
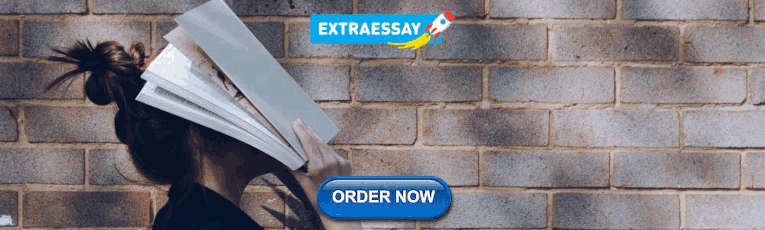
Algorithms of war: The use of artificial intelligence in decision making in armed conflict

In less than a year, Chat-GPT has become a household name, reflecting astonishing advances in artificial intelligence-powered software tools, especially generative AI models. These developments have been accompanied by frequent forecasts that AI will revolutionise warfare. At this stage of AI development, the parameters of what is possible are still being explored, but the military response to AI technology is undeniable. China’s white paper on national defense promoted the theory of the “intelligentization” of warfare, in which leveraging AI is key to the PLA’s modernization plan. The director of the US Cybersecurity and Infrastructure Security Agency Jen Easterly warned that artificial intelligence may be the “ most powerful weapon of our time. ” And whilst autonomous weapon systems have tended to dominate discussions about AI in military applications, less attention has been paid to the use of AI in systems that support human decisions in armed conflicts.
In this post, ICRC Military Adviser Ruben Stewart, and Legal Adviser Georgia Hinds seek to critically examine some of the touted benefits of AI when used to support decisions by armed actors in war. In particular, the areas of civilian harm mitigation and tempo are discussed, with a particular focus on the implications for civilians in armed conflict.
Even before recent hype, you have probably already used AI in various forms, indeed you might be reading this article on a device largely powered by AI. If you have used a fingerprint or face to open your phone, participated on social media, planned journeys using a phone application or purchased anything online from pizzas to books, it has probably involved AI. In many ways we have grown comfortable with AI, adopting it, often unwittingly, into our everyday life.
But what if that facial recognition software was used to identify a person to be attacked? What if, instead of finding the cheapest flight to get you to a destination, software along similar lines was finding aircraft to perform an airstrike on a target. Or, rather than recommending the best pizza place or the closest available taxi, the machine was recommending plans of attack? This is apparently a reality that is ‘coming soon’ from companies developing AI-based decision platforms for defense purposes.
These kinds of AI decision support systems (AI-DSS) are computerised tools that use AI software to display, synthesise and/or analyse data and in some cases make recommendations – even predictions – in order to aid human decision-making in war.
The advantages of AI-DSS are often framed in terms of increased situational awareness and faster decision-making cycles. These claims are unpacked below, in light of both AI system and human limitations, and in the context of the planning processes of modern conflicts.
Minimising risk of harm to civilians in conflict
The advent of new technologies in warfare is often accompanied by assertions that its integration will reduce civilian harm (though this is not always borne out in practice ). In the case of AI-DSS, it has been claimed that such tools could help to better protect civilians in conflict in certain circumstances . Certainly, international humanitarian law (IHL) obliges military commanders and others responsible for attacks to base their decisions on information from all sources available to them at the relevant time. In the context of urban warfare in particular, the ICRC has recommended that information about factors such as the presence of civilians and civilian objects should include open-source repositories such as the internet. Further, specifically considering AI and machine learning, the ICRC has concluded that, to the extent that AI-DSS tools can facilitate quicker and more widespread collection and analysis of this kind of information, they could well enable better decisions in conflict by humans that minimize risks for civilians .
At the same time, any AI-DSS output should be cross-checked between multiple sources to guard against biased or inaccurate information . Whilst this is true of any information source in conflict, it is especially important for AI-DSS; as the ICRC has previously outlined , it may be extremely difficult – if not impossible at times – to verify the accuracy of output due to the system’s functioning and the way in which human users interact with machines. These aspects are expanded below.
System limitations
Recent coverage of AI developments has often included examples of AI failing, sometimes fatally. Examples include software not recognising or misidentifying people with darker skin colour , recommending travel routes that do not take into account updated road conditions , and examples of fatalities resulting from self-driving cars . Some of these failings are explainable, but not excusable, for example, because the data upon which it bases its outputs is biased , corrupted, poisoned or just plainly incorrect. These systems can also still easily be ‘tricked’; techniques can be used to fool the system into misclassifying data . For example, adversarial techniques could conceivably be used in conflict to affect a targeting assistance system’s source code such that it identifies school-buses as enemy vehicles , with devastating consequences.
As AI is used for more complex tasks, and especially when multiple layers of analysis (and possibly decisions and judgements) accumulate, then verifying that final output and the source of any errors that contributed to the final output becomes almost impossible. With increasingly complex systems, the potential for compounding errors increases – a small inadequacy in the first algorithmic recommendation is fed into and skews a second algorithmic process, which feeds into a third, and so on .
Accordingly, AI systems have often exhibited behaviours that cannot be explained by the user or the developer, even after extensive post facto analysis. A study of the high-profile large language model GPT-4 found that its ability to solve a math problem drastically, and inexplicably, reduced from 83.6% to just 35.2% three months later. Unpredictable behaviours can also arise through reinforcement learning , where machines have proven very efficient at adopting and concealing unforeseen, and sometimes negative behaviour to outwit or outplay humans: be that lying to win a negotiation or taking shortcuts to beat a computer games .
Challenges for humans interacting with the machine
AI-DSS do not “make” decisions. However, they do directly and often significantly influence the decisions of humans, including due to humans’ cognitive limitations and tendencies when interacting with machines.
For example, “automation bias” refers to the tendency of humans to not critically challenge a system’s output, or search for contradictory information – especially in time critical situations . This has already been observed in other fields such as healthcare, where the accuracy of diagnoses by experienced radiologists was adversely influenced by false AI outputs .
Inaccurate diagnoses in health settings can be fatal. And so too, in armed conflict over trust can have lethal consequences. In 2003, the US defensive Patriot system twice fired at friendly coalition aircraft based on them being misclassified as attacking missiles. In a subsequent investigation, one of the major shortfalls identified was that “ operators were trained to trust the system’s software .”
These ways of functioning, coupled with these characteristics of human-machine interaction, potentially increase the likelihood of outcomes that diverge from the intention of the human decision-maker. In warfare, this may result in accidental escalation , and in any event will heighten risks for civilians and protected persons .
One touted military advantage of AI is the increase in tempo of decision-making it would give a user over their adversary. Increased tempo often creates additional risks to civilians, which is why techniques that reduce the tempo, such as ‘tactical patience’, are employed to reduce civilian casualties. Slowing the tempo of decision-making, including the processes and assessments that inform the decision, allows both the system and the user the extra time to:
- Understand more; and
- Develop more options.
Importantly, this is true throughout the decision-making chain, not only at the final ‘decision point’. Accordingly, claims that AI-DSS will actually result in greater time for tactical patience, by speeding up time-consuming steps along the way to a final determination of whether to ‘pull the trigger,’ risk oversimplifying the process of targeting and the execution of force in contemporary conflicts.
Extra time allows you to see more
The now infamous drone strike in Kabul on 29 August 2021, during the evacuation of Kabul, which killed 10 civilians was attributed by the commander of Central Command to the fact that “ We did not have the luxury of time to develop pattern of life and to do a number of other things . ”
‘Pattern of life’ analysis is how some militaries describe an assessment of the presence and density of civilians and combatants, their schedules, patterns of movement, etc in and around an area being considered for attack. It is a critical method of reducing civilian harm . However, assessing a pattern of life can only be done in real-time – the time it takes civilians to create such patterns – it cannot be expedited.
Attempts to predict future behavior based on historical trends will not incorporate the current situation. In this example, a review of older intelligence material, especially full motion video of Kabul would not have reflected the changes in situation and behavior occurring because of the Taliban take-over and ongoing evacuation efforts.
As civilian casualty prevention guidance explains “[t] he longer you wait and observe the more you will know about what is going on and be better prepared to make a decision to employ lethal or non-lethal means ” or as Napoleon put it “dress me slowly, I am in a hurry” – sometimes the best results are achieved by doing things deliberately.
Extra time allows a user to understand more
Another reason to slow the tempo of decision-making is that human understanding, especially of a complex and confusing situation takes time to be developed as well as to deliberate on appropriate responses. With less time available, a human’s ability to comprehend the situation will lessen. The military planning process is designed to give commanders and staff the time to consider the operational environment, the adversary, friendly forces and civilians and the advantages and disadvantages of the courses of action being considered. The understanding gleaned from this process of consideration cannot be outsourced for as General Dwight D. Eisenhower explained ”[i] n preparing for battle I have always found that plans are useless, but planning is indispensable.”
This has implications when it comes to human decision-makers considering a course of action generated or ‘recommended’ by an AI-DSS, whose ability to accelerate operational tempo relative to an opponent is probably the most cited reason for it being utilised. Without having undertaken, or even fully understood the process of developing a plan proposed by AI-DSS, the human planner is likely to have a limited understanding of the situation, the various influencing factors, and the actors involved. Indeed, it has been observed that the use of automated aids can reduce the alertness of human users and impair their ability to maintain situational awareness. This should be considered in light of how it affects compliance with IHL obligations; the obligation to do everything feasible to verify targets indicates a requirement to maximise the use of available intelligence, surveillance and reconnaissance assets to gain the most comprehensive situational awareness possible under the circumstances.
Extra time allows a user to develop more options
In addition to allowing a commander to see and understand more, extra time allows commanders to develop tactical alternatives , which could include the decision not to use force or to de-escalate. The extra time allows other units and platforms to disengage, reposition, resupply, plan, and prepare to assist in an upcoming operation. This gives a commander more options, including alternative plans that may better reduce civilian harm. Extra time may allow for additional mitigating measures such as the issuance of warnings and from the civilian perspective it allows them to implement coping mechanisms such as taking shelter, resupplying themselves with food and water or evacuating.
As one example of military planning doctrine explains “ if time is available and there is no advantage to acting more quickly, there can be little excuse for not taking the time to plan adequately. ” For as recalled in NATO’s Protection of Civilians handbook “ [w]hen time is available to deliberately plan, discriminate and precisely target a force or object in accordance with the IHL principles the chances of CIVCAS [civilian casualties] are greatly minimised .”
“War is chaotic, lethal, and a fundamentally human endeavor. It is a clash of wills fought among and between people. All war is inherently about changing human behavior, with each side trying to alter the behavior of the other by force of arms.” Wars result from human disagreement, are waged between groups of humans, are controlled by humans and are concluded by humans who, in the aftermath, have to co-exist. Most importantly, the suffering in conflict is borne by humans.
This reality, and indeed IHL itself, calls for a ‘human-centered’ approach to the development and use of AI in armed conflict – to try to preserve humanity in what is already an inhumane activity. Such an approach has at least two key aspects: (1) a focus on the humans who may be affected; and (2) a focus on the obligations and responsibilities of the humans using or ordering the use of the AI.
When looking at those who may be affected, it is not only about mitigating risks to civilians when using AI-DSS to gain military advantage, there is also the potential to design and use such tools specifically for the objective of civilian protection. Possibilities that have been suggested in this regard include tools to recognise, track and alert forces to the presence of civilian populations, or to recognise distinctive emblems that indicate protected status in armed conflict (see here and here ).
And ensuring that humans can satisfy their obligations under IHL means that AI-DSS should inform but cannot displace human judgment in decisions that pose risks to the life and dignity of people in armed conflict. As much has been widely recognised by states in the context of autonomous weapon systems (see, for example, here , here and here ). The responsibility to comply with IHL lies with individuals and their commanders, not computers. As stated in the US Department of Defense Law of War Manual “ The law of war does not require weapons to make legal determinations… Rather, it is persons who must comply with the law of war. ” China stressed this point more generally in its Ethical Norms for New Generation Artificial Intelligence , with an insistence “that humans are the ultimately responsible entities.”
Assertions that AI-DSS will necessarily result in greater civilian protection and IHL compliance must be critically challenged and measured against these considerations, taking account of what we know about system limitations, human machine interaction, and the effect of increased tempo of operations.
- Tilman Rodenhäuser, Samit D’Cunha, Foghorns of war: IHL and information operations during armed conflict , October 12, 2023
- Fiona Terry, Fabien Dany, Harnessing the power of Artificial Intelligence to uncover patterns of violence , May 25, 2023
- Pierrick Devidal, ‘ Back to basics’ with a digital twist: humanitarian principles and dilemmas in the digital age , February 2, 2023

What can the humanitarian community learn from the Colombian Truth Commission’s LGBTIQ-subchapter?
13 mins read Artificial Intelligence and Armed Conflict / Autonomous Weapons / Humanitarian Action / New Technologies / Technology in Humanitarian Action Elias Dehnen

Falling under the radar: the problem of algorithmic bias and military applications of AI
10 mins read Artificial Intelligence and Armed Conflict / Autonomous Weapons / Humanitarian Action / New Technologies / Technology in Humanitarian Action Ingvild Bode
There are no comments for now.
Leave a comment
Click here to cancel reply.
Email address * This is for content moderation. Your email address will not be made public.
Your comment

- Advisory Board
- Guest Authors
- NEW: Podcast
- Artificial Intelligence
- Climate Change
- Congressional Oversight
- Counterterrorism
- Cybersecurity
- Disinformation
- Human Rights
- Immigration
- Intelligence activities
- International Criminal Law
- Israel-Hamas War
- January 6th Attack on US Capitol
- Law of Armed Conflict
- Local Voices
- Racial Justice
- Social Media Platforms
- United Nations
- Use of Force
- Russia-Ukraine War
- Trump Trials Clearinghouse
Featured Articles
- Write for Just Security
- Signups for A.M. and P.M. emails
AI and the Future of Drone Warfare: Risks and Recommendations
by Brianna Rosen
October 3, 2023
Share this:
- Click to share on Twitter (Opens in new window)
- Click to share on Facebook (Opens in new window)
- Click to share on LinkedIn (Opens in new window)
- Click to share on Reddit (Opens in new window)
- Click to print (Opens in new window)
Filed under:
Artificial Intelligence , Congress , Department of Defense (DoD) , Drones , Emerging technology , Human Rights , International Humanitarian Law (IHL) , LAWS , Russia-Ukraine War
The next phase of drone warfare is here. On Sep. 6, 2023, U.S. Deputy Defense Secretary Kathleen Hicks touted the acceleration of the Pentagon’s Replicator initiative – an effort to dramatically scale up the United States’ use of artificial intelligence on the battlefield. She rightfully called it a “game-changing shift” in national security. Under Replicator, the U.S. military aims to field thousands of autonomous weapons systems across multiple domains in the next 18 to 24 months.
Yet Replicator is only the tip of the iceberg. Rapid advances in AI are giving rise to a new generation of lethal autonomous weapons systems (LAWS) that can identify, track, and attack targets without human intervention. Drones with autonomous capabilities and AI-enabled munitions are already being used on the battlefield, notably in the Russia-Ukraine War . From “killer algorithms” that select targets based on certain characteristics to autonomous drone swarms, the future of warfare looks increasingly apocalyptic.
Amidst the specter of “warbot” armies, it is easy to miss the AI revolution that is underway. Human-centered or “responsible AI,” as the Pentagon refers to it, is designed to keep a human “in the loop” in decision-making to ensure that AI is used in “lawful, ethical, responsible, and accountable ways.” But even with human oversight and strict compliance with the law, there is a growing risk that AI will be used in ways which fundamentally violate international humanitarian law (IHL) and international human rights law (IHRL).
The most immediate threat is not the “AI apocalypse” – where machines take over the world – but humans leveraging AI to establish new patterns of violence and domination over each other.
Drone Wars 2.0
Dubbed the “first full-scale drone war,” the Russia-Ukraine War marks an inflection point where states are testing and fielding LAWS on an increasingly networked battlefield. While autonomous drones reportedly have been used in Libya and Gaza , the war in Ukraine represents an acceleration of the integration of this technology into conventional military operations, with unpredictable and potentially catastrophic results. Those risks are even more pronounced with belligerents who may field drones without the highest level of safeguards due to lack of technological capacity or lack of will.
The lessons from the war in Ukraine include that relatively inexpensive drones can deny adversaries air superiority and provide a decisive military advantage in peer and near-peer conflicts, as well as against non-state actors.
The United States and other countries are taking these lessons seriously. Mass and speed will apparently dominate the future drone wars, as the United States – through Replicator and other initiatives – seeks to develop the capacity to deploy large amounts of cheap, reusable drones that can be put at risk to keep pace with adversaries such as China. Increasingly, discrete drone strikes against non-state actors will be displaced by AI-enabled drone swarms that communicate with each other and work together (and with humans) to destroy critical infrastructure and other targets.
This emerging technology poses even greater risks to civilians than the drone wars of the past. Unlike conventional drone warfare, which is vetted and controlled by human operators, the new drone wars will be more automated. Human-machine collaboration will pervade nearly every stage of the targeting cycle – from the selection and identification of targets to surveillance and attack. The largest shift will be the least visible, as proprietary algorithms sift through reams of intelligence data and drone feeds to compile target lists for human approval.
While humans may continue to sign off on the use of lethal force, AI will play a more pervasive role in shaping underlying choices about who lives and dies and what stands or is destroyed.
As AI reduces human involvement in killing, drone warfare will most likely become less explainable and transparent than it is now. This is true not only for the public – which is already kept in the dark – but also for government officials charged with implementing and overseeing the drone program.
The problem of explainability, where humans cannot fully understand or explain AI-generated outcomes, is a broader issue with AI that is not limited to drone strikes. Computational systems that rely on AI tend to be opaque because they involve proprietary information, evolve as they learn from new data, and are too complex to be understood by any single actor.
But the problem of explainability is particularly acute when it comes to drone warfare.
In the sprawling U.S. interagency process, military and intelligence agencies rely on different information streams, technology, and bureaucratic procedures to support the drone program. These agencies are developing their own AI tools which are highly classified and based on algorithms and assumptions that are not shared with key policymakers or the public. Add to this mix AI systems producing outcomes that cannot be fully understood and it will be impossible for government officials to explain why an individual, for example, was mistakenly targeted and killed.
The problem of explainability will foster a lack of accountability in the coming drone wars – something that is already in short supply. When civilians are mistakenly killed in AI-enabled drone strikes, Pentagon officials will also be able to blame machines for these “ tragic mistakes .” This is especially the case for drone swarms, where drones from different manufacturers may fail to communicate properly, despite the Pentagon spending millions of dollars on the technology. As drones begin to talk to each other as well as to humans, the accountability and legitimacy gap between the human decision to kill and the machines performing the lethal act is likely to grow.
Minding the Gap
These challenges are well known, and the Pentagon has long touted a policy of “ responsible AI ” that aims to address them through a labyrinth of laws and regulations. This sounds good on paper, but the conventional drone program, too, was promoted as being “ legal, ethical, and wise ” before serious concerns about civilian harm surfaced. If the past drone wars are any indication, truly responsible AI drone warfare similarly may prove elusive, particularly where gaps in protection arise in the various legal, ethical, and policy frameworks that govern AI use.
For this reason, several states and the International Committee of the Red Cross (ICRC) have proposed banning weapons systems that lack meaningful human control and are too complex to understand or explain. In the first United Nations Security Council meeting on AI in July, U.N. Secretary-General António Guterres proposed that states adopt within three years a “legally-binding instrument to prohibit lethal autonomous weapons systems that function without human control or oversight, which cannot be used in compliance with international humanitarian law.”
But even if states agree to such a ban in principle, significant questions remain. What legal limits must be placed on autonomous weapons systems to ensure compliance with IHL? What type and degree of human control is needed to ensure that future drone strikes meet the IHL principles of necessity, proportionality, and discrimination, as well as precaution? Is compliance with IHL sufficient or is a new treaty required? While many states have called for such a treaty, the United States , Russia , and India maintain that LAWS should be regulated under existing IHL.
As the new drone wars become more ubiquitous, the exceptional rules that are said to apply in war – notably the lower levels of protections afforded by IHL – risk becoming the default regime. In the long term, the practical effects of this are the continued erosion of the prohibition on the use of force and the adoption of increasingly permissive interpretations of international law. The full costs and consequences of these developments are still emerging, but the precedents set now are likely to undermine individual rights in pernicious and irreversible ways.
To counter this trend, states at a minimum should reaffirm the application of IHRL within and outside of armed conflict. The individualisation and automation of war has prompted a turn toward principles enshrined in IHRL, such as a stricter interpretation of the necessity criterion under certain conditions and similarly the provision that force should be used only if bystanders are unlikely to be harmed. Yet while IHRL offers additional protections beyond IHL, the precise interaction between IHL and IHRL is disputed and varies according to state practice. Fundamentally, these legal regimes were not designed to regulate non-traditional conflicts and non-traditional means of using lethal force, and gaps in legal protections are likely to grow wider in the coming drone wars.
These gaps have prompted the ICRC to emphasize “the need to clarify and strengthen legal protections in line with ethical considerations for humanity.” In cases not covered by existing treaties, Article 1(2) of Additional Protocol I and the preamble of Additional Protocol II to the Geneva Conventions, commonly referred to as the “ Martens Clause ,” provide that individuals should be protected by customary IHL, as well as the “principles of humanity and the dictates of public conscience.”
But ethical considerations may diverge substantially from the law. The relationship between morality and law is a longstanding scholarly debate beyond the scope of this article. Briefly, the law serves a different purpose from morality insofar as it must consider the effect that conventions will have on behavior, degrees of epistemic uncertainty in the real world, and anarchy in the international system. Under these circumstances, the morally optimal laws may be, in the words of Henry Shue, just those that “can produce relatively few mistakes in moral judgment – relatively few wrongs – by angry and frightened mortals wielding awesomely powerful weapons.”
The unpredictable and complex nature of AI, however, complicates efforts to discern, ex ante , the right course of action. Even when humans follow all the legal and policy guidelines, the gap between human decision-making and machine action implies that outcomes may not be moral. Far from it.
What is moral may not be legal or wise – and vice versa.
Policy guidance, meanwhile, is not a substitute for the protections that the law affords. The newly crafted U.S. Presidential Policy Memorandum (PPM), for example, is supposed to provide additional protections above what the laws of war require for direct action, that is, drone strikes and special operations raids. But the policy guidance is not legally binding, can secretly be suspended at any time, contains numerous exemptions for collective and unit self-defense, and applies to only a fraction of U.S. drone strikes outside of “areas of active hostilities,” notably in Iraq and Syria.
Moreover, the policy guidance was written with conventional drone strikes in mind. As the world stands at the precipice of a new phase in AI-driven drone warfare, it is time to rethink the rules.
Walking Back from the Precipice
There have been a number of proposals for regulating lethal autonomous weapons systems, including AI-enabled drones. But if the past drone wars are any indication, these regulations are still likely to fall short. Human oversight and compliance with existing laws and standards is essential, but not sufficient.
To more fully protect civilians in the coming drone wars, U.S. policymakers should take the following steps as a matter of urgency:
- Develop a U.S. government-wide policy on the use of AI in drone warfare. While the Department of Defense has published numerous guidelines on AI and autonomous weapons systems , these directives do not necessarily apply to other agencies, such as those in the U.S. Intelligence Community. This oversight is deeply concerning given the crucial role that these other agencies may play in identifying, vetting, and attacking targets on a routine basis.
- Follow the “two-person rule.” During the Cold War, the two-person rule required two or more authorized individuals to be present when nuclear weapons or material were being repaired, moved, or used. This rule was designed to prevent nuclear accidents or misuse that could pose significant risks to human life. AI-enabled weapons have similar potential for catastrophic results and should follow the same rule for all drone operations.
- Reduce the accountability gap. Increasing autonomy in drone warfare will make strikes more unpredictable, resulting in mistakes that cannot be attributed to any particular individual. To reduce this risk, the timeframe between when humans approve a target for lethal action and when drones take that action should be minimized to mere seconds or minutes, not days or months. Under no circumstances should drones be allowed to independently target individuals who are on a pre-approved (human approved) “kill list.”
- Conduct and publish routine AI health audits. To mitigate the problem of explainability, humans must check AI and AI must check itself. “ Checking AI ” can be a powerful tool in ethical audits, helping humans test AI systems and identify flaws or underlying biases in algorithms. AI health checks must be performed at regular intervals, and the results should be briefed to members of Congress (e.g., the Gang of Eight), and a redacted version should be made available to the public.
Pandora’s box has been opened, but policymakers can still place necessary guardrails on the AI revolution in drone warfare. In the words of Martin Luther King, the United States is “confronted with the fierce urgency of now” and there is “such a thing as being too late.”
Image: Drone swarm (via GettyImages).
About the author(s), brianna rosen.
Brianna Rosen ( @rosen_br ) is a Senior Fellow at Just Security and a Strategy and Policy Fellow at Oxford University's Blavatnik School of Government. She previously served for a decade in the U.S. government, including at the White House National Security Council and Office of the Vice President.
Send A Letter To The Editor
Read these related stories next:
At the supreme court, public corruption jurisprudence and lack of meaningful ethics reform go hand-in-hand.
by Virginia Canter and Gabe Lezra
Apr 15th, 2024
House Meeting on White House AI Overreach Highlights Congressional Inaction
by Melanie Geller and Julian Melendi
Apr 12th, 2024
Russian Human Rights Activist Vladimir Kara-Murza Marks Two Years Behind Bars
by Evgenia Kara-Murza
Apr 10th, 2024
A Quarter Century After the Ottawa Landmine Treaty, the World Needs a UN Fund for Victims
by Ben Keith
Apr 9th, 2024
Deportation, Detention, and Other Crimes: In Ukraine, the Past and Present of International Criminal Law Converge
by Andrew Boyle
In Shifting US Ties with Niger and Africa, Focus on Human Rights and Democracy to Strengthen Partnerships
by Ikechukwu Uzoma
Apr 8th, 2024
Symposium: International Law in Ukraine – The View from Lviv
by Just Security
Apr 5th, 2024
The SAFE Act Is No “Compromise” and Won’t Leave Americans Safer
by George Croner
National Security at the United Nations This Week (Apr. 1-5)
by Mary Szarkowicz
Apr 4th, 2024
Bringing Transparency to National Security Uses of Artificial Intelligence
by Faiza Patel and Patrick C. Toomey
Why We Need a National Data Protection Strategy
by Alex Joel
The Just Security Podcast: The Starvation War Crime in Sudan and Gaza
by Tom Dannenbaum , Tess Bridgeman and Paras Shah
Autonomous drone swarms and the contested imaginaries of artificial intelligence
- Open access
- Published: 11 January 2024
Cite this article
You have full access to this open access article
- Jutta Weber 1
991 Accesses
4 Altmetric
Explore all metrics
AI-based, autonomous weapon systems (AWS) have the potential of weapons of mass destruction and thereby massively add to the intensifying dialectic of fear between ground and space and the pervasive mass human vulnerability of being tracked and targeted from above. Nevertheless, the dangerous effects of the proliferation of AWS have not been and still are not widely acknowledged. On the one hand, the capabilities and effects of AWS are downplayed by the military and the arms industry staging these systems as precise and clean. Recently, it is also argued that they can be built on the basis of a ‘responsible’ or ‘trustworthy’ artificial intelligence (AI). On the other hand, inadequate sociotechnical imaginaries of AI as a conscious, evil super-intelligence circulated by Hollywood blockbuster films such as 'Terminator' or 'Ex Machina' dominate the public discourse. Their massive overstatement of the power of the technology and also their focus on often irrelevant imaginaries such as the ‘Terminator’ hinders a realistic understanding of the AI’s capabilities. Against this background, arms control advocates develop new imaginaries to show the loss of ‘meaningful human control’ (Sharkey 2016) and its problematic consequences. In October 2023, the deployment of autonomous military in the battlefield has already been officially confirmed by an Ukrainian drone company (Hambling 2023).
Similar content being viewed by others
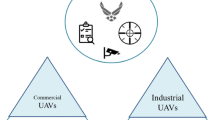
Unmanned aerial vehicles (UAVs): practical aspects, applications, open challenges, security issues, and future trends
Syed Agha Hassnain Mohsan, Nawaf Qasem Hamood Othman, … Muhammad Asghar Khan
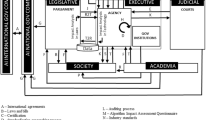
Artificial Intelligence Regulation: a framework for governance
Patricia Gomes Rêgo de Almeida, Carlos Denner dos Santos & Josivania Silva Farias
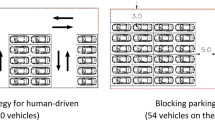
Exploring the implications of autonomous vehicles: a comprehensive review
Kareem Othman
Avoid common mistakes on your manuscript.
Introduction
Negotiations over a ban on lethal AWS have been ongoing at the Convention on Certain Conventional Weapons (CCW) in Geneva since 2014. In parallel, the problematic impact of AWS, as well as tele-operated drones, has been critically debated in academia (Gregory 2011 , Bhuta et al. 2016 , Suchman and Weber 2016 , Weber 2016 ) as well as by non-governmental and investigative journalist organizations, i.e., such as the Campaign to Stop Killer Robots, Code Pink, the Bureau of Investigative Journalism, the International Committee for Robot Arms Control (ICRAC). The massive violations of human rights by tele-operated drones were documented and discussed while the possible severe humanitarian consequences of the deployment of AWS were outlined.
Nevertheless, the efforts of human rights and arms control advocates have had little effect in the public and political arena so far. Against all warnings of the devastating consequences of these systems for civilians - while also ignoring the problem that these systems serve as a trigger for a new global arms race -, drones are staged as ‘precise and clean’ and a remedy to save the lives of one’s own soldiers, by the military, the defense industry, as well as politicians. Against this background, AWS have at the same time been staged for many years as a problem that will only become reality in the far future or that they will always be controllable through a human on the loop or through ‘responsible AI’ (Scharre 2018 ).
The interest in AWS is rising in many nations as loitering munition (so-called kamikaze drones) is increasingly discussed in the media as the decisive game changer for military advantage, for example already in the Nagorno-Karabakh war (Deutsche Welle 2021 ) and today especially in the Russian-Ukraine war (Hambling 2023 ). And again, there is little debate about the tremendous consequences of these weapons for civilians as AWS turn into a permanent threat from above: ‘Some next-generation military drones rely on artificial intelligence to circle over an area, pick out enemy units and destroy them. In the coming years, drone technology will improve, and the cost of drones will decline. As they do, the frightening truth is that troops and civilians in future conflicts will find fewer and fewer places to hide from the gaze of both man and machine’ (Kingsbury 2022 ). The recently started Israel-Gaza war is a terrifying example for this development (Davies et al. 2023 ).
Against this background, several legal, social science and humanities scholars as well as journalists and activists claim that a ‘new human right to protect the freedom to live without physical or psychological threat from above’ (Grief et al. 2018 ) are required. This proposed new human right has been developed in the Airspace Tribunal hearings in London, Sydney, Toronto and Berlin in the last years.
Contested imaginaries of AI
According to some AI experts, an enormous obstacle to a realistic debate about the potentials of AWS is the sociotechnical resp. pop-cultural imaginaries of AI shaped by Hollywood blockbuster films such as the Terminator series, ‘Ex Machine’, or ‘I, Robot’. These films often stage autonomous AI dramatically as a conscious, evil super-intelligence striving for the erasure of the human race. While the power of the technology is massively overstated, it is at the same time neutralized through this overstatement: The popular discourse revolves around the question of whether AI can gain consciousness, while the concrete effects of applied AI, such as the loss of meaningful human control (Sharkey 2016 ) and the reconfiguration of human–machine relations (Suchman and Weber 2016 ), have been mostly overlooked. ‘We have witnessed high-level defense officials dismissing the risk on the grounds that their “experts” do not believe that the “Skynet thing” is likely to happen. Skynet, of course, is the fictional command and control system in the Terminator movies that turns against humanity. The risk of the “Skynet thing” occurring is completely unconnected to the risk of humans using autonomous weapons as WMDs or to any of the other risks […]. If even senior defence officials with responsibility for autonomous weapons programs fail to understand the core issues, then we cannot expect the general public and their elected representatives to make appropriate decisions’ (Russell et al. 2018 ).
‘Enough to kill half a city’
Arms control advocates—from peace researchers to computer scientists—aim to foster a more realistic imaginary of AWS and to illustrate their deadly potential. The Future of Life Institute and well-known AI expert Stuart Russell together with a professional film team developed short YouTube videos to make the consequences explicit for a broader audience. The first video called ‘Slaughterbots’ went viral after its release in 2017. It had received more than two million clicks within a few days, even though it was not an SF trailer but a science communication video. The Slaughterbots video starts with a typical CEO presentation in which the protagonist demonstrates the capabilities of the emergent drone swarms, released in hundreds or thousands from an aeroplane, which allow according to the CEO an ‘airstrike of surgical precision … A 25-million-dollar order now buys this … Enough to kill half a city, the bad half’, because it ‘allows you to separate the good guys from the bad’ (Slaughterbots 2017 ). The drones are equipped with face recognition software to follow and kill selected targets—according to their social media profiles, for example. With this new weapons system, the CEO claims, ‘nuclear is obsolete’ (ibid.). The rest of the video develops two main scenarios in which critical members of parliament and hundreds of politically engaged students are lethally attacked by drone swarms. Stuart Russell warns of the problems and effects of autonomous weapons: ‘What we were trying to show was the property of autonomous weapons to turn into weapons of mass destruction automatically because you can launch as many as you want’ (ibid.). The video impressively sketches the potential for mass destruction of AWS—also in the civilian context. Russell makes clear that the dangerous capabilities of AWS shown in the film are not decades away (as often claimed by some countries at the CCW talks in Geneva), but the ‘results of integrating and militarizing technologies that we already have’ (ibid.)—and that this development needs to be stopped: ‘Allowing machines to choose to kill humans will be devastating to our security and freedom. We have an opportunity to prevent the future you just saw, but the window to act is closing fast’ (ibid.). In the meantime, the first autonomous drones seem to operate. In 2020, an UN report stated that the first autonomous drones have been used in Libya (United Nations, Security Council Report on Second Libyan Civil War 2020 , 17/548) but this statement was never confirmed. In 2023, the Ukrainian drone company Saker proudly announced the use of autonomous drones (Hambling 2023 ).
One of the key issues of understanding AWS is the complex and multiple meanings of autonomy. While in the humanities and social sciences as well as everyday life, autonomy is associated with a free and self-aware subject which acts self-determinedly and consciously. Even though this Kantian concept has been challenged by well-known theorists from Karl Marx to Judith Butler, it still predominates in realms such as ethics, law, economics and everyday life. The concept of autonomy used in AI and robotics has a very different meaning: It follows a cybernetic concept of purposeful behavior in the sense of a pragmatic physiological automated mechanism, like the target-seeking mechanism of a torpedo. Today’s control mechanisms in AI systems are much more sophisticated than traditional servomechanisms but nevertheless AWS do neither follow their ‘own’ rules nor are they capable of decision-making in a wider, (self-)reflective sense. They are determined by norms, values and categories which were programmed into the software by computer scientists—and although the complexity of software layers might lead to unpredictable effects, these are not intentional (Suchman/Weber 2016 ). The Slaughterbots drones can find and follow targets. For example, they use social media profiles to find and follow the people to be killed. But this behaviour is preprogrammed. This sophisticated entanglement of autonomous and preprogrammed behavior in autonomous systems makes it so difficult to understand the challenges they pose.
The arms control imaginary: WMDs
In the Slaughterbots video, it becomes obvious that autonomous drone swarms are not self-determined and self-conscious intelligent ‘organisms.’ The ‘Slaughterbots’ are programmed to select their targets via data analytics according to pre-given criteria: For example, identifying, searching and targeting leftist students engaged in an anti-corruption NGO via their social media profiles. The bots seek their targets using facial recognition and to kill them with explosives. The Slaughterbots may show coordinated, flexible behavior to perform their tasks (avoiding obstacles, following humans, etc.), but these swarms are neither conscious nor capable of setting their own agendas and develop their own goals. The arms control imaginary strives to show the decisive difference between a Hollywood imaginary of the self-conscious, intelligent, autonomous AI and a more realistic Slaughterbots imaginary of AI as a collection of smart software programs.
The imaginary the Slaughterbots’ video emphasizes that today’s AI makes it possible to automate sophisticated and sensible tasks that are normally performed by humans. These software programs are not intelligent in themselves. Nevertheless, adaptive, coordinated drones as well as drone swarms can easily be turned into WMDs.
The arms control advocates’ Slaughterbots video is in my view an important step toward the development of a new AI imaginary that is not build on the old trope of the evil, almighty wrongdoer, but which makes the eminent questions of arms control of AI-based systems and the dimension of lethal autonomous weapon systems as weapons of mass destruction visible. Today autonomous drones are already in operation though many activists are working towards the ban of such systems. This ban is desperately needed as an important contribution to ending the fear of being tracked and targeted from above as demanded by the Airspace Tribunal.
This essay partially relies on an earlier paper: ‘Artificial Intelligence and the Sociotechnical Imaginary: On Skynet, Self-Healing Swarms and Slaughterbots.’ In: Kathrin Maurer, Andreas Immanuel Graae (Eds.): Drone Imaginaries and the Power of Vision. Manchester: Manchester University Press 2021
Autonomous weapons are defined as systems ‘that, once activated can track, identify, and attack targets with violent force without further human interaction’ (Sharkey 2016 , 3)
For the concept of the imaginary see Jasanoff and Kim ( 2009 , 2015 ), Mager and Katzenbach ( 2021 ), McNeil et al. ( 2017 )
Slaughterbots, directed by S. Sugg, written by M. Wood, YouTube ( 2017 ), last accessed 10.10.2022, www.youtube.com .
Bhuta, N., S. Beck, R. Geiss, H.-Y. Liu, and C. Kress, eds. 2016. Autonomous Weapon Systems: Law, Ethics, Policy . Cambridge: Cambridge University Press.
Google Scholar
Davies, Harry., Bethan McKernan, and Dan Sabbagh. 2023. ‘The Gospel’: how Israel uses AI to select bombing targets in Gaza . The Guardian. https://www.theguardian.com/world/2023/dec/01/the-gospel-how-israel-uses-aito-select-bombing-targets . Accessed 20 Dec 2023.
Deutsche Welle. 2021. The Future of Modern Warfare: How Technology is Transforming Conflict. https://www.youtube.com/watch?v=TmlBkW6ANsQ . Accessed 10 Oct 2022.
Graae, A.I., and K. Maurer, eds. 2020. Drone Imaginaries and the Power of Vision . Manchester: Manchester University Press.
Gregory, D. 2011. From a View to a Kill: Drones and Late Modern War. Theory, Culture & Society 28 (7–8): 188–215.
Article Google Scholar
Grief, N., S. Illingworth, A. Hoskins, and C. Conway. 2018. The Airspace Tribunal: Towards a New Human Right to Protect the Freedom to Exist Without a Physical or Psychological Threat from Above. European Human Rights Law Review. 3: 201–207.
Hambling, D. 2023 Ukraine’s AI Drones Seek And Attack Russian Forces Without Human Oversight. https://www.forbes.com/sites/davidhambling/2023/10/17/ukraines-ai-drones-seek-and-attack-russian-forces-without-human-oversight/ . Accessed 16 Nov 2023.
Jasanoff, S., and S.-H. Kim. 2009. Containing the Atom: Sociotechnical Imaginaries and Nuclear Power in the United States and South Korea. Minerva 47 (2): 119–146.
Jasanoff, S., and S.-H. Kim, eds. 2015. Dreamscapes of Modernity: Sociotechnical Imaginaries and the Fabrication of Power , 1–33. Chicago: University of Chicago Press.
Book Google Scholar
Kingsbury, A. 2022. No One Can Hide from this Weapon in the War in Ukraine. New York Times. https://www.nytimes.com/2022/05/30/opinion/drones-ukraine-war.html?searchResultPosition=8 . Accessed 30 May 2022.
Mager, A., and C. Katzenbach. 2021. Future Imaginaries in the Making and Governing of Digital Technology: Multiple, Contested, Commodified. New Media & Society 23 (2): 223–236.
McNeil, M., M. Arribas-Ayllon, J. Haran, A. Mackenzie, and R. Tutton. 2017. Conceptualizing Imaginaries of Science, Technology, and Society. In The Handbook of Science and Technology Studies , 4th ed., ed. U. Felt, R. Fouché, C.A. Miller, and L. Smith-Doerr, 435–463. Cambridge: MIT Press.
Russell, S., Aguirre, A., Conn, A., and Tegmark, M. (2018). Why You Should Fear “Slaughterbots”: A Response. IEEE Spectrum. https://spectrum.ieee.org . Accessed 10 Oct 2022.
Scharre, P. 2018. Army of None. Autonomous Weapons and the Future of Warfare . New York: W.W. Norton & Company.
Sharkey, Noel. 2016. Staying in the Loop: Human Supervisory Control of Weapons. In Autonomous Weapons Systems: Law, Ethics, Policy , ed. Nehal Bhuta, et al., 23–38. Cambridge: Cambridge University Press.
Slaughterbots. 2017. Directed by S. Sugg, Written by M. Wood, YouTube. www.youtube.com . Accessed 10 Oct 2022.
Suchman, L., and J. Weber. 2016. Human-Machine Autonomies. In Autonomous Weapon Systems: Law, Ethics, Policy , ed. N. Bhuta, S. Beck, R. Geiss, H.-Y. Liu, and C. Kress, 75–102. Cambridge: Cambridge University Press.
United Nations, Security Council 2021. Letter dated 8 March 2021 from the Panel of Experts on Libya established pursuant to resolution 1973 (2011) addressed to the President of the Security Council.
Weber, J. 2016. Keep Adding: Kill Lists, Drone Warfare and the Politics of Databases. In: M. DeGoede, A. Leander and G. Sullivan (eds), ‘The Politics of the List: Law, Security, Technology’, Special Issue, Environment and Planning D: Society and Space, vol. 34, no. 1, eds. M. DeGoede, A. Leander and G. Sullivan, 107–125.
Download references
Open Access funding enabled and organized by Projekt DEAL.
Author information
Authors and affiliations.
Institute of Media Studies, Paderborn University, Paderborn, Germany
Jutta Weber
You can also search for this author in PubMed Google Scholar
Corresponding author
Correspondence to Jutta Weber .
Rights and permissions
Open Access This article is licensed under a Creative Commons Attribution 4.0 International License, which permits use, sharing, adaptation, distribution and reproduction in any medium or format, as long as you give appropriate credit to the original author(s) and the source, provide a link to the Creative Commons licence, and indicate if changes were made. The images or other third party material in this article are included in the article's Creative Commons licence, unless indicated otherwise in a credit line to the material. If material is not included in the article's Creative Commons licence and your intended use is not permitted by statutory regulation or exceeds the permitted use, you will need to obtain permission directly from the copyright holder. To view a copy of this licence, visit http://creativecommons.org/licenses/by/4.0/ .
Reprints and permissions
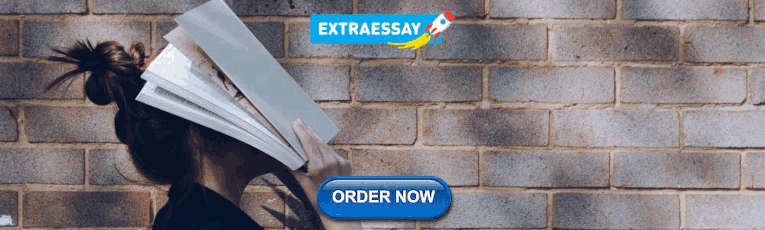
About this article
Weber, J. Autonomous drone swarms and the contested imaginaries of artificial intelligence. Digi War (2024). https://doi.org/10.1057/s42984-023-00076-7
Download citation
Accepted : 17 November 2023
Published : 11 January 2024
DOI : https://doi.org/10.1057/s42984-023-00076-7
Share this article
Anyone you share the following link with will be able to read this content:
Sorry, a shareable link is not currently available for this article.
Provided by the Springer Nature SharedIt content-sharing initiative
- Autonomous weapon system
- Sociotechnical imaginary
- Artificial intelligence
- Mass destruction
- Targeting from above
- Arms control
- Find a journal
- Publish with us
- Track your research

An official website of the United States government
The .gov means it’s official. Federal government websites often end in .gov or .mil. Before sharing sensitive information, make sure you’re on a federal government site.
The site is secure. The https:// ensures that you are connecting to the official website and that any information you provide is encrypted and transmitted securely.
- Publications
- Account settings
Preview improvements coming to the PMC website in October 2024. Learn More or Try it out now .
- Advanced Search
- Journal List
- Elsevier - PMC COVID-19 Collection

Managing the drone revolution: A systematic literature review into the current use of airborne drones and future strategic directions for their effective control
Commercial and private deployment of airborne drones is revolutionising many ecosystems. To identify critical issues and research gaps, our systematic literature review findings suggest that historic issues such as privacy, acceptance and security are increasingly replaced by operational considerations including interaction with and impacts on other airspace users. Recent incidents show that unrestricted drone use can inflict problems on other airspace users like airports and emergency services. Our review of current regulatory approaches shows a need for further policy and management response to both manage rapid and efficient drone usage growth, and facilitate innovation (e.g. intraurban package delivery), with one promising strategic response being low altitude airspace management (LAAM) systems for all drone use cases.
- • Historic issues such as privacy, acceptance and security increasingly replaced by operational and strategy considerations.
- • The literature on drones is wide and not significantly concentrated in any particular source or to any author/ institution.
- • Drone usage can be categorised into 4 uses: monitoring/inspection/data collection, photography, recreation and logistics.
- • Low altitude airspace management (LAAM) as strategic response for all drone use cases.
1. Introduction
Remote technology and automation have been present for centuries, giving human operators safety from harm and enabling new task functionality (increasing capability of individual operations and capacity of the system). Early examples include fireships, a maritime drone, which were used in navies to destroy other ships remotely. In World Wars 1 and 2, airborne drones were used to disrupt airspace above cities, drop ordinance on enemy territory and as target practice for pilots. Railways have for some time used drone (non-crewed) locomotives to support driver occupied locomotives.
While drones have had a long history in military deployment, their increasingly widespread use in non-military roles requires consideration (e.g., Hodgkinson and Johnston, 2018 ). Though current usage is limited whilst the technology is in the development phase, as they possess significant potential versatility drones may transform the way that logistics services are provided. Their use no doubt will lead to the achievement of new business, social, environmental and other goals ( Atwater, 2015 ). However, it also creates a potentially disruptive scenario as their usage expands out of control and causing problems for other parts of the economic system, as illustrated in the rapidly growing literature presented in this paper.
Interestingly, during the COVID-19 crisis drone potential has been further harnessed, using the people free nature of the technology to modify current service delivery to improve safety and capacity levels, including the delivery of face masks to remote islands in Korea and prescription medicines from pharmacies to retirement villages in Florida. It could be argued that COVID-19 has increased technological advancement in many areas and that perhaps drones represent a revolution in how we transport goods and potentially even ourselves (however that is analysis for a future paper).
In that sense, it is important to note that the use of drones in larger commercial applications is also growing (see, e.g. Bartsch et al., 2016 ), with their deployment in remote work leading to significant cost reductions and capability enhancements (such as in mining, engineering and transport network management contexts and agricultural scanning). Their ability to view large areas at a low cost from altitude provides new viewing aspects and new data acquisition ability (or existing data can be sourced at a large scale at a lower cost) to make decisions and manage operations more effectively. Similarly, airborne photography has entered a new stage of development with operators, both large and small, able to give consumers new imagery that had previously been in the domain of birds only. Besides, the recent spurt of the retail sale of drones for recreational and small-scale commercial purposes has pushed airborne drones into the entertainment space.
However, there is a range of other potential uses. Experience in delivering medical supplies in remote African areas gives a potential preview of their role in urban parcel/package delivery, radically changing the way small deliveries are made in urban areas. Commercial and policymaking efforts are turning to contemplate this future and how airborne drones may need control in such uses. This may have significant impacts, not only on delivery cost but on urban congestion and traffic management issues – should they replace land-based journeys. Being in urban areas, implementation issues will arise that require consideration, given the greater risks involved.
While there have been earlier reviews (e.g. a techno-ethical one, Luppicini and So, 2016 ), the commercial use of drones is yet to be written about in any significant volume in the management literature. Preliminary issues like privacy/security received the required attention, given the potential for drones to peer (visually or audially, and intentionally or not) into areas that were previously easy to guard. With increased use, the focus has moved to the engineering literature where a range of computer, materials and design issues are being discussed. Recently, the management literature has begun to case study how drones are used in current commercial contexts, and more importantly, has begun to consider the broader role that drones may play in the logistics industry. What is missing, in our view, is a clear understanding as to where to next, given that increased use cases and traffic volumes might not only significantly disturb other airspace users but also bring the drone ecosystem itself to a standstill (uncontrolled chaos scenario). We aim to investigate whether the emerging body of literature can provide sufficient answers and solutions or at least trending ideas on how to provide drone use with a framework that allows this evolving industry to continue growing at a rapid pace and also to innovatively disturb traditional business models in an economically ordered and safe manner.
This paper reviews the extant literature on the potential implementation of drones into the economic system and specifically how the implementation and ongoing use may be managed. Section 2 outlines our methodology for conducting the systematic review. Section 3 then presents our bibliometric results and discusses the issues being reported in the literature and highlights the four main use cases for drones (based on a content analysis of the reviewed papers) that we see being discussed. Section 4 examples current regulatory steps and then conclude in Section 5 with some discussion and identification of future research avenues, including the need for greater regulation of the drone ecosystem at the macro level and the potential for low altitude air management systems (LAAM).
2. Methodology
Originally developed in the medical literature the systematic literature review (SLR) has been used as a methodology in a range of management papers. In the transport literature it has been deployed in areas such as supply chain (e.g. Perera et al., 2018 ) and in aviation management (e.g. Ginieis et al., 2012 ; Spasojevic et al., 2018 ). Whilst not a strict laboratory controlled study ( Ginieis et al., 2012 ), they do give researchers and practitioners a flavour for the extent and coverage of the literature, and some vision as to where and by whom it is being generated and what it covers.
Drones have received literary attention for some time, primarily in legal/ethical, engineering and computer science fields. For this paper, we have focussed on management literature, given our interest in investigating drone management and related issues. Importantly, we ignore any military/defence use of drones to focus only on civilian applications. While ground-based and maritime drones are also present in the literature ( Pathak et al., 2019 ), the term ‘drones’ is now widely understood to refer to airborne ones, upon which we focus.
For our search, we developed a search string in Scopus composed of a keyword search for ‘drone*‘. We added synonyms like ‘unmanned aviation’, ‘unmanned aircraft*', ‘unmanned aerial vehicle*', ‘UAV’, or ‘remotely piloted aircraft*', which yielded 65,953 documents. We then restricted the results to the Scopus allocated subject areas of ‘Business, Management and Accounting’ (which includes a variety of areas such as innovation, strategy or logistics and supply chain management), or ‘Economics, Econometrics and Finance’ (yielding 1567 documents). Further, we restricted results to articles (published and in press), conference papers or book chapters (1133 documents), and we restricted the search to articles published in the last five years only, since the beginning of 2015 (519 documents). Finally, we limited results to the English language (505 documents).
Using Covidence (an online tool that aids in the faster review of documents through work flowing the review process and collaborative review), we analysed and filtered these articles. This was due to a variety of reasons. Initial screening results showed that for a substantial portion of the papers, drones are not the core focus of the paper and are merely an enabling device for the key topic of the paper, such as strategies for disseminating technology products into the construction sector ( Sepasgozar et al., 2018 ). Where drones were more significant, some articles were operationally (e.g. Zhou et al., 2018 ) or engineering focussed (e.g. Chen et al., 2017 ) with no substantial management consideration. Other articles were excluded as they were not relevant, including other uses for the word ‘drone/s’ (e.g. bees or employees) or UAVs (e.g. corporate finance terms). Articles without full text were also eliminated. Article content was further reviewed through Covidence, and the final sample of 133 articles was derived. Results were then analysed with Excel and Bibexcel ( Persson et al., 2009 ).
The identified papers are a population of different paper types. Some represent operational use case studies. Others are engineering focussed but are contemplative of future management endeavours. There are papers written from other (non-drone) perspectives that provide useful insight into drone deployment more generally. And in addition to bibliographic results, we found that use cases of drones to be a worthy area for discussion, as well as the current issues being experienced, which have expanded past historic issues to cover new ones that had not been encountered.
3.1. Bibliographic results
The following are selected results of our review. As illustrated in Fig. 1 , publications related to drone management (including case studies of their use) have been increasing.

Publication year.
Table 1 provides a summary of the published sources relates to our 133 reviewed drone papers. What is evident is that a few sources account for a significant number of publications on drone management in the investigation period. Also evident is a very long tail of single publication sources. What Table 1 also demonstrates is that drone management is still heavily domained in the technology and engineering literature. However, other types of journals are still present to cover specific drone issues (e.g. security and mining reclamation). As the management of drones appears to be very much about micro-level management instead of macro-level management, it is perhaps natural that technology, engineering and related literature are the major publication areas for drones to date.
Listed publication sources.
In terms of author contribution and potential thought leadership, there are 408 unique authors of the analysed papers, representing a wide and varied number of contributors. Of these, one has produced five publications (Hwang, J), one has made four publications (Liu, Y), three have made three publications ( Abaffy, L, Kim, H and Zhang, X ) and 16 have produced two publications. Aside from a number of author pair or group combinations in clearly linked publications from the same research activity, there does not appear to be any significant grouping/clustering of authors as is evident in other systematic reviews of other topics.
Similarly to authors, contributing institutions are wide and varied in range, with those with three or more contributions shown in Table 2 . Again, a long tail of institutional contribution is present, with some institutions having more concentrated contribution. Note that for these institutions, contribution may be planned but is more often unplanned, with different faculties (e.g. engineering and health) making independent, uncoordinated contributions to the literature. Inspection of the contributing departments reveals substantial contribution from engineering and computer science disciplines or institutes of that nature.
Institution contribution.
Country contribution is shown in Table 3 . Top 10 contributing countries and regions. The US, China, Australia and South Korea are significant contributors. Continentally (see Table 3 .), while Asia and North America are significant (to be expected based on the country results), the diverse efforts of European countries are also evident given Europe's substantial contribution.
Top 10 contributing countries and regions.
Our analysis of author keywords (543 keywords) revealed similarly wide and varied results, reflecting the wide range of contexts of research focus, as shown in Table 4 . Making allowance for similar keywords (e.g. drone delivery and drone-delivery), 442 unique keywords were identified. Excluding keywords used only once (386 keywords) and excluding 91 drone referential keywords that are not descriptive of an issue (e.g. drone, drones, UAV, UAVs, unmanned aerial vehicles), these keywords were identified multiple times. Key issues relating to privacy, security, acceptance and management are evident.
Keyword analysis.
We note that papers from earlier in the literature focus on conceptual issues such as privacy and security and have stood as a warning scene for industry to ensure that these concerns are addressed, and that policy makers will be alert to them. However, and concurrent with greater usage and chance to study this usage, papers later in the date range show a clear trend towards the consideration of more commercial aspects of drone adoption including how they are operated and used.
For example, the keyword ‘privacy’ appears in 2016 (four articles), 2017 (two articles) and 2019 (three articles). ‘Regulation’ appears in 2016 (2 articles), 2018 (one article) and 2019 (2 articles). The keyword ‘ethics’ appears in articles in 2016 (one article) and 2019 (two articles). However, ‘drone delivery’ is top of mind in the research community, quickly followed by how drones are going to navigate their way around. Of the drone delivery keywords, 13 of these (more than 80 percent) were published in 2019 indicating its rather recent focus in the literature, which is consistent with the drone use case discussion presented in section 3.3 .
3.2. Present and emerging issues in civilian drone usage results
In this section, we discuss some of the content of these papers. Operating in new spaces, in a third (vertical) dimension and proximity to other users, drone use is expected to have a significant impact on the quality of life, health, social and economic well-being ( Kyrkou et al., 2019 ). However, this potential disruption will, being a technological development ( Kwon et al., 2017 ), create issues and problems that require management to minimise negative impacts (as well as to maximise positive potential). Notably, however, our review indicates that these security, privacy and acceptance concerns, whist significant and relevant, are not as dominant as they have been in previous periods – with the use of drones in various ecosystems providing an opportunity for researchers to examine their introduction and impact on those with whom they interact.
Security management remains a critical issue. Invasion (intentional or not) of sensitive airspaces, like airports ( Boselli et al., 2017 ) and power stations ( Solodov et al., 2018 ) have the potential to and do cause costly disruption (e.g. the near-total closure of Gatwick Airport and disruption to fire and emergency services work in Tasmania in 2018). Safety is a perennial issue though automation may support improved physical safety outcomes ( Torens et al., 2018 ). Privacy issues remain a concern, particularly from drones that can capture imagery, particularly those that are used close to private personal space such as homes and apartments ( Daly, 2017 ; Aydin, 2019 ), or as drones are used in new ways, including research approaches ( Resnik and Elliott, 2018 ). Drone users, particularly recreational ones, do not have an understanding of the privacy requirements that they are subject to (Finn and Wright, 2016). Therefore, a regulatory response is likely to be required. Ethical issues around the use of drones for surveillance purposes are also present ( West and Bowman, 2016 ). Other amenity issues, such as the impact of noise, are also under consideration (e.g. Chang and Li, 2018 ).
The issue of drone acceptance therefore by the public remains an issue, though different parts of the community are more accepting than others ( Anania et al., 2019 ; Sakiyama et al., 2017 ; Rengarajan et al., 2017 ). Some literature (e.g. Boucher, 2016 ; Khan et al., 2019 ) notes that an outcome of this acceptance debate is that drones are being developed to be accepted, taking into account, instead of enforcing, acceptance of drones by the public, showing the role that ‘social license’ ( Gunningham et al., 2004 ) plays in the acceptance debate. Drones require societal trust ( Nelson and Gorichanaz, 2019 ). The demilitarisation of drones has facilitated trust ( Boucher, 2015 ), and positive media attention to non-controversial use cases has been shown to have had a positive impact on acceptance ( Freeman and Freeland, 2016 ).
The first stages of research into specific consumer reaction to drones have begun to bear fruit. Studies have shown how media positioning frames consumer and public responses to drone technology ( Tham et al., 2017 ). Recent work indicates that consumers may respond positively to drones. The technological aspects of drones have been identified to form a relationship with consumers through changing perceptions of risk, functional benefits and relational attributes ( Ramadan et al., 2017 ). Drones provide a psychological benefit to consumers and generate positive intentions to use drones ( Hwang et al., 2019a ). Perceptions of environmental benefits suggest favourable consumer perceptions of drone use ( Hwang et al., 2019b ). A study of motivated consumer innovations suggests that dimensions of functional, hedonic and social motivatedness are key drivers of attitudes towards consumption using drones ( Hwang et al., 2019c ). Innovativeness is noted as an attraction of drone food delivery services for consumers, with younger and female consumers more likely to be attracted by drones ( Hwang et al., 2019d ). Managing perceived risks associated with drone deliveries is a necessary task for foodservice delivery operators ( Hwang et al., 2019e ). In marketing, aerial drone photography is being well received by targets who respond positively to their inclusion in campaigns/advertisements given its cognitive stimulation ( Royo-Vela and Black, 2018 ). Use of drone imagery in this manner is, therefore expanding ( Stankov et al., 2019 ).
Operational management issues have begun to come to the fore with some studies beginning to examine drone maintenance regimes ( Martinetti et al., 2018 ), battery life management/charging and efficient performance characteristics ( Goss et al., 2017 ; Pinto et al., 2019 ). Importantly, with the move towards logistics, other questions are being raised, including how to optimise delivery strategies (e.g. El-Adle et al., 2019 ). Initial analysis indicates combined truck and drone delivery systems are a more efficient method of logistics delivery systems than current approaches ( Ferrandez et al., 2016 ; Chung, 2018 ; Carlsson and Song, 2017 ; Liu et al., 2018 ), Wang et al. (2019) . However serial delivery systems may be more efficient still ( Sharvarani et al., 2019b ) and overall delivery considerations need further analysis, such as preparation time for deliveries which are different between truck vs. drone delivery ( Swanson, 2019 ). Further research in different urban contexts may yield different results (e.g. dense urban areas with higher density and shorter trip distances). Take-off and landing management processes (Gupta et al., 2019; Papa, 2018a , 2018b ; Papa, 2018a ) and ground handling operations ( Meincke et al., 2018 ) are also evident in the literature. Using longer-range drones for civilian purposes is beginning to be discussed (more so of remotely piloted drones instead of automated ones) ( Tatham et al., 2017a ) and the development of specific, commercial drone aviation parks for large drones has been completed ( Abaffy, 2015a , Abaffy, 2015b ).
Initial strategic impacts are receiving literary attention. Drones are driving entrepreneurial activity ( Giones and Brem, 2017 ). Magistretti and Dell’Era (2019) show that operators use four main types of technology development strategies when using drones: focus (adding drones to current operations), depth (expanding current operations more fully), breadth (expanding operations across new offerings) and holistic (developing wholly new operations or approaches). Both Kim et al. (2016) and Meunier and Bellais (2019) note that drone technology leads to spillover effects in other sectors. Hypothecations of societal impacts of future drone issues are also being made ( Rao et al., 2016 ). Consideration of their use in extra-terrestrial environments is also contemplated ( Pergola and Cipolla, 2016 ; Roma, 2017 ).
In the next section, we analyse drone use through several revealed use cases.
3.3. Primary use cases
A valuable part of our review and a key finding is our contribution to understanding how drones are deployed. A large proportion of reviewed articles are (usage) case studies rather than a systematic analysis of an issue. Through these papers, we can highlight that there are presently four primary categories: monitoring/inspection and data acquisition, photography, logistics (including passenger), and recreation. Even accounting for the lag between events and their academic publication, we view that the categories below are reflective of unpublished but current use types.
3.3.1. Monitoring, inspection and data collection
With lower capital costs and greater capabilities, drones can capture existing data in new ways, or capture uncollected data for new analysis. Industrial users are taking advantage of the new opportunities being offered by the technology to do things in new ways, for the same or better outcome.
Network management businesses, e.g. pipelines or energy transmission ( Li et al., 2018 ), road maintenance ( Abaffy, 2015a , Abaffy, 2015b ) and railway operation ( Vong et al., 2018 ) have swapped costly inspection teams with drones. Some inspection drones have real-time analysis capability and quickly report issues and objects for investigation back to the base rather than involving separate analysis stages. These users mainly deploy drones on their specific network geographies (within a set meterage from the network line) however, in positioning to and from their inspection areas, they may traverse open airspace. These network geographies are often in public spaces and given that powerlines (and sometimes rail/road networks) are placed over private properties via easements, management of drone airspace use is important.
Agricultural (and related) industries are inquisitive when it comes to learning more about the land they manage and naturally have looked at drone technology to capture new information ( Weersink et al., 2018 ). Farming has had a recent history of using satellite information to identify crop health issues, using data collected to more efficiently target the application of fertilisers and pesticides. More recently, drones have acquired this information ( Na et al., 2017 ). This has financial implications, but also environmental impacts, as reduced inputs lead to reduced negative impacts for the same output. Similarly, mining operations have used drones to remotely manage and optimise different elements of their production process ( Wendland and Boxnick, 2017 ), including monitoring stockpiles of ore and leeching pads for maintenance issues and analysing blast ore before its processing ( Bamford et al., 2017 ), accessing waterbodies in hazardous/remote locations to facilitate sampling for environmental management ( Banerjee et al., 2018 ; Langhammer et al., 2018 ) and imaging mines for rehabilitation ( Moudry et al., 2019 ). The construction industry uses drones in planning construction sites cheaper than other means (such as helicopters) and at lower risk to staff ( Abaffy and Sawyer, 2016 ; Li and Liu, 2019 ) and hazardous industrial plants use drones to monitor gas production ( Kovacs et al., 2019 ). Importantly for all of these industries, use of drones takes place largely in the airspace above the mining or farming areas and may have minimal impact on other users (notwithstanding that mining and farming areas are generally quite distant from urban areas).
Drones are also used by government and regulatory agencies for surveillance purposes and to monitor compliance. The technology has, for instance, been used in New South Wales to monitor land clearing, both to ensure that permits are complied with and to check if illegal land clearing has taken place. In hard to access areas, air pollution monitoring has been undertaken with drones ( Alvear et al., 2017 ). Drones were used to assess urban damage in the aftermath of floods, hurricanes and even the 2011 Fukushima nuclear reactor disaster ( Hultquist et al., 2017 ). Drones are also used to assess compliance with rehabilitation performance ( Johansen et al., 2019 ), and just this year have seen use in shark monitoring trials at beaches. Emergency services are making more use of drone technology. While some of this use has overlap with logistics (refer below), using drones in search and rescue is a logical move to increase the capability of rescue activities ( Lygouras et al., 2017 ; Kamlofsky et al., 2018 ). Despite the disruptive potential noted above, the monitoring use of drones is useful to fire management ( Athanasis et al., 2019 ) and surf lifesaving ( Lygouras et al., 2017 ) teams. Drones see use in humanitarian relief uses ( Bravo et al., 2019 ; Carli et al., 2019 ). The use of drones for security monitoring is also increasing ( Anania et al., 2018 ; Sakiyama et al., 2017 ). Sensitive but large area enterprises such as forestry or solar cell farms can monitor and inspect remotely with drones ( Xi et al., 2018 ; Saadat and Sharif, 2017 ). These uses are often performed over public and private property and therefore impact a range of other users. However, they are also supported by regulatory requirements and are often undertaken for public purposes and so might be more accepted by the general public.
3.3.2. Photography/image collection
Photography is another special form of data acquisition. While monitoring/inspection uses by industry might also use photographic means to acquire data, this is to convert visual imagery into data to support decision making. However using photos solely for aesthetic value has become an important use of drones in its own right, mainly for personal use (such as the documentation of a person's special event), but also increasingly for commercial use such as sporting events or in marketing campaigns (e.g. Royo-Vela and Black, 2018 ; Stankov et al., 2019 ). Being able to fly has been a dream of (some) humans since time immemorial, and use of drones to capture imagery from birds-eye-views is attracting substantial interest from some quarters.
Use of drones for this purpose is somewhat ad-hoc, and in a large number of cases involves the use of public space as users document their weddings, family events, naturescapes or other events (either themselves or through a commercial operator). However some uses (e.g. farmers taking drone photography of their farm operations) take place entirely over the privately owned property of the drone operator, and some of the aforementioned events happen over publicly but remote land that is not intensively used like urban public land. For sporting events, such as football matches, golf tournaments and car races, use is largely confined to space above the event and closely managed by the event manager to maximise the photographic potential of the event and avoid event disruption.
3.3.3. Recreation
Drones as recreation is a new use, though mimics things like remote-controlled cars which have provided people with entertainment for many decades. The explosion of recreative use shows how popular the phenomenon is, as people take advantage of the third dimension for leisure, which for a long time has been a luxury only enjoyed by those who could fly (in various forms) or partake in risky sports. Drones are being used, e.g. in tourism activities ( Song and Ko, 2017 ), and there are even competitive drone racing tournaments ( Barin et al., 2017 ). Drones are also being used as three-dimensional art installations to generate linked visual structures with no other purpose than entertainment ( China Global Television Network, 2019 ).
The expansion into recreative space is perhaps linked to the increasing acceptance of drone technology by the public as people become more familiar with the technology and begin considering their potential uses for it. Most recreative use is over public spaces such as parks and other such spaces with some of it in non-urban areas being conducted over farmland and naturescapes (either owned or not by the drone operator), though is limited by the low complexity of drones available to use for this purpose.
3.3.4. Logistics
Perhaps most interesting, and most in need of management consideration is using drones for logistics purposes. In its very early days, this use case has perhaps the most significant potential for disruption. Current discussion contemplates that their use will enhance supply chain efficiency and effectiveness ( Druehl et al., 2018 ). Indeed, currently inside warehouses, logistics firms are using drones to manage inventories ( Xu et al., 2018 ). Externally, drones have been used for medical supplies ( Prasad et al., 2018 ; Tatham et al., 2017b ) and organ deliveries ( Balakrishnan et al., 2016 ) in different contexts so far, but with trials for aerial pesticide application ( Zheng et al., 2019 ) and food deliveries currently underway, their use in broader delivery services (e.g. Drone-as-a-Service Asma et al., 2017 , Kang and Jeon, 2016 Shahzaad et al., 2019 ) may lead to substantial shifts in delivery service execution. Prospective applications also include postage/package delivery, with interest being shown by major logistics firms ( Connolly, 2016 ) and the potential for other drone facilitated household services (e.g. dry-cleaning collection/delivery). But we are sure that this is just the tip of the iceberg of opportunity for drones in the logistics space. Indeed, the potential for personal logistics (i.e. humans) is also a goal of some operators ( Lee et al., 2019 ) which would call for significant regulatory oversight (especially safety). Large scale industrial applications are also being investigated ( Damiani et al., 2015 ). The list of potential uses is extensive, and the development of drones in this way is likely to be revolutionary however initial findings are suggesting that they may only be feasible in congested urban areas ( Yoo and Chankov, 2018 ).
The above use classes show the wide spectrum on which drones are used. Clearly, both the literature and observations of trends outside the literature show that these uses will expand. Several questions in many contexts are open for academic exploration at this time, and a few that are of interest to us we will present here (though our specific areas for further research for our paper topic we will discuss at the end of this paper). In the future logistics space, an important question we believe will arise is who owns drone fleets? Will drones be owned by individuals (e.g. mobile phones and private cars) or will they be owned by fleet management/delivery companies and used in an on-demand manner (as common in traditional wet leased air freight operations; e.g. Merkert et al., 2017 )? A drone premium is likely to be chargeable given the convenience and time-saving factors but who will ultimately pay this premium? Will it be added to the delivery cost of goods and services (as in the current postage cost model) or will goods providers decide to use drones for competitive advantage and absorb the cost as part of their cost structure (offsetting delivery cost savings)?
But the key question on our minds for the remainder of this paper is the management of the significant volume of traffic that these movements will create. Increased and increasing use will be more invasive of airspace than current usage, which if not managed appropriately, and if not managed for community standards (within the license to operate), may lead to rejection of the technology and the benefits that they are purported to bring.
4. Managing the drone revolution – current regulatory approaches
We have alluded to the specific issues that drones will present above. Solodov et al. (2018) describe a range of particular drone threats, in the forms of surveillance, smuggling, kinetic (i.e. collision), electronic and distraction. Solutions to these threats include both non-destructive means (such as software intervention, UAV vs UAV, ground-based capture/interference and bird-based methods) and destructive (including electromagnetism, lasers, firearms, and missiles ( Solodov et al., 2018 ). Some airports are working to manage drones in their airspace (e.g. Sichko, 2019 ; Mackie and Lawrence, 2019 ). Many of these methods are reactive or defensive. Instead, more proactive and preventative methods of management would be warranted. Current regulatory approaches are looking to assign responsibility to the operator, which is, in reality, a concern for both consumer and operator ( Liu and Chen, 2019 ).
But further management of lower airspace is a growing area of policy consideration. Across the globe, laws and regulations will need to be created to manage drone impacts. Jurisdictions across the world are examining the drone use and building regulatory environments around them. Chen (2016) identified that the legal and regulatory framework in the US needs reform to facilitate commercial purposes. Integration of drones into the presently regulated airspace (particularly in urban areas and areas of higher sensitivity) is seen by industry to be a likely policy outcome ( Torens et al., 2018 ). Various consistent jurisdictional approaches to this regulation are under development, some of which appear consistent with that envisaged by Clarke (2016) , and the European approach is said to focus on the operation of the flight, rather than the aircraft itself ( Hirling and Holzapfel, 2017 ). This might be described as an approach to softly regulate the industry as it presently stands to allow for safe participation. These regulatory measures significantly increase the requirements of operators to build cultures of safety into their operations. This approach bears a resemblance to other transport sectors (i.e. non-drone aviation, railways and road vehicle operations) which require pilot/driver licensing and firm accreditation. Regulators worldwide are looking to manage the drone itself (weight and size) who flies the drone (both organisationally and personally), how they fly it (height, day/night, speed, visual line of sight), where they fly it (restricted areas, near people, near private space) and other factors (such as the number of simultaneous drones operated) ( Civil Aviation Safety Authority, 2019 ).
The approach by regulators in most jurisdictions so far to grow regulation with the industry, instead of trying to foresee the future and regulate that, is one that may (and are indeed intended to) be designed to support entrepreneurship, innovation and economic growth ( Chisholm, 2018 ).
However, despite the above, it is clear that even in jurisdictions that are well advanced in terms of established drone governance frameworks, more regulation will no doubt be required. The above framework does not cover the full regulatory gap between current drone use and the non-drone airspace. Operators seeking to operate outside of the limits of the above regulation will arise and require further management. Drone automation will mean that pilot intervention to manage the drone in the event of abnormal operations will be impossible. However, there will remain human-controlled drones (including remote ones) such as for recreation or ad-hoc, customised usage. Manned and unmanned drones will have to operate together, and both modes will involve new levels of complexity, particularly as drone numbers increase. Questions will arise about how to manage drones across the industry, where individual adoption by firms will more than likely require harmonised regulation to support supply chain efficiency ( Druehl et al., 2018 ; Foina et al., 2015 ). And different operators will run subnetworks with different path optimisation plans ( Liu et al., 2019 ; Jeong et al., 2019 ). With the substantial increase in flying, in both time and frequency terms in particular, drones are going to have a far more significant impact than the current regulatory impact can manage.
5. Managing the drone revolution – where to from here?
Given the relatively low level of literary consideration, the opportunities for interesting research into the control and macro-management of drones are significant, wide and varied. However, in the context of this paper, the primary area for further research that we see as relevant is how the new drone management ecosystem is to be managed in the macro sense. There are still a raft of challenges to be overcome ( Zhou et al., 2018 ), however with the prospect that drone flight will be as normal as car trips, and that they will play a role in ‘smart’ cities ( Mohamed et al., 2018 ), how to ensure that this new system is not only safe but also productive is essential.
An Internet-of-Drones ( Edwin et al., 2019 ) is a very potential future. Research into the use of flying ad-hoc networks to monitor and manage deviant drone behaviour ( Bahloul et al., 2017 ; Barka et al., 2018 , Karthikeyan and Vadivel, 2019) are in progress, as are geofencing ( Boselli et al., 2017 ) and signal jamming ( Chowdhury et al., 2017 ) that act on the navigation systems within drones to prevent drone incursion into restricted areas. Though to implement some of these preventative technologies, it is, of course, necessary that the relevant drones have navigation technologies installed to be acted upon by the countermeasures, which for a substantial number of retail drones is not the case. For those that do have navigation technology, research efforts are quite extensive into developing algorithms and programs to facilitate orderly inter-drone coordination like network registration processes ( Agron et al., 2019 ) incorporating obstacle detection, ( Zheng et al., 2016 ; Zhu et al., 2017 ; Choutri et al., 2019 ; Abdullah et al., 2019 ), separation processes and collision avoidance ( Tan et al., 2017 ; Nysetvold and Salmon, 2019 ) the impact of weather on drone performance ( Vural et al., 2019 ), completion of common tasks ( Zhuravska et al., 2018 ; Abraham et al., 2019 ; Fesenko et al., 2019 ; Zhu and Wen, 2019 ), inter-drone information security ( Abughalwa and Hasna, 2019 ) and operation in GPS poor areas ( Siva and Poellabauer, 2019 ), though many of these are conceptual and theoretical deployment (e.g. Kim and Kang, 2019 ). Connecting independent networks of drones (that are expected to exist in the future) is yet to appear in the literature, though some elements of this are developing such as using drones as nodes of a multi-drone communication network ( Kuleshov et al., 2018 ; Smith et al., 2018 ; Xiao and Guo, 2019 ). Though note, these methods are only for local drone coordination of the drone and static obstacles (e.g. buildings) or a few connected drones or drone micro-management – systems and processes being developed to impact the drone from the drone's perspective. However, more thinking about drone macro-management and their broader interaction with the environment needs progression, particularly how to manage drones and their collective impact on the remainder of society so that this impact is positive.
Industry is turning towards this question with operators looking to develop more complex management systems. It is likely that (as for aviation generally) each operator will look to develop a customised way of managing drones to suit their operations, such as for search and rescue systems ( Mohsin et al., 2016 ; Mondal et al., 2018 ), complex distribution networks ( Shavarani, 2019 ) or routings with ad hoc targets ( Suteris et al., 2018 ) which will no doubt be complex given the use of the third dimension ( Pandey et al., 2018 ). The concept of an overarching coordinating network is gaining traction in industry and government - NASA is, for instance, looking to integrate UAS into the national airspace system ( Luxhøj et al., 2017 ; Matus and Hedblom, 2018 ; He et al., 2019 ). Conversely, the industry has a different view. Logistics and technology firms such as Amazon and Google are looking at using drones in their parcel delivery systems and firms such as Uber are looking to introduce point to point passenger drone services. Small scale trials are underway in various locations globally, where industry is developing their navigation systems to manage drone delivery. Industry is making the argument that they would be able to self-regulate their drones with these systems, designing them to communicate between drones of different operators and centralised processors. These systems would look to simultaneously program the most efficient routes for deliveries (taking into account, mitigate and avoid collisions and incursions that may cause damage not only to other drones but also to other non-involved parties).
A competing view is considering whether drones should be integrated into the overall air transport management system ( Zhang et al., 2018 ) and managed using many of the same tools and mechanisms deployed by regular aircraft such as identification and collision avoidance systems ( Lin, 2019 ). There is a view that far more oversight of the sector will be required to ensure that safety conditions can be met, and that airborne drones cannot operate separately to large aircraft with which drones will share airspace. A system through which this control can be exercised is being called by airspace management technology developers ‘low altitude airspace management systems (LAAM)’. LAAM as currently envisaged may replicate the control mechanisms used for general and civil aviation flights. Still, importantly each of these different types of flights, drone and non-drone, will know about all other flights in making flight planning and execution decisions. They will be able to communicate with drones and record their position and use within the network. Other features might also be incorporated into LAAM, including the ability to issue instructions to drones (for say crash avoidance) or the ability to enforce geofencing boundaries to prevent drone incursion into specified issues. They may be able to aid in congestion management, to ensure that all drones can achieve their missions within reasonable parameters and may include mechanisms to facilitate flight planning and operations, consistent with current air and rail control management systems. Real-time management of issues would be an essential feature of LAAMS ( Zheng et al., 2016 ). To us, the debate over centralised or distributed airspace management is quite interesting, not only for the impact that it may have on airspace management for drones but also the precedents it may make for other sectors. The impact of such coordination systems on public drone acceptance would also be of interest for researchers to address considering the involvement of government in such regulation may be trusted more than that of the private sector.
From an engineering and technical perspective, the areas of research that are required are almost endless, as new systems are scoped, designed and developed to integrate within the current regulatory environment and aviation control systems. But from our perspective, that of management, there are a few key areas of research that stem from the question of LAAM implementation. Firstly, the need for LAAM and what they are to do needs better articulation from those who would be impacted by it.
As noted, key potential future users of such systems are discussing their need, but further consultation is required to detail precisely what is needed. There are significant policy and commercial/regulatory discussions, but from an academic perspective, this discussion will provide useful insight into a range of issues. An immediate area to investigate are the perspectives of current recreational and commercial users and their reaction to such a possible integration into LAAM and determining what they may like to see for themselves if LAAM is implemented. Current regulations enforce rules on operators which may not be required with a LAAM. Besides, research into prospective users and their preliminary strategies, pricing and other decisions that firm such as logistics ones will make when using the network. Consideration of overall supply chains and the changes that drones may bring in the context of LAAM, helping to not only enable but cheapen the use of drones and impact a range of upstream and downstream elements. Retail precincts may be impacted by yet more package delivery. Warehouses may look quite different from what they do now. Drones may replace hydrocarbon fuel consumption with electric fuel consumption. They may also remove trucks from roads, particularly urban delivery ones. And individual supply chains and travel patterns may change as drones become part of everyday life.
Other transport management specific questions remain to be answered, as highlighted in the literature. Delivery substitution decisions will also be of interest to academia. Cost will be a driver of these changes, but other factors such as service quality and the types of services offered will become a focus area. Optimal drone network designs will be an interesting avenue of discussion (e.g. Pulver and Wei, 2018 ) which will vary depending on the purpose of the drones employed. Optimising how truck and drone fleets interact may be a useful transitive measure to help improve delivery time and efficiency ( Freitas and Penna, 2018 ). Consideration of other delivery mechanisms is also worth researching, such as replacing the truck with a parent drone ( Kim and Awwad, 2017 ). Medical deliveries will need higher prioritisation on the network to ensure their rapid delivery from the donors to the operating theatres where they are needed, or transit points through which they will need to travel ( Balakrishnan et al., 2016 ). So some form of prioritisation matrix will be required.
A key limitation of our approach and any literature review more principally is the lack of full comprehensiveness as literature in the relevant subject area is a proliferating (past the cut-off date and the publication of the paper) and b) not confined to academic outputs (i.e. those indexed in SCOPUS). During our grey literature review, we noticed a recent surge of consultancy reports on drone use cases in the context of urban air mobility (UAM) as a new mode of transportation (e.g. Baur et al., 2018 (Roland Berger); Booz Allen Hamilton, 2018 ; Grandl et al., 2018 (Porsche Consulting); Thomsen, M., 2017 (Airbus)) which suggests that academic papers covering this topic will follow. Indeed, Fu et al. (2019) is a first in a potential series of such papers and has been included in our review.
In summary, our literature review results suggest that security, privacy and acceptance concerns, whist significant and relevant, are not as dominant as they have been in previous periods – with the use of drones in various ecosystems providing an opportunity for researchers to examine their introduction and impact on those with whom they interact. We conclude that further work is needed to understand potential impacts of drone usage (e.g. fatalities due to accidents), subsequent potential risk trade-offs and adjustment/formulation of new regulation ( Hirling and Holzapfel, 2017 ), The safety/cost trade-off will be an important one to contribute to the setting of appropriate safety rules that facilitate the industry without constraining it unnecessarily, including the development of low altitude airspace management systems to support the increased deployment.
Acknowledgements
We acknowledge the contribution and comments received from participants at the 2019 Air Transport Research Society 23rd World Conference. The comments from two anonymous reviewers have helped us to further improve the paper for which we are thankful. We are grateful for the comments and financial support received from Thales Australia and the University of Sydney Business School through an Industry Partnership Grant.
- Abaffy L. Drones used to conduct bridge inspections in Minnesota. Eng. News Rec. 2015; 274 (41) [ Google Scholar ]
- Abaffy L. Construction begins on drone aviation park in North Dakota. Eng. News Rec. 2015; 274 (31) [ Google Scholar ]
- Abaffy L., Sawyer T. How drones are reformatting photography. Eng. News Rec. 2016; 275 (2) [ Google Scholar ]
- Abdullah A.A., Sahib B.B., Abu N.A. 2019 2nd International Conference of Computer and Informatics Engineering (IC2IE) IEEE; 2019. Investigating connection algorithms among drones in the DRANET system; pp. 175–180. [ Google Scholar ]
- Abraham L., Biju S., Biju F., Jose J., Kalantri R., Rajguru S. 2019 International Conference on Innovative Sustainable Computational Technologies (CISCT) IEEE; 2019. Swarm robotics in disaster management; pp. 1–5. [ Google Scholar ]
- Abughalwa M., Hasna M.O. ICTC 2019 - 10th International Conference On ICT Convergence: ICT Convergence Leading The Autonomous Future. 2019. A comparative secrecy study of flying and ground eavesdropping in UAV based communication systems. [ Google Scholar ]
- Agron D.J.S., Ramli M.R., Lee J.M., Kim D.S. 2019 International Conference on Information and Communication Technology Convergence (ICTC) IEEE; 2019. Secure ground control station-based routing protocol for UAV networks; pp. 794–798. [ Google Scholar ]
- Alvear O., Zema N.R., Natalizio E., Calafate C.T. Using UAV-based systems to monitor air pollution in areas with poor accessibility. J. Adv. Transport. 2017; 17 [ Google Scholar ]
- Anania E.C., Rice S., Pierce M., Winter S.R., Capps J., Walters N.W., Milner M.N. Public support for police drone missions depends on political affiliation and neighborhood demographics. Technol. Soc. 2019; 57 :95–103. [ Google Scholar ]
- Asma T., Addouche S.A., Dellagi S., El Mhamedi A. 2017 IEEE International Conference on Service Operations and Logistics, and Informatics (SOLI) IEEE; 2017. Post-production analysis approach for drone delivery fleet; pp. 150–155. [ Google Scholar ]
- Athanasis N., Themistocleous M., Kalabokidis K., Chatzitheodorou C. European, Mediterranean, and Middle Eastern Conference on Information Systems. Springer; Cham: 2018. Big data analysis in UAV surveillance for wildfire prevention and management; pp. 47–58. [ Google Scholar ]
- Atwater D.M. The commercial global drone market: emerging opportunities for social and environmental uses of UAVs. Graziadio Business Report. 2015; 18 (2) [ Google Scholar ]
- Aydin B. Public acceptance of drones: knowledge, attitudes, and practice. Technol. Soc. 2019; 59 :101180. [ Google Scholar ]
- Bahloul N.E.H., Boudjit S., Abdennebi M., Boubiche D.E. 2017 26th International Conference on Computer Communication and Networks (ICCCN) IEEE; 2017. Bio-inspired on demand routing protocol for unmanned aerial vehicles; pp. 1–6. [ Google Scholar ]
- Balakrishnan N., Devaraj K., Rajan S., Seshadri G. Transportation of organs using UAV. Proc. Int. Conf. Ind. Eng. Oper. Management. 2016; 3090 [ Google Scholar ]
- Bamford T., Esmaeili K., Schoellig A.P. A real-time analysis of post-blast rock fragmentation using UAV technology. Int. J. Min. Reclamat. Environ. 2017; 31 (6):439–456. [ Google Scholar ]
- Banerjee B.P., Raval S., Maslin T.J., Timms W. Development of a UAV-mounted system for remotely collecting mine water samples. Int. J. Min. Reclamat. Environ. 2018:1–12. [ Google Scholar ]
- Barin A., Dolgov I., Toups Z.O. Proceedings of the Annual Symposium on Computer-Human Interaction in Play. 2017. Understanding dangerous play: a grounded theory analysis of high-performance drone racing crashes; pp. 485–496. [ Google Scholar ]
- Barka E., Kerrache C.A., Lagraa N., Lakas A., Calafate C.T., Cano J.C. UNION: a trust model distinguishing intentional and Unintentional misbehavior in inter-UAV communication. J. Adv. Transport. 2018 doi: 10.1155/2018/7475357. [ CrossRef ] [ Google Scholar ]
- Bartsch R., Coyne J., Gray K. Routledge; 2016. Drones in Society – Exploring the Strange New World of Unmanned Aircraft. [ Google Scholar ]
- Baur S., Schickram S., Homulenko A., Martinez N., Dyski A. Urban air mobility the rise of a new mode of transportation; Passenger drones ready for take-off, Roland Berger. 2018. https://www.rolandberger.com/fr/Publications/Passenger-drones-ready-for-take-off.html available at.
- Boselli C., Danis J., McQueen S., Breger A., Jiang T., Looze D., Ni D. Geo-fencing to secure airport perimeter against sUAS. International Journal of Intelligent Unmanned Systems. 2017; 5 (4):102–116. [ Google Scholar ]
- Boucher P. Domesticating the drone: the demilitarisation of unmanned aircraft for civil markets. Sci. Eng. Ethics. 2015; 21 (6):1393–1412. [ PMC free article ] [ PubMed ] [ Google Scholar ]
- Boucher P. ‘You wouldn't have your granny using them’: drawing boundaries between acceptable and unacceptable Applications of civil drones. Sci. Eng. Ethics. 2016; 22 (5):1391–1418. [ PMC free article ] [ PubMed ] [ Google Scholar ]
- Bravo R.Z.B., Leiras A., Cyrino Oliveira F.L. The use of UAV s in humanitarian relief: an application of POMDP‐based methodology for finding victims. Prod. Oper. Manag. 2019; 28 (2):421–440. [ Google Scholar ]
- Carli F., Manzotti M.E., Savoini H. ICT for a Better Life and a Better World. Springer; Cham: 2019. New market creation for technological breakthroughs: commercial drones and the disruption of the emergency market; pp. 335–345. [ Google Scholar ]
- Carlsson J.G., Song S. Coordinated logistics with a truck and a drone. Manag. Sci. 2017; 64 (9):4052–4069. [ Google Scholar ]
- Chang S.J., Li K.W. 2018 5th International Conference on Industrial Engineering and Applications (ICIEA) IEEE; 2018. April). Visual and hearing detection capabilities to discriminate whether a UAV invade a campus airspace; pp. 146–149. [ Google Scholar ]
- Chen G.Y. Reforming the current regulatory framework for commercial drones: retaining American businesses' competitive advantage in the global economy. Northwest Journal of International Law and Business. 2016; 37 :513. [ Google Scholar ]
- Chen P., Zeng W., Yu G., Wang Y. Surrogate safety analysis of pedestrian-vehicle conflict at intersections using unmanned aerial vehicle videos. J. Adv. Transport. 2017; 17 [ Google Scholar ]
- China Global Television Network . 2019. 500 Drones Create Stunning Light Show on AI-Driven Future. https://www.youtube.com/watch?v=LvYNHSf7FbI [ Google Scholar ]
- Chisholm J.D. Drones, dangerous animals and peeping Toms: impact of imposed vs. organic regulation on entrepreneurship, innovation and economic growth. Int. J. Enterpren. Small Bus. 2018; 35 (3):428–451. [ Google Scholar ]
- Choutri K., Lagha M., Dala L. Distributed obstacles avoidance for UAVs formation using consensus-based switching topology. International Journal of Computing and Digital Systems. 2019; 8 :167–178. [ Google Scholar ]
- Chowdhury D., Sarkar M., Haider M.Z.A. Cyber-vigilance system for anti-terrorist drives based on an unmanned aerial vehicular networking signal jammer for specific territorial security. Adv. Sci. Technol. Eng. Syst. J. 2017; 3 (3):43–50. [ Google Scholar ]
- Chung J. Heuristic method for collaborative parcel delivery with drone. J. Distrib. Sci. 2018; 16 (2):19–24. [ Google Scholar ]
- Civil Aviation Safety Authority 2019. www.droneflyer.gov.au
- Clarke R. Appropriate regulatory responses to the drone epidemic. Comput. Law Secur. Rep. 2016; 32 (1):152–155. [ Google Scholar ]
- Connolly K.B. Eyes on the skies: the dream of drone delivery starts to take flight. Packag. Digest. 2016; 53 (3):18–25. [ Google Scholar ]
- Daly A. Privacy in automation: an appraisal of the emerging Australian approach. Comput. Law Secur. Rep. 2017; 33 (6):836–846. [ Google Scholar ]
- Damiani L., Revetria R., Giribone P., Guizzi G. Concomitant 14th International Conference on SoMeT. 2015. Simulative comparison between ship and airship for the transport of waste natural gas from oil wells. [ Google Scholar ]
- Druehl C., Carrillo J., Hsuan J. Collaboration and Strategies; 2018. Technological Innovations: Impacts on Supply Chains. Innovation And Supply Chain Management: Relationship ; pp. 259–281. [ Google Scholar ]
- Edwin E.B., RoshniThanka M., Deula S. An internet of drone (IoD) based data analytics in cloud for emergency services. Int. J. Recent Technol. Eng. 2019; 7 (5S2):263–367. [ Google Scholar ]
- El-Adle A.M., Ghoniem A., Haouari M. Parcel delivery by vehicle and drone. Journal of the Operational Research Society. 2019:1–19. [ Google Scholar ]
- Ferrandez S.M., Harbison T., Weber T., Sturges R., Rich R. Optimization of a truck-drone in tandem delivery network using k-means and genetic algorithm. J. Ind. Eng. Manag. 2016; 9 (2):374–388. [ Google Scholar ]
- Fesenko H., Kharchenko V., Zaitseva E. 2019 International Conference on Information and Digital Technologies (IDT) IEEE; 2019. June). Evaluating reliability of a multi-fleet with a reserve drone fleet: an approach and basic model; pp. 128–132. [ Google Scholar ]
- Foina A.G., Krainer C., Sengupta R. Unmanned Aircraft Systems (ICUAS), 2015 International Conference on. 2015. June). An unmanned aerial traffic management solution for cities using an air parcel model; pp. 1295–1300. [ Google Scholar ]
- Freeman P.K., Freeland R.S. Media framing the reception of unmanned aerial vehicles in the United States of America. Technol. Soc. 2016; 44 :23–29. [ Google Scholar ]
- Freitas J.C., Penna P.H.V. A variable neighborhood search for flying sidekick traveling salesman problem. Int. Trans. Oper. Res. 2018:1–24. [ Google Scholar ]
- Fu M., Rothfeld R., Antoniou C. Exploring preferences for transportation modes in an urban air mobility environment: Munich case study. Transportation Research Record. 2019; 2673 (10):427–442. [ Google Scholar ]
- Ginieis M., Sánchez-Rebull M.V., Campa-Planas F. The academic journal literature on air transport: analysis using systematic literature review methodology. J. Air Transport. Manag. 2012; 19 :31–35. [ Google Scholar ]
- Giones F., Brem A. From toys to tools: the co-evolution of technological and entrepreneurial developments in the drone industry. Bus. Horiz. 2017; 60 (6):875–884. [ Google Scholar ]
- Goss K., Musmeci R., Silvestri S. 2017 26th International Conference on Computer Communication and Networks (ICCCN) IEEE; 2017. Realistic models for characterizing the performance of unmanned aerial vehicles; pp. 1–9. [ Google Scholar ]
- Grandl G., Ostgathe M., Cachay J., Doppler S., Salib J., Ross H. Porsche Consulting; 2018. The future of vertical mobility. https://www.porsche-consulting.com/fileadmin/docs/04_Medien/Publikationen/TT1371_The_Future_of_Vertical_Mobility/The_Future_of_Vertical_Mobility_A_Porsche_Consulting_study__C_2018.pdf available at. [ Google Scholar ]
- Gunningham N., Kagan R.A., Thornton D. Social license and environmental protection: why businesses go beyond compliance. Law Soc. Inq. 2004; 29 (2):307–341. [ Google Scholar ]
- Hamilton Booz Allen. Urban air mobility (UAM) market study, McLean. 2018. https://ntrs.nasa.gov/archive/nasa/casi.ntrs.nasa.gov/20190001472.pdf available at.
- He D., Liu H., Chan S., Guizani M. IEEE Network; 2019. How to Govern the Non-cooperative Amateur Drones? [ Google Scholar ]
- Hirling O., Holzapfel F. O.R.C.U.S. risk assessment tool for operations of light UAS above Germany. International. Journal of Intelligent Unmanned Systems. 2017; 5 (1):2–17. [ Google Scholar ]
- Hodgkinson D., Johnston R. Routledge; 2018. Aviation Law and Drones – Unmanned Aircraft and the Future of Aviation. [ Google Scholar ]
- Hultquist C., Sava E., Cervone G., Waters N. Damage assessment of the urban environment during disasters using volunteered geographic information. Big Data for Regional Science. 2017:214–228. [ Google Scholar ]
- Hwang J., Choe J.Y.J. Exploring perceived risk in building successful drone food delivery services. Int. J. Contemp. Hospit. Manag. 2019; 31 (8):3249–3269. doi: 10.1108/IJCHM-07-2018-0558. [ CrossRef ] [ Google Scholar ]
- Hwang J., Kim H. Consequences of a green image of drone food delivery services: the moderating role of gender and age. Bus. Strat. Environ. 2019; 28 :872–884. [ Google Scholar ]
- Hwang J., Cho S.B., Kim W. Consequences of psychological benefits of using eco-friendly services in the context of drone food delivery services. J. Trav. Tourism Market. 2019:1–12. [ Google Scholar ]
- Hwang J., Kim H., Kim W. Investigating motivated consumer innovativeness in the context of drone food delivery services. J. Hospit. Tourism Manag. 2019; 38 :102–110. [ Google Scholar ]
- Hwang J., Lee J.S., Kim H. Perceived innovativeness of drone food delivery services and its impacts on attitude and behavioral intentions: the moderating role of gender and age. Int. J. Hospit. Manag. 2019; 81 :94–103. [ Google Scholar ]
- Jeong H.Y., Song B.D., Lee S. Truck-drone hybrid delivery routing: payload-energy dependency and No-Fly zones. Int. J. Prod. Econ. 2019; 214 :220–233. [ Google Scholar ]
- Johansen K., Erskine P.D., McCabe M.F. Using Unmanned Aerial Vehicles to assess the rehabilitation performance of open cut coal mines. J. Clean. Prod. 2019; 209 :819–833. [ Google Scholar ]
- Kamlofsky J.A., Naidoo N., Bright G., Bergamini M.L., Zelasco J., Ansaldo F., Stopforth R. 2018. Semi-Autonomous Robot Control System with an Improved 3D Vision Scheme for Search and Rescue Missions. A Joint Research Collaboration between South Africa and Argentina. [ Google Scholar ]
- Kang K., Jeon I. Study on utilization drones in domestic logistics service in Korea. J. Distrib. Sci. 2016; 14 :51–57. [ Google Scholar ]
- Khan R., Tausif S., Javed Malik A. Consumer acceptance of delivery drones in urban areas. Int. J. Consum. Stud. 2019; 43 (1):87–101. [ Google Scholar ]
- Kim K., Awwad M. International Annual Conference of the American Society for Engineering Management. 2017. Modeling Effective Deployment of Airborne Fulfilment Centres. [ Google Scholar ]
- Kim K., Kang Y. 2019 International Conference on Information and Communication Technology Convergence (ICTC) IEEE; 2019. Implementation of UAS identification and authentication on oneM2M IoT platform; pp. 948–950. [ Google Scholar ]
- Kim D.H., Lee B.K., Sohn S.Y. Quantifying technology–industry spillover effects based on patent citation network analysis of unmanned aerial vehicle (UAV) Technol. Forecast. Soc. Change. 2016; 105 :140–157. [ Google Scholar ]
- Kovacs M., Călămar A.N., Toth L., Simion S., Simion A., Kovacs I. Opportunity of using drones equipped with sensors for measurement of combustion gases. Calitatea. 2019; 20 (S1):207. [ Google Scholar ]
- Kuleshov S.V., Zaytseva A.A., Aksenov A.Y. The conceptual view of unmanned aerial vehicle implementation as a mobile communication node of active data transmission network. International Journal of Intelligent Unmanned Systems. 2018; 6 (4):174–183. [ Google Scholar ]
- Kwon H., Kim J., Park Y. Applying LSA text mining technique in envisioning social impacts of emerging technologies: the case of drone technology. Technovation. 2017; 60 :15–28. [ Google Scholar ]
- Kyrkou C., Timotheou S., Kolios P., Theocharides T., Panayiotou C. Drones: augmenting our quality of life. IEEE Potentials. 2019; 38 (1):30–36. [ Google Scholar ]
- Langhammer J., Janský B., Kocum J., Minařík R. 3-D reconstruction of an abandoned montane reservoir using UAV photogrammetry, aerial LiDAR and field survey. Appl. Geogr. 2018; 98 :9–21. [ Google Scholar ]
- Lee J.K., Kim S.H., Sim G.R. Mode choice behavior analysis of air transport on the introduction of remotely piloted passenger aircraft. J. Air Transport. Manag. 2019; 76 :48–55. [ Google Scholar ]
- Li Y., Liu C. Applications of multirotor drone technologies in construction management. International Journal of Construction Management. 2019; 19 (5):401–412. [ Google Scholar ]
- Li Y., Sun Z., Qin R. Proceedings of the International Annual Conference of the American Society for Engineering Management. American Society for Engineering Management (ASEM); 2018. Routing algorithm and cost analysis for using hydrogen fuel cell powered unmanned aerial vehicle in high voltage transmission line inspection; pp. 1–11. [ Google Scholar ]
- Lin L. The design of UAV collision avoidance system based on ADS-B IN. Paper Asia. 2019; 2 :141–144. [ Google Scholar ]
- Liu C.C., Chen J.J. Analysis of the weights of service quality indicators for drone filming and photography by the fuzzy analytic network process. Appl. Sci. 2019; 9 (6):1236. [ Google Scholar ]
- Liu J., Guan Z., Xie X. 2018 8th International Conference on Logistics, Informatics and Service Sciences (LISS) IEEE; 2018. Truck and Drone in Tandem Route Scheduling under Sparse Demand Distribution; pp. 1–6. [ Google Scholar ]
- Liu Y., Liu Z., Shi J., Wu G., Chen C. Journal of Advanced Transportation; 2019. Optimization of base location and patrol routes for unmanned aerial vehicles in border intelligence, surveillance, and reconnaissance. Article ID. [ Google Scholar ]
- Luppicini R., So A. A technoethical review of commercial drone use in the context of governance, ethics, and privacy. Technol. Soc. 2016; 46 :109–119. [ Google Scholar ]
- Luxhøj J.T., Joyce W., Luxhøj C. A ConOps derived UAS safety risk model. J. Risk Res. 2017:1–23. [ Google Scholar ]
- Lygouras E., Dokas I.M., Andritsos K., Tarchanidis K., Gasteratos A. International Conference on Information Systems for Crisis Response and Management in Mediterranean Countries. Springer; Cham: 2017. Identifying hazardous emerging behaviors in search and rescue missions with drones: a proposed methodology; pp. 70–76. [ Google Scholar ]
- Lygouras E., Gasteratos A., Tarchanidis K. International Conference on Information Systems for Crisis Response and Management in Mediterranean Countries. Springer; Cham: 2017. ROLFER: an innovative proactive platform to reserve swimmer's safety; pp. 57–69. [ Google Scholar ]
- Mackie T., Lawrence A. Integrating unmanned aircraft systems into airport operations: from buy-in to public safety. J. Airpt. Manag. 2019; 13 (4):380–390. [ Google Scholar ]
- Magistretti S., Dell'Era C. Unveiling opportunities afforded by emerging technologies: evidences from the drone industry. Technol. Anal. Strat. Manag. 2019; 31 (5):606–623. [ Google Scholar ]
- Martinetti A., Schakel E.J., van Dongen L.A. Flying asset: framework for developing scalable maintenance program for Unmanned Aircraft Systems (UAS) J. Qual. Mainten. Eng. 2018; 24 (2):152–169. [ Google Scholar ]
- Matus F., Hedblom B. 2018 Integrated Communications, Navigation, Surveillance Conference (ICNS) IEEE; 2018. Addressing the Low-Altitude Airspace Integration Challenge—USS or UTM Core? 2F1-1. [ Google Scholar ]
- Meincke P., Asmer L., Geike L., Wiarda H. 2018 8th International Conference on Logistics, Informatics and Service Sciences (LISS) IEEE; 2018. Concepts for cargo ground handling of unmanned cargo aircrafts and their influence on the supply chain; pp. 1–10. [ Google Scholar ]
- Merkert R., Van de Voorde E., de Wit J. Making or breaking - key success factors in the air cargo market. J. Air Transport. Manag. 2017; 61 :1–5. [ Google Scholar ]
- Meunier F.X., Bellais R. Technical systems and cross-sector knowledge diffusion: an illustration with drones. Technol. Anal. Strat. Manag. 2019; 31 (4):433–446. [ Google Scholar ]
- Mohamed N., Al-Jaroodi J., Jawhar I., Idries A., Mohammed F. Unmanned aerial vehicles applications in future smart cities. Technol. Forecast. Soc. Change. 2018 (in press) [ Google Scholar ]
- Mohsin B., Steinhäusler F., Madl P., Kiefel M. An innovative system to enhance situational awareness in disaster response. J. Homel. Secur. Emerg. Manag. 2016; 13 (3):301–327. [ Google Scholar ]
- Mondal T., Bhattacharya I., Pramanik P., Boral N., Roy J., Saha S., Saha S. A multi-criteria evaluation approach in navigation technique for micro-jet for damage & need assessment in disaster response scenarios. Knowl. Base Syst. 2018; 162 :220–237. [ Google Scholar ]
- Moudrý V., Gdulová K., Fogl M., Klápště P., Urban R., Komárek J., Moudrá L., Štroner M., Barták V., Solský M. Comparison of leaf-off and leaf-on combined UAV imagery and airborne LiDAR for assessment of a post-mining site terrain and vegetation structure: prospects for monitoring hazards and restoration success. Appl. Geogr. 2019; 104 :32–41. [ Google Scholar ]
- Na S., Park C., So K., Park J., Lee K. 2017 6th International Conference on Agro-Geoinformatics. IEEE; 2017. Mapping the spatial distribution of barley growth based on unmanned aerial vehicle; pp. 1–5. [ Google Scholar ]
- Nelson J., Gorichanaz T. Technology in Society; 2019. Trust as an Ethical Value in Emerging Technology Governance: the Case of Drone Regulation. (in press) [ Google Scholar ]
- Nysetvold T.B., Salmon J.L. Deconfliction in high-density unmanned aerial vehicle systems. J. Air Transport. 2019; 27 (2):61–69. [ Google Scholar ]
- Pandey P., Shukla A., Tiwari R. Three-dimensional path planning for unmanned aerial vehicles using glowworm swarm optimization algorithm. International Journal of System Assurance Engineering and Management. 2018; 9 (4):836–852. [ Google Scholar ]
- Papa U. Sonar sensor model for safe landing and obstacle detection. Stud. Syst. Decis. Control. 2018; 136 :13–28. [ Google Scholar ]
- Papa U. Optical sensor for UAS aided landing. Stud. Syst. Decis. Control. 2018; 136 :63–79. [ Google Scholar ]
- Pathak P., Damle M., Pal P.R., Yadav V. Humanitarian impact of drones in healthcare and disaster management. Int. J. Recent Technol. Eng. 2019; 7 (5):201–205. [ Google Scholar ]
- Perera H.N., Hurley J., Fahimnia B., Reisi M. The human factor in supply chain forecasting: a systematic review. Eur. J. Oper. Res. 2018; 274 (2):574–600. [ Google Scholar ]
- Pergola P., Cipolla V. Mission architecture for Mars exploration based on small satellites and planetary drones. International Journal of Intelligent Unmanned Systems. 2016; 4 (3):142–162. [ Google Scholar ]
- Persson O., Danell R., Wiborg Schneider J. How to use Bibexcel for various types of bibliometric analysis. In: Åström F., Danell R., Larsen B., Schneider J., editors. Celebrating Scholarly Communication Studies: A Festschrift for Olle Persson at His 60th Birthday. International Society for Scientometrics and Informetrics; Leuven, Belgium: 2009. pp. 9–24. [ Google Scholar ]
- Pinto R., Zambetti M., Lagorio A., Pirola F. A network design model for a meal delivery service using drones. International Journal of Logistics Research and Applications. 2019:1–21. [ Google Scholar ]
- Prasad G., Abishek P., Karthick R. Influence of unmanned aerial vehicle in medical product transport. International Journal of Intelligent Unmanned Systems. 2018; 7 (2):88–94. [ Google Scholar ]
- Pulver A., Wei R. Optimizing the spatial location of medical drones. Appl. Geogr. 2018; 90 :9–16. [ Google Scholar ]
- Ramadan Z.B., Farah M.F., Mrad M. An adapted TPB approach to consumers' acceptance of service-delivery drones. Technol. Anal. Strat. Manag. 2017; 29 (7):817–828. [ Google Scholar ]
- Rao B., Gopi A.G., Maione R. The societal impact of commercial drones. Technol. Soc. 2016; 45 :83–90. [ Google Scholar ]
- Rengarajan V., Alamelu R., Amudha R., Cresenta Shakila Motha L., Sivasundaram Anushan S.C. Youth awareness on drones - a new paradigm in freight logistics. Int. J. Appl. Bus. Econ. Res. 2017; 15 (13):353–361. [ Google Scholar ]
- Resnik D.B., Elliott K.C. Using drones to study human beings: ethical and regulatory issues. Sci. Eng. Ethics. 2018:1–12. [ PMC free article ] [ PubMed ] [ Google Scholar ]
- Roma A. Drones and popularisation of space. Space Pol. 2017; 41 :65–67. [ Google Scholar ]
- Royo-Vela M., Black M. Drone images versus terrain images in advertisements: images' verticality effects and the mediating role of mental simulation on attitude towards the advertisement. J. Market. Commun. 2018:1–19. [ Google Scholar ]
- Saadat N., Sharif M.M.M. 2017 International Conference on Engineering Technology and Technopreneurship (ICE2T) IEEE; 2017. Application framework for forest surveillance and data acquisition using unmanned aerial vehicle system; pp. 1–6. [ Google Scholar ]
- Sakiyama M., Miethe T.D., Lieberman J.D., Heen M.S., Tuttle O. Big hover or big brother? Public attitudes about drone usage in domestic policing activities. Secur. J. 2017; 30 (4):1027–1044. [ Google Scholar ]
- Sepasgozar S.M., Davis S.R., Loosemore M. Dissemination practices of construction sites' technology vendors in technology exhibitions. J. Manag. Eng. 2018; 34 (6) [ Google Scholar ]
- Shahzaad B., Bouguettaya A., Mistry S., Neiat A.G. 2019 IEEE International Conference on Web Services (ICWS) IEEE; 2019. Composing Drone-As-A-Service (DAAS) for Delivery; pp. 28–32. [ Google Scholar ]
- Shavarani S.M. Multi-level facility location-allocation problem for post-disaster humanitarian relief distribution: a case study. J. Humanit. Logist. Supply Chain Manag. 2019; 9 (1):70–81. [ Google Scholar ]
- Shavarani S.M., Golabi M., Izbirak G. A capacitated biobjective location problem with uniformly distributed demands in the UAV‐supported delivery operation. Int. Trans. Oper. Res. 2019 doi: 10.1111/itor.12735. [ CrossRef ] [ Google Scholar ]
- Sichko P. Integrating unmanned aerial system operations into the Dallas/Fort Worth airport environment. J. Airpt. Manag. 2019; 13 (3):206–214. [ Google Scholar ]
- Siva J., Poellabauer C. Mission-oriented Sensor Networks and Systems: Art and Science. 2019. Robot and drone localization in GPS-denied areas; pp. 597–631. [ Google Scholar ]
- Smith P., Hunjet R., Aleti A., Barca J.C. Data transfer via UAV swarm behaviours: rule generation, evolution and learning. Australian Journal of Telecommunications and the Digital Economy. 2018; 6 (2):35. [ Google Scholar ]
- Solodov A., Williams A., Al Hanaei S., Goddard B. Analyzing the threat of unmanned aerial vehicles (UAV) to nuclear facilities. Secur. J. 2018; 31 (1):305–324. [ Google Scholar ]
- Song B.D., Ko Y.D. Quantitative approaches for economic use of emerging technology in the tourism industry: unmanned aerial vehicle systems. Asia Pac. J. Tourism Res. 2017; 22 (12):1207–1220. [ Google Scholar ]
- Spasojevic B., Lohmann G., Scott N. Air transport and tourism–a systematic literature review (2000–2014) Curr. Issues Tourism. 2018; 21 (9):975–997. [ Google Scholar ]
- Stankov U., Kennell J., Morrison A.M., Vujičić M.D. The view from above: the relevance of shared aerial drone videos for destination marketing. J. Trav. Tourism Market. 2019:1–15. [ Google Scholar ]
- Suteris M.S., Rahman F.A., Ismail A. Route schedule optimization method of unmanned aerial vehicle implementation for maritime surveillance in monitoring trawler activities in Kuala Kedah, Malaysia. Int. J. Supply Chain Manag. 2018; 7 (5):245–249. [ Google Scholar ]
- Swanson D. A simulation-based process model for managing drone deployment to minimize total delivery time. IEEE Eng. Manag. Rev. 2019; 47 (3):154–167. [ Google Scholar ]
- Tan D.Y., Chi W., Bin Mohamed Salleh M.F., Low K.H. vol. 5. IOS Press; 2017. Study on impact of separation distance to traffic management for small UAS operations in urban environment; p. 39. (Transdisciplinary Engineering: A Paradigm Shift: Proceedings of the 24th ISPE Inc. International Conference on Transdisciplinary Engineering). July 10-14. [ Google Scholar ]
- Tatham P., Ball C., Wu Y., Diplas P. Long-endurance remotely piloted aircraft systems (LE-RPAS) support for humanitarian logistic operations: the current position and the proposed way ahead. J. Humanit. Logist. Supply Chain Manag. 2017; 7 (1):2–25. [ Google Scholar ]
- Tatham P., Stadler F., Murray A., Shaban R.Z. Flying maggots: a smart logistic solution to an enduring medical challenge. J. Humanit. Logist. Supply Chain Manag. 2017; 7 (2):172–193. [ Google Scholar ]
- Tham A., Ogulin R., Selen W. Taming the wicked problem of a drone ecosystem: the role of the media. Emergence. 2017; 19 (3–4) [ Google Scholar ]
- Thomsen M. Putting urban transport into the third dimension, together, Airbus. 2017. https://www.europeanfiles.eu/digital/putting-urban-transport-third-dimension-together available at.
- Torens C., Dauer J.C., Adolf F. Advances in Aeronautical Informatics. Springer; Cham: 2018. Towards autonomy and safety for unmanned aircraft systems; pp. 105–120. [ Google Scholar ]
- Vong C.H., Ravitharan R., Reichl P., Chevin J., Chung H. IEEE International Conference on Intelligent Rail Transportation (ICIRT) 2017. Ultrasound International; 2018. Small Scale Unmanned Aerial System (UAS) for Railway Culvert and Tunnel Inspection; pp. 1024–1032. [ Google Scholar ]
- Vural D., Dell R.F., Kose E. Locating unmanned aircraft systems for multiple missions under different weather conditions. Operational Research. 2019:1–20. [ Google Scholar ]
- Wang K., Yuan B., Zhao M., Lu Y. Cooperative route planning for the drone and truck in delivery services: a bi-objective optimisation approach. J. Oper. Res. Soc. 2019:1–18. [ Google Scholar ]
- Weersink A., Fraser E., Pannell D., Duncan E., Rotz S. Opportunities and challenges for Big Data in agricultural and environmental analysis. Annual Review of Resource Economics. 2018; 10 :19–37. [ Google Scholar ]
- Wendland E., Boxnick H. Drones in mining: from toy to process optimization. Min. Surf. Min. World Min. 2017; 69 (4):240–241. [ Google Scholar ]
- West J.P., Bowman J.S. The domestic use of drones: an ethical analysis of surveillance issues. Publ. Adm. Rev. 2016; 76 (4):649–659. [ Google Scholar ]
- Xi Z., Lou Z., Sun Y., Li X., Yang Q., Yan W. 2018 17th International Symposium on Distributed Computing and Applications for Business Engineering and Science (DCABES) IEEE; 2018. A vision-based inspection strategy for large-scale photovoltaic farms using an autonomous UAV; pp. 200–203. [ Google Scholar ]
- Xiao Z.M., Guo Z. Research on key technologies of multi-UAV cooperative monitoring for machines faults in paper mills. (2019). Paper Asia. 2019; 2 :152–156. [ Google Scholar ]
- Xu L., Kamat V.R., Menassa C.C. Automatic extraction of 1D barcodes from video scans for drone-assisted inventory management in warehousing applications. International Journal of Logistics Research and Applications. 2018; 21 (3):243–258. [ Google Scholar ]
- Yoo H.D., Chankov S.M. 2018 IEEE International Conference on Industrial Engineering and Engineering Management (IEEM) IEEE; 2018. Drone-delivery Using Autonomous Mobility: an Innovative Approach to Future Last-Mile Delivery Problems; pp. 1216–1220. [ Google Scholar ]
- Zhang X., Liu Y., Zhang Y., Guan X., Delahaye D., Tang L. Safety assessment and risk estimation for unmanned aerial vehicles operating in national airspace system. J. Adv. Transport. 2018 [ Google Scholar ]
- Zheng Z., Liu Y., Zhang X. The more obstacle information sharing, the more effective real-time path planning? Knowl. Base Syst. 2016; 114 :36–46. [ Google Scholar ]
- Zheng S., Wang Z., Wachenheim C.J. Technology adoption among farmers in Jilin Province, China: the case of aerial pesticide application. China Agricultural Economic Review. 2019; 11 (1):206–216. [ Google Scholar ]
- Zhou Z., Irizarry J., Lu Y. A multidimensional framework for unmanned aerial system applications in construction project management. J. Manag. Eng. 2018; 34 (3) [ Google Scholar ]
- Zhu M., Wen Y.Q. Design and analysis of collaborative unmanned surface-aerial vehicle cruise systems. Journal of Advanced Transportation 2019. 2019 [ Google Scholar ]
- Zhu C.C., Liang X.L., Sun Q. Conference Proceedings of the International Symposium on Project Management. 2017. Research on obstacle avoidance of UAV swarm based on cognitive model; pp. 152–158. [ Google Scholar ]
- Zhuravska I., Kulakovska I., Musiyenko M. Development of a method for determining the area of operation of unmanned vehicles formation by using the graph theory. E. Eur. J. Enterprise Technol. 2018; 2 (3–92):4–12. [ Google Scholar ]
- Reference Manager
- Simple TEXT file
People also looked at
Original research article, modeling relation among implementing ai-based drones and sustainable construction project success.

- 1 Department of Civil and Environmental Engineering, Universiti Teknologi PETRONAS, Tronoh, Perak, Malaysia
- 2 Civil Engineering Department, University of Garmian, Kalar, Kurdistan Region, Iraq
- 3 Department of Civil Engineering, Harran University, Şanlıurfa, Türkiye
- 4 Department of Civil and Environmental Engineering, College of Engineering, Majmaah University, Al-Majma’ah, Saudi Arabia
- 5 Department of Civil Engineering, College of Engineering, Taif University, Taif, Saudi Arabia
- 6 Architectural Engineering Department, College of Engineering, Najran University, Najran, Saudi Arabia
- 7 Architectural Engineering Department, College of Engineering, Taibah University, Al-Madinah al-Munawwarah, Saudi Arabia
- 8 Department of Civil Engineering, College of Engineering, Najran University, Najran, Saudi Arabia
- 9 Department of Technology, Faculty of Engineering and Technology, University of Chenab, Gujrat, Pakistan
- 10 Department of Civil Engineering, College of Engineering, Prince Sattam Bin Abdulaziz University, Alkharj, Saudi Arabia
- 11 Department of Architecture and Planning, College of Engineering, King Khalid University, Abha, Saudi Arabia
Project failure is a persistent challenge in the construction industry, rendering it one of the most demanding sectors. Many obstacles, including safety concerns, quality management issues, environmental preservation challenges, economic sustainability, privacy constraints, and legal regulations, weigh heavily on construction projects. However, a beacon of hope emerges in AI-powered drones capable of surmounting these challenges and paving the path to resounding project success. This study employed diverse methodologies, engaging subject-matter experts through interviews and conducting pilot and primary surveys. Our analytical arsenal featured Exploratory Factor Analysis (EFA) for the pilot survey and Structural Equation Modelling (SEM) for the primary survey. Our research revolves around a singular mission: elevating building project success by dismantling the barriers that have impeded the widespread adoption of AI-driven drones in construction. The study’s verdict is clear: privacy and legal constraints, coupled with economic and sustainability challenges, alongside human resource management dilemmas, constitute the formidable triumvirate obstructing the ubiquitous embrace of drones in construction. Yet, the impact of breaching these barriers reverberates far beyond overcoming these hurdles. It cascades into public health and safety, environmental conservation, quality management, and economic sustainability, culminating in an amalgam of enhanced Building Project Success. The implications of our findings are profound for the construction industry. They beckon the sector to confront and surmount the legal and regulatory barriers to adopting AI-based drones. A clarion call to invest in human resources to empower technology integration resounds. And, perhaps most importantly, it beckons the industry to embrace the profound economic and sustainability advantages of embracing these cutting-edge technologies. Furthermore, our study underscores that adopting AI-powered drones in construction is not merely about project success; it catalyzes fostering public health, safeguarding the environment, ensuring top-tier quality management, and fortifying economic sustainability. These interwoven facets illuminate the broader canvas of drone technology’s transformative role in construction.
1 Introduction
The drone, a crewless aerial vehicle, has attracted extensive interest in the construction sector owing to its potential to enhance safety, cut costs, and boost efficiency. According to a survey by market analysts, the worldwide drone services market size in construction was estimated at USD 4.4 billion in 2019 and is predicted to rise at a CAGR of 20.6% to reach USD 11.2 billion by 2024 ( Alsamarraie et al., 2022 ). The report finds many significant drivers pushing the use of drones in construction, including their capacity to conduct various jobs such as surveying, mapping, inspections, and monitoring building progress ( Yang et al., 2021 ). Drones are especially valuable for surveying big or complicated building sites, where they may swiftly and correctly record data that would otherwise be difficult or time-consuming to acquire. In addition, drones outfitted with thermal imaging cameras may be used to discover possible risks, such as heat leaks or electrical issues, that would not be apparent to the human eye. In light of global sustainability concerns, there is an increasing need to transform building practices to conform to ecological and social goals. In this particular setting, incorporating drones that use artificial intelligence (AI) into building procedures presents a potentially revolutionary resolution. The drones have been specifically engineered to carry out various duties independently, such as gathering data, conducting analysis, and making decisions using artificial intelligence algorithms and sensors ( Feng et al., 2013 ; Goessens et al., 2018a ).
The concept of sustainable construction entails a comprehensive perspective on the construction and development of buildings and infrastructure, considering various environmental, social, and economic considerations. The construction industry significantly contributes to global resource consumption and environmental degradation, responsible for around 36% of worldwide energy consumption and 39% of carbon emissions ( Kubo and Okoso, 2019 ; Lahmeri et al., 2021 ; Kim et al., 2022 ). Using drones with artificial intelligence presents a promising opportunity to tackle these difficulties effectively ( Ganesan et al., 2020 ; Gibbin et al., 2023 ). The use of AI in uncrewed aerial vehicles holds promise for substantial improvements in the efficiency of building projects. Modern techniques enable them to efficiently carry out site surveys, monitor project progress, and conduct inspections at a significantly accelerated pace compared to conventional ways.
Even with these advantages, the deployment of drones in construction is challenging, including regulatory and legal limits, lack of knowledge and skills among construction professionals, and budgetary constraints ( Li and Liu, 2019 ). In the United States, for instance, the Federal Aviation Agency (FAA) has established restrictions for the use of drones, including commercial usage guidelines and registration, pilot certification, and flight operations criteria. In addition, many experts in the construction industry may need more expertise and skills to operate drones and evaluate the data they gather. Financial limitations may also prevent construction companies from investing in drone technology ( Pawar, 2020 ).
These drones can offer many benefits, including enhancing safety and quality management, bolstering environmental protection, and improving economic sustainability. Drones equipped with AI capabilities have the potential to redefine the landscape of construction project management ( Yang et al., 2021 ; Alsamarraie et al., 2022 ). They provide real-time data, conduct aerial surveys, and offer precise monitoring capabilities, enabling construction professionals to make informed decisions and optimize project outcomes ( Loveless, 2018 ; Li and Liu, 2019 ). However, this promising trajectory has its challenges. The construction industry grapples with multifarious obstacles, including technical and functional constraints, privacy and legal restrictions, economic sustainability considerations, and organizational resistance to change ( Ateya et al., 2022 ; Ichimura et al., 2022 ). These challenges hinder the widespread adoption of drones and impact critical facets like public health and safety, environmental preservation, quality management, and economic sustainability.
Despite the increased interest in using drones in the construction sector, there still needs to be more knowledge on overcoming the obstacles to their acceptance and ensuring their success in the business. While there have been some studies on the advantages and disadvantages of employing drones in construction, only some have explored the correlation between the obstacles to their adoption and the success of the technology in the sector ( Loveless, 2018 ). This information gap is a substantial challenge for construction industry professionals, policymakers, and academics interested in adopting innovative technology ( Ateya et al., 2022 ; Ichimura et al., 2022 ). Consequently, this expeditious approach results in diminished project durations and cost reductions. The potential advantages of using AI-based drones in sustainable building are readily apparent ( Greene and Myers, 2013 ; Gupta et al., 2021 ). However, there needs to be a more in-depth investigation into the extent to which these drones affect the overall success of construction projects. Previous research often focuses on specific elements of either AI implementation in the building industry or the adoption of sustainable construction practices. A significant research gap needs to be addressed to establish a connection between these two areas and explore the potential synergistic effects that may arise from their integration.
In addition, extant research tends to emphasize individual obstacles or success factors over their interrelation. A more thorough knowledge of the link between barriers and success factors is required to find the most effective techniques for eliminating obstacles and guaranteeing the success of drones in the industry ( Alsamarraie et al., 2022 ). This is especially crucial since the use of drones in buildings continues to increase, and construction companies are under growing pressure to embrace more sustainable and efficient techniques.
The present article seeks to fill this knowledge gap by realizing the full potential of drones in the construction industry. It is essential to comprehend the connection between overcoming these obstacles and the success of drones in business ( Wazid et al., 2020 ). This study intended to provide an intelligent Partial Least Square (PLS) regression model to investigate the link between drone adoption hurdles and their industrial success. The approach is built on two orders, with the first level consisting of adoption obstacles and the second order consisting of success characteristics, including quality, safety, and environmental effects. The data-driven approach enables us to identify the most significant hurdles to the adoption of drones in construction and the elements that contribute to their success, offering a more excellent knowledge of how the barriers affect the success of the technology in building ( Lawani et al., 2022 ).
While facing substantial challenges, the construction industry has shown a growing interest in adopting AI-powered drones. However, the comprehensive examination of the interconnected barriers hindering their widespread integration remains notably limited in the existing literature. Moreover, the extent to which addressing these barriers can significantly impact project success and critical areas like public health and safety, environmental conservation, quality management, and economic sustainability requires deeper investigation. This research seeks to bridge this notable gap by conducting an in-depth analysis that identifies these challenges and elucidates their multifaceted impact on the construction landscape. In doing so, we aim to contribute to a more comprehensive understanding of the potential and challenges associated with AI-based drones in construction while offering practical insights for industry stakeholders and policymakers.
This is, to the best of our knowledge, the first study to develop an intelligent structural equation model to explore the relationship between the barriers to the adoption of drones in construction and their success in the industry, utilizing a comprehensive set of success factors that includes quality, safety, and environmental factors. The model enables us to identify the most critical hurdles to adopting drones in the construction industry and the characteristics contributing to their success. It offers valuable information on overcoming these barriers and guarantees the effective integration of drones into the sector. This unique method for examining the link between obstacles to adoption and the success characteristics of drones in the building will interest construction professionals, policymakers, and scholars. The intended audience for this article consists of building industry specialists with extensive expertise. This study’s results may be of considerable use to construction industry experts, legislators, and scholars interested in adopting developing technology. This research offers a significant road map for enhancing the acceptance and integration of drone technology in construction by identifying the hurdles to drone adoption and the elements that contribute to their success. Using AI-based drones in building projects may result in significant cost reductions, hence offering considerable benefits at a time characterised by limited financial resources and economic instability. The results of this study will provide valuable insights to construction firms and policymakers on the potential of AI-enabled drones, hence facilitating their integration and utilisation within the sector. The research aims to provide a strategic framework for harmonising building practises with sustainability objectives, fostering sustainable development and conscientious resource stewardship.
2 Related works
Many studies have examined the challenges of using drones in construction and the crucial success elements that may assist in overcoming these barriers ( Golpîra, 2021 ; Kim et al., 2022 ). For instance, it was discovered that a lack of knowledge, legal constraints, and data processing difficulties were the most significant obstacles to drone adoption in the construction business ( Yi and Sutrisna, 2021 ). One study observed comparable constraints, such as privacy and security concerns, lack of technical competence, and investment return uncertainty ( Lee and Kwon, 2020 ; Woo et al., 2021 ). Cost and a lack of industry standards were also significant hurdles to adoption by one examination. One study identified regulatory restrictions, restricted drone capabilities, and expensive equipment and maintenance costs as the primary obstacles to the deployment of drones in the construction industry ( Feder, 2020 ; To et al., 2021 ). It was revealed that inadequate awareness and understanding of drone technology, privacy and data security concerns, and drone durability were significant obstacles. It is also identified that the availability of experienced personnel, the price of drone technology, and the aversion to change were significant hurdles to adoption. It is realized that in India, a need for more awareness and expertise regarding drone technology, high prices, and regulatory difficulties were significant obstacles ( Amicone et al., 2021 ; Mahajan, 2021 ). Sawhney et al. (2020) did a thorough literature analysis. They found that the primary hurdles were the absence of clear laws, high expenses, and a lack of knowledge and awareness ( Sawhney et al., 2020 ).
The use of drones within the construction sector has seen substantial expansion in recent years. It is important to comprehend the many aspects that contribute to this acceptance, with a particular focus on the level of maturity in terms of age ( Lee and Kwon, 2020 ; Wazid et al., 2020 ). The construction industry, often seen as conservative, has experienced a growing acceptance of drone technology due to its capacity to potentially transform several facets of project management ( Charlesraj and Rakshith, 2020 ; Kim et al., 2022 ). Nevertheless, the pace of adoption often exhibits variability across people and organizations, with age potentially serving as a relevant element within this context. The available research suggests that individuals belonging to younger professional cohorts, especially those raised in digital advancements, have a greater inclination towards embracing drone technology within the construction industry ( Charlesraj and Rakshith, 2020 ; Feder, 2020 ). Frequently, individuals of this demographic have a higher level of proficiency in assimilating new technical instruments and are more inclined to see drones as beneficial resources for their endeavours. On the other hand, it is worth noting that older professionals may want further training and assistance to effectively adopt and integrate these advancements ( Li and Liu, 2019 ; Alsamarraie et al., 2022 ). Moreover, the extent of expertise within the construction sector significantly influences the impression of crewless aerial vehicles. Experienced individuals who have seen the sector’s progression may possess a heightened understanding of the capabilities of drones, whilst others who are new to the field may need further persuasion ( Loveless, 2018 ; Pawar, 2020 ). The occupation of people also has a significant influence in shaping their perceptions and acceptance of drone technology ( Ateya et al., 2022 ; Ichimura et al., 2022 ). For example, project managers may see drones as instruments for enhancing project efficiency and ensuring quality control, while surveyors may prioritize their capacity for precise data collection.
In a study in Pakistan, the high cost of drone technology and the lack of training and experience were significant obstacles. Agapiou (2020) cited the absence of a regulatory framework, increased expenses, and opposition to change as significant barriers ( Agapiou, 2020 ). It is revealed that the absence of clear rules and policies, the high cost of drones, and a lack of experienced workers were significant impediments to adoption. Kubo and Okoso (2019) researched the challenges and drivers of drone adoption in Saudi Arabia and found that low awareness and understanding, the absence of a regulatory framework, and high prices were the most significant impediments to adoption ( Kubo and Okoso, 2019 ). Kim et al. (2017) indicate similar challenges to using drones in the construction industry, including high prices, a lack of awareness and comprehension, regulatory impediments, and a need for more knowledge and competence ( Kim et al., 2017 ). It highlights the absence of rules and norms, high equipment prices, and technological constraints as the primary obstacles to implementing drones in construction. In the Korean construction business, it was discovered that a lack of competent workers and an inadequate understanding of drone technology were major obstacles ( Goessens et al., 2018a ; Rovira-Sugranes et al., 2022 ). Umar (2021) cited legal hurdles, cost concerns, and a lack of integration with existing processes as the main obstacles to drone adoption in the United Arab Emirates construction sector ( Umar, 2021 ). Mahajan (2021) researched India and discovered that there needed to be a legal framework, high prices, and low awareness and understanding were the primary obstacles to using drones in the construction industry ( Mahajan, 2021 ).
It is also important that the absence of rules, privacy concerns, and the restricted range of drones were the most significant obstacles to adoption in the Middle East. A study in China found the lack of laws, high prices, and lack of knowledge and comprehension of drone technology as the primary impediments ( Li 2019 ). Chung et al. (2020) found that there needed to be a regulatory framework, high prices, and a lack of training and education were the primary obstacles to drone adoption in China ( Chung et al., 2020 ). Research done in the United Kingdom highlighted the lack of standards, the difficulty of integrating drone data with BIM, and the lack of appropriate software tools as the primary obstacles to adoption ( Golpîra, 2021 ; Gibbin et al., 2023 ). Following the research gap, this study has two objectives. The first objective is to identify the barriers to implementing drones in the construction industry. The second objective is to identify the impact of overcoming obstacles of drone implementation on Construction Project Success in the construction industry.
The measurement of environmental advantages is a crucial element in sustainable building. While several research studies have recognised the potential of AI-driven crewless aerial vehicles to mitigate energy consumption and carbon emissions, only a few studies have presented quantifiable data. Nevertheless, this domain needs to be more adequately investigated since a dearth of research provides thorough quantitative evaluations of the environmental consequences ( Albeaino et al., 2022 ; Alsamarraie et al., 2022 ). There is a need for research endeavours that include project-specific variables, including geographical place, magnitude, and intricacy, to provide a complete comprehension of enhancements in efficiency. However, most studies conclude by highlighting these difficulties without providing specific answers or techniques for their reduction ( Beiki and Mosavi, 2020 ; Bera et al., 2022 ). The potential of AI-powered drones to gather extensive quantities of data in construction presents a promising opportunity for decision-making informed by data. Numerous studies have shown the potential of this technology to better project management and optimise resource allocation. One notable deficiency in the existing body of literature is the lack of comprehensive frameworks that guide the incorporation of AI-enabled drones into sustainable building methodologies ( Charlesraj and Rakshith, 2020 ; Chen et al., 2021 ). Most research endeavours concentrate on sure facets, such as efficiency or environmental effect, without presenting a holistic framework for sustainable building. Previous research has put forward a conceptual framework aimed at the seamless integration of drones often called drones, into construction project management. Nevertheless, it is worth noting that this framework needs to improve its emphasis on sustainability, creating an avenue for more investigation in this specific domain ( Ciampa et al., 2019 ; Chung et al., 2020 ).
To assess their sustainability contributions, it is essential to thoroughly understand these technologies’ life cycle effects on building projects. The prevailing body of literature mainly comprises research undertaken in developed areas characterised by established regulatory frameworks and infrastructure. This bias imposes constraints on how the results may be applied to places characterised by distinct socio-economic situations and building practices ( Dillow, 2016 ; Entrop and Vasenev, 2017 ; Elghaish et al., 2021 ). Future studies should include a wide range of scenarios to enhance the comprehensiveness of our knowledge about the worldwide application of AI-based drones in sustainable building. The existing body of research recognises the potential impact of AI-based drones in transforming sustainable building; nevertheless, significant gaps still need to be addressed. The identified gaps encompass various areas that require attention in the academic realm ( Feng et al., 2013 ; Firth, 2018 ; Feder, 2020 ). These gaps pertain to the necessity for thorough quantitative evaluations of environmental impact, a more extensive examination of efficiency improvements and cost reductions, the formulation of feasible strategies to address implementation obstacles, the incorporation of data generated by drones into decision support systems, the establishment of comprehensive sustainability frameworks, and the ongoing monitoring of project performance over an extended period ( Goessens et al., 2018a ; Ganesan et al., 2020 ; Gibbin et al., 2023 ). Rectifying these shortcomings will significantly expand existing knowledge and enhance the seamless incorporation of artificial intelligence-powered uncrewed aerial vehicles into sustainable building methodologies.
Overall, these studies highlight the need for a holistic approach to addressing the barriers to drone adoption in the construction industry, which includes not only addressing regulatory and financial issues but also increasing awareness and knowledge about drone technology and developing the infrastructure and resources required to support its integration into construction workflows. The central hypothesis for the study is H1: Overcoming barriers to implementing AI-based drones has a positive effect on construction project success. This study concentrates on building more precise frameworks and suggestions for overcoming the unique challenges mentioned in different situations and examining the potential advantages of drone adoption in construction beyond cost savings and productivity increases.
3 Identification of model barriers
In the research, 16 semi-structured interviews were used to determine the obstacles to using drones and the success criteria for AI technology in the construction industry. The interviews were conducted to get a more excellent knowledge of the problems and possibilities related to deploying drones and AI technology in the construction sector, as assessed by industry experts.
The semi-structured interview method was selected because it permitted freedom of questions and allowed for prompts and probes. The interviews with construction businesses and stakeholders aimed to get insight into their perspectives and experiences regarding employing drones and AI technologies ( Lee et al., 2019 ; Gupta et al., 2021 ).
The interview data were examined to discover common themes on the obstacles to using drones and the success criteria for AI technology in the construction industry. The data were then utilized to design a Smart PLS model to investigate the association between overcoming obstacles to drone adoption and the effectiveness of drones in construction.
Overall, semi-structured interviews offered a wealth of data that enabled a detailed examination of the obstacles and success factors associated with deploying drones and AI technologies in the construction industry ( Lee et al., 2020 ). This information may be used to guide future industry research and policy choices.
The respondents in the study’s semi-structured interviews noted that environmental protection, public health, and quality management are three crucial elements that must be addressed to guarantee the success of AI technology in buildings ( Mishra, 2019 ; Ullo and Sinha, 2021 ).
By maximizing resource utilization, decreasing waste and emissions, and encouraging green building materials and processes, applying AI technology in construction may facilitate more environmentally friendly and sustainable building practices ( Irizarry et al., 2012 ; Kitjacharoenchai et al., 2020 ). Furthermore, AI technology may improve public health and safety by allowing higher precision and accuracy in project management and delivery, minimizing the likelihood of accidents and injuries on construction sites.
Furthermore, using AI technology may enhance the overall quality of construction projects by allowing higher efficiency and accuracy, minimizing mistakes and delays, and improving project results ( Lee et al., 2021 ; Albeaino et al., 2022 ). Table 1 presents the identified model barriers and Table 2 indicates the factors of project success along with their identified constructs. These success elements are crucial to guaranteeing the broad acceptance and deployment of AI technology in the construction sector, and industry experts and policymakers should prioritize their consideration.
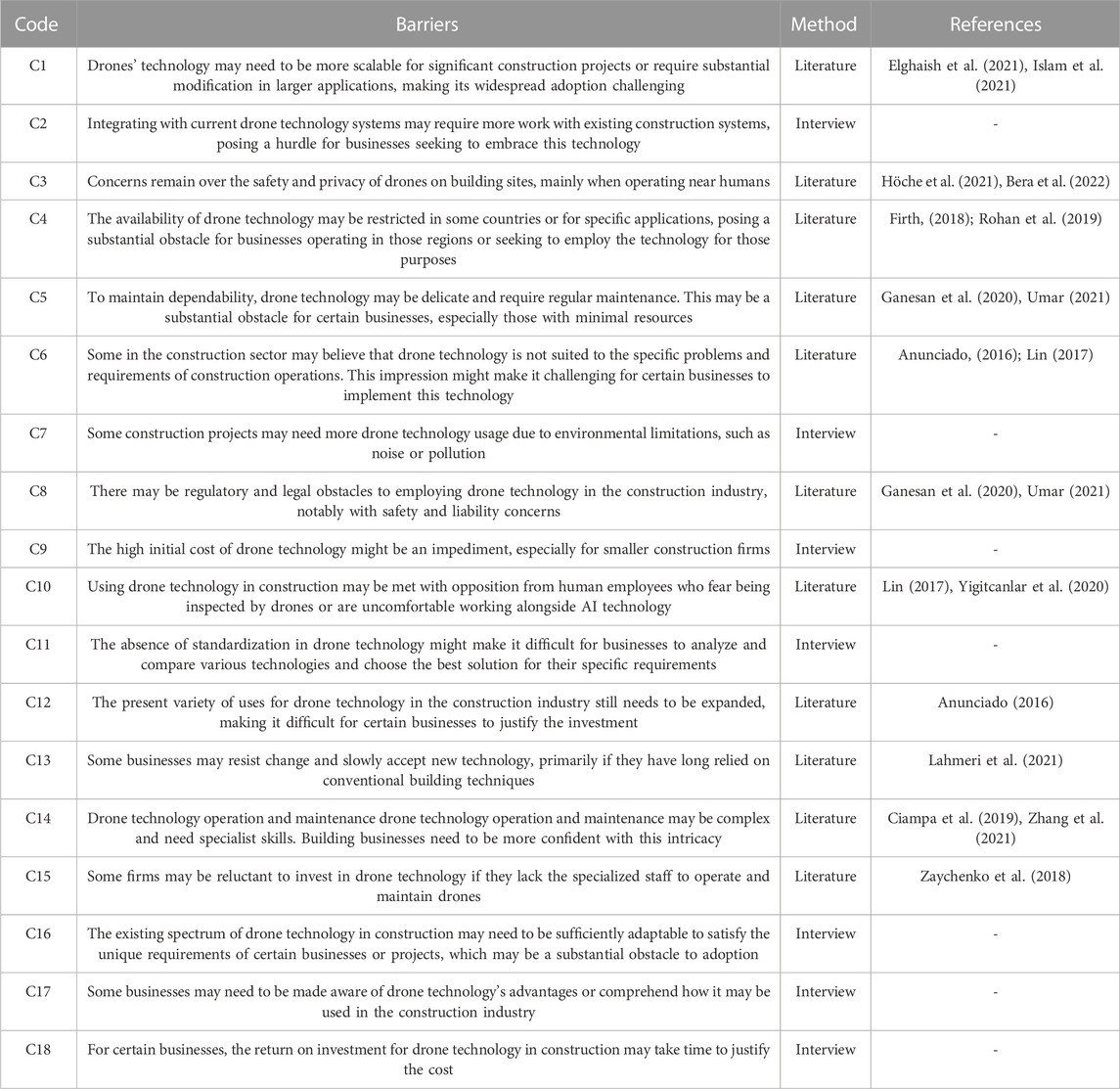
TABLE 1 . Identified barriers to drone implementation.
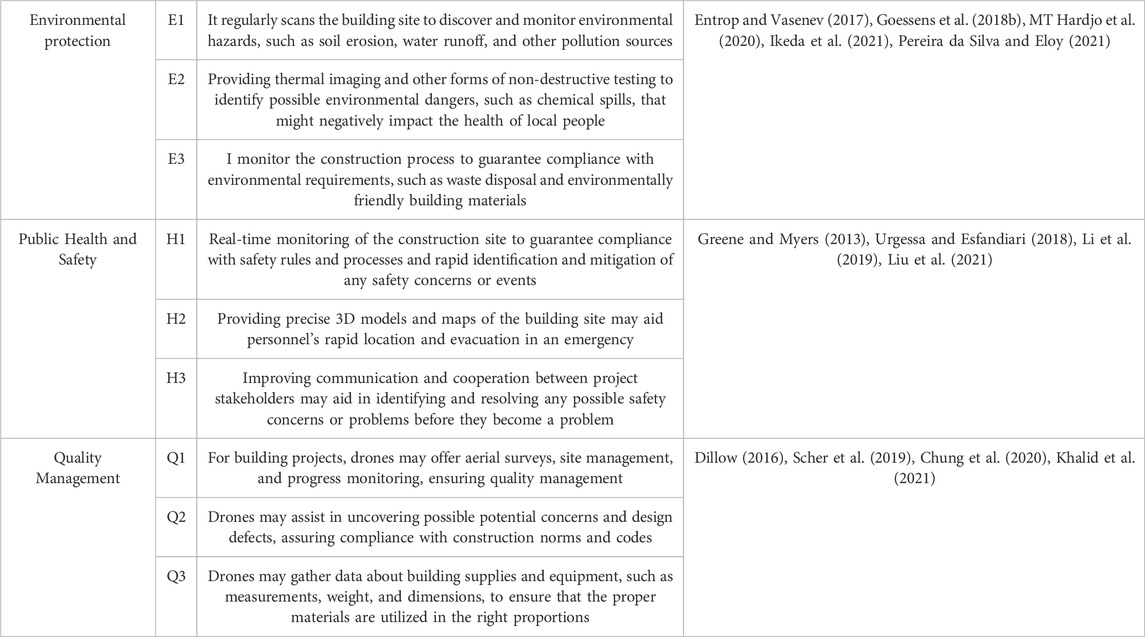
TABLE 2 . Construction project success factors with their constructs.
4 Methodology
Based on the studied literature, 18 hurdles to implementing drones were identified along with nine success factors under three constructs and judged relevant. Figure 1 further demonstrates it. Subsequently, a questionnaire survey was undertaken by distributing a list of obstacles to implementing drones to building industry professionals with relevant construction experience. It was conducted to assess the appropriateness and clarity of drone innovation hurdles that inhibit its adoption and to analyze these barriers and their kinds using exploratory analysis of factors (EFA).
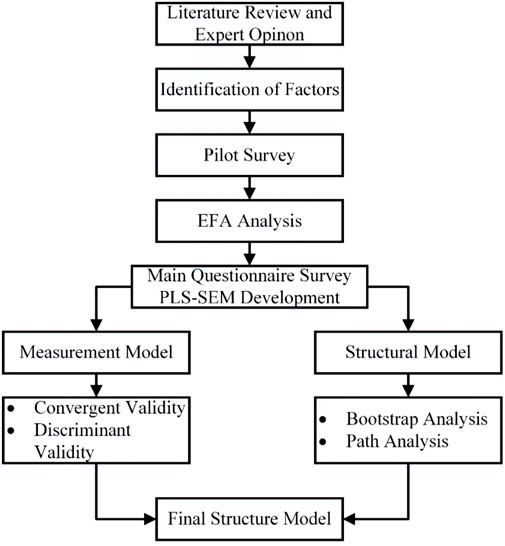
FIGURE 1 . Flow chart of the work.
4.1 Data collection
Using a questionnaire, Malaysian stakeholders in the prospective construction sector were contacted during data collection to analyze the hurdles to drones and associated success factors with their implementation ( Feng et al., 2013 ; Duda et al., 2019 ; Beiki and Mosavi, 2020 ). The survey instrument was separated into four primary components: 1) the respondents’ demographic profile, 2) the drone adoption hurdles, 3) success factors for overcoming the drone implementation barriers, and 4) open-ended questions that allowed experts to add any relevant barriers identified by stakeholders. There were three primary groups questioned. They include customers, consultants, and independent contractors.
They are classified further depending on occupation: mechanical, electrical, structural engineers, architects, and quantity surveyors. Using a five-point Linkert scale, the study population assessed the drone’s adoption hurdles and success based on their experience and knowledge (5 = very high, 4 = high, 3 = average, 2 = low, and 1 = very low). This metric has been widely used in the literature. The drone is a recent development in Malaysia. Hence, a stratified sampling strategy of a particular subgroup has been evaluated [85]. In addition, the sample size selection for this investigation was based on examining the procedural goal [86,87]. Figure 1 presents the stages of the research. According to Kline [88], a multidimensional route model requires at least 200 samples.
In contrast, Yin [89] asserts that at least 100 examples are sufficient for SEM. Due to the use of SEM in this research, 248 respondents were obtained from 335 found initially. The 257 individuals were reached through a self-administered questionnaire with a response rate of 71.81%. It was determined that the rate of return was appropriate for this sort of study [90,91].
4.2 Data analysis
SEM-PLS (Structural Equation Modeling-Partial Least Square) has attracted interest from several fields, particularly the social and economic sciences [59]. Popular SSCI Publications [60–62] have published more studies using the SEM-PLS methodology. The most current edition of the SMART-PLS 4 version was utilized to analyze the collected data and estimate the importance of implementation hurdles and success factors for drones using SEM. The SEM-PLS was initially recognized for its solid predictive capabilities when compared to covariance-based CB(SEM)-structural equation modelling ( Yıldız et al., 2021 ), even though the discrepancy between the two techniques is relatively tiny ( Duda et al., 2019 ). This research’s mathematical analysis comprises analytical and structural modelling evaluation techniques.
4.2.1 Common method variance
Common Method Bias (CMB) results from the standard method variance (CMV). The CMB assists in explaining the mistake (or variance) in the outcomes of an analysis, which is associated with the analytical approach as opposed to the ideas represented by the methodologies [65]. It may be defined as the overlap in variance across ideas ( Yang et al., 2021 ; Alsamarraie et al., 2022 ). Similarly, the CMV is complex whenever data from a specific source, such as self-collected data via a questionnaire, are available [66,67]. In some circumstances, self-collected data might overestimate or misrepresent the number of perceived associations, causing issues [67,68]. That may be crucial, especially for this study, since all data sets are self-collected, unique, and drawn from a single source. Thus, it is vital to address these problems to detect any potential CMV. A valid single-factor test was conducted following the studies undertaken by Harman et al. [69] [70]. Factor analysis yields a single factor that explains most of the variance ( Duda et al., 2019 ; Lewandowski, 2021 ; Yıldız et al., 2021 ).
4.2.2 Analytical model
The analytical model reveals the existing link and underlying structure between variables [71]. The next part evaluated the analytical model’s discriminant and convergent validity.
4.2.2.1 Convergent validity
CV exemplifies the degree of agreement between two or more binary variables (or obstacles) of the same notion or constructs. It is considered a subset of the validity of the concept. The CV of the calculated constructs in PLS may be determined using three tests. I composite reliability ratings (Pc), Cronbach’s alpha, and estimated average variance (AVE). There is a suitable mixed reliability level of 0.70 (Pc). For any research, scores above 0.60 and 0.70 for exploratory studies are considered acceptable. The last test is the AVE, which is regarded as a typical calculation used to assess the CV of the model’s structures. My results are more than 0.50, suggesting an excellent CV.
The following calculations were used for concurrent validity exploration:
where: λ = the factor loading of each indicator on its corresponding construct.ε = the indicator’s unique error variance.where: k = the number of indicators in the construct.ε = the indicator’s unique error variance.ΣX 2 = the total variance of the construct, equal to the sum of the squared factor loadings and error variances of the indicators.
4.2.2.2 Discriminant validity
Discriminant validity (DV) stipulates that the concerns under study are experientially unique and proposes that no dimensions define the construct under investigation in SEM. If the DV is to be determined, the degree of similarity across dispersed measurements must not be excessive. The Fornell-Larcker criteria compare the square root of the AVE for each construct to the construct’s correlations with other constructs in the model ( Feng et al., 2013 ). When the square root of the AVE for a particular construct is more prominent than its correlation with other constructs in the model, it is argued that the construct has discriminant validity ( Makadsi, 2019 ; Kisi et al., 2020 ; Chen et al., 2021 ). The AVE for each structure is computed as follows:
where λ 2 is the factor loading squared, θ is the construct’s error variance.
The correlation between two constructs, i and j, is calculated as:
The square root of the AVE for each construct is then compared to the construct’s correlation with other model constructs. The discriminant validity is established if the square root of the AVE is larger than the correlation with any different concept ( Urgessa and Esfandiari, 2018 ; Beiki and Mosavi, 2020 ). The Heterotrait-Monotrait (HTMT) ratio of correlations is determined by dividing the correlation between two constructs (hetero-trait) by the average correlation of each construct with itself (monotrait). Following is the algorithm for calculating HTMT:
Where r_ij is the correlation between constructs i and j, and ave (r_i,r_j) is the average of the correlations of constructs i and j with themselves. The HTMT threshold value is generally set at 0.9 or less, suggesting that the constructs are sufficiently dissimilar from one another to have discriminant validity ( Li et al., 2019 ; Liu et al., 2021 ). The cross-loading of an item on a structure may be described mathematically as follows: For construct j, the loading of item λ _ij gives me, and the residual variance of item ξ_i gives me. Then, the cross-loading of article i on construct k (k ≠ j) is provided by:
Where cov (i, k) is the covariance between item I and construct k.Var(i) is the variance of item i.
4.2.3 Structural model analysis
This research attempted to demonstrate, using the SEM, the main hurdles to drone implementation in construction projects and its success factors. It may be accomplished by first determining the route coefficients. Hence, a one-way causal link or route relation has been postulated between the concepts of drone’s obstacles (£) and drone’s success adoption barriers (µ) ( Kardasz and Doskocz, 2016 ; Oudjehane et al., 2019 ; Hatfield et al., 2020 ). Thus, the functional relationship between £, µ, and ∈ 1 principle in the structural equation model has been identified as an inner connection that a linear model may represent:
When the route coefficient linking drone, conceptions is β , and it is believed that ∈1 represents the residual correction at the operational level. Hence, the standardized regression load would be identical to the weight of the multiple regression model. Its signals must correspond to the model’s predictions and be statistically significant ( Makadsi, 2019 ; Kisi et al., 2020 ; Chen et al., 2021 ). The issue arises in determining the significance ( β ) of the route coefficients. For the CFA, the bootstrap approach available in the SmartPLS 4 program was used to estimate the standard errors of the route coefficients. Five thousand subsamples were used for this. Hence, the t-statistics of testing the hypothesis have been defined. Three functional formulas for drone ideas were created using the PLS model. It illustrates the underlying connections between ideas and Eq. 8
Using the SmartPLS 4.0 software, a structural equation modelling (SEM) study was undertaken to explore the links between the research model’s components. The bootstrap approach was used to assess the relevance and robustness of the parameter estimations of the model. Bootstrapping entails obtaining random subsamples from the data set and creating a distribution of parameter estimates for each model route ( Greene and Myers, 2013 ; Duda et al., 2019 ). The t-value and p -value show the significance and strength of the association between the constructs for each path coefficient, provided as the results of the bootstrap analysis ( Kardasz and Doskocz, 2016 ; Oudjehane et al., 2019 ; Hatfield et al., 2020 ). A p -value of less than 0.05 was statistically significant. In addition, the coefficient of determination (R-squared) was used to assess how much variation the model explained. R-squared values over 0.3 were regarded as acceptable.
4.2.4 Predictive relevance analysis
After determining the relevance of the route variables, the structural model’s prediction ability was evaluated. The model’s predictive power was assessed using the cross-validated R-squared (Q2) value. The Q2 value represents the amount of predicted variation in the dependent variable. A Q2 value over 0.25 suggests excellent predictive ability, while a Q2 value below 0.1 shows poor predictive power. SmartPLS 4.0’s structural model analysis comprehensively examines the model’s element connections. The structural model is reliable and valid owing to the use of advanced statistical techniques, such as bootstrapping, and the evaluation of its predictive capability.
5.1 EFA analysis
The exploratory factor analysis (EFA) findings suggest that the 18 obstacles to using drones with AI in the construction sector may be broken down into three constructs or components that account for 59.259% of the total variation. Table 3 presents the rotated component matrix from EFA, along with Cronbach Alpha values for each construct.
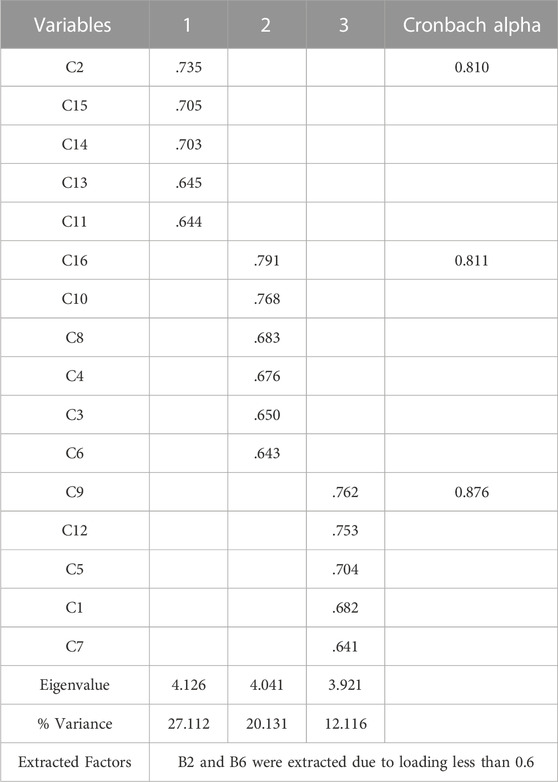
TABLE 3 . Exploratory factor analysis output.
The first part, “Technical and Functional Barriers,” is made up of 5 barriers with high factor loadings, meaning they are highly correlated with each other and can be thought of as obstacles relating to the integration and compatibility of AI-based drone technology with existing construction systems, safety and privacy concerns, regulatory and legal barriers, and a lack of standardization in the technology ( Yıldızel and Calış, 2019 ; Khalid et al., 2021 ).
The second part, titled “Privacy and Legal Barriers,” is comprised of six barriers with high factor loadings; this means that they are highly correlated with each other and can be thought of as barriers relating to the high price of the technology, the need for routine maintenance and specialized skills, the resistance to change, and the lack of technical staff to operate and maintain the drones.
Thirdly, the “Economic and Sustainability” factor includes six obstacles that have high factor loadings, indicating that they are highly correlated with each other and can be thought of as obstacles related to the environmental limitations of using drone technology in construction, employee opposition, and discomfort with AI technology, the difficulty and complexity of operating and maintaining the drones, and the limited variety of uses and awareness of the tec. The strong Cronbach’s alpha values for all three subscales suggest that the individual barriers used to evaluate each subscale are valid and trustworthy indicators of the construct they are meant to assess.
The findings as a whole indicate that there are several obstacles to the successful use of drones powered by artificial intelligence in the construction business. These barriers may be broken down into three categories. The findings of this EFA may guide future studies and practitioners in overcoming obstacles to the widespread use of intelligent drones in the building sector.
The final categorized barriers to AI-based drones in construction are according to EFA results. Businesses may face technical and functional hurdles when trying to embrace and integrate drone technology into their operations, which is what the Technical and Functional Barriers category is all about ( Dillow, 2016 ; Scher et al., 2019 ; Chung et al., 2020 ). Difficulties in integrating drone technology with existing systems, a shortage of specialized staff to operate and maintain the technology, aversion to change and new technology, a lack of standardization in drone technology, and the misconception that drone technology is not suited to the unique demands of construction projects are all obstacles to overcome. Concerns about personal privacy, workplace safety, and legal implications are all factors that might slow down the widespread use of drones in the building. These include the belief that drone technology is not suited for construction operations, the dedication that drones cannot be adapted to meet the unique requirements of certain businesses or projects, opposition from human employees who fear being replaced by drones or working alongside AI technology, and regulatory and legal obstacles. Economic and sustainability concern the potential financial and environmental costs companies may incur using drone technology ( Yıldızel and Calış, 2019 ; Khalid et al., 2021 ). Drone technology has several obstacles, including its high upfront cost, restricted range of applications in construction, its fragile nature and the need for routine maintenance, its inability to scale to more significant building projects, and environmental constraints like noise or pollution. These three frameworks illustrate the many potential challenges companies may face while embracing and using drone technology within the building sector ( Kardasz and Doskocz, 2016 ; Oudjehane et al., 2019 ; Hatfield et al., 2020 ). The EFA findings imply that these obstacles may be broken down into three broad groups, which can guide the development of specific treatments and methods to overcome them.
The components that emerged from the EFA were technological and functional limitations, privacy and legal restrictions, and economic and sustainability concerns. Possible roadblocks to the widespread use of drones powered by artificial intelligence in the building sector include the structures mentioned earlier. Using this data, hypotheses “H2: Technical and functional Barriers have a positive impact on Implementation of AI-Based Drones in the construction industry,” “H3: Privacy and legal barriers have a positive impact on Implementation of AI-Based Drones in the construction industry,” and “H4: Economic and sustainability barriers have a positive impact on Implementation of AI-Based Drones in the construction industry,” were developed. All these show that removing these roadblocks to using AI-powered drones might benefit the construction sector. Figure 2 presents the hypothesized framework of formative constructs and reflective constructs. In particular, organizations looking to embrace this technology may need help combining drone technology with current construction processes. Similar privacy and legal constraints, including safety and liability worries, may slow down the widespread use of drones. Lastly, firms may find the high initial cost and maintenance needs of AI-based drones to hinder their use in construction. After reviewing the available literature and conducting interviews, the authors developed three further hypotheses about the usefulness of drones powered by artificial intelligence in the building industry. The hypotheses were, “H5: Implementation of AI-based drones in construction industry positively impact Construction Project Success by Quality Management,” “H6: Implementation of AI-based drones in construction industry positively impact Construction Project Success by environment protection” and “H7: Implementation of AI-based drones in construction industry positively impact Construction Project Success by public health and safety.” Using AI-powered drones in the construction industry might improve quality control, safeguard the environment, and boost public health and safety. In sum, we utilized the EFA findings to create the six hypotheses about the adoption and effect of AI-based drones in the construction sector, considering the possible challenges and opportunities presented by this emerging technology.
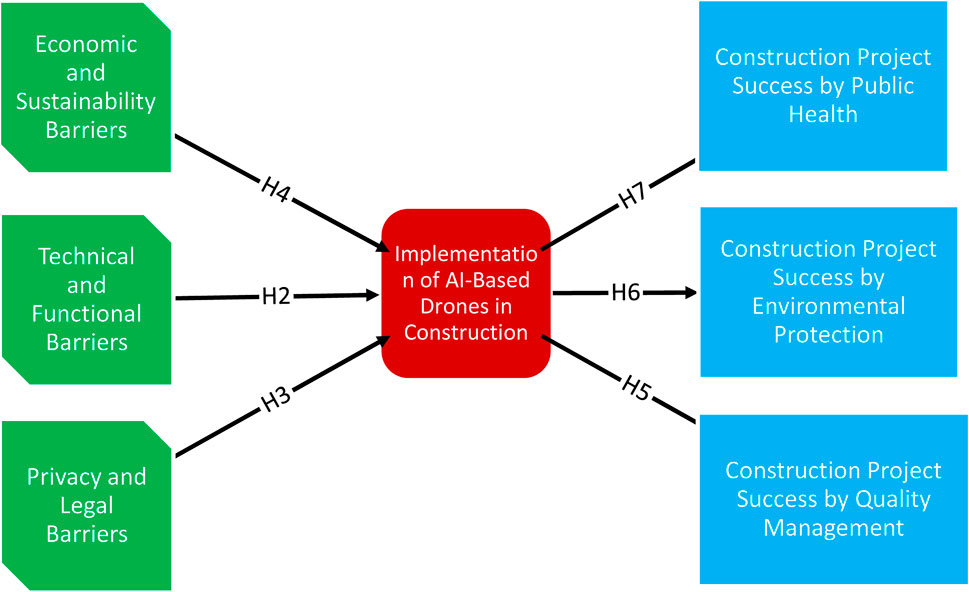
FIGURE 2 . Formative constructs with their hypotheses.
5.1.1 Demographics
The results of the demographics of the primary questionnaire survey are indicated in Figure 3 . Most have a Master’s degree (43%), whereas just 32% have a Bachelor’s degree. The proportion of those with a Ph.D. is lower (14%), while the “Others” group includes 10%. The largest demographic of responders (43%) is comprised of those aged 31–35, followed by those aged 26–30 (22%) and those aged 36–40 (15%). Just 7 per cent of those who answered the survey were 40 or older. In terms of years of experience, the largest share of respondents had worked in the field between 11 and 15 years (44%), followed by those with 5–10 years of experience (21%) and those with 16–20 years of experience (15%). Just seven percent of those polled had more than 20 years of experience. Most responders (74%) are civil engineers; the subsequent most common occupation is the project manager (18%) and safety manager (4%). Architects and those who answered “Other” comprise a smaller sample fraction (3% and 1%, respectively). When taken as a whole, the survey’s respondent pool represents a cross-section of the construction industry’s age, education, experience, and occupational spectrum.
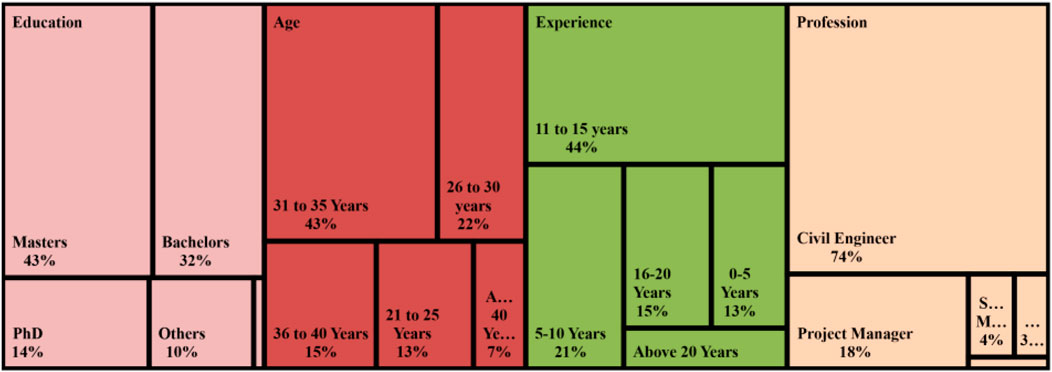
FIGURE 3 . Demographic details.
5.2 Structure equation modelling (SEM) and analysis
Cronbach’s alpha is often used to quantify a measure’s reliability, which is the extent to which it maintains consistent and stable results over time. Internal consistency of items inside a concept is measured by the composite reliability (rho-a and rho-c), with rho-c being the more robust measure of reliability. Figure 4 presents the trend of composite reliability in formative and reflective constructs. With a more considerable value representing more convergent validity, the average variance extracted (AVE) quantifies how well the items in a construct capture the latent variable.
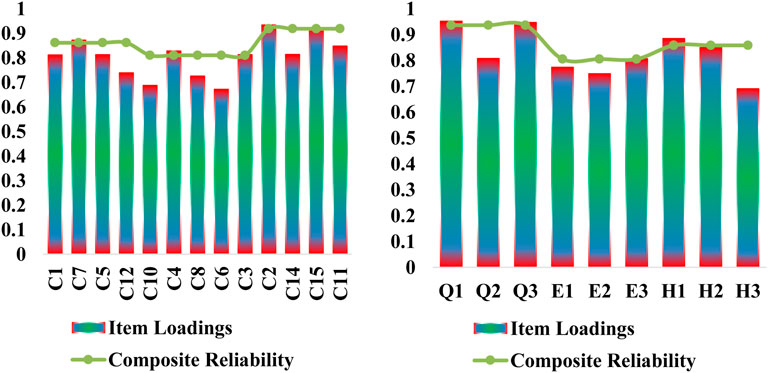
FIGURE 4 . Item loadings with composite reliability for formative and reflective constructs items.
Cronbach’s alpha coefficients for each construct range from 0.701 to 0.902, indicating excellent reliability. The rho-a and rho-c values, which comprise the composite reliability, are relatively high, ranging from 0.805% to 0.936% and 0.852%–0.932%, respectively. Figure 5 presents the trend of composite reliability in formative and reflective constructs. It shows that the components inside each construct are very consistent and trustworthy.
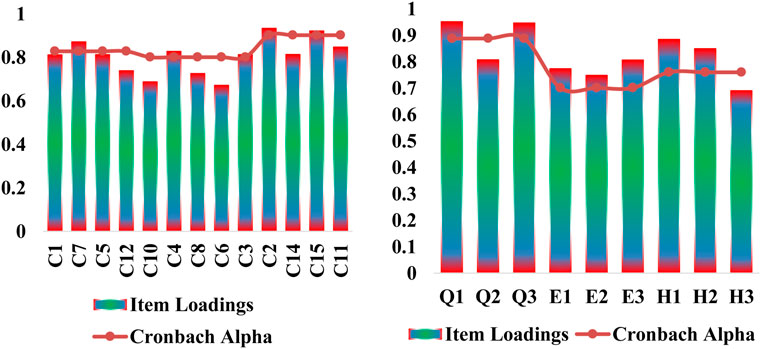
FIGURE 5 . Item loadings vs. Cronbach alpha for formative and reflective construct items.
In addition, the AVE values range from 0.558 to 0.817, much over the minimally acceptable criterion of 0.5. Hence, it can be concluded that the items within each construct have strong convergent validity and are substantially linked with the underlying concept ( Hatfield et al., 2020 ). Figure 6 presents the trend of composite reliability in formative and reflective constructs.
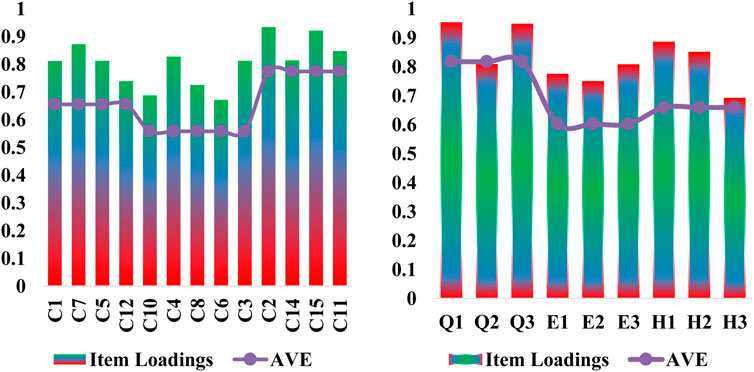
FIGURE 6 . Item loadings vs. AVE for formative and reflective constructs items.
This suggests that the constructs used in the study are reliable and valid, which means they can be used to measure the intended constructs of economic and sustainability barriers, environmental protection, privacy and legal barriers, public health and safety, quality management, and technical and functional barriers. The relationship significance is indicated between constructs and latent variables. Figure 7 demonstrates the path coefficients.
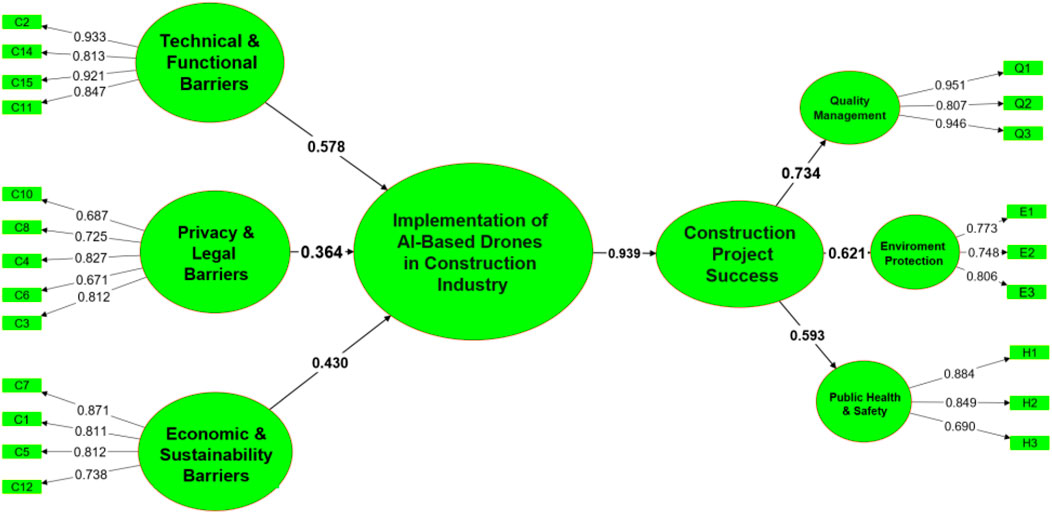
FIGURE 7 . Model with items, item loadings, and path coefficients.
5.2.1 Second order analysis
Table 4 presents the evaluation results for six fundamental constructs (Economic and sustainability Barriers, Privacy and legal Barriers, Public Health and safety, and Technological and functional Barriers) using the Fornell-Larcker criteria. These criteria are essential for assessing the discriminative capability of these constructs effectively. Examining the table’s diagonal reveals each construct’s square roots of the AVE. The AVE quantifies how much a particular concept can account for variance within its indicators. Generally, an AVE value of 0.5 or greater signifies robust convergent validity, indicating the construct adeptly captures the shared variance among its indicators ( Loveless, 2018 ; Li and Liu, 2019 ). Moving on to the off-diagonal values in the table, they depict correlations between different constructs. According to the Fornell-Larcker criteria, for constructs to demonstrate high discriminant validity, the sum of squared correlations between any two constructs should be smaller than the AVE of each concept. This signifies that the constructs should remain distinct, and the shared variance between them should be less than what each construct can explain independently. The findings in Table 4 confirm that all constructs meet the criteria for convergent validity, with AVE values greater than 0.5. Additionally, the AVE values off-diagonal are consistently lower than the AVE values on-diagonal for each concept, indicating robust discriminant validity. This provides strong evidence that the constructs effectively measure separate dimensions of the phenomena under investigation and substantiates the credibility of our study’s construct usage.
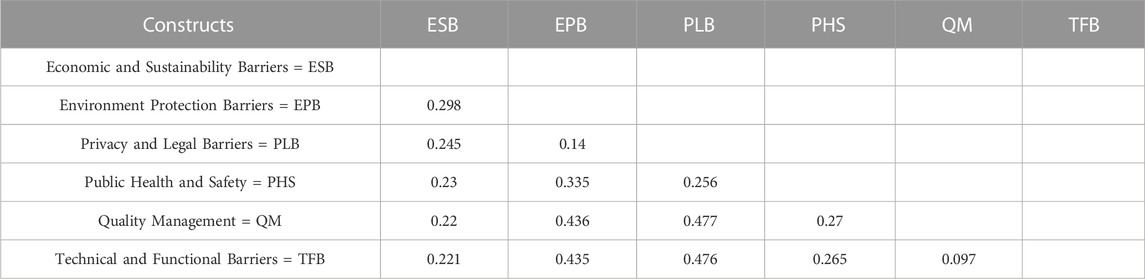
TABLE 4 . Fornell Larker criteria results.
Table 5 provides the HTMT (Heterotrait-Monotrait) ratio data, a commonly used method for assessing the discriminant validity of a measurement model. This analysis is vital to ensure that the constructs within the model are distinct. The table’s diagonal displays the square root of the AVE for each construct, while the numbers below the diagonal represent the HTMT ratios. The HTMT ratio is a crucial metric that must be below 0.90 to confirm discriminant validity. In essence, this metric assesses whether constructs are sufficiently different. In our analysis, all HTMT values are well below the 0.90 threshold, indicating discriminant validity among the constructs ( Wazid et al., 2020 ; Ateya et al., 2022 ). Each construct effectively captures unique aspects of the phenomena under investigation. To further confirm discriminant validity, we compare the square roots of the AVEs with the HTMT values in the relevant rows and columns. The fact that the AVEs are consistently more significant than the HTMT values reinforces that our model accurately distinguishes between various latent variables ( Lee and Kwon, 2020 ; Lawani et al., 2022 ). The HTMT analysis supports the notion that the model’s components are distinct and effectively measure different aspects of the constructs. This demonstrates the model’s accuracy in discerning between various groups and confirms the discriminant validity of our measurement model.
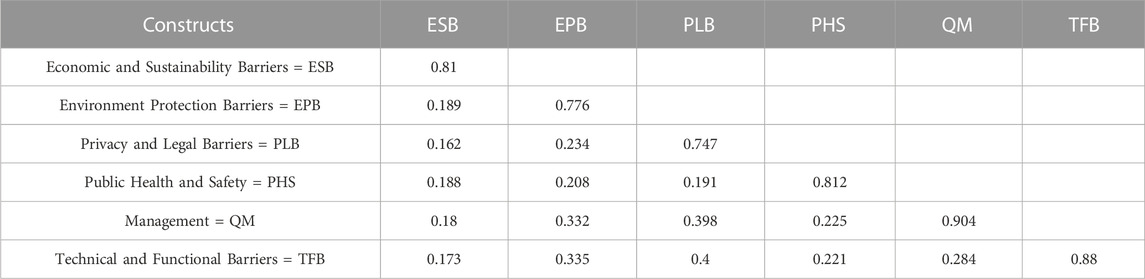
TABLE 5 . HTMT analysis results.
Table 6 illustrates the relationships between individual items and their respective constructs, which are essential for assessing construct validity within our measurement model. It offers insights into the alignment of each item with its intended construct and the potential for capturing unintended aspects. The goal is for items to exhibit strong loadings on their designated constructs and minimal loadings on others ( Li and Liu, 2019 ; Charlesraj and Rakshith, 2020 ). Reviewing the table, it becomes clear that most items demonstrate substantial loadings on their intended constructs. For instance, items associated with the Economic and Sustainability Barriers construct show loadings of 0.811, 0.871, 0.812, and 0.738 for C1, C7, C5, and C12, respectively.
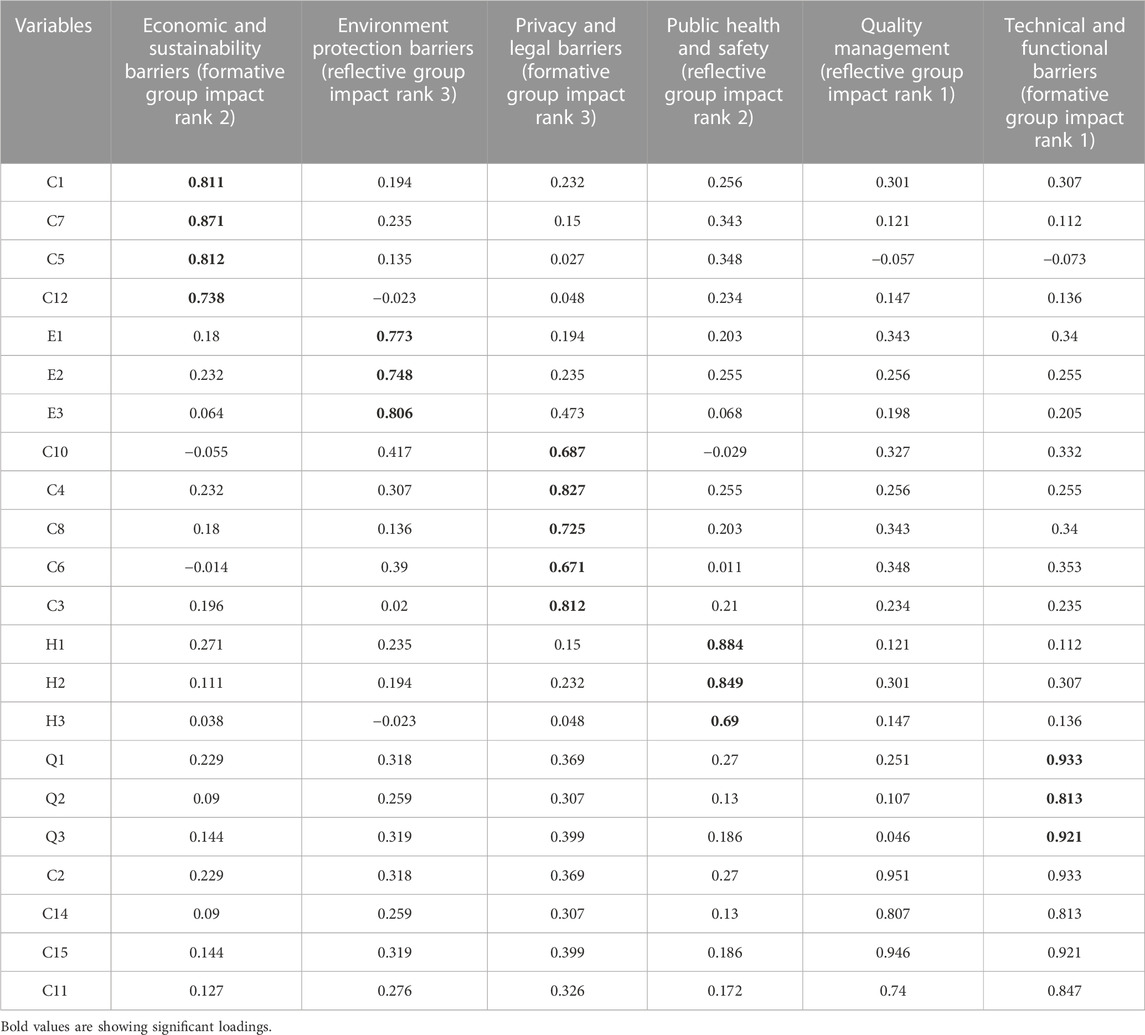
TABLE 6 . Cross loadings of items.
Similarly, items E1, E2, and E3 display loadings of 0.773, 0.748, and 0.806 on the Environmental Protection Barriers construct precisely as intended. However, some exceptions exist where items exhibit significant loadings on additional constructs. For instance, item C2 from the Quality Management construct displays a loading of 0.951 on the Technical and Functional Barriers construct, suggesting it may inadvertently capture aspects of that construct as well. Additionally, item C11 exhibits strong loadings on both the Privacy and legal Barriers and Technical and functional Barriers despite its intended placement in the Quality Management construct. The cross-loadings analysis indicates that, with some notable exceptions, the items effectively measure the targeted constructs rather than unintended ones. These findings inform potential refinements to the measurement scale, ensuring accurate capture of the intended characteristics.
In this scenario, the dependent variable is the frequency with which intelligent drones are used in building projects; hence, the group impact ranking indicates the relative relevance of each construct in making this prediction. Quality Management was shown to have the most significant collective influence, with a grade of 1 ( Oudjehane et al., 2019 ; Hatfield et al., 2020 ). Quality Management is the most influential factor in using drones with artificial intelligence in the building business. Q1 and Q3 have enormous outer weights in the Quality Management construct, suggesting a stronger connection to the construct. With a group effect value of 1, the Technological and Functional Barriers construct similarly significant in foreseeing the use of AI-based drones in the building sector. C2, C15, and C14 are the heaviest outside elements in this framework. With a group effect value of 2, the Economic and Sustainability construct is relatively significant in foreseeing the widespread use of AI-powered drones in the building sector. Items C7 and C5 have the most ideal outside weights in this structure. With a group effect value of 3, the Privacy and Legal Barriers construct is the least relevant in forecasting the adoption of AI-based drones in the construction business. Also, C16 has been eliminated from this construct, suggesting that it did not play a role in the measurement and may need to be included in future studies ( Khalid et al., 2021 ). Lastly, the group impact score of 3 for Environmental Protection and Public Health and Safety indicates that these concepts are relatively relevant in forecasting the use of AI-based drones in the construction business. Items with outer weights of H1 and H2 are the most important to public health and safety, whereas things with outside consequences of E1 and E2 are the most important to the environment.
5.2.2 Path analysis
The results of the route analysis for the formative constructs are listed in Table 7 . Each path’s t-value, p -value, VIF (variance inflation factor), and SE (standard error) are included in the table. Economic and sustainability Barriers, Privacy and legal Barriers, and Technical and functional Barriers all reveal favourable outcomes for deploying AI-based Drones in the Construction Sector. In particular, the p -values for the route coefficients between these three constructs are all less than 0.001, coming in at 0.430, 0.364, and 0.578, respectively. These three elements are crucial to effectively using drones powered by artificial intelligence in the building sector. It is also important to note that the VIF values for all three constructions are less than 1.5, which is the cutoff number for finding multicollinearity ( Feng et al., 2013 ; Duda et al., 2019 ; Beiki and Mosavi, 2020 ). This indicates little correlation between the formative components, suggesting that each adds something novel to the model. Results from the route analysis show that removing economic, sustainability, privacy, legal, technological, and functional hurdles is crucial to expanding the use of drones powered by artificial intelligence in the building sector.
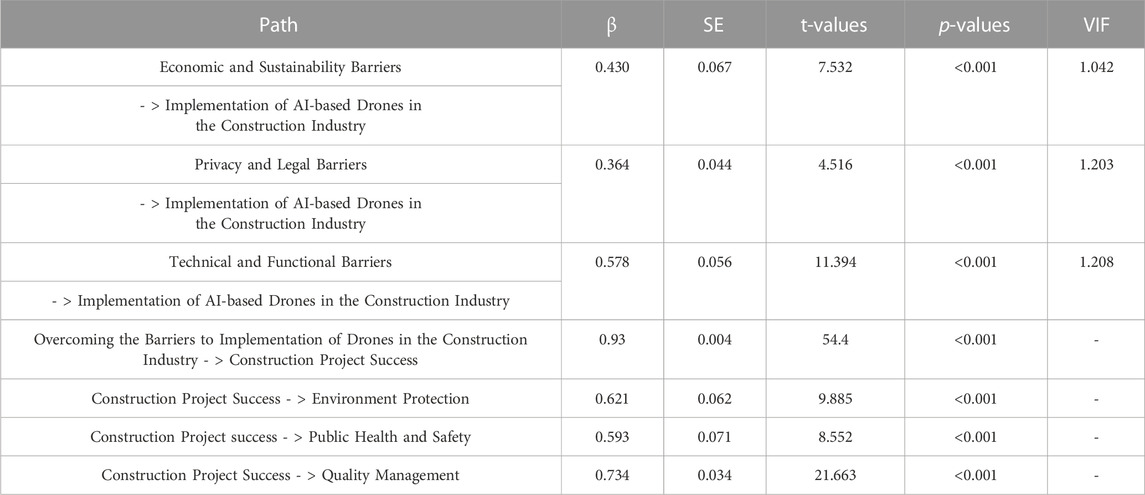
TABLE 7 . Path analysis results of formative constructs.
As seen by the high beta coefficient ( β = 0.93) and the low p -value (0.001), the findings suggest a positive and significant link between the two variables. This indicates that companies are likely to build projects more successfully as they overcome the obstacles of deploying AI-based drones ( Urgessa and Esfandiari, 2018 ; Li et al., 2019 ; Liu et al., 2021 ). Taken as a whole, these findings underline the significance of addressing and removing the many barriers that stand in the way of the widespread use of drones powered by artificial intelligence in the building sector. The reflecting constructions’ route analysis findings illustrate the connection between environmental preservation, public health and safety, and quality management in successful building projects. Path coefficients reveal the nature and direction of the association between the variables. All three route coefficients are positive and statistically significant ( p 0.001), demonstrating that a successful building project significantly improves all three reflective constructs. Success in a building project is most strongly correlated to quality management ( β = 0.734), environmental protection ( β = 0.621), and public health and safety ( β = 0.593). These findings point to a beneficial relationship between environmental protection, public health and safety, quality management, and the success of building projects. The significance of this result in ensuring these goals are attained in the construction sector cannot be overstated. The model with bootstrapping results indicating p -values is presented in Figure 8 .
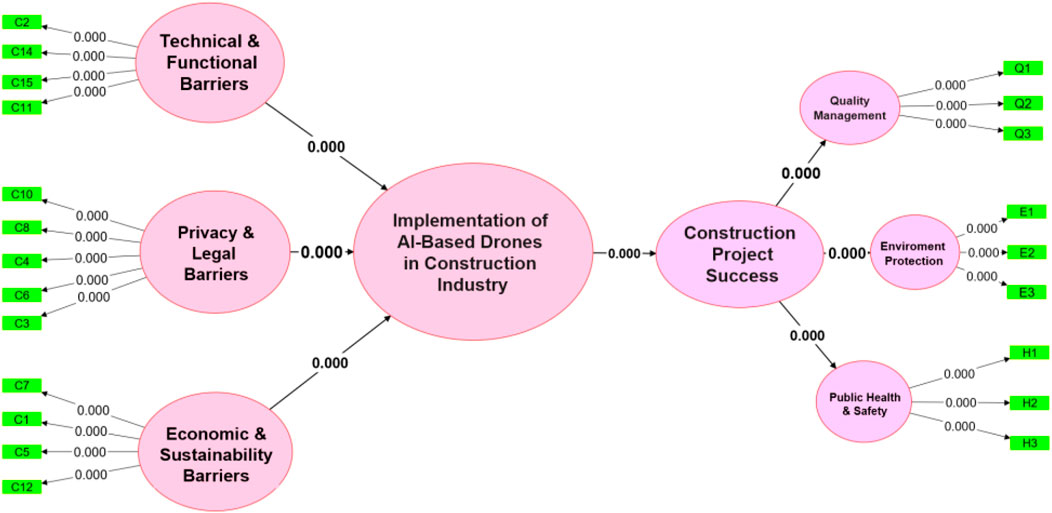
FIGURE 8 . Path significance results after bootstrapping analysis.
The total squares for the Construction Project Success construct are shown in the SS0 column. The sum of squares the model cannot explain is demonstrated in the SSE column. The predicted Construction Project Success value is shown in the Predict-Q 2 column. With an SS0 of 849.000, the model adequately explains a significant fraction of the observed variation in Construction Project Success. With an SSE of 529.479, there is some mystery around the success rate of building projects. Success on a Construction Project is anticipated to be 0.376, as seen in the Predict-Q 2 column. This means the model forecasts a modest degree of Construction Project Success based on the facts. Table 4 shows that the model is a good match for the data and that the other constructs in the model can provide a fair prediction of Construction Project Success ( Greene and Myers, 2013 ). The unaccounted-for variation in Construction Project Success may call for additional research to determine what elements contribute to this concept.
An R2 of 0.881 shows that the model explains 88.1% of the variation in Construction Project Success. Because the model can present a considerable amount of the observed variation in the dependent variable, it increases its predictive power. Adjusted R2 = 0.881 is near the R2 value; hence, the model is probably not overfitting the data. Model fit is supported by an “excellent” explained size and a “highly predictive” prediction of Construction Project Success using the model’s predictors.
The Importance and Performance Index (IPI) of the predictor variable “Overcoming the Barriers to Deployment of AI-based Drones in Construction Industry” is determined from SEM. Respondents placed a high value on this predictor, as demonstrated by its Importance score of 1.810. Respondents with a Performance score of 53.23 think this predictor performs adequately but might need work. The IPI rating is helpful for pinpointing problem spots. With such a high relevance rating, it is clear that removing the obstacles to using drones with artificial intelligence in the building business is crucial. As shown by the score of “moderate,” more effort is required to enhance the performance of this predictor, which might be accomplished by more efficient techniques to overcome the obstacles.
6 Discussion
Technical and Functional Barriers to formative construction include C2 “Integration with current systems drones technology may be difficult to integrate with existing construction systems, posing a hurdle for businesses seeking to embrace this technology,” C15 “Some firms may be reluctant to invest in drones technology if they lack the specialized staff required to operate and maintain the drones. C14 “Drone technology operation and maintenance may be difficult and need specialist skills. Some building businesses may be intimidated by this degree of intricacy,” and C11, “The absence of standardization in drone technology might make it difficult for businesses to analyze and compare various technologies and choose the best solution for their specific requirements.” Four things make up the Technological and Functional Barriers to formative construct, each describing a possible difficulty construction companies may have while attempting to use drones powered by artificial intelligence. Difficulty integrating the technology with preexisting systems, a shortage of qualified personnel to run and maintain the drones, complicated operation and maintenance, and a lack of standardization are all obstacles. The findings support the acceptance of H2: The Deployment of AI-Based Drones in the Construction Industry is Favored by Technological and Functional Barriers. A positive correlation of β = 0.578 ( p 0.001) was found between the constructs of Technical and Functional Barriers and the Implementation of AI-Based Drones in the Construction Industry ( Anunciado, 2016 ; Zaychenko et al., 2018 ; Ciampa et al., 2019 ). This suggests that organizations with more technical and functional hurdles to overcome will have difficulty integrating drones powered by artificial intelligence.
Privacy and Legal Barriers formative construct includes C10 “The use of drones technology in construction may be met with opposition from human employees who fear being inspected by drones or who are uncomfortable working alongside AI technology,” C8 “There may be regulatory and legal obstacles to the employment of drones technology in the construction industry, notably with safety and liability concerns,” C4 “The availability of drones technology may be restricted in some countries or for certain applications, posing a substantial obstacle for businesses operating in those regions or seeking to employ the technology for those purposes,” C3 “Concerns remain over the safety and privacy of drones on building sites, especially when operating near humans,” and C6 “Some in the construction sector may believe that drones technology is not suited to the specific problems and requirements of construction operations. This impression might make it challenging for certain businesses to implement this technology. The Privacy and Legal Barriers framework highlights potential difficulties for construction companies utilizing AI-based drones. Items in this framework indicate worries about legal and regulatory hurdles, safety and privacy issues, and human workers” reluctance to use this technology. These issues may discourage companies from making the first financial investment necessary to use drones in the building sector. According to the data collected and analyzed for this pathway, the Privacy and Legal Barriers construct positively affects the adoption of AI-Based Drones in the Building Sector. This data shows that companies are more likely to adopt drone technology for construction activities in jurisdictions with lower privacy and regulatory restrictions. Politicians and business leaders must thus address these concerns and lay forth precise rules and regulations for the use of drone technology in the building industry. The empirical findings show that the privacy and Legal Barriers construct a favourable influence on the deployment process of AI-based drones in the construction business ( Latteur et al., 2016 ; Charlesraj and Rakshith, 2020 ; Çetin et al., 2020 ). Because of this, we can confidently accept H3, which states that privacy and regulatory restrictions contribute to using AI-based drones in the building business.
Economic and sustainability formative construct include C12: “The present variety of uses for drone technology in the construction industry is still restricted, making it difficult for certain businesses to justify the investment,” C5 “To maintain dependability, drone technology may be delicate and requires regular maintenance. This may be a substantial obstacle for certain businesses, especially those with minimal resources,” C1 “Drones technology may not be scalable for major construction projects or may need substantial modification to be employed in larger applications, making its widespread adoption challenging,” and C7 “Some construction projects may be limited in their usage of drones” technology due to environmental limitations, such as noise or pollution.” Information supplied suggests that constraints on usage, maintenance sensitivity, scalability, and environmental issues all figure into the Economic and Sustainability formative construct. These considerations raise concerns about the widespread use of artificial intelligence (AI) drones in the building sector. Economic and sustainability hurdles substantially influence the adoption of AI-based drones in the construction sector, as the path analysis findings show. As a result, we can confidently believe H4, which claims that the Economic and Sustainability hurdles have a beneficial influence on the application of AI-based drones in the construction business ( Agapiou, 2020 ; Sawhney et al., 2020 ). This indicates that to overcome the constraints and effectively use AI-based drone technology, organizations must consider the economic and sustainability factors, such as the cost-benefit analysis, maintenance needs, scalability, and environmental consequences.
Quality Management reflective construct includes Q1 “For building projects, and drones may offer aerial surveys, site management, and progress monitoring, ensuring quality management,” Q2 “Drones may assist in uncovering possible potential concerns and design defects, assuring compliance with construction norms and codes” and Q3 “Drones may be used to gather data about building supplies and equipment, such as measurements, weight, and dimensions, to ensure that the proper materials are utilized in the proper proportions.” Three indicators make up the Quality Management reflection construct and show how using AI-based drones in the construction sector may improve the overall quality of projects. The first indication, Q1, describes how quality management may be ensured using drones by conducting aerial inspections, managing the site, and monitoring progress ( Goessens et al., 2018a ; Rovira-Sugranes et al., 2022 ). The second indication, Q2, highlights the value of drones in detecting issues and flaws in the design, which is essential for maintaining adherence to building standards and regulations. Q3 demonstrates how drones may collect information on materials and tools in the construction industry, which can then be used to guarantee that the proper resources are utilized. The findings of path analysis support the hypothesis that H5 (Construction Project Success as Influenced by Quality Management) is true when AI-based drones are used in the construction sector. With a beta value of 0.734, the three Quality Management indicators positively and substantially affect the success of construction projects. This indicates that drones equipped with artificial intelligence may be used to enhance quality control and boost the overall success of building projects.
Environmental Protection reflective construct includes E1, “Regularly scanning the building site to discover and monitor any environmental hazards, such as soil erosion, water runoff, and other pollution sources,” E2, “Providing thermal imaging and other forms of non-destructive testing to identify possible environmental dangers, such as chemical spills, that might negatively impact the health of local people,” and E3 “Monitoring the construction process to guarantee compliance with environmental requirements, such as waste disposal and the use of environmentally friendly building materials.” According to the examination of causal relationships, environmental protection is critical in completing construction projects that use reflective constructs. Mainly, it has been established that the three ecological protection indicators of frequent scanning of the construction site, non-destructive testing, and monitoring compliance with environmental regulations all contribute to the success of construction projects ( DeYoung, 2018 ; Li 2019 ). Using drones powered by artificial intelligence in the construction sector might assist in guaranteeing that projects are carried out in an ecologically friendly way, which in turn can lead to better project outcomes. The research results strongly support hypothesis H6, which states that using AI-powered drones improves the likelihood of a building project’s success due to better environmental safeguards.
Public Health and Safety reflective construct include H1, “Real-time monitoring of the construction site to guarantee compliance with safety rules and processes, as well as rapid identification and mitigation of any safety concerns or events,” H2, “Providing precise 3D models and maps of the building site, which may aid in the rapid location and evacuation of personnel in the event of an emergency” and H3 “Improving communication and cooperation between project stakeholders may aid in identifying and resolving any possible safety concerns or problems before they become a problem.” Three indicators are included in the Public Health and Safety reflective construct to show how intelligent drones in the building have contributed to improved public health and safety. The first indication, H1, emphasizes continuous work site monitoring to check for adherence to safety protocols and prompt detection and resolution of potential problems. H2 is the second indication, and its primary goal is to facilitate the speedy location and evacuation of workers in an emergency by giving accurate 3D models and maps of the construction site. The third indication, H3, stresses increased coordination and communication among project participants to foresee and address potential safety issues. The study’s findings support H7, which hypothesizes that using drones equipped with artificial intelligence would improve the success of building projects from the perspective of public health and safety ( Kubo and Okoso, 2019 ; Sawhney et al., 2020 ). Real-time monitoring of the construction site, accurate 3D models and maps of the building site, and enhanced communication and cooperation between project stakeholders are just some of how the study found that using AI-based drones in construction positively affects public health and safety. These elements work together to make for a better construction site in terms of health and safety, making for a more successful project overall.
Technical and functional constraints, privacy and legal barriers, economic and sustainability barriers, and organizational barriers were all noted in the research as obstacles to the widespread use of AI-based drones in the construction sector. According to the findings, clearing these hurdles is beneficial for introducing intelligent drones into the building sector. The research also concluded that public health and safety, environmental protection, quality control, and economic and sustainability benefit from using AI-based drones in the construction sector, contributing to the success of building projects. Consequently, if obstacles to using drones with artificial intelligence were removed, building projects would be more likely to be successful. Thus, it is reasonable to accept H1 as a whole, which asserts that the success of a building project improves when obstacles to the use of AI-based drones are removed.
6.1 Implications
Two basic types of ramifications may be drawn from the findings of this study: practical and theoretical. With the study’s findings in hand, construction companies may better plan for the successful introduction of AI-based drones into their operations. The research’s results may be used to inform the development of policies that promote and enable the usage of drones in the construction sector. The study offers a framework for construction companies to evaluate the costs and advantages of using drones equipped with artificial intelligence. The research emphasizes the need to tackle the numerous challenges of implementing AI-based drone deployment to boost public safety, environmental protection, and project quality and efficiency. By examining the effects of AI-based drone deployment across several facets of construction project management, the research gives a holistic knowledge of the influence of such implementation on project success. This research adds to our understanding of the challenges inherent in introducing drones powered by artificial intelligence to the building sector. The study shows the significance of thinking about technological, functional, legal, economic, environmental, and public safety considerations that may affect the success of AI-based drone applications in the construction business ( DeYoung, 2018 ; Li 2019 ; Rovira-Sugranes et al., 2022 ). The research offers complex data on how removing roadblocks to using AI-based drones may improve the success rate of building projects. In sum, this research sheds light on the possible upsides and downsides of using drones equipped with AI in the building business. Construction companies and politicians may use the results of this research to develop more efficient strategies for using AI-based drones, which can increase project efficiency, quality, safety, and environmental sustainability.
6.2 Managerial recommendations
This research provides evidence that using drones equipped with artificial intelligence may improve the outcome of building projects. Managers in the construction business should consider investing in this technology to enhance the quality of their projects. Legal and privacy issues, economic and sustainability problems, and scepticism about the technology are all highlighted in the research as potential roadblocks to adoption. Managers must endeavour to remove these obstacles using education and training, communication with stakeholders, and collaboration with technology vendors. The research concludes that drones powered by artificial intelligence may aid in quality control by doing airborne inspections, spotting possible issues and design faults, and compiling information about construction materials and machinery. While looking for ways to improve the quality of their projects, managers should consider deploying drones equipped with artificial intelligence for these tasks. The research concludes that drones powered by artificial intelligence may help ensure the public’s wellbeing by keeping tabs on building sites in real time, creating accurate 3D models and maps, and facilitating better communication and collaboration among the project’s many parties. Management should prioritize public health and safety by implementing safety rules and deploying AI-based drones for these tasks. The research concludes that drones powered by artificial intelligence may help safeguard the environment by looking for dangers on construction sites, taking thermal images, doing non-destructive tests, and checking for regulatory compliance. While planning a project, managers should think about how it will affect the environment and how they may deploy intelligent drones to lessen the damage. In conclusion, this research provides strong evidence that drones powered by artificial intelligence may significantly improve the outcome of building projects. Managers in the construction sector may set their businesses up for future success by investing in this technology, removing roadblocks to its adoption, and placing a premium on quality management, public health and safety, and environmental impact.
6.3 Limitations and future direction
Although the results are significant, it is essential to note the study’s limitations. First, this research is limited to discussing the challenges and opportunities of using drones powered by artificial intelligence in the building sector. More attention should be paid to other aspects that, including company culture and preparation, might impact the success of drone adoption. Second, the research was limited in scope since it only polled construction workers in a single nation; thus, its results cannot be extrapolated to the broader construction sector or other countries. Finally, the survey only looked at how experts felt about using drones with AI, and it did not look at how actual technology users felt about incorporating it into their projects.
Even with the earlier constructs, this work points toward some exciting avenues for further investigation. The construction sector might benefit from more studies into how company culture and preparedness affect the use of artificial intelligence-based drones. Second, the implications of AI-based drones in industries other than military and police operations, such as agriculture and shipping, might be the subject of future research. Finally, comparison research comparing construction industry experts with people who have used drones with artificial intelligence in building projects will illuminate the perception gap. Fourth, the impact of rules and laws on intelligent drones in a building might be studied. Lastly, further study might be done on the ethical and legal ramifications of using AI-powered drones in the building sector.
7 Conclusion
This research aimed to determine what was holding back the construction sector from adopting drones powered by artificial intelligence and what effect doing so would have on the success of building projects. The study’s goals were realized using a mixed-methods approach, which included a literature review, in-depth interviews, a pilot survey, and a substantial questionnaire. Barriers to the use of AI drones in construction were highlighted in the research. These included technical and functional hurdles, privacy and legal barriers, and economic and sustainability constraints. The analysis also indicated that construction projects are more likely to be successful if these obstacles can be removed. The study’s findings provide a complete model showing how the success of construction projects may be improved by eliminating barriers to using artificial intelligence-based drones in the industry. Public health and safety, quality management, environmental preservation, and the overall effectiveness of building projects were all shown to benefit from removing these obstacles. The research emphasizes the need to resolve the impediments to using AI-based drones in construction and the potential advantages that may be realized. Construction firms may boost their operations, productivity, and the likelihood of a successful project by identifying and eliminating these obstacles.
Data availability statement
The original contributions presented in the study are included in the article/Supplementary materials, further inquiries can be directed to the corresponding author.
Ethics statement
Ethical review and approval was not required for the study on human participants in accordance with the local legislation and institutional requirements. Written informed consent from the participants was not required to participate in this study in accordance with the national legislation and the institutional requirements.
Author contributions
Conceptualization, AW and HRA; methodology, AW, HRA, and IO; software, OB, BA, MA, and AB; validation, FA, SH, MAA, NHS, and OB; formal analysis, IO, AW, and OB; investigation, AW and HRA; resources, IO, SH, and MAA; data curation, AW and BA; writing–original draft preparation, AW, AA, and NFS; writing–review and editing, IO, AW, MA, FA, NHS, SA, and AB; visualization, IO, OB, BA, AA, and SA; supervision, IO; project administration, AW. All authors contributed to the article and approved the submitted version.
The researchers would like to acknowledge the Deanship of Scientific Research, Taif University for funding this work.
Conflict of interest
The authors declare that the research was conducted without any commercial or financial relationships that could be construed as a potential conflict of interest.
Publisher’s note
All claims expressed in this article are solely those of the authors and do not necessarily represent those of their affiliated organizations, or those of the publisher, the editors and the reviewers. Any product that may be evaluated in this article, or claim that may be made by its manufacturer, is not guaranteed or endorsed by the publisher.
Agapiou, A. (2020). Drones in construction: an international review of the legal and regulatory landscape. Proc. Inst. Civ. Eng. Manag. Procure. Law. 174, 118–125. doi:10.1680/jmapl.19.00041
CrossRef Full Text | Google Scholar
Albeaino, G., Eiris, R., Gheisari, M., and Issa, R. R. (2022). DroneSim: a VR-based flight training simulator for drone-mediated building inspections. Constr. Innov. 22, 831–848. doi:10.1108/CI-03-2021-0049
Alsamarraie, M., Ghazali, F., Hatem, Z. M., and Flaih, A. Y. (2022). A REVIEW ON THE BENEFITS, BARRIERS OF THE DRONE EMPLOYMENT IN THE CONSTRUCTION SITE. J. Teknol. 84, 121–131. doi:10.11113/jurnalteknologi.v84.17503
Amicone, D., Cannas, A., Marci, A., and Tortora, G. (2021). A smart capsule equipped with artificial intelligence for autonomous delivery of medical material through drones. Appl. Sci. 11, 7976. doi:10.3390/app11177976
Anunciado, D. M. (2016). The future of drones in construction . Des. Cost Data.
Google Scholar
Ateya, A. A., Algarni, A. D., Koucheryavy, A., and Soliman, N. F. (2022). Drone-based AI/IoT framework for monitoring, tracking and fighting pandemics. Comput. Mat. Contin. 71, 4677–4699. doi:10.32604/cmc.2022.021850
Beiki, H., and Mosavi, S. J. (2020). Silver nanoparticles-polyurea composite coatings on astm A194 steel: a study of corrosion behavior in chloride medium. J. Bio- Tribo-Corrosion 6, 66. doi:10.1007/s40735-020-00364-9
Bera, B., Wazid, M., Das, A. K., and Rodrigues, J. J. P. C. (2022). Securing internet of drones networks using AI-envisioned smart-contract-based blockchain. IEEE Internet Things Mag. 4, 68–73. doi:10.1109/iotm.001.2100044
Çetin, E., Barrado, C., and Pastor, E. (2020). Counter a drone in a complex neighborhood area by deep reinforcement learning. Sensors Switz. 20, 2320. doi:10.3390/s20082320
Charlesraj, V. P. C., and Rakshith, N. (2020). “Stakeholder perspectives on the adoption of drones in construction projects,” in Proceedings of the 37th International Symposium on Automation and Robotics in Construction, ISARC 2020: From Demonstration to Practical Use - To New Stage of Construction Robot . doi:10.22260/isarc2020/0168
Chen, L. K., Yuan, R. P., Ji, X. J., Lu, X. Y., Xiao, J., Tao, J. B., et al. (2021). Modular composite building in urgent emergency engineering projects: a case study of accelerated design and construction of Wuhan Thunder God Mountain/Leishenshan hospital to COVID-19 pandemic. Autom. Constr. 124, 103555. doi:10.1016/j.autcon.2021.103555
PubMed Abstract | CrossRef Full Text | Google Scholar
Chung, S. H., Sah, B., and Lee, J. (2020). Optimization for drone and drone-truck combined operations: a review of the state of the art and future directions. Comput. Oper. Res. 123, 105004. doi:10.1016/j.cor.2020.105004
Ciampa, E., De Vito, L., and Pecce, M. R. (2019). Practical issues on the use of drones for construction inspections. J. Phys. Conf. Ser. 1249, 012016. doi:10.1088/1742-6596/1249/1/012016
DeYoung, R. (2018). An industrial overview for the standardization of drones in construction. J. Chem. Inf. Model .
Dillow, C. (2016). A drone for every job site . Fortune.
Duda, M., Pach, J., and Lesiuk, G. (2019). Influence of polyurea composite coating on selected mechanical properties of AISI 304 steel. Mater. (Basel) 12, 3137. doi:10.3390/ma12193137
Elghaish, F., Matarneh, S., Talebi, S., Kagioglou, M., Hosseini, M. R., and Abrishami, S. (2021). Toward digitalization in the construction industry with immersive and drones technologies: a critical literature review. Smart Sustain. Built Environ. 10, 345–363. doi:10.1108/SASBE-06-2020-0077
Entrop, A. G., and Vasenev, A. (2017). “Infrared drones in the construction industry: designing a protocol for building thermography procedures,” in Energy procedia . doi:10.1016/j.egypro.2017.09.636
Feder, J. (2020). Drones move from “nice to have” to strategic resources for projects. J. Pet. Technol. 72, 29–30. doi:10.2118/1220-0029-jpt
Feng, J., Liao, L. M., Chen, L., Xiao, C. J., Wang, S. F., and Li, H. (2013). “Research on the formula of polyaspartic ester polyurea concrete coating and its application,” in Applied mechanics and materials . doi:10.4028/www.scientific.net/AMM.423-426.1159
Firth, N. (2018). Drones control construction gear. New Sci. 237, 10. doi:10.1016/s0262-4079(18)30464-0
Ganesan, R., Raajini, X. M., Nayyar, A., Sanjeevikumar, P., Hossain, E., and Ertas, A. H. (2020). Bold: bio-inspired optimized leader election for multiple drones. Sensors Switz. 20, 3134. doi:10.3390/s20113134
Gibbin, R. V., Sigahi, T. F. A. C., Pinto, J. D. S., Rampasso, I. S., and Anholon, R. (2023). Thematic evolution and trends linking sustainability and project management: scientific mapping using SciMAT. J. Clean. Prod. 414, 137753. doi:10.1016/j.jclepro.2023.137753
Goessens, S., Mueller, C., and Latteur, P. (2018a). Feasibility study for drone-based masonry construction of real-scale structures. Autom. Constr. 94, 458–480. doi:10.1016/j.autcon.2018.06.015
Goessens, S., Rogeau, N., Beusscher, G. D., Mueller, C., and Latteur, P. (2018b). Parametric design of drone-compatible architectural timber structures . Iass.
Golpîra, H. (2021). Application of mathematics and optimization in construction project management . doi:10.1007/978-3-030-81123-5
Greene, C. E., and Myers, J. J. (2013). Flexural and shear behavior of reinforced concrete members strengthened with a discrete fiber-reinforced polyurea system. J. Compos. Constr. 17, 108–116. doi:10.1061/(asce)cc.1943-5614.0000308
Gupta, R., Kumari, A., and Tanwar, S. (2021). Fusion of blockchain and artificial intelligence for secure drone networking underlying 5G communications. Trans. Emerg. Telecommun. Technol. 32. doi:10.1002/ett.4176
Hatfield, M. C., Cahill, C. F., and Webley, P. W. (2020). “Drone camp: construction and racing for Pre-college students,” in ASEE Annual Conference and Exposition, Conference Proceedings . doi:10.18260/1-2--34482
Höche, D., Weber, W. E., Gazenbiller, E., Gavras, S., Hort, N., and Dieringa, H. (2021). Novel magnesium based materials: are they reliable drone construction materials? A mini review. Front. Mat. 8. doi:10.3389/fmats.2021.575530
Ichimura, Y., Dalaklis, D., Kitada, M., and Christodoulou, A. (2022). Shipping in the era of digitalization: mapping the future strategic plans of major maritime commercial actors. Digit. Bus. 2, 100022. doi:10.1016/j.digbus.2022.100022
Ikeda, T., Bando, N., and Yamada, H. (2021). Semi-automatic visual support system with drone for teleoperated construction robot. J. Robot. Mechatronics. 33, 313–321. doi:10.20965/jrm.2021.p0313
Irizarry, J., Gheisari, M., and Walker, B. N. (2012). Usability assessment of drone technology as safety inspection tools. Electron. J. Inf. Technol. Constr .
Islam, A., Rahim, T., Masuduzzaman, Muhammad, and Shin, S. Y. (2021). A blockchain-based artificial intelligence-empowered contagious pandemic situation supervision scheme using internet of drone things. IEEE Wirel. Commun. 28, 166–173. doi:10.1109/MWC.001.2000429
Kardasz, P., and Doskocz, J. (2016). Drones and possibilities of their using. J. Civ. Environ. Eng. 6. doi:10.4172/2165-784x.1000233
Khalid, M., Namian, M., and Massarra, C. (2021). The dark side of the drones: a review of emerging safety implications in construction . doi:10.29007/x3vt
Kim, D., Kang, W. J., Koo, Y., Bang, J., Son, K., Hostallero, D., et al. (2017). AI-based drone object tracking system: design and implementation. J. Korean Inst. Commun. Inf. Sci. 42, 2391–2401. doi:10.7840/kics.2017.42.12.2391
Kim, J., Lim, J., Lim, H. C., and Kim, D. Y. (2022). Improving sustainable project success strategies focused on cost and schedule for electrical construction project management. Sustain 14, 2653. doi:10.3390/su14052653
Kisi, K. P., Kayastha, R., Pradhananga, N., Shrestha, J., and Khoteja, D. (2020). Construction research congress 2020: computer applications. Constr. Res. Congr .
Kitjacharoenchai, P., Min, B. C., and Lee, S. (2020). Two echelon vehicle routing problem with drones in last mile delivery. Int. J. Prod. Econ. 225, 107598. doi:10.1016/j.ijpe.2019.107598
Kubo, H., and Okoso, K. (2019). “Business ecosystem strategy using new hydroponic culture method,” in PICMET 2019 - Portland International Conference on Management of Engineering and Technology: Technology Management in the World of Intelligent Systems ( Proceedings ). doi:10.23919/PICMET.2019.8893714
Lahmeri, M. A., Kishk, M. A., and Alouini, M. S. (2021). Artificial intelligence for UAV-enabled wireless networks: a survey. IEEE Open J. Commun. Soc. 2, 1015–1040. doi:10.1109/OJCOMS.2021.3075201
Latteur, P., Goessens, S., and Mueller, C. (2016). Masonry construction with drones. Proc. IASS Annu. Symp .
Lawani, K., Hare, B., Cameron, I., Homatash, H., and Campbell, J. (2022). Designing drone game for construction site inspection. Front. Built Environ. 7. doi:10.3389/fbuil.2021.771703
Lee, D. J., Jung, G. H., Kwon, H. A., and Yang, S. W. (2021). Digital twin-based Anti-drone system construction plan. J. Korean Inst. Def. Technol. 3, 001–006. doi:10.52682/jkidt.2021.3.3.1
Lee, H. S., Park, H., and Kim, J. B. (2019). Development plan of drone-bot network system. Int. J. Innov. Technol. Explor. Eng .
Lee, S., and Kwon, Y. (2020). Safe landing of drone using AI-based obstacle avoidance. Int. J. Mech. Eng. Robot. Res. , 1495–1501. doi:10.18178/ijmerr.9.11.1495-1501
Lee, S. B., Song, M., Kim, S., and Won, J. H. (2020). Change monitoring at expressway infrastructure construction sites using drone. Sensors Mater 32, 3923. doi:10.18494/SAM.2020.2971
Lewandowski, K. (2021). Sustainable usage of freight drones in city centers, proposition of regulations for safe usage of drones. Sustain 13, 8634. doi:10.3390/su13158634
Li, B., Zhang, Z., Wang, X., and Liu, X. (2019). Investigation on the debonding failure model of anchored polyurea coating under a high-velocity water flow and its application. Sustain 11, 1261. doi:10.3390/su11051261
Li, R. Y. M. (2019). “Automated and intelligent tools in the construction industry,” in Construction safety informatics . doi:10.1007/978-981-13-5761-9_9
Li, Y., and Liu, C. (2019). Applications of multirotor drone technologies in construction management. Int. J. Constr. Manag. 19, 401–412. doi:10.1080/15623599.2018.1452101
Lin, A. (2017). Use of drone technology on commercial construction projects . Reseach Gate .
Liu, Q., Guo, B., Chen, P., Su, J., Arab, A., Ding, G., et al. (2021). Investigating ballistic resistance of CFRP/polyurea composite plates subjected to ballistic impact. Thin-Walled Struct. 166, 108111. doi:10.1016/j.tws.2021.108111
Loveless, C. (2018). Drones in construction. SSRN Electron. J . doi:10.2139/ssrn.3111338
Mahajan, G. (2021). Applications of drone technology in construction industry: a study 2012-2021. Int. J. Eng. Adv. Technol. 11, 224–239. doi:10.35940/ijeat.a3165.1011121
Makadsi, I. (2019). An exploratory study of drones used for safety purposes on outdoor construction sites. KTH Ind. Eng. Manag .
Mishra, P. K. (2019). A view of the future: drones in construction industry. Int. J. Manag. IT .
Mt Hardjo, M. F. R., Wahyuni, A., and Rahim, M. R. (2020). Gambaran keselamatan pekerja menggunakan teknologi pemantauan drone pada proyek konstruksi PT. X makassar. Hasanuddin J. Public Heal 1, 142–151. doi:10.30597/hjph.v1i2.9327
Oudjehane, A., Baker, T., and Moeini, S. (2019). “The role and value of integrating AI, drones and associate technologies in construction projects,” in Proceedings, Annual Conference - Canadian Society for Civil Engineering .
Pawar, M. A. (2020). Farmatron - pest detection and treatment using AI based drone. Int. J. Res. Appl. Sci. Eng. Technol. 8, 19–24. doi:10.22214/ijraset.2020.5004
Pereira da Silva, N., and Eloy, S. (2021). “Robotic construction: robotic fabrication experiments for the building construction industry,” in Advances in science, technology and innovation . doi:10.1007/978-3-030-35533-3_14
Rohan, A., Rabah, M., and Kim, S. H. (2019). Convolutional neural network-based real-time object detection and tracking for parrot AR drone 2. IEEE Access 7, 69575–69584. doi:10.1109/ACCESS.2019.2919332
Rovira-Sugranes, A., Razi, A., Afghah, F., and Chakareski, J. (2022). A review of AI-enabled routing protocols for UAV networks: trends, challenges, and future outlook. Ad Hoc Netw. 130, 102790. doi:10.1016/j.adhoc.2022.102790
Sawhney, A., Riley, M., and Irizarry, J. (2020). Introduction and overview of construction 4.0, CPS, digital ecosystem, and innovation .
Scher, C. L., Griffoul, E., and Cannon, C. H. (2019). Drone-based photogrammetry for the construction of high-resolution models of individual trees. Trees - Struct. Funct. 33, 1385–1397. doi:10.1007/s00468-019-01866-x
To, A., Liu, M., Hazeeq Bin Muhammad Hairul, M., Davis, J. G., Lee, J. S. A., Hesse, H., et al. (2021). “Drone-based AI and 3D reconstruction for digital twin augmentation,” in Lecture notes in computer science (including subseries lecture notes in artificial intelligence and lecture notes in bioinformatics . doi:10.1007/978-3-030-77626-8_35
Ullo, S. L., and Sinha, G. R. (2021). Advances in IoT and smart sensors for remote sensing and agriculture applications. Remote Sens. 13, 2585. doi:10.3390/rs13132585
Umar, T. (2021). Applications of drones for safety inspection in the Gulf Cooperation Council construction. Eng. Constr. Archit. Manag. 28, 2337–2360. doi:10.1108/ECAM-05-2020-0369
Urgessa, G. S., and Esfandiari, M. (2018). “Review of polymer coatings used for blast strengthening of reinforced concrete and masonry structures,” in International congress on polymers in concrete ( ICPIC 2018 ). doi:10.1007/978-3-319-78175-4_91
Wazid, M., Bera, B., Mitra, A., Das, A. K., and Ali, R. (2020). “Private blockchain-envisioned security framework for AI-enabled IoT-based drone-aided healthcare services,” in DroneCom 2020 - Proceedings of the 2nd ACM MobiCom Workshop on Drone Assisted Wireless Communications for 5G and Beyond . doi:10.1145/3414045.3415941
Woo, J., Shin, S., Asutosh, A. T., Li, J., and Kibert, C. J. (2021). “An overview of state-of-the-art technologies for data-driven construction,” in Lecture notes in civil engineering . doi:10.1007/978-3-030-51295-8_94
Yang, Y., Chan, A. P. C., Shan, M., Gao, R., Bao, F., Lyu, S., et al. (2021). Opportunities and challenges for construction health and safety technologies under the COVID-19 pandemic in Chinese construction projects. Int. J. Environ. Res. Public Health 18, 13038. doi:10.3390/ijerph182413038
Yi, W., and Sutrisna, M. (2021). Drone scheduling for construction site surveillance. Comput. Civ. Infrastruct. Eng. 36, 3–13. doi:10.1111/mice.12593
Yigitcanlar, T., Kankanamge, N., Regona, M., Maldonado, A. R., Rowan, B., Ryu, A., et al. (2020). Artificial intelligence technologies and related urban planning and development concepts: how are they perceived and utilized in Australia? J. Open Innov. Technol. Mark. Complex 6, 187. doi:10.3390/joitmc6040187
Yıldız, S., Kıvrak, S., and Arslan, G. (2021). Using drone technologies for construction project management: a narrative review. J. Constr. Eng. Manag. Innov. 4, 229–244. doi:10.31462/jcemi.2021.04229244
Yıldızel, S. A., and Calış, G. (2019). Unmanned aerial vehicles for civil engineering: current practises and regulations. Eur. J. Sci. Technol. , 925–932. doi:10.31590/ejosat.565499
Zaychenko, I., Smirnova, A., and Borremans, A. (2018). “Digital transformation: the case of the application of drones in construction,” in MATEC web of conferences . doi:10.1051/matecconf/201819305066
Zhang, G., Soria Zurita, N. F., Stump, G., Song, B., Cagan, J., and McComb, C. (2021). Data on the design and operation of drones by both individuals and teams. Data Br. 36, 107008. doi:10.1016/j.dib.2021.107008
Keywords: AI-based drone, construction industry, construction project success, sustainable construction, modelling
Citation: Waqar A, Othman I, Hamah Sor N, Alshehri AM, Almujibah HR, Alotaibi BS, Abuhussain MA, Bageis AS, Althoey F, Hayat S, Benjeddou O, Alsulamy SH and Aljarbou M (2023) Modeling relation among implementing AI-based drones and sustainable construction project success. Front. Built Environ. 9:1208807. doi: 10.3389/fbuil.2023.1208807
Received: 19 April 2023; Accepted: 18 October 2023; Published: 02 November 2023.
Reviewed by:
Copyright © 2023 Waqar, Othman, Hamah Sor, Alshehri, Almujibah, Alotaibi, Abuhussain, Bageis, Althoey, Hayat, Benjeddou, Alsulamy and Aljarbou. This is an open-access article distributed under the terms of the Creative Commons Attribution License (CC BY). The use, distribution or reproduction in other forums is permitted, provided the original author(s) and the copyright owner(s) are credited and that the original publication in this journal is cited, in accordance with accepted academic practice. No use, distribution or reproduction is permitted which does not comply with these terms.
*Correspondence: Nadhim Hamah Sor, [email protected]
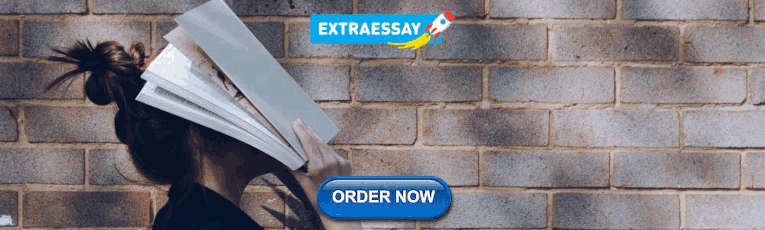
IMAGES
VIDEO
COMMENTS
I. Introduction: the rapid growth of drone technology Unmanned aerial vehicles, commonly referred to as "drones", Footnote 1 offer enormous potential for developing innovative civil applications in a wide variety of sectors. Footnote 2 According to data gathered by the Commission of the European Union (EU), within twenty years the European drone sector alone is expected to employ directly ...
Artificial Intelligence (AI) offers numerous opportunities to improve military Intelligence, Surveillance, and Reconnaissance operations. And, modern militaries recognize the strategic value of reducing civilian harm. Grounded in these two assertions we focus on the transformative potential that AI ISR systems have for improving the respect for and protection of humanitarian relief operations ...
Abstract. The rapid proliferation of a new generation of artificial intelligence (AI)-augmented and -enabled autonomous weapon systems (AWS), most notably drones used in swarming tactics, could have a significant impact on deterrence, nuclear security, escalation and strategic stability in future warfare.
With the advancement of new technologies, drones are now extensively employed in combat operations, unlike surveillance, where it is causing greater violations than expected. Drones reduce human losses and ensure precision target strikes, say advocates. Most modern conflicts occur in metropolitan areas, such as Libya, Syria, Afghanistan, and Ukraine, where civilians and military forces coexist ...
These kinds of AI decision support systems (AI-DSS) are computerised tools that use AI software to display, synthesise and/or analyse data and in some cases make recommendations - even predictions - in order to aid human decision-making in war. The advantages of AI-DSS are often framed in terms of increased situational awareness and faster ...
Artificial intelligence and machine learning in armed conflict: A human-centred approach Note: This is an edited version of a paper published by the ICRC in June 2019. 1. Introduction At a time of increasing conflict and rapid technological change, the International Committee of the Red Cross (ICRC) needs both to understand the impact of new
Drone Wars is an extensive anthology on the current debates surrounding the use of armed aerial drones in contemporary conflicts. The editors compiled a list of twenty-two essays and divided them into four major topic areas: drones on the ground, drones and the law of war, drones and policy, and drones and the future of war.
Keywords: Drone, Security, Artificial Intelligence, Swarming, Surveillance, Suicide Drones, Networks, Autonomous Drones, Lethal Autonomous Weapons (LAWs) Introduction There is no one standard when it comes to the classification of Unmanned Aircraft Systems (UASs), sometimes called Unmanned Aerial Vehicles (UAVs). Defence agencies have their own
Drones and the Future of Armed Conflict: Ethical, Legal and Strategic Implications, David Cortright, Rachel Fairhurst, and Kristen Wall, eds. (Chicago: ... Recommended articles lists articles that we recommend and is powered by our AI driven recommendation engine. Cited by lists all citing articles based on Crossref citations.
The paper presents the possibility of autonomous use of drones with artificial intelligence in combat and non-combat operations of the army. Drones supported by GIS, C5IRS (command, control ...
The use of drones (or unmanned aerial vehicles, UVAs) in humanitarian action has emerged rapidly in the last decade and continues to expand. These so-called 'humanitarian drones' represent the first wave of robotics applied in the humanitarian and development contexts, providing critical information through mapping of crisis-affected areas and timely delivery of aid supplies to populations ...
On the one hand, the war confirmed scholarly expectations about large drones. Far from a drone revolution in military affairs, large drones remain vulnerable to air defenses and have utility primarily in asymmetric conflicts wherein control of skies is uncontested (Horowitz et al., Citation 2016). In addition, rich scholarship on the legal and ...
AI-enabled weapons have similar potential for catastrophic results and should follow the same rule for all drone operations. Reduce the accountability gap. Increasing autonomy in drone warfare will make strikes more unpredictable, resulting in mistakes that cannot be attributed to any particular individual.
This article provides a holistic examination of the international legal frameworks which regulate targeted killings by drones. The article argues that for a particular drone strike to be lawful, it must satisfy the legal requirements under all applicable international legal regimes, namely: the law regulating the use of force (ius ad bellum); international humanitarian law and international ...
Abstract. AI-based, autonomous weapon systems (AWS) have the potential of weapons of mass destruction and thereby massively add to the intensifying dialectic of fear between ground and space and the pervasive mass human vulnerability of being tracked and targeted from above. Nevertheless, the dangerous effects of the proliferation of AWS have ...
There are two broad - and distinct - areas of application of AI and machine learning in which the ICRC has a particular interest: first, its use in the conduct of warfare or in other situations of violence;1 and second, its use in humanitarian action to assist and protect the victims of armed conflict.2 This paper sets out the ICRC's ...
This research paper examines the impact of unmanned aerial vehicles (UAVs), notably drones, on contemporary society and analyses the legal challenges and potential human rights and privacy ...
Additionally, AI is used in lethal autonomous weapons systems (LAWS), which can locate, target, and kill without human involvement. LAWS, for example, could be affixed to an autonomous drone or tank and use recognition technology to identify enemy targets. When a target—say, Mohsen Fakhrizadeh—is located, a weapon could be programmed to fire.
On 24 February 2022, when Russia invaded Ukraine, the art of drone warfare entered a different phase. Thousands of drones are being used on both sides in the war, with Russia using the Orlan-10 and Ukraine relying heavily on the Turkish-made Bayraktar TB2; the latter has become legendary in both Turkey and Ukraine, and it is often the celebrated subject of viral social media posts, including ...
Connecting independent networks of drones (that are expected to exist in the future) is yet to appear in the literature, though some elements of this are developing such as using drones as nodes of a multi-drone communication network (Kuleshov et al., 2018; Smith et al., 2018; Xiao and Guo, 2019). Though note, these methods are only for local ...
Connecting independent networks of drones (that are expected to exist in the future) is yet to appear in the literature, though some elements of this are developing such as using drones as nodes of a multi-drone communication network (Kuleshov et al., 2018; Smith et al., 2018; Xiao and Guo, 2019). Though note, these methods are only for local ...
Ethical analysis of armed drones has to date focused heavily on their use in foreign wars or counterterrorism operations, but it is important also to consider the potential use of armed drones in domestic law enforcement. Governments around the world are already making drones available to police for purposes including border control, criminal ...
Project failure is a persistent challenge in the construction industry, rendering it one of the most demanding sectors. Many obstacles, including safety concerns, quality management issues, environmental preservation challenges, economic sustainability, privacy constraints, and legal regulations, weigh heavily on construction projects. However, a beacon of hope emerges in AI-powered drones ...