- Email Alert
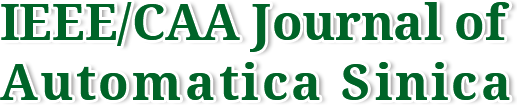
论文 全文 图 表 新闻
- Abstracting/Indexing
- Journal Metrics
- Current Editorial Board
- Early Career Advisory Board
- Previous Editor-in-Chief
- Past Issues
- Current Issue
- Special Issues
- Early Access
- Online Submission
- Information for Authors
- Share facebook twitter google linkedin
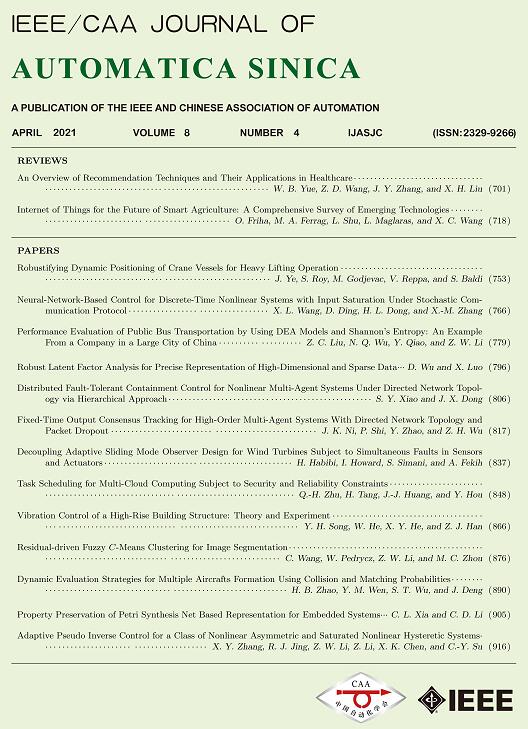
IEEE/CAA Journal of Automatica Sinica
- JCR Impact Factor: 11.8 , Top 4% (SCI Q1) CiteScore: 17.6 , Top 3% (Q1) Google Scholar h5-index: 77, TOP 5
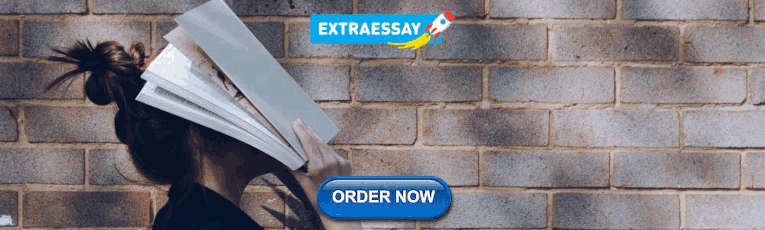
Internet of Things for the Future of Smart Agriculture: A Comprehensive Survey of Emerging Technologies
Doi: 10.1109/jas.2021.1003925.
- Othmane Friha 1 , ,
- Mohamed Amine Ferrag 2 , ,
- Lei Shu 3, 4 , , ,
- Leandros Maglaras 5 , ,
- Xiaochan Wang 6 ,
Networks and Systems Laboratory, University of Badji Mokhtar-Annaba, Annaba 23000, Algeria
Department of Computer Science, Guelma University, Gulema 24000, Algeria
College of Engineering, Nanjing Agricultural University, Nanjing 210095, China
School of Engineering, University of Lincoln, Lincoln LN67TS, UK
School of Computer Science and Informatics, De Montfort University, Leicester LE1 9BH, UK
Department of Electrical Engineering, Nanjing Agricultural University, Nanjing 210095, China
Othmane Friha received the master degree in computer science from Badji Mokhtar-Annaba University, Algeria, in 2018. He is currently working toward the Ph.D. degree in the University of Badji Mokhtar-Annaba, Algeria. His current research interests include network and computer security, internet of things (IoT), and applied cryptography
Mohamed Amine Ferrag received the bachelor degree (June, 2008), master degree (June, 2010), Ph.D. degree (June, 2014), HDR degree (April, 2019) from Badji Mokhtar-Annaba University, Algeria, all in computer science. Since October 2014, he is a Senior Lecturer at the Department of Computer Science, Guelma University, Algeria. Since July 2019, he is a Visiting Senior Researcher, NAULincoln Joint Research Center of Intelligent Engineering, Nanjing Agricultural University. His research interests include wireless network security, network coding security, and applied cryptography. He is featured in Stanford University’s list of the world’s Top 2% Scientists for the year 2019. He has been conducting several research projects with international collaborations on these topics. He has published more than 60 papers in international journals and conferences in the above areas. Some of his research findings are published in top-cited journals, such as the IEEE Communications Surveys and Tutorials , IEEE Internet of Things Journal , IEEE Transactions on Engineering Management , IEEE Access , Journal of Information Security and Applications (Elsevier), Transactions on Emerging Telecommunications Technologies (Wiley), Telecommunication Systems (Springer), International Journal of Communication Systems (Wiley), Sustainable Cities and Society (Elsevier), Security and Communication Networks (Wiley), and Journal of Network and Computer Applications (Elsevier). He has participated in many international conferences worldwide, and has been granted short-term research visitor internships to many renowned universities including, De Montfort University, UK, and Istanbul Technical University, Turkey. He is currently serving on various editorial positions such as Editorial Board Member in Journals (Indexed SCI and Scopus) such as, IET Networks and International Journal of Internet Technology and Secured Transactions (Inderscience Publishers)
Lei Shu (M’07–SM’15) received the B.S. degree in computer science from South Central University for Nationalities in 2002, and the M.S. degree in computer engineering from Kyung Hee University, South Korea, in 2005, and the Ph.D. degree from the Digital Enterprise Research Institute, National University of Ireland, Ireland, in 2010. Until 2012, he was a Specially Assigned Researcher with the Department of Multimedia Engineering, Graduate School of Information Science and Technology, Osaka University, Japan. He is currently a Distinguished Professor with Nanjing Agricultural University and a Lincoln Professor with the University of Lincoln, U.K. He is also the Director of the NAU-Lincoln Joint Research Center of Intelligent Engineering. He has published over 400 papers in related conferences, journals, and books in the areas of sensor networks and internet of things (IoT). His current H-index is 54 and i10-index is 197 in Google Scholar Citation. His current research interests include wireless sensor networks and IoT. He has also served as a TPC Member for more than 150 conferences, such as ICDCS, DCOSS, MASS, ICC, GLOBECOM, ICCCN, WCNC, and ISCC. He was a Recipient of the 2014 Top Level Talents in Sailing Plan of Guangdong Province, China, the 2015 Outstanding Young Professor of Guangdong Province, and the GLOBECOM 2010, ICC 2013, ComManTel 2014, WICON 2016, SigTelCom 2017 Best Paper Awards, the 2017 and 2018 IEEE Systems Journal Best Paper Awards, the 2017 Journal of Network and Computer Applications Best Research Paper Award, and the Outstanding Associate Editor Award of 2017, and the 2018 IEEE ACCESS. He has also served over 50 various Co-Chair for international conferences/workshops, such as IWCMC, ICC, ISCC, ICNC, Chinacom, especially the Symposium Co-Chair for IWCMC 2012, ICC 2012, the General Co-Chair for Chinacom 2014, Qshine 2015, Collaboratecom 2017, DependSys 2018, and SCI 2019, the TPC Chair for InisCom 2015, NCCA 2015, WICON 2016, NCCA 2016, Chinacom 2017, InisCom 2017, WMNC 2017, and NCCA 2018
Leandros Maglaras (SM’15) received the B.Sc. degree from Aristotle University of Thessaloniki, Greece, in 1998, M.Sc. in industrial production and management from University of Thessaly in 2004, and M.Sc. and Ph.D. degrees in electrical & computer engineering from University of Volos in 2008 and 2014, respectively. He is the Head of the National Cyber Security Authority of Greece and a Visiting Lecturer in the School of Computer Science and Informatics at the De Montfort University, U.K. He serves on the Editorial Board of several International peer-reviewed journals such as IEEE Access , Wiley Journal on Security & Communication Networks , EAI Transactions on e-Learning and EAI Transactions on Industrial Networks and Intelligent Systems . He is an author of more than 80 papers in scientific magazines and conferences and is a Senior Member of IEEE. His research interests include wireless sensor networks and vehicular ad hoc networks
Xiaochan Wang is currently a Professor in the Department of Electrical Engineering at Nanjing Agricultural University. His main research fields include intelligent equipment for horticulture and intelligent measurement and control. He is an ASABE Member, and the Vice Director of CSAM (Chinese Society for Agricultural Machinery), and also the Senior Member of Chinese Society of Agricultural Engineering. He was awarded the Second Prize of Science and Technology Invention by the Ministry of Education (2016) and the Advanced Worker for Chinese Society of Agricultural Engineering (2012), and he also gotten the “Blue Project” in Jiangsu province young and middle-aged academic leaders (2010)
- Corresponding author: Lei Shu, e-mail: [email protected]
- Revised Date: 2020-11-25
- Accepted Date: 2020-12-30
- Agricultural internet of things (IoT) ,
- internet of things (IoT) ,
- smart agriculture ,
- smart farming ,
- sustainable agriculture
Proportional views
通讯作者: 陈斌, [email protected].
沈阳化工大学材料科学与工程学院 沈阳 110142
Figures( 12 ) / Tables( 9 )
Article Metrics
- PDF Downloads( 2059 )
- Abstract views( 10829 )
- HTML views( 1389 )
- We review the emerging technologies used by the Internet of Things for the future of smart agriculture.
- We provide a classification of IoT applications for smart agriculture into seven categories, including, smart monitoring, smart water management, agrochemicals applications, disease management, smart harvesting, supply chain management, and smart agricultural practices.
- We provide a taxonomy and a side-by-side comparison of the state-of-the-art methods toward supply chain management based on the blockchain technology for agricultural IoTs.
- We highlight open research challenges and discuss possible future research directions for agricultural IoTs.
- Copyright © 2022 IEEE/CAA Journal of Automatica Sinica
- 京ICP备14019135号-24
- E-mail: [email protected] Tel: +86-10-82544459, 10-82544746
- Address: 95 Zhongguancun East Road, Handian District, Beijing 100190, China
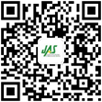
Export File

- Figure 1. The four agricultural revolutions
- Figure 2. Survey structure
- Figure 3. IoT-connected smart agriculture sensors enable the IoT
- Figure 4. The architecture of a typical IoT sensor node
- Figure 5. Fog computing-based agricultural IoT
- Figure 6. SDN/NFV architecture for smart agriculture
- Figure 7. Classification of IoT applications for smart agriculture
- Figure 8. Greenhouse system [ 101 ]
- Figure 9. Aerial-ground robotics system [ 67 ]
- Figure 10. Photovoltaic agri-IoT schematic diagram [ 251 ]
- Figure 11. Smart dairy farming system [ 254 ]
- Figure 12. IoT-based solar insecticidal lamp [ 256 ], [ 257 ]

An official website of the United States government
The .gov means it’s official. Federal government websites often end in .gov or .mil. Before sharing sensitive information, make sure you’re on a federal government site.
The site is secure. The https:// ensures that you are connecting to the official website and that any information you provide is encrypted and transmitted securely.
- Publications
- Account settings
Preview improvements coming to the PMC website in October 2024. Learn More or Try it out now .
- Advanced Search
- Journal List
- Sensors (Basel)

A Systematic Review of IoT Solutions for Smart Farming
Emerson navarro.
1 School of Technology and Management, Computer Science and Communication Research Centre, Polytechnic Institute of Leiria, Campus 2, Morro do Lena—Alto do Vieiro, Apartado 4163, 2411-901 Leiria, Portugal; tp.airielpi.ym@3320812 (E.N.); [email protected] (N.C.)
António Pereira
2 INOV INESC Inovação, Institute of New Technologies, Leiria Office, Campus 2, Morro do Lena—Alto do Vieiro, Apartado 4163, 2411-901 Leiria, Portugal
The world population growth is increasing the demand for food production. Furthermore, the reduction of the workforce in rural areas and the increase in production costs are challenges for food production nowadays. Smart farming is a farm management concept that may use Internet of Things (IoT) to overcome the current challenges of food production. This work uses the preferred reporting items for systematic reviews (PRISMA) methodology to systematically review the existing literature on smart farming with IoT. The review aims to identify the main devices, platforms, network protocols, processing data technologies and the applicability of smart farming with IoT to agriculture. The review shows an evolution in the way data is processed in recent years. Traditional approaches mostly used data in a reactive manner. In more recent approaches, however, new technological developments allowed the use of data to prevent crop problems and to improve the accuracy of crop diagnosis.
1. Introduction
The challenge of food production in the 21st century is an increasingly relevant theme as population growth increases year after year. It is estimated that by 2050 the world will have between 9.4 and 10.1 billion people who depend on the world’s biodiversity to live, increasing the demand for dedicated food production areas—specifically for planting and livestock [ 1 ]. Environmental changes caused by human beings could potentially cause conditions in which the development of new crops is not possible. Likewise, the growing urbanization decreases labor in areas typically involved in food production, increases costs and reduces the productive capacity of the sector [ 2 ]. In view of this, smart farming is a new farm management concept that employs techniques and technologies at various levels and scales of agricultural production, enabling to overcome the challenges in food production demands and reduction in the workforce [ 3 , 4 ]. For example smart farming may use different types of sensors to collect data (e.g., temperature, humidity, light, pressure, presence, etc.), communication networks to send and receive data which is then managed and analyzed by management information systems and data analysis solutions [ 5 ]. This system of interconnected devices is commonly referred to as internet of things (IoT) [ 6 ]. The use of the data provided by smart farming helps boosting productivity and minimizing waste by allowing necessary actions to be carried out at the right time, quantity and place [ 7 ].
Moreover, recent technological developments in areas relevant to IoT facilitates an easier adoption and use of smart farming with IoT [ 8 ]. Such technological developments include, for example, network communications, reduction of hardware size, optimization of power consumption and devices cheapening. Furthermore, the World’s largest agricultural producers are promoting the usage of IoT in smart farming by creating incentive programs and public policies to fund research and training [ 9 , 10 ].
Several reviews have been published on IoT solutions for smart agriculture in recent years which denotes that this research field is being constantly receiving new contributions and constant improvement. Existing reviews usually focus on topics like network technologies, embedded system platforms, unmanned aerial vehicles (UAV) devices, network protocols and topologies and enabling cloud platforms. For instance [ 11 ] focuses on arable farming from year 2008 to 2018 and surveys communication technologies and protocols, the generation and analysis of data, IoT architectures and applications and highlights the challenges and future directions related with the application of IoT technologies on arable farming. Review [ 12 ] presents technologies used for communication and data collection within IoT solutions for smart farming as well as several cloud-based IoT platforms used for IoT solutions for smart farming. Additionally, authors present several use cases for the identified applications of IoT for smart farming. Review [ 13 ] presents a systematic review of papers published between 2006 and 2016 and classifies these papers in application domains, such as monitoring, controlling, logistic and prediction. Within these domains, authors also identified the data visualization strategies and the technologies used for communication and edge computing. Review [ 14 ] presents a review of papers published between 2010 and 2016. The authors rely on an IoT architecture with three layers (perception, network, application) to analyze the reviewed papers in terms of perception devices, network technologies and applications. With this, they identify embedded platforms and communication technologies used in IoT solutions as well as the application of such IoT solutions. Finally, [ 15 ] reviewed papers published between 2010 and 2015 and presents a state-of-the-art of IoT solutions for smart farming and smart agriculture. Authors relied on an IoT architecture with three layers (perception, network and application) to analyze the application of sensor and actuator devices and communication technologies within several farming domains, such as agriculture, food consumption, livestock farming, among others.
This study aims to identify how IoT is used with smart farming by (i) presenting a systematic review of the state of the art of the IoT adoption in smart agriculture and (ii) identifying the most commonly used hardware, platforms, network protocols and technologies and their applicability to the proposed solutions. To give a more up-to-date view to the reader about the smart agriculture research field, this review article surveys literature until year 2020 and evaluates the acceptance of (new) IT technologies, such as big data, computer vision, artificial intelligence, blockchain and fuzzy logic in the smart agriculture field. This review complements the analysis already carried out by the academy by performing a comprehensive review of the state-of-the-art of IoT in smart farming. To do so, we rely on an IoT architecture with 4 layers (perception, network, processing and application) to identify the technologies that enable IoT in smart farming. From this approach, the present work identifies an increasing use of modern techniques and technologies for processing the collected data in recent years, such as big data, machine learning, computer vision and blockchain. Moreover, this review contributes to the existent literature by reporting a change in the treatment of data in recent works: while previous work showed that the majority of decision support systems used simple processing mechanisms to handle data collected in real-time, more recent work showed an increasing number of management systems that use complementary technologies that rely on cloud and big data computing for processing large amounts of data. In terms of research domain, this article addresses the agriculture economic sector, including indoor and outdoor agriculture (greenhouse, hydroponics, crop beds, pots, orchards, permanent crops, and arable lands). However, the livestock farming (both indoors and outdoors) was left out because, due to its specificities like impact on nature, associated mobility, different species (mammals, birds, fishing) deserves a separated and specific review.
For this purpose, the remaining work is organized as follows: Section 2 presents the introductory concepts discussed in this study, Section 3 presents the methodology followed for the systematic review, Section 4 details the results obtained, Section 5 presents the considerations and Section 6 presents the conclusions.
2. Concepts
To better understand the questions related to the use of IoT in agriculture during the 21st century, it is important go deeper in some fundamental concepts.
2.1. Smart Farming
Smart farming can be defined as the application of supplementary technologies to agricultural production techniques to help minimize waste and boost productivity.
For such, intelligent farms use technological resources that help in various stages of the production process, such as monitoring of plantations, soil management, irrigation, pest control, delivery tracking, etc. [ 16 ]. Such resources include, among others, temperature, luminosity, humidity, pressure, ground chemical concentration, unmanned flying equipment, video cameras, agricultural information management systems, global positioning systems (GPS) and communication networks [ 17 ].
The integration of technological resources into the agricultural production process is a relevant issue. From an economic point of view, the precision agriculture market is expected to have a revenue of US$10 billion in 2023 [ 18 ] with opportunities for technology providers, agricultural equipment and machinery providers, producers and others involved in this business. In addition, smart farms are expected to be able to optimize food production by improving the application of nutrients to the soil, reducing the amount of pesticides and water consumption in irrigation [ 19 ].
2.2. Internet of Things
IoT can be understood as a network of interconnected intelligent devices capable of communicating with each other, generating relevant data about the environment in which they operate. Thus, virtually any device capable of establishing a connection to the Internet can be considered a “thing” within the context of IoT, such as household appliances, electronics, furniture, agricultural or industrial machinery and even people [ 6 ].
Although the idea of IoT is not new, its adoption has increased in recent years, mainly thanks to the development of technologies that support it, among which the improvement of hardware—with the consequent reduction in size and power consumption—improvements in connectivity with the Internet and between devices via wireless connection, cloud computing, artificial intelligence and big data. All these technological components help build a network of devices capable of sharing data and information, as well as acting actively based on network inputs [ 20 ].
According to [ 21 ], the architecture of IoT systems is similar to the architecture of other computer systems but it must take into account the particularities of this paradigm, such as the limited computing capabilities of the devices, identification, detection and control of remote objects.
2.3. Intelligent Agriculture
The IoT architecture proposed in [ 22 ] and [ 23 ], and shown in Figure 1 , presents four layers, considering the main components of an IoT solution: devices, network, services and application.

IoT solution architecture that includes 4 layers: perception, transport, processing and application, based on [ 22 , 23 ].
The perception layer relates to the physical devices in the solution and how they interact with each other and with the transport layer. These devices are responsible for collecting data, enabling the communication of the so-called “things”. This can be done by using commercial solutions—such as UAV devices [ 24 ], sensor nodes [ 25 ]—or new devices, developed with components like sensors and single-board computers (SBC)—such as Arduino or Raspberry Pi—to build sensor nodes and communication gateways. Sensor nodes, for example, are used to monitor plant diseases [ 26 ], control environmental variables in greenhouses [ 27 ] and external crops [ 28 , 29 , 30 ], among others. The interaction between the devices that belong to the perception layer and the services that belongs to the processing layer is intermediated by the transport layer and might occur in several ways, such as through the direct communication between sensor nodes and a data processing platform (such as FIWARE [ 31 ], SmartFarmNet [ 32 ] and Thinger.io [ 33 ]) or through a gateway that, besides intermediating the communication between sensor nodes and the internet, acts as a data hub and enables the communication between network protocols that are originally incompatible, such as ZigBee and the Internet [ 28 ].
The transport layer refers to the network and transport capabilities such as network and application protocols [ 22 ]. IoT solutions use network protocols to enable communication between the perception layer and the processing layer. These protocols are used to create the so-called wireless sensor networks (WSN), that allows wireless communication between sensor nodes and applications. Each protocol has important characteristics, such as the data exchange rate, range and power consumption. Based on these characteristics such protocols can be classified in short-range, cellular networks and long-range [ 34 ]. Protocols for short-range networks (e.g., Bluetooth, ZigBee and Wi-Fi) enable communication in short distances. According to [ 34 ], usually such protocols have a high data transmission rate and low power consumption. Therefore, they are used for the communication between devices that are near each other. Protocols for cellular networks (e.g., GPRS, 3G) enable communication in long distances and with a high data transmission rate. However, they have a high power consumption [ 35 ] and costs for licensing [ 34 ]. Protocols for long-range networks (e.g., LoRaWAN and Sigfox) enable communication in very long distances [ 34 ]. These protocols are used to establish the low power wide area networks (LPWAN) due to the fact that they have a low power consumption [ 36 ]. However, the data transmission rate of these protocols is low. Therefore, these protocols are appropriate for use when the solution needs to transmit a few amounts of data in very long distances. Table 1 presents the characteristics of some network technologies used for IoT.
Examples of network technologies used in IoT [ 34 ].
As shown in Table 1 there is a trade-off between coverage, data rate and energy consumption. Considering the technologies for star networks presented in Table 1 , it is possible to notice that energy consumption is higher in technologies with a high data rate and short coverage. On the other hand, LoRa has a small data rate but a large coverage and low power consumption. These questions are especially relevant when considering agriculture because agricultural scenarios often have limited or no energy supply and obstacles for wireless communication.
Different topologies can be used for implementing networks, such as tree, star and mesh. Star networks have a central node and several peripheral nodes. The communication in such topology occurs as follows: peripheral nodes send data directly to the central node. The central node can implement capabilities for routing messages and communicating through multiple network protocols [ 23 ]. Tree networks are composed of router nodes and leaf nodes. Such networks can be understood as a cluster of star networks. Within each cluster, leaf nodes send messages their father node. In mesh networks, in theory, each node can be a router with rerouting capability. Thus, messages in mesh networks are routed hop by hop until reaching the final destination [ 37 ].
Data is sent to the destination through application protocols such as the message queueing telemetry transport (MQTT) [ 38 ] or the constrained application protocol (CoAP) [ 39 ]. MQTT is an open-source messaging protocol that enables communication between constrained devices and in unreliable networks [ 40 ]. MQTT runs over TPC/IP or similar protocols (e.g., Bluetooth) [ 41 ], which makes the use of MQTT appropriate for different IoT solutions. The MQTT protocol, which is based on the publish/subscribe architecture, allows communication between devices to take place in the following way. First, devices publish messages that are structured in topics on a message broker. Then, other devices read these messages by subscribing to relevant topics on the message broker. These topics allow the organization of messages based on categories, subjects, etc. [ 42 ]. The use of MQTT for communication between device allows low coupling between the device that publishes the message and the devices that listen to the messages, the so-called “one-to-many” communication [ 38 ]. Like MQTT, CoAP is a communication protocol optimized for constrained devices and unreliable networks. However, CoAP messages are interchanged using User Datagram Protocol (UDP) and the CoAP protocol is based on the client/server architecture. This architecture requires that a connection is established between devices before any messages are transmitted [ 38 ]. For this reason, communication using CoAP works in the following way. First, the device that sends messages needs to know the address of each device that is expected to receive messages. Then, messages are sent over UDP to the specified address. Due to the use of UDP, CoAP messages are classified accordingly to the required status of confirmation of receival, for example, confirmable or non-confirmable [ 39 ]. The CoAP protocol does not implement a structure of topics for messages. However, a similar approach can be implemented using application programming interface (API). Nonetheless, the use of CoAP creates a high coupling between the device that sends messages and the device that is expected to receive messages, as the communication is “one-to-one” [ 39 ].
The processing layer comprises data storage, visualization and processing resources. In this context, big data allows distributed storage and parallel data processing, enabling the extraction of information in the shortest possible time [ 43 ]. Such information are used as models by artificial intelligence (AI) systems—which, according to [ 44 ], can be understood as the ability of a system to operate as if it had the thinking capacity of a human being—and machine learning—that, according to [ 45 ] is a data processing technique to detect patterns and correlation among complex and unrelated data—for the development of decision support systems and automation of irrigation control systems [ 46 ], monitoring [ 47 ] and diseases detection in crops [ 48 ], for example.
Finally, the application layer comprises IoT applications that, supported by the other mentioned layers, provide management information to farmers, being able to manage the entire production process in the plantations.
Several related works are being developed in recent years. This rich literature has already been analyzed by the academia from multiple perspectives with objective of determining the state of the smart farming development. Thus [ 49 ] presented a systematic review of precision livestock farming in the poultry sector and [ 50 ] made a review of state of the art of technologies used in precision agriculture, focusing in the innovations, measured parameters, technologies and application areas. On the other hand [ 4 ] has focused on the use of big data as a tool to support agriculture, pointing out the main opportunities and challenges of using this technology. Finally, [ 51 ] presented a quantitative literature review on smart farming related papers, helping to outline an overview of academic production related to the subject. In this way, the present work aims to complement such analyses by making a systematic review of IoT solutions applied to smart farming.
To reach the proposed objectives, this study has used the Preferred Reporting Items for Systematic Reviews (PRISMA) methodology, which is a framework developed to support reports and systematic reviews of literature [ 52 ].
As a research strategy, in October 2019 a search was made in the Scopus database through the search tool available on the website. In addition, in June 2020 a new search was made in the same database to include papers published in 2020. The choice of this database took into consideration its scope and relevance in the academia, since this database indexes several journals and catalogues, such as IEEE, ACM and Elsevier, besides being widely used in similar bibliographic reviews, as in [ 4 ] and [ 51 ]. In addition, in February 2020 a new search was performed in the same database. The strategy adopted for the work research in this database looked for terms used to refer to the application of technology in the area of agriculture, such as “Precision Agriculture”, “Precision Farming”, “Smart Farming” and “Smart Agriculture” in association with “IoT” and synonyms terms. The publication date of the articles was not a criterion for ignoring them. The scope of the research was limited to documents such as journal and conference articles, published in English, Portuguese or Spanish, and whose access was fully available. Thus, the resulting search instruction for the database was as follows:
(“Smart Farming” OR “Smart Agriculture” OR “Precision Farming” OR “Precision Agriculture”) AND (“IoT” OR “Internet of Things” OR “internet-of-things”) AND (LIMIT-TO(ACCESSTYPE(OA)))
It should be noted that the quotation marks have the function of ensuring that terms composed of multiple words were searched together, thus preventing words from being considered individually.
After extracting the articles that resulted from the search, they were manually reviewed through the analysis of the title, keywords, abstract and text. Initially, based on this review, the works identified in the researched database were consolidated, thus eliminating duplicate articles.
Subsequently, the articles were validated as to their framing in the objectives proposed for this study and considered valid when: (i) they were not a review or bibliographical research (ii) they were related to theme (iii) they presented a technology or solution based on IoT to solve problems related to agriculture (iv) they were published in English, Portuguese or Spanish. Furthermore, works were also excluded when they were related to livestock activities instead of agriculture.
The process of searching and selecting papers for this study followed the workflow summarized in Figure 2 , where it can be observed that the initial search resulted in a total of 463 articles, which were analyzed, filtered and classified in a narrowing process that culminated in the selection of 159 articles.

PRISMA flowchart of the systematic review on state-of-the-art IoT solutions in smart farming.
In the identification phase 463 articles were selected with the search tool.
During the screening phase, a manual review of the articles was carried out to identify in the titles, abstract and key words the papers adherent to the objectives proposed for this study, following the criteria mentioned in this section. Among these, 257 were considered invalid and discarded. About 62% of the discarded items did not consider smart farming to be the focus of the work, although some presented improvements for IoT that could benefit smart farming indirectly. Additionally, almost 31% of the discarded papers were studies or literature reviews related to smart farming and the use of various technologies. A smaller number of papers related to smart farming but not addressing IoT (about 5%) and papers where the abstract or text were not available (about 2%) were also discarded.
During the eligibility phase, the content of the 206 resulting articles were reviewed and the papers were classified using the same criteria used in the previous step. In this phase 47 articles were discarded. Among the discarded articles 29% were not related to IoT and 30% were not related to smart farming. The other 41% of the discarded papers were paper reviews or papers without content available. This analysis resulted in 159 articles considered eligible which were included as a sample for this study.
4. Discussion
Based on the results obtained in the analysis of the articles considered for this study, it was possible to observe a growth trend in the number of publications related to IoT and smart farming since 2011, with special emphasis from 2016 onwards, as shown in Figure 3 .

Classification of reviewed papers according to the year of publication.
It is possible to observe an expressive increase of 278% in the number of published papers in 2017/2018. It’s also possible to observe a very similar number of published papers in 2019/2020, until the first semester of 2020. The amount of published papers in recent years evidences the increasing in discussion and the relevancy of the topic IoT applied to smart farming.
Within the reviewed papers it was identified the main scenarios and environments of agriculture. As shown in Figure 4 , such scenarios can be divided into indoor and outdoor. Environments for indoor scenario are protected from climatic impacts, such as solar radiation, rain and wind. Examples of environments for indoor scenarios include greenhouse, hydroponics, crop beds, pots, etc. In contrast, environments for outdoor scenario are more susceptible to climatic impacts. Examples of environments for outdoor scenario are arable lands, orchards and generic outdoor plantation.

Typical scenarios in agriculture can be divided in indoor and outdoor. Indoor agriculture includes environments such as greenhouse, hydroponics and crop beds. Outdoor agriculture includes environments such as orchards and arable lands. IoT solutions that may be applied to multiple environments are referred to as “Generic”.
4.1. Application
Within the reviewed papers it was also identified that the most common applications of IoT solutions for smart farming are:
- Chemical control (e.g., pesticides and fertilizers).
- Crop monitoring.
- Disease prevention.
- Irrigation control.
- Soil management.
- Supply chain traceability
- Vehicles and machinery control.
Table 2 presents the reviewed papers, grouped by agricultural environment and application of the IoT solution. It is worth mentioning that several IoT solutions presented on the reviewed papers could be applied to multiple environments ( Figure 4 ). Thus, such IoT solutions are classified as “Generic”. Additionally, the “Others” column in Table 2 includes papers whose IoT solutions were developed for agricultural environments that were less mentioned, such as pots, crop beds, etc. It is possible noting a predominance in projects where the application is for crop monitoring, irrigation management, and disease prevention.
Smart farming, application and environments.
As shown in Table 2 , the most common application of IoT solutions for smart farming is crop monitoring. Moreover, as shown in Table 2 , these solutions have been developed for multiple agricultural environments, such as arable lands, orchards, greenhouses, etc. The fact that this type of application is so common in agriculture can be justified by the relevance that crop monitoring has for farmers. IoT solutions developed for monitoring crops focused on collecting environmental data of plantations (such as temperature, humidity, luminosity, etc.). Farmers can use these data to obtain a better insight of the plantations. For example, such data was used to determine the vigor of rice [ 47 , 58 ], alfalfa [ 30 ] and maize [ 57 ] crops and to control the environmental conditions of greenhouses [ 99 , 100 , 102 , 104 ]. Similarly, IoT solutions for irrigation control has also been developed for multiple agricultural environments, as demonstrated in Table 2 . Such IoT solutions aimed to optimize the use of water resources in agriculture in different ways, such as by simply using sensors for measuring the soil moisture and using these data for controlling the irrigation source [ 34 , 139 ] or in a more sophisticated way, by combining humidity data with datasets of weather to determine the amount of water required during the irrigation [ 140 ]. IoT solutions for disease prevention aimed to identify and prevent diseases on plantations. For this purpose, these IoT solutions collected multiple environmental and plantation data, such as images of plants [ 129 , 132 , 134 ], sounds [ 135 ], temperature, humidity, etc. [ 131 , 137 ]. These data were processed with different approaches, such as image processing [ 129 , 134 ] or artificial intelligence [ 132 , 137 ]. For example, the IoT solution developed in [ 129 ] processes images collected from a sugarcane crop and identifies diseases on the leaves of plants. In addition, [ 135 ] developed an IoT-enabled device that captures sounds produced by larvae inside trees. IoT solutions for chemical control presented in Table 2 aimed to optimize the application of fertilizers and pesticides on plantations. For this purpose, these IoT solutions collect data (such as nitrogen, salinity or PH) from the crops. Based on the collected data, such IoT solutions can identify crop areas that may require the application of fertilizers or pesticides. For example, in [ 54 ] aerial images of crops are processed to determine the nitrogen concentration in a large plantation. These images are useful to determine the specific region that requires fertilizer. In addition, [ 55 ] developed an automated robot that optimizes the application of pesticides in greenhouse cultivations. IoT solutions for soil management aimed to identify different soil attributes used for planting. For example, such IoT solutions are used to measure the soil moisture [ 163 ], to identify the water consumption pattern [ 159 , 161 ] and to identify the nutrients of the soil [ 158 ]. IoT solutions for vehicles and machinery control focused on collecting data of and managing agricultural equipment and machinery such as tractors, harvesters and trucks. For this purpose, IoT solutions had to deal with the characteristics inherent to agricultural equipment, such as mobility. Data from the equipment itself, such as implement status, engine performance, or speed are collected using sensors [ 41 ] to optimize their maintenance cycle. Additionally, due to the mobility of agricultural equipment, opportunistic computing was used to collect data from remote crop areas by using sensors coupled to tractors [ 174 ].
Each agricultural environment presented in Table 2 brings its own challenges for the projects, which includes the environment impact on the communication between sensors, either by the distance between the sensor nodes [ 25 , 105 , 180 ], by the lack of communication in the croplands [ 98 , 174 ] or even by the impact of vegetation in the signal propagation [ 70 , 175 ]. Furthermore, as indicated in [ 28 ], climatic elements—such as rain, snow or solar radiation—have influence on both the planting and the sensor nodes.
To cover these scenarios commercial electronic sensors are used by 96% of the reviewed papers. This expressive usage can be justified by the fact that such sensors are affordable, certified, ready-to-market and meet the main monitoring needs in IoT solutions for smart farming. Such sensors are used for collecting real-time data about multiple agricultural parameters, such as climatic data, substrate information, luminosity, CO2 concentration and images through cameras and multispectral sensors, as shown in Table 3 . Moreover, several papers (4%) focused on developing custom-made sensors for monitoring specific agricultural aspects, such as soil nutrients (e.g., nitrate [ 158 ]) and leaf evapotranspiration for measuring the hydric stress in tobacco crops [ 81 ].
Types of physical sensors and use in smart farming.
Different types of sensors are used in agriculture for collecting data from different aspects of agriculture such as crop monitoring, substrate monitoring and environment monitoring.
As presented in Table 3 , different types of sensors were used in IoT solutions for smart agriculture to collect data from multiple aspects of agriculture, such as the crop, substrate, environment and other. For this purpose, as shown in Table 3 , for environment monitoring electronic sensors were used in IoT solutions to collect environmental data, such as temperature, humidity and luminosity [ 104 , 109 , 114 ]. In addition, for substrate monitoring electronic sensors were used to collect data from the substrate (e.g., soil and water), such as temperature, moisture and nitrogen. Likewise, pH sensors were used for measuring the acidity or the alkalinity of the water in hydroponics cultivations. For crop monitoring, cameras and multispectral sensors were used to collect images of crops. These sensors can be installed on an UAV to obtain aerial images of large plantations [ 47 , 57 , 58 ] or used in robots to retrieve a detailed image of the leaf of a plant [ 111 ].
4.2. Perception
The choice of hardware is a very important aspect of the IoT project development because it impacts the costs and the technologies that can be used. 60% of the reviewed papers mentioned the hardware used to support the IoT solution. Furthermore, SBCs were mentioned by 40% of the reviewed papers. The use of SBCs can be justified by the fact that these devices are affordable and versatile [ 38 ], enabling the development of custom-made IoT devices. For example, some SBCs such as Arduino has an integrated development environment (IDE). This IDE enables the development of custom programs to be installed as firmware on the Arduino boards [ 184 ]. Similarly, Raspberry Pi is compatible with several operating systems, such as Raspbian, Ubuntu Core or Mozilla Web Things [ 185 ]. Some of these operating systems are open-source, which allow for the customization of its source-code. Besides, these operating systems support applications developed with programming languages such as Python [ 26 ]. Furthermore, the capabilities of SBCs can be extended by associating them with other hardware components, such as sensors or transceivers. This characteristic makes SBCs able to work as gateways or sensor nodes in IoT solutions. Among the papers that mentioned SBCs, 82% mentioned the use of Arduino, Raspberry Pi and ESP boards (such as ESP8266, ESP12 and ESP32). Table 4 presents the application of embedded system platforms and UAV devices in smart farming.
Embedded system platforms and UAV devices in smart farming.
As shown in Table 4 , IoT-enabling devices are used for multiple applications on IoT solutions for smart farming. SBCs were used both as sensor nodes and gateways. Table 4 reveals that Arduino was the most commonly used embedded system platform among the reviewed papers. The extensive use of Arduino can be justified by the fact that Arduino is open-source hardware that enables the development of different devices through the use of boards that extend their native functionality. Table 4 also shows that embedded system platforms have been more widely used in IoT solutions for crop monitoring. As sensor nodes, for example, in [ 124 ] sensors for collecting environmental data such as soil humidity, solar radiation and rain are connected to an Arduino Uno. The Arduino is, then, used to monitor the health of a vineyard. Likewise, in [ 117 ] a Raspberry Pi is used to manage the temperature and air humidity of a greenhouse. IoT devices are also used as gateways to connect short-range WSN with the internet by using long-range communication protocols. For example, in [ 127 ] a gateway is used to connect WSNs using 3 different protocols (ZigBee, Bluetooth and Wi-Fi) with a remote server by using 3G. In [ 84 ] a LoRaWAN gateway obtains data from sensor nodes using LoRa and retransmits this data to a cloud-hosted platform by using 4G. 3G and 4G are cellular network technologies that, as discussed in Section 2.3 , enable communication in long distances and with a high data transmission rate. These technologies will be discussed with more details in Section 4.3 .
In addition, Table 4 also reveals that UAV is widely used by IoT solutions for monitoring crops, disease prevention and chemical control. The use of UAV for crop monitoring is due to the fact that UAV has the potential to accelerate and reduce the cost of monitoring extensive crops. For this purpose, cameras and multispectral sensors are attached to UAV devices that are used to obtain aerial images from large crops. Such images are processed by the IoT solution to calculate agricultural parameters, such as the leaf area index (LAI). The LAI is a parameter used to determine the vegetation coverage within a specific area. LAI, combined with other parameters, can be used to evaluate the amount of nitrogen in rice crops [ 58 ], determine the vigor of rice and maize [ 47 , 57 ] crops and detect diseases in sugarcane crops [ 129 ]. Moreover, UAV devices are used in [ 46 ] to optimize the application of pesticides and fertilizers in arable lands.
4.3. Network
Data obtained with sensor nodes are usually sent to the destination (e.g., database, server, IoT platform) through a wired or wireless network. Within the reviewed papers, 60% have mentioned the network protocol used in the IoT solution. Among the mentioned network protocols, CAN and Ethernet were the most used ones for wired networks. Likewise, LoRaWAN and protocols for cellular network (e.g., GPRS, 3G, etc.) were the most used protocols for long-range wireless networks. Analogously, ZigBee, Wi-Fi and Bluetooth were the most used protocols for short and mid-range wireless networks. Table 5 shows network protocols used for the IoT solutions within the reviewed articles.
Use of network protocols in smart farming for different farming scenarios.
As shown in Table 5 , several network protocols are used in different environments of agriculture (e.g., arable land, greenhouse, orchard) to enable communication between IoT solution devices, such as sensor nodes and gateways. Such network protocols enable the creation of short or long-range networks. Table 5 reveals that for short and middle-range communication, IoT solutions of the reviewed papers used different technologies, such as Wi-Fi, ZigBee and Bluetooth. Moreover, it is possible to observe in Table 5 that Wi-Fi is the most common network technology for communication within the analyzed articles. This extensive use of Wi-Fi can be justified by the fact that Wi-Fi is a ubiquitous technology and, therefore, easy to implement. However, due to the higher energy consumption of Wi-Fi, low-energy consumption technologies, such as ZigBee or Bluetooth, are also extensively used. For example, [ 62 ] used ZigBee to send images from a plantation to a remote server and [ 188 ] developed a sensor node that uses Bluetooth to deliver monitoring information from the farm directly to an application installed on a smartphone. Table 5 also demonstrates that IoT solutions of the reviewed papers used cellular networks, Sigfox, or LoRaWAN for long-range networks. Cellular networks are prevalent in IoT solutions for Smart Farming. This can be justified by the fact that cellular networks allow the communication of IoT devices in long distances and with a high data rate. For example, [ 141 ] uses cellular network to send data collected from humidity sensors to a cloud-based platform and to control an irrigation system. Similarly, Sigfox and LoRaWAN enable communication in very long distances while requiring low energy to operate. Based on these characteristics, Sigfox and LoRaWAN were used for long-range communication, as an alternative to cellular networks or in regions where there was no cellular network coverage. Sigfox is used in [ 34 ] as the network protocol of an IoT solution used to control the irrigation of a plantation. Likewise, in [ 137 ] the LoRaWAN is used to send data from multiple sensors installed in a greenhouse to a remote platform.
Besides the distance between sensor nodes, gateways, and other network elements, the vegetation itself can be an obstacle for sensor communication, as demonstrated by [ 175 ] and [ 70 ] who analyzed the impacts on signal propagation on 433 MHz and 2.4 GHz frequencies in rice plantations and an orchard. An additional challenge for greenhouses arises from the high density of sensors, which can lead to interference in the wireless signal due to proximity [ 105 , 112 , 180 ]. To mitigate this problem wired networks, such as CAN [ 100 ] or Ethernet [ 122 ], can be used. As shown in Table 5 , these technologies have been more used in greenhouses, because usually this type of agricultural environment is more appropriated for implementing wired networks. Moreover, [ 112 ] investigated the path loss on wireless signals and concluded that the proper positioning of directional antennas can optimize the number of sensory nodes required for monitoring a greenhouse.
Network topology is another important aspect of an IoT solution. According to [ 61 ] the topology of sensor networks can be star, tree (or cluster) or mesh. The network topology impacts the distance between the sensor nodes and the destination and, consequently, the number of sensor nodes in the WSN [ 190 ]. For example, star networks are composed of a central node (coordinator) and several peripheral nodes. In such topology, peripheral nodes send data to the central node [ 93 ]. Therefore, the maximum distance between the peripheral nodes and the central node is limited by the maximum distance allowed by the physical layer communication standard. On the other hand, as discussed in Section 2.3 , in mesh networks each node has routing capability, hence extending the network coverage by allowing multi-hop communications [ 191 ]. Based on the architecture of the IoT solution and on the project description it was possible to identify the topology adopted by 61% of the reviewed papers. For example, a star topology is used in [ 34 ] for connecting sensor nodes to a central node using the LoRa protocol. This central node acts as a gateway and retransmits messages to a cloud-based application that controls an irrigation system using Sigfox. Also, in [ 107 ] the star topology is used to connect multiple sensors within a greenhouse. Such sensors use the ZigBee protocol to send messages to a central node, which acts as the network gateway. Mesh networks are considered more complex to be implemented but also more reliable due to the redundancy of communication between the sensor nodes [ 105 ]. Such topology is used in [ 105 , 107 ] for monitoring a greenhouse. Tree (or cluster) networks combine multiple star networks. Both [ 61 ] and [ 178 ] implement a cluster network for monitoring crops. In [ 61 ] sensor nodes collect information from a crop and send messages to a router node. This router node acts as the gateway of the cluster and retransmits the message to the main router node of the network. In [ 178 ] several router nodes are deployed in the crop area in order to optimize the energy consumption of sensor nodes.
Furthermore, embedded system platforms have been used to support network topologies. The chart in Figure 5 presents the distribution of embedded system platforms by network topology or device connection type. It is worth mentioning that although point to point is not a network topology, this type of device connection was used in several IoT solutions within the review articles. As shown in Figure 5 , Raspberry Pi is often used in IoT solutions implementing the star network topology. Arduino is the embedded system platform used in multiple types of network topology or device connections. Additionally, Arduino is the most frequently used embedded system platform to support star network topology and point-to-point communication. Finally, ESP-based devices include devices that use system-on-a-chip (SoC) modules such as ESP-32 and ESP8266 (Espressif Systems, Shanghai, China). ESP-based devices are often used in IoT solutions that implement star network topology or point-to-point communication.

Distribution of IoT-enabling devices by network topology or device connection type within the reviewed papers.
As mentioned in Section 4.2 , embedded system platforms can be used to build gateways or sensor nodes. As shown in Figure 5 the use of Raspberry Pi, Arduino and ESP stand out, probably because such embedded system platforms are cost-effective [ 38 ] and enable different network protocols (e.g., ZigBee, Wi-Fi and Bluetooth) with the use of transceivers. This characteristic allows such embedded system platforms to act as sub-nodes and central nodes in a star network [ 27 , 30 , 34 ] or as router nodes in mesh and cluster networks [ 106 , 178 ].
IoT devices transmit information to cloud-based platforms or applications through application protocols [ 109 ]. Such protocols can follow the publisher/subscriber architecture which, as mentioned in Section 2 , are appropriate for devices with limited computing resources. Among the application protocols used in the reviewed papers HTTP, MQTT and CoAP stand out. Such application protocols are useful to enable compatibility between non-standardized IoT devices and IoT platforms. For example, SmarFarmNet developed in [ 32 ] adopts the “bring your own IoT device” concept by implementing loosely coupled application protocols such as MQTT and CoAP. Furthermore, although HTTP is not a specific protocol for machine-to-machine (M2M) communication, its use associated with REST APIs enables low coupling between IoT devices and applications, analogous to MQTT, for example. However, as [ 109 ] concludes, the MQTT protocol is preferable for smart farming applications due to its resiliency, interoperability across different network protocols and transmission rate.
Finally, although the power consumption is not an exclusive topic within the transport layer, according to [ 178 ] the highest power consumption for IoT devices within a WSN occur during the transmission of data. This review identified several approaches for optimizing the power consumption in IoT solutions for smart farming. Among the identified solutions are the use of low energy protocols (e.g., BLE, ZigBee, Sigfox), reduction of data transmission in sensor nodes by an optimized duty cycle [ 177 , 178 , 192 ] and the use of message routing approaches that are more energy-efficient [ 72 , 193 ].
4.4. Processing
Among the analyzed papers it was possible to observe that initially, the main objective of IoT solutions was to collect and store data from sensor nodes. However, in more recent years, it is possible to observe an increasing number of IoT solutions that used supplementary techniques and technologies to treat the collected data, such as cloud computing and big data. Likewise, it is possible to observe an increasing number of works that used simultaneously two or more techniques or technologies for processing data. As shown in Figure 6 , the most cited technologies within the reviewed papers are cloud computing (34%), machine learning (15%), big data (13%), and artificial intelligence (9%).

Techniques and technologies for data-processing in smart farming identified within the reviewed papers. In recent years, the use of modern processing techniques, such as artificial intelligence and big data became more common. IoT applications relies on cloud computing for storing and processing the big data of agricultural information collected by IoT devices.
Table 6 presents IoT solutions that relied on cloud-based platforms for processing data and highlights the main data processing techniques (e.g., Artificial Intelligence, Big Data, etc.). The column “Other/Not identified” comprehends IoT solutions that have used cloud-based platforms but have either (i) used any of the data processing technologies identified by other columns on Table 6 or (ii) not explicitly mentioned the type of data processing technology that was adopted.
IoT-enabling platforms and data processing technologies used in smart farming within the reviewed papers.
Table 6 reveals that the most found cloud-based platforms in the reviewed papers are ThinkgSpeak, FIWARE, Ubidots, SmartFarmNet, AWS IoT and Thinger.io. In particular ThingSpeak is the most used cloud-based platform across all the reviewed papers, due to the fact that this platform is open-source with low infrastructure requirements [ 34 ]. In addition, Table 6 shows that AWS IoT was used with a higher number of data processing techniques. Not all cloud-based platforms offer the same set of functionalities, but in general, they have capabilities for data storage [ 30 , 94 , 102 , 122 , 132 ], processing [ 194 ] and visualization [ 102 ] and action control on farms [ 34 ]. Furthermore, Table 6 also reveals that, even though there are multiple cloud-based platforms, several reviewed papers developed their own cloud-based platform for the IoT solution.
Cloud-based platforms provide scalability for IoT solutions by relying on cloud computing to process and data. For instance, some platforms shown in Table 6 , such as Thinger.io [ 33 ], are built entirely on top of infrastructure services provided by cloud providers (e.g., Amazon AWS and Microsoft Azure). Also supported by such services, the platforms make available data analysis modules with graphics and panels that allow real-time monitoring of the information obtained or the creation of customized panels from the integration of multiple data [ 33 ].
Due to the scalability provided by these platforms, the large amount of data generated by the sensors is stored in databases to form the so-called big data, an unstructured set of information that is used to generate information about crops. According to [ 197 ] big data demands the use of technologies to optimize the processing time due to the large volume of information. For example, Hadoop—a parallel database for big data applications—proved to be efficient when analyzing the rainfall index data from several meteorological stations [ 197 ].
IoT solutions use different types of techniques and technologies for processing the collected data. Table 7 presents commonly used technologies per applications as identified in the reviewed papers. Column “Other Technologies” encompasses all the technologies that are not identified by any of the other columns in Table 7 .
Technologies and application in smart farming.
Table 7 reveals that the most commonly used technologies to support data processing are artificial intelligence, machine learning, and big data. The use of these technologies is related to their ability to process large amounts of information in a short time. In addition, Table 7 also shows that crop monitoring is the most common type of application for IoT solutions that have used data processing technologies. Moreover, crop monitoring is also the type of application that used the most different technologies for data processing. This can be understood by the fact that usually IoT solutions for monitoring crops collect a bunch of data and rely on machine learning and big data to process such data.
As demonstrated in Table 7 , bigdata was used for different applications in IoT solutions, such as crop monitoring, soil management and irrigation control. For example, supported by big data, in [ 142 , 161 , 194 ] the soil moisture data gathered by physical sensors were related to data made available in datasets, such as the NASA Prediction of Worldwide Energy Resources (POWER) [ 198 ]—which contains meteorological data—purchase and sale values of crops, information from the user and government agencies to optimize the amount of water in irrigation cycles, support the farmer in the acquisition of agricultural inputs—such as seeds and fertilizers—and generate information and perspectives about other activities related to agriculture. Big data was also used by [ 56 ] in the development of a decision support system to provide irrigation and monitoring advice to farmers from a knowledge base created with data obtained by physical sensors (e.g., temperature, soil moisture) and virtual sensors (e.g., soil type, season). Virtual sensor is a type of software that, given the available information, processes what a physical sensor otherwise would [ 199 ].
In addition, automatic management with IoT depends on the manipulation of multiple variables. Initially, the simple observation of soil humidity and temperature can be used to trigger irrigation or cooling systems, as proposed by [ 187 ]. Nevertheless, greenhouse management can be more complex. As shown in [ 104 , 107 , 120 ], greenhouse parameters like temperature and humidity are closely tied and changing one of them can affect several others.
Fuzzy logic, as indicated in Table 7 , was used in IoT solutions applications that need to handle multiple variables, such as irrigation control and monitoring crops. For this purpose, [ 120 ] uses fuzzy logic to handle multiple variables of temperature and humidity into a greenhouse and determine when a cooling system and an irrigation system should be started. Similarly, [ 73 ] uses fuzzy logic to optimize the number of sensors for monitoring soil temperature and moisture. Machine learning was also used in data processing by [ 46 ] to predict environmental conditions based on the forecast values of weather, humidity, temperature and water level and thus to control an irrigation system, by [ 47 ] to combine multiple parameters obtained from images, such as color and texture indices and by [ 48 ] to identify marks on the plants and, thus, to identify possible diseases. Similarly, in [ 58 , 125 ] it was used to detect diseases, identify growth stages and the health of plantations.
Similarly, as shown in Table 7 , IoT solutions used computer vision for applications that need to deal with image processing, such as crop monitoring and diseases prevention. It was also possible to observe in the reviewed papers the use of computer vision to identify and classify elements in images obtained by cameras, enabling the identification of fruit in an orchard [ 200 ] or the existence of diseases and pests in plantations [ 48 , 129 , 133 ]. Additionally, in [ 133 ] computer vision was used as a monitoring tool to detect the presence of insects that can cause diseases in olive groves and in [ 48 ] the same technique was employed to analyze diseases that cause morphological deformations in plants. Additionally, computer vision was used in crop management systems, for example in [ 134 ] where it was implanted in a robot equipped with a camera and other sensors, being able to obtain images of vegetation and, through computer vision, detect weeds in plantations and eliminate them. Similarly, in [ 111 ] a robot can identify a plant and interact with the environment to irrigate it, if necessary.
Finally, blockchain proved to be an opportune technology for systems that need to implement traceability of the supply chain, as shown in Table 7 . According to [ 171 ] blockchain is a global public distributed ledger that records all transactions between users. In fact, this type of control is relevant for agriculture in several aspects, such as food safety, guarantee of origin or cost reduction. To ensure information security, this technology was proposed by [ 30 , 167 , 171 ] for agricultural product traceability. For example, in [ 171 ] an IoT solution uses blockchain to record information regarding the tea production based on 5 business processes: production plan, quality inspection, sales processing, product quality inspection and order delivery. In [ 167 ] a production tracking system for agricultural cooperatives have been developed. In [ 30 ] a similar system is being proposed but still in development stage.
5. Considerations
IoT solutions for smart farming take advantage of the scalability provided by platforms and cloud computing to store large amounts of data obtained by sensors. These big data of specific information may be processed with artificial intelligence techniques—such as machine learning—to improve the management of smart farming. For example, the processing of big data may be used to obtain crop insights, optimize water resources and increase the crop quality by preventing disease and reducing the amount of chemical products employed. Crop monitoring solutions use SBC (e.g., Arduino and Raspberry Pi) or UAV (e.g., drones) together with sensors (e.g., humidity, temperature, CO 2 or image) to collect data in indoor or outdoor environments.
Different types of network connections are used for communication between IoT devices, such as wired and wireless connections. The review shows that wired networks, such as CAN and Ethernet, are used for indoor agriculture (e.g., greenhouses). The use of wired network on indoor agriculture may be justified by the fact that in this scenario the physical components of the network are less susceptible to climatic agents impacts. Likewise, generally distance between sensor nodes in indoor agriculture enables this type of connection. Wireless connection, on the other hand, is used both in indoor and outdoor agriculture. Wi-Fi is the most mentioned protocol within the analyzed projects, due to its ubiquitous utilization in the daily life. However, power consumption and signal range characteristics may limit use of Wi-Fi in larger projects or in projects with power restrictions. To overcome the power consumption issue, energy-efficient protocols such as ZigBee, BLE or LoRa are used for communication in wireless networks.
Furthermore, it is worth mentioning that this review investigated papers where the IoT solution for smart farming was applied to agriculture only. However, the use of IoT for smart farming can also be applied to other activities related to farming, such as livestock [ 201 ]. Moreover, despite the fact that power-supply in IoT solutions for smart farming does not represent a specific layer of an IoT solution architecture [ 17 , 18 ], this topic has been covered in some of the reviewed papers. For example, [ 72 , 177 ] proposed improvements in algorithms for message routing and in duty cycles in sensor nodes. These approaches contribute to the reduction of power consumption by IoT devices. Similarly, a mission-based approach was used in [ 53 ] to optimize the power consumption in UAV. This approach was used to identify the most efficient path for a set of drones. Likewise, [ 177 ] proposed an intelligent activity cycle to improve the performance of data aggregators in terms of energy efficiency on cloudy days.
6. Conclusions
This work presented a systematic review of the state-of-the-art of IoT adoption in smart agriculture and identified the main components and applicability of IoT solutions. This review reported a change in the treatment of data in recent works: while previous work showed that the majority of decision support systems used simple processing mechanisms to handle data collected in real-time, more recent work showed an increasing number of management systems that use complementary technologies that rely on cloud and big data computing for processing large amounts of data. Furthermore, it was observed in this review that in recent work the use of artificial intelligence and image processing techniques has become more common to improve the management of smart farming. From the identified applications of IoT for smart farming it was observed that the most common application is the monitoring of crops. This review also showed that different network protocols may be simultaneously used in IoT solutions for smart farming. In addition, the comparison of types of network connections used in IoT solutions for smart farming revealed that wired networks are used in indoor scenarios (e.g., greenhouse) while wireless networks are used both in indoor and outdoor scenarios (e.g., arable lands, orchards). Moreover, the review discussed in this work suggests the increasing relevance of IoT solutions for smart farming. Future work may extend this review by including other relevant articles and complementary analysis of project costs, usability and regional challenges intrinsic to IoT applications. Another important future research direction could be the analysis of the edge and fog computing usage in smart agriculture as a way to deal with challenges associated with traditional centralized cloud solutions such as high communication latencies, lack of support for real-time reaction to detected events, large bandwidths, etc.
Acknowledgments
The authors acknowledge to the Mobile Computing Laboratory of the School of Technology and Management of the Polytechnic Institute of Leiria and to the Computer Science and Communication Research Center for the facilities granted in the implementation of part of this work, in the context of the Smart IoT Ecosystems research line.
Author Contributions
All authors contributed equally to this work. Conceptualization, E.N., N.C., A.P.; data curation, E.N., N.C., A.P.; formal analysis, N.C., A.P.; funding acquisition, A.P.; investigation, E.N., N.C., A.P.; methodology, N.C., A.P.; resources, A.P.; supervision, A.P.; validation, N.C.; writing—original draft, E.N., N.C.; writing—review & editing, E.N., N.C, A.P. All authors have read and agreed to the published version of the manuscript.
This work was partially supported by Portuguese Fundação para a Ciência e a Tecnologia–FCT, I.P., under the project UIDB/04524/2020” and by Portuguese National funds through FITEC–Programa Interface, with reference CIT “INOV–INESC Inovação–Financiamento Base”.
Conflicts of Interest
The authors declare no conflict of interest.
Accessibility Links
- Skip to content
- Skip to search IOPscience
- Skip to Journals list
- Accessibility help
- Accessibility Help
Click here to close this panel.
Purpose-led Publishing is a coalition of three not-for-profit publishers in the field of physical sciences: AIP Publishing, the American Physical Society and IOP Publishing.
Together, as publishers that will always put purpose above profit, we have defined a set of industry standards that underpin high-quality, ethical scholarly communications.
We are proudly declaring that science is our only shareholder.
Smart Agriculture and Smart Farming using IoT Technology
V Dankan Gowda 1 , M Sandeep Prabhu 2 , M Ramesha 3 , Jayashree M Kudari 4 and Ansuman Samal 5
Published under licence by IOP Publishing Ltd Journal of Physics: Conference Series , Volume 2089 , 1st International Conference on Applied Mathematics, Modeling and Simulation in Engineering (AMSE) 2021 15-16 September 2021, India (Virtual) Citation V Dankan Gowda et al 2021 J. Phys.: Conf. Ser. 2089 012038 DOI 10.1088/1742-6596/2089/1/012038
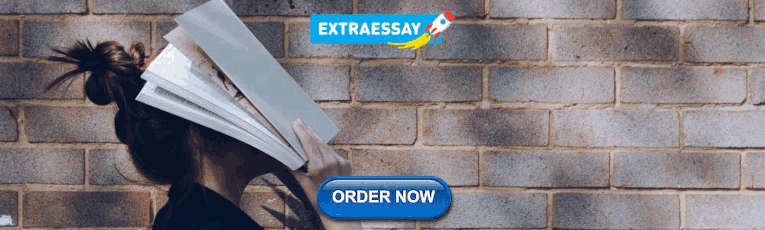
Article metrics
5658 Total downloads
Share this article
Author e-mails.
Author affiliations
1 Department of Electronics and Communication Engineering, B.M.S Institute of Technology and Management, Bangalore, Karnataka, India
2 Department of Electronics and Communication Engineering, Canara Engineering College, Benjanapadavu, Bantwal, Mangalore, Karnataka, India
3 Department of Electronics and Communication Engineering, GITAM School of Technology GITAM(Deemed to be University), Bangalore, Karnataka, India
4 Department of Computer Science and Information Technology, JAIN Deemed to be University, Bangalore, Karnataka, India
5 Faculty of Hospitality and Tourism Management, Siksha O Anusandhan Deemed to be University, Bhubaneshwar, Odisha, India
Buy this article in print
It has become easier to access agriculture data in recent years as a result of a decline in digital breaches between agricultural producers and IoT technologies. These future technologies can be used to boost productivity by cultivating food more sustainably while also preserving the environment, thanks to improved water use and input and treatment optimization. The Internet of Things (IoT) enables the production of agricultural process-supporting systems. Referred to as remote monitoring systems, decision support tools, automated irrigation systems, frost protection systems, and fertilisation systems, respectively. Farmers and researchers must be provided with a detailed understanding of IoT applications in agriculture as a result of the knowledge described above. This study is about using Internet of Things (IoT) technologies and techniques to enhance agriculture. This article is meant to serve as an introduction to IoT-based applications in agriculture by identifying need for such tools and explaining how they support agriculture.
Export citation and abstract BibTeX RIS
Content from this work may be used under the terms of the Creative Commons Attribution 3.0 licence . Any further distribution of this work must maintain attribution to the author(s) and the title of the work, journal citation and DOI.
Internet of Things for the Future of Smart Agriculture: A Comprehensive Survey of Emerging Technologies
Ieee account.
- Change Username/Password
- Update Address
Purchase Details
- Payment Options
- Order History
- View Purchased Documents
Profile Information
- Communications Preferences
- Profession and Education
- Technical Interests
- US & Canada: +1 800 678 4333
- Worldwide: +1 732 981 0060
- Contact & Support
- About IEEE Xplore
- Accessibility
- Terms of Use
- Nondiscrimination Policy
- Privacy & Opting Out of Cookies
A not-for-profit organization, IEEE is the world's largest technical professional organization dedicated to advancing technology for the benefit of humanity. © Copyright 2024 IEEE - All rights reserved. Use of this web site signifies your agreement to the terms and conditions.
Advertisement
A Comprehensive Study on Smart Agriculture Applications in India
- Published: 23 March 2023
- Volume 129 , pages 2345–2385, ( 2023 )
Cite this article
- Neena Alex 3 ,
- C. C. Sobin ORCID: orcid.org/0000-0003-2550-9244 1 &
- Jahfar Ali 2
902 Accesses
7 Citations
Explore all metrics
The rampant adoption of digital technologies made momentous changes in all economic sectors. The agriculture sector cannot abstain from the digital revolution. Agriculture and farming are one of the oldest and most important professions in India. The sector remains the backbone of the Indian rural economy, which desperately demands technological impetus for the socio-economic development of rural areas. Smart agriculture is a revolution in the agriculture industry which helps to guide the actions that are required to modify and reorient the agricultural systems. The paper made an extensive survey of various technologies proposed for the agriculture sector. We have surveyed various smart agricultural applications developed and proposed a taxonomy for classifying them. Network infrastructure and connectivity remains the major challenge for rural areas. The paper explores the viability of deploying IoT-based technologies in agricultural sectors along machine learning techniques to optimize resource utilization, planning and cultivation, marketing, pesticide selection, price prediction, etc. An in-depth coverage of recent research works is also mentioned which will help the future researchers to address specific challenge and adopt suitable technology to help the farmers to improve their productivity and better decision making in cultivation. Apart from listing the applications, we also propose an architecture for smart agriculture and implemented smart price prediction model for crops like cotton and cardamom along with a prototype for smart irrigation.
This is a preview of subscription content, log in via an institution to check access.
Access this article
Price includes VAT (Russian Federation)
Instant access to the full article PDF.
Rent this article via DeepDyve
Institutional subscriptions
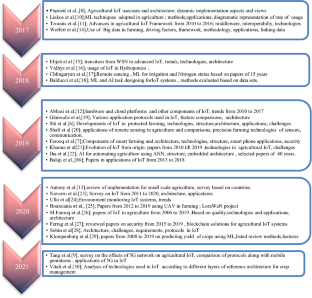
Similar content being viewed by others
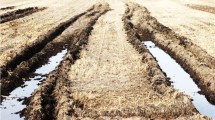
A Review on the Effect of Soil Compaction and its Management for Sustainable Crop Production
Traditional agriculture: a climate-smart approach for sustainable food production.
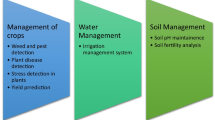
Machine learning in agriculture: a review of crop management applications
Availability of data and materials.
All data generated or analyzed during this study are included in this published article (data transparency).
Code availability
On request (software application or custom code).
(Online) http://www.fao.org/india/fao-in-india/india-at-a-glance/en/ . Accessed 23 Feb 2021
(Online) https://www.statista.com/topics/4868/agricultural-sector-in-india/#dossierSummary . Accessed 23 Feb 2021
(Online) India at a glance|FAO in India|Food and Agriculture Organization of the United Nations. Accessed 15 Oct 2022
(Online) https://www.indiatoday.in/education-today/featurephilia/story/agri-tech-agriculture-technology-indian-entrepreneur-divd-1597632-2019-09-10 . Accessed 23 Feb 2021
(Online) https://www.nasscom.in/sites/default/files/media_pdf/NASSCOM_Press_Release_Agritech_Report_2019.pdf . Accessed 23 Feb 2021
Shi, X., An, X., Zhao, Q., Liu, H., Xia, L., Sun, X., & Guo, Y. (2019). State-of-the-art internet of things in protected agriculture. Sensors, 19 (8), 1833.
Article Google Scholar
Farooq, M. S., Riaz, S., Abid, A., Abid, K., & Naeem, M. A. (2019). A survey on the role of IoT in agriculture for the implementation of smart farming. IEEE Access, 7 , 156237–156271. https://doi.org/10.1109/ACCESS.2019.2949703
Popovic, T., Latinovic, N., Pesic, A., Zecevic, Z., Krstajic, B., & Djukanovic, S. (2017). Architecting an IoT-enabled platform for precision agriculture and ecological monitoring: A case study”. Computers and Electronics in Agriculture, 140 , 255–265.
Tang, Y., Dananjayan, S., Hou, C., Guo, Q., Luo, S., & He, Y. (2021). A survey on the 5G network and its impact on agriculture: Challenges and opportunities. Computers and Electronics in Agriculture, 180 , 105895. https://doi.org/10.1016/j.compag.2020.105895
Liakos, K. G., Busato, P., Moshou, D., Pearson, S., & Bochtis, D. (2018). Machine learning in agriculture: A review. Sensors, 18 (8), 2674. https://doi.org/10.3390/s18082674
Tzounis, A., Katsoulas, N., & Bartzanas, T. (2017). Internet of Things in agriculture, recent advances and future challenges. Biosystems Engineering, 164 , 31–48. https://doi.org/10.1016/j.biosystemseng.2017.09.007
Abbasi, M., Mohammad, Y., & Rahnama, F. (2018). Internet of things in agriculture-survey. In IEEE Conference on IoT, University of Isfahan . https://doi.org/10.1166/jctn.2018.7478
Antony, A. P., Leith, K., Jolley, C., Lu, J., & Sweeney, D. J. (2020). A review of practice and implementation of the internet of things (IoT) for smallholder agriculture. Sustain, 12 , 1–19. https://doi.org/10.3390/su12093750
Wolfert, S., Ge, L., Verdouw, C., & Bogaardt, M. (2017). Big data in smart farming—A review. Agricultural Systems, 153 , 69–80. https://doi.org/10.1016/j.agsy.2017.01.023
Elijah, O., Rahman, T. A., Orikumhi, I., Leow, C. Y., & Hindia, M. N. (2018). An overview of Internet of Things (IoT) and data analytics in agriculture: Benefits and challenges. IEEE Internet of Things Journal, 5 , 3758–3773. https://doi.org/10.1109/JIOT.2018.2844296
R. Vidhya, K. Valarmathi (2018) Survey on automatic monitoring of hydroponics farms using IoT. In Proceedings of the 3rd international conference on communication and electronics systems , pp. 125–128. https://doi.org/10.1109/CESYS.2018.8724103
Chlingaryan, A., Sukkarieh, S., & Whelan, B. (2018). Machine learning approaches for crop yield prediction and nitrogen status estimation in precision agriculture: A review. Computers and Electronics in Agriculture, 151 , 61–69. https://doi.org/10.1016/j.compag.2018.05.012
Balducci, F., Impedovo, D., & Pirlo, G. (2018). Machine learning applications on agricultural datasets for smart farm enhancement. Machines., 6 (3), 38. https://doi.org/10.3390/machines6030038
Glaroudis, D., Iossifides, A., & Chatzimisios, P. (2019). Survey, comparison and research challenges of IoT application protocols for smart farming. Computer Networks, 168 , 107037. https://doi.org/10.1016/j.comnet.2019.107037
Shafi, U., Mumtaz, R., García-Nieto, J., Hassan, S. A., Zaidi, S. A. R., & Iqbal, N. (2019). Precision agriculture techniques and practices: From considerations to applications. Sensors, 19 (17), 3796. https://doi.org/10.3390/s19173796
Khanna, A., & Kaur, S. (2019). Evolution of Internet of Things (IoT) and its significant impact in the field of precision agriculture. Computers and electronics in agriculture, 157 , 218–231. https://doi.org/10.1016/j.compag.2018.12.039
Jha, K., Doshi, A., Patel, P., & Shah, M. (2019). A comprehensive review on automation in agriculture using artificial intelligence. Artificial Intelligence in Agriculture, 2 , 1–12. https://doi.org/10.1016/j.aiia.2019.05.004
Navarro, E., Costa, N., & Pereira, A. (2020). A systematic review of IoT solutions for smart farming. Sensors, 20 (15), 4231. https://doi.org/10.3390/s20154231
Ullo, S. L., & Sinha, G. R. (2020). Advances in smart environment monitoring systems using IoT and sensors. Sensors, 20 (11), 3113. https://doi.org/10.3390/s20113113
Boursianis, A. D., Papadopoulou, M. S., Diamantoulakis, P., Liopa-Tsakalidi, A., Barouchas, P., Salahas, G., Karagiannidis, G., Wan, S., & Goudos, S. K. (2020). Internet of Things (IoT) and Agricultural Unmanned Aerial Vehicles (UAVs) in smart farming: A comprehensive review. Internet of Things, 18 , 100187. https://doi.org/10.1016/j.iot.2020.100187
Farooq, M. S., Riaz, S., & A. Ab id, T. Umer, and Y.B. Zikria,. (2020). Role of IoT technology in agriculture: A systematic literature review. Electronics, 9 (2), 319. https://doi.org/10.3390/electronics9020319
Ferrag, M. A., Shu, L., Yang, X., Derhab, A., & Maglaras, L. (2020). Security and privacy for Green IoT-based agriculture: review, Blockchain solutions, and challenges. IEEE Access, 8 , 32031–32053. https://doi.org/10.1109/ACCESS.2020.2973178
Sobin, C. C. (2020). A survey on architecture, protocols and challenges in IoT. Wireless Personal Communications, 112 , 1383–1429. https://doi.org/10.1007/s11277-020-07108-5
Klompenburg, T., van Kassahun, A., & Catal, C. (2020). Crop yield prediction using machine learning: A systematic literature review. Computers and Electronics in Agriculture, 177 , 105709. https://doi.org/10.1016/j.compag.2020.105709
Vitali, G., Francia, M., Golfarelli, M., & Canavari, M. (2021). Crop management with the IoT: An interdisciplinary survey. Agronomy, 11 , 1–18. https://doi.org/10.3390/agronomy11010181
Vorosmarty, C. J., McIntyre, P. B., Gessner, M. O., Dudgeon, D., Prusevich, A., Green, P., Glidden, S., Bunn, S. E., Sullivan, C. A., Reidy Liermann, C., & Davies, P. M. (2010). Global threats to human water security and river biodiversity. Nature, 467 , 555–561. https://doi.org/10.1038/nature09440
(Online) https://www.gktoday.in/gk/issues-and-challenges-in-irrigation/ . Accessed 3 Mar 2021
(Online) http://www.fao.org/3/s8684e/s8684e08.htm . Accessed 3 Mar 2021
Hsiao, T. C., Steduto, P., & Fereres, E. (2007). A systematic and quantitative approach to improve water use efficiency in agriculture. Irrigation science, 25 (3), 209–231.
Chen, K. T., Zhang, H. H., Wu, T. T., Hu, J., Zhai, C. Y., & Wang, D. (2014). Design of monitoring system for multilayer soil temperature and moisture based on WSN. In 2014 international conference on wireless communication and sensor network (pp. 425–430). https://doi.org/10.1109/WCSN.2014.92
Shock, C., Pereira, A., Feibert, E., Shock, C., Akin, A., & Unlenen, L. (2016). Field comparison of soil moisture sensing using neutron thermalization, frequency domain, tensiometer, and granular matrix sensor devices: relevance to precision irrigation. Journal of Water Resource and Protection, 8 , 154–167. https://doi.org/10.4236/jwarp.2016.82013
(Online) https://www.elprocus.com/smart-irrigation-system-using-iot/ . Accessed 7 Mar 2021
(Online) https://www.instructables.com/id/SMART-IRRIGATION-SYSTEM-Using-IoT/ . Accessed 7 Mar 2021
(Online) https://circuitdigest.com/microcontroller-projects/iot-based-smart-irrigation-system-using-esp8266-and-soil-moisture-sensor . Accessed 7 Mar 2021
Gondchawar, N., & Kawitkar, P. R. S. (2016). IoT based smart agriculture. International Journal of Advanced Research in Computer and Communication Engineering, 5 (6), 838–842. https://doi.org/10.17148/IJARCCE.2016.56188
Prathibha, S. R., Hongal, A., & Jyothi, M. P. (2017). IoT based monitoring system in smart agriculture. In International conference on recent advances in electronics and communication technology ( ICRAECT ), 2017 (pp. 81–84). IEEE. https://doi.org/10.1109/ICRAECT.2017.52
Suma, N., Samson, S. R., Saranya, S., Shanmugapriya, G., & Subhashri, R. (2017). IOT based smart agriculture monitoring system. International Journal on Recent and Innovation Trends in computing and communication, 5 (2), 177–181.
Google Scholar
Rawal, S. (2017). IOT based smart irrigation system. International Journal of Computers and Applications, 159 , 7–11. https://doi.org/10.5120/ijca2017913001
Nesa Sudha, M., Valarmathi, M. L., & Babu, A. S. (2011). Energy efficient data transmission in automatic irrigation system using wireless sensor networks. Computers and Electronics in Agriculture, 78 , 215–221. https://doi.org/10.1016/j.compag.2011.07.009
Kavianand, G., Nivas, V. M., Kiruthika, R., & Lalitha, S. (2016). Smart drip irrigation system for sustainable agriculture. In Proceedings—2016 IEEE international conference on technological innovations in ICT for agriculture and rural development , 2016 (pp. 19–22). https://doi.org/10.1109/TIAR.2016.7801206
Viani, F., Bertolli, M., Salucci, M., & Polo, A. (2017). Low-cost wireless monitoring and decision support for water saving in agriculture. IEEE Sensors Journal, 17 (13), 4299–4309. https://doi.org/10.1109/JSEN.2017.2705043
Tilling, A. K., O’Leary, G. J., Ferwerda, J. G., Jones, S. D., Fitzgerald, G. J., Rodriguez, D., & Belford, R. (2007). Remote sensing of nitrogen and water stress in wheat. Field Crops Research, 104 (1–3), 77–85. https://doi.org/10.1016/j.fcr.2007.03.023
(Online) https://www.nrcs.usda.gov/Internet/FSE_DOCUMENTS/nrcs142p2_053293.pdf . Accessed 15 Mar 2021
(Online) http://soilquality.org.au/factsheets/soil-nitrogen-supply . Accessed 15 Mar 2021
(Online) https://nrcca.cals.cornell.edu/soilFertilityCA/CA1/CA1_print.html . Accessed 15 Mar 2021
(Online) http://aesl.ces.uga.edu/publications/soil/CropSheets.pdf . Accessed 15 Mar 2021
Kumar, K., & Goh, K. M. (1999). Crop residues and management practices: Effects on soil quality, soil nitrogen dynamics, crop yield, and nitrogen recovery. Advances in Agronomy, 68 , 197–319. https://doi.org/10.1016/S0065-2113(08)60846-9
(Online) http://www.fao.org/3/a0443e/a0443e.pdf . Accessed 15 Mar 2021
Chen, W. L., Lin, Y. B., Lin, Y. W., Chen, R., Liao, J. K., Chan, Y., Liu, Y., Wang, C., Chiu, C., & Yen, T. (2019). AgriTalk IoT for precision soil farming of turmeric cultivation. IEEE Internet Things Journal, 2019 (6), 5209–5223. https://doi.org/10.1109/JIOT.2019.2899128
Reeves, D. W. (1997). The role of soil organic matter in maintaining soil quality in continuous cropping systems. Soil and Tillage Research, 43 , 131–167. https://doi.org/10.1016/S0167-1987(97)00038-X
Romero, I., Benito, A., Dominguez, N., Garcia-Escudero, E., & Martin, I. (2014). Leaf blade and petiole nutritional diagnosis for Vitis vinifera L. cv. “Tempranillo” by deviation from optimum percentage method. Spanish Journal of Agricultural Research, 12 , 206–214. https://doi.org/10.5424/sjar/2014121-4308
Kapse, S., & Kale, S. (2020). IOT enable soil testing & NPK nutrient detection. A Journal of Composition Theory, XIII , 310–318.
Barnes, E. M., Sudduth, K. A., Hummel, J. W., Lesch, S. M., Corwin, D. L., Yang, C., Daughtry, C. S. T., & Bausch, W. C. (2003). Remote- and ground-based sensor techniques to map soil properties. Photogrammetric Engineering & Remote Sensing, 69 , 619–630.
Ali, A., Dong, L., Dhau, J., Khosla, A., & Kaushik, A. (2020). Perspective—electrochemical sensors for soil quality assessment. Journal of The Electrochemical Society . https://doi.org/10.1149/1945-7111/ab69fe
Yin, L., & Zhang, Y. (2020). Microprocessors and Microsystems Village precision poverty alleviation and smart agriculture based on FPGA and machine learning. Microprocessors and Microsystems, 1 , 103469. https://doi.org/10.1016/j.micpro.2020.103469
Kumar, P. N., Manikanta, K. B., Venkatesh, B. Y., Kumar, R. N., & Patil, A. M. (2020). Smart agricultural crop prediction using machine learning. Journal of Xi’an University of Architecture & Technology, 12 (V).
Ahila, S. S., Dinesh, G., Kavya, S., & Anandkumar, K. M. (2020). Demand based crop prediction using machine learning algorithm. European Journal of Molecular & Clinical Medicine, 7 (8), 2075–2090.
Adoghe, A. U., Popoola, S. I., Chukwuedo, O. M., Airoboman, A. E., & Atayero, A. A. (2017). Smart weather station for rural agriculture using meteorological sensors and solar energy
Radhika, Y., & Shashi, M. (2009). Atmospheric temperature prediction using support vector machines. International Journal of Computer Theory and Engineering, 1 (1), 55. https://doi.org/10.7763/IJCTE.2009.V1.9
G. Chavan, \& B. Momin ( 2017, February). An integrated approach for weather forecasting over Internet of Things: A brief review. In IEEE international conference on I-SMAC ( IoT in social, mobile, analytics and cloud ), 2017, (pp. 83–88).
Gumaste, S. S., & Kadam, A. J. (2016). Future weather prediction using genetic algorithm and FFT for smart farming. In Proceedings of the second international conference on, communication, control and automation , 2016 (pp. 1–6). https://doi.org/10.1109/ICCUBEA.2016.7860028
Kiran, S., Kanumalli, S. S., Sai Rama Krishna, K. V. S., & Chandra, N. (2021). Internet of things integrated smart agriculture for weather predictions and preventive mechanism. Materials Today Proceedings . https://doi.org/10.1016/j.matpr.2020.11.081
Zhu, N., Liu, X., Liu, Z., Hu, K., Wang, Y., Tan, J., Huang, M., Zhu, Q., Ji, X., Jiang, Y., & Guo, Y. (2018). Deep learning for smart agriculture: Concepts, tools, applications, and opportunities. International Journal of Agricultural and Biological Engineering, 11 , 21–28. https://doi.org/10.25165/j.ijabe.20181104.4475
Demir, K. (2022). Cultivation planning across Europe using machine learning techniques. Avrupa Bilim ve Teknoloji Dergisi, 21 , 697–707. https://doi.org/10.31590/ejosat.822785
Yin, H., Jin, D., Gu, Y. H., Park, C. J., Han, S. K., & Yoo, S. J. (2020). STL-ATTLSTM: Vegetable price forecasting using STL and attention mechanism-based LSTM. Agriculture, 10 (12), 612.
Varun, R., Neema, N., Sahana, H. P., Sathvik, A., & Muddasir, M. (2019). Agriculture commodity price forecasting using ML techniques. The International Journal of Innovative Technology and Exploring Engineering, 9 , 729–732. https://doi.org/10.35940/ijitee.b1226.1292s19
Rajeswari, S., & Suthendran, K. (2019). Developing an agricultural product price prediction model using HADT algorithm. The International Journal of Engineering and Advanced Technology, 9 , 569–575. https://doi.org/10.35940/ijeat.a1126.1291s419
Rohith, R., Vishnu, R., Kishore, A., & Chakkarawarthi, D. (2020). Crop price prediction and forecasting system using supervised machine learning algorithms. International Journal of Advanced Research in Computer and Communication Engineering, 9 , 27–29. https://doi.org/10.17148/IJARCCE.2020.9306
Nasira, G. M., & Hemageetha, N. (2012). Forecasting model for vegetable price using back propagation neural network. International Journal of Computational Intelligence and Informatics, 2 (2), 110–115.
Sabu, K. M., & Kumar, T. K. M. (2020). Predictive analytics in agriculture: Forecasting prices of Arecanuts in Kerala. Procedia Computer Science, 171 , 699–708. https://doi.org/10.1016/j.procs.2020.04.076
Fang, Y., Guan, B., Wu, S., & Heravi, S. (2020). Optimal forecast combination based on ensemble empirical mode decomposition for agricultural commodity futures prices. Journal of Forecasting, 39 , 877–886. https://doi.org/10.1002/for.2665
Article MathSciNet Google Scholar
Kamruzzaman, S. M., Pavel, M. I., & Sabuj, S. R. (2019). Promoting greenness with IoT-based plant growth system: intelligence and promoting greenness with IoT based plant growth system. https://doi.org/10.1007/978-3-030-02674-5
Sarangdhar, A. A., & Pawar, V. R. (2017). Machine learning regression technique for cotton leaf disease detection and controlling using IoT. In Proceedings of the international conference of electronics, communication and aerospace technology ( ICECA ), Coimbatore, India , 20–22 April 2017 (Vol. 2, pp. 449–454).
Truong, T., Dinh, A., & Wahid, K. (2017). An IoT environmental data collection system for fungal detection in crop fields. In 2017 IEEE 30th Canadian conference on electrical and computer engineering ( CCECE ), 2017 (pp. 1–4).
Jean, U., Santos, L., Pessin, G., André, C., & Righi, R. (2018). AgriPrediction: A proactive internet of things model to anticipate problems and improve production in agricultural crops. Computers and Electronics in Agriculture . https://doi.org/10.1016/j.compag.2018.10.010
Jayaraman, P. P., Yavari, A., Georgakopoulos, D., Morshed, A., & Zaslavsky, A. (2016). Internet of things platform for smart farming: Experiences and lessons learnt. Sensors, 16 (11), 1884. https://doi.org/10.3390/s16111884
Adesipo, A., Fadeyi, O., Kuca, K., & Krejcar, O. (2020). Smart and climate-smart agricultural trends as core aspects of smart village functions. Sensors, 20 , 1–22.
Dong, X., Vuran, M. C., & Irmak, S. (2013). Autonomous precision agriculture through integration of wireless underground sensor networks with center pivot irrigation systems. Ad Hoc Networks, 11 (7), 1975–1987. https://doi.org/10.1016/j.adhoc.2012.06.012
(Online) https://www.statista.com/statistics/471264/iot-number-of-connected-devices-worldwide/ . Accessed 5 April 2021
(Online) ITU-T, 2016. Recommendation ITU-T Y.2060, IoT Reference Model, Overview of the Internet of Things. Telecommunication Standardization sector of ITU. http://www.itu.int/itu-t/recommendations/rec.aspx?rec=Y.2060 . Accessed 5 April 2021
Balaji, S., Nathani, K., & Santhakumar, R. (2019). IoT technology, applications and challenges: A contemporary survey. Wireless Personal Communications, 108 (1), 363–388.
Verdouw, C., Sundmaeker, H., Tekinerdogan, B., Conzon, D., & Montanaro, T. (2019). Architecture framework of IoT-based food and farm systems: A multiple case study. Computers and Electronics in Agriculture, 165 , 104939.
P. Fremantle, 2015. A reference architecture for the internet of things. WSO2 White paper.
(Online) https://www.link-labs.com/blog/6lowpan-vs-zigbee . Accessed 19 April 2021
Shelby, Z., & Bormann, C. (2011). 6LoWPAN: The wireless embedded Internet . John Wiley & Sons.
Nicolae, M., Popescu, D., Merezeanu, D., & Ichim, L. (2018). Large scale wireless sensor networks based on fixed nodes and mobile robots in precision agriculture. In International conference on robotics in Alpe-Adria Danube Region (pp. 236–244). Cham: Springer.
Bor, M., Vidler, J. E., & Roedig, U. (2016). LoRa for the Internet of Things.
Nobrega, L., Gonçalves, P., Pedreiras, P., & Pereira, J. (2019). An IoT-based solution for intelligent farming. Sensors, 19 (3), 603. https://doi.org/10.3390/s19030603
(Online) www.networkworld.com/article/3284506/5-reasons-the-iot-needs-its-own-networks.html . Accessed 19 April 2021
(Online) https://behrtech.com/blog/iot-standards-and-protocols-explained/ . Accessed 19 April 2021
(Online) https://mioty-alliance.com/miotytechnology/ . Accessed 7 Mar 2021
Ullah, U., Khan, A., Mahdi, Z., Ihsan, A., Khattak, H. A., & Din, I. U. (2019). Energy-effective cooperative and reliable delivery Routinf protocols for underwater wireless sensor networks. Energies . https://doi.org/10.3390/en12132630
Haseeb, K., Islam, N., Almogren, A., & Din, I. U. D. (2019). Intrusion prevention framework for secure routing in WSN-based mobile Internet of Things. IEEE Access, 7 , 185496–185505. https://doi.org/10.1109/ACCESS.2019.2960633
Enam, R. N., Qureshi, R., & Misbahuddin, S. (2014). A uniform clustering mechanism for wireless sensor networks. International Journal of Distributed Sensor Networks . https://doi.org/10.1155/2014/924012
Zhu, C., Wu, S., Han, G., & Shu, L. E. I. (2015). A tree-cluster-based data-gathering algorithm for industrial WSNs with a mobile sink. IEEE Access, 3 , 381–396. https://doi.org/10.1109/ACCESS.2015.2424452
Heinzelman, W. R., Chandrakasan, A., & Balakrishnan, H. (2000). Energy-efficient communication protocol for wireless microsensor networks. In Proceedings of the IEEE 33rd annual Hawaii international conference on system sciences .
Karaca, O., Sokullu, R., & Prasad, N. R. (2012). Application oriented multi criteria optimization in WSNs using on AHP. Wireless Personal Communications, 65 , 689–712. https://doi.org/10.1007/s11277-011-0280-0
Jain, B., Brar, G., & Malhotra, J. (2018). EKMT-k-means clustering algorithmic solution for low energy consumption for wireless sensor networks based on minimum mean distance from base station. In Networking communication and data knowledge engineering, Ger. 2018, (Vol. 3, pp. 113–123). Berlin: Springer,. https://doi.org/10.1007/978-981-10-4585-1_10
Haseeb, K., Ud Din, I., Almogren, A., & Islam, N. (2020). An energy efficient and secure IoT-based WSN framework: An application to smart agriculture. Sensors, 20 (7), 2081.
L. Touseau, N.L. Sommer. Contribution of the web of things and of the opportunistic computing to the smart agriculture: A practical experiment. Future of the Internet 11:33 https://doi.org/10.3390/fi11020033
Kulatunga, C., Shalloo, L., Donnelly, W., Robson, E., & Ivanov, S. (2017). Opportunistic wireless networking for smart dairy farming. IT Professional, 19 , 16–23. https://doi.org/10.1109/MITP.2017.28
Kamilaris, A., Kartakoullis, A., & Prenafeta-boldu, F. X. (2017). A review on the practice of big data analysis in agriculture. Computers and Electronics in Agriculture, 143 , 23–37. https://doi.org/10.1016/j.compag.2017.09.037
Barbosa, A., Trevisan, R., Hovakimyan, N., & Martin, N. F. (2020). Modeling yield response to crop management using convolutional neural networks. Computers and Electronics in Agriculture, 170 , 105197.
Lin, Y. W., Lin, Y. B., & Liu, C. Y. (2019). AITalk: A tutorial to implement AI as IoT devices. IET Networks, 8 , 195–202. https://doi.org/10.1049/iet-net.2018.5182
Ramos, P. J., Prieto, F. A., Montoya, E. C., & Oliveros, C. E. (2017). Automatic fruit count on coffee branches using computer vision. Computers and Electronics in Agriculture, 137 , 9–22. https://doi.org/10.1016/j.compag.2017.03.010
Avendano, J., Ramos, P. J., & Prieto, F. A. (2017). A system for classifying vegetative structures on coffee branches based on videos recorded in the field by a mobile device. Expert Systems with Applications, 88 , 178–192. https://doi.org/10.1016/j.eswa.2017.06.044
Peng, H., Huang, J., Jin, H., Sun, H., Chai, D., Wang, X., Han, B., Zhou, Z., & Xu, L. (2018, August). Detecting coffee (Coffea arabica L.) sequential flowering events based on image segmentation. In 7th IEEE International Conference on Agro-geoinformatics ( Agro-geoinformatics ) (pp. 1–6). https://doi.org/10.1109/Agro-Geoinformatics.2018.8476057
Tellaeche, A., BurgosArtizzu, X. P., Pajares, G., & Ribeiro, A. (2007). A vision-based hybrid classifier for weeds detection in precision agriculture through the Bayesian and Fuzzy kMeans paradigms. In Innovations in hybrid intelligent systems. Berlin: Springer.
Meyer, G. E., Camargo Neto, J., Jones, D. D., & Hindman, T. W. (2004). Intensified fuzzy clusters for classifying plant, soil, and residue regions of interest from color images. Computers and Electronics in Agriculture, 42 (3), 161–180.
Calo, S. B., Touna, M., Verma, D. C., & Cullen, A. (2017). Edge computing architecture for applying AI to IoT. In Proceedings—2017 IEEE International Conference on Big Data (Big Data), Boston, MA (pp. 3012–3016). https://doi.org/10.1109/BigData.2017.8258272
Debauche, O., Mahmoudi, S., Mahmoudi, S. A., Manneback, P., & Lebeau, F. (2020). A new edge architecture for AI-IoT services deployment. Procedia Computer Science, 175 , 10–19. https://doi.org/10.1016/j.procs.2020.07.006
(Online) https://www.cmswire.com/information-management/edge-computing-vs-fog-computing-whats-the-difference/amp/ . Accessed 24 April 2021
Capra, M., Peloso, R., Masera, G., Roch, M. R., & Martina, M. (2019). Edge computing: A survey on the hardware requirements in the Internet of Things world. Future of the Internet, 11 , 1–25. https://doi.org/10.3390/fi11040100
Dai, B., Xu, G., Huang, B., Qin, P., & Xu, Y. (2017). Enabling network innovation in data center networks with software defined networking: A survey. Journal of Network and Computer Applications, 94 , 33–49. https://doi.org/10.1016/j.jnca.2017.07.004
(Online) https://iot-epi.eu/wp-content/uploads/2018/07/Advancing-IoT-Platform-Interoperability-2018-IoT-EPI.pdf . Accessed 26 April 2021
(Online) Rodriguez, A. Restful web services: The basics. IBM Developer Works. Available online: https://cs.calvin.edu/courses/cs/262/kvlinden/references/rodriguez-restfulWS.pdf , Accessed 26 April 2021
Zhu, Q., Wang, R., Chen, Q., Liu, Y., & Qin, W. (2010) IoT gateway: Bridging wireless sensor networks into internet of things. In Proceedings of the IEEE/IFIP 8th International Conference on Embedded and Ubiquitous Computing, Hong Kong, China , 2011–2010 .
Guoqiang, S., Yanming, C., Chao, Z., Yanxu, Z. (2013). Design and implementation of a smart IoT gateway. In Proceedings of the IEEE international conference on green computing and communications and IEEE internet of things and IEEE cyber, physical and social computing, Beijing, China , 20–23 August 2013 .
Datta, S. K., Bonnet, C., & Nikaein, N. (2014). An IoT gateway centric architecture to provide novel M2M services. In Proceedings of the IEEE world forum on internet of things, Seoul, Korea , 6–8 March 2014.
Desai, P., Sheth, A., & Anantharam, P. (2015). Semantic gateway as a service architecture for IoT interoperability. In 2015 IEEE international conference on mobile services , (pp. 313–319).
Aloi, G., Caliciuri, G., Fortino, G., Gravina, R., Pace, P., Russo, W., & Savaglio, C. (2016). A mobile multi-technology gateway to enable IoT interoperability. In IEEE First International Conference on Internet-of-Things Design and Implementation ( IoTDI ) (pp. 259–264).
Broring, A., Schmid, S., Schindhelm, C. K., Khelil, A., Kabisch, S., Kramer, D., Le Phuoc, D., Mitic, J., Anicic, D., & Teniente, E. (2017). Enabling IoT ecosystems through platform interoperability. IEEE Software, 34 (1), 54–61.
Derhamy, H., Eliasson, J., & Delsing, J. (2017). IoT interoperability—On-demand and low latency transparent multiprotocol translator. IEEE Internet of Things Journal, 4 (5), 1754–1763.
(Online) https://www.onem2m.org/tr-0034/architecture . Accessed 26 April 2021
Al-Osta, M., Ahmed, B., & Abdelouahed, G. (2017). A lightweight semantic web-based approach for data annotation on IoT gateways. Procedia Computer Science, 113 , 186–193.
Cimmino, A., Poveda-Villalon, M., & García-Castro, R. (2020). eWoT: A semantic interoperability approach for heterogeneous IoT ecosystems based on the web of things. Sensors, 20 (3), 822.
(Online) https://www.intel.com/content/dam/www/public/us/en/documents/product-briefs/gateway-solutions-iot-brief.pdf . Accessed 26 April 2021
(Online) https://portal.etsi.org/TB-SiteMap/SmartM2M/SmartM2M-ToR . Accessed 26 April 2021
(Online) https://dcsa.org/wp-content/uploads/2020/09/DCSA-P2-Gateway-Connectivity-Interfaces-Standards_v1.0_FINAL.pdf . Accessed 26 April 2021
(Online) https://omaspecworks.org/what-%20is-oma-specworks/iot/lightweight-m2m-lwm2m/ . Accessed 26 April 2021
Kamienski, C., Kleinschmidt, J. H., Soininen, J., Kolehmainen, K., Roffia, L., Visoli, M., Maia, R. F., & Fernandes, S. (2018). SWAMP: smart water management platform overview and security challenges. In 48th Annual IEEE/IFIP international conference on dependable systems and networks work . (pp. 49–50). https://doi.org/10.1109/DSN-W.2018.00024
Devi, M. S., Suguna, R., Joshi, A. S., & Bagate, R. A. (2019, February). Design of IoT blockchain based smart agriculture for enlightening safety and security. In International conference on emerging technologies in computer engineering (pp. 7–19). Singapore: Springer. https://doi.org/10.1007/978-981-13-8300-7
Frustaci, M., Pace, P., Aloi, G., & Fortino, G. (2017). Evaluating critical security issues of the IoT world: Present and future challenges. IEEE Internet of Things Journal, 5 (4), 2483–2495. https://doi.org/10.1109/JIOT.2017.2767291
Canedo, J., & Skjellum, A. (2016). Using machine learning to secure IoT systems. In 14th IEEE annual conference on privacy, security and trust ( PST ), 2016 , (pp. 219–222).
Giordano, S., Seitanidis, I, Ojo, M., Adami, D., & Vignoli, F. (2018). IoT solutions for crop protection against wild animal attacks. In IEEE international conference on environmental engineering (EE) , March 2018 (pp. 1–5).
Ayele, E. D., Meratnia, N., & Havinga, P. J. (2018). Towards a new opportunistic IoT network architecture for wildlife monitoring system. In 2018 9th IFIP international conference on new technologies, mobility and security ( NTMS ), February 2018 (pp. 1–5). IEEE.
(Online) https://www.financialexpress.com/opinion/blockchain-ai-iot-how-india-can-help-farmers-by-leveraging-these-technologies/1970409/ . Accessed on 3 May 2021.
(Online) https://www.precisionag.com/digital-farming/how-iot-solutions-for-indian-agriculture-are-working-despite-unique-challenges/.Accessed 3 May 2021.
(Online) https://www.precisionag.com/digital-farming/how-digitization-is-moving-indian-agriculture-forward-in-the-wake-of-covid-19/ . Accessed 3 May 2021.
(Online) Monit Khanna, https://www.indiatimes.com/technology/news/sensegrass-farming-iot-india-innnovation-521214.html . Accessed 3 May 2021.
(Online) https://yourstory.com/2021/04/hyderbad-agritech-startup-onebasket-farmers-supply-chain/amp.Accessed 3 May 2021
(Online) https://yourstory.com/2020/12/stellaris-venture-partners-ifc-ai4biz-ai-startups-saas/amp . Accessed 3 May 2021
(Online) https://wap.business-standard.com/article/economy-policy/e-marketplace-for-marine-products-to-raise-farmer-income-piyush-goyal-1210413010461.html . Accessed 3 May 2021
(Online) https://iot.electronicsforu.com/expert-opinion/internet-of-things-in-agriculture-india/ . Accessed 3 May 2021
(Online) https://www.thebetterindia.com/251873/sudhanshu-kumar-bihar-farmer-scientific-technology-earns-lakhs-horticulture-fruit-orchards-agriculture-him16/amp/ . Accessed on 3rd May 2021
(Online) https://agricoop.nic.in/sites/default/files/Guideline%20of%20SMAM%20%20Scheme%2020-21.pdf . Accessed 30 May 2021
(Online) https://krishijagran.com/agripedia/best-government-schemes-and-programmes-in-agriculture-for-farmers/ . Accessed 30 May 2021
(Online) https://ruralmarketing.in/stories/11-government-schemes-in-agriculture-that-every-farmer-need-to-know/ . Accessed 30 May 2021
(Online) https://www.adriindia.org/adri/india_water_facts . Accessed 3 May 2021
(Online) https://machinelearningmastery.com/arima-for-time-series-forecasting-with-python/ . Accessed 3 April 2021
Bacco, M., Barsocchi, P., Ferro, E., Gotta, A., & Ruggeri, M. (2019). The digitisation of agriculture: a survey of research activities on smart farming. Array . https://doi.org/10.1016/j.array.2019.100009
Talavera, J. M., Tobon, L. E., Gomez, J. A., Culman, M. A., Aranda, J. M., Parra, D. T., Quiroz, L. A., Hoyos, A., & Garreta, L. E. (2017). Review of IoT applications in agro-industrial and environmental fields. Computers and Electronics in Agriculture, 142 , 283–297. https://doi.org/10.1016/j.compag.2017.09.015
Gwynn-Jones, D., Dunne, H., Donnison, I., Robson, P., Sanfratello, G. M., Schlarb-Ridley, B., Hughes, K., & Convey, P. (2018). Can the optimisation of pop-up agriculture in remote communities help feed the world? Global Food Security, 18 , 35–43.
Bybee-Finley, K., & Ryan, M. R. (2018). Advancing intercropping research and practices in industrialized agricultural landscapes. Agriculture, 8 (6), 80.
Download references
The authors declared no funding received.
Author information
Authors and affiliations.
Department of CSE, SRM University, Guntur, Andhra Pradesh, India
C. C. Sobin
LTRC, IIIT Hyderabad, Hyderabad, Telangana, India
School of Computing, University of Bolton, Bolton, United Kingdom
You can also search for this author in PubMed Google Scholar
Corresponding author
Correspondence to C. C. Sobin .
Ethics declarations
Conflicts of interest.
The authors declared no potential conflicts of interest with respect to the research, authorship, and/or publication of this article.
Ethics approval
All procedures performed in studies involving human participants were in accordance with the ethical standards of the institutional and/or national research committee and with the 1964 Helsinki declaration and its later amendments or comparable ethical standards.
Consent to participate
Not applicable.
Consent for publication
The Author transfers to Springer (respective to owner if other than Springer and for U.S. government employees: to the extent transferable) the non-exclusive publication rights and he warrants that his/her contribution is original and that he/she has full power to make this grant. The author signs for and accepts responsibility for releasing this material on behalf of any and all co-authors. This transfer of publication rights covers the non-exclusive right to reproduce and distribute the article, including reprints, translations, photographic reproductions, microform, electronic form (offline, online) or any other reproductions of similar nature.
Additional information
Publisher's note.
Springer Nature remains neutral with regard to jurisdictional claims in published maps and institutional affiliations.
Rights and permissions
Springer Nature or its licensor (e.g. a society or other partner) holds exclusive rights to this article under a publishing agreement with the author(s) or other rightsholder(s); author self-archiving of the accepted manuscript version of this article is solely governed by the terms of such publishing agreement and applicable law.
Reprints and permissions
About this article
Alex, N., Sobin, C.C. & Ali, J. A Comprehensive Study on Smart Agriculture Applications in India. Wireless Pers Commun 129 , 2345–2385 (2023). https://doi.org/10.1007/s11277-023-10234-5
Download citation
Accepted : 20 February 2023
Published : 23 March 2023
Issue Date : April 2023
DOI : https://doi.org/10.1007/s11277-023-10234-5
Share this article
Anyone you share the following link with will be able to read this content:
Sorry, a shareable link is not currently available for this article.
Provided by the Springer Nature SharedIt content-sharing initiative
- Smart agriculture
- Internet of things
- Machine learning
- Find a journal
- Publish with us
- Track your research
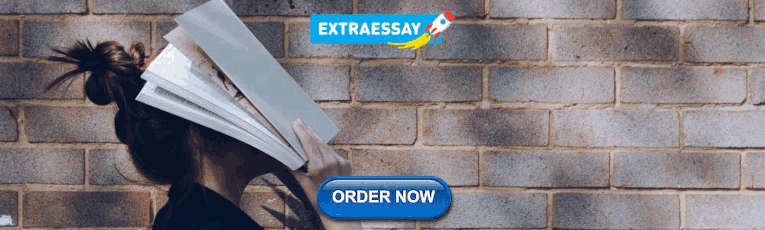
IMAGES
VIDEO
COMMENTS
Smart Agriculture is an evolution of precision agriculture by innovating smart methods to achieve multifunctional regarding the farm management remotely supported by alternatives appropriate solutions of farm management in real-time. ... surveyed 67 research papers that published through 2006 to 2019 on the use of IoT in different agricultural ...
The smart farming is a subset of precision agriculture, which is designed to control the suitable environments. for crops inside a greenhouse by using the least resources. It combines modern ...
agriculture is giving rise to agriculture 4.0, also known as smart a griculture, smart farming, or digital farming (Abbasi et al., 2022 ; Brewster et al., 2017 ). In smart
This paper provides an extensive review of the use of smart technologies in agriculture and elaborates the state-of-the-art technologies for smart agriculture including, Internet of Things, cloud computing, machine learning, and artificial intelligence. The application of smart farming to crop and animal production and post-harvesting is discussed.
Agriculture is backbone of any country. About 60% of our country's population works in agriculture or the primary sector. It contributes more to our country's GDP. It employs the majority of India's population. The internet of things research presents a framework in which farmers may obtain extensive information on the soil, crops growing in specific areas, and agricultural yield and ...
That fall impacts agricultural activity; sometimes, land stays barren and lacks fertility. This paper is focused on the deep analysis of smart farming-related components such as the Internet of Things (IoT), wireless communication technology, sensors, and hardware. ... Lack of smart research laboratories for farming. 6: Over 70% of rural ...
This paper presents a comprehensive review of emerging technologies for the internet of things (IoT)-based smart agriculture. We begin by summarizing the existing surveys and describing emergent technologies for the agricultural IoT, such as unmanned aerial vehicles, wireless technologies, open-source IoT platforms, software defined networking (SDN), network function virtualization (NFV ...
Smart farming is a development that has emphasized information and communication technology used in machinery, equipment, and sensors in network-based hi-tech farm supervision cycles. Innovative technologies, the Internet of Things (IoT), and cloud computing are anticipated to inspire growth and initiate the use of robots and artificial intelligence in farming. Such ground-breaking deviations ...
Finally, reviewed papers published between 2010 and 2015 and presents a state-of-the-art of IoT solutions for smart farming and smart agriculture. Authors relied on an IoT architecture with three layers (perception, network and application) to analyze the application of sensor and actuator devices and communication technologies within several ...
This paper showcases the potential ICT technologies in traditional agriculture, as well as the issues to be encountered when they are applied to farming practices. The challenges of robotics, IoT devices, and machine learning, as well as the roles of machine learning, artificial intelligence, and sensors used in agriculture, are all described ...
Abstract: Despite the perception people may have regarding the agricultural process, the reality is that today's agriculture industry is data-centered, precise, and smarter than ever. The rapid emergence of the Internet-of-Things (IoT) based technologies redesigned almost every industry including "smart agriculture" which moved the industry from statistical to quantitative approaches.
Smart Agriculture and Smart Farming using IoT Technology. V Dankan Gowda 1, M Sandeep Prabhu 2, M Ramesha 3, Jayashree M Kudari 4 and Ansuman Samal 5. Published under licence by IOP Publishing Ltd Journal of Physics: Conference Series, Volume 2089, 1st International Conference on Applied Mathematics, Modeling and Simulation in Engineering (AMSE) 2021 15-16 September 2021, India (Virtual ...
Smart farming with big data and advanced analytics technology includes automation, adding senses and analytics to modern agriculture. ... is a recently contributed package that provides access to real datasets from a large number of published agricultural research papers. The "agridat" datasets are formatted as a data frame .
Smart Farming using IoT, a solution for optimally monitoring farming conditions ... “Next Generation Everywhere Computing†. ‘Internet of Things’ is gaining an important place in research across the world and specially in area of advanced Available online at ... computers and electronics in agriculture original papers IoT ...
Abstract: This paper presents a comprehensive review of emerging technologies for the internet of things (IoT)-based smart agriculture. We begin by summarizing the existing surveys and describing emergent technologies for the agricultural IoT, such as unmanned aerial vehicles, wireless technologies, open-source IoT platforms, software defined networking (SDN), network function virtualization ...
Precision agriculture employs cutting-edge technologies to increase agricultural productivity while reducing adverse impacts on the environment. Precision agriculture is a farming approach that uses advanced technology and data analysis to maximize crop yields, cut waste, and increase productivity. It is a potential strategy for tackling some of the major issues confronting contemporary ...
Responses regarding adoption readiness of the smart farming technologies (SFTs) identified in scientific papers, research projects, and commercial products using the Rogers framework [102] based ...
The rest of this paper is organized as follows; Sect. 2 describes existing taxonomies in smart agriculture and proposed taxonomy is described in Sect. 3.Reference architecture for smart agriculture applications is listed in Sect. 4.Section 5 elaborates technologies used in smart agriculture applications and Sect. 6 mentions other recent technologies used by IoT systems.
The practice of cultivating the soil, producing crops, and keeping livestock is referred to as farming. Agriculture is critical to a country's economic development. Nearly 58 percent of a country's primary source of livelihood is farming. Farmers till date had adopted conventional farming techniques. These techniques were not precise thus ...
Chapter 10. Smart Farming: An Overview. Ahmad Latif Virk, Mehmood Ali Noor, Sajid Fiaz, Saddam Hussain, Ha fiz Athar Hussain, Muzammal Rehman, Muhammad Ahsan. and Wei Ma. Abstract Increasing ...
This research paper delves into the application of Internet of Things (IoT) technology in the context of smart farming within the Indian agricultural landscape. The paper aims to explore the current state of IoT adoption in Indian agriculture, its impact on farming practices, challenges faced, and
2 The state of climate-smart agriculture in the EU today 2.1 Farm economics 2.2 Awareness and knowledge 2.3 Data and technology 2.4 Policy 2.5 Farm diversity 3 The path forward: Opportunity spaces to accelerate the transition to climate-smart agriculture 3.1 Financing and risk management 3.2 Innovation ecosystems 3.3 Education and awareness
Glaroudis et al. (2020) also focused on the research challenges in the utilization of IoT protocols in smart farming and Farooq et al. (2019) studied the "Role of IoT in agriculture for the implementation of smart farming". Bacco et al. (2019) survey, "Research activities on smart farming" specialised in digitization agriculture.
The aim of this research paper is to highlight the concept of Internet of Things (IoT) and in addition to this, a Data Glove-An IoT based smart wearable gadget capable to perform various tasks ...