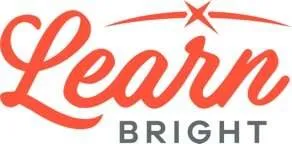
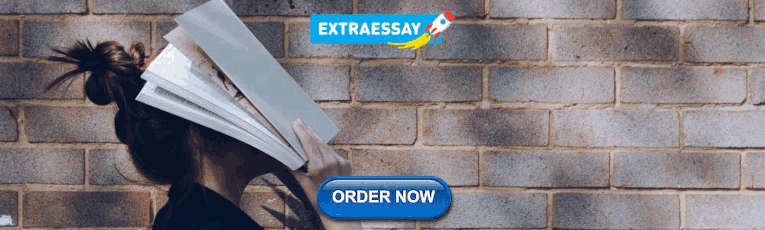
Acids and Bases
Acids and Bases introduces students to pH levels and how to tell the difference between an acid and a base. Students will learn to define and identify both types of substances and explain how they differ. They will also discover why this type of information is important to know.
In the “Options for Lesson” section of the classroom procedure page, you will find several suggestions to add to your lesson delivery. One option is to use litmus paper to test the pH level of a number of substances, including students’ favorite drinks that they bring to class.
Description
Additional information, what our acids and bases lesson plan includes.
Lesson Objectives and Overview: Acids and Bases explores acidic and basic substances and how to measure their pH levels. Students will learn the traits of both an acid and a base. They will be able to explain how the two substances differ. They will also learn what pH means and how it helps scientists measure the hydrogen levels of many substances. This lesson is for students in 5th grade and 6th grade.
Classroom Procedure
Every lesson plan provides you with a classroom procedure page that outlines a step-by-step guide to follow. You do not have to follow the guide exactly. The guide helps you organize the lesson and details when to hand out worksheets. It also lists information in the yellow box that you might find useful. You will find the lesson objectives, state standards, and number of class sessions the lesson should take to complete in this area. In addition, it describes the supplies you will need as well as what and how you need to prepare beforehand. You will need a number of supplies for this lesson—white construction paper, scissors, glue, and colored pencils. If you can, try to obtain litmus paper and a variety of liquids to test their pH values.
Options for Lesson
The “Options for Lesson” section on the classroom procedure page provides a number of extra suggestions for additional activities or ways to alter different parts of the lesson. One suggestion is to get litmus paper and about 10 to 12 substances for students to test and identify as either acids or bases. Another idea is to assign the practice worksheet before the activity rather than after. Students could also bring their favorite drink to class and test whether it is basic or acidic according to its pH level. Another option is to invite a chemist to the class to discuss more about acids and bases with the students.
Teacher Notes
The teacher notes page includes a paragraph that provides a little more information about the lesson or more ideas. It suggests obtaining litmus paper if possible as doing so will greatly enhance the lesson plan and students’ learning about acids and bases. You can use the blank lines on the page to write down any other ideas or thoughts you have prior to the lesson.
ACIDS AND BASES LESSON PLAN CONTENT PAGES
The Acids and Bases lesson plan contains three pages of content. The first page explains why all drinks and some foods have a specific taste. Some are more sour while others are on the bitter side. Examples of sour foods or liquids include buttermilk, lemon juice, and some candies. Other things taste bitter. The foods and drinks that have a sour taste are acidic, and the ones with a bitter taste are more basic.
The lesson provides lists containing facts about acids and bases along with examples of each. Students will learn that the word acid comes from a Latin word that means sour— acere . Some acids are natural, meaning they exist in nature. People often drink liquids that contain these acids. An acid is a molecule that splits apart in water and releases hydrogen ions.
The stomach contains hydrochloric acid, which it uses to digest food and kill disease-causing germs. Batteries of all kinds also contain a type of acid. That includes the batteries you put in toys or remote controls as well as the ones that go inside a real car. Many drinkable liquids also contain acids, like lemon juice, orange juice, and tomato juice.
Bases, on the other hand, have a bitter taste and a soap-like texture. They just happen to feel soapy when rubbing between the fingers. These substances also occur naturally. A base is a molecule that splits apart in water and releases hydroxide ions, which reduces the number of hydrogen ions.
Examples of bases include soapy water, milk, bleach, and Milk of Magnesia. In the body, the pancreas contains a basic substance that helps with digestion. People use bases in household cleaning products and crop fertilizing.
The Indicators
The first person to define these two types of substances was a chemist named Svante Arrhenius in 1887. It would be impossible to determine whether a substance is acidic or basic by tasting every liquid in nature. It would also be dangerous, after all. Instead, there is a special type of substance scientists use to determine whether a liquid is acidic or basic in nature. This special substance is called an indicator.
There are multiple indicators that help scientists with this task. Indicators change color depending on whether the substance is an acid or a base. Three naturally occurring indicators include litmus, turmeric, and China rose. If someone dips any one of these into a liquid, the liquid will change color, which indicates its base or acid level.
Students will discover that the indicator people most commonly use is litmus. Its natural color is purple. When an acidic solution touches it, it will turn red. On the other hand, it will turn blue if someone dips the litmus into a basic solution. Litmus comes from organisms called lichens, which come from both fungi and algae. In addition, litmus can come in either a paper form or as a solution.
The final page of the lesson discusses pH and how this helps determine the a substance’s acidic or basic level. The lesson shows the pH spectrum that all substances fall on, ranging from 0 to 14. The most acidic substances have a low pH, while the most basic substances have a high pH. This means that while two solutions are acidic, one might be more acidic than the other. For instance, black coffee is acidic, and so is the gastric acid in the digestive system. Black coffee, however, is less acidic than gastric acid and thus has a higher pH level.
A pH scale, then, measures how basic or acidic a solution is. It stands for potential of hydrogen, meaning that it measures how many hydrogen ions are in a specific solution. The more hydrogen ions, the more acidic a solution. The more hydroxide ions, the more basic a solution. Liquids that have a pH between 0 and 7 are acidic, and those with 0 are the strongest acids. Bases have pH scores between 7 and 14, with the score of 14 indicating the strongest base. A score of 7 indicates that a solution is neutral, meaning that there is a balance between both the hydrogen and hydroxide ions. Pure water is a neutral substance with a pH of 7.
Acids with a low pH and bases with a high pH are highly dangerous and very reactive. In fact, they could corrode or burn a person’s skin. But people can use the two types to neutralize each other. When a person’s stomach has excess acid, for example, they could drink milk or use a medicine called Milk of Magnesia to neutralize the acid and make their stomach feel better. Students will be interested to learn that toothpaste actually does something similar! Food particles decay in the mouth and increase the acidity levels, leading to tooth decay. Toothpaste helps neutralize the acid and thus prevent tooth decay.
ACIDS AND BASES LESSON PLAN WORKSHEETS
The Acids and Bases lesson plan includes three worksheets: an activity worksheet, a practice worksheet, and a homework assignment. Each worksheet will help reinforce students’ comprehension of the concepts and material they learned throughout the lesson. You can refer to the guide on the classroom procedure page which outlines when to hand out the worksheets.
ACIDIC TO BASIC ACTIVITY WORKSHEET
For the activity, students will cut out 12 pictures of different substances. Using what they learned during the lesson, they will have to order them from most acidic to most basic. They will glue the images onto a piece of construction paper that shows the pH scale.
ACIDS AND BASES PRACTICE WORKSHEET
There are two parts of the practice worksheet. The first part requires students to match statements to the correct term. There are 15 statements and terms to match up. The second section requires them to compare two liquids to each other. There are 10 pairs of liquids to compare in this section.
WHAT DID YOU LEARN HOMEWORK ASSIGNMENT
Students must answer 20 questions that relate to the information they learned throughout the lesson. If you want to allow students to use the content pages for reference, you may do so. You may, however, wish to test their memory of the lesson material instead.
Worksheet Answer Keys
The final pages of the lesson document are answer keys for the worksheets. The activity answer key displays where each liquid should go and orders them from most acidic to most basic. (In the bases section, you will follow from left to right and top to bottom.) The answers on the practice and homework worksheets are in red. For the most part, students’ responses should match exactly. However, a few questions on the homework assignment may include some variation due to the nature of the question. If you choose to administer the lesson pages to your students via PDF, you will need to save a new file that omits these pages. Otherwise, you can simply print out the applicable pages and keep these as reference for yourself when grading assignments.
Thank you for submitting a review!
Your input is very much appreciated. Share it with your friends so they can enjoy it too!
Great curriculum Resource
My students and I enjoy utilizing all of the curriculum resources from Learn Bright!
Acid and base
Excellent, it made my teaching very easy and the student enjoyed the class.
Wow amazing.
This is so informative and fun for my kids. We loved it thank you.
Very useful!
Easy to download and very student friendly materials!
Great teaching resource
I wish I had found this website a month ago! The lesson plans are clear, succinct, and detailed. There are brightly colored pictures, examples, and review questions for the kids and clear instructions for me so that I am no longer stressing about what about the material is important to go over or if I am missing anything. Thank you!
Related products
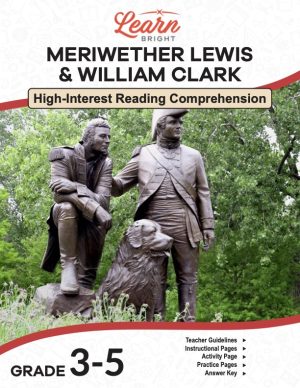
Meriwether Lewis and William Clark
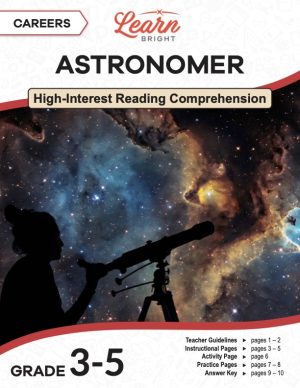
Careers: Astronomer
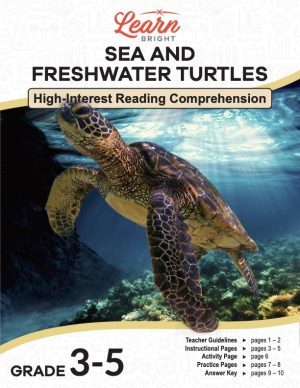
Sea and Freshwater Turtles
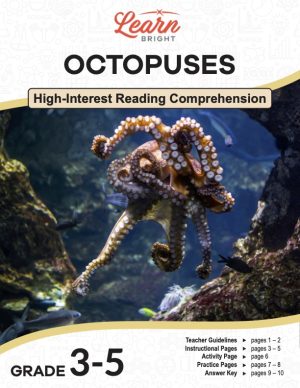
Make Your Life Easier With Our Lesson Plans
Stay up-to-date with new lessons.

- Lesson Plans
- For Teachers
© 2024 Learn Bright. All rights reserved. Terms and Conditions. Privacy Policy.
- Sign Up for Free
Browse Course Material
Course info.
- Prof. Donald Sadoway
Departments
- Materials Science and Engineering
As Taught In
- Chemical Engineering
Learning Resource Types
Introduction to solid state chemistry, 26. acids & bases.
« Previous | Next »
Session Overview
Prerequisites.
Before starting this session, you should be familiar with:
- the Molecules and Bonding module ( Session 7 through Session 12 )
- Solutions ( Session 25 )
Learning Objectives
After completing this session, you should be able to:
- Compare the acid-base models of Arrhenius, Brønsted-Lowry, and Lewis, and know the salient features of each.
- Write the general acid-base reaction.
- Explain the chemical basis of acid strength and the pH measurement.
- Describe the behaviors of conjugate acid-base pairs.
- Explain how dissociation affects ionic compounds.
- Solve specific acid-base reaction problems.
Lecture Video
- Download video
- Download transcript
Lecture Slides (PDF - 2.2MB)
Lecture Summary
This lecture introduces the chemical models and behaviors of acids and bases. Starting from the historical origins (“acid” derives from the Latin acidus , meaning “sour”), Prof. Sadoway discusses the evolving acid-base models of Lavoisier (1776), Arrhenius (1887), Brønsted and Lowry (1923), and Lewis (1923-1938).
The lecture proceeds to cover:
- The general acid-base reaction
- Conjugate acid-base pairs
- The dissociation process of ionic compounds
- Solving acid-base reaction problems
Problems (PDF)
Solutions (PDF)
Textbook Problems
For further study, supplemental readings.
Djerassi, C., and R. Hoffmann. Oxygen: A Play in Two Acts . New York, NY: Wiley-VCH, 2001. ISBN: 9783527304134. See also the study guide and other publisher resources .
Brandis, Kerry. Acid-Base Physiology . See Chapter 1 of this online tutorial/textbook, which applies acid-base chemistry to physiology.
Antoine Lavoisier
Svante Arrhenius — 1903 Nobel Prize in Chemistry
Johannes Nicolaus Brønsted
Martin Lowry
Gilbert N. Lewis
Other OCW and OER Content

You are leaving MIT OpenCourseWare
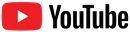
- TPC and eLearning
- Read Watch Interact
- What's NEW at TPC?
- Practice Review Test
- Teacher-Tools
- Subscription Selection
- Seat Calculator
- Ad Free Account
- Edit Profile Settings
- Classes (Version 2)
- Student Progress Edit
- Task Properties
- Export Student Progress
- Task, Activities, and Scores
- Metric Conversions Questions
- Metric System Questions
- Metric Estimation Questions
- Significant Digits Questions
- Proportional Reasoning
- Acceleration
- Distance-Displacement
- Dots and Graphs
- Graph That Motion
- Match That Graph
- Name That Motion
- Motion Diagrams
- Pos'n Time Graphs Numerical
- Pos'n Time Graphs Conceptual
- Up And Down - Questions
- Balanced vs. Unbalanced Forces
- Change of State
- Force and Motion
- Mass and Weight
- Match That Free-Body Diagram
- Net Force (and Acceleration) Ranking Tasks
- Newton's Second Law
- Normal Force Card Sort
- Recognizing Forces
- Air Resistance and Skydiving
- Solve It! with Newton's Second Law
- Which One Doesn't Belong?
- Component Addition Questions
- Head-to-Tail Vector Addition
- Projectile Mathematics
- Trajectory - Angle Launched Projectiles
- Trajectory - Horizontally Launched Projectiles
- Vector Addition
- Vector Direction
- Which One Doesn't Belong? Projectile Motion
- Forces in 2-Dimensions
- Being Impulsive About Momentum
- Explosions - Law Breakers
- Hit and Stick Collisions - Law Breakers
- Case Studies: Impulse and Force
- Impulse-Momentum Change Table
- Keeping Track of Momentum - Hit and Stick
- Keeping Track of Momentum - Hit and Bounce
- What's Up (and Down) with KE and PE?
- Energy Conservation Questions
- Energy Dissipation Questions
- Energy Ranking Tasks
- LOL Charts (a.k.a., Energy Bar Charts)
- Match That Bar Chart
- Words and Charts Questions
- Name That Energy
- Stepping Up with PE and KE Questions
- Case Studies - Circular Motion
- Circular Logic
- Forces and Free-Body Diagrams in Circular Motion
- Gravitational Field Strength
- Universal Gravitation
- Angular Position and Displacement
- Linear and Angular Velocity
- Angular Acceleration
- Rotational Inertia
- Balanced vs. Unbalanced Torques
- Getting a Handle on Torque
- Torque-ing About Rotation
- Properties of Matter
- Fluid Pressure
- Buoyant Force
- Sinking, Floating, and Hanging
- Pascal's Principle
- Flow Velocity
- Bernoulli's Principle
- Balloon Interactions
- Charge and Charging
- Charge Interactions
- Charging by Induction
- Conductors and Insulators
- Coulombs Law
- Electric Field
- Electric Field Intensity
- Polarization
- Case Studies: Electric Power
- Know Your Potential
- Light Bulb Anatomy
- I = ∆V/R Equations as a Guide to Thinking
- Parallel Circuits - ∆V = I•R Calculations
- Resistance Ranking Tasks
- Series Circuits - ∆V = I•R Calculations
- Series vs. Parallel Circuits
- Equivalent Resistance
- Period and Frequency of a Pendulum
- Pendulum Motion: Velocity and Force
- Energy of a Pendulum
- Period and Frequency of a Mass on a Spring
- Horizontal Springs: Velocity and Force
- Vertical Springs: Velocity and Force
- Energy of a Mass on a Spring
- Decibel Scale
- Frequency and Period
- Closed-End Air Columns
- Name That Harmonic: Strings
- Rocking the Boat
- Wave Basics
- Matching Pairs: Wave Characteristics
- Wave Interference
- Waves - Case Studies
- Color Addition and Subtraction
- Color Filters
- If This, Then That: Color Subtraction
- Light Intensity
- Color Pigments
- Converging Lenses
- Curved Mirror Images
- Law of Reflection
- Refraction and Lenses
- Total Internal Reflection
- Who Can See Who?
- Formulas and Atom Counting
- Atomic Models
- Bond Polarity
- Entropy Questions
- Cell Voltage Questions
- Heat of Formation Questions
- Reduction Potential Questions
- Oxidation States Questions
- Measuring the Quantity of Heat
- Hess's Law
- Oxidation-Reduction Questions
- Galvanic Cells Questions
- Thermal Stoichiometry
- Molecular Polarity
- Quantum Mechanics
- Balancing Chemical Equations
- Bronsted-Lowry Model of Acids and Bases
- Classification of Matter
- Collision Model of Reaction Rates
- Density Ranking Tasks
- Dissociation Reactions
- Complete Electron Configurations
- Elemental Measures
- Enthalpy Change Questions
- Equilibrium Concept
- Equilibrium Constant Expression
- Equilibrium Calculations - Questions
- Equilibrium ICE Table
- Ionic Bonding
- Lewis Electron Dot Structures
- Limiting Reactants
- Line Spectra Questions
- Mass Stoichiometry
- Measurement and Numbers
- Metals, Nonmetals, and Metalloids
- Metric Estimations
- Metric System
- Molarity Ranking Tasks
- Mole Conversions
- Name That Element
- Names to Formulas
- Names to Formulas 2
- Nuclear Decay
- Particles, Words, and Formulas
- Periodic Trends
- Precipitation Reactions and Net Ionic Equations
- Pressure Concepts
- Pressure-Temperature Gas Law
- Pressure-Volume Gas Law
- Chemical Reaction Types
- Significant Digits and Measurement
- States Of Matter Exercise
- Stoichiometry Law Breakers
- Stoichiometry - Math Relationships
- Subatomic Particles
- Spontaneity and Driving Forces
- Gibbs Free Energy
- Volume-Temperature Gas Law
- Acid-Base Properties
- Energy and Chemical Reactions
- Chemical and Physical Properties
- Valence Shell Electron Pair Repulsion Theory
- Writing Balanced Chemical Equations
- Mission CG1
- Mission CG10
- Mission CG2
- Mission CG3
- Mission CG4
- Mission CG5
- Mission CG6
- Mission CG7
- Mission CG8
- Mission CG9
- Mission EC1
- Mission EC10
- Mission EC11
- Mission EC12
- Mission EC2
- Mission EC3
- Mission EC4
- Mission EC5
- Mission EC6
- Mission EC7
- Mission EC8
- Mission EC9
- Mission RL1
- Mission RL2
- Mission RL3
- Mission RL4
- Mission RL5
- Mission RL6
- Mission KG7
- Mission RL8
- Mission KG9
- Mission RL10
- Mission RL11
- Mission RM1
- Mission RM2
- Mission RM3
- Mission RM4
- Mission RM5
- Mission RM6
- Mission RM8
- Mission RM10
- Mission LC1
- Mission RM11
- Mission LC2
- Mission LC3
- Mission LC4
- Mission LC5
- Mission LC6
- Mission LC8
- Mission SM1
- Mission SM2
- Mission SM3
- Mission SM4
- Mission SM5
- Mission SM6
- Mission SM8
- Mission SM10
- Mission KG10
- Mission SM11
- Mission KG2
- Mission KG3
- Mission KG4
- Mission KG5
- Mission KG6
- Mission KG8
- Mission KG11
- Mission F2D1
- Mission F2D2
- Mission F2D3
- Mission F2D4
- Mission F2D5
- Mission F2D6
- Mission KC1
- Mission KC2
- Mission KC3
- Mission KC4
- Mission KC5
- Mission KC6
- Mission KC7
- Mission KC8
- Mission AAA
- Mission SM9
- Mission LC7
- Mission LC9
- Mission NL1
- Mission NL2
- Mission NL3
- Mission NL4
- Mission NL5
- Mission NL6
- Mission NL7
- Mission NL8
- Mission NL9
- Mission NL10
- Mission NL11
- Mission NL12
- Mission MC1
- Mission MC10
- Mission MC2
- Mission MC3
- Mission MC4
- Mission MC5
- Mission MC6
- Mission MC7
- Mission MC8
- Mission MC9
- Mission RM7
- Mission RM9
- Mission RL7
- Mission RL9
- Mission SM7
- Mission SE1
- Mission SE10
- Mission SE11
- Mission SE12
- Mission SE2
- Mission SE3
- Mission SE4
- Mission SE5
- Mission SE6
- Mission SE7
- Mission SE8
- Mission SE9
- Mission VP1
- Mission VP10
- Mission VP2
- Mission VP3
- Mission VP4
- Mission VP5
- Mission VP6
- Mission VP7
- Mission VP8
- Mission VP9
- Mission WM1
- Mission WM2
- Mission WM3
- Mission WM4
- Mission WM5
- Mission WM6
- Mission WM7
- Mission WM8
- Mission WE1
- Mission WE10
- Mission WE2
- Mission WE3
- Mission WE4
- Mission WE5
- Mission WE6
- Mission WE7
- Mission WE8
- Mission WE9
- Vector Walk Interactive
- Name That Motion Interactive
- Kinematic Graphing 1 Concept Checker
- Kinematic Graphing 2 Concept Checker
- Graph That Motion Interactive
- Two Stage Rocket Interactive
- Rocket Sled Concept Checker
- Force Concept Checker
- Free-Body Diagrams Concept Checker
- Free-Body Diagrams The Sequel Concept Checker
- Skydiving Concept Checker
- Elevator Ride Concept Checker
- Vector Addition Concept Checker
- Vector Walk in Two Dimensions Interactive
- Name That Vector Interactive
- River Boat Simulator Concept Checker
- Projectile Simulator 2 Concept Checker
- Projectile Simulator 3 Concept Checker
- Hit the Target Interactive
- Turd the Target 1 Interactive
- Turd the Target 2 Interactive
- Balance It Interactive
- Go For The Gold Interactive
- Egg Drop Concept Checker
- Fish Catch Concept Checker
- Exploding Carts Concept Checker
- Collision Carts - Inelastic Collisions Concept Checker
- Its All Uphill Concept Checker
- Stopping Distance Concept Checker
- Chart That Motion Interactive
- Roller Coaster Model Concept Checker
- Uniform Circular Motion Concept Checker
- Horizontal Circle Simulation Concept Checker
- Vertical Circle Simulation Concept Checker
- Race Track Concept Checker
- Gravitational Fields Concept Checker
- Orbital Motion Concept Checker
- Angular Acceleration Concept Checker
- Balance Beam Concept Checker
- Torque Balancer Concept Checker
- Aluminum Can Polarization Concept Checker
- Charging Concept Checker
- Name That Charge Simulation
- Coulomb's Law Concept Checker
- Electric Field Lines Concept Checker
- Put the Charge in the Goal Concept Checker
- Circuit Builder Concept Checker (Series Circuits)
- Circuit Builder Concept Checker (Parallel Circuits)
- Circuit Builder Concept Checker (∆V-I-R)
- Circuit Builder Concept Checker (Voltage Drop)
- Equivalent Resistance Interactive
- Pendulum Motion Simulation Concept Checker
- Mass on a Spring Simulation Concept Checker
- Particle Wave Simulation Concept Checker
- Boundary Behavior Simulation Concept Checker
- Slinky Wave Simulator Concept Checker
- Simple Wave Simulator Concept Checker
- Wave Addition Simulation Concept Checker
- Standing Wave Maker Simulation Concept Checker
- Color Addition Concept Checker
- Painting With CMY Concept Checker
- Stage Lighting Concept Checker
- Filtering Away Concept Checker
- InterferencePatterns Concept Checker
- Young's Experiment Interactive
- Plane Mirror Images Interactive
- Who Can See Who Concept Checker
- Optics Bench (Mirrors) Concept Checker
- Name That Image (Mirrors) Interactive
- Refraction Concept Checker
- Total Internal Reflection Concept Checker
- Optics Bench (Lenses) Concept Checker
- Kinematics Preview
- Velocity Time Graphs Preview
- Moving Cart on an Inclined Plane Preview
- Stopping Distance Preview
- Cart, Bricks, and Bands Preview
- Fan Cart Study Preview
- Friction Preview
- Coffee Filter Lab Preview
- Friction, Speed, and Stopping Distance Preview
- Up and Down Preview
- Projectile Range Preview
- Ballistics Preview
- Juggling Preview
- Marshmallow Launcher Preview
- Air Bag Safety Preview
- Colliding Carts Preview
- Collisions Preview
- Engineering Safer Helmets Preview
- Push the Plow Preview
- Its All Uphill Preview
- Energy on an Incline Preview
- Modeling Roller Coasters Preview
- Hot Wheels Stopping Distance Preview
- Ball Bat Collision Preview
- Energy in Fields Preview
- Weightlessness Training Preview
- Roller Coaster Loops Preview
- Universal Gravitation Preview
- Keplers Laws Preview
- Kepler's Third Law Preview
- Charge Interactions Preview
- Sticky Tape Experiments Preview
- Wire Gauge Preview
- Voltage, Current, and Resistance Preview
- Light Bulb Resistance Preview
- Series and Parallel Circuits Preview
- Thermal Equilibrium Preview
- Linear Expansion Preview
- Heating Curves Preview
- Electricity and Magnetism - Part 1 Preview
- Electricity and Magnetism - Part 2 Preview
- Vibrating Mass on a Spring Preview
- Period of a Pendulum Preview
- Wave Speed Preview
- Slinky-Experiments Preview
- Standing Waves in a Rope Preview
- Sound as a Pressure Wave Preview
- DeciBel Scale Preview
- DeciBels, Phons, and Sones Preview
- Sound of Music Preview
- Shedding Light on Light Bulbs Preview
- Models of Light Preview
- Electromagnetic Radiation Preview
- Electromagnetic Spectrum Preview
- EM Wave Communication Preview
- Digitized Data Preview
- Light Intensity Preview
- Concave Mirrors Preview
- Object Image Relations Preview
- Snells Law Preview
- Reflection vs. Transmission Preview
- Magnification Lab Preview
- Reactivity Preview
- Ions and the Periodic Table Preview
- Periodic Trends Preview
- Reaction Rates Preview
- Ammonia Factory Preview
- Stoichiometry Preview
- Gaining Teacher Access
- Tasks and Classes
- Tasks - Classic
- Subscription
- Subscription Locator
- 1-D Kinematics
- Newton's Laws
- Vectors - Motion and Forces in Two Dimensions
- Momentum and Its Conservation
- Work and Energy
- Circular Motion and Satellite Motion
- Thermal Physics
- Static Electricity
- Electric Circuits
- Vibrations and Waves
- Sound Waves and Music
- Light and Color
- Reflection and Mirrors
- About the Physics Interactives
- Task Tracker
- Usage Policy
- Newtons Laws
- Vectors and Projectiles
- Forces in 2D
- Momentum and Collisions
- Circular and Satellite Motion
- Balance and Rotation
- Electromagnetism
- Waves and Sound
- Forces in Two Dimensions
- Work, Energy, and Power
- Circular Motion and Gravitation
- Sound Waves
- 1-Dimensional Kinematics
- Circular, Satellite, and Rotational Motion
- Einstein's Theory of Special Relativity
- Waves, Sound and Light
- QuickTime Movies
- About the Concept Builders
- Pricing For Schools
- Directions for Version 2
- Measurement and Units
- Relationships and Graphs
- Rotation and Balance
- Vibrational Motion
- Reflection and Refraction
- Teacher Accounts
- Task Tracker Directions
- Kinematic Concepts
- Kinematic Graphing
- Wave Motion
- Sound and Music
- About CalcPad
- 1D Kinematics
- Vectors and Forces in 2D
- Simple Harmonic Motion
- Rotational Kinematics
- Rotation and Torque
- Rotational Dynamics
- Electric Fields, Potential, and Capacitance
- Transient RC Circuits
- Light Waves
- Units and Measurement
- Stoichiometry
- Molarity and Solutions
- Thermal Chemistry
- Acids and Bases
- Kinetics and Equilibrium
- Solution Equilibria
- Oxidation-Reduction
- Nuclear Chemistry
- NGSS Alignments
- 1D-Kinematics
- Projectiles
- Circular Motion
- Magnetism and Electromagnetism
- Graphing Practice
- About the ACT
- ACT Preparation
- For Teachers
- Other Resources
- Newton's Laws of Motion
- Work and Energy Packet
- Static Electricity Review
- Solutions Guide
- Solutions Guide Digital Download
- Motion in One Dimension
- Work, Energy and Power
- Frequently Asked Questions
- Purchasing the Download
- Purchasing the CD
- Purchasing the Digital Download
- About the NGSS Corner
- NGSS Search
- Force and Motion DCIs - High School
- Energy DCIs - High School
- Wave Applications DCIs - High School
- Force and Motion PEs - High School
- Energy PEs - High School
- Wave Applications PEs - High School
- Crosscutting Concepts
- The Practices
- Physics Topics
- NGSS Corner: Activity List
- NGSS Corner: Infographics
- About the Toolkits
- Position-Velocity-Acceleration
- Position-Time Graphs
- Velocity-Time Graphs
- Newton's First Law
- Newton's Second Law
- Newton's Third Law
- Terminal Velocity
- Projectile Motion
- Forces in 2 Dimensions
- Impulse and Momentum Change
- Momentum Conservation
- Work-Energy Fundamentals
- Work-Energy Relationship
- Roller Coaster Physics
- Satellite Motion
- Electric Fields
- Circuit Concepts
- Series Circuits
- Parallel Circuits
- Describing-Waves
- Wave Behavior Toolkit
- Standing Wave Patterns
- Resonating Air Columns
- Wave Model of Light
- Plane Mirrors
- Curved Mirrors
- Teacher Guide
- Using Lab Notebooks
- Current Electricity
- Light Waves and Color
- Reflection and Ray Model of Light
- Refraction and Ray Model of Light
- Classes (Legacy Version)
- Teacher Resources
- Subscriptions

- Newton's Laws
- Einstein's Theory of Special Relativity
- About Concept Checkers
- School Pricing
- Newton's Laws of Motion
- Newton's First Law
- Newton's Third Law
Chemistry: Acids and Bases
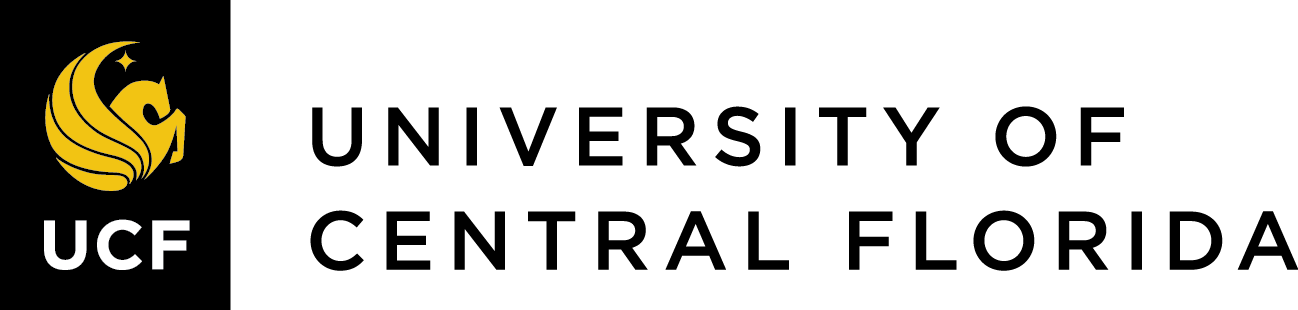
Chapter 15: Acid-Based Equilibria
Chapter 15 Practice
15.1 brønsted-lowry acids and bases [ go to section 15.1 ].
- [latex]\ce{OH–}[/latex]
- [latex]\ce{H2O}[/latex]
- [latex]\ce{HCO3-}[/latex]
- [latex]\ce{NH3}[/latex]
- [latex]\ce{HSO4-}[/latex]
- [latex]\ce{H2O2}[/latex]
- [latex]\ce{HS–}[/latex]
- [latex]\ce{H5N2+}[/latex]
- Write equations that show [latex]\ce{H2PO4^{2-}}[/latex] acting both as an acid and as a base.
- What are amphiprotic species? Illustrate with suitable equations.
- [latex]\ce{HNO3}[/latex]
- [latex]\ce{PH4-}[/latex]
- [latex]\ce{H2S}[/latex]
- [latex]\ce{CH3CH2COOH}[/latex]
- [latex]\ce{H2PO4-}[/latex]
- [latex]\ce{HPO4-}[/latex]
- [latex]\ce{Br–}[/latex]
- [latex]\ce{NH4-}[/latex]
- [latex]\ce{ASO4^{3-}}[/latex]
- [latex]\ce{PO4^{3-}}[/latex]
- [latex]\ce{NH2-}[/latex]
- [latex]\ce{C2H5OH}[/latex]
- [latex]\ce{O^{2–}}[/latex]
- [latex]\ce{H2PO4-}[/latex]
- Identify the conjugate acids of the species in the previous problem.
- [latex]\ce{NO2-} + \ce{H2O} \longrightarrow \ce{HNO2} + \ce{OH-}[/latex]
- [latex]\ce{HBr} + \ce{H2O} \longrightarrow \ce{H3O+} + \ce{Br-}[/latex]
- [latex]\ce{HS-} + \ce{H2O} \longrightarrow \ce{H2S} + \ce{OH-}[/latex]
- [latex]\ce{H2PO4-} + \ce{OH-} \longrightarrow \ce{HPO4^{2-}} + \ce{H2O}[/latex]
- [latex]\ce{H2PO4-} + \ce{HCl} \longrightarrow \ce{H3PO4} + \ce{Cl-}[/latex]
- [latex]\ce{[Fe(H2O)5(OH)]^{2+}} + \ce{[Al(H2O)6]^{3+}} \longrightarrow \ce{[Fe(H2O)6]^{3+}} + \ce{[Al(H2O)5(OH)]^{2+}}[/latex]
- [latex]\ce{CH3OH} + \ce{H-} \longrightarrow \ce{CH3O-} + \ce{H2}[/latex]
- [latex]\ce{HSO3-}[/latex]
- [latex]\ce{HC2O4-}[/latex]
- Is the self ionization of water endothermic or exothermic? The ionization constant for water ( K w ) is 2.9 × 10 –14 at 40 °C and 9.3 × 10 –14 at 60 °C.
- [latex]\ce{H2O}, \hspace{0.25cm} \ce{O^{2–}}[/latex]
- [latex]\ce{H3O+}, \hspace{0.25cm} \ce{OH–}[/latex]
- [latex]\ce{H2CO3}, \hspace{0.25cm} \ce{CO3^{2-}}[/latex]
- [latex]\ce{NH4+}, \hspace{0.25cm} \ce{NH2-}[/latex]
- [latex]\ce{H2SO4}, \hspace{0.25cm} \ce{SO4^{2-}}[/latex]
- [latex]\ce{H3O2+}, \hspace{0.25cm} \ce{HO2-}[/latex]
- [latex]\ce{H2S}, \hspace{0.25cm} \ce{S^{2–}}[/latex]
- [latex]\ce{H6N2^{2+}}, \hspace{0.25cm} \ce{H4N2}[/latex]
- Amphiprotic species may either gain or lose a proton in a chemical reaction, thus acting as a base or an acid. An example is [latex]\ce{H2O}[/latex]. As an acid: [latex]\ce{H2O}(aq) + \ce{NH3}(aq) \longrightarrow \ce{NH4+}(aq) + \ce{OH-}(aq)[/latex]. As a base: [latex]\ce{H2O}(aq) + \ce{HCl}(aq) \longrightarrow \ce{H3O+}(aq) + \ce{Cl-}(aq)[/latex]
- [latex]\ce{NH3} + \ce{H3O+} \longrightarrow \ce{NH4OH} + \ce{H2O}, \hspace{2cm} \ce{NH3} + \ce{OCH3-} \longrightarrow \ce{NH2-} + \ce{CH3OH}[/latex]
- [latex]\ce{HPO4^{2-}} + \ce{OH-} \longrightarrow \ce{PO4^{3-}} + \ce{H2O}, \hspace{2cm} \ce{HPO4^{2-}} + \ce{HClO4} \longrightarrow \ce{H2PO4-} + \ce{ClO4-}[/latex] Not Amphiprotic:
- [latex]\ce{Br-}[/latex]
- [latex]\ce{NH4-}[/latex]
- [latex]\ce{AsO4^{3-}}[/latex]
- [latex]\ce{HPO4^{3-}}[/latex]
- [latex]\ce{C2H5OH2+}[/latex]
- [latex]\ce{OH-}[/latex]
- [latex]\ce{H3PO4}[/latex]
- [latex]\ce{H2O} + \ce{H2O} \leftrightarrows \ce{H3O+} + \ce{OH-}[/latex]
- [latex]\ce{HSO3-} + \ce{H2O} \leftrightarrows \ce{H2SO4-} + \ce{OH-}[/latex]; [latex]\ce{HSO3-} + \ce{H2O} \leftrightarrows \ce{SO3^{2-}} + \ce{H3O+}[/latex]
- [latex]\ce{HC2O4-} + \ce{H2O} \leftrightarrows \ce{H2C2O4} + \ce{OH-}[/latex]; [latex]\ce{HC2O4} + \ce{H2O} \leftrightarrows \ce{C2O4^{2-}} + \ce{H3O+}[/latex]
15.2 pH and pOH [ Go to section 15.2 ]
- What are the hydronium and hydroxide ion concentrations in a solution whose pH is 6.52?
- The ionization constant for water ( K w ) is 2.9 × 10 –14 at 40 °C. Calculate , [latex]\ce{[OH–]}[/latex], pH, and pOH for pure water at 40 °C.
- Calculate the hydronium ion concentration and the hydroxide ion concentration in lime juice from its pH. See Figure 15.2.1 for useful information.
- 0.200 M [latex]\ce{HCl}[/latex]
- 0.0143 M [latex]\ce{NaOH}[/latex]
- 3.0 M [latex]\ce{HNO3}[/latex]
- 0.0031 M [latex]\ce{Ca(OH)2}[/latex]
- [latex]\ce{HBr}[/latex], pH = 2.50
- [latex]\ce{KOH}[/latex], pH = 8.55
- [latex]\ce{HClO4}[/latex], pH = 1.02
- [latex]\ce{LiOH}[/latex], pH = 11.00
- Calculate the hydrogen ion concentration and the hydroxide ion concentration in wine from its pH. See Figure 15.2.1 for useful information.
- Calculate the hydroxide ion concentration for the solutions in problem number 15.
- [latex]\ce{[H3O+]}[/latex] = 3.0 × 10 –7 M ; pOH = 7.48; [latex]\ce{[OH–]}[/latex] = 3.3 × 10 –8 M
- [latex]\ce{[H3O+]}[/latex] = 1 × 10 –2 M ; [latex]\ce{[OH–]}[/latex] = 1 × 10 –12 M
- [latex]\ce{[H3O+]}[/latex] = 3.16 × 10 -3 M
- [latex]\ce{[H3O+]}[/latex] = 2.82 × 10 -9 M
- [latex]\ce{[H3O+]}[/latex] = 0.0955 M
- [latex]\ce{[H3O+]}[/latex] = 1 × 10 -11 M
- [latex]\ce{[OH-]}[/latex] = 3.16 × 10 -12 M
- [latex]\ce{[OH-]}[/latex] = 3.54 × 10 -6 M
- [latex]\ce{[OH-]}[/latex] = 1.05 × 10 -13 M
- [latex]\ce{[OH-]}[/latex] = 1 × 10 -3 M
15.3 Relative Strengths of Acids and Bases [ Go to section 15.3 ]
- Explain why the neutralization reaction of a strong acid and a weak base gives a weakly acidic solution.
- Identify the strong Brønsted-Lowry acids and strong Brønsted-Lowry bases.
- List those compounds in (a) that can behave as Brønsted-Lowry acids with strengths lying between those of [latex]\ce{H3O+}[/latex] and [latex]\ce{H2O}[/latex].
- List those compounds in (a) that can behave as Brønsted-Lowry bases with strengths lying between those of [latex]\ce{H2O}[/latex] and [latex]\ce{OH}[/latex].
- What is the ionization constant at 25 °C for the weak acid [latex]\ce{(CH3)2NH2+}[/latex], the conjugate acid of the weak base [latex]\ce{(CH3)2NH}[/latex], K b = 5.9 × 10 –4 ?
- acids – [latex]\ce{HCl, HNO3, H2SO4}[/latex] bases – [latex]\ce{Ca(OH)2, NaOH}[/latex]
- [latex]\ce{H3PO4, HF, HNO2, CH3CO2H, H2CO3}[/latex]
- [latex]\ce{Na2CO3}[/latex], [latex]\ce{NH3}[/latex]
15.4 Hydrolysis of Salts [ Go to section 15.4 ]
- [latex]\ce{Al(NO3)3}[/latex]
- [latex]\ce{RbI}[/latex]
- [latex]\ce{KHCO2}[/latex]
- [latex]\ce{CH3NH3Br}[/latex]
- [latex]\ce{Al(NO3)3}[/latex] dissociates into [latex]\ce{Al3+}[/latex] ions (acidic metal cation) and [latex]\ce{NO3-}[/latex] ions (the conjugate base of a strong acid and therefore essentially neutral). The aqueous solution is therefore acidic.
- [latex]\ce{RbI}[/latex] dissociates into [latex]\ce{Rb+}[/latex] ions (neutral metal cation) and [latex]\ce{I–}[/latex] ions (the conjugate base of a strong acid and therefore essentially neutral). The aqueous solution is therefore neutral.
15.5 Polyprotic Acids [ Go to section 15.5 ]
- Calculate the concentration of each species present in a 0.050 M solution of [latex]\ce{H2S}[/latex].
- Which of the following species will contribute most significantly to the hydronium ion concentration in a solution of [latex]\ce{H3PO4}[/latex]? [latex]\ce{H3PO4, H2PO4-, HPO42-, PO43-}[/latex]
- [latex]\ce{[H2S]}[/latex] = 0.050 M [latex]\ce{[HS–]}[/latex] = 6.7 × 10 –5 M [latex]\ce{[H3O+]}[/latex]= 6.7 × 10 –5 M [latex]\ce{[S2–]}[/latex] = 1 × 10 –19 M [latex]\ce{[OH–]}[/latex] = 1.5 × 10 –10 M
15.6 Buffers [ Go to section 15.6 ]
- Explain why a buffer can be prepared from a mixture of [latex]\ce{NH4Cl}[/latex] and [latex]\ce{NaOH}[/latex] but not from [latex]\ce{NH3}[/latex] and [latex]\ce{NaOH}[/latex].
- [atex]\ce{OH–}[/latex] is a base, and [latex]\ce{NH4+}[/latex] is a weak acid. They react with one another to form [latex]\ce{NH3}[/latex], thereby setting up the equilibrium [latex]\ce{NH4+}(aq) + \ce{OH-}(aq) \longleftrightarrow \ce{NH3}(aq) + \ce{H2O}(l)[/latex]. Because both the base ([latex]\ce{NH3}[/latex]) and the conjugate acid ([latex]\ce{NH4+}[/latex]) are present, a buffer is formed. However, in the second case, NH3 and OH– are both bases, so no buffer is possible.
15.7 Acid-Base Titrations [ Go to section 15.7 ]
Chemistry Fundamentals Copyright © by Dr. Julie Donnelly, Dr. Nicole Lapeyrouse, and Dr. Matthew Rex is licensed under a Creative Commons Attribution-NonCommercial-ShareAlike 4.0 International License , except where otherwise noted.
Share This Book
Free Printable acids and bases Worksheets for 11th Grade
Science: Discover the fascinating world of acids and bases with our free printable worksheets for Grade 11 students. Enhance learning and explore chemical reactions in a fun and interactive way.
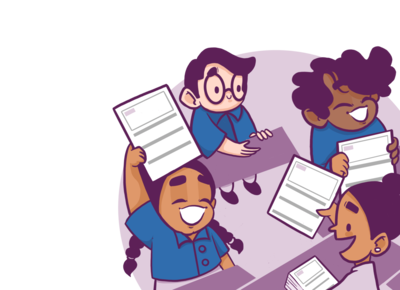
11th - 12th
10th - 11th
Jelajahi acids and bases Lembar Kerja berdasarkan Nilai
Jelajahi lembar kerja mata pelajaran lainnya untuk grade 11.
- social studies
Explore printable acids and bases worksheets for 11th Grade
Acids and bases worksheets for Grade 11 are essential resources for science teachers who aim to provide their students with a comprehensive understanding of the fundamental concepts in chemistry. These worksheets cover a wide range of topics, including the properties of acids and bases, pH scale, neutralization reactions, and titration. Designed specifically for Grade 11 students, these worksheets are tailored to meet the unique learning needs of this age group, ensuring that the content is both engaging and challenging. By incorporating these worksheets into their lesson plans, teachers can effectively reinforce key concepts and promote a deeper understanding of the subject matter.
Quizizz offers an excellent platform for teachers to access a variety of acids and bases worksheets for Grade 11, as well as other resources to supplement their science and chemistry curriculum. In addition to the worksheets, Quizizz provides interactive quizzes, engaging games, and other learning tools that can be easily integrated into the classroom setting. These resources are designed to enhance students' learning experience and help them master the complex concepts related to acids and bases. By utilizing Quizizz, teachers can not only save time in preparing their lessons but also ensure that their students are receiving a well-rounded education in science and chemistry, specifically tailored for Grade 11.
If you're seeing this message, it means we're having trouble loading external resources on our website.
If you're behind a web filter, please make sure that the domains *.kastatic.org and *.kasandbox.org are unblocked.
To log in and use all the features of Khan Academy, please enable JavaScript in your browser.
UP Class 7th Science
Course: up class 7th science > unit 3.
- Litmus paper as an Indicator
Acids, Bases and Salts
- (Choice A) Salt A Salt
- (Choice B) Salt + Oxygen B Salt + Oxygen
- (Choice C) Water C Water
- (Choice D) Salt + Water D Salt + Water
- Chemistry Worksheets
- Class 10 Chemistry Worksheets
- Class 10 Chemistry Worksheet on Chapter 2 Acids, Bases and Salts Set 1
Chemistry Worksheets Class 10 on Chapter 2 Acids, Bases and Salts with Answers - Set 1
Acids are substances that taste sour and are corrosive in nature. It turns blue litmus paper to red. These substances are chemically acidic in nature.E.g.:-orange juice, curd, vinegar, hydrochloric acid etc.
Bases are substances that, in an aqueous solution, are slippery to the touch and bitter in taste. It turns red litmus paper to blue. These substances are chemically basic in nature. Eg:- soap, ammonium hydroxide, calcium hydroxide, etc.
Salt is an ionic compound that results from the neutralization reaction of acids and bases. Salts are constituted of positively charged ions, known as cations, and negatively charged ions, known as anions, which can either be organic or inorganic in nature. These ions are present in a relative amount, thus rendering the nature of the salt neutral.
Download PDF of Class 10 Chemistry Worksheet Chapter 2 Acids, Bases and Salts – Set 1
Download PDF
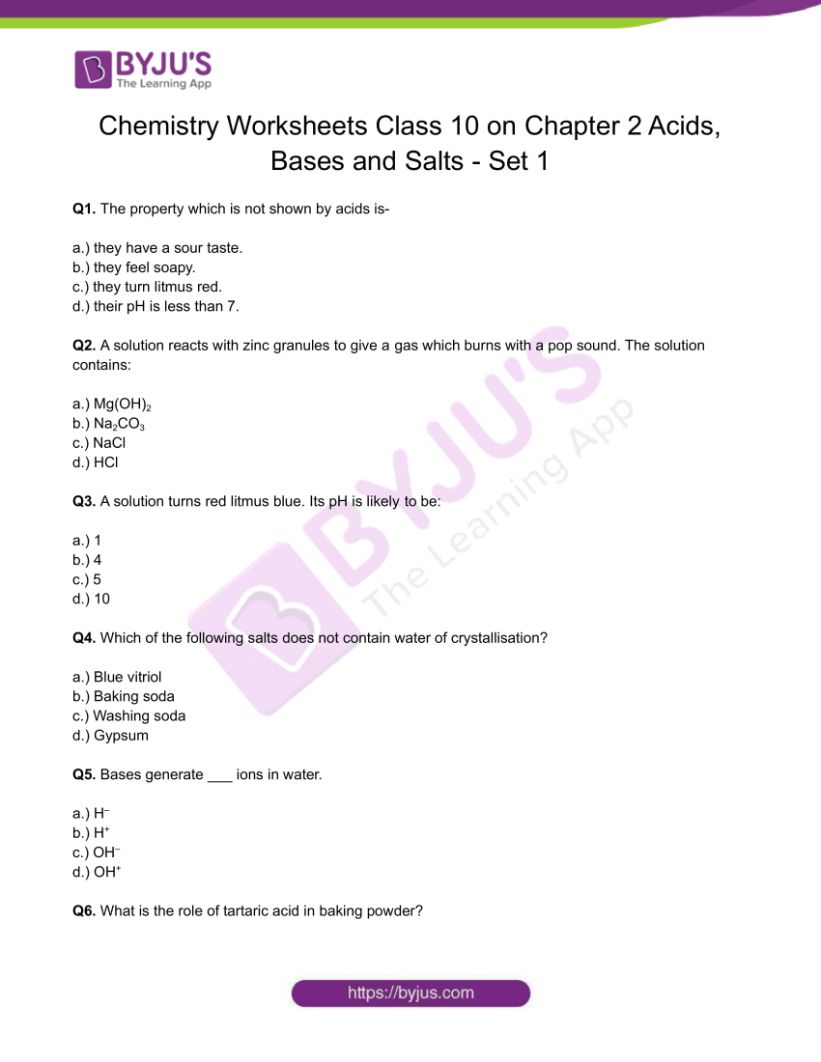
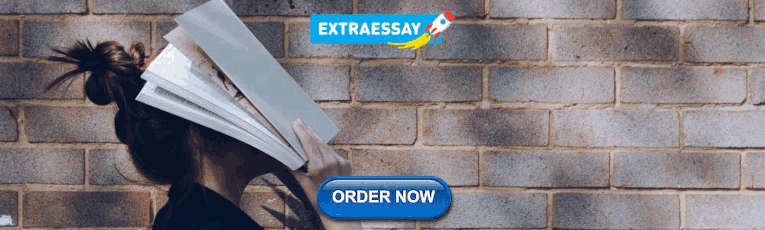
CBSE Class 10 Chemistry Worksheet Chapter 2 Acids, Bases and Salts – Set 1
Q1. The property which is not shown by acids is-
a.) they have a sour taste.
b.) they feel soapy.
c.) they turn litmus red.
d.) their pH is less than 7.
Q2. A solution reacts with zinc granules to give a gas which burns with a pop sound. The solution contains:
a.) Mg(OH) 2
b.) Na 2 CO 3
Q3. A solution turns red litmus blue. Its pH is likely to be:
Q4. Which of the following salts does not contain water of crystallisation?
a.) Blue vitriol
b.) Baking soda
c.) Washing soda
Q5. Bases generate ___ ions in water.
Q6. What is the role of tartaric acid in baking powder?
Q7. What is the chemical formula of blue vitriol?
Q8. What is the role of HCl present in the stomach?
Q9. What is the effect of dilution on an acid or base?
Q10. Explain why bases are not kept in metal containers?
Q11. State the chemical property in each case on which the following uses of baking soda are based:
i.) As an antacid
ii.) As a constituent of baking powder.
Q12. What is the water of crystallisation?
Q13. Define olfactory indicators.
Q14. While diluting an acid why is it recommended that the acid should be added to water and not water to the acid?
Q15. Sea water contains many salts dissolved in it. How can the salt that we use in the food is obtained?
a.) What happens when zinc granules are heated with sodium hydroxide solution?
b.) What does pH stand for? What does it indicate?
Q17. a.) What is gypsum? What happens when gypsum is heated at 100℃?
b.) What is the common name of the compound CaOCl 2 ?
c.) Explain why chlorine is used for sterilising the drinking water supply?
Q18. a.) What is baking powder? How does it make the cake soft and spongy?
b.) In addition to sodium hydrogen carbonate, baking powder contains a substance X. Name the substance X. What is the role of substance X in the baking powder?
c.) State the two uses of sodium hydroxide.
Q19. a.) A knife, which is used to cut fruit, was immediately dipped into water containing drops of blue litmus solution. If the colour of the solution is changed to red, what inference can be drawn about the nature of the fruit and why?
b.) What should be done if someone accidentally touches the leaves of a nettle plant in the wild?
c.) Two solutions X and Y have pH = 4 and pH = 8, respectively. Which solution will give an alkaline reaction and which one acidic?
Q20. Answer the following-
a.) Compounds such as alcohol and glucose also contain hydrogen but are not categorised as acids. Why?
b.) Why do acids not show acidic behaviour in the absence of water?
c.) Why should curd and other sour foodstuffs not be kept in the metal container?
d.) Name three common indicators.
Download PDF to access answers of the Chemistry Worksheet for Class 10 Chemistry Chapter 2: Acids, Bases and Salts Set – 1 Download PDF
- Acids, Bases, and Salts
- Properties of Acids and Bases
- Acids and Bases Questions
- Class 10 Chemistry Chapter 2 – Acids, Bases and Salts Important Questions with Answers
- Class 10 Chemistry Chapter 2 Acids, Bases and Salts MCQs
Recommended Videos
Properties of acids and bases class 10.
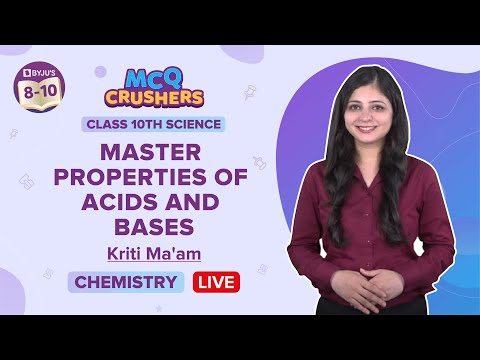
- Share Share
Register with BYJU'S & Download Free PDFs
Register with byju's & watch live videos.

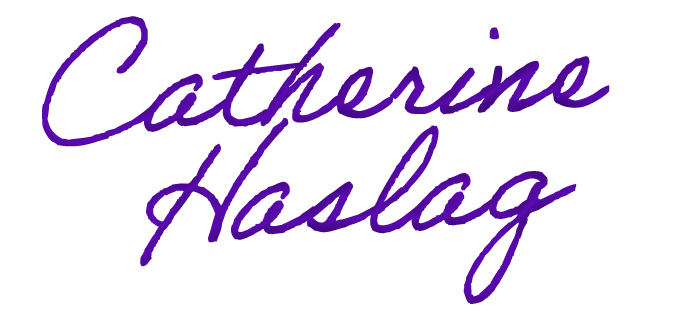
Acid Rain Assignment
- Identify the causes of acid rain.
- Explain how acid rain is formed.
- Explain how acid rain impacts the environment.
- Identify ways to prevent the formation of acid rain.
- Explain sources of human impact on the environment from chemical disposal/production.
- Balance a chemical equation.
- Identify types of chemical reactions.
- Predict the products of chemical reactions.
- Calculate pH from concentration and vice versa.
- Calculate concentration using percent by mass and by volume.
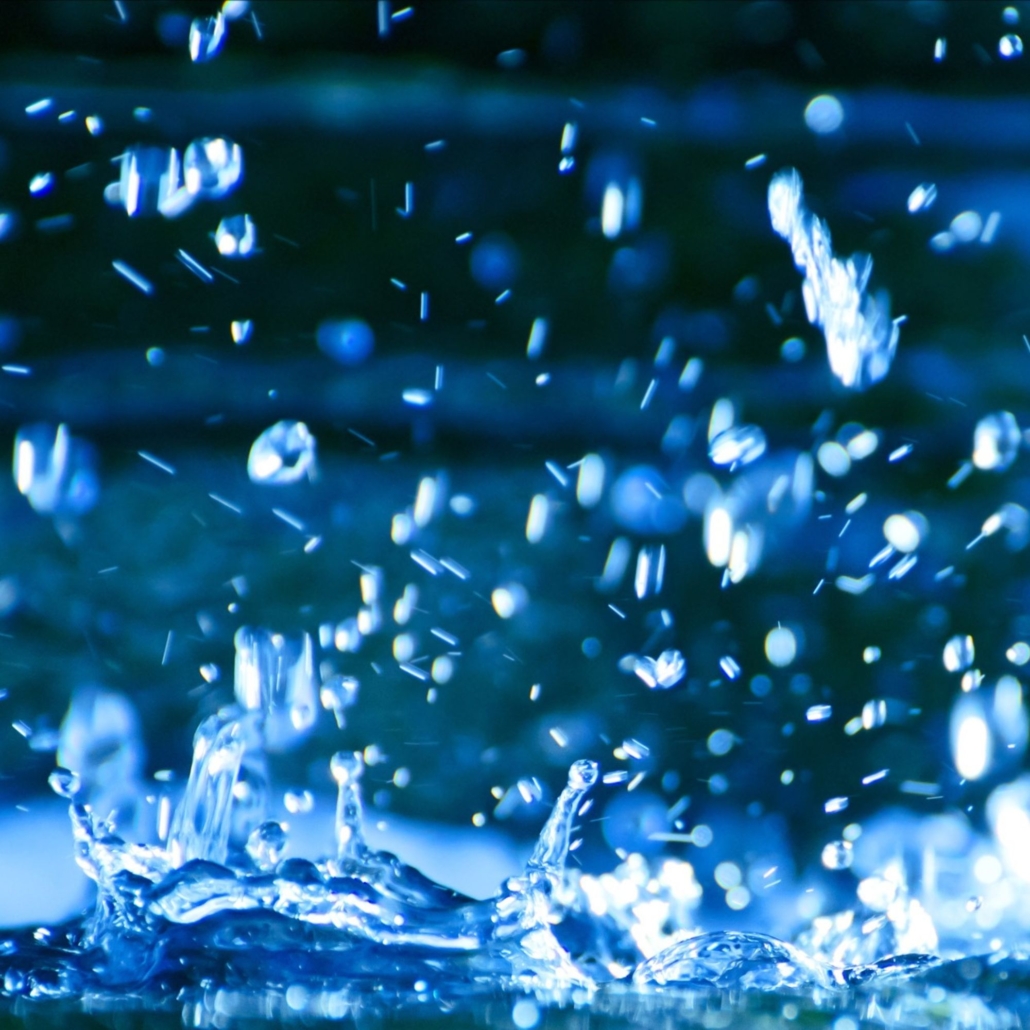
Related Textbook
Please read section 17.9 of your textbook before completing this assignment. The information provided here will help you complete this assignment.
Introduction
Acid rain is caused by burning fossil fuels containing nitrogen and sulfur. When burned, fossil fuels release nitrogen and sulfur, which react with oxygen to form sulfur oxides (such as sulfur dioxide and sulfur trioxide) and nitrogen oxides (such as nitrogen monoxide and nitrogen dioxide). These chemicals then react with the water in the air to produce sulfurous acid, sulfuric acid, nitrous acid, and nitric acid. The presence of these acids in rainwater lowers the pH, making the rain more acidic.
According to the U.S. Energy Information Administration website, petroleum, natural gas, and coal, all of which are fossil fuels, have provided more than 80% of the total US energy consumption for the past century. In 2015, 81.5% of US energy consumption was met by the use of fossil fuels.
The resources below explain in more detail how acid rain is formed, the impacts of acid rain, and how to prevent the formation of acid rain. Review these resources to learn more about acid rain.
Environmental Protection Agency Information on Acid Rain:
- What is acid rain: https://www.epa.gov/acidrain/what-acid-rain
- Effects of Acid Rain: https://www.epa.gov/acidrain/effects-acid-rain
Acid Rain by Fuse School
Reducing acid rain and it’s effects by fuse school.
Answer the following questions using the information provided in the assignment introduction, your textbook, and the concepts we have learned in this class. Your assignment must be handwritten. Typed submissions will not be accepted and will earn a zero. You must show all of your work on the calculations to earn credit. Upload your completed assignment to the “Acid Rain assignment folder” on Brightspace. This assignment is worth 25 points.
- Explain how fossil fuels, which are made of carbon and hydrogen, contain sulfur compounds. (2 points)
- Explain how nitrogen is released into the air to form nitric acid and nitrous acid, causing acid rain. (2 points)
- What are 3 specific ways acid rain impacts the environment? (3 points)
- What are 3 specific ways to reduce the amount of acid rain produced? Be sure to address both the production of sulfur oxides and nitrogen oxides in your answer. (3 points)
- Acid rain is formed when sulfur dioxide or nitrogen dioxide is released into the air from burning fossil fuels and then reacts with the water in the air. Balance the equations for the formation of sulfur dioxide and nitric acid, illustrated below: (2 points each)
Reaction 1: FeS 2(s) + O 2(g) → Fe 2 O 3(s) + SO 2(g)
Reaction 2: NO (g) + O 2(g) → NO 2(g)
- What type of chemical reaction (single replacement, double replacement, combination, decomposition, combustion) is illustrated in Reaction 2 above? (1 point)
- Calcium oxide or sodium carbonate can be used to scrub the sulfur dioxide from emissions from power plants. One of the videos illustrated a reaction between calcium oxide and sulfur dioxide to produce calcium sulfite. When sulfur dioxide reacts with sodium carbonate, sodium sulfite and carbon dioxide gas are produced. Using this information, write the balanced chemical equation for the reaction of sulfur dioxide and sodium carbonate. (4 points)
- The pH of a sample of rainwater water is 5.6. What is the hydronium concentration in this sample of rainwater? (0.5 point)
- The pH of a sample of acid rain is 4.3. What is the hydronium concentration of this sample of acid rain? (0.5 point)
- According to data obtained from Statista.com, the United States burned 587 tons (532.5 metric tons) of coal in 2019. If coal contains 3.5% sulfur, how many grams of sulfur are released by coal burning in 2019 in the US? Write your answer in scientific notation. You must show your work to receive credit for this problem. (1 metric ton = 1,000,000 grams) (5 points)
“Acid Rain.” YouTube , Fuse School, 10 Aug. 2014, www.youtube.com/watch?v=Nf8cuvl62Vc .
“Reducing Acid Rain Or Its Effect.” YouTube , Fuse School, 10 Aug. 2014, www.youtube.com/watch?v=VILCk2CpUCw .
“Effects of Acid Rain.” EPA , Environmental Protection Agency, 4 May 2020, www.epa.gov/acidrain/effects-acid-rain .
Sönnichsen, Published by N., and Jul 14. “Coal Consumption United States 2006-2019.” Statista , 14 July 2020, www.statista.com/statistics/243934/coal-consumption-in-the-united-states/ .
“U.S. Energy Information Administration – EIA – Independent Statistics and Analysis.” Fossil Fuels Still Dominate U.S. Energy Consumption despite Recent Market Share Decline – Today in Energy – U.S. Energy Information Administration (EIA) , 1 July 2016, www.eia.gov/todayinenergy/detail.php?id=26912 .
“What Is Acid Rain?” EPA , Environmental Protection Agency, 12 May 2020, www.epa.gov/acidrain/what-acid-rain .
This page was created on February 15, 2023, and was last updated on August 4, 2023.
©2023 Catherine Haslag. All Rights Reserved.

- school Campus Bookshelves
- menu_book Bookshelves
- perm_media Learning Objects
- login Login
- how_to_reg Request Instructor Account
- hub Instructor Commons
- Download Page (PDF)
- Download Full Book (PDF)
- Periodic Table
- Physics Constants
- Scientific Calculator
- Reference & Cite
- Tools expand_more
- Readability
selected template will load here
This action is not available.
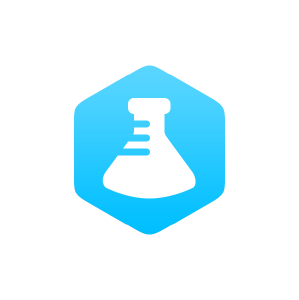
5: Acids and Bases
- Last updated
- Save as PDF
- Page ID 322486
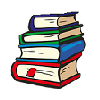
This chapter will illustrate the chemistry of acid-base reactions and equilibria, and provide you with tools for quantifying the concentrations of acids and bases in solutions.
- 5.1: Brønsted-Lowry Acids and Bases Compounds that donate a proton (a hydrogen ion) to another compound is called a Brønsted-Lowry acid. The compound that accepts the proton is called a Brønsted-Lowry base. The species remaining after a Brønsted-Lowry acid has lost a proton is the conjugate base of the acid. The species formed when a Brønsted-Lowry base gains a proton is the conjugate acid of the base. Amphiprotic species can act as both proton donors and proton acceptors. Water is the most important amphiprotic species.
- 5.2: pH and pOH The concentration of hydronium ion in a solution of an acid in water is greater than 1.0×10⁻⁷M at 25 °C. The concentration of hydroxide ion in a solution of a base in water is greater than 1.0×10⁻⁷M M at 25 °C. The concentration of H₃O⁺ in a solution can be expressed as the pH of the solution; pH=−log H₃O⁺. The concentration of OH⁻ can be expressed as the pOH of the solution: pOH=−log[OH⁻].
- 5.3: Relative Strengths of Acids and Bases The strengths of Brønsted-Lowry acids and bases in aqueous solutions can be determined by their acid or base ionization constants. Stronger acids form weaker conjugate bases, and weaker acids form stronger conjugate bases. Thus strong acids are completely ionized in aqueous solution because their conjugate bases are weaker bases than water. Weak acids are only partially ionized because their conjugate bases are compete successfully with water for possession of protons.
- 5.4: Hydrolysis of Salt Solutions The characteristic properties of aqueous solutions of Brønsted-Lowry acids are due to the presence of hydronium ions; those of aqueous solutions of Brønsted-Lowry bases are due to the presence of hydroxide ions. The neutralization that occurs when aqueous solutions of acids and bases are combined results from the reaction of the hydronium and hydroxide ions to form water. Some salts formed in neutralization reactions may make the product solutions slightly acidic or slightly basic.
- 5.5: Polyprotic Acids An acid that contains more than one ionizable proton is a polyprotic acid. The protons of these acids ionize in steps. The differences in the acid ionization constants for the successive ionizations of the protons in a polyprotic acid usually vary by roughly five orders of magnitude. As long as the difference between the successive values of Ka of the acid is greater than about a factor of 20, it is appropriate to break down the calculations of the concentrations sequentially.
- 5.6: Lewis Acids and Bases A Lewis acid is a species that can accept an electron pair, whereas a Lewis base has an electron pair available for donation to a Lewis acid. Complex ions are examples of Lewis acid-base adducts. In a complex ion, we have a central atom, often consisting of a transition metal cation, which acts as a Lewis acid, and several neutral molecules or ions surrounding them called ligands that act as Lewis bases. Complex ions form by sharing electron pairs to form coordinate covalent bonds.
Contributors and Attributions
Paul Flowers (University of North Carolina - Pembroke), Klaus Theopold (University of Delaware) and Richard Langley (Stephen F. Austin State University) with contributing authors. Textbook content produced by OpenStax College is licensed under a Creative Commons Attribution License 4.0 license. Download for free at http://cnx.org/contents/[email protected] ).
Thank you for visiting nature.com. You are using a browser version with limited support for CSS. To obtain the best experience, we recommend you use a more up to date browser (or turn off compatibility mode in Internet Explorer). In the meantime, to ensure continued support, we are displaying the site without styles and JavaScript.
- View all journals
- My Account Login
- Explore content
- About the journal
- Publish with us
- Sign up for alerts
- Open access
- Published: 18 October 2022
Rapid protein assignments and structures from raw NMR spectra with the deep learning technique ARTINA
- Piotr Klukowski ORCID: orcid.org/0000-0003-1045-3487 1 ,
- Roland Riek ORCID: orcid.org/0000-0002-6333-066X 1 &
- Peter Güntert ORCID: orcid.org/0000-0002-2911-7574 1 , 2 , 3
Nature Communications volume 13 , Article number: 6151 ( 2022 ) Cite this article
11k Accesses
25 Citations
30 Altmetric
Metrics details
- Machine learning
- Solution-state NMR
Nuclear Magnetic Resonance (NMR) spectroscopy is a major technique in structural biology with over 11,800 protein structures deposited in the Protein Data Bank. NMR can elucidate structures and dynamics of small and medium size proteins in solution, living cells, and solids, but has been limited by the tedious data analysis process. It typically requires weeks or months of manual work of a trained expert to turn NMR measurements into a protein structure. Automation of this process is an open problem, formulated in the field over 30 years ago. We present a solution to this challenge that enables the completely automated analysis of protein NMR data within hours after completing the measurements. Using only NMR spectra and the protein sequence as input, our machine learning-based method, ARTINA, delivers signal positions, resonance assignments, and structures strictly without human intervention. Tested on a 100-protein benchmark comprising 1329 multidimensional NMR spectra, ARTINA demonstrated its ability to solve structures with 1.44 Å median RMSD to the PDB reference and to identify 91.36% correct NMR resonance assignments. ARTINA can be used by non-experts, reducing the effort for a protein assignment or structure determination by NMR essentially to the preparation of the sample and the spectra measurements.
Similar content being viewed by others
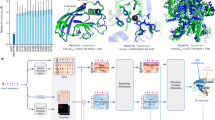
Highly accurate protein structure prediction with AlphaFold
John Jumper, Richard Evans, … Demis Hassabis
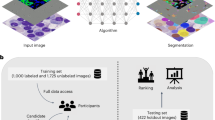
The multimodality cell segmentation challenge: toward universal solutions
Jun Ma, Ronald Xie, … Bo Wang
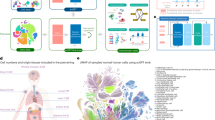
scGPT: toward building a foundation model for single-cell multi-omics using generative AI
Haotian Cui, Chloe Wang, … Bo Wang
Introduction
Studying structures of proteins and ligand-protein complexes is one of the most influential endeavors in molecular biology and rational drug design. All key structure determination techniques, X-ray crystallography, electron microscopy, and NMR spectroscopy, have led to remarkable discoveries, but suffer from their respective experimental limitations. NMR can elucidate structures and dynamics of small and medium size proteins in solution 1 and even in living cells 2 . However, the analysis of NMR spectra and the resonance assignment, which are indispensable for NMR studies, remain time-consuming even for a skilled and experienced spectroscopist. Attributed to this, the percentage of NMR protein structures in the Protein Data Bank (PDB) has decreased from a maximum of 14.6% in 2007 to 7.3% in 2021 ( https://www.rcsb.org/stats ). The problem has sparked research towards automating different tasks in NMR structure determination 3 , 4 , including peak picking 5 , 6 , 7 , 8 , 9 , resonance assignment 10 , 11 , 12 , and the identification of distance restraints 13 , 14 . Several of these methods are available as webservers 15 , 16 . This enabled semi-automatic 17 , 18 but not yet unsupervised automation of the entire NMR structure determination process, except for a very small number of favorable proteins 7 , 19 .
The advance of machine learning techniques 20 now offers unprecedented possibilities for reliably replacing decisions of human experts by efficient computational tools. Here, we present a method that achieves this goal for NMR assignment and structure determination. We show for a diverse set of 100 proteins that NMR resonance assignments and protein structures can be determined within hours after completing the NMR measurements. Our method, Art ificial I ntelligence for N MR A pplications, ARTINA (Fig. 1 ), combines machine learning for tasks that are difficult to model otherwise with existing algorithms—evolutionary optimization for resonance assignment with FLYA 12 , chemical shift database searches for torsion angle restraint generation with TALOS-N 21 , ambiguous distance restraints, network-anchoring and constraint combination for NOESY assignment 14 , 22 and simulated annealing by torsion angle dynamics for structure calculation with CYANA 23 . Machine learning is used in multiple flavors—deep residual neural networks 24 for visual spectrum analysis to identify peak positions (pp-ResNet) and to deconvolve overlapping signals (deconv-ResNet) in 25 different types of spectra (Supplementary Table 1 ), kernel density estimation (KDE) to reconstruct original peak positions in folded spectra, a deep graph neural network 25 , 26 (GNN) for chemical shift estimation within the refinement of chemical shift assignments, and a gradient boosted trees 27 (GBT) model for the selection of structure proposals.
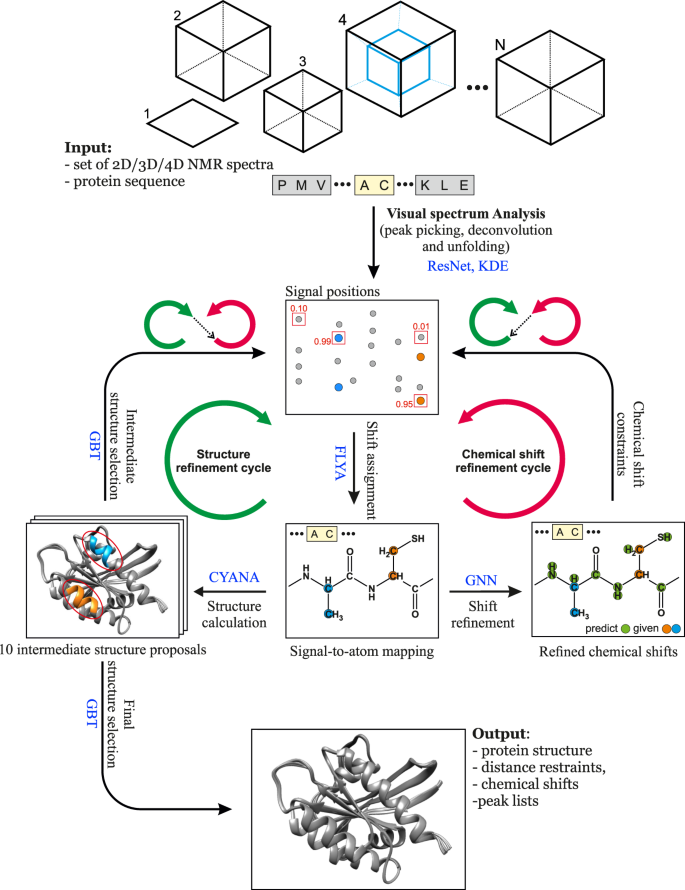
The flowchart presents the interplay between the main components of the automated protein structure determination workflow: Residual Neural Network (ResNet), FLYA automated chemical shift assignment, Graph Neural Network (GNN), Gradient Boosted Trees (GBT), and CYANA structure calculation.
A major challenge in developing ARTINA was the collection and preparation of a large training data set that is required for machine learning, because, in contrast to assignments and structures, NMR spectra are generally not archived in public data repositories. Instead, we were obliged to collect from different sources and standardize complete sets of multidimensional NMR spectra for the assignment and structure determination of 100 proteins.
In the following work, we describe the algorithm, training and test data, and results of ARTINA automated structure determination, which are on par with those achieved in weeks or months of human experts’ labor.
Benchmark dataset
One of the major obstacles for developing deep learning solutions for protein NMR spectroscopy is the lack of a large-scale standardized benchmark dataset of protein NMR spectra. To date, published manuscripts presenting the most notable methods for computational NMR, typically refer to less than 50 2D/3D/4D NMR spectra in their experimental sections. Even the well-recognized CASD-NMR competition cannot serve as a major source of training data for deep learning, since only the NOESY spectra of 10 proteins were used in the last round of the event 28 .
To make our study possible, we established a standardized benchmark of 1329 2D/3D/4D NMR spectra, which allows 100 proteins to be recalculated using their original spectral data (Fig. 2 and Supplementary Table 2 ). Each protein record in our dataset contains 5–20 spectra together with manually identified chemical shifts (usually depositions at the Biological Magnetic Resonance Data Bank, BMRB) and the previously determined (“ground truth”) protein structure (PDB record; Supplementary Table 3 ). The benchmark covers protein sizes typically studied by NMR spectroscopy with sequence lengths between 35 and 175 residues (molecular mass 4–20 kDa).
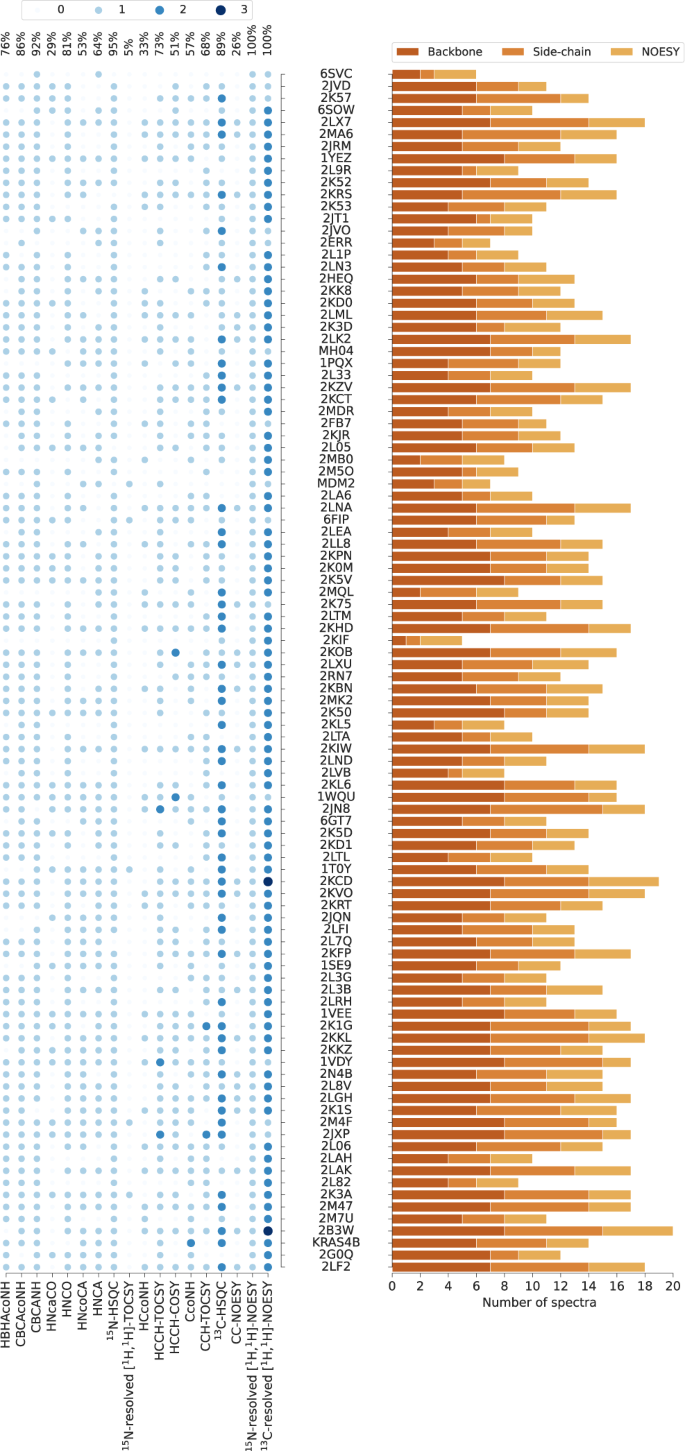
PDB codes (or names, MH04, MDM2, KRAS4B, if PDB code unavailable) of the 100 benchmark proteins are ordered by the number of residues. The histogram shows the number of spectra for backbone assignment, side-chain assignment, and NOE measurement. Spectrum types in each data set are shown by light to dark blue circles indicating the number of individual spectra of the given type. The percentages of benchmark records that contain a given spectrum type are given at the top. Spectrum types present in less than 5% of the data sets have been omitted.
Automated protein structure determination
The accuracy of protein structure determination with ARTINA was evaluated in a 5-fold cross-validation experiment with the aforementioned benchmark dataset. Five instances of pp-ResNet and GBT were trained, each one using data from about 80% of the proteins for training and the remaining ones for testing. Since each protein was present exactly once in the test set, reported quality metrics were obtained directly in the cross-validation experiment, and no averaging between data splits was required. To deploy pp-ResNet and GBT models in our online system, we constructed an ensemble by averaging predictions of all 5 cross-validation models. The other models were trained only once using either generated data (deconv-ResNet, Supplementary Fig. 1 ) or BMRB depositions excluding all benchmark proteins (GNN, KDE).
In this experiment, we reproduced 100 structures in fully automated manner using only NMR spectra and the protein sequences as input. Since ARTINA has no tunable parameters and does not require any manual curation of data, each structure was calculated by a single execution of the ARTINA workflow. All benchmark datasets were analyzed by ARTINA in parallel with execution times of 4–20 h per protein.
All automatically determined structures, overlaid with the corresponding reference structures from the PDB, are visualized in Fig. 3 , Supplementary Fig. 2 , and Supplementary Movie 1 . ARTINA was able to reproduce the reference structures with a median backbone root-mean-square deviation (RMSD) of 1.44 Å between the mean coordinates of the ARTINA structure bundle and the mean coordinates of the corresponding reference PDB structure bundle for the backbone atoms N, C α , C’ in the residue ranges determined by CYRANGE 29 (Fig. 4a and Supplementary Table 4 ). ARTINA automatically identified between 459 and 4678 distance restraints (2198 on average over 100 proteins), which corresponds to 4.25–33.20 restraints per residue (Fig. 4b ). This number is mainly influenced by the extent of unstructured regions and the quality of the NOESY spectra. In agreement with earlier findings 30 , it correlates only weakly with the backbone RMSD to reference (linear correlation coefficient −0.38). As a more expressive validation measure for the structures from ARTINA, we computed a predicted RMSD to the PDB reference structure on the basis of the RMSDs between the 10 candidate structure bundles calculated in ARTINA (see “Methods”, Fig. 5 , and Supplementary Table 5 ). The average deviation between actual and predicted RMSDs for the 100 proteins in this study is 0.35 Å, and their linear correlation coefficient is 0.77 (Fig. 5 ). In no case, the true RMSD exceeds the predicted one by more than 1 Å.
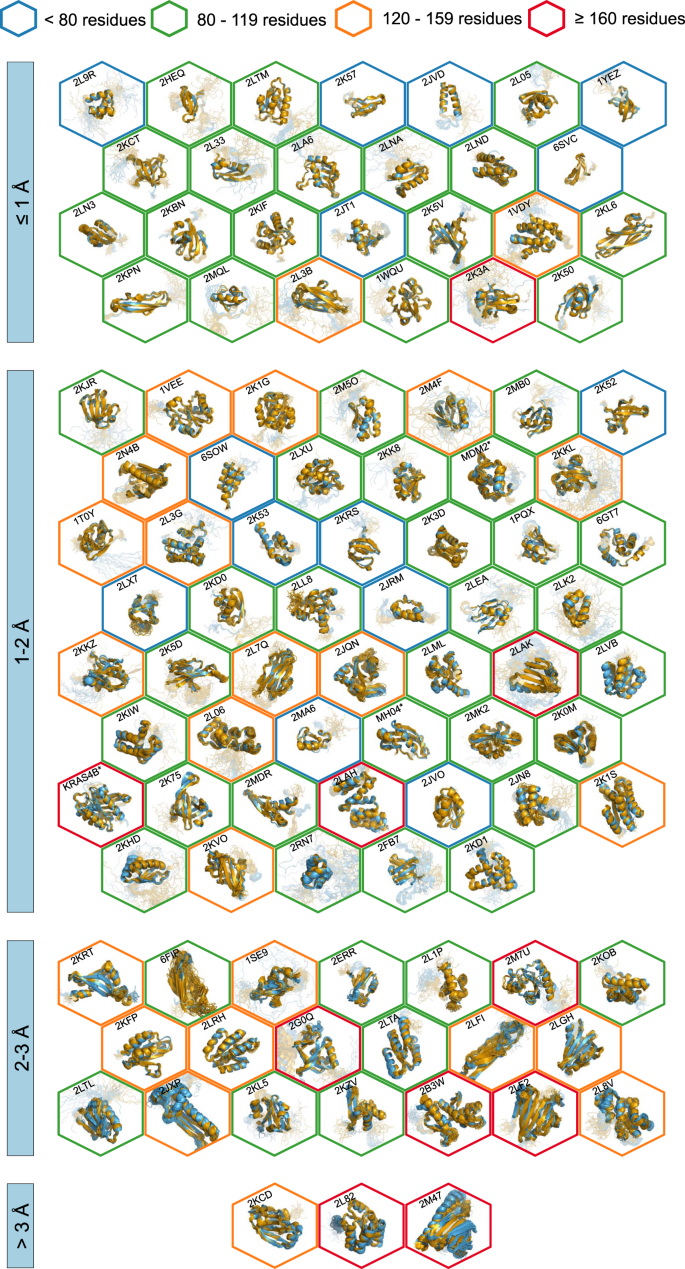
The structures are aligned with the RMSD to reference range as indicated on the left and hexagonal frames color-coded by their size as indicated above. Structures with no corresponding PDB depositions are marked by an asterisk.
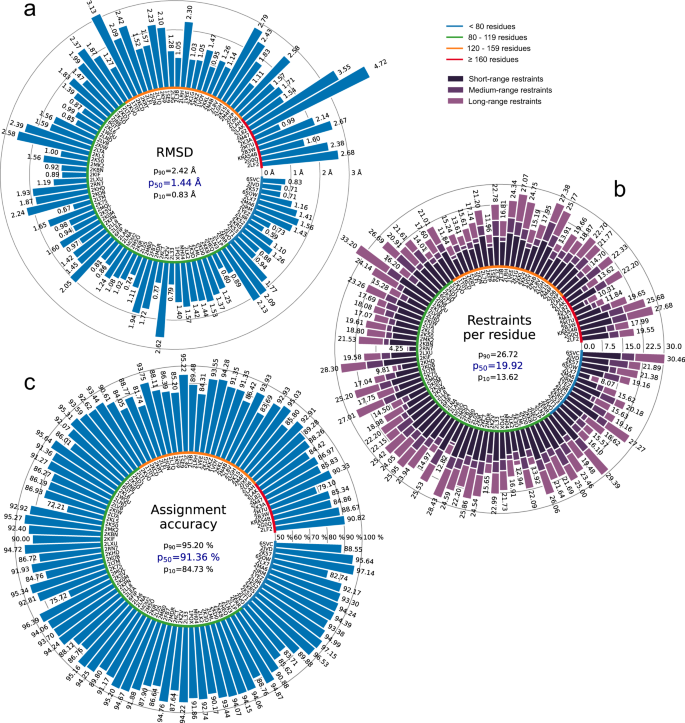
a Backbone RMSD to reference. b Number of distance restraints per residue. c Chemical shift assignment accuracy. Bars represent quantity values for benchmark proteins, identified by PDB codes (or protein names). Proteins are ordered by size, which is indicated by a color-coded circle. Values in the center of each panel are 10th, 50th, and 90th percentiles of values presented in the bar plot. Short/medium/long-range restraints are between residues i and j with | i – j | ≤ 1, 2 ≤ | i – j | ≤ 4, and | i – j | ≥ 5, respectively.
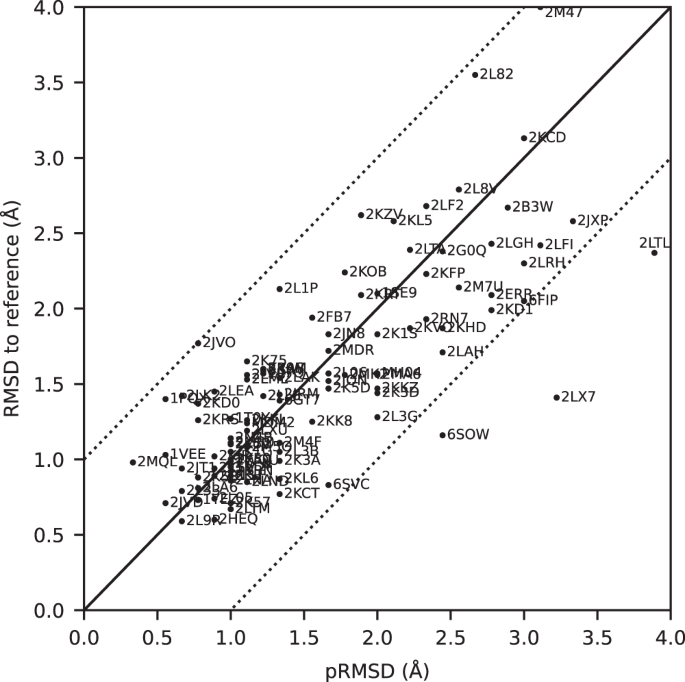
The predicted RMSD to reference (pRMSD) is calculated from the ARTINA results without knowledge of the reference PDB structure (see “Methods”) and, by definition, always in the range of 0–4 Å. For comparability, actual RMSD values to reference are also truncated at 4 Å (protein 2M47 with RMSD 4.47 Å). The dotted lines represent deviations of ±1 Å between the two RMSD quantities.
Additional structure validation scores obtained from ANSSUR 31 (Supplementary Table 6 ), RPF 32 (Supplementary Table 7 ), and consensus structure bundles 33 (Supplementary Table 8 ) confirm that overall the ARTINA structures and the corresponding reference PDB structures are of equivalent quality. Energy refinement of the ARTINA structures in explicit water using OPALp 34 (not part of the standard ARTINA workflow) does not significantly alter the agreement with the PDB reference structures (Supplementary Table 9 ). The benchmark data set comprises 78 protein structures determined by the Northeast Structural Genomics Consortium (NESG). On average, ARTINA yielded structures of the same accuracy for NESG targets (median RMSD to reference 1.44 Å) as for proteins from other sources (1.42 Å).
On average, ARTINA correctly assigned 90.39% of the chemical shifts (Fig. 4c ), as compared to the manually prepared assignments, including both “strong” (high-reliability) and “weak” (tentative) FLYA assignments 12 . Backbone chemical shifts were assigned more accurately (96.03%) than side-chain ones (86.50%), which is mainly due to difficulties in assigning lysine/arginine (79.97%) and aromatic (76.87%) side-chains. Further details on the assignment accuracy for individual amino acid types in the protein cores (residues with less than 20% solvent accessibility) are given in Supplementary Table 10 . Assignments for core residues, which are important for the protein structure, are generally more accurate than for the entire protein, in particular for core Ala, Cys, and Asp residues, which show a median assignment accuracy of 100% over the 100 proteins. The lowest accuracies are observed for core His (83.3%), Phe (83.3%), and Arg (87.5%) residues. The three proteins with highest RMSD to reference, 2KCD, 2L82, and 2M47 (see below), show 68.2, 83.8, and 75.7% correct aromatic assignments, respectively, well below the corresponding median of 85.5%. On the other hand, the assignment accuracies for the methyl-containing residues Ala, Ile, Val are above average and reach a median of 100, 97.6, and 98.6%, respectively.
The quality of automated structure determination and chemical shift assignment reflects the performance of deep learning-based visual spectrum analysis, presented qualitatively in Figs. 6 – 7 , Supplementary Fig. 3 , and Supplementary Movies 2 – 4 . In this experiment, our models (pp-ResNet, deconv-ResNet) automatically identified 1,168,739 cross-peaks with high confidence (≥0.50) in the benchmark spectra. All 1329 peak lists, together with automatically determined protein structures and chemical shift lists, are available for download.
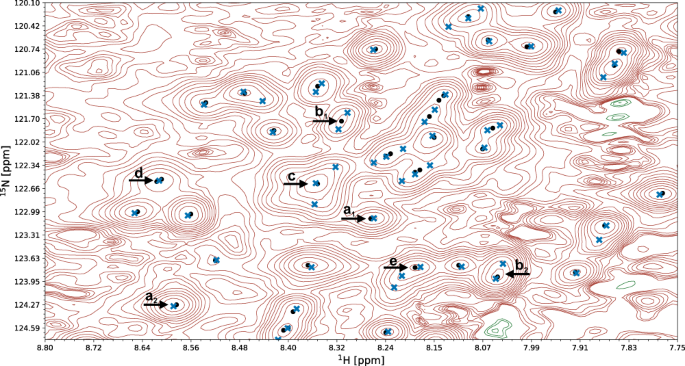
A fragment of a 15 N-HSQC spectrum of the protein 1T0Y is shown. Initial signal positions identified by the peak picking model pp-ResNet (black dots) are deconvolved by deconv-ResNet, yielding the final coordinates used for automated assignment and structure determination (blue crosses). a 1 , a 2 Initial peak picking marker position is refined by the deconvolution model. b 1 , b 2 pp-ResNet output is deconvolved into two components. c The deconvolution model supports maximally 3 components per initial signal. d Two peak picking markers are merged by the deconvolution model. e Peak picking output deconvolved into three components.
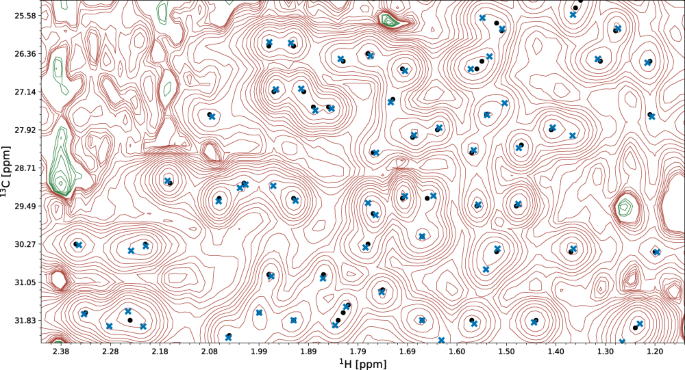
A fragment of the 13 C-HSQC spectrum of protein 2K0M is shown. Initial signal positions identified by the peak picking model pp-ResNet (black dots) are deconvolved by deconv-ResNet, yielding the final coordinates used for automated assignment and structure determination (blue crosses).
Error analysis
The largest deviations from the PDB reference structure were observed for the proteins 2KCD, 2L82, and 2M47, for which the pRMSD consistently indicated low accuracy (Fig. 5 ). Significant deviations are mainly due to displacements of terminal secondary structure elements (e.g., a tilted α-helix near a chain terminus), or inaccurate loop conformations (e.g., more flexible than in the PDB deposition). We investigated the origin of these discrepancies.
2KCD is a 120-residue (14.4 kDa) protein from Staphylococcus saprophyticus with an α-β roll architecture. Its dataset comprises 19 spectra (8 backbone, 6 side-chain, and 5 NOESY). The ARTINA structure has a backbone RMSD to PDB reference of 3.13 Å, which is caused by the displacement of the C-terminal α-helix (residues 105–109; Supplementary Fig. 4a ). Excluding this 5-residue fragment decreases the RMSD to 2.40 Å (Supplementary Table 11 ). The positioning of this helix appears to be uncertain, since an ARTINA calculation without the 4D CC-NOESY spectrum yields a significantly lower RMSD of 1.77 Å (Supplementary Table 12 ).
2L82 is a de novo designed protein of 162 residues (19.7 kDa) with an αβ 3-layer (αβα) sandwich architecture. Although only 9 spectra (4 backbone, 2 side-chain and 3 NOESY) are available, ARTINA correctly assigned 97.87% backbone and 81.05% side-chain chemical shifts. The primary reason for the high RMSD value of 3.55 Å is again a displacement of the C-terminal α-helix (residues 138–153). The remainder of the protein matches closely the PDB deposition (1.04 Å RMSD, Supplementary Fig. 4b ).
The protein with highest RMSD to reference (4.72 Å) in our benchmark dataset is 2M47, a 163-residue (18.8 kDa) protein from Corynebacterium glutamicum with an α-β 2-layer sandwich architecture, for which 17 spectra (7 backbone, 7 side chain and 3 NOESY) are available. The main source of discrepancy are two α-helices spanning residues 111–157 near the C-terminus. Nevertheless, the residues contributing to the high RMSD value are distributed more extensively than in 2L82 and 2KCD just discussed. Interestingly, 2 of the 10 structure proposals calculated by ARTINA have an RMSD to reference below 2 Å (1.66 Å and 1.97 Å). In the final structure selection step, our GBT model selected the 4.72 Å RMSD structure as the first choice and 1.66 Å as the second one (Supplementary Fig. 4c ). Such results imply that the automated structure determination of this protein is unstable. Since ARTINA returns the two structures selected by GBT with the highest confidence, the user can, in principle, choose the better structure based on contextual information.
In addition to these three case studies, we performed a quantitative analysis of all regular secondary structure elements and flexible loops present in our 100-protein benchmark in order to assess their impact on the backbone RMSD to reference (Supplementary Table 11 ). All residues in the structurally well-defined regions determined by CYRANGE 29 were assigned to 6 partially overlapping sets: (a) first secondary structure element, (b) last secondary structure element, (c) α-helices, (d) β-sheets, (e) α-helices and β-sheets, and (f) loops. Then, the RMSD to reference was calculated 6 times, each time with one set excluded. In total, for 66 of the 100 proteins the lowest RMSD was obtained if set (f) was excluded from RMSD calculation, and 13% benefited most from removal of the first or last secondary structure element (a or b). Moreover, for 18 out of the 19 proteins with more than 0.5 Å RMSD decrease compared to the RMSD for all well-defined residues, (a), (b), or (f) was the primary source of discrepancy. These results are consistent with our earlier statement that deviations in automatically determined protein structures are mainly caused by terminal secondary structure elements or inaccurate loop conformations.
Ablation studies
During the experiment, we captured the state of each structure determination at 9 time-points, 3 per structure determination cycle: (a) after the initial FLYA shift assignment, (b) after GNN shift refinement, and (c) after structure calculation (Fig. 1 ). Comparative analysis of these states allowed us to quantify the contribution of different ARTINA components to the structure determination process (Table 1 ).
The results show a strong benefit of the refinement cycles, as quantities reported in Table 1 consistently improve from cycle 1 to 3. The majority of benchmark proteins converge to the correct fold after the first cycle (1.56 Å median backbone RMSD to reference), which is further refined to 1.52 Å in cycle 2 and 1.44 Å in cycle 3. Additionally, within each chemical shift refinement cycle, improvements in assignment accuracy resulting from the GNN predictions are observed. This quantity also increases consistently across all refinement cycles, in particular for side-chains. Refinement cycles are particularly advantageous for large and challenging systems, such as 2LF2, 2M7U, or 2B3W, which benefit substantially in cycles 2 and 3 from the presence of the approximate protein fold in the chemical shift assignment step.
Impact of 4D NOESY experiments
As presented in Fig. 2 , 26 out of 100 benchmark datasets contain 4D CC-NOESY spectra, which require long measurement times and were used in the manual structure determination. To quantify their impact, we performed automated structure determinations of these 26 proteins with and without the 4D CC-NOESY spectra (Supplementary Table 12 ).
On average, the presence of 4D CC-NOESY improves the backbone RMSD to reference by 0.15 Å (decrease from 1.88 to 1.73 Å) and has less than 1% impact on chemical shift assignment accuracy. However, the impact is non-uniform. For three proteins, 2KIW, 2L8V, and 2LF2, use of the 4D CC-NOESY decreased the RMSD by more than 1 Å. On the other hand, there is also one protein, 2KCD, for which the RMSD decreased by more than 1 Å by excluding the 4D CC-NOESY.
These results suggest that overall the amount of information stored in 2D/3D experiments is sufficient for ARTINA to reach close to optimal performance, and only modest improvement can be achieved by introducing additional information redundancy from 4D CC-NOESY spectra.
Automated chemical shift assignment
Apart from structure determination, our data analysis pipeline for protein NMR spectroscopy can address an array of problems that are nowadays approached manually or semi-manually. For instance, ARTINA can be stopped after visual spectrum analysis, returning positions and intensities of cross-peaks that can be utilized for any downstream task, not necessarily related to protein structure determination.
Alternatively, a single chemical shift refinement cycle can be performed to get automatically assigned cross-peaks from spectra and sequence. We evaluated this approach with three sets of spectra: (i) Exclusively backbone assignment spectra were used to assign N, C α , C β , C’, and H N shifts. With this input, ARTINA assigned 92.40% (median value) of the backbone shifts correctly. (ii) All through-bond but no NOESY spectra were used to assign the backbone and side-chain shifts. This raised the percentage of correct backbone assignments to 94.20%. (iii) The full data set including NOESY yielded 96.60% correct assignments of the backbone shifts. These three experiments were performed for the 45 benchmark proteins, for which CBCANH and CBCAcoNH, as well as either HNCA and HNcoCA or HNCO and HNcaCO experiments were available. The availability of NOESY spectra had a large impact on the side-chain assignments: 86.00% were correct for the full spectra set iii, compared to 73.70% in the absence of NOESY spectra (spectra set ii). The presence of NOESY spectra consistently improved the chemical shift assignment accuracy of all amino acid types (Supplementary Tables 13 and 14 ). The improvement is particularly strong for aromatic residues (Phe, 61.6 to 76.5%, Trp 52.5 to 80%, and Tyr 71.4 to 89.7%), but not limited to this group.
The results obtained with ARTINA differ in several aspects substantially from previous approaches towards automating protein NMR analysis 3 , 4 , 7 , 12 , 17 , 18 , 19 , 35 . First, ARTINA comprehends the entire workflow from spectra to structures rather than individual steps in it, and there are strictly no manual interventions or protein-specific parameters to be adapted. Second, the quality of the results regarding peak identification, resonance assignments, and structures have been assessed on a large and diverse set of 100 proteins; for the vast majority of which they are on par with what can be achieved by human experts. Third, the method provides a two-orders-of-magnitude leap in efficiency by providing assignments and a structure within hours of computation time rather than weeks or months of human work. This reduces the effort for a protein structure determination by NMR essentially to the preparation of the sample and the measurement of the spectra. Its implementation in the https://nmrtist.org webserver (Supplementary Movie 5 ) encapsulates its complexity, eliminates any intermediate data and format conversions by the user, and enables the use of different types of high-performance hardware as appropriate for each of the subtasks. ARTINA is not limited to structure determination but can be used equally well for peak picking and resonance assignment in NMR studies that do not aim at a structure, such as investigations of ligand binding or dynamics.
Although ARTINA has no parameters to be optimized by the user, care should be given to the preparation of the input data, i.e., the choice, measurement, processing, and specification of the spectra. Spectrum type, axes, and isotope labeling declarations must be correct, and chemical shift referencing consistent over the entire set of spectra. Slight variations of corresponding chemical shifts within the tolerances of 0.03 ppm for 1 H and 0.4 ppm for 13 C/ 15 N can be accommodated, but larger deviations, resulting, for instance, from the use of multiple samples, pH changes, protein degradation, or inaccurate referencing, can be detrimental. Where appropriate, ARTINA proposes corrections of chemical shift referencing 36 . Furthermore, based on the large training data set, which comprises a large variety of spectral artifacts, ARTINA largely avoids misinterpreting artifacts as signals. However, with decreasing spectral quality, ARTINA, like a human expert, will progressively miss real signals.
Regarding protein size and spectrum quality, limitations of ARTINA are similar to those encountered by a trained spectroscopist. Machine-learning-based visual analysis of spectra requires signals to be present and distinguishable in the spectra. ARTINA does not suffer from accidental oversight that may affect human spectra analysis. On the other hand, human experts may exploit contextual information to which the automated system currently has no access because it identifies individual signals by looking at relatively small, local excerpts of spectra.
In this paper, we used all spectra that are available from the earlier manual structure determination. For most of the 100 proteins, the spectra data set has significant redundancy regarding information for the resonance assignment. Our results indicate that one can expect to obtain good assignments and structures also from smaller sets of spectra 37 , with concomitant savings of NMR measurement time. We plan to investigate this in a future study.
The present version of ARTINA can be enhanced in several directions. Besides improving individual models and algorithms, it is conceivable to integrate the so far independently trained collection of machine learning models, plus additional models that replace conventional algorithms, into a coherent system that is trained as a whole. Furthermore, the reliability of machine learning approaches depends strongly on the quantity and quality of training data available. While the collection of the present training data set for ARTINA was cumbersome, from now on it can be expected to expand continuously through the use of the https://nmrtist.org website, both quantitatively and qualitatively with regard to greater variability in terms of protein types. spectral quality, source laboratory, data processing (including non-linear sampling), etc., which can be exploited in retraining the models. ARTINA can also be extended to use additional experimental input data, e.g., known partial assignments, stereospecific assignments, 3 J couplings, residual dipolar couplings, paramagnetic data, and H-bonds. Structural information, e.g., from AlphaFold 38 , can be used in combination with reduced sets of NMR spectra for rapid structure-based assignment. Finally, the range of application of ARTINA can be generalized to small molecule-protein complexes relevant for structure-activity relationship studies in drug research, protein-protein complexes, RNA, solid state, and in-cell NMR.
Overall, ARTINA stands for a paradigm change in biomolecular NMR from a time-consuming technique for specialists to a fast method open to researchers in molecular biology and medicinal chemistry. At the same time, in a larger perspective, the appearance of generally highly accurate structure predictions by AlphaFold 38 is revolutionizing structural biology. Nevertheless, there remains space for the experimental methods, for instance, to elucidate various states of proteins under different conditions or in dynamic exchange, or for studying protein-ligand interaction. Regarding ARTINA, one should keep in mind that its applications extend far beyond structure determination. It will accelerate virtually any biological NMR studies that require the analysis of multidimensional NMR spectra and chemical shift assignments. Protein structure determination is just one possible ARTINA application, which is both demanding in terms of the amount and quality of required experimental data and amenable to quantitative evaluation.
Spectrum benchmark collection
To collect the benchmark of NMR spectra (Fig. 2 and Supplementary Table 2 ), we implemented a crawler software, which systematically scanned the FTP server of the BMRB data bank 39 , identifying data files relevant to our study. Additional datasets were obtained by setting up a website for the deposition of published data ( https://nmrdb.ethz.ch ), from our collaboration network, or had been acquired internally in our laboratory. NMR data was collected from these channels either in the form of processed spectra (Sparky 40 , NMRpipe 41 , XEASY 42 , Bruker formats), or in the form of time-domain data accompanied by depositor-supplied NMRpipe processing scripts. No additional spectra processing (e.g., baseline correction) was performed as part of this study.
The most challenging aspects of the benchmark collection process were: scarcity of data—only a small fraction of all BMRB depositions are accompanied by uploaded spectra (or time-domain data), lack of standards for NMR data depositions—each protein data set had to be prepared manually, as the original data was stored in different formats (spectra name conventions, axis label standards, spectra data format), and difficulties in correlating data files deposited in the BMRB FTP site with contextual information about the spectrum and the sample (e.g., sample characteristics, measurement conditions, instrument used). Manually prepared (mostly NOESY) peak lists, which are available from the BMRB for some of the proteins in the benchmark, were not used for this study.
Different approaches to 3D 13 C-NOESY spectra measurement had to be taken into account: (i) Two separate 13 C NOESY for aliphatic and aromatic signals. These were analyzed by ARTINA without any special treatment. We used ALI , ARO tags (Supplementary Movie S5 ) to provide the information that only either aliphatic or aromatics shifts are expected in a given spectrum. (ii) Simultaneous NC-NOESY. These spectra were processed twice to have proper scaling of the 13 C and 15 N axes in ppm units, and cropped to extract 15 N-NOESY and 13 C-NOESY spectra. If nitrogen and carbon cross-peak amplitudes have different signs, we used POS , NEG tags to provide the information that only either positive or negative signals should be analyzed. (iii) Aliphatic and aromatic signals in a single 13 C-NOESY spectrum. These measurements do not require any special treatment, but proper cross-peak unfolding plays a vital role in aromatic signals analysis.
Overview of the ARTINA algorithm
ARTINA uses as input only the protein sequence and a set of NMR spectra, which may contain any combination of 25 experiments currently supported by the method (Supplementary Table 1 ). Within 4–20 h of computation time (depending on protein size, number of spectra, and computing hardware load), ARTINA determines: (a) cross-peak positions for each spectrum, (b) chemical shift assignments, (c) distance restraints from NOESY spectra, and (d) the protein structure. The whole process does not require any human involvement, allowing rapid protein NMR assignment and structure determination by non-experts.
The ARTINA workflow starts with visual spectrum analysis (Fig. 1 ), wherein cross-peak positions are identified in frequency-domain NMR spectra using deep residual neural networks (ResNet) 24 . Coordinates of signals in the spectra are passed as input to the FLYA automated assignment algorithm 12 , yielding initial chemical shift assignments . In the subsequent chemical shift refinement step, we bring to the workflow contextual information about thousands of protein structures solved by NMR in the past using a deep GNN 25 that was trained on BMRB/PDB depositions. Its goal is to predict expected values of yet missing chemical shifts, given the shifts that have already been confidently and unambiguously assigned by FLYA. With these GNN predictions as additional input, the cross-peak positions are reassessed in a second FLYA call, which completes the chemical shift refinement cycle (Fig. 1 ).
In the structure refinement cycle , 10 variants of NOESY peak lists are generated, which differ in the number of cross-peaks selected from the output of the visual spectrum analysis by varying the confidence threshold of a signal selected by ResNet between 0.05 and 0.5. Each set of NOESY peak lists is used in an independent CYANA structure calculation 22 , 23 , yielding 10 intermediate structure proposals (Fig. 1 ). The structure proposals are ranked in the intermediate structure selection step based on 96 features with a dedicated GBT model. The selected best structure proposal is used as contextual information in a consecutive FLYA run, which closes the structure refinement cycle .
After the two initial steps of visual spectrum analysis and initial chemical shift assignment, ARTINA interchangeably executes refinement cycles. The chemical shift refinement cycle provides FLYA with tighter restraints on expected chemical shifts, which helps to assign ambiguous cross-peaks. The structure refinement cycle provides information about possible through-space contacts, allowing identified cross-peaks (especially in NOESY) to be reassigned. The high-level concept behind the interchangeable execution of refinement cycles is to iteratively update the protein structure given fixed chemical shifts, and update chemical shifts given the fixed protein structure. Both refinement cycles are executed three times.
Automated visual analysis of the spectrum
We established two machine learning models for the visual analysis of multidimensional NMR spectra (see downloads in the Code availability section). In their design, we made no assumptions about the downstream task and the 2D/3D/4D experiment type. Therefore, the proposed models can be used as the starting point of our automated structure determination procedure, as well as for any other task that requires cross-peak coordinates.
The automated visual analysis starts by selecting all extrema \({{{{{\boldsymbol{x}}}}}}=\left\{{{{{{{\boldsymbol{x}}}}}}}_{1},{{{{{{\boldsymbol{x}}}}}}}_{2},\ldots,{{{{{{\boldsymbol{x}}}}}}}_{N}\right\}\) , \({{{{{{\boldsymbol{x}}}}}}}_{n}\in {{\mathbb{N}}}^{D}\) in the NMR spectrum, which is represented as a D -dimensional regular grid storing signal intensities at discrete frequencies. We formulated the peak picking task as an object detection problem, where possible object positions are confined to \({{{{{\boldsymbol{x}}}}}}\) . This task was addressed by training a deep residual neural network 24 , in the following denoted as peak picking ResNet (pp-ResNet), which learns a mapping \({{{{{{\boldsymbol{x}}}}}}}_{n}\to[0,\,1]\) that assigns to each signal extremum a real-valued score, which resembles its probability of being a true signal rather than an artefact.
Our network architecture is strongly linked to ResNet-18 24 . It contains 8 residual blocks, followed by a single fully connected layer with sigmoidal activation. After weight initialization with Glorot Uniform 43 , the architecture was trained by optimizing a binary cross-entropy loss using Adam 44 with learning rate 10 –4 and gradient clipping of 0.5.
To establish an experimental training dataset for pp-ResNet, we normalized the 1329 spectra in our benchmark with respect to resolution (adjusting the number of data grid points per unit chemical shift (ppm) using linear interpolation) and signal amplitude (scaling the spectrum by a constant). Subsequently, 675,423 diverse 2D fragments of size 256 × 32 × 1 were extracted from the normalized spectra and manually annotated, yielding 98,730 positive and 576,693 negative class training examples. During the training process, we additionally augmented this dataset by flipping spectrum fragments along the second dimension (32 pixels), stretching them by 0–30% in the first and second dimensions, and perturbing signal intensities with Gaussian noise addition.
The role of the pp-ResNet is to quickly iterate over signal extrema in the spectrum, filtering out artefacts and selecting approximate cross-peak positions for the downstream task. The relatively small network architecture (8 residual blocks) and input size of 2D 256 × 32 image patches make it possible to analyze large 3D 13 C-resolved NOESY spectra in less than 5 min on a high-end desktop computer. Simultaneously, the first dimension of the image patch (256 pixels) provides long-range contextual information on the possible presence of signals aligned with the current extremum (e.g., C α , C β cross-peaks in an HNCACB spectrum).
Extrema classified with high confidence as true signals by pp-ResNet undergo subsequent analysis with a second deep residual neural network (deconv-ResNet). Its objective is to perform signal deconvolution, based on a 3D spectrum fragment (64 × 32 × 5 voxels) that is cropped around a signal extremum selected by pp-ResNet. This task is defined as a regression problem, where deconv-ResNet outputs a 3 × 3 matrix storing 3D coordinates of up to 3 deconvolved peak components, relative to the center of the input image. To ensure permutation invariance with respect to the ordering of components in the output coordinate matrix, and to allow for a variable number of 1–3 peak components, the architecture was trained with a Chamfer distance loss 45 .
Since deconv-ResNet deals only with true signals and their local neighborhood, its training dataset can be conveniently generated. We established a spectrum fragment generator, based on rules reflecting the physics of NMR, which produced 110,000 synthetic training examples (Supplementary Fig. 1 ) having variable (a) numbers of components to deconvolve (1–3), (b) signal-to-noise ratio, (c) component shapes (Gaussian, Lorentzian, and mixed), (d) component amplitude ratios, (e) component separation, and (f) component neighborhood type (i.e., NOESY-like signal strips or HSQC-like 2D signal clusters). The deconv-ResNet model was thus trained on fully synthetic data.
Signal unaliasing
To use ResNet predictions in automated chemical shift assignment and structure calculation, detected cross-peak coordinates must be transformed from the spectrum coordinate system to their true resonance frequencies. We addressed the problem of automated signal unfolding with the classical machine learning approach to density estimation.
At first, we generated 10 5 cross-peaks associated with each experiment type supported by ARTINA (Supplementary Table 1 ). In this process, we used randomly selected chemical shift lists deposited in the BMRB database, excluding depositions associated with our benchmark proteins. Subsequently, we trained a Kernel Density Estimator (KDE):
which captures the distribution \({p}_{e}\left({{{{{\boldsymbol{x}}}}}}\right)\) of true peaks being present at position \({{{{{\boldsymbol{x}}}}}}\) in spectrum type \(e\) , based on N e = 10 5 cross-peaks coordinates \({{{{{{\boldsymbol{x}}}}}}}_{i}^{(e)}\) generated with BMRB data, and \(\kappa\) being the Gaussian kernel.
Unfolding a k -dimensional spectrum is defined as a discrete optimization problem, solved independently for each cross-peak \({{{{{{\boldsymbol{x}}}}}}}_{j}^{\left(e\right)}\) observed in a spectrum of type \(e\) :
where \({{{{{\boldsymbol{w}}}}}}\in{{\mathbb{R}}}^{k}\) is a vector storing the spectral widths in each dimension (ppm units), \({{\circ }}\) is element-wise multiplication, \({{{{{\boldsymbol{s}}}}}}\in \,{{\mathbb{Z}}}^{k}\) is a vector indicating how many times the cross-peak is unfolded in each dimension, and \({{{{{{\boldsymbol{s}}}}}}}^{{{{{{\boldsymbol{*}}}}}}}\in {{\mathbb{Z}}}^{k}\) is the optimal cross-peak unfolding.
As long as regular and folded signals do not overlap or have different signs in the spectrum, KDE can unfold the peak list regardless of spectrum dimensionality. The spectrum must not be cropped in the folded dimension, i.e., the folding sweep width must equal the width of the spectrum in the corresponding dimension.
All 2D/3D spectra in our benchmark were folded in at most one dimension and satisfy the aforementioned requirements. However, the 4D CC-NOESY spectra satisfy neither, as regular and folded peaks both overlap and have the same signal amplitude sign. This introduces ambiguity in the spectrum unfolding that prevents direct use of the KDE technique. To retrieve original signal positions, 4D CC-NOESY cross-peaks were unfolded to overlap with signals detected in 3D 13 C-NOESY. In consequence, 4D CC-NOESY unfolding depended on other experiments, and individual 4D cross-peaks were retained only if they were confirmed in a 3D experiment.
Chemical shift assignment
Chemical shift assignment is performed with the existing FLYA algorithm 12 that uses a genetic algorithm combined with local optimization to find an optimal matching between expected and observed peaks. FLYA uses as input the protein sequence, lists of peak positions from the available spectra, chemical shift statistics, either from the BMRB 39 or the GNN described in the next section, and, if available, the structure from the previous refinement cycle. The tolerance for the matching of peak positions and chemical shifts was set to 0.03 ppm for 1 H, and 0.4 ppm for 13 C/ 15 N shifts. Each FLYA execution comprises 20 independent runs with identical input data that differ in the random numbers used in the optimization algorithm. Nuclei for which at least 80% of the 20 runs yield, within tolerance, the same chemical shift value are classified as reliably assigned 12 and used as input for the following chemical shift refinement step.
Chemical shift refinement
We used a graph data structure to combine FLYA-assigned shifts with information from previously assigned proteins (BMRB records) and possible spatial interactions. Each node corresponds to an atom in the protein sequence, and is represented by a feature vector composed of (a) a one-hot encoded atom type code (e.g., C α , H β ), (b) a one-hot encoded amino acid type, (c) the value of the chemical shift assigned by FLYA (only if a confident assignment is available, zero otherwise), (d) atom-specific BMRB shift statistics (mean and standard deviation), and (e) 30 chemical shift values obtained from BMRB database fragments. The latter feature is obtained by searching BMRB records for assigned 2–3-residue fragments that match the local protein sequence and have minimal mean-squared-error (MSE) to shifts confidently assigned by FLYA (non-zero values of feature (c) in the local neighborhood of the atom). The edges of the graph correspond to chemical bonds or skip connections. The latter connect the C β atom of a given residue with C β atoms 2, 3, and 5 residues apart in the amino acid sequence, and have the purpose to capture possible through-space influence on the chemical shift that is typically observed in secondary structure elements.
The chemical shift refinement task is defined as a node regression problem, where an expected value of the chemical shift is predicted for each atom that lacks a confident FLYA assignment. This task is addressed with a DeepGCN model 25 , 26 that was trained on 28,400 graphs extracted from 2840 referenced BMRB records 39 . Each training example was created by building a fully assigned graph out of a single BMRB record, and dropping chemical shift values (feature (c) above) for randomly chosen atoms that FLYA typically assigns either with low confidence or inaccurately.
Our DeepGCN model is designed specifically for de novo structure determination, as it uses only the protein sequence and partial shift assignments to estimate values of missing chemical shifts. Its predictions are used to guide the FLYA genetic algorithm optimization 12 by reducing its search range for assignments. The precise final chemical shift value is always determined by the position of a signal in the spectrum, rather than the model prediction alone.
Torsion angle restraints
Before each structure calculation step, torsion angle restraints for the ϕ and ψ angles of the polypeptide backbone were obtained from the current backbone chemical shifts using the program TALOS-N 21 . Restraints were only generated if TALOS-N classified the prediction as ‘Good’, ‘Strong’, or ‘Generous’. Given a TALOS-N torsion angle prediction of ϕ ± Δ ϕ , the allowed range of the torsion angle was set to ϕ ± max(Δ ϕ , 10°) for ‘Good’ and ‘Strong’ predictions, and ϕ ± 1.5 max(Δ ϕ , 10°) for ‘Generous’ predictions, and likewise for ψ .
Structure calculation and selection
Given the chemical shift assignments and NOESY cross-peak positions and intensities, the structure is calculated with CYANA 23 using the established method 22 that comprises 7 cycles of NOESY cross-peak assignment and structure calculation, followed by a final structure calculation. In total, 8 × 100 conformers are calculated for a given input data set using 30,000 torsion angle dynamics steps per conformer. The 20 conformers with the lowest final target function value are chosen to represent the solution structure proposal. The entire combined NOESY assignment and structure calculation procedure is executed independently 10 times based on 10 variants of NOESY peak lists, which differ in the number of cross-peaks selected from the output of the visual spectrum analysis. The first set generously includes all signals selected by ResNet with confidence ≥0.05. The other variants of NOESY peak lists follow the same principle with increasingly restrictive confidence thresholds of 0.1, 0.15, …, 0.5.
The CYANA structures calculations are followed by a structure selection step, wherein the 10 intermediate structure proposals are compared pairwise by a Gradient Boosted Tree (GBT) model that uses 96 features from each structure proposal (including the CYANA target function value 23 , number of long-range distance restraints, etc.; for details, see downloads in the Code availability section) to rank the structures by their expected accuracy. The best structure from the ranking is subsequently used as contextual information for the chemical shift refinement cycle (Fig. 1 ), or returned as the final outcome of ARTINA. The second-best final structure is also returned for comparison.
To train GBT, we collected a set of successful and unsuccessful structure calculations with CYANA. Each training example was a tuple ( s i , r i ), where s i is the vector of features extracted from the CYANA structure calculation output, and r i is the RMSD of the output structure to the PDB reference. The GBT was trained to take the features s i and s j of two structure calculations with CYANA as input, and to predict a binary order variable o ij , such that o ij = 1 if r i < r j , and 0 otherwise. Importantly, the deposited PDB reference structures were not used directly in the GBT model training (they are used only to calculate the RMSDs). Consequently, the GBT model is unaffected by methodology and technicalities related to PDB deposition (e.g., the structure calculation software used to calculate the deposited reference structure).
Structure accuracy estimate
As an accuracy estimate for the final ARTINA structure, a predicted RMSD to reference (pRMSD) is calculated from the ARTINA results (without knowledge of the reference PDB structure). It aims at reproducing the actual RMSD to reference, which is the RMSD between the mean coordinates of the ARTINA structure bundle and the mean coordinates of the corresponding reference PDB structure bundle for the backbone atoms N, C α , C’ in the residue ranges as given in Supplementary Table 4 . The predicted RMSD is given by pRMSD = (1 – t ) × 4 Å, where, in analogy to the GDT_HA value 46 , t is the average fraction of the RMSDs ≤ 0.5, 1, 2, 4 Å between the mean coordinates of the best ARTINA candidate structure bundle and the mean coordinates of the structure bundles of the 9 other structure proposals. Since t ∈ [0, 1], the pRMSD is always in the range of 0–4 Å, grouping all “bad” structures with expected RMSD to reference ≥ 4 Å at pRMSD = 4 Å.
Reporting summary
Further information on research design is available in the Nature Research Reporting Summary linked to this article.
Data availability
References structures: PDB Protein Data Bank ( https://www.rcsb.org/ ; accession codes in Fig. 2 and Supplementary Table 3 ).
Spectra and reference assignments: BMRB Biological Magnetic Resonance Data Bank ( https://bmrb.io/ ; entry IDs in Supplementary Table 3 ).
Peak lists, assignments, and structures: https://nmrtist.org/static/public/publications/artina/ARTINA_results.zip and in the ETH Research Collection under DOI 10.3929/ethz-b-000568621.
Source data for Figs. 2 , 4 , and 5 is available in Supplementary Tables 2 , 4 , and 5, respectively.
Code availability
The ARTINA algorithm is available as a webserver at https://nmrtist.org . pp-ResNet, deconv-ResNet, GNN, and GBT are available for download in binary form, together with architecture schemes, example input data, model input description, and source code that allows to read model files and make predictions ( https://github.com/PiotrKlukowski/ARTINA , https://nmrtist.org/static/public/publications/artina/models/ {ARTINA_peak_picking.zip, ARTINA_peak_deconvolution.zip, ARTINA_shift_prediction.zip, ARTINA_structure_ranking.zip}). These files provide a full technical specification of the components developed within ARTINA, and allow for their independent use in Python.
Existing software used: Python ( https://www.python.org/ ), CYANA ( https://www.las.jp/ ), TALOS-N ( https://spin.niddk.nih.gov/bax/software/TALOS-N ).
Wüthrich, K. NMR studies of structure and function of biological macromolecules (Nobel Lecture). Angew. Chem. Int. Ed. 42 , 3340–3363 (2003).
Article CAS Google Scholar
Sakakibara, D. et al. Protein structure determination in living cells by in-cell NMR spectroscopy. Nature 458 , 102–105 (2009).
Article ADS CAS Google Scholar
Guerry, P. & Herrmann, T. Advances in automated NMR protein structure determination. Q. Rev. Biophys. 44 , 257–309 (2011).
Güntert, P. Automated structure determination from NMR spectra. Eur. Biophys. J. 38 , 129–143 (2009).
Garrett, D. S., Powers, R., Gronenborn, A. M. & Clore, G. M. A common sense approach to peak picking two-, three- and four-dimensional spectra using automatic computer analysis of contour diagrams. J. Magn. Reson. 95 , 214–220 (1991).
ADS CAS Google Scholar
Koradi, R., Billeter, M., Engeli, M., Güntert, P. & Wüthrich, K. Automated peak picking and peak integration in macromolecular NMR spectra using AUTOPSY. J. Magn. Reson. 135 , 288–297 (1998).
Würz, J. M. & Güntert, P. Peak picking multidimensional NMR spectra with the contour geometry based algorithm CYPICK. J. Biomol. NMR 67 , 63–76 (2017).
Klukowski, P. et al. NMRNet: A deep learning approach to automated peak picking of protein NMR spectra. Bioinformatics 34 , 2590–2597 (2018).
Li, D. W., Hansen, A. L., Yuan, C. H., Bruschweiler-Li, L. & Brüschweiler, R. DEEP picker is a deep neural network for accurate deconvolution of complex two-dimensional NMR spectra. Nat. Commun. 12 , 5229 (2021).
Bartels, C., Güntert, P., Billeter, M. & Wüthrich, K. GARANT—A general algorithm for resonance assignment of multidimensional nuclear magnetic resonance spectra. J. Comput. Chem. 18 , 139–149 (1997).
Zimmerman, D. E. et al. Automated analysis of protein NMR assignments using methods from artificial intelligence. J. Mol. Biol. 269 , 592–610 (1997).
Schmidt, E. & Güntert, P. A new algorithm for reliable and general NMR resonance assignment. J. Am. Chem. Soc. 134 , 12817–12829 (2012).
Linge, J. P., O’Donoghue, S. I. & Nilges, M. Automated assignment of ambiguous nuclear overhauser effects with ARIA. Methods Enzymol. 339 , 71–90 (2001).
Herrmann, T., Güntert, P. & Wüthrich, K. Protein NMR structure determination with automated NOE assignment using the new software CANDID and the torsion angle dynamics algorithm DYANA. J. Mol. Biol. 319 , 209–227 (2002).
Allain, F., Mareuil, F., Ménager, H., Nilges, M. & Bardiaux, B. ARIAweb: a server for automated NMR structure calculation. Nucleic Acids Res. 48 , W41–W47 (2020).
Lee, W. et al. I-PINE web server: Aan integrative probabilistic NMR assignment system for proteins. J. Biomol. NMR 73 , 213–222 (2019).
Huang, Y. P. J. et al. An integrated platform for automated analysis of protein NMR structures. Methods Enzymol. 394 , 111–141 (2005).
Kobayashi, N. et al. KUJIRA, a package of integrated modules for systematic and interactive analysis of NMR data directed to high-throughput NMR structure studies. J. Biomol. NMR 39 , 31–52 (2007).
López-Méndez, B. & Güntert, P. Automated protein structure determination from NMR spectra. J. Am. Chem. Soc. 128 , 13112–13122 (2006).
Murphy, K. P. Probabilistic Machine Learning: An Introduction (MIT Press, 2022).
Shen, Y. & Bax, A. Protein backbone and sidechain torsion angles predicted from NMR chemical shifts using artificial neural networks. J. Biomol. NMR 56 , 227–241 (2013).
Güntert, P. & Buchner, L. Combined automated NOE assignment and structure calculation with CYANA. J. Biomol. NMR 62 , 453–471 (2015).
Güntert, P., Mumenthaler, C. & Wüthrich, K. Torsion angle dynamics for NMR structure calculation with the new program DYANA. J. Mol. Biol. 273 , 283–298 (1997).
Article Google Scholar
Kaiming, H., Xiangyu, Z., Shaoqing, R. & Jian, S. Deep residual learning for image recognition. In Proc. 2016 IEEE Conference on Computer Vision and Pattern Recognition (CVPR) 770–778 (2016).
Kipf, T. N. & Welling, M. Semi-supervised classification with graph convolutional networks. Preprint at https://arxiv.org/abs/1609.02907 (2016).
Chiang, W. L. et al. Cluster-GCN: An efficient algorithm for training deep and large graph convolutional networks. In Proc. 25th ACM SIGKDD International Conference on Knowledge Discovery & Data Mining (KDD) 257–266 (2019).
Prokhorenkova, L., Gusev, G., Vorobev, A., Dorogush, A. V. & Gulin, A. CatBoost: Unbiased boosting with categorical features. In Proc. 32nd Conference on Neural Information Processing Systems (NIPS) (2018).
Rosato, A. et al. The second round of Critical Assessment of Automated Structure Determination of Proteins by NMR: CASD-NMR-2013. J. Biomol. NMR 62 , 413–424 (2015).
Kirchner, D. K. & Güntert, P. Objective identification of residue ranges for the superposition of protein structures. BMC Bioinform. 12 , 170 (2011).
Buchner, L. & Güntert, P. Systematic evaluation of combined automated NOE assignment and structure calculation with CYANA. J. Biomol. NMR 62 , 81–95 (2015).
Fowler, N. J., Sljoka, A. & Williamson, M. P. A method for validating the accuracy of NMR protein structures. Nat. Commun . 11 , 6321 (2020).
Huang, Y. J., Powers, R. & Montelione, G. T. Protein NMR recall, precision, and F-measure scores (RPF scores): Structure quality assessment measures based on information retrieval statistics. J. Am. Chem. Soc. 127 , 1665–1674 (2005).
Buchner, L. & Güntert, P. Increased reliability of nuclear magnetic resonance protein structures by consensus structure bundles. Structure 23 , 425–434 (2015).
Koradi, R., Billeter, M. & Güntert, P. Point-centered domain decomposition for parallel molecular dynamics simulation. Comput. Phys. Commun. 124 , 139–147 (2000).
Herrmann, T., Güntert, P. & Wüthrich, K. Protein NMR structure determination with automated NOE-identification in the NOESY spectra using the new software ATNOS. J. Biomol. NMR 24 , 171–189 (2002).
Buchner, L., Schmidt, E. & Güntert, P. Peakmatch: A simple and robust method for peak list matching. J. Biomol. NMR 55 , 267–277 (2013).
Scott, A., López-Méndez, B. & Güntert, P. Fully automated structure determinations of the Fes SH2 domain using different sets of NMR spectra. Magn. Reson. Chem. 44 , S83–S88 (2006).
Jumper, J. et al. Highly accurate protein structure prediction with AlphaFold. Nature 596 , 583–589 (2021).
Ulrich, E. L. et al. BioMagResBank. Nucleic Acids Res. 36 , D402–D408 (2008).
Goddard, T. D. & Kneller, D. G. Sparky 3. (University of California, San Francisco, 2001).
Delaglio, F. et al. NMRPipe—A multidimensional spectral processing system based on Unix pipes. J. Biomol. NMR 6 , 277–293 (1995).
Bartels, C., Xia, T. H., Billeter, M., Güntert, P. & Wüthrich, K. The program XEASY for computer-supported NMR spectral analysis of biological macromolecules. J. Biomol. NMR 6 , 1–10 (1995).
Glorot, X. & Bengio, Y. Understanding the difficulty of training deep feedforward neural networks. Proc. Mach. Learn. Res. 9 , 249–256 (2010).
Google Scholar
Kingma, D. P. & Ba, J. Adam: A method for stochastic optimization. Preprint at https://arxiv.org/abs/1412.6980 (2015).
Davies, E. R. Computer Vision (Academic Press, 2018).
Kryshtafovych, A. et al. New tools and expanded data analysis capabilities at the protein structure prediction center. Proteins 69 , 19–26 (2007).
Download references
Acknowledgements
We thank Drs. Frédéric Allain, Fred Damberger, Hideo Iwai, Harindranath Kadavath, Julien Orts, and Dean Strotz for providing unpublished spectra. This project has received funding from the European Union’s Horizon 2020 research and innovation program under the Marie Sklodowska-Curie grant agreement No 891690 (P.K.), and a Grant-in-Aid for Scientific Research of the Japan Society for the Promotion of Science (P.G., 20 K06508).
Author information
Authors and affiliations.
Laboratory of Physical Chemistry, ETH Zurich, Vladimir-Prelog-Weg 2, 8093, Zurich, Switzerland
Piotr Klukowski, Roland Riek & Peter Güntert
Institute of Biophysical Chemistry, Goethe University Frankfurt, Max-von-Laue-Str. 9, 60438, Frankfurt am Main, Germany
- Peter Güntert
Department of Chemistry, Tokyo Metropolitan University, 1-1 Minami-Osawa, Hachioji, 192-0397, Tokyo, Japan
You can also search for this author in PubMed Google Scholar
Contributions
P.K. prepared training and test data sets, designed and trained machine learning models, performed experiments described in the manuscript, and implemented ARTINA within the nmrtist.org web platform. P.K. and P.G. wrote the software. P.K., R.R., and P.G. conceived the project, analyzed the results, and wrote the manuscript.
Corresponding authors
Correspondence to Piotr Klukowski , Roland Riek or Peter Güntert .
Ethics declarations
Competing interests.
The authors declare no competing interests.
Peer review
Peer review information.
Nature Communications thanks Benjamin Bardiaux, Gaetano Montelione, Theresa Ramelot, and the other, anonymous, reviewer(s) for their contribution to the peer review of this work. Peer reviewer reports are available.
Additional information
Publisher’s note Springer Nature remains neutral with regard to jurisdictional claims in published maps and institutional affiliations.
Supplementary information
Supplementary info file #1, description of additional supplementary files, supplementary movie 1, supplementary movie 2, supplementary movie 3, supplementary movie 4, supplementary movie 5, reporting summary, peer review file, rights and permissions.
Open Access This article is licensed under a Creative Commons Attribution 4.0 International License, which permits use, sharing, adaptation, distribution and reproduction in any medium or format, as long as you give appropriate credit to the original author(s) and the source, provide a link to the Creative Commons license, and indicate if changes were made. The images or other third party material in this article are included in the article’s Creative Commons license, unless indicated otherwise in a credit line to the material. If material is not included in the article’s Creative Commons license and your intended use is not permitted by statutory regulation or exceeds the permitted use, you will need to obtain permission directly from the copyright holder. To view a copy of this license, visit http://creativecommons.org/licenses/by/4.0/ .
Reprints and permissions
About this article
Cite this article.
Klukowski, P., Riek, R. & Güntert, P. Rapid protein assignments and structures from raw NMR spectra with the deep learning technique ARTINA. Nat Commun 13 , 6151 (2022). https://doi.org/10.1038/s41467-022-33879-5
Download citation
Received : 28 March 2022
Accepted : 30 September 2022
Published : 18 October 2022
DOI : https://doi.org/10.1038/s41467-022-33879-5
Share this article
Anyone you share the following link with will be able to read this content:
Sorry, a shareable link is not currently available for this article.
Provided by the Springer Nature SharedIt content-sharing initiative
This article is cited by
The 100-protein nmr spectra dataset: a resource for biomolecular nmr data analysis.
- Piotr Klukowski
- Fred F. Damberger
Scientific Data (2024)
Overlay databank unlocks data-driven analyses of biomolecules for all
- Anne M. Kiirikki
- Hanne S. Antila
- O. H. Samuli Ollila
Nature Communications (2024)
5D solid-state NMR spectroscopy for facilitated resonance assignment
- Alexander Klein
- Suresh K. Vasa
- Rasmus Linser
Journal of Biomolecular NMR (2023)
By submitting a comment you agree to abide by our Terms and Community Guidelines . If you find something abusive or that does not comply with our terms or guidelines please flag it as inappropriate.
Quick links
- Explore articles by subject
- Guide to authors
- Editorial policies
Sign up for the Nature Briefing newsletter — what matters in science, free to your inbox daily.

Nucleic Acid
Nucleic acids are large molecules where genetic information is stored. They are macromolecules that store genetic information and enable protein production. They are composed of individual acid units termed nucleotides. There are two types of nucleic acids: deoxyribonucleic acid, better known as DNA and ribonucleic acid, better known as RNA. They carry the genetic blueprint of a cell and carry instructions for the functioning of the cell.
The nucleic acids are made of nucleotides. DNA and RNA are made up of monomers also known as nucleotides. A nucleotide is made of a nitrogenous base, sugar with five carbon atoms and a phosphate group. This compound was neither a protein nor lipid nor a carbohydrate; therefore, it was a novel type of biological molecule. Each nucleotide is made up of three components: a nitrogenous base, a pentose sugar, and a phosphate group. They are the most important macromolecules for the continuity of life.
Nucleic acids allow organisms to transfer genetic information from one generation to the next. When a cell divides, its DNA is copied and passed from one cell generation to the next generation. DNA is composed of a phosphate-deoxyribose sugar backbone and the nitrogenous bases adenine (A), guanine (G), cytosine (C), and thymine (T). It is found in the nucleus of eukaryotes and in the organelles, chloroplasts, and mitochondria. The order, or sequence, of the nucleotides in DNA allows nucleic acid to encode an organism’s genetic blueprint.
DNA is organized into chromosomes and found within the nucleus of our cells. Its function in any cell is to carry the sequence of bases which will be transcribed into RNA. The function of RNA is much more difficult to explain. RNA has ribose sugar and the nitrogenous bases A, G, C, and uracil (U). One well-known function is for messenger RNA to be translated into proteins, mainly enzymes. But there are other types of RNA, and these are called non-coding RNAs.
Inert Gas – Zero Group Elements
Chemical nomenclature, suspension (definition, properties), soil contamination, tobacco hawkmoths benefit from learning to select a nectar source and oviposition site more efficiently, a protocol for using robotic pets in memory care is being introduced, biography of johan cruyff, the leap at rhodes, intercropping – an agricultural practice, latest post, urban climatology, oceanic trenches, conformational isomerism – in chemistry, researchers are harnessing rna in a new strategy to combating hiv, antibiotic resistance genes have significantly increased in both humans and cattle, cyclopentyne.
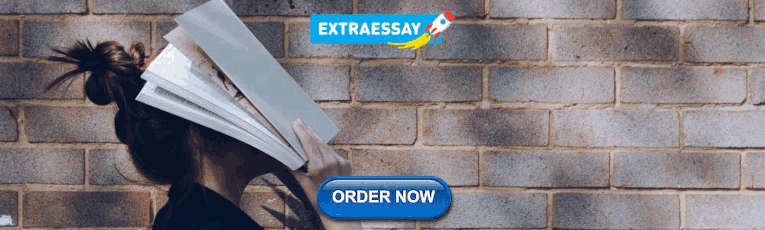
IMAGES
VIDEO
COMMENTS
Brønsted-Lowry. The Brønsted-Lowry definition of acids and bases liberates the acid-base concept from its limitation to aqueous solutions, as well as the requirement that bases contain the hydroxyl group. A Brønsted-Lowry acid is a hydrogen-containing species which is capable of acting as a proton (hydrogen ion) donor. A Brønsted-Lowry base is a species which is capable of acting ...
The O atom is donating an electron pair to the H + ion, making the base an electron pair donor and the acid an electron pair acceptor. The N atom is donating a lone pair to B in BF 3, Hence NH 3 is the Lewis base and BF 3 is the Lewis acid. 11.E: Acids and Bases (Exercises) Problems and select solutions to the chapter.
Brønsted-Lowry acids and bases. Autoionization of water. Water autoionization and Kw. Definition of pH. Strong acid solutions. Strong base solutions. Acid strength, anion size, and bond energy. Weak acid-base equilibria. Conjugate acid-base pairs.
The sodium hydroxide, calcium carbonate and potassium oxide are examples of bases. A base is a substance that reacts with hydrogen ions and can neutralize the acid. Most bases are minerals which form water and salts by reacting with acids. Bases include the metal oxides, hydroxides, and carbonates. Q8.
Some acids are natural, meaning they exist in nature. People often drink liquids that contain these acids. An acid is a molecule that splits apart in water and releases hydrogen ions. ... The Acids and Bases lesson plan includes three worksheets: an activity worksheet, a practice worksheet, and a homework assignment. Each worksheet will help ...
This page contains materials for the session on acid-base chemistry. It features a 1-hour lecture video, and also presents the prerequisites, learning objectives, reading assignment, lecture slides, homework with solutions, and resources for further study.
The Arrhenius definition of acid-base reactions is a development of the "hydrogen theory of acids". It was used to provide a modern definition of acids and bases, and followed from Arrhenius's work with Friedrich Wilhelm Ostwald in establishing the presence of ions in aqueous solution in 1884. This led to Arrhenius receiving the Nobel Prize in ...
Acid-base neutralization reactions and titration studies will also be addressed. Problems will range from the very easy plug-and-chug to the more difficult analysis of multi-step problems. Click a link to open a publicly-available problem set. If you are a Task Tracker student, open the assignment using the link on the Task Tracker assignment ...
O 2 -. H 2PO − 4. H 2 PO − 4. Identify the conjugate acids of the species in the previous problem. Identify and label the Brønsted-Lowry acid, its conjugate base, the Brønsted-Lowry base, and its conjugate acid in each of the following equations: NO − 2 + H 2O HNO 2 + OH −. NO − 2 + H 2 O HNO 2 + OH −. HBr+ H 2O H 3O + + Br −.
Acids and bases worksheets for Grade 11 are essential resources for science teachers who aim to provide their students with a comprehensive understanding of the fundamental concepts in chemistry. These worksheets cover a wide range of topics, including the properties of acids and bases, pH scale, neutralization reactions, and titration.
Salt + Water. Report a problem. Learn for free about math, art, computer programming, economics, physics, chemistry, biology, medicine, finance, history, and more. Khan Academy is a nonprofit with the mission of providing a free, world-class education for anyone, anywhere.
Identify two acids and two bases that you use or come into contact with in an average week. Identify uses for each substance. I use citric acid everyday when I eat foods with preservatives and citrus fruits. I use magnesium hydroxide everyday when I put on my deodorant.
acid, any substance that in water solution tastes sour, changes the colour of certain indicators (e.g., reddens blue litmus paper), reacts with some metals (e.g., iron) to liberate hydrogen, reacts with bases to form salts, and promotes certain chemical reactions (acid catalysis). Examples of acids include the inorganic substances known as the ...
There are three primary theories of acid-base chemistry that are often taught together: Arrhenius theory, Brønsted-Lowry theory, and Lewis acid-base theory. Each theory is introduced below. Figure 7.1A. 1 7.1 A. 1: Hierarchal definitions of acids and bases via the three primary theories. These theories are designed to be "superset" of the ...
The pH scale ranges from 0 to 14. Substances with a pH between 0 and less than 7 are acids (pH and [H +] are inversely related - lower pH means higher [H +] and a stronger acid). Substances with a pH greater than 7 and up to 14 are bases (the higher the pH, the stronger the base). Right in the middle, at pH = 7, are neutral substances, for ...
Chemistry Worksheets Class 10 on Chapter 2 Acids, Bases and Salts with Answers - Set 1. Acids are substances that taste sour and are corrosive in nature. It turns blue litmus paper to red. These substances are chemically acidic in nature.E.g.:-orange juice, curd, vinegar, hydrochloric acid etc. Bases are substances that, in an aqueous solution ...
Acidity is measured on a scale called pH, as shown in Figure below. Pure water has a pH of 7, so the point of neutrality on the pH scale is 7. Acidity and the pH scale: Water has a pH of 7, so this is the point of neutrality on the pH scale. Acids have a pH less than 7, and bases have a pH greater than 7. Approximate pH of examples are shown.
nucleic acid, naturally occurring chemical compound that is capable of being broken down to yield phosphoric acid, sugars, and a mixture of organic bases (purines and pyrimidines).Nucleic acids are the main information-carrying molecules of the cell, and, by directing the process of protein synthesis, they determine the inherited characteristics of every living thing.
A substance with a pH of 2 has a 10 times higher concentration of H+ than a substance with a pH of 3. Study with Quizlet and memorize flashcards containing terms like pure water, pH = 7.0 lake water, pH = 6.5 baking soda solution, pH = 9 soapy water, pH = 12, rainwater, pOH = 8.5 cola, pOH = 11 tomato juice, pOH = 10 liquid drain cleaner, pOH ...
Upload your completed assignment to the "Acid Rain assignment folder" on Brightspace. This assignment is worth 25 points. Explain how fossil fuels, which are made of carbon and hydrogen, contain sulfur compounds. (2 points) Explain how nitrogen is released into the air to form nitric acid and nitrous acid, causing acid rain. (2 points)
5.6: Lewis Acids and Bases. A Lewis acid is a species that can accept an electron pair, whereas a Lewis base has an electron pair available for donation to a Lewis acid. Complex ions are examples of Lewis acid-base adducts. In a complex ion, we have a central atom, often consisting of a transition metal cation, which acts as a Lewis acid, and ...
Further details on the assignment accuracy for individual amino acid types in the protein cores (residues with less than 20% solvent accessibility) are given in Supplementary Table 10. Assignments ...
Nucleic acids are large molecules where genetic information is stored. They are macromolecules that store genetic information and enable protein production. They are composed of individual acid units termed nucleotides. There are two types of nucleic acids: deoxyribonucleic acid, better known as DNA and ribonucleic acid, better known as RNA.