- Search by keyword
- Search by citation
Page 1 of 30
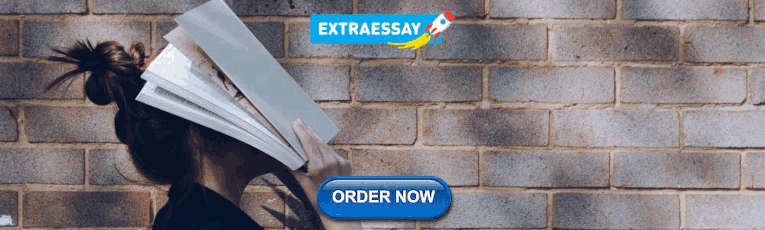
Predicting preoperative muscle invasion status for bladder cancer using computed tomography-based radiomics nomogram
The aim of the study is to assess the efficacy of the established computed tomography (CT)-based radiomics nomogram combined with radiomics and clinical features for predicting muscle invasion status in bladde...
- View Full Text
Quantitative analysis of the eyelid curvature in patients with blepharoptosis
The aim of this study was to evaluate the ability of two novel eyelid curvature measurements to distinguish between normal eyes and different severities of blepharoptosis.
MRCT and CT in the diagnosis of pediatric disease imaging: assessing imaging performance and clinical effects
This study focused on analyzing the clinical value and effect of magnetic resonance imaging plus computed tomography (MRCT) and CT in the clinical diagnosis of cerebral palsy in children.
Imaging segmentation mechanism for rectal tumors using improved U-Net
In radiation therapy, cancerous region segmentation in magnetic resonance images (MRI) is a critical step. For rectal cancer, the automatic segmentation of rectal tumors from an MRI is a great challenge. There...
Kidney dynamic SPECT acquisition on a CZT swiveling-detector ring camera: an in vivo pilot study
Large field of view CZT SPECT cameras with a ring geometry are available for some years now. Thanks to their good sensitivity and high temporal resolution, general dynamic SPECT imaging may be performed more e...
Comparison of vestibular aqueduct visualization on computed tomography and magnetic resonance imaging in patients with Ménière’s disease
The vestibular aqueduct (VA) serves an essential role in homeostasis of the inner ear and pathogenesis of Ménière’s disease (MD). The bony VA can be clearly depicted by high-resolution computed tomography (HRC...
Deep learning model for pleural effusion detection via active learning and pseudo-labeling: a multisite study
The study aimed to develop and validate a deep learning-based Computer Aided Triage (CADt) algorithm for detecting pleural effusion in chest radiographs using an active learning (AL) framework. This is aimed a...
Lymph node metastasis prediction and biological pathway associations underlying DCE-MRI deep learning radiomics in invasive breast cancer
The relationship between the biological pathways related to deep learning radiomics (DLR) and lymph node metastasis (LNM) of breast cancer is still poorly understood. This study explored the value of DLR based...
Construction of a nomogram for predicting compensated cirrhosis with Wilson’s disease based on non-invasive indicators
Wilson’s disease (WD) often leads to liver fibrosis and cirrhosis, and early diagnosis of WD cirrhosis is essential. Currently, there are few non-invasive prediction models for WD cirrhosis. The purpose of thi...
Ultrasound-based deep learning radiomics model for differentiating benign, borderline, and malignant ovarian tumours: a multi-class classification exploratory study
Accurate preoperative identification of ovarian tumour subtypes is imperative for patients as it enables physicians to custom-tailor precise and individualized management strategies. So, we have developed an u...
The feasibility of half-dose contrast-enhanced scanning of brain tumours at 5.0 T: a preliminary study
This study investigated and compared the effects of Gd enhancement on brain tumours with a half-dose of contrast medium at 5.0 T and with a full dose at 3.0 T.
Role of radiomics in staging liver fibrosis: a meta-analysis
Fibrosis has important pathoetiological and prognostic roles in chronic liver disease. This study evaluates the role of radiomics in staging liver fibrosis.
Remote sensing image information extraction based on Compensated Fuzzy Neural Network and big data analytics
Medical imaging AI systems and big data analytics have attracted much attention from researchers of industry and academia. The application of medical imaging AI systems and big data analytics play an important...
Amide proton transfer weighted and diffusion weighted imaging based radiomics classification algorithm for predicting 1p/19q co-deletion status in low grade gliomas
1p/19q co-deletion in low-grade gliomas (LGG, World Health Organization grade II and III) is of great significance in clinical decision making. We aim to use radiomics analysis to predict 1p/19q co-deletion in...
Radiomics-based discrimination of coronary chronic total occlusion and subtotal occlusion on coronary computed tomography angiography
Differentiating chronic total occlusion (CTO) from subtotal occlusion (SO) is often difficult to make from coronary computed tomography angiography (CCTA). We developed a CCTA-based radiomics model to differen...
Deep learning-based image annotation for leukocyte segmentation and classification of blood cell morphology
The research focuses on the segmentation and classification of leukocytes, a crucial task in medical image analysis for diagnosing various diseases. The leukocyte dataset comprises four classes of images such ...
Deep transfer learning with fuzzy ensemble approach for the early detection of breast cancer
Breast Cancer is a significant global health challenge, particularly affecting women with higher mortality compared with other cancer types. Timely detection of such cancer types is crucial, and recent researc...
Correction: Evaluating the consistency in different methods for measuring left atrium diameters
The original article was published in BMC Medical Imaging 2024 24 :57
Dynamic radiomics based on contrast-enhanced MRI for predicting microvascular invasion in hepatocellular carcinoma
To exploit the improved prediction performance based on dynamic contrast-enhanced (DCE) MRI by using dynamic radiomics for microvascular invasion (MVI) in hepatocellular carcinoma (HCC).
A survey of the impact of self-supervised pretraining for diagnostic tasks in medical X-ray, CT, MRI, and ultrasound
Self-supervised pretraining has been observed to be effective at improving feature representations for transfer learning, leveraging large amounts of unlabelled data. This review summarizes recent research int...
Renal interstitial fibrotic assessment using non-Gaussian diffusion kurtosis imaging in a rat model of hyperuricemia
To investigate the feasibility of Diffusion Kurtosis Imaging (DKI) in assessing renal interstitial fibrosis induced by hyperuricemia.
Preoperative prediction of microsatellite instability status in colorectal cancer based on a multiphasic enhanced CT radiomics nomogram model
To investigate the value of a nomogram model based on the combination of clinical-CT features and multiphasic enhanced CT radiomics for the preoperative prediction of the microsatellite instability (MSI) statu...
Dynamic contrast-enhanced MR imaging in identifying active anal fistula after surgery
It is challenging to identify residual or recurrent fistulas from the surgical region, while MR imaging is feasible. The aim was to use dynamic contrast-enhanced MR imaging (DCE-MRI) technology to distinguish ...
The use of individual-based FDG-PET volume of interest in predicting conversion from mild cognitive impairment to dementia
Based on a longitudinal cohort design, the aim of this study was to investigate whether individual-based 18 F fluorodeoxyglucose positron emission tomography ( 18 F-FDG-PET) regional signals can predict dementia con...
The value of a neural network based on multi-scale feature fusion to ultrasound images for the differentiation in thyroid follicular neoplasms
The objective of this research was to create a deep learning network that utilizes multiscale images for the classification of follicular thyroid carcinoma (FTC) and follicular thyroid adenoma (FTA) through pr...
Correction: Evaluating renal iron overload in diabetes mellitus by blood oxygen level-dependent magnetic resonance imaging: a longitudinal experimental study
The original article was published in BMC Medical Imaging 2022 22 :200
Classification of cognitive ability of healthy older individuals using resting-state functional connectivity magnetic resonance imaging and an extreme learning machine
Quantitative determination of the correlation between cognitive ability and functional biomarkers in the older brain is essential. To identify biomarkers associated with cognitive performance in the older, thi...
Characteristics of high frame frequency contrast-enhanced ultrasound in renal tumors
This study aims to analyze the characteristics of high frame rate contrast-enhanced ultrasound (H-CEUS) in renal lesions and to improve the ability for differential diagnosis of renal tumors.
Comparison of ASL and DSC perfusion methods in the evaluation of response to treatment in patients with a history of treatment for malignant brain tumor
Perfusion MRI is of great benefit in the post-treatment evaluation of brain tumors. Interestingly, dynamic susceptibility contrast-enhanced (DSC) perfusion has taken its place in routine examination for this p...
Predictive value of cyst/tumor volume ratio of pituitary adenoma for tumor cell proliferation
MRI has been widely used to predict the preoperative proliferative potential of pituitary adenoma (PA). However, the relationship between the cyst/tumor volume ratio (C/T ratio) and the proliferative potential...
Malignancy diagnosis of liver lesion in contrast enhanced ultrasound using an end-to-end method based on deep learning
Contrast-enhanced ultrasound (CEUS) is considered as an efficient tool for focal liver lesion characterization, given it allows real-time scanning and provides dynamic tissue perfusion information. An accurate...
Contrast-enhanced to non-contrast-enhanced image translation to exploit a clinical data warehouse of T1-weighted brain MRI
Clinical data warehouses provide access to massive amounts of medical images, but these images are often heterogeneous. They can for instance include images acquired both with or without the injection of a gad...
Altered trends of local brain function in classical trigeminal neuralgia patients after a single trigger pain
To investigate the altered trends of regional homogeneity (ReHo) based on time and frequency, and clarify the time-frequency characteristics of ReHo in 48 classical trigeminal neuralgia (CTN) patients after a ...
Development and validation of a multi-modal ultrasomics model to predict response to neoadjuvant chemoradiotherapy in locally advanced rectal cancer
To assess the performance of multi-modal ultrasomics model to predict efficacy to neoadjuvant chemoradiotherapy (nCRT) in patients with locally advanced rectal cancer (LARC) and compare with the clinical model.
Combining radiomics with thyroid imaging reporting and data system to predict lateral cervical lymph node metastases in medullary thyroid cancer
Medullary Thyroid Carcinoma (MTC) is a rare type of thyroid cancer. Accurate prediction of lateral cervical lymph node metastases (LCLNM) in MTC patients can help guide surgical decisions and ensure that patie...
Unified deep learning models for enhanced lung cancer prediction with ResNet-50–101 and EfficientNet-B3 using DICOM images
Significant advancements in machine learning algorithms have the potential to aid in the early detection and prevention of cancer, a devastating disease. However, traditional research methods face obstacles, a...
Hybrid transformer convolutional neural network-based radiomics models for osteoporosis screening in routine CT
Early diagnosis of osteoporosis is crucial to prevent osteoporotic vertebral fracture and complications of spine surgery. We aimed to conduct a hybrid transformer convolutional neural network (HTCNN)-based rad...
Improving the diagnosis and treatment of congenital heart disease through the combination of three-dimensional echocardiography and image guided surgery
The paper aimed to improve the accuracy limitations of traditional two-dimensional ultrasound and surgical procedures in the diagnosis and management of congenital heart disease (chd), and to improve the diagn...
A noninvasive method for predicting clinically significant prostate cancer using magnetic resonance imaging combined with PRKY promoter methylation level: a machine learning study
Traditional process for clinically significant prostate cancer (csPCA) diagnosis relies on invasive biopsy and may bring pain and complications. Radiomic features of magnetic resonance imaging MRI and methylat...
Artificial intelligence in tongue diagnosis: classification of tongue lesions and normal tongue images using deep convolutional neural network
This study aims to classify tongue lesion types using tongue images utilizing Deep Convolutional Neural Networks (DCNNs).
A rapid multi-parametric quantitative MR imaging method to assess Parkinson’s disease: a feasibility study
MULTIPLEX is a single-scan three-dimensional multi-parametric MRI technique that provides 1 mm isotropic T1-, T2*-, proton density- and susceptibility-weighted images and the corresponding quantitative maps. T...
Evaluating the consistency in different methods for measuring left atrium diameters
The morphological information of the pulmonary vein (PV) and left atrium (LA) is of immense clinical importance for effective atrial fibrillation ablation. The aim of this study is to examine the consistency i...
The Correction to this article has been published in BMC Medical Imaging 2024 24 :81
Deep learning–based automatic segmentation of meningioma from T1-weighted contrast-enhanced MRI for preoperative meningioma differentiation using radiomic features
This study aimed to establish a dedicated deep-learning model (DLM) on routine magnetic resonance imaging (MRI) data to investigate DLM performance in automated detection and segmentation of meningiomas in com...
Development of a prediction model for facilitating the clinical application of transcranial color-coded duplex ultrasonography
Transcranial color-coded duplex ultrasonography (TCCD) is an important diagnostic tool in the investigation of cerebrovascular diseases. TCCD is often hampered by the temporal window that ultrasound cannot pen...
Transfer learning–based PET/CT three-dimensional convolutional neural network fusion of image and clinical information for prediction of EGFR mutation in lung adenocarcinoma
To introduce a three-dimensional convolutional neural network (3D CNN) leveraging transfer learning for fusing PET/CT images and clinical data to predict EGFR mutation status in lung adenocarcinoma (LADC).
Evaluation of post-dilatation on longitudinal stent deformation and postprocedural stent malapposition in the left main artery by optical coherence tomography (OCT): an in vitro study
The diameter of the ostial and proximal left main coronary artery can be greater than 5.0 mm. However, the diameters of the mostly available coronary drug-eluting stents (DESs) are ≤ 4.0 mm. Whether high-press...
Craniofacial phenotyping with fetal MRI: a feasibility study of 3D visualisation, segmentation, surface-rendered and physical models
This study explores the potential of 3D Slice-to-Volume Registration (SVR) motion-corrected fetal MRI for craniofacial assessment, traditionally used only for fetal brain analysis. In addition, we present the ...
PulmoNet: a novel deep learning based pulmonary diseases detection model
Pulmonary diseases are various pathological conditions that affect respiratory tissues and organs, making the exchange of gas challenging for animals inhaling and exhaling. It varies from gentle and self-limit...
Automated machine learning for the identification of asymptomatic COVID-19 carriers based on chest CT images
Asymptomatic COVID-19 carriers with normal chest computed tomography (CT) scans have perpetuated the ongoing pandemic of this disease. This retrospective study aimed to use automated machine learning (AutoML) ...
Influence of spectral shaping and tube voltage modulation in ultralow-dose computed tomography of the abdomen
Unenhanced abdominal CT constitutes the diagnostic standard of care in suspected urolithiasis. Aiming to identify potential for radiation dose reduction in this frequent imaging task, this experimental study c...
Important information
Editorial board
For authors
For editorial board members
For reviewers
- Manuscript editing services
Annual Journal Metrics
2022 Citation Impact 2.7 - 2-year Impact Factor 2.7 - 5-year Impact Factor 0.983 - SNIP (Source Normalized Impact per Paper) 0.535 - SJR (SCImago Journal Rank)
2023 Speed 34 days submission to first editorial decision for all manuscripts (Median) 177 days submission to accept (Median)
2023 Usage 951,496 downloads 161 Altmetric mentions
- More about our metrics
Peer-review Terminology
The following summary describes the peer review process for this journal:
Identity transparency: Single anonymized
Reviewer interacts with: Editor
Review information published: Review reports. Reviewer Identities reviewer opt in. Author/reviewer communication
More information is available here
- Follow us on Twitter
BMC Medical Imaging
ISSN: 1471-2342
- General enquiries: [email protected]
- U.S. Department of Health & Human Services
- National Institutes of Health

En Español | Site Map | Staff Directory | Contact Us
Medical Imaging and Data Resource Center
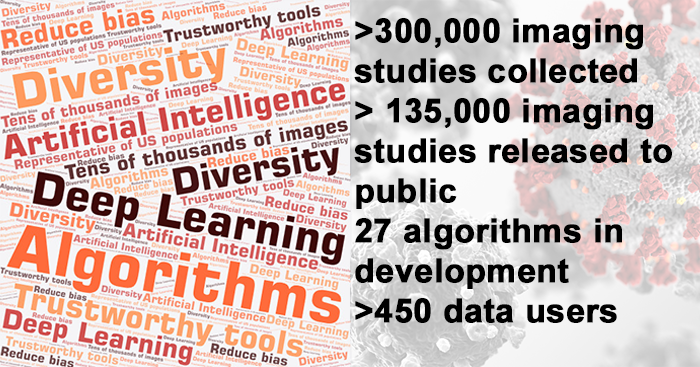
The Medical Imaging and Data Resource Center (MIDRC) is a collaboration of leading medical imaging organizations launched in August 2020 as part of NIBIB's response to the COVID-19 pandemic. MIDRC aims to develop a high-quality repository for medical images related to COVID-19 and associated clinical data, and develop and foster medical image-based artificial intelligence (AI) for use in the detection, diagnosis, prognosis, and monitoring of COVID-19.
NIBIB joined with the American College of Radiology (ACR), the Radiological Society of North America (RSNA), the American Association of Physicists in Medicine (AAPM), and the University of Chicago to create this free resource for researchers to use in the development of AI and deep learning tools and serve as a reference to enhance clinical recognition of COVID-19. Imaging data is contributed to MIDRC from multiple clinical sites throughout the country.
Learn more about MIDRC .
Access to the MIDRC data commons is now open.
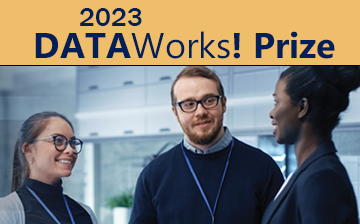
MIDRC wins distinction in 2023 DataWorks! Prize
MIDRC has received a Distinguished Achievement Award in the 2023 DataWorks! Prize. The prize is a partnership between the NIH Office of Data Science Strategy and the Federation of American Societies for Experimental Biology (FASEB) to incentivize effective practices and increase community engagement around data sharing and reuse. MIDRC was recognized for its collaboration to create a curated, diverse commons for medical imaging AI research and translation. All of MIDRC’s procedures, data models, data harmonization, annotation, and data management strategies are publicly available.
MIDRC lends medical imaging expertise to ARPA-H program
MIDRC was selected in September 2023 as one of several performers for the ARPA-H Biomedical Data Fabric (BDF) Toolbox. The ARPA-H BDF is an initiative to de-risk technologies for an easily deployable, multi-modal, multi-scale, connected data ecosystem for biomedical data. MIDRC will provide domain expertise and data commons technology development in medical imaging. MIDRC is funded by NIH NIBIB, co-led by investigators from the American Association of Physicists in Medicine (AAPM), the Radiological Society of North America (RSNA), and the American College of Radiology (ACR), hosted at the University of Chicago (MIDRC Central), and exists on the Gen3 data ecosystem.
ARPA-H is enlisting multiple performers who are experts in their fields to build components of the ARPA-H BDF Toolbox. MIDRC’s expertise stems from developing the repository in which MIDRC imaging data are harmonized and vetted by clinical, artificial intelligence (AI)/machine learning (ML), and data science domain experts and are aligned with a common data model. The public open data commons was created “at scale” and is interoperable with other data commons to enable multi-modal, multi-omics research as well as rigorous statistical evaluations. MIDRC also includes a user portal for cohort building and downloading, allowing for multiple re-uses of the data by various AI/ML developers for various specific tasks. Importantly, MIDRC has also produced various resources such as a metrology decision tree and a bias awareness tool for aiding in bias mitigation as well as multiple algorithms/software.
The ARPA-H BDF toolbox will help make research data easier and more reliable to use, reduce effort for data integration, and enable new capabilities and models that can be applied across disciplines and generalized across disease domains. “MIDRC’s involvement in the ARPA-H BDF Toolbox will include development and deployment of medical imaging data commons architectures and resources to support various diseases and applications for the toolbox.” said MIDRC principal investigator Maryellen L. Giger, PhD, the A.N. Pritzker Distinguished Service Professor of Radiology, Committee on Medical Physics at the University of Chicago.
For more on how you can get involved, go to ARPA-H BDF ; for more on how you can submit and/or access data, go to MIDRC .
Announcements
MIDRC and NIBIB are hosting a workshop to review the history of MIDRC and its impact on medical imaging and trustworthy Artificial Intelligence (AI) development, and to look forward to the future of MIDRC and AI.
Date: April 26, 2024
Time: 9:00 AM to 12 PM EDT
Location: NIH main campus in Bethesda, MD and virtually by Zoom
Registration is now closed.
Publications
Find publications on the MIDRC website .
- International Society for Optics and Photonics (SPIE)
- America Society for Neuroradiology (ASNR)
- American Institute of Ultrasound in Medicine (AIUM)
- Academy for Radiology and Biomedical Imaging Research
- Society of Imaging Informatics in Medicine (SIIM)
- >300,000 imaging studies collected
- >135,000 imaging studies released to the public
- 27 algorithms in development
- >450 data users
Related News & Events
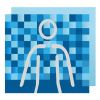
This interview with Maryellen Giger, PhD, delves into the creation of the MIDRC imaging repository, how its data can be used to develop and evaluate AI algorithms, ways that bias can be introduced—and potentially mitigated—in medical imaging models, and what the future may hold.
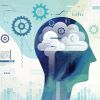
Researchers have found that AI models could accurately predict self-reported race in several types of medical images, suggesting that race information could be unknowingly incorporated into image analysis models.
After less than two years of data collection and processing, RSNA has successfully delivered over 30,000 de-identified imaging studies to the Medical Imaging and Data Resource Center (MIDRC) project, an open-access platform which publishes data to be used for research. MIDRC is funded by NIBIB. Source: RSNA News
MIDRC's June 2023 monthly seminar will focus on how MIDRC can help investigators satisfy the new NIH Data Management and Sharing Policy.
Presenting the medical imaging community with new tools and resources from MIDRC to facilitate rapid and flexible collection, AI research, and dissemination of imaging and associated data.
Virtual event: February 21, 2023 speaker: Dr. Paul Kinahan, University of Washington, on Curating the MIDRC collection of DICOM images for use in AI research.
A series of webinars about topics related to the Medical Imaging and Data Resource Center (MIDRC).
Explore More
Heath Topics
Research Topics
- Artificial Intelligence
- Computed Tomography (CT)
- Machine Learning
Scientific Program Areas
- Division of Applied Science & Technology (Bioimaging)
- Division of Discovery Science & Technology (Bioengineering)
- Division of Health Informatics Technologies (Informatics)
- Division of Interdisciplinary Training (DIDT)
Inside NIBIB
- Director's Corner
- Funding Policies
- NIBIB Fact Sheets
- Press Releases
- Brownell Lab
- Brugarolas Lab
- Elmaleh Lab
- Johnson Lab
- Normandin Lab
- Santarnecchi Lab
- Sepulcre Lab
- Technical Staff
- Administrative Staff
- Collaborators
- Publications
- Image Generation and Clinical Assessment
- PET and SPECT Instrumentation
- Multimodal MRI Research
- Quantitative PET-MR Imaging
- Quantitative PET/SPECT
- Machine Learning for Anatomical imaging
- Therapy Imaging Program
- Pharmaco-Kinetic Modeling
- Monitoring Radiotherapy with PET
- Radiochemistry Discovery
- Precision Neuroscience & Neuromodulation Program
- Video Lectures
- T32 Postgraduate Training Program in Medical Imaging (PTPMI)
- The Gordon Speaker Series
- Translation
- Covid-19 response
- Recent Seminars
- Awards and Honors
- Recent Publications
- Other News Items
- Grant Proposals
- Grant Related FAQs
- Travel Policies
- Purchasing and Other Daily Operations
- Human Resources
- Contact Forms
- Science Resources
- Computing Resources
- File Repository
- Seminar Sign Up
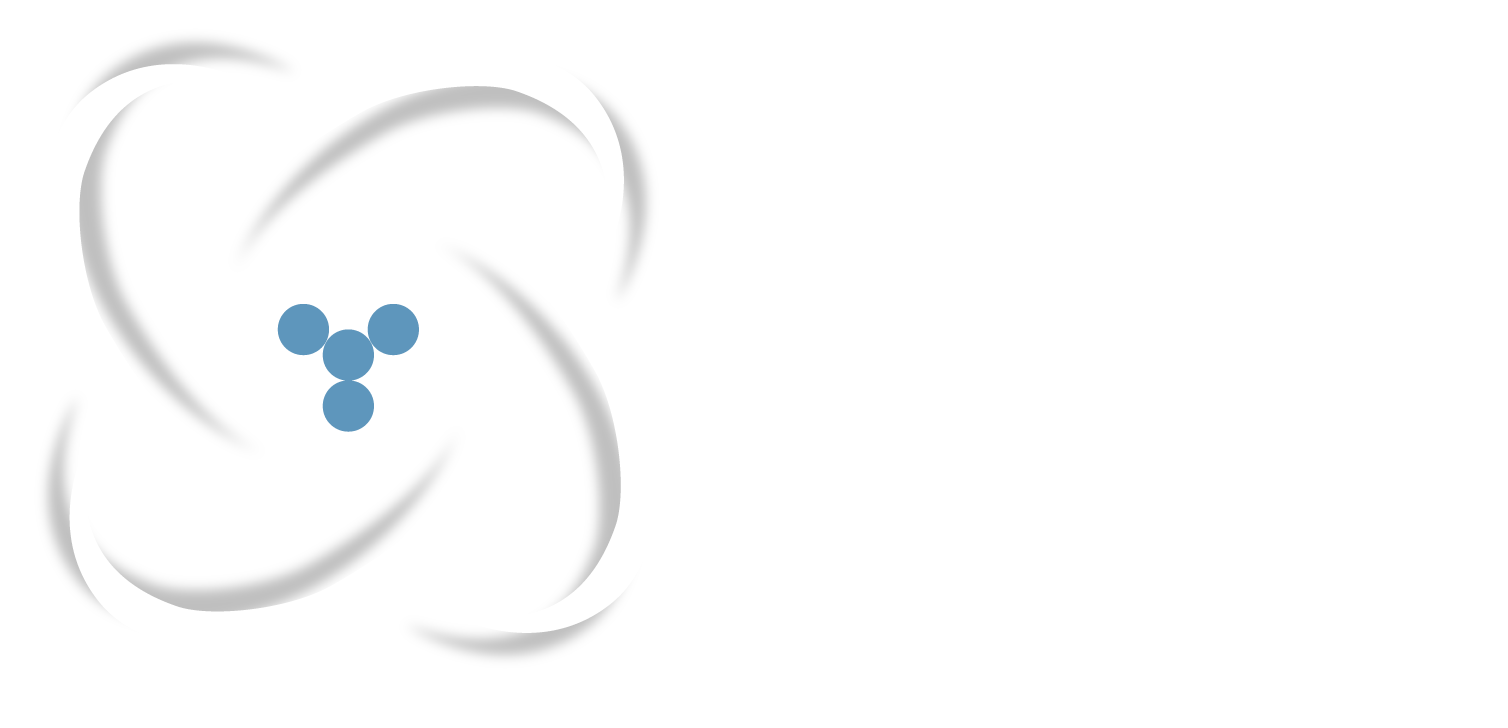
Welcome to the Gordon Center for Medical Imaging
한국어 中文 Français Español
The Gordon Center for Medical Imaging at Massachusetts General Hospital (MGH) and Harvard Medical School is a multidisciplinary research center dedicated to improving patient care by developing and promoting new biomedical imaging technologies used in both diagnosis and therapy.
Our main activities include research , training , and translation of innovative research into clinical applications. Investigators from the academic community, research laboratories, and industry are welcome to use the Center’s medical imaging equipment and clinical research facilities available through PET Core .
Created in 2015 with an endowment from the Bernard and Sophia Gordon Foundation, the Gordon Center for Medical Imaging is a direct continuation of MGH’s Division of Radiological Sciences where the first positron-imaging device was invented. As early as the 1950s, a series of important milestones were achieved at MGH, including the MGH-positron (2D) cameras, the filtered backprojection algorithm (Chesler) and multiple gated cardiac imaging (Alpert).
Today, the Gordon Center focuses on research, translation to clinical application and training of the next generation of scientists and engineers in medical imaging. These activities stress academic research sponsored by federal agencies and industrial collaborations. Predoctoral students and postdoctoral fellows gain experience, mainly aimed toward fostering their academic careers, by participating in ongoing research, educational symposia and tutorials. In parallel, promising scientists and engineers, who are interested in combining entrepreneurship, engineering and academic research in support of the public good, can benefit from the Center’s support to translate their transformative research.
Upcoming Seminars and Events
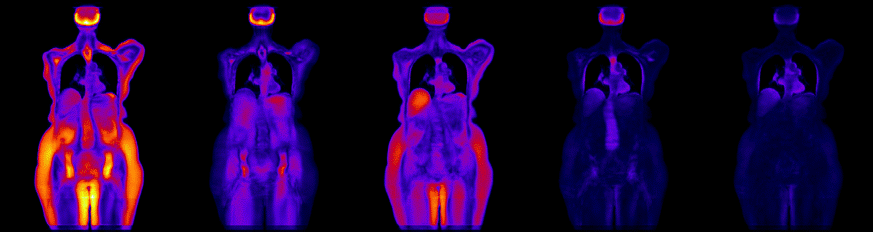
Our research spans a variety of imaging modalities from CT to PET to MRI to optical. We have expertise in a variety of techniques from reconstruction and kinetic modeling to radiotracer development
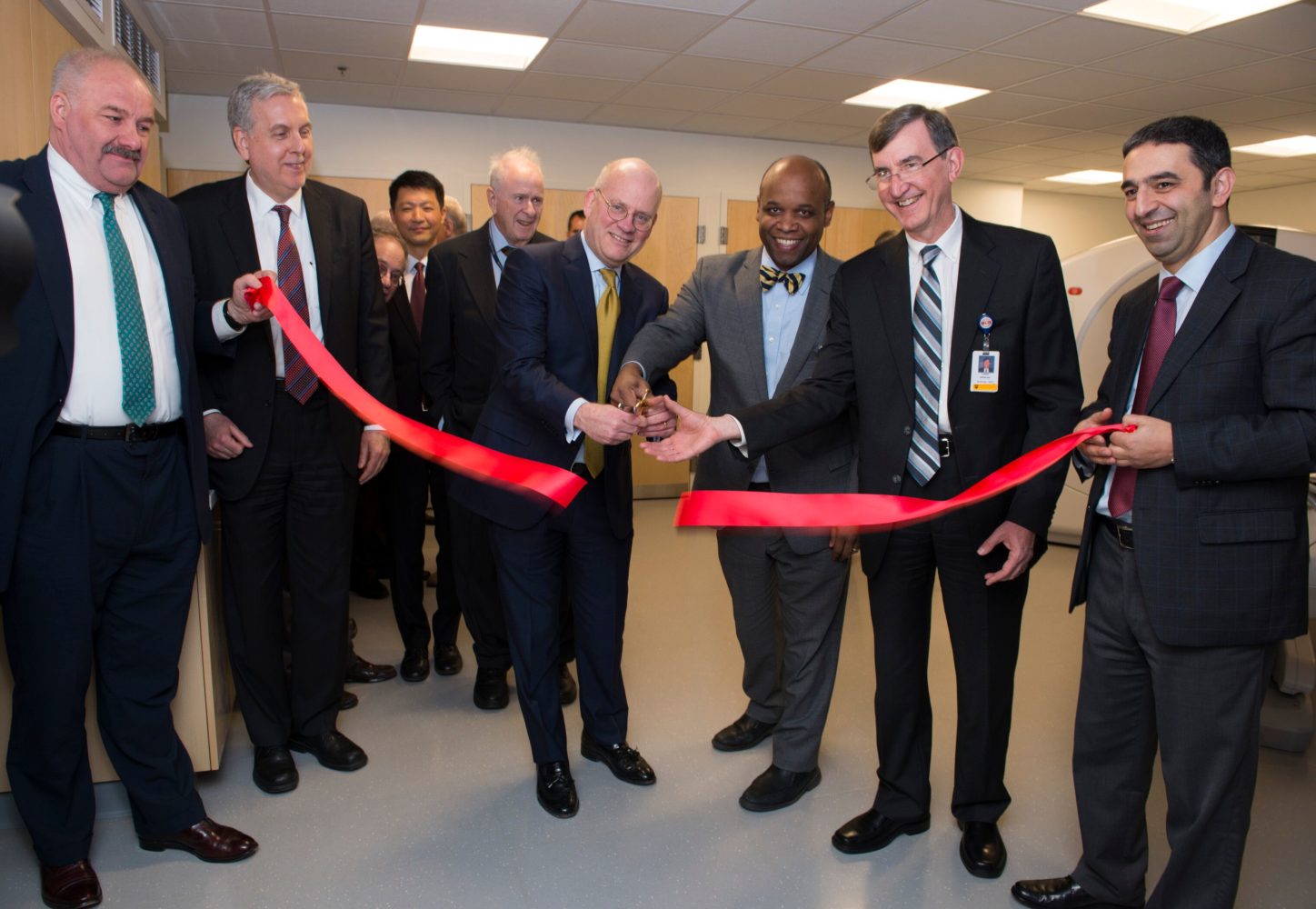
See updates on recent publications, Gordon Lecture Series seminars on a variety of medical imaging topics from experts around the world, and other news items such as awards and promotions.
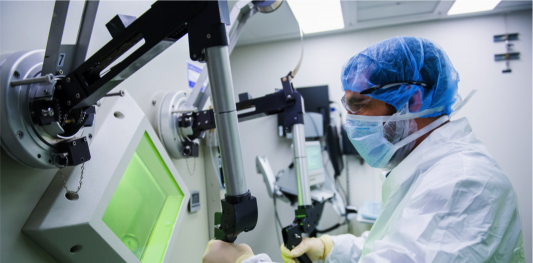
The MGH Gordon PET Core is cGMP/FDA registered positron emission tomography drug manufacturer that operates at cost to provide investigators with services to design and conduct research studies.
Medical Imaging
Our research in this area covers a wide range of topics, from development of imaging devices, image reconstruction, contrast media and kinetic modeling, image processing and analysis, and patient modeling. In addition to traditional clinical imaging modalities, such as CT, MRI, PET and ultrasound, we are also interested in emerging technologies (e.g., functional and biological imaging techniques) for cancer detection, tumor target delineation and therapeutic assessment. We are also working on four-dimensional imaging techniques so that the change of 3D patient anatomy with time can be visualized and analyzed.
4D CT Sample 1

Conventional 3D PET
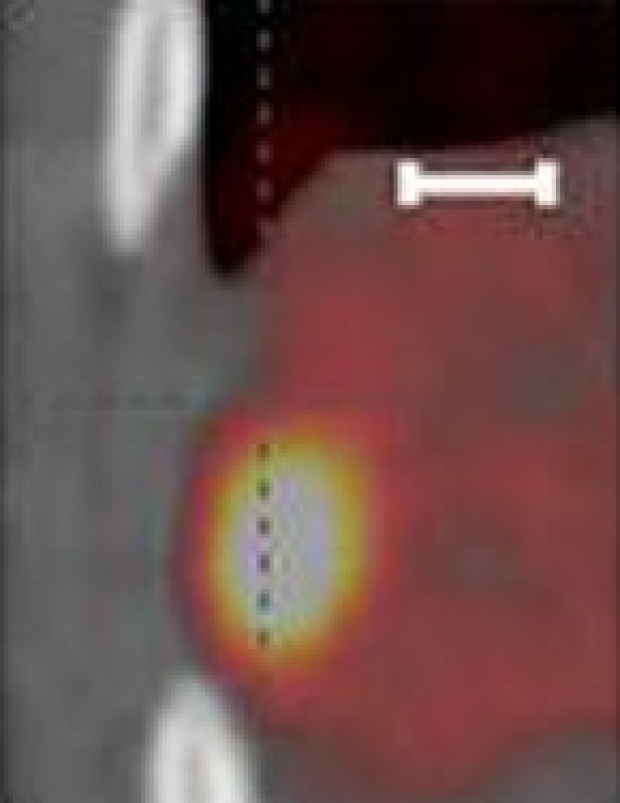

The AIMI Center
Stanford has established the AIMI Center to develop, evaluate, and disseminate artificial intelligence systems to benefit patients. We conduct research that solves clinically important problems using machine learning and other AI techniques.

Director's Welcome
Back in 2017, I tweeted “radiologists who use AI will replace radiologists who don’t.” The tweet has taken on a life of its own, perhaps because it has a double meaning.

Register for #AIMI24
AIMI Dataset Index
AIMI has launched a community-driven resource of health AI datasets for machine learning in healthcare as part of our vision of catalyze sharing well curated, de-identified clinical data sets
AIMI Summer Research Internship & AI Bootcamp
Inviting high school students to join us for a two-week virtual journey delving into the intersection of AI and healthcare through our summer programs. Applications due March 31, 2024.
AIMI Datasets for Research & Commercial Use
The AIMI Center is helping to catalyze outstanding open science by publicly releasing 20+ AI-ready clinical data sets (many with code and AI models) for research and commercial use.

Upcoming Events
Aimi symposium 2024, ibiis-aimi seminar: mildred cho, phd, ibiis-aimi seminar: bo wang, phd.
- Alzheimer's disease & dementia
- Arthritis & Rheumatism
- Attention deficit disorders
- Autism spectrum disorders
- Biomedical technology
- Diseases, Conditions, Syndromes
- Endocrinology & Metabolism
- Gastroenterology
- Gerontology & Geriatrics
- Health informatics
- Inflammatory disorders
- Medical economics
- Medical research
- Medications
- Neuroscience
- Obstetrics & gynaecology
- Oncology & Cancer
- Ophthalmology
- Overweight & Obesity
- Parkinson's & Movement disorders
- Psychology & Psychiatry
- Radiology & Imaging
- Sleep disorders
- Sports medicine & Kinesiology
- Vaccination
- Breast cancer
- Cardiovascular disease
- Chronic obstructive pulmonary disease
- Colon cancer
- Coronary artery disease
- Heart attack
- Heart disease
- High blood pressure
- Kidney disease
- Lung cancer
- Multiple sclerosis
- Myocardial infarction
- Ovarian cancer
- Post traumatic stress disorder
- Rheumatoid arthritis
- Schizophrenia
- Skin cancer
- Type 2 diabetes
- Full List »
share this!
April 25, 2024
This article has been reviewed according to Science X's editorial process and policies . Editors have highlighted the following attributes while ensuring the content's credibility:
fact-checked
peer-reviewed publication
trusted source
Researchers create an AI-powered digital imaging system to speed up cancer biopsy results
by University of Waterloo
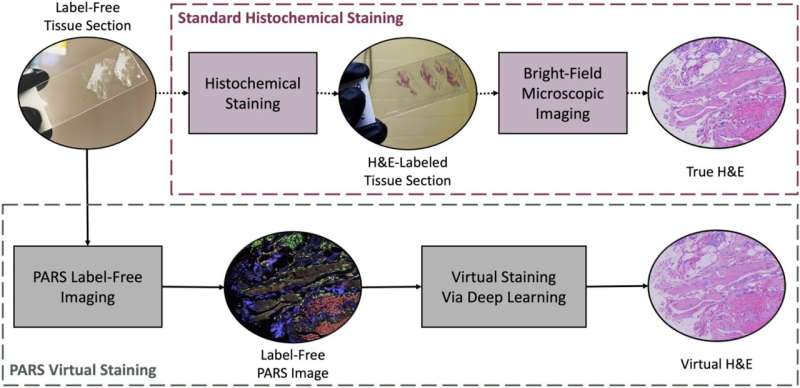
University of Waterloo researchers have invented a digital medical imaging system that significantly improves the cancer detection process to deliver immediate results and enable swift, effective treatment for all types of cancer.
The Photon Absorption Remote Sensing (PARS) system, an innovative, built-from-scratch technology, is faster than traditional cancer-detection methods and aims to deliver a diagnosis in minutes—enabling prompt surgical intervention. Currently, patients can wait weeks or even months to receive biopsy results, leading to delays in treatment and increased patient anxiety . The work is published in the journals IEEE Transactions on Biomedical Engineering and Scientific Reports .
The new system is also highly accurate. Clinical studies using human breast tissue showed that pathologists were unable to distinguish between images generated by the PARS system and those produced by conventional methods. The technology boasts a 98 percent correlation with traditional diagnostic techniques.
"Our primary goal is to provide patients with timely and accurate diagnoses, reducing the need for multiple surgeries and minimizing the risk of cancer spread," said Dr. Parsin Haji Reza, lead researcher and a professor in Waterloo's Department of Systems Design Engineering.
"This invention will transform digital pathology, enabling surgeons to obtain multiple results simultaneously with just one biopsy and provide accurate diagnoses within minutes. It also ensures thorough removal of cancerous tissue before closing the incision, mitigating the need for further surgeries."
The biopsy process is a crucial step in determining the presence of cancer. The traditional histopathology method involves taking a tissue sample and preparing it for analysis—cutting it into sections, staining those sections with different dyes, mounting those sections onto slides, and analyzing those slides under a microscope to identify cancer markers.
By replacing traditional methods with high-resolution imaging powered by artificial intelligence (AI), the PARS system accelerates the diagnostic process, saving both time and resources. Lasers are applied to a tissue sample which generates a unique and detailed set of data in high resolution.
The data is fed into the AI system which translates it into a standard histopathology image for the pathologist to read. It removes the need for multiple slide preparation by applying digitized image filters to the one tissue sample providing multiple reads—all without damaging the sample which allows for further analysis if needed.
"In addition to reducing patients' stress, this technology will save billions of dollars for the health care system," said Reza. "Surgeon and pathologists' time costs money, each slide and special dye costs money, the hospitals' facilities cost money. We can now reduce all those costs with faster biopsy results that are just as accurate."
illumiSonics, a Waterloo-based startup, has been established to commercialize the research with plans to bring the technology to market within two years. The company offers graduate students involved in the research employment opportunities to continue to steer the system's development.
Boktor, M., et al. Multi-channel feature extraction for virtual histological staining of photon absorption remote sensing images. Scientific Reports (2024). DOI: 10.1038/s41598-024-52588-1
Explore further
Feedback to editors

Research shows 'profound' link between dietary choices and brain health
3 hours ago

Component of keto diet plus immunotherapy may reduce prostate cancer
7 hours ago

Study finds big jump in addiction treatment at community health clinics

Positive childhood experiences can boost mental health and reduce depression and anxiety in teens

Gene linked to epilepsy and autism decoded in new study
Apr 26, 2024

Blood test finds knee osteoarthritis up to eight years before it appears on X-rays

Researchers find pregnancy cytokine levels impact fetal brain development and offspring behavior

Study finds biomarkers for psychiatric symptoms in patients with rare genetic condition 22q

Clinical trial evaluates azithromycin for preventing chronic lung disease in premature babies

Scientists report that new gene therapy slows down amyotrophic lateral sclerosis disease progression
Related stories.

'Virtual biopsy' lets clinicians analyze skin noninvasively
Apr 10, 2024

Deep learning–based virtual staining of tissue facilitates rapid assessment of breast cancer biomarker
Oct 27, 2022

Digital pathology cleared for use in UK cancer screening programs
Jan 25, 2024

A touchless technology for early detection of eye diseases
Jun 14, 2021

New imaging technology could 'revolutionize' cancer surgery
Sep 16, 2019

AI taps human wisdom for faster, better cancer diagnosis
Mar 11, 2020
Recommended for you

Study identifies driver of liver cancer that could be target for treatment

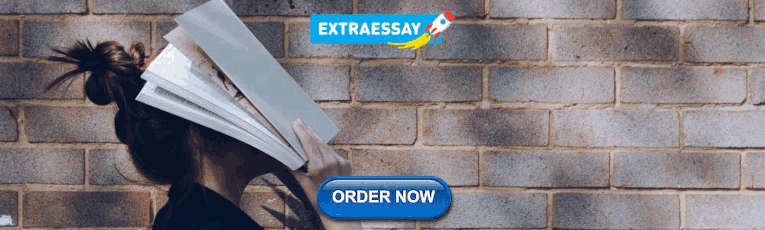
Analysis identifies 50 new genomic regions associated with kidney cancer risk

Biomarkers identified for successful treatment of bone marrow tumors

Study finds vitamin D alters mouse gut bacteria to give better cancer immunity
Apr 25, 2024

Targeting specific protein regions offers a new treatment approach in medulloblastoma
Let us know if there is a problem with our content.
Use this form if you have come across a typo, inaccuracy or would like to send an edit request for the content on this page. For general inquiries, please use our contact form . For general feedback, use the public comments section below (please adhere to guidelines ).
Please select the most appropriate category to facilitate processing of your request
Thank you for taking time to provide your feedback to the editors.
Your feedback is important to us. However, we do not guarantee individual replies due to the high volume of messages.
E-mail the story
Your email address is used only to let the recipient know who sent the email. Neither your address nor the recipient's address will be used for any other purpose. The information you enter will appear in your e-mail message and is not retained by Medical Xpress in any form.
Newsletter sign up
Get weekly and/or daily updates delivered to your inbox. You can unsubscribe at any time and we'll never share your details to third parties.
More information Privacy policy
Donate and enjoy an ad-free experience
We keep our content available to everyone. Consider supporting Science X's mission by getting a premium account.
E-mail newsletter
- Reference Manager
- Simple TEXT file
People also looked at
Mini review article, a history of cardiovascular magnetic resonance imaging in clinical practice and population science.
- 1 William Harvey Research Institute, Queen Mary University of London, London, United Kingdom
- 2 Barts Heart Centre, Barts Health NHS Trust, London, United Kingdom
- 3 Division of Cardiology, Department of Medicine, Johns Hopkins University, Baltimore, MD, United States
- 4 Department of Radiology, Johns Hopkins University, Baltimore, MD, United States
- 5 Department of Radiology, University of Wisconsin School of Medicine and Public Heath, Madison, WI, United States
Cardiovascular magnetic resonance (CMR) imaging has become an invaluable clinical and research tool. Starting from the discovery of nuclear magnetic resonance, this article provides a brief overview of the key developments that have led to CMR as it is today, and how it became the modality of choice for large-scale population studies.
Introduction
Even the most ardent cardiac imager may be forgiven for not having read C. J. Gorter's 1936 manuscript in Physica in which he described unsuccessfully attempting to find resonance of lithium-7 nuclei in crystalline lithium fluoride ( 1 ). However, it is from here that the story of cardiovascular magnetic resonance (CMR) can trace its origins. Whilst the latest guidelines and research articles make up the bricks of our clinical and academic practice, the mortar—the tales of how these edifices were constructed—can often be even more compelling. In this review article, we embark on a whistlestop tour of the history of CMR in clinical practice and its strides into population science. We hope that state-of-the-art CMR and future directions will be better understood and appreciated when contextualised within the tapestry of innovation and discovery that has preceded it.
Early NMR research
The phenomenon of nuclear magnetic resonance (NMR) sought by Gorter was discovered independently by Felix Bloch and Edwin Purcell in 1946 ( 2 , 3 ). They determined that when certain nuclei were placed in a magnetic field and subjected to radiofrequency energy, they absorbed energy and re-emitted this energy when the nuclei returned to their original state. For this discovery, they were awarded the 1952 Nobel Prize in physics. From this point, the field of NMR saw a host of investigations in non-biological and biological samples. The work of Richard Ernst in 1966 resulted in a step change in the field when he demonstrated that it was possible to markedly improve the sensitivity of NMR signals generated by applying the Fourier transformation ( 4 ). This mathematical operation, named after the French physicist Jean-Baptiste Joseph Fourier, is now the bedrock upon which magnetic resonance output can be interpreted.
In 1971, Raymond Damadian measured T1 and T2 relaxation times of normal and cancerous ex vivo rat tissue, demonstrating that tumours had longer relaxation times ( 5 ). However, all NMR experiments until the early 1970's were one-dimensional—no spatial information was available to ascertain where within the sample the NMR signal originated from. The problem of spatial localisation was solved independently by Paul Lauterbur and Peter Mansfield in 1974 ( 6 , 7 ). They discovered that the addition of a varying magnetic field (a “gradient”) altered the resonance frequency of nuclei in proportion to their position in the magnetic field. By understanding how these gradients could be applied in different orientations, spatial information could be encoded in three dimensions and thereby provide the requisite information for generating an image. Ernst, having heard Lauterbur describe this at a conference in North Carolina in 1974 ( 8 ), understood the applicability of his work from NMR spectroscopy and wrote a 1975 publication with Anil Kumar which described a practical method to rapidly reconstruct an image from NMR signals ( 9 ). Ernst received the Nobel Prize in chemistry in 1991 and Lauterbur and Mansfield the Nobel Prize in medicine in 2003.
Towards cardiovascular NMR
With the discovery of spatial localisation, a Rubicon had been crossed. The first anatomical structure imaged in detail was a cross-section of a finger in 1977 by Mansfield and Andrew Maudsley ( 10 ). Later the same year, Damadian and colleagues had generated a cross-sectional image of the human chest ( 11 ) and by 1978, Hugh Clow and Ian Young had reported the first transverse image of the head ( 12 ).
Whilst cardiac structures were visible on these early NMR images, Robert Hawkes and colleagues in Nottingham published results of NMR imaging directed specifically at the heart in 1981 ( 13 ). In this manuscript they presciently remarked that electrocardiogram (ECG) gating might improve scan quality and the great potential of cardiovascular NMR given its lack of ionising radiation. By this time, the original term NMR imaging had been replaced by the rather less precise magnetic resonance imaging (MRI) and applications of MRI accelerated rapidly. In 1983, Herfkens et al. published cardiovascular MRI findings at 0.35 Tesla (T) in 244 individuals—a remarkably large sample even by comparison to the size of modern day CMR cohorts ( 14 ).
Limitations in early MRI scanners meant the process of generation the NMR signal was slow—to the order of one to several seconds. Due to heart motion during long acquisitions, investigators recognised that synchronisation of the NMR signal to the cardiac cycle was necessary. Higgins and colleagues tested three different triggering methods: peripheral pulse signals, Doppler flow signals and ECG signals. The ECG gating method was demonstrably superior to other methods and became widely adopted in the field.
Structure and function
Mansfield and his team reported the first real time cine CMR image of a live rabbit heart in 1982 using an echo planar imaging technique they developed ( 15 ). CMR was recognised to be useful to provide accurate estimates of cardiac function and left ventricular mass ( 16 ) as well as estimating regurgitant fractions in patients with mitral or aortic regurgitation ( 17 ). A major disadvantage of early CMR was long acquisition times (e.g., two minutes for each phase of the cardiac cycle). This was solved in the early 1990's by Atkinson and colleagues who introduction segmented data acquisition. With this method, cine CMR image acquisition was accelerated in inverse proportion to the acquired number of frames per cardiac cycle. Importantly, ECG segmented acquisitions combined with fast gradient echo acquisition allowed single breath-hold, two-dimensional cine imaging of the heart ( 18 ).
Hawkes, who generated perhaps the first set of dedicated cardiac images using NMR, also worked on the earliest versions of the steady-state free precession imaging sequence (SSFP) in the early 1980's. The SSFP technique temporarily faded in relevance due to its relative inferior image quality compared to the gradient echo imaging techniques. However, by the turn of the millennium, better magnet field homogeneity and improved gradient hardware with rapid switching had remarkably improved image quality. By the late 1990s, the SSFP method was providing far clearer blood pool-to-myocardium and epicardial fat-to-myocardium delineation and is currently the workhorse for both clinical and research cine CMR.
From the early 1980's, the use of exogenous intravenously administered contrast agents had been explored in conjunction with MRI, particularly for imaging the brain. MRI contrast agents were found to improve the detection of multiple pathological abnormalities in the brain, including better depiction of solid tissue from adjacent oedema. Similar results were subsequently identified when imaging the heart with CMR. In 1982, intravenous administration of a manganese chelate contrast agent was demonstrated in differentiating ischaemic myocardium (“a dark spot”) from normal tissue in a canine model. Manganese had a limited clinical role due to its toxicity, however, other agents such as gadopentetate dimeglumine were investigated in the early 1980's. At present, gadolinium-based contrast agents remain the cornerstone of CMR to improve the depiction of multiple cardiovascular abnormalities, ranging from perfusion deficits and myocardial infarction to myocardial tumours and inflammation.
In 1989, de Roos and colleagues described the different enhancement patterns in patients with occlusive vs. reperfused infarction ( 19 ). Over the following decade, improvements in CMR technology and sequences improving the contrast between normal and infarcted myocardium, in concert with a proliferation of gadolinium-based contrast agents, led to gadolinium-enhanced MR becoming a cornerstone of CMR's clinical utility as a modality. In 2000, a seminal paper described the use of a 180 degree inversion-recovery preparatory pulse combined with a subsequent gradient echo image acquisition. In this method, normal myocardial signal relaxed to steady state at a different rate than infarcted tissue due to their difference in T1 relaxation times. Image acquisition at the inversion time when normal tissue had zero signal (the “null” point after the inversion pulse) provided a substantial improvement in contrast to noise ratio between the infarcted myocardial tissue and the adjacent normal myocardium ( 20 ). Images were acquired under steady state conditions (e.g., 10–30 min) after gadolinium contrast administration. The so-called “late gadolinium enhancement” or LGE CMR method led to a critical study by Kim et al. showing contrast-enhanced CMR could differentiate irreversible from reversible myocardial dysfunction prior to patients proceeding to percutaneous or surgical revascularisation ( 21 ).
Interpreting late-gadolinium enhanced images was extended to other pathologies, including infiltrative diseases and tumours. Patterns of mural distribution on LGE CMR images began to be described for both ischaemic and non-ischaemic cardiomyopathies. The extent of myocardial LGE appeared related to the risk of adverse cardiovascular events as well as to specific genotypes in the cases of Mendelian disease. At present, the LGE CMR method has become central to the characterisation of multiple types of acquired and genetic cardiac diseases.
Myocardial perfusion
Myocardial perfusion evaluation by CMR was first described in 1990 also by Atkinson and colleagues. They observed the first pass kinetics of a rapid bolus of gadolinium-DTPA through the cardiac chambers and myocardium ( 22 ). This technique was subsequently augmented by the introduction of common pharmacological cardiac agents such as dipyridamole ( 23 ) and dobutamine ( 24 ) through the 1990's. Today, a vasodilator approach using adenosine or regadenoson is preferred. Further technical developments in the pulse sequence design and contrast agents have led to stress perfusion now being a key component of the assessment of coronary artery disease using CMR. Stress CMR has been demonstrated to be a feasible and efficient tool for the diagnosis of myocardial ischaemia when compared to other non-invasive modalities such as SPECT in large prospective trials ( 25 ). Current advances in stress CMR have focused on quantification of myocardial blood flow in order to supplement the qualitative interpretation of images ( 26 ).
Quantitative CMR
The bulk of CMR interpretation relies on visual identification of varying myocardial signal intensity. However, these signals may also be quantified. Signal quantification in CMR is a technique that provides pixel-by-pixel representation of absolutely denominated numerical T1 or T2 properties, expressed in units of time (ms). These quantitative imaging approaches produce parametric maps of the entire myocardium, allowing rapid assessment of both diffuse and focal myocardial abnormalities.
The roots of parametric mapping emerged very early in the development of NMR. In particular, T1 relaxation experiments were slow, requiring many minutes (or even hours) to acquire depending on the signal magnitude. David Look and Donald Locker solved this in 1970 whilst grappling with methods to automate the challenge of calculating short T1 values ( 27 ). They published their method in 1970. Remarkably, Look and Locker only learnt of the new life their method had taken on within medical MRI in 2014 via a serendipitous email. To their surprise, the term “Look-Locker” had already been mentioned in more than 2,300 papers and 140 patents ( 28 ).
The Look-Locker technique was modified to perform measurement of myocardial T1 relaxation times within a single breath-hold by Messroghli and colleagues in 2004. Their pulse sequence, named MOLLI (MOdified Look-Locker Inversion recovery) ( 29 ), acquired an image where the signal intensity of each pixel corresponds to the T1 relaxation time of that pixel. Subsequent modifications of the original MOLLI sequence have led to the more exotic acronyms in the field of CMR such as shMOLLI, SASHA and SAPPHIRE, each representing modifications or alternatives to the original MOLLI scheme. With non-diseased myocardium having a predictable T1 relaxation time which becomes altered in the presence of pathology such as oedema, fibrosis, and infiltrative diseases, T1 mapping is now relatively routine within clinical workflows to detect focal or diffuse disease. Extensions of the approach such as extracellular myocardial volume quantification and T2 and T2* mapping also have now been incorporated into workstreams depending on the myocardial disease being studied.
The bridge to population science
The advancements described above have catapulted CMR from the relatively esoteric to being a mainstream clinical diagnostic modality. Moreover, with its accurate quantification came the opportunity to evaluate early, asymptomatic tissue remodelling not evident with other non-invasive techniques. It was quickly apparent that CMR had a role to play in assessing subclinical cardiovascular disease and how these insights could be used in risk assessment and prognostication.
By the 1980s, cardiovascular risk factors in the general population that led to clinical cardiovascular events were identified, most notably from work in the Framingham Study. In particular, age, gender, blood pressure and cholesterol elevation as well as diabetes were termed “traditional” risk factors that identified individuals at high risk for subsequent cardiovascular disease. Notably, most early population-based studies such as Framingham evaluated European ancestry populations, typically enrolling men. By the mid-1990s, the concept of “novel” risk factors took hold, with the premise that subclinical cardiovascular disease could be detected with new methods, and potentially modified to reduce clinical disease expression. Besides serum and genetic testing, advanced imaging methods were identified as methods to phenotype subclinical cardiovascular disease. In the United States, this led to the National Heart, Lung and Blood Institute (NHLBI)-sponsored Multi-Ethnic Study of Atherosclerosis (MESA) population-based cohort. The MESA study was also designed to reflect ethnic diversity of the United States population at that time. MESA investigators sought to maximise the statistical opportunities for risk factor association and prediction; this led to all MESA participants undergoing the same exhaustive battery of tests, often acquired over a multiple day interval.
The CMR component of MESA was led by David Bluemke and João Lima ( 30 ). More than 5,000 men and women without clinical cardiovascular disease underwent CMR examination at six university sites in the United States. Given the relative shortage of MRI scanner capacity, this represented an enormous commitment of scarce hospital resources to imaging apparently healthy individuals at a time when MRI was otherwise reserved for the most complex and in-need patients.
When the CMR MESA protocol was finalised (1999), Hawkes' SSFP cine sequences were non-standard and commercially available at only half of the CMR MESA sites. In order to provide standardisation of methods, the initial MESA CMR protocol used the better validated fast gradient-recalled echo cine imaging. MESA permitted large-scale exploration of cardiac structure and function and demonstrated its association with cardiovascular outcomes in the general population. As examples, novel insights into the importance of mass:volume ratio ( 31 ) as well as the relevance of the right ventricle ( 32 ), independent of the left ventricle, as biomarkers of subclinical cardiovascular disease in healthy individuals were novel. In 2010, the NHLBI authorised additional funding for re-examining a large portion of the original MESA cohort using CMR and other phenotypic and genotyping methods. This second CMR MESA examination added the use of gadolinium contrast to the base CMR protocol. LGE CMR contributed to understanding regarding unrecognised myocardial scar—demonstrating the strong association between scar and adverse cardiovascular events even in those without known myocardial infarction ( 33 ). In addition, gadolinium administration allowed pre- and post-contrast T1 mapping to be performed in more than 1,000 study participants.
As well as the scientific insights MESA has continued to provide since its conception a quarter of a century ago, another of its legacies is how it has allowed CMR to proliferate as the modality of choice in large-scale, prospective population studies across the world. Where MESA led, cohorts such as the Jackson Heart Study, Study of Health in Pomerania, Framingham Heart Study Offspring Cohort, Dallas Heart Study and AGES Reykjavik incorporated CMR into their population studies. The largest effort, however, was yet to come, spurred by the concept of genotype-phenotype association.
In the early 2000's, the Medical Research Council and Wellcome Trust decided to establish the UK Biobank cohort to investigate risk factors for diseases of middle and old age. Between 2,006–10, 500,000 individuals were recruited and extensive questionnaire data, physical measurements and biological samples collected. The desire to better understand the role of genetics in phenotypic and disease variation has been a major driver of the UK Biobank project and its sample size. As such, a key component has been the collection of whole-genome genetic data in every participant.
In 2009, three years since the first participants had begun being recruited, a proposal was submitted for conducting imaging assessments as an enhancement to the UK Biobank study. This was to be done in 100,000 of the 500,000 participants and that these were to be performed in regional, non-medical settings. This ambitious, perhaps even radical, plan was initially deferred by the funders but the UK Biobank imaging working group, in collaboration with international experts, returned with a bolstered case and were awarded funding for this unprecedented task. The CMR component of the imaging enhancement was led by Steffen Petersen, Stefan Piechnik and Stefan Neubauer. A pilot phase consisting of performing 5,000 examinations was successfully completed in 2014 with approval to proceed to 100,000; to date, over 60,000 participants have been imaged with funding recently agreed to additionally perform repeat examinations in 10,000 individuals.
CMR in the UK Biobank has provided many novel insights regarding associations with traditional and non-traditional cardiovascular risk factors, allowed development of novel imaging biomarkers and seen the publication of the first genome-wide association studies of CMR phenotypes ( 34 – 38 ). However, just as a legacy of MESA is in its inspiration of using CMR in population studies, it can be argued that the UK Biobank CMR effort too has a legacy of equal note to the science it has produced.
By 2015, the UK Biobank core lab had commenced work on manually segmenting the 5,000 pilot cases however, it was always clear that manual analysis of the intended 100,000 CMR examinations was never going to be feasible. Thus, UK Biobank provided not only the supply of ground truth but also the demand and impetus for a solution to be found. The manual segmentation by the core lab led to automated algorithms using deep learning methods for CMR analysis, first solved by Bai ( 39 ) and subsequently others ( 40 – 42 ). The ability to segment all four chambers, in any view, in every frame of the cardiac cycle, without any human input and all of this within seconds has been a profound legacy that the UK Biobank has left upon the field of CMR. This development led to another significant advance with the possibility of relating detailed CMR-derived imaging phenotypes to paired UK Biobank genomics, proteomics and metabolomics data.
It is nearly 90 years since Bloch and Purcell discovered NMR and just over 50 since Lauterbur and Mansfield recognised its application to medical imaging. In Look's personal reflections on the sequence that bears his name, he concludes by saying “the cost of research is small, but the long-term payoff can be huge”. It is a fool's errand to predict where CMR might be 50 years hence but as long as it continues to be supported and attracts individuals willing to innovate and push boundaries, it will continue to offer new solutions to the age-old challenge of cardiovascular disease.
Author contributions
MMS: Writing – original draft, Writing – review & editing. JACL: Writing – review & editing. DAB: Writing – review & editing. SEP: Writing – review & editing.
The author(s) declare that financial support was received for the research, authorship, and/or publication of this article.
MMS is supported by a British Heart Foundation Clinical Research Training Fellowship (FS/CRTF/22/24353). This work acknowledges the support of the National Institute for Health and Care Research Barts Biomedical Research Centre (NIHR203330); a delivery partnership of Barts Health NHS Trust, Queen Mary University of London, St George's University Hospitals NHS Foundation Trust and St George's University of London.
Conflict of interest
The authors declare that the research was conducted in the absence of any commercial or financial relationships that could be construed as a potential conflict of interest.
The reviewer PD declared a past co-authorship with the author SEP to the handling editor.
Publisher's note
All claims expressed in this article are solely those of the authors and do not necessarily represent those of their affiliated organizations, or those of the publisher, the editors and the reviewers. Any product that may be evaluated in this article, or claim that may be made by its manufacturer, is not guaranteed or endorsed by the publisher.
1. Gorter CJ. Negative result of an attempt to detect nuclear magnetic spins. Physica . (1936) 3:995–8. doi: 10.1016/S0031-8914(36)80324-3
Crossref Full Text | Google Scholar
2. Bloch F. Nuclear induction. Phys Rev . (1946) 70:460–74. doi: 10.1103/PhysRev.70.460
3. Purcell EM, Torrey HC, Pound RV. Resonance absorption by nuclear magnetic moments in a solid. Phys Rev . (1946) 69:37–8. doi: 10.1103/PhysRev.69.37
4. Ernst RR, Anderson WA. Application of fourier transform spectroscopy to magnetic resonance. Rev Sci Instrum . (1966) 37:93–102. doi: 10.1063/1.1719961
5. Damadian R. Tumor detection by nuclear magnetic resonance. Science . (1971) 171:1151–3. doi: 10.1126/science.171.3976.1151
PubMed Abstract | Crossref Full Text | Google Scholar
6. Lauterbur PC. Image formation by induced local interactions: examples employing nuclear magnetic resonance. Nature . (1973) 242:190–1. doi: 10.1038/242190a0
7. Mansfield P, Grannell PK. NMR “diffraction” in solids? J Phys C Solid State Phys . (1973) 6:L422. doi: 10.1088/0022-3719/6/22/007
8. Ernst RR. NMR fourier zeugmatography. J Magn Reson . (2011) 213:510–2. doi: 10.1016/j.jmr.2011.08.006
9. Kumar A, Welti D, Ernst RR. NMR fourier zeugmatography. J Magn Reson . (1975) 18:69–83. doi: 10.1016/0022-2364(75)90224-3
10. Mansfield P, Maudsley AA. Medical imaging by NMR. Br J Radiol . (1977) 50:188–94. doi: 10.1259/0007-1285-50-591-188
11. Damadian R, Goldsmith M, Minkoff L. NMR In cancer: XVI. FONAR image of the live human body. Physiol Chem Phys . (1977) 9:97–100. 108.909957
PubMed Abstract | Google Scholar
12. Clow H, Young IR. Britain’s brains produce first NMR scans. New Sci . (1978) 80:588.
Google Scholar
13. Hawkes RC, Holland GN, Moore WS, Roebuck EJ, Worthington BS. Nuclear magnetic resonance (NMR) tomography of the normal heart. J Comput Assist Tomogr . (1981) 5:605–12. doi: 10.1097/00004728-198110000-00001
14. Herfkens RJ, Higgins CB, Hricak H, Lipton MJ, Crooks LE, Lanzer P, et al. Nuclear magnetic resonance imaging of the cardiovascular system: normal and pathologic findings. Radiology . (1983) 147(3):749–59. doi: 10.1148/radiology.147.3.6601813
15. Stehling MK, Turner R, Mansfield P. Echo-planar imaging: magnetic resonance imaging in a fraction of a second. Science . (1991) 254:43–50. doi: 10.1126/science.1925560
16. Katz J, Milliken MC, Stray-Gundersen J, Buja LM, Parkey RW, Mitchell JH, et al. Estimation of human myocardial mass with MR imaging. Radiology . (1988) 169:495–8. doi: 10.1148/radiology.169.2.2971985
17. Klipstein RH, Firmin DN, Underwood SR, Nayler GL, Rees RS, Longmore DB. Colour display of quantitative blood flow and cardiac anatomy in a single magnetic resonance cine loop. Br J Radiol . (1987) 60:105–11. doi: 10.1259/0007-1285-60-710-105
18. Atkinson DJ, Edelman RR. Cineangiography of the heart in a single breath hold with a segmented turboFLASH sequence. Radiology . (1991) 178:357–60. doi: 10.1148/radiology.178.2.1987592
19. de Roos A, van Rossum AC, van der Wall E, Postema S, Doornbos J, Matheijssen N, et al. Reperfused and nonreperfused myocardial infarction: diagnostic potential of Gd-DTPA–enhanced MR imaging. Radiology . (1989) 172:717–20. doi: 10.1148/radiology.172.3.2772179
20. Simonetti OP, Kim RJ, Fieno DS, Hillenbrand HB, Wu E, Bundy JM, et al. An improved MR imaging technique for the visualization of myocardial infarction. Radiology . (2001) 218:215–23. doi: 10.1148/radiology.218.1.r01ja50215
21. Kim RJ, Wu E, Rafael A, Chen E-L, Parker MA, Simonetti O, et al. The use of contrast-enhanced magnetic resonance imaging to identify reversible myocardial dysfunction. N Engl J Med . (2000) 343:1445–53. doi: 10.1056/NEJM200011163432003
22. Atkinson DJ, Burstein D, Edelman RR. First-pass cardiac perfusion: evaluation with ultrafast MR imaging. Radiology . (1990) 174:757–62. doi: 10.1148/radiology.174.3.2305058
23. Pennell DJ, Underwood SR, Ell PJ, Swanton RH, Walker JM, Longmore DB. Dipyridamole magnetic resonance imaging: a comparison with thallium-201 emission tomography. Br Heart J . (1990) 64:362–9. doi: 10.1136/hrt.64.6.362
24. Pennell DJ, Underwood SR, Manzara CC, Swanton RH, Walker JM, Ell PJ, et al. Magnetic resonance imaging during dobutamine stress in coronary artery disease. Am J Cardiol . (1992) 70:34–40. doi: 10.1016/0002-9149(92)91386-i
25. Greenwood JP, Maredia N, Younger JF, Brown JM, Nixon J, Everett CC, et al. Cardiovascular magnetic resonance and single-photon emission computed tomography for diagnosis of coronary heart disease (CE-MARC): a prospective trial. Lancet Lond Engl . (2012) 379:453–60. doi: 10.1016/S0140-6736(11)61335-4
26. Hsu L-Y, Jacobs M, Benovoy M, Ta AD, Conn HM, Winkler S, et al. Diagnostic performance of fully automated pixel-wise quantitative myocardial perfusion imaging by cardiovascular magnetic resonance. JACC Cardiovasc Imaging . (2018) 11:697–707. doi: 10.1016/j.jcmg.2018.01.005
27. Look D, Locker D. Time saving in measurement of NMR and EPR relaxation times. Rev Sci Instrum . (1970) 41:250–1. doi: 10.1063/1.1684482
28. Look DC. The Look-Locker Method in Magnetic Resonance Imaging: A Brief, Personal History.
29. Messroghli DR, Radjenovic A, Kozerke S, Higgins DM, Sivananthan MU, Ridgway JP. Modified look-locker inversion recovery (MOLLI) for high-resolution T1 mapping of the heart. Magn Reson Med . (2004) 52:141–6. doi: 10.1002/mrm.20110
30. Bild DE, Bluemke DA, Burke GL, Detrano R, Diez Roux AV, Folsom AR, et al. Multi-ethnic study of atherosclerosis: objectives and design. Am J Epidemiol . (2002) 156:871–81. doi: 10.1093/aje/kwf113
31. Bluemke DA, Kronmal RA, Lima JAC, Liu K, Olson J, Burke GL, et al. The relationship of left ventricular mass and geometry to incident cardiovascular events: the MESA (multi-ethnic study of atherosclerosis) study. J Am Coll Cardiol . (2008) 52:2148–55. doi: 10.1016/j.jacc.2008.09.014
32. Kawut SM, Barr RG, Lima JAC, Praestgaard A, Johnson WC, Chahal H, et al. Right ventricular structure is associated with the risk of heart failure and cardiovascular death: the multi-ethnic study of atherosclerosis (MESA)–right ventricle study. Circulation . (2012) 126:1681–8. doi: 10.1161/CIRCULATIONAHA.112.095216
33. Ambale-Venkatesh B, Liu C-Y, Liu Y-C, Donekal S, Ohyama Y, Sharma RK, et al. Association of myocardial fibrosis and cardiovascular events: the multi-ethnic study of atherosclerosis. Eur Heart J Cardiovasc Imaging . (2019) 20:168–76. doi: 10.1093/ehjci/jey140
34. Petersen SE, Sanghvi MM, Aung N, Cooper JA, Paiva JM, Zemrak F, et al. The impact of cardiovascular risk factors on cardiac structure and function: insights from the UK biobank imaging enhancement study. PLoS One . (2017) 12:e0185114. doi: 10.1371/journal.pone.0185114
35. Sanghvi MM, Aung N, Cooper JA, Paiva JM, Lee AM, Zemrak F, et al. The impact of menopausal hormone therapy (MHT) on cardiac structure and function: insights from the UK biobank imaging enhancement study. PLoS One . (2018) 13:e0194015. doi: 10.1371/journal.pone.0194015
36. Aung N, Sanghvi MM, Piechnik SK, Neubauer S, Munroe PB, Petersen SE. The effect of blood lipids on the left ventricle: a mendelian randomization study. J Am Coll Cardiol . (2020) 76:2477–88. doi: 10.1016/j.jacc.2020.09.583
37. Aung N, Vargas JD, Yang C, Cabrera CP, Warren HR, Fung K, et al. Genome-wide analysis of left ventricular image-derived phenotypes identifies fourteen loci associated with cardiac morphogenesis and heart failure development. Circulation . (2019) 140:1318–30. doi: 10.1161/CIRCULATIONAHA.119.041161
38. Aung N, Vargas JD, Yang C, Fung K, Sanghvi MM, Piechnik SK, et al. Genome-wide association analysis reveals insights into the genetic architecture of right ventricular structure and function. Nat Genet . (2022) 54:783–91. doi: 10.1038/s41588-022-01083-2
39. Bai W, Sinclair M, Tarroni G, Oktay O, Rajchl M, Vaillant G, et al. Automated cardiovascular magnetic resonance image analysis with fully convolutional networks. J Cardiovasc Magn Reson Off J Soc Cardiovasc Magn Reson . (2018) 20:65. doi: 10.1186/s12968-018-0471-x
40. Attar R, Pereañez M, Gooya A, Albà X, Zhang L, de Vila MH, et al. Quantitative CMR population imaging on 20,000 subjects of the UK biobank imaging study: LV/RV quantification pipeline and its evaluation. Med Image Anal . (2019) 56:26–42. doi: 10.1016/j.media.2019.05.006
41. Davies RH, Augusto JB, Bhuva A, Xue H, Treibel TA, Ye Y, et al. Precision measurement of cardiac structure and function in cardiovascular magnetic resonance using machine learning. J Cardiovasc Magn Reson . (2022) 24:16. doi: 10.1186/s12968-022-00846-4
42. Chen C, Qin C, Qiu H, Tarroni G, Duan J, Bai W, et al. Deep learning for cardiac image segmentation: a review. Front Cardiovasc Med . (2020) 7:25. doi: 10.3389/fcvm.2020.00025
Keywords: history of medicine, magnetic resonance imaging, cardiovascular magnetic resonance (CMR) imaging, nuclear magnetic resonance, Multi-Ethnic Study of Atherosclerosis (MESA), UK Biobank
Citation: Sanghvi MM, Lima JAC, Bluemke DA and Petersen SE (2024) A history of cardiovascular magnetic resonance imaging in clinical practice and population science. Front. Cardiovasc. Med. 11:1393896. doi: 10.3389/fcvm.2024.1393896
Received: 29 February 2024; Accepted: 8 April 2024; Published: 19 April 2024.
Reviewed by:
© 2024 Sanghvi, Lima, Bluemke and Petersen. This is an open-access article distributed under the terms of the Creative Commons Attribution License (CC BY) . The use, distribution or reproduction in other forums is permitted, provided the original author(s) and the copyright owner(s) are credited and that the original publication in this journal is cited, in accordance with accepted academic practice. No use, distribution or reproduction is permitted which does not comply with these terms.
*Correspondence: Steffen E. Petersen [email protected]
This article is part of the Research Topic
Glimpse to the Past – the Evolution of the Role of Imaging in Cardio Therapeutics

An official website of the United States government
The .gov means it’s official. Federal government websites often end in .gov or .mil. Before sharing sensitive information, make sure you’re on a federal government site.
The site is secure. The https:// ensures that you are connecting to the official website and that any information you provide is encrypted and transmitted securely.
- Publications
- Account settings
Preview improvements coming to the PMC website in October 2024. Learn More or Try it out now .
- Advanced Search
- Journal List
- v.2(1); 2020

The role of artificial intelligence in medical imaging research
Xiaoli tang.
1 Yale New Haven Hospital, New Haven, USA
Without doubt, artificial intelligence (AI) is the most discussed topic today in medical imaging research, both in diagnostic and therapeutic. For diagnostic imaging alone, the number of publications on AI has increased from about 100–150 per year in 2007–2008 to 1000–1100 per year in 2017–2018. Researchers have applied AI to automatically recognizing complex patterns in imaging data and providing quantitative assessments of radiographic characteristics. In radiation oncology, AI has been applied on different image modalities that are used at different stages of the treatment. i.e . tumor delineation and treatment assessment. Radiomics, the extraction of a large number of image features from radiation images with a high-throughput approach, is one of the most popular research topics today in medical imaging research. AI is the essential boosting power of processing massive number of medical images and therefore uncovers disease characteristics that fail to be appreciated by the naked eyes. The objectives of this paper are to review the history of AI in medical imaging research, the current role, the challenges need to be resolved before AI can be adopted widely in the clinic, and the potential future.
A brief overview of the history
A handful of scientists from a variety of fields (mathematics, psychology, engineering, economics and political science) began to discuss the possibility of creating an artificial brain. They gathered together at a workshop held on the campus of Dartmouth College during the summer of 1956. This is widely known as Dartmouth Workshop, and it founded a society of artificial intelligence (AI). 1 The field then went through its peaks and valleys several cycles. MIT cognitive scientist Marvin Minsky along with other attendees at the Dartmouth Workshop were extremely optimistic about AI’s future. They believed that AI will substantially be solved within a generation. However, no significant progress was made. After several criticizing reports and ongoing pressure from congress, government funding and interests dropped off. 1974–90 became the first AI winter. In the 80’s, due to the competition of the British and Japan, AI revived. 1983–93 was a major winter for AI, coinciding with the collapse of the market for the needed computer power, which led to withdrawal of funding again. Research began to pick up again after that. One well-known event was IBM’s Deep Blue—the first computer beat a chess champion. In 2011, the computer giant’s question answering system Watson won the quiz show Jeopardy, and this marked the newest wave of AI booming. In Parallel of recent 10 years in medical imaging research, the amount of imaging data has grown exponentially. This has increased the burden to physicians to process the images. They need to read images with higher efficiency while maintain the same or better accuracy. At the same time, fortunately, computational power has also grown exponentially. These challenges and opportunities have formed the perfect foundation for the AI to be blossomed in the medical imaging research.
Researchers have successfully applied AI in radiology to identify findings either detectable or not by the human eye. Radiology is now moving from a subjective perceptual skill to a more objective science. 2,3 In Radiation Oncology, AI has been successfully applied to automatic tumor and organ segmentation, 4–6 7 8 and tumor monitoring during the treatment for adaptive treatment. In 2012, a Dutch researcher, Lambin P, proposed the concept of “Radiomics” for the first time and defined it as follows: the extraction of a large number of image features from radiation images with a high-throughput approach. 9 As AI became more popular and also more medical images than ever have been generated, these are good reason for radiomics to evolve rapidly. Radiomics is a novel approach for solving the issue of precision medicine. These researches have demonstrated a great potential of the role of AI in medical imaging. In fact, it has sparkled one of the ongoing discussions—will AI replace clinicians entirely? We believe it will not. For short term, AI is constrained by a lack of high quality, high volume, longitudinal, outcomes data, a constraint that is further exacerbated by the competing need for strict privacy protection. 10 There were approaches to address the privacy threat, like distributed learning. However, in a 2017 paper, it was argued that any distributed, federated, or decentralized deep learning approach is susceptible to attacks that reveal information about participant information from the training set. 11 For long term, we believe that AI will continue to underperform human level accuracy in medical decision making. Fundamentally, medicine is art, not science. AI might be able to outperform human in terms of quantitative tasks. Overall medical decision, however, will still depend on human evaluation to achieve the optimal results for a given patient.
Current role of AI in radiology
Machine learning, as a subset of AI, also called the traditional AI, was applied on diagnostic imaging started 1980’s. 12 Users first predefine explicit parameters and features of the imaging based on expert knowledge. For instance, the shapes, areas, histogram of image pixels of the regions-of-interest ( i.e. tumor regions) can be extracted. Usually, for a given number of available data entries, part of them are used as training and the rest would be for testing. Certain machine learning algorithm is selected for the training to understand the features. Some examples of the algorithms are principal component analysis (PCA), support vector machines (SVM), convolutional neural networks (CNN), etc. Then, for a given testing image, the trained algorithm is supposed to recognize the features and classify the image.
One of the problems of machine learning is that users need to select the features which define the class of the image it belongs to. However, this might miss some contributing factors. 12,13 For instance, lung tumor diagnosis requires user to segment the tumor region as structure features. Due to the patient and user variation, the consistency of the manual feature selection has always been a challenge. Deep learning, however, does not require explicit user input of the features. As its name suggests, deep learning learns from significantly more amount of data. It uses models of deep artificial neural networks. Deep learning uses multiple layers to progressively extract higher level features from raw image input. It helps to disentangle the abstractions and picks out the features that can improve performance. The concept of deep learning was proposed decades ago. Only till recent decade, the application of deep learning became feasible due to enormous number of medical images being produced and advancements in the development of hardware, like graphics processing units (GPU). 14 However, with machine learning gaining its relevance and importance every day, even GPU became somewhat lacking. To combat this situation, Google developed an AI accelerator integrated circuit which would be used by its TensorFlow AI framework—tensor processing unit (TPU). TPU is designed specifically for neural network machine learning and would have potential to be applied on medical imaging research as well.
The main research area in diagnostic imaging is detection. Researchers started developing computer-aided detection (CAD) systems in the 1980s. Traditional machine learning algorithms were applied on image modalities like CT, MRI, and mammography. Despite a lot of effort made in the research area, the real clinical applications were not promising. Several large trials came to the conclusion that CAD has at best delivered no benefit 15 and at worst has actually reduced radiology accuracy, 16 resulting in higher recall and biopsy rates. 17,18
The new era of AI—the deep learning has so far demonstrated promising improvements in the research area over the traditional machine learning. As an example, Ardila et al proposed a deep learning algorithm that uses a patient’s current and prior CT volumes to predict the risk of lung cancer. 19 The model achieved a state-of-the-art performance (94.4% area under the curve) on 6716 national lung cancer screening trial cases and performed similarly on an independent clinical validation set of 1139 cases. As a comparison of conventional screening by low-dose CT, per cancer.gov, 20 there are several associated harms: false-positive exams, overdiagnosis, complications of diagnostic evaluation, increase in lung cancer mortality, and radiation exposure. One false-positive exam example provided on the web site was 60%. Overdiagnosis was estimated at 67%. There is also radiation induced risk to develop lung cancer or other types of cancer later in life. AI-based diagnosis reduced these risks.
In fact, deep learning algorithms have become a methodology of choice for radiology imaging analysis. 20 This includes different image modalities like CT, MRI, PET, ultrasonography etc and different tasks like tumor detection, segmentation, disease prediction etc. Researches have shown that AI/deep learning-based methods have substantial performance improvements over the conventional machine learning algorithms. 21 Similar to human learning, deep learning learns from enormous amount of image examples. However, it might take much less time, as it solely depends on curated data and the corresponding metadata rather than the domain expertise, which usually takes years to develop. 12 As the traditional AI requires predefined features and have shown plateauing performance over recent years, and with the current success of AI/deep learning in image research, it is expected that AI will further dominate the image research in radiology.
Current role of AI in radiation oncology
In radiation oncology imaging research, AI has been applied in organ and lesion segmentation, image registration, fiducial/marker detection, radiomics etc. Similar to radiology, it started with traditional AI and now with deep learning. 3,22–24 25 26 In the most recent Medical Physics journal (May 2019, Volume 46, Issue 5), there were 16/51 papers on deep learning-based imaging research. As we know, imaging research is only one subsection of the entire radiation oncology research. The large portion of the published deep learning imaging research articles demonstrates the important role AI is now playing in the field.
For organ and lesion segmentation, the main goal is to segment the organs at risk automatically for treatment planning. Deep learning algorithms have been applied to segment head and neck organs, brain, lung, prostate, kidney, pelvis etc. Lesion segmentation applications include bladder, breast, bone, brain, head and neck, liver, lung, lymph nodes, rectum etc. Sahiner et al 23 has summarized the segmentation object, deep learning methods used, data set used, and the corresponding performance. One algorithm used often was U-net. 27 Unlike traditional AI, U-nets consist of several convolution layers, followed by deconvolution layers, with connections between the opposing convolution and deconvolution layers. The network can therefore analyze the entire image during training and allow for obtaining segmentation likelihood maps directly.
Dong et al applied U-net-generative adversarial network (U-Net-GAN) to train deep neural networks for the segmentation of multiple organs on thoracic CT images. 28 U-Net-GAN jointly trains a set of U-Nets as generators and fully convolutional networks (FCNs) as discriminators. The generator and discriminator compete against each other in an adversarial learning process to produce the optimal segmentation map of multiple organs. The proposed algorithm was demonstrated feasible and reliable in segmenting five different organs. Similarly, Feng et al successfully applied deep convolutional neural networks (DCNN) for thoracic organs at risks segmentation using cropped hree-dimensional images. 29 CNN has also been used on head and neck organ segmentation. 30
Holistically nested networks (HNN) uses side outputs of the convolutional layers, and it has been applied on prostate and brain tumor segmentation. 31,32
In radiation therapy, often there are needs to register one image modality to another (multimodal) or an image on a one day to another (monomodal). To avoid traditional AI which required handcrafted features, an unsupervised deep learning feature selection framework was proposed. It implemented a convolutional stacked auto-encoder network to identify the intrinsic features in image patches. 33 The algorithm demonstrated better Dice ratio scores compares to state of the art. These can be applied on both multimodal and monomodal image registrations. Sloan et al 34 have proposed a novel method of image registration by regressing the transformation parameters using a convolutional neural network (CNN). This was applied on both mono- and multimodal applications. With the promising result AI has demonstrated so far in the research domain, we hope the AI-based image registration can be applied in the clinic soon. This is an important step towards real-time adaptive treatment planning and delivery.
The automatic fiducial/marker detection is needed for real time tracking of the treatment area during the delivery. Most common methods require prior knowledge of the marker properties to construct a template. Recent proposed deep learning CNN framework requires no prior knowledge of marker properties or additional learning periods to segment cylindrical and arbitrarily shaped fiducial markers. 22 The algorithm achieved high classification performance.
Radiomics, one of the most advanced AI applications in medical imaging research, is a novel approach towards the precision medicine. 35 Radiomics consists two steps. First step is feature extraction. Images from multiple modalities might be included. Image segmentation algorithms are applied to segment the volumes of interest. After the segmentation, features will be extracted. Common features include texture, geometric information, tumor volume, shape, density, pixel intensity etc. The second step is to incorporate the extracted features into mathematical models to decoding the phenotype of the tumor for treatment outcome prediction. A successful outcome prediction can provide valuable information for precise treatment design. For instance, different lung cancer patients might share many similarities like histology and age. However, the images of the tumor might appear different, and the survival time might be very different. 36 If radiomics can take the image information, decode the phenotype, and therefore predict the survival time or prognosis prior to the treatment, different treatment regimens might be chosen. This is called personalized or precision medicine. 35 Traditionally, precision medicine depended on biomarkers to estimate patient different prognosis or subtype, which usually required invasive biopsy. Radiomics, on the other hand, does not require invasive procedures. It was shown that features extracted from CT images of lung cancer patients alone correlate well with gene mutations and have prognostic powers. 37 The success of radiomics can potentially avoid undesirable complications caused by biopsy 38,39 and achieve the same or better prediction outcome.
Aerts et al 37 built a radiomic signature, assessed on an independent lung data set. It demonstrated the translational capability of radiomics across different cancers. Authors also showed significant associations between the radiomic features and gene-expression patterns. Some researchers did radiomics modeling using positron emission tomography (PET) images, 40 PET/CT or PET/MRI. 41 Most applications were on lung cancer. There are also applications on head and neck 42 and prostate cancers. 43 All these models have achieved reasonable prediction power.
Challenges need to be resolved before clinical implementation
Despite the excitement AI has generated in the medical imaging research, there are challenges before it can become more robust and be widely adopted in the clinic. AI is constrained by a lack of high quality, high volume, longitudinal, outcomes data. Even the same image modality on the same disease site, the parameters of the imaging setting and protocols might be different in different clinical settings. Each set of images is associated with a clinical scenario. The number of potential clinical scenarios and the variety of tasks that each of the image might contain is astronomical and might be impossible to be tacked by one organization with any AI algorithm. Each patient cohort associated with a clinic is different. The way each clinic practices is also different. How to organize the data generated from different practices in a more standard way is a big challenge on AI-based medical imaging research. Medical imaging data organization itself might deserve to be a major research field.
There are challenges associated with medical imaging data curation. 44 45 Data curation is an important step. Accurate labeling therefore is a key. As the exponentially growth of the number of images, clinicians have challenges to process them with the same efficiency and accuracy. It usually takes years to train people to become experts. Therefore, the lack of ability to keep up labeling enormous number of images imposes limitations of the data curation.
On the policy level, there are increasing concerns on patient privacy. Patient-related health information was protected by tight privacy policies, which limited cross-institution image sharing. Recently, there were several headline news level healthcare data breaches and security attacks. As a result, hospitals are now more than ever concerning about securities and liabilities and have tightened up security and data sharing policies. However, the success implementations of AI needs large amount of data from multiple institutions. How to share images without compromising security is a challenge.
The future of AI in medical imaging research
Two challenges need to be resolved before AI can be more widely implemented in medical imaging research. First, how to organize and pre-process data generated from different institutions. Miotto et al stated in their breakthrough work “deep patient”—challenges in summarizing and representing patient data prevent widespread practice of predictive modeling using electronic health records. They presented a novel unsupervised deep feature learning method to derive a general-purpose patient representation from electronic health record data that facilitates clinical predictive modeling. 46 Authors have successfully derived patient representations from a large-scale data set that were not optimized for any specific task and can fit different clinical applications. However, their data are from one institution. Tackling data set from multiple institutions in fact is a much more challenging task. Even for the same procedure, different institution might implement differently. Patient cohorts might also be different. All these will need to be addressed when pre-process data for AI algorithm.
Second, on a policy or infrastructure level, how to encourage more image data sharing is also a challenge. Currently, image data sharing is very limited. HIPAA compliant is one concern, and lack of infrastructure is another. The medical data security needs to work with the emerging needs of data sharing. Corresponding infrastructure also needs to be built.
On the long run, how AI can become true “intelligent” at the human level is a key to the question if AI can replace human in medical imaging. Unlike pure quantitative task, the knowledge involved in medical imaging related decision making require life experience and philosophy. For the machine to behave in human level, there are not only challenges on data collection and algorithm development, but also on ethical regulations.
Conclusions
AI is playing a significant role in medical imaging researches. It changed the way people process the enormous number of images. There are still challenges to be resolved before AI can eventually impact clinical practices.
Thank you for visiting nature.com. You are using a browser version with limited support for CSS. To obtain the best experience, we recommend you use a more up to date browser (or turn off compatibility mode in Internet Explorer). In the meantime, to ensure continued support, we are displaying the site without styles and JavaScript.
- View all journals
- My Account Login
- Explore content
- About the journal
- Publish with us
- Sign up for alerts
- Review Article
- Open access
- Published: 12 April 2022
Machine learning for medical imaging: methodological failures and recommendations for the future
- Gaël Varoquaux 1 , 2 , 3 &
- Veronika Cheplygina ORCID: orcid.org/0000-0003-0176-9324 4
npj Digital Medicine volume 5 , Article number: 48 ( 2022 ) Cite this article
62k Accesses
184 Citations
280 Altmetric
Metrics details
- Computer science
- Medical research
- Research data
Research in computer analysis of medical images bears many promises to improve patients’ health. However, a number of systematic challenges are slowing down the progress of the field, from limitations of the data, such as biases, to research incentives, such as optimizing for publication. In this paper we review roadblocks to developing and assessing methods. Building our analysis on evidence from the literature and data challenges, we show that at every step, potential biases can creep in. On a positive note, we also discuss on-going efforts to counteract these problems. Finally we provide recommendations on how to further address these problems in the future.
Similar content being viewed by others
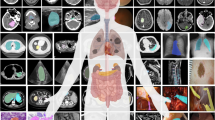
Segment anything in medical images
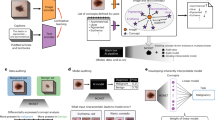
Transparent medical image AI via an image–text foundation model grounded in medical literature
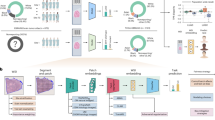
Demographic bias in misdiagnosis by computational pathology models
Introduction.
Machine learning, the cornerstone of today’s artificial intelligence (AI) revolution, brings new promises to clinical practice with medical images 1 , 2 , 3 . For example, to diagnose various conditions from medical images, machine learning has been shown to perform on par with medical experts 4 . Software applications are starting to be certified for clinical use 5 , 6 . Machine learning may be the key to realizing the vision of AI in medicine sketched several decades ago 7 .
The stakes are high, and there is a staggering amount of research on machine learning for medical images. But this growth does not inherently lead to clinical progress. The higher volume of research could be aligned with the academic incentives rather than the needs of clinicians and patients. For example, there can be an oversupply of papers showing state-of-the-art performance on benchmark data, but no practical improvement for the clinical problem. On the topic of machine learning for COVID, Robert et al. 8 reviewed 62 published studies, but found none with potential for clinical use.
In this paper, we explore avenues to improve clinical impact of machine learning in medical imaging. After sketching the situation, documenting uneven progress in Section It’s not all about larger datasets, we study a number of failures frequent in medical imaging papers, at different steps of the “publishing lifecycle”: what data to use (Section Data, an imperfect window on the clinic), what methods to use and how to evaluate them (Section Evaluations that miss the target), and how to publish the results (Section Publishing, distorted incentives). In each section, we first discuss the problems, supported with evidence from previous research as well as our own analyses of recent papers. We then discuss a number of steps to improve the situation, sometimes borrowed from related communities. We hope that these ideas will help shape research practices that are even more effective at addressing real-world medical challenges.
It’s not all about larger datasets
The availability of large labeled datasets has enabled solving difficult machine learning problems, such as natural image recognition in computer vision, where datasets can contain millions of images. As a result, there is widespread hope that similar progress will happen in medical applications, algorithm research should eventually solve a clinical problem posed as discrimination task. However, medical datasets are typically smaller, on the order of hundreds or thousands: 9 share a list of sixteen “large open source medical imaging datasets”, with sizes ranging from 267 to 65,000 subjects. Note that in medical imaging we refer to the number of subjects, but a subject may have multiple images, for example, taken at different points in time. For simplicity here we assume a diagnosis task with one image/scan per subject.
Few clinical questions come as well-posed discrimination tasks that can be naturally framed as machine-learning tasks. But, even for these, larger datasets have to date not lead to the progress hoped for. One example is that of early diagnosis of Alzheimer’s disease (AD), which is a growing health burden due to the aging population. Early diagnosis would open the door to early-stage interventions, most likely to be effective. Substantial efforts have acquired large brain-imaging cohorts of aging individuals at risk of developing AD, on which early biomarkers can be developed using machine learning 10 . As a result, there have been steady increases in the typical sample size of studies applying machine learning to develop computer-aided diagnosis of AD, or its predecessor, mild cognitive impairment. This growth is clearly visible in publications, as on Fig. 1 a, a meta-analysis compiling 478 studies from 6 systematic reviews 4 , 11 , 12 , 13 , 14 , 15 .
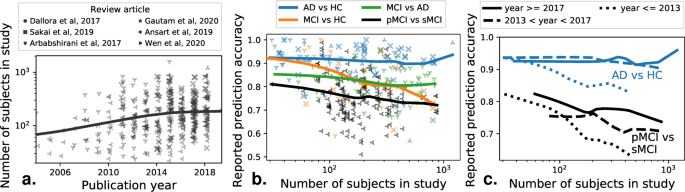
A meta-analysis across 6 review papers, covering more than 500 individual publications. The machine-learning problem is typically formulated as distinguishing various related clinical conditions, Alzheimer’s Disease (AD), Healthy Control (HC), and Mild Cognitive Impairment, which can signal prodromal Alzheimer’s . Distinguishing progressive mild cognitive impairment (pMCI) from stable mild cognitive impairment (sMCI) is the most relevant machine-learning task from the clinical standpoint. a Reported sample size as a function of the publication year of a study. b Reported prediction accuracy as a function of the number of subjects in a study. c Same plot distinguishing studies published in different years.
However, the increase in data size (with the largest datasets containing over a thousand subjects) did not come with better diagnostic accuracy, in particular for the most clinically relevant question, distinguishing pathological versus stable evolution for patients with symptoms of prodromal Alzheimer’s (Fig. 1 b). Rather, studies with larger sample sizes tend to report worse prediction accuracy. This is worrisome, as these larger studies are closer to real-life settings. On the other hand, research efforts across time did lead to improvements even on large, heterogeneous cohorts (Fig. 1 c), as studies published later show improvements for large sample sizes (statistical analysis in Supplementary Information) . Current medical-imaging datasets are much smaller than those that brought breakthroughs in computer vision. Although a one-to-one comparison of sizes cannot be made, as computer vision datasets have many classes with high variation (compared to few classes with less variation in medical imaging), reaching better generalization in medical imaging may require assembling significantly larger datasets, while avoiding biases created by opportunistic data collection, as described below.
Data, an imperfect window on the clinic
Datasets may be biased: reflect an application only partly.
Available datasets only partially reflect the clinical situation for a particular medical condition, leading to dataset bias 16 . As an example, a dataset collected as part of a population study might have different characteristics that people who are referred to the hospital for treatment (higher incidence of a disease). As the researcher may be unaware of the corresponding dataset bias is can lead to important that shortcomings of the study. Dataset bias occurs when the data used to build the decision model (the training data), has a different distribution than the data on which it should be applied 17 (the test data). To assess clinically-relevant predictions, the test data must match the actual target population, rather than be a random subset of the same data pool as the train data, the common practice in machine-learning studies. With such a mismatch, algorithms which score high in benchmarks can perform poorly in real world scenarios 18 . In medical imaging, dataset bias has been demonstrated in chest X-rays 19 , 20 , 21 , retinal imaging 22 , brain imaging 23 , 24 , histopathology 25 , or dermatology 26 . Such biases are revealed by training and testing a model across datasets from different sources, and observing a performance drop across sources.
There are many potential sources of dataset bias in medical imaging, introduced at different phases of the modeling process 27 . First, a cohort may not appropriately represent the range of possible patients and symptoms, a bias sometimes called spectrum bias 28 . A detrimental consequence is that model performance can be overestimated for different groups, for example between male and female individuals 21 , 26 . Yet medical imaging publications do not always report the demographics of the data.
Imaging devices or procedures may lead to specific measurement biases. A bias particularly harmful to clinically relevant automated diagnosis is when the data capture medical interventions. For instance, on chest X-ray datasets, images for the “pneumothorax” condition sometimes show a chest drain, which is a treatment for this condition, and which would not yet be present before diagnosis 29 . Similar spurious correlations can appear in skin lesion images due to markings placed by dermatologists next to the lesions 30 .
Labeling errors can also introduce biases. Expert human annotators may have systematic biases in the way they assign different labels 31 , and it is seldom possible to compensate with multiple annotators. Using automatic methods to extract labels from patient reports can also lead to systematic errors 32 . For example, a report on a follow-up scan that does not mention previously-known findings, can lead to an incorrect “negative” labels.
Dataset availability distorts research
The availability of datasets can influence which applications are studied more extensively. A striking example can be seen in two applications of oncology: detecting lung nodules, and detecting breast tumors in radiological images. Lung datasets are widely available on Kaggle or grand-challenge.org , contrasted with (to our knowledge) only one challenge focusing on mammograms. We look at the popularity of these topics, here defined by the fraction of papers focusing on lung or breast imaging, either in literature on general medical oncology, or literature on AI. In medical oncology this fraction is relatively constant across time for both lung and breast imaging, but in the AI literature lung imaging publications show a substantial increase in 2016 (Fig. 2 , methodological details in Supplementary Information ). We suspect that the Kaggle lung challenges published around that time contributed to this disproportional increase. A similar point on dataset trends has been made throughout the history of machine learning in general 33 .
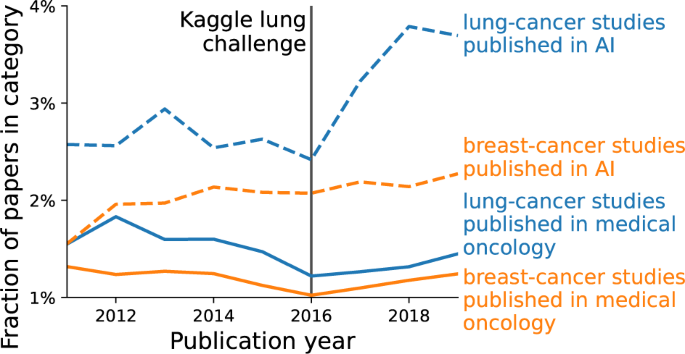
We show the percentage of papers on lung cancer (in blue) vs breast cancer (in red), relative to all papers within two fields: medical oncology (solid line) and AI (dotted line). Details on how the papers are selected are given in the Supplementary Information) . The percentages are relatively constant, except lung cancer in AI, which shows an increase after 2016.
Let us build awareness of data limitations
Addressing such problems arising from the data requires critical thinking about the choice of datasets, at the project level, i.e. which datasets to select for a study or a challenge, and at a broader level, i.e. which datasets we work on as a community.
At the project level, the choice of the dataset will influence the models trained on the data, and the conclusions we can draw from the results. An important step is using datasets from multiple sources, or creating robust datasets from the start when feasible 9 . However, existing datasets can still be critically evaluated for dataset bias 34 , hidden subgroups of patients 29 , or mislabeled instances 35 . A checklist for such evaluation on computer vision datasets is presented in Zendel et al. 18 . When problems are discovered, relabeling a subset of the data can be a worthwhile investment 36 .
At the community level, we should foster understanding of the datasets’ limitations. Good documentation of datasets should describe their characteristics and data collection 37 . Distributed models should detail their limitations and the choices made to train them 38 .
Meta-analyses which look at evolution of dataset use in different areas are another way to reflect on current research efforts. For example, a survey of crowdsourcing in medical imaging 39 shows a different distribution of applications than surveys focusing on machine learning 1 , 2 . Contrasting more clinically-oriented venues to more technical venues can reveal opportunities for machine learning research.
Evaluations that miss the target
Evaluation error is often larger than algorithmic improvements.
Research on methods often focuses on outperforming other algorithms on benchmark datasets. But too strong a focus on benchmark performance can lead to diminishing returns , where increasingly large efforts achieve smaller and smaller performance gains. Is this also visible in the development of machine learning in medical imaging?
We studied performance improvements in 8 Kaggle medical-imaging challenges, 5 on detection of diagnosis of diseases and 3 on image segmentation (details in Supplementary Information) . We use the differences in algorithms performance between the public and private leaderboards (two test sets used in the challenge) to quantify the evaluation noise –the spread of performance differences between the public and private test sets–, in Fig. 3 . We compare its distribution to the winner gap —the difference in performance between the best algorithm, and the “top 10%” algorithm.
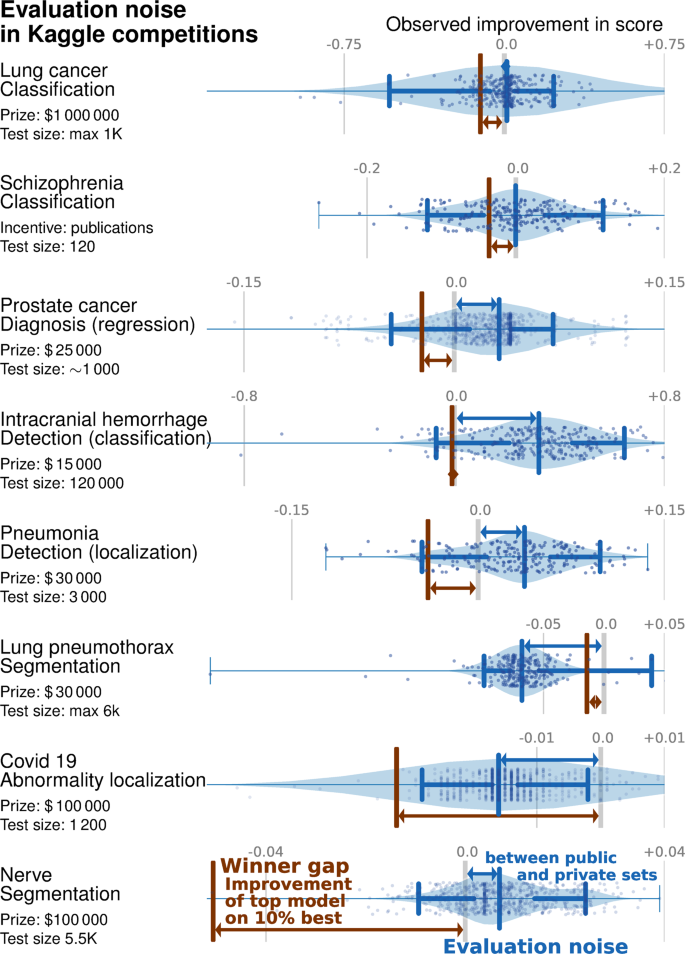
The blue violin plot shows the evaluation noise —the distribution of differences between public and private leaderboards. A systematic shift between public and private set (positive means that the private leaderboard is better than the public leaderboard) indicates overfitting or dataset bias. The width of this distribution shows how noisy the evaluation is, or how representative the public score is for the private score. The brown bar is the winner gap , the improvement between the top-most model (the winner) and the 10% best model. It is interesting to compare this improvement to the shift and width in the difference between the public and private sets: if the winner gap is smaller, the 10% best models reached diminishing returns and did not lead to a actual improvement on new data.
Overall, 6 of the 8 challenges are in the diminishing returns category. For 5 challenges—lung cancer, schizophrenia, prostate cancer diagnosis and intracranial hemorrhage detection—the evaluation noise is worse than the winner gap. In other words, the gains made by the top 10% of methods are smaller than the expected noise when evaluating a method.
For another challenge, pneumothorax segmentation, the performance on the private set is worse than on the public set, revealing an overfit larger than the winner gap. Only two challenges (covid 19 abnormality and nerve segmentation) display a winner gap larger than the evaluation noise, meaning that the winning method made substantial improvements compared to the 10% competitor.
Improper evaluation procedures and leakage
Unbiased evaluation of model performance relies on training and testing the models with independent sets of data 40 . However incorrect implementations of this procedure can easily leak information, leading to overoptimistic results. For example some studies classifying ADHD based on brain imaging have engaged in circular analysis 41 , performing feature selection on the full dataset, before cross-validation. Another example of leakage arises when repeated measures of an individual are split across train and test set, the algorithm then learning to recognize the individual patient rather than markers of a condition 42 .
A related issue, yet more difficult to detect, is what we call “overfitting by observer”: even when using cross-validation, overfitting may still occur by the researcher adjusting the method to improve the observed cross-validation performance, which essentially includes the test folds into the validation set of the model. Skocik et al. 43 provide an illustration of this phenomenon by showing how by adjusting the model this way can lead to better-than-random cross-validation performance for randomly generated data. This can explain some of the overfitting visible in challenges (Section Evaluation error is often larger than algorithmic improvements), though with challenges a private test set reveals the overfitting, which is often not the case for published studies. Another recommendation for challenges would be to hold out several datasets (rather than a part of the same dataset), as is for example done in the Decathlon challenge 44 .
Metrics that do not reflect what we want
Evaluating models requires choosing a suitable metric. However, our understanding of “suitable” may change over time. For example, an image similarity metric which was widely used to evaluate image registration algorithms, was later shown to be ineffective as scrambled images could lead to high scores 45 .
In medical image segmentation, Maier-Hein et al. 46 review 150 challenges and show that the typical metrics used to rank algorithms are sensitive to different variants of the same metric, casting doubt on the objectivity of any individual ranking.
Important metrics may be missing from evaluation. Next to typical classification metrics (sensitivity, specificity, area under the curve), several authors argue for a calibration metric that compares the predicted and observed probabilities 28 , 47 .
Finally, the metrics used may not be synonymous with practical improvement 48 , 49 . For example, typical metrics in computer vision do not reflect important aspects of image recognition, such as robustness to out-of-distribution examples 49 . Similarly, in medical imaging, improvements in traditional metrics may not necessarily translate to different clinical outcomes, e.g. robustness may be more important than an accurate delineation in a segmentation application.
Incorrectly chosen baselines
Developing new algorithms builds upon comparing these to baselines. However, if these baselines are poorly chosen, the reported improvement may be misleading.
Baselines may not properly account for recent progress, as revealed in machine-learning applications to healthcare 50 , but also other applications of machine learning 51 , 52 , 53 .
Conversely, one should not forget simple approaches effective for the problem at hand. For example, Wen et al. 14 show that convolutional neural networks do not outperform support vector machines for Alzheimer’s disease diagnosis from brain imaging.
Finally, minute implementation details of algorithms may be important and many are not aware of implementation factors 54 .
Statistical significance not tested, or misunderstood
Experimental results are by nature noisy: results may depend on which specific samples were used to train the models, the random initializations, small differences in hyper-parameters 55 . However, benchmarking predictive models currently lacks well-adopted statistical good practices to separate out noise from generalizable findings.
A first, well-documented, source of brittleness arises from machine-learning experiments with too small sample sizes 56 . Indeed, testing predictive modeling requires many samples, more than conventional inferential studies, else the measured prediction accuracy may be a distant estimation of real-life performance. Sample sizes are growing, albeit slowly 57 . On a positive note, a meta-analysis of public vs private leaderboards on Kaggle 58 suggests that overfitting is less of an issue with “large enough” test data (at least several thousands).
Another challenge is that strong validation of a method requires it to be robust to details of the data. Hence validation should go beyond a single dataset, and rather strive for statistical consensus across multiple datasets 59 . Yet, the corresponding statistical procedures require dozens of datasets to establish significance and are seldom used in practice. Rather, medical imaging research often reuses the same datasets across studies, which raises the risk of finding an algorithm that performs well by chance, in an implicit multiple comparison problem 60 .
But overall medical imaging research seldom analyzes how likely empirical results are to be due to chance: only 6% of segmentation challenges surveyed 61 , and 15% out of 410 popular computer science papers published by ACM used a statistical test 62 .
However, null-hypothesis tests are often misinterpreted 63 , with two notable challenges: (1) the lack of statistically significant results does not demonstrate the absence of effect, and (2) any trivial effect can be significant given enough data 64 , 65 . For these reasons, Bouthiellier et al. 66 recommend to replace traditional null-hypothesis testing with superiority testing , testing that the improvement is above a given threshold.
Let us redefine evaluation
Higher standards for benchmarking.
Good machine-learning benchmarks are difficult. We compile below several recognized best practices for medical machine learning evaluation 28 , 40 , 67 , 68 :
Safeguarding from data leakage by separating out all test data from the start, before any data transformation.
A documented way of selecting model hyper-parameters (including architectural parameters for neural networks, the use of additional (unlabeled) dataset or transfer learning 2 ), without ever using data from the test set.
Enough data in the test set to bring statistical power, at least several hundreds samples, ideally thousands or more 9 , and confidence intervals on the reported performance metric—see Supplementary Information . In general, more research on appropriate sample sizes for machine learning studies would be helpful.
Rich data to represent the diversity of patients and disease heterogeneity, ideally multi-institutional data including all relevant patient demographics and disease state, with explicit inclusion criteria; other cohorts with different recruitment go the extra mile to establish external validity 69 , 70 .
Strong baselines that reflect the state of the art of machine-learning research, but also historical solutions including clinical methodologies not necessarily relying on medical imaging.
A discussion the variability of the results due to arbitrary choices (random seeds) and data sources with an eye on statistical significance—see Supplementary Information .
Using different quantitative metrics to capture the different aspects of the clinical problem and relating them to relevant clinical performance metrics. In particular, the potential health benefits from a detection of the outcome of interest should be used to choose the right trade off between false detections and misses 71 .
Adding qualitative accounts and involving groups that will be most affected by the application in the metric design 72 .
More than beating the benchmark
Even with proper validation and statistical significance testing, measuring a tiny improvement on a benchmark is seldom useful. Rather, one view is that, beyond rejecting a null, a method should be accepted based on evidence that it brings a sizable improvement upon the existing solutions. This type of criteria is related to superiority tests sometimes used in clinical trials 73 , 74 , 75 . These tests are easy to implement in predictive modeling benchmarks, as they amount to comparing the observed improvement to variation of the results due to arbitrary choices such as data sampling or random seeds 55 .
Organizing blinded challenges, with a hidden test set, mitigate the winner’s curse. But to bring progress, challenges should not only focus on the winner. Instead, more can be learned by comparing the competing methods and analyzing the determinants of success, as well as failure cases.
Evidence-based medicine good practices
A machine-learning algorithm deployed in clinical practice is a health intervention. There is a well-established practice to evaluate the impact of health intervention, building mostly on randomized clinical trials 76 . These require actually modifying patients’ treatments and thus should be run only after thorough evaluation on historical data.
A solid trial evaluates a well-chosen measure of patient health outcome, as opposed to predictive performance of an algorithm. Many indirect mechanisms may affect this outcome, including how the full care processes adapts to the computer-aided decision. For instance, a positive consequence of even imperfect predictions may be reallocating human resources to complex cases. But a negative consequence may be over-confidence leading to an increase in diagnostic errors. Cluster randomized trials can account for how modifications at the level of care unit impact the individual patient: care units, rather than individuals are randomly allocated to receive the intervention (the machine learning algorithm) 77 . Often, double blind is impossible: the care provider is aware of which arm of the study is used, the baseline condition or the system evaluated. Providers’ expectations can contribute to the success of a treatment, for instance via indirect placebo or nocebo effects 78 , making objective evaluation of the health benefits challenging, if these are small.
Publishing, distorted incentives
No incentive for clarity.
The publication process does not create incentives for clarity. Efforts to impress may give rise to unnecessary “mathiness” of papers or suggestive language 79 (such as “human-level performance”).
Important details may be omitted, from ablation experiments showing what part of the method drives improvements 79 , to reporting how algorithms were evaluated in a challenge [ 46 ]. This in turn undermines reproducibility: being able to reproduce the exact results or even draw the same conclusions 80 , 81 .
Optimizing for publication
As researchers our goal should be to solve scientific problems. Yet, the reality of the culture we exist in can distort this objective. Goodhart’s law summarizes well the problem: when a measure becomes a target, it ceases to be a good measure . As our academic incentive system is based publications, it erodes their scientific content via Goodhart’s law.
Methods publication are selected for their novelty. Yet, comparing 179 classifiers on 121 datasets shows no statistically significant differences between the top methods [ 82 ]. In order to sustain novelty, researchers may be introducing unnecessary complexity into the methods, that do not improve their prediction but rather contribute to technical debt, making systems harder to maintain and deploy 83 .
Another metric emphasized is obtaining “state-of-the-art” results, which leads to several of the evaluation problems outlined in Section Evaluations that miss the target. The pressure to publish “good” results can aggravate methodological loopholes 84 , for instance gaming the evaluation in machine learning 85 . It is then all too appealing to find after-the-fact theoretical justifications of positive yet fragile empirical findings. This phenomenon, known as HARKing (hypothesizing after the results are known) 86 , has been documented in machine learning 87 and computer science in general 62 .
Finally, the selection of publications creates the so-called “file drawer problem” 88 : positive results, some due to experimental flukes, are more likely to be published than corresponding negative findings. For example, in 410 most downloaded papers from the ACM, 97% of the papers which used significance testing had a finding with p -value of less than 0.05 62 . It seems highly unlikely that only 3% of the initial working hypotheses—even for impactful work—turned out not confirmed.
Let us improve our publication norms
Fortunately there are various alleys to improve reporting and transparency. For instance, the growing set of open datasets could be leveraged for collaborative work beyond the capacities of a single team 89 . The set of metrics studied could then be broadened, shifting the publication focus away from a single-dimension benchmark. More metrics can indeed help understanding a method’s strengths and weaknesses 41 , 90 , 91 , exploring for instance calibration metrics 28 , 47 , 92 or learning curves 93 . The medical-research literature has several reporting guidelines for prediction studies 67 , 94 , 95 . They underline many points raised in previous sections: reporting on how representative the study sample is, on the separation between train and test data, on the motivation for the choice of outcome, evaluation metrics, and so forth. Unfortunately, algorithmic research in medical imaging seldom refers to these guidelines.
Methods should be studied on more than prediction performance: reproducibility 81 , carbon footprint 96 , or a broad evaluation of costs should be put in perspective with the real-world patient outcomes, from a putative clinical use of the algorithms 97 .
Preregistration or registered reports can bring more robustness and trust: the motivation and experimental setup of a paper are to be reviewed before empirical results are available, and thus the paper is be accepted before the experiments are run 98 . Translating this idea to machine learning faces the challenge that new data is seldom acquired in a machine learning study, yet it would bring sizeable benefits 62 , 99 .
More generally, accelerating the progress in science calls for accepting that some published findings are sometimes wrong 100 . Popularizing different types of publications may help, for example publishing negative results 101 , replication studies 102 , commentaries 103 and reflections on the field 68 or the recent NeurIPS Retrospectives workshops. Such initiatives should ideally be led by more established academics, and be welcoming of newcomers 104 .
Conclusions
Despite great promises, the extensive research in medical applications of machine learning seldom achieves a clinical impact. Studying the academic literature and data-science challenges reveals troubling trends: accuracy on diagnostic tasks progresses slower on research cohorts that are closer to real-life settings; methods research is often guided by dataset availability rather than clinical relevance; many developments of model bring improvements smaller than the evaluation errors. We have surveyed challenges of clinical machine-learning research that can explain these difficulties. The challenges start with the choice of datasets, plague model evaluation, and are amplified by publication incentives. Understanding these mechanisms enables us to suggest specific strategies to improve the various steps of the research cycle, promoting publications best practices 105 . None of these strategies are silver-bullet solutions. They rather require changing procedures, norms, and goals. But implementing them will help fulfilling the promises of machine-learning in healthcare: better health outcomes for patients with less burden on the care system.
Data availability
For reproducibility, all data used in our analyses are available on https://github.com/GaelVaroquaux/ml_med_imaging_failures .
Code availability
For reproducibility, all code for our analyses is available on https://github.com/GaelVaroquaux/ml_med_imaging_failures .
Litjens, G. et al. A survey on deep learning in medical image analysis. Med. Image Anal. 42 , 60–88 (2017).
Article PubMed Google Scholar
Cheplygina, V., de Bruijne, M. & Pluim, J. P. W. Not-so-supervised: a survey of semi-supervised, multi-instance, and transfer learning in medical image analysis. Med. Image Anal. 54 , 280–296 (2019).
Zhou, S. K. et al. A review of deep learning in medical imaging: Image traits, technology trends, case studies with progress highlights, and future promises. Proceedings of the IEEE1-19 (2020).
Liu, X. et al. A comparison of deep learning performance against health-care professionals in detecting diseases from medical imaging: a systematic review and meta-analysis. The Lancet Digital Health (2019).
Topol, E. J. High-performance medicine: the convergence of human and artificial intelligence. Nat. Med. 25 , 44–56 (2019).
Article CAS PubMed Google Scholar
Sendak, M. P. et al. A path for translation of machine learning products into healthcare delivery. Eur. Med. J. Innov. 10 , 19–00172 (2020).
Google Scholar
Schwartz, W. B., Patil, R. S. & Szolovits, P. Artificial intelligence in medicine (1987).
Roberts, M. et al. Common pitfalls and recommendations for using machine learning to detect and prognosticate for COVID-19 using chest radiographs and CT scans. Nat. Mach. Intell. 3 , 199–217 (2021).
Article Google Scholar
Willemink, M. J. et al. Preparing medical imaging data for machine learning. Radiology192224 (2020).
Mueller, S. G. et al. Ways toward an early diagnosis in Alzheimer’s disease: the Alzheimer’s Disease Neuroimaging Initiative (ADNI). Alzheimer’s Dement. 1 , 55–66 (2005).
Dallora, A. L., Eivazzadeh, S., Mendes, E., Berglund, J. & Anderberg, P. Machine learning and microsimulation techniques on the prognosis of dementia: A systematic literature review. PLoS ONE 12 , e0179804 (2017).
Article PubMed PubMed Central Google Scholar
Arbabshirani, M. R., Plis, S., Sui, J. & Calhoun, V. D. Single subject prediction of brain disorders in neuroimaging: Promises and pitfalls. NeuroImage 145 , 137–165 (2017).
Sakai, K. & Yamada, K. Machine learning studies on major brain diseases: 5-year trends of 2014–2018. Jpn. J. Radiol. 37 , 34–72 (2019).
Wen, J. et al. Convolutional neural networks for classification of Alzheimer’s disease: overview and reproducible evaluation. Medical Image Analysis 101694 (2020).
Ansart, M. et al. Predicting the progression of mild cognitive impairment using machine learning: a systematic, quantitative and critical review. Medical Image Analysis 101848 (2020).
Torralba, A. & Efros, A. A. Unbiased look at dataset bias. In Computer Vision and Pattern Recognition (CVPR) , 1521–1528 (2011).
Dockès, J., Varoquaux, G. & Poline, J.-B. Preventing dataset shift from breaking machine-learning biomarkers. GigaScience 10 , giab055 (2021).
Zendel, O., Murschitz, M., Humenberger, M. & Herzner, W. How good is my test data? introducing safety analysis for computer vision. Int. J. Computer Vis. 125 , 95–109 (2017).
Pooch, E. H., Ballester, P. L. & Barros, R. C. Can we trust deep learning models diagnosis? the impact of domain shift in chest radiograph classification. In MICCAI workshop on Thoracic Image Analysis (Springer, 2019).
Zech, J. R. et al. Variable generalization performance of a deep learning model to detect pneumonia in chest radiographs: a cross-sectional study. PLoS Med. 15 , e1002683 (2018).
Larrazabal, A. J., Nieto, N., Peterson, V., Milone, D. H. & Ferrante, E. Gender imbalance in medical imaging datasets produces biased classifiers for computer-aided diagnosis. Proceedings of the National Academy of Sciences (2020).
Tasdizen, T., Sajjadi, M., Javanmardi, M. & Ramesh, N. Improving the robustness of convolutional networks to appearance variability in biomedical images. In International Symposium on Biomedical Imaging (ISBI), 549–553 (IEEE, 2018).
Wachinger, C., Rieckmann, A., Pölsterl, S. & Initiative, A. D. N. et al. Detect and correct bias in multi-site neuroimaging datasets. Med. Image Anal. 67 , 101879 (2021).
Ashraf, A., Khan, S., Bhagwat, N., Chakravarty, M. & Taati, B. Learning to unlearn: building immunity to dataset bias in medical imaging studies. In NeurIPS workshop on Machine Learning for Health (ML4H) (2018).
Yu, X., Zheng, H., Liu, C., Huang, Y. & Ding, X. Classify epithelium-stroma in histopathological images based on deep transferable network. J. Microsc. 271 , 164–173 (2018).
Abbasi-Sureshjani, S., Raumanns, R., Michels, B. E., Schouten, G. & Cheplygina, V. Risk of training diagnostic algorithms on data with demographic bias. In Interpretable and Annotation-Efficient Learning for Medical Image Computing , 183–192 (Springer, 2020).
Suresh, H. & Guttag, J. V. A framework for understanding unintended consequences of machine learning. arXiv preprint arXiv:1901.10002 (2019).
Park, S. H. & Han, K. Methodologic guide for evaluating clinical performance and effect of artificial intelligence technology for medical diagnosis and prediction. Radiology 286 , 800–809 (2018).
Oakden-Rayner, L., Dunnmon, J., Carneiro, G. & Ré, C. Hidden stratification causes clinically meaningful failures in machine learning for medical imaging. In ACM Conference on Health, Inference, and Learning, 151–159 (2020).
Winkler, J. K. et al. Association between surgical skin markings in dermoscopic images and diagnostic performance of a deep learning convolutional neural network for melanoma recognition. JAMA Dermatol. 155 , 1135–1141 (2019).
Joskowicz, L., Cohen, D., Caplan, N. & Sosna, J. Inter-observer variability of manual contour delineation of structures in CT. Eur. Radiol. 29 , 1391–1399 (2019).
Oakden-Rayner, L. Exploring large-scale public medical image datasets. Academic Radiol. 27 , 106–112 (2020).
Langley, P. The changing science of machine learning. Mach. Learn. 82 , 275–279 (2011).
Rabanser, S., Günnemann, S. & Lipton, Z. C. Failing loudly: an empirical study of methods for detecting dataset shift. In Neural Information Processing Systems (NeurIPS) (2018).
Rädsch, T. et al. What your radiologist might be missing: using machine learning to identify mislabeled instances of X-ray images. In Hawaii International Conference on System Sciences (HICSS) (2020).
Beyer, L., Hénaff, O. J., Kolesnikov, A., Zhai, X. & Oord, A. v. d. Are we done with ImageNet? arXiv preprint arXiv:2006.07159 (2020).
Gebru, T. et al. Datasheets for datasets. In Workshop on Fairness, Accountability, and Transparency in Machine Learning (2018).
Mitchell, M. et al. Model cards for model reporting. In Fairness, Accountability, and Transparency (FAccT) , 220–229 (ACM, 2019).
Ørting, S. N. et al. A survey of crowdsourcing in medical image analysis. Hum. Comput. 7 , 1–26 (2020).
Poldrack, R. A., Huckins, G. & Varoquaux, G. Establishment of best practices for evidence for prediction: a review. JAMA Psychiatry 77 , 534–540 (2020).
Pulini, A. A., Kerr, W. T., Loo, S. K. & Lenartowicz, A. Classification accuracy of neuroimaging biomarkers in attention-deficit/hyperactivity disorder: Effects of sample size and circular analysis. Biol. Psychiatry.: Cogn. Neurosci. Neuroimaging 4 , 108–120 (2019).
Saeb, S., Lonini, L., Jayaraman, A., Mohr, D. C. & Kording, K. P. The need to approximate the use-case in clinical machine learning. Gigascience 6 , gix019 (2017).
Hosseini, M. et al. I tried a bunch of things: The dangers of unexpected overfitting in classification of brain data. Neuroscience & Biobehavioral Reviews (2020).
Simpson, A. L. et al. A large annotated medical image dataset for the development and evaluation of segmentation algorithms. arXiv preprint arXiv:1902.09063 (2019).
Rohlfing, T. Image similarity and tissue overlaps as surrogates for image registration accuracy: widely used but unreliable. IEEE Trans. Med. Imaging 31 , 153–163 (2011).
Maier-Hein, L. et al. Why rankings of biomedical image analysis competitions should be interpreted with care. Nat. Commun. 9 , 5217 (2018).
Article CAS PubMed PubMed Central Google Scholar
Van Calster, B., McLernon, D. J., Van Smeden, M., Wynants, L. & Steyerberg, E. W. Calibration: the Achilles heel of predictive analytics. BMC Med. 17 , 1–7 (2019).
Wagstaff, K. L. Machine learning that matters. In International Conference on Machine Learning (ICML), 529–536 (2012).
Shankar, V. et al. Evaluating machine accuracy on imagenet. In International Conference on Machine Learning (ICML) (2020).
Bellamy, D., Celi, L. & Beam, A. L. Evaluating progress on machine learning for longitudinal electronic healthcare data. arXiv preprint arXiv:2010.01149 (2020).
Oliver, A., Odena, A., Raffel, C., Cubuk, E. D. & Goodfellow, I. J. Realistic evaluation of semi-supervised learning algorithms. In Neural Information Processing Systems (NeurIPS) (2018).
Dacrema, M. F., Cremonesi, P. & Jannach, D. Are we really making much progress? a worrying analysis of recent neural recommendation approaches. In ACM Conference on Recommender Systems, 101–109 (2019).
Musgrave, K., Belongie, S. & Lim, S.-N. A metric learning reality check. In European Conference on Computer Vision, 681–699 (Springer, 2020).
Pham, H. V. et al. Problems and opportunities in training deep learning software systems: an analysis of variance. In IEEE/ACM International Conference on Automated Software Engineering, 771–783 (2020).
Bouthillier, X. et al. Accounting for variance in machine learning benchmarks. In Machine Learning and Systems (2021).
Varoquaux, G. Cross-validation failure: small sample sizes lead to large error bars. NeuroImage 180 , 68–77 (2018).
Szucs, D. & Ioannidis, J. P. Sample size evolution in neuroimaging research: an evaluation of highly-cited studies (1990–2012) and of latest practices (2017–2018) in high-impact journals. NeuroImage117164 (2020).
Roelofs, R. et al. A meta-analysis of overfitting in machine learning. In Neural Information Processing Systems (NeurIPS), 9179–9189 (2019).
Demšar, J. Statistical comparisons of classifiers over multiple data sets. J. Mach. Learn. Res. 7 , 1–30 (2006).
Thompson, W. H., Wright, J., Bissett, P. G. & Poldrack, R. A. Meta-research: dataset decay and the problem of sequential analyses on open datasets. eLife 9 , e53498 (2020).
Maier-Hein, L. et al. Is the winner really the best? a critical analysis of common research practice in biomedical image analysis competitions. Nature Communications (2018).
Cockburn, A., Dragicevic, P., Besançon, L. & Gutwin, C. Threats of a replication crisis in empirical computer science. Commun. ACM 63 , 70–79 (2020).
Gigerenzer, G. Statistical rituals: the replication delusion and how we got there. Adv. Methods Pract. Psychol. Sci. 1 , 198–218 (2018).
Benavoli, A., Corani, G. & Mangili, F. Should we really use post-hoc tests based on mean-ranks? J. Mach. Learn. Res. 17 , 152–161 (2016).
Berrar, D. Confidence curves: an alternative to null hypothesis significance testing for the comparison of classifiers. Mach. Learn. 106 , 911–949 (2017).
Bouthillier, X., Laurent, C. & Vincent, P. Unreproducible research is reproducible. In International Conference on Machine Learning (ICML), 725–734 (2019).
Norgeot, B. et al. Minimum information about clinical artificial intelligence modeling: the MI-CLAIM checklist. Nat. Med. 26 , 1320–1324 (2020).
Drummond, C. Machine learning as an experimental science (revisited). In AAAI workshop on evaluation methods for machine learning, 1–5 (2006).
Steyerberg, E. W. & Harrell, F. E. Prediction models need appropriate internal, internal–external, and external validation. J. Clin. Epidemiol. 69 , 245–247 (2016).
Woo, C.-W., Chang, L. J., Lindquist, M. A. & Wager, T. D. Building better biomarkers: brain models in translational neuroimaging. Nat. Neurosci. 20 , 365 (2017).
Van Calster, B. et al. Reporting and interpreting decision curve analysis: a guide for investigators. Eur. Urol. 74 , 796 (2018).
Thomas, R. & Uminsky, D. The problem with metrics is a fundamental problem for AI. arXiv preprint arXiv:2002.08512 (2020).
for the Evaluation of Medicinal Products, E. A. Points to consider on switching between superiority and non-inferiority. Br. J. Clin. Pharmacol. 52 , 223–228 (2001).
D’Agostino Sr, R. B., Massaro, J. M. & Sullivan, L. M. Non-inferiority trials: design concepts and issues–the encounters of academic consultants in statistics. Stat. Med. 22 , 169–186 (2003).
Christensen, E. Methodology of superiority vs. equivalence trials and non-inferiority trials. J. Hepatol. 46 , 947–954 (2007).
Hendriksen, J. M., Geersing, G.-J., Moons, K. G. & de Groot, J. A. Diagnostic and prognostic prediction models. J. Thrombosis Haemost. 11 , 129–141 (2013).
Campbell, M. K., Elbourne, D. R. & Altman, D. G. Consort statement: extension to cluster randomised trials. BMJ 328 , 702–708 (2004).
Blasini, M., Peiris, N., Wright, T. & Colloca, L. The role of patient–practitioner relationships in placebo and nocebo phenomena. Int. Rev. Neurobiol. 139 , 211–231 (2018).
Lipton, Z. C. & Steinhardt, J. Troubling trends in machine learning scholarship: some ML papers suffer from flaws that could mislead the public and stymie future research. Queue 17 , 45–77 (2019).
Tatman, R., VanderPlas, J. & Dane, S. A practical taxonomy of reproducibility for machine learning research. In ICML workshop on Reproducibility in Machine Learning (2018).
Gundersen, O. E. & Kjensmo, S. State of the art: Reproducibility in artificial intelligence. In AAAI Conference on Artificial Intelligence (2018).
Fernández-Delgado, M., Cernadas, E., Barro, S., Amorim, D. & Amorim Fernández-Delgado, D. Do we need hundreds of classifiers to solve real world classification problems? J. Mach. Learn. Res. 15 , 3133–3181 (2014).
Sculley, D. et al. Hidden technical debt in machine learning systems. In Neural Information Processing Systems (NeurIPS), 2503–2511 (2015).
Ioannidis, J. P. A. Why most published research findings are false. PLoS Med. 2 , e124 (2005).
Teney, D. et al. On the value of out-of-distribution testing: an example of Goodhart’s Law. In Neural Information Processing Systems (NeurIPS) (2020).
Kerr, N. L. HARKing: hypothesizing after the results are known. Personal. Soc. Psychol. Rev. 2 , 196–217 (1998).
Article CAS Google Scholar
Gencoglu, O. et al. HARK side of deep learning–from grad student descent to automated machine learning. arXiv preprint arXiv:1904.07633 (2019).
Rosenthal, R. The file drawer problem and tolerance for null results. Psychological Bull. 86 , 638 (1979).
Kellmeyer, P. Ethical and legal implications of the methodological crisis in neuroimaging. Camb. Q. Healthc. Ethics 26 , 530–554 (2017).
Japkowicz, N. & Shah, M. Performance evaluation in machine learning. In Machine Learning in Radiation Oncology , 41–56 (Springer, 2015).
Santafe, G., Inza, I. & Lozano, J. A. Dealing with the evaluation of supervised classification algorithms. Artif. Intell. Rev. 44 , 467–508 (2015).
Han, K., Song, K. & Choi, B. W. How to develop, validate, and compare clinical prediction models involving radiological parameters: study design and statistical methods. Korean J. Radiol. 17 , 339–350 (2016).
Richter, A. N. & Khoshgoftaar, T. M. Sample size determination for biomedical big data with limited labels. Netw. Modeling Anal. Health Inform. Bioinforma. 9 , 12 (2020).
Collins, G. S., Reitsma, J. B., Altman, D. G. & Moons, K. G. Transparent reporting of a multivariable prediction model for individual prognosis or diagnosis (tripod): the tripod statement. J. Br. Surg. 102 , 148–158 (2015).
Wolff, R. F. et al. Probast: a tool to assess the risk of bias and applicability of prediction model studies. Ann. Intern. Med. 170 , 51–58 (2019).
Henderson, P. et al. Towards the systematic reporting of the energy and carbon footprints of machine learning. J. Mach. Learn. Res. 21 , 1–43 (2020).
Bowen, A. & Casadevall, A. Increasing disparities between resource inputs and outcomes, as measured by certain health deliverables, in biomedical research. Proc. Natl Acad. Sci. 112 , 11335–11340 (2015).
Chambers, C. D., Dienes, Z., McIntosh, R. D., Rotshtein, P. & Willmes, K. Registered reports: realigning incentives in scientific publishing. Cortex 66 , A1–A2 (2015).
Forde, J. Z. & Paganini, M. The scientific method in the science of machine learning. In ICLR workshop on Debugging Machine Learning Models (2019).
Firestein, S.Failure: Why science is so successful (Oxford University Press, 2015).
Borji, A. Negative results in computer vision: a perspective. Image Vis. Comput. 69 , 1–8 (2018).
Voets, M., Møllersen, K. & Bongo, L. A. Replication study: Development and validation of deep learning algorithm for detection of diabetic retinopathy in retinal fundus photographs. arXiv preprint arXiv:1803.04337 (2018).
Wilkinson, J. et al. Time to reality check the promises of machine learning-powered precision medicine. The Lancet Digital Health (2020).
Whitaker, K. & Guest, O. #bropenscience is broken science. Psychologist 33 , 34–37 (2020).
Kakarmath, S. et al. Best practices for authors of healthcare-related artificial intelligence manuscripts. NPJ Digital Med. 3 , 134–134 (2020).
Download references
Acknowledgements
We would like to thank Alexandra Elbakyan for help with the literature review. We thank Pierre Dragicevic for providing feedback on early versions of this manuscript, and Pierre Bartet for comments on the preprint. We also thank the reviewers, Jack Wilkinson and Odd Erik Gundersen, for excellent comments which improved our manuscript. GV acknowledges funding from grant ANR-17-CE23-0018, DirtyData.
Author information
Authors and affiliations.
INRIA, Versailles, France
Gaël Varoquaux
McGill University, Montreal, Canada
Mila, Montreal, Canada
IT University of Copenhagen, Copenhagen, Denmark
Veronika Cheplygina
You can also search for this author in PubMed Google Scholar
Contributions
Both V.C. and G.V. collected the data; conceived, designed, and performed the analysis; reviewed the literature; and wrote the paper.
Corresponding authors
Correspondence to Gaël Varoquaux or Veronika Cheplygina .
Ethics declarations
Competing interests.
The authors declare that there are no competing interests.
Additional information
Publisher’s note Springer Nature remains neutral with regard to jurisdictional claims in published maps and institutional affiliations.
Supplementary information
Latex source files, rights and permissions.
Open Access This article is licensed under a Creative Commons Attribution 4.0 International License, which permits use, sharing, adaptation, distribution and reproduction in any medium or format, as long as you give appropriate credit to the original author(s) and the source, provide a link to the Creative Commons license, and indicate if changes were made. The images or other third party material in this article are included in the article’s Creative Commons license, unless indicated otherwise in a credit line to the material. If material is not included in the article’s Creative Commons license and your intended use is not permitted by statutory regulation or exceeds the permitted use, you will need to obtain permission directly from the copyright holder. To view a copy of this license, visit http://creativecommons.org/licenses/by/4.0/ .
Reprints and permissions
About this article
Cite this article.
Varoquaux, G., Cheplygina, V. Machine learning for medical imaging: methodological failures and recommendations for the future. npj Digit. Med. 5 , 48 (2022). https://doi.org/10.1038/s41746-022-00592-y
Download citation
Received : 21 June 2021
Accepted : 09 March 2022
Published : 12 April 2022
DOI : https://doi.org/10.1038/s41746-022-00592-y
Share this article
Anyone you share the following link with will be able to read this content:
Sorry, a shareable link is not currently available for this article.
Provided by the Springer Nature SharedIt content-sharing initiative
This article is cited by
Enhancing prediction accuracy of coronary artery disease through machine learning-driven genomic variant selection.
Journal of Translational Medicine (2024)
A survey of the impact of self-supervised pretraining for diagnostic tasks in medical X-ray, CT, MRI, and ultrasound
- Blake VanBerlo
- Alexander Wong
BMC Medical Imaging (2024)
Deep representation learning of tissue metabolome and computed tomography annotates NSCLC classification and prognosis
- Marc Boubnovski Martell
- Kristofer Linton-Reid
- Eric O. Aboagye
npj Precision Oncology (2024)
Electronic health records and stratified psychiatry: bridge to precision treatment?
- Adrienne Grzenda
- Alik S. Widge
Neuropsychopharmacology (2024)
Diagnostic performance of artificial intelligence-assisted PET imaging for Parkinson’s disease: a systematic review and meta-analysis
npj Digital Medicine (2024)
Quick links
- Explore articles by subject
- Guide to authors
- Editorial policies
Sign up for the Nature Briefing: AI and Robotics newsletter — what matters in AI and robotics research, free to your inbox weekly.


- button-radnet EXPLORE RADNET.COM
- button-locator Find an Imaging Center
- SCHEDULE APPOINTMENT

- News & Press Releases
- Investor Relations
- search-solid
- facebook-solid
- twitter-solid
- linkedin-solid
- youtube-solid
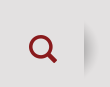
- Outpatient Imaging Centers
- Technology Solutions
- Centers of Excellence
- Hospital Partnerships
- Payor Solutions
- Quality Assurance
- Insurance Solutions
- RadNet Direct
- MammogramNow
- Find an Imaging Center
- Our Services
- Why Choose RadNet?
- Musculoskeletal MRI Fellowship
- Contact RadNet
- Media Appearances
- Board of Directors
Partnering For The Future
In partnership with international pharmaceutical and biotechnical companies, we are participating in research to improve the diagnosis and treatment of challenging diseases.
The Future of Care—In the Community
Under the leadership of Dr. Judith Rose, the research team participates in clinical research trials that capitalize on our PET/CT, and Nuclear Medicine expertise. Our experience in these advanced modalities—combined with our rigorous methodology and our relationship to the communities we serve—makes us a sought-after partner for drug and device manufacturers as well as for contract research organizations (CROs). In collaboration with RadNet, an outpatient imaging provider with a national profile, we are able to conduct research consistently across a broad patient population, while controlling quality and efficiency.
As sophisticated radiopharmaceuticals are developed to identify disease markers (such as beta-amyloid, a biological marker of Alzheimer’s Disease), testing depends on imaging to track metabolic activity in the areas targeted by those drugs. A natural follow-on is the development of drugs that can treat pathology in those same areas, once it’s discovered, and advanced imaging techniques are again required. They enable researchers to evaluate how or if the new drugs are working. We are making important contributions in that field across RadNet’s large network.
Quality & Experience
The research team has participated in multiple clinical trials that use advanced imaging, from molecular diagnostics to dosimetry, and with partners like GE, Eli Lilly, Eisai, Merck, and many others. Our protocol includes standardization across multiple states, and reading workflows that ensure quality and continuous education. In one study, for example, more than a dozen RadNet radiologists went through special training for reading exams that made use of a new tracer—training recommended by the drug manufacturer. Dr. Rose instituted a double-read protocol, and all certified radiologists could view the exams on a special centralized server. Quality measures are built in to all our research activities.
Partnering with Community Physicians
We team with physicians in the community to provide quality imaging for their clinical trials. We have experience in following protocols, contributing data, understanding research budgets, and performing high quality reads in advanced modalities. Physicians know that they are teaming with a well-developed research group that can play a valuable role in the imaging component of their clinical trials, and that we offer a familiar and comfortable local setting for their patients.
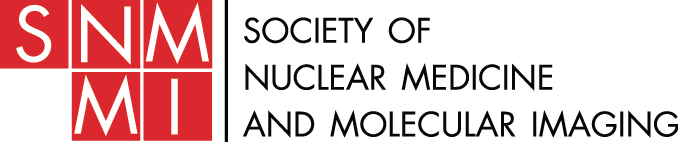
Society for Nuclear Medicine Clinical Trials Network
Standardization in molecular imaging is critical for a clinical trial to be successful. To achieve CTN validation, a site must demonstrate that it complies with common quality control metrics and that it is able to acquire high-quality PET images and interpret them correctly. RadNet has achieved SNM validation at nine of our imaging centers across the U.S.
Are you a clinical research site interested in using RadNet-owned and -operated imaging centers for clinical trials? Contact our study start-up team.
CONNECT WITH US
West Coast Operations 1510 Cotner Avenue Los Angeles, CA 90025 Phone: 310-445-2800 Fax: 310-445-2980
East Coast Operations BECO Towers 10461 Mill Run Circle, Suite 1100 Owings Mills, MD 21117 Phone: 443-436-1100 Fax: 443-436-1500
Shiba P. Kuanar
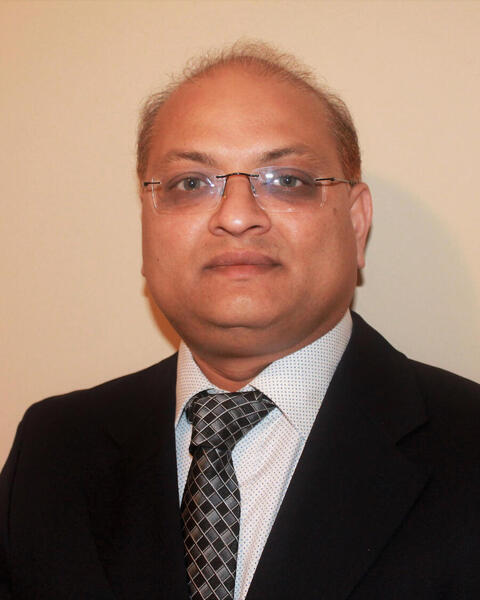
Shiba P. Kuanar, Ph.D., explores advancements in the field of medical imaging analysis using artificial intelligence (AI) and machine learning (ML) methodologies in cancer research. He enhances disease understanding by integrating data from various medical specialties into AI models. Dr. Kuanar's primary focus revolves around the development and validation of deep learning and probability algorithms tailored to detect and assess disease severity with an emphasis on people's care.
In a recent shift, Dr. Kuanar has broadened his research scope to incorporate electronic health record text analysis, employing generative large language models (LLMs). By integrating these models with imaging pipelines and statistical learning theories, he aims to extract crucial feature information for healthcare data analysis.
Focus areas
- Abdominal image analysis. Dr. Kuanar applies deep learning pipelines to detect clinically significant prostate cancer and predicts outcomes through multiparametric Magnetic Resonance Imaging (MRI). Dr. Kuanar believes that these models could enhance the interpretation of MRI images and provide valuable supplementary insights to aid radiologists in their diagnostic process. Additionally, Dr. Kuanar's research explores biomarkers such as transition-zone PSA-density as potential alternatives to the conventional prostate-specific antigen test technique for screening prostate cancer.
- Unsupervised and active learning. Dr. Kuanar's recent work focuses on fine-tuning LLMs to accurately predict different class labels in clinical text within electronic health records, specifically tailored to address people's safety challenges. In an active learning context, Dr. Kuanar endeavors to leverage generative models to summarize the text and enhance the data labeling process.
- Multi-Modal Learning. Dr. Kuanar utilizes multimodal methods to integrate data from diverse sources, employing both traditional and deep learning techniques. Employing feature-level, decision-level and model-level fusion techniques, Dr. Kuanar endeavors to uncover essential insights within images, mitigating biases and improving model interpretability.
- Federated learning. Decentralized federated learning addresses people's privacy, computational and storage challenges in managing large medical archives across multiple hospitals. This collaborative effort seeks to assess the feasibility of using AI-assisted annotation to streamline federated learning for automated image segmentation tasks. The goal is to enhance overall model performance and mitigate bias stemming from interobserver variability in image annotation.
Significance to patient care
Medical images and clinical texts have important information for healthcare applications. Despite increasing digitization, the complexity and variability of clinical text and image modalities pose challenges for traditional ML. Dr. Kuanar's research explores AI/ML techniques to understand medical data better, tackle safety challenges, expedite decision-making and improve people's care.
Professional highlights
- Member, Society for Imaging Informatics in Medicine, Machine Learning Tools and Research Sub-Committee, 2022-present.
- Member, American Association for Cancer Research, 2021-present.
- Member, Coalition for Health Artificial Intelligence Team, Mayo Clinic, 2024.
- Member, Program Committee Deep Generative Models Workshop, Medical Image Computing and Computer Assisted Intervention Society, 2022.
PROFESSIONAL DETAILS
Academic rank.
- Assistant Professor of Radiology
- Doctorate - in Electrical Engineering The University of Texas at Arlington
- Master of Science - in Electrical Engineering The University of Texas at Arlington
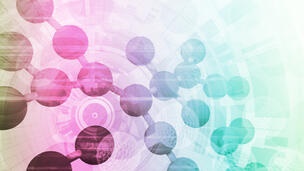
Clinical Studies
Learn about clinical trials that address specific scientific questions about human health and disease.
Explore all research studies at Mayo Clinic.
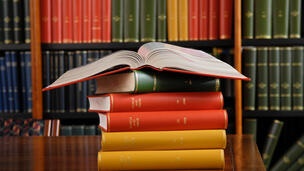
- Publications
See the peer-reviewed findings I have published as a result of my research.
More about research at Mayo Clinic
- Research Faculty
- Laboratories
- Core Facilities
- Centers & Programs
- Departments & Divisions
- Clinical Trials
- Institutional Review Board
- Postdoctoral Fellowships
- Training Grant Programs
Mayo Clinic Footer
- Request Appointment
- About Mayo Clinic
- About This Site
Legal Conditions and Terms
- Terms and Conditions
- Privacy Policy
- Notice of Privacy Practices
- Notice of Nondiscrimination
- Manage Cookies
Advertising
Mayo Clinic is a nonprofit organization and proceeds from Web advertising help support our mission. Mayo Clinic does not endorse any of the third party products and services advertised.
- Advertising and sponsorship policy
- Advertising and sponsorship opportunities
Reprint Permissions
A single copy of these materials may be reprinted for noncommercial personal use only. "Mayo," "Mayo Clinic," "MayoClinic.org," "Mayo Clinic Healthy Living," and the triple-shield Mayo Clinic logo are trademarks of Mayo Foundation for Medical Education and Research.
This page uses frames, but your browser doesn't support them.
However, you can visit the no-framed part .
- Now Trending:
- Lighting Up Surgery and ...
- Pancreatic Cancer: Light...
- Pancreatic Cancer: Guidi...
- The Color of Colon Cance...
Photoimmune Discoveries
- About the Authors
Andrei Reshetnickov, PhD , is a bioorganic chemist who has synthesized numerous photosensitizing agents and developed a wide range of light-based medical technologies. His pioneering insights into the use of light for therapeutic purposes have guided the emergence of more effective treatment protocols and helped inform a new direction for cancer medicine.
Dr. Reshetnickov obtained his doctoral degree from M. V. Lomonosov Institute of Fine Chemical Technology in Moscow, Russia in 1999. His primary area of specialization was biotechnology before eventually bringing all of his research expertise to bear on the chemistry of physiologically active and natural compounds.
In the past decade, Dr. Reshetnickov has synthesized and studied the structure and properties of well over a hundred new photosensitizers. Some of these have become medicines while others are currently undergoing testing as candidates for future medicines. Clinical trials are ongoing to demonstrate the efficacy of these agents as adjuncts to innovative cancer treatment.
Dr. Reshetnickov has published a number of scientific reports about these agents in peer-reviewed journals and received 31 patents related to PDT and innovative ways to use this and other light treatment modalities to enhance health and healing. His participation in clinical trials and ongoing communications with physicians and cancer researchers have helped him stay on the cutting edge of developments in the burgeoning field of photomedicine.
Dr. Reshetnickov is the discoverer of several leading-edge photosensitizers, optical imaging probes, and tumor-selective MRI contrast agents. Among these discoveries are Bremachlorin®, Radachlorin®, Fotonaflor®, Magnetaflor®, Thermochlorin®, Oscirad®, Sonorad® and FloraDynamica®. In addition, he serves as a reviewer for Photodiagnosis and Photodynamic Therapy, one of the leading international journals for sharing scientific knowledge and clinical developments of light-based medical applications.
Throughout the 1990s, Mead served as a contributing editor to Natural Health, a popular health magazine based in the United States, and wrote for various publications including American Health, Alternative Medicine Digest, The Progressive, Utne Reader, Whole Earth Review, and many other publications. He has coauthored a number of health-related books, including The Rapid Recovery Handbook (HarperCollins 2006), with a foreword by Mehmet Oz, MD. After writing The Alternative Medicine Definitive Guide to Cancer for W. John Diamond, MD (Future Medicine Publishing, 1997), he contributed to Breast Cancer Beyond Convention (Pocket Books, 2002) and Life Over Cancer by Keith Block, MD (Bantam, 2009).
He also worked as an integrative medicine research consultant for four integrative medicine clinics in the United States. Mead has a passion for guiding cancer patients and educating health care professionals about the therapeutic potential of innovative, holistic cancer therapies. He continues to serve as an editor for Integrative Cancer Therapies and resides with his family in Durham, North Carolina.
- Which Cancers?
- Which Infections?
- Photosensitizers
- Photodynamic Therapy
- Photoimmune Therapies
- Fluorescence-Guided Surgery
- Photodiagnosis
- Other Innovative Therapies
- Chemotherapy
- Radiotherapy
- Molecular-Targeting Therapies
- Europe & Russia
- USA & Canada
- New Zealand
- The Medicine of Light
- Expert Comments
- Photoimmune Discoveries eBook Series
- About Photoimmune Discoveries
- Our Projects
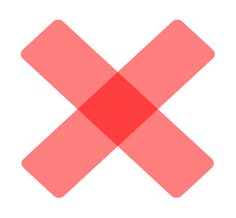
Something went wrong
An error has prevented the portal from working properly.
Please contact us .
You reached this page when trying to access MDI3MDQ5ZTAzYjcxLTcyNDgtZjNlNC03NDRiLWRhYzdkOTUy from 193.7.198.129 on April 27 2024, 19:35:10 UTC

Automated machine learning robot unlocks new potential for genetics research
This technology will save labs time and money while enabling large-scale experiments.
University of Minnesota Twin Cities researchers have constructed a robot that uses machine learning to fully automate a complicated microinjection process used in genetic research.
In their experiments, the researchers were able to use this automated robot to manipulate the genetics of multicellular organisms, including fruit fly and zebrafish embryos. The technology will save labs time and money while enabling them to more easily conduct new, large-scale genetic experiments that were not possible previously using manual techniques
The research is featured on the cover of the April 2024 issue of GENETICS , a peer-reviewed, open access, scientific journal. The work was co-led by two University of Minnesota mechanical engineering graduate students Andrew Alegria and Amey Joshi. The team is also working to commercialize this technology to make it widely available through the University of Minnesota start-up company, Objective Biotechnology.
Microinjection is a method for introducing cells, genetic material, or other agents directly into embryos, cells, or tissues using a very fine pipette. The researchers have trained the robot to detect embryos that are one-hundredth the size of a grain of rice. After detection, the machine can calculate a path and automate the process of the injections.
"This new process is more robust and reproducible than manual injections," said Suhasa Kodandaramaiah, a University of Minnesota mechanical engineering associate professor and senior author of the study. "With this model, individual laboratories will be able to think of new experiments that you couldn't do without this type of technology."
Typically, this type of research requires highly skilled technicians to perform the microinjection, which many laboratories do not have. This new technology could expand the ability to perform large experiments in labs, while reducing time and costs.
"This is very exciting for the world of genetics. Writing and reading DNA have drastically improved in recent years, but having this technology will increase our ability to perform large-scale genetic experiments in a wide range of organisms," said Daryl Gohl, a co-author of the study, the group leader of the University of Minnesota Genomics Center's Innovation Lab and research assistant professor in the Department of Genetics, Cell Biology, and Development.
Not only can this technology be used in genetic experiments, but it can also help to preserve endangered species through cryopreservation, a preservation technique conducted at ultra-low temperatures.
"You can use this robot to inject nanoparticles into cells and tissues that helps in cryopreservation and in the process of rewarming afterwards," Kodandaramaiah explained.
Other team members highlighted other applications for the technology that could have even more impact.
"We hope that this technology could eventually be used for in vitro fertilization, where you could detect those eggs on the microscale level," said Andrew Alegria, co-lead author on the paper and University of Minnesota mechanical engineering graduate research assistant in the Biosensing and Biorobotics Lab.
- Medical Devices
- Personalized Medicine
- Medical Imaging
- Educational Technology
- Artificial Intelligence
- Robot calibration
- Computational neuroscience
- Industrial robot
- Molecular biology
- Weight machine
- Computer vision
Story Source:
Materials provided by University of Minnesota . Note: Content may be edited for style and length.
Journal Reference :
- Andrew D Alegria, Amey S Joshi, Jorge Blanco Mendana, Kanav Khosla, Kieran T Smith, Benjamin Auch, Margaret Donovan, John Bischof, Daryl M Gohl, Suhasa B Kodandaramaiah. High-throughput genetic manipulation of multicellular organisms using a machine-vision guided embryonic microinjection robot . GENETICS , 2024; 226 (4) DOI: 10.1093/genetics/iyae025
Cite This Page :
Explore More
- Collisions of Neutron Stars and Black Holes
- Advance in Heart Regenerative Therapy
- Bioluminescence in Animals 540 Million Years Ago
- Profound Link Between Diet and Brain Health
- Loneliness Runs Deep Among Parents
- Food in Sight? The Liver Is Ready!
- Acid Reflux Drugs and Risk of Migraine
- Do Cells Have a Hidden Communication System?
- Mice Given Mouse-Rat Brains Can Smell Again
- How Do Birds Flock? New Aerodynamics
Trending Topics
Strange & offbeat.
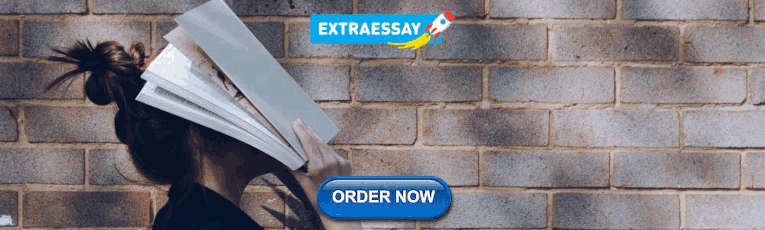
IMAGES
VIDEO
COMMENTS
Medical imaging comprises different imaging modalities and processes to image human body for diagnostic and treatment purposes. It is also used to follow the course of a disease already diagnosed ...
Today medical imaging is an essential component of the entire health-care continuum, from wellness and screening, to early diagnosis, treatment selection, and follow-up. ... Robust research initiatives with fMRI and diffusion tensor imaging to study brain and spine nerve fiber tracts are underway. 16, 17 Magnetic resonance spectroscopy is used ...
AI for medical imaging goes deep. An artificial intelligence (AI) using a deep-learning approach can classify retinal images from optical coherence tomography for early diagnosis of retinal ...
Medical imaging techniques such as computed tomography (CT), magnetic resonance imaging (MRI), and positron emission tomography (PET) play a pivotal role in providing clinicians with detailed and comprehensive visual information about the human body. ... Consequently, future research endeavors can explore its applicability to diverse diseases ...
Medical imaging informatics has been driving clinical research, translation, and practice for over three decades. Advances in associate research branches highlighted in this study promise to revolutionize imaging informatics as known today across the healthcare continuum enabling informed, more accurate diagnosis, timely prognosis, and ...
BMC Medical Imaging is an open access journal publishing original peer-reviewed research articles in the development, evaluation, and use of imaging techniques and image processing tools to diagnose and manage disease. Become an Editorial Board Member. Dmitriy Gutarev from Pixabay.
This study focused on analyzing the clinical value and effect of magnetic resonance imaging plus computed tomography (MRCT) and CT in the clinical diagnosis of cerebral palsy in children. Xiaofei Wang and Wen Hu. BMC Medical Imaging 2024 24 :96. Research Published on: 25 April 2024. Full Text.
We included all research articles in all languages that applied deep learning models for clinical outcome prediction or diagnosis using a combination of medical imaging modalities and EHR data.
The Medical Imaging and Data Resource Center (MIDRC) is a collaboration of leading medical imaging organizations launched in August 2020 as part of NIBIB's response to the COVID-19 pandemic. ... MIDRC was recognized for its collaboration to create a curated, diverse commons for medical imaging AI research and translation. All of MIDRC's ...
Overview. Mayo Clinic's Center for Advanced Imaging Research (CAIR) aims to advance the science of imaging techniques, such as MRI and CT, to deliver more accurate diagnostics and a better quality of care. The center brings together clinicians and scientists from across Mayo Clinic in unique collaborations to enhance innovations in medical ...
Through interdisciplinary collaboration, Stanford Radiology's 3DQ Imaging Lab develops and applies innovative techniques for the efficient quantitative analysis and display of medical imaging data used in training, research, and the delivery of patient care.
The Gordon Center for Medical Imaging at Massachusetts General Hospital (MGH) and Harvard Medical School is a multidisciplinary research center dedicated to improving patient care by developing and promoting new biomedical imaging technologies used in both diagnosis and therapy. Our main activities include research, training, and translation of ...
Medical Imaging Our research in this area covers a wide range of topics, from development of imaging devices, image reconstruction, contrast media and kinetic modeling, image processing and analysis, and patient modeling. In addition to traditional clinical imaging modalities, such as CT, MRI, PET and ultrasound, we are also interested in ...
Fostering Research Advances And Innovations. The Johns Hopkins Department of Radiology is internationally recognized for its leadership in innovation and leading-edge research in all aspects of molecular, functional, and anatomic imaging. This is reflected in the funding it attracts, the number of Gold Medals awarded to faculty, the impact of ...
Stanford has established the AIMI Center to develop, evaluate, and disseminate artificial intelligence systems to benefit patients. We conduct research that solves clinically important problems using machine learning and other AI techniques. Director's Welcome. Back in 2017, I tweeted "radiologists who use AI will replace radiologists who don ...
University of Waterloo researchers have invented a digital medical imaging system that significantly improves the cancer detection process to deliver immediate results and enable swift, effective ...
Cardiovascular magnetic resonance (CMR) imaging has become an invaluable clinical and research tool. Starting from the discovery of nuclear magnetic resonance, this article provides a brief overview of the key developments that have led to CMR as it is today, and how it became the modality of choice for large-scale population studies.
Abstract. Without doubt, artificial intelligence (AI) is the most discussed topic today in medical imaging research, both in diagnostic and therapeutic. For diagnostic imaging alone, the number of publications on AI has increased from about 100-150 per year in 2007-2008 to 1000-1100 per year in 2017-2018.
In this interview, News Medical speaks to Isaac Chiu, Ph.D and Daping Yang, Ph.D. of Harvard Medical School, about their latest research, revealing the surprising properties of pain.
But overall medical imaging research seldom analyzes how likely empirical results are to be due to chance: only 6% of segmentation challenges surveyed 61, and 15% out of 410 popular computer ...
The research team has participated in multiple clinical trials that use advanced imaging, from molecular diagnostics to dosimetry, and with partners like GE, Eli Lilly, Eisai, Merck, and many others. Our protocol includes standardization across multiple states, and reading workflows that ensure quality and continuous education.
Shiba P. Kuanar, Ph.D., explores advancements in the field of medical imaging analysis using artificial intelligence (AI) and machine learning (ML) methodologies in cancer research. He enhances disease understanding by integrating data from various medical specialties into AI models. Dr.
Fig. Development of High Frame Rate Ultrasound Imaging System - On the left, it shows an eight-layer printed circuit board (PCB) used primarily for multi-channel ultrasound signal reception and storage.The board was designed in the Ultrasound Lab at the University of Toledo, led by Dr. Jian-yu Lu. It is one of the many PCBs designed for a high frame rate medical ultrasound imaging system.
Andrei Reshetnickov, PhD, is a bioorganic chemist who has synthesized numerous photosensitizing agents and developed a wide range of light-based medical technologies. His pioneering insights into the use of light for therapeutic purposes have guided the emergence of more effective treatment protocols and helped inform a new direction for cancer medicine. Dr. Reshetnickov obtained his doctoral
United Kingdom: +44 753-710-5080. United States: +1 302-232-5106. Email: [email protected]. Website: www.thenicheresearch.com. Global Medical Image Management Market has been ...
6Center of Bioimaging, Institute for Regenerative Medicine, Sechenov First Moscow State Medical University, Moscow, Russia E-mail: [email protected] Received 6 July 2016, revised 8 November 2016 Accepted for publication 9 November 2016 Published 8 December 2016 Abstract The mechanism of upconversion at the nanoscale is still under ...
MOSCOW--(BUSINESS WIRE)--GE Healthcare, a unit of General Electric Company (NYSE:GE), and a leading Russian medical imaging manufacturer Medical Technologies Limited (MTL), today announced a strategic partnership to manufacture diagnostic imaging products in Russia, starting with GE Healthcare's CT BrightSpeed™ Elite system1, in line with GE's healthymagination strategy.
physical research institutes. For example in Germany, the Pilot project for proton therapy was realized in GSI on a basis of research synchrotron prior to construct the Heidelberg ion beam medical centre. In Russia, three proton therapy centres are now in action in nuclear research institutes (see Table 1) and the fourth is now
University of Minnesota. "Automated machine learning robot unlocks new potential for genetics research." ScienceDaily. ScienceDaily, 26 April 2024. <www.sciencedaily.com / releases / 2024 / 04 ...