Regression Analysis
- Reference work entry
- First Online: 03 December 2021
- Cite this reference work entry
- Bernd Skiera 4 ,
- Jochen Reiner 4 &
- Sönke Albers 5
7345 Accesses
3 Citations
Linear regression analysis is one of the most important statistical methods. It examines the linear relationship between a metric-scaled dependent variable (also called endogenous, explained, response, or predicted variable) and one or more metric-scaled independent variables (also called exogenous, explanatory, control, or predictor variable). We illustrate how regression analysis work and how it supports marketing decisions, e.g., the derivation of an optimal marketing mix. We also outline how to use linear regression analysis to estimate nonlinear functions such as a multiplicative sales response function. Furthermore, we show how to use the results of a regression to calculate elasticities and to identify outliers and discuss in details the problems that occur in case of autocorrelation, multicollinearity and heteroscedasticity. We use a numerical example to illustrate in detail all calculations and use this numerical example to outline the problems that occur in case of endogeneity.
This is a preview of subscription content, log in via an institution to check access.
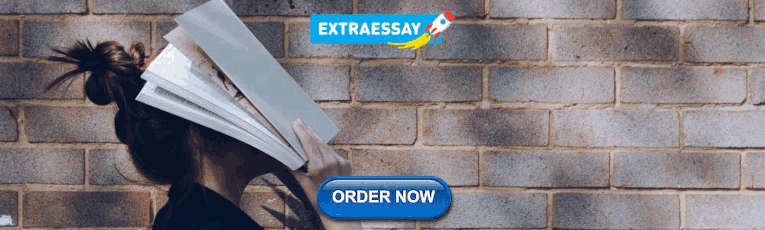
Access this chapter
- Available as PDF
- Read on any device
- Instant download
- Own it forever
- Available as EPUB and PDF
- Durable hardcover edition
- Dispatched in 3 to 5 business days
- Free shipping worldwide - see info
Tax calculation will be finalised at checkout
Purchases are for personal use only
Institutional subscriptions
Albers, S. (2012). Optimizable and implementable aggregate response modeling for marketing decision support. International Journal of Research in Marketing, 29 (2), 111–122.
Article Google Scholar
Albers, S., Mantrala, M. K., & Sridhar, S. (2010). Personal selling elasticities: A meta-analysis. Journal of Marketing Research, 47 (5), 840–853.
Assmus, G., Farley, J. W., & Lehmann, D. R. (1984). How advertising affects sales: A meta-analysis of econometric results. Journal of Marketing Research, 21 (1), 65–74.
Bijmolt, T. H. A., van Heerde, H., & Pieters, R. G. M. (2005). New empirical generalizations on the determinants of price elasticity. Journal of Marketing Research, 42 (2), 141–156.
Chatterjee, S., & Hadi, A. S. (1986). Influential observations, high leverage points, and outliers in linear regressions. Statistical Science, 1 (3), 379–416.
Google Scholar
Greene, W. H. (2008). Econometric analysis (6th ed.). Upper Saddle River: Pearson.
Gujarati, D. N. (2003). Basic econometrics (4th ed.). New York: McGraw Hill.
Hair, J. F., Black, W. C., Babin, J. B., & Anderson, R. E. (2014). Multivariate data analysis (7th ed.). Upper Saddle River: Pearson.
Hair, J. F., Hult, G. T. M., Ringle, C. M., & Sarstedt, M. (2017). A primer on partial least squares structural equation modeling (PLS-SEM) (2nd ed.). Thousand Oaks: Sage.
Hanssens, D. M., Parsons, L. J., & Schultz, R. L. (1990). Market response models: Econometric and time series analysis . Boston: Springer.
Hsiao, C. (2014). Analysis of panel data (3rd ed.). Cambridge: Cambridge University Press.
Book Google Scholar
Irwin, J. R., & McClelland, G. H. (2001). Misleading heuristics and moderated multiple regression models. Journal of Marketing Research, 38 (1), 100–109.
Koutsoyiannis, A. (1977). Theory of econometrics (2nd ed.). Houndmills: MacMillan.
Laurent, G. (2013). EMAC distinguished marketing scholar 2012: Respect the data! International Journal of Research in Marketing, 30 (4), 323–334.
Leeflang, P. S. H., Wittink, D. R., Wedel, M., & Neart, P. A. (2000). Building models for marketing decisions . Berlin: Kluwer.
Lodish, L. L., Abraham, M. M., Kalmenson, S., Livelsberger, J., Lubetkin, B., Richardson, B., & Stevens, M. E. (1995). How TV advertising works: A meta-analysis of 389 real world split cable T. V. advertising experiments. Journal of Marketing Research, 32 (2), 125–139.
Pindyck, R. S., & Rubenfeld, D. (1998). Econometric models and econometric forecasts (4th ed.). New York: McGraw-Hill.
Sethuraman, R., Tellis, G. J., & Briesch, R. A. (2011). How well does advertising work? Generalizations from meta-analysis of brand advertising elasticities. Journal of Marketing Research, 48 (3), 457–471.
Snijders, T. A. B., & Bosker, R. J. (2012). Multilevel analysis: An introduction to basic and advanced multilevel modeling (2nd ed.). London: Sage.
Stock, J., & Watson, M. (2015). Introduction to econometrics (3rd ed.). Upper Saddle River: Pearson.
Tellis, G. J. (1988). The price sensitivity of selective demand: A meta-analysis of econometric models of sales. Journal of Marketing Research, 25 (4), 391–404.
White, H. (1980). A heteroskedasticity-consistent covariance matrix estimator and a direct test for heteroskedasticity. Econometrica, 48 (4), 817–838.
Wooldridge, J. M. (2009). Introductory econometrics: A modern approach (4th ed.). Mason: South-Western Cengage.
Download references
Author information
Authors and affiliations.
Goethe University Frankfurt, Frankfurt, Germany
Bernd Skiera & Jochen Reiner
Kuehne Logistics University, Hamburg, Germany
Sönke Albers
You can also search for this author in PubMed Google Scholar
Corresponding author
Correspondence to Bernd Skiera .
Editor information
Editors and affiliations.
Department of Business-to-Business Marketing, Sales, and Pricing, University of Mannheim, Mannheim, Germany
Christian Homburg
Department of Marketing & Sales Research Group, Karlsruhe Institute of Technology (KIT), Karlsruhe, Germany
Martin Klarmann
Marketing & Sales Department, University of Mannheim, Mannheim, Germany
Arnd Vomberg
Rights and permissions
Reprints and permissions
Copyright information
© 2022 Springer Nature Switzerland AG
About this entry
Cite this entry.
Skiera, B., Reiner, J., Albers, S. (2022). Regression Analysis. In: Homburg, C., Klarmann, M., Vomberg, A. (eds) Handbook of Market Research. Springer, Cham. https://doi.org/10.1007/978-3-319-57413-4_17
Download citation
DOI : https://doi.org/10.1007/978-3-319-57413-4_17
Published : 03 December 2021
Publisher Name : Springer, Cham
Print ISBN : 978-3-319-57411-0
Online ISBN : 978-3-319-57413-4
eBook Packages : Business and Management Reference Module Humanities and Social Sciences Reference Module Business, Economics and Social Sciences
Share this entry
Anyone you share the following link with will be able to read this content:
Sorry, a shareable link is not currently available for this article.
Provided by the Springer Nature SharedIt content-sharing initiative
- Publish with us
Policies and ethics
- Find a journal
- Track your research

An official website of the United States government
The .gov means it’s official. Federal government websites often end in .gov or .mil. Before sharing sensitive information, make sure you’re on a federal government site.
The site is secure. The https:// ensures that you are connecting to the official website and that any information you provide is encrypted and transmitted securely.
- Publications
- Account settings
Preview improvements coming to the PMC website in October 2024. Learn More or Try it out now .
- Advanced Search
- Journal List
- Lippincott Open Access

Linear Regression in Medical Research
Patrick schober.
From the * Department of Anesthesiology, Amsterdam UMC, Vrije Universiteit Amsterdam, Amsterdam, the Netherlands
Thomas R. Vetter
† Department of Surgery and Perioperative Care, Dell Medical School at the University of Texas at Austin, Austin, Texas.
Related Article, see p 110
Linear regression is used to quantify the relationship between ≥1 independent (predictor) variables and a continuous dependent (outcome) variable.
In this issue of Anesthesia & Analgesia , Müller-Wirtz et al 1 report results of a study in which they used linear regression to assess the relationship in a rat model between tissue propofol concentrations and exhaled propofol concentrations (Figure.

Table 2 given in Müller-Wirtz et al, 1 showing the estimated relationships between tissue (or plasma) propofol concentrations and exhaled propofol concentrations. The authors appropriately report the 95% confidence intervals as a measure of the precision of their estimates, as well as the coefficient of determination ( R 2 ). The presented values indicate, for example, that (1) the exhaled propofol concentrations are estimated to increase on average by 4.6 units, equal to the slope (regression) coefficient, for each 1-unit increase of plasma propofol concentration; (2) the “true” mean increase could plausibly be expected to lie anywhere between 3.6 and 5.7 units as indicated by the slope coefficient’s confidence interval; and (3) the R 2 suggests that about 71% of the variability in the exhaled concentration can be explained by its relationship with plasma propofol concentrations.
Linear regression is used to estimate the association of ≥1 independent (predictor) variables with a continuous dependent (outcome) variable. 2 In the most simple case, thus referred to as “simple linear regression,” there is only one independent variable. Simple linear regression fits a straight line to the data points that best characterizes the relationship
between the dependent ( Y ) variable and the independent ( X ) variable, with the y -axis intercept ( b 0 ), and the regression coefficient being the slope ( b 1 ) of this line:

A model that includes several independent variables is referred to as “multiple linear regression” or “multivariable linear regression.” Even though the term linear regression suggests otherwise, it can also be used to model curved relationships.
Linear regression is an extremely versatile technique that can be used to address a variety of research questions and study aims. Researchers may want to test whether there is evidence for a relationship between a categorical (grouping) variable (eg, treatment group or patient sex) and a quantitative outcome (eg, blood pressure). The 2-sample t test and analysis of variance, 3 which are commonly used for this purpose, are essentially special cases of linear regression. However, linear regression is more flexible, allowing for >1 independent variable and allowing for continuous independent variables. Moreover, when there is >1 independent variable, researchers can also test for the interaction of variables—in other words, whether the effect of 1 independent variable depends on the value or level of another independent variable.
Linear regression not only tests for relationships but also quantifies their direction and strength. The regression coefficient describes the average (expected) change in the dependent variable for each 1-unit change in the independent variable for continuous independent variables or the expected difference versus a reference category for categorical independent variables. The coefficient of determination, commonly referred to as R 2 , describes the proportion of the variability in the outcome variable that can be explained by the independent variables. With simple linear regression, the coefficient of determination is also equal to the square of the Pearson correlation between the x and y values.
When including several independent variables, the regression model estimates the effect of each independent variable while holding the values of all other independent variables constant. 4 Thus, linear regression is useful (1) to distinguish the effects of different variables on the outcome and (2) to control for other variables—like systematic confounding in observational studies or baseline imbalances due to chance in a randomized controlled trial. Ultimately, linear regression can be used to predict the value of the dependent outcome variable based on the value(s) of the independent predictor variable(s).
Valid inferences from linear regression rely on its assumptions being met, including
- the residuals are the differences between the observed values and the values predicted by the regression model, and the residuals must be approximately normally distributed and have approximately the same variance over the range of predicted values;
- the residuals are also assumed to be uncorrelated. In simple language, the observations must be independent of each other; for example, there must not be repeated measurements within the same subjects. Other techniques like linear mixed-effects models are required for correlated data 5 ; and
- the model must be correctly specified, as explained in more detail in the next paragraph.
Whereas Müller-Wirtz et al 1 used simple linear regression to address their research question, researchers often need to specify a multivariable model and make choices on which independent variables to include and on how to model the functional relationship between variables (eg, straight line versus curve; inclusion of interaction terms).
Variable selection is a much-debated topic, and the details are beyond the scope of this Statistical Minute. Basically, variable selection depends on whether the purpose of the model is to understand the relationship between variables or to make predictions. This is also predicated on whether there is informed a priori theory to guide variable selection and on whether the model needs to control for variables that are not of primary interest but are confounders that could distort the relationship between other variables.
Omitting important variables or interactions can lead to biased estimates and a model that poorly describes the true underlying relationships, whereas including too many variables leads to modeling the noise (sampling error) in the data and reduces the precision of the estimates. Various statistics and plots, including adjusted R 2 , Mallows C p , and residual plots are available to assess the goodness of fit of the chosen linear regression model.
Thank you for visiting nature.com. You are using a browser version with limited support for CSS. To obtain the best experience, we recommend you use a more up to date browser (or turn off compatibility mode in Internet Explorer). In the meantime, to ensure continued support, we are displaying the site without styles and JavaScript.
- View all journals
- Explore content
- About the journal
- Publish with us
- Sign up for alerts
- Published: 29 October 2015
Points of Significance
Simple linear regression
- Naomi Altman 1 &
- Martin Krzywinski 2
Nature Methods volume 12 , pages 999–1000 ( 2015 ) Cite this article
50k Accesses
81 Citations
29 Altmetric
Metrics details
- Research data
- Statistical methods
“The statistician knows...that in nature there never was a normal distribution, there never was a straight line, yet with normal and linear assumptions, known to be false, he can often derive results which match, to a useful approximation, those found in the real world.” 1
You have full access to this article via your institution.
We have previously defined association between X and Y as meaning that the distribution of Y varies with X . We discussed correlation as a type of association in which larger values of Y are associated with larger values of X (increasing trend) or smaller values of X (decreasing trend) 2 . If we suspect a trend, we may want to attempt to predict the values of one variable using the values of the other. One of the simplest prediction methods is linear regression, in which we attempt to find a 'best line' through the data points.
Correlation and linear regression are closely linked—they both quantify trends. Typically, in correlation we sample both variables randomly from a population (for example, height and weight), and in regression we fix the value of the independent variable (for example, dose) and observe the response. The predictor variable may also be randomly selected, but we treat it as fixed when making predictions (for example, predicted weight for someone of a given height). We say there is a regression relationship between X and Y when the mean of Y varies with X .
In simple regression, there is one independent variable, X , and one dependent variable, Y . For a given value of X , we can estimate the average value of Y and write this as a conditional expectation E( Y | X ), often written simply as μ ( X ). If μ ( X ) varies with X , then we say that Y has a regression on X ( Fig. 1 ). Regression is a specific kind of association and may be linear or nonlinear ( Fig. 1c,d ).
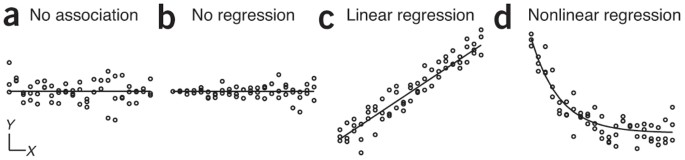
( a ) If the properties of Y do not change with X , there is no association. ( b ) Association is possible without regression. Here E( Y | X ) is constant, but the variance of Y increases with X . ( c ) Linear regression E( Y | X ) = β 0 + β 1 X . ( d ) Nonlinear regression E( Y | X ) = exp( β 0 + β 1 X ).
The most basic regression relationship is a simple linear regression. In this case, E( Y | X ) = μ ( X ) = β 0 + β 1 X , a line with intercept β 0 and slope β 1 . We can interpret this as Y having a distribution with mean μ ( X ) for any given value of X . Here we are not interested in the shape of this distribution; we care only about its mean. The deviation of Y from μ( X ) is often called the error, ε = Y – μ ( X ). It's important to realize that this term arises not because of any kind of error but because Y has a distribution for a given value of X . In other words, in the expression Y = μ ( X ) + ε , μ ( X ) specifies the location of the distribution, and ε captures its shape. To predict Y at unobserved values of X , one substitutes the desired values of X in the estimated regression equation. Here X is referred to as the predictor, and Y is referred to as the predicted variable.
Consider a relationship between weight Y (in kilograms) and height X (in centimeters), where the mean weight at a given height is μ ( X ) = 2 X /3 – 45 for X > 100. Because of biological variability, the weight will vary—for example, it might be normally distributed with a fixed σ = 3 ( Fig. 2a ). The difference between an observed weight and mean weight at a given height is referred to as the error for that weight.
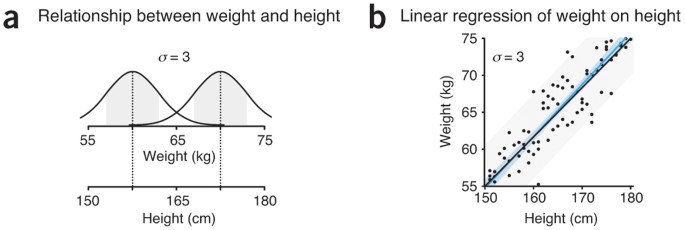
( a ) At each height, weight is distributed normally with s.d. σ = 3. ( b ) Linear regression of n = 3 weight measurements for each height. The mean weight varies as μ (Height) = 2 × Height/3 – 45 (black line) and is estimated by a regression line (blue line) with 95% confidence interval (blue band). The 95% prediction interval (gray band) is the region in which 95% of the population is predicted to lie for each fixed height.

The LSE of the regression line has favorable properties for very general error distributions, which makes it a popular estimation method. When Y values are selected at random from the conditional distribution E( Y | X ), the LSEs of the intercept, slope and fitted values are unbiased estimates of the population value regardless of the distribution of the errors, as long as they have zero mean. By “unbiased,” we mean that although they might deviate from the population values in any sample, they are not systematically too high or too low. However, because the LSE is very sensitive to extreme values of both X (high leverage points) and Y (outliers), diagnostic outlier analyses are needed before the estimates are used.
In the context of regression, the term “linear” can also refer to a linear model, where the predicted values are linear in the parameters. This occurs when E( Y|X ) is a linear function of a known function g ( X ), such as β 0 + β 1 g ( X ). For example, β 0 + β 1 X 2 and β 0 + β 1 sin( X ) are both linear regressions, but exp( β 0 + β 1 X ) is nonlinear because it is not a linear function of the parameters β 0 and β 1 . Analysis of variance (ANOVA) is a special case of a linear model in which the t treatments are labeled by indicator variables X 1 . . . X t , E( Y|X 1 . . . X t ) = μ i is the ith treatment mean, and the LSE predicted values are the corresponding sample means 3 .

If the errors are normally distributed, so are b 0 , b 1 and (ŷ(x)) . Even if the errors are not normally distributed, as long as they have zero mean and constant variance, we can apply a version of the central limit theorem for large samples 4 to obtain approximate normality for the estimates. In these cases the SE is very helpful in testing hypotheses. For example, to test that the slope is β 1 = 2/3, we would use t * = ( b 1 – β 1 )/SE( b 1 ); when the errors are normal and the null hypothesis true, t * has a t -distribution with d.f. = n – 2. We can also calculate the uncertainty of the regression parameters using confidence intervals, the range of values that are likely to contain β i (for example, 95% of the time) 5 . The interval is b i ± t 0.975 SE( b i ), where t 0.975 is the 97.5% percentile of the t -distribution with d.f. = n – 2.
When the errors are normally distributed, we can also use confidence intervals to make statements about the predicted value for a fixed value of X . For example, the 95% confidence interval for μ ( x ) is b 0 + b 1 x ± t 0.975 SE (ŷ(x)) ( Fig. 2b ) and depends on the error variance ( Fig. 3a ). This is called a point-wise interval because the 95% coverage is for a single fixed value of X . One can compute a band that covers the entire line 95% of the time by replacing t 0.975 with W 0.975 = √(2 F 0.975 ), where F 0.975 is the critical value from the F 2, n –2 distribution. This interval is wider because it must cover the entire regression line, not just one point on the line.
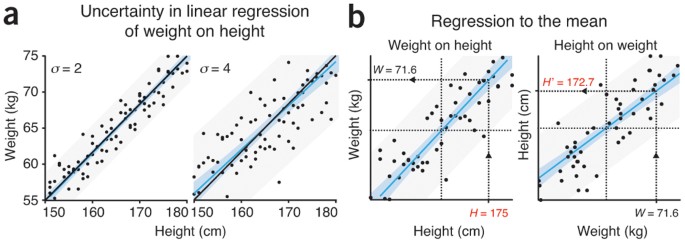
( a ) Uncertainty in a linear regression relationship can be expressed by a 95% confidence interval (blue band) and 95% prediction interval (gray band). Shown are regressions for the relationship in Figure 2a using different amounts of scatter (normally distributed with s.d. σ). ( b ) Predictions using successive regressions X → Y → X ′ to the mean. When predicting using height H = 175 cm (larger than average), we predict weight W = 71.6 kg (dashed line). If we then regress H on W at W = 71.6 kg, we predict H ′ = 172.7 cm, which is closer than H to the mean height (64.6 cm). Means of height and weight are shown as dotted lines.

Linear regression is readily extended to multiple predictor variables X 1 , . . ., X p , giving E( Y | X 1 , . . ., X p ) = β 0 + ∑ β i X i . Clever choice of predictors allows for a wide variety of models. For example, X i = X i yields a polynomial of degree p . If there are p + 1 groups, letting X i = 1 when the sample comes from group i and 0 otherwise yields a model in which the fitted values are the group means. In this model, the intercept is the mean of the last group, and the slopes are the differences in means.
A common misinterpretation of linear regression is the 'regression fallacy'. For example, we might predict weight W = 71.6 kg for a larger than average height H = 175 cm and then predict height H ′ = 172.7 cm for someone with weight W = 71.6 kg ( Fig. 3b ). Here we will find H ′ < H . Similarly, if H is smaller than average, we will find H ′> H . The regression fallacy is to ascribe a causal mechanism to regression to the mean, rather than realizing that it is due to the estimation method. Thus, if we start with some value of X , use it to predict Y , and then use Y to predict X , the predicted value will be closer to the mean of X than the original value ( Fig. 3b ).
Estimating the regression equation by LSE is quite robust to non-normality of and correlation in the errors, but it is sensitive to extreme values of both predictor and predicted. Linear regression is much more flexible than its name might suggest, including polynomials, ANOVA and other commonly used statistical methods.
Box, G. J. Am. Stat. Assoc. 71 , 791–799 (1976).
Article Google Scholar
Altman, N. & Krzywinski, M. Nat. Methods 12 , 899–900 (2015).
Article CAS Google Scholar
Krzywinski, M. & Altman, N. Nat. Methods 11 , 699–700 (2014).
Krzywinski, M. & Altman, N. Nat. Methods 10 , 809–810 (2013).
Krzywinski, M. & Altman, N. Nat. Methods 10 , 1041–1042 (2013).
Download references
Author information
Authors and affiliations.
Naomi Altman is a Professor of Statistics at The Pennsylvania State University.,
- Naomi Altman
Martin Krzywinski is a staff scientist at Canada's Michael Smith Genome Sciences Centre.,
- Martin Krzywinski
You can also search for this author in PubMed Google Scholar
Ethics declarations
Competing interests.
The authors declare no competing financial interests.
Rights and permissions
Reprints and permissions
About this article
Cite this article.
Altman, N., Krzywinski, M. Simple linear regression. Nat Methods 12 , 999–1000 (2015). https://doi.org/10.1038/nmeth.3627
Download citation
Published : 29 October 2015
Issue Date : November 2015
DOI : https://doi.org/10.1038/nmeth.3627
Share this article
Anyone you share the following link with will be able to read this content:
Sorry, a shareable link is not currently available for this article.
Provided by the Springer Nature SharedIt content-sharing initiative
This article is cited by
Errors in predictor variables.
Nature Methods (2024)
Comparing classifier performance with baselines
- Fadel M. Megahed
- Ying-Ju Chen
Grazing by nano- and microzooplankton on heterotrophic picoplankton dominates the biological carbon cycling around the Western Antarctic Peninsula
- Sebastian Böckmann
- Scarlett Trimborn
- Florian Koch
Polar Biology (2024)
A multi-scenario multi-model analysis of regional climate projections in a Central–Eastern European agricultural region: assessing shallow groundwater table responses using an aggregated vertical hydrological model
- László Koncsos
- Gábor Murányi
Applied Water Science (2024)
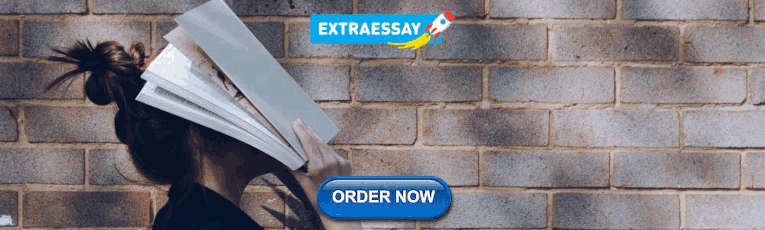
Neural networks primer
- Alexander Derry
Nature Methods (2023)
Quick links
- Explore articles by subject
- Guide to authors
- Editorial policies
Sign up for the Nature Briefing newsletter — what matters in science, free to your inbox daily.

Subscribe to the PwC Newsletter
Join the community, edit method, add a method collection.
- GENERALIZED LINEAR MODELS
Remove a collection
- GENERALIZED LINEAR MODELS -
Add A Method Component
Remove a method component, linear regression.
Linear Regression is a method for modelling a relationship between a dependent variable and independent variables. These models can be fit with numerous approaches. The most common is least squares , where we minimize the mean square error between the predicted values $\hat{y} = \textbf{X}\hat{\beta}$ and actual values $y$: $\left(y-\textbf{X}\beta\right)^{2}$.
We can also define the problem in probabilistic terms as a generalized linear model (GLM) where the pdf is a Gaussian distribution, and then perform maximum likelihood estimation to estimate $\hat{\beta}$.
Image Source: Wikipedia
Usage Over Time
Categories edit add remove.
A comparative analysis on linear regression and support vector regression
Ieee account.
- Change Username/Password
- Update Address
Purchase Details
- Payment Options
- Order History
- View Purchased Documents
Profile Information
- Communications Preferences
- Profession and Education
- Technical Interests
- US & Canada: +1 800 678 4333
- Worldwide: +1 732 981 0060
- Contact & Support
- About IEEE Xplore
- Accessibility
- Terms of Use
- Nondiscrimination Policy
- Privacy & Opting Out of Cookies
A not-for-profit organization, IEEE is the world's largest technical professional organization dedicated to advancing technology for the benefit of humanity. © Copyright 2024 IEEE - All rights reserved. Use of this web site signifies your agreement to the terms and conditions.
multiple linear regression Recently Published Documents
Total documents.
- Latest Documents
- Most Cited Documents
- Contributed Authors
- Related Sources
- Related Keywords
The Effect of Conflict and Termination of Employment on Employee's Work Spirit
This study aims to find out the conflict and termination of employment both partially and simultaneously have a significant effect on the morale of employees at PT. The benefits of Medan Technique and how much it affects. The method used in this research is quantitative method with several tests namely reliability analysis, classical assumption deviation test and linear regression. Based on the results of primary data regression processed using SPSS 20, multiple linear regression equations were obtained as follows: Y = 1,031 + 0.329 X1+ 0.712 X2.In part, the conflict variable (X1)has a significant effect on the employee's work spirit (Y) at PT. Medan Technical Benefits. This means that the hypothesis in this study was accepted, proven from the value of t calculate > t table (3,952 < 2,052). While the variable termination of employment (X2) has a significant influence on the work spirit of employees (Y) in PT. Medan Technical Benefits. This means that the hypothesis in this study was accepted, proven from the value of t calculate > t table (7,681 > 2,052). Simultaneously, variable conflict (X1) and termination of employment (X2) have a significant influence on the morale of employees (Y) in PT. Medan Technical Benefits. This means that the hypothesis in this study was accepted, as evidenced by the calculated F value > F table (221,992 > 3.35). Conflict variables (X1) and termination of employment (X2) were able to contribute an influence on employee morale variables (Y) of 94.3% while the remaining 5.7% was influenced by other variables not studied in this study. From the above conclusions, the author advises that employees and leaders should reduce prolonged conflict so that the spirit of work can increase. Leaders should be more selective in severing employment relationships so that decent employees are not dismissed unilaterally. Employees should work in a high spirit so that the company can see the quality that employees have.
Prediction of Local Government Revenue using Data Mining Method
Local Government Revenue or commonly abbreviated as PAD is part of regional income which is a source of regional financing used to finance the running of government in a regional government. Each local government must plan Local Government Revenue for the coming year so that a forecasting method is needed to determine the Local Government Revenue value for the coming year. This study discusses several methods for predicting Local Government Revenue by using data on the realization of Local Government Revenue in the previous years. This study proposes three methods for forecasting local Government revenue. The three methods used in this research are Multiple Linear Regression, Artificial Neural Network, and Deep Learning. In this study, the data used is Local Revenue data from 2010 to 2020. The research was conducted using RapidMiner software and the CRISP-DM framework. The tests carried out showed an RMSE value of 97 billion when using the Multiple Linear Regression method and R2 of 0,942, the ANN method shows an RMSE value of 135 billion and R2 of 0.911, and the Deep Learning method shows the RMSE value of 104 billion and R2 of 0.846. This study shows that for the prediction of Local Government Revenue, the Multiple Linear Regression method is better than the ANN or Deep Learning method. Keywords— Local Government Revenue, Multiple Linear Regression, Artificial Neural Network, Deep Learning, Coefficient of Determination
Analisis Peran Motivasi sebagai Mediasi Pengaruh Trilogi Kepemimpinan dan Kepuasan Kerja terhadap Produktivitas Kerja Karyawan PT. Mataram Tunggal Garment
The purpose of this study is to find out the motivation to mediate the leadership trilogy and job satisfaction to employee work productivity at PT. Mataram Tunggal Garment. The method used in this study is quantitative. Primary data was obtained from questionnaires with 78 respondents with saturated sample techniques. Then the data is analyzed using descriptive analysis, multiple linear regression tests, t (partial) tests, coesifisien determination (R2) and sobel tests. The results showed that job satisfaction had a significant influence on motivation, leadership trilogy and job satisfaction had a significant influence on employee work productivity, leadership trilogy and motivation had no significant effect on employee work productivity, motivation mediated leadership trilogy and job satisfaction had no insignificant effect on employee work productivity. Keywords: Leadership Trilogy, Motivation, Job Satisfaction and Employee Productivity.
Prevalence of asymptomatic hyperuricemia and its association with prediabetes, dyslipidemia and subclinical inflammation markers among young healthy adults in Qatar
Abstract Aim The aim of this study is to investigate the prevalence of asymptomatic hyperuricemia in Qatar and to examine its association with changes in markers of dyslipidemia, prediabetes and subclinical inflammation. Methods A cross-sectional study of young adult participants aged 18 - 40 years old devoid of comorbidities collected between 2012 and 2017. Exposure was defined as uric acid level, and outcomes were defined as levels of different blood markers. De-identified data were collected from Qatar Biobank. T-tests, correlation tests and multiple linear regression were all used to investigate the effects of hyperuricemia on blood markers. Statistical analyses were conducted using STATA 16. Results The prevalence of asymptomatic hyperuricemia is 21.2% among young adults in Qatar. Differences between hyperuricemic and normouricemic groups were observed using multiple linear regression analysis and found to be statistically and clinically significant after adjusting for age, gender, BMI, smoking and exercise. Significant associations were found between uric acid level and HDL-c p = 0.019 (correlation coefficient -0.07 (95% CI [-0.14, -0.01]); c-peptide p = 0.018 (correlation coefficient 0.38 (95% CI [0.06, 0.69]) and monocyte to HDL ratio (MHR) p = 0.026 (correlation coefficient 0.47 (95% CI [0.06, 0.89]). Conclusions Asymptomatic hyperuricemia is prevalent among young adults and associated with markers of prediabetes, dyslipidemia, and subclinical inflammation.
Screen Time, Age and Sunshine Duration Rather Than Outdoor Activity Time Are Related to Nutritional Vitamin D Status in Children With ASD
Objective: This study aimed to investigate the possible association among vitamin D, screen time and other factors that might affect the concentration of vitamin D in children with autism spectrum disorder (ASD).Methods: In total, 306 children with ASD were recruited, and data, including their age, sex, height, weight, screen time, time of outdoor activity, ASD symptoms [including Autism Behavior Checklist (ABC), Childhood Autism Rating Scale (CARS) and Autism Diagnostic Observation Schedule–Second Edition (ADOS-2)] and vitamin D concentrations, were collected. A multiple linear regression model was used to analyze the factors related to the vitamin D concentration.Results: A multiple linear regression analysis showed that screen time (β = −0.122, P = 0.032), age (β = −0.233, P < 0.001), and blood collection month (reflecting sunshine duration) (β = 0.177, P = 0.004) were statistically significant. The vitamin D concentration in the children with ASD was negatively correlated with screen time and age and positively correlated with sunshine duration.Conclusion: The vitamin D levels in children with ASD are related to electronic screen time, age and sunshine duration. Since age and season are uncontrollable, identifying the length of screen time in children with ASD could provide a basis for the clinical management of their vitamin D nutritional status.
Determining Factors of Fraud in Local Government
The objectives of this research are to analyze determining factors of fraud in local government. This study used internal control effectiveness, compliance with accounting rules, compensation compliance, and unethical behavior as an independent variable, while fraud as the dependent variable. The research was conducted at Bantul local government (OPD). The sample of this research were 86 respondents. The sample uses a purposive sampling method. The respondent data is analyzed with multiple linear regression. The results showed: Internal control effectiveness has an impact on fraud. Compliance with accounting rules does not affect fraud. Compensations compliance does not affect fraud. Unethical behavior has an impact on fraud.
PENGARUH TINGKAT EFEKTIVITAS PERPUTARAN KAS, PIUTANG, DAN MODAL KERJA TERHADAP RENTABILITAS EKONOMI PADA KOPERASI PEDAGANG PASAR GROGOLAN BARU (KOPPASGOBA) PERIODE 2016-2020
This study aims to test and analyze the effect of effectiveness of cash turnover, receivables,and working capital on economic rentability in the New Grogolan Market Traders Cooperative of Pekalongan City from 2016 to 2020. The method used in this study was quantitative research method with documentation techniques and analyzed used multiple linear regression analysis. The results of this study showed (1) the effectiveness of cash turnover has no significant effect on economic rentability, (2) the effectiveness of receivables turnover has no significant effect on economic rentability, (3) the effectiveness of working capital turnover has a positive and significant effect on economic rentability, and (4) there is a positive and significant effect on the effectiveness of cash turnover, receivables, and working capital together on economic rentability. Keywords: Turnover of cash, turnover of receivables, turnover of working capital, and economic rentability.
Improvement of AHMES Using AI Algorithms
This research aims to improve the rationality and intelligence of AUTOMATICALLY HIGHER MATHEMATICALLY EXAM SYSTEM (AHMES) through some AI algorithms. AHMES is an intelligent and high-quality higher math examination solution for the Department of Computer Engineering at Pai Chai University. This research redesigned the difficulty system of AHMES and used some AI algorithms for initialization and continuous adjustment. This paper describes the multiple linear regression algorithm involved in this research and the AHMES learning (AL) algorithm improved by the Q-learning algorithm. The simulation test results of the upgraded AHMES show the effectiveness of these algorithms.
ANALISIS PENGARUH KUALITAS PELAYANAN, PROMOSI DAN HARGA TERHADAP KEPUASAN PELANGGAN PADA JASA PENGIRIMAN BARANG JNE DI BESUKI
This research was conducted to see the effect of service quality, promotion and price on customer satisfaction. This research was conducted at the Besuki branch of JNE. Sampling was done by random sampling technique where all the population was taken at random to be the research sample. This is done to increase customer satisfaction at JNE Besuki branch through service quality, promotion and price. The analytical tool used is multiple linear regression to determine service quality, promotion and price on customer satisfaction. The results show that service quality affects customer satisfaction, promotion affects customer satisfaction, price affects customer satisfaction. Keyword : service quality, promotion, price, customer satisfaction
PENGARUH KUALITAS PRODUK, HARGA DAN INFLUENCER MARKETING TERHADAP KEPUTUSAN PEMBELIAN SCARLETT BODY WHITENING
This research aimed to figure out the influence between product quality, price and marketing influencer with the purchasing decision of Scarlett Body Whitening in East Java. The research instrument employed questionnaire to collect data from Scarlett Body Whitening consumers in East Java. Since there was no valid data for number of the consumers, the research used Roscoe method to take the sample. Data analyzed using multiple linear regression test. Product quality and price have a positive and significant effect on purchasing decisions. Meanwhile, the marketing influencer had no significant effect on purchase decision for Scarlett Body Whitening. Need further research to ensure that marketing influencer had an effect on purchase decision. Keywords: Product quality, price, marketing influencer, buying decision
Export Citation Format
Share document.
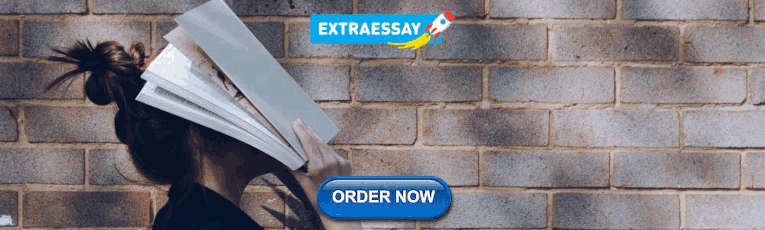
IMAGES
VIDEO
COMMENTS
Linear regression refers to the mathematical technique of fitting given data to a function of a certain type. It is best known for fitting straight lines. In this paper, we explain the theory behind linear regression and illustrate this technique with a real world data set. This data relates the earnings of a food truck and the population size of the city where the food truck sells its food.
Linear regression... | Find, read and cite all the research you need on ResearchGate. Article PDF Available. ... (PRISMA) method was adopted to select papers for review. The study reviewed 44 ...
Example: regression line for a multivariable regression Y = -120.07 + 100.81 × X 1 + 0.38 × X 2 + 3.41 × X 3, where. X 1 = height (meters) X 2 = age (years) ... The study of relationships between variables and the generation of risk scores are very important elements of medical research. The proper performance of regression analysis ...
The linear regression has two types: simple regression and multiple regression (MLR). This paper discusses various works by different researchers on linear regression and polynomial regression and ...
Linear regression is used to quantify a linear relationship or association between a continuous response/outcome variable or ... Logistic regression in medical research. Anesth Analg. 2021;132:365 ...
The aim of linear regression analysis is to estimate the coefficients of the regression equation b 0 and b k (k∈K) so that the sum of the squared residuals (i.e., the sum over all squared differences between the observed values of the i th observation of y i and the corresponding predicted values \( {\hat{y}}_i \)) is minimized.The lower part of Fig. 1 illustrates this approach, which is ...
When we use the regression sum of squares, SSR = Σ ( ŷi − Y−) 2, the ratio R2 = SSR/ (SSR + SSE) is the amount of variation explained by the regression model and in multiple regression is ...
Linear regression is an extremely versatile technique that can be used to address a variety of research questions and study aims. Researchers may want to test whether there is evidence for a relationship between a categorical (grouping) variable (eg, treatment group or patient sex) and a quantitative outcome (eg, blood pressure).
Least squares linear regression is one of the oldest and widely used data analysis tools. Although the theoretical analysis of the ordinary least squares (OLS) estimator is as old, several fundamental questions are yet to be answered. Suppose regression observations $(X_1,Y_1),\\ldots,(X_n,Y_n)\\in\\mathbb{R}^d\\times\\mathbb{R}$ (not necessarily independent) are available. Some of the ...
The most basic regression relationship is a simple linear regression. In this case, E ( Y | X) = μ ( X) = β0 + β1X, a line with intercept β0 and slope β1. We can interpret this as Y having a ...
Linear regression is used to quantify the relationship between ≥1 independent (predictor) variables and a continuous dependent (outcome) variable. In this issue of Anesthesia & Analgesia, Müller-Wirtz et al 1 report results of a study in which they used linear regression to assess the relationship in a rat model between tissue propofol ...
Regression analysis allows researchers to understand the relationship between two or more variables by estimating the mathematical relationship between them (Sarstedt & Mooi, 2014). In this case ...
The method used in this research is quantitative method with several tests namely reliability analysis, classical assumption deviation test and linear regression. Based on the results of primary data regression processed using SPSS 20, multiple linear regression equations were obtained as follows: Y = 1,031 + 0.329 X1+ 0.712 X2.In part, the ...
In both cases, we still use the term 'linear' because we assume that the response variable is directly related to a linear combination of the explanatory variables. The equation for multiple linear regression has the same form as that for simple linear regression but has more terms: = 0 +. 1 +. 2 + ⋯ +.
Linear Regression is a method for modelling a relationship between a dependent variable and independent variables. These models can be fit with numerous approaches. ... Stay informed on the latest trending ML papers with code, research developments, libraries, methods, and datasets. ... Papers With Code is a free resource with all data licensed ...
In this study, data for multilinear regression analysis is occur from Sakarya University Education Faculty student's lesson (measurement and evaluation, educational psychology, program development, counseling and instructional techniques) scores and their 2012- KPSS score. Assumptions of multilinear regression analysis- normality, linearity, no ...
Regression allows you to estimate how a dependent variable changes as the independent variable (s) change. Simple linear regression example. You are a social researcher interested in the relationship between income and happiness. You survey 500 people whose incomes range from 15k to 75k and ask them to rank their happiness on a scale from 1 to ...
Abstract—In this paper, market values of the football players in the forward positions are estimated using multiple. linear regression by including the physical and performance factors in 2017-2018 season. Players from 4 major. leagues of Europe are examined, and by applying Breusch - Pagan test for homoscedasticity, a reasonable regression.
Quality (SACMEQ). The data were submitted to linear regression analysis through structural equation modelling using AMOS 4.0. In our results, we showed that a proxy for SES was the strongest predictor of reading achievement. Zimbabwe, reading achievement, home environment, linear regression, structural equation modelling INTRODUCTION
In this paper, linear regression and support vector regression model is compared using the training data set in order to use the correct model for better prediction and accuracy. Published in: 2016 Online International Conference on Green Engineering and Technologies (IC-GET)
Regression methods are then discussed with fair length focusing on linear regression. We conclude the research with an application of a real-life regression problem. Example of association learning
This paper describes the multiple linear regression algorithm involved in this research and the AHMES learning (AL) algorithm improved by the Q-learning algorithm. The simulation test results of the upgraded AHMES show the effectiveness of these algorithms. Download Full-text.
Based on near-infrared (NIR) spectroscopy, a new quantitative calibration algorithm, called "Partial Least Squares Regression Residual Extreme Learning Machine (PLSRR-ELM)", was proposed for fast determination of research octane number (RON) for blended gasoline. In this algorithm, partial least square (PLS) cooperates with non-linear extreme learning machine (ELM) to separate the relationship ...
This is paper presented a multiple linear regression model and logistic regression model, according to assumptions of both models. The paper depended on logistic regression model because the ...