- Our Mission
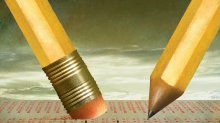
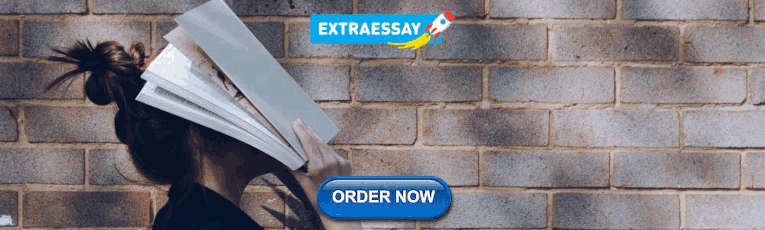
What Does the Research Say About Testing?
There’s too much testing in schools, most teachers agree, but well-designed classroom tests and quizzes can improve student recall and retention.
For many teachers, the image of students sitting in silence filling out bubbles, computing mathematical equations, or writing timed essays causes an intensely negative reaction.
Since the passage of the No Child Left Behind Act (NCLB) in 2002 and its 2015 update, the Every Student Succeeds Act (ESSA), every third through eighth grader in U.S. public schools now takes tests calibrated to state standards, with the aggregate results made public. In a study of the nation’s largest urban school districts , students took an average of 112 standardized tests between pre-K and grade 12.
This annual testing ritual can take time from genuine learning, say many educators , and puts pressure on the least advantaged districts to focus on test prep—not to mention adding airless, stultifying hours of proctoring to teachers’ lives. “Tests don’t explicitly teach anything. Teachers do,” writes Jose Vilson , a middle school math teacher in New York City. Instead of standardized tests, students “should have tests created by teachers with the goal of learning more about the students’ abilities and interests,” echoes Meena Negandhi, math coordinator at the French American Academy in Jersey City, New Jersey.
The pushback on high-stakes testing has also accelerated a national conversation about how students truly learn and retain information. Over the past decade and a half, educators have been moving away from traditional testing —particularly multiple choice tests—and turning to hands-on projects and competency-based assessments that focus on goals such as critical thinking and mastery rather than rote memorization.
But educators shouldn’t give up on traditional classroom tests so quickly. Research has found that tests can be valuable tools to help students learn , if designed and administered with format, timing, and content in mind—and a clear purpose to improve student learning.
Not All Tests Are Bad
One of the most useful kinds of tests are the least time-consuming: quick, easy practice quizzes on recently taught content. Tests can be especially beneficial if they are given frequently and provide near-immediate feedback to help students improve. This retrieval practice can be as simple as asking students to write down two to four facts from the prior day or giving them a brief quiz on a previous class lesson.
Retrieval practice works because it helps students retain information in a better way than simply studying material, according to research . While reviewing concepts can help students become more familiar with a topic, information is quickly forgotten without more active learning strategies like frequent practice quizzes.
But to reduce anxiety and stereotype threat—the fear of conforming to a negative stereotype about a group that one belongs to—retrieval-type practice tests also need to be low-stakes (with minor to no grades) and administered up to three times before a final summative effort to be most effective.
Timing also matters. Students are able to do fine on high-stakes assessment tests if they take them shortly after they study. But a week or more after studying, students retain much less information and will do much worse on major assessments—especially if they’ve had no practice tests in between.
A 2006 study found that students who had brief retrieval tests before a high-stakes test remembered 60 percent of material, while those who only studied remembered 40 percent. Additionally, in a 2009 study , eighth graders who took a practice test halfway through the year remembered 10 percent more facts on a U.S. history final at the end of the year than peers who studied but took no practice test.
Short, low-stakes tests also help teachers gauge how well students understand the material and what they need to reteach. This is effective when tests are formative —that is, designed for immediate feedback so that students and teachers can see students’ areas of strength and weakness and address areas for growth. Summative tests, such as a final exam that measures how much was learned but offers no opportunities for a student to improve, have been found to be less effective.
Testing Format Matters
Teachers should tread carefully with test design, however, as not all tests help students retain information. Though multiple choice tests are relatively easy to create, they can contain misleading answer choices—that are either ambiguous or vague—or offer the infamous all-, some-, or none-of-the-above choices, which tend to encourage guessing.
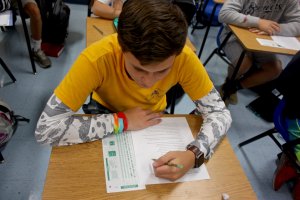
While educators often rely on open-ended questions, such short-answer questions, because they seem to offer a genuine window into student thinking, research shows that there is no difference between multiple choice and constructed response questions in terms of demonstrating what students have learned.
In the end, well-constructed multiple choice tests , with clear questions and plausible answers (and no all- or none-of-the-above choices), can be a useful way to assess students’ understanding of material, particularly if the answers are quickly reviewed by the teacher.
All students do not do equally well on multiple choice tests, however. Girls tend to do less well than boys and perform better on questions with open-ended answers , according to a 2018 study by Stanford University’s Sean Reardon, which found that test format alone accounts for 25 percent of the gender difference in performance in both reading and math. Researchers hypothesize that one explanation for the gender difference on high-stakes tests is risk aversion, meaning girls tend to guess less .
Giving more time for fewer, more complex or richer testing questions can also increase performance, in part because it reduces anxiety. Research shows that simply introducing a time limit on a test can cause students to experience stress, so instead of emphasizing speed, teachers should encourage students to think deeply about the problems they’re solving.
Setting the Right Testing Conditions
Test achievement often reflects outside conditions, and how students do on tests can be shifted substantially by comments they hear and what they receive as feedback from teachers.
When teachers tell disadvantaged high school students that an upcoming assessment may be a challenge and that challenge helps the brain grow, students persist more, leading to higher grades, according to 2015 research from Stanford professor David Paunesku. Conversely, simply saying that some students are good at a task without including a growth-mindset message or the explanation that it’s because they are smart harms children’s performance —even when the task is as simple as drawing shapes.
Also harmful to student motivation are data walls displaying student scores or assessments. While data walls might be useful for educators, a 2014 study found that displaying them in classrooms led students to compare status rather than improve work.
The most positive impact on testing comes from peer or instructor comments that give the student the ability to revise or correct. For example, questions like , “Can you tell me more about what you mean?” or “Can you find evidence for that?” can encourage students to improve engagement with their work. Perhaps not surprisingly, students do well when given multiple chances to learn and improve—and when they’re encouraged to believe that they can.
Created by the Great Schools Partnership , the GLOSSARY OF EDUCATION REFORM is a comprehensive online resource that describes widely used school-improvement terms, concepts, and strategies for journalists, parents, and community members. | Learn more »

Summative Assessment
Summative assessments are used to evaluate student learning, skill acquisition, and academic achievement at the conclusion of a defined instructional period—typically at the end of a project, unit, course, semester, program, or school year. Generally speaking, summative assessments are defined by three major criteria:
- The tests, assignments, or projects are used to determine whether students have learned what they were expected to learn. In other words, what makes an assessment “summative” is not the design of the test, assignment, or self-evaluation, per se, but the way it is used—i.e., to determine whether and to what degree students have learned the material they have been taught.
- Summative assessments are given at the conclusion of a specific instructional period, and therefore they are generally evaluative, rather than diagnostic—i.e., they are more appropriately used to determine learning progress and achievement, evaluate the effectiveness of educational programs, measure progress toward improvement goals, or make course-placement decisions, among other possible applications.
- Summative-assessment results are often recorded as scores or grades that are then factored into a student’s permanent academic record, whether they end up as letter grades on a report card or test scores used in the college-admissions process. While summative assessments are typically a major component of the grading process in most districts, schools, and courses, not all assessments considered to be summative are graded.
Summative assessments are commonly contrasted with formative assessments , which collect detailed information that educators can use to improve instruction and student learning while it’s happening. In other words, formative assessments are often said to be for learning, while summative assessments are of learning. Or as assessment expert Paul Black put it, “When the cook tastes the soup, that’s formative assessment. When the customer tastes the soup, that’s summative assessment.” It should be noted, however, that the distinction between formative and summative is often fuzzy in practice, and educators may have divergent interpretations and opinions on the subject.
Some of the most well-known and widely discussed examples of summative assessments are the standardized tests administered by states and testing organizations, usually in math, reading, writing, and science. Other examples of summative assessments include:
- End-of-unit or chapter tests.
- End-of-term or semester tests.
- Standardized tests that are used to for the purposes of school accountability, college admissions (e.g., the SAT or ACT), or end-of-course evaluation (e.g., Advanced Placement or International Baccalaureate exams).
- Culminating demonstrations of learning or other forms of “performance assessment,” such as portfolios of student work that are collected over time and evaluated by teachers or capstone projects that students work on over extended periods of time and that they present and defend at the conclusion of a school year or their high school education.
While most summative assessments are given at the conclusion of an instructional period, some summative assessments can still be used diagnostically. For example, the growing availability of student data, made possible by online grading systems and databases, can give teachers access to assessment results from previous years or other courses. By reviewing this data, teachers may be able to identify students more likely to struggle academically in certain subject areas or with certain concepts. In addition, students may be allowed to take some summative tests multiple times, and teachers might use the results to help prepare students for future administrations of the test.
It should also be noted that districts and schools may use “interim” or “benchmark” tests to monitor the academic progress of students and determine whether they are on track to mastering the material that will be evaluated on end-of-course tests or standardized tests. Some educators consider interim tests to be formative, since they are often used diagnostically to inform instructional modifications, but others may consider them to be summative. There is ongoing debate in the education community about this distinction, and interim assessments may defined differently from place to place. See formative assessment for a more detailed discussion.
While educators have arguably been using “summative assessments” in various forms since the invention of schools and teaching, summative assessments have in recent decades become components of larger school-improvement efforts. As they always have, summative assessments can help teachers determine whether students are making adequate academic progress or meeting expected learning standards, and results may be used to inform modifications to instructional techniques, lesson designs, or teaching materials the next time a course, unit, or lesson is taught. Yet perhaps the biggest changes in the use of summative assessments have resulted from state and federal policies aimed at improving public education—specifically, standardized high-stakes tests used to make important decisions about schools, teachers, and students.
While there is little disagreement among educators about the need for or utility of summative assessments, debates and disagreements tend to center on issues of fairness and effectiveness, especially when summative-assessment results are used for high-stakes purposes. In these cases, educators, experts, reformers, policy makers, and others may debate whether assessments are being designed and used appropriately, or whether high-stakes tests are either beneficial or harmful to the educational process. For more detailed discussions of these issues, see high-stakes test , measurement error , test accommodations , test bias , score inflation , standardized test , and value-added measures .

Alphabetical Search
- Reference Manager
- Simple TEXT file
People also looked at
Conceptual analysis article, the past, present and future of educational assessment: a transdisciplinary perspective.
- 1 Department of Applied Educational Sciences, Umeå Universitet, Umeå, Sweden
- 2 Faculty of Education and Social Work, The University of Auckland, Auckland, New Zealand
To see the horizon of educational assessment, a history of how assessment has been used and analysed from the earliest records, through the 20th century, and into contemporary times is deployed. Since paper-and-pencil assessments validity and integrity of candidate achievement has mattered. Assessments have relied on expert judgment. With the massification of education, formal group-administered testing was implemented for qualifications and selection. Statistical methods for scoring tests (classical test theory and item response theory) were developed. With personal computing, tests are delivered on-screen and through the web with adaptive scoring based on student performance. Tests give an ever-increasing verisimilitude of real-world processes, and analysts are creating understanding of the processes test-takers use. Unfortunately testing has neglected the complicating psychological, cultural, and contextual factors related to test-taker psychology. Computer testing neglects school curriculum and classroom contexts, where most education takes place and where insights are needed by both teachers and learners. Unfortunately, the complex and dynamic processes of classrooms are extremely difficult to model mathematically and so remain largely outside the algorithms of psychometrics. This means that technology, data, and psychometrics have become increasingly isolated from curriculum, classrooms, teaching, and the psychology of instruction and learning. While there may be some integration of these disciplines within computer-based testing, this is still a long step from where classroom assessment happens. For a long time, educational, social, and cultural psychology related to learning and instruction have been neglected in testing. We are now on the cusp of significant and substantial development in educational assessment as greater emphasis on the psychology of assessment is brought into the world of testing. Herein lies the future for our field: integration of psychological theory and research with statistics and technology to understand processes that work for learning, identify how well students have learned, and what further teaching and learning is needed. The future requires greater efforts by psychometricians, testers, data analysts, and technologists to develop solutions that work in the pressure of living classrooms and that support valid and reliable assessment.
Introduction
In looking to the horizon of educational assessment, I would like to take a broad chronological view of where we have come from, where we are now, and what the horizons are. Educational assessment plays a vital role in the quality of student learning experiences, teacher instructional activities, and evaluation of curriculum, school quality, and system performance. Assessments act as a lever for both formative improvement of teaching and learning and summative accountability evaluation of teachers, schools, and administration. Because it is so powerful, a nuanced understanding of its history, current status, and future possibilities seems a useful exercise. In this overview I begin with a brief historical journey from assessments past through the last 3000 years and into the future that is already taking place in various locations and contexts.
Early records of the Chinese Imperial examination system can be found dating some 2,500 to 3,000 years ago ( China Civilisation Centre, 2007 ). That system was used to identify and reward talent wherever it could be found in the sprawling empire of China. Rather than rely solely on recommendations, bribery, or nepotism, it was designed to meritocratically locate students with high levels of literacy and memory competencies to operate the Emperor’s bureaucracy of command and control of a massive population. To achieve those goals, the system implemented standardised tasks (e.g., completing an essay according to Confucian principles) under invigilated circumstances to ensure integrity and comparability of performances ( Feng, 1995 ). The system had a graduated series of increasingly more complex and demanding tests until at the final examination no one could be awarded the highest grade because it was reserved for the Emperor alone. Part of the rationale for this extensive technology related to the consequences attached to selection; not only did successful candidates receive jobs with substantial economic benefits, but they were also recognised publicly on examination lists and by the right to wear specific colours or badges that signified the level of examination the candidate had passed. Unsurprisingly, given the immense prestige and possibility of social advancement through scholarship, there was an industry of preparing cheat materials (e.g., miniature books that replicated Confucian classics) and catching cheats (e.g., ranks of invigilators in high chairs overlooking desks at which candidates worked; Elman, 2013 ).
In contrast, as described by Encyclopedia Brittanica (2010a) , European educational assessment grew out of the literary and oratorical remains of the Roman empire such as schools of grammarians and rhetoricians. At the same time, schools were formed in the various cathedrals, monasteries (especially, the Benedictine monasteries), and episcopal schools throughout Europe. Under Charlemagne, church priests were required to master Latin so that they could understand scripture correctly, leading to more advanced religious and academic training. As European society developed in the early Renaissance, schools were opened under the authority of a bishop or cathedral officer or even from secular guilds to those deemed sufficiently competent to teach. Students and teachers at these schools were given certain protection and rights to ensure safe travel and free thinking. European universities from the 1100s adopted many of the clerical practices of reading important texts and scholars evaluating the quality of learning by student performance in oral disputes, debates, and arguments relative to the judgement of higher ranked experts. The subsequent centuries added written tasks and performances to the oral disputes as a way of judging the quality of learning outcomes. Nonetheless, assessment was based, as the Chinese Imperial system, on the expertise and judgment of more senior scholars or bureaucrats.
These mechanisms were put in place to meet the needs of society or religion for literate and numerate bureaucrats, thinkers, and scholars. The resource of further education, or even basic education, was generally rationed and limited. Standardised assessments, even if that were only the protocol rather than the task or the scoring, were carried out to select candidates on a relatively meritocratic basis. Families and students engaged in these processes because educational success gave hope of escape from lives of poverty and hard labour. Consequently, assessment was fundamentally a summative judgement of the student’s abilities, schooling was preparation for the final examination, and assessments during the schooling process were but mimicry of a final assessment.
With the expansion of schooling and higher education through the 1800s, more efficient methods were sought to the workload surrounding hearing memorized recitations ( Encyclopedia Brittanica, 2010b ). This led to the imposition of leaving examinations as an entry requirement to learned professions (e.g., being a teacher), the civil service, and university studies. As more and more students attended universities in the 1800s, more efficient ways collecting information were established, most especially the essay examination and the practice of answering in writing by oneself without aids. This tradition can still be seen in ordered rows of desks in examination halls as students complete written exam papers under scrutiny and time pressure.
The 20th century
By the early 1900s, however, it became apparent that the scoring of these important intellectual exercises was highly problematic. Markers did not agree with each other nor were they consistent within themselves across items or tasks and over time so that their scores varied for the same work. Consequently, early in the 20th century, multiple-choice question tests were developed so that there would be consistency in scoring and efficiency in administration ( Croft and Beard, 2022 ). It is also worth noting that considerable cost and time efficiencies were obtained through using multiple-choice test methods. This aspect led, throughout the century, to increasingly massive use of standardised machine scoreable tests for university entrance, graduate school selection, and even school evaluation. The mechanism of scoring items dichotomously (i.e., right or wrong), within classical test theory statistical modelling, resulted in easy and familiar numbers (e.g., mean, standard deviation, reliability, and standard error of measurement; Clauser, 2022 ).
As the 20th century progressed, the concepts of validity have grown increasingly expansive, and the methods of validation have become increasingly complex and multi-faceted to ensure validity of scores and their interpretation ( Zumbo and Chan, 2014 ). These included scale reliability, factor analysis, item response theory, equating, norming, and standard setting, among others ( Kline, 2020 ). It is worth noting here that statistical methods for test score analysis grew out of the early stages of the discipline of psychology. As psychometric methods became increasingly complex, the world of educational testing began to look much more like the world of statistics. Indeed, Cronbach (1954) noted that the world of psychometrics (i.e., statistical measurement of psychological phenomena) was losing contact with the world of psychology which was the most likely user of psychometric method and research. Interestingly, the world of education makes extensive use of assessment, but few educators are adept at the statistical methods necessary to evaluate their own tests, let alone those from central authorities. Indeed, few teachers are taught statistical test analysis techniques, even fewer understand them, and almost none make use of them.
Of course, assessment is not just a scored task or set of questions. It is legitimately an attempt to operationalize a sample of a construct or content or curriculum domain. The challenge for assessment lies in the conundrum that the material that is easy to test and score tends to be the material that is the least demanding or valuable in any domain. Learning objectives for K-12 schooling, let alone higher education, expect students to go beyond remembering, recalling, regurgitating lists of terminology, facts, or pieces of data. While recall of data pieces is necessary for deep processing, recall of those details is not sufficient. Students need to exhibit complex thinking, problem-solving, creativity, and analysis and synthesis. Assessment of such skills is extremely complex and difficult to achieve.
However, with the need to demonstrate that teachers are effective and that schools are achieving society’s goals and purposes it becomes easy to reduce the valued objectives of society to that which can be incorporated efficiently into a standardised test. Hence, in many societies the high-stakes test becomes the curriculum. If we could be sure that what was on the test is what society really wanted, this would not be such a bad thing; what Resnick and Resnick (1989) called measurement driven reform. However, research over extensive periods since the middle of the 20 th century has shown that much of what we test does not add value to the learning of students ( Nichols and Harris, 2016 ).
An important development in the middle of the 20th century was Scriven’s (1967) work on developing the principles and philosophy of evaluation. A powerful aspect to evaluation that he identified was the distinction between formative evaluation taking place early enough in a process to make differences to the end points of the process and summative evaluation which determined the amount and quality or merit of what the process produced. The idea of formative evaluation was quickly adapted into education as a way of describing assessments that teachers used within classrooms to identify which children needed to be taught what material next ( Bloom et al., 1971 ). This contrasted nicely with high-stakes end-of-unit, end-of-course, or end-of-year formal examinations that summatively judged the quality of student achievement and learning. While assessment as psychometrically validated tests and examinations historically focused on the summative experience, Scriven’s formative evaluation led to using assessment processes early in the educational course of events to inform learners as to what they needed to learn and instructors as to what they needed to teach.
Nonetheless, since the late 1980s (largely thanks to Sadler, 1989 ) the distinction between summative and formative transmogrified from timing to one of type. Formative assessments began to be only those which were not formal tests but were rather informal interactions in classrooms. This perspective was extended by the UK Assessment Reform Group (2002) which promulgated basic principles of formative assessment around the world. Those classroom assessment practices focused much more on what could be seen as classroom teaching practices ( Brown, 2013 , 2019 , 2020a ). Instead of testing, teachers interacted with students on-the-fly, in-the-moment of the classroom through questions and feedback that aimed to help students move towards the intended learning outcomes established at the beginning of lessons or courses. Thus, assessment for learning has become a child-friendly approach ( Stobart, 2006 ) to involving learners in their learning and developing rich meaningful outcomes without the onerous pressure of testing. Much of the power of this approach was that it came as an alternative to the national curriculum of England and Wales that incorporated high-stakes standardised assessment tasks of children at ages 7, 9, 11, and 14 (i.e., Key Stages 1 to 4; Wherrett, 2004 ).
In line with increasing access to schooling worldwide throughout the 20 th century, there is concern that success on high-consequence, summative tests simply reinforced pre-existing social status and hierarchy ( Bourdieu, 1974 ). This position argues tests are not neutral but rather tools of elitism ( Gipps, 1994 ). Unfortunately, when assessments have significant consequences, much higher proportions of disadvantaged students (e.g., minority students, new speakers of the language-medium of assessment, special needs students, those with reading difficulties, etc.) do not experience such benefits ( Brown, 2008 ). This was a factor in the development of using assessment high-quality formative assessment to accelerate the learning progression of disadvantaged students. Nonetheless, differences in group outcomes do not always mean tests are the problem; group score differences can point out that there is sociocultural bias in the provision of educational resources in the school system ( Stobart, 2005 ). This would be rationale for system monitoring assessments, such as Hong Kong’s Territory Wide System Assessment, 1 the United States’ National Assessment of Educational Progress, 2 or Australia’s National Assessment Program Literacy and Numeracy. 3 The challenge is how to monitor a system without blaming those who have been let down by it.
Key Stage tests were put in place, not only to evaluate student learning, but also to assure the public that teachers and schools were achieving important goals of education. This use of assessment put focus on accountability, not for the student, but for the school and teacher ( Nichols and Harris, 2016 ). The decision to use tests of student learning to evaluate schools and teachers was mimicked, especially in the United States, in various state accountability tests, the No Child Left Behind legislation, and even such innovative programs of assessment as Race to the Top and PARCC. It should be noted that the use of standardised tests to evaluate teachers and schools is truly a global phenomenon, not restricted to the UK and the USA ( Lingard and Lewis, 2016 ). In this context, testing became a summative evaluation of teachers and school leaders to demonstrate school effectiveness and meet accountability requirements.
The current situation is that assessment is perceived quite differently by experts in different disciplines. Psychometricians tend to define assessment in terms of statistical modelling of test scores. Psychologists use assessments for diagnostic description of client strengths or needs. Within schooling, leaders tend to perceive assessment as jurisdiction or state-mandated school accountability testing, while teachers focus on assessment as interactive, on-the-fly experiences with their students, and parents ( Buckendahl, 2016 ; Harris and Brown, 2016 ) understand assessment as test scores and grades. The world of psychology has become separated from the worlds of classroom teaching, curriculum, psychometrics and statistics, and assessment technologies.
This brief history bringing us into early 21 st century shows that educational assessment is informed by multiple disciplines which often fail to talk with or even to each other. Statistical analysis of testing has become separated from psychology and education, psychology is separated from curriculum, teaching is separated from testing, and testing is separated from learning. Hence, we enter the present with many important facets that inform effective use of educational assessment siloed from one another.
Now and next
Currently the world of educational statistics has become engrossed in the large-scale data available through online testing and online learning behaviours. The world of computational psychometrics seeks to move educational testing statistics into the dynamic analysis of big data with machine learning and artificial intelligence algorithms potentially creating a black box of sophisticated statistical models (e.g., neural networks) which learners, teachers, administrators, and citizens cannot understand ( von Davier et al., 2019 ). The introduction of computing technologies means that automation of item generation ( Gierl and Lai, 2016 ) and scoring of performances ( Shin et al., 2021 ) is possible, along with customisation of test content according to test-taker performance ( Linden and Glas, 2000 ). The Covid-19 pandemic has rapidly inserted online and distance testing as a commonplace practice with concerns raised about how technology is used to assure the integrity of student performance ( Dawson, 2021 ).
The ecology of the classroom is not the same as that of a computerised test. This is especially notable when the consequence of a test (regardless of medium) has little relevance to a student ( Wise and Smith, 2016 ). Performance on international large-scale assessments (e.g., PISA, TIMSS) may matter to government officials ( Teltemann and Klieme, 2016 ) but these tests have little value for individual learners. Nonetheless, governmental responses to PISA or TIMSS results may create policies and initiatives that have trickle-down effect on schools and students ( Zumbo and Forer, 2011 ). Consequently, depending on the educational and cultural environment, test-taking motivation on tests that have consequences for the state can be similar to a test with personal consequence in East Asia ( Zhao et al., 2020 ), but much lower in a western democracy ( Zhao et al., 2022 ). Hence, without surety that in any educational test learners are giving full effort ( Thorndike, 1924 ), the information generated by psychometric analysis is likely to be invalid. Fortunately, under computer testing conditions, it is now possible to monitor reduced or wavering effort during an actual test event and provide support to such a student through a supervising proctor ( Wise, 2019 ), though this feature is not widely prevalent.
Online or remote teaching, learning, and assessment have become a reality for many teachers and students, especially in light of our educational responses to the Covid-19 pandemic. Clearly, some families appreciate this because their children can progress rapidly, unencumbered by the teacher or classmates. For such families, continuing with digital schooling would be seen as a positive future. However, reliance on a computer interface as the sole means of assessment or teaching may dehumanise the very human experience of learning and teaching. As Asimov (1954) described in his short story of a future world in which children are taught individually by machines, Margie imagined what it must have been like to go to school with other children:
Margie …was thinking about the old schools they had when her grandfather's grandfather was a little boy. All the kids from the whole neighborhood came, laughing and shouting in the schoolyard, sitting together in the schoolroom, going home together at the end of the day. They learned the same things so they could help one another on the homework and talk about it.
And the teachers were people...
The mechanical teacher was flashing on the screen: "When we add the fractions ½ and ¼ -"
Margie was thinking about how the kids must have loved it in the old days. She was thinking about the fun they had.
As Brown (2020b) has argued the option of a de-schooled society through computer-based teaching, learning, and assessment is deeply unattractive on the grounds that it is likely to be socially unjust. The human experience of schooling matters to the development of humans. We learn through instruction ( Bloom, 1976 ), culturally located experiences ( Cole et al., 1971 ), inter-personal interaction with peers and adults ( Vygotsky, 1978 ; Rogoff, 1991 ), and biogenetic factors ( Inhelder and Piaget, 1958 ). Schooling gives us access to environments in which these multiple processes contribute to the kinds of citizens we want. Hence, we need confidence in the power of shared schooling to do more than increase the speed by which children acquire knowledge and learning; it helps us be more human.
This dilemma echoes the tension between in vitro and in vivo biological research. Within the controlled environment of a test tube (vitro) organisms do not necessarily behave the same way as they do when released into the complexity of human biology ( Autoimmunity Research Foundation, 2012 ). This analogy has been applied to educational assessment ( Zumbo, 2015 ) indicating that how students perform in a computer-mediated test may not have validity for how students perform in classroom interactions or in-person environments.
The complexity of human psychology is captured in Hattie’s (2004) ROPE model which posits that the various aspects of human motivation, belief, strategy, and values interact as threads spun into a rope. This means it is hard to analytically separate the various components and identify aspects that individually explain learning outcomes. Indeed, Marsh et al. (2006) showed that of the many self-concept and control beliefs used to predict performance on the PISA tests, almost all variables have relations to achievement less than r = 0.35. Instead, interactions among motivation, beliefs about learning, intelligence, assessment, the self, and attitudes with and toward others, subjects, and behaviours all matter to performance. Aspects that create growth-oriented pathways ( Boekaerts and Niemivirta, 2000 ) and strategies include inter alia mastery goals ( Deci and Ryan, 2000 ), deep learning ( Biggs et al., 2001 ) beliefs, malleable intelligence ( Duckworth et al., 2011 ) beliefs, improvement-oriented beliefs about assessment ( Brown, 2011 ), internal, controllable attributes ( Weiner, 1985 ), effort ( Wise and DeMars, 2005 ), avoiding dishonesty ( Murdock et al., 2016 ), trusting one’s peers ( Panadero, 2016 ), and realism in evaluating one’s own work ( Brown and Harris, 2014 ). All these adaptive aspects of learning stand in contrast to deactivating and maladaptive beliefs, strategies, and attitudes that serve to protect the ego and undermine learning. What this tells us that psychological research matters to understanding the results of assessment and that no one single psychological construct is sufficient to explain very much of the variance in student achievement. However, it seems we are as yet unable to identify which specific processes matter most to better performance for all students across the ability spectrum, given that almost all the constructs that have been reported in educational psychology seem to have a positive contribution to better performance. Here is the challenge for educational psychology within an assessment setting —which constructs are most important and effectual before, during, and after any assessment process ( Mcmillan, 2016 ) and how should they be operationalised.
A current enthusiasm is to use ‘big data’ from computer-based assessments to examine in more detail how students carry out the process of responding to tasks. Many large-scale testing programs through computer testing collect, utilize, and report on test-taker engagement as part of their process data collection (e.g., the United States National Assessment of Educational Progress 4 ). These test systems provide data about what options were clicked on, in what order, what pages were viewed, and the timings of these actions. Several challenges to using big data in educational assessment exist. First, computerised assessments need to capture the processes and products we care about. That means we need a clear theoretical model of the underlying cognitive mechanisms or processes that generate the process data itself ( Zumbo et al., in press ). Second, we need to be reminded that data do not explain themselves; theory and insight about process are needed to understand data ( Pearl and Mackenzie, 2018 ). Examination of log files can give some insight into effective vs. ineffective strategies, once the data were analysed using theory to create a model of how a problem should be done ( Greiff et al., 2015 ). Access to data logs that show effort and persistence on a difficult task can reveal that, despite failure to successfully resolve a problem, such persistence is related to overall performance ( Lundgren and Eklöf, 2020 ). But data by itself will not tell us how and why students are successful and what instruction might need to do to encourage students to use the scientific method of manipulating one variable at a time or not giving up quickly.
Psychometric analyses of assessments can only statistically model item difficulty, item discrimination, and item chance parameters to estimate person ability ( Embretson and Reise, 2000 ). None of the other psychological features of how learners relate to themselves and their environment are included in score estimation. In real classroom contexts, teachers make their best efforts to account for individual motivation, affect, and cognition to provide appropriate instruction, feedback, support, and questioning. However, the nature of these factors varies across time (cohorts), locations (cultures and societies), policy priorities for schooling and assessment, and family values ( Brown and Harris, 2009 ). This means that what constitutes a useful assessment to inform instruction in a classroom context (i.e., identify to the teacher who needs to be taught what next) needs to constantly evolve and be incredibly sensitive to individual and contextual factors. This is difficult if we keep psychology, curriculum, psychometrics, and technology in separate silos. It seems highly desirable that these different disciplines interact, but it is not guaranteed that the technology for psychometric testing developments will cross-pollinate with classroom contexts where teachers have to relate to and monitor student learning across all important curricular domains.
It is common to treat what happens in the minds and emotions of students when they are assessed as a kind of ‘black box’ implying that the processes are opaque or unknowable. This is an approach I have taken previously in examining what students do when asked to self-assess ( Yan and Brown, 2017 ). However, the meaning of a black box is quite different in engineering. In aeronautics, the essential constructs related to flight (e.g., engine power, aileron settings, pitch and yaw positions, etc.) are known very deeply, otherwise flight would not happen. The black box in an airplane records the values of those important variables and the only thing unknown (i.e., black) is what the values were at the point of interest. If we are to continue to use this metaphor as a way of understanding what happens when students are assessed or assess, then we need to agree on what the essential constructs are that underlie learning and achievement. Our current situation seems to be satisfied with everything is correlated and everything matters. It may be that data science will help us sort through the chaff for the wheat provided we design and implement sensors appropriate to the constructs we consider hypothetically most important. It may be that measuring timing of mouse clicks and eye tracking do connect to important underlying mechanisms, but at this stage data science in testing seems largely a case of crunch the ‘easy to get’ numbers and hope that the data mean something.
To address this concern, we need to develop for education’s sake, assessments that have strong alignment with curricular ambitions and values and which have applicability to classroom contexts and processes ( Bennett, 2018 ). This will mean technology that supports what humans must do in schooling rather than replace them with teaching/testing machines. Fortunately, some examples of assessment technology for learning do exist. One supportive technology is PeerWise ( Denny et al., 2008 ; Hancock et al., 2018 ) in which students create course related multiple-choice questions and use them as a self-testing learning strategy. A school-based technology is the e-asTTle computer assessment system that produces a suite of diagnostic reports to support teachers’ planning and teaching in response to what the system indicated students need to be taught ( Hattie and Brown, 2008 ; Brown and Hattie, 2012 ; Brown et al., 2018 ). What these technologies do is support rather than supplant the work that teachers and learners need to do to know what they need to study or teach and to monitor their progress. Most importantly they are well-connected to what students must learn and what teachers are teaching. Other detailed work uses organised learning models or dynamic learning maps to mark out routes for learners and teachers using cognitive and curriculum insights with psychometric tools for measuring status and progress ( Kingston et al., 2022 ). The work done by Wise (2019) shows that it is possible in a computer assisted testing environment to monitor student effort based on their speed of responding and give prompts that support greater effort and less speed.
Assessment needs to exploit more deeply the insights educational psychology has given us into human behavior, attitudes, inter- and intra-personal relations, emotions, and so on. This was called for some 20 years ago ( National Research Council, 2001 ) but the underlying disciplines that inform this integration seem to have grown away from each other. Nonetheless, the examples given above suggest that the gaps can be closed. But assessments still do not seem to consider and respond to these psychological determinants of achievement. Teachers have the capability of integrating curriculum, testing, psychology, and data at a superficial level but with some considerable margin of error ( Meissel et al., 2017 ). To overcome their own error, teachers need technologies that support them in making useful and accurate interpretations of what students need to be taught next that work with them in the classroom. As Bennett (2018) pointed out more technology will happen, but perhaps not more tests on computers. This is the assessment that will help teachers rather than replace them and give us hope for a better future.
Author contributions
GB wrote this manuscript and is solely responsible for its content.
Support for the publication of this paper was received from the Publishing and Scholarly Services of the Umeå University Library.
Acknowledgments
A previous version of this paper was presented as a keynote address to the 2019 biennial meeting of the European Association for Research in Learning and Instruction, with the title Products, Processes, Psychology, and Technology: Quo Vadis Educational Assessment ?
Conflict of interest
The author declares that the research was conducted in the absence of any commercial or financial relationships that could be construed as a potential conflict of interest.
Publisher’s note
All claims expressed in this article are solely those of the authors and do not necessarily represent those of their affiliated organizations, or those of the publisher, the editors and the reviewers. Any product that may be evaluated in this article, or claim that may be made by its manufacturer, is not guaranteed or endorsed by the publisher.
1. ^ https://www.hkeaa.edu.hk/en/sa_tsa/tsa/
2. ^ https://www.nationsreportcard.gov/
3. ^ https://nap.edu.au/
4. ^ https://www.nationsreportcard.gov/process_data/
Asimov, I. (1954). Oh the fun they had. Fantasy Sci. Fiction 6, 125–127.
Google Scholar
Assessment Reform Group (2002). Assessment for learning: 10 principles Research-based Principles to Guide Classroom Practice Cambridge: Assessment Reform Group.
Autoimmunity Research Foundation. (2012). Differences between in vitro, in vivo, and in silico studies [online]. The Marshall Protocol Knowledge Base. Available at: http://mpkb.org/home/patients/assessing_literature/in_vitro_studies (Accessed November 12, 2015).
Bennett, R. E. (2018). Educational assessment: what to watch in a rapidly changing world. Educ. Meas. Issues Pract. 37, 7–15. doi: 10.1111/emip.12231
CrossRef Full Text | Google Scholar
Biggs, J., Kember, D., and Leung, D. Y. (2001). The revised two-factor study process questionnaire: R-SPQ-2F. Br. J. Educ. Psychol. 71, 133–149. doi: 10.1348/000709901158433
PubMed Abstract | CrossRef Full Text | Google Scholar
Bloom, B. S. (1976). Human Characteristics and School Learning . New York: McGraw-Hill.
Bloom, B., Hastings, J., and Madaus, G. (1971). Handbook on Formative and Summative Evaluation of Student Learning . New York:McGraw Hill.
Boekaerts, M., and Niemivirta, M. (2000). “Self-regulated learning: finding a balance between learning goals and ego-protective goals,” in Handbook of Self-regulation . eds. M. Boekaerts, P. R. Pintrich, and M. Zeidner (San Diego, CA: Academic Press).
Bourdieu, P. (1974). “The school as a conservative force: scholastic and cultural inequalities,” in Contemporary Research in the Sociology of Education . ed. J. Eggleston (London: Methuen).
Brown, G. T. L. (2008). Conceptions of Assessment: Understanding what Assessment Means to Teachers and Students . New York: Nova Science Publishers.
Brown, G. T. L. (2011). Self-regulation of assessment beliefs and attitudes: a review of the Students' conceptions of assessment inventory. Educ. Psychol. 31, 731–748. doi: 10.1080/01443410.2011.599836
Brown, G. T. L. (2013). “Assessing assessment for learning: reconsidering the policy and practice,” in Making a Difference in Education and Social Policy . eds. M. East and S. May (Auckland, NZ: Pearson).
Brown, G. T. L. (2019). Is assessment for learning really assessment? Front. Educ. 4:64. doi: 10.3389/feduc.2019.00064
Brown, G. T. L. (2020a). Responding to assessment for learning: a pedagogical method, not assessment. N. Z. Annu. Rev. Educ. 26, 18–28. doi: 10.26686/nzaroe.v26.6854
Brown, G. T. L. (2020b). Schooling beyond COVID-19: an unevenly distributed future. Front. Educ. 5:82. doi: 10.3389/feduc.2020.00082
Brown, G. T. L., and Harris, L. R. (2009). Unintended consequences of using tests to improve learning: how improvement-oriented resources heighten conceptions of assessment as school accountability. J. MultiDisciplinary Eval. 6, 68–91.
Brown, G. T. L., and Harris, L. R. (2014). The future of self-assessment in classroom practice: reframing self-assessment as a core competency. Frontline Learn. Res. 3, 22–30. doi: 10.14786/flr.v2i1.24
Brown, G. T. L., O'leary, T. M., and Hattie, J. A. C. (2018). “Effective reporting for formative assessment: the asTTle case example,” in Score Reporting: Research and Applications . ed. D. Zapata-Rivera (New York: Routledge).
Brown, G. T., and Hattie, J. (2012). “The benefits of regular standardized assessment in childhood education: guiding improved instruction and learning,” in Contemporary Educational Debates in Childhood Education and Development . eds. S. Suggate and E. Reese (New York: Routledge).
Buckendahl, C. W. (2016). “Public perceptions about assessment in education,” in Handbook of Human and Social Conditions in Assessment . eds. G. T. L. Brown and L. R. Harris (New York: Routledge).
China Civilisation Centre (2007). China: Five Thousand Years of History and Civilization . Hong Kong: City University of Hong Kong Press.
Clauser, B. E. (2022). “A history of classical test theory,” in The History of Educational Measurement: Key Advancements in Theory, Policy, and Practice . eds. B. E. Clauser and M. B. Bunch (New York: Routledge).
Cole, M., Gay, J., Glick, J., and Sharp, D. (1971). The Cultural Context of Learning and Thinking: An Exploration in Experimental Anthropology . New York: Basic Books.
Croft, M., and Beard, J. J. (2022). “Development and evolution of the SAT and ACT,” in The History of Educational Measurement: Key Advancements in Theory, Policy, and Practice . eds. B. E. Clauser and M. B. Bunch (New York: Routledge).
Cronbach, L. J. (1954). Report on a psychometric mission to Clinicia. Psychometrika 19, 263–270. doi: 10.1007/BF02289226
Dawson, P. (2021). Defending Assessment Security in a Digital World: Preventing e-cheating and Supporting Academic Integrity in Higher Education . London: Routledge.
Deci, E. L., and Ryan, R. (2000). Self-determination theory and the facilitation of intrinsic motivation, social development, and well-being. Am. Psychol. 55, 68–78.
Denny, P., Hamer, J., Luxton-Reilly, A., and Purchase, H. PeerWise: students sharing their multiple choice questions. ICER '08: Proceedings of the Fourth international Workshop on Computing Education Research; September6–7 (2008). Sydney, Australia, 51-58.
Duckworth, A. L., Quinn, P. D., and Tsukayama, E. (2011). What no child left behind leaves behind: the roles of IQ and self-control in predicting standardized achievement test scores and report card grades. J. Educ. Psychol. 104, 439–451. doi: 10.1037/a0026280
Elman, B. A. (2013). Civil Examinations and Meritocracy in Late IMPERIAL China . Cambridge: Harvard University Press.
Embretson, S. E., and Reise, S. P. (2000). Item Response Theory for Psychologists . Mahwah: LEA.
Encyclopedia Brittanica (2010a). Europe in the middle ages: the background of early Christian education. Encyclopedia Britannica.
Encyclopedia Brittanica (2010b). Western education in the 19th century. Encyclopedia Britannica.
Feng, Y. (1995). From the imperial examination to the national college entrance examination: the dynamics of political centralism in China's educational enterprise. J. Contemp. China 4, 28–56. doi: 10.1080/10670569508724213
Gierl, M. J., and Lai, H. (2016). A process for reviewing and evaluating generated test items. Educ. Meas. Issues Pract. 35, 6–20. doi: 10.1111/emip.12129
Gipps, C. V. (1994). Beyond Testing: Towards a Theory of Educational Assessment . London: Falmer Press.
Greiff, S., Wüstenberg, S., and Avvisati, F. (2015). Computer-generated log-file analyses as a window into students' minds? A showcase study based on the PISA 2012 assessment of problem solving. Comput. Educ. 91, 92–105. doi: 10.1016/j.compedu.2015.10.018
Hancock, D., Hare, N., Denny, P., and Denyer, G. (2018). Improving large class performance and engagement through student-generated question banks. Biochem. Mol. Biol. Educ. 46, 306–317. doi: 10.1002/bmb.21119
Harris, L. R., and Brown, G. T. L. (2016). “Assessment and parents,” in Encyclopedia of Educational Philosophy And theory . ed. M. A. Peters (Springer: Singapore).
Hattie, J. Models of self-concept that are neither top-down or bottom-up: the rope model of self-concept. 3rd International Biennial Self Research Conference; July, (2004). Berlin, DE.
Hattie, J. A., and Brown, G. T. L. (2008). Technology for school-based assessment and assessment for learning: development principles from New Zealand. J. Educ. Technol. Syst. 36, 189–201. doi: 10.2190/ET.36.2.g
Inhelder, B., and Piaget, J. (1958). The Growth of Logical Thinking from Childhood to Adolescence . New York; Basic Books
Kingston, N. M., Alonzo, A. C., Long, H., and Swinburne Romine, R. (2022). Editorial: the use of organized learning models in assessment. Front. Education 7:446. doi: 10.3389/feduc.2022.1009446
Kline, R. B. (2020). “Psychometrics,” in SAGE Research Methods Foundations . eds. P. Atkinson, S. Delamont, A. Cernat, J. W. Sakshaug, and R. A. Williams (London: Sage).
Linden, W. J. V. D., and Glas, G. A. W. (2000). Computerized Adaptive Testing: Theory and Practice . London: Kluwer Academic Publishers.
Lingard, B., and Lewis, S. (2016). “Globalization of the Anglo-American approach to top-down, test-based educational accountability,” in Handbook of Human and Social Conditions in Assessment . eds. G. T. L. Brown and L. R. Harris (New York: Routledge).
Lundgren, E., and Eklöf, H. (2020). Within-item response processes as indicators of test-taking effort and motivation. Educ. Res. Eval. 26, 275–301. doi: 10.1080/13803611.2021.1963940
Marsh, H. W., Hau, K.-T., Artelt, C., Baumert, J., and Peschar, J. L. (2006). OECD's brief self-report measure of educational psychology's most useful affective constructs: cross-cultural, psychometric comparisons across 25 countries. Int. J. Test. 6, 311–360. doi: 10.1207/s15327574ijt0604_1
Mcmillan, J. H. (2016). “Section discussion: student perceptions of assessment,” in Handbook of Human and Social Conditions in Assessment . eds. G. T. L. Brown and L. R. Harris (New York: Routledge).
Meissel, K., Meyer, F., Yao, E. S., and Rubie-Davies, C. M. (2017). Subjectivity of teacher judgments: exploring student characteristics that influence teacher judgments of student ability. Teach. Teach. Educ. 65, 48–60. doi: 10.1016/j.tate.2017.02.021
Murdock, T. B., Stephens, J. M., and Groteweil, M. M. (2016). “Student dishonesty in the face of assessment: who, why, and what we can do about it,” in Handbook of Human and Social Conditions in assessment . eds. G. T. L. Brown and L. R. Harris (New York: Routledge).
National Research Council (2001). Knowing what students know: The science and design of educational assessment. The National Academies Press.
Nichols, S. L., and Harris, L. R. (2016). “Accountability assessment’s effects on teachers and schools,” in Handbook of human and Social Conditions in Assessment . eds. G. T. L. Brown and L. R. Harris (New York: Routledge).
Panadero, E. (2016). “Is it safe? Social, interpersonal, and human effects of peer assessment: a review and future directions,” in Handbook of Human and Social Conditions in Assessment . eds. G. T. L. Brown and L. R. Harris (New York: Routledge).
Pearl, J., and Mackenzie, D. (2018). The Book of why: The New Science of Cause and Effect . New York: Hachette Book Group.
Resnick, L. B., and Resnick, D. P. (1989). Assessing the Thinking Curriculum: New Tools for Educational Reform . Washington, DC: National Commission on Testing and Public Policy.
Rogoff, B. (1991). “The joint socialization of development by young children and adults,” in Learning to Think: Child Development in Social Context 2 . eds. P. Light, S. Sheldon, and M. Woodhead (London: Routledge).
Sadler, D. R. (1989). Formative assessment and the design of instructional systems. Instr. Sci. 18, 119–144. doi: 10.1007/BF00117714
Scriven, M. (1967). “The methodology of evaluation,” in Perspectives of Curriculum Evaluation . eds. R. W. Tyler, R. M. Gagne, and M. Scriven (Chicago, IL: Rand McNally).
Shin, J., Guo, Q., and Gierl, M. J. (2021). “Automated essay scoring using deep learning algorithms,” in Handbook of Research on Modern Educational Technologies, Applications, and Management . ed. D. B. A. M. Khosrow-Pour (Hershey, PA, USA: IGI Global).
Stobart, G. (2005). Fairness in multicultural assessment systems. Assess. Educ. Principles Policy Pract. 12, 275–287. doi: 10.1080/09695940500337249
Stobart, G. (2006). “The validity of formative assessment,” in Assessment and Learning . ed. J. Gardner (London: Sage).
Teltemann, J., and Klieme, E. (2016). “The impact of international testing projects on policy and practice,” in Handbook of Human and Social Conditions in Assessment . eds. G. T. L. Brown and L. R. Harris (New York: Routledge).
Thorndike, E. L. (1924). Measurement of intelligence. Psychol. Rev. 31, 219–252. doi: 10.1037/h0073975
Von Davier, A. A., Deonovic, B., Yudelson, M., Polyak, S. T., and Woo, A. (2019). Computational psychometrics approach to holistic learning and assessment systems. Front. Educ. 4:69. doi: 10.3389/feduc.2019.00069
Vygotsky, L. S. (1978). Mind in Society: The Development of Higher Psychological Processes . Cambridge, MA:Harvard University Press.
Weiner, B. (1985). An Attributional theory of achievement motivation and emotion. Psychol. Rev. 92, 548–573. doi: 10.1037/0033-295X.92.4.548
Wherrett, S. (2004). The SATS story. The Guardian, 24 August.
Wise, S. L. (2019). Controlling construct-irrelevant factors through computer-based testing: disengagement, anxiety, & cheating. Educ. Inq. 10, 21–33. doi: 10.1080/20004508.2018.1490127
Wise, S. L., and Demars, C. E. (2005). Low examinee effort in low-stakes assessment: problems and potential solutions. Educ. Assess. 10, 1–17. doi: 10.1207/s15326977ea1001_1
Wise, S. L., and Smith, L. F. (2016). “The validity of assessment when students don’t give good effort,” in Handbook of Human and Social Conditions in Assessment . eds. G. T. L. Brown and L. R. Harris (New York: Routledge).
Yan, Z., and Brown, G. T. L. (2017). A cyclical self-assessment process: towards a model of how students engage in self-assessment. Assess. Eval. High. Educ. 42, 1247–1262. doi: 10.1080/02602938.2016.1260091
Zhao, A., Brown, G. T. L., and Meissel, K. (2020). Manipulating the consequences of tests: how Shanghai teens react to different consequences. Educ. Res. Eval. 26, 221–251. doi: 10.1080/13803611.2021.1963938
Zhao, A., Brown, G. T. L., and Meissel, K. (2022). New Zealand students’ test-taking motivation: an experimental study examining the effects of stakes. Assess. Educ. 29, 1–25. doi: 10.1080/0969594X.2022.2101043
Zumbo, B. D. (2015). Consequences, side effects and the ecology of testing: keys to considering assessment in vivo. Plenary Address to the 2015 Annual Conference of the Association for Educational Assessment—Europe (AEA-E). Glasgow, Scotland.
Zumbo, B. D., and Chan, E. K. H. (2014). Validity and Validation in Social, Behavioral, and Health Sciences . Cham, CH: Springer Press.
Zumbo, B. D., and Forer, B. (2011). “Testing and measurement from a multilevel view: psychometrics and validation,” in High Stakes Testing in Education-Science and Practice in K-12 Settings . eds. J. A. Bovaird, K. F. Geisinger, and C. W. Buckendahl (Washington: American Psychological Association Press).
Zumbo, B. D., Maddox, B., and Care, N. M. (in press). Process and product in computer-based assessments: clearing the ground for a holistic validity framework. Eur. J. Psychol. Assess.
Keywords: assessment, testing, technology, psychometrics, psychology, curriculum, classroom
Citation: Brown GTL (2022) The past, present and future of educational assessment: A transdisciplinary perspective. Front. Educ . 7:1060633. doi: 10.3389/feduc.2022.1060633
Received: 03 October 2022; Accepted: 25 October 2022; Published: 11 November 2022.
Reviewed by:
Copyright © 2022 Brown. This is an open-access article distributed under the terms of the Creative Commons Attribution License (CC BY) . The use, distribution or reproduction in other forums is permitted, provided the original author(s) and the copyright owner(s) are credited and that the original publication in this journal is cited, in accordance with accepted academic practice. No use, distribution or reproduction is permitted which does not comply with these terms.
*Correspondence: Gavin T. L. Brown, [email protected] ; [email protected]
This article is part of the Research Topic
Horizons in Education 2022

An official website of the United States government
The .gov means it’s official. Federal government websites often end in .gov or .mil. Before sharing sensitive information, make sure you’re on a federal government site.
The site is secure. The https:// ensures that you are connecting to the official website and that any information you provide is encrypted and transmitted securely.
- Publications
- Account settings
Preview improvements coming to the PMC website in October 2024. Learn More or Try it out now .
- Advanced Search
- Journal List
- Am J Pharm Educ
- v.77(9); 2013 Nov 12
Educational Testing and Validity of Conclusions in the Scholarship of Teaching and Learning
Michael j. peeters.
a College of Pharmacy and Pharmaceutical Sciences, University of Toledo, Toledo, Ohio
Svetlana A. Beltyukova
b Judith Herb College of Education, University of Toledo, Toledo, Ohio
Beth A. Martin
c School of Pharmacy, University of Wisconsin-Madison, Madison, Wisconsin
Validity and its integral evidence of reliability are fundamentals for educational and psychological measurement, and standards of educational testing. Herein, we describe these standards of educational testing, along with their subtypes including internal consistency, inter-rater reliability, and inter-rater agreement. Next, related issues of measurement error and effect size are discussed. This article concludes with a call for future authors to improve reporting of psychometrics and practical significance with educational testing in the pharmacy education literature. By increasing the scientific rigor of educational research and reporting, the overall quality and meaningfulness of SoTL will be improved.
INTRODUCTION
The rigor of education research, including research in medical education, has been under scrutiny for years. 1,2 On the technical side, issues raised include lack of examination of the psychometric properties of assessment instruments and/or insufficient reporting of validity and reliability. 3-5 On the applied side, researchers have frequently based their conclusions on significance without addressing the practical implications of their findings. 6 These issues appear even more pronounced in the pharmacy education literature. In a review of over 300 articles published in pharmacy and medical education journals using educational tests, Hoover and colleagues found that pharmacy education articles much more often lacked evidence of reliability (and consequently validity) than did medical education articles, while neither consistently reported validity evidence. 7 While not specifically evaluated in that study, few pharmacy education articles reported an effect size of their studied intervention (MJ Hoover, e-mail, April 17, 2013).
It is encouraging that diverse pharmacy education instructors have authored many of the reviewed articles, representing a scholarship of teaching and learning (SoTL). However, authors still need to actively pursue psychometric evaluation of their student-learning assessments and examine the practical significance of the results. Increasing the technical rigor of research and reporting effect sizes will increase the overall quality and meaningfulness of SoTL. While doing so can be challenging, it can be accomplished without formal training. Just as scientists would not conduct experiments without verifying that their instruments were properly calibrated and would not claim that an experiment worked without indicating the magnitude of the effect, a SoTL investigator should not presume an assessment instrument’s reliability and validity but rather should seek evidence of both prior to attempting statistical analyses and interpret the results of those analyses from the perspective of educational significance (ie, effect size). This should be standard practice not only for standardized tests but also for other types of assessments of student knowledge and abilities, including performance-based assessments (eg, objective structured clinical examinations [OSCEs]) and traditional classroom assessments (eg, assessments with true/false, multiple-choice questions, case clinical notes, short-answer questions, and essay questions) used in SoTL.
This paper can be seen as an extension of a measurement series in Medical Education 8 for a SoTL audience, wherein it explicitly discusses the interrelatedness of psychometrics, statistics, and validity of conclusions. It is intended as a less-technical review of several established practices related to reporting educational test psychometrics and effect sizes, while also explaining how addressing both will contribute important evidence to the overall validity of data-based conclusions. Some of these practices involve statistical computations while others are based on logic. Following these practices should help SoTL investigators, who may not have formal training in psychometrics or statistics, to increase the rigor of their scholarship. We also offer a brief overview of some major advanced psychometric models that can be used to obtain further validity evidence. It is beyond the scope and focus of this paper to show how to create and administer assessments or how to calculate most statistics. We hope that the level of language, ideas, and examples herein will be relevant to the diverse readership. Examples from published studies, mainly in pharmacy education, are provided to illustrate some of the ways in which SoTL researchers could report findings.
By its traditional definition, validity refers to the degree to which a test accurately and meaningfully measures what it is supposed to measure. The seminal work, Test Validity and the Ethics of Assessment reminds us that validity also refers to the appropriateness of inferences or conclusions from assessment data and emphasizes that it is an ethical responsibility of researchers to provide evidence in support of their inferences. 9 The more convincing the evidence, the stronger the validity of inferences and the higher the degree to which researchers’ interpretations of assessment results are justifiable and defensible. With the focus on the nature of evidence underlying validity of inferences, the unitary validity framework presented in this text forms the basis of current testing standards. 10
Differing from an older framework comprised of 3 separate types of validity (ie, content, criterion, and construct), Messick argues that “different kinds of inferences … require different kinds of evidence, not different kinds of validity” 9 and presents the current standards according to which researchers should think of validity as 1 unifying concept instead of several separate types of validity. 9 Further, researchers are advised to consider reliability as evidence of validity and not as a separate statistic. Approaching validity as 1 holistic concept allows researchers to focus on the evidence they need to collect to be confident in the quality of their assessment instrument. This evidence typically involves reliability or stability of the instrument, discussion of the content relevance of the items, evidence that the items form a stable linear measure that is able to differentiate more-able from less-able persons in a way that is meaningful and consistent with the theory. It is also sometimes necessary to establish that an assessment produces results comparable to some other well-known instrument or functions the same way with different subgroups; that is, researchers must frequently consider multiple sources of validity evidence to be able to argue with confidence that their assessment instrument generates meaningful data from which justifiable conclusions can be drawn. No single validity source can provide such evidence. Taking an evidence-seeking approach to validity also implies that validity is contextual and that gathering such evidence is a process wherein researchers seek their own evidence each time they use an assessment based on this proposed purpose, use, and interpretation. For this reason, researchers should not solely rely on validity evidence reported by others. As overwhelming as this may sound, it is a doable task that does not necessarily require advanced psychometric training. Validity is a matter of degree and researchers can always find ways to gather validity evidence at the level of their own expertise and may seek help from a psychometrician when needed. Much of validity evidence comes in the form of words and logical arguments, while some (eg, reliability) may involve statistical applications and even advanced psychometric analyses.
For example, every researcher should be able to provide evidence of content relevance and content coverage, and the process of gathering this evidence should start prior to administering an educational test. As part of the process, the researcher should operationally define the knowledge, skills, or abilities that are being measured. This does not require psychometric expertise and is deeply grounded in content expertise of the investigator(s) or other subject matter experts. To illustrate, let us examine an assessment of a physician’s ability to practice medicine. To determine if the assessment we are considering is the right one to use, we need to reflect on how we define this ability and then draw on existing evidence and theories. Alternatively, we could match test items to a blueprint created after surveying numerous other content experts in the practice of medicine. If we define a physician’s ability to practice medicine as the ability to apply knowledge, concepts, and principles, and to demonstrate fundamental patient-centered skills, the United States Medical Licensing Examination Step 3 would be a test of choice 11 ; however, this examination would not be appropriate to use in nurse or pharmacist licensing because validity is contextual. For reporting purposes, an operational definition of what is being measured should be explicitly presented, given that inferences about the construct evaluated are based on this definition. The operational definition also becomes the driving factor in determining whether the right questions are included and whether they are constructed in such a way that they will elicit the needed information. Later in the research process, all of this knowledge about content relevance and coverage becomes evidence that is used to argue the validity of test-score interpretations and inferences about the construct after the statistical analyses are completed. In the example above, the term “validity of an examination” was deliberately avoided; instead, the focus was on “validity of inferences ” or “validity of conclusions ” from the data generated by an examination in this sample of participants.
Gathering validity evidence does not stop at the item review and instrument selection stages. Although evidence of content coverage and relevance contributes important information about the construct being measured and might influence the nature of inferences, it cannot be used in direct support of inferences from the scores. 9 Therefore, while it is important to collect content-related evidence, investigators also need to seek other evidence after participants have completed the test, focusing on internal structure evidence, including reliability . Collection of this evidence involves investigating the extent to which survey instrument items function well together to measure the underlying construct in a meaningful way, and for this task, researchers typically consider several different options, such as computing reliability indices, conducting an item analysis, using factor analysis, using generalizability theory, and applying item response theory. If problems such as low internal consistency or inter-rater reliability, lack of the meaning of a variable, poor item fit, multidimensionality, lack of variance explained, or inconsistent item functioning across different subgroups of respondents are discovered, interpretations of the results and inferences about the construct should not be attempted. Instead, the investigator should go back to the drawing board, revisit the theory behind the construct, reexamine content relevance and coverage, and start the process again until the content and structure-related evidence points to good psychometric properties of an assessment instrument.
RELIABILITY
Reliability — reproducibility or consistency of assessment scores within a sample of participants — is a major initial quantitative “quality index” of the assessment data as well as essential evidence toward the overall validity. However, as with any other validity evidence, its use alone is not sufficient for arguing overall validity. Reliability alone has limited usefulness because it indicates only that an assessment’s items measure something consistently. Reliability does not indicate what knowledge, skills and/or abilities are being measured. Thus, along with reliability, other validity evidence is crucial before any validity conclusions can be made.
While seeking reliability evidence, researchers should select the most appropriate type and explicitly identify this type while reporting a SoTL study. Two common types of reliability include internal consistency reliability and inter-rater reliability. The first is particularly relevant for many SoTL investigators . The internal consistency reliability index shows the extent to which patterns of responses are consistent across items on a single test occasion, whereas the inter-rater reliability index indicates consistency across raters and is reported when judges or scorers are involved. That said, it is also possible and sometimes necessary to report more than 1 reliability index (eg, when using multiple educational tests). In many instances, reliability is specific to each use of an assessment and can change when the assessment is used with another group or when an assessment is even slightly modified. Thus, it is prudent to report reliability for every occasion within any scholarship. 12
Reliability can be relative or absolute. 13,14 When repeated measurements are used, relative reliability refers to a consistency or reproducibility of rankings of scores in a sample, whereas absolute reliability refers to the degree to which individual scores are reproduced (eg, when judges agree on 1 specific score). A relative reliability index can be helpful when student performances are ranked in relation to 1 another, whereas absolute reliability could be helpful when the focus is on specific scores from an evaluation. Of the 2, absolute reliability is often omitted, even though it is considered by some to be of greater clinical 15 and educational 16-18 value. Standard error of measurement (SEM) is a recommended absolute reliability index to report, whereas internal consistency reliability would be a choice as relative reliability index. That said, both absolute and relative reliability should be assessed when appropriate.
Internal Consistency Reliability
Internal consistency reliability is the relative reliability of items within an assessment. It is most easily obtained for a quiz or examination wherein all the items are on the same scale and in the same format (eg, multiple-choice, short-answer, or longer-answer clinical cases). Cronbach alpha is typically calculated when the questions on a test are on a rating scale, while a Kuder-Richardson formula 20 (KR-20) is applied to dichotomous (yes/no) or multiple-choice testing with only 1 correct answer. Commonly accepted ranges for internal consistency reliability are widely available, and often a coefficient >0.7 is sufficient for a classroom test used in SoTL (ie, some random inconsistency is not only expected but also allowed because no measurement can ever be perfect). However, life-changing decisions necessitate higher reproducibility of test scores, and internal consistency should be >0.80 or >0.90 on high or very high-stakes assessments. 19 In an example of reporting this relative internal consistency reliability type, the authors not only reported Cronbach’s alpha for each of their 4 assessments but also evaluated their indices against their minimum a priori Cronbach alpha level. 20
When internal consistency reliability is low, there are several ways to improve it. The most common strategies include: increasing the number of response options/distractors (eg, 5 possible answers instead of 3), and using a greater number of related questions on a test, including items that range in difficulty so that the test would not discourage low-ability students but would still be challenging enough for high-ability students. Notable sources suggest that the number of response options should be limited 21 and that reliability may actually decrease if those extra distractors are not plausible. Some of the above strategies require only content expertise while others rely on the researcher’s knowledge of computing item-discrimination and item-difficulty indices. A detailed discussion of these item-analysis indices is beyond the scope of this paper but can be found in other sources. 22 Researchers are strongly encouraged to evaluate and, if necessary, improve internal consistency reliability of their tests to improve precision of their assessments and ultimately influence the accuracy of conclusions and decisions based on the results.
Standard Error of Measurement
Test scores are not perfect representations of student knowledge, skills, or abilities, and SEM is capable of capturing this inherent imprecision in scores. Assessing the extent of measurement error is important for the validity of inferences from the scores. SEM is an absolute reliability index that can be used when seeking reliability evidence, as it shows the extent to which individual scores would be repeated if students were tested again and again. As such, it should not be confused with the standard error of the mean (also commonly and confusingly abbreviated as SEM), which refers to groups rather than individuals and shows the extent to which a sample/group mean score would be reproducible if the test were administered again and again to different same-sized samples.
When the focus is on individual student scores rather than group means, SEM can be quite helpful. The following formula may facilitate understanding of the concept of SEM, 17,18 and can employ either an internal consistency or inter-rater reliability coefficient.

As suggested by the formula, SEM is directly related to a test’s reliability, uses that test’s standard deviation (SD), and is reported in the units of each specific test. Once computed, SEM can be used to develop confidence intervals for interpretation of test scores and thus represents important validity evidence. By convention, ±1 SEM around a test score yields a 68% confidence interval around that test score, and ±2 SEM yields a 95% confidence interval. The latter is the most typical in medical research and may be the most applicable for SoTL research as well. The choice of interval depends on the desired level of precision, with greater confidence being expected in high-stakes testing situations, wherein SEM has most often been used. We could not find an example of reporting SEM with internal consistency reliability in the pharmacy education literature. An example from medical education can be found in an evaluation of SEM in borderline (pass/fail) scores of medical students within a medical school’s integrated assessment program. 23
Inter-rater Reliability
As the name suggests, inter-rater reliability would be the choice if an assessment involves multiple raters or judges. Investigators typically need to consider how consistent the judges are and how much agreement they demonstrate when, for example, observing a skill performance. Judge consistency, or inter-rater consistency , reflects the extent to which multiple raters are in consensus about which examinees are more knowledgeable, able, or skilled, and which are less so. Therefore, high inter-rater consistency means that judges produce similar rankings of examinees’ scores. As such, this type of reliability would be an example of relative reliability. Judge agreement, or inter-rater agreement , on the other hand, represents absolute reliability, 15 showing the extent to which raters give the same (and accurate) rating to the same examinee’s skill performance.
Depending on the data, there are different statistics that can be computed for determining inter-rater consistency. The most known indices include an ICC for continuous data, and a Cohen kappa for categorical data. In the pharmacy education literature, there is an example of using an ICC to determine the degree of agreement between the analytical checklist scores obtained in 2 different conditions (real-time and video). 24 The ICC was 0.951, which the authors interpreted as high agreement (values of less than 0.4 indicated poor agreement; between 0.4 and 0.8, fair to good agreement; and greater than 0.8, excellent agreement). An example of Cohen kappa can be found in an analysis of letter grades from the 2008-2009 academic year using 2 independent faculty evaluations representing categorical-level data. 25 In this paper, the researchers reported a Cohen kappa of 0.98 as evidence of inter-rater reliability.
There are several ways to address problems with inter-rater reliability. The most common strategies include increasing the number of observations by each rater, providing raters with detailed scoring descriptions in a rubric, and using a larger scoring scale. Increasing observations and increasing the scoring scale are similar to common strategies for improving internal consistency reliability of an assessment. A similar warning applies to the use of a larger scoring scale: while a larger scoring scale can be more reliable, raters may be less likely to agree with 1 another. Consequently, they may be imprecise in their evaluation of a examinee’s performance, which would result in low inter-rater agreement (and absolute reliability). An example of seeking inter-rater consistency and inter-rater agreement is provided in Table 1 , which also illustrates an impact of the length of the scoring scale using ICC and percent agreement. Including both consistency and agreement is not common in clinical research. 13,15,26 However, given that doing so is highly recommended, SoTL investigators should attempt to report both when student performances were judged. The pharmacy education literature includes a study in which both inter-rater consistency and agreement were reported. When scoring a PharmD program’s admission essays, a balance between the 2 indices was sought, the result being a minimal SEM and an acceptably high ICC. 27 While use of ICC alone favored 1 rubric, including SEM showed a more complete picture, resulting in more confident evaluations with less measurement error and alterations in rubric design toward a more holistic scoring.
Examples of Inter-rater Reliability and Inter-rater Agreement*

ADVANCED PSYCHOMETRIC MODELS
Complexity of research projects often calls for more advanced approaches to gathering validity evidence Table 2 . As previously mentioned, some of the most popular approaches include generalizability theory, factor analysis, and item response theory.
Methods of Collecting Validity Evidence and Enhancing the Quality of Scholarship of Teaching and Learning (SoTL)

Generalizability Theory
If a SoTL researcher is using a test that is similar to an OSCE, in which multiple raters are scoring items while nested in different stations, the reliability indices discussed above would not be sufficient, even if more than 1 is reported. While researchers could evaluate the internal consistency of the items at each station or the inter-rater reliability among 2 or more raters at each station, those reliability indices would quantify only the measurement error specific to the items or the raters at that 1 station but would fall short of capturing the reliability of all items and raters across all the stations. Generalizability theory (G-theory) is a model that could be considered, as it simultaneously models variation in data from multiple sources (eg, stations, raters, and items) and provides 1 overall (combined) process reliability index for an entire multi-station assessment. In other words, this index includes both internal consistency and inter-rater reliability information. A further advantage of using G-theory is that subsequent decision-study analyses could identify which changes in the number of items, raters, and/or stations would improve the process reliability most beneficially for future assessments. As such, a distinct benefit with G-theory is that it can help optimize the number of raters and stations needed for a reliable test, which can be an important consideration given the limited curricular resources (ie, faculty, space, finances) available to pharmacy colleges and schools for assessment of student learning.
The G-theory model is widely accepted in medical education research and OSCEs are a noteworthy application of G-theory. 28 Using G-theory, important findings from OSCE-based evaluations have revealed that a global rating scale for each station (instead of a detailed checklist) was at least as reliable as a checklist over the multiple OSCE stations. 29 Regarding validity evidence, they also demonstrated that, among medical students, residents and physicians, global rating scales were able to detect changes in growing clinical expertise that checklists could not capture. 30 An example of using G-theory in pharmacy education can be found in research that analyzed interview data from pharmacy resident candidates. 31 Each candidate was interviewed by 8 interviewers, with 1 or 2 interviewers nested within their own interview session (or station); subsequent decision studies showed that placing one interviewer into multiple, separate stations was much more reliable than simply adding more interviewers to an existing station (or panel).
Factor Analysis
Factor analysis is another approach wherein investigators analyze correlations among test (or instrument) items. These correlations can be used as the internal structure evidence to support validity of conclusions. Within this analysis, inter-item correlations are uncovered, and items are grouped to represent different meaningful factors (or domains). 32 The most commonly reported statistics for factor analysis include inter-item correlations, eigenvalues, explained variance, and reliability indices for all factors and the entire assessment. In 1 use of factor analysis with data from 7 institutions, the researchers developed a professionalism assessment tool and examined its internal structure. 33 Although the 33-item instrument was generally used to assess an overall level of student pharmacist professionalism, 5 more-specific factors were unveiled through exploratory factor analysis, with subsequent confirmation within another larger cohort of students. This allowed the authors to better understand the specific dimensions of professionalism captured by their instrument as well as to assess the internal consistency reliability for each identified domain using a KR-20 statistic.
Rasch Analysis
Unlike factor analysis or G-theory, item response theory and specifically the Rasch model represent an advanced alternative to classical test theory. This model takes into consideration each student’s ability and each item’s difficulty, and examines how students interact with each item based on their abilities. 34 The Rasch model produces several useful statistics that provide rich evidence of validity. The most commonly reported statistics include: different reliability indices (Rasch reliability and separation), item fit (ie, how well the items function together as a unidimensional measure of some underlying construct), item-person map (ie, a visual “ruler” that allows researchers to determine qualitatively if the meaning of the measure matches the theory), scoring scale functioning (particularly useful with rating scales in that it shows empirically if all the categories in the scale are used by examinees consistently), and judging bias. The Rasch model generates estimates of item difficulty and person abilities and is sample-independent. As such, the Rasch model is used in high-stakes testing for physicians, chiropractors, and nurses, as well as in high school graduation examinations by numerous state departments of education. Reproaching the example from kappa above, that article is also an example of a Rasch analysis of a PharmD student presentation rubric and judge bias. 24 In that study, a Many-Facets Rasch model was used “to determine the rubric’s reliability, quantify the contribution of evaluator harshness/leniency in scoring, and assess grading validity by comparing the current grading method with a criterion-referenced grading scheme.” The researchers reported high rubric reliability (r=0.98) and recommended that several grades be adjusted to eliminate evaluator leniency, although they concluded that evaluator leniency appeared minimal. 24
EFFECT SIZE
Once investigators are confident that they have assessed student performances accurately and meaningfully (ie, determined that their assessment instrument has good psychometric properties), they can conduct statistical analyses of the data and proceed with the interpretation of the results from the standpoint of both statistical and educational significance. The former involves reporting a p value while the latter requires reporting an effect size. 6 Together with statistical significance, effect sizes provide powerful evidence of the validity of conclusions. For example, they may be particularly informative when significance is not found but a large change or difference is observed or when significance is found but the effect size is negligible. Without noting the size of the effect, the researchers may falsely conclude no effect of the intervention in the first situation and effect of the intervention in the second. In reality, however, the sample size may have been too small to reach statistical significance or the assessment tool may not have been sensitive enough to capture change or difference.
Two of the most common indices for effect size are Cohen’s d and R 2 . Cohen’s d is used when researchers compare 2 groups using standard deviation units. With the help of 1 of numerous online calculators, it can be easily calculated once an investigator knows the means and standard deviations for each group. By convention, effect sizes of <0.2 are considered small; 0.5, medium; and 0.8, large. Since many meaningful educational interventions have had a medium-large effect size, 35 reporting an effect size value may assist readers in identifying interventions that might also be promising in their comparable educational settings. That said, effect size does not guarantee generalizability, although the likelihood that interventions with large effect sizes and proper experimental controls will replicate is high. An example of reporting an effect size can be seen in a study evaluating a prescribing error module. 36 In this study, the researchers compared 2 groups on 3 worksheets and noted a large effect of 0.85 associated with a significant difference on 1 of the worksheets.
The second index, R 2 , also known as coefficient of determination, shows a percentage of variance shared by 2 or more variables and, as such, is easy to use and interpret. By convention, accounting for less than 2% is considered a small effect; up to 13%, a medium effect; and for 25% or more, a large effect. Researchers can also easily convert between a Cohen’s d and R 2 using the following formula: d=2r/sqrt(1-R 2 ). 37
This primer was written to encourage a more rigorous and scholarly approach to SoTL research that uses student-learning assessments. The key message is that researchers should aim to provide evidence to maximize the validity of their conclusions. This evidence should include both psychometrics of the instruments used and practical significance of the test results. By increasing the scientific rigor of educational research and reporting, the overall quality and meaningfulness of SoTL will be improved.
ACKNOWLEDGEMENT
The authors did not have grant support for this manuscript nor do they have any financial conflicts of interest to report. All authors substantially contributed to the writing and editing of this manuscript.
- Skip to Content
- Skip to Main Navigation
- Skip to Search

Indiana University Bloomington Indiana University Bloomington IU Bloomington

- Course Development Institute
- Programmatic Assessment
- Instructional Technology
- Class Observations and Feedback
- Online Course Review and Feedback
- New Faculty Programs
- History of SoTL
- SOTL Resources
- IUB Database
- Featured SoTL Activity
- Intensive Writing
- Faculty Liaison
- Incorporating and Grading Writing
- Writing Tutorial Services
- Cel Conference
- CEL Course Development Institute
- ACE Program
- Community Partners
- CEL Course Designation
- CEL during COVID-19
- Annual AI Orientation
- Annual Classroom Climate Workshop
- GTAP Awardees
- Graduate Student Learning Communities
- Pedagogy Courses for Credit
- Diversity Statements
- Learning Communities
- Active Learning
- Frequent and Targeted Feedback
- Spaced Practice
- Transparency in Learning and Teaching
- Faculty Spotlights
- Preparing to Teach
- Decoding the Disciplines
- Backward Course Design
- Developing Learning Outcomes
- Syllabus Construction
- How to Productively Address AI-Generated Text in Your Classroom
- Accurate Attendance & Participation with Tophat
- Designing Assignments to Encourage Integrity
- DEI and Student Evals
- Engaging Students with Mental Health Issues
- Inclusive and Equitable Syllabi
- Creating Accessible Classrooms
- Proctoring and Equity
- Equitable Assignment Design
- Making Teaching Transparent
- DEIJ Institute
- Sense of Belonging
- Trauma-Informed Teaching
- Managing Difficult Classroom Discussions
- Technology to Support Equitable and Inclusive Teaching
- Teaching during a Crisis
- Teaching for Equity
- Supporting Religious Observances
- DEIJ Resources
Test Construction
- Summative and Formative Assessment
- Classroom Assessment Techniques
- Authentic Assessment
- Alternatives to Traditional Exams and Papers
- Assessment for General Education and Programmatic Review
- Rubric Creation and Use
- Google Suite
- Third Party Services: Legal, Privacy, and Instructional Concerns
- eTexts and Unizin Engage
- Next@IU Pilot Projects
- Web Conferencing
- Student Response Systems
- Mid-Semester Evaluations
- Teaching Statements & Philosophies
- Peer Review of Teaching
- Teaching Portfolios
- Administering and Interpreting Course Evaluations
- Temporary Online Teaching
- Attendance Policies and Student Engagement
- Teaching in the Face of Tragedy
- Application for an Active Learning Classroom
- Cedar Hall Classrooms
- Reflection in Service Learning
- Discussions
- Incorporating Writing
- Team-Based Learning
- First Day Strategies
- Flipping the Class
- Holding Students Accountable
- Producing Video for Courses
- Effective Classroom Management
- Games for Learning
- Quick Guides
- Mosaic Initiative
- Kelley Office of Instructional Consulting and Assessment
Center for Innovative Teaching and Learning
- Teaching Resources
- Assessing Student Learning
Most tests are a form of summative assessment; that is, they measure students’ performance on a given task. (For more information on summative assessment, see the CITL resource on formative and summative assessment .) McKeachie (2010) only half-jokes that “Unfortunately, it appears to be generally true that the examinations that are the easiest to construct are the most difficult to grade.” The inverse is also true: time spent constructing a clear exam will save time in the grading of it.
Closed-answer or “objective” tests
By “objective” this handbook refers to tests made up of multiple choice (or “multi-op”), matching, fill-in, true/false, fill-in-the-blank, or short-answer items as objective tests. Objective tests have the advantages of allowing an instructor to assess a large and potentially representative sample of course material and allow for reliable and efficient scoring. The disadvantages of objective tests include a tendency to emphasize only “recognition” skills, the ease with which correct answers can be guessed on many item types, and the inability to measure students’ organization and synthesis of material
Since the practical arguments for giving objective exams are compelling, we offer a few suggestions for writing multiple-choice items. The first is to find and adapt existing test items. Teachers’ manuals containing collections of items accompany many textbooks. However, the general rule is “adapt rather than adopt.” Existing items will rarely fit your specific needs; you should tailor them to more adequately reflect your objectives.
Objective-answer tests can be constructed to require students to apply concepts, or synthesize and analyze data and text. Consider using small “cases studies,” problems or situations. Provide a small collection of data, such as a description of a situation, a series of graphs, quotes, a paragraph, or any cluster of the kinds of raw information that might be appropriate material for the activities of your discipline. Then develop a series of questions based on that material, the answers to which require students to process and think through the material and question significantly before answering.
Here are a few additional guidelines to keep in mind when writing multiple-choice tests:
- As much of the question as possible should be included in the stem.
- Make sure there is only one clearly correct answer (unless you are instructing students to select more than one).
- Make sure the correct answer is not given away by its being noticeably shorter, longer, or more complex than the distractors.
- Make the wording in the response choices consistent with the item stem.
- Beware of using answers such as “none of these” or “all of the above.”
- Use negatives sparingly in the question or stem; do not use double negatives.
- Beware of using sets of opposite answers unless more than one pair is presented (e.g., go to work, not go to work).
Essay exams
Conventional wisdom accurately portrays short-answer and essay examinations as the easiest to write and the most difficult to grade, particularly if they are graded well. You should give students an exam question for each crucial concept that they must understand.
If you want students to study in both depth and breadth, don't give them a choice among topics. This allows them to choose not to answer questions about those things they didn’t study. Instructors generally expect a great deal from students, but remember that their mastery of a subject depends as much on prior preparation and experience as it does on diligence and intelligence; even at the end of the semester some students will be struggling to understand the material. Design your questions so that all students can answer at their own levels.
The following are some suggestions that may enhance the quality of the essay tests that you produce
- Have in mind the processes that you want measured (e.g., analysis, synthesis).
- Start questions with words such as “compare,” “contrast,” “explain why.” Don’t use “what,” “when,” or “list.” (These latter types are better measured with objective-type items).
- Write items that define the parameters of expected answers as clearly as possible.
- Make sure that the essay question is specific enough to invite the level of detail you expect in the answer. A question such as “Discuss the causes of the American Civil War,” might get a wide range of answers, and therefore be impossible to grade reliably. A more controlled question would be, “Explain how the differing economic systems of the North and South contributed to the conflicts that led to the Civil War.
- Design the question to prompt students’ organization of the answer. For example, a question like “Which three economic factors were most influential in the formation of the League of Nations?”
- Don’t have too many questions for the time available.
- For take-home exams, indicate whether or not students may collaborate and whether the help of a Writing Tutorial Services tutor is permissible.
Grading essay exams
A more detailed discussion of grading student work is offered in evaluating student written work and applies to handling essay exams as well.
However, unlike formal essays, essay exams are usually written in class under a time limit; they often fall at particularly busy times of the year like mid-term and finals week. Consequently, they are differently stressful for students, and as a result you may encounter errors and oversights that do not appear in formal essays. Similarly, it is not unusual to find essays that do not provide responses we have anticipated.
Your grading changes in response. Adjustments to the grading scale may be necessary in light of exam essays that provide answers you had not anticipated. Comments may be briefer, and focused primarily on the product students have produced; that is, exams do not require suggestions for revision.
Center for Innovative Teaching & Learning social media channels
Useful indiana university information.
- Campus Policies
- Knowledge Base
- University Information Technology Services
- Office of the Vice Provost for Undergraduate Education
- Office of the Vice Provost for Faculty and Academic Affairs
- Faculty Academy on Excellence in Teaching
- Wells Library, 2nd Floor, East Tower 1320 East Tenth Street Bloomington, IN 47405
- Phone: 812-855-9023
Advertisement
School testing culture and teacher satisfaction
- Open access
- Published: 05 November 2020
- Volume 32 , pages 461–479, ( 2020 )
Cite this article
You have full access to this open access article
- William C. Smith 1 &
- Jessica Holloway 2
9617 Accesses
8 Citations
11 Altmetric
Explore all metrics
Teachers, as frontline providers of education, are increasingly targets of accountability reforms. Such reforms often narrowly define ‘teacher quality’ around performative terms. Past research suggests holding teachers to account for student performance measures (i.e. test scores) damages their job satisfaction, including increasing stress and burnout. This article examines whether the relationship between test-based accountability and teacher satisfaction can be, in part, explained by the emphasis of student test scores in teacher appraisals. Although historically used for formative purposes, recent research demonstrates that across a large range of countries, nearly all teachers work in a system where their appraisal is based, in part, on students’ test scores. Using data from the 2013 Teaching and Learning International Survey, we pool data from 33 countries to evaluate the direct and indirect effect of school testing culture on teacher satisfaction. Results suggest that there is a direct relationship between the intensity of the testing culture and the satisfaction of teachers, as well as an indirect relationship with test score emphasis in teacher appraisals suppressing potential positive effects of appraisals on teacher satisfaction.
Similar content being viewed by others
Exploring Diversity in the Relationships Between Teacher Quality and Job Satisfaction in the Nordic Countries—Insights from TALIS 2013 and 2018
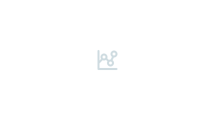
The influences of teachers’ perceptions of using student achievement data in evaluation and their self-efficacy on job satisfaction: evidence from China
Potential psychosocial and instructional consequences of the common core state standards: implications for research and practice.
Avoid common mistakes on your manuscript.
Recent decades have brought about a sharp increase in teacher-focused accountability policies and practices. This global phenomenon (Holloway et al. 2017 ; Verger and Parcerisa 2017 ) has relied heavily on the numerical measures of ‘teacher quality’, as various forms of standardised achievement tests grow in prominence. Large-scale international achievement tests, such the Organisation for Economic Co-operation and Development’s (OECD’s) Programme for International Student Assessment (PISA), as well as national (e.g. NAPLAN in Australia) and subnational tests (e.g. state-level tests in the USA), have helped facilitate the incorporation of student test scores into accountability systems around the world. While most of these standardised tests were never designed or intended to be used for measuring teacher quality or effectiveness, it is becoming increasingly common for schools to incorporate student test scores in their teacher-level appraisal/evaluation systems. Indeed, Smith and Kubacka ( 2017 ) analysed international data from the OECD’s Teaching and Learning International Survey (TALIS) and found that nearly all teachers (i.e. 97%) reported that their appraisals included some form of student test scores. While multiple measures of teacher performance are typically included in these systems, overall, teachers reported that student test scores have been increasingly prioritized in terms of appraisal focus, and thus supplanted other forms of more meaningful feedback (e.g. teacher portfolios, observations). According to leading organisations, such as the OECD ( 2014 ) and the American Education Research Association (AERA 2015 ), multiple measures of teacher performance are necessary for achieving the fair and valid appraisal/evaluation systems deemed so important.
Unfortunately, the disproportionate emphasis on student test scores has led to the production of what some have identified as a ‘testing culture’, where the professional identities and work of teachers are being fundamentally changed. This transformation is exacerbated when high stakes are attached to appraisal outcomes (Certo 2006 ; Larsen 2005 ), which is also increasingly common across most education systems worldwide (Smith and Kubacka 2017 ). Past literature using the lens of organizational theory and sociological institutionalism has highlighted the importance of environmental factors, such as occupational stress (Xiaofu and Qiwen 2007 ), work pressure, and practical support (Aldridge and Fraser 2016 ), on teacher satisfaction. Within the school climate, interpersonal relationships are also important (Price 2012 ). Grayson and Alvarez ( 2008 ) identified poor relationships between teachers and their principal as one of main factors predicting teachers feeling of depersonalization and cynicism in their job. Furthermore, expectations for teachers are shaped by emerging institutional norms (Booher-Jennings 2005 ; Smith 2016 ) that lay out appropriate scripts for behaviour. Increasingly, these require teachers to embrace the preparation, application, and interpretation of student test scores (Holloway 2019 ). Shaping the experiences of teachers, the school testing culture reinforces these emerging norms, while permeating the teaching and learning environment, and influencing interpersonal relationships.
In this paper, we seek to investigate the relationship between the school testing culture and teacher satisfaction, with a particular focus on how teacher appraisals may moderate the relationship. Specifically, we are investigating whether teacher appraisals can provide an explanation for the reported relationship between test-based accountability and teacher satisfaction. In the following section, we start with an overview of the literature, focusing on the varied ways that education systems have incorporated student test scores into teacher appraisal, and then we move towards a more specific focus on how these systems have affected teacher satisfaction.
1 Use of student test scores in teacher accountability
Test-based accountability, or testing for accountability (Smith 2014 ), is present when student test scores are used as one input to hold teachers or schools accountable. For teachers, this often comes in the form of performance-based pay, where the results of student test scores influence whether the teacher continues in their current position and what their cumulative income equals. Although the current push originated in the USA and UK, this type of test-based accountability has expanded to countries around the globe (UNESCO 2017 ). For instance, in Portugal, salary scales were redesigned in 2007 to include student test scores, and in Chile up to 30% of teachers’ salaries may be based on student test scores (Barnes et al. 2016 ).
The effects of test-based accountability have been studied in a variety of ways, most of which have focused on the general effects of high-stakes accountability, such as decreased teacher morale (Certo 2006 ; Larsen 2005 ), limited pedagogical approaches (e.g. narrowing of the curriculum, teaching to the test; Polesel et al. 2014 ; Warren and Ward 2018 ), and other intended and unintended consequences that have resulted from increased testing programmes (e.g. policy responses to PISA test results, see Breakspear 2014 for a review). Another large area of research focus has been on the measurement issues associated with using student test scores to measure teacher effectiveness (Amrein-Beardsley 2014 ; Hanushek and Rivkin 2010 ; Rothstein 2010 ). This body of research has primarily stemmed from the USA, as the USA has developed the most sophisticated method for directly linking student test scores to teacher effects via the student growth model (SGM) or value-added model (VAM). VAMs are statistical tools designed to capture and compare the predicted and real effects that individual teachers have had on their students’ annual, standardized achievement tests. While there are technical differences between the SGM and VAM methodologies, these differences are irrelevant for the present study; therefore, for the sake of clarity, the term ‘VAM’ will be used throughout the rest of the paper to mean any form of student growth or value-added model.
These models have been extensively covered in the literature, with a particular focus on the methodological and logistical issues related to VAM measurement and use, such as studies on the validity, reliability, bias, and fairness of VAM-based evaluation and policy (Ballou and Springer 2015 ; Koedel and Betts 2011 ; Moore Johnson 2015 ; Rothstein 2010 ). There have been several reviews of these issues, from varied disciplines, see Amrein-Beardsley and Holloway ( 2017 ) for a review from an educational research perspective, Koedel et al. ( 2015 ) for an economic perspective, and Darling-Hammond ( 2015 ), Everson ( 2017 ), and the AERA official statement (AERA 2015 ) for general reviews of VAMs and VAM use. Together, these reviews demonstrate that VAMs are more sophisticated than previously-used status models—or models designed to measure student proficiency at a given time—as they measure growth in learning over time and are able to theoretically mitigate the effects of extraneous variables, such as socioeconomic status, English language status, and prior testing performance. However, the vast conditions that must first be met to guarantee that VAMs are valid, reliable, unbiased, and fair for teacher evaluation are nearly impossible in actual practice. As described in the AERA Statement ( 2015 ):
Even if all of the technical requirements…are met, the validity of inferences from VAM scores depends on the ability to isolate the contributions of teachers and leaders to student learning from the contributions of other factors not under their control. This is very difficult, not only because of data limitations but also because of the highly nonrandom sorting of students and teachers into schools and classes within schools (p. 449).
Given these challenges, the general consensus is that VAMs should not be used for high-stakes purposes, though this warning has had little effect on most US states’ adoption and use of VAMs in their high-stakes teacher evaluation systems (Collins and Amrein-Beardsley 2014 ; Close et al. 2019 ). While the global trend appears to be increased use of test-based accountability, caution in implementing VAMs for accountability purposes is still exercised in many countries. One notable exception is England, which has used various iterations of VAMs for school- and system-level accountability (Sørensen 2016 ).
This particular dimension of test-based teacher accountability is important for the current paper because it underlines the potential problems associated with the use of student test scores in teacher appraisals. In the USA specifically, and in the UK to some extent, the ways in which test scores have been used for high-stakes accountability purposes have led to problems with trust, utility, and satisfaction (Collins 2014 ; Garver 2019 ; Pizmony-Levy and Woolsey 2017 ). As mentioned previously, this has impacted teachers’ interpersonal relationships (between colleagues and teachers and their supervisors), as well as made it difficult for teachers to see the value in the test scores for informing their instruction. As we will revisit throughout the paper, there are multiple ways that test scores can be used in appraisals, which might have bearing on whether teachers see such uses as beneficial or not. Worth considering here is how test-based accountability interacts with the ‘global testing culture’, which we describe next.
2 The global testing culture and its influence on teachers
The incorporation of test scores into teacher accountability schemes and the underlying belief that student test scores represent an objective, accurate account of student learning reflects a larger global testing culture (Smith 2016 ). The global testing culture is based on the assumptions of positivism and individualism. In agreeing with these assumptions, there is an almost unconscious belief that quantitative measures, such as test scores, represent the reality of the situation and that the outcomes of education are the result of individual actions and are not influenced by larger societal context or family circumstances. Based on sociological institutionalism, within this culture, behavioural expectations are laid out, including that teachers do everything in their power to help students succeed on the test.
The consistent pressure to improve test scores contributes to reshaping the ‘possibilities by which the teaching profession, and teaching professionals, can be known and valued, and the ways that teachers can ultimately be and associate themselves in relation to their work’ (Lewis and Holloway 2019 , p. 48). Muller and Boutte ( 2019 ) further deconstruct the global testing culture by using the work of Paulo Friere to draw equivalences between standardized testing and the oppression of teachers. The divide and conquer dimension of oppression is clearly seen in past research that points to teachers blaming those in earlier grades for inadequately preparing students (Wiggins and Tymms 2000 ) and concerns that teachers will be stigmatized for not buying into the school’s focus on student test scores (Booher-Jennings 2005 ).
A handful of studies (Holloway and Brass 2018 ; Perryman and Calvert 2019 ; Warren and Ward 2018 ) have explored how the cultural expectations of teachers, and the prevailing testing culture, are associated with an increase in teacher workload, and, consequently, work-related pressure, personal stress, and decreased job satisfaction. Perryman and Calvert ( 2019 ) have linked high-stakes accountability to excessive burnout and teacher turnover, arguing that their participants illustrated ‘a discourse of disappointment, the reality of teaching being worse than expected, and the nature (rather than the quantity) of the workload, linked to notions of performativity and accountability, being a crucial factor’ (p. 2) for why teachers were leaving the profession. Similarly, Garver ( 2019 ), who conducted an in-depth ethnographic study of a US middle school’s use of a test-based teacher evaluation system, found that teachers experienced feelings of anxiety, distrust, and vulnerability. Wronowski and Urick ( 2019 ) found that although stress and worry were associated with the intent to leave their position, the factors only predicted actual departure for teachers frustrated by the accountability system.
Bringing together the statistical issues with using student test scores in teacher accountability, with the creeping pressures that are often associated with such systems, we argue that the testing culture is producing an environment where teacher satisfaction is potentially compromised. The relationship between satisfaction and appraisal has been studied in different contexts, and we see our study as extending this literature in important ways. First, though, we identify some of the studies that have explored similar questions.
3 Teacher appraisals and teacher satisfaction
Teacher appraisals have become the dominant tool for administering the accountability of teachers. Although initially separate from summative teacher evaluations, the inclusion of high-stakes and links to student test scores (Murphy et al. 2013 ; Xu et al. 2016 ) have made teacher evaluations and teacher appraisals practically indistinguishable (Smith and Kubacka 2017 ). Past research suggests that teacher appraisals, and how appraisals are experienced by teachers, are an artefact of the school climate and can impact individual job satisfaction. Past studies that have examined the role of teacher appraisals/evaluations on satisfaction have focused on general perceptions that the process was fair or inclusive (Brezicha et al. 2019 ). Ford et al. ( 2018 ) found that when the teachers viewed the evaluations as being part of a supportive process, and when the evaluations led to meaningful changes in their practice, teachers were more likely to report feelings of satisfaction. The authors emphasized that the utility of the evaluation was important for teachers to feel satisfied with their work and with their profession. In China, Liu et al. ( 2018 ) found that teachers who believed their evaluation to be inaccurate or subjective were more likely to have lower levels of teacher satisfaction.
What has received less empirical attention is the perspective of teachers on the use of student test scores in their appraisals/evaluations. In their international, large-scale study of TALIS data, Smith and Kubacka ( 2017 ) found that the overemphasis of student test scores in teacher appraisals was related to increased perceptions of the appraisal being an administrative task that carries little relevance for classroom practice. This result is similar to what other studies from the USA have found. Collins ( 2014 ) surveyed the teachers of Houston Independent School District, which is known for having one of the strongest high-stakes teacher evaluation systems in the USA, about their experiences with their VAM-based teacher evaluation. One of the most prominent things she found was that teachers perceived little to no utility associated with their VAM scores or reports. The teachers claimed the reports to be too vague or unclear to produce any meaningful guidance for classroom practice. In fact, ‘almost 60% of the teachers in this study reported that they do not use their SAS EVAAS® data for formative purposes whatsoever’ (Collins 2014 , p. 22). The participants also reported that VAM does not improve working conditions or enhance the school environment. Pizmony-Levy and Woolsey ( 2017 ) found similar results in their survey research with New Jersey teachers about their high-stakes teacher evaluation system. Their participants noted effects on classroom practice. They felt the emphasis on test scores forced them to teach to the test and remove non-tested content from their lessons. They also expressed concerns about the validity and fairness of evaluating teachers on student achievement scores.
Similarly, Hewitt ( 2015 ) looked at teachers’ perceptions of a teacher evaluation system that included VAM scores in North Carolina. She found that, amongst other things, such systems had a profound impact on levels of stress, pressure, and anxiety. She also noted that a majority of teachers did not fully understand VAM or how to incorporate VAM outputs into their decisions about how to improve their practice. Overall, her participants reported feeling sceptical about the utility, fairness, or accuracy of VAM.
These issues point to potential problems principals must consider when thinking of incorporating and emphasizing student test scores in teacher appraisals. Broadly, when schools use test scores and appraisals in formative ways, there is a greater chance that teachers appreciate the feedback as a useful data point. Otherwise, when the scores are used in high-stakes and summative ways to label teacher quality and determine personnel decisions, teachers seem to feel greater pressure and more frustration. Building from these previous findings, we sought to more explicitly investigate the relationship between teacher satisfaction and the use of student test scores in teacher appraisal.
4 This study
Teacher appraisals/evaluations represent a relatively unexamined pathway that could help explain the relationship between test-based accountability and teacher satisfaction. Results from Burns and Darling-Hammond ( 2014 ), suggesting that low levels of feedback utility are associated with reduced teacher satisfaction, hint at this connection. In a rare study, Lacierno-Paquet and colleagues (Lacireno-Paquet et al. 2016 ) found that teachers in the USA were 2.5 times less likely to be satisfied with the evaluation process when it included student test scores.
This study further explores whether teacher appraisals are one potential path to explain the reported relationship between test-based accountability and teacher satisfaction. The two primary research questions include:
What is the relationship between school testing culture and teacher satisfaction?
Is this relationship mediated by feedback received on teacher appraisals?
5 Data and methods
Data from the 2013 Teaching and Learning International Survey (TALIS) was used in this study. TALIS is administered by the OECD and includes a cross-national survey of teachers and school environments, focusing on lower secondary education. As the largest international survey of teachers, TALIS has been used extensively to research factors associated with teacher satisfaction at the global (OECD 2016 ), regional (for Eastern Europe example see Smith and Persson 2016 ), and national level (for USA example, see Ford et al. 2018 ; for Spain, see Gil-Flores 2017 ). Essential for this study, TALIS teacher and principal questionnaires include information capturing the primary independent variable, school testing culture, the dependent variable, teacher satisfaction, and information on the proposed mediation path, teachers’ perspectives on their appraisal. This study draws on information from the initial wave of participants from the 2013 TALIS, in which 33 countries or participating economies completed teacher and principal questionnaires. The stratified samples are nationally representative, with teachers nested in schools. Following Dicke et al. ( 2020 ) and Sun and Xia ( 2018 ), country surveys are combined into one pooled sample. Cases missing values on teacher satisfaction were dropped and missing data for the remaining analysis was dealt with through listwise deletion, producing a functional pooled sample for the final model of 66,592 teachers.
5.1 Dependent variable
The TALIS teacher questionnaire contains information on both general satisfaction with the profession and specific satisfaction with the school. Given that the school testing culture is unique to the school environment in which the teacher is employed, this study is limited to the latter. Following the approach of Smith and Persson ( 2016 ), teacher responses to three statements are included in the final job satisfaction variable: (1) ‘I would like to change to another school if possible’, (2) ‘I enjoy working at this school’, and (3) ‘I would recommend my school as a good place to work’. Statements are reverse coded as needed so that a score of 1 indicates satisfaction with the current place of employment. The aggregated variable has a range of 0 (not satisfied at all) to 3 (satisfaction indicated in all three statements). For path analysis, the teacher satisfaction variable is standardized with a mean of zero and a standard deviation of one. Coefficients for each pathway are then interpreted as a one-unit increase in the corresponding variable is associated with a change in teacher satisfaction, relative to the standard deviation (i.e. a coefficient of 0.2 suggests a one-unit increase in the corresponding variables is associated with a 0.2 standard deviation increase in teacher satisfaction).
5.2 Predictor variables
Two variables are used to measure the presence of a school testing culture. First, a dichotomous variable captures whether student test scores are included in the teacher’s appraisal (1 = yes; 0 = no). However, given recent research indicating that over 95% of teacher appraisals include student test scores (Smith and Kubacka 2017 ), a second measure is included for a more fine-grained analysis. To capture the extent to which teachers are held responsible for student test scores, principal responses to the statement ‘I took actions to ensure that teachers feel responsible for their students’ learning outcomes’ are included. The teachers are responsible variable ranges from 0 (principal never or rarely took action) to 3 (principal very often took action). An independent samples t test identified a significant relationship between the two school testing culture variables ( t = − 24.417, df = 91,088, p < .01) in the expected direction, suggesting the two variables capture a similar construct. While many structural equation models include a large set of variables in their measurement models, given the strength of the relationship between the school testing culture variables and the suggestions by Hayduk and Littvay ( 2012 ) when considering whether many or few indicators should be included in structural equation modeling that ‘using the few best indicators...encourages development of theoretically sophisticated models’ (p. 1), we are confident that these variables capture, at a minimum, a key part of the pressure felt by teachers in test-based accountability systems.
The mediation pathway through teacher appraisal feedback consists of two variables. To capture whether student test scores are emphasized in appraisal feedback, this study follows that of Smith and Kubacka ( 2017 ). To identify which parts of teacher appraisal are emphasized, teachers are asked to evaluate eleven potential areas of feedback. Each area is coded on a Likert scale from 0 (not considered at all when feedback is received) to 3 (considered with high importance). The relative emphasis score is then calculated by taking the difference between the score related to student achievement and the mean score of the ten other potential areas of emphasis (see Eq. 1 ). Values over 0, therefore, indicate that student test scores were relatively more emphasized in teacher appraisal feedback, in comparison to the average score of other areas.
The second variable in the teacher appraisal pathway captures the extent teachers feel the feedback they received had a direct, positive effect on their job satisfaction. Teacher responses ranged from 0 (feedback had no positive change on my job satisfaction) to 3 (feedback had a largely positive change on my job satisfaction).
5.3 Control variables
Four control variables are included at the teacher level: sex, age, years of education, and education level. Teacher’s sex is coded 1 for female and 0 for male. Years of experience is a continuous variable that captures the years the teacher has spent at their current school. Age is a continuous variable that captures the age of the teacher. Education level is treated as an ordinal variable and coded from 1 for below ISCED level 5 (completion of secondary or below) to 4 for ISCED level 6 or above (completion of bachelors’ degree or above).
5.4 Analytic strategy
Descriptive statistics were calculated to provide an initial illustration of all key variables. This was followed by a preliminary bivariate analysis to evaluate the initial association between independent and mediating variables with teacher satisfaction. Independent t tests were performed to examine the mean difference in satisfaction by whether test scores were included as a component in the teacher appraisal. Pearson correlation coefficients are calculated to compare all continuous variables.
Multi-level structural equation modeling (SEM) is employed for the primary analysis. The approach in this study is similar to Sun and Xia ( 2018 ) who draw on a pooled sample of teachers across all participating countries in the 2013 TALIS and apply multi-level SEM to predict the relationship between distributed leadership and teacher job satisfaction. Multi-level SEM is appropriate for this analysis as it takes into consideration the nested characteristic of the data—with teachers nested in schools nested in countries (Hox 2013 ). Additionally, SEM allows us to distinguish between (a) the direct effect of school testing culture on teacher satisfaction and (b) the indirect effects of school testing culture on teacher satisfaction through teacher appraisal feedback (Schumacker and Lomax 2004 ). All results are presented graphically to ease interpretation (Hox 2010 ) and computed using the gsem option in Stata v14 (Huber 2013 ).
The full model (shown in Fig. 1 ) is completed through three additive steps. The baseline model predicts the direct effect of school testing culture on teacher satisfaction (paths A and B) and includes teacher-level control variables (path X). The second model adds the impact of teachers’ positive perception of appraisal feedback (path G) to evaluate whether teacher appraisal feedback is a potential mediating mechanism. Model 3 completes the full multi-level model by adding test score emphasis in appraisal feedback and school and country-level error terms. To aid convergence, covariance of exogenous upper-level latent variables (school and country) are constrained (Huber 2013 ) Footnote 1 and not displayed in the results. Each model assumes exogenous variables are correlated. Error terms for each endogenous variable are included for each model and provided in the notes for each figure. The final total effect of school testing culture on teacher satisfaction is calculated as follows:
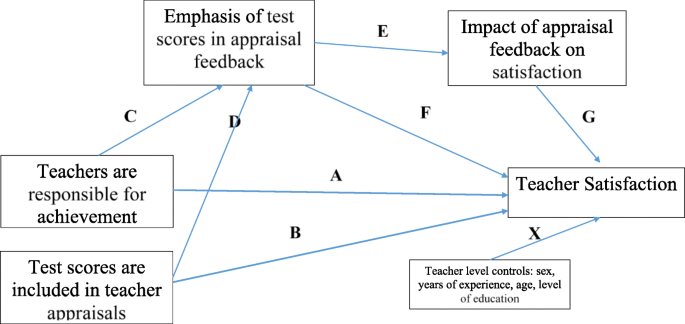
Full path analysis
5.5 Goodness of fit
As teacher satisfaction is the primary endogenous variable of interest, an r-squared or equation level goodness of fit was calculated to evaluate the precision of each model in predicting teacher satisfaction. While the gsem command in Stata provides flexibility in allowing the inclusion of multiple levels, it has limited options for model fit. Given the minimal differences in output using gsem and alternative approaches (see footnote 1), a single-level model was assumed to calculate the r-squared. Included in the notes for each figure, the r-squared illustrates the amount of variance in teacher satisfaction captured by each model.
6.1 Preliminary analysis
Descriptive statistics are found in Table 1 . In the pooled sample, teachers report relatively high levels of satisfaction with their current place of employment (mean = 2.528, SD = .849). Additionally, a fairly substantial school testing culture appears to be the norm. The mean value (1.976, SD = .719) suggests that the average principal often takes actions to ensure teachers know they are responsible for student outcomes and nearly 97% of teachers have student test scores incorporated into their teacher appraisal. Finally, for the teacher appraisal mediation pathway, the emphasis on student test scores is higher than the mean of other potential pieces of feedback (mean = .363, SD = .643) and teachers, on average, report feedback resulting in a small to moderate change in their satisfaction (mean = 1.796, SD = .987).
Bivariate analysis revealed few initial relationships between school testing culture variables or teacher appraisal feedback variables and teacher satisfaction. An independent samples t test found no significant relationship between including student test score in the appraisal and teacher satisfaction ( t = 1.454, df = 86,853, p = .93). Amongst Pearson correlation coefficients, no relationships are significant and the only correlation above ± .2 is the correlation between whether the teacher believed the appraisal feedback had a positive impact on their satisfaction and their overall satisfaction level ( r = .229).
6.2 Primary analysis
Our baseline path analysis (Fig. 2 ) reveals coefficients in the expected direction, but neither ensuring teachers know they are responsible for student achievement ( β = − .003, p = .51) or the inclusion of test scores in appraisals ( β = − .032, p = .11) are significantly related to teacher satisfaction, after controlling for teacher demographic variables. Female ( β = .058, p < .01) and older teachers ( β = .004, p < .01) tend to be more satisfied. In addition, those with lower levels of education are more satisfied ( β = − .096, p < .01). Teacher years of education are not significantly related to their satisfaction. Direction, magnitude, and significance levels of all control variables remained largely consistent across all models.
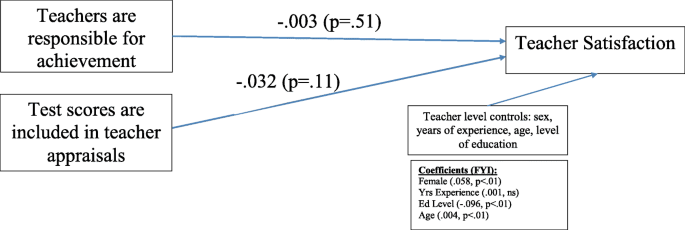
Baseline analysis—Direct effect of school testing culture on teacher satisfaction ( n = 81,361). Notes : r-squared for teacher satisfaction = .004. Measurement error for teacher satisfaction ( β = 1.010)
In the second model, we add part of the teacher appraisal feedback pathway to examine the potential benefits of feedback on teacher satisfaction. The results (Fig. 3 ) illustrate that teachers that view their feedback as positively impacting their satisfaction are more likely to report higher levels of overall satisfaction ( β = .235, p < .01). The increased magnitude of school testing culture coefficients and change from non-significant to significantly related to teacher satisfaction suggest that one avenue the overall school climate is influencing teacher satisfaction is through their individual interaction with appraisals and appraisal feedback.
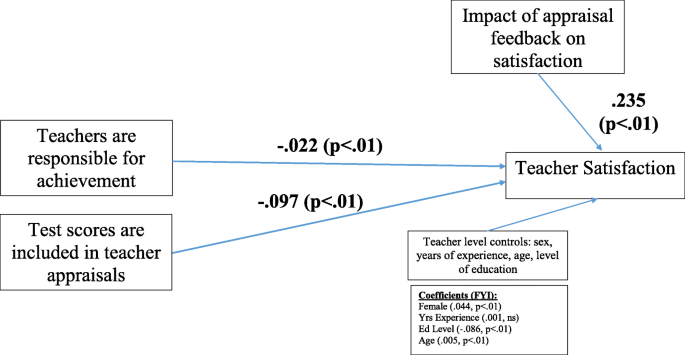
Model 2—Exploring the Potential benefits of appraisal feedback ( n = 70,613). Notes : r-squared for teacher satisfaction = .059. Measurement error for teacher satisfaction ( β = .921)
The full model (Fig. 4 ) completes the hypothesized mediation pathway by including whether student test scores are emphasized in appraisal feedback and provides, marginally, the best fit for predicting teacher satisfaction (r-squared = .060). Here, it is clear that school testing culture has both direct effects on teacher satisfaction and indirect effects on teacher satisfaction through the teacher appraisal feedback pathway. In the full model, which controls for teacher demographics, the inclusion of test scores in teacher appraisals is directly related to a .103 ( p < .01) standard deviation reduction in teacher satisfaction. Furthermore, a one-unit increase in principals ensuring teachers are responsible for student outcomes is associated with a .023 ( p < .01) standard deviation decrease in teacher satisfaction.
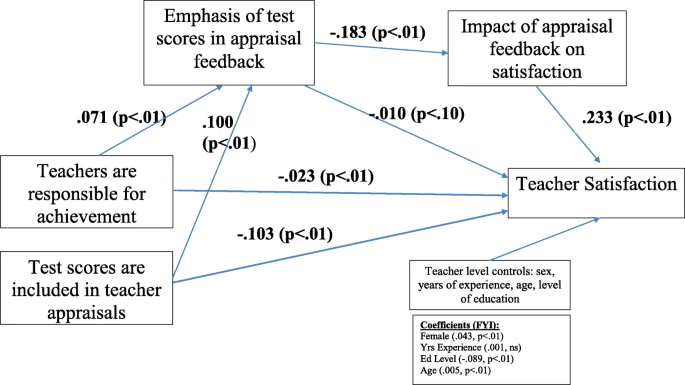
Full model—Direct and indirect effect of school testing culture on teacher satisfaction ( n = 66,592). Notes : Full model controls for school- and country-level effects. Error terms for both levels are regressed on teacher satisfaction and constrained to 1 (see “Data and Methods” section for more information). R-squared for teacher satisfaction = .060. Measurement error for teacher satisfaction ( β = .924). Measurement error for emphasis on test scores ( β = .409). Measurement error for the impact of appraisal on satisfaction ( β = .958)
Teachers in school testing cultures are more likely to have student test scores emphasized in their appraisal feedback (ensuring teachers are responsible, β = .071, p < .01; test scores in appraisal, β = .100, p < .01). Emphasizing test scores above other areas in teacher appraisal feedback is associated with a .010 ( p < .10) standard deviation decrease in satisfaction and reduces the likelihood that the teacher would state their feedback positively impacts their satisfaction ( β = − .183, p < .01) reducing any potential benefits from the teacher appraisal pathway.
Figure 5 provides the total unstandardized effect of school testing culture on teacher satisfaction by intensity of testing culture. Total effects are calculated using Eq. 2 (see above). The figure predicts teacher satisfaction by setting all control variables to the mean and assuming the teacher’s sex is female. The first bar indicates that a female teacher of average age, years of experience, and education level would have a satisfaction score of 2.16. As the school testing culture intensifies the predicted satisfaction score decreases from 2.16 to 1.87. Of the net drop of 0.29 points, the direct effect of school testing culture accounts for 59% of the reduction while the indirect effect through teacher appraisal feedback accounts for approximately 41%.
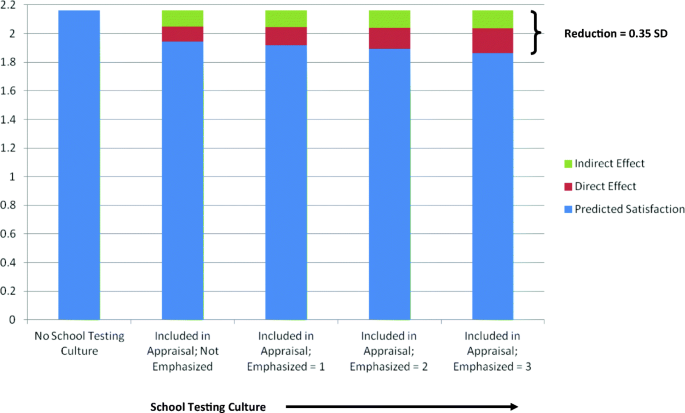
Total unstandardized effect of school testing culture on teacher satisfaction
In terms of standard deviation, the difference between no school testing culture and the most intense testing culture is 0.35. Although an effect size of 0.35 standard deviations would be considered between small and medium by Cohen ( 1969 ), it would be considered ‘substantively important’ (SD > .25) by the What Works Clearinghouse (WWC 2014 , p. 23). While teacher satisfaction and student achievement are distinct dependent variables making it difficult to compare, the total effect size is also larger than the average effect size (SD = .28) on student achievement across 124 random trials (Lipsey et al. 2012 ) or reported effects of individualized tutoring (SD = .23, Cook et al. 2015 ) or universal free school lunch (SD = .09, Frisvold 2015 ) on math test score.
7 Concluding discussion
The global increase in the use of student test scores to hold teachers’ accountability has seen a rush of scholars working to understand how the trend has impacted policy and practice. There has been a great deal of empirical studies that have looked specifically at the effects of teachers, ranging from large-scale survey studies (e.g. Collins 2014 ; Pizmony-Levy and Woolsey 2017 ) to small-scale qualitative studies (e.g. Garver 2019 ; Hardy 2018 ; Perryman 2009 ). There have also been a number of studies that have looked at measurement issues related to using student test scores to measure teacher quality (see Darling-Hammond 2015 for a review). While these studies focus on a wide range of topics and contexts, what most of them have in common is that their findings and conclusions indicate a troubled relationship between the use of student test scores in teacher accountability and how teachers feel about their practice and work-place conditions. This is particularly pronounced in systems where the stakes for teachers are high, such as in the USA and the UK. Not only are researchers finding that teachers are significantly modifying their practice in response to these sorts of accountability systems, which has been a long-standing concern about testing more generally (Amrein and Berliner 2002 ; Nichols and Berliner 2007 ; Ravitch 2016 ), but it has also begun to influence the way teachers feel about their work and their professional identity (Brass and Holloway 2019 ; Garver 2019 ; Perryman 2009 ).
Our research extends past studies, providing a more nuanced view of the relationship between test-based accountability and teacher satisfaction. First, our results support past research that draws a direct line between an increased focus on student testing and decreased teacher satisfaction. Second, it is clear that the use and perception of teacher appraisals have an important role to play regarding teacher satisfaction. Our findings suggest that teacher appraisals are not pre-destined to have a negative impact on overall teacher satisfaction. In fact, when not emphasizing student test scores, teacher appraisals can boost teacher satisfaction. This is an important finding because it aligns with what many scholars and education leaders have argued for a while now—that appraisal and accountability are not in and of themselves bad for teachers and schooling (Darling-Hammond 2014 ; Darling-Hammond et al. 2012 ). However, what many of these researchers and their more critical colleagues (e.g. Perryman 2009 ; Perryman and Calvert 2019 ; Holloway and Brass 2018 ; Hursh 2007 ; Lipman 2004 ) have argued is that it is the pervasiveness of the testing culture, and the overemphasis on student test scores in teacher appraisals, that is having a profoundly negative effect on teachers and their practice. Our current study provides another layer to our understanding about this phenomenon. Once established, the school testing culture appears to both directly relate to teacher dissatisfaction and reduce the potential benefits of teacher appraisals by indirectly and negatively influencing teacher satisfaction by warping the teacher appraisal process. This is similar to what Ford et al. ( 2018 ) found in their study—that the degree to which teachers view the utility of appraisal and feedback is closely related to their satisfaction. Ultimately, as the testing culture intensifies, teachers’ overall satisfaction decreases.
These findings are not without limitations. First, while the results make clear that the common global trends associated with the increased emphasis on student test scores appear to be reflected in the school testing culture, potentially harming teacher satisfaction, the school testing culture and school appraisal mediation pathway only capture a limited level of variance in teacher satisfaction (r-squared = .060). While school testing culture may play a small, but important role, many more factors such as self-efficacy (Kasalak and Dağyar 2020 ), teachers involvement in decision-making (Smith and Persson 2016 ) and distributed leadership within the school (Sun and Xia 2018 ) should be considered to get a full understanding of teacher satisfaction. Additionally, TALIS data includes information from both the principal (i.e. school testing variables) and teacher (i.e. appraisal and teacher satisfaction variables). Past research has suggested that principals and teachers have different perceptions related to school climate (Casteel 1994 ), including whether or not teachers are satisfied (Dicke et al. 2020 ). Teacher self-reported satisfaction is used in this study and we believe appropriately captures the affective relationship between the teacher and the school. Still, the perceptions of teachers may not represent those of other actors within the school.
Given our results, we strongly urge school leaders to consider carefully the ways they use student test scores, as well as appraisals more broadly. Situating student test scores amongst multiple indicators can partially mitigate, but is unlikely to remove, the pressure felt by the school testing culture. Even appraisals that include multiple metrics, appearing more holistic, often end with principals emphasizing student test scores above other components (Smith and Kubacka 2017 ). School leaders need to be cautious when including student test scores. If used, they need to be treated as a source of formative feedback, rather than as a summative judgment about the teacher’s quality or ability. This has serious implications for policy and practice, which are described next.
7.1 Implications for policy, practice, and future research
While this study adds to our overall understanding about the impact of appraisal on teacher satisfaction, it also prompts further questions about the utility of evaluation and the use of student test scores in holding teachers accountable. This is especially important if we consider this in line with current trends that prioritize numerical data for making sense of school and teacher quality more broadly. In this way, we argue that there is a critical need for school leaders to grapple with the various approaches to appraisal, as well as how appraisals and student test scores might be used in more formative ways. One way this could be achieved is through training and ongoing professional development for principals and other school leaders. Training on topics such as data literacy, assessment, and accountability might incorporate sections on how to use such techniques in ways that support teacher development. This might help leaders navigate the complicated relationship between being not only evidence-driven but also supportive of teacher wellbeing and growth.
However, training and professional development can only achieve so much if policies continue to prioritize high-stakes testing as a means for identifying school and teacher quality. Principals are left with little discretionary space if there are policies that require them to use test scores and appraisals for making personnel decisions (e.g. promotion, performance-based pay). This is where countries like the USA and the UK might benefit from considering how other countries are taking a more holistic and formative approach to test score use.
We acknowledge that ‘satisfaction’ is a difficult construct to measure, and knowing specifically how satisfaction might affect teacher practice or subsequent decisions about whether to remain in the classroom is hard to say at this time. There is a growing criticism of the testing culture, coming from a variety of perspectives (e.g. from governments to teacher organizations; see Strauss 2012a , b ), with a particular warning about how these conditions are creating a dire environment for teachers (Perryman and Calvert 2019 ). For example, concerns about teacher shortages, decreased interest in becoming teachers amongst young people, and teachers’ personal and professional wellbeing, have all been highlighted in calls for reduction to the widespread testing culture. Therefore, we need more research about how the testing culture is changing the make-up of the profession (e.g. are teacher shortages related to increased accountability and testing?), especially with regard to student test scores. We add the findings of the current study to these considerations by urging school leaders and policymakers to weigh critically the purpose and consequences of test-based appraisals. As we have shown, it is possible for teachers to have high levels of satisfaction within schools that use teacher appraisals. However, this relationship changes as the intensity of the testing culture increases, which signals time for reflection on how the pervasiveness of the testing culture can be challenged.
An alternative two-level approach using the vce (cluster) command at the school level to relax the assumption of independence of observations and adjust standard errors did not substantially change the value of coefficients or the level of significance of results.
Aldridge, J. M., & Fraser, B. J. (2016). Teachers’ views of their school climate and its relationship with teacher self-efficacy and job satisfaction. Learning Environments Research, 19 , 291–307.
Article Google Scholar
American Educational Research Association. (2015). AERA statement on use of value-added models (VAM) for the evaluation of educators and educator preparation programs. Educational Researcher, 44 (8), 448–452.
Amrein-Beardsley, A. (2014). Rethinking value-added models in education: critical perspectives on tests and assessment-based accountability . London: Routledge.
Amrein-Beardsley, A., & Holloway, J. (2017). Value-added models for teacher evaluation and accountability: commonsense assumptions. Educational Policy, 33 (3), 516–542.
Amrein, A. L., & Berliner, D. C. (2002). High-stakes testing & student learning. Education policy analysis archives, 10 , 18.
Ballou, D., & Springer, M. G. (2015). Using student test scores to measure teacher performance: Some problems in the design and implementation of evaluation systems. Educational Researcher, 44 (2), 77–86.
Barnes, S.-A., Lyonette, C., Atfield, G., & Owen, D. (2016). Teachers’ pay and equality: a literature review – longitudinal research into the impact of changes to teachers’ pay on equality in schools in England . Warwickshire: Warwick Institute for Employment Research.
Google Scholar
Booher-Jennings, J. (2005). Below the bubble: ‘Educational triage’ and the Texas accountability system. American Education Research Journal, 42 (2), 231–268.
Brass, J., & Holloway, J. (2019). Re-professionalizing teaching: the new professionalism in the United States. Critical Studies in Education , 1–18.
Breakspear, S. (2014). How does PISA shape education policy making. In Why how we measure learning determines what counts in education, CSE Seminar series (Vol. 240). Melbourne: Centre for Strategic Education.
Brezicha, K. F., Ikoma, S., Park, H., & LeTendre, G. K. (2019). The ownership perception gap: exploring teacher satisfaction and its relationship to teachers’ and principals’ perception of decision-making opportunities. International Journal of Leadership in Education , 1–29.
Burns, D., & Darling-Hammond, L. (2014). Teaching around the world: what can TALIS tell us . Stanford: Stanford Center for Opportunity Policy in Education.
Casteel, D. B. (1994). Principal and teacher perceptions of school climate related to value-added assessment and selected school contextual effects in the First Tennessee District. PhD Dissertation. East Tennessee State University.
Certo, J. L. (2006). Beginning teacher concerns in an accountability-based testing environment. Journal of Research in Childhood Education, 20 (4), 331–349.
Close, K., Amrein-Beardsley, A., & Collins, C. (2019). Mapping America’s teacher evaluation plans under ESSA. Phi Delta Kappan, 101 (2), 22–26.
Cohen, J. (1969). Statistical power analysis for the behavioral sciences (1st ed.). New York: Academic Press.
Collins, C. (2014). Houston, we have a problem: teachers find no value in the SAS education value-added assessment system (EVAAS®). Education Policy Analysis Archives, 22 , 98.
Collins, C., & Amrein-Beardsley, A. (2014). Putting growth and value-added models on the map: a national overview. Teachers College Record, 116 (1), 1–32.
Cook, P. J., Dodge, K., Farkas, G., Fryer, R. G., Guryan, J., Ludwig, J., & Mayer, S. (2015). Not too late: improving academic outcomes for disadvantaged youth. Working paper WP-15-01. Northwestern University: Institute for Policy Research.
Darling-Hammond, L. (2014). One piece of the whole: teacher evaluation as part of a comprehensive system for teaching and learning. American Educator, 38 (1), 4.
Darling-Hammond, L. (2015). Can value added add value to teacher evaluation? Educational Researcher, 44 (2), 132–137.
Darling-Hammond, L., Amrein-Beardsley, A., Haertel, E., & Rothstein, J. (2012). Evaluating teacher evaluation. Phi Delta Kappan, 93 (6), 8–15.
Dicke, T., Marsh, H. W., Parker, P. D., Guo, J., Riley, P., & Waldeyer, J. (2020). Job satisfaction of teachers and their principals in relation to climate and student achievement. Journal of Educational Psychology, 112 (5), 1061–1073.
Everson, K. C. (2017). Value-added modeling and educational accountability: are we answering the real questions? Review of Educational Research, 87 (1), 35–70.
Ford, T. G., Urick, A., & Wilson, A. S. (2018). Exploring the effect of supportive teacher evaluation experiences on US teachers’ job satisfaction. Education Policy Analysis Archives, 26 , 59.
Frisvold, D. E. (2015). Nutrition and cognitive achievement: an evaluation of the school breakfast program. Journal of Public Economics, 124 , 91–104.
Garver, R. (2019). Evaluative relationships: teacher accountability and professional culture. Journal of Education Policy , 1–25.
Gil-Flores, J. (2017). The role of personal characteristics and school characteristics in explaining teacher job satisfaction. Revista de Psicodidáctica/Journal of Psychodidactics, 22 (1), 16–22.
Grayson, J. L., & Alvarez, H. K. (2008). School climate factors related to teacher burnout: a mediator model. Teaching and Teacher Education, 24 , 1349–1363.
Hanushek, E. A., & Rivkin, S. G. (2010). Generalizations about using value-added measures of teacher quality. American Economic Review, 100 (2), 267–271.
Hardy, I. (2018). Governing teacher learning: understanding teachers’ compliance with and critique of standardization. Journal of Education Policy, 33 (1), 1–22.
Hayduk, L. A., & Littvay, L. (2012). Should researchers use single indicators, best indicators, or multiple indicators in structural equation models? BMC Medical Research Methodology, 12 , 159.
Hewitt, K. K. (2015). Educator evaluation policy that incorporates EVAAS value-added measures: undermined intentions and exacerbated inequities. Education Policy Analysis Archives, 23 (76).
Holloway, J. (2019). Teacher evaluation as an onto-epistemic framework. British Journal of Sociology of Education, 40 (2), 174–189.
Holloway, J., & Brass, J. (2018). Making accountable teachers: the terrors and pleasures of performativity. Journal of Education Policy, 33 (3), 361–382.
Holloway, J., Sørensen, T. B., & Verger, A. (2017). Global perspectives on high-stakes teacher accountability policies: an introduction. Education Policy Analysis Archives, 25 (85), 1–18.
Hox, J. J. (2013). Multilevel regression and multilevel structural equation modeling. The Oxford handbook of quantitative methods, 2 (1), 281–294.
Hox, J. J. (2010). Multilevel analysis: Techniques and applications (Second ed.). New York: Routledge.
Book Google Scholar
Huber, C. (2013). Generalized structure equation modelling using Stata. Presentation at Italian Stata Users Group Meeting (Florence, Italy) , November 14–15, 2013.
Hursh, D. (2007). Assessing no child left behind and the rise of neoliberal education policies. American Educational Research Journal, 44 (3), 493–518.
Johnson, S. M. (2015). Will VAMS reinforce the walls of the egg-crate school? Educational Researcher, 44 (2), 117–126.
Kasalak, G., & Dağyar, M. (2020). The relationship between teacher self-efficacy and teacher job satisfaction: a meta-analysis of the Teaching and Learning International Survey (TALIS). Educational Sciences: Theory and Practice, 20 (3), 16–33.
Koedel, C., & Betts, J. R. (2011). Does student sorting invalidate value-added models of teacher effectiveness? An extended analysis of the Rothstein critique. Education Finance and policy, 6 (1), 18–42.
Koedel, C., Mihaly, K., & Rockoff, J. E. (2015). Value-added modeling: A review. Economics of Education Review, 47 , 180–195.
Lacireno-Paquet, N., Bocala, C., & Bailey, J. (2016). Relationship between school professional climate and teachers’ satisfaction with the evaluation process. (REL 2016–133) . Washington, DC: U.S. Department of Education, Institute of Education Sciences, National Center for Education Evaluation and Regional Assistance, Regional Educational Laboratory Northeast & Islands.
Larsen, M. A. (2005). A critical analysis of teacher evaluation policy trends. Australian Journal of Education, 49 (3), 292–305.
Lewis, S., & Holloway, J. (2019). Datafying the teaching ‘profession’: remaking the professional teacher in the image of data. Cambridge Journal of Education, 49 (1), 35–51.
Lipman, P. (2004). High stakes education: inequality, globalization, and urban school reform . London: Routledge.
Lipsey, M. W., Puzio, K., Yun, C., Hebert, M. A., Steinka-Fry, K., Cole, M. W., et al. (2012). Translating the statistical representation of the effects of education interventions into more readily interpretable forms . Washington, D.C.: National Center for Special Education Research.
Liu, S., Xu, X., & Stronge, J. (2018). The influences of teachers’ perceptions of using student achievement data in evaluation and their self-efficacy on job satisfaction: evidence from China. Asia Pacific Education Review, 19 , 493–509.
Muller, M., & Boutte, G. S. (2019). A framework for helping teachers interrupt oppression in their classrooms. Journal for Multicultural Education., 13 , 94–105. https://doi.org/10.1108/JME-09-2017-0052 .
Murphy, J., Hallinger, P., & Heck, R. H. (2013). Leading via teacher evaluation. Educational Researcher, 42 , 349–354.
Nichols, S. L., & Berliner, D. C. (2007). Collateral damage: how high-stakes testing corrupts America’s schools . Cambridge, MA: Harvard Education Press.
OECD. (2014). TALIS 2013 results: an international perspective on teaching and learning . Paris: Organisation for Economic Cooperation and Development.
Perryman, J., & Calvert, G. (2019). What motivates people to teach, and why do they leave? Accountability, performativity and teacher retention. British Journal of Educational Studies , 68(1), 3–23.
OECD. (2016). Supporting teacher professionalism . Paris: OECD.
Perryman, J. (2009). Inspection and the fabrication of professional and performative processes. Journal of Education Policy, 24 (5), 611–631.
Pizmony-Levy, O., & Woolsey, A. (2017). Politics of education and teachers’ support for high-stakes teacher accountability policies. Education Policy Analysis Archives, 25 , 87.
Polesel, J., Rice, S., & Dulfer, N. (2014). The impact of high-stakes testing on curriculum and pedagogy: a teacher perspective from Australia. Journal of Education Policy, 29 (5), 640–657.
Price, H. E. (2012). Principal-teacher interactions: how affective relationships shape principal and teacher attitudes. Educational Administration Quarterly, 48 (1), 39–85.
Ravitch, D. (2016). The death and life of the great American school system: how testing and choice are undermining education . New York: Basic Books.
Rothstein, J. (2010). Teacher quality in educational production: tracking, decay, and student achievement. The Quarterly Journal of Economics, 125 (1), 175–214.
Schumacker, R. E., & Lomax, R. G. (2004). A beginner’s guide to structural equation modeling . London: Psychology Press.
Smith, W. C. (2014). The global transformation toward testing for accountability. Education Policy Analysis Archives, 22 (116).
Smith, W. C. (2016). The global testing culture: shaping education policy, perceptions, and practice . Oxford: Symposium Books.
Smith, W. C., & Kubacka, K. (2017). The emphasis of student test scores in teacher appraisal systems. Education Policy Analysis Archives, 25 (86).
Smith, W. C., & Persson, A. M. (2016). Teacher satisfaction in high poverty schools: searching for policy relevant interventions in Estonia, Georgia, and Latvia. Educational Studies Moscow, 2 , 146–182.
Sørensen, T.B. (2016). Value-added measurement or modelling (VAM). Education international discussion paper. Available at: https://worldsofeducation.org/en/woe_homepage/woe_detail/14860/discussion-paper-value-added-measurement-or-modelling-vam
Strauss, V. (2012a). Moco schools chief calls for three-year moratorium on standardized testing. Washington Post. Available at: https://www.washingtonpost.com/news/answer-sheet/wp/2012/12/10/moco-schools-chief-calls-for-three-year-moratorium-on-standardized-testing/ . Accessed 23 Mar 2020.
Strauss, V. (2012b). Texas schools chief calls testing obsession a ‘perversion’. Washington Post. Available at: https://www.washingtonpost.com/blogs/answer-sheet/post/texas-schools-chief-calls-testing-obsession-a-perversion/2012/02/05/gIQA5FUWvQ_blog.html . Accessed 23 Mar 2020.
Sun, A., & Xia, J. (2018). Teacher-perceived distributed leadership, teacher self-efficacy and job satisfaction: a multilevel SEM approach using TALIS 2013 data. International Journal of Educational Research, 92 , 86–97.
UNESCO. (2017). Accountability in education: meeting our commitments . Paris: UNESCO.
Verger, A., & Parcerisa, L. (2017). A difficult relationship: accountability policies and teachers—International Evidence and Premises for Future Research. In Akiba, M. & LeTendre, G. K. (eds.), International handbook of teacher quality and policy (pp. 241–254). London: Routledge.
Warren, A. N., & Ward, N. A. (2018). ‘This is my new normal’: teachers’ accounts of evaluation policy at local school board meetings. Journal of Education Policy, 33 (6), 840–860.
WWC (What Works Clearinghouse). (2014). WWC procedures and standards handbook (Version 3.0) . Washington, DC: U.S. Department of Education, Institute of Education Sciences, National Center for Education Evaluation and Regional Assistance, What Works Clearinghouse.
Wiggins, A. & Tymms, P. (2000). Dysfunctional effects of public performance indicator systems: a comparison between English and Scottish primary schools. Paper presented at the European Conference on Educational Research (Edinburgh, UK) , 20-23 September, 2000.
Wronowski, M. L., & Urick, A. (2019). Examining the relationship of teacher perception of accountability and assessment policies on teacher turnover during NCLB. Education Policy Analysis Archives, 27 (86).
Xiaofu, P., & Qiwen, Q. (2007). An analysis of the relation between secondary school organizational climate and teacher job satisfaction. Chinese Education & Society, 40 (5), 65–77.
Xu, X., Grant, L. W., & Ward, T. J. (2016). Validation of a statewide teacher evaluation system. NASSP Bulletin, 100 (4), 203–222.
Download references
Author information
Authors and affiliations.
Moray House School of Education and Sport, University of Edinburgh, Edinburgh, UK
William C. Smith
Research for Educational Impact (REDI) Centre, Deakin University, Melbourne, Australia
Jessica Holloway
You can also search for this author in PubMed Google Scholar
Corresponding author
Correspondence to William C. Smith .
Additional information
Publisher’s note.
Springer Nature remains neutral with regard to jurisdictional claims in published maps and institutional affiliations.
Rights and permissions
Open Access This article is licensed under a Creative Commons Attribution 4.0 International License, which permits use, sharing, adaptation, distribution and reproduction in any medium or format, as long as you give appropriate credit to the original author(s) and the source, provide a link to the Creative Commons licence, and indicate if changes were made. The images or other third party material in this article are included in the article's Creative Commons licence, unless indicated otherwise in a credit line to the material. If material is not included in the article's Creative Commons licence and your intended use is not permitted by statutory regulation or exceeds the permitted use, you will need to obtain permission directly from the copyright holder. To view a copy of this licence, visit http://creativecommons.org/licenses/by/4.0/ .
Reprints and permissions
About this article
Smith, W.C., Holloway, J. School testing culture and teacher satisfaction. Educ Asse Eval Acc 32 , 461–479 (2020). https://doi.org/10.1007/s11092-020-09342-8
Download citation
Received : 03 February 2020
Accepted : 28 October 2020
Published : 05 November 2020
Issue Date : November 2020
DOI : https://doi.org/10.1007/s11092-020-09342-8
Share this article
Anyone you share the following link with will be able to read this content:
Sorry, a shareable link is not currently available for this article.
Provided by the Springer Nature SharedIt content-sharing initiative
- Teacher satisfaction
- Accountability
- Testing culture
- Teacher appraisal
- Find a journal
- Publish with us
- Track your research
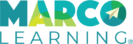
Importance of Validity and Reliability in Classroom Assessments
One of the following tests is reliable but not valid and the other is valid but not reliable. Can you figure out which is which?
- You want to measure student intelligence so you ask students to do as many push-ups as they can every day for a week.
- You want to measure students’ perception of their teacher using a survey but the teacher hands out the evaluations right after she reprimands her class, which she doesn’t normally do.
Continue reading to find out the answer–and why it matters so much.
Validity and Reliability in Education
Schools all over the country are beginning to develop a culture of data , which is the integration of data into the day-to-day operations of a school in order to achieve classroom, school, and district-wide goals. One of the biggest difficulties that comes with this integration is determining what data will provide an accurate reflection of those goals.
Such considerations are particularly important when the goals of the school aren’t put into terms that lend themselves to cut and dry analysis; school goals often describe the improvement of abstract concepts like “school climate.”
Schools interested in establishing a culture of data are advised to come up with a plan before going off to collect it. They need to first determine what their ultimate goal is and what achievement of that goal looks like. An understanding of the definition of success allows the school to ask focused questions to help measure that success, which may be answered with the data.
For example, if a school is interested in increasing literacy, one focused question might ask: which groups of students are consistently scoring lower on standardized English tests? If a school is interested in promoting a strong climate of inclusiveness, a focused question may be: do teachers treat different types of students unequally?
These focused questions are analogous to research questions asked in academic fields such as psychology, economics, and, unsurprisingly, education. However, the question itself does not always indicate which instrument (e.g. a standardized test, student survey, etc.) is optimal.
If the wrong instrument is used, the results can quickly become meaningless or uninterpretable, thereby rendering them inadequate in determining a school’s standing in or progress toward their goals.
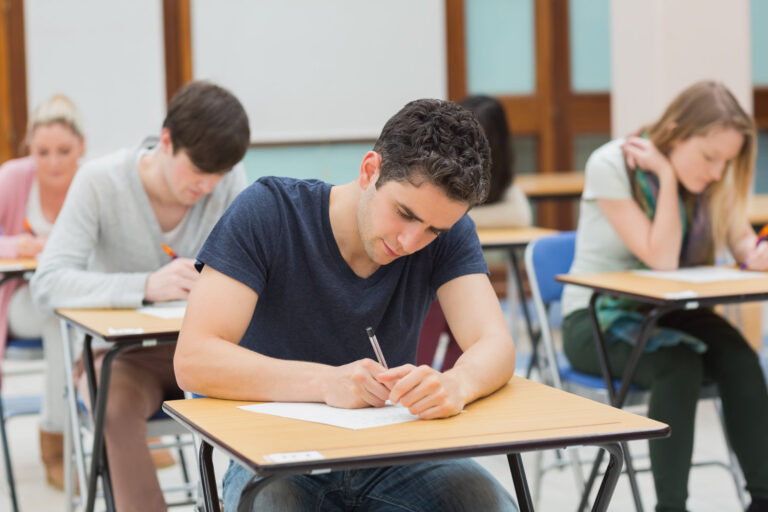
Differences Between Validity and Reliability
When creating a question to quantify a goal, or when deciding on a data instrument to secure the results to that question, two concepts are universally agreed upon by researchers to be of pique importance.
These two concepts are called validity and reliability, and they refer to the quality and accuracy of data instruments.
WHAT IS VALIDITY?
The validity of an instrument is the idea that the instrument measures what it intends to measure.
Validity pertains to the connection between the purpose of the research and which data the researcher chooses to quantify that purpose.
For example, imagine a researcher who decides to measure the intelligence of a sample of students. Some measures, like physical strength, possess no natural connection to intelligence. Thus, a test of physical strength, like how many push-ups a student could do, would be an invalid test of intelligence.
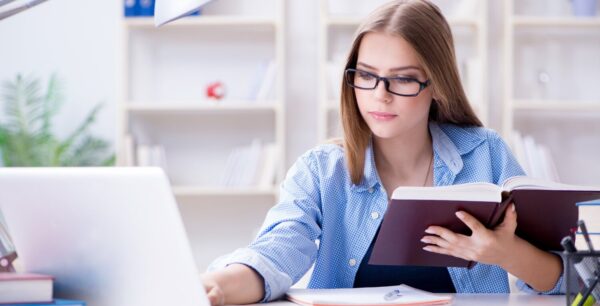
WHAT IS RELIABILITY?
Reliability , on the other hand, is not at all concerned with intent, instead asking whether the test used to collect data produces accurate results. In this context, accuracy is defined by consistency (whether the results could be replicated).
The property of ignorance of intent allows an instrument to be simultaneously reliable and invalid.
Returning to the example above, if we measure the number of pushups the same students can do every day for a week (which, it should be noted, is not long enough to significantly increase strength) and each person does approximately the same amount of pushups on each day, the test is reliable. But, clearly, the reliability of these results still does not render the number of pushups per student a valid measure of intelligence.
Because reliability does not concern the actual relevance of the data in answering a focused question, validity will generally take precedence over reliability . Moreover, schools will often assess two levels of validity:
- the validity of the research question itself in quantifying the larger, generally more abstract goal
- the validity of the instrument chosen to answer the research question
See the diagram below as an example:
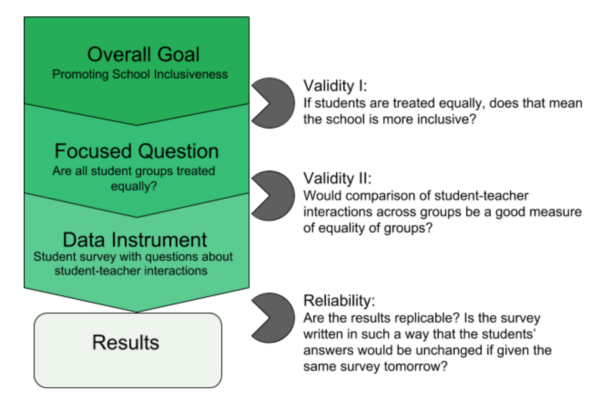
Although reliability may not take center stage, both properties are important when trying to achieve any goal with the help of data. So how can schools implement them? In research, reliability and validity are often computed with statistical programs. However, even for school leaders who may not have the resources to perform proper statistical analysis, an understanding of these concepts will still allow for intuitive examination of how their data instruments hold up, thus affording them the opportunity to formulate better assessments to achieve educational goals. So, let’s dive a little deeper.
A Deeper Look at Validity
The most basic definition of validity is that an instrument is valid if it measures what it intends to measure . It’s easier to understand this definition through looking at examples of invalidity. Colin Foster, an expert in mathematics education at the University of Nottingham, gives the example of a reading test meant to measure literacy that is given in a very small font size. A highly literate student with bad eyesight may fail the test because they can’t physically read the passages supplied. Thus, such a test would not be a valid measure of literacy (though it may be a valid measure of eyesight). Such an example highlights the fact that validity is wholly dependent on the purpose behind a test. More generally, in a study plagued by weak validity , “it would be possible for someone to fail the test situation rather than the intended test subject.” Validity can be divided into several different categories, some of which relate very closely to one another. We will discuss a few of the most relevant categories in the following paragraphs.
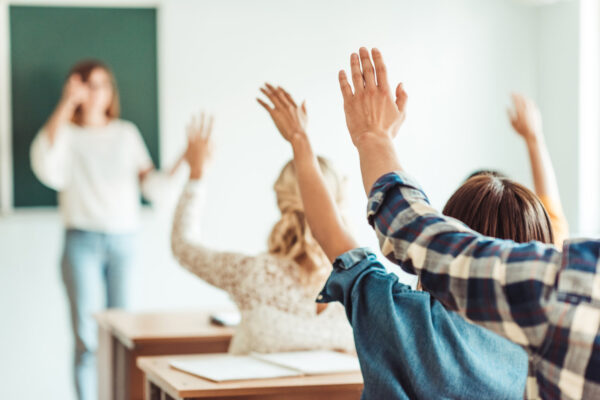
Types of Validity
WHAT IS CONSTRUCT VALIDITY?
Construct validity refers to the general idea that the realization of a theory should be aligned with the theory itself . If this sounds like the broader definition of validity, it’s because construct validity is viewed by researchers as “a unifying concept of validity” that encompasses other forms, as opposed to a completely separate type.
It is not always cited in the literature, but, as Drew Westen and Robert Rosenthal write in “ Quantifying Construct Validity: Two Simple Measures ,” construct validity “is at the heart of any study in which researchers use a measure as an index of a variable that is itself not directly observable.”
The ability to apply concrete measures to abstract concepts is obviously important to researchers who are trying to measure concepts like intelligence or kindness. However, it also applies to schools, whose goals and objectives (and therefore what they intend to measure) are often described using broad terms like “effective leadership” or “challenging instruction.”
Construct validity ensures the interpretability of results, thereby paving the way for effective and efficient data-based decision making by school leaders.
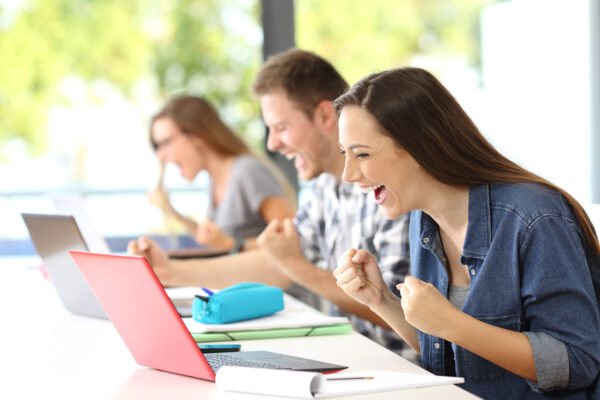
WHAT IS CRITERION VALIDITY?
Criterion validity refers to the correlation between a test and a criterion that is already accepted as a valid measure of the goal or question . If a test is highly correlated with another valid criterion, it is more likely that the test is also valid.
Criterion validity tends to be measured through statistical computations of correlation coefficients, although it’s possible that existing research has already determined the validity of a particular test that schools want to collect data on.
WHAT IS CONTENT VALIDITY?
Content validity refers to the actual content within a test. A test that is valid in content should adequately examine all aspects that define the objective.
Content validity is not a statistical measurement, but rather a qualitative one. For example, a standardized assessment in 9th-grade biology is content-valid if it covers all topics taught in a standard 9th-grade biology course.
Warren Schillingburg, an education specialist and associate superintendent, advises that determination of content-validity “should include several teachers (and content experts when possible) in evaluating how well the test represents the content taught.”
While this advice is certainly helpful for academic tests, content validity is of particular importance when the goal is more abstract, as the components of that goal are more subjective.
School inclusiveness, for example, may not only be defined by the equality of treatment across student groups, but by other factors, such as equal opportunities to participate in extracurricular activities.
Despite its complexity, the qualitative nature of content validity makes it a particularly accessible measure for all school leaders to take into consideration when creating data instruments.
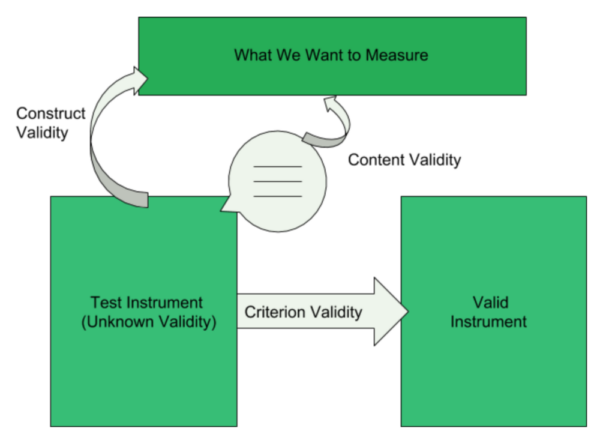
A CASE STUDY ON VALIDITY
To understand the different types of validity and how they interact, consider the example of Baltimore Public Schools trying to measure school climate.
School climate is a broad term, and its intangible nature can make it difficult to determine the validity of tests that attempt to quantify it. Baltimore Public Schools found research from The National Center for School Climate (NCSC) which set out five criterion that contribute to the overall health of a school’s climate. These criteria are safety, teaching and learning, interpersonal relationships, environment, and leadership, which the paper also defines on a practical level.
Because the NCSC’s criterion were generally accepted as valid measures of school climate, Baltimore City Schools sought to find tools that “are aligned with the domains and indicators proposed by the National School Climate Center.” This is essentially asking whether the tools Baltimore City Schools used were criterion-valid measures of school climate.
Baltimore City Schools introduced four data instruments, predominantly surveys, to find valid measures of school climate based on these criterion. They found that “each source addresses different school climate domains with varying emphasis,” implying that the usage of one tool may not yield content-valid results, but that the usage of all four “can be construed as complementary parts of the same larger picture.” Thus, sometimes validity can be achieved by using multiple tools from multiple viewpoints.
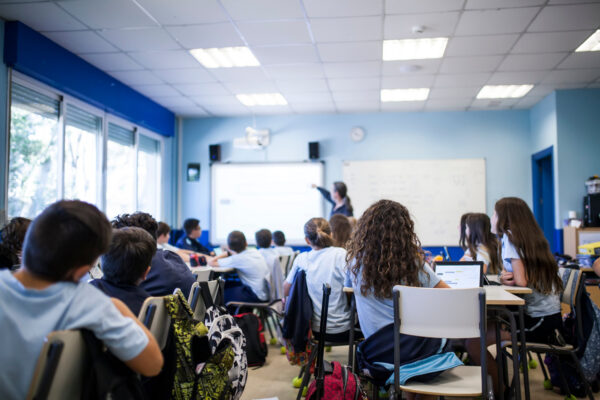
A Deeper Look at Reliability
TYPES OF RELIABILITY
The reliability of an assessment refers to the consistency of results. The most basic interpretation generally references something called test-retest reliability , which is characterized by the replicability of results. That is to say, if a group of students takes a test twice, both the results for individual students, as well as the relationship among students’ results, should be similar across tests.
However, there are two other types of reliability: alternate-form and internal consistency. Alternate form is a measurement of how test scores compare across two similar assessments given in a short time frame . Alternate form similarly refers to the consistency of both individual scores and positional relationships. Internal consistency is analogous to content validity and is defined as a measure of how the actual content of an assessment works together to evaluate understanding of a concept .
LIMITATIONS OF RELIABILITY
The three types of reliability work together to produce, according to Schillingburg , “confidence… that the test score earned is a good representation of a child’s actual knowledge of the content.” Reliability is important in the design of assessments because no assessment is truly perfect. A test produces an estimate of a student’s “true” score, or the score the student would receive if given a perfect test; however, due to imperfect design, tests can rarely, if ever, wholly capture that score. Thus, tests should aim to be reliable, or to get as close to that true score as possible.
Imperfect testing is not the only issue with reliability. Reliability is sensitive to the stability of extraneous influences, such as a student’s mood. Extraneous influences could be particularly dangerous in the collection of perceptions data, or data that measures students, teachers, and other members of the community’s perception of the school, which is often used in measurements of school culture and climate.
Uncontrollable changes in external factors could influence how a respondent perceives their environment, making an otherwise reliable instrument seem unreliable. For example, if a student or class is reprimanded the day that they are given a survey to evaluate their teacher, the evaluation of the teacher may be uncharacteristically negative. The same survey given a few days later may not yield the same results. However, most extraneous influences relevant to students tend to occur on an individual level, and therefore are not a major concern in the reliability of data for larger samples.
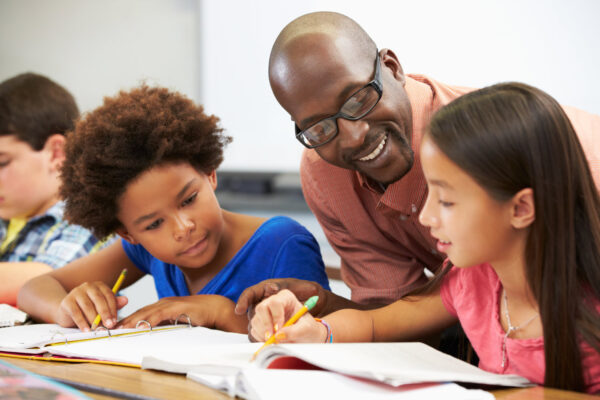
HOW TO IMPROVE RELIABILITY
On the other hand, extraneous influences relevant to other agents in the classroom could affect the scores of an entire class.
If the grader of an assessment is sensitive to external factors, their given grades may reflect this sensitivity, therefore making the results unreliable. Assessments that go beyond cut-and-dry responses engender a responsibility for the grader to review the consistency of their results.
Some of this variability can be resolved through the use of clear and specific rubrics for grading an assessment . Rubrics limit the ability of any grader to apply normative criteria to their grading, thereby controlling for the influence of grader biases . However, rubrics, like tests, are imperfect tools and care must be taken to ensure reliable results.
How does one ensure reliability? Measuring the reliability of assessments is often done with statistical computations.
The three measurements of reliability discussed above all have associated coefficients that standard statistical packages will calculate. However, schools that don’t have access to such tools shouldn’t simply throw caution to the wind and abandon these concepts when thinking about data.
Schillingburg advises that at the classroom level, educators can maintain reliability by:
- Creating clear instructions for each assignment
- Writing questions that capture the material taught
- Seeking feedback regarding the clarity and thoroughness of the assessment from students and colleagues.
With such care, the average test given in a classroom will be reliable. Moreover, if any errors in reliability arise, Schillingburg assures that class-level decisions made based on unreliable data are generally reversible, e.g. assessments found to be unreliable may be rewritten based on feedback provided.
However, reliability, or the lack thereof, can create problems for larger-scale projects, as the results of these assessments generally form the basis for decisions that could be costly for a school or district to either implement or reverse.
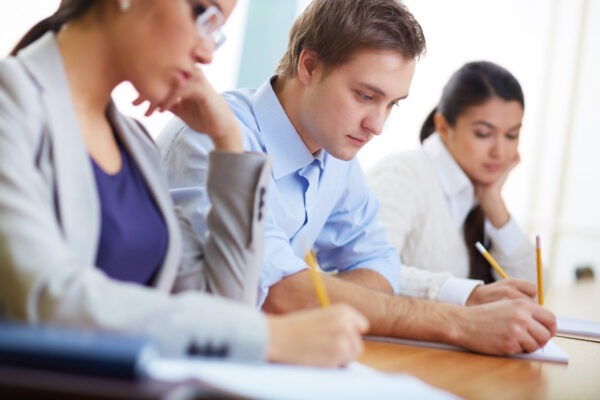
Validity and reliability are meaningful measurements that should be taken into account when attempting to evaluate the status of or progress toward any objective a district, school, or classroom has.
If precise statistical measurements of these properties are not able to be made, educators should attempt to evaluate the validity and reliability of data through intuition, previous research, and collaboration as much as possible.
An understanding of validity and reliability allows educators to make decisions that improve the lives of their students both academically and socially, as these concepts teach educators how to quantify the abstract goals their school or district has set.
To learn more about how Marco Learning can help your school meet its goals, check out our information page here .
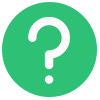
Please read Marco Learning’s Terms and Conditions, click to agree, and submit to continue to your content.
Please read Marco Learning’s Terms and Conditions, click to agree, and submit at the bottom of the window.
MARCO LEARNING TERMS OF USE
Last Modified: 1/24/2023
Acceptance of the Terms of Use
These terms of use are entered into by and between You and Marco Learning LLC (“ Company “, “ we “, or “ us “). The following terms and conditions (these “ Terms of Use “), govern your access to and use of Marco Learning , including any content, functionality, and services offered on or through Marco Learning (the “ Website “), whether as a guest or a registered user.
Please read the Terms of Use carefully before you start to use the Website. By using the Website or by clicking to accept or agree to the Terms of Use when this option is made available to you, you accept and agree to be bound and abide by these Terms of Use. You may not order or obtain products or services from this website if you (i) do not agree to these Terms of Use, or (ii) are prohibited from accessing or using this Website or any of this Website’s contents, goods or services by applicable law . If you do not want to agree to these Terms of Use, you must not access or use the Website.
This Website is offered and available to users who are 13 years of age or older, and reside in the United States or any of its territories or possessions. Any user under the age of 18 must (a) review the Terms of Use with a parent or legal guardian to ensure the parent or legal guardian acknowledges and agrees to these Terms of Use, and (b) not access the Website if his or her parent or legal guardian does not agree to these Terms of Use. By using this Website, you represent and warrant that you meet all of the foregoing eligibility requirements. If you do not meet all of these requirements, you must not access or use the Website.
Changes to the Terms of Use
We may revise and update these Terms of Use from time to time in our sole discretion. All changes are effective immediately when we post them, and apply to all access to and use of the Website thereafter.
These Terms of Use are an integral part of the Website Terms of Use that apply generally to the use of our Website. Your continued use of the Website following the posting of revised Terms of Use means that you accept and agree to the changes. You are expected to check this page each time you access this Website so you are aware of any changes, as they are binding on you.
Accessing the Website and Account Security
We reserve the right to withdraw or amend this Website, and any service or material we provide on the Website, in our sole discretion without notice. We will not be liable if for any reason all or any part of the Website is unavailable at any time or for any period. From time to time, we may restrict access to some parts of the Website, or the entire Website, to users, including registered users.
You are responsible for (i) making all arrangements necessary for you to have access to the Website, and (ii) ensuring that all persons who access the Website through your internet connection are aware of these Terms of Use and comply with them.
To access the Website or some of the resources it offers, you may be asked to provide certain registration details or other information. It is a condition of your use of the Website that all the information you provide on the Website is correct, current, and complete. You agree that all information you provide to register with this Website or otherwise, including but not limited to through the use of any interactive features on the Website, is governed by our Marco Learning Privacy Policy , and you consent to all actions we take with respect to your information consistent with our Privacy Policy.
If you choose, or are provided with, a user name, password, or any other piece of information as part of our security procedures, you must treat such information as confidential, and you must not disclose it to any other person or entity. You also acknowledge that your account is personal to you and agree not to provide any other person with access to this Website or portions of it using your user name, password, or other security information. You agree to notify us immediately of any unauthorized access to or use of your user name or password or any other breach of security. You also agree to ensure that you exit from your account at the end of each session. You should use particular caution when accessing your account from a public or shared computer so that others are not able to view or record your password or other personal information.
We have the right to disable any user name, password, or other identifier, whether chosen by you or provided by us, at any time in our sole discretion for any or no reason, including if, in our opinion, you have violated any provision of these Terms of Use.
Intellectual Property Rights
The Website and its entire contents, features, and functionality (including but not limited to all information, software, text, displays, images, graphics, video, other visuals, and audio, and the design, selection, and arrangement thereof) are owned by the Company, its licensors, or other providers of such material and are protected by United States and international copyright, trademark, patent, trade secret, and other intellectual property or proprietary rights laws. Your use of the Website does not grant to you ownership of any content, software, code, date or materials you may access on the Website.
These Terms of Use permit you to use the Website for your personal, non-commercial use only. You must not reproduce, distribute, modify, create derivative works of, publicly display, publicly perform, republish, download, store, or transmit any of the material on our Website, except as follows:
- Your computer may temporarily store copies of such materials in RAM incidental to your accessing and viewing those materials.
- You may store files that are automatically cached by your Web browser for display enhancement purposes.
- You may print or download one copy of a reasonable number of pages of the Website for your own personal, non-commercial use and not for further reproduction, publication, or distribution.
- If we provide desktop, mobile, or other applications for download, you may download a single copy to your computer or mobile device solely for your own personal, non-commercial use, provided you agree to be bound by our end user license agreement for such applications.
- If we provide social media features with certain content, you may take such actions as are enabled by such features.
You must not:
- Modify copies of any materials from this site.
- Use any illustrations, photographs, video or audio sequences, or any graphics separately from the accompanying text.
- Delete or alter any copyright, trademark, or other proprietary rights notices from copies of materials from this site.
You must not access or use for any commercial purposes any part of the Website or any services or materials available through the Website.
If you wish to make any use of material on the Website other than that set out in this section, please contact us
If you print, copy, modify, download, or otherwise use or provide any other person with access to any part of the Website in breach of the Terms of Use, your right to use the Website will stop immediately and you must, at our option, return or destroy any copies of the materials you have made. No right, title, or interest in or to the Website or any content on the Website is transferred to you, and all rights not expressly granted are reserved by the Company. Any use of the Website not expressly permitted by these Terms of Use is a breach of these Terms of Use and may violate copyright, trademark, and other laws.
Trademarks, logos, service marks, trade names, and all related names, logos, product and service names, designs, and slogans are trademarks of the Company or its affiliates or licensors (collectively, the “ Trademarks ”). You must not use such Trademarks without the prior written permission of the Company. All other names, logos, product and service names, designs, and slogans on this Website are the trademarks of their respective owners.
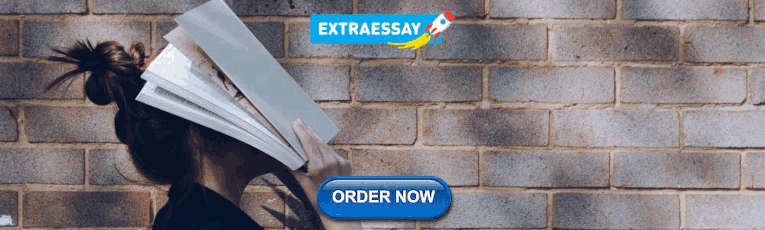
Prohibited Uses
You may use the Website only for lawful purposes and in accordance with these Terms of Use. You agree not to use the Website:
- In any way that violates any applicable federal, state, local, or international law or regulation (including, without limitation, any laws regarding the export of data or software to and from the US or other countries).
- For the purpose of exploiting, harming, or attempting to exploit or harm minors in any way by exposing them to inappropriate content, asking for personally identifiable information, or otherwise.
- To send, knowingly receive, upload, download, use, or re-use any material that does not comply with the Content Standards set out in these Terms of Use.
- To transmit, or procure the sending of, any advertising or promotional material, including any “junk mail”, “chain letter”, “spam”, or any other similar solicitation.
- To impersonate or attempt to impersonate the Company, a Company employee, another user, or any other person or entity (including, without limitation, by using email addresses or screen names associated with any of the foregoing).
- To engage in any other conduct that restricts or inhibits anyone’s use or enjoyment of the Website, or which, as determined by us, may harm the Company or users of the Website or expose them to liability.
Additionally, you agree not to:
- Use the Website in any manner that could disable, overburden, damage, or impair the site or interfere with any other party’s use of the Website, including their ability to engage in real time activities through the Website.
- Use any robot, spider, or other automatic device, process, or means to access the Website for any purpose, including monitoring or copying any of the material on the Website.
- Use any manual process to monitor or copy any of the material on the Website or for any other unauthorized purpose without our prior written consent.
- Use any device, software, or routine that interferes with the proper working of the Website.
- Introduce any viruses, Trojan horses, worms, logic bombs, or other material that is malicious or technologically harmful.
- Attempt to gain unauthorized access to, interfere with, damage, or disrupt any parts of the Website, the server on which the Website is stored, or any server, computer, or database connected to the Website.
- Attack the Website via a denial-of-service attack or a distributed denial-of-service attack.
- Otherwise attempt to interfere with the proper working of the Website.
If you use, or assist another person in using the Website in any unauthorized way, you agree that you will pay us an additional $50 per hour for any time we spend to investigate and correct such use, plus any third party costs of investigation we incur (with a minimum $300 charge). You agree that we may charge any credit card number provided for your account for such amounts. You further agree that you will not dispute such a charge and that we retain the right to collect any additional actual costs.
User Contributions
The Website may contain message boards, chat rooms, personal web pages or profiles, forums, bulletin boards, and other interactive features (collectively, “ Interactive Services “) that allow users to post, submit, publish, display, or transmit to other users or other persons (hereinafter, “ post “) content or materials (collectively, “ User Contributions “) on or through the Website.
All User Contributions must comply with the Content Standards set out in these Terms of Use.
Any User Contribution you post to the site will be considered non-confidential and non-proprietary. By providing any User Contribution on the Website, you grant us and our affiliates and service providers, and each of their and our respective licensees, successors, and assigns the right to use, reproduce, modify, perform, display, distribute, and otherwise disclose to third parties any such material for any purpose.
You represent and warrant that:
- You own or control all rights in and to the User Contributions and have the right to grant the license granted above to us and our affiliates and service providers, and each of their and our respective licensees, successors, and assigns.
- All of your User Contributions do and will comply with these Terms of Use.
You understand and acknowledge that you are responsible for any User Contributions you submit or contribute, and you, not the Company, have full responsibility for such content, including its legality, reliability, accuracy, and appropriateness.
For any academic source materials such as textbooks and workbooks which you submit to us in connection with our online tutoring services, you represent and warrant that you are entitled to upload such materials under the “fair use” doctrine of copyright law. In addition, if you request that our system display a representation of a page or problem from a textbook or workbook, you represent and warrant that you are in proper legal possession of such textbook or workbook and that your instruction to our system to display a page or problem from your textbook or workbook is made for the sole purpose of facilitating your tutoring session, as “fair use” under copyright law.
You agree that we may record all or any part of any live online classes and tutoring sessions (including voice chat communications) for quality control and other purposes. You agree that we own all transcripts and recordings of such sessions and that these Terms of Use will be deemed an irrevocable assignment of rights in all such transcripts and recordings to us.
We are not responsible or liable to any third party for the content or accuracy of any User Contributions posted by you or any other user of the Website.
Monitoring and Enforcement: Termination
We have the right to:
- Remove or refuse to post any User Contributions for any or no reason in our sole discretion.
- Take any action with respect to any User Contribution that we deem necessary or appropriate in our sole discretion, including if we believe that such User Contribution violates the Terms of Use, including the Content Standards, infringes any intellectual property right or other right of any person or entity, threatens the personal safety of users of the Website or the public, or could create liability for the Company.
- Disclose your identity or other information about you to any third party who claims that material posted by you violates their rights, including their intellectual property rights or their right to privacy.
- Take appropriate legal action, including without limitation, referral to law enforcement, for any illegal or unauthorized use of the Website.
- Terminate or suspend your access to all or part of the Website for any or no reason, including without limitation, any violation of these Terms of Use.
Without limiting the foregoing, we have the right to cooperate fully with any law enforcement authorities or court order requesting or directing us to disclose the identity or other information of anyone posting any materials on or through the Website. YOU WAIVE AND HOLD HARMLESS THE COMPANY AND ITS AFFILIATES, LICENSEES, AND SERVICE PROVIDERS FROM ANY CLAIMS RESULTING FROM ANY ACTION TAKEN BY ANY OF THE FOREGOING PARTIES DURING, OR TAKEN AS A CONSEQUENCE OF, INVESTIGATIONS BY EITHER SUCH PARTIES OR LAW ENFORCEMENT AUTHORITIES.
However, we do not undertake to review material before it is posted on the Website, and cannot ensure prompt removal of objectionable material after it has been posted. Accordingly, we assume no liability for any action or inaction regarding transmissions, communications, or content provided by any user or third party. We have no liability or responsibility to anyone for performance or nonperformance of the activities described in this section.
Content Standards
These content standards apply to any and all User Contributions and use of Interactive Services. User Contributions must in their entirety comply with all applicable federal, state, local, and international laws and regulations. Without limiting the foregoing, User Contributions must not:
- Contain any material that is defamatory, obscene, indecent, abusive, offensive, harassing, violent, hateful, inflammatory, or otherwise objectionable.
- Promote sexually explicit or pornographic material, violence, or discrimination based on race, sex, religion, nationality, disability, sexual orientation, or age.
- Infringe any patent, trademark, trade secret, copyright, or other intellectual property or other rights of any other person.
- Violate the legal rights (including the rights of publicity and privacy) of others or contain any material that could give rise to any civil or criminal liability under applicable laws or regulations or that otherwise may be in conflict with these Terms of Use and our Privacy Policy .
- Be likely to deceive any person.
- Promote any illegal activity, or advocate, promote, or assist any unlawful act.
- Cause annoyance, inconvenience, or needless anxiety or be likely to upset, embarrass, alarm, or annoy any other person.
- Impersonate any person, or misrepresent your identity or affiliation with any person or organization.
- Involve commercial activities or sales, such as contests, sweepstakes, and other sales promotions, barter, or advertising.
- Give the impression that they emanate from or are endorsed by us or any other person or entity, if this is not the case.
(collectively, the “ Content Standards ”)
Copyright Infringement
If you believe that any User Contributions violate your copyright, please contact us and provide the following information:
- An electronic or physical signature of the person authorized to act on behalf of the owner of the copyright interest;
- A description of the copyrighted work that you claim has been infringed;
- A description of where the material you claim is infringing is located on the website (and such description must reasonably sufficient to enable us to find the alleged infringing material);
- Your address, telephone number and email address;
- A written statement by you that you have a good faith belief that the disputed use is not authorized by the copyright owner, its agent, or the law; and
- A statement by you, made under the penalty of perjury, that the above information in your notice is accurate and that you are the copyright owner or authorized to act on the copyright owner’s behalf.
We may terminate the accounts of any infringers.
Reliance on Information Posted
From time to time, we may make third party opinions, advice, statements, offers, or other third party information or content available on the Website or from tutors under tutoring services (collectively, “Third Party Content”). All Third Party Content is the responsibility of the respective authors thereof and should not necessarily be relied upon. Such third party authors are solely responsible for such content. WE DO NOT (I) GUARANTEE THE ACCURACY, COMPLETENESS OR USEFULNESS OF ANY THIRD PARTY CONTENT ON THE SITE OR ANY VERIFICATION SERVICES DONE ON OUR TUTORS OR INSTRUCTORS, OR (II) ADOPT, ENDORSE OR ACCEPT RESPONSIBILITY FOR THE ACCURACY OR RELIABILITY OF ANY OPINION, ADVICE, OR STATEMENT MADE BY ANY TUTOR OR INSTRUCTOR OR ANY PARTY THAT APPEARS ON THE WEBSITE. UNDER NO CIRCUMSTANCES WILL WE BE RESPONSBILE OR LIABLE FOR ANY LOSS OR DAMAGE RESULTING FROM YOUR RELIANCE ON INFORMATION OR OTHER CONENT POSTED ON OR AVAILBLE FROM THE WEBSITE.
Changes to the Website
We may update the content on this Website from time to time, but its content is not necessarily complete or up-to-date. Any of the material on the Website may be out of date at any given time, and we are under no obligation to update such material.
Information About You and Your Visits to the Website
All information we collect on this Website is subject to our Privacy Policy . By using the Website, you consent to all actions taken by us with respect to your information in compliance with the Privacy Policy.
Online Purchases and Other Terms and Conditions
All purchases through our site or other transactions for the sale of services and information formed through the Website or resulting from visits made by you are governed by our Terms of Sale, which are hereby incorporated into these Terms of Use.
Additional terms and conditions may also apply to specific portions, services, or features of the Website. All such additional terms and conditions are hereby incorporated by this reference into these Terms of Use.
Linking to the Website and Social Media Features
You may link to our homepage, provided you do so in a way that is fair and legal and does not damage our reputation or take advantage of it, but you must not establish a link in such a way as to suggest any form of association, approval, or endorsement on our part without our express written consent.
This Website may provide certain social media features that enable you to:
- Link from your own or certain third-party websites to certain content on this Website.
- Send emails or other communications with certain content, or links to certain content, on this Website.
- Cause limited portions of content on this Website to be displayed or appear to be displayed on your own or certain third-party websites.
You may use these features solely as they are provided by us, and solely with respect to the content they are displayed with and otherwise in accordance with any additional terms and conditions we provide with respect to such features. Subject to the foregoing, you must not:
- Establish a link from any website that is not owned by you.
- Cause the Website or portions of it to be displayed on, or appear to be displayed by, any other site, for example, framing, deep linking, or in-line linking.
- Link to any part of the Website other than the homepage.
- Otherwise take any action with respect to the materials on this Website that is inconsistent with any other provision of these Terms of Use.
The website from which you are linking, or on which you make certain content accessible, must comply in all respects with the Content Standards set out in these Terms of Use.
You agree to cooperate with us in causing any unauthorized framing or linking immediately to stop. We reserve the right to withdraw linking permission without notice.
We may disable all or any social media features and any links at any time without notice in our discretion.
Links from the Website
If the Website contains links to other sites and resources provided by third parties (“ Linked Sites ”), these links are provided for your convenience only. This includes links contained in advertisements, including banner advertisements and sponsored links. You acknowledge and agree that we have no control over the contents, products, services, advertising or other materials which may be provided by or through those Linked sites or resources, and accept no responsibility for them or for any loss or damage that may arise from your use of them. If you decide to access any of the third-party websites linked to this Website, you do so entirely at your own risk and subject to the terms and conditions of use for such websites.
You agree that if you include a link from any other website to the Website, such link will open in a new browser window and will link to the full version of an HTML formatted page of this Website. You are not permitted to link directly to any image hosted on the Website or our products or services, such as using an “in-line” linking method to cause the image hosted by us to be displayed on another website. You agree not to download or use images hosted on this Website or another website, for any purpose, including, without limitation, posting such images on another website. You agree not to link from any other website to this Website in any manner such that the Website, or any page of the Website, is “framed,” surrounded or obfuscated by any third party content, materials or branding. We reserve all of our rights under the law to insist that any link to the Website be discontinued, and to revoke your right to link to the Website from any other website at any time upon written notice to you.
Geographic Restrictions
The owner of the Website is based in the state of New Jersey in the United States. We provide this Website for use only by persons located in the United States. We make no claims that the Website or any of its content is accessible or appropriate outside of the United States. Access to the Website may not be legal by certain persons or in certain countries. If you access the Website from outside the United States, you do so on your own initiative and are responsible for compliance with local laws.
Disclaimer of Warranties
You understand that we cannot and do not guarantee or warrant that files available for downloading from the internet or the Website will be free of viruses or other destructive code. You are responsible for implementing sufficient procedures and checkpoints to satisfy your particular requirements for anti-virus protection and accuracy of data input and output, and for maintaining a means external to our site for any reconstruction of any lost data. TO THE FULLEST EXTENT PROVIDED BY LAW, WE WILL NOT BE LIABLE FOR ANY LOSS OR DAMAGE CAUSED BY A DISTRIBUTED DENIAL-OF-SERVICE ATTACK, VIRUSES, OR OTHER TECHNOLOGICALLY HARMFUL MATERIAL THAT MAY INFECT YOUR COMPUTER EQUIPMENT, COMPUTER PROGRAMS, DATA, OR OTHER PROPRIETARY MATERIAL DUE TO YOUR USE OF THE WEBSITE OR ANY SERVICES OR ITEMS OBTAINED THROUGH THE WEBSITE OR TO YOUR DOWNLOADING OF ANY MATERIAL POSTED ON IT, OR ON ANY WEBSITE LINKED TO IT.
YOUR USE OF THE WEBSITE, ITS CONTENT, AND ANY SERVICES OR ITEMS OBTAINED THROUGH THE WEBSITE IS AT YOUR OWN RISK. THE WEBSITE, ITS CONTENT, AND ANY SERVICES OR ITEMS OBTAINED THROUGH THE WEBSITE ARE PROVIDED ON AN “AS IS” AND “AS AVAILABLE” BASIS, WITHOUT ANY WARRANTIES OF ANY KIND, EITHER EXPRESS OR IMPLIED. NEITHER THE COMPANY NOR ANY PERSON ASSOCIATED WITH THE COMPANY MAKES ANY WARRANTY OR REPRESENTATION WITH RESPECT TO THE COMPLETENESS, SECURITY, RELIABILITY, QUALITY, ACCURACY, OR AVAILABILITY OF THE WEBSITE. WITHOUT LIMITING THE FOREGOING, NEITHER THE COMPANY NOR ANYONE ASSOCIATED WITH THE COMPANY REPRESENTS OR WARRANTS THAT THE WEBSITE, ITS CONTENT, OR ANY SERVICES OR ITEMS OBTAINED THROUGH THE WEBSITE WILL BE ACCURATE, RELIABLE, ERROR-FREE, OR UNINTERRUPTED, THAT DEFECTS WILL BE CORRECTED, THAT OUR SITE OR THE SERVER THAT MAKES IT AVAILABLE ARE FREE OF VIRUSES OR OTHER HARMFUL COMPONENTS, OR THAT THE WEBSITE OR ANY SERVICES OR ITEMS OBTAINED THROUGH THE WEBSITE WILL OTHERWISE MEET YOUR NEEDS OR EXPECTATIONS.
TO THE FULLEST EXTENT PROVIDED BY LAW, THE COMPANY HEREBY DISCLAIMS ALL WARRANTIES OF ANY KIND, WHETHER EXPRESS OR IMPLIED, STATUTORY, OR OTHERWISE, INCLUDING BUT NOT LIMITED TO ANY WARRANTIES OF MERCHANTABILITY, NON-INFRINGEMENT, AND FITNESS FOR PARTICULAR PURPOSE.
THE FOREGOING DOES NOT AFFECT ANY WARRANTIES THAT CANNOT BE EXCLUDED OR LIMITED UNDER APPLICABLE LAW.
Limitation on Liability
TO THE FULLEST EXTENT PROVIDED BY LAW, IN NO EVENT WILL THE COMPANY, ITS AFFILIATES, OR THEIR LICENSORS, SERVICE PROVIDERS, EMPLOYEES, AGENTS, OFFICERS, OR DIRECTORS BE LIABLE FOR DAMAGES OF ANY KIND, UNDER ANY LEGAL THEORY, ARISING OUT OF OR IN CONNECTION WITH YOUR USE, OR INABILITY TO USE, THE WEBSITE, ANY WEBSITES LINKED TO IT, ANY CONTENT ON THE WEBSITE OR SUCH OTHER WEBSITES, INCLUDING ANY DIRECT, INDIRECT, SPECIAL, INCIDENTAL, CONSEQUENTIAL, OR PUNITIVE DAMAGES, INCLUDING BUT NOT LIMITED TO, PERSONAL INJURY, PAIN AND SUFFERING, EMOTIONAL DISTRESS, LOSS OF REVENUE, LOSS OF PROFITS, LOSS OF BUSINESS OR ANTICIPATED SAVINGS, LOSS OF USE, LOSS OF GOODWILL, LOSS OF DATA, AND WHETHER CAUSED BY TORT (INCLUDING NEGLIGENCE), BREACH OF CONTRACT, OR OTHERWISE, EVEN IF FORESEEABLE.
THE FOREGOING DOES NOT AFFECT ANY LIABILITY THAT CANNOT BE EXCLUDED OR LIMITED UNDER APPLICABLE LAW.
Indemnification
You agree to defend, indemnify, and hold harmless the Company, its affiliates, licensors, and service providers, and its and their respective officers, directors, employees, contractors, agents, licensors, suppliers, successors, and assigns from and against any claims, liabilities, damages, judgments, awards, losses, costs, expenses, or fees (including reasonable attorneys’ fees) arising out of or relating to your violation of these Terms of Use or your use of the Website, including, but not limited to, your User Contributions, any use of the Website’s content, services, and products other than as expressly authorized in these Terms of Use or your use of any information obtained from the Website.
Governing Law and Jurisdiction
All matters relating to the Website and these Terms of Use and any dispute or claim arising therefrom or related thereto (in each case, including non-contractual disputes or claims), shall be governed by and construed in accordance with the internal laws of the State of New Jersey without giving effect to any choice or conflict of law provision or rule (whether of the State of New Jersey or any other jurisdiction).
Any legal suit, action, or proceeding arising out of, or related to, these Terms of Use or the Website shall be instituted exclusively in the federal courts of the United States or the courts of the State of New Jersey in each case located in the County of Monmouth although we retain the right to bring any suit, action, or proceeding against you for breach of these Terms of Use in your country of residence or any other relevant country. You waive any and all objections to the exercise of jurisdiction over you by such courts and to venue in such courts. You may not under any circumstances commence or maintain against us any class action, class arbitration, or other representative action or proceeding.
Arbitration
By using this Website, you agree, at Company’s sole discretion, that it may require you to submit any disputes arising from the use of these Terms of Use or the Website, including disputes arising from or concerning their interpretation, violation, invalidity, non-performance, or termination, to final and binding arbitration under the Rules of Arbitration of the American Arbitration Association applying New Jersey law. In doing so, YOU GIVE UP YOUR RIGHT TO GO TO COURT to assert or defend any claims between you and us. YOU ALSO GIVE UP YOUR RIGHT TO PARTICIPATE IN A CLASS ACTION OR OTHER CLASS PROCEEDING. Your rights may be determined by a NEUTRAL ARBITRATOR, NOT A JUDGE OR JURY. You are entitled to a fair hearing before the arbitrator. The arbitrator can grant any relief that a court can, but you should note that arbitration proceedings are usually simpler and more streamlined than trials and other judicial proceedings. Decisions by the arbitrator are enforceable in court and may be overturned by a court only for very limited reasons.
Any proceeding to enforce this arbitration provision, including any proceeding to confirm, modify, or vacate an arbitration award, may be commenced in any court of competent jurisdiction. In the event that this arbitration provision is for any reason held to be unenforceable, any litigation against Company must be commenced only in the federal or state courts located in Monmouth County, New Jersey. You hereby irrevocably consent to the jurisdiction of those courts for such purposes.
Limitation on Time to File Claims
ANY CAUSE OF ACTION OR CLAIM YOU MAY HAVE ARISING OUT OF OR RELATING TO THESE TERMS OF USE OR THE WEBSITE MUST BE COMMENCED WITHIN ONE (1) YEAR AFTER THE CAUSE OF ACTION ACCRUES, OTHERWISE, SUCH CAUSE OF ACTION OR CLAIM IS PERMANENTLY BARRED.
Waiver and Severability
No waiver by the Company of any term or condition set out in these Terms of Use shall be deemed a further or continuing waiver of such term or condition or a waiver of any other term or condition, and any failure of the Company to assert a right or provision under these Terms of Use shall not constitute a waiver of such right or provision.
If any provision of these Terms of Use is held by a court or other tribunal of competent jurisdiction to be invalid, illegal, or unenforceable for any reason, such provision shall be eliminated or limited to the minimum extent such that the remaining provisions of the Terms of Use will continue in full force and effect.
Entire Agreement
The Terms of Use, our Privacy Policy, and Terms of Sale constitute the sole and entire agreement between you and Marco Learning LLC regarding the Website and supersede all prior and contemporaneous understandings, agreements, representations, and warranties, both written and oral, regarding the Website.
Communications and Miscellaneous
If you provide us your email address, you agree and consent to receive email messages from us. These emails may be transaction or relationship communications relating to the products or services we offer, such as administrative notices and service announcements or changes, or emails containing commercial offers, promotions or special offers from us.
Your Comments and Concerns
This website is operated by Marco Learning LLC, a New Jersey limited liability company with an address of 113 Monmouth Road, Suite 1, Wrightstown, New Jersey 08562.
Please contact us for all other feedback, comments, requests for technical support, and other communications relating to the Website.
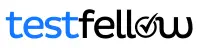
What is a Test? 10 Types of Tests in Education
In the world of education, tests are something we all encounter at some point, whether in school, college, or university. They’re a fundamental part of learning, but have you ever wondered what tests really are and how they affect our education? In this article, we’ll break down the concept of tests and explore the different types used in education. Beyond mere assessments , tests serve as vital instruments for comprehension and advancement along the educational pathway. So, let’s dive into this essential aspect of education and discover its significance in various learning contexts.
What is a Test?
A test serves as a formal method for assessing your knowledge and understanding of a particular subject or topic. It typically consists of questions or tasks designed to gauge your grasp of the material. Tests take various forms, such as written questions with multiple-choice options or practical demonstrations of your skills. Their primary purpose is to evaluate how well you know the subject, your abilities, or your performance in a structured manner.
Tests go beyond merely assigning a grade; they offer valuable insights to teachers and educators about their student’s learning progress and areas where additional support might be necessary. Tests play a crucial role in the learning process by pinpointing strengths and areas requiring further practice. Thus, tests act as educational milestones, helping both learners and educators track progress and enhance learning outcomes.
Types of Tests in Education
In the diverse world of education, tests come in various shapes and sizes, each serving a distinct purpose in assessing learning and knowledge. In this section, we will explore different types of tests commonly used in education.
1. Standardized Test
Standardized tests are designed to evaluate a student’s knowledge, skills, or abilities using a uniform set of questions and scoring methods. These tests are administered consistently to a broad group of students, often on a regional, national, or international level. Standardized tests aim to provide a fair and equitable way to compare students’ performance and assess educational systems. An example of a standardized test is the SAT (Scholastic Assessment Test), which is widely used for college admissions in the United States.
2. Diagnostic Test
Diagnostic tests are tailored assessments that aim to identify a student’s strengths and weaknesses in a particular subject or skill. They serve as diagnostic tools for educators to understand where a student might be struggling and where additional support is needed. For instance, in mathematics, a diagnostic test might reveal that a student excels in algebra but struggles with geometry, allowing educators to provide targeted assistance to improve geometry skills.
3. Proficiency Test
Proficiency tests evaluate a student’s level of expertise or mastery in a specific subject or skill area. These tests assess how well a student has acquired the knowledge or competencies required for a particular academic level or professional field. For example, language proficiency tests like the TOEFL (Test of English as a Foreign Language) gauge an individual’s ability to communicate effectively in English, crucial for academic and professional purposes.
4. Placement Test
Placement tests are used to determine a student’s appropriate level or placement within an educational program or course. These tests help ensure that students are neither overwhelmed by material too advanced nor held back by material too basic for their abilities. In language education, a placement test may assess a student’s language skills to determine which level of language course they should enroll in, ensuring an optimal learning experience for each student.
5. Achievement Test
An achievement test measures your grasp of specific subject matter you’ve been taught. It’s like a snapshot of your understanding in a particular course or program. For instance, consider an end-of-term science exam. Its purpose is to gauge how well you’ve absorbed the scientific knowledge covered during that term. Achievement tests are vital tools for showcasing your academic progress.
6. Aptitude Test
Aptitude tests evaluate your potential to excel in particular areas. They serve as indicators of your inherent abilities and talents rather than assessing what you’ve already learned. Think of them as a compass guiding you toward fields where your strengths naturally shine. Career aptitude tests, for example, help uncover professions that align with your unique strengths and interests, aiding in informed career choices.
7. Objective Test
Objective tests have clear-cut right and wrong answers. They employ formats such as multiple-choice questions or true/false statements, leaving no room for subjective interpretation. Imagine a history quiz featuring multiple-choice questions. It’s considered an objective test because each question has a definitive correct answer. These tests are efficient tools for assessing factual knowledge and specific information.
8. Subjective Test
A subjective test operates in shades of interpretation rather than black and white. It’s less about facts and more about your perspective, analysis, and personal insights. In a subjective test, you’re asked to express your opinions, provide explanations, or even present arguments. Essay questions are a classic example of subjective testing. They require you to delve into your understanding of a topic, offering reasoned explanations and arguments, often leaving room for varied responses based on individual viewpoints.
9. Personality Test
Personality tests aim to uncover your unique traits, behaviors, and characteristics. These tests delve into your emotional responses, social tendencies, and preferences to create a comprehensive picture of your personality. They don’t have right or wrong answers; instead, they provide insights into your individuality. For instance, the Myers-Briggs Type Indicator (MBTI) is a well-known personality test that categorizes individuals into specific personality types based on their preferences in areas like extroversion/introversion or thinking/feeling.
10. Intelligence Test
Intelligence tests, as their name implies, delve into your cognitive capabilities and intellectual potential. Their purpose is to gauge your capacity for tasks like problem-solving, logical reasoning, and abstract thinking. Unlike assessments that measure your existing knowledge, these tests focus on your ability to learn and adapt to fresh challenges. For instance, the Stanford-Binet Intelligence Scales and the Wechsler Adult Intelligence Scale (WAIS) are renowned examples of such assessments that aid in evaluating an individual’s intellectual aptitude. Intelligence tests offer valuable insights into cognitive strengths and areas where one may excel.
Conclusion
In the journey through “What is a Test? 10 Types of Tests in Education,” we’ve explored the fundamental concept of tests and delved into the diverse range of test types used to evaluate knowledge, skills, and abilities. Tests are not mere assessments; they are versatile tools that serve various purposes in education. Understanding these test types empowers both students and educators to excel in their educational journey
FAQs about Tests
1. what is the purpose of a test.
Tests serve to evaluate and measure various aspects of knowledge, skills, or abilities. They are used in education and other fields to assess learning, make informed decisions, and provide feedback.
2. Are all tests standardized?
No, not all tests are standardized. Standardized tests have a consistent format and scoring system, making it easy to compare results across a group. However, there are also non-standardized tests, which may have more flexible formats and are often used for specific purposes within an educational context.
3. What are some common types of standardized tests?
Common standardized tests include the SAT, ACT, GRE, and TOEFL for academic purposes, as well as IQ tests for cognitive assessment. These tests follow a uniform structure and scoring system.
4. What is the difference between a test and an assessment?
A test and an assessment share the common goal of evaluating knowledge and performance, but they differ in scope and purpose. An assessment is a broader term encompassing various methods and tools used to measure understanding, skills, or abilities. It can include observations, discussions, projects, and tests.
On the other hand, a test is a specific type of assessment characterized by structured questions or tasks with predetermined answers. Tests often have a more defined format, like multiple-choice questions or essay prompts, and are typically used to measure a specific aspect of knowledge or skill in a standardized way.
5. What is the difference between a test and a quiz?
The distinction between a test and a quiz primarily lies in their context, scope, and purpose. A test is a comprehensive assessment that covers a broader range of topics or material. It often carries higher stakes and may be used for grading, certification, or evaluation of a significant portion of a course or curriculum.
In contrast, a quiz is usually a shorter, less comprehensive assessment that focuses on a specific section or topic within a course. Quizzes are often used for practice, review, or to assess understanding of recently covered material. While both tests and quizzes aim to evaluate knowledge, quizzes tend to be less extensive and carry lower weight in the overall assessment.
6. Why are there various types of tests available worldwide?
The reason we have many different types of tests around the world is because schools and colleges in different places have different needs and goals for their students. Also, what students learn can be different from one place to another. So, there isn’t one type of test that fits everyone. Different types of tests were created to match these specific needs. Some tests are used to compare students across a whole country, while others help figure out what each student needs to learn better. Additionally, the field of education continually evolves, and new assessment methods are developed to align with emerging teaching and learning
Related Articles:
- What Are Assessments? 14 Types with Examples
- A Comprehensive Guide to 23 Types of Questions
- What Is a Psychometric Test – A Comprehensive Guide
- What is an IQ Test – A Comprehensive Guide
- What are Personality Tests – A Comprehensive Guide
- What are Aptitude Tests – A Comprehensive Guide
- What are MCQs? 24 Types with Examples
- Top 10 Tips to Prepare for a Psychometric Test
- How to Pass an Aptitude Test: Top 10 Effective Tips
- How to Prepare for Personality Tests: 10 Expert Tips
- How to Answer Multiple Choice Questions: 10 Tips

Statistics Made Easy
How to Write Hypothesis Test Conclusions (With Examples)
A hypothesis test is used to test whether or not some hypothesis about a population parameter is true.
To perform a hypothesis test in the real world, researchers obtain a random sample from the population and perform a hypothesis test on the sample data, using a null and alternative hypothesis:
- Null Hypothesis (H 0 ): The sample data occurs purely from chance.
- Alternative Hypothesis (H A ): The sample data is influenced by some non-random cause.
If the p-value of the hypothesis test is less than some significance level (e.g. α = .05), then we reject the null hypothesis .
Otherwise, if the p-value is not less than some significance level then we fail to reject the null hypothesis .
When writing the conclusion of a hypothesis test, we typically include:
- Whether we reject or fail to reject the null hypothesis.
- The significance level.
- A short explanation in the context of the hypothesis test.
For example, we would write:
We reject the null hypothesis at the 5% significance level. There is sufficient evidence to support the claim that…
Or, we would write:
We fail to reject the null hypothesis at the 5% significance level. There is not sufficient evidence to support the claim that…
The following examples show how to write a hypothesis test conclusion in both scenarios.
Example 1: Reject the Null Hypothesis Conclusion
Suppose a biologist believes that a certain fertilizer will cause plants to grow more during a one-month period than they normally do, which is currently 20 inches. To test this, she applies the fertilizer to each of the plants in her laboratory for one month.
She then performs a hypothesis test at a 5% significance level using the following hypotheses:
- H 0 : μ = 20 inches (the fertilizer will have no effect on the mean plant growth)
- H A : μ > 20 inches (the fertilizer will cause mean plant growth to increase)
Suppose the p-value of the test turns out to be 0.002.
Here is how she would report the results of the hypothesis test:
We reject the null hypothesis at the 5% significance level. There is sufficient evidence to support the claim that this particular fertilizer causes plants to grow more during a one-month period than they normally do.
Example 2: Fail to Reject the Null Hypothesis Conclusion
Suppose the manager of a manufacturing plant wants to test whether or not some new method changes the number of defective widgets produced per month, which is currently 250. To test this, he measures the mean number of defective widgets produced before and after using the new method for one month.
He performs a hypothesis test at a 10% significance level using the following hypotheses:
- H 0 : μ after = μ before (the mean number of defective widgets is the same before and after using the new method)
- H A : μ after ≠ μ before (the mean number of defective widgets produced is different before and after using the new method)
Suppose the p-value of the test turns out to be 0.27.
Here is how he would report the results of the hypothesis test:
We fail to reject the null hypothesis at the 10% significance level. There is not sufficient evidence to support the claim that the new method leads to a change in the number of defective widgets produced per month.
Additional Resources
The following tutorials provide additional information about hypothesis testing:
Introduction to Hypothesis Testing 4 Examples of Hypothesis Testing in Real Life How to Write a Null Hypothesis
Featured Posts

Hey there. My name is Zach Bobbitt. I have a Masters of Science degree in Applied Statistics and I’ve worked on machine learning algorithms for professional businesses in both healthcare and retail. I’m passionate about statistics, machine learning, and data visualization and I created Statology to be a resource for both students and teachers alike. My goal with this site is to help you learn statistics through using simple terms, plenty of real-world examples, and helpful illustrations.
Leave a Reply Cancel reply
Your email address will not be published. Required fields are marked *
You are using an older unsupported browser. We cannot guarantee this site will work properly for you. Please upgrade to a more recent browser or install Google Chrome Frame to experience this site.
- Active Programs
Colorado Parks & Wildlife
Upcoming events, colorado hunter education internet conclusion course.
Internet Course + Conclusion Class - Students complete a portion of the course online at their convenience, and then attend a shorter class than required by the traditional course. This option is not available in all parts of the state, so register for an available class before starting the online course.
Show additional information
The Colorado INTERNET-BASED Hunter Education Course is necessary for students to qualify for a Colorado Hunter Education Certificate. The major portion of this course is taken online, allowing the student to complete the mandatory homework. Students must complete the online course before they attend this in-person class session.
Internet Course
The internet course is available at Hunter-ed.com . There is an additional cost for the online course, in addition to any fee for the classroom portion of the course. Learn more about Internet Courses for Hunter Education on the Colorado Parks and Wildlife website.
Find events near you
Internet conclusion course.
Full Event with Wait List 0 of 36 seats remaining
Location & Schedule
Skyline Hunting and Fishing Club Littleton, CO 80127
- Saturday, May 11, 2024 7:30am - 4:00pm
Full Event with Wait List 0 of 35 seats remaining
GJ Colorado Parks and Wildlife Hunter Ed Building Grand Junction, CO 81505
- Saturday, May 11, 2024 7:30am - 5:00pm
Full Event with Wait List 0 of 70 seats remaining
Colorado Parks and Wildlife Hunter Education Bldg Denver, CO 80216
- Saturday, May 11, 2024 7:30am - 3:00pm
Full Event with Wait List 0 of 30 seats remaining
High Plains Shooting Range Eaton, CO 80550
- Saturday, May 11, 2024 8:30am - 4:00pm
Registration Open 27 of 50 seats remaining
Eaton Public Library Eaton, CO 80615
- Saturday, May 11, 2024 10:15am - 3:15pm
- Plus, 1 additional day.
Full Event with Wait List 0 of 39 seats remaining
- Saturday, May 18, 2024 8:00am - 4:00pm
Full Event with Wait List 0 of 75 seats remaining
Thompson Rivers Parks and Recreation Miliken, CO 80543
- Saturday, May 18, 2024 9:00am - 2:00pm
JAX Outdoor Gear - Fort Collins Fort Collins, CO 80524
- Saturday, May 18, 2024 10:15am - 3:15pm
Full Event with Wait List 0 of 50 seats remaining
Bass Pro Outdoor World Colorado Springs, CO 80921
- Saturday, May 25, 2024 9:00am - 5:00pm
Registration Open 26 of 70 seats remaining
- Saturday, June 8, 2024 8:00am - 3:00pm

Kalkomey is the official provider of recreational safety education materials for all 50 states.
Online Boating and Hunting Licenses and Recreational Safety Education
- boat-ed.com
- hunter-ed.com
- bowhunter-ed.com
- offroad-ed.com
- snowmobile-ed.com
© 1998 - 2024 All rights reserved. View Privacy and Terms of Use.
Blog The Education Hub
https://educationhub.blog.gov.uk/2024/04/26/when-are-year-6-sats-2024-key-dates-for-parents-and-pupils/
When are year 6 SATs 2024? Key dates for parents and pupils
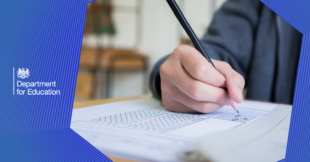
Year 6 pupils in England will soon be taking the key stage 2 (KS2) national curriculum tests, which are often referred to as SATs.
The assessments are used to measure school performance and to make sure individual pupils are being supported in the best way possible as they move into secondary school .
When are SATs?
This year, SATs will take place over four days from 13 May to 16 May 2024.
The timetable is as follows:
What are the tests on?
While pupils won’t be able to see what’s on the test beforehand, t he assessments only include questions on things that children should already have been taught as part of the national curriculum.
You can find past papers on GOV.UK .
As usual, there won’t be a test for English writing or science. Instead, this will be reported as a teacher assessment judgement.
This is a judgement teachers will make based on your child’s work at the end of KS2.
Does my child need to revise for SATs?
Children shouldn’t be made to feel any unnecessary pressure when it comes to the KS2 assessments and t eachers will make sure that all pupils in their class are prepared.
You should follow their general advice about supporting your child’s education throughout the year and ahead of the tests.
While it is statutory for schools to hold the assessments, headteachers make the final decision about whether a pupil participates in them.
Some pupils – for example those with special education needs or disabilities – may be assessed under different arrangements if these are more appropriate.
If you have concerns about your child participating in the KS2 tests, you should speak to your school in the first instance.
What if my child finds the SATs tests too difficult?
It’s important to remember that one of the purposes of the key stage 2 assessments is to identify each pupil's strengths and the areas where they may have fallen behind in their learning as they head into secondary school.
The results will help their new school determine in which areas your child needs the most support.
The tests are designed to be challenging to measure attainment, including stretching the most able children. It means some pupils will find them harder than others.
It takes three years to create appropriate tests. During the process, they’re rigorously trialled with year 6 pupils and reviewed by education and inclusion experts to make sure they’re the right difficulty level.
The Standards and Testing Agency (STA) is responsible for developing the tests, and Ministers don't have any influence on their content.
When will we find out the results of SATs?
Schools will receive test results on Tuesday 9 July 2024.
Before the end of the summer term, your child’s school will send you a report which will include test results and teacher assessment judgements.
This should provide you with a good sense of the standard at which your child is working in each subject.
The school will report your child’s test results as a scaled score for each subject. This is created from the number of marks your child scores in a particular test. A scaled score:
- below 100 means that your child may need more support to help them reach the expected standard;
- of 100 or more means that your child is working at, or above, the expected standard for the key stage.
If your child is working below the overall standard of the key stage, or they have special educational needs, reporting will be different, and you should speak to your child’s teacher for more information.
You can also find more information about results at the end of key stage 2 on GOV.UK.
You may also be interested in:
- How we are helping to inspire primary school children about their future careers
- What is the multiplication tables check and why is it important?
- SATs leaflet for parents
Tags: KS2 , primary school , SATs , SATs 2023 , SATs results , Secondary School
Sharing and comments
Share this page, related content and links, about the education hub.
The Education Hub is a site for parents, pupils, education professionals and the media that captures all you need to know about the education system. You’ll find accessible, straightforward information on popular topics, Q&As, interviews, case studies, and more.
Please note that for media enquiries, journalists should call our central Newsdesk on 020 7783 8300. This media-only line operates from Monday to Friday, 8am to 7pm. Outside of these hours the number will divert to the duty media officer.
Members of the public should call our general enquiries line on 0370 000 2288.
Sign up and manage updates
Follow us on social media, search by date, comments and moderation policy.
iOS 18 release date: When to expect the betas and public launch
We’re not far from the first official look at iOS 18 at WWDC 2024 on June 10. Whether you’d like to test out the new OS as soon as the dev beta is available or you’ll wait for the public beta or public launch, follow along for when to expect the iOS 18 release date.
Table of contents
Ios 18 release date: developer beta, public beta, official public release, ios 18 release date conclusion.
Apple historically reveals its major updates of iOS during the WWDC keynote and launches the first developer beta within hours.
Next, the first free iOS public beta usually launches about a month later with the official public release coming shortly after the fall iPhone event.
The upcoming major new iOS release is expected to come with big AI upgrades including on-device processing, a revamped version of Siri, smart integration with Messages, Apple Music, iWork, and more.
Other changes should include RCS support, new customization options for the Home Screen and the Calculator app finally landing on iPad (and Mac). Read more in our full guide:
- iOS 18: Here’s everything we know so far
iOS 18 release date: When does iOS 18 come out?
- iOS 18 developer beta – expected June 10 release at WWDC
- iOS 18 public beta – expected between the end of June and mid-July
- iOS 18 public release – expected in mid to late September
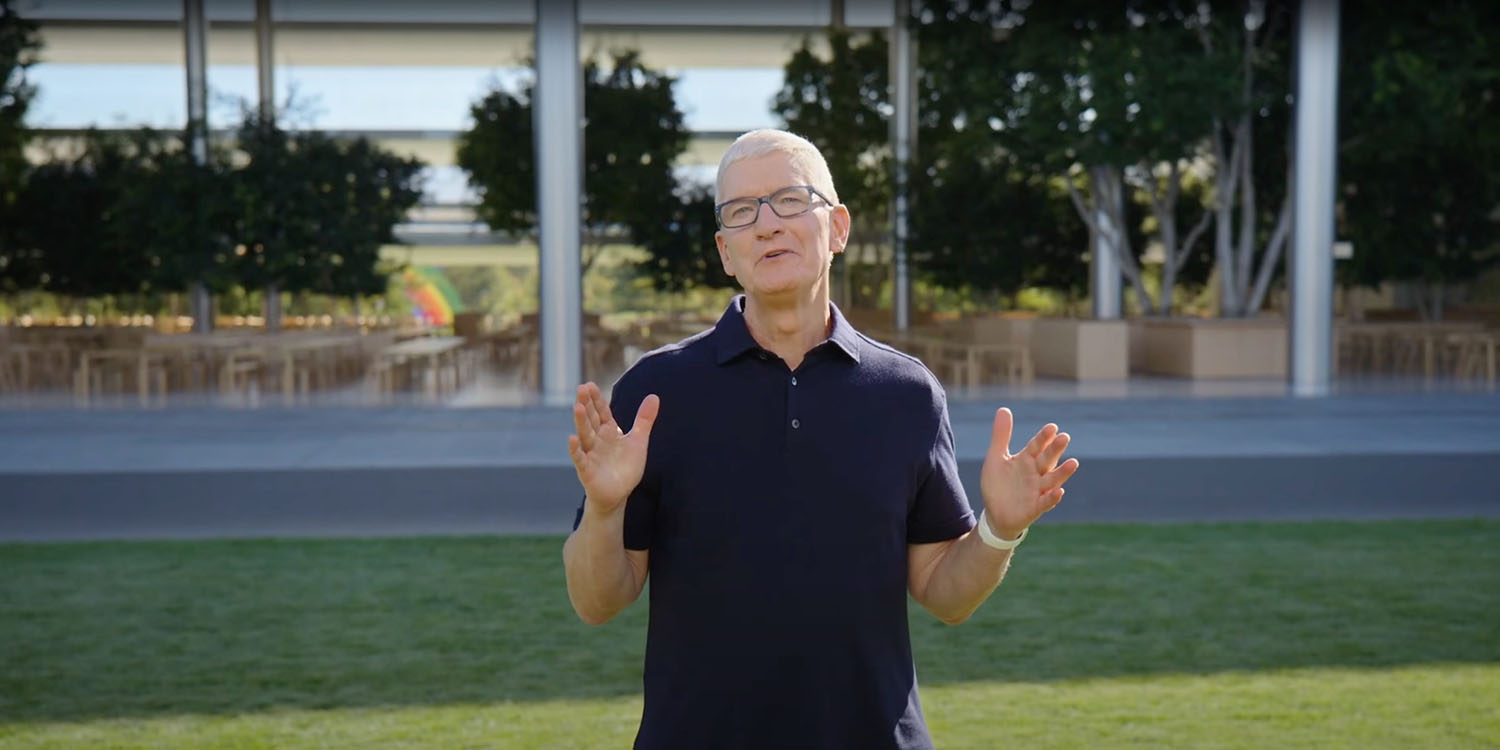
Apple has made a tradition of releasing the first developer beta of each major iOS update on the same day as the yearly WWDC keynote.
- WWDC kicks off with the keynote on June 10 , expect the first iOS 18 developer beta to be released shortly afterward unless Apple switches things up
Here’s a look at the past five years of dev beta releases:
- iOS 17 developer beta 1: June 5
- iOS 16 developer beta 1: June 6
- iOS 15 developer beta 1: June 7
- iOS 14 developer beta 1: June 22 (delay due to pandemic)
- iOS 13 developer beta 1: June 3
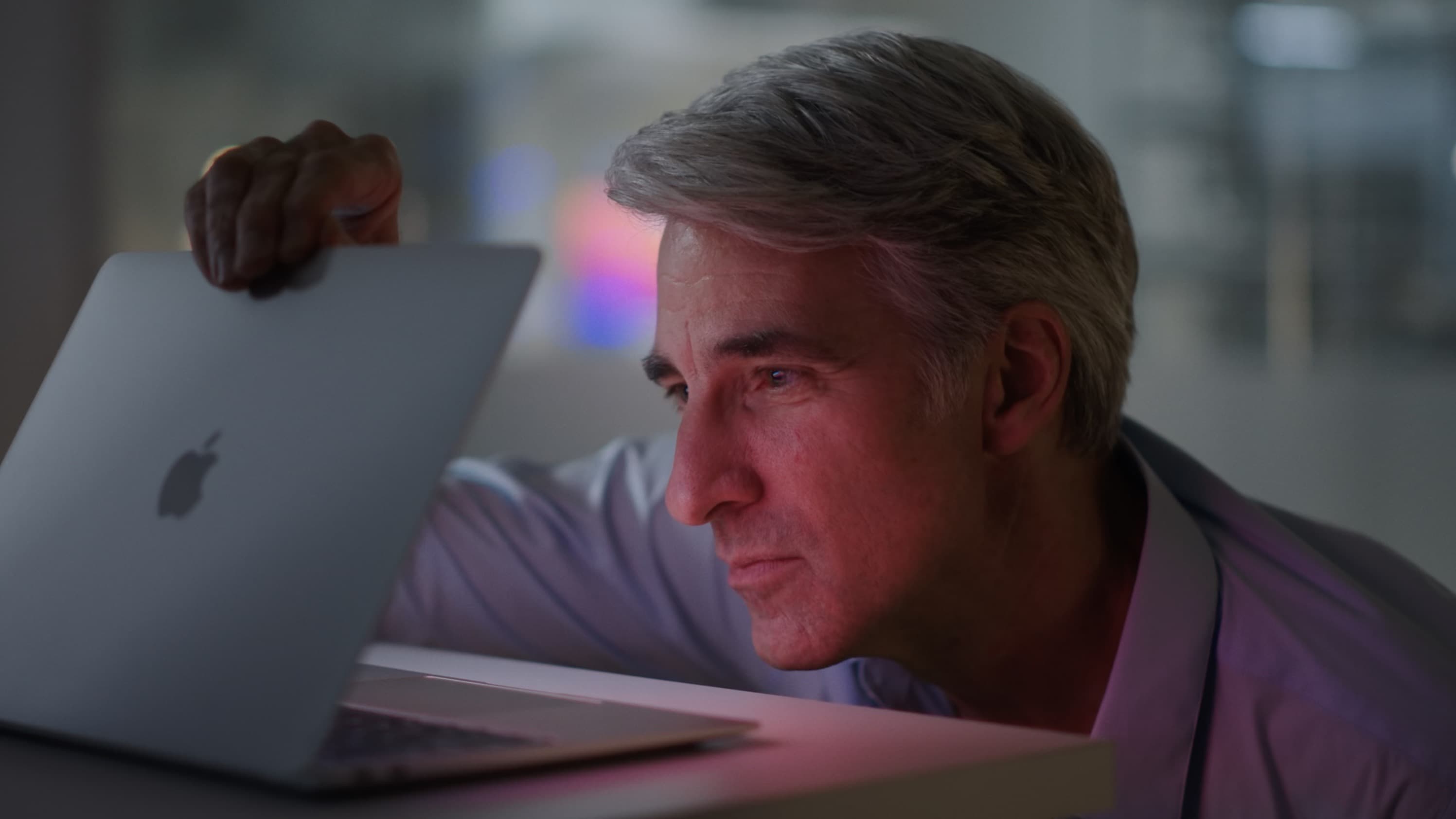
- The first iOS public beta typically arrives four to five weeks after the first developer beta launches in June. Historically that’s been between late June and early July .
Here’s a look at the last few years:
- iOS 17 public beta 1 : July 12, 2023 (after June 5 developer beta release)
- iOS 16 public beta 1 : July 11, 2022 (after June 6 developer beta release)
- iOS 15 public beta 1 : June 30, 2021 (after June 7 developer beta release)
- iOS 14 public beta 1 : July 9, 2020 (after June 22 developer beta release)
- iOS 13 public beta 1 : June 24, 2019 (after June 3 developer beta release)
- It’s likely iOS 18 will come out in mid to late September unless Apple throws us a curveball
- If Apple has its iPhone 16 event between September 9-17, we could see iOS 18 officially launch between September 16-23.
Here’s a look at the last four years of iOS releases:
- iOS 17 : September 18, 2023 (after September 12 event)
- iOS 16 : September 16, 2022 (after September 7 event)
- iOS 15 : September 20, 2021 (after September 14 event)
- iOS 14 : September 16, 2020 (after September 15 event)
- iOS 13 : September 19, 2019 (after September 10 event)
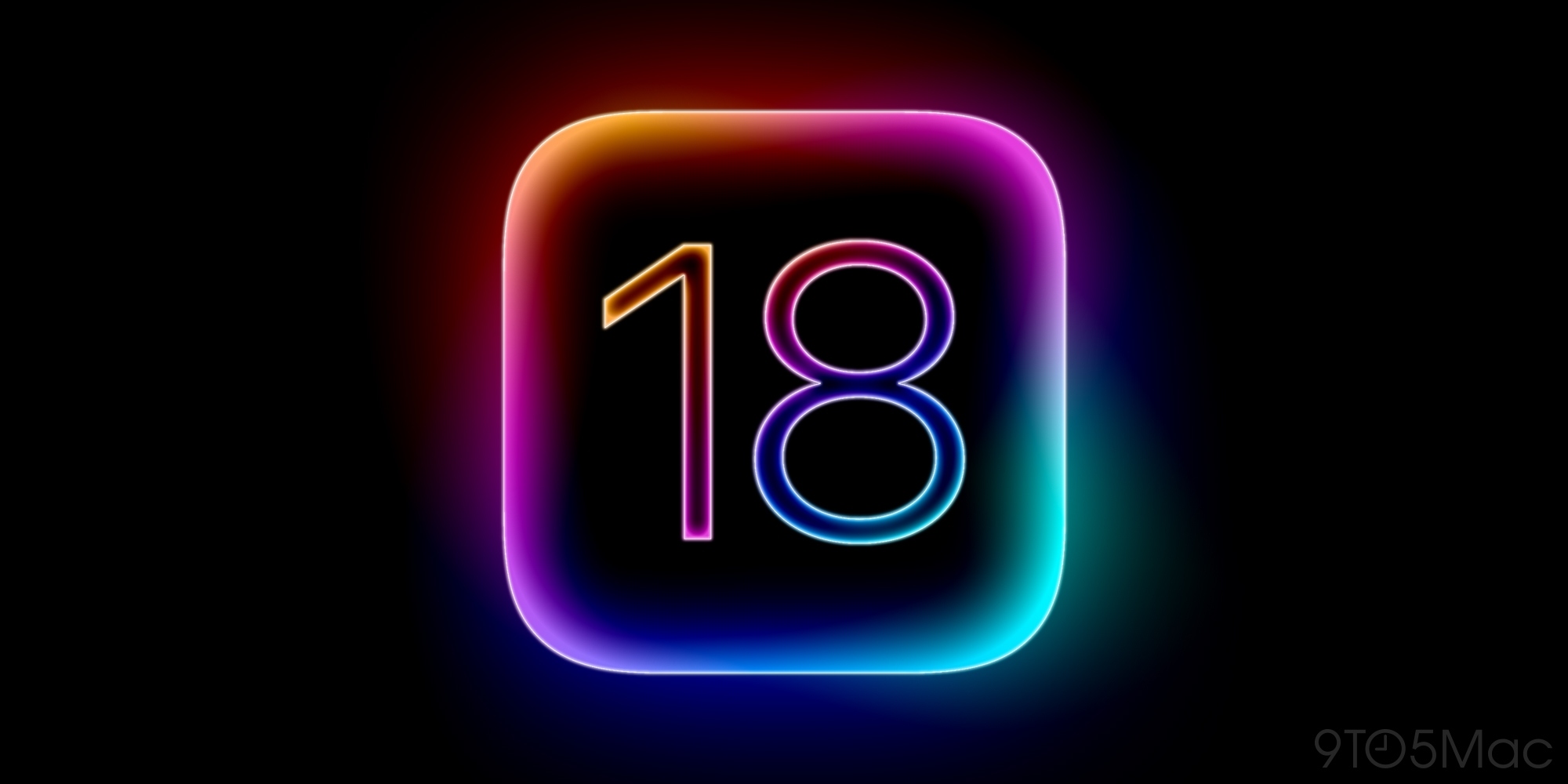
Since you can install iOS betas right within iPhone Settings (since iOS 16.4), it will be smooth and easy to try out iOS 18 come June.
Are you planning to run the upcoming beta or will you wait for the polished, public release? Share your thoughts in the comments!
Thanks for reading our guide on the iOS 18 release date! Check out more details on what to expect with iOS 18:
- iOS 18: The latest on Apple’s plans for on-device AI
- iOS 18 reportedly bringing these two upgrades to Apple Maps
- iOS 18 again said to be ‘the most ambitious overhaul’ to the iPhone ever
- Gurman: iOS 18 AI features to be powered by ‘entirely on-device’ LLM, offering privacy and speed benefits
Top image by 9to5Mac
FTC: We use income earning auto affiliate links. More.

Check out 9to5Mac on YouTube for more Apple news:
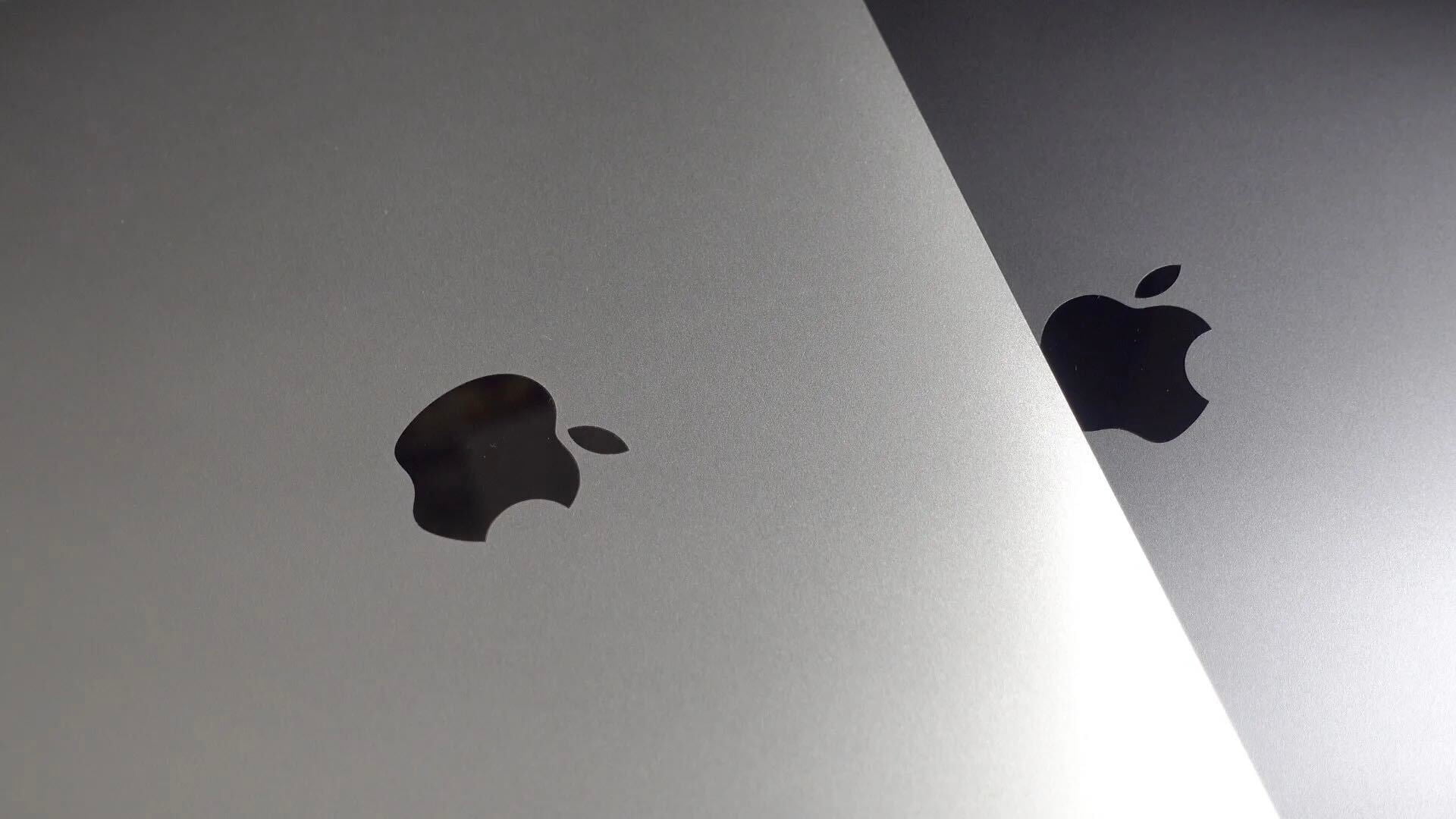
A collection of tutorials, tips, and tricks from…
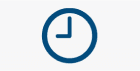
Michael is an editor for 9to5Mac. Since joining in 2016 he has written more than 3,000 articles including breaking news, reviews, and detailed comparisons and tutorials.
Michael Potuck's favorite gear

Satechi USB-C Charger (4 ports)
Really useful USB-C + USB-A charger for home/work and travel.

Apple Leather MagSafe Wallet
My slim wallet of choice for iPhone 12

Manage push notifications

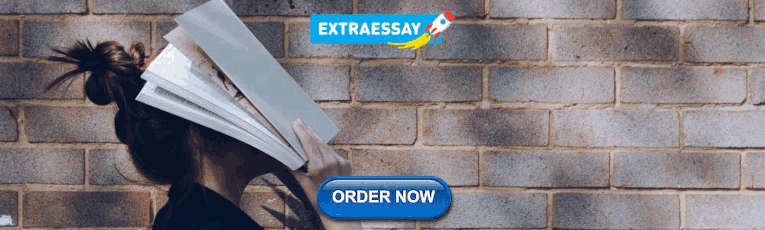
IMAGES
VIDEO
COMMENTS
the schematic representation of the constructs of evaluation, measurement, and testing as applied in education. The conceptual framework sought to illustrate the superordinate-subordinate relationship between these concepts and demonstrate the areas of overlap. Figure 1. Lynch's model of evaluation, measurement, and testing [4]
Giving more time for fewer, more complex or richer testing questions can also increase performance, in part because it reduces anxiety. Research shows that simply introducing a time limit on a test can cause students to experience stress, so instead of emphasizing speed, teachers should encourage students to think deeply about the problems they ...
CONCLUSION 5-1 The studies conducted to assess content validity are in line with those called for in the Standards for Educational and Psychological Testing in place in 1992 and currently in 2016. The results of these studies suggested that changes in the achievement-level descriptors (ALDs) were needed, and they were subsequently made.
Summative assessments are used to evaluate student learning, skill acquisition, and academic achievement at the conclusion of a defined instructional period—typically at the end of a project, unit, course, semester, program, or school year. Generally speaking, summative assessments are defined by three major criteria: The tests, assignments, or projects are used to determine whether students
Test, measurement, and evaluation are concepts used in education to explain. how the progress of learning and the final learning outcomes of students are. assessed. However, the terms are often ...
To see the horizon of educational assessment, a history of how assessment has been used and analysed from the earliest records, through the 20th century, and into contemporary times is deployed. Since paper-and-pencil assessments validity and integrity of candidate achievement has mattered. Assessments have relied on expert judgment. With the massification of education, formal group ...
Two test developers—Educational Testing Service (ETS) and National Evaluation Systems (NES)—develop the vast majority of these tests. Conclusions Because a teacher's work is complex, even a set of well-designed tests cannot measure all of the prerequisites of competent beginning teaching.
Citation: Phelps, R.P. (2016). Teaching to the test: A very large red herring. Nonpartisan Education Review/Essays, 12(1). According to Literary Devices "Red herring is a kind of fallacy that is an irrelevant topic introduced in an argument to divert the attention of listeners or readers from the original issue.
Federal K-12 education laws and testing. Simply put, today's federal K-12 education laws ask states, in exchange for federal funding, to ensure that students are meeting grade-level benchmarks ...
INTRODUCTION. The rigor of education research, including research in medical education, has been under scrutiny for years. 1,2 On the technical side, issues raised include lack of examination of the psychometric properties of assessment instruments and/or insufficient reporting of validity and reliability. 3-5 On the applied side, researchers have frequently based their conclusions on ...
The ubiquity of multiple-choice testing in education today stems from the many advantages that it offers relative to other assessment formats. For example, multiple-choice tests are relatively easy to score, offer greater objectivity in grading, and allow more content to be covered by reducing the time it takes test-takers to respond to questions.
Again, standardized tests are a good measure of a student's achievement, the standardized tests and increased testing are a better college preparation, and the testing is not too stressful for students. Immediately, we need to call the United States Department of Education and tell them that standardized tests should be kept in schools. Sources.
Test Construction. Most tests are a form of summative assessment; that is, they measure students' performance on a given task. (For more information on summative assessment, see the CITL resource on formative and summative assessment.)McKeachie (2010) only half-jokes that "Unfortunately, it appears to be generally true that the examinations that are the easiest to construct are the most ...
Teachers, as frontline providers of education, are increasingly targets of accountability reforms. Such reforms often narrowly define 'teacher quality' around performative terms. Past research suggests holding teachers to account for student performance measures (i.e. test scores) damages their job satisfaction, including increasing stress and burnout. This article examines whether the ...
Reliability is important in the design of assessments because no assessment is truly perfect. A test produces an estimate of a student's "true" score, or the score the student would receive if given a perfect test; however, due to imperfect design, tests can rarely, if ever, wholly capture that score.
Step 1: Return to your thesis. To begin your conclusion, signal that the essay is coming to an end by returning to your overall argument. Don't just repeat your thesis statement —instead, try to rephrase your argument in a way that shows how it has been developed since the introduction. Example: Returning to the thesis.
Many, as well, focus on facts and details rather than larger themes of causes and consequences of events. The shortfalls of these approaches are not apparent if the only test of learning involves tests of memory, but when the transfer of learning is measured, the advantages of learning with understanding are likely to be revealed.
For instance, in mathematics, a diagnostic test might reveal that a student excels in algebra but struggles with geometry, allowing educators to provide targeted assistance to improve geometry skills. 3. Proficiency Test. Proficiency tests evaluate a student's level of expertise or mastery in a specific subject or skill area.
A hypothesis test is used to test whether or not some hypothesis about a population parameter is true.. To perform a hypothesis test in the real world, researchers obtain a random sample from the population and perform a hypothesis test on the sample data, using a null and alternative hypothesis:. Null Hypothesis (H 0): The sample data occurs purely from chance.
The U.S. Army used a multiple-choice test to measure soldiers' mental abilities for the purpose of sorting, assigning, and discharging them during World War I. This test would become the model for future standardized assessments.17 In 1919, Terman transformed the Army Alpha test into the National Intelligence Tests for school
A standardized diagnostic test uses well-defined testing methods to evaluate students' knowledge. This assessment provides an in-depth analysis of a student's strengths and weaknesses. Standardized diagnostic evaluations usually follow informal evaluations. The teacher starts by gathering unprompted information on the student's level of ...
Conclusion. As has ever been true, edtech holds vast potential to improve learning and teaching for every student and teacher in the United States. In recent years, driven by the emergency of a pandemic, schools have found themselves with more connectivity, devices, and digital resources than at any other moment in history. This current context ...
The Colorado INTERNET-BASED Hunter Education Course is necessary for students to qualify for a Colorado Hunter Education Certificate. The major portion of this course is taken online, allowing the student to complete the mandatory homework. Students must complete the online course before they attend this in-person class session.
Hong Kong education authorities will replace a local assessment for English teachers with an internationally recognised test, prompting a lawmaker to raise concerns that the move might make it ...
The Education Hub is a site for parents, pupils, education professionals and the media that captures all you need to know about the education system. You'll find accessible, straightforward information on popular topics, Q&As, interviews, case studies, and more. ... The Standards and Testing Agency (STA) is responsible for developing the ...
Apple historically reveals its major updates of iOS during the WWDC keynote and launches the first developer beta within hours. Next, the first free iOS public beta usually launches about a month ...