Projects Based on Hadoop
- Projects Using Hadoop
- Projects in Hadoop
- open source project related to hadoop
- big data based projects
- big data projects list
- interesting big data projects
- projects on big data
- big data projects for beginners
- big data open source projects
- big data project topics
- open source big data projects
- simple big data projects
- projects based on big data
- big data real time projects
- big data research projects
- big data analysis open source projects
- big data projects for final year
- big data mini projects
- ieee projects on big data
- ieee big data projects
- cool big data projects
- big data student projects
- project ideas on big data
- big data ieee projects
- projects in big data
- big data related projects
- big data project titles
- project topics on big data
- apache projects for big data
- projects related to big data
- dissertation topics on big data
- phd thesis big data
- phd thesis on big data analytics
- thesis on big data analytics
- projects in big data analytics
- Projects on Hadoop
- data analytics projects
Achievements – Hadoop Solutions
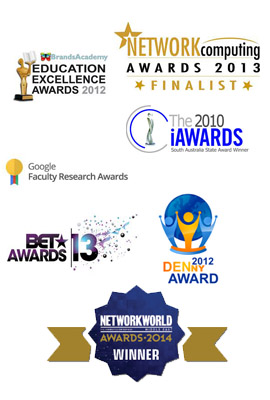
YouTube Channel
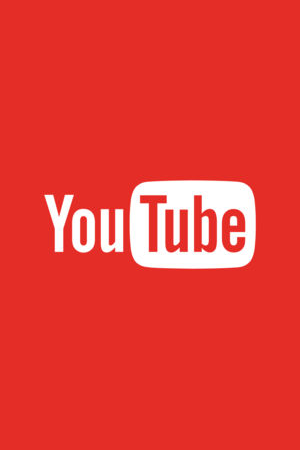
Customer Review

Other Pages
Quick links.
- Hadoop Projects
- Big Data Projects
- Hadoop Thesis
- MapReduce Project Ideas
- Big Data Analytics Projects
Support Through
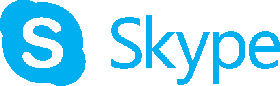
© 2015 HADOOP SOLUTIONS|Theme Developed By Hadoop Solutions | Dissertation project topics
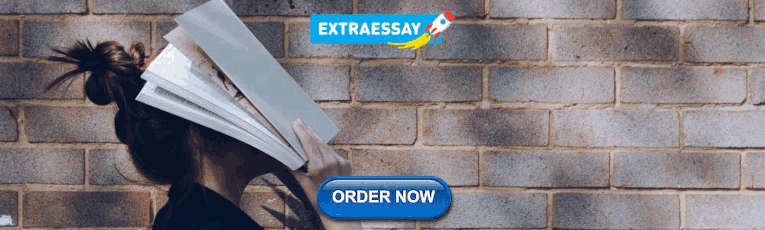
IMAGES
VIDEO
COMMENTS
These 15 topics will help you to dive into interesting research. You may even build on research done by other scholars. Evaluate the data mining process. The influence of the various dimension reduction methods and techniques. The best data classification methods. The simple linear regression modeling methods.
Here are some of the best data mining project topics that learners can consider. Big data mining techniques and tools. Model-based clustering of texts. Describe the concept of data spectroscopic clustering. Parallel spectral clustering within a distributed system.
Data Science-Related Research Topics. Developing machine learning models for real-time fraud detection in online transactions. The use of big data analytics in predicting and managing urban traffic flow. Investigating the effectiveness of data mining techniques in identifying early signs of mental health issues from social media usage.
These ideas have been drawn from the 8 v's of big data namely Volume, Value, Veracity, Visualization, Variety, Velocity, Viscosity, and Virility that provide interesting and challenging research areas for prospective researches in their masters or PhD thesis . Overall, the general big data research topics can be divided into distinct ...
166 Latest Big Data Research Topics And Fascinating Ideas. Big data refers to a huge volume of data, whether organized or unorganized, whose analysis shapes technologies and methodologies. Big data is so massive and complicated that it cannot be handled using ordinary application software. For instance, some frameworks, such as Hadoop, are ...
To write an excellent computer science thesis on big data, you must have a valid research topic. As big data is a broad subject, choosing a new trending research topic is a challenging task. So, to help you, here, in this blog post, we have listed the top interesting big data topics for you to consider for research or academic writing.
The term big data refers to the technology which processes a huge amount of data in various formats within a fraction of seconds.Big data handles the research domains by means of managing their data loads. Big data dissertation helps to convey the perceptions on the proposed research problems. It is also known as the new generation technology which could compatible with high-speed data ...
In this article, we have listed 10 such research and thesis topic ideas to take up as data science projects in 2022. Handling practical video analytics in a distributed cloud: With increased dependency on the internet, sharing videos has become a mode of data and information exchange. The role of the implementation of the Internet of Things ...
1. Introduction. Statistics and data science are more popular than ever in this era of data explosion and technological advances. Decades ago, John Tukey (Brillinger, 2014) said, "The best thing about being a statistician is that you get to play in everyone's backyard."More recently, Xiao-Li Meng (2009) said, "We no longer simply enjoy the privilege of playing in or cleaning up everyone ...
22.) Cybersecurity. Cybersecurity is a relatively new research topic in data science and in general, but it's already garnering a lot of attention from businesses and organizations. After all, with the increasing number of cyber attacks in recent years, it's clear that we need to find better ways to protect our data.
This dissertation explores three topics related to random forests: tree aggregation, variable importance, and robustness. 10. Climate Data Computing: Optimal Interpolation, Averaging, Visualization and Delivery. This dissertation solves two important problems in the modern analysis of big climate data.
The field of data science in 2024 is characterized by a convergence of cutting-edge technologies and the imperative to address real-world challenges. The ten compelling thesis topics outlined above offer students the opportunity to embark on a journey of exploration and innovation. Whether unravelling the intricacies of deep learning, combating ...
The handout provides a curated list of 20 data science dissertation topics for students in 2023. It emphasizes the importance of selecting a research area aligned with personal interests and addressing current trends and challenges in the field. The topics cover machine learning, data mining, artificial intelligence, and big data analytics.
Massive parallelism, machine learnin g, and AI. High-speed networking and high-performance computation. Hadoop, Spark-based big data analytics technologies. For quantitative, analytical, theoretical, and coding platforms related to all these methodologies, you can approach us for great big data master thesis writing.
Topic. 1: Blockchain and data security. Topic. 2: Significance of data security and privacy in health care. Topic. 3: Data security and privacy in banks. Topic. 4: Why it is imperative for businesses to ensure the data security of their clients. Topic. 5: Challenges of cyberattacks for state organisations.
The main thesis topics in Big Data and Hadoop include applications, architecture, Big Data in IoT, MapReduce, Big Data Maturity Model etc. Latest Thesis and Research Topics in Big Data. There are a various thesis and research topics in big data for M.Tech and Ph.D. Following is the list of good topics for big data for masters thesis and research:
Here are the hand-picked dissertation topics for data science that can help you grab the reader's attention quickly and without too much effort. 1. Compare the implementation of data science in various investigations concerning wildfires. 2. Explain the K-means clustering from the perspective of online spherical.
Major Real-Time Applications of Big Data Analytics. Intelligent Traffic Control. Smart City Surveillance. Healthcare Monitoring. Risk and also fraud Management. Predictive Product Grading. In this, PhD research topics in Big Data Analytics are very much familiar to access also a various "online repositories of datasets".
Apache Flink. Apache Flink is an open-source big data processing framework that provides scalable, high-throughput, and fault-tolerant data stream processing capabilities. It offers low-latency data processing and provides APIs for batch processing, stream processing, and graph processing. 25. Apache Storm.
The time required to write a master's level full dissertation varies, but it typically takes 6-12 months, depending on research complexity and individual pace. This is a sample Masters' level full dissertation in the area of big data, developed by our experts and demonstrates the quality of our work.
38 min. read. Wr1ter. team. 18 January 2024. last updated. Dissertation topics encapsulate the individual's interests and passion while simultaneously making a noteworthy contribution to the respective field of study. Potential topics span a wide range of disciplines and interests, from an exploration of recent advancements in artificial ...
Big Data Dissertation Ideas. Big Data is shortly referred to as large-scale data which is collected from different sources with mixed data types. The collection and arrangement of vast data is quite a complex task in conventional methods. It may create more technical issues while processing large data.
The following are some Dissertation topics on Big Data which we currently working on: Learning Platform for Primary school pupils. Data Centre Consolidation based on open source cloud platforms. Benchmarking the clouds. Building a secure distributed environment for information sharing across organizations.