Society Homepage About Public Health Policy Contact
Data-driven hypothesis generation in clinical research: what we learned from a human subject study, article sidebar.
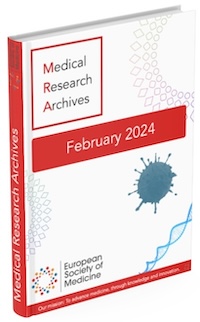
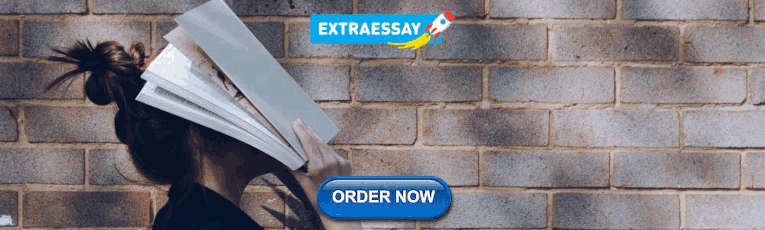
Submit your own article
Register as an author to reserve your spot in the next issue of the Medical Research Archives.
Join the Society
The European Society of Medicine is more than a professional association. We are a community. Our members work in countries across the globe, yet are united by a common goal: to promote health and health equity, around the world.
Join Europe’s leading medical society and discover the many advantages of membership, including free article publication.
Main Article Content
Hypothesis generation is an early and critical step in any hypothesis-driven clinical research project. Because it is not yet a well-understood cognitive process, the need to improve the process goes unrecognized. Without an impactful hypothesis, the significance of any research project can be questionable, regardless of the rigor or diligence applied in other steps of the study, e.g., study design, data collection, and result analysis. In this perspective article, the authors provide a literature review on the following topics first: scientific thinking, reasoning, medical reasoning, literature-based discovery, and a field study to explore scientific thinking and discovery. Over the years, scientific thinking has shown excellent progress in cognitive science and its applied areas: education, medicine, and biomedical research. However, a review of the literature reveals the lack of original studies on hypothesis generation in clinical research. The authors then summarize their first human participant study exploring data-driven hypothesis generation by clinical researchers in a simulated setting. The results indicate that a secondary data analytical tool, VIADS—a visual interactive analytic tool for filtering, summarizing, and visualizing large health data sets coded with hierarchical terminologies, can shorten the time participants need, on average, to generate a hypothesis and also requires fewer cognitive events to generate each hypothesis. As a counterpoint, this exploration also indicates that the quality ratings of the hypotheses thus generated carry significantly lower ratings for feasibility when applying VIADS. Despite its small scale, the study confirmed the feasibility of conducting a human participant study directly to explore the hypothesis generation process in clinical research. This study provides supporting evidence to conduct a larger-scale study with a specifically designed tool to facilitate the hypothesis-generation process among inexperienced clinical researchers. A larger study could provide generalizable evidence, which in turn can potentially improve clinical research productivity and overall clinical research enterprise.
Article Details
The Medical Research Archives grants authors the right to publish and reproduce the unrevised contribution in whole or in part at any time and in any form for any scholarly non-commercial purpose with the condition that all publications of the contribution include a full citation to the journal as published by the Medical Research Archives .
ORIGINAL RESEARCH article
Temporal dynamics of hypothesis generation: the influences of data serial order, data consistency, and elicitation timing.

- 1 Department of Psychological Sciences, Birkbeck College, University of London, London, UK
- 2 Department of Psychology, University of Oklahoma, Norman, OK, USA
The pre-decisional process of hypothesis generation is a ubiquitous cognitive faculty that we continually employ in an effort to understand our environment and thereby support appropriate judgments and decisions. Although we are beginning to understand the fundamental processes underlying hypothesis generation, little is known about how various temporal dynamics, inherent in real world generation tasks, influence the retrieval of hypotheses from long-term memory. This paper presents two experiments investigating three data acquisition dynamics in a simulated medical diagnosis task. The results indicate that the mere serial order of data, data consistency (with previously generated hypotheses), and mode of responding influence the hypothesis generation process. An extension of the HyGene computational model endowed with dynamic data acquisition processes is forwarded and explored to provide an account of the present data.
Hypothesis generation is a pre-decisional process by which we formulate explanations and beliefs regarding the occurrences we observe in our environment. The hypotheses we generate from long-term memory (LTM) bring structure to many of the ill-structured decision making tasks we commonly encounter. As such, hypothesis generation represents a fundamental and ubiquitous cognitive faculty on which we constantly rely in our day-to-day lives. Given the regularity with which we employ this process, it is no surprise that hypothesis generation forms a core component of several professions. Auditors, for instance, must generate hypotheses regarding abnormal financial patterns, mechanics must generate hypotheses concerning car failure, and intelligence analysts must interpret the information they receive. Perhaps the clearest example, however, is that of medical diagnosis. A physician observes a pattern of symptoms presented by a patient (i.e., data) and uses this information to generate likely diagnoses (i.e., hypotheses) in an effort to explain the patient’s presenting symptoms. Given these examples, the importance of developing a full understanding of the processes underlying hypothesis generation is clear, as the consequences of impoverished or inaccurate hypothesis generation can be injurious.
Issues of temporality pervade hypothesis generation and its underlying information acquisition processes. Hypothesis generation is a task situated at the confluence of external environmental dynamics and internal cognitive dynamics. External dynamics in the environment dictate the manifestation of the information we acquire and use as cues to retrieve likely hypotheses from LTM. Internal cognitive dynamics then determine how this information is used in service of the generation process and how the resulting hypotheses are maintained over the further course of time as judgments and decisions are rendered. Additionally, these further internal processes are influenced by and interact with the ongoing environmental dynamics as new information is acquired. These complicated interactions govern the beliefs (i.e., hypotheses) we entertain over time. It is likely that these factors interact in such a manner that would cause the data acquisition process to deviate from normative prescriptions.
Important to the present work is the fact that data acquisition generally occurs serially over some span of time. This, in turn, dictates that individual pieces of data are acquired in some relative temporal relation to one another. These constraints, individual data acquisition over time and the relative ordering of data, are likely to have significant consequences for hypothesis generation processes. Given these basic constraints, it is intuitive that temporal dynamics must form an integral part of any comprehensive account of hypothesis generation processes. At present there exists only a scant amount of data concerning the temporal dynamics of hypothesis generation. Thus, the influences of the constraints operating over these processes are not yet well understood. Until such influences are addressed more deeply at an empirical and theoretical level, a full understanding of hypothesis generation processes will remain speculative.
The empirical paradigm used in the following experiments is a simulated diagnosis task comprised of two main phases. The first phase represents a form of category learning in which the participant learns the conditional probabilities of medical symptoms (i.e., data) and fictitious diseases (i.e., hypotheses), from experience over time by observing a large sample of hypothetical pre-diagnosed patients. The second phase of the task involves presenting symptoms to the participant whose task it is to generate (i.e., retrieve) likely disease states from memory. At a broader level, such experiments involving a learning phase followed by a decision making phase have been utilized widely in previous experiments (e.g., McKenzie, 1998 ; Cooper et al., 2003 ; Nelson et al., 2010 ; Sprenger and Dougherty, 2012 ). In the to-be-presented experiments, we presented the symptoms sequentially and manipulated the symptom’s sequence structures in the “decision making phase.” As the data acquisition unfolds over time, the results of these experiments provide insight into the dynamic data acquisition and hypothesis generation processes operating over time that are important for computational models.
In this paper, we present a novel extension of an existing computational model of hypothesis generation. This extension is designed to capture the working memory dynamics operating during data acquisition and how these factors contribute to the process of hypothesis generation. Additionally, two experiments exploring three questions of interest to dynamic hypothesis generation are described whose results are captured by this model. Experiment 1 utilized an adapted generalized order effects paradigm to assess how the serial position of an informative piece of information (i.e., a diagnostic datum), amongst uninformative information (i.e., non-diagnostic data), influences its contribution to the generation process. Experiment 2 investigated (1) how the acquisition of data inconsistent with previously generated hypotheses influences further generation and maintenance processes and (2) if generation behavior differs when it is based on the acquisition of a set of data vs. when those same pieces of data are acquired in isolation and generation is carried out successively as each datum is acquired. This distinction underscores different scenarios in which it is advantageous to maintain previously acquired data vs. previously generated hypotheses over time.
HyGene: A Computational Model of Hypothesis Generation
HyGene ( Thomas et al., 2008 ; Dougherty et al., 2010 ), short for hypothesis generation, is a computational architecture addressing hypothesis generation, evaluation, and testing. This framework has provided a useful account through which to understand the cognitive mechanisms underlying these processes. This process model is presented in Figure 1 .
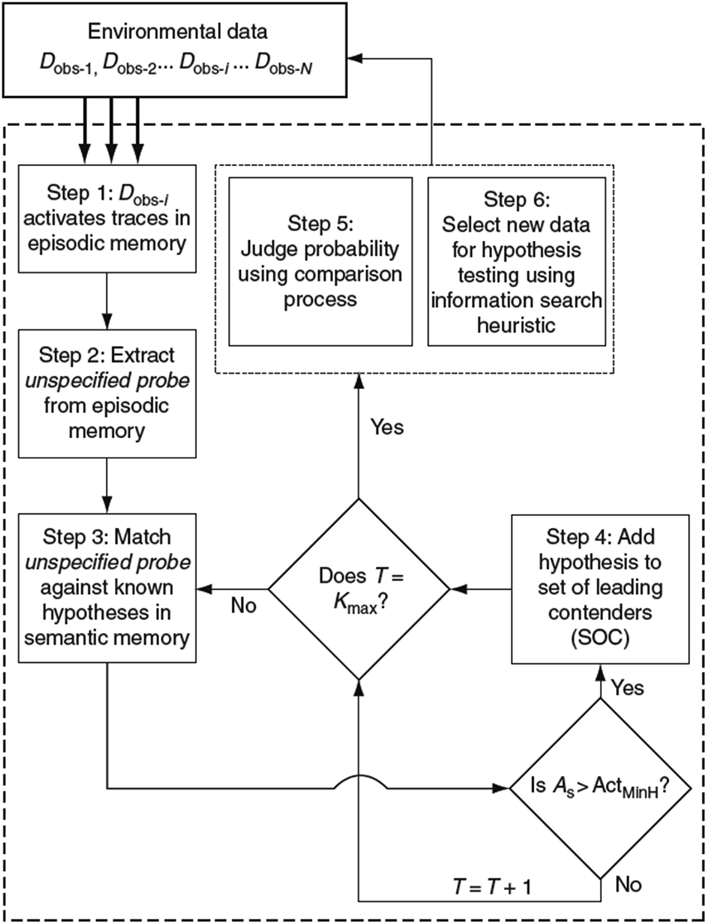
Figure 1. Flow diagram of the HyGene model of hypothesis generation, judgment, and testing . A s , semantic activation of retrieved hypothesis; Act MinH , minimum semantic activation criterion for placement of hypothesis in SOC; T , total number of retrieval failures; and K max , number of retrieval failures allowed before terminating hypothesis generation.
HyGene rests upon three core principles. First, as underscored by the above examples, it is assumed that hypothesis generation represents a generalized case of cued recall. That is, the data observed in the environment (D obs ), which one would like to explain, act as cues prompting the retrieval of hypotheses from LTM. For instance, when a physician examines a patient, he/she uses the symptoms expressed by the patient as cues to related experiences stored in LTM. These cues activate a subset of related memories from which hypotheses are retrieved. These retrieval processes are indicated in Steps 1, 2, and 3 shown in Figure 1 . Step 1 represents the environmental data being matched against episodic memory. In step 2, the instances in episodic memory that are highly activated by the environmental data contribute to the extraction of an unspecified probe representing a prototype of these highly activated episodic instances. This probe is then matched against all known hypotheses in semantic memory as indicated in Step 3. Hypotheses are then sampled into working memory based on their activations resulting from this semantic memory match.
As viable hypotheses are retrieved from LTM, they are placed in the Set of Leading Contenders (SOC) as demonstrated in Step 4. The SOC represents HyGene’s working memory construct to which HyGene’s second principle applies. The second principle holds that the number of hypotheses that can be maintained at one time is constrained by cognitive limitations (e.g., working memory capacity) as well as task characteristics (e.g., divided attention, time pressure). Accordingly, the more working memory resources that one has available to devote to the generation and maintenance of hypotheses, the greater the number of additional hypotheses can be placed in the SOC. Working memory capacity places an upper bound on the amount of hypotheses and data that one will be able to maintain at any point in time. In many circumstances, however, attention will be divided by a secondary task. Under such conditions this upper bound is reduced as the alternative task siphons resource that would otherwise allow the population of the SOC to its unencumbered capacity ( Dougherty and Hunter, 2003a , b ; Sprenger and Dougherty, 2006 ; Sprenger et al., 2011 ).
The third principle states that the hypotheses maintained in the SOC form the basis from which probability judgments are derived and provide the basis from which hypothesis testing is implemented. This principle underscores the function of hypothesis generation as a pre-decisional process underlying higher-level decision making tasks. The tradition of much of the prior research on probability judgment and hypothesis testing has been to provide the participant with the options to be judged or tested. HyGene highlights this as somewhat limiting the scope of the conclusions drawn from such procedures, as decision makers in real world tasks must generally generate the to-be-evaluated hypotheses themselves. As these higher-level tasks are contingent upon the output of the hypothesis generation process, any conclusions drawn from such experimenter-provided tasks are likely limited to such conditions.
Hypothesis Generation Processes in HyGene
The representation used by HyGene was borrowed from the multiple-trace global matching memory model MINERVA II ( Hintzman, 1986 , 1988 ) and the decision making model MINERVA-DM ( Dougherty et al., 1999 ) 1 . Memory traces are represented in the model as a series of concatenated minivectors arbitrarily consisting of 1, 0, and −1 s where each minivector represents either a hypothesis or a piece of data (i.e., a feature of the memory). Separate episodic and semantic memory stores are present in HyGene which are made up of separate instances of such concatenated feature minivectors. While semantic memory contains prototypes of each disease, episodic memory contains individual traces for every experience the model acquires.
Retrieval is initiated when D obs are matched against each of data minivectors in episodic LTM. This returns an LTM activation value for each trace in episodic LTM whereby greater overlap of features present in the trace and present in the D obs results in greater activation. A threshold is applied to these episodic activation values such that only traces with long-term episodic activation values exceeding this threshold contribute to additional processing in the model. A prototype is extracted from this subset of traces which is then used as a cue to semantic memory for the retrieval of hypotheses. We refer to this cue as the unspecified probe . This unspecified probe is matched against all hypotheses in semantic memory which returns an activation value for each known hypothesis. The activation values for each hypothesis serve as input into retrieval through sampling via Luce’s choice rule. Generation proceeds in this way until a stopping rule is reached based on the total number of resamples of previously generated hypotheses (i.e., retrieval failures).
In its current form, the HyGene model is static with regards to data acquisition and utilization. The model receives all available data from the environment simultaneously and engages in only a single iteration of hypothesis generation. Given the static nature of the model, each piece of data used to cue LTM contributes equally to the recall process. Based on effects observed in related domains, however, it seems reasonable to suspect that all available data do not contribute equally in hypothesis generation tasks. For example, Anderson (1965) , for instance, observed primacy weightings in an impression formation task in which attributes describing a person were revealed sequentially. Moreover, recent work has demonstrated biases in the serial position of data used to support hypothesis generation tasks ( Sprenger and Dougherty, 2012 ). By ignoring differential use of available data in the generation process, HyGene, as previously implemented, ignores temporal dynamics influencing hypothesis generation tasks. In our view, what is needed is an understanding of working memory dynamics as data acquisition, hypothesis generation, and maintenance processes unfold and evolve over time in hypothesis generation tasks.
Dynamic Working Memory Buffer of the Context-Activation Model
The context-activation model of memory ( Davelaar et al., 2005 ) is one of the most comprehensive models of memory recall to date. It is a dual-trace model of list memory accounting for a large set of data from various recall paradigms. Integral to the model’s behavior are the activation-based working memory dynamics of its buffer. The working memory buffer of the model dictates that the activations of the items in working memory systematically fluctuate over time as the result of competing processes described by Eq. 1.
Equation 1: activation calculation of the context-activation model
The activation level of each item, x i , is determined by the item’s activation on the previous time step, self-recurrent excitation that each item recycles onto itself α, inhibition from the other active items β, and zero-mean Gaussian noise N with standard deviation σ. Lastly, λ is the Euler integration constant that discretizes the differential equation. Note, however, that as this equation is applied in the present model, noise was only applied to an item’s activation value once it was presented to the model 2 .
Figure 2 illustrates the interplay between the competitive buffer dynamics in a noiseless run of the buffer when four pieces of data have been presented to the model successively. The activation of each datum rises as it is presented to the model and its bottom-up sensory input contributes to the activation. These activations are then dampened in the absence of bottom-up input as inhibition from the other items drive activation down. Self-recurrency can keep an item in the buffer in the absence of bottom-up input, but this ability is in proportion to the amount of competition from other items in the buffer. The line at 0.2 represents the model’s working memory threshold. In the combined dynamic HyGene model (utilizing the dynamics of the buffer to determine the weights of the data) this WM threshold separates data that are available to contribute to generation (>0.2) from those that will not (<0.2). That is, if a piece of data’s activation is greater than this threshold at the time of generation then it contributes to the retrieval of hypotheses from LTM and is weighted by its amount of activation. However, if, on the other hand, a piece of data falls below the WM threshold then it is weighted zero and as a result does not contribute to the hypothesis retrieval.
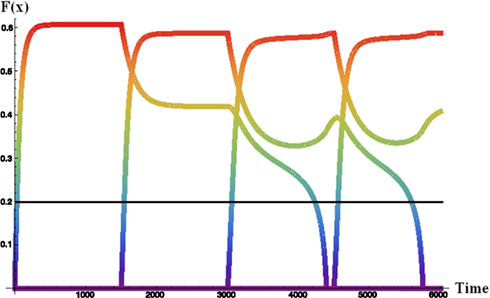
Figure 2. Noiseless activation trajectories for four sequentially received data in the dynamic activation-based buffer . Each item presented to the buffer for 1500 iterations. F ( x ) = memory activation.
The activations of individual items are sensitive the amount of recurrency (alpha) and inhibition (beta) operating in the buffer. Figure 3 demonstrates differential sensitivity to values of alpha and beta by item presentation serial position (1 through 4 in this case). This plot was generated by running the working memory buffer across a range of alpha and beta values for 50 runs at each parameter combination. Each panel presents the activation of an item in a four-item sequence after the final item has been presented. The activation levels vary with serial position, as shown by the differences among the four panels and with the value of the alpha and beta parameters, as shown within each panel. It can be seen that items one and two are mainly sensitive to the value of alpha. As alpha is increased, these items are more likely to maintain high activation values at the end of the data presentation. Item three demonstrates a similar pattern under low values of beta, but under higher values of beta this item only achieves modest activation as it cannot overcome the strong competition exerted by item one and two. Item four demonstrates a pattern distinct from the others. Like the previous three items the value of alpha limits the influence of beta up to a certain point. At moderate to high values of alpha, however, beta has a large impact on the activation value of the fourth item. At very low values of beta (under high alpha) this item is able to attain high activation, but quickly moves to very low activation values with modest increases in beta. These modest increases in beta are enough to make the competition from the three preceding items severe enough that the fourth item cannot overcome it.
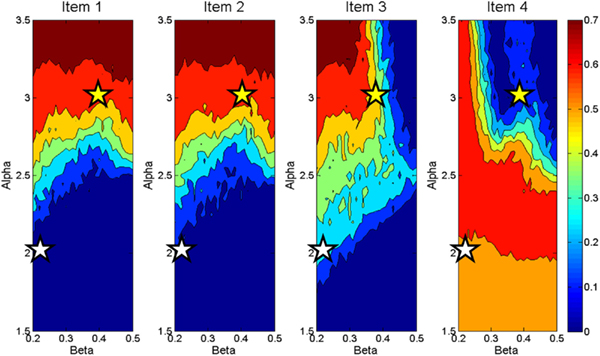
Figure 3. Contour plot displaying activation values of four items at end of data presentation across a range of Beta ( X axes) and Alpha ( Y axes) demonstrating differences in activation weight gradients produced by the working memory buffer .
Taken as a whole, these plots describe differences in the activation gradients (profiles of activation across all four items) taken on by the buffer across various values of alpha and beta. For instance, the stars in the plot represent two different settings of alpha and beta which result in different activation gradients across the items. The settings of alpha = 2 and beta = 0.2 represented by the white stars, for instance, represent an instance of recency in the item activations. That is, the earlier items have only slight activation, the third item modest activation, and the last item is highly active relative to the others. Tracing the activations across the settings of alpha = 3 and beta = 0.4 represented by the yellow stars, on the other hand, shows a primacy gradient in which the earlier items are highly active, item three is less so, and the last item’s activation is very low. As will be seen, this pattern of activation values across different values of alpha and beta will become important for the computational account of Experiment 2. At a broader level, however, this plot shows possible activation gradients that can be obtained with the working memory buffer. In general, the activation gradients produce recency, but primacy gradients are also possible. Additionally, there are patterns of activation across items that the buffer cannot produce. For instance an inverted U shape of item activations would not result from the buffer’s processes.
These dynamics are theoretically meaningful as they produce data patterns which item-based working memory buffers (e.g., SAM; Raaijmakers and Shiffrin, 1981 ) cannot account for. For example, the buffer dynamics of the context-activation model dictate that items presented early in a sequence will remain high in activation (i.e., remain in working memory) under fast presentation rates. That is, under fast presentation rates the model predicts a primacy effect. Such effects have been observed in cued recall ( Davelaar et al., 2005 ), free recall ( Usher et al., 2008 ), and in a hypothesis generation task ( Lange et al., 2012 ). Given these findings and the unique ability of the activation-based buffer to account for these effects, we have selected the activation-based buffer as our starting point for endowing the HyGene model with dynamic data acquisition processes.
A Dynamic Model of Hypothesis Generation: Endowing HyGene with Dynamic Data Acquisition
The competitive working memory processes of the context-activation model’s dynamic buffer provide a principled means for incorporating fine-grained temporal dynamics into currently static portions of HyGene. As a first step in incorporating the dynamic working memory processes of the working memory buffer, we use the buffer as a means to endow HyGene with dynamic data acquisition. In so doing, the HyGene architecture gains two main advantages. As pointed out by Sprenger and Dougherty (2012) , any model of hypothesis generation seeking to account for situations in which data are presented sequentially needs a means of weighting the contribution of individual data. In using the buffer’s output as weights on the generation process we provide such a weighting mechanism. Additionally, as a natural consequence of utilizing the buffer to provide weights on data observed in the environment, working memory capacity constraints are imposed on the amount of data that can contribute to the generation process. As data acquisition was not a focus of the original instantiation of HyGene, capacity limitations in this part of the generation process were not addressed. However, recent data suggest that capacity constraints operating over data acquisition influence hypothesis generation ( Lange et al., 2012 ). Lastly, at a less pragmatic level, this integration provides insight into the working memory dynamics unfolding throughout the data acquisition period thereby providing a window into processing occurring over this previously unmodeled epoch of the hypothesis generation process.
In order to endow HyGene with dynamic data acquisition, each run of the model begins with the context-activation model being sequentially presented with a series of items. In the context of this model these items are the environmental data the model has observed. The activation values for each piece of data at the end of the data acquisition period are then used as the weights on the generation process. A working memory threshold is imposed on the data activations such that data with activations falling below 0.2 are weighted with a zero rather than their actual activation value 3 . Specifically, the global memory match performed between the current D obs and episodic memory in HyGene is weighted by the individual item activations in the dynamic working memory buffer (with the application of the working memory threshold). As each trace in HyGene’s episodic memory is made up of concatenated minivectors, each representing a particular data feature (e.g., fever vs. normal temperature), this weighting is applied in a feature by feature manner in the global matching process. From this point on in the model everything operates in accordance with the original instantiation of HyGene. That is, a subset of the highly activated traces in episodic memory is then used as the basis for the extraction of the unspecified probe . This probe is then matched against semantic memory from which hypotheses are serially retrieved into working memory for further processing.
In order to demonstrate how the integrated dynamic HyGene model responds to variation in the buffer dynamics a simulation was run in which alpha and beta were manipulated at the two levels highlighted above in Figure 3 . In this simulation, the model was sequentially presented with four pieces of data. Only one of these pieces of data was diagnostic whereas the remaining three were completely non-diagnostic. An additional independent variable in this simulation was the serial position in which the diagnostic piece of data was placed. Displayed in Figure 4 is the model’s generation of the most likely hypothesis (i.e., the hypothesis suggested by the diagnostic piece of data) across that data’s serial position plotted by the two levels of alpha (recurrent activation) and beta (global lateral inhibition). What this plot demonstrates, in effect, is how the contribution of each data’s serial position to the model’s generation process is influenced by alpha and beta. As displayed on the left side of the plot, at the lower value of alpha there are clear recency effects. This is due to the buffer dynamics which under these settings predict an “early in – early out” cycling of items through the buffer as shown in Figure 2 . The recency effects emerge as earlier data are less likely to reside in the buffer at the time of generation than later data. It should be noted that these parameters (alpha = 2, beta = 0.2) have been used in previous work accounting for the data from multiple list recall paradigms ( Davelaar et al., 2005 ). By means of preview, we utilize the model’s prediction of recency under these standard parameter settings in guiding our expectations and the implementation of Experiment 1.
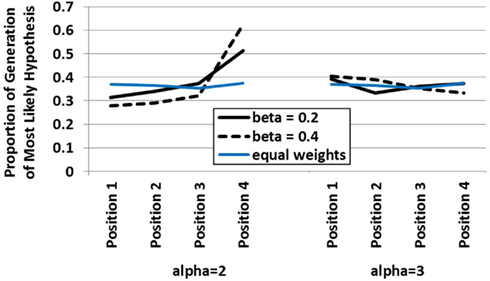
Figure 4. Influence of data serial position on the hypothesis generation behavior of the dynamic HyGene model at two levels of alpha and beta (and the performance of an equal weighted model in blue) . Data plotted represents the proportion of simulation runs on which the most likely hypothesis was generated.
Under the higher value of alpha however, recency does not obtain. In this case, the serial position function flattens substantially as the increased recurrency allows more items to be available to contribute to generation at the end of the sequential data presentation. That is, even when the diagnostic datum appears early, it is maintained long enough in the buffer to be incorporated into the cue to episodic memory. Under the higher value of beta, we see this flattening out transition to a mild primacy gradient. This results from the increased inhibition making it more difficult for the later items to gain enough activation in working memory to contribute to the retrieval process. The greater amount of inhibition essentially renders the later items uncompetitive as they face more competition than they are able, in general, to overcome. Figure 4 additionally plots a line in blue demonstrating the generation level of the static HyGene model in which, rather than utilizing the weights produced by the buffer, each piece of data was weighted equally with a value of one. It can be seen that this line of performance is intermediate under low alpha, but somewhat consistent with the high alpha condition in which more data contribute to the generation process more regularly.
Experiment 1: Data Serial Position
Order effects are pervasive in investigations of memory and decision making ( Murdock, 1962 ; Weiss and Anderson, 1969 ; Hogarth and Einhorn, 1992 ; Page and Norris, 1998 ). Such effects have even been obtained in a hypothesis generation task specifically. Although observed under different conditions than addressed by the present experiment, Sprenger and Dougherty, 2012 , Experiments 1 and 3) found that people sometimes tend to generate hypotheses suggested by more recent cues.
The generalized order effect paradigm was developed by Anderson (1965 , 1973 ) and couched within the algebra of information integration theory to derive weight estimates for individual pieces of information presented in impression formation tasks (e.g., adjectives describing a person). This procedure involved embedding a fixed list of information with a critical piece of information at various serial positions. The differences in the serial position occupied by the piece of critical information thus defined the independent variable, and given that all other information was held constant between conditions, the differences in final judgment were attributable to this difference in serial position. The present experiment represents an adaptation of this paradigm to assess the impact of data serial position on hypothesis generation.
Participants
Seventy-two participants from the University of Oklahoma participated in this experiment for course credit.
Design and procedure
The design of Experiment 1 was a one-way within-subjects design with symptom order as the independent variable. The statistical ecology for this experiment, as defined by the conditional probabilities between the various diseases and symptoms, is shown in Table 1 . Each of the values appearing in this table represents the probability that the symptom will be positive (e.g., fever) given the disease [where the complementary probability represents the probability of the symptom being negative (e.g., normal temperature) given the disease]. The only diagnostic (i.e., informative) symptom is S1 whereas the remaining symptoms, S2–S4, are non-diagnostic (uninformative).
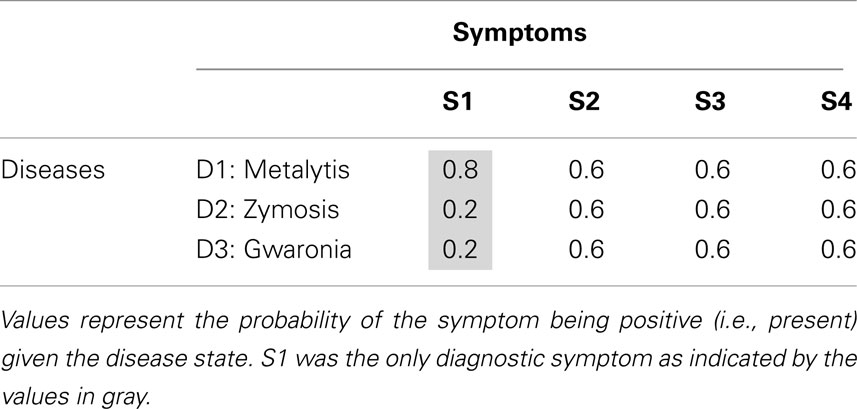
Table 1 . Disease × Symptom ecology of Experiment 1 .
Table 2 displays the four symptom orders. Each of these orders was identical (S2 → S3 → S4) except for the position of S1 within them. All participants received and judged all four symptom orders.
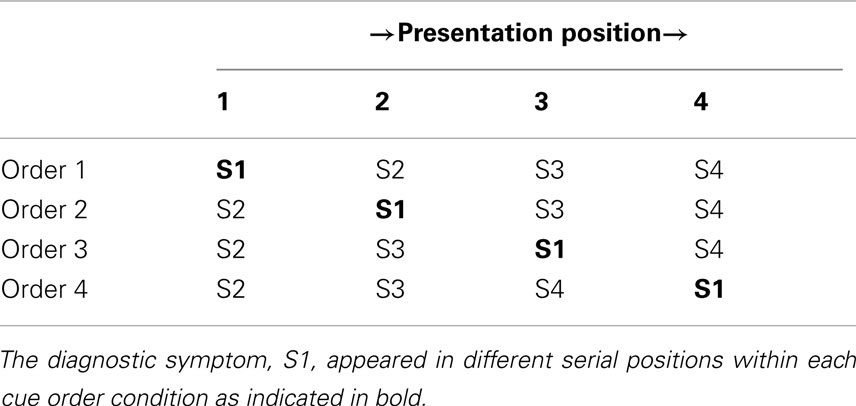
Table 2 . Symptom presentation orders used in Experiment 1 .
There were three main phases to the experiment, an exemplar training phase to learn the contingencies displayed in Table 1 , a learning test to allow discrimination of participants that had learned in the training from those that had not, and an elicitation phase in which the symptom order manipulation was applied in a diagnosis task in which the patient’s symptoms were presented sequentially. The procedure began with the exemplar training phase in which a series of hypothetical pre-diagnosed patients was presented to the participant in order for them to learn, through experience, the contingencies between the diseases and symptoms. Each of these patients was represented by a diagnosis at the top of the screen and a series of test results (i.e., symptoms) pertaining to the columns of S1, S2, S3, and S4 as can be seen in the example displayed by Figure 5 .
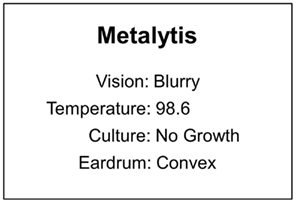
Figure 5. Example exemplar used in Experiment 1 .
Each participant saw 50 exemplars of each disease for a total of 150 exemplars, thus making the base rates of the diseases equal. The specific results of these tests respected the probabilities in Table 1 . The exemplars were drawn in blocks of 10 in which the symptoms would be drawn from the fixed distribution of symptom states given that disease. These symptom states were sampled independently without replacement from exemplar to exemplar. Therefore over the 10 exemplars presented in each individual disease block, the symptoms observed by the participant perfectly represented the distribution of symptoms for that disease. The disease blocks were randomly sampled without replacement which was repeated after the third disease block was presented. Thus, over the course of training the participants were repeatedly presented with the exact probabilities displayed in Table 1 . Each exemplar appeared on the screen for a minimum of 5000 ms at which point they could continue studying the current exemplar or advance to the next exemplar by entering (on the keyboard) the first letter of the current disease exemplar. This optional prolonged studying made the training pseudo-self-paced. Prior to beginning the exemplar training phase, the participants were informed that they had an opportunity to earn a $5.00 gift card to Wal-Mart if they performed well enough in the task.
The diagnosis test phase directly followed exemplar training. This test was included to allow discrimination of participants that learned the contingencies between the symptoms and the diseases in the training phase 4 . The participants were presented with the symptoms of a series of 12 patients (four of each disease) as defined principally by the presence or absence of S1. That is, four of the patients had S1 present (suffering from Metalytis) and the remaining eight had S1 absent (four suffering from Zymosis and four suffering from Gwaronia). The remaining symptoms for the four patients of each disease were the same across the three diseases. On one patient these symptoms were all positive. On the remaining three patients one of these symptoms (S2, S3, S4) was selected without replacement to be absent while the other two were present. Note that as S2, S3, and S4 were completely non-diagnostic as the presence or absence of their symptoms does not influence the likelihood of the disease state. The disease likelihood is completely dependent on the state of S1. The symptoms of each of the patients were presented simultaneously on a single screen. The participants’ task was to correctly diagnose the patients with the disease of greatest posterior probability given their presenting symptoms. No feedback on this test performance was provided. As only S1 was diagnostic, the participants’ scores on this test were tallied based on their correct discrimination of each patient as Metalytis vs. Gwaronia or Zymosis. There were 12 test patients in this diagnosis test. If the participant scored greater than 60% on a diagnosis test they were awarded the gift card at the end of the experiment 5 . Prior to the end of the experiment, the participants were not informed of their performance on the diagnosis test. The participant then completed a series of arithmetic distracters in order to clear working memory of information processed during the diagnosis test phase. The distracter task consisted of a series of 15 arithmetic equations for which the correctness or incorrectness was to be reported (e.g., 15/3 + 2 = 7? Correct or Incorrect?). This distracter task was self-paced.
The elicitation phase then proceeded. First, the diagnosis task was described to the participants as follows: “You will now be presented with additional patients that need to be diagnosed. Each symptom of the patient will be presented one at a time. Following the last symptom you will be asked to diagnose the patient based on their symptoms. Keep in mind that sometimes the symptoms will help you narrow down the list of likely diagnoses to a single disease and other times the symptoms may not help you narrow down the list of likely diagnoses at all. It is up to you to determine if the patient is likely to be suffering from 1 disease, 2 diseases, or all 3 diseases. When you input your response make sure that you respond with the most likely disease first. You will then be asked if you think there is another likely disease. If you think so then you will enter the next most likely disease second. If you do not think there is another likely disease then just hit the Spacebar. You will then have the option to enter a third disease or hit the Spacebar in the same manner. To input the diseases you will use the first letter of the disease, just as you have been during the training and previous test.”
The participant was then presented with the first patient and triggered the onset of the stream of symptoms themselves when they were ready. Each of the four symptoms was presented individually for 1.5 s with a 250 ms interstimulus interval following each symptom. The order in which the symptoms were presented was determined by the order condition as shown in Table 2 . Additionally, all of the patient symptoms presented in this phase positive (i.e., present, as the values in Table 2 represent the likelihood of the symptoms being present given the disease state). The Bayesian posterior probability of D1 was 0.67 whereas the posterior probability of either D2 or D3 was 0.17. Following the presentation of the last symptom the participant responded to two sets of prompts: the diagnosis prompts (as previously described in the instructions to the participants) and a single probability judgment of their highest ranked diagnosis. The probability judgment was elicited with the following prompt: “If you were presented 100 patients with the symptoms of the patient you just observed how many would have [INSERT HIGHEST RANKED DISEASE]?” The participant was then presented with the remaining symptom orders in the same manner with distracter tasks intervening between each trial. The first order received by each participant was randomized between participants and the sequence of the remaining three orders was randomized within participants. Eighteen participants received each symptom order first.
Hypotheses and Predictions
A recency effect was predicted on the grounds that more recent cues would be more active in working memory and contribute to the hypothesis generation process to a greater degree than less recent cues. Given that the activation of the diagnostic symptom (S1) in working memory at the time of generation was predicted to increase in correspondence with its serial position, increases in the generation of Metalytis were predicted to be observed with greater recency of S1. As suggested by Figure 2 , the context-activation model, under parameters based on previous work in list recall paradigms ( Davelaar et al., 2005 ) predicts this generally recency effect as later items are more often more active in memory at the end of list presentation. Correspondingly, decreases in the generation of the alternatives to Metalytis were expected with increases in the serial position of S1. This prediction stems directly from the buffer activation dynamics of the context-activation model.
The main DV for the analyses was the discrete generation vs. non-generation of Metalytis as the most likely disease (i.e., first disease generated). All participants were included in the analyses regardless of performance in the diagnosis test phase and there were no differences in results based on learning. Carry-over effects were evident as demonstrated by a significant interaction between order condition and trial, χ 2 (3) = 12.68, p < 0.016 6 . In light of this, only the data from the first trial for each participant was subjected to further analysis as it was assumed that this was the only uncontaminated trial for each subject. Nominal logistic regression was used to examine the effect of data serial position on the generation of Metalytis (the disease with the greatest posterior probability given the data). A logistic regression contrast test demonstrated a trend for the generation of Metalytis as it was more often generated as the most likely hypothesis with increases in the serial position of the diagnostic data, χ 2 (1) = 4.32, p < 0.05. The number of hypotheses generated between order conditions did not differ, F (3,68) = 0.567, p = 0.64, η p 2 = 0.02 , ranging from an average of 1.67–1.89 hypotheses. There were no differences in the probability judgments of Metalytis as a function of data order when it was generated as the most likely hypothesis (with group means ranging from 56.00 to 67.13), F (3,33) = 0.66, p = 0.58, η p 2 = 0.06 .
Simulating Experiment 1
To simulate Experiment 1, the model’s episodic memory was endowed with the Disease-Symptom contingencies described in Table 1 . On each trial, each symptom was presented to the buffer for 1500 iterations (mapping onto the presentation duration of 1500 ms) and the order of the symptoms was manipulated to match the symptom orders used in the experiment. 1000 iterations of the entire simulation were run for each condition 7 . The primary model output of interest was the first hypothesis generated on each trial. As is demonstrated in Figure 6 , the model is able to capture the qualitative trend in the empirical data quite well. Although the rate of generation is slightly less for the model, the model clearly captures the recency trend as observed in the empirical data. Increased generation of the most likely hypothesis corresponded to the recency of the diagnostic datum. This effect is directly attributable to the buffer activation weights being applied to the generation process. Although Figure 10 will become more pertinent later, the left hand side of this figure demonstrates the recency gradient in the data activation weights produced by the model under these parameter settings. Inspection of the average weights for the first two data acquired show them to be below the working memory threshold of 0.2. Therefore, on a large proportion of trials the model relied on only the third and fourth piece of data (or just the last piece). This explains why the model performs around chance under the first two data orders and only deviates under orders three and four. Additionally, it should be noted that the model could provide a suitable quantitative fit to the empirical data by incorporating an assumption concerning the rate of guessing in the task or potentially by manipulating the working memory threshold. Although the aim of the current paper is to capture the qualitative effects evidenced in the data, future work may seek more precise quantitative fits.
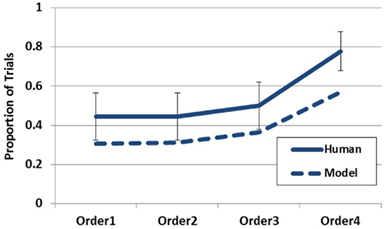
Figure 6. Empirical data (solid line) and model data (dashed line) for Experiment 1 plotting the probability of reporting D1 (Metalytis) as most likely across order conditions . Error bars represent standard errors of the mean.
The primary prediction of the experiment was confirmed. The generation of the most likely hypothesis increased in correspondence with increasing recency of the diagnostic data (i.e., symptom). This finding clearly demonstrates that not all available data contribute equally to the hypothesis generation process (i.e., some data are weighted more heavily than others) and that the serial position of a datum can be an important factor governing the weight allocated to it in the generation process. Furthermore, these results are consistent with the notion that the data weightings utilized in the generation process are governed by the amount of working memory activation possessed by each datum.
There are, however, two alternative explanations for the present finding to consider that do not necessarily implicate unequal weightings of data in working memory as governing generation. First, it could be the case that all data resident in working memory at the time of generation were equally weighted, but that the likelihood of S1 dropping out of working memory increased with its distance in time from the generation prompt. Such a discrete utilization (i.e., all that matters is that data are in or out of working memory regardless of the activation associated with individual data) would likely result in a more gradual recency effect than seen in the data. Future investigations measuring working memory capacity could provide illuminating tests of this account. If generation is sensitive to only the presence or absence of data in working memory (as opposed to graded activations of the data in working memory) it could be expected that participants with higher capacity would be less biased by serial order (as shown in Lange et al., 2012 ) or would demonstrate the bias at a different serial position relative to those with lower capacity.
A second alternative explanation could be that the participants engaged in spontaneous rounds of generation following each piece of data as it was presented. Because the hypothesis generation performance was only assessed after the final piece of data in the present experiment, such “step-by-step” generation would result in stronger generation of Metalytis as the diagnostic data is presented closer to the end of the list. For instance, if spontaneous generation occurs as each piece of data is being presented, then when the diagnostic datum is presented first, there remains three more rounds of generation (based on non-diagnostic data in this case) that could obscure the generation of the initial round. As the diagnostic data moves closer to the end of the data stream the likelihood that that particular round of generation will be obscured by forthcoming rounds diminishes. It is likely that the present data represents a mixture of participants that engaged in such spontaneous generation and those that did not engage in generation until prompted. This is likely the reason for the quantitative discrepancy between the model and empirical data. Future investigations could attempt to determine the likelihood that a participant will engage in such spontaneous generation and the conditions making it more or less likely.
The probability judgments observed in the present experiments did not differ across order conditions. Because the probability judgments were only elicited for the highest ranked hypothesis, the conditions under which the probability judgments were collected were highly constrained. It should be noted that the focus of the present experiment was to address generation behavior and the collection of the judgment data was ancillary. An independent experiment manipulating serial order in the manner done here and designed explicitly for the examination of judgment behavior would be useful for examining the influence of specific data serial positions on probability judgments. This would be interesting as HyGene predicts the judged probability of a hypothesis to be directly influenced by the relative support for the hypotheses currently in working memory. In so far as serial order influences the hypotheses generated into working memory, effects of serial position on probability judgment are likely to be observed as well.
The goal of Experiment 1 was to determine how relative data serial position affects the contribution of individual data to hypothesis generation processes. It was predicted that data presented later in the sequence would be more active in working memory and would thereby contribute more to the generation process based on the dynamics of the context-activation buffer. Such an account predicts a recency profile for the generation of hypotheses from LTM. This effect was obtained and is well-captured by our model in which such differences in the working memory activation possessed by individual data govern the generation process. Despite these positive results, however, the specific processes underlying this data are not uniquely discernible in the present experiment as the aforementioned alternative explanations likely predict similar results. Converging evidence for the notion that data activation plays a governing role in the generation process should be sought.
Experiment 2: Data Maintenance and Data Consistency
When acquiring information from the world that we may use as cues for the generation of hypotheses we acquire these cues in variously sized sets. In some cases we might receive several pieces of environmental data over a brief period, such as when a patient rattles off a list of symptoms to a physician. At other times, however, we receive cues in isolation across time and generate hypotheses based on the first cue and update this set of hypotheses as further data are acquired, such as when an underlying cause of car failure reveals itself over a few weeks. Such circumstances are more complicated as additional processes come into play as further data are received and previously generated hypotheses are evaluated in light of the new data. Hogarth and Einhorn (1992) refer to this task characteristic as the response mode.
In the context of understanding dynamic hypothesis generation this distinction is of interest as it contrasts hypothesis generation following the acquisition of a set of data with a situation in which hypotheses are generated (and updated or discarded) while further data is acquired and additional hypotheses generated. An experiment manipulating this response mode variable in a hypothesis generation task was conducted by Sprenger and Dougherty, 2012 , Experiment 3) in which people hypothesized about which psychology courses were being described by various keywords. The two response modes are step-by-step (SbS), in which a response is elicited following each piece of incoming data, and end-of-sequence (EoS), in which a response is made only after all the data has been acquired as a grouped set. Following the last piece of data, the SbS conditions exhibited clear recency effects whereas EoS conditions, on the other hand, did not demonstrate reliable order effects. A careful reader may notice a discrepancy between the lack of order effects in their EoS condition and the recency effect in the present Experiment 1 (which essentially represents an EoS mode condition). In the Sprenger and Dougherty experiment, the participants received nine cues from which to generate hypotheses as opposed to the four cues in our Experiment 1. As the amount of data in their experiment exceeded working memory capacity (more severely) it is likely that the cue usage strategies utilized by the participants differed between the two experiments. Indeed, it is important to gain a deeper understanding of such cue usage strategies in order to develop a better understanding of dynamic hypothesis generation.
The present experiment compared response modes to examine differences between data maintenance prior to generation (EoS mode) and generation that does not encourage the maintenance of multiple pieces of data (SbS mode). Considered in another light, SbS responding can be thought of as encouraging an anchoring and adjustment process where the set of hypotheses generated in response to the first piece of data supply the set of beliefs in which forthcoming data may be interpreted. The EoS condition, on the other hand, does not engender such belief anchoring as generation is not prompted until all data have been observed. As such, the SbS conditions provide investigation of a potential propensity to discard previously generated hypotheses and/or generate new hypotheses in the face of inconsistent data.
One hundred fifty-seven participants from the University of Oklahoma participated in this experiment for course credit.
As previously mentioned, the first independent variable was the timing of the generation and judgment promptings provided to the participant as dictated by the response mode condition. This factor was manipulated within-subject. The second independent variable, manipulated between-subjects, was the consistency of the second symptom (S2) with the hypotheses likely to be entertained by the participant following the first symptom. This consistency or inconsistency was manipulated within the ecologies learned by the participants as displayed in Table 3 . In addition, this table demonstrates the temporal order in which the symptoms were presented in the elicitation phase of this experiment (i.e., S1 → S2 → S3 → S4). Note that only positive symptom (i.e., symptom present) states were presented in the elicitation phase. The only difference between the ecologies was the conditional probability of S2 being positive under D1. This probability was 0.9 in the “consistent ecology” and 0.1 in the “inconsistent ecology.” Given that S1 should prompt the generation of D1 and D2, this manipulation of the ecology can be realized to govern the consistency of S2 with the hypothesis(es) currently under consideration following S1. This can be seen in Table 4 displaying the Bayesian posterior probabilities for each disease following each symptom. Seventy-nine participants were in the consistent ecology condition and 78 participants were in the inconsistent ecology condition. Response mode was counter-balanced within ecology condition.
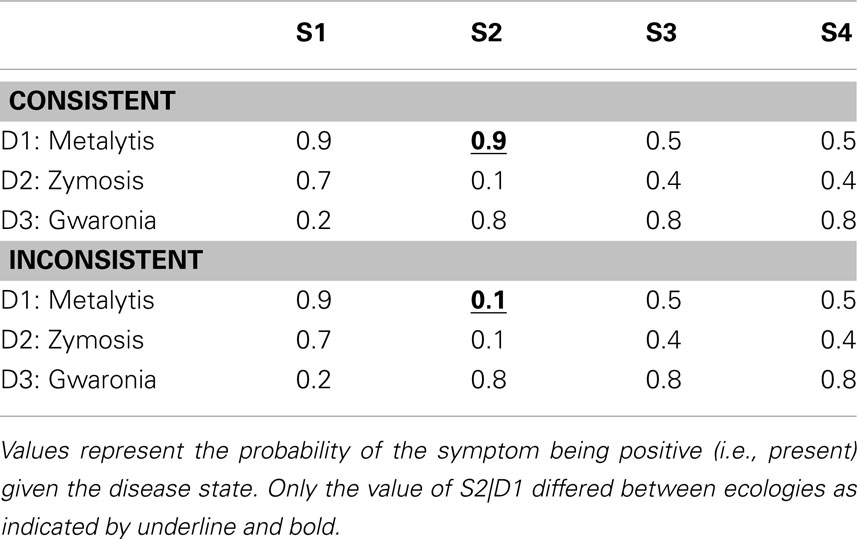
Table 3 . Disease × Symptom ecologies of Experiment 2 .
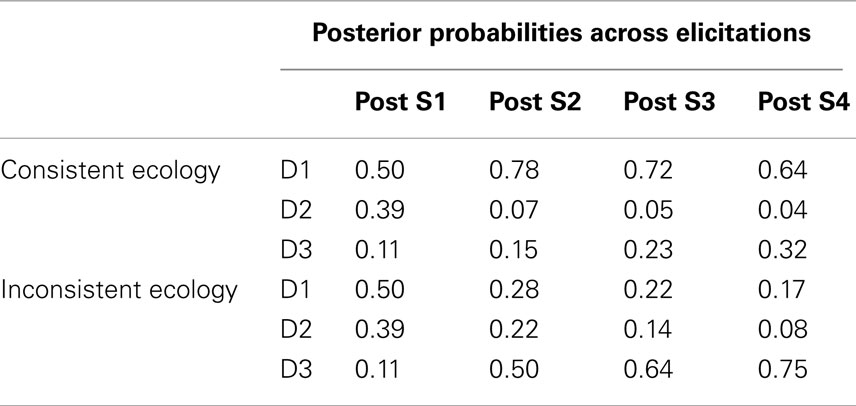
Table 4 . Bayesian posterior probabilities as further symptoms are acquired within each ecology of Experiment 2 .
The procedure was much like that of Experiment 1: exemplar training to learn the probability distributions, a test to verify learning (for which a $5.00 gift card could be earned for performance greater than 60%) 8 , and a distractor task prior to elicitation. The experiment was again cast in terms of medical diagnosis where D1, D2, and D3 represented fictitious disease states and S1–S4 represented various test results (i.e., symptoms).
There were slight differences in each phase of the procedure however. The exemplars presented in the exemplar training phase of were simplified and consisted of the disease name and a single test result (as opposed to all four). This change was made in an effort to enhance learning. Exemplars were blocked by disease such that a disease was selected at random without replacement. For each disease the participant would be presented with 40 exemplars selected at random without replacement. Therefore over the course of these 40 exemplars the entire (and exact) distribution of symptoms would be presented for that disease. This was then done for the remaining two diseases and the entire process was repeated two more times. Therefore the participant observed 120 exemplars per disease (inducing equal base rates for each disease) and observed the entire distribution three times. Each exemplar was again pseudo-self-paced and displayed on the screen for 1500 ms per exemplar prior to the participant being able to proceed to the next exemplar by pressing the first letter of the disease. Patient cases in the diagnosis test phase presented with only individual symptoms as well. Each of the eight possible symptom states were individually presented to the participants and they were asked to report the most likely disease given that particular symptom. Diseases with a posterior probability greater than or equal to 0.39 were tallied as correct responses.
In the elicitation phase, the prompts for hypothesis generation were the same as those used in Experiment 1, but the probability judgment prompt differed slightly. The judgment prompt used in the present experiment was as follows: “How likely is it that the patient has [INSERT HIGHEST RANKED DISEASE]? (Keep in mind that an answer of 0 means that there is NO CHANCE that the patient has [INSERT HIGHEST RANKED DISEASE] and that 100 means that you are ABSOLUTELY CERTAIN that the patient has [INSERT HIGHEST RANKED DISEASE].) Type in your answer from 1 to 100 and press Enter to continue.” Probability judgments were taken following each generation sequence in the SbS condition (i.e., there were four probability judgments taken, one for the disease ranked highest on each round of generation).
Hypotheses and predictions
The general prediction for the end-of-sequence response mode was that recency would be demonstrated in both ecologies as the more recent symptoms should contribute more strongly to the generation process as seen in Experiment 1. Therefore, greater generation of D3 relative to the alternatives was expected in both ecologies. The focal predictions for the SbS conditions concerned the generation behavior following S2. It was predicted that participants in the consistent ecology would generate D1 to a greater extent than those in the inconsistent ecology who were expected to purge D1 from their hypothesis set in response to its inconsistency with S2. It was additionally predicted that those in the inconsistent ecology would generate D3 to a greater extent at this point than those in the consistent ecology as they would utilize S2 to repopulate working memory with a viable hypothesis.
As no interactions with trial order were detected, both trials from each subject were used in the present analyses and no differences in results were found with differences in learning. The main dependent variable analyzed for this experiment was the hypothesis generated as most likely on each round of elicitation. All participants were included in the analyses regardless of performance in the diagnosis test phase. In order to test if a recency effect obtained following the last symptom (S4), comparisons between the rates of generation of each disease were carried out within each of the four ecology-by-response mode conditions. Within the step-by-step conditions the three diseases were generated at different rates in the consistent ecology according to Cochran’s Q Test, χ 2 (2) = 9.14, p < 0.05, but not in the inconsistent ecology χ 2 (2) = 1, p = 0.61. In the end-of-sequence conditions, significant differences in generation rates were revealed in both the consistent ecology, χ 2 (2) = 17.04, p < 0.001, and the inconsistent ecology, χ 2 (2) = 7.69, p < 0.05.
As D2 was very unlikely in both ecologies the comparison of interest in all cases is between D1 and D3. This pairwise comparison was carried out within each of the ecology-by-response mode conditions and reached significance only in the EoS mode in the consistent ecology, χ 2 (1) = 6.79, p < 0.01, as D1 was generated to a greater degree than D3 according to Cochran’s Q Test. These results, displayed in Figure 7 , demonstrate the absence of a recency effect in the present experiment. This difference between the EoS and SbS ecology is additionally observed by comparing rates of D1 generation across the entire design demonstrating a main effect of ecology, χ 2 (1) = 8.87, p < 0.01, but no effect of mode, χ 2 (1) = 0.987, p = 0.32, and no interaction, χ 2 (1) = 0.554, p = 0.457.
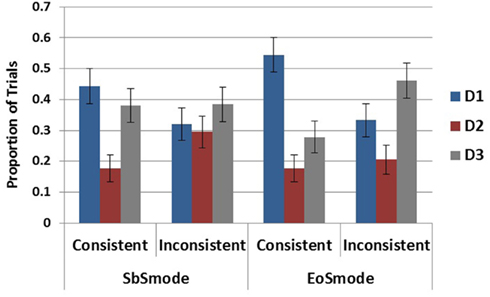
Figure 7. Proportion of generation for each disease by response mode and ecology conditions . Error bars represent standard errors of the mean.
To test the influence of the inconsistent cue on the maintenance of D1 (the most likely disease in both ecologies following S1) in the SbS conditions, elicitation round (post S1 and post S2) was entered as an independent variable with ecology and tested in a 2 × 2 logistic regression. As plotted in Figure 8 , this revealed a main effect of elicitation round, χ 2 (1) = 10.51, p < 0.01, an effect of ecology, χ 2 (1) = 6.65, p < 0.05, and a marginal interaction, χ 2 (1) = 3.785, p = 0.052. When broken down by ecology it is evident that the effect of round and the marginal interaction were due to the decreased generation of D1 following S2 in the inconsistent ecology, χ 2 (1) = 10.51, p < 0.01, as there was no difference between rounds in the consistent ecology, χ 2 (1) = 0.41, p = 0.524.
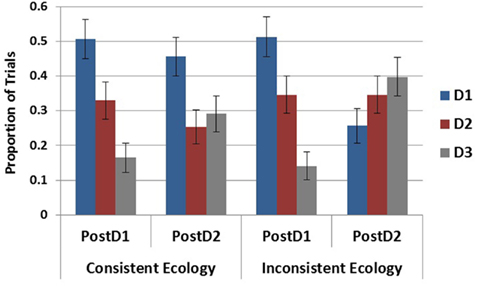
Figure 8. Proportion of generation for each disease within the SbS condition following S1 and S2 . Error bars represent standard errors of the mean.
This same analysis was done with D3 to examine potential differences in its rate of generation over these two rounds of generation. This test revealed a main effect of elicitation round, χ 2 (1) = 12.135, p < 0.001, but no effect of ecology, χ 2 (1) = 1.953, p = 0.162, and no interaction, χ 2 (1) = 1.375, p = 0.241.
Simulating Experiment 2
To model the EoS conditions, the model was presented all four symptoms in sequence and run in conditions in which the model was endowed with either the consistent or inconsistent ecology. This simulation was run for 1000 iterations in each condition. As is intuitive from the computational results of Experiment 1, when the model is run with the same parameters utilized in the previous simulation it predicts greater generation for D3 in both ecologies (i.e., recency) which was not observed in the present experiment. However, the model is able to capture the data of the EoS mode quite well by increasing the amount of recurrent activation that each piece of data recycles onto itself (alpha parameter) and the amount of lateral inhibition applied to each piece of data (beta parameter) as it is acquired prior to generation. These results appear alongside the empirical results in Figure 9 . Although the model is able the capture the qualitative pattern in the data in the inconsistent ecology reasonably well with either set of parameters, the model produces divergent results under the two alpha and beta levels in the consistent ecology. Only when recurrency and inhibition are increased does the model capture the data from both ecologies.
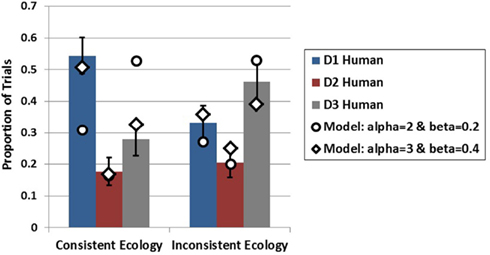
Figure 9. Empirical data (bars) from Experiment 2 for the EoS conditions in both ecologies plotted with model data (diamonds and circles) at two levels of alpha and beta . Error bars represent standard errors of the mean.
Examination of how the data activations are influenced by the increased alpha and beta levels reveals the underlying cause for this difference in generation. As displayed in Figure 10 , there is a steep recency gradient for the data activations under alpha = 2 and beta = 0.2 (parameters from Experiment 1), but there is a markedly different pattern of activations under alpha = 3 and beta = 0.4 9 . Most notably, these higher alpha and beta levels cause the earlier pieces of data to reach high levels of activation which then suppress the activation levels of later data. This is due to the competitive dynamics of the buffer which restrict rise of activation for later items under high alpha and beta values resulting in a primacy gradient in the activation values as opposed to the recency gradient observed under the lower values.
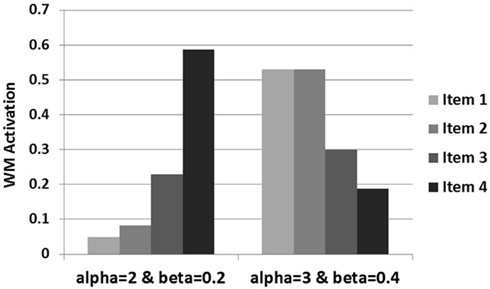
Figure 10. Individual data activations under both levels of alpha and beta .
To capture the SbS conditions for generation following S1 and generation following S2, the model was presented with different amounts of data on different trials. Specifically, the model was presented with S1 only, capturing the situation in which only the first piece of data had been received, or the model was presented with S1 and S2 successively in order to capture the SbS condition following the second piece of data. This was done for both ecologies in order to assess the effects of data inconsistency on the model’s generation behavior 10 . As can be seen in Figure 11 the model is able to capture the empirical data quite well following S1 while providing a decent, although imperfect, account of the post S2 data as well 11 . Focally, the model as implemented captures the influences of S2 on the hypothesis sets generated in response to S1. Following S2 in the inconsistent ecology D1 decreases substantially capturing its purging from working memory. Additionally, the increases in the generation of D3 are present in both ecologies.
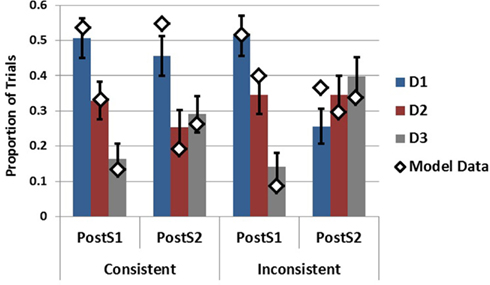
Figure 11. Empirical data (bars) from Experiment 2 in the SbS conditions following S1 and S2 plotted with model data (diamonds) . Error bars represent standard errors of the mean.
The present experiment has provided a window into two distinct processing dynamics. The first dynamic under investigation was how generation differs when based on the acquisition of a set of data (EoS condition) vs. when each piece of data is acquired in isolation (SbS condition). The generation behavior between these conditions was somewhat similar overall, as neither D1 nor D3 dominated generation in three of the four conditions. The EoS consistent ecology condition, however, was clearly dominated by D1. This result obtained in contrast to the prediction of recency in the EoS conditions, which would have been evidenced by higher rankings for D3 (for both ecologies).
The divergence between the recency effect in Experiment 1 and the absence of recency effect in the EoS conditions of Experiment 2 is surprising. In order for the model to account for the amelioration of the recency effect an adjustment was made to the alpha and beta parameters governing how much activation each piece of data is able to recycle onto itself and the level of competition thereby eliminating the recency gradient in the activations. Moreover, the last piece of data did not contribute as often or as strongly to the cue to LTM under these settings. Therefore, rather than a recency effect, the model suggests a primacy effect whereby the earlier cues contributed more to generation than the later cues. As we have not manipulated serial order in the present experiment, it is difficult to assert a primacy effect based on the empirical data alone. The model’s account of the current data, however, certainly suggests that a primacy gradient is needed to capture the results. Additionally, a recent experiment in a similar paradigm utilizing an EoS response mode demonstrated a primacy effect in a diagnostic reasoning task ( Rebitschek et al., 2012 ) suggesting that primacy may be somewhat prevalent under EoS data acquisition situations.
As for why the earlier cues may have enjoyed greater activation in the present experiment relative to Experiment 1 we need to consider the main difference between these paradigms. The largest difference was that in the present experiment each piece of data present in the ecology carried a good amount of informational value whereas in Experiment 1 80% of the data in the ecology was entirely non-diagnostic. It is possible that this information rich vs. information scarce ecological difference unintentionally led to a change in how the participants allocated their attention over the course of the data streams between the two experiments. As all of the data in Experiment 2 was somewhat useful, the participants may have used this as a cue to utilize as much of the information as possible thereby rehearsing/reactivating the data as much as possible prior to generation. In contrast, being in the information scarce ecology of Experiment 1 would not have incentivized such maximization of the data activations for most of the data. Future experiments could address how the complexity of the ecology might influence dynamic attentional allocation during data acquisition.
The second dynamic explored was how inconsistent data influences the hypotheses currently under consideration. In the step-by-step conditions it was observed that a previously generated hypothesis was purged from working memory in response to the inconsistency of a newly received cue. This can be viewed as consistent with an extension of the consistency checking mechanism employed in the original HyGene framework. The present data suggests that hypotheses currently under consideration are checked against newly acquired data and are purged in accordance with their degree of (in)consistency. This is different from, although entirely compatible with, the operation of the original consistency checking mechanism operating over a single round of hypothesis generation. The consistency checking operation within the original version of HyGene checks each hypothesis retrieved into working memory for its consistency with the data used as a cue to its retrieval as the SOCs is populated. The consistency checking mechanism exposed in the present experiment, however, suggests that people check the consistency of newly acquired data against hypotheses generated from previous rounds of generation as well. If the previously generated hypotheses fall below some threshold of agreement with the newly acquired data they are purged from working memory. Recent work by Mehlhorn et al. (2011) also investigated the influence of consistent and inconsistent cues on the memory activation of hypotheses. They utilized a clever adaptation of the lexical decision task to assess the automatic memory activation of hypotheses as data were presented and found memory activation sensitivity to the consistency of the data. As the present experiment utilized overt report, these findings complement one another quite well as automatic memory activation can be understood as a precursor to the generation of hypotheses into working memory. The present experiment additionally revealed that S2 was used to re-cue LTM as evidenced by increased generation of D3 following S2. In contrast to the prediction that this would occur only in the inconsistent ecology, this recuing was observed in both ecologies. Lastly, although the model as currently implemented represents a simplification of the participant’s task in the SbS conditions, it was able to capture these effects.
General Discussion
This paper presented a model of dynamic data acquisition and hypothesis generation which was then used to account for data from two experiments investigating three consequences of hypothesis generation being extended over time. Experiment 1 varied the serial position of a diagnostic datum and demonstrated a recency effect whereby the hypothesis implied by this datum was generated more often when the datum appeared later in the data stream. Experiment 2 examined how generation might differ when it is based on isolated data acquired one at a time (step-by-step response mode) vs. when generation is based upon the acquisition of the entire set of data (end-of-sequence response mode). Secondly, the influence of an inconsistent cue (conflicting with hypotheses suggested by the first datum) was investigated by manipulating a single contingency of the data-hypothesis ecology in which the participants were trained. It was found that the different response modes did not influence hypothesis generation a great deal as the two most likely hypotheses were generated at roughly the same rates in most cases. The difference that was observed however was that the most likely hypothesis was favored in the EoS condition within the consistent ecology. This occurred in contrast to the prediction of recency for both EoS conditions, thereby suggesting that the participants weighted the data more equally than in Experiment 1 or perhaps may have weighted the earlier cues slightly more heavily. Data from the SbS conditions following the acquisition of the inconsistent cue revealed that this cue caused participants to purge a previously generated hypothesis from working memory that was incompatible with the newly acquired data. Moreover, this newly acquired data was utilized to re-cue LTM. Interestingly, this re-cueing was demonstrated in both ecologies and was therefore not contingent on the purging of hypotheses from working memory.
Given that the EoS conditions of Experiment 2 were procedurally very similar to the procedure used in Experiment 1 it becomes important to reconcile their contrasting results. As discussed above, the main factor distinguishing these conditions was the statistical ecology defining their respective data-hypothesis contingencies. The ecology of the first experiment contained mostly non-diagnostic data whereas each datum in the ecology utilized in Experiment 2 carried information as to the relative likelihood of each hypothesis. It is possible that this difference of relative information scarcity and information richness influenced the processing of the data streams between the two experiments. In order to capture the data from Experiment 2 with our model, the level of recurrent activation recycled by each piece of data was adjusted upwards and lateral inhibition increased thereby giving the early items a large processing advantage over the later pieces of data. Although post hoc , this suggests the presence of a primacy bias. It is then perhaps of additional interest to note that the EoS results resemble the SbS results following D2 and this is particularly so within the consistent ecology. This could be taken to suggest that those in the EoS condition were utilizing the initial cues more greatly than the later cues. Fisher (1987) suggested that people tend to use a subset of the pool of provided data and estimated that people generally use two cues when three are available and three cues when four are available. Interestingly the model forwarded in the present paper provides support for this estimate as it used three of the four available cues in accounting for the EoS data in Experiment 2. While the utilization of three as opposed to four data could be understood as resulting from working memory constraints, the determinants of why people would fail to utilize three pieces of data when only three data are available is less clear. Future investigation of the conditions under which people underutilize available data in three and four data-hypothesis generation problems could be illuminating for the working memory dynamics of these tasks.
It is also important to compare the primacy effect in the EoS conditions with the results of Sprenger and Dougherty (2012) in which the SbS conditions revealed recency (Experiments 1 and 3) and no order effects were revealed in the EoS conditions (only implemented in Experiment 3). As for why the SbS results of the present experiment do not demonstrate recency as in their Experiments 1 and 3 is unclear. The ecologies used in these experiments were quite different, however, and it could be the case that the ecology implemented in their experiment was better able to capture this effect. Moreover, they explicitly manipulated data serial order and it was through this manipulation that the recency effect was observed. As serial order was not manipulated in the present experiment we did not have the opportunity to observe recency in the same fashion and instead relied on relative rates of generation given one data ordering. Perhaps the manipulation of serial order within the present ecology would uncover recency as well.
In comparing the present experiment to the procedure of Sprenger and Dougherty’s Experiment 3 a clearer reason for diverging results is available. In their experiment, the participants were presented with a greater pool of data from which to generate hypotheses, nine pieces in total. Participants in the present experiment, on the other hand, were only provided with four cues. It is quite possible that people’s strategies for cue usage would differ between these conditions. Whereas the present experiment provided enough data to fill working memory to capacity (or barely breach it), Sprenger and Dougherty’s experiment provided an abundance of data thereby providing insight into a situation in which the data could not be held in working memory at once. It is possible that the larger pool of data engendered a larger pool of strategies to be employed than in the present study. Understanding the strategies that people employ and the retrieval plans developed under such conditions ( Raaijmakers and Shiffrin, 1981 ; Gillund and Shiffrin, 1984 ; Fisher, 1987 ) as well as how these processes contrast with situations in which fewer cues are available is a crucial aspect of dynamic memory retrieval in need of better understanding.
The model presented in the present work represents a fusion of the HyGene model ( Thomas et al., 2008 ) with the activation dynamics of the context-activation model of memory ( Davelaar et al., 2005 ). As the context-activation model provides insight into the working memory dynamics underlying list memory tasks, it provides a suitable guidepost for understanding some of the likely working memory dynamics supporting data acquisition and hypothesis generation over time. The present model acquires data over time whose activations systematically ebb and flow in concert with the competitive buffer dynamics borrowed from the context-activation model. The resulting activation levels possessed by each piece of data are then used as weights in the retrieval of hypotheses from LTM. In addition to providing an account of the data from the present experiments this model has demonstrated further usefulness by suggesting potentially fruitful areas of future investigation.
The modeling presented here represents the first step of a work in progress. As we are working toward a fully dynamical model of data acquisition, hypothesis generation, maintenance, and use in decision making tasks, additional facets clearly still await inclusion. Within the current implementation of the model it is only the environmental data that are subject to the working memory activation dynamics of the working memory buffer. In future work, hypotheses generated into working memory (HyGene’s SOCs) will additionally be sensitive to these dynamics. This will provide us with the means of fully capturing hypothesis maintenance dynamics (e.g., step-by-step generation) that the present model ignores. Moreover, by honoring such dynamic maintenance processes we may be able to address considerations of what information people utilize at different portions of a hypothesis generation task. For instance, when data is acquired over long lags (e.g., minutes), it is unclear what information people use to populate working memory with hypotheses at different points in the task. If someone is reminded of the diagnostic problem they are trying to solve, do they recall the hypotheses directly (e.g., via contextual retrieval) or do they sometimes recall previous data to be combined with new data and re-generate the current set of hypotheses? Presumably both strategies are prevalent, but the conditions under which they are more or less likely to manifest is unclear. It is hoped that this more fully specified model may provide insight into situations favoring one over the other.
As pointed out by Sprenger and Dougherty (2012) a fuller understanding of hypothesis generation dynamics will entail learning about how working memory resources are dynamically allocated between data and hypotheses over time. One-way that this could be achieved in the forthcoming model would be to have two sets of information available for use at any given time, one of which would be the set of relevant data (RED) and the other would be the SOC hypotheses. The competitive dynamics of the buffer could be brought to bear between these sets of items by allowing them to inhibit one another, thereby instantiating competition between the items in these sets for the same limited resource. Setting up the model in this or similar manners would be informative for addressing dynamic working memory tradeoffs that are struck between data and hypotheses over time.
In addition, this more fully elaborated model could inform maintenance dynamics as hypotheses are utilized to render judgments and decisions. The output of the judgment and decision processes could cohabitate the working memory buffer and its maintenance and potential influence on other items’ activations could be gauged across time. Lastly, as the model progresses in future work it will be important and informative to examine the model’s behavior more broadly. For the present paper we have focused on the first hypothesis generated in each round of generation. The generation behavior of people and the model of course furnishes more than one hypothesis into working memory. Further work with this model has the potential to provide a richer window into hypothesis generation behavior by taking a greater focus on the full hypothesis sets considered over time.
Developing an understanding of the temporal dynamics governing the rise and fall of beliefs over time is a complicated problem in need of further investigation and theoretical development. This paper has presented an initial model of how data acquisition dynamics influence the generation of hypotheses from LTM and two experiments considering three distinct processing dynamics. It was found that the recency of the data, sometimes but not always, biases the generation of hypotheses. Additionally, it was found that previously generated hypotheses are purged from working memory in light of new data with which they are inconsistent. Future work will develop a more fully specified model of dynamic hypothesis generation, maintenance, and use in decision making tasks.
Conflict of Interest Statement
The authors declare that the research was conducted in the absence of any commercial or financial relationships that could be construed as a potential conflict of interest.
- ^ For a more thorough treatment of HyGene’s computational architecture please see Thomas et al. (2008) or Dougherty et al. (2010) .
- ^ This was done from the pragmatic view that the buffer cannot apply noise to an item representation that does not yet exist in the environment or in the system. A full and systematic analysis of how this assumption affects the behavior of the buffer has not been carried out as of yet, but in the context of the current simulations preliminary analysis suggests that this change affects the activation values produced by the buffer only slightly.
- ^ This working memory threshold has been carried over from the context-activation model as it proved valuable for that model’s account of data from a host of list recall paradigms ( Davelaar et al., 2005 ).
- ^ Previous investigations in our lab utilizing exemplar training tasks have demonstrated variation in conclusions drawn from results conditionalized on such learning data against entire non-conditionalized data set. Therefore including this learning test allows us a check on the presence of such discrepancies in addition to obtaining data that may inform how greater or lesser learning influences the generation process.
- ^ Thirty-five participants (48%) exceeded this 60% criterion.
- ^ This carry-over effect was not entirely surprising as the same symptom states were presented for every patient and our manipulation of serial order was likely transparent on later trials.
- ^ The parameters used for this simulation were the following. Original HyGene parameters: L = 0.85, Ac = 0.1, Phi = 4, KMAX = 8. Context-activation model parameters: Alpha = 2.0, Beta = 0.2, Lambda = 0.98, Delta = 1. Note, these parameters were based on values utilized in previous work and were not chosen based on fitting the model to the current data.
- ^ Eighty-eight participants (56%) exceeded this 60% criterion.
- ^ These parameter values were based on a grid search to examine the neighborhood of values capturing the qualitative patterns in the data and not based on a quantitative fit to the empirical data.
- ^ This is, of course, a simplification of the participant’s task in the SbS condition. This is addressed in the general discussion.
- ^ This simulation was run with alpha = 3 and beta = 0.4.
Anderson, N. H. (1965). Primacy effects in personality impression formation using a generalized order effect paradigm. J. Pers. Soc. Psychol. 2, 1–9.
CrossRef Full Text
Anderson, N. H. (1973). Serial position curves in impression formation. J. Exp. Psychol. 97, 8–12.
Cooper, R. P., Yule, P., and Fox, J. (2003). Cue selection and category learning: a systematic comparison of three theories. Cogn. Sci. Q. 3, 143–182.
Davelaar, E. J., Goshen-Gottstein, Y., Ashkenazi, A., Haarmann, H. J., and Usher, M. (2005). The demise of short term memory revisited: empirical and computational investigations of recency effects. Psychol. Rev. 112, 3–42.
Pubmed Abstract | Pubmed Full Text | CrossRef Full Text
Dougherty, M. R. P., Gettys, C. F., and Ogden, E. E. (1999). A memory processes model for judgments of likelihood. Psychol. Rev. 106, 180–209.
Dougherty, M. R. P., and Hunter, J. E. (2003a). Probability judgment and subadditivity: the role of WMC and constraining retrieval. Mem. Cognit. 31, 968–982.
Dougherty, M. R. P., and Hunter, J. E. (2003b). Hypothesis generation, probability judgment, and working memory capacity. Acta Psychol . (Amst.) 113, 263–282.
Dougherty, M. R. P., Thomas, R. P., and Lange, N. (2010). Toward an integrative theory of hypothesis generation, probability judgment, and hypothesis testing. Psychol. Learn. Motiv. 52, 299–342.
Fisher, S. D. (1987). Cue selection in hypothesis generation: Reading habits, consistency checking, and diagnostic scanning. Organ. Behav. Hum. Decis. Process. 40, 170–192.
Gillund, G., and Shiffrin, R. M. (1984). A retrieval model for both recognition and recall. Psychol. Rev. 91, 1–67.
Hintzman, D. L. (1986). “Schema Abstraction” in a multiple-trace memory model. Psychol. Rev. 93, 411–428.
Hintzman, D. L. (1988). Judgments of frequency and recognition memory in a multiple-trace memory model. Psychol. Rev. 95, 528–551.
Hogarth, R. M., and Einhorn, H. J. (1992). Order effects in belief updating: the belief-adjustment model. Cogn. Psychol. 24, 1–55.
Lange, N. D., Thomas, R. P., and Davelaar, E. J. (2012). “Data acquisition dynamics and hypothesis generation,” in Proceedings of the 11th International Conference on Cognitive Modelling , eds N. Rußwinkel, U. Drewitz, J. Dzaack, H. van Rijn, and F. Ritter (Berlin: Universitaetsverlag der TU), 31–36.
McKenzie, C. R. M. (1998). Taking into account the strength of an alternative hypothesis. J. Exp. Psychol. Learn. Mem. Cogn. 24, 771–792.
Mehlhorn, K., Taatgen, N. A., Lebiere, C., and Krems, J. F. (2011). Memory activation and the availability of explanations in sequential diagnostic reasoning. J. Exp. Psychol. Learn. Mem. Cogn. 37, 1391–1411.
Murdock, B. B. (1962). The serial position effect of free recall. J. Exp. Psychol. 64, 482–488.
Nelson, J. D., McKenzie, C. R. M., Cottrell, G. W., and Sejnowski, T. J. (2010). Experience matters: information acquisition optimizes probability gain. Psychol. Sci. 21, 960–969.
Page, M. P. A., and Norris, D. (1998). The primacy model: a new model of immediate serial recall. Psychol. Rev. 105, 761–781.
Raaijmakers, J. G. W., and Shiffrin, R. M. (1981). Search of associative memory. Psychol. Rev. 88, 93–134.
Rebitschek, F., Scholz, A., Bocklisch, F., Krems, J. F., and Jahn, G. (2012). “Order effects in diagnostic reasoning with four candidate hypotheses,” in Proceedings of the 34th Annual Conference of the Cognitive Science Society , eds N. Miyake, D. Peebles, and R. P. Cooper (Austin, TX: Cognitive Science Society) (in press).
Sprenger, A., and Dougherty, M. P. (2012). Generating and evaluating options for decision making: the impact of sequentially presented evidence. J. Exp. Psychol. Learn. Mem. Cogn. 38, 550–575.
Sprenger, A., and Dougherty, M. R. P. (2006). Differences between probability and frequency judgments: the role of individual differences in working memory capacity. Organ. Behav. Hum. Decis. Process. 99, 202–211.
Sprenger, A. M., Dougherty, M. R., Atkins, S. M., Franco-Watkins, A. M., Thomas, R. P., Lange, N. D., and Abbs, B. (2011). Implications of cognitive load for hypothesis generation and probability judgment. Front. Psychol. 2:129. doi:10.3389/fpsyg.2011.00129
Thomas, R. P., Dougherty, M. R., Sprenger, A. M., and Harbison, J. I. (2008). Diagnostic hypothesis generation and human judgment. Psychol. Rev. 115, 155–185.
Usher, M., Davelaar, E. J., Haarmann, H., and Goshen-Gottstein, Y. (2008). Short term memory after all: comment on Sederberg, Howard, and Kahana (2008). Psychol. Rev. 115, 1108–1118.
Weiss, D. J., and Anderson, N. H. (1969). Subjective averaging of length with serial position. J. Exp. Psychol. 82, 52–63.
Keywords: hypothesis generation, temporal dynamics, working memory, information acquisition, decision making
Citation: Lange ND, Thomas RP and Davelaar EJ (2012) Temporal dynamics of hypothesis generation: the influences of data serial order, data consistency, and elicitation timing. Front. Psychology 3 :215. doi: 10.3389/fpsyg.2012.00215
Received: 24 January 2012; Accepted: 09 June 2012; Published online: 29 June 2012.
Reviewed by:
Copyright: © 2012 Lange, Thomas and Davelaar. This is an open-access article distributed under the terms of the Creative Commons Attribution Non Commercial License , which permits non-commercial use, distribution, and reproduction in other forums, provided the original authors and source are credited.
*Correspondence: Nicholas D. Lange, Department of Psychological Sciences, Birkbeck College, University of London, Malet Street, London WC1E 7HX, UK. e-mail: ndlange@gmail.com
Disclaimer: All claims expressed in this article are solely those of the authors and do not necessarily represent those of their affiliated organizations, or those of the publisher, the editors and the reviewers. Any product that may be evaluated in this article or claim that may be made by its manufacturer is not guaranteed or endorsed by the publisher.
Automating Psychological Hypothesis Generation with AI: Large Language Models Meet Causal Graph
Leveraging the synergy between causal knowledge graphs and a large language model (LLM), our study introduces a groundbreaking approach for computational hypothesis generation in psychology. We analyzed 43,312 psychology articles using a LLM to extract causal relation pairs. This analysis produced a specialized causal graph for psychology. Applying link prediction algorithms, we generated 130 potential psychological hypotheses focusing on ‘well-being’, then compared them against research ideas conceived by doctoral scholars and those produced solely by the LLM. Interestingly, our combined approach of a LLM and causal graphs mirrored the expert-level insights in terms of novelty, clearly surpassing the LLM-only hypotheses ( t (59) = 3.34, p =0.007 and t (59) = 4.32, p <0.001, respectively). This alignment was further corroborated using deep semantic analysis. Our results show that combining LLM with machine learning techniques such as causal knowledge graphs can revolutionize automated discovery in psychology, extracting novel insights from the extensive literature. This work stands at the crossroads of psychology and artificial intelligence, championing a new enriched paradigm for data-driven hypothesis generation in psychological research.
Keywords : Hypothesis Generation, Causal Reasoning, Large Language Model, Psychological Science, Scientific Discovery
1 Introduction
In an age in which the confluence of artificial intelligence (AI) with various subjects profoundly shapes sectors ranging from academic research to commercial enterprises, dissecting the interplay of these disciplines becomes paramount [ williams2023investigating ] . In particular, psychology, which serves as a nexus between the humanities and natural sciences, consistently endeavors to demystify the complex web of human behaviors and cognition [ hergenhahn2013introduction ] . Its profound insights have significantly enriched academia, inspiring innovative applications in AI design. For example, AI models have been molded on hierarchical brain structures [ cichy2016comparison ] and human attention systems [ vaswani2017attention ] . Additionally , these AI models reciprocally offer a rejuvenated perspective, deepening our understanding from the foundational cognitive taxonomy to nuanced aesthetic perceptions [ battleday2020capturing , tong2021putative ] . Nevertheless , the multifaceted domain of psychology, particularly social psychology, has exhibited a measured evolution compared to its tech-centric counterparts. This can be attributed to its enduring reliance on conventional theory-driven methodologies [ henrich2010most , shah2015big ] , a characteristic that stands in stark contrast to the burgeoning paradigms of AI and data-centric research [ wang2023scientific , bechmann2019unsupervised ] .
In the journey of psychological research, each exploration originates from a spark of innovative thought. These research trajectories may arise from established theoretical frameworks, daily event insights, anomalies within data, or intersections of interdisciplinary discoveries [ jaccard2019theory ] . Hypothesis generation is pivotal in psychology [ koehler1994hypothesis , mcguire1973yin ] , as it facilitates the exploration of multifaceted influencers of human attitudes, actions, and beliefs. The HyGene model [ thomas2008diagnostic ] elucidated the intricacies of hypothesis generation, encompassing the constraints of working memory and the interplay between ambient and semantic memories. Recently, causal graphs have provided psychology with a systematic framework that enables researchers to construct and simulate intricate systems for a holistic view of “bio-psycho-social” interactions [ borsboom2021network , crielaard2022refining ] . Yet , the labor-intensive nature of the methodology poses challenges, which requires multidisciplinary expertise in algorithmic development, exacerbating the complexities [ crielaard2022refining ] . Meanwhile, advancements in AI, exemplified by models such as the generative pretrained transformer (GPT), present new avenues for creativity and hypothesis generation [ wang2023scientific ] .
Building on this , notably large language models (LLMs) such as GPT-3, GPT-4, and Claude-2, which demonstrate profound capabilities to comprehend and infer causality from natural language texts, a promising path has emerged to extract causal knowledge from vast textual data [ gu2023conceptgraphs , binz2023using ] . Exciting possibilities are seen specific scenarios in which LLMs and causal graphs manifest complementary strengths [ pan2023unifying ] . Their synergistic combination converges human analytical and systemic thinking, echoing the holistic versus analytic cognition delineated in social psychology [ nisbett2001culture ] . This amalgamation enables fine-grained semantic analysis and conceptual understanding via LLMs, while causal graphs offer a global perspective on causality, alleviating the interpretability challenges of AI [ pan2023unifying ] . This integrated methodology efficiently counters the inherent limitations of working and semantic memories in hypothesis generation and, as previous academic endeavors indicate, has proven efficacious across disciplines. For example, a groundbreaking study in physics synthesized 750,000 physics publications, utilizing cutting-edge natural language processing to extract 6,368 pivotal quantum physics concepts, culminating in a semantic network forecasting research trajectories [ krenn2020predicting ] . Additionally, by integrating knowledge-based causal graphs into the foundation of the LLM, the LLM’s capability for causative inference significantly improves [ kiciman2023causal ] .
To this end , our study seeks to build a pioneering analytical framework, combining the semantic and conceptual extraction proficiency of LLMs with the systemic thinking of the causal graph, with the aim of crafting a comprehensive causal network of semantic concepts within psychology. We meticulously analyzed 43,312 psychological articles, devising an automated method to construct a causal graph, and systematically mining causative concepts and their interconnections. Specifically , the initial sifting and preparation of the data ensures a high-quality corpus, and is followed by employing advanced extraction techniques to identify standardized causal concepts. This results in a graph database that serves as a reservoir of causal knowledge. In conclusion, using node embedding and similarity-based link prediction, we unearthed potential causal relationships, and thus generated the corresponding hypotheses.
To gauge the pragmatic value of our network, we selected 130 hypotheses on ‘well-being’ generated by our framework, comparing them with hypotheses crafted by novice experts (doctoral students in psychology) and the LLM models . The results are encouraging: Our algorithm matches the caliber of novice experts, outshining the hypotheses generated solely by the LLM models in novelty. Additionally, through deep semantic analysis, we demonstrated that our algorithm contains more profound conceptual incorporations and a broader semantic spectrum.
Our study advances the field of psychology in two significant ways. Firstly, it extracts invaluable causal knowledge from the literature and converts it to visual graphics. These aids can feed algorithms to help deduce more latent causal relations and guide models in generating a plethora of novel causal hypotheses. Secondly, our study furnishes novel tools and methodologies for causal analysis and scientific knowledge discovery, representing the seamless fusion of modern AI with traditional research methodologies. This integration serves as a bridge between conventional theory-driven methodologies in psychology and the emerging paradigms of data-centric research, thereby enriching our understanding of the factors influencing psychology, especially within the realm of social psychology.
2 Methodological Framework for Hypothesis Generation
The proposed LLM-based causal graph (LLMCG) framework encompasses three steps: literature retrieval, causal pair extraction, and hypothesis generation , as illustrated in Figure 1 . In the literature gathering phase, approximately 140K psychology-related articles were downloaded from public databases. In step two, GPT-4 were used to distill causal relationships from these articles , culminating in the creation of a causal relationship network based on 43,312 selected articles. In the third step, an in-depth examination of these data was executed, adopting link prediction algorithms to forecast the dynamics within the causal relationship network for searching the highly potential causality concept pairs.

2.1 Step 1: Literature Retrieval
The primary data source for this study was a public repository of scientific articles, the PMC Open Access Subset. Our decision to utilize this repository was informed by several key attributes that it possesses. The PMC Open Access Subset boasts an expansive collection of over 2 million full-text XML science and medical articles, providing a substantial and diverse base from which to derive insights for our research. Furthermore, the open-access nature of the articles not only enhances the transparency and reproducibility of our methodology, but also ensures that the results and processes can be independently accessed and verified by other researchers. Notably , the content within this subset originates from recognized journals, all of which have undergone rigorous peer review, lending credence to the quality and reliability of the data we leveraged. Finally , an added advantage was the rich metadata accompanying each article. These metadata were instrumental in refining our article selection process, ensuring coherent thematic alignment with our research objectives in the domains of psychology.
To identify articles relevant to our study, we applied a series of filtering criteria. First , the presence of certain keywords within article titles or abstracts was mandatory. Some examples of these keywords include ‘psychol’, ‘clin psychol’, and ‘biol psychol’. Second, we exploited the metadata accompanying each article. The classification of articles based on these metadata ensured alignment with recognized thematic standards in the domains of psychology and neuroscience. Upon the application of these criteria, we managed to curate a subset of approximately 140K articles that most likely discuss causal concepts in both psychology and neuroscience.
2.2 Step 2: Causal Pair Extraction
The process of extracting causal knowledge from vast troves of scientific literature is intricate and multifaceted. Our methodology distills this complex process into four coherent steps, each serving a distinct purpose. (1) Article selection and cost analysis: Determines the feasibility of processing a specific volume of articles, ensuring optimal resource allocation. (2) Text extraction and analysis: Ensures the purity of the data that enter our causal extraction phase by filtering out nonrelevant content. (3) Causal knowledge extraction: Uses advanced language models to detect, classify, and standardize causal factors relationships present in texts. (4) Graph database storage: Facilitates structured storage, easy retrieval, and the possibility of advanced relational analyses for future research. This streamlined approach ensures accuracy, consistency, and scalability in our endeavor to understand the interplay of causal concepts in psychology and neuroscience.
2.2.1 Text extraction and cleaning.
After a meticulous cost analysis detailed in Appendix A.1 , our selection process identified 43,312 articles. This selection was strategically based on the criterion that the journal titles must incorporate the term ‘Psychol’, signifying their direct relevance to the field of psychology. The distributions of publication sources and years can be found in Table 1 . Extracting the full texts of the articles from their PDF sources was an essential initial step, and, for this purpose, the PyPDF2 Python library was used. This library allowed us to seamlessly extract and concatenate titles, abstracts, and main content from each PDF article. However, a challenge arose with the presence of extraneous sections such as references or tables, in the extracted texts. The implemented procedure, employing regular expressions in Python, was not only adept at identifying variations of the term ‘references’ but also ascertained whether this section appeared as an isolated segment. This check was critical to ensure that the identified that the ‘references’ section was indeed distinct, marking the start of a reference list without continuation into other text. Once identified as a standalone entity, the next step in the method was to efficiently remove the reference section and its subsequent content.
2.2.2 Causal knowledge extraction method.
In our effort to extract causal knowledge, the choice of GPT-4 was not arbitrary. While several models were available for such tasks, GPT-4 emerged as a frontrunner due to its advanced capabilities [ wu2023comparative ] , extensive training on diverse data, with its proven proficiency in understanding context, especially in complex scientific texts [ cheng2023exploring , sanderson2023gpt ] . Other models were indeed considered; however, the capacity of GPT-4 to generate coherent , contextually relevant responses gave our project an edge in its specific requirements.
The extraction process commenced with the segmentation of the articles. Due to the token constraints inherent to GPT-4, it was imperative to break down the articles into manageable chunks, specifically those of 4,000 tokens or fewer. This approach ensured a comprehensive interpretation of the content without omitting any potential causal relationships. The next phase was prompt engineering. To effectively guide the extraction capabilities of GPT-4, we crafted explicit prompts. A testament to this meticulous engineering is demonstrated in a directive in which we asked the model to elucidate causal pairs in a predetermined JSON format. For a clearer understanding, readers are referred to Table 2 , which elucidates the example prompt and the subsequent model response. After extraction, the outputs were not immediately cataloged. A filtering process was initiated to ascertain the standardization of the concept pairs. This process weeded out suboptimal outputs. Aiding in this quality control, GPT-4 played a pivotal role in the verification of causal pairs, determining their relevance, causality, and ensuring correct directionality. Finally, while extracting knowledge, we were aware of the constraints imposed by the GPT-4 API. There was a conscious effort to ensure that we operated within the bounds of 60 requests and 150k tokens per minute. This interplay of prompt engineering and stringent filtering was productive.
In addition, we conducted an exploratory study to assess GPT-4’s discernment between ‘causality’ and ‘correlation’ involved four graduate students (mean age 31 ± plus-or-minus \pm ± 10.23), each evaluating relationship pairs extracted from their familiar psychology articles. The experimental details and results can be found in Appendix A.1 and Table 8 . The results showed that out of 289 relationships identified by GPT-4, 87.54% were validated. Notably, when GPT-4 classified relationships as causal, only 13.02 % (31/238) were recognized as non-relationship, while 65.55% (156/238) agreed upon as causality. This shows that GPT-4 can accurately extract relationships (causality or correlation) in psychological texts, underscoring the potential as a tool for the construction of causal graphs.
To enhance the robustness of the extracted causal relationships and minimize biases, we adopted a multifaceted approach. Recognizing the indispensable role of human judgment, we periodically subjected random samples of extracted causal relationships to the scrutiny of domain experts. Their valuable feedback was instrumental in the real-time fine-tuning the extraction process. Instead of heavily relying on referenced hypotheses, our focus was on extracting causal pairs, primarily from the findings mentioned in the main texts. This systematic methodology ultimately resulted in a refined text corpus distilled from 43,312 articles, which contained many conceptual insights and were primed for rigorous causal extraction.
2.2.3 Graph database storage.
Our decision to employ Neo4j as the database system was strategic. Neo4j, as a graph database [ thomer2020relational ] , is inherently designed to capture and represent complex relationships between data points, an attribute that is essential for understanding intricate causal relationships. Beyond its technical prowess, Neo4j provides advantages such as scalability, resilience, and efficient querying capabilities [ webber2012programmatic ] . It is particularly adept at traversing interconnected data points, making it an excellent fit for our causal relationship analysis. The mined causal knowledge finds its abode in the Neo4j graph database. Each pair of causal concepts is represented as a node, with its directionality and interpretations stored as attributes. Relationships provide related concepts together. Storing the knowledge graph in Neo4j allows for the execution of the graph algorithms to analyze concept interconnectivity and reveal potential relationships.
The graph database contains 197K concepts and 235K connections. Table 3 encapsulates the core concepts and provides a vivid snapshot of the most recurring themes; helping us to understand the central topics that dominate the current psychological discourse. A comprehensive examination of the core concepts extracted from 43,312 psychological papers, several distinct patterns and focal areas emerged. In particular, there is a clear balance between health and illness in psychological research. The prominence of terms such as ‘depression’, ‘anxiety’, and ‘symptoms of depression magnifies the commitment in the discipline to understanding and addressing mental illnesses. However , juxtaposed against these are positive terms such as ‘life satisfaction’ and ‘sense of happiness’, suggesting that psychology not only fixates on challenges but also delves deeply into the nuances of positivity and well-being. Furthermore, the significance given to concepts such as ‘life satisfaction’, ‘sense of happiness’, and ‘job satisfaction’ underscores an increasing recognition of emotional well-being and job satisfaction as integral to overall mental health. Intertwining the realms of psychology and neuroscience, terms such as ‘microglial cell activation’, ‘cognitive impairment’, and ‘neurodegenerative changes’ signal a growing interest in understanding the neural underpinnings of cognitive and psychological phenomena. In addition, the emphasis on ‘self-efficacy’, ‘positive emotions’, and ‘self-esteem’ reflect the profound interest in understanding how self-perception and emotions influence human behavior and well-being. Concepts such as ‘age’, ‘resilience’, and ‘creativity’ further expand the canvas, showcasing the eclectic and comprehensive nature of inquiries in the field of psychology.
Overall , this analysis paints a vivid picture of modern psychological research, illuminating its multidimensional approach. It demonstrates a discipline that is deeply engaged with both the challenges and triumphs of human existence, offering holistic insight into the human mind and its myriad complexities.
2.3 Step 3: Hypothesis Generation using Link Prediction
In the quest to uncover novel causal relationships beyond direct extraction from texts, the technique of link prediction emerges as a pivotal methodology. It hinges on the premise of proposing potential causal ties between concepts that our knowledge graph does not explicitly connect. The process intricately weaves together vector embedding, similarity analysis, and probability-based ranking. Initially, concepts are transposed into a vector space using node2vec, which is valued for its ability to capture topological nuances. Here, every pair of unconnected concepts is assigned a similarity score, and pairs that do not meet a set benchmark are quickly discarded. As we dive deeper into the higher echelons of these scored pairs, the likelihood of their linkage is assessed using the Jaccard similarity of their neighboring concepts. Subsequently, these potential causal relationships are organized in descending order of their derived probabilities, and the elite pairs are selected.
An illustration of this approach is provided in the case highlighted in Figure 5 . For instance , the behavioral inhibition system (BIS) exhibits ties to both the behavioral activation system (BAS) and the subsequent behavioral response of the BAS when encountering reward stimuli, termed the BAS reward response. Simultaneously, another concept, interference, finds itself bound to both the BAS and the BAS Reward Response. This configuration hints at a plausible link between the BIS and interference. Such highly probable causal pairs are not mere intellectual curiosity . They act as springboards, catalyzing the genesis of new experimental designs or research hypotheses ripe for empirical probing. In essence, this capability equips researchers with a cutting-edge instrument, empowering them to navigate the unexplored waters of the psychological and neurological domains.
Using pairs of highly probable causal concepts, we pushed GPT-4 to conjure novel causal hypotheses that bridge concepts. To further elucidate the process of this method, Table 4 provides some examples of hypotheses generated from the process. Such hypotheses, as exemplified in the last row, underscore the potential and power of our method for generating innovative causal propositions.
3 Hypotheses Evaluation and Results
In this section, we present an analysis focusing on quality in terms of novelty and usefulness of the hypotheses generated. According to existing literature, these dimensions are instrumental in encapsulating the essence of inventive ideas [ boden2009computer , mccarthy2018novelty , miron2015motivating ] . These parameters have not only been quintessential for gauging creative concepts, but they have also been adopted to evaluate the caliber of research hypotheses [ oleinik2019neural , dowling2023chatgpt , krenn2020predicting ] . Specifically, we evaluate the quality of the hypotheses generated by the proposed LLMCG algorithm in relation to those generated by PhD students from an elite university who represent human junior experts, the LLM model , which represents advanced AI systems, and the research ideas refined by psychological researchers which represents cooperation between AI and humans .
The evaluation comprises three main stages. In the first stage, the hypotheses are generated by all contributors, including steps taken to ensure fairness and relevance for comparative analysis. In the second stage, the hypotheses from the first stage are independently and blindly reviewed by experts who represent the human academic community. These experts are asked to provide hypothesis ratings using a specially designed questionnaire to ensure statistical validity . The third stage delves deeper by transforming each research idea into the semantic space of a bidirectional encoder representations from transformers (BERT) [ lee2023natural ] , allowing us to intricately analyze the intrinsic reasons behind the rating disparities among the groups. This semantic mapping not only pinpoints the nuanced differences, but also provides potential insights into the cognitive constructs of each hypothesis.
3.1 Evaluation Procedure
3.1.1 selection of the focus area for hypothesis generation..
Selecting an appropriate focus area for hypothesis generation is crucial to ensure a balanced and insightful comparison of the hypothesis generation capacities between various contributors. In this study, our goal is to gauge the quality of hypotheses derived from four distinct contributors, with measures in place to mitigate potential confounding variables that might skew the results among groups [ rubin2005causal ] . Our choice of domain is informed by two pivotal criteria: the intricacy and subtlety of the subject matter and familiarity with the domain. It is essential that our chosen domain boasts sufficient complexity to prompt meaningful hypothesis generation and offer a robust assessment of both AI and human contributors’ depth of understanding and creativity. Furthermore, while human contributors should be well-acquainted with the domain, their expertise need not match the vast corpus knowledge of the AI.
In terms of overarching human pursuits such as the search for happiness, positive psychology distinguishes itself by avoiding narrowly defined, individual-centric challenges [ seligman2000positive ] . This alignment with our selection criteria is epitomized by well-being, a salient concept within positive psychology, as shown in Table 3 . Well-being, with its multidimensional essence that encompass emotional, psychological, and social facets, and its central stature in both research and practical applications of positive psychology [ seligman2000positive , fredrickson2001role , diener2010new ] , becomes the linchpin of our evaluation. The growing importance of well-being in the current global context offers myriad novel avenues for hypothesis generation and theoretical advancement [ otu2020mental , madill2022mainstreaming , forgeard2011doing ] . Adding to our rationale, the Positive Psychology Research Center at Tsinghua University is a globally renowned hub for cutting-edge research in this domain. Leveraging this stature, we secured participation from specialized PhD students, reinforcing positive psychology as the most fitting domain for our inquiry.
3.1.2 Hypotheses comparison.
In our study, the generated psychological hypotheses were categorized into four distinct groups, consisting of two experimental groups and two control groups. The experimental groups encapsulate hypotheses generated by our algorithm, either through random selection or handpicking by experts from a pool of generated hypotheses. On the other hand, control groups comprise research ideas that were meticulously crafted by doctoral students with substantial academic expertise in the domains and hypotheses generated by representative LLMs. In the following, we elucidate the methodology and underlying rationale for each group:
LLMCG algorithm output (Random-selected LLMCG) : Following to the requirement of generating hypotheses centered on well-being, the LLMCG algorithm crafted 130 unique hypotheses. These hypotheses were derived by LLMCG’s evaluation of the most likely causal relationships related to well-being that had not been previously documented research literature dataset . From this refined pool, 30 research ideas were chosen at random for this experimental group. These hypotheses represent the algorithm’s ability to identify causal relationships and formulate pertinent hypotheses.
LLMCG expert-vetted hypotheses (Expert-selected LLMCG) : For this group, two seasoned psychological researchers, one male aged 47 and one female aged 46, in-depth expertise in the realm of Positive Psychology, conscientiously handpicked 30 of the most promising hypotheses from the refined pool, excluding those from the Random-selected LLMCG category. The selection criteria centered on a holistic understanding of both the novelty and practical relevance of each hypothesis. With an illustrious postdoctoral journey and a robust portfolio of publications in positive psychology to their names, they rigorously sifted through the hypotheses, pinpointing those that showcased a perfect confluence of originality and actionable insight. These hypotheses were meticulously appraised for their relevance, structural coherence, and potential academic value, representing the nexus of machine intelligence and seasoned human discernment.
PhD students’ output (Control-Human) : We enlisted the expertise of 16 doctoral students from the Positive Psychology Research Center at Tsinghua University. Under the guidance of their supervisor, each student was provided with a questionnaire geared toward research on well-being. The participants were given a period of four working days to complete and return the questionnaire, which was distributed during vacation to ensure minimal external disruptions and commitments. The specific instructions provided in the questionnaire is detailed in Table 9 , and each participant was asked to complete 3-4 research hypotheses. By the stipulated deadline, we received responses from 13 doctoral students, with a mean age of 31.92 years (SD = 7.75 years), cumulatively presenting 41 hypotheses related to well-being. To maintain uniformity with the other groups, a random selection was made to shortlist 30 hypotheses for further analysis. These hypotheses reflect the integration of core theoretical concepts with the latest insights into the domain, presenting an academic interpretation rooted in their rigorous training and education. Including this group in our study not only provides a natural benchmark for human ingenuity and expertise but also underscores the invaluable contribution of human cognition in research ideation, serving as a pivotal contrast to AI-generated hypotheses. This juxtaposition illuminates the nuanced differences between human intellectual depth and AI’s analytical progress , enriching the comparative dimensions of our study.
Claude model output (Control-Claude) : This group exemplifies the pinnacle of current LLM technology in generating research hypotheses. Since LLMCG is a nascent technology, its assessment requires a comparative study with well-established counterparts, creating a key paradigm in comparative research. Currently, Claude-2 and GPT-4 represent the apex of AI technology. For example, Claude-2, with an accuracy rate of 54. 4% excels in reasoning and answering questions, substantially outperforming other models such as Falcon, Koala and Vicuna, which have accuracy rates of 17.1%-25.5% [ wu2023comparative ] . To facilitate a more comprehensive evaluation of the new model by researchers and to increase the diversity and breadth of comparison, we chose Claude-2 as the control model. Using the detailed instructions provided in Table 10 , Claude-2 was iteratively prompted to generate research hypotheses, generating ten hypotheses per prompt, culminating in a total of 50 hypotheses. Although the sheer number and range of these hypotheses accentuate the capabilities of Claude-2, to ensure compatibility in terms of complexity and depth between all groups, a subsequent refinement was considered essential. With minimal human intervention, GPT-4 was used to evaluate these 50 hypotheses and select the top 30 that exhibited the most innovative, relevant, and academically valuable insights. This process ensured the infusion of both the LLM’s analytical prowess and a layer of qualitative rigor, thus giving rise to a set of hypotheses that not only align with the overarching theme of well-being but also resonate with current academic discourse.
3.1.3 Hypotheses assessment.
The assessment of the hypotheses encompasses two key components: the evaluation conducted by eminent psychology professors emphasizing novelty and utility, and the deep semantic analysis involving BERT and t 𝑡 t italic_t -distributed stochastic neighbor embedding ( t 𝑡 t italic_t -SNE) visualization to discern semantic structures and disparities among hypotheses.
Human academic community. The review task was entrusted to three eminent psychology professors (all male, mean age = 42.33), who have a decade-long legacy in guiding doctoral and master’s students in positive psychology and editorial stints in renowned journals; their task was to conduct a meticulous evaluation of the 120 hypotheses . Importantly, to ensure unbiased evaluation, the hypotheses were presented to them in a completely randomized order in the questionnaire.
Our emphasis was undeniably anchored to two primary tenets: novelty and utility [ yu2016semantic , shardlow2018identification , thompson2023scope , cohen2017should ] , as shown in Table 11 . Utility in hypothesis crafting demands that our propositions extend beyond mere factual accuracy; they must resonate deeply with academic investigations, ensuring substantial practical implications. Given the inherent challenges of research, marked by constraints in time, manpower, and funding, it is essential to design hypotheses that optimize the utilization of these resources. On the novelty front , we strive to introduce innovative perspectives that have the power to challenge and expand upon existing academic theories. This not only propels the discipline forward but also ensures that we do not inadvertently tread on ground already covered by our contemporaries.
Deep semantic analysis. While human evaluations provide invaluable insight into the novelty and utility of hypotheses, to objectively discern and visualize semantic structures and the disparities among them, we turn to the realm of deep learning. Specifically, we employ the power of BERT [ devlin2018bert ] . BERT, as highlighted by [ lee2023natural ] , had a remarkable potential to assess the innovation of ideas. By translating each hypothesis into a high-dimensional vector in the BERT domain, we obtain the profound semantic core of each statement. However , such granularity in dimensions presents challenges when aiming for visualization.
To alleviate this and to intuitively understand the clustering and dispersion of these hypotheses in semantic space, we deploy the t 𝑡 t italic_t -SNE ( t 𝑡 t italic_t -distributed Stochastic Neighbor Embedding) technique [ van2008visualizing ] , which is adept at reducing the dimensionality of the data while preserving the relative pairwise distances between the items. Thus, when we map our BERT-encoded hypotheses onto a 2D t 𝑡 t italic_t -SNE plane, an immediate visual grasp on how closely or distantly related our hypotheses are in terms of their semantic content. Our intent is twofold: to understand the semantic terrains carved out by the different groups and to infer the potential reasons for some of the hypotheses garnered heightened novelty or utility ratings from experts. The convergence of human evaluations and semantic layouts, as delineated by Algorithm 1 , reveal the interplay between human intuition and the inherent semantic structure of the hypotheses.
3.2 Results
3.2.1 qualitative analysis by topic analysis.
To better understand the underlying thought processes and the topical emphasis of both PhD students and the LLMCG model, qualitative analyses were performed using visual tools such as word clouds and connection graphs, as detailed in Appendix B.1 . The word cloud, as a graphical representation, effectively captures the frequency and importance of terms, providing direct visualization of the dominant themes. Connection graphs, on the other hand, elucidate the relationships and interplay between various themes and concepts. Using these visual tools, we aimed to achieve a more intuitive and clear representation of the data, allowing for easy comparison and interpretation.
Observations drawn from both the word clouds and the connection graphs in Figures 6 and 7 provide us with a rich tapestry of insights into the thought processes and priorities of PhD students and the LLMCG model. For instance, the emphasis in the Control-Human word cloud on terms such as ‘robot’ and ‘AI’ indicates a strong interest among PhD students in the nexus between technology and psychology. It is particularly fascinating to see a group of academically trained individuals focusing on the real world implications and intersections of their studies, as shown by their apparent draw toward trending topics. This not only underscores their adaptability but also emphasizes the importance of contextual relevance. Conversely , the LLMCG groups , particularly the Expert-selected LLMCG group, emphasize the community, collective experiences, and the nuances of social interconnectedness. This denotes a deep-rooted understanding and application of higher-order social psychological concepts, reflecting the model’s ability to dive deep into the intricate layers of human social behavior.
Furthermore , the connection graphs support these observations. The Control-Human graph, with its exploration of themes such as ‘Robot Companionship’ and its relation to factors such as ‘heart rate variability (HRV)’, demonstrates a confluence of technology and human well-being. The other groups, especially the Random-selected LLMCG group, yield themes that are more societal and structural, hinting at broader determinants of individual well-being.
3.2.2 Analysis of human evaluations
To quantify the agreement among the raters, we employed Spearman correlation coefficients. The results, as shown in Table 13 , reveal a spectrum of agreement levels between the reviewer pairs, showcasing the subjective dimension intrinsic to the evaluation of novelty and usefulness. In particular, the correlation between reviewer 1 and reviewer 2 in novelty (Spearman r 𝑟 r italic_r = 0.387, p < 𝑝 absent p< italic_p < 0.0001 ) and between reviewer 2 and reviewer 3 in usefulness (Spearman r 𝑟 r italic_r = 0.376, p < 𝑝 absent p< italic_p < 0.0001) suggests a meaningful level of consensus, particularly highlighting their capacity to identify valuable insights when evaluating hypotheses.
The variations in correlation values, such as between reviewer 2 and reviewer 3 ( r 𝑟 r italic_r = 0.069, p 𝑝 p italic_p = 0.453), can be attributed to the diverse research orientations and backgrounds of each reviewer. Reviewer 1 focuses on social ecology, reviewer 3 specializes in neuroscientific methodologies, and reviewer 2 integrates various views using technologies like virtual reality, and computational methods. In our evaluation, we present specific hypotheses cases to illustrate the differing perspectives between reviewers, as detailed in Table 12 and Figure 8 . For example, C5 introduces the novel concept of ‘Virtual Resilience’. Reviewers 1 and 3 highlighted its originality and utility, while reviewer 2 rated it lower in both categories. Meanwhile, C6, which focuses on social neuroscience, resonated with reviewer 3, while reviewers 1 and 2 only partially affirmed it. These differences underscore the complexity of evaluating scientific contributions and highlight the importance of considering a range of expert opinions for a comprehensive evaluation.
This assessment is divided into two main sections: Novelty analysis and usefulness analysis.
Novelty analysis : In the dynamic realm of scientific research, measuring and analyzing novelty is gaining paramount importance [ shin2022scientific ] . ANOVA was used to analyze the novelty scores represented in Figure 2a , and we identified a significant influence of the group factor on the mean novelty score between different reviewers. The clear distinctions between the groups, as visualized in the boxplots, are statistically underpinned by the results in Table 5 . The ANOVA results revealed a pronounced effect of the grouping factor ( F (3,116)=6.92, p = 0.0002), with variance explained by the grouping factor (R-squared) of 15.19%.

Further pairwise comparisons using the Bonferroni method, as delineated in Table 5 and visually corroborated by Figure 2a ; significant disparities were discerned between Random-selected LLMCG and Control-Claude ( t (59) = 3.34, p =0.007) and between Control-Human and Control-Claude ( t (59) = 4.32, p <0.001). Importantly, when considering the cumulative distribution plots to the right of Figure 2a , we observe the distributional characteristics of the novel scores. For example, it can be observed that the Expert-selected LLMCG curve portrays a greater concentration in the middle score range when compared to the Control-Claude , curve but dominates in the high novelty scores (highlighted in dashed rectangle). Moreover, comparisons involving Control-Human with both Random-selected LLMCG and Expert-selected LLMCG did not manifest statistically significant variances, indicating aligned novelty perceptions among these groups. Finally , the comparisons between Expert-selected LLMCG and Control-Claude ( t (59) = 2.49, p =0.085) suggest a trend toward significance, underscoring the nuanced differences between these groups in the novelty dimension.
To mitigate potential biases due to individual reviewer inclinations, we expanded our evaluation to encompass both median and maximum values. These multifaceted analyses enhance the robustness of our results by minimizing the influence of extreme values and potential outliers. First, when analyzing the median novelty scores, the ANOVA test demonstrated a notable association with the grouping factor ( F (3,116)=6.54, p =0.0004), which explained 14.41% of the variance . As illustrated in Table 5 , pairwise evaluations revealed significant disparities between Control-Human and Control-Claude ( t (59) = 4.01, p =0.001) as well as between Random-selected LLMCG and Control-Claude ( t (59) = 3.40, p =0.006). Interestingly, the comparison of Expert-selected LLMCG with Control-Claude ( t (59) = 1.70, p =0.550) and other group pairings were not included statistically significant differences.
Subsequently, turning our attention to maximum novelty scores provided crucial insights, especially where outlier scores may carry significant weight. The influence of the grouping factor was evident ( F (3,116)=7.20, p =0.0002), indicating an explained variance of 15.70%. In particular, clear differences emerged between Control-Human and Control-Claude ( t (59) = 4.36, p <0.001), and between Random-selected LLMCG and Control-Claude ( t (59) = 3.47, p =0.004). A particularly intriguing observation was the significant difference between Expert-selected LLMCG and Control-Claude ( t (59) = 3.12, p =0.014). Together, these analyses offer a multifaceted perspective on novelty evaluations. Specifically, the results of the median analysis echo and support those of the mean, reinforcing the reliability of our assessments. The discerned significance between Control-Claude and Expert-selected LLMCG in the median data emphasizes the intricate differences, while also pointing to broader congruence in novelty perceptions.
Usefulness analysis. Evaluating the practical impact of hypotheses is crucial in scientific research assessments. In the mean useful spectrum, the grouping factor did not exert a significant influence ( F (3,116)=5.25, p = 0.553). Figure 2b presents the utility score distributions between groups. The narrow interquartile range of Control-Human suggests a relatively consistent assessment among reviewers. On the other hand, the spread and outliers in the Control-Claude distribution hint at varied utility perceptions. Both LLMCG groups cover a broad score range, demonstrating a mixture of high and low utility scores, while the Expert-selected LLMCG gravitates more toward higher usefulness scores. The smoothed line plots accompanying Figure 2b further detail the score densities. For instance, Random-selected LLMCG boasts several high utility scores, counterbalanced by a smattering of low scores. Interestingly, the distributions for Control-Human and Expert-selected LLMCG appear to be closely aligned. While mean utility scores provide an overarching view, the nuances within the boxplots and smoothed plots offer deeper insights. This comprehensive understanding can guide future endeavors in content generation and evaluation, spotlighting key areas of focus and potential improvements.

3.2.3 Deep semantic analysis
The t 𝑡 t italic_t -SNE visualizations (Figure 3 ) illustrate the semantic relationships between different groups, capturing the patterns of novelty and usefulness. Notably, a distinct clustering among PhD students suggests shared academic influences, while the LLMCG groups display broader topic dispersion, hinting at a wider semantic understanding. The size of the bubbles reflects the novelty and usefulness scores, emphasizing the diverse perceptions of what is considered innovative versus beneficial. Additionally, the numbers near the yellow dots represent the participant IDs, which demonstrated that the semantics of the same participant, such as H05 or H06, are closely aligned. In Figure 9 , a distinct clustering of examples is observed, particularly highlighting the close proximity of hypotheses C3, C4 and C8 within the semantic space. This observation is further elucidated in the Appendix A.2 , enhancing the comprehension of BERT’s semantic representation. Instead of solely depending on superficial textual descriptions, this analysis penetrates into the underlying understanding of concepts within the semantic space, a topic also explored in recent research [ johnson2023divergent ] .
In the distribution of semantic distances (Figure 4 ), we observed that the Control-Human group exhibits a distinctively greater semantic distance in comparison to the other groups, emphasizing their unique semantic orientations. The statistical support for this observation is derived from the ANOVA results, with a significant F-statistic ( F (3,1652)=84.1611, p 𝑝 p italic_p < 0.00001), underscoring the impact of the grouping factor. This factor explains a remarkable 86.96% of the variance, as indicated by the R 𝑅 R italic_R -squared value. Multiple comparisons, as shown in Table 6 , further elucidate the subtleties of these group differences. Control-Human and Control-Claude exhibit a significant contrast in their semantic distances, as highlighted by the t 𝑡 t italic_t -value of 16.41 and the adjusted p 𝑝 p italic_p -value ( < 0.0001 absent 0.0001 <0.0001 < 0.0001 ). This difference indicates distinct thought patterns or emphasis in the two groups. a comparison of Control-Human with the LLMCG models shows divergent semantic orientations. The group has statistically significant differences with Random-selected LLMCG ( p 𝑝 p italic_p =0.0036) and a trend toward difference with Expert-selected LLMCG ( p 𝑝 p italic_p =0.0687). A comparison of the Control-Claude and LLMCG models reveals pronounced differences, more so with the Expert-selected LLMCG ( p 𝑝 p italic_p < 0.0001 0.0001 0.0001 0.0001 ). Intriguingly, the two LLMCG groups —— Random-selected and Expert-selected —— exhibit similar semantic distances, as evidenced by a nonsignificant p 𝑝 p italic_p -value of 0.4362. Furthermore, the significant distinctions we observed, particularly between the control-human and other groups, align with human evaluations of novelty. This coherence indicates that the BERT space representation coupled with statistical analyses could effectively mimic human judgment. Such results underscore the potential of this approach for automated hypothesis testing, paving the way for more efficient and streamlined semantic evaluations in the future.
In general, visual and statistical analyses reveal the nuanced semantic landscapes of each group. While the PhD students’ shared background influences their clustering, the machine models exhibit a comprehensive grasp of topics, emphasizing the intricate interplay of individual experiences, academic influences, and algorithmic understanding in shaping semantic representations.
This investigation carried out a detailed evaluation of the various hypothesis contributors, blending both quantitative and qualitative analyses . In terms of topic analysis, distinct variations were observed between Control-Human and LLMCG, the latter presenting more expansive thematic coverage. For human evaluation, hypotheses from PhD students paralleled the LLMCG in novelty, reinforcing AI’s growing competence in mirroring human innovative thinking. Furthermore, when juxtaposed with AI models such as Control-Claude , the LLMCG exhibited increased novelty. Deep semantic analysis via t 𝑡 t italic_t -SNE and BERT representations allowed us to intuitively grasp semantic essence of hypotheses , signaling the possibility of future automated hypothesis assessments. Interestingly, LLMCG appeared to encompass broader complementary domains compared to human input. Taken together, these findings highlight the emerging role of AI in hypothesis generation and provides key insights into hypothesis evaluation across diverse origins.
3.2.4 Comparison Between the LLMCG and GPT-4
To evaluate the impact of integrating a causal graph with GPT-4, we performed an ablation study comparing the hypotheses generated by GPT-4 alone and those of the proposed LLMCG framework. For this experiment, 60 hypotheses were created using GPT-4, following the detailed instructions in Table 10 . Furthermore, 60 hypotheses for the LLMCG group were randomly selected from the remaining pool of 70 hypotheses. Subsequently, both set of hypotheses were assessed by three independent reviewers for novelty and usefulness, as previously described.
Table 7 shows a comparison between the GPT-4 and LLMCG groups, highlighting a significant difference in novelty scores (mean value: t(119) = 6.60, p < 0.0001 𝑝 0.0001 p<0.0001 italic_p < 0.0001 ) but not in usefulness scores (mean value: t(119) = 1.31, p = 0.1937 𝑝 0.1937 p=0.1937 italic_p = 0.1937 ). This indicates that the LLMCG framework significantly enhances hypothesis novelty without affecting usefulness compared to the GPT-4 group. Figure 11 visually contrasts these findings, underlining the causal graph’s unique role in fostering novel hypothesis generation when integrated with GPT-4.
4 General Discussion
This research delves into the synergistic relationship between LLM and causal graphs in the hypothesis generation process. Our findings underscore the ability of LLM, when integrated with causal graph techniques, to produce meaningful hypotheses with increased efficiency and quality. By centering our investigation on ‘well-being’ we emphasize its pivotal role in psychological studies and highlight the potential convergence of technology and society. A multifaceted assessment approach to evaluate quality by topic analysis, human evaluation and deep semantic analysis demonstrates that AI-augmented methods not only outshine LLM-only techniques in generating hypotheses with superior novelty and show a quality on par with human expertise but also boast the capability for more profound conceptual incorporations and a broader semantic spectrum. Such a multifaceted lens of assessment introduces a novel perspective for the scholarly realm, equipping researchers with an enriched understanding and an innovative toolset for hypothesis generation. At its core, the melding of LLM and causal graphs signals a promising frontier, especially in regard to dissecting cornerstone psychological constructs such as ‘well-being’. This marriage of methodologies, enriched by the comprehensive assessment angle, deepens our comprehension of both the immediate and broader ramifications of our research endeavors.
The prominence of causal graphs in psychology is profound, they offer researchers a unified platform for synthesizing and hypothesizing diverse psychological realms [ uleman2021mapping , borsboom2021network ] . Our study echoes this, producing groundbreaking hypotheses comparable in depth to early expert propositions. Deep semantic analysis bolstered these findings, emphasizing that our hypotheses have distinct cross-disciplinary merits, particularly when compared to those of individual doctoral scholars. However, the traditional use of causal graphs in psychology presents challenges due to its demanding nature, often requiring insights from multiple experts [ crielaard2022refining ] . Our research, however, harnesses LLM’s causal extraction, automating causal pair derivation and, in turn, minimizing the need for extensive expert input. The union of the causal graphs’ systematic approach with AI-driven creativity, as seen with LLMs, paves the way for the future in psychological inquiry. Thanks to advancements in AI, barriers once created by causal graphs’ intricate procedures are being dismantled. Furthermore, as the era of big data dawns, the integration of AI and causal graphs in psychology augments research capabilities, but also brings into focus the broader implications for society. This fusion provides a nuanced understanding of the intricate sociopsychological dynamics, emphasizing the importance of adapting research methodologies in tandem with technological progress.
In the realm of research, LLMs serve a unique purpose, often by acting as the foundation or baseline against which newer methods and approaches are assessed. The demonstrated productivity enhancements by generative AI tools, as evidenced by [ noy2023experimental ] , indicate the potential of such LLMs. In our investigation, we pit the hypotheses generated by such substantial models against our integrated LLMCG approach. Intriguingly, while these LLMs showcased admirable practicality in their hypotheses, they substantially lagged behind in terms of innovation when juxtaposed with the doctoral student and LLMCG group. This divergence in results can be attributed to the causal network curated from 43k research papers, funneling the vast knowledge reservoir of the LLM squarely into the realm of scientific psychology. The increased precision in hypothesis generation by these models fits well within the framework of generative networks. [ tong2021putative ] highlighted that, by integrating structured constraints, conventional neural networks can accurately generate semantically relevant content. One of the salient merits of the causal graph, in this context, is its ability to alleviate inherent ambiguity or interpretability challenges posed by LLMs. By providing a systematic and structured framework, the causal graph aids in unearthing the underlying logic and rationale of the outputs generated by LLMs. Notably, this finding echoes the perspective of [ pan2023unifying ] , where the integration of structured knowledge from knowledge graphs was shown to provide an invaluable layer of clarity and interpretability to LLMs, especially in complex reasoning tasks. Such structured approaches not only boost the confidence of researchers in the hypotheses derived, but also augment the transparency and understandability of LLM outputs. In essence, leveraging causal graphs may very well herald a new era in model interpretability, serving as a conduit to unlock the black box that large models often represent in contemporary research.
In the ever-evolving tapestry of research, every advancement invariably comes with its unique set of constraints, and our study was no exception. On the technical front, a pivotal challenge stemmed from the opaque inner workings of the GPT. Determining the exact machinations within the GPT that lead to the formation of specific causal pairs remains elusive, thereby reintroducing the age-old issue of AI’s inherent lack of transparency [ cao2023extrapolation , buruk2023academic ] . This opacity is magnified in our sparse causal graph, which, while expansive, is occasionally riddled with concepts that, while semantically distinct, converge in meaning. In tangible applications, a careful and meticulous algorithmic evaluation would be imperative to construct an accurate psychological conceptual landscape. Delving into psychology, which bridges humanities and natural sciences, it continuously aims to unravel human cognition and behavior [ hergenhahn2013introduction ] . Despite the dominance of traditional methodologies [ henrich2010most , shah2015big ] , the present data-centric era amplifies the synergy of technology and humanities, resonating with Hasok Chang’s vision of enriched science [ chang2007scientific ] . This symbiosis is evident when assessing structural holes in social networks [ burt2004structural ] and viewing novelty as a bridge across these divides [ foster2021surprise ] . Such perspectives emphasize the importance of thorough algorithmic assessments, highlighting potential avenues in humanities research, especially when incorporating large language models for innovative hypothesis crafting and verification.
However, there are some limitations for this research. Firstly, we acknowledge that constructing causal relationship graphs has potential inaccuracies, with approximately 13% relationship pairs not aligning with human expert estimations. Enhancing the estimation of relationship extraction could be a pathway to improve the accuracy of the causal graph, potentially leading to more robust hypotheses. Secondly, our validation process was limited to 130 hypotheses, however, the vastness of our conceptual landscape suggests the countless possibilities. As an exemplar, the twenty pivotal psychological concepts highlighted in Table 3 alone could spawn an extensive array of hypotheses. However, the validation of these surrounding hypotheses would unquestionably lead to a multitude of speculations. A striking observation during our validation was the inconsistency in the evaluations of the senior expert panels (as shown in Table 13 ). This shift underscores a pivotal insight: our integration of AI has transitioned the dependency on scarce expert resources from hypothesis generation to evaluation. In the future, rigorous evaluations ensuring both novelty and utility could become a focal point of exploration. The promising path forward necessitates a thoughtful integration of technological innovation and human expertise to fully realize the potential suggested by our study.
In conclusion, our research provides pioneering insight into the symbiotic fusion of LLMs, which are epitomized by GPT, and causal graphs from the realm of psychological hypothesis generation, especially emphasizing ‘well-being’. Importantly, as highlighted by [ cao2023extrapolation ] , ensuring a synergistic alignment between domain knowledge and AI extrapolation is crucial. This synergy serves as the foundation for maintaining AI models within their conceptual limits, thus bolstering the validity and reliability of the hypotheses generated. Our approach intricately interweaves the advanced capabilities of LLMs with the methodological prowess of causal graphs, thereby optimizing while also refining the depth and precision of hypothesis generation. The causal graph, of paramount importance in psychology due to its cross-disciplinary potential, often demands vast amounts of expert involvement. Our innovative approach addresses this by utilizing LLM’s exceptional causal extraction abilities, effectively facilitating the transition of intense expert engagement from hypothesis creation to evaluation . Therefore, our methodology combined LLM with causal graphs, propelling psychological research forward by improving hypothesis generation and offering tools to blend theoretical and data-centric approaches. This synergy particularly enriches our understanding of social psychology’s complex dynamics, such as happiness research, demonstrating the profound impact of integrating AI with traditional research frameworks.
5 Authorship Contribution Statement
Song Tong : Data analysis, Experiments, Writing - original draft & review. Kai Mao : Designed the causality graph methodology, Generated AI hypotheses, Developed hypothesis generation techniques, Writing - review & editing. Zhen Huang : Statistical Analysis, Experiments, Writing - review & editing. Yukun Zhao : Conceptualization, Project administration, Supervision, Writing - review & editing. Kaiping Peng : Conceptualization, Writing - review & editing.
6 Declaration of Competing Interest
The author(s) declared no potential conflicts of interest with respect to the research, authorship, and/or publication of this article.
7 Acknowledgments
The authors thank Dr. Honghong Bai (Radboud University), Dr. ChienTe Wu (The University of Tokyo), Dr. Peng Cheng (Tsinghua University), and Yusong Guo (Tsinghua University) for their great comments on the earlier version of this manuscript. This research has been generously funded by personal contributions, with special acknowledgment to Kai Mao. Additionally, he conceived and developed the causality graph and AI hypothesis generation technology presented in this paper from scratch, and generated all AI hypotheses and paid for its costs.
The authors sincerely thank Kai Mao for fully funding the development of hypothesis generation techniques and providing the generated AI hypotheses that enabled this research. His generous support and pioneering work in these areas made this collaborative project possible.
Appendix A Method
A.1 article selection & cost analysis..
Before delving into the specifics of the extraction process, it’s crucial to emphasize the importance of cost analysis, especially when dealing with large-scale data processing. In the realm of data-intensive research, the sheer volume of articles and the intricate nature of text extraction entail significant computational and financial resources. Understanding the associated costs is paramount not only for optimal resource allocation but also for ensuring the scalability and feasibility of the project. With these considerations in mind, extracting causal knowledge from texts requires the utilization of language models, such as GPT-4, to process each paper. Given the costs associated with API usage, we projected the total expenses for different corpus sizes. Key determinants of the costs include: Token Count and API Constraints. Total number of tokens (word segments) across all texts. GPT-4 charges per thousand tokens for both inputs and outputs. In addition, GPT-4 capped at 60 requests per minute and 150k tokens per minute at the time of this research. Extraction procedures must comply with these thresholds. For our curated subset of 140k articles, with each abstract at 500 words and main content at 5,000 words, the estimates are 40 million tokens for 40k articles. The GPT-4 pricing is approximate to 40,000 USD. Based on this analysis, our choice was to extract 43,312 articles, representing around 40 million tokens. This strikes a balance between comprehensive coverage and cost-efficiency.
A.2 Differentiating Causality and Correlation by GPT-4
A experiment is conducted to investigate the capability of GPT-4 for differentiating ‘causality’ and ‘correlation’. The experiment involved four graduate students (1 male, mean age 31 ± plus-or-minus \pm ± 10.23), each well acquainted with a collection of six psychological articles. This familiarity was intended to mitigate the inherent difficulty in assessing the presence of causality or correlation within academic texts. From an initial set of 21 articles, after excluding amenable to effective processing by PyPDF and excluding review articles, GPT-4 extracted 238 causal and 51 correlational concept pairs. Detailed methodologies and GPT prompt are provided in Section ‘Causal knowledge extraction method’ and Table 2 . This resulted in an average of 11.33 pairs of causal concepts per article (SD = 7.20).
The students as evaluators were then surveyed to assess these pairs of identified concepts within the articles they provided, assigned with categorizing each as ‘existent or not’ and as ‘causal or correlational’, based on the descriptions of the articles. This unique perspective aimed to leverage their detailed understanding of the content to validate extracted concept pairs. The evaluation results are in Table 8 . The preliminary statistical analysis of the 289 relationships highlighted that 87.54% (253/289) were recognized as existing, with 74.31% (188/289) classified as causality by evaluators. Notably, when GPT-4 identified a concept pair as causality, 86.98% (207/238) of the relationships were acknowledged, with 65.55% (156/238) agreed upon as causality, and only 13.02% (31/238) potentially not mentioned in the papers.

Appendix B Results
B.1 details for topic analysis, b.1.1 word cloud comparison..
Figure 6 showcases visual representations of term and theme frequencies for different models and groups. A preliminary analysis suggests:
Control-Human (a): This word cloud seems to emphasize terms related to individual well-being and psychological health. Notable terms include ‘relationship’, ‘happiness’, ‘self’, and ‘experience’. The presence of ‘robot’ and ‘AI’ suggests topics about technology’s relationship with human psychology was atrracted by Ph.D students.
Control-Claude (b): The themes here seem to be oriented around positivity and growth. Key terms such as ‘will’, ‘increase’, ‘greater’, and ‘positive’ stand out.
Random-selected LLMCG (c): The terms in this word cloud underscore social connections, individual autonomy, and competence. Words such as ‘social’, ‘individual’, ‘autonomy’, and ‘competence’ are dominant. Themes of satisfaction, resilience, and cultural aspects can also be deciphered.
Expert-selected LLMCG (d): Here, the emphasis seems to be on community, personal feelings, and shared experiences. ‘Support’, ‘sense’, ‘one’, ‘we’, and ‘social’ are recurrent terms, highlighting collective experiences and social interconnectedness.
B.1.2 Connection graph analysis.
The connection graphs in Figure 7 depict relationships between various themes and concepts for different groups.
Control-Human (a): The graph for this model suggests a notable interplay between artificial intelligence themes, such as ‘Robot Companionship’ and ‘AI generating music/classic music’, and human well-being factors like ‘Heart rate variability (HRV) and electrodermal activity measures’ and ‘Life quality based ESM data’. This suggests research or perspectives focusing on how AI, robotics or algorithms can impact and measure human well-being.
Control-Claude (b): This graph emphasizes different facets of well-being, from ‘Emotional Well-being’ to ‘Workplace Well-being’. It also considers both positive elements, such as ‘Growth mindset’ and ‘Shared novel experiences’, and potential challenges, like ‘Reducing anxiety symptoms’. This indicates a holistic view of well-being.
Random-selected LLMCG (c): There’s a strong focus on societal and structural determinants of well-being, such as ‘Economic condition and financial hardship’, ‘Autonomy/Competence’, and ‘Management of health-related issues’. It seems to highlight the broader environmental and cultural factors affecting individual well-being.
Expert-selected LLMCG (d): This graph reflects more nuanced interconnections between personal experiences and environmental factors. Notable themes include the ‘Living in walkable, mixed-use neighborhoods’, ‘Exposure to nature’, and the ‘Integration of all influences into empowerment’. It suggests a focus on how diverse life experiences, settings, and exposures can interplay to shape an individual’s well-being.
B.2 Deep Semantic Analysis on Hypothesis Examples
Figure 9 illustrates the grouping of various cases shown in Table 12 , highlighting the proximity of hypotheses C4 and C8 within the deep semantic space of BERT (conner, bottom left). Both C4 and C8 involve interactions with technological entities that provide social support, suggesting a thematic overlap and highlighting the impact of social support mechanisms on well-being. Expanding this group to include C3, which also revolves around the theme of narrative-based therapeutic recovery, suggesting a broader category of technologically mediated psychological support. In contrast, the distance between the C7 and C4 and C8 group could indicate that their thematic links are less direct, which introduces an entirely different element, humor, combined with mindfulness, representing another separate avenue to improve well-being.
F g i superscript subscript 𝐹 𝑔 𝑖 F_{g}^{i} italic_F start_POSTSUBSCRIPT italic_g end_POSTSUBSCRIPT start_POSTSUPERSCRIPT italic_i end_POSTSUPERSCRIPT : The 2D t 𝑡 t italic_t -SNE feature representation of the i t h superscript 𝑖 𝑡 ℎ i^{th} italic_i start_POSTSUPERSCRIPT italic_t italic_h end_POSTSUPERSCRIPT idea in group g 𝑔 g italic_g , derived from the respective high-dimensional embedding.
G 𝐺 G italic_G : The set of all groups, with g 𝑔 g italic_g representing an individual group.
D g subscript 𝐷 𝑔 D_{g} italic_D start_POSTSUBSCRIPT italic_g end_POSTSUBSCRIPT : A dictionary containing lists of pairwise distances for each group g 𝑔 g italic_g .

- Search Menu
- Browse content in A - General Economics and Teaching
- Browse content in A1 - General Economics
- A11 - Role of Economics; Role of Economists; Market for Economists
- Browse content in B - History of Economic Thought, Methodology, and Heterodox Approaches
- Browse content in B4 - Economic Methodology
- B49 - Other
- Browse content in C - Mathematical and Quantitative Methods
- Browse content in C0 - General
- C00 - General
- C01 - Econometrics
- Browse content in C1 - Econometric and Statistical Methods and Methodology: General
- C10 - General
- C11 - Bayesian Analysis: General
- C12 - Hypothesis Testing: General
- C13 - Estimation: General
- C14 - Semiparametric and Nonparametric Methods: General
- C18 - Methodological Issues: General
- Browse content in C2 - Single Equation Models; Single Variables
- C21 - Cross-Sectional Models; Spatial Models; Treatment Effect Models; Quantile Regressions
- C23 - Panel Data Models; Spatio-temporal Models
- C26 - Instrumental Variables (IV) Estimation
- Browse content in C3 - Multiple or Simultaneous Equation Models; Multiple Variables
- C30 - General
- C31 - Cross-Sectional Models; Spatial Models; Treatment Effect Models; Quantile Regressions; Social Interaction Models
- C32 - Time-Series Models; Dynamic Quantile Regressions; Dynamic Treatment Effect Models; Diffusion Processes; State Space Models
- C35 - Discrete Regression and Qualitative Choice Models; Discrete Regressors; Proportions
- Browse content in C4 - Econometric and Statistical Methods: Special Topics
- C40 - General
- Browse content in C5 - Econometric Modeling
- C52 - Model Evaluation, Validation, and Selection
- C53 - Forecasting and Prediction Methods; Simulation Methods
- C55 - Large Data Sets: Modeling and Analysis
- Browse content in C6 - Mathematical Methods; Programming Models; Mathematical and Simulation Modeling
- C63 - Computational Techniques; Simulation Modeling
- C67 - Input-Output Models
- Browse content in C7 - Game Theory and Bargaining Theory
- C71 - Cooperative Games
- C72 - Noncooperative Games
- C73 - Stochastic and Dynamic Games; Evolutionary Games; Repeated Games
- C78 - Bargaining Theory; Matching Theory
- C79 - Other
- Browse content in C8 - Data Collection and Data Estimation Methodology; Computer Programs
- C83 - Survey Methods; Sampling Methods
- Browse content in C9 - Design of Experiments
- C90 - General
- C91 - Laboratory, Individual Behavior
- C92 - Laboratory, Group Behavior
- C93 - Field Experiments
- C99 - Other
- Browse content in D - Microeconomics
- Browse content in D0 - General
- D00 - General
- D01 - Microeconomic Behavior: Underlying Principles
- D02 - Institutions: Design, Formation, Operations, and Impact
- D03 - Behavioral Microeconomics: Underlying Principles
- D04 - Microeconomic Policy: Formulation; Implementation, and Evaluation
- Browse content in D1 - Household Behavior and Family Economics
- D10 - General
- D11 - Consumer Economics: Theory
- D12 - Consumer Economics: Empirical Analysis
- D13 - Household Production and Intrahousehold Allocation
- D14 - Household Saving; Personal Finance
- D15 - Intertemporal Household Choice: Life Cycle Models and Saving
- D18 - Consumer Protection
- Browse content in D2 - Production and Organizations
- D20 - General
- D21 - Firm Behavior: Theory
- D22 - Firm Behavior: Empirical Analysis
- D23 - Organizational Behavior; Transaction Costs; Property Rights
- D24 - Production; Cost; Capital; Capital, Total Factor, and Multifactor Productivity; Capacity
- Browse content in D3 - Distribution
- D30 - General
- D31 - Personal Income, Wealth, and Their Distributions
- D33 - Factor Income Distribution
- Browse content in D4 - Market Structure, Pricing, and Design
- D40 - General
- D41 - Perfect Competition
- D42 - Monopoly
- D43 - Oligopoly and Other Forms of Market Imperfection
- D44 - Auctions
- D47 - Market Design
- D49 - Other
- Browse content in D5 - General Equilibrium and Disequilibrium
- D50 - General
- D51 - Exchange and Production Economies
- D52 - Incomplete Markets
- D53 - Financial Markets
- D57 - Input-Output Tables and Analysis
- Browse content in D6 - Welfare Economics
- D60 - General
- D61 - Allocative Efficiency; Cost-Benefit Analysis
- D62 - Externalities
- D63 - Equity, Justice, Inequality, and Other Normative Criteria and Measurement
- D64 - Altruism; Philanthropy
- D69 - Other
- Browse content in D7 - Analysis of Collective Decision-Making
- D70 - General
- D71 - Social Choice; Clubs; Committees; Associations
- D72 - Political Processes: Rent-seeking, Lobbying, Elections, Legislatures, and Voting Behavior
- D73 - Bureaucracy; Administrative Processes in Public Organizations; Corruption
- D74 - Conflict; Conflict Resolution; Alliances; Revolutions
- D78 - Positive Analysis of Policy Formulation and Implementation
- Browse content in D8 - Information, Knowledge, and Uncertainty
- D80 - General
- D81 - Criteria for Decision-Making under Risk and Uncertainty
- D82 - Asymmetric and Private Information; Mechanism Design
- D83 - Search; Learning; Information and Knowledge; Communication; Belief; Unawareness
- D84 - Expectations; Speculations
- D85 - Network Formation and Analysis: Theory
- D86 - Economics of Contract: Theory
- D89 - Other
- Browse content in D9 - Micro-Based Behavioral Economics
- D90 - General
- D91 - Role and Effects of Psychological, Emotional, Social, and Cognitive Factors on Decision Making
- D92 - Intertemporal Firm Choice, Investment, Capacity, and Financing
- Browse content in E - Macroeconomics and Monetary Economics
- Browse content in E0 - General
- E00 - General
- E01 - Measurement and Data on National Income and Product Accounts and Wealth; Environmental Accounts
- E02 - Institutions and the Macroeconomy
- E03 - Behavioral Macroeconomics
- Browse content in E1 - General Aggregative Models
- E10 - General
- E12 - Keynes; Keynesian; Post-Keynesian
- E13 - Neoclassical
- Browse content in E2 - Consumption, Saving, Production, Investment, Labor Markets, and Informal Economy
- E20 - General
- E21 - Consumption; Saving; Wealth
- E22 - Investment; Capital; Intangible Capital; Capacity
- E23 - Production
- E24 - Employment; Unemployment; Wages; Intergenerational Income Distribution; Aggregate Human Capital; Aggregate Labor Productivity
- E25 - Aggregate Factor Income Distribution
- Browse content in E3 - Prices, Business Fluctuations, and Cycles
- E30 - General
- E31 - Price Level; Inflation; Deflation
- E32 - Business Fluctuations; Cycles
- E37 - Forecasting and Simulation: Models and Applications
- Browse content in E4 - Money and Interest Rates
- E40 - General
- E41 - Demand for Money
- E42 - Monetary Systems; Standards; Regimes; Government and the Monetary System; Payment Systems
- E43 - Interest Rates: Determination, Term Structure, and Effects
- E44 - Financial Markets and the Macroeconomy
- Browse content in E5 - Monetary Policy, Central Banking, and the Supply of Money and Credit
- E50 - General
- E51 - Money Supply; Credit; Money Multipliers
- E52 - Monetary Policy
- E58 - Central Banks and Their Policies
- Browse content in E6 - Macroeconomic Policy, Macroeconomic Aspects of Public Finance, and General Outlook
- E60 - General
- E62 - Fiscal Policy
- E66 - General Outlook and Conditions
- Browse content in E7 - Macro-Based Behavioral Economics
- E71 - Role and Effects of Psychological, Emotional, Social, and Cognitive Factors on the Macro Economy
- Browse content in F - International Economics
- Browse content in F0 - General
- F00 - General
- Browse content in F1 - Trade
- F10 - General
- F11 - Neoclassical Models of Trade
- F12 - Models of Trade with Imperfect Competition and Scale Economies; Fragmentation
- F13 - Trade Policy; International Trade Organizations
- F14 - Empirical Studies of Trade
- F15 - Economic Integration
- F16 - Trade and Labor Market Interactions
- F18 - Trade and Environment
- Browse content in F2 - International Factor Movements and International Business
- F20 - General
- F21 - International Investment; Long-Term Capital Movements
- F22 - International Migration
- F23 - Multinational Firms; International Business
- Browse content in F3 - International Finance
- F30 - General
- F31 - Foreign Exchange
- F32 - Current Account Adjustment; Short-Term Capital Movements
- F34 - International Lending and Debt Problems
- F35 - Foreign Aid
- F36 - Financial Aspects of Economic Integration
- Browse content in F4 - Macroeconomic Aspects of International Trade and Finance
- F40 - General
- F41 - Open Economy Macroeconomics
- F42 - International Policy Coordination and Transmission
- F43 - Economic Growth of Open Economies
- F44 - International Business Cycles
- Browse content in F5 - International Relations, National Security, and International Political Economy
- F50 - General
- F51 - International Conflicts; Negotiations; Sanctions
- F52 - National Security; Economic Nationalism
- F55 - International Institutional Arrangements
- Browse content in F6 - Economic Impacts of Globalization
- F60 - General
- F61 - Microeconomic Impacts
- F63 - Economic Development
- Browse content in G - Financial Economics
- Browse content in G0 - General
- G00 - General
- G01 - Financial Crises
- G02 - Behavioral Finance: Underlying Principles
- Browse content in G1 - General Financial Markets
- G10 - General
- G11 - Portfolio Choice; Investment Decisions
- G12 - Asset Pricing; Trading volume; Bond Interest Rates
- G14 - Information and Market Efficiency; Event Studies; Insider Trading
- G15 - International Financial Markets
- G18 - Government Policy and Regulation
- G19 - Other
- Browse content in G2 - Financial Institutions and Services
- G20 - General
- G21 - Banks; Depository Institutions; Micro Finance Institutions; Mortgages
- G22 - Insurance; Insurance Companies; Actuarial Studies
- G23 - Non-bank Financial Institutions; Financial Instruments; Institutional Investors
- G24 - Investment Banking; Venture Capital; Brokerage; Ratings and Ratings Agencies
- G28 - Government Policy and Regulation
- Browse content in G3 - Corporate Finance and Governance
- G30 - General
- G31 - Capital Budgeting; Fixed Investment and Inventory Studies; Capacity
- G32 - Financing Policy; Financial Risk and Risk Management; Capital and Ownership Structure; Value of Firms; Goodwill
- G33 - Bankruptcy; Liquidation
- G34 - Mergers; Acquisitions; Restructuring; Corporate Governance
- G38 - Government Policy and Regulation
- Browse content in G4 - Behavioral Finance
- G40 - General
- G41 - Role and Effects of Psychological, Emotional, Social, and Cognitive Factors on Decision Making in Financial Markets
- Browse content in G5 - Household Finance
- G50 - General
- G51 - Household Saving, Borrowing, Debt, and Wealth
- Browse content in H - Public Economics
- Browse content in H0 - General
- H00 - General
- Browse content in H1 - Structure and Scope of Government
- H10 - General
- H11 - Structure, Scope, and Performance of Government
- Browse content in H2 - Taxation, Subsidies, and Revenue
- H20 - General
- H21 - Efficiency; Optimal Taxation
- H22 - Incidence
- H23 - Externalities; Redistributive Effects; Environmental Taxes and Subsidies
- H24 - Personal Income and Other Nonbusiness Taxes and Subsidies; includes inheritance and gift taxes
- H25 - Business Taxes and Subsidies
- H26 - Tax Evasion and Avoidance
- Browse content in H3 - Fiscal Policies and Behavior of Economic Agents
- H31 - Household
- Browse content in H4 - Publicly Provided Goods
- H40 - General
- H41 - Public Goods
- H42 - Publicly Provided Private Goods
- H44 - Publicly Provided Goods: Mixed Markets
- Browse content in H5 - National Government Expenditures and Related Policies
- H50 - General
- H51 - Government Expenditures and Health
- H52 - Government Expenditures and Education
- H53 - Government Expenditures and Welfare Programs
- H54 - Infrastructures; Other Public Investment and Capital Stock
- H55 - Social Security and Public Pensions
- H56 - National Security and War
- H57 - Procurement
- Browse content in H6 - National Budget, Deficit, and Debt
- H63 - Debt; Debt Management; Sovereign Debt
- Browse content in H7 - State and Local Government; Intergovernmental Relations
- H70 - General
- H71 - State and Local Taxation, Subsidies, and Revenue
- H73 - Interjurisdictional Differentials and Their Effects
- H75 - State and Local Government: Health; Education; Welfare; Public Pensions
- H76 - State and Local Government: Other Expenditure Categories
- H77 - Intergovernmental Relations; Federalism; Secession
- Browse content in H8 - Miscellaneous Issues
- H81 - Governmental Loans; Loan Guarantees; Credits; Grants; Bailouts
- H83 - Public Administration; Public Sector Accounting and Audits
- H87 - International Fiscal Issues; International Public Goods
- Browse content in I - Health, Education, and Welfare
- Browse content in I0 - General
- I00 - General
- Browse content in I1 - Health
- I10 - General
- I11 - Analysis of Health Care Markets
- I12 - Health Behavior
- I13 - Health Insurance, Public and Private
- I14 - Health and Inequality
- I15 - Health and Economic Development
- I18 - Government Policy; Regulation; Public Health
- Browse content in I2 - Education and Research Institutions
- I20 - General
- I21 - Analysis of Education
- I22 - Educational Finance; Financial Aid
- I23 - Higher Education; Research Institutions
- I24 - Education and Inequality
- I25 - Education and Economic Development
- I26 - Returns to Education
- I28 - Government Policy
- Browse content in I3 - Welfare, Well-Being, and Poverty
- I30 - General
- I31 - General Welfare
- I32 - Measurement and Analysis of Poverty
- I38 - Government Policy; Provision and Effects of Welfare Programs
- Browse content in J - Labor and Demographic Economics
- Browse content in J0 - General
- J00 - General
- J01 - Labor Economics: General
- J08 - Labor Economics Policies
- Browse content in J1 - Demographic Economics
- J10 - General
- J12 - Marriage; Marital Dissolution; Family Structure; Domestic Abuse
- J13 - Fertility; Family Planning; Child Care; Children; Youth
- J14 - Economics of the Elderly; Economics of the Handicapped; Non-Labor Market Discrimination
- J15 - Economics of Minorities, Races, Indigenous Peoples, and Immigrants; Non-labor Discrimination
- J16 - Economics of Gender; Non-labor Discrimination
- J18 - Public Policy
- Browse content in J2 - Demand and Supply of Labor
- J20 - General
- J21 - Labor Force and Employment, Size, and Structure
- J22 - Time Allocation and Labor Supply
- J23 - Labor Demand
- J24 - Human Capital; Skills; Occupational Choice; Labor Productivity
- Browse content in J3 - Wages, Compensation, and Labor Costs
- J30 - General
- J31 - Wage Level and Structure; Wage Differentials
- J33 - Compensation Packages; Payment Methods
- J38 - Public Policy
- Browse content in J4 - Particular Labor Markets
- J40 - General
- J42 - Monopsony; Segmented Labor Markets
- J44 - Professional Labor Markets; Occupational Licensing
- J45 - Public Sector Labor Markets
- J48 - Public Policy
- J49 - Other
- Browse content in J5 - Labor-Management Relations, Trade Unions, and Collective Bargaining
- J50 - General
- J51 - Trade Unions: Objectives, Structure, and Effects
- J53 - Labor-Management Relations; Industrial Jurisprudence
- Browse content in J6 - Mobility, Unemployment, Vacancies, and Immigrant Workers
- J60 - General
- J61 - Geographic Labor Mobility; Immigrant Workers
- J62 - Job, Occupational, and Intergenerational Mobility
- J63 - Turnover; Vacancies; Layoffs
- J64 - Unemployment: Models, Duration, Incidence, and Job Search
- J65 - Unemployment Insurance; Severance Pay; Plant Closings
- J68 - Public Policy
- Browse content in J7 - Labor Discrimination
- J71 - Discrimination
- J78 - Public Policy
- Browse content in J8 - Labor Standards: National and International
- J81 - Working Conditions
- J88 - Public Policy
- Browse content in K - Law and Economics
- Browse content in K0 - General
- K00 - General
- Browse content in K1 - Basic Areas of Law
- K14 - Criminal Law
- K2 - Regulation and Business Law
- Browse content in K3 - Other Substantive Areas of Law
- K31 - Labor Law
- Browse content in K4 - Legal Procedure, the Legal System, and Illegal Behavior
- K40 - General
- K41 - Litigation Process
- K42 - Illegal Behavior and the Enforcement of Law
- Browse content in L - Industrial Organization
- Browse content in L0 - General
- L00 - General
- Browse content in L1 - Market Structure, Firm Strategy, and Market Performance
- L10 - General
- L11 - Production, Pricing, and Market Structure; Size Distribution of Firms
- L13 - Oligopoly and Other Imperfect Markets
- L14 - Transactional Relationships; Contracts and Reputation; Networks
- L15 - Information and Product Quality; Standardization and Compatibility
- L16 - Industrial Organization and Macroeconomics: Industrial Structure and Structural Change; Industrial Price Indices
- L19 - Other
- Browse content in L2 - Firm Objectives, Organization, and Behavior
- L21 - Business Objectives of the Firm
- L22 - Firm Organization and Market Structure
- L23 - Organization of Production
- L24 - Contracting Out; Joint Ventures; Technology Licensing
- L25 - Firm Performance: Size, Diversification, and Scope
- L26 - Entrepreneurship
- Browse content in L3 - Nonprofit Organizations and Public Enterprise
- L33 - Comparison of Public and Private Enterprises and Nonprofit Institutions; Privatization; Contracting Out
- Browse content in L4 - Antitrust Issues and Policies
- L40 - General
- L41 - Monopolization; Horizontal Anticompetitive Practices
- L42 - Vertical Restraints; Resale Price Maintenance; Quantity Discounts
- Browse content in L5 - Regulation and Industrial Policy
- L50 - General
- L51 - Economics of Regulation
- Browse content in L6 - Industry Studies: Manufacturing
- L60 - General
- L62 - Automobiles; Other Transportation Equipment; Related Parts and Equipment
- L63 - Microelectronics; Computers; Communications Equipment
- L66 - Food; Beverages; Cosmetics; Tobacco; Wine and Spirits
- Browse content in L7 - Industry Studies: Primary Products and Construction
- L71 - Mining, Extraction, and Refining: Hydrocarbon Fuels
- L73 - Forest Products
- Browse content in L8 - Industry Studies: Services
- L81 - Retail and Wholesale Trade; e-Commerce
- L83 - Sports; Gambling; Recreation; Tourism
- L84 - Personal, Professional, and Business Services
- L86 - Information and Internet Services; Computer Software
- Browse content in L9 - Industry Studies: Transportation and Utilities
- L91 - Transportation: General
- L93 - Air Transportation
- L94 - Electric Utilities
- Browse content in M - Business Administration and Business Economics; Marketing; Accounting; Personnel Economics
- Browse content in M1 - Business Administration
- M11 - Production Management
- M12 - Personnel Management; Executives; Executive Compensation
- M14 - Corporate Culture; Social Responsibility
- Browse content in M2 - Business Economics
- M21 - Business Economics
- Browse content in M3 - Marketing and Advertising
- M31 - Marketing
- M37 - Advertising
- Browse content in M4 - Accounting and Auditing
- M42 - Auditing
- M48 - Government Policy and Regulation
- Browse content in M5 - Personnel Economics
- M50 - General
- M51 - Firm Employment Decisions; Promotions
- M52 - Compensation and Compensation Methods and Their Effects
- M53 - Training
- M54 - Labor Management
- Browse content in N - Economic History
- Browse content in N0 - General
- N00 - General
- N01 - Development of the Discipline: Historiographical; Sources and Methods
- Browse content in N1 - Macroeconomics and Monetary Economics; Industrial Structure; Growth; Fluctuations
- N10 - General, International, or Comparative
- N11 - U.S.; Canada: Pre-1913
- N12 - U.S.; Canada: 1913-
- N13 - Europe: Pre-1913
- N17 - Africa; Oceania
- Browse content in N2 - Financial Markets and Institutions
- N20 - General, International, or Comparative
- N22 - U.S.; Canada: 1913-
- N23 - Europe: Pre-1913
- Browse content in N3 - Labor and Consumers, Demography, Education, Health, Welfare, Income, Wealth, Religion, and Philanthropy
- N30 - General, International, or Comparative
- N31 - U.S.; Canada: Pre-1913
- N32 - U.S.; Canada: 1913-
- N33 - Europe: Pre-1913
- N34 - Europe: 1913-
- N36 - Latin America; Caribbean
- N37 - Africa; Oceania
- Browse content in N4 - Government, War, Law, International Relations, and Regulation
- N40 - General, International, or Comparative
- N41 - U.S.; Canada: Pre-1913
- N42 - U.S.; Canada: 1913-
- N43 - Europe: Pre-1913
- N44 - Europe: 1913-
- N45 - Asia including Middle East
- N47 - Africa; Oceania
- Browse content in N5 - Agriculture, Natural Resources, Environment, and Extractive Industries
- N50 - General, International, or Comparative
- N51 - U.S.; Canada: Pre-1913
- Browse content in N6 - Manufacturing and Construction
- N63 - Europe: Pre-1913
- Browse content in N7 - Transport, Trade, Energy, Technology, and Other Services
- N71 - U.S.; Canada: Pre-1913
- Browse content in N8 - Micro-Business History
- N82 - U.S.; Canada: 1913-
- Browse content in N9 - Regional and Urban History
- N91 - U.S.; Canada: Pre-1913
- N92 - U.S.; Canada: 1913-
- N93 - Europe: Pre-1913
- N94 - Europe: 1913-
- Browse content in O - Economic Development, Innovation, Technological Change, and Growth
- Browse content in O1 - Economic Development
- O10 - General
- O11 - Macroeconomic Analyses of Economic Development
- O12 - Microeconomic Analyses of Economic Development
- O13 - Agriculture; Natural Resources; Energy; Environment; Other Primary Products
- O14 - Industrialization; Manufacturing and Service Industries; Choice of Technology
- O15 - Human Resources; Human Development; Income Distribution; Migration
- O16 - Financial Markets; Saving and Capital Investment; Corporate Finance and Governance
- O17 - Formal and Informal Sectors; Shadow Economy; Institutional Arrangements
- O18 - Urban, Rural, Regional, and Transportation Analysis; Housing; Infrastructure
- O19 - International Linkages to Development; Role of International Organizations
- Browse content in O2 - Development Planning and Policy
- O23 - Fiscal and Monetary Policy in Development
- O25 - Industrial Policy
- Browse content in O3 - Innovation; Research and Development; Technological Change; Intellectual Property Rights
- O30 - General
- O31 - Innovation and Invention: Processes and Incentives
- O32 - Management of Technological Innovation and R&D
- O33 - Technological Change: Choices and Consequences; Diffusion Processes
- O34 - Intellectual Property and Intellectual Capital
- O38 - Government Policy
- Browse content in O4 - Economic Growth and Aggregate Productivity
- O40 - General
- O41 - One, Two, and Multisector Growth Models
- O43 - Institutions and Growth
- O44 - Environment and Growth
- O47 - Empirical Studies of Economic Growth; Aggregate Productivity; Cross-Country Output Convergence
- Browse content in O5 - Economywide Country Studies
- O52 - Europe
- O53 - Asia including Middle East
- O55 - Africa
- Browse content in P - Economic Systems
- Browse content in P0 - General
- P00 - General
- Browse content in P1 - Capitalist Systems
- P10 - General
- P16 - Political Economy
- P17 - Performance and Prospects
- P18 - Energy: Environment
- Browse content in P2 - Socialist Systems and Transitional Economies
- P26 - Political Economy; Property Rights
- Browse content in P3 - Socialist Institutions and Their Transitions
- P37 - Legal Institutions; Illegal Behavior
- Browse content in P4 - Other Economic Systems
- P48 - Political Economy; Legal Institutions; Property Rights; Natural Resources; Energy; Environment; Regional Studies
- Browse content in P5 - Comparative Economic Systems
- P51 - Comparative Analysis of Economic Systems
- Browse content in Q - Agricultural and Natural Resource Economics; Environmental and Ecological Economics
- Browse content in Q1 - Agriculture
- Q10 - General
- Q12 - Micro Analysis of Farm Firms, Farm Households, and Farm Input Markets
- Q13 - Agricultural Markets and Marketing; Cooperatives; Agribusiness
- Q14 - Agricultural Finance
- Q15 - Land Ownership and Tenure; Land Reform; Land Use; Irrigation; Agriculture and Environment
- Q16 - R&D; Agricultural Technology; Biofuels; Agricultural Extension Services
- Browse content in Q2 - Renewable Resources and Conservation
- Q25 - Water
- Browse content in Q3 - Nonrenewable Resources and Conservation
- Q32 - Exhaustible Resources and Economic Development
- Q34 - Natural Resources and Domestic and International Conflicts
- Browse content in Q4 - Energy
- Q41 - Demand and Supply; Prices
- Q48 - Government Policy
- Browse content in Q5 - Environmental Economics
- Q50 - General
- Q51 - Valuation of Environmental Effects
- Q53 - Air Pollution; Water Pollution; Noise; Hazardous Waste; Solid Waste; Recycling
- Q54 - Climate; Natural Disasters; Global Warming
- Q56 - Environment and Development; Environment and Trade; Sustainability; Environmental Accounts and Accounting; Environmental Equity; Population Growth
- Q58 - Government Policy
- Browse content in R - Urban, Rural, Regional, Real Estate, and Transportation Economics
- Browse content in R0 - General
- R00 - General
- Browse content in R1 - General Regional Economics
- R11 - Regional Economic Activity: Growth, Development, Environmental Issues, and Changes
- R12 - Size and Spatial Distributions of Regional Economic Activity
- R13 - General Equilibrium and Welfare Economic Analysis of Regional Economies
- Browse content in R2 - Household Analysis
- R20 - General
- R23 - Regional Migration; Regional Labor Markets; Population; Neighborhood Characteristics
- R28 - Government Policy
- Browse content in R3 - Real Estate Markets, Spatial Production Analysis, and Firm Location
- R30 - General
- R31 - Housing Supply and Markets
- R38 - Government Policy
- Browse content in R4 - Transportation Economics
- R40 - General
- R41 - Transportation: Demand, Supply, and Congestion; Travel Time; Safety and Accidents; Transportation Noise
- R48 - Government Pricing and Policy
- Browse content in Z - Other Special Topics
- Browse content in Z1 - Cultural Economics; Economic Sociology; Economic Anthropology
- Z10 - General
- Z12 - Religion
- Z13 - Economic Sociology; Economic Anthropology; Social and Economic Stratification
- Advance Articles
- Editor's Choice
- Author Guidelines
- Submission Site
- Open Access Options
- Self-Archiving Policy
- Why Submit?
- About The Quarterly Journal of Economics
- Editorial Board
- Advertising and Corporate Services
- Journals Career Network
- Dispatch Dates
- Journals on Oxford Academic
- Books on Oxford Academic
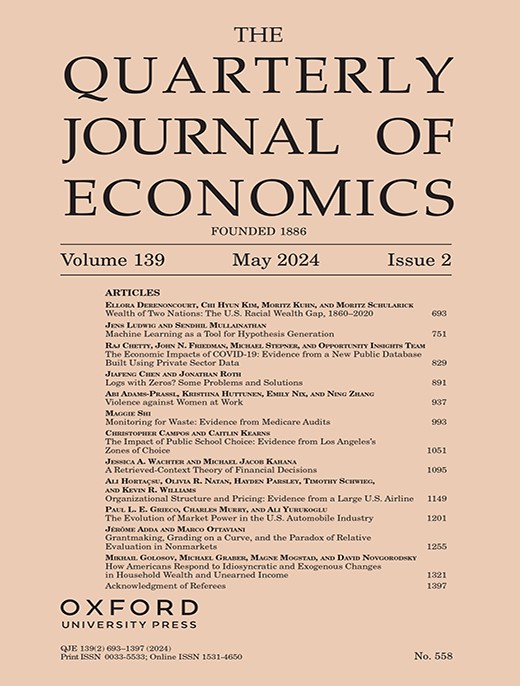
Article Contents
I. introduction, ii. a simple framework for discovery, iii. application and data, iv. the surprising importance of the face, v. algorithm-human communication, vi. evaluating these new hypotheses, vii. conclusion, data availability.
- < Previous
Machine Learning as a Tool for Hypothesis Generation *
- Article contents
- Figures & tables
- Supplementary Data
Jens Ludwig, Sendhil Mullainathan, Machine Learning as a Tool for Hypothesis Generation, The Quarterly Journal of Economics , Volume 139, Issue 2, May 2024, Pages 751–827, https://doi.org/10.1093/qje/qjad055
- Permissions Icon Permissions
While hypothesis testing is a highly formalized activity, hypothesis generation remains largely informal. We propose a systematic procedure to generate novel hypotheses about human behavior, which uses the capacity of machine learning algorithms to notice patterns people might not. We illustrate the procedure with a concrete application: judge decisions about whom to jail. We begin with a striking fact: the defendant’s face alone matters greatly for the judge’s jailing decision. In fact, an algorithm given only the pixels in the defendant’s mug shot accounts for up to half of the predictable variation. We develop a procedure that allows human subjects to interact with this black-box algorithm to produce hypotheses about what in the face influences judge decisions. The procedure generates hypotheses that are both interpretable and novel: they are not explained by demographics (e.g., race) or existing psychology research, nor are they already known (even if tacitly) to people or experts. Though these results are specific, our procedure is general. It provides a way to produce novel, interpretable hypotheses from any high-dimensional data set (e.g., cell phones, satellites, online behavior, news headlines, corporate filings, and high-frequency time series). A central tenet of our article is that hypothesis generation is a valuable activity, and we hope this encourages future work in this largely “prescientific” stage of science.
Science is curiously asymmetric. New ideas are meticulously tested using data, statistics, and formal models. Yet those ideas originate in a notably less meticulous process involving intuition, inspiration, and creativity. The asymmetry between how ideas are generated versus tested is noteworthy because idea generation is also, at its core, an empirical activity. Creativity begins with “data” (albeit data stored in the mind), which are then “analyzed” (through a purely psychological process of pattern recognition). What feels like inspiration is actually the output of a data analysis run by the human brain. Despite this, idea generation largely happens off stage, something that typically happens before “actual science” begins. 1 Things are likely this way because there is no obvious alternative. The creative process is so human and idiosyncratic that it would seem to resist formalism.
That may be about to change because of two developments. First, human cognition is no longer the only way to notice patterns in the world. Machine learning algorithms can also find patterns, including patterns people might not notice themselves. These algorithms can work not just with structured, tabular data but also with the kinds of inputs that traditionally could only be processed by the mind, like images or text. Second, data on human behavior is exploding: second-by-second price and volume data in asset markets, high-frequency cellphone data on location and usage, CCTV camera and police bodycam footage, news stories, children’s books, the entire text of corporate filings, and so on. The kind of information researchers once relied on for inspiration is now machine readable: what was once solely mental data is increasingly becoming actual data. 2
We suggest that these changes can be leveraged to expand how hypotheses are generated. Currently, researchers do of course look at data to generate hypotheses, as in exploratory data analysis, but this depends on the idiosyncratic creativity of investigators who must decide what statistics to calculate. In contrast, we suggest capitalizing on the capacity of machine learning algorithms to automatically detect patterns, especially ones people might never have considered. A key challenge is that we require hypotheses that are interpretable to people. One important goal of science is to generalize knowledge to new contexts. Predictive patterns in a single data set alone are rarely useful; they become insightful when they can be generalized. Currently, that generalization is done by people, and people can only generalize things they understand. The predictors produced by machine learning algorithms are, however, notoriously opaque—hard-to-decipher “black boxes.” We propose a procedure that integrates these algorithms into a pipeline that results in human-interpretable hypotheses that are both novel and testable.
While our procedure is broadly applicable, we illustrate it in a concrete application: judicial decision making. Specifically we study pretrial decisions about which defendants are jailed versus set free awaiting trial, a decision that by law is supposed to hinge on a prediction of the defendant’s risk ( Dobbie and Yang 2021 ). 3 This is also a substantively interesting application in its own right because of the high stakes involved and mounting evidence that judges make these decisions less than perfectly ( Kleinberg et al. 2018 ; Rambachan et al. 2021 ; Angelova, Dobbie, and Yang 2023 ).
We begin with a striking fact. When we build a deep learning model of the judge—one that predicts whether the judge will detain a given defendant—a single factor emerges as having large explanatory power: the defendant’s face. A predictor that uses only the pixels in the defendant’s mug shot explains from one-quarter to nearly one-half of the predictable variation in detention. 4 Defendants whose mug shots fall in the bottom quartile of predicted detention are 20.4 percentage points more likely to be jailed than those in the top quartile. By comparison, the difference in detention rates between those arrested for violent versus nonviolent crimes is 4.8 percentage points. Notice what this finding is and is not. We are not claiming the mug shot predicts defendant behavior; that would be the long-discredited field of phrenology ( Schlag 1997 ). We instead claim the mug shot predicts judge behavior: how the defendant looks correlates strongly with whether the judge chooses to jail them. 5
Has the algorithm found something new in the pixels of the mug shot or simply rediscovered something long known or intuitively understood? After all, psychologists have been studying people’s reactions to faces for at least 100 years ( Todorov et al. 2015 ; Todorov and Oh 2021 ), while economists have shown that judges are influenced by factors (like race) that can be seen from someone’s face ( Arnold, Dobbie, and Yang 2018 ; Arnold, Dobbie, and Hull 2020 ). When we control for age, gender, race, skin color, and even the facial features suggested by previous psychology research (dominance, trustworthiness, attractiveness, and competence), none of these factors (individually or jointly) meaningfully diminishes the algorithm’s predictive power (see Figure I , Panel A). It is perhaps worth noting that the algorithm on its own does rediscover some of the signal from these features: in fact, collectively these known features explain |$22.3\%$| of the variation in predicted detention (see Figure I , Panel B). The key point is that the algorithm has discovered a great deal more as well.

Correlates of Judge Detention Decision and Algorithmic Prediction of Judge Decision
Panel A summarizes the explanatory power of a regression model in explaining judge detention decisions, controlling for the different explanatory variables indicated at left (shaded tiles), either on their own (dark circles) or together with the algorithmic prediction of the judge decisions (triangles). Each row represents a different regression specification. By “other facial features,” we mean variables that previous psychology research suggests matter for how faces influence people’s reactions to others (dominance, trustworthiness, competence, and attractiveness). Ninety-five percent confidence intervals around our R 2 estimates come from drawing 10,000 bootstrap samples from the validation data set. Panel B shows the relationship between the different explanatory variables as indicated at left by the shaded tiles with the algorithmic prediction itself as the outcome variable in the regressions. Panel C examines the correlation with judge decisions of the two novel hypotheses generated by our procedure about what facial features affect judge detention decisions: well-groomed and heavy-faced.
Perhaps we should control for something else? Figuring out that “something else” is itself a form of hypothesis generation. To avoid a possibly endless—and misleading—process of generating other controls, we take a different approach. We show mug shots to subjects and ask them to guess whom the judge will detain and incentivize them for accuracy. These guesses summarize the facial features people readily (if implicitly) believe influence jailing. Although subjects are modestly good at this task, the algorithm is much better. It remains highly predictive even after controlling for these guesses. The algorithm seems to have found something novel beyond what scientists have previously hypothesized and beyond whatever patterns people can even recognize in data (whether or not they can articulate them).
What, then, are the novel facial features the algorithm has discovered? If we are unable to answer that question, we will have simply replaced one black box (the judge’s mind) with another (an algorithmic model of the judge’s mind). We propose a solution whereby the algorithm can communicate what it “sees.” Specifically, our procedure begins with a mug shot and “morphs” it to create a mug shot that maximally increases (or decreases) the algorithm’s predicted detention probability. The result is pairs of synthetic mug shots that can be examined to understand and articulate what differs within the pairs. The algorithm discovers, and people name that discovery. In principle we could have just shown subjects actual mug shots with higher versus lower predicted detention odds. But faces are so rich that between any pair of actual mug shots, many things will happen to be different and most will be unrelated to detention (akin to the curse of dimensionality). Simply looking at pairs of actual faces can, as a result, lead to many spurious observations. Morphing creates counterfactual synthetic images that are as similar as possible except with respect to detention odds, to minimize extraneous differences and help focus on what truly matters for judge detention decisions.
Importantly, we do not generate hypotheses by looking at the morphs ourselves; instead, they are shown to independent study subjects (MTurk or Prolific workers) in an experimental design. Specifically, we showed pairs of morphed images and asked participants to guess which image the algorithm predicts to have higher detention risk. Subjects were given both incentives and feedback, so they had motivation and opportunity to learn the underlying patterns. While subjects initially guess the judge’s decision correctly from these morphed mug shots at about the same rate as they do when looking at “raw data,” that is, actual mug shots (modestly above the |$50\%$| random guessing mark), they quickly learn from these morphed images what the algorithm is seeing and reach an accuracy of nearly |$70\%$| . At the end, participants are asked to put words to the differences they see across images in each pair, that is, to name what they think are the key facial features the algorithm is relying on to predict judge decisions. Comfortingly, there is substantial agreement on what subjects see: a sizable share of subjects all name the same feature. To verify whether the feature they identify is used by the algorithm, a separate sample of subjects independently coded mug shots for this new feature. We show that the new feature is indeed correlated with the algorithm’s predictions. What subjects think they’re seeing is indeed what the algorithm is also “seeing.”
Having discovered a single feature, we can iterate the procedure—the first feature explains only a fraction of what the algorithm has captured, suggesting there are many other factors to be discovered. We again produce morphs, but this time hold the first feature constant: that is, we orthogonalize so that the pairs of morphs do not differ on the first feature. When these new morphs are shown to subjects, they consistently name a second feature, which again correlates with the algorithm’s prediction. Both features are quite important. They explain a far larger share of what the algorithm sees than all the other variables (including race and skin color) besides gender. These results establish our main goals: show that the procedure produces meaningful communication, and that it can be iterated.
What are the two discovered features? The first can be called “well-groomed” (e.g., tidy, clean, groomed, versus unkept, disheveled, sloppy look), and the second can be called “heavy-faced” (e.g., wide facial shape, puffier face, wider face, rounder face, heavier). These features are not just predictive of what the algorithm sees, but also of what judges actually do ( Figure I , Panel C). We find that both well-groomed and heavy-faced defendants are more likely to be released, even controlling for demographic features and known facial features from psychology. Detention rates of defendants in the top and bottom quartile of well-groomedness differ by 5.5 percentage points ( |$24\%$| of the base rate), while the top versus bottom quartile difference in heavy-facedness is 7 percentage points (about |$30\%$| of the base rate). Both differences are larger than the 4.8 percentage points detention rate difference between those arrested for violent versus nonviolent crimes. Not only are these magnitudes substantial, these hypotheses are novel even to practitioners who work in the criminal justice system (in a public defender’s office and a legal aid society).
Establishing whether these hypotheses are truly causally related to judge decisions is obviously beyond the scope of the present article. But we nonetheless present a few additional findings that are at least suggestive. These novel features do not appear to be simply proxies for factors like substance abuse, mental health, or socioeconomic status. Moreover, we carried out a lab experiment in which subjects are asked to make hypothetical pretrial release decisions as if they were a judge. They are shown information about criminal records (current charge, prior arrests) along with mug shots that are randomly morphed in the direction of higher or lower values of well-groomed (or heavy-faced). Subjects tend to detain those with higher-risk structured variables (criminal records), all else equal, suggesting they are taking the task seriously. These same subjects, though, are also more likely to detain defendants who are less heavy-faced or well-groomed, even though these were randomly assigned.
Ultimately, though, this is not a study about well-groomed or heavy-faced defendants, nor are its implications limited to faces or judges. It develops a general procedure that can be applied wherever behavior can be predicted using rich (especially high-dimensional) data. Development of such a procedure has required overcoming two key challenges.
First, to generate interpretable hypotheses, we must overcome the notorious black box nature of most machine learning algorithms. Unlike with a regression, one cannot simply inspect the coefficients. A modern deep-learning algorithm, for example, can have tens of millions of parameters. Noninspectability is especially problematic when the data are rich and high dimensional since the parameters are associated with primitives such as pixels. This problem of interpretation is fundamental and remains an active area of research. 6 Part of our procedure here draws on the recent literature in computer science that uses generative models to create counterfactual explanations. Most of those methods are designed for AI applications that seek to automate tasks humans do nearly perfectly, like image classification, where predictability of the outcome (is this image of a dog or a cat?) is typically quite high. 7 Interpretability techniques are used to ensure the algorithm is not picking up on spurious signal. 8 We developed our method, which has similar conceptual underpinnings to this existing literature, for social science applications where the outcome (human behavior) is typically more challenging to predict. 9 To what degree existing methods (as they currently stand or with some modification) could perform as well or better in social science applications like ours is a question we leave to future work.
Second, we must overcome what we might call the Rorschach test problem. Suppose we, the authors, were to look at these morphs and generate a hypothesis. We would not know if the procedure played any meaningful role. Perhaps the morphs, like ink blots, are merely canvases onto which we project our creativity. 10 Put differently, a single research team’s idiosyncratic judgments lack the kind of replicability we desire of a scientific procedure. To overcome this problem, it is key that we use independent (nonresearcher) subjects to inspect the morphs. The fact that a sizable share of subjects all name the same discovery suggests that human-algorithm communication has occurred and the procedure is replicable, rather than reflecting some unique spark of creativity.
At the same time, the fact that our procedure is not fully automatic implies that it will be shaped and constrained by people. Human participants are needed to name the discoveries. So whole new concepts that humans do not yet understand cannot be produced. Such breakthroughs clearly happen (e.g., gravity or probability) but are beyond the scope of procedures like ours. People also play a crucial role in curating the data the algorithm sees. Here, for example, we chose to include mug shots. The creative acquisition of rich data is an important human input into this hypothesis generation procedure. 11
Our procedure can be applied to a broad range of settings and will be particularly useful for data that are not already intrinsically interpretable. Many data sets contain a few variables that already have clear, fixed meanings and are unlikely to lead to novel discoveries. In contrast, images, text, and time series are rich high-dimensional data with many possible interpretations. Just as there is an ocean of plausible facial features, these sorts of data contain a large set of potential hypotheses that an algorithm can search through. Such data are increasingly available and used by economists, including news headlines, legislative deliberations, annual corporate reports, Federal Open Market Committee statements, Google searches, student essays, résumés, court transcripts, doctors’ notes, satellite images, housing photos, and medical images. Our procedure could, for example, raise hypotheses about what kinds of news lead to over- or underreaction of stock prices, which features of a job interview increase racial disparities, or what features of an X-ray drive misdiagnosis.
Central to this work is the belief that hypothesis generation is a valuable activity in and of itself. Beyond whatever the value might be of our specific procedure and empirical application, we hope these results also inspire greater attention to this traditionally “prescientific” stage of science.
We develop a simple framework to clarify the goals of hypothesis generation and how it differs from testing, how algorithms might help, and how our specific approach to algorithmic hypothesis generation differs from existing methods. 12
II.A. The Goals of Hypothesis Generation
What criteria should we use for assessing hypothesis generation procedures? Two common goals for hypothesis generation are ones that we ensure ex post. First is novelty. In our application, we aim to orthogonalize against known factors, recognizing that it may be hard to orthogonalize against all known hypotheses. Second, we require that hypotheses be testable ( Popper 2002 ). But what can be tested is hard to define ex ante, in part because it depends on the specific hypothesis and the potential experimental setups. Creative empiricists over time often find ways to test hypotheses that previously seemed untestable. 13 To these, we add two more: interpretability and empirical plausibility.
What do we mean by empirically plausible? Let y be some outcome of interest, which for simplicity we assume is binary, and let h ( x ) be some hypothesis that maps the features of each instance, x , to [0,1]. By empirical plausibility we mean some correlation between y and h ( x ). Our ultimate aim is to uncover causal relationships. But causality can only be known after causal testing. That raises the question of how to come up with ideas worth causally testing, and how we would recognize them when we see them. Many true hypotheses need not be visible in raw correlations. Those can only be identified with background knowledge (e.g., theory). Other procedures would be required to surface those. Our focus here is on searching for true hypotheses that are visible in raw correlations. Of course not every correlation will turn out to be a true hypothesis, but even in those cases, generating such hypotheses and then invalidating them can be a valuable activity. Debunking spurious correlations has long been one of the most useful roles of empirical work. Understanding what confounders produce those correlations can also be useful.
We care about our final goal for hypothesis generation, interpretability, because science is largely about helping people make forecasts into new contexts, and people can only do that with hypotheses they meaningfully understand. Consider an uninterpretable hypothesis like “this set of defendants is more likely to be jailed than that set,” but we cannot articulate a reason why. From that hypothesis, nothing could be said about a new set of courtroom defendants. In contrast an interpretable hypothesis like “skin color affects detention” has implications for other samples of defendants and for entirely different settings. We could ask whether skin color also affects, say, police enforcement choices or whether these effects differ by time of day. By virtue of being interpretable, these hypotheses let us use a wider set of knowledge (police may share racial biases; skin color is not as easily detected at night). 14 Interpretable descriptions let us generalize to novel situations, in addition to being easier to communicate to key stakeholders and lending themselves to interpretable solutions.
II.B. Human versus Algorithmic Hypothesis Generation
Human hypothesis generation has the advantage of generating hypotheses that are interpretable. By construction, the ideas that humans come up with are understandable by humans. But as a procedure for generating new ideas, human creativity has the drawback of often being idiosyncratic and not necessarily replicable. A novel hypothesis is novel exactly because one person noticed it when many others did not. A large body of evidence shows that human judgments have a great deal of “noise.” It is not just that different people draw different conclusions from the same observations, but the same person may notice different things at different times ( Kahneman, Sibony, and Sunstein 2022 ). A large body of psychology research shows that people typically are not able to introspect and understand why we notice specific things those times we do notice them. 15
There is also no guarantee that human-generated hypotheses need be empirically plausible. The intuition is related to “overfitting.” Suppose that people look at a subset of all data and look for something that differentiates positive ( y = 1) from negative ( y = 0) cases. Even with no noise in y , there is randomness in which observations are in the data. That can lead to idiosyncratic differences between y = 0 and y = 1 cases. As the number of comprehensible hypotheses gets large, there is a “curse of dimensionality”: many plausible hypotheses for these idiosyncratic differences. That is, many different hypotheses can look good in sample but need not work out of sample. 16
In contrast, supervised learning tools in machine learning are designed to generate predictions in new (out-of-sample) data. 17 That is, algorithms generate hypotheses that are empirically plausible by construction. 18 Moreover, machine learning can detect patterns in data that humans cannot. Algorithms can notice, for example, that livestock all tend to be oriented north ( Begall et al. 2008 ), whether someone is about to have a heart attack based on subtle indications in an electrocardiogram ( Mullainathan and Obermeyer 2022 ), or that a piece of machinery is about to break ( Mobley 2002 ). We call these machine learning prediction functions m ( x ), which for a binary outcome y map to [0, 1].
The challenge is that most m ( x ) are not interpretable. For this type of statistical model to yield an interpretable hypothesis, its parameters must be interpretable. That can happen in some simple cases. For example, if we had a data set where each dimension of x was interpretable (such as individual structured variables in a tabular data set) and we used a predictor such as OLS (or LASSO), we could just read the hypotheses from the nonzero coefficients: which variables are significant? Even in that case, interpretation is challenging because machine learning tools, built to generate accurate predictions rather than apportion explanatory power across explanatory variables, yield coefficients that can be unstable across realizations of the data ( Mullainathan and Spiess 2017 ). 19 Often interpretation is much less straightforward than that. If x is an image, text, or time series, the estimated models (such as convolutional neural networks) can have literally millions of parameters. The models are defined on granular inputs with no particular meaning: if we knew m ( x ) weighted a particular pixel, what have we learned? In these cases, the estimated model m ( x ) is not interpretable. Our focus is on these contexts where algorithms, as black-box models, are not readily interpreted.
Ideally one might marry people’s unique knowledge of what is comprehensible with an algorithm’s superior capacity to find meaningful correlations in data: to have the algorithm discover new signal and then have humans name that discovery. How to do so is not straightforward. We might imagine formalizing the set of interpretable prediction functions, and then focus on creating machine learning techniques that search over functions in that set. But mathematically characterizing those functions is typically not possible. Or we might consider seeking insight from a low-dimensional representation of face space, or “eigenfaces,” which are a common teaching tool for principal components analysis ( Sirovich and Kirby 1987 ). But those turn out not to provide much useful insight for our purposes. 20 In some sense it is obvious why: the subset of actual faces is unlikely to be a linear subspace of the space of pixels. If we took two faces and linearly interpolated them the resulting image would not look like a face. Some other approach is needed. We build on methods in computer science that use generative models to generate counterfactual explanations.
II.C. Related Methods
Our hypothesis generation procedure is part of a growing literature that aims to integrate machine learning into the way science is conducted. A common use (outside of economics) is in what could be called “closed world problems”: situations where the fundamental laws are known, but drawing out predictions is computationally hard. For example, the biochemical rules of how proteins fold are known, but it is hard to predict the final shape of a protein. Machine learning has provided fundamental breakthroughs, in effect by making very hard-to-compute outcomes computable in a feasible timeframe. 21
Progress has been far more limited with applications where the relationship between x and y is unknown (“open world” problems), like human behavior. First, machine learning here has been useful at generating unexpected findings, although these are not hypotheses themselves. Pierson et al. (2021) show that a deep-learning algorithm is better able to predict patient pain from an X-ray than clinicians can: there are physical knee defects that medicine currently does not understand. But that study is not able to isolate what those defects are. 22 Second, machine learning has also been used to explore investigator-generated hypotheses, such as Mullainathan and Obermeyer (2022) , who examine whether physicians suffer from limited attention when diagnosing patients. 23
Finally, a few papers take on the same problem that we do. Fudenberg and Liang (2019) and Peterson et al. (2021) have used algorithms to predict play in games and choices between lotteries. They inspected those algorithms to produce their insights. Similarly, Kleinberg et al. (2018) and Sunstein (2021) use algorithmic models of judges and inspect those models to generate hypotheses. 24 Our proposal builds on these papers. Rather than focusing on generating an insight for a specific application, we suggest a procedure that can be broadly used for many applications. Importantly, our procedure does not rely on researcher inspection of algorithmic output. When an expert researcher with a track record of generating scientific ideas uses some procedure to generate an idea, how do we know whether the result is due to the procedure or the researcher? By relying on a fixed algorithmic procedure that human subjects can interface with, hypothesis generation goes from being an idiosyncratic act of individuals to a replicable process.
III.A. Judicial Decision Making
Although our procedure is broadly applicable, we illustrate it through a specific application to the U.S. criminal justice system. We choose this application partly because of its social relevance. It is also an exemplar of the type of application where our hypothesis generation procedure can be helpful. Its key ingredients—a clear decision maker, a large number of choices (over 10 million people are arrested each year in the United States) that are recorded in data, and, increasingly, high-dimensional data that can also be used to model those choices, such as mug shot images, police body cameras, and text from arrest reports or court transcripts—are shared with a variety of other applications.
Our specific focus is on pretrial hearings. Within 24–48 hours after arrest, a judge must decide where the defendant will await trial, in jail or at home. This is a consequential decision. Cases typically take 2–4 months to resolve, sometimes up to 9–12 months. Jail affects people’s families, their livelihoods, and the chances of a guilty plea ( Dobbie, Goldin, and Yang 2018 ). On the other hand, someone who is released could potentially reoffend. 25
While pretrial decisions are by law supposed to hinge on the defendant’s risk of flight or rearrest if released ( Dobbie and Yang 2021 ), studies show that judges’ decisions deviate from those guidelines in a number of ways. For starters, judges seem to systematically mispredict defendant risk ( Jung et al. 2017 ; Kleinberg et al. 2018 ; Rambachan 2021 ; Angelova, Dobbie, and Yang 2023 ), partly because judges overweight the charge for which people are arrested ( Sunstein 2021 ). Judge decisions can also depend on extralegal factors like race ( Arnold, Dobbie, and Yang 2018 ; Arnold, Dobbie, and Hull 2020 ), whether the judge’s favorite football team lost ( Eren and Mocan 2018 ), weather ( Heyes and Saberian 2019 ), the cases the judge just heard ( Chen, Moskowitz, and Shue 2016 ), and if the hearing is on the defendant’s birthday ( Chen and Philippe 2023 ). These studies test hypotheses that some human being was clever enough to think up. But there remains a great deal of unexplained variation in judges’ decisions. The challenge of expanding the set of hypotheses for understanding this variation without losing the benefit of interpretability is the motivation for our own analysis here.
III.B. Administrative Data
We obtained data from Mecklenburg County, North Carolina, the second most populated county in the state (over 1 million residents) that includes North Carolina’s largest city (Charlotte). The county is similar to the rest of the United States in terms of economic conditions (2021 poverty rates were |$11.0\%$| versus |$11.4\%$| , respectively), although the share of Mecklenburg County’s population that is non-Hispanic white is lower than the United States as a whole ( |$56.6\%$| versus |$75.8\%$| ). 26 We rely on three sources of administrative data: 27
The Mecklenburg County Sheriff’s Office (MCSO) publicly posts arrest data for the past three years, which provides information on defendant demographics like age, gender, and race, as well as the charge for which someone was arrested.
The North Carolina Administrative Office of the Courts (NCAOC) maintains records on the judge’s pretrial decisions (detain, release, etc.).
Data from the North Carolina Department of Public Safety includes information about the defendant’s prior convictions and incarceration spells, if any.
We also downloaded photos of the defendants from the MCSO public website (so-called mug shots), 28 which capture a frontal view of each person from the shoulders up in front of a gray background. These images are 400 pixels wide by 480 pixels high, but we pad them with a black boundary to be square 512 × 512 images to conform with the requirements of some of the machine learning tools. In Figure II , we give readers a sense of what these mug shots look like, with two important caveats. First, given concerns about how the overrepresentation of disadvantaged groups in discussions of crime can contribute to stereotyping ( Bjornstrom et al. 2010 ), we illustrate the key ideas of the paper using images for non-Hispanic white males. Second, out of sensitivity to actual arrestees, we do not wish to display actual mug shots (which are available at the MCSO website). 29 Instead, the article only shows mug shots that are synthetic, generated using generative adversarial networks as described in Section V.B .

Illustrative Facial Images
This figure shows facial images that illustrate the format of the mug shots posted publicly on the Mecklenberg County, North Carolina, sheriff’s office website. These are not real mug shots of actual people who have been arrested, but are synthetic. Moreover, given concerns about how the overrepresentation of disadvantaged groups in discussions of crime can exacerbate stereotyping, we illustrate the our key ideas using images for non-Hispanic white men. However, in our human intelligence tasks that ask participants to provide labels (ratings for different image features), we show images that are representative of the Mecklenberg County defendant population as a whole.
These data capture much of the information the judge has available at the time of the pretrial hearing, but not all of it. Both the judge and the algorithm see structured variables about each defendant like defendant demographics, current charge, and prior record. Because the mug shot (which the algorithm uses) is taken not long before the pretrial hearing, it should be a reasonable proxy for what the judge sees in court. The additional information the judge has but the algorithm does not includes the narrative arrest report from the police and what happens in court. While pretrial hearings can be quite brief in many jurisdictions (often not more than just a few minutes), the judge may nonetheless hear statements from police, prosecutors, defense lawyers, and sometimes family members. Defendants usually have their lawyers speak for them and do not say much at these hearings.
We downloaded 81,166 arrests made between January 18, 2017, and January 17, 2020, involving 42,353 unique defendants. We apply several data filters, like dropping cases without mugshots ( Online Appendix Table A.I ), leaving 51,751 observations. Because our goal is inference about new out-of-sample (OOS) observations, we partition our data as follows:
A train set of N = 22,696 cases, constructed by taking arrests through July 17, 2019, grouping arrests by arrestee, 30 randomly selecting |$70\%$| to the training-plus-validation data set, then randomly selecting |$70\%$| of those arrestees for the training data specifically.
A validation set of N = 9,604 cases used to report OOS performance in the article’s main exhibits, consisting of the remaining |$30\%$| in the combined training-plus-validation data frame.
A lock-box hold-out set of N = 19,009 cases that we did not touch until the article was accepted for final publication, to avoid what one might call researcher overfitting: we run lots of models over the course of writing the article, and the results on the validation data set may overstate our findings. This data set consists of the N = 4,759 valid cases for the last six months of our data period (July 17, 2019, to January 17, 2020) plus a random sample of |$30\%$| of those arrested before July 17, 2019, so that we can present results that are OOS with respect to individuals and time. Once this article was officially accepted, we replicated the findings presented in our main exhibits (see Online Appendix D and Online Appendix Tables A.XVIII–A.XXXII ). We see that our core findings are qualitatively similar. 31
Descriptive statistics are shown in Table I . Relative to the county as a whole, the arrested population substantially overrepresents men ( |$78.7\%$| ) and Black residents ( |$69.4\%$| ). The average age of arrestees is 31.8 years. Judges detain |$23.3\%$| of cases, and in |$25.1\%$| of arrests the person is rearrested before their case is resolved (about one-third of those released). Randomization of arrestees to the training versus validation data sets seems to have been successful, as shown in Table I . None of the pairwise comparisons has a p -value below .05 (see Online Appendix Table A.II ). A permutation multivariate analysis of variance test of the joint null hypothesis that the training-validation differences for all variables are all zero yields p = .963. 32 A test for the same joint null hypothesis for the differences between the training sample and the lock-box hold-out data set (out of sample by individual) yields a test statistic of p = .537.
Summary Statistics for Mecklenburg County NC Data, 2017–2020
Notes. This table reports descriptive statistics for our full data set and analysis subsets, which cover the period January 18, 2017, through January 17, 2020, from Mecklenburg County, NC. The lock-box hold-out data set consists of data from the last six months of our study period (July 17, 2019–January 17, 2020) plus a subset of cases through July 16, 2019, selected by randomly selecting arrestees. The remainder of the data set is then randomly assigned by arrestee to our training data set (used to build our algorithms) or to our validation set (which we use to report results in the article’s main exhibits). For additional details of our data filters and partitioning procedures, see Online Appendix Table A.I . We define pretrial release as being released on the defendant’s own recognizance or having been assigned and then posting cash bail requirements within three days of arrest. We define rearrest as experiencing a new arrest before adjudication of the focal arrest, with detained defendants being assigned zero values for the purposes of this table. Arrest charge categories reflect the most serious criminal charge for which a person was arrested, using the FBI Uniform Crime Reporting hierarchy rule in cases where someone is arrested and charged with multiple offenses. For analyses of variance for the test of the joint null hypothesis that the difference in means across each variable is zero, see Online Appendix Table A.II .
III.C. Human Labels
The administrative data capture many key features of each case but omit some other important ones. We solve these data insufficiency problems through a series of human intelligence tasks (HITs), which involve having study subjects on one of two possible platforms (Amazon’s Mechanical Turk or Prolific) assign labels to each case from looking at the mug shots. More details are in Online Appendix Table A.III . We use data from these HITs mostly to understand how the algorithm’s predictions relate to already-known determinants of human decision making, and hence the degree to which the algorithm is discovering something novel.
One set of HITs filled in demographic-related data: ethnicity; skin tone (since people are often stereotyped on skin color, or “colorism”; Hunter 2007 ), reported on an 18-point scale; the degree to which defendants appear more stereotypically Black on a 9-point scale ( Eberhardt et al. 2006 show this affects criminal justice decisions); and age, to compare to administrative data for label quality checks. 33 Because demographics tend to be easy for people to see in images, we collect just one label per image for each of these variables. To confirm one label is enough, we repeated the labeling task for 100 images but collected 10 labels for each image; we see that additional labels add little information. 34 Another data quality check comes from the fact that the distributions of skin color ratings do systematically differ by defendant race ( Online Appendix Figure A.III ).
A second type of HIT measured facial features that previous psychology research has shown affect human judgments. The specific set of facial features we focus on come from the influential study by Oosterhof and Todorov (2008) of people’s perceptions of the facial features of others. When subjects are asked to provide descriptions of different faces, principal components analysis suggests just two dimensions account for about |$80\%$| of the variation: (i) trustworthiness and (ii) dominance. We also collected data on two other facial features shown to be associated with real-world decisions like hiring or whom to vote for: (iii) attractiveness and (iv) competence ( Frieze, Olson, and Russell 1991 ; Little, Jones, and DeBruine 2011 ; Todorov and Oh 2021 ). 35
We asked subjects to rate images for each of these psychological features on a nine-point scale. Because psychological features may be less obvious than demographic features, we collected three labels per training–data set image and five per validation–data set image. 36 There is substantial variation in the ratings that subjects assign to different images for each feature (see Online Appendix Figure A.VI ). The ratings from different subjects for the same feature and image are highly correlated: interrater reliability measures (Cronbach’s α) range from 0.87 to 0.98 ( Online Appendix Figure A.VII ), similar to those reported in studies like Oosterhof and Todorov (2008) . 37 The information gain from collecting more than a few labels per image is modest. 38 For summary statistics, see Online Appendix Table A.IV .
Finally, we also tried to capture people’s implicit or tacit understanding of the determinants of judges’ decisions by asking subjects to predict which mug shot out of a pair would be detained, with images in each pair matched on gender, race, and five-year age brackets. 39 We incentivized study subjects for correct predictions and gave them feedback over the course of the 50 image pairs to facilitate learning. We treat the first 10 responses per subject as a “learning set” that we exclude from our analysis.
The first step of our hypothesis generation procedure is to build an algorithmic model of some behavior, which in our case is the judge’s detention decision. A sizable share of the predictable variation in judge decisions comes from a surprising source: the defendant’s face. Facial features implicated by past research explain just a modest share of this predictable variation. The algorithm seems to have found a novel discovery.
IV.A. What Drives Judge Decisions?
We begin by predicting judge pretrial detention decisions ( y = 1 if detain, y = 0 if release) using all the inputs available ( x ). We use the training data set to construct two separate models for the two types of data available. We apply gradient-boosted decision trees to predict judge decisions using the structured administrative data (current charge, prior record, age, gender), m s ( x ); for the unstructured data (raw pixel values from the mug shots), we train a convolutional neural network, m u ( x ). Each model returns an estimate of y (a predicted detention probability) for a given x . Because these initial steps of our procedure use standard machine learning methods, we relegate their discussion to the Online Appendix .
We pool the signal from both models to form a single weighted-average model |$m_p(x)=[\hat{\beta _s} m_s(x) + \hat{\beta _u} m_u(x)]$| using a so-called stacking procedure where the data are used to estimate the relevant weights. 40 Combining structured and unstructured data is an active area of deep-learning research, often called fusion modeling ( Yuhas, Goldstein, and Sejnowski 1989 ; Lahat, Adali, and Jutten 2015 ; Ramachandram and Taylor 2017 ; Baltrušaitis, Ahuja, and Morency 2019 ). We have tried several of the latest fusion architectures; none improve on our ensemble approach.
Judge decisions do have some predictable structure. We report predictive performance as the area under the receiver operating characteristic curve, or AUC, which is a measure of how well the algorithm rank-orders cases with values from 0.5 (random guessing) to 1.0 (perfect prediction). Intuitively, AUC can be thought of as the chance that a uniformly randomly selected detained defendant has a higher predicted detention likelihood than a uniformly randomly selected released defendant. The algorithm built using all candidate features, m p ( x ), has an AUC of 0.780 (see Online Appendix Figure A.X ).
What is the algorithm using to make its predictions? A single type of input captures a sizable share of the total signal: the defendant’s face. The algorithm built using only the mug shot image, m u ( x ), has an AUC of 0.625 (see Online Appendix Figure A.X ). Since an AUC of 0.5 represents random prediction, in AUC terms the mug shot accounts for |$\frac{0.625-0.5}{0.780-0.5}=44.6\%$| of the predictive signal about judicial decisions.
Another common way to think about predictive accuracy is in R 2 terms. While our data are high dimensional (because the facial image is a high-dimensional object), the algorithm’s prediction of the judge’s decision based on the facial image, m u ( x ), is a scalar and can be easily included in a familiar regression framework. Like AUC, measures like R 2 and mean squared error capture how well a model rank-orders observations by predicted probabilities, but R 2 , unlike AUC, also captures how close predictions are to observed outcomes (calibration). 41 The R 2 from regressing y against m s ( x ) and m u ( x ) in the validation data is 0.11. Regressing y against m u ( x ) alone yields an R 2 of 0.03. So depending on how we measure predictive accuracy, around a quarter ( |$\frac{0.03}{0.11}=27.3\%)$| to a half ( |$44.6\%$| ) of the predicted signal about judges’ decisions is captured by the face.
Average differences are another way to see what drives judges’ decisions. For any given feature x k , we can calculate the average detention rate for different values of the feature. For example, for the variable measuring whether the defendant is male ( x k = 1) versus female ( x k = 0), we can calculate and plot E [ y | x k = 1] versus E [ y | x k = 0]. As shown in Online Appendix Figure A.XI , the difference in detention rates equals 4.8 percentage points for those arrested for violent versus nonviolent crimes, 10.2 percentage points for men versus women, and 4.3 percentage points for bottom versus top quartile of skin tone, which are all sizable relative to the baseline detention rate of |$23.3\%$| in our validation data set. By way of comparison, average detention rates for the bottom versus top quartile of the mug shot algorithm’s predictions, m u ( x ), differ by 20.4 percentage points.
In what follows, we seek to understand more about the mug shot–based prediction of the judge’s decision, which we refer to simply as m ( x ) in the remainder of the article.
IV.B. Judicial Error?
So far we have shown that the face predicts judges’ behavior. Are judges right to use face information? To be precise, by “right” we do not mean a broader ethical judgment; for many reasons, one could argue it is never ethical to use the face. But suppose we take a rather narrow (exceedingly narrow) formulation of “right.” Recall the judge is meant to make jailing decisions based on the defendant’s risk. Is the use of these facial characteristics consistent with that objective? Put differently, if we account for defendant risk differences, do these facial characteristics still predict judge decisions? The fact that judges rely on the face in making detention decisions is in itself a striking insight regardless of whether the judges use appearance as a proxy for risk or are committing a cognitive error.
At first glance, the most straightforward way to answer this question would be to regress rearrest against the algorithm’s mug shot–based detention prediction. That yields a statistically significant relationship: The coefficient (and standard error) for the mug shot equals 0.6127 (0.0460) with no other explanatory variables in the regression versus 0.5735 (0.0521) with all the explanatory variables (as in the final column, Table III ). But the interpretation here is not so straightforward.
The challenge of interpretation comes from the fact that we have only measured crime rates for the released defendants. The problem with having measured crime, not actual crime, is that whether someone is charged with a crime is itself a human choice, made by police. If the choices police make about when to make an arrest are affected by the same biases that might afflict judges, then measured rearrest rates may correlate with facial characteristics simply due to measurement bias. The problem created by having measures of rearrest only for released defendants is that if judges have access to private information (defendant characteristics not captured by our data set), and judges use that information to inform detention decisions, then the released and detained defendants may be different in unobservable ways that are relevant for rearrest risk ( Kleinberg et al. 2018 ).
With these caveats in mind, at least we can perform a bounding exercise. We created a predictor of rearrest risk (see Online Appendix B ) and then regress judges’ decisions on predicted rearrest risk. We find that a one-unit change in predicted rearrest risk changes judge detention rates by 0.6103 (standard error 0.0213). By comparison, we found that a one-unit change in the mug shot (by which we mean the algorithm’s mug shot–based prediction of the judge detention decision) changes judge detention rates by 0.6963 (standard error 0.0383; see Table III , column (1)). That means if the judges were reacting to the defendant’s face only because the face is a proxy for rearrest risk, the difference in rearrest risk for those with a one-unit difference in the mug shot would need to be |$\frac{0.6963}{0.6103} = 1.141$| . But when we directly regress rearrest against the algorithm’s mug shot–based detention prediction, we get a coefficient of 0.6127 (standard error 0.0460). Clearly 0.6127 < 1.141; that is, the mug shot does not seem to be strongly related enough to rearrest risk to explain the judge’s use of it in making detention decisions. 42
Of course this leaves us with the second problem with our data: we only have crime data on the released. It is possible the relationship between the mug shot and risk could be very different among the |$23.3\%$| of defendants who are detained (which we cannot observe). Put differently, the mug shot–risk relationship among the |$76.7\%$| of the defendants who are released is 0.6127; and let A be the (unknown) mug shot–risk relationship among the jailed. What we really want to know is the mug shot–risk relationship among all defendants, which equals (0.767 · 0.6127) + (0.233 · A ). For this mug shot–risk relationship among all defendants to equal 1.141, A would need to be 2.880, nearly five times as great among the detained defendants as among the released. This would imply an implausibly large effect of the mug shot on rearrest risk relative to the size of the effects on rearrest risk of other defendant characteristics. 43
In addition, the results from Section VI.B call into question that these characteristics are well-understood proxies for risk. As we show there, experts who understand pretrial (public defenders and legal aid society staff) do not recognize the signal about judge decision making that the algorithm has discovered in the mug shot. These considerations as a whole—that measured rearrest is itself biased, the bounding exercise, and the failure of experts to recreate this signal—together lead us to tentatively conclude that it is unlikely that what the algorithm is finding in the face is merely a well-understood proxy for risk, but reflects errors in the judicial decision-making process. Of course, that presumption is not essential for the rest of the article, which asks: what exactly has the algorithm discovered in the face?
IV.C. Is the Algorithm Discovering Something New?
Previous studies already tell us a number of things about what shapes the decisions of judges and other people. For example, we know people stereotype by gender ( Avitzour et al. 2020 ), age ( Neumark, Burn, and Button 2016 ; Dahl and Knepper 2020 ), and race or ethnicity ( Bertrand and Mullainathan 2004 ; Arnold, Dobbie, and Yang 2018 ; Arnold, Dobbie, and Hull 2020 ; Fryer 2020 ; Hoekstra and Sloan 2022 ; Goncalves and Mello 2021 ). Is the algorithm just rediscovering known determinants of people’s decisions, or discovering something new? We address this in two ways. We first ask how much of the algorithm’s predictions can be explained by already-known features ( Table II ). We then ask how much of the algorithm’s predictive power in explaining actual judges’ decisions is diminished when we control for known factors ( Table III ). We carry out both analyses for three sets of known facial features: (i) demographic characteristics, (ii) psychological features, and (iii) incentivized human guesses. 44
Is the Algorithm Rediscovering Known Facial Features?
Notes. The table presents the results of regressing an algorithmic prediction of judge detention decisions against each of the different explanatory variables as listed in the rows, where each column represents a different regression specification (the specific explanatory variables in each regression are indicated by the filled-in coefficients and standard errors in the table). The algorithm was trained using mug shots from the training data set; the regressions reported here are carried out using data from the validation data set. Data on skin tone, attractiveness, competence, dominance, and trustworthiness comes from asking subjects to assign feature ratings to mug shot images from the Mecklenburg County, NC, Sheriff’s Office public website (see the text). The human guess about the judges’ decision comes from showing workers on the Prolific platform pairs of mug shot images and asking them to report which defendant they believe the judge would be more likely to detain. Regressions follow a linear probability model and also include indicators for unknown race and unknown gender. * p < .1; ** p < .05; *** p < .01.
Does the Algorithm Predict Judge Behavior after Controlling for Known Factors?
Notes. This table reports the results of estimating a linear probability specification of judges’ detain decisions against different explanatory variables in the validation set described in Table I . Each row represents a different explanatory variable for the regression, while each column reports the results of a separate regression with different combinations of explanatory variables (as indicated by the filled-in coefficients and standard errors in the table). The algorithmic predictions of the judges’ detain decision come from our convolutional neural network algorithm built using the defendants’ face image as the only feature, using data from the training data set. Measures of defendant demographics and current arrest charge come from government administrative data obtained from a combination of Mecklenburg County, NC, and state agencies. Measures of skin tone, attractiveness, competence, dominance, and trustworthiness come from subject ratings of mug shot images (see the text). Human guess variable comes from showing subjects pairs of mug shot images and asking subjects to identify the defendant they think the judge would be more likely to detain. Regression specifications also include indicators for unknown race and unknown gender. * p < .1; ** p < .05; *** p < .01.
Table II , columns (1)–(3) show the relationship of the algorithm’s predictions to demographics. The predictions vary enormously by gender (men have predicted detention likelihoods 11.9 percentage points higher than women), less so by age, 45 and by different indicators of race or ethnicity. With skin tone scored on a 0−1 continuum, defendants whom independent raters judge to be at the lightest end of the continuum are 4.4 percentage points less likely to be detained than those rated to have the darkest skin tone (column (3)). Conditional on skin tone, Black defendants have a 1.9 percentage point lower predicted likelihood of detention compared with whites. 46
Table II , column (4) shows how the algorithm’s predictions relate to facial features implicated by past psychological studies as shaping people’s judgments of one another. These features also help explain the algorithm’s predictions of judges’ detention decisions: people judged by independent raters to be one standard deviation more attractive, competent, or trustworthy have lower predicted likelihood of detention equal to 0.55, 0.91, and 0.48 percentage points, respectively, or |$2.2\%$| , |$3.6\%$| , and |$1.8\%$| of the base rate. 47 Those whom subjects judge are one standard deviation more dominant-looking have a higher predicted likelihood of detention of 0.37 percentage points (or |$1.5\%)$| .
How do we know we have controlled for everything relevant from past research? The literature on what shapes human judgments in general is vast; perhaps there are things that are relevant for judges’ decisions specifically that we have inadvertently excluded? One way to solve this problem would be to do a comprehensive scan of past studies of human judgment and decision making, and then decide which results from different non–criminal justice contexts might be relevant for criminal justice. But that itself is a form of human-driven hypothesis generation, bringing us right back to where we started.
To get out of this box, we take a different approach. Instead of enumerating individual characteristics, we ask people to embody their beliefs in a guess, which ought to be the compound of all these characteristics. Then we can ask whether the algorithm has rediscovered this human guess (and later whether it has discovered more). We ask independent subjects to look at pairs of mug shots matched by gender, race, and five-year age bins and forecast which defendant is more likely to be detained by a judge. We provide a financial incentive for accurate guesses to increase the chances that subjects take the exercise seriously. 48 We also provide subjects with an opportunity to learn by showing subjects 50 image pairs with feedback after each pair about which defendant the judge detained. We treat the first 10 image pairs from each subject as learning trials and only use data from the last 40 image pairs. This approach is intended to capture anything that influences judges’ decisions that subjects could recognize, from subtle signs of things like socioeconomic status or drug use or mood, to things people can recognize but not articulate.
It turns out subjects are modestly good at this task ( Table II ). Participants guess which mug shot is more likely to be detained at a rate of |$51.4\%$| , which is different to a statistically significant degree from the |$50\%$| random-guessing threshold. When we regress the algorithm’s predicted detention rate against these subject guesses, the coefficient is 3.99 percentage points, equal to |$17.1\%$| of the base rate.
The findings in Table II are somewhat remarkable. The only input the algorithm had access to was the raw pixel values of each mug shot, yet it has rediscovered findings from decades of previous research and human intuition.
Interestingly, these features collectively explain only a fraction of the variation in the algorithm’s predictions: the R 2 is only 0.2228. That by itself does not necessarily mean the algorithm has discovered additional useful signal. It is possible that the remaining variation is prediction error—components of the prediction that do not explain actual judges’ decisions.
In Table III , we test whether the algorithm uncovers any additional signal for actual judge decisions, above and beyond the influence of these known factors. The algorithm by itself produces an R 2 of 0.0331 (column (1)), substantially higher than all previously known features taken together, which produce an R 2 of 0.0162 (column (5)), or the human guesses alone which produce an R 2 of 0.0025 (so we can see the algorithm is much better at predicting detention from faces than people are). Another way to see that the algorithm has detected signal above and beyond these known features is that the coefficient on the algorithm prediction when included alone in the regression, 0.6963 (column (1)), changes only modestly when we condition on everything else, now equal to 0.6171 (column (7)). The algorithm seems to have discovered some novel source of signal that better predicts judge detention decisions. 49
The algorithm has made a discovery: something about the defendant’s face explains judge decisions, above and beyond the facial features implicated by existing research. But what is it about the face that matters? Without an answer, we are left with a discovery of an unsatisfying sort. We have simply replaced one black box hypothesis generation procedure (human creativity) with another (the algorithm). In what follows we demonstrate how existing methods like saliency maps cannot solve this challenge in our application and then discuss our solution to that problem.
V.A. The Challenge of Explanation
The problem of algorithm-human communication stems from the fact that we cannot simply look inside the algorithm’s “black box” and see what it is doing because m ( x ), the algorithmic predictor, is so complicated. A common solution in computer science is to forget about looking inside the algorithmic black box and focus instead on drawing inferences from curated outputs of that box. Many of these methods involve gradients: given a prediction function m ( x ), we can calculate the gradient |$\nabla m(x) = \frac{\mathrm{d}{m}}{\mathrm{d}x}(x)$| . This lets us determine, at any input value, what change in the input vector maximally changes the prediction. 50 The idea of gradients is useful for image classification tasks because it allows us to tell which pixel image values are most important for changing the predicted outcome.
For example, a widely used method known as saliency maps uses gradient information to highlight the specific pixels that are most important for predicting the outcome of interest ( Baehrens et al. 2010 ; Simonyan, Vedaldi, and Zisserman 2014 ). This approach works well for many applications like determining whether a given picture contains a given type of animal, a common task in ecology ( Norouzzadeh et al. 2018 ). What distinguishes a cat from a dog? A saliency map for a cat detector might highlight pixels around, say, the cat’s head: what is most cat-like is not the tail, paws, or torso, but the eyes, ears, and whiskers. But more complicated outcomes of the sort social scientists study may depend on complicated functions of the entire image.
Even if saliency maps were more selective in highlighting pixels in applications like ours, for hypothesis generation they also suffer from a second limitation: they do not convey enough information to enable people to articulate interpretable hypotheses. In the cat detector example, a saliency map can tell us that something about the cat’s (say) whiskers are key for distinguishing cats from dogs. But what about that feature matters? Would a cat look more like a dog if its whiskers were longer? Or shorter? More (or less?) even in length? People need to know not just what features matter but how they must change to change the prediction. For hypothesis generation, the saliency map undercommunicates with humans.
To test the ability of saliency maps to help with our application, we focused on a facial feature that people already understand and can easily recognize from a photo: age. We first build an algorithm that predicts each defendant’s age from their mug shot. For a representative image, as in the top left of Figure III , we can highlight which pixels are most important for predicting age, shown in the top right. 51 A key limitation of saliency maps is easy to see: because age (like many human facial features) is a function of almost every part of a person’s face, the saliency map highlights almost everything.

Candidate Algorithm-Human Communication Vehicles for a Known Facial Feature: Age
Panel A shows a randomly selected point in the GAN latent space for a non-Hispanic white male defendant. Panel B shows a saliency map that highlights the pixels that are most important for an algorithmic model that predicts the defendant’s age from the mug shot image. Panel C shows an image changed or “morphed” in the direction of older age, based on the gradient of the image-based age prediction, using the “naive” morphing procedure that does not constrain the new image to lie on the face manifold (see the text). Panel D shows the image morphed to the maximum age using our actual preferred morphing procedure.
An alternative to simply highlighting high-leverage pixels is to change them in the direction of the gradient of the predicted outcome, to—ideally—create a new face that now has a different predicted outcome, what we call “morphing.” This new image answers the counterfactual question: “How would this person’s face change to increase their predicted outcome?” Our approach builds on the ability of people to comprehend ideas through comparisons, so we can show morphed image pairs to subjects to have them name the differences that they see. Figure IV summarizes our semiautomated hypothesis generation pipeline. (For more details see Online Appendix B .) The benefit of morphed images over actual mug shot images is to isolate the differences across faces that matter for the outcome of interest. By reducing noise, morphing also reduces the risk of spurious discoveries.

Hypothesis Generation Pipeline
The diagram illustrates all the algorithmic components in our procedure by presenting a full pipeline for algorithmic interpretation.
Figure V illustrates how this morphing procedure works in practice and highlights some of the technical challenges that arise. Let the box in the top panel represent the space of all possible images—all possible combinations of pixel values for, say, a 512 × 512 image. Within this space, we can apply our mug shot–based predictor of the known facial feature, age, to identify all images with the same predicted age, as shown by the contour map of the prediction function. Imagine picking some random initial mug shot image. We could follow the gradient to find an image with a higher predicted value of the outcome y .

Morphing Images for Detention Risk On and Off the Face Manifold
The figure shows the difference between an unconstrained (naive) morphing procedure and our preferred new morphing approach. In both panels, the background represents the image space (set of all possible pixel values) and the blue line (color version available online) represents the set of all pixel values that correspond to any face image (the face manifold). The orange lines show all images that have the same predicted outcome (isoquants in predicted outcome). The initial face (point on the outermost contour line) is a randomly selected face in GAN face space. From there we can naively follow the gradients of an algorithm that predicts some outcome of interest from face images. As shown in Panel A, this takes us off the face manifold and yields a nonface image. Alternatively, with a model of the face manifold, we can follow the gradient for the predicted outcome while ensuring that the new image is again a realistic instance as shown in Panel B.
The challenge is that most points in this image space are not actually face images. Simply following the gradient will usually take us off the data distribution of face images, as illustrated abstractly in the top panel of Figure V . What this means in practice is shown in the bottom left panel of Figure III : the result is an image that has a different predicted outcome (in the figure, illustrated for age) but no longer looks like a real instance—that is, no longer looks like a realistic face image. This “naive” morphing procedure will not work without some way to ensure the new point we wind up on in image space corresponds to a realistic face image.
V.B. Building a Model of the Data Distribution
To ensure morphing leads to realistic face images, we need a model of the data distribution p ( x )—in our specific application, the set of images that are faces. We rely on an unsupervised learning approach to this problem. 52 Specifically, we use generative adversarial networks (GANs), originally introduced to generate realistic new images for a variety of tasks (see Goodfellow et al. 2014 ). 53
A GAN is built by training two algorithms that “compete” with each another, the generator G and the classifier C : the generator creates synthetic images and the classifier (or “discriminator”), presented with synthetic or real images, tries to distinguish which is which. A good discriminator pressures the generator to produce images that are harder to distinguish from real; in turn, a good generator pressures the classifier to get better at discriminating real from synthetic images. Data on actual faces are used to train the discriminator, which results in the generator being trained as it seeks to fool the discriminator. With machine learning, the performance of C and G improve with successive iterations of training. A perfect G would output images where the classifier C does no better than random guessing. Such a generator would by definition limit itself to the same input space that defines real images, that is, the data distribution of faces. (Additional discussion of GANs in general and how we construct our GAN specifically are in Online Appendix B .)
To build our GAN and evaluate its expressiveness we use standard training metrics, which turn out to compare favorably to what we see with other widely used GAN models on other data sets (see Online Appendix B.C for details). A more qualitative way to judge our GAN comes from visual inspection; some examples of synthetic face images are in Figure II . Most importantly, the GAN we build (as is true of GANs in general) is not generic. GANs are specific. They do not generate “faces” but instead seek to match the distribution of pixel combinations in the training data. For example, our GAN trained using mug shots would never generate generic Facebook profile photos or celebrity headshots.
Figure V illustrates how having a model such as the GAN lets morphing stay on the data distribution of faces and produce realistic images. We pick a random point in the space of faces (mug shots) and then use the algorithmic predictor of the outcome of interest m ( x ) to identify nearby faces that are similar in all respects except those relevant for the outcome. Notice this procedure requires that faces closer to one another in GAN latent space should look relatively more similar to one another to a human in pixel space. Otherwise we might make a small movement along the gradient and wind up with a face that looks different in all sorts of other ways that are irrelevant to the outcome. That is, we need the GAN not just to model the support of the data but also to provide a meaningful distance metric.
When we produce these morphs, what can possibly change as we morph? In principle there is no limit. The changes need not be local: features such as skin color, which involves many pixels, could change. So could features such as attractiveness, where the pixels that need to change to make a face more attractive vary from face to face: the “same” change may make one face more attractive and another less so. Anything represented in the face could change, as could anything else in the image beyond the face that matters for the outcome (if, for example, localities varied in both detention rates and the type of background they have someone stand in front of for mug shots).
In practice, though, there is a limit. What can change depends on how rich and expressive the estimated GAN is. If the GAN fails to capture a certain kind of face or a dimension of the face, then we are unlikely to be able to morph on that dimension. The morphing procedure is only as complete as the GAN is expressive. Assuming the GAN expresses a feature, then if m ( x ) truly depends on that feature, morphing will likely display it. Nor is there any guarantee that in any given application the classifier m ( x ) will find novel signal for the outcome y , or that the GAN successfully learns the data distribution ( Nalisnick et al. 2018 ), or that subjects can detect and articulate whatever signal the classifier algorithm has discovered. Determining the general conditions under which our procedure will work is something we leave to future research. Whether our procedure can work for the specific application of judge decisions is the question to which we turn next. 54
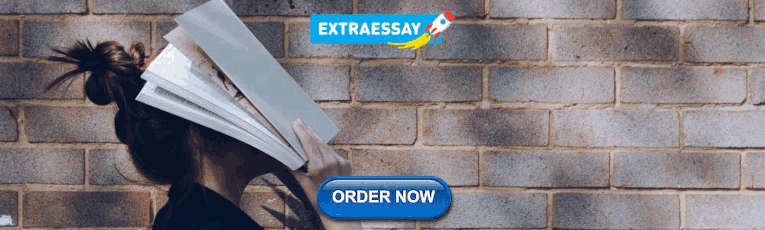
V.C. Validating the Morphing Procedure
We return to our algorithmic prediction of a known facial feature—age—and see what morphing by age produces as a way to validate or test our procedure. When we follow the gradient of the predicted outcome (age), by constraining ourselves to stay on the GAN’s latent space of faces we wind up with a new age-morphed face that does indeed look like a realistic face image, as shown in the bottom right of Figure III . We seem to have successfully developed a model of the data distribution and a way to move around on that surface to create realistic new instances.
To figure out if algorithm-human communication occurs, we run these age-morphed image pairs through our experimental pipeline ( Figure IV ). Our procedure is only useful if it is replicable—that is, if it does not depend on the idiosyncratic insights of any particular person. For that reason, the people looking at these images and articulating what they see should not be us (the investigators) but a sample of external, independent study subjects. In our application, we use Prolific workers (see Online Appendix Table A.III ). Reliability or replicability is indicated by the agreement in the subject responses: lots of subjects see and articulate the same thing in the morphed images.
We asked subjects to look at 50 age-morphed image pairs selected at random from a population of 100 pairs, and told them the images in each pair differ on some hidden dimension but did not tell them what that was. 55 We asked subjects to guess which image expresses that hidden feature more, gave them feedback about the right answer, treated the first 10 image pairs as learning examples, and calculated accuracy on the remaining 40 images. Subjects correctly selected the older image |$97.8\%$| of the time.
The final step was to ask subjects to name what differs in image pairs. Making sense of these responses requires some way to group them into semantic categories. Each subject comment could include several concepts (e.g., “wrinkles, gray hair, tired”). We standardized these verbal descriptions by removing punctuation, using only lowercase characters, and removing stop words. We gave three research assistants not otherwise involved in the project these responses and asked them to create their own categories that would capture all the responses (see Online Appendix Figure A.XIII ). We also gave them an illustrative subject comment and highlighted the different “types” of categories (descriptive physical features, i.e., “thick eyebrows,” descriptive impression category, i.e., “energetic,” but also an illustration of a category of comment that is too vague to lend itself to useful measurement, i.e., “ears”). In our validation exercise |$81.5\%$| of subject reports fall into the semantic categories of either age or the closely related feature of hair color. 56
V.D. Understanding the Judge Detention Predictor
Having validated our algorithm-human communication procedure for the known facial feature of age, we are ready to apply it to generate a new hypothesis about what drives judge detention decisions. To do this we combine the mug shot algorithm predictor of judges’ detention decisions, m ( x ), with our GAN of the data distribution of mug shot images, then create new synthetic image pairs morphed with respect to the likelihood the judge would detain the defendant (see Figure IV ).
The top panel of Figure VI shows a pair of such images. Underneath we show an “image strip” of intermediate steps, along with each image’s predicted detention rate. With an overall detention rate of |$23.3\%$| in our validation data set, morphing takes us from about one-half the base rate ( |$13\%$| ) up to nearly twice the base rate ( |$41\%$| ). Additional examples of morphed image pairs are shown in Figure VII .

Illustration of Morphed Faces along the Detention Gradient
Panel A shows the result of selecting a random point on the GAN latent face space for a white non-Hispanic male defendant, then using our new morphing procedure to increase the predicted detention risk of the image to 0.41 (left) or reduce the predicted detention risk down to 0.13 (right). The overall average detention rate in the validation data set of actual mug shot images is 0.23 by comparison. Panel B shows the different intermediate images between these two end points, while Panel C shows the predicted detention risk for each of the images in the middle panel.

Examples of Morphing along the Gradients of the Face-Based Detention Predictor
We showed 54 subjects 50 detention-risk-morphed image pairs each, asked them to predict which defendant would be detained, offered them financial incentives for correct answers, 57 and gave them feedback on the right answer. Online Appendix Figure A.XV shows how accurate subjects are as they get more practice across successive morphed image pairs. With the initial image-pair trials, subjects are not much better than random guessing, in the range of what we see when subjects look at pairs of actual mugshots (where accuracy is |$51.4\%$| across the final 40 mug shot pairs people see). But unlike what happens when subjects look at actual images, when looking at morphed image pairs subjects seem to quickly learn what the algorithm is trying to communicate to them. Accuracy increased by over 10 percentage points after 20 morphed image pairs and reached |$67\%$| after 30 image pairs. Compared to looking at actual mugshots, the morphing procedure accomplished its goal of making it easier for subjects to see what in the face matters most for detention risk.
We asked subjects to articulate the key differences they saw across morphed image pairs. The result seems to be a reliable hypothesis—a facial feature that a sizable share of subjects name. In the top panel of Figure VIII , we present a histogram of individual tokens (cleaned words from worker comments) in “word cloud” form, where word size is approximately proportional to frequency. 58 Some of the most common words are “shaved,” “cleaner,” “length,” “shorter,” “moustache,” and “scruffy.” To form semantic categories, we use a procedure similar to what we describe for our validation exercise for the known feature of age. 59 Grouping tokens into semantic categories, we see that nearly |$40\%$| of the subjects see and name a similar feature that they think helps explain judge detention decisions: how well-groomed the defendant is (see the bottom panel of Figure VIII ). 60

Subject Reports of What They See between Detention-Risk-Morphed Image Pairs
Panel A shows a word cloud of subject reports about what they see as the key difference between image pairs where one is a randomly selected point in the GAN latent space and the other is morphed in the direction of a higher predicted detention risk. Words are approximately proportionately sized to the frequency of subject mentions. Panel B shows the frequency of semantic groupings of those open-ended subject reports (see the text for additional details).
Can we confirm that what the subjects think the algorithm is seeing is what the algorithm actually sees? We asked a separate set of 343 independent subjects (MTurk workers) to label the 32,881 mug shots in our combined training and validation data sets for how well-groomed each image was perceived to be on a nine-point scale. 61 For data sets of our size, these labeling costs are fairly modest, but in principle those costs could be much more substantial (or even prohibitive) in some applications.
Table IV suggests algorithm-human communication has successfully occurred: our new hypothesis, call it h 1 ( x ), is correlated with the algorithm’s prediction of the judge, m ( x ). If subjects were mistaken in thinking they saw well-groomed differences across images, there would be no relationship between well-groomed and the detention predictions. Yet what we actually see is the R 2 from regressing the algorithm’s predictions against well-groomed equals 0.0247, or |$11\%$| of the R 2 we get from a model with all the explanatory variables (0.2361). In a bivariate regression the coefficient (−0.0172) implies that a one standard deviation increase in well-groomed (1.0118 points on our 9-point scale) is associated with a decline in predicted detention risk of 1.74 percentage points, or |$7.5\%$| of the base rate. Another way to see the explanatory power of this hypothesis is to note that this coefficient hardly changes when we add all the other explanatory variables to the regression (equal to −0.0153 in the final column) despite the substantial increase in the model’s R 2 .
Correlation between Well-Groomed and the Algorithm’s Prediction
Notes. This table shows the results of estimating a linear probability specification regressing algorithmic predictions of judges’ detain decision against different explanatory variables, using data from the validation set of cases from Mecklenburg County, NC. Each row of the table represents a different explanatory variable for the regression, while each column reports the results of a separate regression with different combinations of explanatory variables (as indicated by the filled-in coefficients and standard errors in the table). Algorithmic predictions of judges’ decisions come from applying an algorithm built with face images in the training data set to validation set observations. Data on well-groomed, skin tone, attractiveness, competence, dominance, and trustworthiness come from subject ratings of mug shot images (see the text). Human guess variable comes from showing subjects pairs of mug shot images and asking subjects to identify the defendant they think the judge would be more likely to detain. Regression specifications also include indicators for unknown race and unknown gender. * p < .1; ** p < .05; *** p < .01.
V.E. Iteration
Our procedure is iterable. The first novel feature we discovered, well-groomed, explains some—but only some—of the variation in the algorithm’s predictions of the judge. We can iterate our procedure to generate hypotheses about the remaining residual variation as well. Note that the order in which features are discovered will depend on how important each feature is in explaining the judge’s detention decision and on how salient each feature is to the subjects who are viewing the morphed image pairs. So explanatory power for the judge’s decisions need not monotonically decline as we iterate and discover new features.
To isolate the algorithm’s signal above and beyond what is explained by well-groomed, we wish to generate a new set of morphed image pairs that differ in predicted detention but hold well-groomed constant. That would help subjects see other novel features that might differ across the detention-risk-morphed images, without subjects getting distracted by differences in well-groomed. 62 But iterating the procedure raises several technical challenges. To see these challenges, consider what would in principle seem to be the most straightforward way to orthogonalize, in the GAN’s latent face space:
use training data to build predictors of detention risk, m ( x ), and the facial features to orthogonalize against, h 1 ( x );
pick a point on the GAN latent space of faces;
collect the gradients with respect to m ( x ) and h 1 ( x );
use the Gram-Schmidt process to move within the latent space toward higher predicted detention risk m ( x ), but orthogonal to h 1 ( x ); and
show new morphed image pairs to subjects, have them name a new feature.
The challenge with implementing this playbook in practice is that we do not have labels for well-groomed for the GAN-generated synthetic faces. Moreover, it would be infeasible to collect this feature for use in this type of orthogonalization procedure. 63 That means we cannot orthogonalize against well-groomed, only against predictions of well-groomed. And orthogonalizing with respect to a prediction is an error-prone process whenever the predictor is imperfect (as it is here). 64 The errors in the process accumulate as we take many morphing steps. Worse, that accumulated error is not expected to be zero on average. Because we are morphing in the direction of predicted detention and we know predicted detention is correlated with well-groomed, the prediction error will itself be correlated with well-groomed.
Instead we use a different approach. We build a new detention-risk predictor with a curated training data set, limited to pairs of images matched on the features to be orthogonalized against. For each detained observation i (such that y i = 1), we find a released observation j (such that y j = 0) where h 1 ( x i ) = h 1 ( x j ). In that training data set y is now orthogonal to h 1 ( x ), so we can use the gradient of the orthogonalized detention risk predictor to move in GAN latent space to create new morphed images with different detention odds but are similar with respect to well-groomed. 65 We call these “orthogonalized morphs,” which we then feed into the experimental pipeline shown in Figure IV . 66 An open question for future work is how many iterations are possible before the dimensionality of the matching problem required for this procedure would create problems.
Examples from this orthogonalized image-morphing procedure are in Figure IX . Changes in facial features across morphed images are notably different from those in the first iteration of morphs as in Figure VI . From these examples, it appears possible that orthogonalization may be slightly imperfect; sometimes they show subtle differences in “well-groomed” and perhaps age. As with the first iteration of the morphing procedure, the second (orthogonalized) iteration of the procedure again generates images that vary substantially in their predicted risk, from 0.07 up to 0.27 (see Online Appendix Figure A.XVIII ).

Examples of Morphing along the Orthogonal Gradients of the Face-Based Detention Predictor
Still, there is a salient new signal: when presented to subjects they name a second facial feature, as shown in Figure X . We showed 52 subjects (Prolific workers) 50 orthogonalized morphed image pairs and asked them to name the differences they see. The word cloud shown in the top panel of Figure X shows that some of the most common terms reported by subjects include “big,” “wider,” “presence,” “rounded,” “body,” “jaw,” and “head.” When we ask independent research assistants to group the subject tokens into semantic groups, we can see as in the bottom of the figure that a sizable share of subject comments (around |$22\%$| ) refer to a similar facial feature, h 2 ( x ): how “heavy-faced” or “full-faced” the defendant is.

Subject Reports of What They See between Detention-Risk-Morphed Image Pairs, Orthogonalized to the First Novel Feature Discovered (Well-Groomed)
Panel A shows a word cloud of subject reports about what they see as the key difference between image pairs, where one is a randomly selected point in the GAN latent space and the other is morphed in the direction of a higher predicted detention risk, where we are moving along the detention gradient orthogonal to well-groomed and skin tone (see the text). Panel B shows the frequency of semantic groupings of these open-ended subject reports (see the text for additional details).
This second facial feature (like the first) is again related to the algorithm’s prediction of the judge. When we ask a separate sample of subjects (343 MTurk workers, see Online Appendix Table A.III ) to independently label our validation images for heavy-facedness, we can see the R 2 from regressing the algorithm’s predictions against heavy-faced yields an R 2 of 0.0384 ( Table V , column (1)). With a coefficient of −0.0182 (0.0009), the results imply that a one standard deviation change in heavy-facedness (1.1946 points on our 9-point scale) is associated with a reduced predicted detention risk of 2.17 percentage points, or |$9.3\%$| of the base rate. Adding in other facial features implicated by past research substantially boosts the adjusted R 2 of the regression but barely changes the coefficient on heavy-facedness.
Correlation between Heavy-Faced and the Algorithm’s Prediction
Notes. This table shows the results of estimating a linear probability specification regressing algorithmic predictions of judges’ detain decision against different explanatory variables, using data from the validation set of cases from Mecklenburg County, NC. Each row of the table represents a different explanatory variable for the regression, while each column reports the results of a separate regression with different combinations of explanatory variables (as indicated by the filled-in coefficients and standard errors in the table). Algorithmic predictions of judges’ decisions come from applying the algorithm built with face images in the training data set to validation set observations. Data on heavy-faced, well-groomed, skin tone, attractiveness, competence, dominance, and trustworthiness come from subject ratings of mug shot images (see the text). Human guess variable comes from showing subjects pairs of mug shot images and asking subjects to identify the defendant they think the judge would be more likely to detain. Regression specifications also include indicators for unknown race and unknown gender. * p < .1; ** p < .05; *** p < .01.
In principle, the procedure could be iterated further. After all, well-groomed, heavy-faced plus previously known facial features all together still only explain |$27\%$| of the variation in the algorithm’s predictions of the judges’ decisions. As long as there is residual variation, the hypothesis generation crank could be turned again and again. Because our goal is not to fully explain judges’ decisions but to illustrate that the procedure works and is iterable, we leave this for future work (ideally done on data from other jurisdictions as well).
Here we consider whether the new hypotheses our procedure has generated meet our final criterion: empirical plausibility. We show that these facial features are new not just to the scientific literature but also apparently to criminal justice practitioners, before turning to whether these correlations might reflect some underlying causal relationship.
VI.A. Do These Hypotheses Predict What Judges Actually Do?
Empirical plausibility need not be implied by the fact that our new facial features are correlated with the algorithm’s predictions of judges’ decisions. The algorithm, after all, is not a perfect predictor. In principle, well-groomed and heavy-faced might be correlated with the part of the algorithm’s prediction that is unrelated to judge behavior, or m ( x ) − y .
In Table VI , we show that our two new hypotheses are indeed empirically plausible. The adjusted R 2 from regressing judges’ decisions against heavy-faced equals 0.0042 (column (1)), while for well-groomed the figure is 0.0021 (column (2)) and for both together the figure equals 0.0061 (column (3)). As a benchmark, the adjusted R 2 from all variables (other than the algorithm’s overall mug shot–based prediction) in explaining judges’ decisions equals 0.0218 (column (6)). So the explanatory power of our two novel hypotheses alone equals about |$28\%$| of what we get from all the variables together.
Do Well-Groomed and Heavy-Faced Correlate with Judge Decisions?
Notes. This table reports the results of estimating a linear probability specification of judges’ detain decisions against different explanatory variables in the validation set described in Table I . The algorithmic predictions of the judges’ detain decision come from our convolutional neural network algorithm built using the defendants’ face image as the only feature, using data from the training data set. Measures of defendant demographics and current arrest charge come from Mecklenburg County, NC, administrative data. Data on heavy-faced, well-groomed, skin tone, attractiveness, competence, dominance, and trustworthiness come from subject ratings of mug shot images (see the text). Human guess variable comes from showing subjects pairs of mug shot images and asking subjects to identify the defendant they think the judge would be more likely to detain. Regression specifications also include indicators for unknown race and unknown gender. * p < .1; ** p < .05; *** p < .01.
For a sense of the magnitude of these correlations, the coefficient on heavy-faced of −0.0234 (0.0036) in column (1) and on well-groomed of −0.0198 (0.0043) in column (2) imply that one standard deviation changes in each variable are associated with reduced detention rates equal to 2.8 and 2.0 percentage points, respectively, or |$12.0\%$| and |$8.9\%$| of the base rate. Interestingly, column (7) shows that heavy-faced remains statistically significant even when we control for the algorithm’s prediction. The discovery procedure led us to a facial feature that, when measured independently, captures signal above and beyond what the algorithm found. 67
VI.B. Do Practitioners Already Know This?
Our procedure has identified two hypotheses that are new to the existing research literature and to our study subjects. Yet the study subjects we have collected data from so far likely have relatively little experience with the criminal justice system. A reader might wonder: do experienced criminal justice practitioners already know that these “new” hypotheses affect judge decisions? The practitioners might have learned the influence of these facial features from day-to-day experience.
To answer this question, we carried out two smaller-scale data collections with a sample of N = 15 staff at a public defender’s office and a legal aid society. We first asked an open-ended question: on what basis do judges decide to detain versus release defendants pretrial? Practitioners talked about judge misunderstandings of the law, people’s prior criminal records, and judge underappreciation for the social contexts in which criminal records arise. Aside from the defendant’s race, nothing about the appearance of defendants was mentioned.
We showed practitioners pairs of actual mug shots and asked them to guess which person is more likely to be detained by a judge (as we had done with MTurk and Prolific workers). This yields a sample of 360 detention forecasts. After seeing these mug shots practitioners were asked an open-ended question about what they think matters about the defendant’s appearance for judge detention decisions. There were a few mentions of well-groomed and one mention of something related to heavy-faced, but these were far from the most frequently mentioned features, as seen in Online Appendix Figure A.XX .
The practitioner forecasts do indeed seem to be more accurate than those of “regular” study subjects. Table VII , column (5) shows that defendants whom the practitioners predict will be detained are 29.2 percentage points more likely to actually be detained, even after controlling for the other known determinants of detention from past research. This is nearly four times the effect of forecasts made by Prolific workers, as shown in the last column of Table VI . The practitioner guesses (unlike the regular study subjects) are even about as accurate as the algorithm; the R 2 from the practitioner guess (0.0165 in column (1)) is similar to the R 2 from the algorithm’s predictions (0.0166 in column (6)).
Results from the Criminal Justice Practitioner Sample
Notes. This table shows the results of estimating judges’ detain decisions using a linear probability specification of different explanatory variables on a subset of the validation set. The criminal justice practitioner’s guess about the judge’s decision comes from showing 15 different public defenders and legal aid society members actual mug shot images of defendants and asking them to report which defendant they believe the judge would be more likely to detain. The pairs are selected to be congruent in gender and race but discordant in detention outcome. The algorithmic predictions of judges’ detain decisions come from applying the algorithm, which is built with face images in the training data set, to validation set observations. Measures of defendant demographics and current arrest charge come from Mecklenburg County, NC, administrative data. Data on heavy-faced, well-groomed, skin tone, attractiveness, competence, dominance, and trustworthiness come from subject ratings of mug shot images (see the text). Regression specifications also include indicators for unknown race and unknown gender. * p < .1; ** p < .05; *** p < .01.
Yet practitioners do not seem to already know what the algorithm has discovered. We can see this in several ways in Table VII . First, the sum of the adjusted R 2 values from the bivariate regressions of judge decisions against practitioner guesses and judge decisions against the algorithm mug shot–based prediction is not so different from the adjusted R 2 from including both variables in the same regression (0.0165 + 0.0166 = 0.0331 from columns (1) plus (6), versus 0.0338 in column (7)). We see something similar for the novel features of well-groomed and heavy-faced specifically as well. 68 The practitioners and the algorithm seem to be tapping into largely unrelated signal.
VI.C. Exploring Causality
Are these novel features actually causally related to judge decisions? Fully answering that question is clearly beyond the scope of the present article. But we can present some additional evidence that is at least suggestive.
For starters we can rule out some obvious potential confounders. With the specific hypotheses in hand, identifying the most important concerns with confounding becomes much easier. In our application, well-groomed and heavy-faced could in principle be related to things like (say) the degree to which the defendant has a substance-abuse problem, is struggling with mental health, or their socioeconomic status. But as shown in a series of Online Appendix tables, we find that when we have study subjects independently label the mug shots in our validation data set for these features and then control for them, our novel hypotheses remain correlated with the algorithmic predictions of the judge and actual judge decisions. 69 We might wonder whether heavy-faced is simply a proxy for something that previous mock-trial-type studies suggest might matter for criminal justice decisions, “baby-faced” ( Berry and Zebrowitz-McArthur 1988 ). 70 But when we have subjects rate mug shots for baby-facedness, our full-faced measure remains strongly predictive of the algorithm’s predictions and actual judge decisions; see Online Appendix Tables A.XII and A.XVI .
In addition, we carried out a laboratory-style experiment with Prolific workers. We randomly morphed synthetic mug shot images in the direction of either higher or lower well-groomed (or full-faced), randomly assigned structured variables (current charge and prior record) to each image, explained to subjects the detention decision judges are asked to make, and then asked them which from each pair of subjects they would be more likely to detain if they were the judge. The framework from Mobius and Rosenblat (2006) helps clarify what this lab experiment gets us: appearance might affect how others treat us because others are reacting to something about our own appearance directly, because our appearance affects our own confidence, or because our appearance affects our effectiveness in oral communication. The experiment’s results shut down these latter two mechanisms and isolate the effects of something about appearance per se, recognizing it remains possible well-groomed and heavy-faced are correlated with some other aspect of appearance. 71
The study subjects recommend for detention those subjects with higher-risk structured variables (like current charge and prior record), which at the very least suggests they are taking the task seriously. Holding these other case characteristics constant, we find that the subjects are more likely to recommend for detention those defendants who are less well-groomed or less heavy-faced (see Online Appendix Table A.XVII ). Qualitatively, these results support the idea that well-groomed and heavy-faced could have a causal effect. It is not clear that the magnitudes in these experiments necessarily have much meaning: the subjects are not actual judges, and the context and structure of choice is very different from real detention decisions. Still, it is worth noting that the magnitudes implied by our results are nontrivial. Changing well-groomed or heavy-faced has the same effect on subject decisions as a movement within the predicted rearrest risk distribution of 4 and 6 percentile points, respectively (see Online Appendix C for details). Of course only an actual field experiment could conclusively determine causality here, but carrying out that type of field experiment might seem more worthwhile to an investigator in light of the lab experiment’s results.
Is this enough empirical support for these hypotheses to justify incurring the costs of causal testing? The empirical basis for these hypotheses would seem to be at least as strong as (or perhaps stronger than) the informal standard currently used to decide whether an idea is promising enough to test, which in our experience comes from some combination of observing the world, brainstorming, and perhaps some exploratory investigator-driven correlational analysis.
What might such causal testing look like? One possibility would follow in the spirit of Goldin and Rouse (2000) and compare detention decisions in settings where the defendant is more versus less visible to the judge to alter the salience of appearance. For example, many jurisdictions have continued to use some version of virtual hearings even after the pandemic. 72 In Chicago the court system has the defendant appear virtually but everyone else is in person, and the court system of its own volition has changed the size of the monitors used to display the defendant to court participants. One could imagine adding some planned variation to screen size or distance or angle to the judge. These video feeds could in principle be randomly selected for AI adjustment to the defendant’s level of well-groomedness or heavy-facedness (this would probably fall into a legal gray area). In the case of well-groomed, one could imagine a field experiment that changed this aspect of the defendant’s actual appearance prior to the court hearing. We are not claiming these are the right designs but intend only to illustrate that with new hypotheses in hand, economists are positioned to deploy the sort of creativity and rigorous testing that have become the hallmark of the field’s efforts at causal inference.
We have presented a new semi-automated procedure for hypothesis generation. We applied this new procedure to a concrete, socially important application: why judges jail some defendants and not others. Our procedure suggests two novel hypotheses: some defendants appear more well-groomed or more heavy-faced than others.
Beyond the specific findings from our illustrative application, our empirical analysis also illustrates a playbook for other applications. Start with a high-dimensional predictor m ( x ) of some behavior of interest. Build an unsupervised model of the data distribution, p ( x ). Then combine the models for m ( x ) and p ( x ) in a morphing procedure to generate new instances that answer the counterfactual question: what would a given instance look like with higher or lower likelihood of the outcome? Show morphed pairs of instances to participants and get them to name what they see as the differences between morphed instances. Get others to independently rate instances for whatever the new hypothesis is; do these labels correlate with both m ( x ) and the behavior of interest, y ? If so, we have a new hypothesis worth causal testing. This playbook is broadly applicable whenever three conditions are met.
The first condition is that we have a behavior we can statistically predict. The application we examine here fits because the behavior is clearly defined and measured for many cases. A study of, say, human creativity would be more challenging because it is not clear that it can be measured ( Said-Metwaly, Van den Noortgate, and Kyndt 2017 ). A study of why U.S. presidents use nuclear weapons during wartime would be challenging because there have been so few cases.
The second condition relates to what input data are available to predict behavior. Our procedure is likely to add only modest value in applications where we only have traditional structured variables, because those structured variables already make sense to people. Moreover the structured variables are usually already hypothesized to affect different behaviors, which is why economists ask about them on surveys. Our procedure will be more helpful with unstructured, high-dimensional data like images, language, and time series. The deeper point is that the collection of such high-dimensional data is often incidental to the scientific enterprise. We have images because the justice system photographs defendants during booking. Schools collect text from students as part of required assignments. Cellphones create location data as part of cell tower “pings.” These high-dimensional data implicitly contain an endless number of “features.”
Such high-dimensional data have already been found to predict outcomes in many economically relevant applications. Student essays predict graduation. Newspaper text predicts political slant of writers and editors. Federal Open Market Committee notes predict asset returns or volatility. X-ray images or EKG results predict doctor diagnoses (or misdiagnoses). Satellite images predict the income or health of a place. Many more relationships like these remain to be explored. From such prediction models, one could readily imagine human inspection of morphs leading to novel features. For example, suppose high-frequency data on volume and stock prices are used to predict future excess returns, for example, to understand when the market over- or undervalues a stock. Morphs of these time series might lead us to discover the kinds of price paths that produce overreaction. After all, some investors have even named such patterns (e.g., “head and shoulders,” “double bottom”) and trade on them.
The final condition is to be able to morph the input data to create new cases that differ in the predicted outcome. This requires some unsupervised learning technique to model the data distribution. The good news is that a number of such techniques are now available that work well with different types of high-dimensional data. We happen to use GANs here because they work well with images. But our procedure can accomodate a variety of unsupervised models. For example for text we can use other methods like Bidirectional Encoder Representations from Transformers ( Devlin et al. 2018 ), or for time series we could use variational auto-encoders ( Kingma and Welling 2013 ).
An open question is the degree to which our experimental pipeline could be changed by new technologies, and in particular by recent innovations in generative modeling. For example, several recent models allow people to create new synthetic images from text descriptions, and so could perhaps (eventually) provide alternative approaches to the creation of counterfactual instances. 73 Similarly, recent generative language models appear to be able to process images (e.g., GPT-4), although they are only recently publicly available. Because there is inevitably some uncertainty in forecasting what those tools will be able to do in the future, they seem unlikely to be able to help with the first stage of our procedure’s pipeline—build a predictive model of some behavior of interest. To see why, notice that methods like GPT-4 are unlikely to have access to data on judge decisions linked to mug shots. But the stage of our pipeline that GPT-4 could potentially be helpful for is to substitute for humans in “naming” the contrasts between the morphed pairs of counterfactual instances. Though speculative, such innovations potentially allow for more of the hypothesis generation procedure to be automated. We leave the exploration of these possibilities to future work.
Finally, it is worth emphasizing that hypothesis generation is not hypothesis testing. Each follows its own logic, and one procedure should not be expected to do both. Each requires different methods and approaches. What is needed to creatively produce new hypotheses is different from what is needed to carefully test a given hypothesis. Testing is about the curation of data, an effort to compare comparable subsets from the universe of all observations. But the carefully controlled experiment’s focus on isolating the role of a single prespecified factor limits the ability to generate new hypotheses. Generation is instead about bringing as much data to bear as possible, since the algorithm can only consider signal within the data available to it. The more diverse the data sources, the more scope for discovery. An algorithm could have discovered judge decisions are influenced by football losses, as in Eren and Mocan (2018) , but only if we thought to merge court records with massive archives of news stories as for example assembled by Leskovec, Backstrom, and Kleinberg (2009) . For generating ideas, creativity in experimental design useful for testing is replaced with creativity in data assembly and merging.
More generally, we hope to raise interest in the curious asymmetry we began with. Idea generation need not remain such an idiosyncratic or nebulous process. Our framework hopefully illustrates that this process can also be modeled. Our results illustrate that such activity could bear actual empirical fruit. At a minimum, these results will hopefully spur more theoretical and empirical work on hypothesis generation rather than leave this as a largely “prescientific” activity.
This is a revised version of Chicago Booth working paper 22-15 “Algorithmic Behavioral Science: Machine Learning as a Tool for Scientific Discovery.” We gratefully acknowledge support from the Alfred P. Sloan Foundation, Emmanuel Roman, and the Center for Applied Artificial Intelligence at the University of Chicago, and we thank Stephen Billings for generously sharing data. For valuable comments we thank Andrei Shleifer, Larry Katz, and five anonymous referees, as well as Marianne Bertrand, Jesse Bruhn, Steven Durlauf, Joel Ferguson, Emma Harrington, Supreet Kaur, Matteo Magnaricotte, Dev Patel, Betsy Levy Paluck, Roberto Rocha, Evan Rose, Suproteem Sarkar, Josh Schwartzstein, Nick Swanson, Nadav Tadelis, Richard Thaler, Alex Todorov, Jenny Wang, and Heather Yang, plus seminar participants at Bocconi, Brown, Columbia, ETH Zurich, Harvard, the London School of Economics, MIT, Stanford, the University of California Berkeley, the University of Chicago, the University of Pennsylvania, the University of Toronto, the 2022 Behavioral Economics Annual Meetings, and the 2022 NBER Summer Institute. For invaluable assistance with the data and analysis we thank Celia Cook, Logan Crowl, Arshia Elyaderani, and especially Jonas Knecht and James Ross. This research was reviewed by the University of Chicago Social and Behavioral Sciences Institutional Review Board (IRB20-0917) and deemed exempt because the project relies on secondary analysis of public data sources. All opinions and any errors are our own.
The question of hypothesis generation has been a vexing one in philosophy, as it appears to follow a process distinct from deduction and has been sometimes called “abduction” (see Schickore 2018 for an overview). A fascinating economic exploration of this topic can be found in Heckman and Singer (2017) , which outlines a strategy for how economists should proceed in the face of surprising empirical results. Finally, there is a small but growing literature that uses machine learning in science. In the next section we discuss how our approach is similar in some ways and different in others.
See Einav and Levin (2014) , Varian (2014) , Athey (2017) , Mullainathan and Spiess (2017) , Gentzkow, Kelly, and Taddy (2019) , and Adukia et al. (2023) on how these changes can affect economics.
In practice, there are a number of additional nuances, as discussed in Section III.A and Online Appendix A.A .
This is calculated for some of the most commonly used measures of predictive accuracy, area under the curve (AUC) and R 2 , recognizing that different measures could yield somewhat different shares of variation explained. We emphasize the word predictable here: past work has shown that judges are “noisy” and decisions are hard to predict ( Kahneman, Sibony, and Sunstein 2022 ). As a consequence, a predictive model of the judge can do better than the judge themselves ( Kleinberg et al. 2018 ).
In Section IV.B , we examine whether the mug shot’s predictive power can be explained by underlying risk differences. There, we tentatively conclude that the predictive power of the face likely reflects judicial error, but that working assumption is not essential to either our results or the ultimate goal of the article: uncovering hypotheses for later careful testing.
For reviews of the interpretability literature, see Doshi-Velez and Kim (2017) and Marcinkevičs and Vogt (2020) .
See Liu et al. (2019) , Narayanaswamy et al. (2020) , Lang et al. (2021) , and Ghandeharioun et al. (2022) .
For example, if every dog photo in a given training data set had been taken outdoors and every cat photo was taken indoors, the algorithm might learn what animal is in the image based in part on features of the background, which would lead the algorithm to perform poorly in a new data set of more representative images.
For example, for canonical computer science applications like image classification (does this photo contain an image of a dog or of a cat?), predictive accuracy (AUC) can be on the order of 0.99. In contrast, our model of judge decisions using the face only achieves an AUC of 0.625.
Of course even if the hypotheses that are generated are the result of idiosyncratic creativity, this can still be useful. For example, Swanson (1986 , 1988) generated two novel medical hypotheses: the possibility that magnesium affects migraines and that fish oil may alleviate Raynaud’s syndrome.
Conversely, given a data set, our procedure has a built-in advantage: one could imagine a huge number of hypotheses that, while possible, are not especially useful because they are not measurable. Our procedure is by construction guaranteed to generate hypotheses that are measurable in a data set.
For additional discussion, see Ludwig and Mullainathan (2023a) .
For example, isolating the causal effects of gender on labor market outcomes is a daunting task, but the clever test in Goldin and Rouse (2000) overcomes the identification challenges by using variation in screening of orchestra applicants.
See the clever paper by Grogger and Ridgeway (2006) that uses this source of variation to examine this question.
This is related to what Autor (2014) called “Polanyi’s paradox,” the idea that people’s understanding of how the world works is beyond our capacity to explicitly describe it. For discussions in psychology about the difficulty for people to access their own cognition, see Wilson (2004) and Pronin (2009) .
Consider a simple example. Suppose x = ( x 1 , …, x k ) is a k -dimensional binary vector, all possible values of x are equally likely, and the true function in nature relating x to y only depends on the first dimension of x so the function h 1 is the only true hypothesis and the only empirically plausible hypothesis. Even with such a simple true hypothesis, people can generate nonplausible hypotheses. Imagine a pair of data points ( x 0 , 0) and ( x 1 , 1). Since the data distribution is uniform, x 0 and x 1 will differ on |$\frac{k}{2}$| dimensions in expectation. A person looking at only one pair of observations would have a high chance of generating an empirically implausible hypothesis. Looking at more data, the probability of discovering an implausible hypothesis declines. But the problem remains.
Some canonical references include Breiman et al. (1984) , Breiman (2001) , Hastie et al. (2009) , and Jordan and Mitchell (2015) . For discussions about how machine learning connects to economics, see Belloni, Chernozhukov, and Hansen (2014) , Varian (2014) , Mullainathan and Spiess (2017) , Athey (2018) , and Athey and Imbens (2019) .
Of course there is not always a predictive signal in any given data application. But that is equally an issue for human hypothesis generation. At least with machine learning, we have formal procedures for determining whether there is any signal that holds out of sample.
The intuition here is quite straightforward. If two predictor variables are highly correlated, the weight that the algorithm puts on one versus the other can change from one draw of the data to the next depending on the idiosyncratic noise in the training data set, but since the variables are highly correlated, the predicted outcome values themselves (hence predictive accuracy) can be quite stable.
See Online Appendix Figure A.I , which shows the top nine eigenfaces for the data set we describe below, which together explain |$62\%$| of the variation.
Examples of applications of this type include Carleo et al. (2019) , He et al. (2019) , Davies et al. (2021) , Jumper et al. (2021) , and Pion-Tonachini et al. (2021) .
As other examples, researchers have found that retinal images alone can unexpectedly predict gender of patient or macular edema ( Narayanaswamy et al. 2020 ; Korot et al. 2021 ).
Sheetal, Feng, and Savani (2020) use machine learning to determine which of the long list of other survey variables collected as part of the World Values Survey best predict people’s support for unethical behavior. This application sits somewhat in between an investigator-generated hypothesis and the development of an entirely new hypothesis, in the sense that the procedure can only choose candidate hypotheses for unethical behavior from the set of variables the World Values Survey investigators thought to include on their questionnaire.
Closest is Miller et al. (2019) , which morphs EKG output but stops at the point of generating realistic morphs and does not carry this through to generating interpretable hypotheses.
Additional details about how the system works are found in Online Appendix A .
For Black non-Hispanics, the figures for Mecklenburg County versus the United States were |$33.3\%$| versus |$13.6\%$| . See https://www.census.gov/programs-surveys/sis/resources/data-tools/quickfacts.html .
Details on how we operationalize these variables are found in Online Appendix A .
The mug shot seems to have originated in Paris in the 1800s ( https://law.marquette.edu/facultyblog/2013/10/a-history-of-the-mug-shot/ ). The etymology of the term is unclear, possibly based on “mug” as slang for either the face or an “incompetent person” or “sucker” since only those who get caught are photographed by police ( https://www.etymonline.com/word/mug-shot ).
See https://mecksheriffweb.mecklenburgcountync.gov/ .
We partition the data by arrestee, not arrest, to ensure people show up in only one of the partitions to avoid inadvertent information “leakage” across data partitions.
As the Online Appendix tables show, while there are some changes to a few of the coefficients that relate the algorithm’s predictions to factors known from past research to shape human decisions, the core findings and conclusions about the importance of the defendant’s appearance and the two specific novel facial features we identify are similar.
Using the data on arrests up to July 17, 2019, we randomly reassign arrestees to three groups of similar size to our training, validation, and lock-box hold-out data sets, convert the data to long format (with one row for each arrest-and-variable) and calculate an F -test statistic for the joint null hypothesis that the difference in baseline characteristics are all zero, clustering standard errors by arrestee. We store that F -test statistic, rerun this procedure 1,000 times, and then report the share of splits with an F -statistic larger than the one observed for the original data partition.
For an example HIT task, see Online Appendix Figure A.II .
For age and skin tone, we calculated the average pairwise correlation between two labels sampled (without replacement) from the 10 possibilities, repeated across different random pairs. The Pearson correlation was 0.765 for skin tone, 0.741 for age, and between age assigned labels versus administrative data, 0.789. The maximum correlation between the average of the first k labels collected and the k + 1 label is not all that much higher for k = 1 than k = 9 (0.733 versus 0.837).
For an example of the consent form and instructions given to labelers, see Online Appendix Figures A.IV and A.V .
We actually collected at least three and at least five, but the averages turned out to be very close to the minimums, equal to 3.17 and 5.07, respectively.
For example, in Oosterhof and Todorov (2008) , Supplemental Materials Table S2, they report Cronbach’s α values of 0.95 for attractiveness, and 0.93 for both trustworthy and dominant.
See Online Appendix Figure A.VIII , which shows that the change in the correlation between the ( k + 1)th label with the mean of the first k labels declines after three labels.
For an example, see Online Appendix Figure A.IX .
We use the validation data set to estimate |$\hat{\beta }$| and then evaluate the accuracy of m p ( x ). Although this could lead to overfitting in principle, since we are only estimating a single parameter, this does not matter much in practice; we get very similar results if we randomly partition the validation data set by arrestee, use a random |$30\%$| of the validation data set to estimate the weights, then measure predictive performance in the other random |$70\%$| of the validation data set.
The mean squared area for a linear probability model’s predictions is related to the Brier score ( Brier 1950 ). For a discussion of how this relates to AUC and calibration, see Murphy (1973) .
Note how this comparison helps mitigate the problem that police arrest decisions could depend on a person’s face. When we regress rearrest against the mug shot, that estimated coefficient may be heavily influenced by how police arrest decisions respond to the defendant’s appearance. In contrast when we regress judge detention decisions against predicted rearrest risk, some of the variation across defendants in rearrest risk might come from the effect of the defendant’s appearance on the probability a police officer makes an arrest, but a great deal of the variation in predicted risk presumably comes from people’s behavior.
The average mug shot–predicted detention risk for the bottom and top quartiles equal 0.127 and 0.332; that difference times 2.880 implies a rearrest risk difference of 59.0 percentage points. By way of comparison, the difference in rearrest risk between those who are arrested for a felony crime rather than a less serious misdemeanor crime is equal to just 7.8 percentage points.
In our main exhibits, we impose a simple linear relationship between the algorithm’s predicted detention risk and known facial features like age or psychological variables, for ease of presentation. We show our results are qualitatively similar with less parametric specifications in Online Appendix Tables A.VI, A.VII, and A.VIII .
With a coefficient value of 0.0006 on age (measured in years), the algorithm tells us that even a full decade’s difference in age has |$5\%$| the impact on detention likelihood compared to the effects of gender (10 × 0.0006 = 0.6 percentage point higher likelihood of detention, versus 11.9 percentage points).
Online Appendix Table A.V shows that Hispanic ethnicity, which we measure from subject ratings from looking at mug shots, is not statistically significantly related to the algorithm’s predictions. Table II , column (2) showed that conditional on gender, Black defendants have slightly higher predicted detention odds than white defendants (0.3 percentage points), but this is not quite significant ( t = 1.3). Online Appendix Table A.V , column (1) shows that conditioning on Hispanic ethnicity and having stereotypically Black facial features—as measured in Eberhardt et al. (2006) —increases the size of the Black-white difference in predicted detention odds (now equal to 0.8 percentage points) as well as the difference’s statistical significance ( t = 2.2).
This comes from multiplying the effect of each 1 unit change in our 9-point scale associated, equal to 0.55, 0.91, and 0.48 percentage points, respectively, with the standard deviation of the average label for each psychological feature for each image, which equal 0.923, 0.911, and 0.844, respectively.
As discussed in Online Appendix Table A.III , we offer subjects a |${\$}$| 3.00 base rate for participation plus an incentive of 5 cents per correct guess. With 50 image pairs shown to each participant, they could increase their earnings by another |${\$}$| 2.50, or up to |$83\%$| above the base compensation.
Table III gives us another way to see how much of previously known features are rediscovered by the algorithm. That the algorithm’s prediction plus all previously known features yields an R 2 of just 0.0380 (column (7)), not much larger than with the algorithm alone, suggests the algorithm has discovered most of the signal in these known features. But not necessarily all: these other known features often do remain statistically significant predictors of judges’ decisions even after controlling for the algorithm’s predictions (last column). One possible reason is that, given finite samples, the algorithm has only imperfectly reconstructed factors such as “age” or “human guess.” Controlling for these factors directly adds additional signal.
Imagine a linear prediction function like |$m(x_1,x_2) = \widehat{\beta }_1 x_1 + \widehat{\beta }_2 x_2$| . If our best estimates suggested |$\widehat{\beta }_2=0$| , the maximum change to the prediction comes from incrementally changing x 1 .
As noted already, to avoid contributing to the stereotyping of minorities in discussions of crime, in our exhibits we show images for non-Hispanic white men, although in our HITs we use images representative of the larger defendant population.
Modeling p ( x ) through a supervised learning task would involve assembling a large set of images, having subjects label each image for whether they contain a realistic face, and then predicting those labels using the image pixels as inputs. But this supervised learning approach is costly because it requires extensive annotation of a large training data set.
Kaji, Manresa, and Pouliot (2020) and Athey et al. (2021 , 2022) are recent uses of GANs in economics.
Some ethical issues are worth considering. One is bias. With human hypothesis generation there is the risk people “see” an association that impugns some group yet has no basis in fact. In contrast our procedure by construction only produces empirically plausible hypotheses. A different concern is the vulnerability of deep learning to adversarial examples: tiny, almost imperceptible changes in an image changing its classification for the outcome y , so that mug shots that look almost identical (that is, are very “similar” in some visual image metric) have dramatically different m ( x ). This is a problem because tiny changes to an image don’t change the nature of the object; see Szegedy et al. (2013) and Goodfellow, Shlens, and Szegedy (2014) . In practice such instances are quite rare in nature, indeed, so rare they usually occur only if intentionally (maliciously) generated.
Online Appendix Figure A.XII gives an example of this task and the instructions given to participating subjects to complete it. Each subject was tested on 50 image pairs selected at random from a population of 100 images. Subjects were told that for every pair, one image was higher in some unknown feature, but not given details as to what the feature might be. As in the exercise for predicting detention, feedback was given immediately after selecting an image, and a 5 cent bonus was paid for every correct answer.
In principle this semantic grouping could be carried out in other ways, for example, with automated procedures involving natural-language processing.
See Online Appendix Table A.III for a high-level description of this human intelligence task, and Online Appendix Figure A.XIV for a sample of the task and the subject instructions.
We drop every token of just one or two characters in length, as well as connector words without real meaning for this purpose, like “had,” “the,” and “and,” as well as words that are relevant to our exercise but generic, like “jailed,” “judge,” and “image.”
We enlisted three research assistants blinded to the findings of this study and asked them to come up with semantic categories that captured all subject comments. Since each assistant mapped each subject comment to |$5\%$| of semantic categories on average, if the assistant mappings were totally uncorrelated, we would expect to see agreement of at least two assistant categorizations about |$5\%$| of the time. What we actually see is if one research assistant made an association, |$60\%$| of the time another assistant would make the same association. We assign a comment to a semantic category when at least two of the assistants agree on the categorization.
Moreover what subjects see does not seem to be particularly sensitive to which images they see. (As a reminder, each subject sees 50 morphed image pairs randomly selected from a larger bank of 100 morphed image pairs). If we start with a subject who says they saw “well-groomed” in the morphed image pairs they saw, for other subjects who saw 21 or fewer images in common (so saw mostly different images) they also report seeing well-groomed |$31\%$| of the time, versus |$35\%$| among the population. We select the threshold of 21 images because this is the smallest threshold in which at least 50 pairs of raters are considered.
See Online Appendix Table A.III and Online Appendix Figure A.XVI . This comes to a total of 192,280 individual labels, an average of 3.2 labels per image in the training set and an average of 10.8 labels per image in the validation set. Sampling labels from different workers on the same image, these ratings have a correlation of 0.14.
It turns out that skin tone is another feature that is correlated with well-groomed, so we orthogonalize on that as well as well-groomed. To simplify the discussion, we use “well-groomed” as a stand-in for both features we orthogonalize against, well-groomed plus skin tone.
To see why, consider the mechanics of the procedure. Since we orthogonalize as we create morphs, we would need labels at each morphing step. This would entail us producing candidate steps (new morphs), collecting data on each of the candidates, picking one that has the same well-groomed value, and then repeating. Moreover, until the labels are collected at a given step, the next step could not be taken. Since producing a final morph requires hundreds of such intermediate morphing steps, the whole process would be so time- and resource-consuming as to be infeasible.
While we can predict demographic features like race and age (above/below median age) nearly perfectly, with AUC values close to 1, for predicting well-groomed, the mean absolute error of our OOS prediction is 0.63, which is plus or minus over half a slider value for this 9-point-scaled variable. One reason it is harder to predict well-groomed is because the labels, which come from human subjects looking at and labeling mug shots, are themselves noisy, which introduces irreducible error.
For additional details see Online Appendix Figure A.XVII and Online Appendix B .
There are a few additional technical steps required, discussed in Online Appendix B . For details on the HIT we use to get subjects to name the new hypothesis from looking at orthogonalized morphs, and the follow-up HIT to generate independent labels for that new hypothesis or facial feature, see Online Appendix Table A.III .
See Online Appendix Figure A.XIX .
The adjusted R 2 of including the practitioner forecasts plus well-groomed and heavy-facedness together (column (3), equal to 0.0246) is not that different from the sum of the R 2 values from including just the practitioner forecasts (0.0165 in column (1)) plus that from including just well-groomed and heavy-faced (equal to 0.0131 in Table VII , column (2)).
In Online Appendix Table A.IX we show that controlling for one obvious indicator of a substance abuse issue—arrest for drugs—does not seem to substantially change the relationship between full-faced or well-groomed and the predicted detention decision. Online Appendix Tables A.X and A.XI show a qualitatively similar pattern of results for the defendant’s mental health and socioeconomic status, which we measure by getting a separate sample of subjects to independently rate validation–data set mug shots. We see qualitatively similar results when the dependent variable is the actual rather than predicted judge decision; see Online Appendix Tables A.XIII, A.XIV, and A.XV .
Characteristics of having a baby face included large eyes, narrow chin, small nose, and high, raised eyebrows. For a discussion of some of the larger literature on how that feature shapes the reactions of other people generally, see Zebrowitz et al. (2009) .
For additional details, see Online Appendix C .
See https://www.nolo.com/covid-19/virtual-criminal-court-appearances-in-the-time-of-the-covid-19.html .
See https://stablediffusionweb.com/ and https://openai.com/product/dall-e-2 .
The data underlying this article are available in the Harvard Dataverse, https://doi.org/10.7910/DVN/ILO46V ( Ludwig and Mullainathan 2023b ).
Adukia Anjali , Eble Alex , Harrison Emileigh , Birali Runesha Hakizumwami , Szasz Teodora , “ What We Teach about Race and Gender: Representation in Images and Text of Children’s Books ,” Quarterly Journal of Economics , 138 ( 2023 ), 2225 – 2285 . https://doi.org/10.1093/qje/qjad028
Google Scholar
Angelova Victoria , Dobbie Will S. , Yang Crystal S. , “ Algorithmic Recommendations and Human Discretion ,” NBER Working Paper no. 31747, 2023 . https://doi.org/10.3386/w31747
Arnold David , Dobbie Will S. , Hull Peter , “ Measuring Racial Discrimination in Bail Decisions ,” NBER Working Paper no. 26999, 2020. https://doi.org/10.3386/w26999
Arnold David , Dobbie Will , Yang Crystal S. , “ Racial Bias in Bail Decisions ,” Quarterly Journal of Economics , 133 ( 2018 ), 1885 – 1932 . https://doi.org/10.1093/qje/qjy012
Athey Susan , “ Beyond Prediction: Using Big Data for Policy Problems ,” Science , 355 ( 2017 ), 483 – 485 . https://doi.org/10.1126/science.aal4321
Athey Susan , “ The Impact of Machine Learning on Economics ,” in The Economics of Artificial Intelligence: An Agenda , Ajay Agrawal, Joshua Gans, and Avi Goldfarb, eds. (Chicago: University of Chicago Press , 2018 ), 507 – 547 .
Athey Susan , Imbens Guido W. , “ Machine Learning Methods That Economists Should Know About ,” Annual Review of Economics , 11 ( 2019 ), 685 – 725 . https://doi.org/10.1146/annurev-economics-080217-053433
Athey Susan , Imbens Guido W. , Metzger Jonas , Munro Evan , “ Using Wasserstein Generative Adversarial Networks for the Design of Monte Carlo Simulations ,” Journal of Econometrics , ( 2021 ), 105076. https://doi.org/10.1016/j.jeconom.2020.09.013
Athey Susan , Karlan Dean , Palikot Emil , Yuan Yuan , “ Smiles in Profiles: Improving Fairness and Efficiency Using Estimates of User Preferences in Online Marketplaces ,” NBER Working Paper no. 30633 , 2022 . https://doi.org/10.3386/w30633
Autor David , “ Polanyi’s Paradox and the Shape of Employment Growth ,” NBER Working Paper no. 20485 , 2014 . https://doi.org/10.3386/w20485
Avitzour Eliana , Choen Adi , Joel Daphna , Lavy Victor , “ On the Origins of Gender-Biased Behavior: The Role of Explicit and Implicit Stereotypes ,” NBER Working Paper no. 27818 , 2020 . https://doi.org/10.3386/w27818
Baehrens David , Schroeter Timon , Harmeling Stefan , Kawanabe Motoaki , Hansen Katja , Müller Klaus-Robert , “ How to Explain Individual Classification Decisions ,” Journal of Machine Learning Research , 11 ( 2010 ), 1803 – 1831 .
Baltrušaitis Tadas , Ahuja Chaitanya , Morency Louis-Philippe , “ Multimodal Machine Learning: A Survey and Taxonomy ,” IEEE Transactions on Pattern Analysis and Machine Intelligence , 41 ( 2019 ), 423 – 443 . https://doi.org/10.1109/TPAMI.2018.2798607
Begall Sabine , Červený Jaroslav , Neef Julia , Vojtěch Oldřich , Burda Hynek , “ Magnetic Alignment in Grazing and Resting Cattle and Deer ,” Proceedings of the National Academy of Sciences , 105 ( 2008 ), 13451 – 13455 . https://doi.org/10.1073/pnas.0803650105
Belloni Alexandre , Chernozhukov Victor , Hansen Christian , “ High-Dimensional Methods and Inference on Structural and Treatment Effects ,” Journal of Economic Perspectives , 28 ( 2014 ), 29 – 50 . https://doi.org/10.1257/jep.28.2.29
Berry Diane S. , Zebrowitz-McArthur Leslie , “ What’s in a Face? Facial Maturity and the Attribution of Legal Responsibility ,” Personality and Social Psychology Bulletin , 14 ( 1988 ), 23 – 33 . https://doi.org/10.1177/0146167288141003
Bertrand Marianne , Mullainathan Sendhil , “ Are Emily and Greg More Employable than Lakisha and Jamal? A Field Experiment on Labor Market Discrimination ,” American Economic Review , 94 ( 2004 ), 991 – 1013 . https://doi.org/10.1257/0002828042002561
Bjornstrom Eileen E. S. , Kaufman Robert L. , Peterson Ruth D. , Slater Michael D. , “ Race and Ethnic Representations of Lawbreakers and Victims in Crime News: A National Study of Television Coverage ,” Social Problems , 57 ( 2010 ), 269 – 293 . https://doi.org/10.1525/sp.2010.57.2.269
Breiman Leo , “ Random Forests ,” Machine Learning , 45 ( 2001 ), 5 – 32 . https://doi.org/10.1023/A:1010933404324
Breiman Leo , Friedman Jerome H. , Olshen Richard A. , Stone Charles J. , Classification and Regression Trees (London: Routledge , 1984 ). https://doi.org/10.1201/9781315139470
Google Preview
Brier Glenn W. , “ Verification of Forecasts Expressed in Terms of Probability ,” Monthly Weather Review , 78 ( 1950 ), 1 – 3 . https://doi.org/10.1175/1520-0493(1950)078<0001:VOFEIT>2.0.CO;2
Carleo Giuseppe , Cirac Ignacio , Cranmer Kyle , Daudet Laurent , Schuld Maria , Tishby Naftali , Vogt-Maranto Leslie , Zdeborová Lenka , “ Machine Learning and the Physical Sciences ,” Reviews of Modern Physics , 91 ( 2019 ), 045002 . https://doi.org/10.1103/RevModPhys.91.045002
Chen Daniel L. , Moskowitz Tobias J. , Shue Kelly , “ Decision Making under the Gambler’s Fallacy: Evidence from Asylum Judges, Loan Officers, and Baseball Umpires ,” Quarterly Journal of Economics , 131 ( 2016 ), 1181 – 1242 . https://doi.org/10.1093/qje/qjw017
Chen Daniel L. , Philippe Arnaud , “ Clash of Norms: Judicial Leniency on Defendant Birthdays ,” Journal of Economic Behavior & Organization , 211 ( 2023 ), 324 – 344 . https://doi.org/10.1016/j.jebo.2023.05.002
Dahl Gordon B. , Knepper Matthew M. , “ Age Discrimination across the Business Cycle ,” NBER Working Paper no. 27581 , 2020 . https://doi.org/10.3386/w27581
Davies Alex , Veličković Petar , Buesing Lars , Blackwell Sam , Zheng Daniel , Tomašev Nenad , Tanburn Richard , Battaglia Peter , Blundell Charles , Juhász András , Lackenby Marc , Williamson Geordie , Hassabis Demis , Kohli Pushmeet , “ Advancing Mathematics by Guiding Human Intuition with AI ,” Nature , 600 ( 2021 ), 70 – 74 . https://doi.org/10.1038/s41586-021-04086-x
Devlin Jacob , Chang Ming-Wei , Lee Kenton , Toutanova Kristina , “ BERT: Pre-Training of Deep Bidirectional Transformers for Language Understanding ,” arXiv preprint arXiv:1810.04805 , 2018 . https://doi.org/10.48550/arXiv.1810.04805
Dobbie Will , Goldin Jacob , Yang Crystal S. , “ The Effects of Pretrial Detention on Conviction, Future Crime, and Employment: Evidence from Randomly Assigned Judges ,” American Economic Review , 108 ( 2018 ), 201 – 240 . https://doi.org/10.1257/aer.20161503
Dobbie Will , Yang Crystal S. , “ The US Pretrial System: Balancing Individual Rights and Public Interests ,” Journal of Economic Perspectives , 35 ( 2021 ), 49 – 70 . https://doi.org/10.1257/jep.35.4.49
Doshi-Velez Finale , Kim Been , “ Towards a Rigorous Science of Interpretable Machine Learning ,” arXiv preprint arXiv:1702.08608 , 2017 . https://doi.org/10.48550/arXiv.1702.08608
Eberhardt Jennifer L. , Davies Paul G. , Purdie-Vaughns Valerie J. , Lynn Johnson Sheri , “ Looking Deathworthy: Perceived Stereotypicality of Black Defendants Predicts Capital-Sentencing Outcomes ,” Psychological Science , 17 ( 2006 ), 383 – 386 . https://doi.org/10.1111/j.1467-9280.2006.01716.x
Einav Liran , Levin Jonathan , “ The Data Revolution and Economic Analysis ,” Innovation Policy and the Economy , 14 ( 2014 ), 1 – 24 . https://doi.org/10.1086/674019
Eren Ozkan , Mocan Naci , “ Emotional Judges and Unlucky Juveniles ,” American Economic Journal: Applied Economics , 10 ( 2018 ), 171 – 205 . https://doi.org/10.1257/app.20160390
Frieze Irene Hanson , Olson Josephine E. , Russell June , “ Attractiveness and Income for Men and Women in Management ,” Journal of Applied Social Psychology , 21 ( 1991 ), 1039 – 1057 . https://doi.org/10.1111/j.1559-1816.1991.tb00458.x
Fryer Roland G., Jr , “ An Empirical Analysis of Racial Differences in Police Use of Force: A Response ,” Journal of Political Economy , 128 ( 2020 ), 4003 – 4008 . https://doi.org/10.1086/710977
Fudenberg Drew , Liang Annie , “ Predicting and Understanding Initial Play ,” American Economic Review , 109 ( 2019 ), 4112 – 4141 . https://doi.org/10.1257/aer.20180654
Gentzkow Matthew , Kelly Bryan , Taddy Matt , “ Text as Data ,” Journal of Economic Literature , 57 ( 2019 ), 535 – 574 . https://doi.org/10.1257/jel.20181020
Ghandeharioun Asma , Kim Been , Li Chun-Liang , Jou Brendan , Eoff Brian , Picard Rosalind W. , “ DISSECT: Disentangled Simultaneous Explanations via Concept Traversals ,” arXiv preprint arXiv:2105.15164 2022 . https://doi.org/10.48550/arXiv.2105.15164
Goldin Claudia , Rouse Cecilia , “ Orchestrating Impartiality: The Impact of ‘Blind’ Auditions on Female Musicians ,” American Economic Review , 90 ( 2000 ), 715 – 741 . https://doi.org/10.1257/aer.90.4.715
Goncalves Felipe , Mello Steven , “ A Few Bad Apples? Racial Bias in Policing ,” American Economic Review , 111 ( 2021 ), 1406 – 1441 . https://doi.org/10.1257/aer.20181607
Goodfellow Ian , Pouget-Abadie Jean , Mirza Mehdi , Xu Bing , Warde-Farley David , Ozair Sherjil , Courville Aaron , Bengio Yoshua , “ Generative Adversarial Nets ,” Advances in Neural Information Processing Systems , 27 ( 2014 ), 2672 – 2680 .
Goodfellow Ian J. , Shlens Jonathon , Szegedy Christian , “ Explaining and Harnessing Adversarial Examples ,” arXiv preprint arXiv:1412.6572 , 2014 . https://doi.org/10.48550/arXiv.1412.6572
Grogger Jeffrey , Ridgeway Greg , “ Testing for Racial Profiling in Traffic Stops from Behind a Veil of Darkness ,” Journal of the American Statistical Association , 101 ( 2006 ), 878 – 887 . https://doi.org/10.1198/016214506000000168
Hastie Trevor , Tibshirani Robert , Friedman Jerome H. , Friedman Jerome H. , The Elements of Statistical Learning: Data Mining, Inference, and Prediction , vol. 2 (Berlin: Springer , 2009 ).
He Siyu , Li Yin , Feng Yu , Ho Shirley , Ravanbakhsh Siamak , Chen Wei , Póczos Barnabás , “ Learning to Predict the Cosmological Structure Formation ,” Proceedings of the National Academy of Sciences , 116 ( 2019 ), 13825 – 13832 . https://doi.org/10.1073/pnas.1821458116
Heckman James J. , Singer Burton , “ Abducting Economics ,” American Economic Review , 107 ( 2017 ), 298 – 302 . https://doi.org/10.1257/aer.p20171118
Heyes Anthony , Saberian Soodeh , “ Temperature and Decisions: Evidence from 207,000 Court Cases ,” American Economic Journal: Applied Economics , 11 ( 2019 ), 238 – 265 . https://doi.org/10.1257/app.20170223
Hoekstra Mark , Sloan CarlyWill , “ Does Race Matter for Police Use of Force? Evidence from 911 Calls ,” American Economic Review , 112 ( 2022 ), 827 – 860 . https://doi.org/10.1257/aer.20201292
Hunter Margaret , “ The Persistent Problem of Colorism: Skin Tone, Status, and Inequality ,” Sociology Compass , 1 ( 2007 ), 237 – 254 . https://doi.org/10.1111/j.1751-9020.2007.00006.x
Jordan Michael I. , Mitchell Tom M. , “ Machine Learning: Trends, Perspectives, and Prospects ,” Science , 349 ( 2015 ), 255 – 260 . https://doi.org/10.1126/science.aaa8415
Jumper John , Evans Richard , Pritzel Alexander , Green Tim , Figurnov Michael , Ronneberger Olaf , Tunyasuvunakool Kathryn , Bates Russ , Žídek Augustin , Potapenko Anna et al. , “ Highly Accurate Protein Structure Prediction with AlphaFold ,” Nature , 596 ( 2021 ), 583 – 589 . https://doi.org/10.1038/s41586-021-03819-2
Jung Jongbin , Concannon Connor , Shroff Ravi , Goel Sharad , Goldstein Daniel G. , “ Simple Rules for Complex Decisions ,” SSRN working paper , 2017 . https://doi.org/10.2139/ssrn.2919024
Kahneman Daniel , Sibony Olivier , Sunstein C. R , Noise (London: HarperCollins , 2022 ).
Kaji Tetsuya , Manresa Elena , Pouliot Guillaume , “ An Adversarial Approach to Structural Estimation ,” University of Chicago, Becker Friedman Institute for Economics Working Paper No. 2020-144 , 2020 . https://doi.org/10.2139/ssrn.3706365
Kingma Diederik P. , Welling Max , “ Auto-Encoding Variational Bayes ,” arXiv preprint arXiv:1312.6114 , 2013 . https://doi.org/10.48550/arXiv.1312.6114
Kleinberg Jon , Lakkaraju Himabindu , Leskovec Jure , Ludwig Jens , Mullainathan Sendhil , “ Human Decisions and Machine Predictions ,” Quarterly Journal of Economics , 133 ( 2018 ), 237 – 293 . https://doi.org/10.1093/qje/qjx032
Korot Edward , Pontikos Nikolas , Liu Xiaoxuan , Wagner Siegfried K. , Faes Livia , Huemer Josef , Balaskas Konstantinos , Denniston Alastair K. , Khawaja Anthony , Keane Pearse A. , “ Predicting Sex from Retinal Fundus Photographs Using Automated Deep Learning ,” Scientific Reports , 11 ( 2021 ), 10286 . https://doi.org/10.1038/s41598-021-89743-x
Lahat Dana , Adali Tülay , Jutten Christian , “ Multimodal Data Fusion: An Overview of Methods, Challenges, and Prospects ,” Proceedings of the IEEE , 103 ( 2015 ), 1449 – 1477 . https://doi.org/10.1109/JPROC.2015.2460697
Lang Oran , Gandelsman Yossi , Yarom Michal , Wald Yoav , Elidan Gal , Hassidim Avinatan , Freeman William T , Isola Phillip , Globerson Amir , Irani Michal , et al. , “ Explaining in Style: Training a GAN to Explain a Classifier in StyleSpace ,” paper presented at the IEEE/CVF International Conference on Computer Vision , 2021. https://doi.org/10.1109/ICCV48922.2021.00073
Leskovec Jure , Backstrom Lars , Kleinberg Jon , “ Meme-Tracking and the Dynamics of the News Cycle ,” paper presented at the 15th ACM SIGKDD international conference on Knowledge discovery and data mining, 2009. https://doi.org/10.1145/1557019.1557077
Little Anthony C. , Jones Benedict C. , DeBruine Lisa M. , “ Facial Attractiveness: Evolutionary Based Research ,” Philosophical Transactions of the Royal Society B: Biological Sciences , 366 ( 2011 ), 1638 – 1659 . https://doi.org/10.1098/rstb.2010.0404
Liu Shusen , Kailkhura Bhavya , Loveland Donald , Han Yong , “ Generative Counterfactual Introspection for Explainable Deep Learning ,” paper presented at the IEEE Global Conference on Signal and Information Processing (GlobalSIP) , 2019. https://doi.org/10.1109/GlobalSIP45357.2019.8969491
Ludwig Jens , Mullainathan Sendhil , “ Machine Learning as a Tool for Hypothesis Generation ,” NBER Working Paper no. 31017 , 2023a . https://doi.org/10.3386/w31017
Ludwig Jens , Mullainathan Sendhil , “ Replication Data for: ‘Machine Learning as a Tool for Hypothesis Generation’ ,” ( 2023b ), Harvard Dataverse. https://doi.org/10.7910/DVN/ILO46V .
Marcinkevičs Ričards , Vogt Julia E. , “ Interpretability and Explainability: A Machine Learning Zoo Mini-Tour ,” arXiv preprint arXiv:2012.01805 , 2020 . https://doi.org/10.48550/arXiv.2012.01805
Miller Andrew , Obermeyer Ziad , Cunningham John , Mullainathan Sendhil , “ Discriminative Regularization for Latent Variable Models with Applications to Electrocardiography ,” paper presented at the International Conference on Machine Learning , 2019.
Mobius Markus M. , Rosenblat Tanya S. , “ Why Beauty Matters ,” American Economic Review , 96 ( 2006 ), 222 – 235 . https://doi.org/10.1257/000282806776157515
Mobley R. Keith , An Introduction to Predictive Maintenance (Amsterdam: Elsevier , 2002 ).
Mullainathan Sendhil , Obermeyer Ziad , “ Diagnosing Physician Error: A Machine Learning Approach to Low-Value Health Care ,” Quarterly Journal of Economics , 137 ( 2022 ), 679 – 727 . https://doi.org/10.1093/qje/qjab046
Mullainathan Sendhil , Spiess Jann , “ Machine Learning: an Applied Econometric Approach ,” Journal of Economic Perspectives , 31 ( 2017 ), 87 – 106 . https://doi.org/10.1257/jep.31.2.87
Murphy Allan H. , “ A New Vector Partition of the Probability Score ,” Journal of Applied Meteorology and Climatology , 12 ( 1973 ), 595 – 600 . https://doi.org/10.1175/1520-0450(1973)012<0595:ANVPOT>2.0.CO;2
Nalisnick Eric , Matsukawa Akihiro , Whye Teh Yee , Gorur Dilan , Lakshminarayanan Balaji , “ Do Deep Generative Models Know What They Don’t Know? ,” arXiv preprint arXiv:1810.09136 , 2018 . https://doi.org/10.48550/arXiv.1810.09136
Narayanaswamy Arunachalam , Venugopalan Subhashini , Webster Dale R. , Peng Lily , Corrado Greg S. , Ruamviboonsuk Paisan , Bavishi Pinal , Brenner Michael , Nelson Philip C. , Varadarajan Avinash V. , “ Scientific Discovery by Generating Counterfactuals Using Image Translation ,” in International Conference on Medical Image Computing and Computer-Assisted Intervention , (Berlin: Springer , 2020), 273 – 283 . https://doi.org/10.1007/978-3-030-59710-8_27
Neumark David , Burn Ian , Button Patrick , “ Experimental Age Discrimination Evidence and the Heckman Critique ,” American Economic Review , 106 ( 2016 ), 303 – 308 . https://doi.org/10.1257/aer.p20161008
Norouzzadeh Mohammad Sadegh , Nguyen Anh , Kosmala Margaret , Swanson Alexandra , S. Palmer Meredith , Packer Craig , Clune Jeff , “ Automatically Identifying, Counting, and Describing Wild Animals in Camera-Trap Images with Deep Learning ,” Proceedings of the National Academy of Sciences , 115 ( 2018 ), E5716 – E5725 . https://doi.org/10.1073/pnas.1719367115
Oosterhof Nikolaas N. , Todorov Alexander , “ The Functional Basis of Face Evaluation ,” Proceedings of the National Academy of Sciences , 105 ( 2008 ), 11087 – 11092 . https://doi.org/10.1073/pnas.0805664105
Peterson Joshua C. , Bourgin David D. , Agrawal Mayank , Reichman Daniel , Griffiths Thomas L. , “ Using Large-Scale Experiments and Machine Learning to Discover Theories of Human Decision-Making ,” Science , 372 ( 2021 ), 1209 – 1214 . https://doi.org/10.1126/science.abe2629
Pierson Emma , Cutler David M. , Leskovec Jure , Mullainathan Sendhil , Obermeyer Ziad , “ An Algorithmic Approach to Reducing Unexplained Pain Disparities in Underserved Populations ,” Nature Medicine , 27 ( 2021 ), 136 – 140 . https://doi.org/10.1038/s41591-020-01192-7
Pion-Tonachini Luca , Bouchard Kristofer , Garcia Martin Hector , Peisert Sean , Bradley Holtz W. , Aswani Anil , Dwivedi Dipankar , Wainwright Haruko , Pilania Ghanshyam , Nachman Benjamin et al. “ Learning from Learning Machines: A New Generation of AI Technology to Meet the Needs of Science ,” arXiv preprint arXiv:2111.13786 , 2021 . https://doi.org/10.48550/arXiv.2111.13786
Popper Karl , The Logic of Scientific Discovery (London: Routledge , 2nd ed. 2002 ). https://doi.org/10.4324/9780203994627
Pronin Emily , “ The Introspection Illusion ,” Advances in Experimental Social Psychology , 41 ( 2009 ), 1 – 67 . https://doi.org/10.1016/S0065-2601(08)00401-2
Ramachandram Dhanesh , Taylor Graham W. , “ Deep Multimodal Learning: A Survey on Recent Advances and Trends ,” IEEE Signal Processing Magazine , 34 ( 2017 ), 96 – 108 . https://doi.org/10.1109/MSP.2017.2738401
Rambachan Ashesh , “ Identifying Prediction Mistakes in Observational Data ,” Harvard University Working Paper, 2021 . www.nber.org/system/files/chapters/c14777/c14777.pdf
Said-Metwaly Sameh , Van den Noortgate Wim , Kyndt Eva , “ Approaches to Measuring Creativity: A Systematic Literature Review ,” Creativity: Theories–Research-Applications , 4 ( 2017 ), 238 – 275 . https://doi.org/10.1515/ctra-2017-0013
Schickore Jutta , “ Scientific Discovery ,” in The Stanford Encyclopedia of Philosophy , Edward N. Zalta, ed. (Stanford, CA: Stanford University , 2018).
Schlag Pierre , “ Law and Phrenology ,” Harvard Law Review , 110 ( 1997 ), 877 – 921 . https://doi.org/10.2307/1342231
Sheetal Abhishek , Feng Zhiyu , Savani Krishna , “ Using Machine Learning to Generate Novel Hypotheses: Increasing Optimism about COVID-19 Makes People Less Willing to Justify Unethical Behaviors ,” Psychological Science , 31 ( 2020 ), 1222 – 1235 . https://doi.org/10.1177/0956797620959594
Simonyan Karen , Vedaldi Andrea , Zisserman Andrew , “ Deep Inside Convolutional Networks: Visualising Image Classification Models and Saliency Maps ,” paper presented at the Workshop at International Conference on Learning Representations , 2014.
Sirovich Lawrence , Kirby Michael , “ Low-Dimensional Procedure for the Characterization of Human Faces ,” Journal of the Optical Society of America A , 4 ( 1987 ), 519 – 524 . https://doi.org/10.1364/JOSAA.4.000519
Sunstein Cass R. , “ Governing by Algorithm? No Noise and (Potentially) Less Bias ,” Duke Law Journal , 71 ( 2021 ), 1175 – 1205 . https://doi.org/10.2139/ssrn.3925240
Swanson Don R. , “ Fish Oil, Raynaud’s Syndrome, and Undiscovered Public Knowledge ,” Perspectives in Biology and Medicine , 30 ( 1986 ), 7 – 18 . https://doi.org/10.1353/pbm.1986.0087
Swanson Don R. , “ Migraine and Magnesium: Eleven Neglected Connections ,” Perspectives in Biology and Medicine , 31 ( 1988 ), 526 – 557 . https://doi.org/10.1353/pbm.1988.0009
Szegedy Christian , Zaremba Wojciech , Sutskever Ilya , Bruna Joan , Erhan Dumitru , Goodfellow Ian , Fergus Rob , “ Intriguing Properties of Neural Networks ,” arXiv preprint arXiv:1312.6199 , 2013 . https://doi.org/10.48550/arXiv.1312.6199
Todorov Alexander , Oh DongWon , “ The Structure and Perceptual Basis of Social Judgments from Faces. in Advances in Experimental Social Psychology , B. Gawronski, ed. (Amsterdam: Elsevier , 2021 ), 189–245.
Todorov Alexander , Olivola Christopher Y. , Dotsch Ron , Mende-Siedlecki Peter , “ Social Attributions from Faces: Determinants, Consequences, Accuracy, and Functional Significance ,” Annual Review of Psychology , 66 ( 2015 ), 519 – 545 . https://doi.org/10.1146/annurev-psych-113011-143831
Varian Hal R. , “ Big Data: New Tricks for Econometrics ,” Journal of Economic Perspectives , 28 ( 2014 ), 3 – 28 . https://doi.org/10.1257/jep.28.2.3
Wilson Timothy D. , Strangers to Ourselves (Cambridge, MA: Harvard University Press , 2004 ).
Yuhas Ben P. , Goldstein Moise H. , Sejnowski Terrence J. , “ Integration of Acoustic and Visual Speech Signals Using Neural Networks ,” IEEE Communications Magazine , 27 ( 1989 ), 65 – 71 . https://doi.org/10.1109/35.41402
Zebrowitz Leslie A. , Luevano Victor X. , Bronstad Philip M. , Aharon Itzhak , “ Neural Activation to Babyfaced Men Matches Activation to Babies ,” Social Neuroscience , 4 ( 2009 ), 1 – 10 . https://doi.org/10.1080/17470910701676236
Supplementary data
Email alerts, citing articles via.
- Recommend to Your Librarian
Affiliations
- Online ISSN 1531-4650
- Print ISSN 0033-5533
- Copyright © 2024 President and Fellows of Harvard College
- About Oxford Academic
- Publish journals with us
- University press partners
- What we publish
- New features
- Open access
- Institutional account management
- Rights and permissions
- Get help with access
- Accessibility
- Advertising
- Media enquiries
- Oxford University Press
- Oxford Languages
- University of Oxford
Oxford University Press is a department of the University of Oxford. It furthers the University's objective of excellence in research, scholarship, and education by publishing worldwide
- Copyright © 2024 Oxford University Press
- Cookie settings
- Cookie policy
- Privacy policy
- Legal notice
This Feature Is Available To Subscribers Only
Sign In or Create an Account
This PDF is available to Subscribers Only
For full access to this pdf, sign in to an existing account, or purchase an annual subscription.
Making Sense of the Relationship Between Adaptive Thinking and Heuristics in Evolutionary Psychology
- Original Article
- Open access
- Published: 09 February 2021
- Volume 16 , pages 16–29, ( 2021 )
Cite this article
You have full access to this open access article
- Shunkichi Matsumoto ORCID: orcid.org/0000-0002-3333-2963 1
4710 Accesses
2 Citations
11 Altmetric
Explore all metrics
In recent years, quite a few evolutionary psychologists have come to embrace a heuristic interpretation of the discipline. They claim that, no matter how methodologically incomplete, adaptive thinking works fine as a good heuristic that effectively reduces the hypothesis space by generating novel and promising hypotheses that can eventually be empirically tested. The purpose of this article is to elucidate the use of heuristics in evolutionary psychology, thereby clarifying the role adaptive thinking has to play. To that end, two typical heuristic interpretations—Machery’s "bootstrap strategy" and Goldfinch’s heuristically streamlined evolutionary psychology—are examined, focusing on the relationship between adaptive thinking and heuristics. The article draws two primary conclusions. The first is that the reliability of the heuristic hypothesis generation procedure (in the context of discovery) should count no less than the conclusiveness of the final testing procedure (in the context of justification) in establishing scientific facts; nature does not always get the last word. Philosophy also counts. The second is that adaptive thinking constitutes a core heuristic in evolutionary psychology that provides the discipline with its raison d'être , but this is only possible when adaptive thinking is substantiated with sufficient historical underpinnings.
Similar content being viewed by others
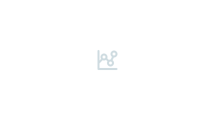
Statistical tests, P values, confidence intervals, and power: a guide to misinterpretations
What is the function of confirmation bias.
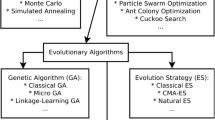
Evolutionary algorithms and their applications to engineering problems
Avoid common mistakes on your manuscript.
Introduction
The controversy revolving around evolutionary psychology does not seem to be subsiding; however, the focus of the debate has been gradually shifting. Before, the trend used to be that the debate primarily revolved around the objections raised by critics from a methodological point of view. Some problematized the stability of the Pleistocene environment as the human Environment of Evolutionary Adaptedness (EEA) that is necessary for natural selection to work out robust solutions over an evolutionary time scale (Sterelny 1995 ; Sterelny and Griffiths 1999 ; Buller 2005 ; Richerson and Boyd 2005 ).
Some others cast a question about the grain with which the ancient adaptive problems should be identified: does fear in general constitute a single adaptive problem, or should the fear of predators and that of heights be considered as separate problems to be subsumed under a related domain (Sterelny and Griffiths 1999 ; Buller 2005 )?
Still others doubted the feasibility or logical consistency of adaptive thinking. For the purpose of identifying ancient adaptive problems with sufficient precision to be able to pick out only relevant aspects of the environment while screening out unnecessary information, we need to know quite a good deal about the trait in advance (Griffiths 1996 ; Buller 2005 ; Laland and Brown 2011 ).
In recent years, however, quite a few evolutionary psychologists or their defenders have come to emphasize evolutionary psychology as a scientific discipline based on heuristic predictions and eventual confirmation (Gigerenzer and Selten 2001 ; Andrews et al. 2002 ; Goldfinch 2015 ; Hagen 2016 ; Machery forthcoming). According to them, no matter how methodologically incomplete, adaptive thinking (a core methodology of evolutionary psychology to be clarified later) works fine as a good heuristic in effectively reducing the hypothesis space. The methodological objections raised by critics we just synopsized above do not doom evolutionary psychology, because they all concern the context of discovery, not the context of justification: if the hypotheses discovered ought to have been justified in terms of methodological consistency in advance of their final testing, those objections would surely be crucial. However, if the truthfulness of the hypotheses is to be entirely determined by final testing, it will not make any serious difference which methodology is employed in the process of discovering hypotheses, or where they come from. After all, it is not philosophy (methodology) but nature that gets the last word (Symons 1992 ).
For example, Edouard Machery advocates such a heuristic interpretation. According to him, what he calls "the forward-looking heuristic" ( adaptive thinking , in our terms) assumes a central place in evolutionary psychology reasoning. Yet, at the same time, he remarks that although it is useful for discovering our psychological traits, it need not be necessary. Sometimes it is supplemented by a backward-looking reasoning, and at other times its speculative character needs to be constrained by some other non-evolutionary sources of information. Since the forward-looking heuristic is just a heuristic, it need not stand on its own as a complete and self-contained hypothesis generator (Machery forthcoming).
Andrew Goldfinch brings such a heuristic aspect to the fore and argues that it is this aspect that evolutionary psychology as a scientific practice conducted by today’s most pragmatic researchers on a daily basis ought to be identified with. According to his diagnosis, the reason why evolutionary psychology at its early stage provoked such fierce antipathy from critics is because its leading pioneers such as Leda Cosmides, John Tooby, David Buss, and others ventured to sell "a package of strong views" that presented it as a "game-changer," a "scientific revolution" in psychology, the unifying principle in behavioral and social sciences, or even having a bearing on public policy making. Instead, Goldfinch insists that evolutionary psychology be "streamlined" by letting go of these sorts of excessive promises unlikely ever to be fulfilled, in order to circumvent irrelevant criticisms against it. Evolutionary psychology should rather be taken as a hypothesis-driven empirical science, the daily practice of which consists in a kind of adaptationist version of the hypothetico-deductive method; that is, focusing on adaptive problems, hypothesizing dedicated solutions to the problems, and then subjecting these hypotheses to testing (Goldfinch 2015 , p. 132).
However, as we will argue later, heuristics come with their own problems. Using heuristics to find solutions to given problems means committing ourselves to more or less reductive explanations to make them tractable by reducing the complexity of the system concerned. But this, in turn, makes it prone to oversimplified conceptions of its components, contexts, environments, and their interactions, likely resulting in "reductionist biases" (Wimsatt 2007 ).
This is especially true of evolutionary psychology where, as we will see later, adaptive thinking is feasible only in so far as some drastic simplifying assumptions are in place, such as those concerning the existence of nonselective forces, persistence of ancient selection pressures, effects of epistatic interactions, or existence of developmental or phylogenetic constraints.
The purpose of this article is to elucidate the use of heuristics in evolutionary psychology and thereby clarify the role adaptive thinking has to play. To that end, in the next section, the situation will be reviewed in which the pioneers of evolutionary psychology tried to advertise adaptive thinking as the proprietary methodology that enabled them with its heuristic function to enjoy a methodological advantage over that of beleaguered sociobiologists.
In the third section, I will take up one major methodological objection to evolutionary psychology as a case example—the charge of circular reasoning in identifying adaptive problems—and examine whether Machery’s idea of "bootstrap strategy" as a response to it—that adaptive thinking can get away with the charge by being supplemented by reverse engineering—can address it properly.
In the following section, I will turn to Goldfinch’s proposal of heuristically streamlined evolutionary psychology. There I will focus on his proposal of division of labor between evolutionary psychology as managing heuristic hypothesis generation and adjacent relevant fields as justifying them in order to see if it can circumvent the conventional charge that evolutionary psychological hypothesization wants evidential supports.
In the fifth section, I will introduce Matthew Rellihan’s analysis of the type of adaptive thinking employed in evolutionary psychology (Rellihan 2012 ) in order to clarify the role of adaptive thinking and thereby identify one of the core (biasing) assumptions inherent in the program.
In the sixth section, I will readdress the initial issue of the possibility of construing evolutionary psychology as a heuristic project and what to make of the relationship between adaptive thinking and heuristics.
Two primary points will be drawn by the end of this article. The first is that the reliability of the heuristic hypothesis generation procedure (in the context of discovery) should count no less than the conclusiveness of the final testing procedure (in the context of justification) in establishing scientific facts; nature does not necessarily get the last word. Philosophy also counts. The second is that adaptive thinking constitutes a core heuristic in evolutionary psychology that provides the discipline with its raison d'être but that this is only possible when adaptive thinking is substantiated with sufficient historical underpinnings.
Adaptive Thinking in Evolutionary Psychology
In this brief section, for the argument to follow, I will preliminarily delineate what adaptive thinking means in evolutionary psychology and how its pioneers appealed to it to establish their methodological advantage over other approaches.
Adaptive thinking is a type of reasoning in which, on the basis of prespecified selection pressures, structures or behaviors of the organism that must have been evolved as adaptive responses are inferred; it is a forward-looking inference from past functions (survival values) to current forms. Usually adaptive thinking is contrasted with reverse engineering, which infers backwardly from current forms to past functions. Put another way, "Reverse engineering infers the adaptive problem from the solution which was adopted. Adaptive thinking infers the solution from the adaptive problem" (Griffiths 1996 , p. 514).
The pioneers of evolutionary psychology initially advertised their methodological advantage over that of sociobiologists of older generations by appealing to adaptive thinking, or what they called "evolutionary functional analysis" (Tooby and Cosmides 1992 ). Sociobiologists used to be accused of untestable post hoc storytelling about the historical origins they conjectured by the reverse engineering of currently observed traits. In contrast, evolutionary psychologists are supposed to be exempted from such accusations because the end-products of their forward-looking reasoning, namely, the psychological mechanisms possessed by modern humans, can directly be put to empirical testing. This way, adaptive thinking can better lend itself to the typical formula of hypothesis-driven scientific reasoning—focusing on adaptive problems, hypothesizing solutions to them, and finally confirming them empirically.
For example, Cosmides, Tooby, and Barkow ( 1992 , p. 11) states that, "One virtue of this approach is that it is immune to the usual (but often vacuous) accusation of post hoc storytelling: The researcher has predicted in advance the properties of the mechanism."
Adaptive thinking is also supposed to have heuristic value. By figuring out the solutions that might have solved postulated adaptive problems, adaptive thinking is expected to lead to the discovery of previously unknown features. Thus, "an explanation for a fact by a theory cannot be post hoc if the fact was unknown until after it was predicted by the theory and if the reason the fact is known at all is because of the theory" (Tooby and Cosmides 1992 , p. 75).
At the same time, it can serve as a kind of winnow to narrow down the vast hypothesis space by identifying "out of the millions of possible theories" those "that are more likely to be true" (Tooby and Cosmides 1998 , p. 197), namely, by sorting out a handful of promising hypotheses from the rest of those unworthy of serious consideration in accordance with whether they make evolutionary sense.
The Charge of Circular Reasoning in Identifying Adaptive Problems and Machery’s Idea of the "Bootstrap Strategy"
Many different types of criticism have been levelled against adaptive thinking as a central methodology of evolutionary psychology. Here, I will pick out one, for it concerns the feasibility—or internal logical consistency—of the forward-looking inference itself, and thus I think is central among all. That is the problem related to identifying adaptations in our ancestral past (Rose and Lauder 1996 ; Buller 2005 ; Richardson 2007 ; Fox and Westneat 2010 ; Laland and Brown 2011 ). It may not be impossible to infer whether a given trait is an adaptation by conjecturing which traits might have been favored by natural selection in the past, provided that sufficient knowledge of evolutionary processes and ancestral environmental conditions are available (Cosmides and Tooby 1987 ; Tooby and Cosmides 1990 ). However, whether such conjectures can be meaningfully made in practice is a matter of controversy. Since researchers are rarely completely ignorant of the features of the trait in question, they may be in a position to cheat and fudge an evolutionary scenario that predicts features of the trait that are already known (Laland and Brown 2011 , p. 133). If this is the case, the credibility of the confirmation process of those predictions where they are confronted with the current data—be it through experiments, questionnaires, or cross-cultural studies—will be compromised.
Against this conventional criticism, Machery argues that evolutionary psychologists can escape the charge by construing the whole reasoning procedure as a "bootstrap strategy" in which the preceding reverse-engineering and the following adaptive thinking work together in tandem. He writes,
Moreover, the forward-looking heuristic is often complemented by a bootstrap strategy. Evolutionary psychologists often use the knowledge accumulated by psychologists about the structure of known psychological traits to infer what past selective pressures might have been (backward-looking reasoning). These hypotheses about past selective pressures are then used to develop novel hypotheses about some properties of these known psychological traits or to attempt to discover new psychological traits (forward-looking reasoning). (Machery forthcoming, p. 8).
Edward Hagen also endorses Machery’s view:
Used separately, these two types of arguments each do have limitations. Used together, however, and in combination with well-tested theories from evolutionary biology, they are able to make genuine contributions to understanding human evolution. (Hagen 2016 , p. 149)
The point is that the hypotheses about past selective pressures reached by backward-looking reasoning can then serve as a springboard for further conducting a forward-looking one for developing novel hypotheses about some properties of the traits in question. Forward-looking reasoning cannot stand alone, indeed. But with the auxiliary help of backward-looking reasoning based on already known traits—on top of other circumstantial evidence available—it can "boot up" and perform the desired function.
Herein lies the problem: is it really a virtuous circle as Machery and Hagen envisage, or might it be perhaps a vicious circle as critics suspect? (Caporael 1989 ; Davies 1999 ; Buller 2005 ) Even those proponents admit that both forward- and backward-looking reasonings are in themselves incomplete—a forward-looking one being beset with the problem of the incomplete identifiability of the EEA adaptive problems at the outset, and a backward-looking one being saddled with the problem of underdetermination by available evidence among the multiple competing hypotheses consistent with what we observe now. Footnote 1 If so, can two in-themselves incomplete methods complement each other to form a more reliable one? Or might it not be the case that an uncertain inference method that builds on an in-itself uncertain premise will end up with something like a house of cards?
Since Machery does not give us concrete examples of how this strategy works, let us consider instead the case Hagen makes. Following the above quote, Hagen argues as follows to instantiate the bootstrap strategy:
The universal aspects of mate preferences of contemporary women provide a decent hypothesis for the mate preferences of ancestral women, for instance, …. These hypothesized ancestral female preferences are then essential components of the EEA of male-mating strategies of humans …. (Hagen 2016 , p. 149)
This sounds slightly simplistic. First, how can he assert that those aspects of women currently observed are "universal"? He seems to neglect the variations existing among contemporary women (e.g., not all women prefer high-status men). Second, he identifies the ancestral female preferences hypothesized through backward-looking reasoning immediately with the essential components of the EEA constituting the male adaptive problems from which to start forward-looking reasoning. However, the "hypothesized" preferences are not the actual ones, unless confirmed so.
Finally, if we reconstruct the reasoning presented in his sketchy argument, using standard evolutionary psychology doctrine to fill in the missing links, we would have the following chain of reasoning:
The universal features of modern women that prefer certain types of male behavioral patterns (industriousness, strife for high status, etc.) can be projected onto those of ancestral women using backward-looking reasoning.
These projected female features in turn can be used to infer the sorts of selection pressures that contemporary men were forced to face in order to survive the intrasexual competition of the time.
These ancient selection pressures, combined with what is predicted from Trivers’s parental investment theory that men were placed under severer intrasexual competition (Trivers 1972 ), are supposed to serve as a springboard for the subsequent forward-looking reasoning to hypothesize the specialized psychological mechanisms that our male ancestors should have evolved by the end of the Pleistocene, in regard to a mating strategy.
These evolved male mechanisms are what modern men are supposed to inherit virtually unchanged due to the lack of necessary time for evolution of complex adaptations after the end of the Pleistocene. Footnote 2
This explains why modern men are innately disposed to behave in a way that conforms to preferences of modern women observed at the outset.
Now, whether this chain of reasoning as a composite of backward- and forward-looking reasonings proves to be a successful case of the bootstrap strategy to yield a novel prediction or collapses into an unproductive circularity seems to hinge upon whether there is any chance to subject the end products of this chain (i.e., predicted male mechanisms) to empirical confirmation that can be designed independently of the corresponding behavioral patterns supposed to supervene on those mechanisms (in terms of, say, identifying the underlying neuronal circuits responsible for those patterns). If, on the other hand, the intended confirmation was a mere reassurance of those patterns observed at the outset, then the whole detour to and from the ancestral environments would be redundant. Yet, at least up to the present point in time, the alleged confirmation conducted by evolutionary psychologists has not met this requirement.
For instance, let us take up Buss’s well-received theory of jealousy (Buss et al. 1992 ; Buss 2000 , 2008 ). This is a partial application of Trivers’s theory of parental investment and sexual selection as a middle-range evolutionary theory to a specifically human case (Trivers 1972 ). Footnote 3 According to it, the sex that is more heavily investing in offspring tends to be choosier in mate selection; whereas the less-investing sex tends to be more promiscuous and simultaneously forced into competitive intrasexual selection.
Now, on the one hand, human females are, as in most other mammalian and bird species, investing more than males; therefore, Trivers’s theory applies to humans. However, on the other, there are some peculiarities among humans; as female ovulation is concealed, paternity uncertainty becomes a problem among males. In addition, human males are, differently from other primate relatives, considerably committed to parental investment, especially postnatally. Trivers’s theory predicts that these factors can lead men to be "choosier" in their own manner, namely, more vigilant about the reproductive activities of their mates than other primate counterparts. If a man’s partner has an affair with another man, it poses a serious threat to his reproductive prospect as he is not certain about the paternity of the child his partner bears, and, hence, he risks misallocating his resources on a child he did not father. In contrast, his partner’s emotional attachment to another man is less serious as long as she is sexually faithful. On the other hand, for a woman (as the higher-investing sex and, therefore, in need of resources), her partner’s emotional attachment to another woman poses a serious threat to her reproductive prospects, for then part of the resources she was supposed to receive will likely be allocated to another woman. In contrast, her partner having brief extramarital affairs is of lesser concern as long as he is emotionally faithful.
Buss predicts, from these considerations, that human males must have evolved an innate jealousy module that makes them more alert to their mates’ sexual infidelities, whereas their female counterparts must have evolved one that makes them more alert to their mates’ emotional infidelities.
Now let us turn to the hitherto attempted verification of this prediction. Buss and others have conducted it primarily counting on either self-reports on forced-choice questionnaires or the measurement of the physiological stress responses of male and female test subjects who were asked to imagine an uncomfortable scene where their partner, with whom the subject is deeply involved, is being (emotionally or sexually) unfaithful with another person. The researchers then reported that their predictions about sex-biased jealousy sensitivity were confirmed (Buss et al. 1992 ).
The problem I see is, however, whatever the result—whether those predictions be positively or negatively confirmed—what is sought to be verified here is whether the relevant jealous emotions (or some associated bodily responses) are aroused in subjects, not whether they are brought about by some underlying mechanisms . Buss should indeed be credited for designing experiments to confirm, in a quantitative measure, the extent to which the types of jealous emotions entertained by the different sexes differ. Still, until it is demonstrated—or, at least, the experimental design is proposed to demonstrate—that the behavioral differences are caused by some underlying modules hardwired differently between the sexes, the alleged confirmation of the sex-biased sensitivity will remain as a mere reassurance or an accommodation of known facts, rather than a prediction of novel phenomena, albeit adding some quantitative underpinning.
This situation is typical of hypothesization and confirmation in evolutionary psychology; it is usually the case that the required mechanisms are presumed to lie at the underlying information-processing level as something responsible for the corresponding behavioral outputs, namely, they are postulated just as hypothetical placeholders for what we can currently observe. They do not have any chance to play substantial roles in the confirmation of hypotheses, at least for the time being, and thus are theoretically unnecessary. This will make the detour to and from the ancient EEA seem redundant; Machery's remedy against the charge of circularity does not seem promising.
Goldfinch’s Proposal of Heuristically Streamlined Evolutionary Psychology
Goldfinch adds another twist to this issue. He admits that if given an explanatory interpretation, evolutionary psychology may well end up with a circular explanation consisting in projecting forward into the present that which was once obtained by projecting back into the past that which is currently observed. However, evolutionary psychology can manage to break loose from this vicious circle charge by being interpreted as a heuristic project, not as an explanatory project.
The key to this interpretation is a distinction between explanations and heuristic hypothesis generations. According to Goldfinch, evolutionary psychology should not be considered to provide final explanations of phenomena; rather, it should be regarded as just producing hypotheses to be confirmed later. The difference between the two can be put as follows: while explanations are expected not only to provide hypotheses but also to eventually justify them, heuristic projects can stop short of this justificatory procedure.
For instance, if one is to propose via adaptive thinking that trait T is an adaptation for X , all that is required of heuristic projects is to make the following inference in the form of a conditional (here X refers to some adaptive problem, T some trait as a solution to X , C some properties exhibited by T , and P some observable phenomena derived from C ): "If trait T is an adaptation for X , trait T should have configuration C , and so we should find phenomenon P " (Goldfinch 2015 , p. 144). Making a further factual claim that trait T is actually an adaptation for X is not in the purview of a heuristic project, much less justifying it.
According to Goldfinch, it is because evolutionary psychology hypotheses have been unduly deemed as self-contained final pronouncements that unnecessary objections expressing doubt about them are raised. Instead, if they are considered to be just hypotheses waiting (and wanting) to be verified, then those objections will disappear, and other adjacent relevant disciplines will take up the baton and put them to the test.
I wonder if we can separate hypotheses from explanations in such a dichotomous manner. In my eyes, they are more or less mutually exchangeable concepts. In science, every time a new thus-far-unknown phenomenon is discovered, scientists try to explain it, no matter how tentative that explanation may be. Any and all explanations are fallible and left open to revision, thus assuming a hypothetical character. On the other hand, any hypotheses are products of the attempt to explain thus-far-unexplained phenomena and, therefore, are themselves already kinds of explanations, with their provisional character being emphasized. It is not that mere hypotheses waiting to be tested and full-blown explanations established as true are qualitatively separated. Hence, it does not seem that, by simply renaming the concept from "explanations" to "hypotheses," the situation will change so drastically that the critical backlash from skeptics will subside.
The situation will rather be that the probability of hypotheses becoming true propositions is a function of both the reliability of the procedure generating them (i.e., context of discovery) and the conclusiveness of the final testing procedure (i.e., context of justification). The more reliable the former procedure already is, the more likely to be true the hypothesis generated will be, and the less crucial role the latter procedure will have. In contrast, if the former is error-prone in some way or another, the evidential criteria for the eventual confirmation will have to be all the more demanding. Furthermore, it is often the case that the initial errors made in the context of discovery have an overarching biasing effect on practices done in the context of justification without being noticed by practitioners. We can substantiate this point by referring to Wimsatt’s argument about the "reductionist problem-solving heuristics" (Wimsatt 2007 ; see also Tversky and Kahneman 1974 ). Footnote 4
According to Wimsatt, using heuristics is applying a kind of reductionistic research strategy for reducing the complexity of the system to a tractable level by introducing simplifications, idealizations, or approximations. Footnote 5 As such, it is prone to the same kind of errors or biases of reductionism in general. Among them, the most relevant to our current argument is that, "The errors produced by using a heuristic are not random but systematically biased" (Wimsatt 2007 , p. 76). That is, even different heuristics slightly modified by different practitioners to get better fits tend to "generate errors in the same direction" (2007, p. 84) if they share the initial biasing assumptions introduced by the originators of the research program.
Wimsatt goes on to argue that "heuristics can hide their tracks" (2007, p. 86). That is, those multiple reductionist models sharing the initial basic assumptions have a generic tendency to constrain the overall direction along which to expect the results in such a way that each model covers up each other's inadequacies; rather than producing independent results either confirming or disconfirming a hypothesis, heuristic models frequently create pseudo-robust conclusions confirming initial theoretical biases. Therefore, instead of fulfilling the expected function of significantly reducing the hypothesis space, the use of heuristics may often end up entrenching the underlying biases and thus compromising the falsifiability, as it were, of the research program.
In this same vein, Paul Griffiths ( 1996 ) notes a "negative heuristic effect" of adaptive thinking to draw attention not just to the ease with which an adaptive hypothesis can be invoked to accommodate existing or novel findings but, more importantly, to its tendency to rule out other equally plausible hypotheses borne out by different sets of findings once a particular hypothesis has become predominant. As an example, he raises the case for parent/offspring conflict that was first put forward by Trivers ( 1974 ) and immediately gained considerable momentum among sociobiologists (and has remained in some circles up to today). Although the idea that the parent wants to conserve its resources for future offspring, whereas the offspring wants as much as it can get now, is quite appealing, Griffiths notes, empirical evidence for parent/offspring tug-of-war (especially over weaning) is very weak. On the contrary, he cites Bateson's ( 1994 ) review of a number of studies that failed to find aggressive interactions at weaning in various species; namely, studies that report voluntary weaning on offspring's part or ones that found both parties signaling to each other in order to coordinate peaceful weaning, although these studies have largely been underappreciated. The point here is that a predominant hypothesis can suppress others by heuristically (i.e., selectively) picking out evidence that fits it most.
These points of argument have a great bearing on Goldfinch's proposal of division of labor between evolutionary psychology as engaging in just hypothesis generation and adjacent relevant fields as undertaking the task of justifying them: the task ought to be taken on by evolutionary psychologists themselves of systematically investigating heuristic biases and their adverse effects inherent in the program. If it is delegated to practitioners in other fields, they will more likely try to collect evidences either confirming or disconfirming the artifacts created by those biases rather than detect the underlying biases themselves that even evolutionary psychologists could not notice.
In order to substantiate these points, in the next section I will look at Rellihan’s argument on the nature of "Adaptationism and Adaptive Thinking in Evolutionary Psychology" (Rellihan 2012 ) and bring out one of the core biasing assumptions initially introduced by the pioneers into the program.
Rellihan’s Analysis of Adaptationism in Evolutionary Psychology
According to Rellihan, the type of adaptive thinking typical of evolutionary psychology is in fact what can be termed "strong adaptationism." This is the idea that the force of natural selection is so powerful and overwhelming to any obstacles that, once given perennial selection pressures, the destination of adaptive evolution is uniquely predictable no matter what phenotypes a given population may have started with in the distant past—a much stronger version than the one evolutionary psychologists typically think themselves committed to.
Rellihan notes that the usual justification by evolutionary psychologists for the use of adaptive thinking is given by appealing to a rather modest form of adaptationism, to the effect that "the mind’s adaptive complexity reveals it to be a product of selection" (Rellihan 2012 , p. 245). But he argues that this justification is insufficient, for the mind’s being an adaptation is only a necessary and not a sufficient condition for the validity of adaptive thinking. Even granted that most of our mind's features are designed to perform fine-tuned adaptive functions, it does not warrant the deducibility of those functions from hypothesized initial conditions. Therefore, much stronger assumptions are needed in order to be able to predict the psychological mechanisms possessed by modern humans on the basis of knowledge about the selection pressures faced by our ancestors. Footnote 6 Then what are those assumptions?
First, Rellihan defines adaptive thinking succinctly as an inference strategy in accordance with the following formula:
From the fact that there was a significant selection pressure for organism O to evolve trait T , infer that O has evolved T. (Rellihan 2012 , p. 249) Footnote 7
Then he introduces the notion of an "adaptive landscape" as a graphical way to represent what this inference strategy will amount to. Imagine an N -dimensional graph with a separate axis for each conceivable phenotypic property. Movement along an axis corresponds to quantitative change of the value of its associated property. Thus, such phenotypic properties as height, beak size, linguistic capacity, speaking in general terms, are represented by corresponding axes. By adding one extra axis representing the relative fitness of the organism that comprises those properties, we will then get an adaptive landscape for the species concerned.
In this landscape, organisms are represented as points on the surface, populations as clusters of associated points, evolution as the process in which these clusters travel across the surface, and evolution by natural selection as the process in which populations ascend fitness peaks. Nonadaptive evolutionary change such as through genetic drift is represented as wandering about along a contour line. And saltatory evolution, say by means of macromutations, if any, is represented as a leap to a different position far from the current one. Thus, the power of selection can be thought of as the extent to which a population's evolutionary trajectory is determined by the surrounding topography as a gradual hill-climbing process without leaps. Since adaptationism is a position that sees the power of selection as by far the most predominant of all the factors influencing evolution, adaptationists insist the trajectory be mostly (if not exclusively) determined by the topography (Orzack and Sober 1994 ).
Now evolutionary psychology is committed to adaptive thinking, a special type of adaptationism with a predictive focus, according to which a population’s evolutionary trajectory, and hence its destination all the way from its current position, can be predicted mostly by taking the power of selection into account. Therefore, according to Rellihan, in order to justify the use of adaptive thinking, we must presuppose the validity of what he calls "strong adaptationism," defined as follows:
The evolutionary path of a population across the adaptive landscape is largely determined by (and therefore predictable on the basis of) the population’s current position on the landscape together with the neighboring topography of the landscape. (Rellihan 2012 , p. 256)
However, when we begin to take into account the actual constraints of the epistatic interaction between component phenotypes—what Kauffman ( 1995 ) calls "conflicting constraints"—the fitness contribution of one trait becomes contingent upon the presence or absence of another one, thus, contributions by different traits become more and more nonadditive. Accordingly, the landscape becomes increasingly rugged with many a local optimum appearing here and there.
In such a situation, it will be difficult to predict the evolutionary destination (and the trajectory leading to it) solely on the basis of the landscape’s topography plus the current position of the population. If the landscape were simple and smooth, such that there were only one global peak as with Mt. Fuji, we would not have to specify the point of departure and the intermediary pathway in order to predict that a population would eventually arrive at the peak; from anywhere on the landscape there could always be found a continuously uphill route leading to the peak. In contrast, if the landscape gets more and more rugged as a result of epistasis, it gets increasingly harder to predict to which peak a population will eventually ascend and along what route.
What does this all amount to for evolutionary psychology? If the actual landscape involved in the evolutionary history of the human mind happens to be simple and smooth, with a single optimal solution specifiable to the ancient problems, no matter what psychological phenotypes our ancestral population was initially possessed of, it is assured of evolving that solution over time, as the orthodoxy of evolutionary psychology teaches us. On the other hand, if the landscape becomes more or less rugged, just being able to specify the initial problems is not nearly sufficient to predict the end products unless at the same time sufficient information is provided both about the state of ancestral phenotypes and the sequential intermediary stages of their evolution.
What then does the actual landscape look like? Rellihan argues that there is evidence that it has always been considerably rugged. In the original "NK model" put forward by Kauffman and Levin ( 1987 ), where N represents the number of distinct components of the system—genes in the case of a genotype, traits in the case of a phenotype—and K the degree of epistatic interaction between them, K = 0 corresponds to the case where the landscape is smooth, containing a single global peak, whereas at K = 2 "the landscape already begins to resemble the French Alps," and at the extreme of K = N -1 "it looks more like a bed of nails." The number of peaks increases exponentially as either K or N increases. According to Kauffman and Levin’s mathematical model, there will be 10 28 peaks when N = 100 and K = 99, and 10 48 peaks when N = 1024 and K = 1. In contrast, the human genome contains around 25,000 to 30,000 genes and our phenotypes consists of thousands of distinguishable traits (Rellihan 2012 , p. 260).
The lesson to be drawn from the consideration above is that although the use of adaptive thinking is essential in evolutionary psychology theorizing, the condition in which it can be justified is extremely limited.
Recall Wimsatt's argument that using heuristics is applying a kind of reductionistic research strategy for reducing the complexity of the system by introducing simplifications or idealizations. This disregard of the effects of epistasis constitutes one of the core simplifying assumptions set in the discipline by its pioneers and henceforth having been inherited by inertia , as it were, by their followers. This is not an innocuous but a pernicious type of simplification, for it misleads us into accepting a caricatured picture of evolution on the grounds of the irresistibility of a naive intuition that the mind’s adaptive complexity reveals it to be a product of selection.
Adaptive thinking is an inference justifiable only in idealized conditions: the extent to which epistatic interaction occurs should be extremely low, as we just saw above. Besides that, evolutionary forces other than selection should be negligible, ancient selection pressures should have remained robust at least until the relevant psychological adaptations were set in place, and there should not be any major developmental constraints that compromise the optimizing force of natural selection. Accordingly, if adaptive thinking is to serve as an effective heuristic that can significantly reduce the hypothesis space by picking out promising candidates worthy of serious consideration, those idealized conditions must have approximated the historical conditions in which evolution of the human mind has actually taken place. On the other hand, if these conditions are too ideal for any actual historical condition to come close to, adaptive thinking will not be serviceable even as an effective heuristic.
This state of affairs may be better understood with the help of the following analogous situation. Galileo’s law of free fall obtains only in idealized conditions where there are no other forces than gravity that act on the object. The reason that this law can approximate the behavior of an actual object falling in the air is because the effect of air resistance is negligible compared to the force of gravity. However, the stronger the viscosity of the surrounding medium becomes, the less reliable will the application of this idealization to an actual condition be, such that the law can no longer predict the movement of an object sinking in the water, for instance.
The Relationship Between Adaptive Thinking and Heuristics
Now we will get back to the initial issue of whether evolutionary psychology can be construed as a heuristic program. What has become of the claim that since empirical data gets the last word in confirming hypotheses, adaptive thinking can settle for the minor status of just a heuristic device?
First, we want to ensure that adaptive thinking constitutes a core heuristic in evolutionary psychology, which even its proponents would willingly endorse.
For instance, Machery argues that tracing back to the historical origins of the trait by means of adaptationist thinking—whether it be in a forward- or backward-looking manner—is what provides evolutionary psychology with its "originality" or raison d'être:
So far, there is no difference between evolutionary psychologists’ hypotheses and the hypotheses developed by other psychologists. What distinguishes the structure of evolutionary psychologists’ theories is a third, distinctive level of hypothesis: Evolutionary psychologists attempt to identify the origins of the psychological traits under consideration . (Machery forthcoming, p. 15; emphasis in original)
That is, without an adaptationist perspective, evolutionary psychology would not deserve the name of evolutionary psychology, for then it would be deprived of the critical tool to identify the historical origins.
Of course, heuristics in evolutionary psychology do not have to be confined to adaptive thinking. A variety of sources of information can serve as heuristics so long as they can generate some testable hypotheses. Machery mentions the usefulness of nonselectionist sources of information coming from such areas as cross-species comparisons, hunter-gatherer studies, and paleoanthropology. Nonetheless, he treats them as "constraints" that only play supplementary roles to curb the speculative character of adaptive thinking. Footnote 8 This suggests that unless adaptive thinking constitutes an integral part that binds up all these auxiliary sources, evolutionary psychology may end up with a mere hodgepodge of heterogeneous bodies of knowledge, such that its disciplinary integration will be jeopardized.
Second, as we noted time and again, one of the important functions heuristics are expected to perform is to narrow down the hypothesis space by sorting out a handful of promising hypotheses more likely to be true from the rest of the worthless ones. But then it follows that even heuristics already have to have some justificatory function—not just a discovering one. Therefore, if adaptive thinking functions as a core heuristic in evolutionary psychology, as we noted above, it cannot just settle for an innocuous role as generator of whatever hypotheses make evolutionary sense; rather, it has to take on a more active role in turning how-possibly explanations to how-actually ones as much as possible in advance of final testing. This is a reassurance of our previous point that the probability of hypotheses becoming true is a function of both the reliability of the context of discovery and the conclusiveness of that of justification and hence that we cannot draw a sharp line between the two. Footnote 9
Since Hans Reichenbach ( 1938 ) proposed it, the notion of the "context distinction" between those of discovery and justification had been predominant in the mainstream philosophy of science throughout the 20th century (Schickore 2018 ). In actual practice in science, however, the distinction cannot necessarily be drawn that neatly; this distinction has rather been utilized for sanctifying the role of philosophy of science à la logical positivism than for describing real scientific practices.
For example, getting back to Goldfinch’s formula of the adaptationist version of the hypothetico-deductive method—that "if trait T is an adaptation for X , trait T should have configuration C , and so we should find phenomenon P "—it can be schematically represented as follows:
X → T → C → P .
Leaving off the intermediary stage C and dividing the whole into two qualitatively distinct stages of the generation and confirmation of hypotheses, it can be represented as:
where the first part X → T may be called the context of discovery and the second part T → P the context of justification.
Here it might be argued that what happens in the context of justification screens off the information about what had happened in the context of discovery. Footnote 10 That is, no matter in what way T had been derived from X , once T is proposed at all, all the relevant information for designing and conducting confirmatory research of T should be sought in the semantic content of T alone, thereby rendering the information about how T is generated in the first place irrelevant. Footnote 11
This seems to be what Goldfinch actually has in mind. For, in his argumentation, the part of the predictive project X → T is supposed to be carried on almost automatically: once an adaptive problem ( X ) is given, somehow the necessary solutions to it ( T ) are almost bound to be forthcoming. The explanatory gap between X and T is too easily bridged. Compared to all the weight he places on and pages he allocates to describing how the confirmation of the hypotheses heuristically generated by evolutionary psychology should be carried out reliably in relevant adjacent fields (Goldfinch 2015 , Chap. 4), his lack of interest in this phase of how the solutions to given problems should be predicted reliably is noteworthy.
But proposing some trait as a candidate adaptation is not an easy task. As George Williams argued, "adaptation is a special and onerous concept that should be used only where it is really necessary" (Williams 1966 , p. 4); that is, it should not be invoked when less onerous and more parsimonious explanations are sufficient to do the trick. Therefore, this way of tipping the scale of the weight of establishing scientific facts exclusively to the side of final testing, thereby downplaying the weight to be carried by reliable hypothesis generation, is unbalanced.
Goldfinch’s underappreciation of the necessity of providing reliable evidential particulars in generating adaptationist hypotheses is understandable, considering that one of his primary targets is Robert Richardson’s Evolutionary Psychology as Maladapted Psychology (2007), which presented an exactly opposite case to his position, and therefore it appears that Goldfinch could not take a sympathetic stance toward what Richardson had emphasized. In that book, Richardson dismisses evolutionary psychology as a collection of unfounded speculations, because evolutionary psychologists seldom provide historical details only with which their adaptationist hypotheses can be substantiated. He draws on Brandon’s ( 1990 ) analysis on the evidential criteria that any "adaptation explanations" have to meet to qualify as reliable ones. They consist in providing historically informed evidential details concerning the following five conditions: (1) selection, (2) ecological factors, (3) heritability, (4) population structure, and (5) trait polarity. Without the information about, at least, several of these conditions, any adaptation explanations will remain as unreliable stories (Brandon 1990 , Chap. 5; Richardson 2007 , pp. 99f.). Footnote 12
The same case for the necessity of basing hypotheses on reliable historical underpinnings could be made by the following consideration. One of the rivals of evolutionary psychology in its budding stage in the 1980s through the 1990s was contemporary cognitive psychology (on top of sociobiology, as I argued in the second section). The pioneers of evolutionary psychology of the time had to demonstrate their methodological superiority to cognitive psychologists by claiming that only an evolutionary perspective could provide deeper insights into the historical origin of the now apparently synchronic constitution of the human mind. This was supposed to be possible by having access to the vantage point of the ancient selection pressures imposed on our ancestors.
In those days, the brain structure remained a "black box" with the neural circuits inside almost invisible. Although cognitive psychology had developed powerful techniques that provided clues to understanding it at levels above individual neurons, it still counted on quite indirect ways of investigation, such as stimulating the brain with images, sounds, or questions and inferring its structure from the corresponding outputs such as buttons pressed or boxes checked (Hagen 2002 ). Under such a situation, an evolutionary perspective could be a promising alternative for better approaching the structure and functions of the brain. The currently synchronic functional organization of the brain may be a close reflection of the survival and reproductive necessities in our ancestral environments. Studying the past remained much easier than studying brain wiring. It should be an evolutionary perspective "that sets the agenda for cognitive science, telling it what to look for and how to interpret what it finds" (Griffiths 2011 , p. 405). That is, conjecturing past adaptive problems and hypothesizing solutions to them could provide heuristically useful targets for later more rigorous empirical research to zero in on in a search space otherwise too vast to search exhaustively. Thus, "The major insight of evolutionary psychology is that if you want to understand the brain, look deeply at the environment of our ancestors as focused through the lens of reproduction" (Hagen 2002 , p. 520).
For example, in an effort to explain the so-called content effect on the Wason selection task, Cosmides and Tooby attempted to establish the methodological advantage of their "Social Contract Theory" over contemporary rival theories such as the "Pragmatic Reasoning Schemas" put forward by cognitive psychologists Patricia Cheng and Keith Holyoak (Cheng and Holyoak 1985 ). In doing so, they relied heavily on an evolutionary perspective for eliminating their rival theories; in one context, Cosmides argues that her social contract theory is based on the idea of domain-specific mechanisms while the rival theory is based on domain-general ones, and that evolutionary theory adjudicates in favor of the former:
The more important the adaptive problem, the more intensely selection should have specialized and improved the performance of the mechanism for solving it […]. Thus, the realization that the human mind evolved to accomplish adaptive ends indicates that natural selection would have produced special-purpose, domain-specific mental algorithms including rules of inference for solving important and recurrent adaptive problems (such as learning a language […]). (Cosmides 1989 , p. 193)
I will not delve here into whether or not this way of appealing to evolutionary theory was legitimate, an issue that has already been given exhaustive consideration in the literature. Footnote 13 What I want to stress here instead is that this way of discriminating its proprietary methodology from that of its rivals by appealing to an evolutionary (adaptationist) perspective was built in by its pioneers as one of the core identities of evolutionary psychology without which the discipline would not deserve the title. At the same time, however, this is only possible when adaptive thinking is substantiated with as sufficient historical underpinnings as possible.
Evolutionary psychology is, prima facie, going along the right track as a steady scientific discipline. A variety of psychological and behavioral traits of humans have been given evolutionary interpretations. Further, its methodology is extending beyond psychology into such surrounding areas as mental health, study of religion, criminology, consumer psychology, and so forth (cf. Buss 2016 ). For instance, the reinterpretations of mental disorders (on top of other diseases) in evolutionary medicine may be promising in that they can provide ultimate, etiological explanations for "why we get sick" (Nesse and Williams 1994 ), distinguished from, say, the typological classifications by methods of traditional psychiatry such as given in the DSM (Diagnostic and Statistical Manual of Mental Disorders). I am one of those who have a positive expectation that evolutionary psychology could eventually provide deeper understanding of our psychology and behaviors by bringing ultimate, evolutionary inquiries to bear on the study of proximate, mechanical causes.
Nevertheless, it is also true that pop hypotheses that attract media coverage have been constantly generated in some circles and disseminated without being put through rigorous tests. More importantly, even those hypotheses allegedly having been put through scientific confirmation are oftentimes more of a sort of reassurance of findings that are supposed to supervene on (or just correlate with) the hypothesized entities, rather than the confirmation of those entities themselves (like in the confirmation of jealousy modules in Buss et al. 1992 ). Otherwise, the alleged confirmations are often artifacts resulting from using theoretical models as what Wimsatt calls "pattern-matching templates": in an attempt to test a theoretical model, more often than not the researcher tends to use it as a pattern to organize phenomena by classifying results according to whether or not they fit the model, thereby choosing the parameters to be measured not independently of the model (Wimsatt 2007 ).
Then, can this state of affairs be attributed to the issue of the research ethics or morality of some researchers who lack sufficient methodological awareness? Not necessarily. My view tends to be rather that some kind of vulnerability or instability is inherent in the methodology of evolutionary psychology itself that makes it prone to those kinds of errors. That is, it appears that practitioners in evolutionary psychology today are still being largely constrained by theoretical presuppositions that the pioneers of the discipline had to incorporate, rather hastily, in need of confronting their rivals in traditional psychology of the time by demonstrating the superiority of their methodology.
Since the end of the 20th, however, situations surrounding evolutionary biology from which evolutionary psychology heavily draws as its theoretical authority have changed drastically. The initial biasing assumptions inherent in the Modern Synthesis itself have been brought to the fore, such that its received view of evolution cannot be taken at face value today.
For instance, the theory of niche construction, or cultural evolution in general, teaches us that we humans can reconstruct our social, cultural, or even ecological environments in such a way that the altered environments can in turn exert feedback effects on the selection pressures relevant to our evolution, especially of our cognitive capacities (Odling-Smee et al. 2003 ). This can happen in such a relatively short time in evolutionary terms that Mother Nature has to adopt a tinkering expedient to exapt (or co-opt ) preexisting structures for meeting the novel and urgent needs rather than creating adaptations from scratch (Gould and Vrba 1982 ). This makes the relevance of the Pleistocene EEA to the evolution of the human mind less significant than postulated by evolutionary psychologists.
In addition, research in epigenetics brought out that DNA modifications triggered by environmental changes organisms encounter pre- or postnatally play important roles in the developmental plasticity of various morphological and behavioral traits of animals, including human brain structures, and that some of these effects can be transmitted across generations without underlying changes in the DNA sequence (Jablonka and Lamb 1995 , 2005 ; Meaney 2001 ; McGowan et al. 2009 ).
Adding further to the list of new trends of research that are diametrically opposed to the nativist leaning of evolutionary psychology, the discovery of neuroplasticity in neuroscience revealed that, rather than comprising full-blown domain-specific cognitive modules, the human brain houses rudimentary module-like neuronal assemblies that become the substrate for developmental processes to mold into individually idiosyncratic neuronal pattern by the dynamic reassembling mediated through learning or experiences (Merzenich and Jenkins 1995 ; Panksepp and Panksepp 2000 ).
Therefore, there is less and less need for present-day evolutionary psychologists to continue to be constrained by the historical limitations that the pioneers of the discipline had to settle for in order to weather the initial predicaments they faced by superficially assimilating the orthodoxies of Modern Synthesis at the time, before such new trends of life and behavioral sciences as touched on above began to truly have bearing on the study of human cognition and emotions. Nevertheless, many of today's pragmatically minded evolutionary psychologists seem to be indifferent to these kind of basic issues while engaging in the so-called puzzle-solving in the phase of normal science à la Kuhn.
With respect to the above-mentioned use of theoretical models as "pattern-matching templates," trying to fit the data to the models rather than the other way around, Wimsatt ( 2007 , pp. 88–89) further states as follows:
these kinds of promotion of a theoretical or experimental model to a paradigm … can defer for a long time the noticing or analyzing of questions that were far more obvious at the start of this line of investigation. This phenomenon—the increasing entrenchment of a theoretical or experimental paradigm—in part serves to explain why disciples of an approach are often far less flexible and far less methodologically conscious than the originators of that approach.
This statement is not explicitly addressed to evolutionary psychology, but the extent to which it is applicable to it is remarkable. Unless evolutionary psychologists become more aware of these issues and embark on pursuing more reality-oriented—rather than doctrine-oriented—ways of establishing the science of the human mind, it may someday end up being remembered in history as one of the exemplary cases of degenerative research programs in the Lakatosian sense, comparable to phrenology. For a research program's being carried out in line with the typical formula of hypothesis-driven scientific reasoning is just a necessary—but not a sufficient—reason for it to qualify as a science in a productive and progressive state.
Bringing up one example of this latter problem (an example of the former problem is to be discussed shortly), the Archaeopteryx foot exhibits a design for grasping, but this observation alone is insufficient for determining whether it evolved to grasp branches (i.e., to perch), implying that Archaeopteryx was adapted for flight, or to grab prey, implying that it was a terrestrial predator (Richardson 2007 ; Hagen 2016 ).
According to Smith (2020), even this assumption of the sameness of the traits of our ancestors and those of modern humans naively postulated and shared by evolutionary psychologists (implicit in the first and fourth links in this chain) is enough to make us doubt the possibility of evolutionary psychology. For, without the explicit demonstration that the modern trait is descended from the ancestral one along the same lineage and therefore that the function that affects the fitness of the modern trait is nothing but the function that caused the ancient trait to be selected for—what she dubs "strong vertical homology"— the whole research program of evolutionary psychology would collapse. I agree that this is an aspect that has been overlooked even by critics, let alone evolutionary psychologists, that has a serious consequence. I will remain neutral on this issue for the time, however, just for the sake of my current argument.
See also note 8.
I am grateful to an anonymous reviewer for enabling me to elaborate my argument into the current form by drawing my attention to these points.
A generic conception of heuristics will be that of rules of thumb that "serve as guidelines for finding a solution to a given problem quickly and efficiently," at the expense of giving up making "exhaustive random trial and error searches," in a problem space comprising all possible configurations in a relevant domain (Schickore 2018 ; see also Gigerenzer and Selten 2001 ). One important feature resulting from this conception is that, differently from truth-preserving algorithms, heuristics make no guarantees that they will produce a solution (let alone a correct solution) to the problem. This further indicates that the use of heuristics does not always guarantee an effective reduction of hypothesis space but instead can make it even more confounding by adding to spurious hypotheses (cf. Tversky and Kahneman 1974 ), the point to be addressed in what follows.
In this sense, the issue concerning the accuracy with which to identify those ancient problems is not as fundamental to Rellihan as this issue of predictability (deducibility) of solutions via adaptive thinking. Even if Machery’s idea of a bootstrap strategy makes it plausible that backward-looking reverse engineering can assist forward-looking adaptive thinking in better identifying the initial conditions of human evolution, it will not affect his point here that "even if we can identify these initial conditions, very little can be inferred about our evolved psychology" (Rellihan 2012 , p. 273).
In contrast, reverse engineering is defined by the following formula: "From the fact that trait T is well designed for Φ -ing, infer that T is an adaptation for Φ -ing" (Rellihan 2012 , p. 248).
He also includes "middle-range evolutionary theories" as one of the constraints to a forward-looking heuristic. I will leave it out here, however, for they seem not so much constraints to adaptive thinking as more basic evolutionary theories themselves. For instance, Trivers’s theory of parental investment (Trivers 1972 ), which Machery takes as an exemplar of middle-range evolutionary theories that constrain forward-looking heuristics such as Buss’s theory of the human mating strategy, seems to actually function as a major premise to deduce Buss’s theory combined with a minor premise of specifically human cases, rather than as a constraint imposed on it from without.
Rellihan argues in this context, "One and the same inference procedure [i.e., adaptive thinking as a theory-driven inference strategy] would be considered reliable if it produced true beliefs with an eighty percent frequency and merely an effective heuristic if it produced true beliefs with, say, a twenty percent frequency. Heuristics are simply less reliable inference strategies; inference strategies are simply more reliable heuristics" (Rellihan 2012 , p. 253; clarification added). This is another way of stating that adopting "Oh, it is just a heuristic!" tactics cannot be an excuse for having yet to provide sufficient grounds for accepting a hypothesis.
For the idea of "screening-off" refer to Brandon ( 1982 ); Salmon ( 1971 ).
A typical example is the well-known case of discovery of the benzene ring by August Kekule: although it is reported that Kekule hit upon the idea of the benzene ring from a dream he had during his slumber, that episode is irrelevant to the scientific legitimacy of the idea so long as it is confirmed by a rigorous testing procedure.
Griffiths's 1996 quite lucid piece titled "The Historical Turn in the Study of Adaptation" is also written throughout with the same spirit of stressing the need to heavily incorporate historical information into the study of adaptation, such as from the comparative method or cladistics, to give substance and credibility to adaptationist storytelling including evolutionary psychological ones (Griffiths 1996 ).
Having said that, Elisabeth Lloyd’s critical analysis of the argumentation of Cosmides and Tooby in this context is noteworthy. Lloyd argues that, although Cosmides and Tooby try to establish Cosmides’s experiments designed to demonstrate the reality of the cheater detection module as crucial experiments that managed to decisively eliminate rival hypotheses (Cosmides 1989 ; Cosmides and Tooby 1994 ; Tooby and Cosmides 1989 ), "the ostensible links to evolutionary biology—rather than the experimental evidence—are doing much of the work of eliminating rival psychological hypotheses. Once the exaggerated and ill-reasoned claims are removed, the experiments appear to support a non-evolutionary psychological theory at least as strongly" (Lloyd 1999 , p. 213).
Andrews PW, Gangestad SW, Matthews D (2002) Adaptationism: how to carry out an exaptationist program. Behav Brain Sci 25(4):489–504
Article Google Scholar
Bateson P (1994) The dynamics of parent-offspring relationships in mammals. Trends Ecol Evol 9:399–403
Brandon RN (1982) The levels of selection. PSA: Proceedings of the biennial meeting of the Philosophy of Science Association. 1982:315–323
Brandon RN (1990) Adaptation and environment. Princeton University Press, Princeton
Google Scholar
Buller DJ (2005) Adapting minds: evolutionary psychology and the persistent quest for human nature. MIT Press, Cambridge
Buss DM (2000) The dangerous passion: why jealousy is as necessary as love and sex. Free Press, New York
Buss DM (2008) Evolutionary psychology: The new science of the mind, 3rd edn. Pearson/Allyn and Bacon, Boston
Buss DM, Larsen RJ, Westen D, Semmelroth J (1992) Sex differences in jealousy: evolution, physiology, and psychology. Psychol Sci 3:251–256
Buss DM (ed) (2016) The handbook of evolutionary psychology, vol 2: integrations. 2nd edn. Wiley, Hoboken
Caporael LR (1989) Mechanisms matter: the difference between socioblology and evolutionary psychology. Behav Brain Sci 12:17–18
Cheng PW, Holyoak KJ (1985) Pragmatic reasoning schemas. Cogn Psychol 17:391–416
Cosmides L (1989) The logic of social exchange: has natural selection shaped how humans reason? Studies with the Wason selection task. Cognition 31:187–276
Cosmides L, Tooby J (1987) From evolution to behavior: evolutionary psychology as the missing link. In: Dupré J (ed) The latest on the best: essays on evolution and optimality. MIT Press, Cambridge, pp 276–306
Cosmides L, Tooby J (1994) Beyond intuition and instinct blindness: toward an evolutionarily rigorous cognitive science. Cognition 50:41–77
Cosmides L, Tooby J, Barkow JH (1992) Introduction: evolutionary psychology and conceptual integration. In: Barkow J, Cosmides L, Tooby J (eds) The adapted mind: evolutionary psychology and the generation of culture. Oxford University Press, Oxford, pp 3–15
Davies PS (1999) The conflict of evolutionary psychology. Where biology meets psychology: philosophical essays. MIT Press, Cambridge, pp 67–81
Fox CW, Westneat DF (2010) Adaptation. In: Westneat DF, Fox CW (eds) Evolutionary behavioral ecology. Oxford University Press, Oxford, pp 16–25
Gigerenzer G, Selten R (2001) Bounded rationality: the adaptive toolbox. MIT Press, Cambridge
Goldfinch A (2015) Rethinking evolutionary psychology. Palgrave Macmillan, London
Book Google Scholar
Gould SJ, Vrba ES (1982) Exaptation: a missing term in the science of form. Paleobiology 8:4–15
Griffiths PE (1996) The historical turn in the study of adaptation. Br J Philos Sci 47:511–532
Griffiths PE (2011) Ethology, sciobiology, and evolutionary psychology. In: Sarkar S, Plutynski A (eds) A companion to the philosophy of biology. Wiley-Blackwell, Malden, pp 393–414
Hagen EH (2002) Special design’s centuries of success. Behav Brain Sci 25:519–520
Hagen EH (2016) Evolutionary psychology and its critics. In: Buss D (ed) The handbook of evolutionary psychology, vol 1: foundation, 2nd edn. Wiley, Hoboken, pp 136–160
Jablonka E, Lamb M (1995) Epigenetic inheritance and evolution: the Lamarckian dimension. Oxford University Press, Oxford
Jablonka E, Lamb M (2005) Evolution in four dimensions: genetic, epigenetic, behavioral, and symbolic variation in the history of life. MIT Press, Cambridge
Kauffman SA (1995) At home in the universe: the search for laws of self-organization and complexity. Oxford University Press, New York
Kauffman SA, Levin S (1987) Towards a general theory of adaptive walks on rugged landscapes. J Theor Biol 128:11–45
Laland KN, Brown GR (2011) Sense and nonsense: evolutionary perspectives on human behaviour, 2nd edn. Oxford University Press, New York
Lloyd EA (1999) Evolutionary psychology: the burdens of proof. Biol Philos 14:211–233
Machery E (forthcoming) Discovery and confirmation in evolutionary psychology. In: Prinz JJ (ed) The Oxford handbook of philosophy of psychology. Oxford University Press, Oxford
McGowan PO, Sasaki A, D’Alessio AC, Dymov S, Labonté B, Szyf M, Turecki G, Meaney MJ (2009) Epigenetic regulation of the glucocorticoid receptor in human brain associates with childhood abuse. Nat Neurosci 12:342–348
Meaney MJ (2001) Maternal care, gene expression, and the transmission of individual differences in stress reactivity across generations. Annu Rev Neurosci 24:1161–1192
Merzenich MM, Jenkins WM (1995) Cortical plasticity, learning and learning dysfunction. In: Julesz B, Kovacs I (eds) Maturational windows and adult cortical plasticity. Addison-Wesley, New York, pp 247–272
Nesse RM, Williams GC (1994) Why we get sick: the new science of Darwinian medicine. Vintage, New York
Odling-Smee FJ, Laland KN, Feldman MW (2003) Niche construction: the neglected process in evolution. Princeton University Press, Princeton
Orzack SH, Sober E (1994) Optimality models and the test of adaptationism. Am Nat 143:361–380
Panksepp J, Panksepp JB (2000) The seven sins of evolutionary psychology. Evol Cogn 6:108–131
Reichenbach H (1938) Experience and prediction: an analysis of the foundations and the structure of knowledge. University of Chicago Press, Chicago
Rellihan M (2012) Adaptationism and adaptive thinking in evolutionary psychology. Philos Psychol 25:245–277
Richardson RC (2007) Evolutionary psychology as maladapted psychology. MIT Press, Cambridge
Richerson P, Boyd R (2005) Not by genes alone: how culture transformed human evolution. University of Chicago Press, Chicago
Rose MR, Lauder GV (1996) Adaptation. Academic Press, San Diego
Salmon WC (1971) Statistical explanation. In: Salmon WC, Jeffrey RC, Greeno JG (eds) Statistical explanation and statistical relevance. University of Pittsburgh Press, Pittsburgh, pp 29–88
Chapter Google Scholar
Schickore J (2018) Scientific discovery. The Stanford Encyclopedia of Philosophy (Summer 2018 Edition) https://plato.stanford.edu/archives/sum2018/entries/scientific-discovery . Accessed 2 Sept 2019
Smith S (2019) Is evolutionary psychology possible? Biol Theory 15:39–49
Sterelny K (1995) The adapted mind. Biol Philos 10:365–380
Sterelny K, Griffiths PE (1999) Sex and death: an introduction to philosophy of biology. University of Chicago Press, Chicago
Symons D (1992) On the use and misuse of Darwinism in the study of human behavior. In: Barkow JH, Cosmides L, Tooby J (eds) The adapted mind: evolutionary psychology and the generation of culture. Oxford University Press, Oxford, pp 137–159
Tooby J, Cosmides L (1989) Evolutionary psychology and the generation of culture, part I: theoretical considerations. Ethol Sociobiol 10:29–49
Tooby J, Cosmides L (1990) The past explains the present: emotional adaptations and the structure of ancestral environments. Ethol Sociobiol 11:375–424
Tooby J, Cosmides L (1992) The psychological foundations of culture. In: Barkow JH, Cosmides L, Tooby J (eds) The adapted mind: evolutionary psychology and the generation of culture. Oxford University Press, Oxford, pp 19–136
Tooby J, Cosmides L (1998) Evolutionizing the cognitive sciences: a reply to Shapiro and Epstein. Mind Lang 13:195–204
Trivers R (1972) Parental investment and sexual selection. In: Campbell B (ed) Sexual selection and the descent of man. Aldine DeGruyter, Chicago, pp 136–179
Trivers R (1974) Parent-offspring conflict Am Zool 14:249–264
Tversky A, Kahneman D (1974) Judgment under uncertainty: heuristics and biases. Science 185:1124–1131
Williams GC (1966) Adaptation and natural selection: a critique of some current evolutionary thought. Princeton University Press, Princeton
Wimsatt WC (2007) Re-engineering philosophy for limited beings: piecewise approximations to reality. Harvard University Press, Cambridge
Download references
Acknowledgments
This work was supported by a Grant-in-Aid for Scientific Research (C) from the Ministry of Education, Culture, Sports, Science and Technology of Japan (Grant Number: JP19K00277). I would like to thank the Numerical Algorithms Group and Editage for language and editing services.
Author information
Authors and affiliations.
Center for Liberal Arts, Tokai University, Kanagawa, Japan
Shunkichi Matsumoto
You can also search for this author in PubMed Google Scholar
Corresponding author
Correspondence to Shunkichi Matsumoto .
Additional information
Publisher's note.
Springer Nature remains neutral with regard to jurisdictional claims in published maps and institutional affiliations.
Rights and permissions
Open Access This article is licensed under a Creative Commons Attribution 4.0 International License, which permits use, sharing, adaptation, distribution and reproduction in any medium or format, as long as you give appropriate credit to the original author(s) and the source, provide a link to the Creative Commons licence, and indicate if changes were made. The images or other third party material in this article are included in the article's Creative Commons licence, unless indicated otherwise in a credit line to the material. If material is not included in the article's Creative Commons licence and your intended use is not permitted by statutory regulation or exceeds the permitted use, you will need to obtain permission directly from the copyright holder. To view a copy of this licence, visit http://creativecommons.org/licenses/by/4.0/ .
Reprints and permissions
About this article
Matsumoto, S. Making Sense of the Relationship Between Adaptive Thinking and Heuristics in Evolutionary Psychology. Biol Theory 16 , 16–29 (2021). https://doi.org/10.1007/s13752-020-00369-0
Download citation
Received : 27 February 2020
Accepted : 24 October 2020
Published : 09 February 2021
Issue Date : March 2021
DOI : https://doi.org/10.1007/s13752-020-00369-0
Share this article
Anyone you share the following link with will be able to read this content:
Sorry, a shareable link is not currently available for this article.
Provided by the Springer Nature SharedIt content-sharing initiative
- Adaptive thinking
- Bootstrap strategy
- Contexts of discovery and justification
- Division of labor
- Evolutionary psychology
- Find a journal
- Publish with us
- Track your research
Research Hypothesis In Psychology: Types, & Examples
Saul Mcleod, PhD
Editor-in-Chief for Simply Psychology
BSc (Hons) Psychology, MRes, PhD, University of Manchester
Saul Mcleod, PhD., is a qualified psychology teacher with over 18 years of experience in further and higher education. He has been published in peer-reviewed journals, including the Journal of Clinical Psychology.
Learn about our Editorial Process
Olivia Guy-Evans, MSc
Associate Editor for Simply Psychology
BSc (Hons) Psychology, MSc Psychology of Education
Olivia Guy-Evans is a writer and associate editor for Simply Psychology. She has previously worked in healthcare and educational sectors.
On This Page:
A research hypothesis, in its plural form “hypotheses,” is a specific, testable prediction about the anticipated results of a study, established at its outset. It is a key component of the scientific method .
Hypotheses connect theory to data and guide the research process towards expanding scientific understanding
Some key points about hypotheses:
- A hypothesis expresses an expected pattern or relationship. It connects the variables under investigation.
- It is stated in clear, precise terms before any data collection or analysis occurs. This makes the hypothesis testable.
- A hypothesis must be falsifiable. It should be possible, even if unlikely in practice, to collect data that disconfirms rather than supports the hypothesis.
- Hypotheses guide research. Scientists design studies to explicitly evaluate hypotheses about how nature works.
- For a hypothesis to be valid, it must be testable against empirical evidence. The evidence can then confirm or disprove the testable predictions.
- Hypotheses are informed by background knowledge and observation, but go beyond what is already known to propose an explanation of how or why something occurs.
Predictions typically arise from a thorough knowledge of the research literature, curiosity about real-world problems or implications, and integrating this to advance theory. They build on existing literature while providing new insight.
Types of Research Hypotheses
Alternative hypothesis.
The research hypothesis is often called the alternative or experimental hypothesis in experimental research.
It typically suggests a potential relationship between two key variables: the independent variable, which the researcher manipulates, and the dependent variable, which is measured based on those changes.
The alternative hypothesis states a relationship exists between the two variables being studied (one variable affects the other).
A hypothesis is a testable statement or prediction about the relationship between two or more variables. It is a key component of the scientific method. Some key points about hypotheses:
- Important hypotheses lead to predictions that can be tested empirically. The evidence can then confirm or disprove the testable predictions.
In summary, a hypothesis is a precise, testable statement of what researchers expect to happen in a study and why. Hypotheses connect theory to data and guide the research process towards expanding scientific understanding.
An experimental hypothesis predicts what change(s) will occur in the dependent variable when the independent variable is manipulated.
It states that the results are not due to chance and are significant in supporting the theory being investigated.
The alternative hypothesis can be directional, indicating a specific direction of the effect, or non-directional, suggesting a difference without specifying its nature. It’s what researchers aim to support or demonstrate through their study.
Null Hypothesis
The null hypothesis states no relationship exists between the two variables being studied (one variable does not affect the other). There will be no changes in the dependent variable due to manipulating the independent variable.
It states results are due to chance and are not significant in supporting the idea being investigated.
The null hypothesis, positing no effect or relationship, is a foundational contrast to the research hypothesis in scientific inquiry. It establishes a baseline for statistical testing, promoting objectivity by initiating research from a neutral stance.
Many statistical methods are tailored to test the null hypothesis, determining the likelihood of observed results if no true effect exists.
This dual-hypothesis approach provides clarity, ensuring that research intentions are explicit, and fosters consistency across scientific studies, enhancing the standardization and interpretability of research outcomes.
Nondirectional Hypothesis
A non-directional hypothesis, also known as a two-tailed hypothesis, predicts that there is a difference or relationship between two variables but does not specify the direction of this relationship.
It merely indicates that a change or effect will occur without predicting which group will have higher or lower values.
For example, “There is a difference in performance between Group A and Group B” is a non-directional hypothesis.
Directional Hypothesis
A directional (one-tailed) hypothesis predicts the nature of the effect of the independent variable on the dependent variable. It predicts in which direction the change will take place. (i.e., greater, smaller, less, more)
It specifies whether one variable is greater, lesser, or different from another, rather than just indicating that there’s a difference without specifying its nature.
For example, “Exercise increases weight loss” is a directional hypothesis.
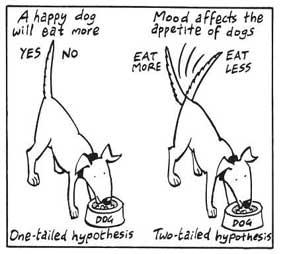
Falsifiability
The Falsification Principle, proposed by Karl Popper , is a way of demarcating science from non-science. It suggests that for a theory or hypothesis to be considered scientific, it must be testable and irrefutable.
Falsifiability emphasizes that scientific claims shouldn’t just be confirmable but should also have the potential to be proven wrong.
It means that there should exist some potential evidence or experiment that could prove the proposition false.
However many confirming instances exist for a theory, it only takes one counter observation to falsify it. For example, the hypothesis that “all swans are white,” can be falsified by observing a black swan.
For Popper, science should attempt to disprove a theory rather than attempt to continually provide evidence to support a research hypothesis.
Can a Hypothesis be Proven?
Hypotheses make probabilistic predictions. They state the expected outcome if a particular relationship exists. However, a study result supporting a hypothesis does not definitively prove it is true.
All studies have limitations. There may be unknown confounding factors or issues that limit the certainty of conclusions. Additional studies may yield different results.
In science, hypotheses can realistically only be supported with some degree of confidence, not proven. The process of science is to incrementally accumulate evidence for and against hypothesized relationships in an ongoing pursuit of better models and explanations that best fit the empirical data. But hypotheses remain open to revision and rejection if that is where the evidence leads.
- Disproving a hypothesis is definitive. Solid disconfirmatory evidence will falsify a hypothesis and require altering or discarding it based on the evidence.
- However, confirming evidence is always open to revision. Other explanations may account for the same results, and additional or contradictory evidence may emerge over time.
We can never 100% prove the alternative hypothesis. Instead, we see if we can disprove, or reject the null hypothesis.
If we reject the null hypothesis, this doesn’t mean that our alternative hypothesis is correct but does support the alternative/experimental hypothesis.
Upon analysis of the results, an alternative hypothesis can be rejected or supported, but it can never be proven to be correct. We must avoid any reference to results proving a theory as this implies 100% certainty, and there is always a chance that evidence may exist which could refute a theory.
How to Write a Hypothesis
- Identify variables . The researcher manipulates the independent variable and the dependent variable is the measured outcome.
- Operationalized the variables being investigated . Operationalization of a hypothesis refers to the process of making the variables physically measurable or testable, e.g. if you are about to study aggression, you might count the number of punches given by participants.
- Decide on a direction for your prediction . If there is evidence in the literature to support a specific effect of the independent variable on the dependent variable, write a directional (one-tailed) hypothesis. If there are limited or ambiguous findings in the literature regarding the effect of the independent variable on the dependent variable, write a non-directional (two-tailed) hypothesis.
- Make it Testable : Ensure your hypothesis can be tested through experimentation or observation. It should be possible to prove it false (principle of falsifiability).
- Clear & concise language . A strong hypothesis is concise (typically one to two sentences long), and formulated using clear and straightforward language, ensuring it’s easily understood and testable.
Consider a hypothesis many teachers might subscribe to: students work better on Monday morning than on Friday afternoon (IV=Day, DV= Standard of work).
Now, if we decide to study this by giving the same group of students a lesson on a Monday morning and a Friday afternoon and then measuring their immediate recall of the material covered in each session, we would end up with the following:
- The alternative hypothesis states that students will recall significantly more information on a Monday morning than on a Friday afternoon.
- The null hypothesis states that there will be no significant difference in the amount recalled on a Monday morning compared to a Friday afternoon. Any difference will be due to chance or confounding factors.
More Examples
- Memory : Participants exposed to classical music during study sessions will recall more items from a list than those who studied in silence.
- Social Psychology : Individuals who frequently engage in social media use will report higher levels of perceived social isolation compared to those who use it infrequently.
- Developmental Psychology : Children who engage in regular imaginative play have better problem-solving skills than those who don’t.
- Clinical Psychology : Cognitive-behavioral therapy will be more effective in reducing symptoms of anxiety over a 6-month period compared to traditional talk therapy.
- Cognitive Psychology : Individuals who multitask between various electronic devices will have shorter attention spans on focused tasks than those who single-task.
- Health Psychology : Patients who practice mindfulness meditation will experience lower levels of chronic pain compared to those who don’t meditate.
- Organizational Psychology : Employees in open-plan offices will report higher levels of stress than those in private offices.
- Behavioral Psychology : Rats rewarded with food after pressing a lever will press it more frequently than rats who receive no reward.

- Bipolar Disorder
- Therapy Center
- When To See a Therapist
- Types of Therapy
- Best Online Therapy
- Best Couples Therapy
- Best Family Therapy
- Managing Stress
- Sleep and Dreaming
- Understanding Emotions
- Self-Improvement
- Healthy Relationships
- Student Resources
- Personality Types
- Guided Meditations
- Verywell Mind Insights
- 2023 Verywell Mind 25
- Mental Health in the Classroom
- Editorial Process
- Meet Our Review Board
- Crisis Support
How to Write a Great Hypothesis
Hypothesis Definition, Format, Examples, and Tips
Kendra Cherry, MS, is a psychosocial rehabilitation specialist, psychology educator, and author of the "Everything Psychology Book."
:max_bytes(150000):strip_icc():format(webp)/IMG_9791-89504ab694d54b66bbd72cb84ffb860e.jpg)
Amy Morin, LCSW, is a psychotherapist and international bestselling author. Her books, including "13 Things Mentally Strong People Don't Do," have been translated into more than 40 languages. Her TEDx talk, "The Secret of Becoming Mentally Strong," is one of the most viewed talks of all time.
:max_bytes(150000):strip_icc():format(webp)/VW-MIND-Amy-2b338105f1ee493f94d7e333e410fa76.jpg)
Verywell / Alex Dos Diaz
- The Scientific Method
Hypothesis Format
Falsifiability of a hypothesis.
- Operationalization
Hypothesis Types
Hypotheses examples.
- Collecting Data
A hypothesis is a tentative statement about the relationship between two or more variables. It is a specific, testable prediction about what you expect to happen in a study. It is a preliminary answer to your question that helps guide the research process.
Consider a study designed to examine the relationship between sleep deprivation and test performance. The hypothesis might be: "This study is designed to assess the hypothesis that sleep-deprived people will perform worse on a test than individuals who are not sleep-deprived."
At a Glance
A hypothesis is crucial to scientific research because it offers a clear direction for what the researchers are looking to find. This allows them to design experiments to test their predictions and add to our scientific knowledge about the world. This article explores how a hypothesis is used in psychology research, how to write a good hypothesis, and the different types of hypotheses you might use.
The Hypothesis in the Scientific Method
In the scientific method , whether it involves research in psychology, biology, or some other area, a hypothesis represents what the researchers think will happen in an experiment. The scientific method involves the following steps:
- Forming a question
- Performing background research
- Creating a hypothesis
- Designing an experiment
- Collecting data
- Analyzing the results
- Drawing conclusions
- Communicating the results
The hypothesis is a prediction, but it involves more than a guess. Most of the time, the hypothesis begins with a question which is then explored through background research. At this point, researchers then begin to develop a testable hypothesis.
Unless you are creating an exploratory study, your hypothesis should always explain what you expect to happen.
In a study exploring the effects of a particular drug, the hypothesis might be that researchers expect the drug to have some type of effect on the symptoms of a specific illness. In psychology, the hypothesis might focus on how a certain aspect of the environment might influence a particular behavior.
Remember, a hypothesis does not have to be correct. While the hypothesis predicts what the researchers expect to see, the goal of the research is to determine whether this guess is right or wrong. When conducting an experiment, researchers might explore numerous factors to determine which ones might contribute to the ultimate outcome.
In many cases, researchers may find that the results of an experiment do not support the original hypothesis. When writing up these results, the researchers might suggest other options that should be explored in future studies.
In many cases, researchers might draw a hypothesis from a specific theory or build on previous research. For example, prior research has shown that stress can impact the immune system. So a researcher might hypothesize: "People with high-stress levels will be more likely to contract a common cold after being exposed to the virus than people who have low-stress levels."
In other instances, researchers might look at commonly held beliefs or folk wisdom. "Birds of a feather flock together" is one example of folk adage that a psychologist might try to investigate. The researcher might pose a specific hypothesis that "People tend to select romantic partners who are similar to them in interests and educational level."
Elements of a Good Hypothesis
So how do you write a good hypothesis? When trying to come up with a hypothesis for your research or experiments, ask yourself the following questions:
- Is your hypothesis based on your research on a topic?
- Can your hypothesis be tested?
- Does your hypothesis include independent and dependent variables?
Before you come up with a specific hypothesis, spend some time doing background research. Once you have completed a literature review, start thinking about potential questions you still have. Pay attention to the discussion section in the journal articles you read . Many authors will suggest questions that still need to be explored.
How to Formulate a Good Hypothesis
To form a hypothesis, you should take these steps:
- Collect as many observations about a topic or problem as you can.
- Evaluate these observations and look for possible causes of the problem.
- Create a list of possible explanations that you might want to explore.
- After you have developed some possible hypotheses, think of ways that you could confirm or disprove each hypothesis through experimentation. This is known as falsifiability.
In the scientific method , falsifiability is an important part of any valid hypothesis. In order to test a claim scientifically, it must be possible that the claim could be proven false.
Students sometimes confuse the idea of falsifiability with the idea that it means that something is false, which is not the case. What falsifiability means is that if something was false, then it is possible to demonstrate that it is false.
One of the hallmarks of pseudoscience is that it makes claims that cannot be refuted or proven false.
The Importance of Operational Definitions
A variable is a factor or element that can be changed and manipulated in ways that are observable and measurable. However, the researcher must also define how the variable will be manipulated and measured in the study.
Operational definitions are specific definitions for all relevant factors in a study. This process helps make vague or ambiguous concepts detailed and measurable.
For example, a researcher might operationally define the variable " test anxiety " as the results of a self-report measure of anxiety experienced during an exam. A "study habits" variable might be defined by the amount of studying that actually occurs as measured by time.
These precise descriptions are important because many things can be measured in various ways. Clearly defining these variables and how they are measured helps ensure that other researchers can replicate your results.
Replicability
One of the basic principles of any type of scientific research is that the results must be replicable.
Replication means repeating an experiment in the same way to produce the same results. By clearly detailing the specifics of how the variables were measured and manipulated, other researchers can better understand the results and repeat the study if needed.
Some variables are more difficult than others to define. For example, how would you operationally define a variable such as aggression ? For obvious ethical reasons, researchers cannot create a situation in which a person behaves aggressively toward others.
To measure this variable, the researcher must devise a measurement that assesses aggressive behavior without harming others. The researcher might utilize a simulated task to measure aggressiveness in this situation.
Hypothesis Checklist
- Does your hypothesis focus on something that you can actually test?
- Does your hypothesis include both an independent and dependent variable?
- Can you manipulate the variables?
- Can your hypothesis be tested without violating ethical standards?
The hypothesis you use will depend on what you are investigating and hoping to find. Some of the main types of hypotheses that you might use include:
- Simple hypothesis : This type of hypothesis suggests there is a relationship between one independent variable and one dependent variable.
- Complex hypothesis : This type suggests a relationship between three or more variables, such as two independent and dependent variables.
- Null hypothesis : This hypothesis suggests no relationship exists between two or more variables.
- Alternative hypothesis : This hypothesis states the opposite of the null hypothesis.
- Statistical hypothesis : This hypothesis uses statistical analysis to evaluate a representative population sample and then generalizes the findings to the larger group.
- Logical hypothesis : This hypothesis assumes a relationship between variables without collecting data or evidence.
A hypothesis often follows a basic format of "If {this happens} then {this will happen}." One way to structure your hypothesis is to describe what will happen to the dependent variable if you change the independent variable .
The basic format might be: "If {these changes are made to a certain independent variable}, then we will observe {a change in a specific dependent variable}."
A few examples of simple hypotheses:
- "Students who eat breakfast will perform better on a math exam than students who do not eat breakfast."
- "Students who experience test anxiety before an English exam will get lower scores than students who do not experience test anxiety."
- "Motorists who talk on the phone while driving will be more likely to make errors on a driving course than those who do not talk on the phone."
- "Children who receive a new reading intervention will have higher reading scores than students who do not receive the intervention."
Examples of a complex hypothesis include:
- "People with high-sugar diets and sedentary activity levels are more likely to develop depression."
- "Younger people who are regularly exposed to green, outdoor areas have better subjective well-being than older adults who have limited exposure to green spaces."
Examples of a null hypothesis include:
- "There is no difference in anxiety levels between people who take St. John's wort supplements and those who do not."
- "There is no difference in scores on a memory recall task between children and adults."
- "There is no difference in aggression levels between children who play first-person shooter games and those who do not."
Examples of an alternative hypothesis:
- "People who take St. John's wort supplements will have less anxiety than those who do not."
- "Adults will perform better on a memory task than children."
- "Children who play first-person shooter games will show higher levels of aggression than children who do not."
Collecting Data on Your Hypothesis
Once a researcher has formed a testable hypothesis, the next step is to select a research design and start collecting data. The research method depends largely on exactly what they are studying. There are two basic types of research methods: descriptive research and experimental research.
Descriptive Research Methods
Descriptive research such as case studies , naturalistic observations , and surveys are often used when conducting an experiment is difficult or impossible. These methods are best used to describe different aspects of a behavior or psychological phenomenon.
Once a researcher has collected data using descriptive methods, a correlational study can examine how the variables are related. This research method might be used to investigate a hypothesis that is difficult to test experimentally.
Experimental Research Methods
Experimental methods are used to demonstrate causal relationships between variables. In an experiment, the researcher systematically manipulates a variable of interest (known as the independent variable) and measures the effect on another variable (known as the dependent variable).
Unlike correlational studies, which can only be used to determine if there is a relationship between two variables, experimental methods can be used to determine the actual nature of the relationship—whether changes in one variable actually cause another to change.
The hypothesis is a critical part of any scientific exploration. It represents what researchers expect to find in a study or experiment. In situations where the hypothesis is unsupported by the research, the research still has value. Such research helps us better understand how different aspects of the natural world relate to one another. It also helps us develop new hypotheses that can then be tested in the future.
Thompson WH, Skau S. On the scope of scientific hypotheses . R Soc Open Sci . 2023;10(8):230607. doi:10.1098/rsos.230607
Taran S, Adhikari NKJ, Fan E. Falsifiability in medicine: what clinicians can learn from Karl Popper [published correction appears in Intensive Care Med. 2021 Jun 17;:]. Intensive Care Med . 2021;47(9):1054-1056. doi:10.1007/s00134-021-06432-z
Eyler AA. Research Methods for Public Health . 1st ed. Springer Publishing Company; 2020. doi:10.1891/9780826182067.0004
Nosek BA, Errington TM. What is replication ? PLoS Biol . 2020;18(3):e3000691. doi:10.1371/journal.pbio.3000691
Aggarwal R, Ranganathan P. Study designs: Part 2 - Descriptive studies . Perspect Clin Res . 2019;10(1):34-36. doi:10.4103/picr.PICR_154_18
Nevid J. Psychology: Concepts and Applications. Wadworth, 2013.
By Kendra Cherry, MSEd Kendra Cherry, MS, is a psychosocial rehabilitation specialist, psychology educator, and author of the "Everything Psychology Book."
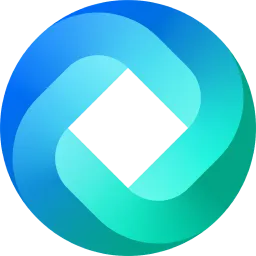
Hypothesis Maker
Ai-powered research hypothesis generator.
- Scientific Research: Generate a hypothesis for your experimental or observational study based on your research question.
- Academic Studies: Formulate a hypothesis for your thesis, dissertation, or academic paper.
- Market Research: Develop a hypothesis for your market research study to understand consumer behavior or market trends.
- Social Science Research: Create a hypothesis for your social science research to explore societal or behavioral patterns.
New & Trending Tools
Ai text generator, webpage text extractor ai.
- International edition
- Australia edition
- Europe edition
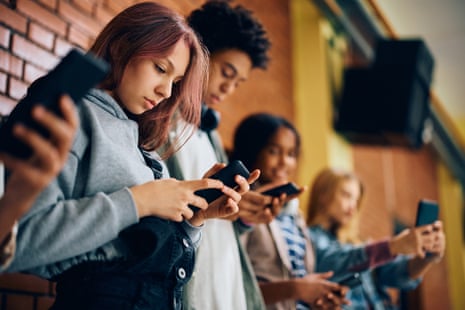
The Anxious Generation wants to save teens. But the bestseller’s anti-tech logic is skewed
There’s no doubt about the mental health crisis facing young people. Jonathan Haidt blames our devices – which oversimplifies the problem
I n the introduction to his new book The Anxious Generation , titled “Growing up on Mars”, Jonathan Haidt tells a fanciful piece of science fiction about a child conscripted into a dangerous mission to the red planet that will deform the young person as they grow. The journey is undertaken without the parents’ consent. The ham-fisted metaphor is that technology companies have done the same to children and teenagers by putting smartphones into their hands.
Haidt, a New York University professor of ethical leadership who researches social psychology and morality, goes on to argue that smartphones ignited a wildfire of anxiety and depression in gen Z around the world, by granting them “continuous access to social media, online video games, and other internet-based activities”. He says there are four foundational harms in this degradation of youth: social deprivation, sleep deprivation, attention fragmentation, and addiction.
“This great rewiring of childhood, I argue, is the single largest reason for the tidal wave of adolescent mental illness that began in the early 2010s,” he writes.
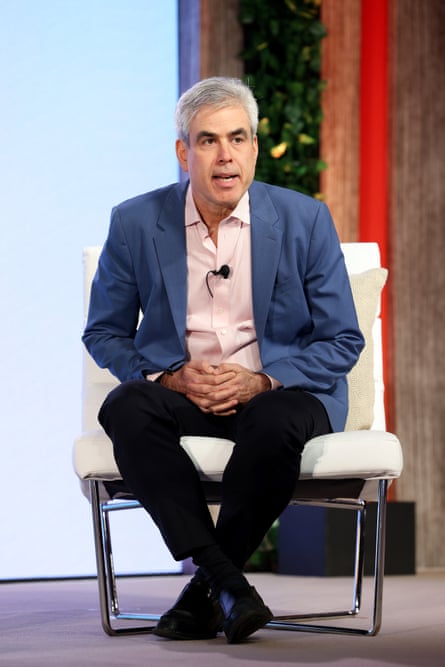
The Anxious Generation has squatted atop the New York Times bestseller list for four weeks now and garnered florid, positive reviews – it hit a nerve . But it has also sparked fierce debate over the effects of our now ubiquitous devices, the causes of mental illness, and just what to do about the kids. Haidt’s critics argue that he took advantage of very real phenomena – depressed and anxious children, overattachment to technology, disconnection from other humans – to make a broad indictment of smartphones, when it’s not as simple as that.
We can split The Anxious Generation into two parts: the first details the supposed digital destruction of childhood around the world, while the second recommends ways to fix it.
There is, in fact, a crashing wave of teenage anguish. Studies in Haidt’s book and elsewhere show an alarming surge in teenage depression, anxiety and suicide attempts from 2010 to 2023. This is happening at the same time as widespread social media and smartphone adoption. The psychologist Jean Twenge, an associate of Haidt, asked in 2017 on the cover of the Atlantic: “ Have smartphones destroyed a generation? ” In the fall of 2021, a “national emergency in child and adolescent mental health” was declared by the American Academy of Pediatrics, the American Academy of Child and Adolescent Psychiatry and the Children’s Hospital Association.
But as the University of California, Irvine, psychology professor Candice Odgers asked in her critique of The Anxious Generation in Nature , “Is social media really behind an epidemic of teenage mental illness?”
The answer, per Odgers, is no. Blisteringly, she accuses Haidt of “making up stories by simply looking at trend lines” and says his book’s core argument “is not supported by science”. Haidt makes the basic error of mistaking correlation with causation, she says.
In a review of 40 previous studies published in 2020, Odgers found no cause-effect relationship between smartphone ownership, social media usage and adolescents’ mental health. A 2023 analysis of wellbeing and Facebook adoption in 72 countries cited by Odgers delivered no evidence connecting the spread of social media with mental illness. (Those researchers even found that Facebook adoption predicted some positive trends in wellbeing among young people.) Another survey of more than 500 teens and over 1,000 undergraduates conducted over two and six years, respectively, found that increased social media use did not precede the onset of depression.
For Haidt to draw such a sweeping conclusion as “teens troubled, ergo smartphones bad” from such unsettled science is wrong, Odgers argues. He engages in post hoc, ergo propter hoc reasoning: after this, therefore because of this. The irony is palpable –Haidt himself has argued in his own academic research that “moral reasoning is usually a post hoc construction” that follows a judgment already made. His fellow scientists now say his book falls into the same trap in pronouncing that immoral technology has corrupted the youth of today. The Oxford psychology professor Andrew Przybylski told the tech newsletter Platformer : “Extraordinary claims require extraordinary evidence. Right now, I’d argue he doesn’t have that.” The Stetson University psychology professor Christopher Ferguson said Haidt’s book was fomenting moral panic about social media reminiscent of the debate over video games and real-world violence.
“Overall, as has been the case for previous media such as video games, concerns about screen time and mental health are not based in reliable data,” Ferguson noted in a 2021 meta-analysis of more than 30 studies that found no link between smartphone or social media use and poor mental health or suicidal ideation.
Responding to social scientists’ critiques of his book on the New Yorker Radio Hour, Haidt said, “I keep asking for alternative theories. You don’t think it’s the smartphones and social media – what is it?”
Haidt was making an appeal to ignorance, a logical fallacy: an alternative is absent, ergo my hypothesis is correct. Simply because there are no other explanations for the deterioration of the mental health of teenagers on the bestseller list right now does not mean his book is right – a drought of certainty does not mean the first idea we find is water. And scientists and doctors have, in fact, put forward ideas that compete with his , or else acknowledged smartphones as part, but not all, of the problem.
What’s more, The Anxious Generation barely acknowledges the effect of school closures during the pandemic had on kids and teens’ mental health and development, the Washington Post technology reporter Taylor Lorenz pointed out on her podcast . The Anxious Generation includes graphs showing that adolescent mental health grew even worse beginning in 2020, but Haidt insists that the pandemic was only an accelerant to an already raging fire caused by smartphones.
“The mess is not because of Covid. It was baked in before Covid. Covid didn’t actually have a long-lasting impact,” he said in a podcast interview with a fellow NYU professor, Scott Galloway.
A rebuttal in the language of a TikToker: be so for real. Studies definitively say that school closures due to the coronavirus pandemic caused and continue to inflame mental distress among children and teenagers. These disruptions hindered students’ social and emotional development, academic progress and physical health, multiple researchers have found, without equivocation . Worse still, studies have found that these measures did little to limit the spread of coronavirus as much as they hurt young students, an ineffective tradeoff.
Haidt needed to substantively contend with the problems caused by lockdowns and school closures, which are correlated with the worst period of teen suffering in the last 15 years, to give real, current solutions to the mental health crisis among youth. He offers little in that regard.
I f the first part of Haidt’s book – teens suffering, phones to blame – reads as sensational generalization, the second half is full of recommendations you have probably heard before, because Haidt cites nationwide professional associations of doctors and authorities.
The Anxious Generation proposes four solutions to the epidemic: “No smartphones before high school. No social media before 16. Phone-free schools. Far more unsupervised play and childhood independence.” With the exception of age-gating policies, these are not unreasonable things. Schools have seen remarkable results when they ban smartphones . Many educators are in favor of such prohibitions. Teenagers do struggle with appropriate use of social media , and many say it makes them feel worse about themselves. Allowing children playtime free of surveillance does not seem beyond the pale. Parents limiting children’s phone use before bed and in the early morning, as Haidt advises, is decent counsel.
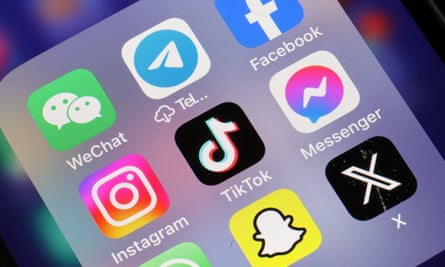
The American Academy of Child and Adolescent Psychiatry goes a step further, advising that parents themselves should attempt to model the habits of screen time they wish to see in their children.
That same organization that declared a mental health emergency among young people offers a measured approach to technology and teens in general: “Screens are here to stay and can offer many positives,” its website reads. But Haidt can see none of these positives in smartphones or social media, an unrealistic attitude. He rightfully points out that social media can be a nightmare of compare and despair, of the fear of missing out. The other side of the same coin is that it forms aspirational and inspirational communities, and outlets for creativity. Smartphones are likewise tools of productivity for young people: in 2012, squarely in the years that Haidt says the ruination of childhood began, Reuters reported that more than a third of surveyed American teenagers were doing homework on their phones. “Influencer” has become a derisive term, but the job of creating content for social media has minted a generation of young business owners . And how do you think the teenage students of Marjory Stoneman Douglas high school organized a global movement against gun violence?
Children have always inhabited worlds that seem foreign and foreboding to their parents – the internet is one such place. It is unsettling and unfamiliar to those who did not grow up with it. What The Anxious Generation does successfully is smooth on a salve over the hurt of being disregarded by a loved one in favor of a phone. It provides an answer to the painful parental question of “Why is my child ignoring me? Why are they spending so much time online and alone in their room?”
But the question of teen mental health is complicated and resistant to any single explanation. And overlooking all that smartphones can be for teens and adults – maps, digital cameras, novels, encyclopedias, Walkmen and whatever else Haidt dismisses as “other internet-based activities”– is a reductive understanding of our devices as mere gaming and gabbing machines. In 2024, these devices contain our lives .
I was reminded of Haidt’s book on the subway the other night. A woman asked her daughter in the seat next to her a question. Her daughter did not answer; she was staring at her phone playing a game. The woman’s smile faded. They did not speak for another minute. Then the daughter handed her mother the phone and looked her in the eyes: it was the mother’s turn in the game. The woman looked at the phone and laughed at something the young girl had done, some funny misstep or a clever move. They both smiled. Though only an anecdote, it did remind me of the possibility of connection, both online and off.
- Social media
- Smartphones
- Mobile phones
- Mental health
- Parents and parenting
Most viewed
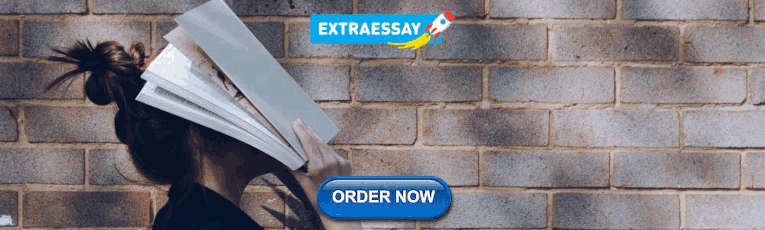
IMAGES
VIDEO
COMMENTS
Hypothesis generation is an early and critical step in any hypothesis-driven clinical research project. Because it is not yet a well-understood cognitive process, the need to improve the process goes unrecognized. ... The Quarterly journal of experimental psychology A, Human experimental psychology. 1998;51(4):819-852. doi:10.1080/713755788 40 ...
Abstract To correct a common imbalance in methodology courses, focusing almost entirely on hypothesis-testing issues to the neglect of hypothesis-generating issues which are at least as important, 49 creative heuristics are described, divided into 5 categories and 14 subcategories. Each of these heuristics has often been used to generate hypotheses in psychological research, and each is ...
creative hypothesis generating, but despair of teaching or even describing it. I have contended (McGuire 1973, 1983) that creative hypothesis-generating aspects of research on both strategic and tactical levels can be taught. While in the past (McGuire 1989) I have discussed creative hypothesis generation on
Principle 1 suggests that hypothesis-generation processes are a general case of cued recall in that the data or symptoms observed cue the retrieval of diagnostic hypotheses from either episodic long-term memory or knowledge. Note, however, that the retrieval goals in a hypothesis-generation task differ from the retrieval goals in the typical ...
1 Department of Psychological Sciences, Birkbeck College, University of London, London, UK; 2 Department of Psychology, University of Oklahoma, Norman, OK, USA; The pre-decisional process of hypothesis generation is a ubiquitous cognitive faculty that we continually employ in an effort to understand our environment and thereby support appropriate judgments and decisions.
Leveraging the synergy between causal knowledge graphs and a large language model (LLM), our study introduces a groundbreaking approach for computational hypothesis generation in psychology. We analyzed 43,312 psychology articles using a LLM to extract causal relation pairs. This analysis produced a specialized causal graph for psychology. Applying link prediction algorithms, we generated 130 ...
Toward an integrative theory of hypothesis generation, probability judgment, and hypothesis testing. In B. H. Ross (Ed.), The psychology of learning and motivation: Advances in research and theory (pp. 299-342).
I have contended (McGuire 1973, 1983) that creative hypothesis-generating aspects of research on both strategic and tactical levels can be taught. While in the past (McGuire 1989) I have discussed creative hypothesis generation on the strategic level, here I address it at the tactical level by describing a variety of creative heuristics ...
Creative hypothesis generating in psychology: some useful heuristics. W. Mcguire. Published in Annual Review of Psychology 1997. Psychology. To correct a common imbalance in methodology courses, focusing almost entirely on hypothesis-testing issues to the neglect of hypothesis-generating issues which are at least as important, 49 creative….
Stanley D Fisher. Published 3 March 1980. Psychology. Abstract : This project was devoted to a study of hypothesis generation. Hypothesis generation is the process by which the decision maker specifies possible hypotheses, or states of the world, that are relevant in a decision problem. View via Publisher.
We find that explanation supports learners' generation of broad and abstract hypotheses but does not impact their evaluation of them. These results provide a more precise account of the process by which explanation impacts learning and offer additional support for the claim that hypothesis generation and evaluation play distinct roles in ...
Hypothesis generation is pivotal in psychology [koehler1994hypothesis, mcguire1973yin], as it facilitates the exploration of multifaceted influencers of human attitudes, actions, and beliefs. The HyGene model [ thomas2008diagnostic ] elucidated the intricacies of hypothesis generation, encompassing the constraints of working memory and the ...
While hypothesis testing is a highly formalized activity, hypothesis generation remains largely informal. We propose a systematic procedure to generate novel hypotheses about human behavior, which uses the capacity of machine learning algorithms to notice patterns people might not.
The first is that the reliability of the heuristic hypothesis generation procedure (in the context of discovery) should count no less than the conclusiveness of the final testing procedure (in the context of justification) in establishing scientific facts; nature does not necessarily get the last word. ... Evolutionary psychology and the ...
Abstract. The extent to which hypothesis generation affects hypothesis-testing performance was examined in a rule-discovery task. One hundred eight undergraduates enrolled in introductory psychology were randomly assigned to conditions in which the participants, experimenter, other participants, or no one generated hypotheses before the participants were tested on three different tasks.
Examples. A research hypothesis, in its plural form "hypotheses," is a specific, testable prediction about the anticipated results of a study, established at its outset. It is a key component of the scientific method. Hypotheses connect theory to data and guide the research process towards expanding scientific understanding.
A hypothesis is a tentative statement about the relationship between two or more variables. It is a specific, testable prediction about what you expect to happen in a study. It is a preliminary answer to your question that helps guide the research process. Consider a study designed to examine the relationship between sleep deprivation and test ...
Hypothesis Generation Model. The unit of observation in social psychology is a human who is less consistent in their. behavior and who interacts with their surroundings and other people in quite a ...
The generation effect is a phenomenon whereby information is better remembered if it is generated from one's own mind rather than simply read. ... The generation effect is typically achieved in cognitive psychology experiments by asking participants to generate words from word fragments. ... Lexical activation hypothesis
MACHINE-ASSISTED HYPOTHESIS GENERATION 5 fine-tuned specifically on several thousands of abstracts gathered from 50 social psychology journals over more than 55 years as well as pre-prints such as PsyArXiv. Second, we use the GPT-4 large language model to generate hypotheses based on specific prompts.
5. Phrase your hypothesis in three ways. To identify the variables, you can write a simple prediction in if…then form. The first part of the sentence states the independent variable and the second part states the dependent variable. If a first-year student starts attending more lectures, then their exam scores will improve.
Create a hypothesis for your research based on your research question. HyperWrite's Hypothesis Maker is an AI-driven tool that generates a hypothesis based on your research question. Powered by advanced AI models like GPT-4 and ChatGPT, this tool can help streamline your research process and enhance your scientific studies.
My Research and Language Selection Sign into My Research Create My Research Account English; Help and support. Support Center Find answers to questions about products, access, use, setup, and administration.; Contact Us Have a question, idea, or some feedback? We want to hear from you.
Haidt studies social psychology at New York University. Photograph: Alexander Tamargo/Getty Images for Vox Media. The Anxious Generation has squatted atop the New York Times bestseller list for ...