Have a language expert improve your writing
Run a free plagiarism check in 10 minutes, generate accurate citations for free.
- Knowledge Base
Methodology
- Textual Analysis | Guide, 3 Approaches & Examples
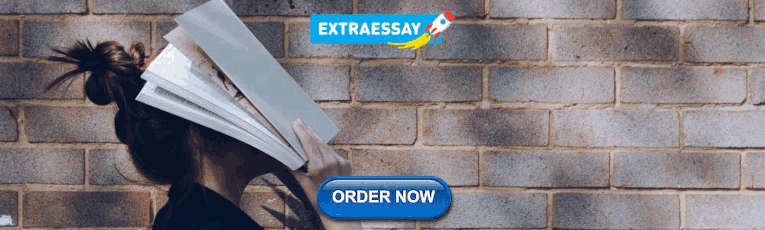
Textual Analysis | Guide, 3 Approaches & Examples
Published on November 8, 2019 by Jack Caulfield . Revised on June 22, 2023.
Textual analysis is a broad term for various research methods used to describe, interpret and understand texts. All kinds of information can be gleaned from a text – from its literal meaning to the subtext, symbolism, assumptions, and values it reveals.
The methods used to conduct textual analysis depend on the field and the aims of the research. It often aims to connect the text to a broader social, political, cultural, or artistic context. Relatedly, it’s good to be careful of confirmation bias when conducting these sorts of analyses, grounding your observations in clear and plausible ways.
Table of contents
What is a text, textual analysis in cultural and media studies, textual analysis in the social sciences, textual analysis in literary studies, other interesting articles.
The term “text” is broader than it seems. A text can be a piece of writing, such as a book, an email, or a transcribed conversation. But in this context, a text can also be any object whose meaning and significance you want to interpret in depth: a film, an image, an artifact, even a place.
The methods you use to analyze a text will vary according to the type of object and the purpose of your analysis:
- Analysis of a short story might focus on the imagery, narrative perspective and structure of the text.
- To analyze a film, not only the dialogue but also the cinematography and use of sound could be relevant to the analysis.
- A building might be analyzed in terms of its architectural features and how it is navigated by visitors.
- You could analyze the rules of a game and what kind of behaviour they are designed to encourage in players.
While textual analysis is most commonly applied to written language, bear in mind how broad the term “text” is and how varied the methods involved can be.
Receive feedback on language, structure, and formatting
Professional editors proofread and edit your paper by focusing on:
- Academic style
- Vague sentences
- Style consistency
See an example
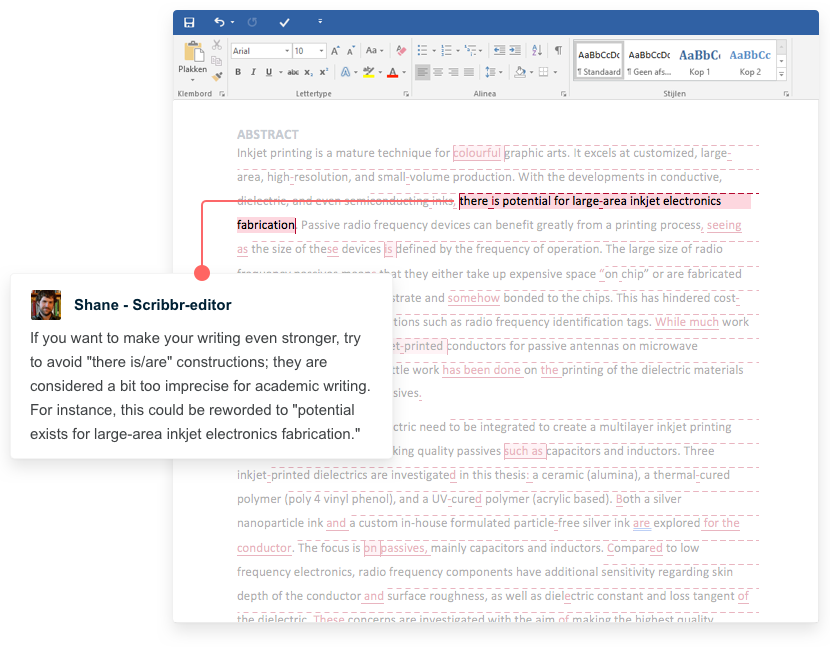
In the fields of cultural studies and media studies, textual analysis is a key component of research. Researchers in these fields take media and cultural objects – for example, music videos, social media content, billboard advertising – and treat them as texts to be analyzed.
Usually working within a particular theoretical framework (for example, using postcolonial theory, media theory, or semiotics), researchers seek to connect elements of their texts with issues in contemporary politics and culture. They might analyze many different aspects of the text:
- Word choice
- Design elements
- Location of the text
- Target audience
- Relationship with other texts
Textual analysis in this context is usually creative and qualitative in its approach. Researchers seek to illuminate something about the underlying politics or social context of the cultural object they’re investigating.
In the social sciences, textual analysis is often applied to texts such as interview transcripts and surveys , as well as to various types of media. Social scientists use textual data to draw empirical conclusions about social relations.
Textual analysis in the social sciences sometimes takes a more quantitative approach , where the features of texts are measured numerically. For example, a researcher might investigate how often certain words are repeated in social media posts, or which colors appear most prominently in advertisements for products targeted at different demographics.
Some common methods of analyzing texts in the social sciences include content analysis , thematic analysis , and discourse analysis .
Textual analysis is the most important method in literary studies. Almost all work in this field involves in-depth analysis of texts – in this context, usually novels, poems, stories or plays.
Because it deals with literary writing, this type of textual analysis places greater emphasis on the deliberately constructed elements of a text: for example, rhyme and meter in a poem, or narrative perspective in a novel. Researchers aim to understand and explain how these elements contribute to the text’s meaning.
However, literary analysis doesn’t just involve discovering the author’s intended meaning. It often also explores potentially unintended connections between different texts, asks what a text reveals about the context in which it was written, or seeks to analyze a classic text in a new and unexpected way.
Some well-known examples of literary analysis show the variety of approaches that can be taken:
- Eve Kosofky Sedgwick’s book Between Men analyzes Victorian literature in light of more contemporary perspectives on gender and sexuality.
- Roland Barthes’ S/Z provides an in-depth structural analysis of a short story by Balzac.
- Harold Bloom’s The Anxiety of Influence applies his own “influence theory” to an analysis of various classic poets.
Prevent plagiarism. Run a free check.
If you want to know more about statistics , methodology , or research bias , make sure to check out some of our other articles with explanations and examples.
- Normal distribution
- Measures of central tendency
- Chi square tests
- Confidence interval
- Quartiles & Quantiles
- Cluster sampling
- Stratified sampling
- Thematic analysis
- Cohort study
- Peer review
- Ethnography
Research bias
- Implicit bias
- Cognitive bias
- Conformity bias
- Hawthorne effect
- Availability heuristic
- Attrition bias
- Social desirability bias
Cite this Scribbr article
If you want to cite this source, you can copy and paste the citation or click the “Cite this Scribbr article” button to automatically add the citation to our free Citation Generator.
Caulfield, J. (2023, June 22). Textual Analysis | Guide, 3 Approaches & Examples. Scribbr. Retrieved April 1, 2024, from https://www.scribbr.com/methodology/textual-analysis/
Is this article helpful?
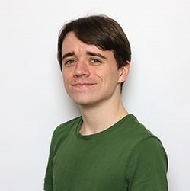
Jack Caulfield
Other students also liked, what is qualitative research | methods & examples, critical discourse analysis | definition, guide & examples, how to do thematic analysis | step-by-step guide & examples, what is your plagiarism score.
Literature Review: What Is Textual Analysis, and Can We Use It to Measure Corporate Culture?
- First Online: 25 April 2023
Cite this chapter
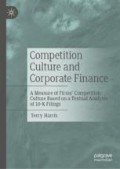
- Terry Harris 2
288 Accesses
The textual analysis approach also sometimes referred to as content analysis, computational linguistics, information retrieval, natural language processing, etc., refers to the systematic and objective quantification of the semantic content contained in a body of text. This notion of the parsing text to discover patterns allows for the unearthing of valuable information in text and has a long history and has been applied in many different contexts and disciplines.
This is a preview of subscription content, log in via an institution to check access.
Access this chapter
- Available as PDF
- Read on any device
- Instant download
- Own it forever
- Available as EPUB and PDF
- Durable hardcover edition
- Dispatched in 3 to 5 business days
- Free shipping worldwide - see info
Tax calculation will be finalised at checkout
Purchases are for personal use only
Institutional subscriptions
In later work, Bodnaruk et al. ( 2015 ) adopt a similar methodology to that used in Loughran and McDonald ( 2011 ) and propose “constraining” words, which are those words that suggest some financial constraint, for inclusion in the Loughran and McDonald word list.
Ahern, K. R., & Sosyura, D. (2014). Who Writes the News? Corporate Press Releases During Merger Negotiations. The Journal of Finance, 69 , 241–291.
Article Google Scholar
Andreou, P. C., Fiordelisi, F., Harris, T., & Philip, D. (2021). Institutional Ownership and Firms’ Thrust to Compete. British Journal of Management, 33 , 1346–1370.
Andreou, P. C., Harris, T., & Philip, D. (2020). Measuring Firms’ Market Orientation Using Textual Analysis of 10-K Filings. British Journal of Management, 31 , 872–895.
Antweiler, W., & Frank, M. Z. (2004). Is All That Talk Just Noise? The Information Content of Internet Stock Message Boards. The Journal of Finance, 59 , 1259–1294.
Audi, R., Loughran, T., & McDonald, B. (2016). Trust, but Verify: MD&A Language and the Role of Trust in Corporate Culture. Journal of Business Ethics, 139 , 551–561.
Barth, A. (2016). The Role of Corporate Culture in the Financial Industry . Available at SSRN 2707078.
Google Scholar
Bellstam, G., Bhagat, S., & Cookson, J. A. (2019). A Text-Based Analysis of Corporate Innovation . Available at SSRN 2803232.
Bhandari, A., Mammadov, B., Thevenot, M., & Vakilzadeh, S. H. (2017). The Invisible Hand: Corporate Culture and Its Implications for Earnings Management (Working Paper). Warrington College of Business, Gainesville, FL.
Biddle, G. C., Hilary, G., & Verdi, R. S. (2009). How does Financial Reporting Quality Relate to Investment Efficiency? Journal of Accounting and Economics, 48 , 112–131.
Blei, D. M., Ng, A. Y., & Jordan, M. I. (2003). Latent Dirichlet Allocation. Journal of Machine Learning Research, 3 , 993–1022.
Bodnaruk, A., Loughran, T., & McDonald, B. (2015). Using 10-K Text to Gauge Financial Constraints. Journal of Financial and Quantitative Analysis, 50 , 623–646.
Bonsall, S. B., IV., Leone, A. J., Miller, B. P., & Rennekamp, K. (2017). A Plain English Measure of Financial Reporting Readability. Journal of Accounting and Economics, 63 , 329–357.
Boukus, E., & Rosenberg, J. V. (2006). The Information Content of FOMC Minutes . Available at SSRN 922312.
Bushman, R. M., Hendricks, B. E., & Williams, C. D. (2016). Bank Competition: Measurement, Decision-Making, and Risk-Taking. Journal of Accounting Research, 54 , 777–826.
Chen, H., De, P., Hu, Y. J., & Hwang, B. H. (2014). Wisdom of Crowds: The Value of Stock Opinions Transmitted Through Social Media. The Review of Financial Studies , 27 , 1367–1403.
Cohen, L., Malloy, C., & Nguyen, Q. (2020). Lazy prices. The Journal of Finance, 75 , 1371–1415.
Da, Z., Engelberg, J., & Gao, P. (2015). The Sum of All FEARS Investor Sentiment and Asset Prices. The Review of Financial Studies, 28 , 1–32.
Davis, A. K., Piger, J. M., & Sedor, L. M. (2012). Beyond the Numbers: Measuring the Information Content of Earnings Press Release Language. Contemporary Accounting Research, 29 , 845–868.
Davis, A. K., & Tama-Sweet, I. (2012). Managers’ Use of Language Across Alternative Disclosure Outlets: Earnings Press Releases Versus MD&A. Contemporary Accounting Research, 29 , 804–837.
Das, S. R., & Chen, M. Y. (2007). Yahoo! for Amazon: Sentiment Extraction from Small Talk on the Web. Management Science, 53 , 1375–1388.
Doran, J. S., Peterson, D. R., & Price, S. M. (2012). Earnings Conference Call Content and Stock Price: The Case of REITs. The Journal of Real Estate Finance and Economics, 45 , 402–434.
Dougal, C., Engelberg, J., Garcia, D., & Parsons, C. A. (2012). Journalists and the Stock Market. The Review of Financial Studies, 25 , 639–679.
Dyer, T., Lang, M., & Stice-Lawrence, L. (2017). The Evolution of 10-K Textual Disclosure: Evidence from Latent Dirichlet Allocation. Journal of Accounting and Economics, 64 , 221–245.
Feldman, R., Govindaraj, S., Livnat, J., & Segal, B. (2010). Management’s Tone Change, Post Earnings Announcement Drift and Accruals. Review of Accounting Studies, 15 , 915–953.
Ferris, S. P., Hao, Q., & Liao, M. Y. (2013). The Effect of Issuer Conservatism on IPO Pricing and Performance. Review of Finance, 17 , 993–1027.
Fiordelisi, F., & Ricci, O. (2014). Corporate Culture and CEO Turnover. Journal of Corporate Finance, 28 , 66–82.
Fiordelisi, F., & Ricci, O. (2021). Corrigendum to “Corporate Culture and CEO Turnover”. Journal of Corporate Finance, 70 , 102064.
Fiordelisi, F., Renneboog, L., Ricci, O., & Lopes, S. S. (2019). Creative Corporate Culture and Innovation. Journal of International Financial Markets, Institutions and Money, 63 , 101137.
Garcia, D. (2013). Sentiment During Recessions. The Journal of Finance, 68 , 1267–1300.
Graham, J. R., Harvey, C. R., Grennen, J., & Rajgopal, S. (2022). Corporate Culture: Evidence from the Field. Journal of Financial Economics, 146 , 552–593.
Grennan, J. (2019). A Corporate Culture Channel: How Increased Shareholder Governance Reduces Firm Value . Available at SSRN 2345384.
Guiso, L., Sapienza, P., & Zingales, L. (2015a). Corporate Culture, Societal Culture, and Institutions. American Economic Review, 105 , 336–339.
Guiso, L., Sapienza, P., & Zingales, L. (2015b). The Value of Corporate Culture. Journal of Financial Economics, 117 , 60–76.
Guo, H., Yin, C., & Zeng, Y. (2022). How Does Firm‐Specific Investor Sentiment Affect the Value of Corporate Cash Holdings? British Journal of Management .
Hanley, K. W., & Hoberg, G. (2010). The Information Content of IPO Prospectuses. The Review of Financial Studies, 23 , 2821–2864.
Henry, E. (2008). Are Investors Influenced by How Earnings Press Releases Are Written? The Journal of Business Communication, 45 , 363–407.
Heston, S. L., & Sinha, N. R. (2015). News Versus Sentiment: Predicting Stock Returns from News Stories . Available at SSRN 2311310.
Hillert, A., Niessen-Ruenzi, A., & Ruenzi, S. (2014). Mutual Fund Shareholder Letter Tone-Do Investors Listen? (Working Paper). University of Mannheim, Mannheim, Germany.
Hoberg, G., & Phillips, G. (2010). Product Market Synergies and Competition in Mergers and Acquisitions: A Text-Based Analysis. Review of Financial Studies, 23 , 3773–3811.
Hoberg, G., & Phillips, G. (2016). Text-Based Network Industries and Endogenous Product Differentiation. Journal of Political Economy, 124 , 1423–1465.
Hoberg, G., Phillips, G., & Prabhala, N. (2014). Product Market Threats, Payouts, and Financial Flexibility. The Journal of Finance, 69 , 293–324.
Huang, A. H., Zang, A. Y., & Zheng, R. (2014a). Evidence on the Information Content of Text in Analyst Reports. The Accounting Review, 89 , 2151–2180.
Huang, X., Teoh, S. H., & Zhang, Y. (2014b). Tone Management. The Accounting Review, 89 , 1083–1113.
Kearney, C., & Liu, S. (2014). Textual Sentiment in Finance: A survey of Methods and Models. International Review of Financial Analysis, 33 , 171–185.
Kothari, S. P., Li, X., & Short, J. E. (2009). The Effect of Disclosures by Management, Analysts, and Business Press on Cost of Capital, Return Volatility, and Analyst Forecasts: A Study Using Content Analysis. The Accounting Review, 84 , 1639–1670.
Lawrence, A. (2013). Individual Investors and Financial Disclosure. Journal of Accounting and Economics, 56 , 130–147.
Lehavy, R., Li, F., & Merkley, K. (2011). The Effect of Annual Report Readability on Analyst Following and the Properties of Their Earnings Forecasts. The Accounting Review, 86 , 1087–1115.
Li, E. X., & Ramesh, K. (2009). Market Reaction Surrounding the Filing of Periodic SEC Reports. The Accounting Review, 84 , 1171–1208.
Li, F. (2008). Annual Report Readability, Current Earnings, and Earnings Persistence. Journal of Accounting and Economics, 45 , 221–247.
Li, F. (2010a). Textual Analysis of Corporate Disclosures: A Survey of the Literature. Journal of Accounting Literature, 29 , 143.
Li, F. (2010b). The Information Content of Forward-Looking Statements in Corporate Filings: A Naive Bayesian Machine Learning Approach. Journal of Accounting Research, 48 , 1049–1102.
Li, F. (2011). Managers’ Self-serving Attribution Bias, Overconfidence, and Corporate Financial Policies (Working Paper). University of Michigan, Ann Arbor, MI.
Li, F., Lundholm, R., & Minnis, M. (2013a). A Measure of Competition Based on 10-K Filings. Journal of Accounting Research, 51 , 399–436.
Li, K., Griffin, D., Yue, H., & Zhao, L. (2013b). How Does Culture Influence Corporate Risk-Taking? Journal of Corporate Finance, 23 , 1–22.
Li, K., Liu, X., Mai, F., & Zhang, T. (2020). The Role of Corporate Culture in Bad Times, Evidence from the COVID-19 Pandemic. Journal of Financial and Quantitative Analysis , 1–68.
Li, X. (2010c). The Impacts of Product Market Competition on the Quantity and Quality of Voluntary Disclosures. Review of Accounting Studies, 15 , 663–711.
Loughran, T., McDonald, B., & Yun, H. (2009). A Wolf in Sheep’s Clothing: The Use of Ethics-Related Terms in 10-K Reports. Journal of Business Ethics, 89 , 39–49.
Loughran, T., & McDonald, B. (2011). When Is a Liability Not a Liability? Textual Analysis, Dictionaries, and 10-Ks. The Journal of Finance, 66 , 35–65.
Loughran, T., & McDonald, B. (2013). IPO First-Day Returns, Offer Price Revisions, Volatility, and Form S-1 Language. Journal of Financial Economics, 109 , 307–326.
Loughran, T., & McDonald, B. (2014). Measuring Readability in Financial Disclosures. The Journal of Finance, 69 , 1643–1671.
Loughran, T., & McDonald, B. (2016). Textual Analysis in Accounting and Finance: A Survey. Journal of Accounting Research, 54 , 1187–1230.
Luu, H., Nguyen, L., & Wilson, J. O. S. (2022). Organizational Culture, Competition and Bank Loan Loss Provisions . Available at SSRN 3921043.
Mayew, W. J., & Venkatachalam, M. (2012). The Power of Voice: Managerial Affective States and Future Firm Performance. The Journal of Finance, 67 , 1–43.
Miller, B. P. (2010). The Effects of Reporting Complexity on Small and Large Investor Trading. The Accounting Review, 85 , 2107–2143.
Mosteller, F., & Wallace, D. (1964). Inference and Disputed Authorship: The Federalist . Addison-Wesley.
Nelson, K. K., & Pritchard, A. C. (2007). Litigation Risk and Voluntary Disclosure: The Use of Meaningful Cautionary Language. Available at SSRN 998590.
Nguyen, D. D., Hagendorff, J., & Eshraghi, A. (2018). Does a CEO’s Cultural Heritage Affect Performance Under Competitive Pressure? The Review of Financial Studies, 31 , 97–141.
Nguyen, D. D., Nguyen, L., & Sila, V. (2019). Does Corporate Culture Affect Bank Risk-Taking? Evidence from Loan-Level Data. British Journal of Management, 30 , 106–133.
Price, S. M., Doran, J. S., Peterson, D. R., & Bliss, B. A. (2012). Earnings Conference Calls and Stock Returns: The Incremental Informativeness of Textual Tone. Journal of Banking & Finance, 36 , 992–1011.
Rogers, J. L., Van Buskirk, A., & Zechman, S. L. (2011). Disclosure Tone and Shareholder Litigation. The Accounting Review, 86 , 2155–2183.
Simon, A. (1997). Administrative Behaviour (4th ed.). The Free Press.
Solomon, D. H., Soltes, E., & Sosyura, D. (2014). Winners in the Spotlight: Media Coverage of Fund Holdings as a Driver of Flows. Journal of Financial Economics, 113 , 53–72.
Tetlock, P. C. (2007). Giving Content to Investor Sentiment: The Role of Media in the Stock Market. The Journal of Finance, 62 , 1139–1168.
Tetlock, P. C., Saar-Tsechansky, M., & Macskassy, S. (2008). More Than Words: Quantifying Language to Measure Firms’ Fundamentals. The Journal of Finance, 63 , 1437–1467.
Download references
Author information
Authors and affiliations.
Accounting Department, Durham University, Durham, UK
Terry Harris
You can also search for this author in PubMed Google Scholar
Corresponding author
Correspondence to Terry Harris .
Rights and permissions
Reprints and permissions
Copyright information
© 2023 The Author(s), under exclusive license to Springer Nature Switzerland AG
About this chapter
Harris, T. (2023). Literature Review: What Is Textual Analysis, and Can We Use It to Measure Corporate Culture?. In: Competition Culture and Corporate Finance. Palgrave Macmillan, Cham. https://doi.org/10.1007/978-3-031-30156-8_3
Download citation
DOI : https://doi.org/10.1007/978-3-031-30156-8_3
Published : 25 April 2023
Publisher Name : Palgrave Macmillan, Cham
Print ISBN : 978-3-031-30155-1
Online ISBN : 978-3-031-30156-8
eBook Packages : Economics and Finance Economics and Finance (R0)
Share this chapter
Anyone you share the following link with will be able to read this content:
Sorry, a shareable link is not currently available for this article.
Provided by the Springer Nature SharedIt content-sharing initiative
- Publish with us
Policies and ethics
- Find a journal
- Track your research

Textual Analysis: Definition, Types & 10 Examples
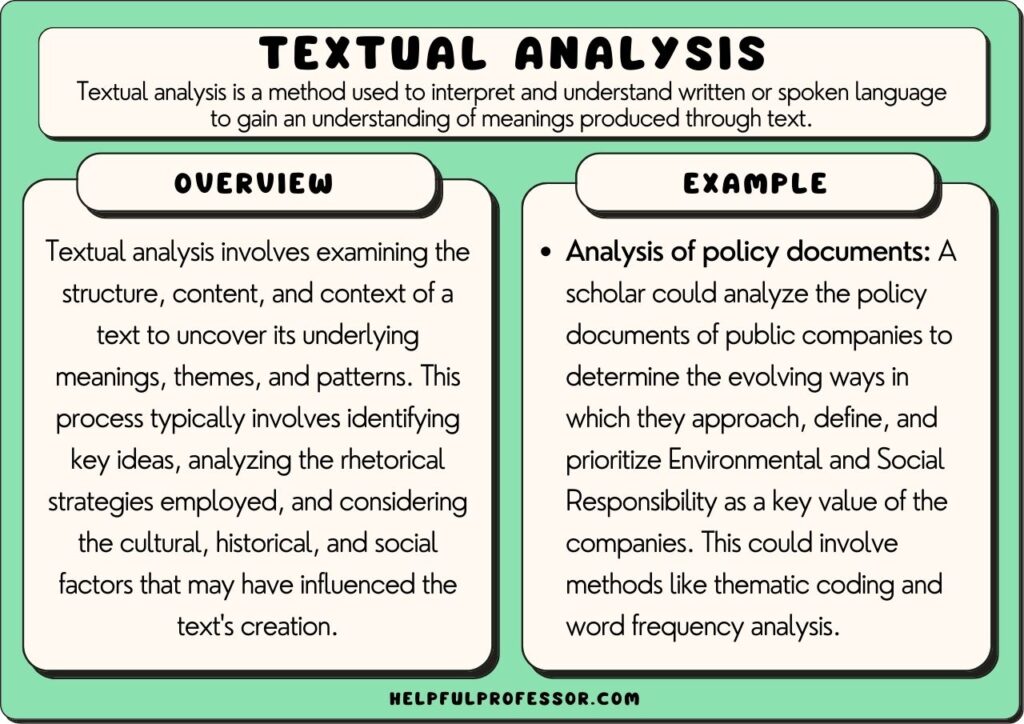
Textual analysis is a research methodology that involves exploring written text as empirical data. Scholars explore both the content and structure of texts, and attempt to discern key themes and statistics emergent from them.
This method of research is used in various academic disciplines, including cultural studies, literature, bilical studies, anthropology , sociology, and others (Dearing, 2022; McKee, 2003).
This method of analysis involves breaking down a text into its constituent parts for close reading and making inferences about its context, underlying themes, and the intentions of its author.
Textual Analysis Definition
Alan McKee is one of the preeminent scholars of textual analysis. He provides a clear and approachable definition in his book Textual Analysis: A Beginner’s Guide (2003) where he writes:
“When we perform textual analysis on a text we make an educated guess at some of the most likely interpretations that might be made of the text […] in order to try and obtain a sense of the ways in which, in particular cultures at particular times, people make sense of the world around them.”
A key insight worth extracting from this definition is that textual analysis can reveal what cultural groups value, how they create meaning, and how they interpret reality.
This is invaluable in situations where scholars are seeking to more deeply understand cultural groups and civilizations – both past and present (Metoyer et al., 2018).
As such, it may be beneficial for a range of different types of studies, such as:
- Studies of Historical Texts: A study of how certain concepts are framed, described, and approached in historical texts, such as the Bible.
- Studies of Industry Reports: A study of how industry reports frame and discuss concepts such as environmental and social responsibility.
- Studies of Literature: A study of how a particular text or group of texts within a genre define and frame concepts. For example, you could explore how great American literature mythologizes the concept of the ‘The American Dream’.
- Studies of Speeches: A study of how certain politicians position national identities in their appeals for votes.
- Studies of Newspapers: A study of the biases within newspapers toward or against certain groups of people.
- Etc. (For more, see: Dearing, 2022)
McKee uses the term ‘textual analysis’ to also refer to text types that are not just written, but multimodal. For a dive into the analysis of multimodal texts, I recommend my article on content analysis , where I explore the study of texts like television advertisements and movies in detail.
Features of a Textual Analysis
When conducting a textual analysis, you’ll need to consider a range of factors within the text that are worthy of close examination to infer meaning. Features worthy of considering include:
- Content: What is being said or conveyed in the text, including explicit and implicit meanings, themes, or ideas.
- Context: When and where the text was created, the culture and society it reflects, and the circumstances surrounding its creation and distribution.
- Audience: Who the text is intended for, how it’s received, and the effect it has on its audience.
- Authorship: Who created the text, their background and perspectives, and how these might influence the text.
- Form and structure: The layout, sequence, and organization of the text and how these elements contribute to its meanings (Metoyer et al., 2018).
Textual Analysis Coding Methods
The above features may be examined through quantitative or qualitative research designs , or a mixed-methods angle.
1. Quantitative Approaches
You could analyze several of the above features, namely, content, form, and structure, from a quantitative perspective using computational linguistics and natural language processing (NLP) analysis.
From this approach, you would use algorithms to extract useful information or insights about frequency of word and phrase usage, etc. This can include techniques like sentiment analysis, topic modeling, named entity recognition, and more.
2. Qualitative Approaches
In many ways, textual analysis lends itself best to qualitative analysis. When identifying words and phrases, you’re also going to want to look at the surrounding context and possibly cultural interpretations of what is going on (Mayring, 2015).
Generally, humans are far more perceptive at teasing out these contextual factors than machines (although, AI is giving us a run for our money).
One qualitative approach to textual analysis that I regularly use is inductive coding, a step-by-step methodology that can help you extract themes from texts. If you’re interested in using this step-by-step method, read my guide on inductive coding here .
See more Qualitative Research Approaches Here
Textual Analysis Examples
Title: “Discourses on Gender, Patriarchy and Resolution 1325: A Textual Analysis of UN Documents” Author: Nadine Puechguirbal Year: 2010 APA Citation: Puechguirbal, N. (2010). Discourses on Gender, Patriarchy and Resolution 1325: A Textual Analysis of UN Documents, International Peacekeeping, 17 (2): 172-187. doi: 10.1080/13533311003625068
Summary: The article discusses the language used in UN documents related to peace operations and analyzes how it perpetuates stereotypical portrayals of women as vulnerable individuals. The author argues that this language removes women’s agency and keeps them in a subordinate position as victims, instead of recognizing them as active participants and agents of change in post-conflict environments. Despite the adoption of UN Security Council Resolution 1325, which aims to address the role of women in peace and security, the author suggests that the UN’s male-dominated power structure remains unchallenged, and gender mainstreaming is often presented as a non-political activity.
Title: “Racism and the Media: A Textual Analysis” Author: Kassia E. Kulaszewicz Year: 2015 APA Citation: Kulaszewicz, K. E. (2015). Racism and the Media: A Textual Analysis . Dissertation. Retrieved from: https://sophia.stkate.edu/msw_papers/477
Summary: This study delves into the significant role media plays in fostering explicit racial bias. Using Bandura’s Learning Theory, it investigates how media content influences our beliefs through ‘observational learning’. Conducting a textual analysis, it finds differences in representation of black and white people, stereotyping of black people, and ostensibly micro-aggressions toward black people. The research highlights how media often criminalizes Black men, portraying them as violent, while justifying or supporting the actions of White officers, regardless of their potential criminality. The study concludes that news media likely continues to reinforce racism, whether consciously or unconsciously.
Title: “On the metaphorical nature of intellectual capital: a textual analysis” Author: Daniel Andriessen Year: 2006 APA Citation: Andriessen, D. (2006). On the metaphorical nature of intellectual capital: a textual analysis. Journal of Intellectual capital , 7 (1), 93-110.
Summary: This article delves into the metaphorical underpinnings of intellectual capital (IC) and knowledge management, examining how knowledge is conceptualized through metaphors. The researchers employed a textual analysis methodology, scrutinizing key texts in the field to identify prevalent metaphors. They found that over 95% of statements about knowledge are metaphor-based, with “knowledge as a resource” and “knowledge as capital” being the most dominant. This study demonstrates how textual analysis helps us to understand current understandings and ways of speaking about a topic.
Title: “Race in Rhetoric: A Textual Analysis of Barack Obama’s Campaign Discourse Regarding His Race” Author: Andrea Dawn Andrews Year: 2011 APA Citation: Andrew, A. D. (2011) Race in Rhetoric: A Textual Analysis of Barack Obama’s Campaign Discourse Regarding His Race. Undergraduate Honors Thesis Collection. 120 . https://digitalcommons.butler.edu/ugtheses/120
This undergraduate honors thesis is a textual analysis of Barack Obama’s speeches that explores how Obama frames the concept of race. The student’s capstone project found that Obama tended to frame racial inequality as something that could be overcome, and that this was a positive and uplifting project. Here, the student breaks-down times when Obama utilizes the concept of race in his speeches, and examines the surrounding content to see the connotations associated with race and race-relations embedded in the text. Here, we see a decidedly qualitative approach to textual analysis which can deliver contextualized and in-depth insights.
Sub-Types of Textual Analysis
While above I have focused on a generalized textual analysis approach, a range of sub-types and offshoots have emerged that focus on specific concepts, often within their own specific theoretical paradigms. Each are outlined below, and where I’ve got a guide, I’ve linked to it in blue:
- Content Analysis : Content analysis is similar to textual analysis, and I would consider it a type of textual analysis, where it’s got a broader understanding of the term ‘text’. In this type, a text is any type of ‘content’, and could be multimodal in nature, such as television advertisements, movies, posters, and so forth. Content analysis can be both qualitative and quantitative, depending on whether it focuses more on the meaning of the content or the frequency of certain words or concepts (Chung & Pennebaker, 2018).
- Discourse Analysis : Emergent specifically from critical and postmodern/ poststructural theories, discourse analysis focuses closely on the use of language within a social context, with the goal of revealing how repeated framing of terms and concepts has the effect of shaping how cultures understand social categories. It considers how texts interact with and shape social norms, power dynamics, ideologies, etc. For example, it might examine how gender is socially constructed as a distinct social category through Disney films. It may also be called ‘critical discourse analysis’.
- Narrative Analysis: This approach is used for analyzing stories and narratives within text. It looks at elements like plot, characters, themes, and the sequence of events to understand how narratives construct meaning.
- Frame Analysis: This approach looks at how events, ideas, and themes are presented or “framed” within a text. It explores how these frames can shape our understanding of the information being presented. While similar to discourse analysis, a frame analysis tends to be less associated with the loaded concept of ‘discourse’ that exists specifically within postmodern paradigms (Smith, 2017).
- Semiotic Analysis: This approach studies signs and symbols, both visual and textual, and could be a good compliment to a content analysis, as it provides the language and understandings necessary to describe how signs make meaning in cultural contexts that we might find with the fields of semantics and pragmatics . It’s based on the theory of semiotics, which is concerned with how meaning is created and communicated through signs and symbols.
- Computational Textual Analysis: In the context of data science or artificial intelligence, this type of analysis involves using algorithms to process large amounts of text. Techniques can include topic modeling, sentiment analysis, word frequency analysis, and others. While being extremely useful for a quantitative analysis of a large dataset of text, it falls short in its ability to provide deep contextualized understandings of words-in-context.
Each of these methods has its strengths and weaknesses, and the choice of method depends on the research question, the type of text being analyzed, and the broader context of the research.
See More Examples of Analysis Here
Strengths and Weaknesses of Textual Analysis
When writing your methodology for your textual analysis, make sure to define not only what textual analysis is, but (if applicable) the type of textual analysis, the features of the text you’re analyzing, and the ways you will code the data. It’s also worth actively reflecting on the potential weaknesses of a textual analysis approach, but also explaining why, despite those weaknesses, you believe this to be the most appropriate methodology for your study.
Chung, C. K., & Pennebaker, J. W. (2018). Textual analysis. In Measurement in social psychology (pp. 153-173). Routledge.
Dearing, V. A. (2022). Manual of textual analysis . Univ of California Press.
McKee, A. (2003). Textual analysis: A beginner’s guide. Textual analysis , 1-160.
Mayring, P. (2015). Qualitative content analysis: Theoretical background and procedures. Approaches to qualitative research in mathematics education: Examples of methodology and methods , 365-380. doi: https://doi.org/10.1007/978-94-017-9181-6_13
Metoyer, R., Zhi, Q., Janczuk, B., & Scheirer, W. (2018, March). Coupling story to visualization: Using textual analysis as a bridge between data and interpretation. In 23rd International Conference on Intelligent User Interfaces (pp. 503-507). doi: https://doi.org/10.1145/3172944.3173007
Smith, J. A. (2017). Textual analysis. The international encyclopedia of communication research methods , 1-7.
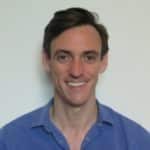
Chris Drew (PhD)
Dr. Chris Drew is the founder of the Helpful Professor. He holds a PhD in education and has published over 20 articles in scholarly journals. He is the former editor of the Journal of Learning Development in Higher Education. [Image Descriptor: Photo of Chris]
- Chris Drew (PhD) https://helpfulprofessor.com/author/chris-drew-phd/ 5 Top Tips for Succeeding at University
- Chris Drew (PhD) https://helpfulprofessor.com/author/chris-drew-phd/ 50 Durable Goods Examples
- Chris Drew (PhD) https://helpfulprofessor.com/author/chris-drew-phd/ 100 Consumer Goods Examples
- Chris Drew (PhD) https://helpfulprofessor.com/author/chris-drew-phd/ 30 Globalization Pros and Cons
Leave a Comment Cancel Reply
Your email address will not be published. Required fields are marked *
- Subject List
- Take a Tour
- For Authors
- Subscriber Services
- Publications
- African American Studies
- African Studies
- American Literature
- Anthropology
- Architecture Planning and Preservation
- Art History
- Atlantic History
- Biblical Studies
- British and Irish Literature
- Childhood Studies
- Chinese Studies
- Cinema and Media Studies
Communication
- Criminology
- Environmental Science
- Evolutionary Biology
- International Law
- International Relations
- Islamic Studies
- Jewish Studies
- Latin American Studies
- Latino Studies
- Linguistics
- Literary and Critical Theory
- Medieval Studies
- Military History
- Political Science
- Public Health
- Renaissance and Reformation
- Social Work
- Urban Studies
- Victorian Literature
- Browse All Subjects
How to Subscribe
- Free Trials
In This Article Expand or collapse the "in this article" section Textual Analysis and Communication
Introduction, theoretical background.
- General Introductions
- Analytical Strategies
- Methodological Antecedents
- Methodological Debate
- Types of Textual Analysis
- Qualitative Research in Media and Communication Studies
Related Articles Expand or collapse the "related articles" section about
About related articles close popup.
Lorem Ipsum Sit Dolor Amet
Vestibulum ante ipsum primis in faucibus orci luctus et ultrices posuere cubilia Curae; Aliquam ligula odio, euismod ut aliquam et, vestibulum nec risus. Nulla viverra, arcu et iaculis consequat, justo diam ornare tellus, semper ultrices tellus nunc eu tellus.
- Approaches to Multimodal Discourse Analysis
- Critical and Cultural Studies
- Feminist Theory
- Food Studies and Communication
- Gender and the Media
- Rhetoric and Communication
- Symbolic Interactionism in Communication
Other Subject Areas
Forthcoming articles expand or collapse the "forthcoming articles" section.
- Culture Shock and Communication
- LGBTQ+ Family Communication
- Queerbaiting
- Find more forthcoming titles...
- Export Citations
- Share This Facebook LinkedIn Twitter
Textual Analysis and Communication by Elfriede Fürsich LAST MODIFIED: 25 September 2018 DOI: 10.1093/obo/9780199756841-0216
Textual analysis is a qualitative method used to examine content in media and popular culture, such as newspaper articles, television shows, websites, games, videos, and advertising. The method is linked closely to cultural studies. Based on semiotic and interpretive approaches, textual analysis is a type of qualitative analysis that focuses on the underlying ideological and cultural assumptions of a text. In contrast to systematic quantitative content analysis, textual analysis reaches beyond manifest content to understand the prevailing ideologies of a particular historical and cultural moment that make a specific coverage possible. Critical-cultural scholars understand media content and other cultural artifacts as indicators of how realities are constructed and which ideas are accepted as normal. Following the French cultural philosopher Roland Barthes, content is understood as “text,” i.e., not as a fixed entity but as a complex set of discursive strategies that is generated in a special social, political, historic, and cultural context ( Barthes 2013 , cited under Theoretical Background ). Any text can be interpreted in multiple ways; the possibility of multiple meanings within a text is called “polysemy.” The goal of textual analysis is not to find one “true” interpretation—in contrast to traditional hermeneutic approaches to text exegesis—but to explain the variety of possible meanings inscribed in the text. Researchers who use textual analysis do not follow a single established approach but employ a variety of analysis types, such as ideological, genre, narrative, rhetorical, gender, or discourse analysis. Therefore, the term “textual analysis” could also be understood as a collective term for a variety of qualitative, interpretive, and critical content analysis techniques of popular culture artifacts. This method, just as cultural studies itself, draws on an eclectic mix of disciplines, such as anthropology, literary studies, rhetorical criticism, and cultural sociology, along with intellectual traditions, such as semiotics, (post)structuralism, and deconstruction. What distinguishes textual analysis from other forms of qualitative content analysis in the sociological tradition is its critical-cultural focus on power and ideology. Moreover, textual analysts normally do not use linguistic aspects as central evidence (such as in critical discourse analysis), nor do they use a pre-established code book, such as some traditional qualitative content methods. Textual analysis follows an inductive, interpretive approach by finding patterns in the material that lead to “readings” grounded in the back and forth between observation and contextual analysis. Of central interest is the deconstruction of representations (especially but not always of Others with regard to race, class, gender, sexuality, and ability) because these highlight the relationship of media and content to overall ideologies. The method is based in a constructionist framework. For textual analysts, media content does not simply reflect reality; instead, media, popular culture, and society are mutually constituted. Media and popular culture are arenas in which representations and ideas about reality are produced, maintained, and also challenged.
Central to textual analysis is the idea that content as “text” is a coming together of multiple meanings in a specific moment. Barthes 2013 and Barthes 1977 discuss this idea in detail and provide groundbreaking analysis of cultural phenomena in postwar France. Fiske 1987 , Fiske 2010 , and Fiske 2011 provide the standard on how popular culture can be “read,” i.e., interpreted for its ideological assumptions. Because the central aim of textual analysis is to understand how representations are produced in media content, Stuart Hall’s chapter 1 “The Work of Representation” in the renowned textbook Hall, et al. 2013 delivers a compact but comprehensive explanation of representation as a concept. To understand the shift to post-structuralism and concepts, such as discourse, hegemony, and the relationship between language and power, that are central to textual work, one can turn to the works of original theorists such as Foucault 1972 as well as Best and Kellner 1991 for contextualized clarification. Moreover, Deleuze and Guattari 2004 is a foundational post-structural text that radically rethinks the relationship between meaning and practice.
Barthes, Roland. 2013. Mythologies . New York: Hill and Wang.
English translation by Richard Howard and Annette Lavers of the original book published in 1957. Part 1: “Mythologies” consists of a series of short essayistic analyses of cultural phenomena, such as the Blue Guide travel books or advertising for detergents. Part 2: “Myth Today” lays out Barthes’s semiotic-structural approach. Although Barthes later acknowledged the historic contingencies of his interpretations, they remain important as they provided relevant perspective and methodological vocabulary for textual analysis for years to come.
Barthes, Roland. 1977. Image, music, text . Essays selected and translated by Stephen Heath. New York: Hill and Wang.
Classic collection of Barthes’s writing on semiotics and structuralism. For methodological considerations, the chapters “Introduction to the Structural Analysis of Narrative” and “The Death of the Author” are especially relevant.
Best, Steven, and Douglas Kellner. 1991. Postmodern theory: Critical interventions . New York: Guilford.
DOI: 10.1007/978-1-349-21718-2
Accessible introduction to leading postmodern and post-structuralist theorists. For textual analysis, especially the chapter 2 “Foucault and the Critique of Modernity,” chapter 4 “Baudrillard en route to Postmodernity,” and chapter 5 “Lyotard and Postmodern Gaming” provide relevant context for understanding post-structural and postmodern principles.
Deleuze, Gilles, and Félix Guattari. 2004. A thousand plateaus . Translated by Brian Massumi. London and New York: Continuum.
This book is the second part of Deleuze and Guattari’s groundbreaking philosophical project, “Capitalism and Schizophrenia.” Originally published in 1980, it explains central post-structural concepts such as rhizomes, multiplicity, and nomadic thought. Foundational for understanding the production of knowledge and meaning, these ideas have stood the test of time and resonate in networked and digitalized societies in the early 21st century.
Fiske, John. 1987. Television culture . New York: Routledge.
A classic book by Fiske. His “codes of television” (pp. 4–20) explain even for beginning researchers the important relationship among reality, representation, and ideology that is foundational for the textual analysis of any media content even beyond television.
Fiske, John. 2010. Understanding popular culture . 2d ed. London and New York: Routledge.
Important work by Fiske, originally published in 1989, that lays out the theoretical foundations for cultural analysis.
Fiske, John. 2011. Reading the popular . 2d ed. London and New York: Routledge.
Recently reissued companion book to Fiske 2010 . Provides a variety of examples for cultural analysis ranging from Madonna and shopping malls to news and quiz shows.
Foucault, Michel. 1972. The discourse on language. In The archeology of knowledge and the discourse of language . By Michel Foucault, 215–237. Translated by A. M. Sheridan Smith. New York: Pantheon.
Based on the author’s inaugural lecture at the Collège de France in 1970, this appendix provides a fairly succinct introduction to Foucault’s scholarly program and outlines his specific concepts of “discourse.” The author begins to connect discourses to structures of power and knowledge, an argument that becomes more central in his later writings.
Hall, Stuart, Jessica Evans, and Sean Nixon, eds. 2013. Representation: Cultural representations and signifying practices . 2d ed. London and Thousand Oaks, CA: SAGE.
One of the central goals of textual analysis is to understand and interpret media representations. Chapter 1 “The Work of Representation,” by Stuart Hall, is the most comprehensive introduction to this central post-structural cultural studies concept.
back to top
Users without a subscription are not able to see the full content on this page. Please subscribe or login .
Oxford Bibliographies Online is available by subscription and perpetual access to institutions. For more information or to contact an Oxford Sales Representative click here .
- About Communication »
- Meet the Editorial Board »
- Accounting Communication
- Acculturation Processes and Communication
- Action Assembly Theory
- Action-Implicative Discourse Analysis
- Activist Media
- Adherence and Communication
- Adolescence and the Media
- Advertisements, Televised Political
- Advertising
- Advertising, Children and
- Advertising, International
- Advocacy Journalism
- Agenda Setting
- Annenberg, Walter H.
- Apologies and Accounts
- Applied Communication Research Methods
- Argumentation
- Artificial Intelligence (AI) Advertising
- Attitude-Behavior Consistency
- Audience Fragmentation
- Audience Studies
- Authoritarian Societies, Journalism in
- Bakhtin, Mikhail
- Bandwagon Effect
- Baudrillard, Jean
- Blockchain and Communication
- Bourdieu, Pierre
- Brand Equity
- British and Irish Magazine, History of the
- Broadcasting, Public Service
- Capture, Media
- Castells, Manuel
- Celebrity and Public Persona
- Civil Rights Movement and the Media, The
- Co-Cultural Theory and Communication
- Codes and Cultural Discourse Analysis
- Cognitive Dissonance
- Collective Memory, Communication and
- Comedic News
- Communication Apprehension
- Communication Campaigns
- Communication, Definitions and Concepts of
- Communication History
- Communication Law
- Communication Management
- Communication Networks
- Communication, Philosophy of
- Community Attachment
- Community Journalism
- Community Structure Approach
- Computational Journalism
- Computer-Mediated Communication
- Content Analysis
- Corporate Social Responsibility and Communication
- Crisis Communication
- Critical Race Theory and Communication
- Cross-tools and Cross-media Effects
- Cultivation
- Cultural and Creative Industries
- Cultural Imperialism Theories
- Cultural Mapping
- Cultural Persuadables
- Cultural Pluralism and Communication
- Cyberpolitics
- Death, Dying, and Communication
- Debates, Televised
- Deliberation
- Developmental Communication
- Diffusion of Innovations
- Digital Divide
- Digital Gender Diversity
- Digital Intimacies
- Digital Literacy
- Diplomacy, Public
- Distributed Work, Comunication and
- Documentary and Communication
- E-democracy/E-participation
- E-Government
- Elaboration Likelihood Model
- Electronic Word-of-Mouth (eWOM)
- Embedded Coverage
- Entertainment
- Entertainment-Education
- Environmental Communication
- Ethnic Media
- Ethnography of Communication
- Experiments
- Families, Multicultural
- Family Communication
- Federal Communications Commission
- Feminist and Queer Game Studies
- Feminist Data Studies
- Feminist Journalism
- Focus Groups
- Freedom of the Press
- Friendships, Intercultural
- Gatekeeping
- Global Englishes
- Global Media, History of
- Global Media Organizations
- Glocalization
- Goffman, Erving
- Habermas, Jürgen
- Habituation and Communication
- Health Communication
- Hermeneutic Communication Studies
- Homelessness and Communication
- Hook-Up and Dating Apps
- Hostile Media Effect
- Identification with Media Characters
- Identity, Cultural
- Image Repair Theory
- Implicit Measurement
- Impression Management
- Infographics
- Information and Communication Technology for Development
- Information Management
- Information Overload
- Information Processing
- Infotainment
- Innis, Harold
- Instructional Communication
- Integrated Marketing Communications
- Interactivity
- Intercultural Capital
- Intercultural Communication
- Intercultural Communication, Tourism and
- Intercultural Communication, Worldview in
- Intercultural Competence
- Intercultural Conflict Mediation
- Intercultural Dialogue
- Intercultural New Media
- Intergenerational Communication
- Intergroup Communication
- International Communications
- Interpersonal Communication
- Interpersonal LGBTQ Communication
- Interpretation/Reception
- Interpretive Communities
- Journalism, Accuracy in
- Journalism, Alternative
- Journalism and Trauma
- Journalism, Citizen
- Journalism, Citizen, History of
- Journalism Ethics
- Journalism, Interpretive
- Journalism, Peace
- Journalism, Tabloid
- Journalists, Violence against
- Knowledge Gap
- Lazarsfeld, Paul
- Leadership and Communication
- Mass Communication
- McLuhan, Marshall
- Media Activism
- Media Aesthetics
- Media and Time
- Media Convergence
- Media Credibility
- Media Dependency
- Media Ecology
- Media Economics
- Media Economics, Theories of
- Media, Educational
- Media Effects
- Media Ethics
- Media Events
- Media Exposure Measurement
- Media, Gays and Lesbians in the
- Media Literacy
- Media Logic
- Media Management
- Media Policy and Governance
- Media Regulation
- Media, Social
- Media Sociology
- Media Systems Theory
- Merton, Robert K.
- Message Characteristics and Persuasion
- Mobile Communication Studies
- Multimodal Discourse Analysis, Approaches to
- Multinational Organizations, Communication and Culture in
- Murdoch, Rupert
- Narrative Engagement
- Narrative Persuasion
- Net Neutrality
- News Framing
- News Media Coverage of Women
- NGOs, Communication and
- Online Campaigning
- Open Access
- Organizational Change and Organizational Change Communicat...
- Organizational Communication
- Organizational Communication, Aging and
- Parasocial Theory in Communication
- Participation, Civic/Political
- Participatory Action Research
- Patient-Provider Communication
- Peacebuilding and Communication
- Perceived Realism
- Personalized Communication
- Persuasion and Social Influence
- Persuasion, Resisting
- Photojournalism
- Political Advertising
- Political Communication, Normative Analysis of
- Political Economy
- Political Knowledge
- Political Marketing
- Political Scandals
- Political Socialization
- Polls, Opinion
- Product Placement
- Public Interest Communication
- Public Opinion
- Public Relations
- Public Sphere
- Queer Intercultural Communication
- Queer Migration and Digital Media
- Race and Communication
- Racism and Communication
- Radio Studies
- Reality Television
- Reasoned Action Frameworks
- Religion and the Media
- Reporting, Investigative
- Rhetoric and Intercultural Communication
- Rhetoric and Social Movements
- Rhetoric, Religious
- Rhetoric, Visual
- Risk Communication
- Rumor and Communication
- Schramm, Wilbur
- Science Communication
- Scripps, E. W.
- Selective Exposure
- Sense-Making/Sensemaking
- Sesame Street
- Sex in the Media
- Small-Group Communication
- Social Capital
- Social Change
- Social Cognition
- Social Construction
- Social Identity Theory and Communication
- Social Interaction
- Social Movements
- Social Network Analysis
- Social Protest
- Sports Communication
- Stereotypes
- Strategic Communication
- Superdiversity
- Surveillance and Communication
- Synchrony in Intercultural Communication
- Tabloidization
- Telecommunications History/Policy
- Television, Cable
- Textual Analysis and Communication
- Third Culture Kids
- Third-Person Effect
- Time Warner
- Transgender Media Studies
- Transmedia Storytelling
- Two-Step Flow
- United Nations and Communication
- Urban Communication
- Uses and Gratifications
- Video Deficit
- Video Games and Communication
- Violence in the Media
- Virtual Reality and Communication
- Visual Communication
- Web Archiving
- Whistleblowing
- Whiteness Theory in Intercultural Communication
- Youth and Media
- Zines and Communication
- Privacy Policy
- Cookie Policy
- Legal Notice
- Accessibility
Powered by:
- [66.249.64.20|81.177.182.159]
- 81.177.182.159
- Privacy Policy
Buy Me a Coffee

Home » Textual Analysis – Types, Examples and Guide
Textual Analysis – Types, Examples and Guide
Table of Contents

Textual Analysis
Textual analysis is the process of examining a text in order to understand its meaning. It can be used to analyze any type of text, including literature , poetry, speeches, and scientific papers. Textual analysis involves analyzing the structure, content, and style of a text.
Textual analysis can be used to understand a text’s author, date, and audience. It can also reveal how a text was constructed and how it functions as a piece of communication.
Textual Analysis in Research
Textual analysis is a valuable tool in research because it allows researchers to examine and interpret text data in a systematic and rigorous way. Here are some ways that textual analysis can be used in research:
- To explore research questions: Textual analysis can be used to explore research questions in various fields, such as literature, media studies, and social sciences. It can provide insight into the meaning, interpretation, and communication patterns of text.
- To identify patterns and themes: Textual analysis can help identify patterns and themes within a set of text data, such as analyzing the representation of gender or race in media.
- To evaluate interventions: Textual analysis can be used to evaluate the effectiveness of interventions, such as analyzing the language and messaging of public health campaigns.
- To inform policy and practice: Textual analysis can provide insights that inform policy and practice, such as analyzing legal documents to inform policy decisions.
- To analyze historical data: Textual analysis can be used to analyze historical data, such as letters, diaries, and newspapers, to provide insights into historical events and social contexts.
Textual Analysis in Cultural and Media Studies
Textual analysis is a key tool in cultural and media studies as it enables researchers to analyze the meanings, representations, and discourses present in cultural and media texts. Here are some ways that textual analysis is used in cultural and media studies:
- To analyze representation: Textual analysis can be used to analyze the representation of different social groups, such as gender, race, and sexuality, in media and cultural texts. This analysis can provide insights into how these groups are constructed and represented in society.
- To analyze cultural meanings: Textual analysis can be used to analyze the cultural meanings and symbols present in media and cultural texts. This analysis can provide insights into how culture and society are constructed and understood.
- To analyze discourse: Textual analysis can be used to analyze the discourse present in cultural and media texts. This analysis can provide insights into how language is used to construct meaning and power relations.
- To analyze media content: Textual analysis can be used to analyze media content, such as news articles, TV shows, and films, to understand how they shape our understanding of the world around us.
- To analyze advertising : Textual analysis can be used to analyze advertising campaigns to understand how they construct meanings, identities, and desires.
Textual Analysis in the Social Sciences
Textual analysis is a valuable tool in the social sciences as it enables researchers to analyze and interpret text data in a systematic and rigorous way. Here are some ways that textual analysis is used in the social sciences:
- To analyze interview data: Textual analysis can be used to analyze interview data, such as transcribed interviews, to identify patterns and themes in the data.
- To analyze survey responses: Textual analysis can be used to analyze survey responses to identify patterns and themes in the data.
- To analyze social media data: Textual analysis can be used to analyze social media data, such as tweets and Facebook posts, to identify patterns and themes in the data.
- To analyze policy documents: Textual analysis can be used to analyze policy documents, such as government reports and legislation, to identify discourses and power relations present in the policy.
- To analyze historical data: Textual analysis can be used to analyze historical data, such as letters and diaries, to provide insights into historical events and social contexts.
Textual Analysis in Literary Studies
Textual analysis is a key tool in literary studies as it enables researchers to analyze and interpret literary texts in a systematic and rigorous way. Here are some ways that textual analysis is used in literary studies:
- To analyze narrative structure: Textual analysis can be used to analyze the narrative structure of a literary text, such as identifying the plot, character development, and point of view.
- To analyze language and style: Textual analysis can be used to analyze the language and style used in a literary text, such as identifying figurative language, symbolism, and rhetorical devices.
- To analyze themes and motifs: Textual analysis can be used to analyze the themes and motifs present in a literary text, such as identifying recurring symbols, themes, and motifs.
- To analyze historical and cultural context: Textual analysis can be used to analyze the historical and cultural context of a literary text, such as identifying how the text reflects the social and political context of its time.
- To analyze intertextuality: Textual analysis can be used to analyze the intertextuality of a literary text, such as identifying how the text references or is influenced by other literary works.
Textual Analysis Methods
Textual analysis methods are techniques used to analyze and interpret various types of text, including written documents, audio and video recordings, and online content. These methods are commonly used in fields such as linguistics, communication studies, sociology, psychology, and literature.
Some common textual analysis methods include:
Content Analysis
This involves identifying patterns and themes within a set of text data. This method is often used to analyze media content or other types of written materials, such as policy documents or legal briefs.
Discourse Analysis
This involves examining how language is used to construct meaning in social contexts. This method is often used to analyze political speeches or other types of public discourse.
Critical Discourse Analysis
This involves examining how power and social relations are constructed through language use, particularly in political and social contexts.
Narrative Analysis
This involves examining the structure and content of stories or narratives within a set of text data. This method is often used to analyze literary texts or oral histories.
This involves analyzing the meaning of signs and symbols within a set of text data. This method is often used to analyze advertising or other types of visual media.
Text mining
This involves using computational techniques to extract patterns and insights from large sets of text data. This method is often used in fields such as marketing and social media analysis.
Close Reading
This involves a detailed and in-depth analysis of a particular text, focusing on the language, style, and literary techniques used by the author.
How to Conduct Textual Analysis
Here are some general steps to conduct textual analysis:
- Choose your research question: Define your research question and identify the text or set of texts that you want to analyze.
- F amiliarize yourself with the text: Read and re-read the text, paying close attention to its language, structure, and content. Take notes on key themes, patterns, and ideas that emerge.
- Choose your analytical approach: Select the appropriate analytical approach for your research question, such as close reading, thematic analysis, content analysis, or discourse analysis.
- Create a coding scheme: If you are conducting content analysis, create a coding scheme to categorize and analyze the content of the text. This may involve identifying specific words, themes, or ideas to code.
- Code the text: Apply your coding scheme to the text and systematically categorize the content based on the identified themes or patterns.
- Analyze the data: Once you have coded the text, analyze the data to identify key patterns, themes, or trends. Use appropriate software or tools to help with this process if needed.
- Draw conclusions: Draw conclusions based on your analysis and answer your research question. Present your findings and provide evidence to support your conclusions.
- R eflect on limitations and implications: Reflect on the limitations of your analysis, such as any biases or limitations of the selected method. Also, discuss the implications of your findings and their relevance to the broader research field.
When to use Textual Analysis
Textual analysis can be used in various research fields and contexts. Here are some situations when textual analysis can be useful:
- Understanding meaning and interpretation: Textual analysis can help understand the meaning and interpretation of text, such as literature, media, and social media.
- Analyzing communication patterns: Textual analysis can be used to analyze communication patterns in different contexts, such as political speeches, social media conversations, and legal documents.
- Exploring cultural and social contexts: Textual analysis can be used to explore cultural and social contexts, such as the representation of gender, race, and identity in media.
- Examining historical documents: Textual analysis can be used to examine historical documents, such as letters, diaries, and newspapers.
- Evaluating marketing and advertising campaigns: Textual analysis can be used to evaluate marketing and advertising campaigns, such as analyzing the language, symbols, and imagery used.
Examples of Textual Analysis
Here are a few examples:
- Media Analysis: Textual analysis is frequently used in media studies to examine how news outlets and social media platforms frame and present news stories. Researchers can use textual analysis to examine the language and images used in news articles, tweets, and other forms of media to identify patterns and biases.
- Customer Feedback Analysis: Textual analysis is often used by businesses to analyze customer feedback, such as online reviews or social media posts, to identify common themes and areas for improvement. This allows companies to make data-driven decisions and improve their products or services.
- Political Discourse Analysis: Textual analysis is commonly used in political science to analyze political speeches, debates, and other forms of political communication. Researchers can use this method to identify the language and rhetoric used by politicians, as well as the strategies they employ to appeal to different audiences.
- Literary Analysis: Textual analysis is a fundamental tool in literary criticism, allowing scholars to examine the language, structure, and themes of literary works. This can involve close reading of individual texts or analysis of larger literary movements.
- Sentiment Analysis: Textual analysis is used to analyze social media posts, customer feedback, or other sources of text data to determine the sentiment of the text. This can be useful for businesses or organizations to understand how their brand or product is perceived in the market.
Purpose of Textual Analysis
There are several specific purposes for using textual analysis, including:
- To identify and interpret patterns in language use: Textual analysis can help researchers identify patterns in language use, such as common themes, recurring phrases, and rhetorical devices. This can provide insights into the values and beliefs that underpin the text.
- To explore the cultural context of the text: Textual analysis can help researchers understand the cultural context in which the text was produced, including the historical, social, and political factors that shaped the language and messages.
- To examine the intended and unintended meanings of the text: Textual analysis can help researchers uncover both the intended and unintended meanings of the text, and to explore how the language is used to convey certain messages or values.
- To understand how texts create and reinforce social and cultural identities: Textual analysis can help researchers understand how texts contribute to the creation and reinforcement of social and cultural identities, such as gender, race, ethnicity, and nationality.
Applications of Textual Analysis
Here are some common applications of textual analysis:
Media Studies
Textual analysis is frequently used in media studies to analyze news articles, advertisements, and social media posts to identify patterns and biases in media representation.
Literary Criticism
Textual analysis is a fundamental tool in literary criticism, allowing scholars to examine the language, structure, and themes of literary works.
Political Science
Textual analysis is commonly used in political science to analyze political speeches, debates, and other forms of political communication.
Marketing and Consumer Research
Textual analysis is used to analyze customer feedback, such as online reviews or social media posts, to identify common themes and areas for improvement.
Healthcare Research
Textual analysis is used to analyze patient feedback and medical records to identify patterns in patient experiences and improve healthcare services.
Social Sciences
Textual analysis is used in various fields within social sciences, such as sociology, anthropology, and psychology, to analyze various forms of data, including interviews, field notes, and documents.
Linguistics
Textual analysis is used in linguistics to study language use and its relationship to social and cultural contexts.
Advantages of Textual Analysis
There are several advantages of textual analysis in research. Here are some of the key advantages:
- Systematic and objective: Textual analysis is a systematic and objective method of analyzing text data. It enables researchers to analyze text data in a consistent and rigorous way, minimizing the risk of bias or subjectivity.
- Versatile : Textual analysis can be used to analyze a wide range of text data, including interview transcripts, survey responses, social media data, policy documents, and literary texts.
- Efficient : Textual analysis can be a more efficient method of data analysis compared to manual coding or other methods of qualitative analysis. With the help of software tools, researchers can process large volumes of text data more quickly and accurately.
- Allows for in-depth analysis: Textual analysis enables researchers to conduct in-depth analysis of text data, uncovering patterns and themes that may not be visible through other methods of data analysis.
- Can provide rich insights: Textual analysis can provide rich and detailed insights into complex social phenomena. It can uncover subtle nuances in language use, reveal underlying meanings and discourses, and shed light on the ways in which social structures and power relations are constructed and maintained.
Limitations of Textual Analysis
While textual analysis can provide valuable insights into the ways in which language is used to convey meaning and create social and cultural identities, it also has several limitations. Some of these limitations include:
- Limited Scope : Textual analysis is only able to analyze the content of written or spoken language, and does not provide insights into non-verbal communication such as facial expressions or body language.
- Subjectivity: Textual analysis is subject to the biases and interpretations of the researcher, as well as the context in which the language was produced. Different researchers may interpret the same text in different ways, leading to inconsistencies in the findings.
- Time-consuming: Textual analysis can be a time-consuming process, particularly if the researcher is analyzing a large amount of text. This can be a limitation in situations where quick analysis is necessary.
- Lack of Generalizability: Textual analysis is often used in qualitative research, which means that its findings cannot be generalized to larger populations. This limits the ability to draw conclusions that are applicable to a wider range of contexts.
- Limited Accessibility: Textual analysis requires specialized skills and training, which may limit its accessibility to researchers who are not trained in this method.
About the author
Muhammad Hassan
Researcher, Academic Writer, Web developer
You may also like

Cluster Analysis – Types, Methods and Examples

Questionnaire – Definition, Types, and Examples

Discriminant Analysis – Methods, Types and...

Case Study – Methods, Examples and Guide

MANOVA (Multivariate Analysis of Variance) –...

Observational Research – Methods and Guide
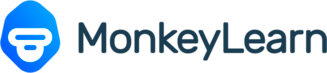
The Practical Guide to Textual Analysis
- Getting Started
- How Does It Work?
- Use Cases & Applications
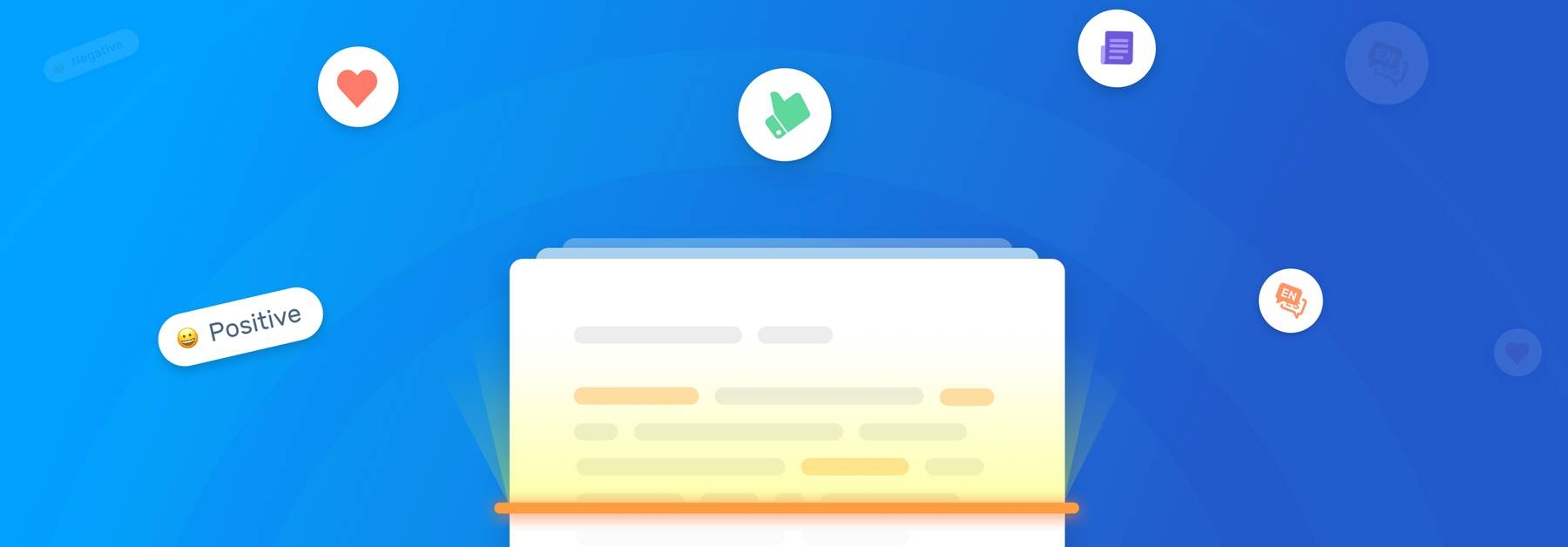
Textual analysis is the process of gathering and examining qualitative data to understand what it’s about.
But making sense of qualitative information is a major challenge. Whether analyzing data in business or performing academic research, manually reading, analyzing, and tagging text is no longer effective – it’s time-consuming, results are often inaccurate, and the process far from scalable.
Fortunately, developments in the sub-fields of Artificial Intelligence (AI) like machine learning and natural language processing (NLP) are creating unprecedented opportunities to process and analyze large collections of text data.
Thanks to algorithms trained with machine learning it is possible to perform a myriad of tasks that involve analyzing text, like topic classification (automatically tagging texts by topic), feature extraction (identifying specific characteristics in a text) and sentiment analysis (recognizing the emotions that underlie a given text).
Below, we’ll dive into textual analysis with machine learning, what it is and how it works, and reveal its most important applications in business and academic research:
Getting started with textual analysis
- What is textual analysis?
- Difference between textual analysis and content analysis?
- What is computer-assisted textual analysis?
- Methods and techniques
- Why is it important?
How does textual analysis work?
- Text classification
- Text extraction
Use cases and applications
- Customer service
- Customer feedback
- Academic research
Let’s start with the basics!
Getting Started With Textual Analysis
What is textual analysis.
While similar to text analysis , textual analysis is mainly used in academic research to analyze content related to media and communication studies, popular culture, sociology, and philosophy.
In this case, the purpose of textual analysis is to understand the cultural and ideological aspects that underlie a text and how they are connected with the particular context in which the text has been produced. In short, textual analysis consists of describing the characteristics of a text and making interpretations to answer specific questions.
One of the challenges of textual analysis resides in how to turn complex, large-scale data into manageable information. Computer-assisted textual analysis can be instrumental at this point, as it allows you to perform certain tasks automatically (without having to read all the data) and makes it simple to observe patterns and get unexpected insights. For example, you could perform automated textual analysis on a large set of data and easily tag all the information according to a series of previously defined categories. You could also use it to extract specific pieces of data, like names, countries, emails, or any other features.
Companies are using computer-assisted textual analysis to make sense of unstructured business data , and find relevant insights that lead to data-driven decisions. It’s being used to automate everyday tasks like ticket tagging and routing, improving productivity, and saving valuable time.
Difference Between Textual Analysis and Content Analysis?
When we talk about textual analysis we refer to a data-gathering process for analyzing text data. This qualitative methodology examines the structure, content, and meaning of a text, and how it relates to the historical and cultural context in which it was produced. To do so, textual analysis combines knowledge from different disciplines, like linguistics and semiotics.
Content analysis can be considered a subcategory of textual analysis, which intends to systematically analyze text, by coding the elements of the text to get quantitative insights. By coding text (that is, establishing different categories for the analysis), content analysis makes it possible to examine large sets of data and make replicable and valid inferences.
Sitting at the intersection between qualitative and quantitative approaches, content analysis has proved to be very useful to study a wide array of text data ― from newspaper articles to social media messages ― within many different fields, that range from academic research to organizational or business studies.
What is Computer-Assisted Textual Analysis?
Computer-assisted textual analysis involves using a software, digital platform, or computational tools to perform tasks related to text analysis automatically.
The developments in machine learning make it possible to create algorithms that can be trained with examples and learn a series of tasks, from identifying topics on a given text to extracting relevant information from an extensive collection of data. Natural Language Processing (NLP), another sub-field of AI, helps machines process unstructured data and transform it into manageable information that’s ready to analyze.
Automated textual analysis enables you to analyze large amounts of data that would require a significant amount of time and resources if done manually. Not only is automated textual analysis fast and straightforward, but it’s also scalable and provides consistent results.
Let’s look at an example. During the US elections 2016, we used MonkeyLearn to analyze millions of tweets referring to Donald Trump and Hillary Clinton . A text classification model allowed us to tag each Tweet into the two predefined categories: Trump and Hillary. The results showed that, on an average day, Donald Trump was getting around 450,000 Twitter mentions while Hillary Clinton was only getting about 250,000. And that was just the tip of the iceberg! What was really interesting was the nuances of those mentions: were they favorable or unfavorable? By performing sentiment analysis , we were able to discover the feelings behind those messages and gain some interesting insights about the polarity of those opinions.
For example, this is how Trump’s Tweets looked like when counted by sentiment:
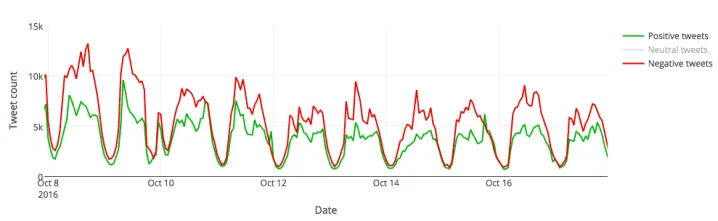
And this graphic shows the same for Hillary Clinton:
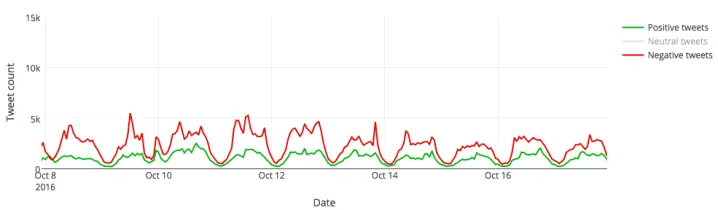
There are many methods and techniques for automated textual analysis. In the following section, we’ll take a closer look at each of them so that you have a better idea of what you can do with computer-assisted textual analysis.
Textual Analysis Methods & Techniques
- Word frequency
Collocation
Concordance, basic methods, word frequency.
Word frequency helps you find the most recurrent terms or expressions within a set of data. Counting the times a word is mentioned in a group of texts can lead you to interesting insights, for example, when analyzing customer feedback responses. If the terms ‘hard to use’ or ‘complex’ often appear in comments about your product, it may indicate you need to make UI/UX adjustments.
By ‘collocation’ we mean a sequence of words that frequently occur together. Collocations are usually bigrams (a pair of words) and trigrams (a combination of three words). ‘Average salary’ , ‘global market’ , ‘close a deal’ , ‘make an appointment’ , ‘attend a meeting’ are examples of collocations related to business.
In textual analysis, identifying collocations is useful to understand the semantic structure of a text. Counting bigrams and trigrams as one word improves the accuracy of the analysis.
Human language is ambiguous: depending on the context, the same word can mean different things. Concordance is used to identify instances in which a word or a series of words appear, to understand its exact meaning. For example, here are a few sentences from product reviews containing the word ‘time’:
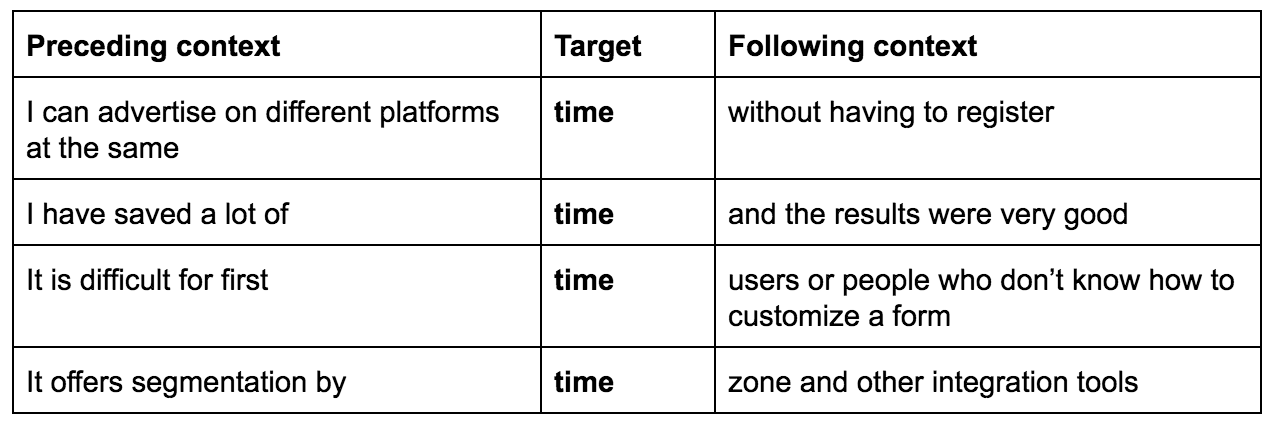
Advanced Methods
Text classification.
Text classification is the process of assigning tags or categories to unstructured data based on its content.
When we talk about unstructured data we refer to all sorts of text-based information that is unorganized, and therefore complex to sort and manage. For businesses, unstructured data may include emails, social media posts, chats, online reviews, support tickets, among many others. Text classification ― one of the essential tasks of Natural Language Processing (NLP) ― makes it possible to analyze text in a simple and cost-efficient way, organizing the data according to topic, urgency, sentiment or intent. We’ll take a closer look at each of these applications below:
Topic Analysis consists of assigning predefined tags to an extensive collection of text data, based on its topics or themes. Let’s say you want to analyze a series of product reviews to understand what aspects of your product are being discussed, and a review reads ‘the customer service is very responsive, they are always ready to help’ . This piece of feedback will be tagged under the topic ‘Customer Service’ .
Sentiment Analysis , also known as ‘opinion mining’, is the automated process of understanding the attributes of an opinion, that is, the emotions that underlie a text (e.g. positive, negative, and neutral). Sentiment analysis provides exciting opportunities in all kinds of fields. In business, you can use it to analyze customer feedback, social media posts, emails, support tickets, and chats. For instance, you could analyze support tickets to identify angry customers and solve their issues as a priority. You may also combine topic analysis with sentiment analysis (it is called aspect-based sentiment analysis ) to identify the topics being discussed about your product, and also, how people are reacting towards those topics. For example, take the product review we mentioned earlier for topic analysis: ‘the customer service is very responsive, they are always ready to help’ . This statement would be classified as both Positive and Customer Service .
Language detection : this allows you to classify a text based on its language. It’s particularly useful for routing purposes. For example, if you get a support ticket in Spanish, it could be automatically routed to a Spanish-speaking customer support team.
Intent detection : text classifiers can also be used to recognize the intent of a given text. What is the purpose behind a specific message? This can be helpful if you need to analyze customer support conversations or the results of a sales email campaign. For example, you could analyze email responses and classify your prospects based on their level of interest in your product.
Text Extraction
Text extraction is a textual analysis technique which consists of extracting specific terms or expressions from a collection of text data. Unlike text classification, the result is not a predefined tag but a piece of information that is already present in the text. For example, if you have a large collection of emails to analyze, you could easily pull out specific information such as email addresses, company names or any keyword that you need to retrieve. In some cases, you can combine text classification and text extraction in the same analysis.
The most useful text extraction tasks include:
Named-entity recognition : used to extract the names of companies , people , or organizations from a set of data.
Keyword extraction : allows you to extract the most relevant terms within a text. You can use keyword extraction to index data to be searched, create tags clouds, summarize the content of a text, among many other things.
Feature extraction : used to identify specific characteristics within a text. For example, if you are analyzing a series of product descriptions, you could create customized extractors to retrieve information like brand, model, color, etc .
Why is Textual Analysis Important?
Every day, we create a colossal amount of digital data. In fact, in the last two years alone we generated 90% percent of all the data in the world . That includes social media messages, emails, Google searches, and every other source of online data.
At the same time, books, media libraries, reports, and other types of databases are now available in digital format, providing researchers of all disciplines opportunities that didn’t exist before.
But the problem is that most of this data is unstructured. Since it doesn’t follow any organizational criteria, unstructured text is hard to search, manage, and examine. In this scenario, automated textual analysis tools are essential, as they help make sense of text data and find meaningful insights in a sea of information.
Text analysis enables businesses to go through massive collections of data with minimum human effort, saving precious time and resources, and allowing people to focus on areas where they can add more value. Here are some of the advantages of automated textual analysis:
Scalability
You can analyze as much data as you need in just seconds. Not only will you save valuable time, but you’ll also make your teams much more productive.
Real-time analysis
For businesses, it is key to detect angry customers on time or be warned of a potential PR crisis. By creating customized machine learning models for text analysis, you can easily monitor chats, reviews, social media channels, support tickets and all sorts of crucial data sources in real time, so you’re ready to take action when needed.
Academic researchers, especially in the political science field , may find real-time analysis with machine learning particularly useful to analyze polls, Twitter data, and election results.
Consistent criteria
Routine manual tasks (like tagging incoming tickets or processing customer feedback, for example) often end up being tedious and time-consuming. There are more chances of making mistakes and the criteria applied within team members often turns out to be inconsistent and subjective. Machine learning algorithms, on the other hand, learn from previous examples and always use the same criteria to analyze data .
How does Textual Analysis Work?
Computer-assisted textual analysis makes it easy to analyze large collections of text data and find meaningful information. Thanks to machine learning, it is possible to create models that learn from examples and can be trained to classify or extract relevant data.
But how easy is to get started with textual analysis?
As with most things related to artificial intelligence (AI), automated text analysis is perceived as a complex tool, only accessible to those with programming skills. Fortunately, that’s no longer the case. AI platforms like MonkeyLearn are actually very simple to use and don’t require any previous machine learning expertise. First-time users can try different pre-trained text analysis models right away, and use them for specific purposes even if they don’t have coding skills or have never studied machine learning.
However, if you want to take full advantage of textual analysis and create your own customized models, you should understand how it works.
There are two steps you need to follow before running an automated analysis: data gathering and data preparation. Here, we’ll explain them more in detail:
Data gathering : when we think of a topic we want to analyze, we should first make sure that we can obtain the data we need. Let’s say you want to analyze all the customer support tickets your company has received over a designated period of time. You should be able to export that information from your software and create a CSV or an Excel file. The data can be either internal (that is, data that’s only available to your business, like emails, support tickets, chats, spreadsheets, surveys, databases, etc) or external (like review sites, social media, news outlets or other websites).
Data preparation : before performing automated text analysis it’s necessary to prepare the data that you are going to use. This is done by applying a series of Natural Language Processing (NLP) techniques. Tokenization , parsing , lemmatization , stemming and stopword removal are just a few of them.
Once these steps are complete, you will be all set up for the data analysis itself. In this section, we’ll refer to how the most common textual analysis methods work: text classification and text extraction.
Text classification is the process of assigning tags to a collection of data based on its content.
When done manually, text categorization is a time-consuming task that often leads to mistakes and inaccuracies. By doing this automatically, it is possible to obtain very good results while spending less time and resources. Automatic text classification consists of three main approaches: rule-based, machine learning and hybrid.
Rule-based systems
Rule-based systems follow an ‘if-then’ (condition-action) structure based on linguistic rules. Basically, rules are human-made associations between a linguistic pattern on a text and a predefined tag. These linguistic patterns often refer to morphological, syntactic, lexical, semantic, or phonological aspects.
For instance, this could be a rule to classify a series of laptop descriptions:
( Lenovo | Sony | Hewlett Packard | Apple ) → Brand
In this case, when the text classification model detects any of those words within a text (the ‘if’ portion), it will assign the predefined tag ‘brand’ to them (the ‘then’ portion).
One of the main advantages of rule-based systems is that they are easy to understand by humans. On the downside, creating complex systems is quite tricky, because you need to have good knowledge of linguistics and of the topics present in the text that you want to analyze. Besides, adding new rules can be tough as it requires several tests, making rule-based systems hard to scale.
Machine learning-based systems
Machine learning-based systems are trained to make predictions based on examples. This means that a person needs to provide representative and consistent samples and assign the expected tags manually so that the system learns to make its own predictions from those past observations. The collection of manually tagged data is called training data .
But how does machine learning actually work?
Suppose you are training a machine learning-based classifier. The system needs to transform the training data into something it can understand: in this case, vectors (an array of numbers with encoded data). Vectors contain a set of relevant features from the given text, and use them to learn and make predictions on future data.
One of the most common methods for text vectorization is called bag of words and consists of counting how many times a particular word (from a predetermined list of words) appears in the text you want to analyze.
So, the text is transformed into vectors and fed into a machine learning algorithm along with its expected tags, creating a text classification model:
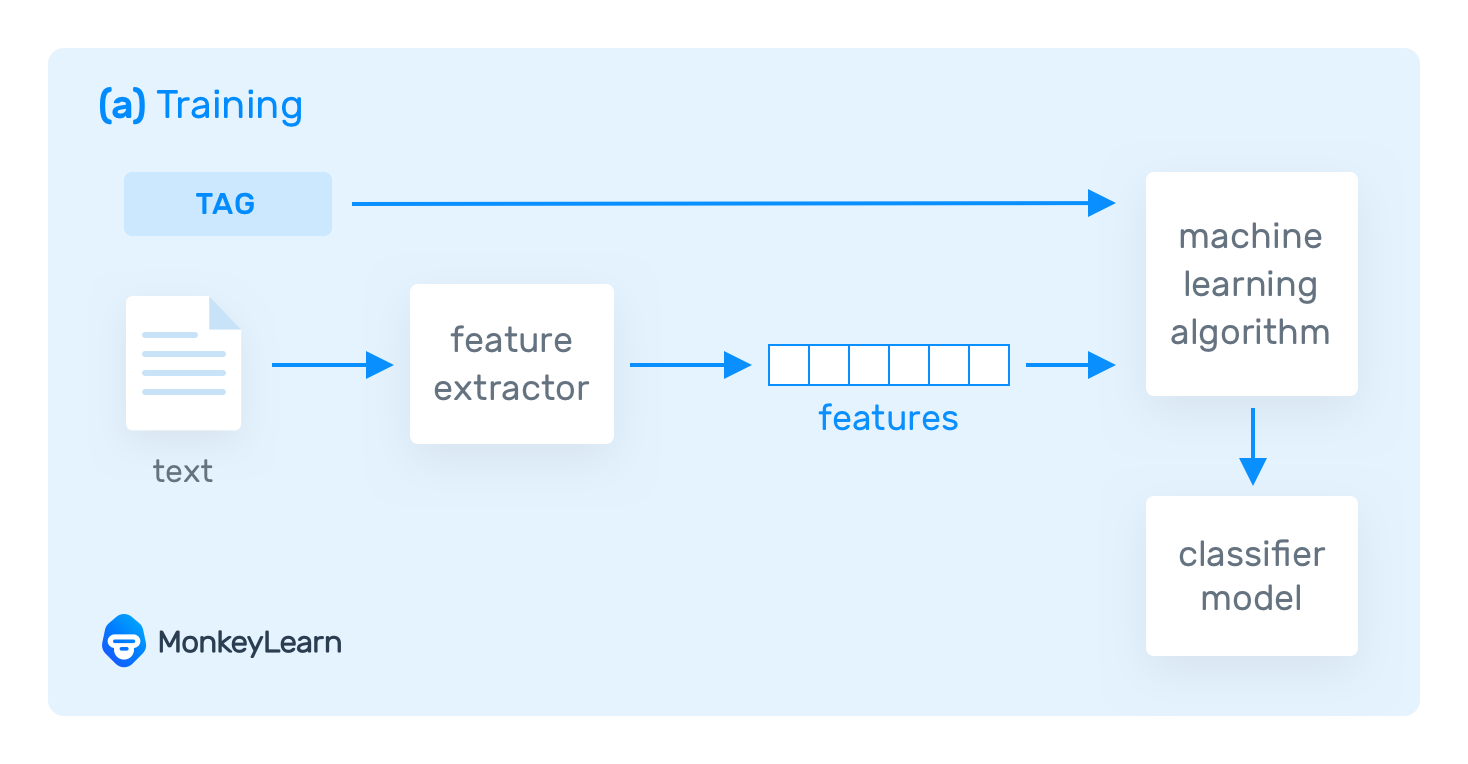
After being trained, the model can make predictions over unseen data:
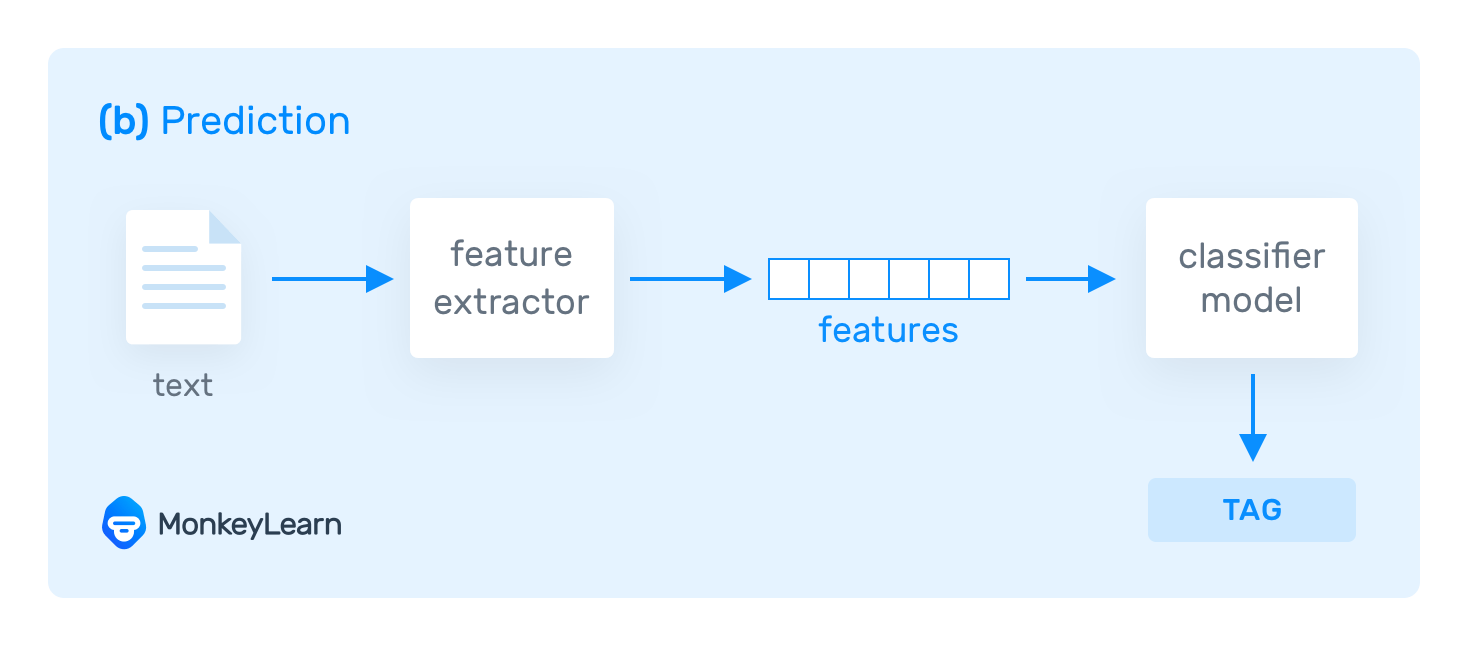
Machine learning algorithms
The most common algorithms used in text classification are Naive Bayes family of algorithms (NB), Support Vector Machines (SVM), and deep learning algorithms .
Naive Bayes family of algorithms (NB) is a probabilistic algorithm that uses Bayes’ theorem to calculate the probability of each tag for a given text. It then provides the tag with the highest likelihood of occurrence. This algorithm provides good results as long as the training data is scarce.
Support Vector Machines (SVM) is a machine learning algorithm that divides vectors into two different groups within a three-dimensional space. In one group, you have vectors that belong to a given tag, and in the other group vectors that don’t belong to that tag. Using this algorithm requires more coding skills, but the results are better than the ones with Naive Bayes.
Deep learning algorithms try to emulate the way the human brain thinks. They use millions of training examples and generate very rich representations of texts, leading to much more accurate predictions than other machine learning algorithms. The downside is that they need vast amounts of training data to provide accurate results and require intensive coding.
Hybrid systems
These systems combine rule-based systems and machine learning-based systems to obtain more accurate predictions.
There are different parameters to evaluate the performance of a text classifier: accuracy , precision , recall , and F1 score .
You can measure how your text classifier works by comparing it to a fixed testing set (that is, a group of data that already includes its expected tags) or by using cross-validation, a process that divides your training data into two groups – one used to train the model, and the other used to test the results.
Let’s go into more detail about each of these parameters:
Accuracy : this is the number of correct predictions that the text classifier makes divided by the total number of predictions. However, accuracy alone is not the best parameter to analyze the performance of a text classifier. When the number of examples is imbalanced (for example, a lot of the data belongs to one of the categories) you may experience an accuracy paradox , that is, a model with high accuracy, but one that’s not necessarily able to make accurate predictions for all tags. In this case, it’s better to look at precision and recall, and F1 score.
Precision : this metric indicates the number of correct predictions for a given tag, divided by the total number of correct and incorrect predictions for that tag. In this case, a high precision level indicates there were less false positives. For some tasks ― like sending automated email responses ― you will need text classification models with a high level of precision, that will only deliver an answer when it’s highly likely that the recipient belongs to a given tag.
Recall : it shows the number of correct predictions for a given tag, over the number of predictions that should have been predicted as belonging to that tag. High recall metrics indicate there were less false negatives and, if routing support tickets for example, it means that tickets will be sent to the right teams.
F1 score : this metric considers both precision and recall results, and provides an idea of how well your text classifier is working. It allows you to see how accurate is your model for all the tags you’re using.
Cross-validation
Cross-validation is a method used to measure the accuracy of a text classifier model. It consists of splitting the training dataset into a number of equal-length subsets, in a random way. For instance, let’s imagine you have four subsets and each of them contains 25% of your training data.
All of those subsets except one are used to train the text classifier. Then, the classifier is used to make predictions over the remaining subset. After this, you need to compile all the metrics we mentioned before (accuracy, precision, recall, and F1 score), and start the process all over again, until all the subsets have been used for testing. Finally, all the results are compiled to obtain the average performance of each metric.
Text extraction is the process of identifying specific pieces of text from unstructured data. This is very useful for a variety of purposes, from extracting company names from a Linkedin dataset to pulling out prices on product descriptions.
Text extraction allows to automatically visualize where the relevant terms or expressions are, without needing to read or scan all the text by yourself. And that is particularly relevant when you have massive databases, which would otherwise take ages to analyze manually.
There are different approaches to text extraction. Here, we’ll refer to the most commonly used and reliable:
Regular expressions
Regular expressions are similar to rules for text classification models. They can be defined as a series of characters that define a pattern.
Every time the text extractor detects a coincidence with a pattern, it assigns the corresponding tag.
This approach allows you to create text extractors quickly and with good results, as long as you find the right patterns for the data you want to analyze. However, as it gets more complex, it can be hard to manage and scale.
Conditional Random Fields
Conditional Random Fields (CRF) is a statistical approach used for text extraction with machine learning. It identifies different patterns by assigning a weight to each of the word sequences within a text. CRF’s also allow you to create additional parameters related to the patterns, based on syntactic or semantic information.
This approach creates more complex and richer patterns than regular expressions and can encode a large volume of information. However, if you want to train the text extractor properly, you will need to have in-depth NLP and computing knowledge.
You can use the same performance metrics that we mentioned for text classification (accuracy, precision, recall, and F1 score), although these metrics only consider exact matches as positive results, leaving partial matches aside.
If you want partial matches to be included in the results, you should use a performance metric called ROUGE (Recall-Oriented Understudy for Gisting Evaluation). This group of metrics measures lengths and numbers of sequences to make a match between the source text and the extraction performed by the model.
The parameters used to compare these two texts need to be defined manually. You may define ROUGE-n metrics (n is the length of the units you want to measure) or ROUGE-L metrics (to compare the longest common sentence).
Use Cases and Applications
Automated textual analysis is the process of obtaining meaningful information out of raw data. Considering unstructured data is getting closer to 80% of the existing information in the digital world , it’s easy to understand why this brings outstanding opportunities for businesses, organizations, and academic researchers.
For companies, it is now possible to obtain real-time insights on how their users feel about their products and make better business decisions based on data. Shifting to a data-driven approach is one of the main challenges of businesses today.
Textual analysis has many exciting applications across different areas of a company, like customer service, marketing, product, or sales. By allowing the automation of specific tasks that used to be manual, textual analysis is helping teams become more productive and efficient, and allowing them to focus on areas where they can add real value.
In the academic research field, computer-assisted textual analysis (and mainly, machine learning-based models) are expanding the horizons of investigation, by providing new ways of processing, classifying, and obtaining relevant data.
In this section, we’ll describe the most significant applications related to customer service, customer feedback, and academic research.
Customer Service
It’s not all about having an amazing product or investing a lot of money on advertising. What really tips the balance when it comes to business success is to provide high-quality customer service. Stats claim that 70% of the customer journey is defined by how people feel they are being treated .
So, how can textual analysis help companies deliver a better customer service experience?
Automatically tag support tickets
Every time a customer sends a request, comment, or complain, there’s a new support ticket to be processed. Customer support teams need to categorize every incoming message based on its content, a routine task that can be boring, time-consuming, and inconsistent if done manually.
Textual analysis with machine learning allows you automatically identify the topic of each support ticket and tag it accordingly. How does it work?
- First, a person defines a set of categories and trains a classifier model by applying the appropriate tags to a number of representative samples.
- The model analyzes the words and expressions used in each ticket. For example: ‘I’m having problems when paying with my credit card’ , and it compares it with previous examples.
- Finally, it automatically tags the ticket according to its content. In this case, the ticket would be tagged as Payment Issues .
Automatically route and triage support tickets
Once support tickets are tagged, they need to be routed to the appropriate team in charge to deal with that issue. Machine learning enables teams to send a ticket to the right person in real-time , based on the ticket’s topic, language or complexity. For example, a ticket previously tagged as Payment Issues will be automatically routed to the Billing Area .
Detect the urgency of a ticket
A simple task, like being able to prioritize tickets based on their urgency, can have a substantial positive impact on your customer service. By analyzing the content of each ticket, a textual analysis model can let you assess which of them are more critical and prioritize accordingly . For instance, a ticket containing the words or expressions ‘as soon as possible’ or ‘immediately’ would be automatically classified as Urgent .
Get insights from ticket analytics
The performance of customer service teams is usually measured by KPI’s, like first response time, the average time of resolution, and customer satisfaction (CSAT).
Textual analysis algorithms can be used to analyze the different interactions between customers and the customer service area, like chats, support tickets, emails, and customer satisfaction surveys.
You can use aspect-based sentiment analysis to understand the main topics discussed by your customers and how they are feeling about those topics. For example, you may have a lot of mentions referring to the topic ‘UI/UX’ . But, are all those customers’ opinions positive, negative, or neutral? This type of analysis can provide a more accurate perspective of what they think about your product and get a deeper understanding the overall customer satisfaction.
Customer Feedback
Listening to the Voice of Customer (VoC) is critical to understand the customers’ expectations, experience and opinion about your brand. Two of the most common tools to monitor and examine customer feedback are customer surveys and product reviews.
By analyzing customer feedback data, companies can detect topics for improvement, spot product flaws, get a better understanding of your customer’s needs and measure their level of satisfaction, among many other things.
But how do you process and analyze tons of reviews or thousands of customer surveys? Here are some ideas of how you can use textual analysis algorithms to analyze different kinds of customer feedback:
Analyze NPS Responses
Net Promoter Score (NPS) is the most popular tool to measure customer satisfaction. The first part of the survey involves giving the brand a score from 0 to 10 based on the question: 'How likely is it that you would recommend [brand] to a friend or colleague?' . The results allow you to classify your customers as promoters , passives , and detractors .
Then, there’s a follow-up question, inquiring about the reasons for your previous score. These open-ended responses often provide the most insightful information about your company. At the same time, it’s the most complex data to process. Yes, you could read and tag each of the responses manually, but what if there are thousands of them?
Textual analysis with machine learning enables you to detect the main topics that your customers are referring to, and even extract the most relevant keywords related to those topics . To make the most of your data, you could also perform sentiment analysis and find out if your customers are talking about a given topic positively or negatively.
Analyze Customer Surveys
Besides NPS, textual analysis algorithms can help you analyze all sorts of customer surveys. Using a text classification model to tag your responses can make you save a lot of valuable time and resources while allowing you to obtain consistent results.
Analyze Product Reviews
Product reviews are a significant factor when buying a product. Prospective buyers read at least 10 reviews before feeling they can trust a local business and that’s just one of the (many) reasons why you should keep a close eye on what people are saying about your brand online.
Analyzing product reviews can give you an idea of what people love and hate the most about your product and service. It can provide useful insights and opportunities for improvement. And it can show you what to do to get one step ahead of your competition.
The truth is that going through pages and pages of product reviews is not a very exciting task. Categorizing all those opinions can take teams hours and in the end, it becomes an expensive and unproductive process. That’s why automated textual analysis is a game-changer.
Imagine you want to analyze a set of product reviews from your SaaS company in G2 Crowd. A textual analysis model will allow you to tag g each review based on topic, like Ease of Use , Price , UI/UX , Integrations . You could also run a sentiment analysis to discover how your customers feel about those topics: do they think the price is suitable or too expensive? Do they find it too complex or easy to use?
Thanks to textual analysis algorithms, you can get powerful information to help you make data-driven decisions, and empower your teams to be more productive by reducing manual tasks to a minimum.
Academic Research
What if you were able to sift through tons of papers and journals, and discover data that is relevant to your research in just seconds? Just imagine if you could easily classify years of news articles and extract meaningful keywords from them, or analyze thousands of tweets after a significant political change .
Even though machine learning applications in business and science seem to be more frequent, social science research is also benefiting from ML to perform tasks related to the academic world.
Social science researchers need to deal with vast volumes of unstructured data. Therefore, one of the major opportunities provided by computer-assisted textual analysis is being able to classify data, extract relevant information, or identify different groups in extensive collections of data.
Another application of textual analysis with machine learning is supporting the coding process . Coding is one of the early steps of any qualitative textual analysis. It involves a detailed examination of what you want to analyze to become familiar with the data. When done manually, this task can be very time consuming and often inaccurate or inconsistent. Fortunately, machine learning algorithms (like text classifier models) can help you do this in very little time and allow you to scale up the coding process easily.
Finally, using machine learning algorithms to scan large amounts of papers, databases, and journal articles can lead to new investigation hypotheses .
Final Words
In a world overloaded with data, textual analysis with machine learning is a powerful tool that enables you to make sense of unstructured information and find what’s relevant in just seconds.
With promising use cases across many fields from marketing to social science research, machine learning algorithms are far from being a niche technology only available for a few. Moreover, they are turning into user-friendly applications that are dominated by workers with little or no coding skills.
Thanks to text analysis models, teams are becoming more productive by being released from manual and routine tasks that used to take valuable time from them. At the same time, companies can make better decisions based on valuable, real-time insights obtained from data.
By now, you probably have an idea of what textual analysis is with machine learning and how you can use it to make your everyday tasks more efficient and straightforward. Ready to get started? MonkeyLearn makes it very simple to take your first steps. Just contact us and get a personalized demo from one of our experts!
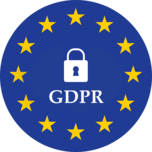
MonkeyLearn Inc. All rights reserved 2024
Table of Contents
Collaboration, information literacy, writing process, textual analysis – how to engage in textual analysis.
- © 2023 by Jennifer Janechek - IBM Quantum
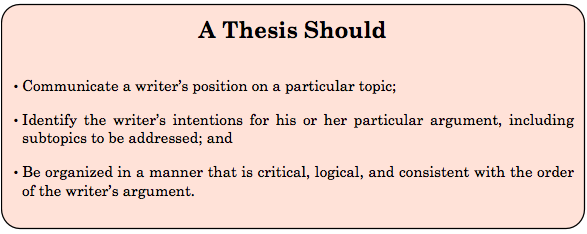
As a reader, a developing writer, and an informed student and citizen, you need to be able to locate, understand, and critically analyze others’ purposes in communicating information. Being able to identify and articulate the meaning of other writers’ arguments and theses enables you to engage in intelligent, meaningful, and critical knowledge exchanges. Ultimately, regardless of the discipline you choose to participate in, textual analysis —the summary, contextualization, and interpretation of a writer’s effective or ineffective delivery of their perspective on a topic, statement of thesis, and development of an argument—will be an invaluable skill. Your ability to critically engage in knowledge exchanges—through the analysis of others’ communication—is integral to your success as a student and as a citizen.
Step 1: What Is The Thesis?
In order to learn how to better recognize a thesis in a written text, let’s consider the following argument:
So far, [Google+] does seem better than Facebook, though I’m still a rookie and don’t know how to do even some basic things.
It’s better in design terms, and also much better with its “circles” allowing you to target posts to various groups.
Example: following that high school reunion, the overwhelming majority of my Facebook friends list (which I’m barely rebuilding after my rejoin) are people from my own hometown. None of these people are going to care too much when my new book comes out from Edinburgh. Likewise, not too many of you would care to hear inside jokes about our old high school teachers, or whatever it is we banter about.
Another example: people I know only from exchanging a couple of professional emails with them ask to be Facebook friends. I’ve never met these people and have no idea what they’re really like, even if they seem nice enough on email. Do I really want to add them to my friends list on the same level as my closest friends, brothers, valued colleagues, etc.? Not yet. But then there’s the risk of offending people if you don’t add them. On Google+ you can just drop them in the “acquaintances” circle, and they’ll never know how they’re classified.
But they won’t be getting any highly treasured personal information there, which is exactly the restriction you probably want for someone you’ve never met before.
I also don’t like too many family members on my Facebook friends list, because frankly they don’t need to know everything I’m doing or chatting about with people. But on Google+ this problem will be easily manageable. (Harman)
The first sentence, “[Google+] does seem better than Facebook” (Harman), doesn’t communicate the writer’s position on the topic; it is merely an observation . A position, also called a “claim,” often includes the conjunction “because,” providing a reason why the writer’s observation is unique, meaningful, and critical.https://www.youtube.com/embed/rwSFfnlwtjY?rel=0&feature=youtu.beTherefore, if the writer’s sentence, “[Google+] does seem better than Facebook” (Harman), is simply an observation, then in order to identify the writer’s position, we must find the answer to “because, why?” One such answer can be found in the author’s rhetorical question/answer, “Do I really want to add them to my friends list on the same level as my closest friends, brothers, valued colleagues, etc.? Not yet” (Harman). The writer’s “because, why?” could be “because Google+ allows me to manage old, new, and potential friends and acquaintances using separate circles, so that I’m targeting posts to various, separate groups.” Therefore, the writer’s thesis—their position—could be something like, “Google+ is better than Facebook because its design enables me to manage my friends using separate circles, so that I’m targeting posts to various, separate groups instead of posting the same information for everyone I’ve added to my network.”
In addition to communicating a position on a particular topic, a writer’s thesis outlines what aspects of the topic they will address. Outlining intentions within a thesis is not only acceptable, but also one of a writer’s primary obligations, since the thesis relates their general argument. In a sense, you could think of the thesis as a responsibility to answer the question, “What will you/won’t you be claiming and why?”
To explain this further, let’s consider another example. If someone were to ask you what change you want to see in the world, you probably wouldn’t readily answer “world peace,” even though you (and many others) may want that. Why wouldn’t you answer that way? Because such an answer is far too broad and ambiguous to be logically argued. Although world peace may be your goal, for logic’s sake, you would be better off articulating your answer as “a peaceful solution to the violence currently occurring on the border of southern Texas and Mexico,” or something similarly specific. The distinction between the two answers should be clear: the first answer, “world peace,” is broad, ambiguous, and not a fully developed claim (there wouldn’t be many, if any, people who would disagree with this statement); the second answer is narrower, more specific, and a fully developed claim. It confines the argument to a particular example of violence, but still allows you to address what you want, “world peace,” on a smaller, more manageable, and more logical scale.
Since a writer’s thesis functions as an outline of what they will address in an argument, it is often organized in the same manner as the argument itself. Let’s return to the argument about Google+ for an example. If the author stated their position as suggested—“Google+ is better than Facebook because its design enables me to manage my friends using separate circles, so that I’m targeting posts to various, separate groups instead of posting the same information I’ve added to my network”—we would expect them to first address the similarities and differences between the designs of Google+ and Facebook, and then the reasons why they believe Google+ is a more effective way of sharing information. The organization of their thesis should reflect the overall order of their argument. Such a well-organized thesis builds the foundation for a cohesive and persuasive argument.
Textual Analysis: How is the Argument Structured?
“Textual analysis” is the term writers use to describe a reader’s written explanation of a text. The reader’s textual analysis ought to include a summary of the author’s topic, an analysis or explanation of how the author’s perspective relates to the ongoing conversation about that particular topic, an interpretation of the effectiveness of the author’s argument and thesis , and references to specific components of the text that support his or her analysis or explanation.
An effective argument generally consists of the following components:
- A thesis. Communicates the writer’s position on a particular topic.
- Acknowledgement of opposition. Explains existing objections to the writer’s position.
- Clearly defined premises outlining reasoning. Details the logic of the writer’s position.
- Evidence of validating premises. Proves the writer’s thorough research of the topic.
- A conclusion convincing the audience of the argument’s soundness/persuasiveness. Argues the writer’s position is relevant, logical, and thoroughly researched and communicated.
An effective argument also is specifically concerned with the components involved in researching, framing, and communicating evidence:
- The credibility and breadth of the writer’s research
- The techniques (like rhetorical appeals) used to communicate the evidence (see “The Rhetorical Appeals”)
- The relevance of the evidence as it reflects the concerns and interests of the author’s targeted audience
To identify and analyze a writer’s argument, you must critically read and understand the text in question. Focus and take notes as you read, highlighting what you believe are key words or important phrases. Once you are confident in your general understanding of the text, you’ll need to explain the author’s argument in a condensed summary. One way of accomplishing this is to ask yourself the following questions:
- What topic has the author written about? (Explain in as few words as possible.)
- What is the author’s point of view concerning their topic?
- What has the author written about the opposing point of view? (Where does it appear as though the author is “giving credit” to the opposition?)
- Does the author offer proof (either in reference to another published source or from personal experience) supporting their stance on the topic?
- As a reader, would you say that the argument is persuasive? Can you think of ways to strengthen the argument? Using which evidence or techniques?
Your articulation of the author’s argument will most likely derive from your answers to these questions. Let’s reconsider the argument about Google+ and answer the reflection questions listed above:
The author’s topic is two social networks—Google+ and Facebook.
The author is “for” the new social network Google+.
The author makes a loose allusion to the opposing point of view in the explanation, “I’m still a rookie and don’t know how to do even some basic things” (Harman). (The author alludes to his inexperience and, therefore, the potential for the opposing argument to have more merit.)
Yes, the author offers proof from personal experience, particularly through their first example: “following that high school reunion, the overwhelming majority of my Facebook friends list (which I’m barely rebuilding after my rejoin) are people from my hometown” (Harman). In their second example, they cite that “[o]n Google+ you can just drop [individuals] in the ‘acquaintances’ circle, and they’ll never even know how they’re classified” (Harman) in order to offer even more credible proof, based on the way Google+ operates instead of personal experience.
Yes, I would say that this argument is persuasive, although if I wanted to make it even stronger, I would include more detailed information about the opposing point of view. A balanced argument—one that fairly and thoroughly articulates both sides—is often more respected and better received because it proves to the audience that the writer has thoroughly researched the topic prior to making a judgment in favor of one perspective or another.
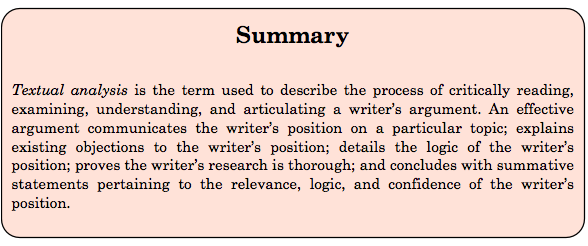
Works Cited
Harman, Graham. Object-Oriented Philosophy. WordPress, n.d. Web. 15 May 2012.
Related Articles:
Annotating the margins, textual analysis - how to analyze ads, suggested edits.
- Please select the purpose of your message. * - Corrections, Typos, or Edits Technical Support/Problems using the site Advertising with Writing Commons Copyright Issues I am contacting you about something else
- Your full name
- Your email address *
- Page URL needing edits *
- Phone This field is for validation purposes and should be left unchanged.
- Jennifer Janechek
Featured Articles
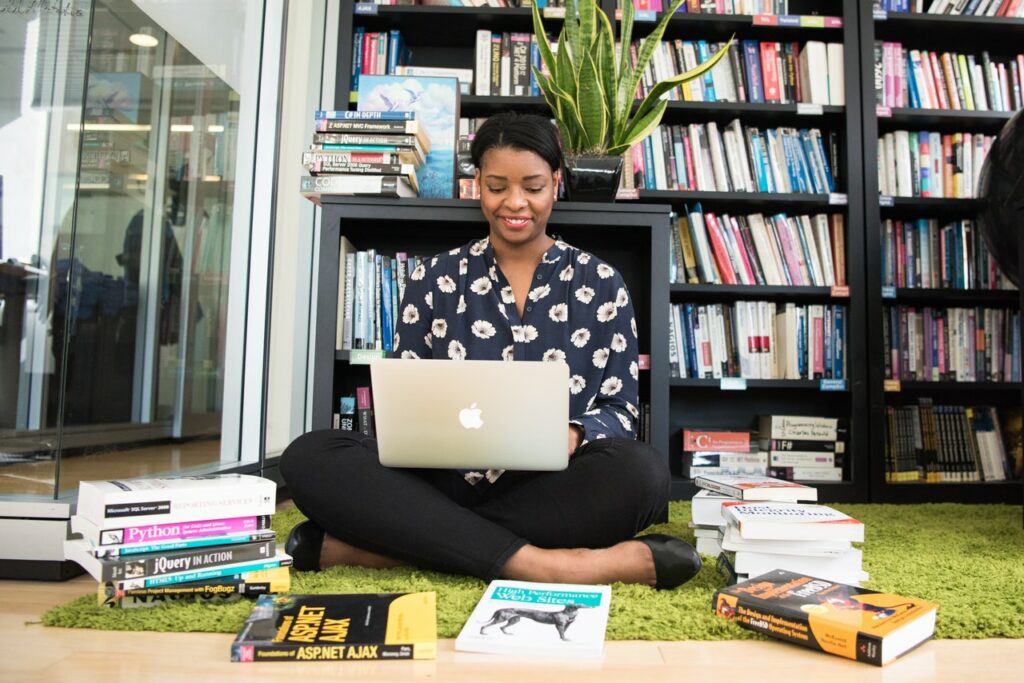
Academic Writing – How to Write for the Academic Community
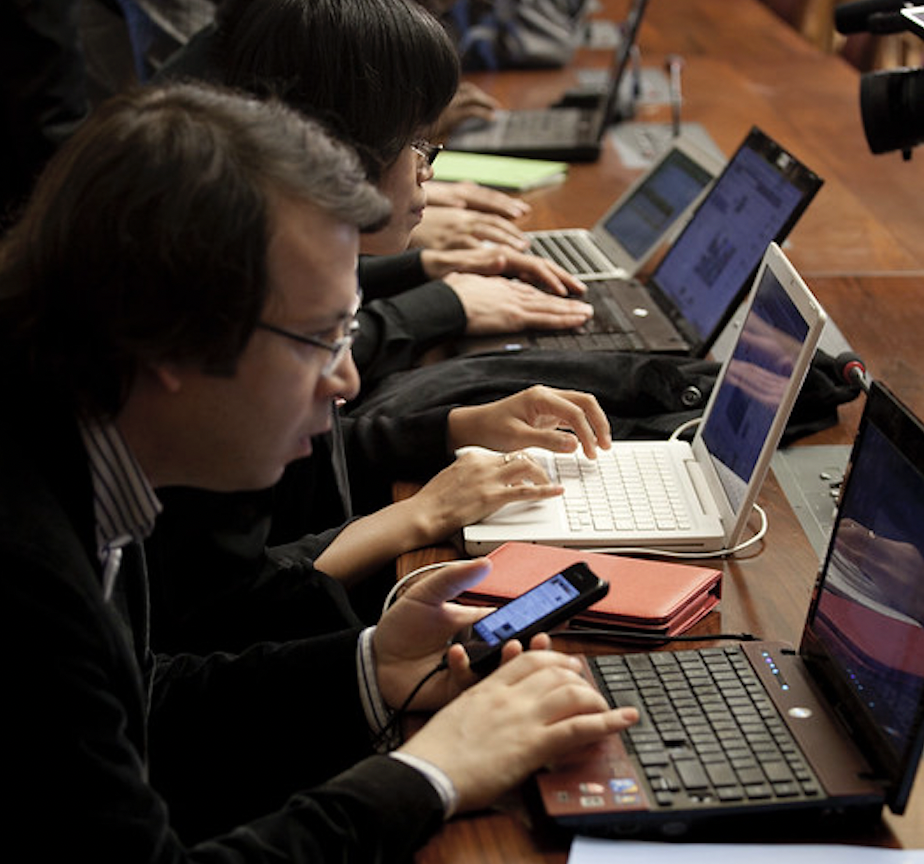
Professional Writing – How to Write for the Professional World
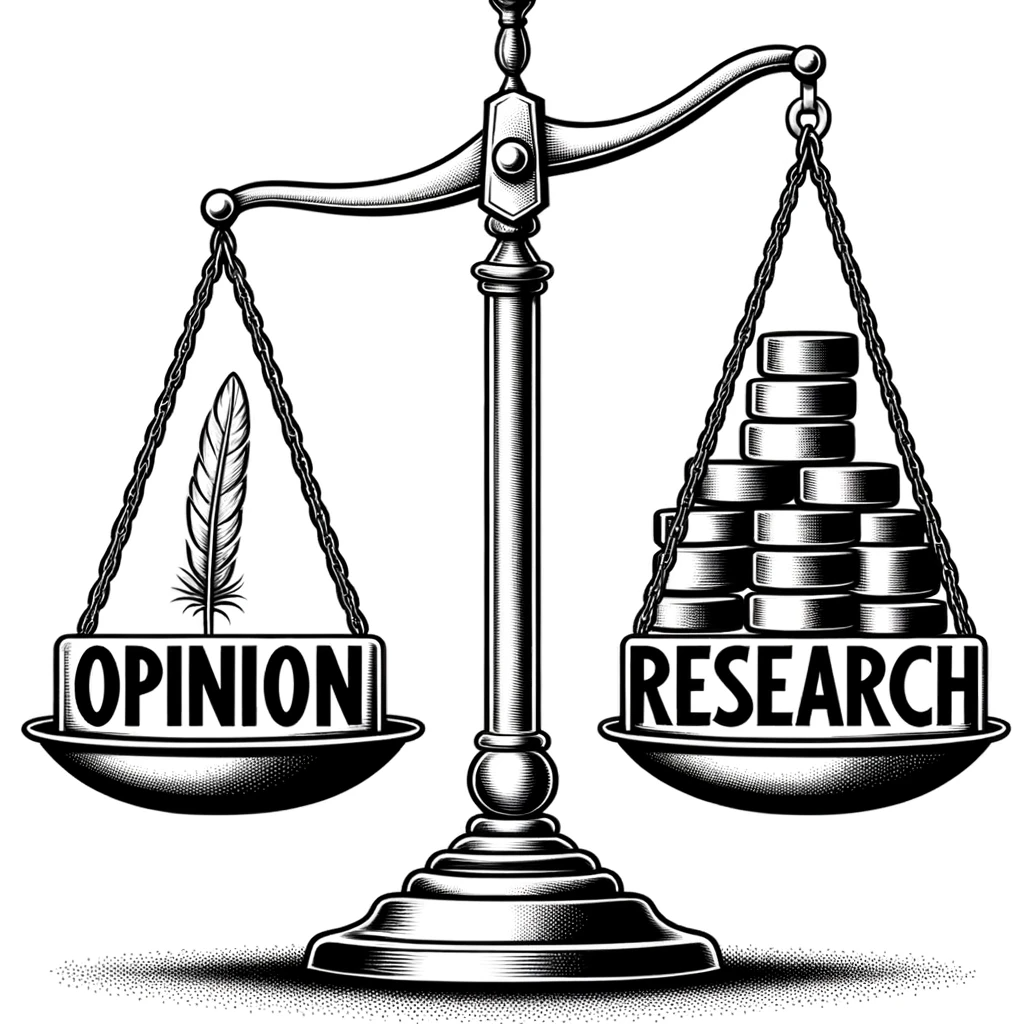
Authority – How to Establish Credibility in Speech & Writing
No internet connection.
All search filters on the page have been cleared., your search has been saved..
- All content
- Dictionaries
- Encyclopedias
- Expert Insights
- Foundations
- How-to Guides
- Journal Articles
- Little Blue Books
- Little Green Books
- Project Planner
- Tools Directory
- Sign in to my profile My Profile
- Sign in Signed in
- My profile My Profile
Textual Analysis
- By: Alan McKee
- Publisher: SAGE Publications Ltd
- Publication year: 2003
- Online pub date: January 01, 2011
- Discipline: Anthropology
- Methods: Textual analysis , Qualitative interviewing , Programming
- DOI: https:// doi. org/10.4135/9780857020017
- Keywords: discipline , film , films , identity , knowledge , sense-making , television Show all Show less
- Print ISBN: 9780761949930
- Online ISBN: 9780857020017
- Buy the book icon link
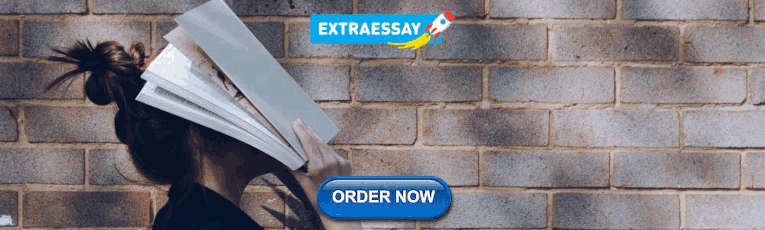
Subject index
`Alan McKee presents a student friendly introduction to the analysis of cultural texts. The book highlights the cultural differences in interpretation with an array of fascinating examples. Textual Analysis is written in an accessible style with several useful case studies. Each chapter also includes exercises for classroom' - Jane Stokes, London Metropolitan University. `McKee is a gifted practitioner of the skills he would teach in this book, as well as a lively and engaging writer and one who has a real commitment to making his ideas available to a larger public' - Henry Jenkins, Massachusetts Institute of Technology. This book provides an indispensable basic introduction to textual analysis. McKee starts from the most basic philosophical foundations that underlie the practice and explains why texts are important and what they tell us about the world they represent. Textual Analysis guides students away from finding the `correct' interpretation of a text and explains why we can't simply ask audiences about the interpretations they make of texts. Textual Analysis: - points to the importance of context, genre and modality - uses excellent examples drawn from popular culture - provides students with a solid grounding on many of the important concepts underlying media and cultural studies. Written in an accessible and straightforward style Textual Analysis : A Beginners Guide will be essential reading for all students of media, cultural and communication studies.
Front Matter
- Acknowledgements
- What Is Textual Analysis?
- Does It Really Matter How People Make Sense of the World?
- What's Interpretation Got to Do with It?
- How Do I Know What's a Likely Interpretation?
- Can't We Make It a Bit More Scientific?
- Is That It?
Back Matter
Sign in to access this content, get a 30 day free trial, more like this, sage recommends.
We found other relevant content for you on other Sage platforms.
Have you created a personal profile? Login or create a profile so that you can save clips, playlists and searches
- Sign in/register
Navigating away from this page will delete your results
Please save your results to "My Self-Assessments" in your profile before navigating away from this page.
Sign in to my profile
Sign up for a free trial and experience all Sage Learning Resources have to offer.
You must have a valid academic email address to sign up.
Get off-campus access
- View or download all content my institution has access to.
Sign up for a free trial and experience all Sage Research Methods has to offer.
- view my profile
- view my lists
Analyzing Text Data
- Overview of Text Analysis and Text Mining
Choosing a Method
How much data do you need, word frequency analysis, machine learning/natural language processing, sentiment analysis.
- Library Databases
- Social Media
- Open Source
- Language Corpora
- Web Scraping
- Software for Text Analysis
- Text Data Citation
Library Data Services
Choosing the right text mining method is crucial because it significantly impacts the quality of insights and information you can extract from your text data. Each method provides different insights and requires different amounts of data, training, and iteration. Before you search for data, it is essential that you:
- identify the goals of your analysis
- determine the method you will use to meet those goals
- identify how much data you need for that method
- develop a sampling plan to build a data set that accurately represents your object of study.
Starting with this information in mind will make your project go more quickly and smoothly, and help you overcome a lot of hurdles such as incomplete data, too much or too little data, or problems with access to data.
More Resources:
- Content Analysis Method and Examples, from Mailman School of Public Health, Columbia University
- Qualitative Research Methods Overview, from Northeastern University
Before you start collecting data, think about how much data you really need. New researchers in text analysis often want to collect every source mentioning their topic, but this is usually not the best approach. Collecting so much data takes a lot of time, uses many computational resources, often goes against platform terms of service, and doesn't necessarily improve analysis.
In text analysis, an essential idea is saturation , where adding more data doesn't significantly improve performance. Saturation is when the model has learned as much as it can from the available data, and no new patterns are themes are emerging with additional data. Researchers often use experimentation and learning curves to determine when saturation occurs; you can start by analyzing a small or mid-sized dataset and see what happens if you add more data.
Once you know your research question, the next step is to create a sampling plan . In text analysis, sampling means selecting a representative subset of data from a larger dataset for analysis. This subset, called the sample, aims to capture the diversity of sentiments in the overall dataset. The goal is to analyze this smaller portion to draw conclusions about the information in the entire dataset.
For example, in a large collection of customer reviews, sampling may involve randomly selecting a subset for sentiment analysis instead of analyzing every single review. This approach saves computational resources and time while still providing insights into the overall sentiment distribution of the entire dataset. It's crucial to ensure that the sample accurately reflects the diversity of sentiments in the complete dataset for valid and reliable generalizations.
Example Sampling Plans
Sampling plans for text analysis involve selecting a subset of text data for analysis rather than analyzing the entire dataset. Here are two common sampling plans for text analysis:
Random Sampling:
- Description: Randomly select a subset of text documents from the entire dataset.
- Process: Assign each document a unique identifier and use a random number generator to choose documents for inclusion in the sample.
Stratified Sampling:
- Description: Divide the dataset into distinct strata or categories based on certain characteristics (e.g., product types, genres, age groups, race or ethnicity). Then, randomly sample from each stratum.
- Process: Divide the dataset into strata, and within each stratum, use random sampling to select a representative subset.
Remember, the choice of sampling plan depends on the specific goals of the analysis and the characteristics of the dataset. Random sampling is straightforward and commonly used when there's no need to account for specific characteristics in the dataset. Stratified sampling is useful when the dataset has distinct groups, and you want to ensure representation from each group in the sample.
Exactly How Many Sources do I need?
Determining the amount of data needed for text analysis involves a balance between having enough data to train a reliable model and avoiding unnecessary computational costs. The ideal dataset size depends on several factors, including the complexity of the task, the diversity of the data, and the specific algorithms or models being used.
- Task Complexity: If you are doing a simple task, like sentiment analysis or basic text classification, a few dozen articles might be enough. More complex tasks, like language translation or summarization, often require datasets on the scale of tens of thousands to millions.
- Model Complexity: Simple models like Naive Bayes often perform well with smaller datasets, whereas complex models, such as deep learning models with many parameters, will require larger datasets for effective training.
- Data Diversity: Ensure that the dataset is diverse and representative of the various scenarios the model will encounter. A more diverse dataset can lead to a more robust and generalizable model. A large dataset that is not diverse will yield worse results than a smaller, more diverse dataset.
- Domain-Specific Considerations: Sometimes there is not a lot of data available, and it is okay to make do with what you have!
Start by taking a look at articles in your field that have done a similar analysis. What approaches did they take? You can also schedule an appointment with a Data Services Librarian to get you started.
More Readings on Sampling Plans for Text Analysis:
- How to Choose a Sample Size in Qualitative Research, from LinkedIn Learning (members of the GW community have free access to LinkedIn Learning using their GW email account)
- Sampling in Qualitative Research , from Saylor Academy
- Lowe, A., Norris, A. C., Farris, A. J., & Babbage, D. R. (2018). Quantifying Thematic Saturation in Qualitative Data Analysis. Field Methods, 30(3), 191-207. https://doi.org/10.1177/1525822X17749386

Software for Word Frequency Analysis
- NVivo via GW's Virtual Computer Lab NVivo is a software package used for qualitative data analysis. It includes tools to support the analysis of textual data in a wide array of formats, as well as and audio, video, and image data. NVivo is available through the Virtual Computer Lab. Faculty and staff may find NVivo available for download from GW's Software Center.
- Analyzing Word and Document Frequency in R This chapter explains how to use tidy to analyze word and document frequency using Tidy Data in R.
- word clouds in R R programming functionality to create pretty word clouds, visualize differences and similarities between documents, and avoid over-plotting in scatter plots with text.
- ATLAS.ti Trial version of qualitative analysis workbench for processing text, image, audio, and video data. (Note: Health science students may have access to full version through Himmelfarb Library)
Related Tools Available Online
- Google ngram Viewer When you enter phrases into the Google Books Ngram Viewer, it displays a graph showing how those phrases have occurred in a corpus of books (e.g., "British English", "English Fiction", "French") over the selected years.
- HathiTrust This link opens in a new window HathiTrust is a partnership of academic and research institutions, offering a collection of millions of titles digitized from libraries around the world. To log in, select The George Washington University as your institution, then log in with your UserID and regular GW password.
- Voyant Voyant is an online point-and-click tool for text analysis. While the default graphics are impressive, it allows limited customizing of analysis and graphs and may be most useful for exploratory visualization.
Related Library Resources
- HathiTrust and Text Mining at GWU HathiTrust is an international community of research libraries committed to the long-term curation and availability of the cultural record. Through their common efforts and deep commitment to the public good, the libraries support the teaching and learning activities of the faculty, students or researchers at their home institutions, and the scholarly needs of the broader public as well.
- HathiTrust+Bookworn From the University of Illinois Library: HathiTrust+Bookworm is an online tool for visualizing trends in language over time. Developed by the HathiTrust Research Center using textual data from the HathiTrust Digital Library, it allows you to track changes in word use based on publication country, genre of works, and more.
- Python for Natural Language Processing A workshop offered through GW Libraries on natural language processing using Python.
- Text Mining Tutorials in R A collection of text mining course materials and tutorials developed for humanists and social scientists interested to learn R.
- Oxford English Dictionary This link opens in a new window The Oxford English Dictionary database will provide a word frequency analysis over time, drawing both from Google ngrams and the OED's own databases.
Example Projects Using Word Frequency Analysis
Robinson, J. S. and D. (n.d.). 3 Analyzing word and document frequency: Tf-idf | Text Mining with R . Retrieved November 21, 2023, from https://www.tidytextmining.com/tfidf.html
- Zhang, Z. (n.d.). Text Mining for Social and Behavioral Research Using R . Retrieved November 21, 2023, from https://books.psychstat.org/textmining/index.html
- Exploring Fascinating Insights with Word Frequency Analysis In the realm of data analysis, words hold immense power. They convey meaning, express ideas, and shape our understanding of the world. In this article, we’ll explore the fascinating world of textual data analysis by examining word frequencies. By counting the occurrence of words in a text, we can uncover interesting insights and gain a deeper understanding of the underlying themes and patterns. Join us on this word-centric journey as we dive into the realm of word frequency analysis using Python.
Machine learning for text analysis is a technology that teaches computers to understand and interpret written language by exposing them to examples. There are two types of machine learning for text analysis: supervised learning, in which a human helps to train the computer to detect patterns, and unsupervised learning, which enables computers to automatically categorize, analyze, and extract information from text without needing explicit programming.
One type of machine learning for text analysis is Natural Language Processing (NLP). NLP for text analysis is a field of artificial intelligence that involves the development and application of algorithms to automatically process, understand, and extract meaningful information from human language in textual form. NLP techniques are used to analyze and derive insights from large volumes of text data, enabling tasks such as sentiment analysis, named entity recognition, text classification, and language translation. The aim is to equip computers with the capability to comprehend and interpret written language, making it possible to automate various aspects of text-based information processing.
Software for Natural Language Processing
- NLTK for Python NLTK is a leading platform for building Python programs to work with human language data. It provides easy-to-use interfaces to over 50 corpora and lexical resources such as WordNet, along with a suite of text processing libraries for classification, tokenization, stemming, tagging, parsing, and semantic reasoning, wrappers for industrial-strength NLP libraries, and an active discussion forum.
- scikit Simple and efficient tools for predictive data analysis, using Python.
Related Resources Available Online
- [Large Language Models] LLMs in Scientific Research
- HathiTrust and Text Mining at GWU Information on text data mining using HathiTrust
- Social Feed Manager Social Feed Manager software was developed to support campus research about social media including Twitter, Tumblr, Flickr, and Sina Weibo platforms. It can be used to track mentions of you or your articles and other research products for the previous seven days and on into the future.. Email [email protected] to get started with Social Feed Manager or to schedule a consultation
Example Projects using Natural Language Processing
- Redd D, Workman TE, Shao Y, Cheng Y, Tekle S, Garvin JH, Brandt CA, Zeng-Treitler Q. Patient Dietary Supplements Use: Do Results from Natural Language Processing of Clinical Notes Agree with Survey Data? Medical Sciences . 2023; 11(2):37. https://doi.org/10.3390/medsci11020037
- Nguyen D, Liakata M, DeDeo S, Eisenstein J, Mimno D, Tromble R, Winters J. How We Do Things With Words: Analyzing Text as Social and Cultural Data. Front Artif Intell. 2020 Aug 25;3:62. doi: 10.3389/frai.2020.00062.
Sentiment analysis is a method of analyzing text to determine whether the emotional tone or sentiment expressed in a piece of text is positive, negative, or neutral. Sentiment analysis is commonly used in businesses to gauge customer feedback, social media monitoring, and market research.
Software for Sentiment Analysis
- Sentiment Analysis using NLTK for Python NLTK is a leading platform for building Python programs to work with human language data. It provides easy-to-use interfaces to over 50 corpora and lexical resources such as WordNet, along with a suite of text processing libraries for classification, tokenization, stemming, tagging, parsing, and semantic reasoning, wrappers for industrial-strength NLP libraries, and an active discussion forum.
- Sentiment Analysis with TidyData in R This chapter shows how to implement sentiment analysis using tidy data principles in R.
- Tableau Tableau works with numeric and categorical data to produce advanced graphics. Browse the Tableau public gallery to see examples of visuals and dashboards. Tableau offers free one-year Tableau licenses to students at accredited academic institutions, including GW. Visit https://www.tableau.com/academic/students for more about the program or to request a license.
- Qualtrics Text iQ Qualtrics is a powerful tool for collecting and analyzing survey data. Qualtrics Text iQ automatically performs sentiment analysis on collected data.
Related Resources Available Online
- finnstats. (2021, May 16). Sentiment analysis in R | R-bloggers. https://www.r-bloggers.com/2021/05/sentiment-analysis-in-r-3/
Example Projects Using Sentiment Analysis
- Duong, V., Luo, J., Pham, P., Yang, T., & Wang, Y. (2020). The Ivory Tower Lost: How College Students Respond Differently than the General Public to the COVID-19 Pandemic. 2020 IEEE/ACM International Conference on Advances in Social Networks Analysis and Mining (ASONAM), 126–130. https://doi.org/10.1109/ASONAM49781.2020.9381379
- Ali, R. H., Pinto, G., Lawrie, E., & Linstead, E. J. (2022). A large-scale sentiment analysis of tweets pertaining to the 2020 US presidential election. Journal of Big Data, 9(1). https://doi.org/10.1186/s40537-022-00633-z
- << Previous: Overview of Text Analysis and Text Mining
- Next: Finding Text Data >>
- Last Updated: Feb 7, 2024 10:26 AM
- URL: https://libguides.gwu.edu/textanalysis
- How it works
A Quick Guide to Textual Analysis – Definition & Steps
Published by Alvin Nicolas at August 16th, 2021 , Revised On August 29, 2023
Textual analysis is the method of analysing and understanding the text. It includes the close reading of fictional texts, images symbols, and all forms of written literary texts. A researcher needs to look keenly at the text to identify the writer’s context and its message.
What is a Text?
- A text can be anything from which you can extract any meaning or information.
- A text can be a written book, records, journals, newspapers, magazines, ornaments, objects, emails, or transcribed conversations.
- It also includes analysing events, places, videos, images, or movies to get in-depth knowledge about its creation and purpose.
Why do we Call it a Text?
You might be thinking, why don’t we call it a book, video, or magazine? Why do we call it a text ?
The word text means the post-structuralist approach to thinking about the development of meaning.
The Purpose of Textual Analysis
The purpose of your textual analysis depends on the object of your analysis, such as:
- Analysis of a video or movie may aim to determine the target audience, dialogues, cinematography, visual and sound effects, and the message conveyed through his work.
- Analysis of texts and short stories focus on the language, narrative, choice of words, imagery, perception of the writer, and organisation of the text.
- You can analyse a monument based on its architectural history, stories related to it, and visitors’ navigation.
- You can analyse images in terms of the photographer’s creativity, use of a camera, sense of selecting locations, skills of capturing images, etc.
- You can analyse paintings in terms of their artistic level, use of colours, perception of the painters, the message hidden in the image, etc.
Confused between qualitative and quantitative methods of data analysis? No idea what discourse and content analysis are?
We hear you.
- Whether you want a full dissertation written or need help forming a dissertation proposal, we can help you with both.
- Get different dissertation services at ResearchProspect and score amazing grades!
Textual Analysis of Cultural and Media Studies
A researcher uses videos, social media content, newspapers, maps, buildings, advertisements, and images. Textual analysis plays a key role in the fields of cultural and media studies. A researcher aims to analyse, interpret, and analyse the controversial issues and messages embedded within the text and tries to connect them with other texts or events.
These kinds of texts are analysed from the following aspects:
- Content of the texts
- Language and word choice
- Elements of creativity
- Target audience
- Association with other texts or events
It focuses primarily on the creative and qualitative approach of the text. A researcher identifies the context and hidden message of the text.
Textual Analysis in the Social Sciences
It includes the textual analysis of the interview transcripts and surveys, and other media, including TV programs, chats, social media content. It takes a quantitative approach to measure the characteristics and organisation of the text. You can measure the text’s repetitions, types of colours displayed, tone of the speakers, etc.
Content analysis , thematic analysis , and discourse analysis are the most frequent ways to analyse these texts.
Literary Text Analysis
The literary text analysis is breaking a text’s structure and content into smaller parts to seek in-depth understanding. A researcher It includes stories, poetry, plays, novels, plays, and books.
Also read about: Correlational research , a comprehensive guide on its types with examples
It focuses on identifying:
- The association between various individual texts.
- The similarities between the concepts of the two separate texts.
- The relationship between the texts and political, social, aesthetic, economic, or religious contexts.
Frequently Asked Questions
How to describe textual analysis.
Textual analysis examines written, visual, or spoken material to uncover layers of meaning, themes, and patterns. It dissects language, structure, and context to reveal how elements convey messages, ideologies, and cultural insights, often contributing to a deeper understanding of the content’s significance.
You May Also Like
A variable is a characteristic that can change and have more than one value, such as age, height, and weight. But what are the different types of variables?
A hypothesis is a research question that has to be proved correct or incorrect through hypothesis testing – a scientific approach to test a hypothesis.
Content analysis is used to identify specific words, patterns, concepts, themes, phrases, or sentences within the content in the recorded communication.
USEFUL LINKS
LEARNING RESOURCES
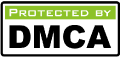
COMPANY DETAILS
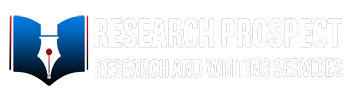
- How It Works
- Architecture and Design
- Asian and Pacific Studies
- Business and Economics
- Classical and Ancient Near Eastern Studies
- Computer Sciences
- Cultural Studies
- Engineering
- General Interest
- Geosciences
- Industrial Chemistry
- Islamic and Middle Eastern Studies
- Jewish Studies
- Library and Information Science, Book Studies
- Life Sciences
- Linguistics and Semiotics
- Literary Studies
- Materials Sciences
- Mathematics
- Social Sciences
- Sports and Recreation
- Theology and Religion
- Publish your article
- The role of authors
- Promoting your article
- Abstracting & indexing
- Publishing Ethics
- Why publish with De Gruyter
- How to publish with De Gruyter
- Our book series
- Our subject areas
- Your digital product at De Gruyter
- Contribute to our reference works
- Product information
- Tools & resources
- Product Information
- Promotional Materials
- Orders and Inquiries
- FAQ for Library Suppliers and Book Sellers
- Repository Policy
- Free access policy
- Open Access agreements
- Database portals
- For Authors
- Customer service
- People + Culture
- Journal Management
- How to join us
- Working at De Gruyter
- Mission & Vision
- De Gruyter Foundation
- De Gruyter Ebound
- Our Responsibility
- Partner publishers

Your purchase has been completed. Your documents are now available to view.
9. Textual Analysis as a Research Method
From the book research methods for english studies.
- Catherine Belsey
- X / Twitter
Supplementary Materials
Please login or register with De Gruyter to order this product.
Chapters in this book (17)
- Open access
- Published: 28 March 2024
Using the consolidated Framework for Implementation Research to integrate innovation recipients’ perspectives into the implementation of a digital version of the spinal cord injury health maintenance tool: a qualitative analysis
- John A Bourke 1 , 2 , 3 ,
- K. Anne Sinnott Jerram 1 , 2 ,
- Mohit Arora 1 , 2 ,
- Ashley Craig 1 , 2 &
- James W Middleton 1 , 2 , 4 , 5
BMC Health Services Research volume 24 , Article number: 390 ( 2024 ) Cite this article
69 Accesses
Metrics details
Despite advances in managing secondary health complications after spinal cord injury (SCI), challenges remain in developing targeted community health strategies. In response, the SCI Health Maintenance Tool (SCI-HMT) was developed between 2018 and 2023 in NSW, Australia to support people with SCI and their general practitioners (GPs) to promote better community self-management. Successful implementation of innovations such as the SCI-HMT are determined by a range of contextual factors, including the perspectives of the innovation recipients for whom the innovation is intended to benefit, who are rarely included in the implementation process. During the digitizing of the booklet version of the SCI-HMT into a website and App, we used the Consolidated Framework for Implementation Research (CFIR) as a tool to guide collection and analysis of qualitative data from a range of innovation recipients to promote equity and to inform actionable findings designed to improve the implementation of the SCI-HMT.
Data from twenty-three innovation recipients in the development phase of the SCI-HMT were coded to the five CFIR domains to inform a semi-structured interview guide. This interview guide was used to prospectively explore the barriers and facilitators to planned implementation of the digital SCI-HMT with six health professionals and four people with SCI. A team including researchers and innovation recipients then interpreted these data to produce a reflective statement matched to each domain. Each reflective statement prefaced an actionable finding, defined as alterations that can be made to a program to improve its adoption into practice.
Five reflective statements synthesizing all participant data and linked to an actionable finding to improve the implementation plan were created. Using the CFIR to guide our research emphasized how partnership is the key theme connecting all implementation facilitators, for example ensuring that the tone, scope, content and presentation of the SCI-HMT balanced the needs of innovation recipients alongside the provision of evidence-based clinical information.
Conclusions
Understanding recipient perspectives is an essential contextual factor to consider when developing implementation strategies for healthcare innovations. The revised CFIR provided an effective, systematic method to understand, integrate and value recipient perspectives in the development of an implementation strategy for the SCI-HMT.
Trial registration
Peer Review reports
Injury to the spinal cord can occur through traumatic causes (e.g., falls or motor vehicle accidents) or from non-traumatic disease or disorder (e.g., tumours or infections) [ 1 ]. The onset of a spinal cord injury (SCI) is often sudden, yet the consequences are lifelong. The impact of a SCI is devastating, with effects on sensory and motor function, bladder and bowel function, sexual function, level of independence, community participation and quality of life [ 2 ]. In order to maintain good health, wellbeing and productivity in society, people with SCI must develop self-management skills and behaviours to manage their newly acquired chronic health condition [ 3 ]. Given the increasing emphasis on primary health care and community management of chronic health conditions, like SCI, there is a growing responsibility on all parties to promote good health practices and minimize the risks of common health complications in their communities.
To address this need, the Spinal Cord Injury Health Maintenance Tool (SCI-HMT) was co-designed between 2018 and 2023 with people living with SCI and their General Practitioners (GPs) in NSW, Australia [ 4 ] The aim of the SCI-HMT is to support self-management of the most common and arguably avoidable potentially life-threatening complications associated with SCI, such as mental health crises, autonomic dysreflexia, kidney infections and pressure injuries. The SCI-HMT provides comprehensible information with resources about the six highest priority health areas related to SCI (as indicated by people with SCI and GPs) and was developed over two phases. Phase 1 focused on developing a booklet version and Phase 2 focused on digitizing this content into a website and smartphone app [ 4 , 5 ].
Enabling the successful implementation of evidence-based innovations such as the SCI-HMT is inevitably influenced by contextual factors: those dynamic and diverse array of forces within real-world settings working for or against implementation efforts [ 6 ]. Contextual factors often include background environmental elements in which an intervention is situated, for example (but not limited to) demographics, clinical environments, organisational culture, legislation, and cultural norms [ 7 ]. Understanding the wider context is necessary to identify and potentially mitigate various challenges to the successful implementation of those innovations. Such work is the focus of determinant frameworks, which focus on categorising or classing groups of contextual determinants that are thought to predict or demonstrate an effect on implementation effectiveness to better understand factors that might influence implementation outcomes [ 8 ].
One of the most highly cited determinant frameworks is the Consolidated Framework for Implementation Research (CFIR) [ 9 ], which is often posited as an ideal framework for pre-implementation preparation. Originally published in 2009, the CFIR has recently been subject to an update by its original authors, which included a literature review, survey of users, and the creation of an outcome addendum [ 10 , 11 ]. A key contribution from this revision was the need for a greater focus on the place of innovation recipients, defined as the constituency for whom the innovation is being designed to benefit; for example, patients receiving treatment, students receiving a learning activity. Traditionally, innovation recipients are rarely positioned as key decision-makers or innovation implementers [ 8 ], and as a consequence, have not often been included in the application of research using frameworks, such as the CFIR [ 11 ].
Such power imbalances within the intersection of healthcare and research, particularly between those receiving and delivering such services and those designing such services, have been widely reported [ 12 , 13 ]. There are concerted efforts within health service development, health research and health research funding, to rectify this power imbalance [ 14 , 15 ]. Importantly, such efforts to promote increased equitable population impact are now being explicitly discussed within the implementation science literature. For example, Damschroder et al. [ 11 ] has recently argued for researchers to use the CFIR to collect data from innovation recipients, and that, ultimately, “equitable population impact is only possible when recipients are integrally involved in implementation and all key constituencies share power and make decisions together” (p. 7). Indeed, increased equity between key constituencies and partnering with innovation recipients promotes the likelihood of sustainable adoption of an innovation [ 4 , 12 , 14 ].
There is a paucity of work using the updated CFIR to include and understand innovation recipients’ perspectives. To address this gap, this paper reports on a process of using the CFIR to guide the collection of qualitative data from a range of innovation recipients within a wider co-design mixed methods study examining the development and implementation of SCI-HMT. The innovation recipients in our research are people living with SCI and GPs. Guided by the CFIR domains (shown in the supplementary material), we used reflexive thematic analysis [ 16 ]to summarize data into reflective summaries, which served to inform actionable findings designed to improve implementation of the SCI-HMT.
The procedure for this research is multi-stepped and is summarized in Fig. 1 . First, we mapped retrospective qualitative data collected during the development of the SCI-HMT [ 4 ] against the five domains of the CFIR in order to create a semi-structured interview guide (Step 1). Then, we used this interview guide to collect prospective data from health professionals and people with SCI during the development of the digital version of the SCI-HMT (Step 2) to identify implementation barriers and facilitators. This enabled us to interpret a reflective summary statement for each CFIR domain. Lastly, we developed an actionable finding for each domain summary. The first (RESP/18/212) and second phase (2019/ETH13961) of the project received ethical approval from The Northern Sydney Local Health District Human Research Ethics Committee. The reporting of this study was conducted in line with the consolidated Criteria for Reporting Qualitative Research (COREQ) guidelines [ 17 ]. All methods were performed in accordance with the relevant guidelines and regulations.
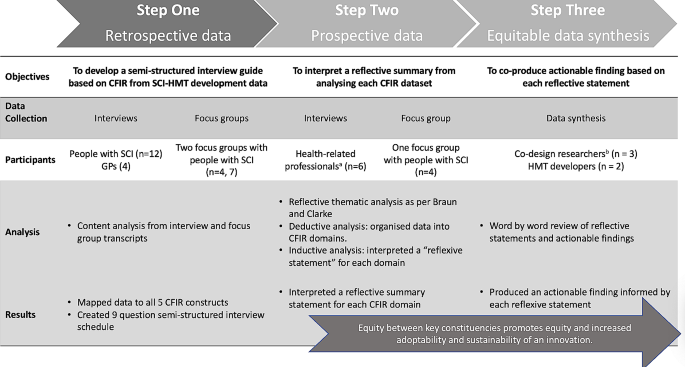
Procedure of synthesising datasets to inform reflective statements and actionable findings. a Two health professionals had a SCI (one being JAB); b Two co-design researchers had a SCI (one being JAB)
Step one: retrospective data collection and analysis
We began by retrospectively analyzing the data set (interview and focus group transcripts) from the previously reported qualitative study from the development phase of the SCI-HMT [ 4 ]. This analysis was undertaken by two team members (KASJ and MA). KASJ has a background in co-design research. Transcript data were uploaded into NVivo software (Version 12: QSR International Pty Ltd) and a directed content analysis approach [ 18 ] was applied to analyze categorized data a priori according to the original 2009 CFIR domains (intervention characteristics, outer setting, inner setting, characteristics of individuals, and process of implementation) described by Damschroder et al. [ 9 ]. This categorized data were summarized and informed the specific questions of a semi-structured interview guide. The final output of step one was an interview guide with context-specific questions arranged according to the CFIR domains (see supplementary file 1). The interview was tested with two people with SCI and one health professional.
Step two: prospective data collection and analysis
In the second step, semi-structured interviews were conducted by KASJ (with MA as observer) with consenting healthcare professionals who had previously contributed to the development of the SCI-HMT. Healthcare professionals included GPs, Nurse Consultants, Specialist Physiotherapists, along with Health Researchers (one being JAB). In addition, a focus group was conducted with consenting individuals with SCI who had contributed to the SCI-HMT design and development phase. The interview schedule designed in step one above guided data collection in all interviews and the focus group.
The focus group and interviews were conducted online, audio recorded, transcribed verbatim and uploaded to NVivo software (Version 12: QSR International Pty Ltd). All data were subject to reflexive, inductive and deductive thematic analysis [ 16 , 19 ] to better understand participants’ perspectives regarding the potential implementation of the SCI-HMT. First, one team member (KASJ) read transcripts and began a deductive analysis whereby data were organized into CFIR domains-specific dataset. Second, KASJ and JAB analyzed this domain-specific dataset to inductively interpret a reflective statement which served to summarise all participant responses to each domain. The final output of step two was a reflective summary statement for each CFIR domain.
Step three: data synthesis
In the third step we aimed to co-create an actionable finding (defined as tangible alteration that can be made to a program, in this case the SCI-HMT [ 20 ]) based on each domain-specific reflective statement. To achieve this, three codesign researchers (KAS and JAB with one person with SCI from Step 2 (deidentified)) focused on operationalising each reflective statement into a recommended modification for the digital version of the SCI-HMT. This was an iterative process guided by the specific CFIR domain and construct definitions, which we deemed salient and relevant to each reflective statement (see Table 2 for example). Data synthesis involved line by line analysis, group discussion, and repeated refinement of actionable findings. A draft synthesis was shared with SCI-HMT developers (JWM and MA) and refinement continued until consensus was agreed on. The final outputs of step three were an actionable finding related to each reflective statement for each CFIR domain.
The characteristics of both the retrospective and prospective study participants are shown in Table 1 . The retrospective data included data from a total of 23 people: 19 people with SCI and four GPs. Of the 19 people with SCI, 12 participated in semi-structured interviews, seven participated in the first focus group, and four returned to the second focus group. In step 2, four people with SCI participated in a focus group and six healthcare professionals participated in one-on-one semi-structured interviews. Two of the healthcare professionals (a GP and a registrar) had lived experience of SCI, as did one researcher (JAB). All interviews and focus groups were conducted either online or in-person and ranged in length between 60 and 120 min.
In our overall synthesis, we actively interpreted five reflective statements based on the updated CFIR domain and construct definitions by Damschroder et al. [ 11 ]. Table 2 provides a summary of how we linked the updated CFIR domain and construct definitions to the reflective statements. We demonstrate this process of co-creation below, including illustrative quotes from participants. Importantly, we guide readers to the actionable findings related to each reflective statement in Table 2 . Each actionable statement represents an alteration that can be made to a program to improve its adoption into practice.
Participants acknowledged that self-management is a major undertaking and very demanding, as one person with SCI said, “ we need to be informed without being terrified and overwhelmed”. Participants felt the HMT could indeed be adapted, tailored, refined, or reinvented to meet local needs. For example, another person with SCI remarked:
“Education needs to be from the get-go but in bite sized pieces from all quarters when readiness is most apparent… at all time points , [not just as a] a newbie tool or for people with [long-term impairment] ” (person with SCI_02).
Therefore, the SCI-HMT had to balance complexity of content while still being accessible and engaging, and required input from both experts in the field and those with lived experience of SCI, for example, a clinical nurse specialist suggested:
“it’s essential [the SCI-HMT] is written by experts in the field as well as with collaboration with people who have had a, you know, the lived experience of SCI” (healthcare professional_03).
Furthermore, the points of contact with healthcare for a person with SCI can be challenging to navigate and the SCI-HMT has the potential to facilitate a smoother engagement process and improve communication between people with SCI and healthcare services. As a GP suggested:
“we need a tool like this to link to that pathway model in primary health care , [the SCI-HMT] it’s a great tool, something that everyone can read and everyone’s reading the same thing” (healthcare professional_05).
Participants highlighted that the ability of the SCI-HMT to facilitate effective communication was very much dependent on the delivery format. The idea of digitizing the SCI-HMT garnered equal support from people with SCI and health care professionals, with one participant with SCI deeming it to be “ essential” ( person with SCI_01) and a health professional suggesting a “digitalized version will be an advantage for most people” (healthcare professional_02).
Outer setting
There was strong interest expressed by both people with SCI and healthcare professionals in using the SCI-HMT. The fundamental premise was that knowledge is power and the SCI-HMT would have strong utility in post-acute rehabilitation services, as well as primary care. As a person with SCI said,
“ we need to leave the [spinal unit] to return to the community with sufficient knowledge, and to know the value of that knowledge and then need to ensure primary healthcare provider [s] are best informed” (person with SCI_04).
The value of the SCI-HMT in facilitating clear and effective communication and shared decision-making between healthcare professionals and people with SCI was also highlighted, as shown by the remarks of an acute nurse specialist:
“I think this tool is really helpful for the consumer and the GP to work together to prioritize particular tests that a patient might need and what the regularity of that is” (healthcare professional_03).
Engaging with SCI peer support networks to promote the SCI-HMT was considered crucial, as one person with SCI emphasized when asked how the SCI-HMT might be best executed in the community, “…peers, peers and peers” (person with SCI_01). Furthermore, the layering of content made possible in the digitalized version will allow for the issue of approachability in terms of readiness for change, as another person with SCI said:
“[putting content into a digital format] is essential and required and there is a need to put summarized content in an App with links to further web-based information… it’s not likely to be accessed otherwise” (person with SCI_02).
Inner setting
Participants acknowledged that self-management of health and well-being is substantial and demanding. It was suggested that the scope, tone, and complexity of the SCI-HMT, while necessary, could potentially be resisted by people with SCI if they felt overwhelmed, as one person with SCI described:
“a manual that is really long and wordy, like, it’s [a] health metric… they maybe lack the health literacy to, to consume the content then yes, it would impede their readiness for [self-management]” (person with SCI_02).
Having support from their GPs was considered essential, and the HMT could enable GP’s, who are under time pressure, to provide more effective health and advice to their patients, as one GP said:
“We GP’s are time poor, if you realize then when you’re time poor you look quickly to say oh this is a patient tool - how can I best use this?” (healthcare professional_05).
Furthermore, health professional skills may be best used with the synthesis of self-reported symptoms, behaviors, or observations. A particular strength of a digitized version would be its ability to facilitate more streamlined communication between a person with SCI and their primary healthcare providers developing healthcare plans, as an acute nurse specialist reflected, “ I think that a digitalized version is essential with links to primary healthcare plans” (healthcare professional_03).
Efficient communication with thorough assessment is essential to ensure serious health issues are not missed, as findings reinforce that the SCI-HMT is an educational tool, not a replacement for healthcare services, as a clinical nurse specialist commented, “ remember, things will go wrong– people end up very sick and in acute care “ (healthcare professional_02).
The SCI-HMT has the potential to provide a pathway to a ‘hope for better than now’ , a hope to ‘remain well’ and a hope to ‘be happy’ , as the informant with SCI (04) declared, “self-management is a long game, if you’re keeping well, you’ve got that possibility of a good life… of happiness”. Participants with SCI felt the tool needed to be genuine and
“acknowledge the huge amount of adjustment required, recognizing that dealing with SCI issues is required to survive and live a good life” (person with SCI_04).
However, there is a risk that an individual is completely overwhelmed by the scale of the SCI-HMT content and the requirement for lifelong vigilance. Careful attention and planning were paid to layering the information accordingly to support self-management as a ‘long game’, which one person with SCI reflected in following:
“the first 2–3 year [period] is probably the toughest to get your head around the learning stuff, because you’ve got to a stage where you’re levelling out, and you’ve kind of made these promises to yourself and then you realize that there’s no quick fix” (person with SCI_01).
It was decided that this could be achieved by providing concrete examples and anecdotes from people with SCI illustrating that a meaningful, healthy life is possible, and that good health is the bedrock of a good life with SCI.
There was universal agreement that the SCI-HMT is aspirational and that it has the potential to improve knowledge and understanding for people with SCI, their families, community workers/carers and primary healthcare professionals, as a GP remarked:
“[different groups] could just read it and realize, ‘Ahh, OK that’s what that means… when you’re doing catheters. That’s what you mean when you’re talking about bladder and bowel function or skin care” (healthcare professional_04).
Despite the SCI-HMT providing an abundance of information and resources to support self-management, participants identified four gaps: (i) the priority issue of sexuality, including pleasure and identity, as one person with SCI remarked:
“ sexuality is one of the biggest issues that people with SCI often might not speak about that often cause you know it’s awkward for them. So yeah, I think that’s a that’s a serious issue” (person with SCI_03).
(ii) consideration of the taboo nature of bladder and bowel topics for indigenous people, (iii) urgent need to ensure links for SCI-HMT care plans are compatible with patient management systems, and (iv) exercise and leisure as a standalone topic taking account of effects of physical activity, including impact on mental health and wellbeing but more especially for fun.
To ensure longevity of the SCI-HMT, maintaining a partnership between people with SCI, SCI community groups and both primary and tertiary health services is required for liaison with the relevant professional bodies, care agencies, funders, policy makers and tertiary care settings to ensure ongoing education and promotion of SCI-HMT is maintained. For example, delivery of ongoing training of healthcare professionals to both increase the knowledge base of primary healthcare providers in relation to SCI, and to promote use of the tools and resources through health communities. As a community nurse specialist suggested:
“ improving knowledge in the health community… would require digital links to clinical/health management platforms” (healthcare professional_02).
In a similar vein, a GP suggested:
“ our common GP body would have continuing education requirements… especially if it’s online, in particular for the rural, rural doctors who you know, might find it hard to get into the city” (healthcare professional_04).
The successful implementation of evidence-based innovations into practice is dependent on a wide array of dynamic and active contextual factors, including the perspectives of the recipients who are destined to use such innovations. Indeed, the recently updated CFIR has called for innovation recipient perspectives to be a priority when considering contextual factors [ 10 , 11 ]. Understanding and including the perspectives of those the innovation is being designed to benefit can promote increased equity and validation of recipient populations, and potentially increase the adoption and sustainability of innovations.
In this paper, we have presented research using the recently updated CFIR to guide the collection of innovation recipients’ perspectives (including people with SCI and GPs working in the community) regarding the potential implementation barriers and facilitators of the digital version of the SCI-HMT. Collected data were synthesized to inform actionable findings– tangible ways in which the SCI-HMT could be modified according of the domains of the CFIR (e.g., see Keith et al. [ 20 ]). It is important to note that we conducted this research using the original domains of the CFIR [ 9 ] prior to Damschroder et al. publishing the updated CFIR [ 11 ]. However, in our analysis we were able to align our findings to the revised CFIR domains and constructs, as Damschroder [ 11 ] suggests, constructs can “be mapped back to the original CFIR to ensure longitudinal consistency” (p. 13).
One of the most poignant findings from our analyses was the need to ensure the content of the SCI-HMT balanced scientific evidence and clinical expertise with lived experience knowledge. This balance of clinical and experiential knowledge demonstrated genuine regard for lived experience knowledge, and created a more accessible, engaging, useable platform. For example, in the innovation and individual domains, the need to include lived experience quotes was immediately apparent once the perspective of people with SCI was included. It was highlighted that while the SCI-HMT will prove useful to many parties at various stages along the continuum of care following onset of SCI, there will be those individuals that are overwhelmed by the scale of the content. That said, the layering of information facilitated by the digitalized version is intended to provide an ease of navigation through the SCI-HMT and enable a far greater sense of control over personal health and wellbeing. Further, despite concerns regarding e-literacy the digitalized version of the SCI-HMT is seen as imperative for accessibility given the wide geographic diversity and recent COVID pandemic [ 21 ]. While there will be people who are challenged by the technology, the universally acceptable use of the internet is seen as less of a barrier than printed material.
The concept of partnership was also apparent within the data analysis focusing on the outer and inner setting domains. In the outer setting domain, our findings emphasized the importance of engaging with SCI community groups, as well as primary and tertiary care providers to maximize uptake at all points in time from the phase of subacute rehabilitation onwards. While the SCI-HMT is intended for use across the continuum of care from post-acute rehabilitation onwards, it may be that certain modules are more relevant at different times, and could serve as key resources during the hand over between acute care, inpatient rehabilitation and community reintegration.
Likewise, findings regarding the inner setting highlighted the necessity of a productive partnership between GPs and individuals with SCI to address the substantial demands of long-term self-management of health and well-being following SCI. Indeed, support is crucial, especially when self-management is the focus. This is particularly so in individuals living with complex disability following survival after illness or injury [ 22 ], where health literacy has been found to be a primary determinant of successful health and wellbeing outcomes [ 23 ]. For people with SCI, this tool potentially holds the most appeal when an individual is ready and has strong partnerships and supportive communication. This can enable potential red flags to be recognized earlier allowing timely intervention to avert health crises, promoting individual well-being, and reducing unnecessary demands on health services.
While the SCI-HMT is an educational tool and not meant to replace health services, findings suggest the current structure would lead nicely to having the conversation with a range of likely support people, including SCI peers, friends and family, GP, community nurses, carers or via on-line support services. The findings within the process domain underscored the importance of ongoing partnership between innovation implementers and a broad array of innovation recipients (e.g., individuals with SCI, healthcare professionals, family, funding agencies and policy-makers). This emphasis on partnership also addresses recent discussions regarding equity and the CFIR. For example, Damschroder et al. [ 11 ] suggests that innovation recipients are too often not included in the CFIR process, as the CFIR is primarily seen as a tool intended “to collect data from individuals who have power and/or influence over implementation outcomes” (p. 5).
Finally, we feel that our inclusion of innovation recipients’ perspectives presented in this article begins to address the notion of equity in implementation, whereby the inclusion of recipient perspectives in research using the CFIR both validates, and increases, the likelihood of sustainable adoption of evidence-based innovations, such as the SCI-HMT. We have used the CFIR in a pragmatic way with an emphasis on meaningful engagement between the innovation recipients and the research team, heeding the call from Damschroder et al. [ 11 ], who recently argued for researchers to use the CFIR to collect data from innovation recipients. Adopting this approach enabled us to give voice to innovation recipient perspectives and subsequently ensure that the tone, scope, content and presentation of the SCI-HMT balanced the needs of innovation recipients alongside the provision of evidence-based clinical information.
Our research is not without limitations. While our study was successful in identifying a number of potential barriers and facilitators to the implementation of the SCI-HMT, we did not test any implementation strategies to impact determinants, mechanisms, or outcomes. This will be the focus of future research on this project, which will investigate the impact of implementation strategies on outcomes. Focus will be given to the context-mechanism configurations which give rise to particular outcomes for different groups in certain circumstances [ 7 , 24 ]. A second potential concern is the relatively small sample size of participants that may not allow for saturation and generalizability of the findings. However, both the significant impact of secondary health complications for people with SCI and the desire for a health maintenance tool have been established in Australia [ 2 , 4 ]. The aim our study reported in this article was to achieve context-specific knowledge of a small sample that shares a particular mutual experience and represents a perspective, rather than a population [ 25 , 26 ]. We feel our findings can stimulate discussion and debate regarding participant-informed approaches to implementation of the SCI-HMT, which can then be subject to larger-sample studies to determine their generalisability, that is, their external validity. Notably, future research could examine the interaction between certain demographic differences (e.g., gender) of people with SCI and potential barriers and facilitators to the implementation of the SCI-HMT. Future research could also include the perspectives of other allied health professionals working in the community, such as occupational therapists. Lastly, while our research gave significant priority to recipient viewpoints, research in this space would benefit for ensuring innovation recipients are engaged as genuine partners throughout the entire research process from conceptualization to implementation.
Employing the CFIR provided an effective, systematic method for identifying recipient perspectives regarding the implementation of a digital health maintenance tool for people living with SCI. Findings emphasized the need to balance clinical and lived experience perspectives when designing an implementation strategy and facilitating strong partnerships with necessary stakeholders to maximise the uptake of SCI-HMT into practice. Ongoing testing will monitor the uptake and implementation of this innovation, specifically focusing on how the SCI-HMT works for different users, in different contexts, at different stages and times of the rehabilitation journey.
Data availability
The datasets supporting the conclusions of this article are available available upon request and with permission gained from the project Steering Committee.
Abbreviations
spinal cord injury
HMT-Spinal Cord Injury Health Maintenance Tool
Consolidated Framework for Implementation Research
Kirshblum S, Vernon WL. Spinal Cord Medicine, Third Edition. New York: Springer Publishing Company; 2018.
Middleton JW, Arora M, Kifley A, Clark J, Borg SJ, Tran Y, et al. Australian arm of the International spinal cord Injury (Aus-InSCI) Community Survey: 2. Understanding the lived experience in people with spinal cord injury. Spinal Cord. 2022;60(12):1069–79.
Article PubMed PubMed Central Google Scholar
Craig A, Nicholson Perry K, Guest R, Tran Y, Middleton J. Adjustment following chronic spinal cord injury: determining factors that contribute to social participation. Br J Health Psychol. 2015;20(4):807–23.
Article PubMed Google Scholar
Middleton JW, Arora M, Jerram KAS, Bourke J, McCormick M, O’Leary D, et al. Co-design of the Spinal Cord Injury Health Maintenance Tool to support Self-Management: a mixed-methods Approach. Top Spinal Cord Injury Rehabilitation. 2024;30(1):59–73.
Article Google Scholar
Middleton JW, Arora M, McCormick M, O’Leary D. Health maintenance Tool: how to stay healthy and well with a spinal cord injury. A tool for consumers by consumers. 1st ed. Sydney, NSW Australia: Royal Rehab and The University of Sydney; 2020.
Google Scholar
Nilsen P, Bernhardsson S. Context matters in implementation science: a scoping review of determinant frameworks that describe contextual determinants for implementation outcomes. BMC Health Serv Res. 2019;19(1):189.
Jagosh J. Realist synthesis for Public Health: building an Ontologically Deep understanding of how Programs Work, for whom, and in which contexts. Annu Rev Public Health. 2019;40(1):361–72.
Nilsen P. Making sense of implementation theories, models and frameworks. Implement Sci. 2015;10(1):53.
Damschroder LJ, Aron DC, Keith RE, Kirsh SR, Alexander JA, Lowery JC. Fostering implementation of health services research findings into practice: a consolidated framework for advancing implementation science. Implement Sci. 2009;4(1):50.
Damschroder LJ, Reardon CM, Opra Widerquist MA, Lowery JC. Conceptualizing outcomes for use with the Consolidated Framework for Implementation Research (CFIR): the CFIR outcomes Addendum. Implement Sci. 2022;17(1):7.
Damschroder LJ, Reardon CM, Widerquist MAO, Lowery JC. The updated Consolidated Framework for Implementation Research based on user feedback. Implement Sci. 2022;17(1):75.
Plamondon K, Ndumbe-Eyoh S, Shahram S. 2.2 Equity, Power, and Transformative Research Coproduction. Research Co-Production in Healthcare2022. p. 34–53.
Verville L, Cancelliere C, Connell G, Lee J, Munce S, Mior S, et al. Exploring clinicians’ experiences and perceptions of end-user roles in knowledge development: a qualitative study. BMC Health Serv Res. 2021;21(1):926.
Gainforth HL, Hoekstra F, McKay R, McBride CB, Sweet SN, Martin Ginis KA, et al. Integrated Knowledge Translation Guiding principles for conducting and Disseminating Spinal Cord Injury Research in Partnership. Arch Phys Med Rehabil. 2021;102(4):656–63.
Langley J, Knowles SE, Ward V. Conducting a Research Coproduction Project. Research Co-Production in Healthcare2022. p. 112– 28.
Braun V, Clarke V. One size fits all? What counts as quality practice in (reflexive) thematic analysis? Qualitative Research in Psychology. 2020:1–25.
Tong A, Sainsbury p, Craig J. Consolidated criteria for reporting qulaitative research (COREQ): a 32-item checklist for interviews and focus groups. Int J Qual Health Care. 2007;19(6):349–57.
Bengtsson M. How to plan and perform a qualitative study using content analysis. NursingPlus Open. 2016;2:8–14.
Braun V, Clarke V. Using thematic analysis in psychology. Qualitative Res Psychol. 2006;3(2):77–101.
Keith RE, Crosson JC, O’Malley AS, Cromp D, Taylor EF. Using the Consolidated Framework for Implementation Research (CFIR) to produce actionable findings: a rapid-cycle evaluation approach to improving implementation. Implement Science: IS. 2017;12(1):15.
Choukou M-A, Sanchez-Ramirez DC, Pol M, Uddin M, Monnin C, Syed-Abdul S. COVID-19 infodemic and digital health literacy in vulnerable populations: a scoping review. Digit HEALTH. 2022;8:20552076221076927.
PubMed PubMed Central Google Scholar
Daniels N. Just Health: Meeting Health needs fairly. Cambridge University Press; 2007. p. 397.
Parker SM, Stocks N, Nutbeam D, Thomas L, Denney-Wilson E, Zwar N, et al. Preventing chronic disease in patients with low health literacy using eHealth and teamwork in primary healthcare: protocol for a cluster randomised controlled trial. BMJ Open. 2018;8(6):e023239–e.
Salter KL, Kothari A. Using realist evaluation to open the black box of knowledge translation: a state-of-the-art review. Implement Sci. 2014;9(1):115.
Sebele-Mpofu FY. The Sampling Conundrum in qualitative research: can Saturation help alleviate the controversy and alleged subjectivity in Sampling? Int’l J Soc Sci Stud. 2021;9:11.
Malterud K, Siersma VD, Guassora AD. Sample size in qualitative interview studies: guided by Information Power. Qual Health Res. 2015;26(13):1753–60.
Download references
Acknowledgements
Authors of this study would like to thank all the consumers with SCI and healthcare professionals for their invaluable contribution to this project. Their participation and insights have been instrumental in shaping the development of the SCI-HMT. The team also acknowledges the support and guidance provided by the members of the Project Steering Committee, as well as the partner organisations, including NSW Agency for Clinical Innovation, and icare NSW. Author would also like to acknowledge the informant group with lived experience, whose perspectives have enriched our understanding and informed the development of SCI-HMT.
The SCI Wellness project was a collaborative project between John Walsh Centre for Rehabilitation Research at The University of Sydney and Royal Rehab. Both organizations provided in-kind support to the project. Additionally, the University of Sydney and Royal Rehab received research funding from Insurance and Care NSW (icare NSW) to undertake the SCI Wellness Project. icare NSW do not take direct responsibility for any of the following: study design, data collection, drafting of the manuscript, or decision to publish.
Author information
Authors and affiliations.
John Walsh Centre for Rehabilitation Research, Northern Sydney Local Health District, St Leonards, NSW, Australia
John A Bourke, K. Anne Sinnott Jerram, Mohit Arora, Ashley Craig & James W Middleton
The Kolling Institute, Faculty of Medicine and Health, The University of Sydney, Sydney, NSW, Australia
Burwood Academy Trust, Burwood Hospital, Christchurch, New Zealand
John A Bourke
Royal Rehab, Ryde, NSW, Australia
James W Middleton
State Spinal Cord Injury Service, NSW Agency for Clinical Innovation, St Leonards, NSW, Australia
You can also search for this author in PubMed Google Scholar
Contributions
Project conceptualization: KASJ, MA, JWM; project methodology: JWM, MA, KASJ, JAB; data collection: KASJ and MA; data analysis: KASJ, JAB, MA, JWM; writing—original draft preparation: JAB; writing—review and editing: JAB, KASJ, JWM, MA, AC; funding acquisition: JWM, MA. All authors contributed to the revision of the paper and approved the final submitted version.
Corresponding author
Correspondence to John A Bourke .
Ethics declarations
Ethics approval and consent to participate.
The first (RESP/18/212) and second phase (2019/ETH13961) of the project received ethical approval from The Northern Sydney Local Health District Human Research Ethics Committee. All participants provided informed, written consent. All data were to be retained for 7 years (23rd May 2030).
Consent for publication
Not applicable.
Competing interests
MA part salary (from Dec 2018 to Dec 2023), KASJ part salary (July 2021 to Dec 2023) and JAB part salary (Jan 2022 to Aug 2022) was paid from the grant monies. Other authors declare no conflicts of interest.
Additional information
Publisher’s note.
Springer Nature remains neutral with regard to jurisdictional claims in published maps and institutional affiliations.
Electronic supplementary material
Below is the link to the electronic supplementary material.
Supplementary Material 1
Rights and permissions.
Open Access This article is licensed under a Creative Commons Attribution 4.0 International License, which permits use, sharing, adaptation, distribution and reproduction in any medium or format, as long as you give appropriate credit to the original author(s) and the source, provide a link to the Creative Commons licence, and indicate if changes were made. The images or other third party material in this article are included in the article’s Creative Commons licence, unless indicated otherwise in a credit line to the material. If material is not included in the article’s Creative Commons licence and your intended use is not permitted by statutory regulation or exceeds the permitted use, you will need to obtain permission directly from the copyright holder. To view a copy of this licence, visit http://creativecommons.org/licenses/by/4.0/ . The Creative Commons Public Domain Dedication waiver ( http://creativecommons.org/publicdomain/zero/1.0/ ) applies to the data made available in this article, unless otherwise stated in a credit line to the data.
Reprints and permissions
About this article
Cite this article.
Bourke, J.A., Jerram, K.A.S., Arora, M. et al. Using the consolidated Framework for Implementation Research to integrate innovation recipients’ perspectives into the implementation of a digital version of the spinal cord injury health maintenance tool: a qualitative analysis. BMC Health Serv Res 24 , 390 (2024). https://doi.org/10.1186/s12913-024-10847-x
Download citation
Received : 14 August 2023
Accepted : 11 March 2024
Published : 28 March 2024
DOI : https://doi.org/10.1186/s12913-024-10847-x
Share this article
Anyone you share the following link with will be able to read this content:
Sorry, a shareable link is not currently available for this article.
Provided by the Springer Nature SharedIt content-sharing initiative
- Spinal Cord injury
- Self-management
- Innovation recipients
- Secondary health conditions
- Primary health care
- Evidence-based innovations
- Actionable findings
- Consolidated Framework for implementation research
BMC Health Services Research
ISSN: 1472-6963
- General enquiries: [email protected]
Help | Advanced Search
Computer Science > Computer Vision and Pattern Recognition
Title: mm1: methods, analysis & insights from multimodal llm pre-training.
Abstract: In this work, we discuss building performant Multimodal Large Language Models (MLLMs). In particular, we study the importance of various architecture components and data choices. Through careful and comprehensive ablations of the image encoder, the vision language connector, and various pre-training data choices, we identified several crucial design lessons. For example, we demonstrate that for large-scale multimodal pre-training using a careful mix of image-caption, interleaved image-text, and text-only data is crucial for achieving state-of-the-art (SOTA) few-shot results across multiple benchmarks, compared to other published pre-training results. Further, we show that the image encoder together with image resolution and the image token count has substantial impact, while the vision-language connector design is of comparatively negligible importance. By scaling up the presented recipe, we build MM1, a family of multimodal models up to 30B parameters, including both dense models and mixture-of-experts (MoE) variants, that are SOTA in pre-training metrics and achieve competitive performance after supervised fine-tuning on a range of established multimodal benchmarks. Thanks to large-scale pre-training, MM1 enjoys appealing properties such as enhanced in-context learning, and multi-image reasoning, enabling few-shot chain-of-thought prompting.
Submission history
Access paper:.
- Download PDF
- Other Formats
References & Citations
- Google Scholar
- Semantic Scholar
BibTeX formatted citation

Bibliographic and Citation Tools
Code, data and media associated with this article, recommenders and search tools.
- Institution
arXivLabs: experimental projects with community collaborators
arXivLabs is a framework that allows collaborators to develop and share new arXiv features directly on our website.
Both individuals and organizations that work with arXivLabs have embraced and accepted our values of openness, community, excellence, and user data privacy. arXiv is committed to these values and only works with partners that adhere to them.
Have an idea for a project that will add value for arXiv's community? Learn more about arXivLabs .
- Open access
- Published: 01 April 2024
Midwives’ lived experiences of caring for women with mobility disabilities during pregnancy, labour and puerperium in Eswatini: a qualitative study
- Annie M. Temane 1 ,
- Fortunate N. Magagula 2 &
- Anna G. W. Nolte 1
BMC Women's Health volume 24 , Article number: 207 ( 2024 ) Cite this article
Metrics details
Midwives encounter various difficulties while aiming to achieve excellence in providing maternity care to women with mobility disabilities. The study aimed to explore and describe midwives’ experiences of caring for women with mobility disabilities during pregnancy, labour and puerperium in Eswatini.
A qualitative, exploratory, descriptive, contextual research design with a phenomenological approach was followed. Twelve midwives working in maternal health facilities in the Hhohho and Manzini regions in Eswatini were interviewed. Purposive sampling was used to select midwives to participate in the research. In-depth phenomenological interviews were conducted, and Giorgi’s descriptive phenomenological method was used for data analysis.
Three themes emerged from the data analysis: midwives experienced physical and emotional strain in providing maternity care to women with mobility disabilities, they experienced frustration due to the lack of equipment to meet the needs of women with mobility disabilities, and they faced challenges in providing support and holistic care to women with mobility disabilities during pregnancy, labour and puerperium.
Conclusions
Midwives experienced challenges caring for women with mobility disabilities during pregnancy, labour and the puerperium in Eswatini. There is a need to develop and empower midwives with the knowledge and skill to implement guidelines and enact protocols. Moreover, equipment and infrastructure are required to facilitate support and holistic maternity care for women with mobility disabilities.
Peer Review reports
Globally, few studies have focused on midwives’ views of providing maternity care to women with mobility disabilities during pregnancy, labour and the puerperium [ 1 ]. In The Disabled World [ 2 ], the World Health Organisation (WHO) defines ‘disability’ as an umbrella term covering impairments, activity limitations, and participation restrictions. Furthermore, the WHO defines an ‘impairment’ as a problem in bodily function or structure; an ‘activity limitation’ as a difficulty encountered by an individual in executing a task or action; and ‘participation restriction’ as a problem experienced by an individual in various life situations [ 2 ]. In this study, mobility disabilities refer to an impairment in the functioning of the upper and lower extremities as experienced by women during pregnancy, labour and the puerperium.
Midwives, as frontline workers in the delivery of maternity care [ 3 ] responsible for the lives of the mother and the baby, are accountable for providing competent and holistic care for women during pregnancy, labour and puerperium. As part of healthcare provision, midwives play an important role in ensuring that every woman, including women with mobility disabilities, receives the best maternity care during pregnancy, labour and puerperium. Moridi et al. [ 4 ] state that women with mobility disabilities are entitled to feel safe, respected and well cared for by midwives, who must be sufficiently prepared to care for these women.
According to the Global Population Report, [ 5 ] more than one billion people have some form of disability. Eswatini is classified as a middle-income setting in the southern African region, measuring 17 000 square kilometres with a population of 1 093 238. Of the population, 76.2% reside in rural areas (833 472), and 23.8% (259 766) reside in urban areas [ 6 ]. The economy is largely agricultural as most industries manufacture agricultural products [ 7 ]. Of the Eswatini population, 146 554 (13%) live with disabilities, with most being women (87 258; 16%), 22,871 (14.1%) and 26,270 (14.3%) of them reside in the Hhohho and Manzini regions respectively [ 8 ]. 15% (125 545) of people with disabilities live in rural areas, and 85% of the disabled population is unemployed [ 8 ], which means most of these individuals are economically disadvantaged. Furthermore, according to the Eswatini Central Statistics Office, 8 26.5% of people with disabilities have a mobility (walking) disability, with 63.5% of these being women.
Midwives may encounter difficulties while aiming to achieve excellence in providing maternity care to women with mobility disabilities in what may be challenging circumstances [ 9 ]. The WHO [ 10 ] claims people with disabilities do not receive the health services they need and are thus likely to find healthcare providers have inadequate skills. Lawler et al. [ 11 ] argue that ineffective interactions and poor communication with women needing care, particularly among health professionals engaged in providing maternity services, limit these women’s opportunities to participate in decision-making processes during pregnancy, childbirth, and postpartum care. According to the University of Johannesburg, [ 12 ] the midwife, together with the mother, have to engage collaboratively in order to come up with opportunities to promote health while removing any challenges that could impede the achievement thereof.
Walsh-Gallagher et al. [ 13 ] postulate that healthcare professionals tend to view women with disabilities as liabilities and regard them as high risk; they often exclude them from the individualised plan of care, which leads to an increase in these women’s fears about their maternity care. These challenges frequently result in health disparities and prevent women with mobility disabilities from receiving optimal maternity care. By exploring midwives’ experiences of this phenomenon, guidelines for support can be developed to extend available knowledge on maternity care for women with mobility disabilities during pregnancy, labour and puerperium.
Study design
The aim of the study was to explore and describe midwives’ experiences of caring for women with mobility disabilities during pregnancy, labour and puerperium in the Hhohho and Manzini regions of Eswatini. A qualitative, [ 14 ] exploratory, [ 15 ] descriptive, [ 16 ] contextual [ 17 ] research design with a phenomenological approach [ 18 ] was applied for this study to gain insight and understanding of the research phenomenon [ 19 ]. The phenomenon under study was midwives’ lived experiences caring for women with mobility disabilities during pregnancy, labour and puerperium. The participants were approached face-to-face to participate in the study. The researchers followed the Consolidated Criteria for Reporting Qualitative Research (COREQ) to report on this qualitative study [ 20 ].
The setting for the study was the Hhohho and Manzini regions of Eswatini. The researcher collected data at the site where participants experienced the phenomenon, as emphasised by Yildiz, [ 21 ] within the context in which they were comfortable to be interviewed [ 22 ]. This setting included maternal health facilities in hospitals and public health units.
Population and sampling
The study’s population comprised midwives working in maternal health facilities in hospitals and public health units, that is, one referral hospital and one public health unit in the Hhohho region and two referral hospitals and one public health unit in the Manzini region of Eswatini. Purposive sampling was used to select midwives to participate in the study; [ 16 ] 12 midwives from both regions were included. The midwives were between the ages of 35 and 55, and all midwives were black in race and identified as females. The years of experience in the field ranged between 5 and 15 years. The criteria for inclusion were midwives who had provided maternity care to women with mobility disabilities during pregnancy, labour and puerperium for a period of not more than two to three years, willing to participate in the study. The sample size was determined by repetitions of key statements about the research phenomenon during data collection, termed data saturation [ 23 ]. None of the participants refused to participate in the study.
Table 1 summarises the participants’ demographic characteristics.
Data collection
In-depth phenomenological, face-to-face, individual interviews were conducted to collect data [ 17 ]. The researcher who was a Midwifery lecturer held a Master’s Degree in Maternal and Neonatal science at the time of the study requested approval from the Unit manager to seek permission from the midwives to take part in the study. The midwives were given an information letter which included objectives of the study and the reasons for conducting the study. After recruiting midwives and obtaining their written consent to participate in the study and permission to audio-record the interviews, the researcher set up appointments with them for the interviews, and the data collection process commenced. The central question posed to participants was: How was it for you to care for a woman with a mobility disability during pregnancy, labour and puerperium? A pilot of the tool was performed on the first participant who met the inclusion criteria and possessed the same characteristics as those of the study sample. The pre-testing question yielded positive results, the participant responded to the question asked and there was no need to rephrase it or further test it.
The interviews were conducted from March 2019 to July 2019 and lasted 30–45 min. The researcher conducted interviews until the data became redundant and repetitive, reflecting that saturation had been reached, in congruence with Fouché et al. [ 25 ] In addition, field notes were recorded in a notebook after each in-depth phenomenological interview. No repeat interviews were held. The researcher ensured bracketing by omitting any perceptions from her past experiences that were likely to influence her interpretation of the research findings.
Data analysis
Before data analysis commenced, data were organised in computer files after being transcribed and translated into narrative form. Data from each participant were coded and stored in the relevant file and kept in a safe place; only the researcher could access the information. Back-up copies were made of all the data, and the master copies were stored in a safe to which only the researcher had access.
Data collection and analysis occurred concurrently. The researcher was guided by Giorgi et al.’s [ 26 ] five-step method of data analysis. This entailed the researcher reading all the transcribed data and the entire ‘naïve description’ provided by the participants during the interviews. The demarcation of ‘meaning units’ within narratives followed. In addition, the researcher marked where meaning shifts occurred and transformed meaning units into descriptive expressions. The researcher laid out the general structure of midwives’ experiences. Moreover, an independent coder was provided with the raw data (after signing a confidentiality agreement) to analyse the findings. The researcher and independent coder analysed the data separately and met for a consensus discussion. Both agreed on all the units of analysis, with an inter-coder reliability of 100%.
Measures of trustworthiness
The research was informed by Guba and Lincoln’s [ 27 ] model in relation to credibility, transferability, dependability and confirmability. For credibility, the researcher ensured prolonged engagement in the field [ 28 ], peer debriefing, [ 29 ] member checking, and an external auditor was used [ 25 ]. The study was also presented at a national conference. Transferability refers to the ability to extend the findings of one’s study to comparable environments or participants, as stated by Pitney et al. [ 30 ] The researcher ensured the study’s transferability by providing a richly documented account and in-depth description of all aspects and processes of the study protocol. Data saturation also confirmed transferability [ 23 ]. Dependability is evident in a study when other researchers are able to follow the researcher’s decision trail [ 31 ]. The researcher ensured dependability by densely describing the research process in congruence with Fouché et al.’s [ 25 ] guidelines, so that other researchers can follow similar steps of the same research methodology. Confirmability occurs when the research is judged by the way in which the findings and conclusions achieve their aim and are not the result of the researcher’s prior assumptions and preconceptions [ 32 ]. The researcher ensured this by remaining true to the research process through reflexivity and not compromising the research process in any way [ 28 ]. In addition, the researcher engaged an independent coder and provided a chain of evidence of the entire research process to enable an audit. Therefore, all forms of collected data, including raw data, reflexive journals, [ 29 ] notes and transcriptions, were recorded.
Ethical clearance to conduct this study was obtained from the University of Johannesburg Faculty of Health Sciences Higher Degrees Committee (ref. no. HDC-01-50-2018), University of Johannesburg Faculty of Health Research Ethics Committee (ref. no. REC-01-82-2018), and the Eswatini National Health Research Review Board (ref. no. NHRRB982/2018). The researcher applied and adhered to the four principles to be considered when conducting research: autonomy, beneficence, non-maleficence and justice [ 33 ]. Autonomy was adhered to by affording the participants the right to choose to participate in the study and by signing a written informed consent form a week after it was given to them before the interviews commenced. Beneficence was ensured through doing good and doing no harm to participants by prioritising the participants’ interests above those of the researcher, and did not engage in any practice that jeopardised their rights. Non-maleficence was observed by eradicating any possible harmful risks in the study; the researcher ensured the safety of the participants by conducting interviews in a familiar, private environment where they felt free and safe from harm. Furthermore, justice was observed by treating all participants equally regardless of their biographical, social and economic status.
Three themes and categories emerged from the data analysis. Table 2 summarises the themes and categories of midwives’ lived experiences caring for women with mobility disabilities during pregnancy, labour and puerperium in Eswatini.
Theme 1: physical and emotional efforts required from midwives to provide maternity care to women with mobility disabilities
Category 1.1: midwives experienced that woman with mobility disabilities needed assistance getting onto the bed during labour and delivery.
According to the participants, caring for women with mobility disabilities weighed heavily on them physically as they were required to assist the women onto delivery beds, which were too high for the women to climb up on their own:
“The beds are too high, they need to be adjustable…unless you change her to another room, we only have one in the other room…but to be honest she delivered on the same high bed with the help…It’s uncomfortable even with me who is normal, how about someone who has a disability? Getting the woman onto the bed is also uncomfortable for us we end up having pain on our backs.” (M3) . “The challenge is that I couldn’t help her to climb on to the bed, because I needed someone to assist when she came for postnatal care as she was even carrying 3 babies, I didn’t know what to do…I eventually went out and asked for assistance from my colleague…” (M10) . “I believe that the equipment should accommodate the women with disability, however, ours is not accommodative to the women…there are no special delivery beds, specifically designed for them because in my opinion the beds have to be shorter so they can be able to get on to them easily…yes so that they can be able to climb on the beds” (M1) .
Category 1.2: midwives experienced challenges in manoeuvring women with mobility disabilities during labour
Midwives reported it was difficult to perform some procedures while progressing these women during labour and delivery. This situation called for some adjustment and improvisation on their part, and they were unsure if it was the right thing to do.
“Even though she was a bit uncomfortable and anxious because the leg was just straight and could not bend, I reassured her…She had to remove the artificial leg and remain with the stump. I placed her on the lithotomy position. With the other hand she had to hold on to the ankle of the normal foot, even though it was awkward and difficult to manoeuvre, she managed to deliver the baby.” (M1) . “Luckily for us, she didn’t sustain a tear and we were saved from suturing her cause we foresaw difficulties as how we could have done it as she couldn’t open her thighs well due to the disability…yes I had to get a partner to assist, since she couldn’t even open her thighs. She also couldn’t cooperate possibly because of the pain that is also more reason I asked for my colleague to assist.” (M6) . “…yes…let me make an example, in my case she had a fracture, even if the pelvis was gynaecoid, there were problems of finding the right position for her during delivery, when she had to push the baby out…” (M8) . “The one that I saw did not have one leg. She had come for her postnatal care. We assisted and her on the couch, with my colleague. Since she couldn’t keep her legs open, I asked my colleague to keep one of her legs open whilst I examined her.” (M12) .
Category 1.3: midwives experienced anxiety and the need to exercise patience when caring for women with mobility disabilities
The participants experienced an emotional and psychological burden when caring for women with mobility disabilities. They felt unqualified and foresaw difficulties that triggered anxiety, which led to them not knowing what to do and how to handle these women.
“It was during labour…the woman was limping the woman she was on crutches. The moment she came into the ward I am a human being I just felt sorry for her kutsi (as to) how is she going to take care of the baby, and the hand was somehow deformed.” (M3) . “At first its emotionally draining as an individual you cause you start sympathising…(other midwife chips in)…yes you even find yourself saying things just because you pity her, and in the process they get hurt.” (M6) . “It came as a shock and it was my first experience, it came as a shock as to how I was going to help her as even my experience was limited in that area.” (M7) . “As I was taking care of her it became necessary for me to put myself into her shoes and to bear with her considering her situation….When you see her for the first time you would pity her yet she is now used to it.” (M1) .
Theme 2: lack of equipment to meet the needs of women with mobility disabilities
Category 2.1: midwives reported a lack of special beds and infrastructure to meet the needs of women with mobility disabilities.
Midwives reported their frustration at the lack of sufficient equipment like special beds and examination tables, tailored for women with mobility disabilities. It was a challenge to provide maternity care for women without this equipment.
“I believe that the infrastructure and equipment should accommodate the women with mobility disability, however, ours is not accommodative to the women…Usually we don’t have the prenatal ward in the maternity, most women who come in the latent phase have to ambulate, or go to the waiting huts and come back when the labour pains are stronger…There are no special delivery beds, specifically designed for them because in my opinion the beds have to be shorter so they can be able to get on to them easily. We do not even have toilets meant for them.” (M1) . “I was anxious as to how was she going to push how to push cause we do not have the right beds when it was time for pushing I asked for assistance…” (M2) . “The challenge is that I couldn’t help her to climb on to the bed, because I needed someone to assist when she came for postnatal care…the beds need to be adjustable so that they are able to be pushed lower for the mother to move from wheel chair to the bed and we pull the bed up again to examine her.” (M11) .
Theme 3: challenges in providing holistic care to women with mobility disabilities during pregnancy, labour, and puerperium
Category 3.1: midwives reported a lack of guidelines and protocols in caring holistically for women with mobility disabilities.
Midwives emphasised a lack of guidelines, protocols and knowledge about caring holistically for women with mobility disabilities. This resulted in everyone making their own decisions and doing as they saw fit in caring for these women:
“I think during antenatal care they (the women with mobility disabilities) need to be prepared for labour cause for others the pain is extraordinary, apart from the pain threshold, they also face self-esteem issues, they are looked down upon…I only saw that she was disabled during assessment cause nothing was recorded on the antenatal care card.” (M2) . “I was not aware of the disability at first, I only discovered when she was pushing…she was admitted and progressed by another midwife, I only attended to her when she was pushing… there was nothing written on the nurse’s notes/ handover notes about her disability.” (M5) . “There is no normal practice for a woman with mobility disability when they come and they are in labour, I usually admit regardless of the stage of labour or dilatation…It is not a protocol, it’s a midwife’s prerogative.” (M1) . “We assess and come up with our own discretion even in terms of admitting them (women with mobility disability). Some midwives will admit them regardless of the stage of labour and disregard the protocol that women who come into labour have to ambulate if they are in the latent phase.” (M8) . “There is one that came the past 3 days she has 3 children now and we just scheduled her for c/section because we know that she has been having c/section since she started. Just from looking at the way she walked, we could tell that she couldn’t deliver normally.” (M9) .
Category 3.2: midwives experienced challenges in allowing significant others to support women with mobility disabilities during labour and delivery
Consequent to the challenges in providing holistic care to women with mobility disabilities, midwives experienced challenges in allowing significant others to support these women during labour and delivery.
“It can depend on the patients themselves, they should decide and we need to be flexible for it to happen…as you can see our labour room also has the issue of privacy…we would need to restructure cause we have beds for 5 or more women in labour room…and then bringing someone from outside could be tricky” (M6) . “Maybe…not sure though, that they can bring their relatives, but maybe, considering staffing limitation…also the issue of discrimination and privacy, they (the women with disabilities) might feel we discriminate against them because they are disabled we now treat them differently.” (M7) . “Maybe if she can (bring her relative) but that’s not necessary, because I can always ask my colleague to assist, unless there is no one…” (M12) .
Childbirth is a special experience that requires a personal connection between the midwife and the woman giving birth, characterised by successful communication and respect [ 34 ]. However, the themes identified in the study indicated that midwives experienced challenges caring for women with mobility disabilities during pregnancy, labour and puerperium based on their limited capacity and preparedness, and lack of protocols to care for these women. They also reported a lack of supportive equipment for women with mobility disabilities. This posed a challenge for them in attending to these women’s specific needs, and they did not always know how to handle the situation appropriately.
One of the themes centred on midwives’ experiences of the physical and emotional efforts required of them to provide maternity care to women with mobility disabilities. They explained women with mobility disabilities required assistance getting onto the bed during labour and delivery, and more manoeuvring was expected of them (as midwives) as they had to adjust their performance and some procedures. The midwives also reported challenges in providing holistic care to women with mobility disabilities during pregnancy, labour and puerperium. Konig-Bachmann et al. [ 35 ] reiterate that caring for women with disabilities requires a level of flexibility, adaptation beyond routine procedures, and demands a high degree of improvisation from healthcare providers to ensure high-quality care. Morrison et al. [ 36 ] also found that healthcare providers reported difficulties with equipment when providing healthcare for women with physical disabilities; particularly the beds being too high for them to access. Smeltzer et al. [ 37 ] similarly allude to the importance of educating and training clinicians to equip them with knowledge and technical skills to provide more effective care to women with physical disabilities.
The midwives also shared that labour and deliveries were further complicated by some women with mobility disabilities not being able to cooperate due to the pain they experienced; others could not change position due to their disability. In a study by Sonalkar et al., [ 38 ] healthcare providers described the gynaecologic examination as challenging to complete as it required patience and the ability to be adaptable to different methods and positioning. Similarly, Konig-Bachmann et al. [ 35 ] indicate that in order to provide high-quality care for women with disabilities, healthcare providers need to exercise strong flexibility, adapt beyond routine procedures, and engage in a high degree of improvisation. Byrnes and Hickey [ 39 ] concur with this study’s findings and state that due to mobility restrictions, it may be difficult to assess the fundal height and foetal growth in women with physical disabilities.
Some midwives reported their caregiving role was emotionally draining as they felt sorry and pitied the women with mobility disabilities; thus, they needed to show compassion and reassure them. According to Mgwili et al., [ 40 ] psychoanalytic thinkers associate pity among staff members upon first contact with a physically disabled person as being instigated by personal feelings, stimulated by the disability. The midwives in this study stated they needed to be more patient and adjust their approach to caring for these women. Tarasoff [ 41 ] and Schildberger et al. [ 42 ] reiterated that healthcare providers seemed uncomfortable with women’s disability, consequently failing to offer needed support. According to Sonalkar et al., [ 38 ] healthcare providers reported there would be less fear and concern about hurting women with disabilities if midwives had increased training. Similarly, Mitra et al. [ 43 ] mentioned that healthcare providers had a general lack of confidence in their ability to provide adequate maternity care for women with physical disabilities.
Another theme was midwives’ challenges in providing competent and quality care for women with mobility disabilities due to a lack of equipment, including special beds and examination tables to meet these women’s needs. The examination, labour and delivery beds were too high and could not be adjusted for the women to get on by themselves, or even with the assistance of a midwife. In addition, the midwives reported there was no prenatal ward or waiting huts where they could place these women during the latent phase of labour. The midwives further emphasised there were no special toilets for women with mobility disabilities, which made it hazardous and difficult for them. Mitra et al. [ 43 ] concur on the barriers to providing maternity care to women with physical disabilities presented from health professionals’ perspectives. The authors indicated that participants from their study reported inaccessible equipment, including examination tables, as a barrier, making it more difficult and time-consuming to care for women with physical disabilities. In addition, Sonalkar et al. [ 38 ] said healthcare providers shared their concern about the lack of adjustable examination tables and transfer equipment, thus presenting a barrier to equitable care for women with disabilities.
Midwives further reported a lack of guidelines and protocols. This resulted in everyone making their own decisions and doing as they saw fit in caring for these women, and, in most instances, not recording the disability at all during antenatal care and admission into labour records. They often only discovered that the woman had a mobility disability at a later stage, when they were in labour. Sonalkar et al. [ 38 ] reported that healthcare providers felt frustrated and overwhelmed by the uncertainty of whether they made the correct decisions when caring for women with physical disabilities due to the lack of guidelines forcing them to use their own judgement. Mitra et al. [ 43 ] determined that most healthcare providers reported a lack of maternity practice guidelines for women with physical disabilities. Also, healthcare providers highlighted the importance of learning about disabilities and having a better understanding of a condition, particularly if it is likely to be exacerbated during pregnancy [ 44 ]. The need to make and read the notes on these women’s antenatal care cards or reports was emphasised.
Due to the lack of clear guidelines and protocols in caring for women with mobility disabilities, the midwives reported they sometimes admitted the woman into the labour ward regardless of the stage of labour, while other midwives did not and wanted them to walk around and come back for admission once they are in the active phase of labour. Furthermore, the midwives explained they often referred these women for caesarean sections right away, regardless of whether the woman could deliver normally due to mere panic from just seeing the disability or based on a previous record of surgery. Smeltzer et al. [ 45 ] researched obstetric clinicians’ experiences and educational preparation in caring for pregnant women with physical disabilities, and they agree on the lack of knowledge among health professionals caring for women with mobility disability.
Devkota et al. [ 46 ] also agree regarding midwives’ inefficiency in providing quality care for women with mobility disabilities. They claim healthcare providers often struggle to understand women with disabilities’ needs as they are not formally trained to provide services to this population. These healthcare providers were found to be undertrained in specific skills that would equip them to provide better and more targeted services for women with disabilities.
Consequent to the challenges in providing holistic care to women with mobility disabilities during pregnancy, labour and puerperium, midwives experienced challenges in allowing significant others to support these women. They reported that as much as they needed assistance caring for these women, and as much as the women would prefer to have their family members or significant others assisting them, this is not possible due to the lack of privacy, especially in public health facilities. Walsh-Gallager et al.’s [ 13 ] study on the ambiguity of disabled women’s experiences of pregnancy, childbirth and motherhood resonate with this study’s findings. The authors reported that women with disabilities’ partners were denied access or had their visits curtailed on several occasions due to inflexible hospital visiting policies. Redshaw et al. [ 47 ] reiterated the same in their study; disabled women were less likely to say their companion or partner was welcome to visit, let alone provide any form of assistance. In addition, a study by Bassoumah and Mohammad [ 48 ] reported that women with disabilities were denied their spouses’ support while receiving maternity care. Byrnes and Hickey [ 39 ] also concur that every effort should be made to allow women with disabilities who are in labour to receive support from significant others, and they should be active partners in the labour process.
Limitations
The study was limited to two of the four regions of Eswatini, namely Hhohho and Manzini; hence, the results could not be generalised for the whole country. The study also only focused on mobility disabilities due to time constraints and limited funds. Future research could be conducted to cover all other forms of disabilities.
This study focused on midwives’ lived experiences caring for women with mobility disabilities during pregnancy, labour and puerperium in Eswatini. In-depth phenomenological interviews were conducted, the findings were analysed, and themes were established. The findings illustrate that midwives experienced challenges caring for women with mobility disabilities during pregnancy, labour and puerperium in Eswatini. There is a need to develop and implement guidelines to empower midwives with knowledge and skill to provide support and holistic maternity care, and enact protocols. They should also have access to appropriate equipment and infrastructure specifically tailored towards promoting optimal health for women with mobility disabilities.
Data availability
The data analysed is available from the corresponding author upon reasonable request.
González-Timoneda A, Hernández Hernández V, Pardo Moya S, Alfaro Blazquez R. Experiences and attitudes of midwives during the birth of a pregnant woman with COVID-19 infection: a qualitative study. Women Birth. 2021;34(5):467.
Article Google Scholar
Disabled World. Definitions of disability [home page on the internet]. C2009 [updated 2021; cited 2023 July 26]. Available from: https://disabled-world.com/definitions/disability-definitions.php .
Aune I, Tysland T, Vollheim SA. Norwegian midwives’ experiences of relational continuity of midwifery care in the primary health care service: a qualitative descriptive study. Nordic J Nurs Res. 2021;4(1):5–13.
Moridi M, Pazandeh F, Hajian S, Potrata B. Midwives’ perspectives of respectful maternity care during childbirth: a qualitative study. PLoS ONE. 2020;15(3):1–12. https://doi.org/10.1371/journal.pone.0229941 .
Article CAS Google Scholar
United Nations. Background: International Day of Persons with Disabilities. [homepage on the internet]. c2022 [updated 2022 December 1; cited 2023 July 20]. Available from: https://un.org/en/observances/day-of-persons-with-disabilities/background .
Central Statistics Office. Population and housing census: 2017. Volume 3. Mbabane: Swaziland Government Printing Office; 2019a.
Google Scholar
Central Statistics Office. National accounts estimates. Mbabane: Swaziland Government Printing Office; 2018.
Central Statistics Office. Population and housing census: 2017. Volume 6. Mbabane: Swaziland Government Printing Office; 2019b.
Magqadiyane S. Experiences of midwives for caring un-booked pregnant mothers in a maternity unit at a district hospital in the Eastern Cape Province. Advances in reproductive sciences [serial online]. 2020. [cited 2021 August 5];8:186–200. https://doi.org/10.4236/arsci.2020.84016 .
World Health Organisation (WHO). Global report on health equity for persons with disabilities. [homepage on the internet]. c2022 [updated 2022 December 2; cited 2023 July 20]. Available from: https://who.int/health-topics/disability#tab=tab_1 .
Lawler D, Lalor J, Begley C. Access to maternity services for women with physical disability: a systematic review of literature. Int J Childbirth. 2013;3(4):203–17.
University of Johannesburg. Department of nursing paradigm. Johannesburg: University of Johannesburg;2017.
Walsh-Gallagher D, McConkey R, Sinclair M, Clarke R. Normalising birth for women with a disability: the challenges facing practitioners. Midwifery. 2013;29:294–9.
Article PubMed Google Scholar
Silverman D, editor. Qualitative research. 5th ed. Los Angeles: SAGE; 2021.
Nassaji H. Good qualitative research. Language Teaching Research [serial online]. 2020. [cited 2021 August 6];24(4):427–431. Available from: https://journals.sagepub.com/doi/pdf/10.1177/1362168820941288 .
Doyle L, McCabe C, Keogh B, Brady A, McCann M. An overview of the qualitative design within nursing research. Journal of Research in Nursing [serial online]. 2020. [cited 2021 August 6];25(5):444–446. Available from: https://journals.sagepub.com/doi/pdf/10.1177/1744987119880234 .
Hennink M, Hutter I, Bailey A. Qualitative research methods. 2nd ed. London: SAGE; 2020.
Frechette J, Bitzas V, Aubry M, Kilpatrick K, Lavoie-Tremblay M. Capturing lived experience: methodological considerations for interpretive phenomenological inquiry. Int J Qual Meth. 2022;19:2–11.
Flick U. The SAGE handbook of qualitative research design. London: SAGE; 2022.
Book Google Scholar
Tong A, Sainsbury P, Craig J. Consolidated criteria for reporting qualitative research (COREQ): a 32-item checklist for interviews and focus groups. Int J Qual Health Care. 2007;19(6):349–57. https://doi.org/10.1093/intqhc/mzm042 .
Yildiz A. A discussion on accurate and effective data collection for qualitative research. J Curr Researches Educational Stud. 2020;10(2):17–24.
Papakitsou V. Qualitative research: narrative approaches in sciences. Dialogues Clin Neurosci Mental Health. 2020;3(1):63–70.
Johnson JL, Adkins D, Chauvin S. Qualitative research in pharmacy education: a review of the quality indicators of rigor in qualitative research. Am J Pharm Educ. 2020;84(1):138–46.
Magagula T. The guidelines of maternity care of women with mobility disabilities in the Hhohho and Manzini regions: Eswatini [unpublished thesis]. University of Johannesburg, Johannesburg; 2021.
Fouché CB, Strydom H, Roestenburg WJH, editors. Research at grassroots for social sciences and human services professions. 5th ed. Pretoria: Van Schaik; 2021.
Giorgi A, Giorgi B, Morley J. The descriptive phenomenological psychological method. In: The SAGE handbook of qualitative research in psychology. 2nd edition. Los Angeles: SAGE; 2017.
Guba EG, Lincoln YS. Fourth generation evaluation. Newbury Park, CA: SAGE; 1989.
Rose J, Johnson W. Contextualising reliability and validity in qualitative research: toward more rigorous and trustworthy qualitative social science in leisure research. J Leisure Res. 2020;1:10–3. https://doi.org/10.1080/00222216.2020.1722042 .
Creswell JW, Creswell JD. Research Design: qualitative, quantitative and mixed methods approaches. 5th Ed. California: SAGE; 2018.
Pitney WA, Parker J, Singe SM, Potteiger K. Qualitative research in health professions. Thorofare: SLACK Incorporated; 2020.
Leavy P, editor. The Oxford handbook of qualitative research. New York: Oxford University Press; 2020.
Kyngäs H, Mikkonen K, Kääriäinen M, editors. The application of content analysis in nursing science research. 2020. [cited 2022 April 27]. Available from: https://dl1tarjomac.ir/nursing-ebooks/TPC202203.pdf .
Dhai A, McQuoid-Mason DJ. Bioethics, human rights and health law: principles and practice. Cape Town: Juta; 2020.
Hallam J, Howard C, Locke A, Thomas M. Communicating choice: an exploration of mothers’ experiences of birth. J Reprod Infant Psyc. 2016;34(2):175–84.
König-Bachmann M, Zenzmaier C, Schildberger B. Health professionals’ views on maternity care for women with physical disabilities: a qualitative study. BMC Health Serv Res. 2019;19(551):1–11.
Morrison J, Basnet M, Buthathoki B, et al. Disabled women’s maternal and newborn health care in rural Nepal: a qualitative study. Midwifery. 2014;30:1132–9.
Article PubMed PubMed Central Google Scholar
Smeltzer S, Wint A, Ecker J, Iezzoni L. Labor, delivery, and anaesthesia experiences of women with physical disability. Birth. 2017;44(4):315–24.
Sonalkar S, Chavez V, McClusky J, Hunter TA, Mollen CJ. Gynaecologic care for women with physical disabilities: a qualitative study of patients and providers. Women Health Iss. 2020;30(2):136–41.
Byrnes L, Hickey M. Perinatal care for women with disabilities: clinical considerations. J Nurse Practitioners. 2016;12(8):506–7.
Mgwili VN, Watermayer B. Physically disabled women and discrimination in reproductive health care: Psychoanalytic reflections. In: Disability and Social Change: A South African agenda [serial online]. 2006. [cited 2020 June 01]. Available from: https://www.hsrcpress.ac.za .
Tarasoff LA. Improving perinatal care for women with physical disabilities [Abstract]. J Obstet Gynaecol Can. 2016;38(5):501.
Schildberger B, Zenzmaier C, König-Bachmann M. Experiences of Austrian mothers with mobility or sensory impairments during pregnancy, childbirth and the puerperium: a qualitative study. BMC Pregnancy Childb. 2017;17(201):1–11.
Mitra M, Akobirshoev I, Moring N, et al. Access to and satisfaction with prenatal care among pregnant women with physical disabilities: findings from a national survey. J Womens Health. 2017;26(12):1356–63.
Hall J, Hundley V, Collins B, Ireland J. Dignity and respect during pregnancy and childbirth: a survey of experience of disabled women. BMC Pregnancy Childb. 2018;18(328):1–13.
Smeltzer S, Mitra M, Long-Bellil L, Iezzoni L, Smith L. Obstetric clinicians’ experiences and educational preparation for caring for pregnant women with physical disabilities: a qualitative study. Disabil Health J. 2018;11(1):8–13.
Devkota HR, Murray EA, Kett M, Groce N. Health care provider’s attitude towards disability and experience of women with disabilities in the use of maternal healthcare service in rural Nepal. Reprod Health. 2017;14(79):1–14.
Redshaw M, Malouf R, Gao H, Gray R. Women with disability: the experience of maternity care during pregnancy, labour and birth and the postnatal period. BMC Pregnancy Childb. 2013;13(174):1–14.
Bassoumah B, Mohammed A. The socio-cultural challenges to maternal and neonatal care: the views of women with disabilities receiving maternity care in the Chereponi district of Northern Ghana. Sci Afr. 2020;7:1–10.
Download references
Acknowledgements
The authors would like to acknowledge the midwives in the Hhohho and Manzini regions of Eswatini who participated in the study and provided their own experiences of providing maternity care to women with mobility disabilities during pregnancy, labour and puerperium.
The research received funding from the University of Johannesburg Postgraduate Supervisor-linked Bursary.
Author information
Authors and affiliations.
Health Sciences, University of Johannesburg, Johannesburg, South Africa
Annie M. Temane & Anna G. W. Nolte
Mother and Child Nursing, University of Eswatini, Kwaluseni, Eswatini
Fortunate N. Magagula
You can also search for this author in PubMed Google Scholar
Contributions
F.N.M conducted the research and wrote the manuscript. A.M.T supervised, reviewed, and finalised the manuscript. A.G.W.N co-supervised the study and edited the manuscript for final submission.
Corresponding author
Correspondence to Annie M. Temane .
Ethics declarations
Ethical approval and consent to participate.
Ethical clearance to conduct this study was obtained from the University of Johannesburg Faculty of Health Sciences Higher Degrees Committee (ref. no. HDC-01-50-2018), University of Johannesburg Faculty of Health Research Ethics Committee (ref. no. REC-01-82-2018) and the Eswatini National Health Research Review Board (ref. no. NHRRB982/2018). Participation in this study was voluntary, and informed consent was obtained from participants before the interviews commenced.
Consent for publication
Not applicable.
Competing interests
The authors declare no competing interests.
Additional information
Publisher’s note.
Springer Nature remains neutral with regard to jurisdictional claims in published maps and institutional affiliations.
Rights and permissions
Open Access This article is licensed under a Creative Commons Attribution 4.0 International License, which permits use, sharing, adaptation, distribution and reproduction in any medium or format, as long as you give appropriate credit to the original author(s) and the source, provide a link to the Creative Commons licence, and indicate if changes were made. The images or other third party material in this article are included in the article’s Creative Commons licence, unless indicated otherwise in a credit line to the material. If material is not included in the article’s Creative Commons licence and your intended use is not permitted by statutory regulation or exceeds the permitted use, you will need to obtain permission directly from the copyright holder. To view a copy of this licence, visit http://creativecommons.org/licenses/by/4.0/ . The Creative Commons Public Domain Dedication waiver ( http://creativecommons.org/publicdomain/zero/1.0/ ) applies to the data made available in this article, unless otherwise stated in a credit line to the data.
Reprints and permissions
About this article
Cite this article.
Temane, A.M., Magagula, F.N. & Nolte, A.G.W. Midwives’ lived experiences of caring for women with mobility disabilities during pregnancy, labour and puerperium in Eswatini: a qualitative study. BMC Women's Health 24 , 207 (2024). https://doi.org/10.1186/s12905-024-03032-z
Download citation
Received : 18 August 2023
Accepted : 18 March 2024
Published : 01 April 2024
DOI : https://doi.org/10.1186/s12905-024-03032-z
Share this article
Anyone you share the following link with will be able to read this content:
Sorry, a shareable link is not currently available for this article.
Provided by the Springer Nature SharedIt content-sharing initiative
- Experiences
- Maternity care
- Women with mobility disabilities
- Labour and the puerperium
BMC Women's Health
ISSN: 1472-6874
- Submission enquiries: [email protected]
- General enquiries: [email protected]
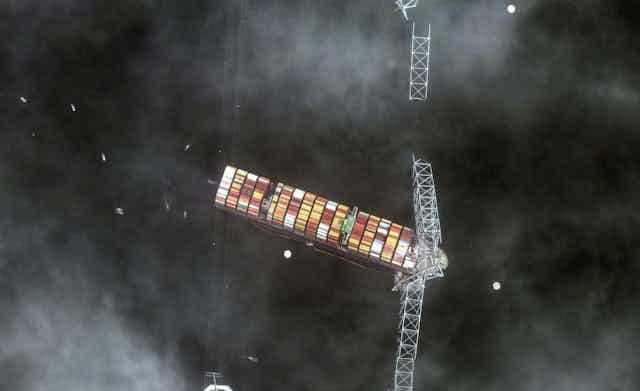
Baltimore bridge collapse: a bridge engineer explains what happened, and what needs to change
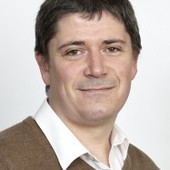
Associate Professor, Civil Engineering, Monash University
Disclosure statement
Colin Caprani receives funding from the Department of Transport (Victoria) and the Level Crossing Removal Project. He is also Chair of the Confidential Reporting Scheme for Safer Structures - Australasia, Chair of the Australian Regional Group of the Institution of Structural Engineers, and Australian National Delegate for the International Association for Bridge and Structural Engineering.
Monash University provides funding as a founding partner of The Conversation AU.
View all partners
When the container ship MV Dali, 300 metres long and massing around 100,000 tonnes, lost power and slammed into one of the support piers of the Francis Scott Key Bridge in Baltimore, the bridge collapsed in moments . Six people are presumed dead, several others injured, and the city and region are expecting a months-long logistical nightmare in the absence of a crucial transport link.
It was a shocking event, not only for the public but for bridge engineers like me. We work very hard to ensure bridges are safe, and overall the probability of being injured or worse in a bridge collapse remains even lower than the chance of being struck by lightning.
However, the images from Baltimore are a reminder that safety can’t be taken for granted. We need to remain vigilant.
So why did this bridge collapse? And, just as importantly, how might we make other bridges more safe against such collapse?
A 20th century bridge meets a 21st century ship
The Francis Scott Key Bridge was built through the mid 1970s and opened in 1977. The main structure over the navigation channel is a “continuous truss bridge” in three sections or spans.
The bridge rests on four supports, two of which sit each side of the navigable waterway. It is these two piers that are critical to protect against ship impacts.
And indeed, there were two layers of protection: a so-called “dolphin” structure made from concrete, and a fender. The dolphins are in the water about 100 metres upstream and downstream of the piers. They are intended to be sacrificed in the event of a wayward ship, absorbing its energy and being deformed in the process but keeping the ship from hitting the bridge itself.
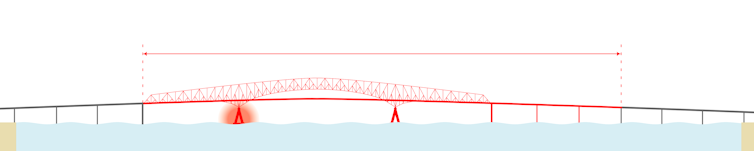
The fender is the last layer of protection. It is a structure made of timber and reinforced concrete placed around the main piers. Again, it is intended to absorb the energy of any impact.
Fenders are not intended to absorb impacts from very large vessels . And so when the MV Dali, weighing more than 100,000 tonnes, made it past the protective dolphins, it was simply far too massive for the fender to withstand.
Read more: I've captained ships into tight ports like Baltimore, and this is how captains like me work with harbor pilots to avoid deadly collisions
Video recordings show a cloud of dust appearing just before the bridge collapsed, which may well have been the fender disintegrating as it was crushed by the ship.
Once the massive ship had made it past both the dolphin and the fender, the pier – one of the bridge’s four main supports – was simply incapable of resisting the impact. Given the size of the vessel and its likely speed of around 8 knots (15 kilometres per hour), the impact force would have been around 20,000 tonnes .
Bridges are getting safer
This was not the first time a ship hit the Francis Scott Bridge. There was another collision in 1980 , damaging a fender badly enough that it had to be replaced.
Around the world, 35 major bridge collapses resulting in fatalities were caused by collisions between 1960 and 2015, according to a 2018 report from the World Association for Waterborne Transport Infrastructure. Collisions between ships and bridges in the 1970s and early 1980s led to a significant improvement in the design rules for protecting bridges from impact.
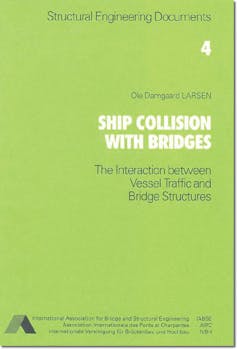
Further impacts in the 1970s and early 1980s instigated significant improvements in the design rules for impact.
The International Association for Bridge and Structural Engineering’s Ship Collision with Bridges guide, published in 1993, and the American Association of State Highway and Transporation Officials’ Guide Specification and Commentary for Vessel Collision Design of Highway Bridges (1991) changed how bridges were designed.
In Australia, the Australian Standard for Bridge Design (published in 2017) requires designers to think about the biggest vessel likely to come along in the next 100 years, and what would happen if it were heading for any bridge pier at full speed. Designers need to consider the result of both head-on collisions and side-on, glancing blows. As a result, many newer bridges protect their piers with entire human-made islands.
Of course, these improvements came too late to influence the design of the Francis Scott Key Bridge itself.
Lessons from disaster
So what are the lessons apparent at this early stage?
First, it’s clear the protection measures in place for this bridge were not enough to handle this ship impact. Today’s cargo ships are much bigger than those of the 1970s, and it seems likely the Francis Scott Key Bridge was not designed with a collision like this in mind.
So one lesson is that we need to consider how the vessels near our bridges are changing. This means we cannot just accept the structure as it was built, but ensure the protection measures around our bridges are evolving alongside the ships around them.
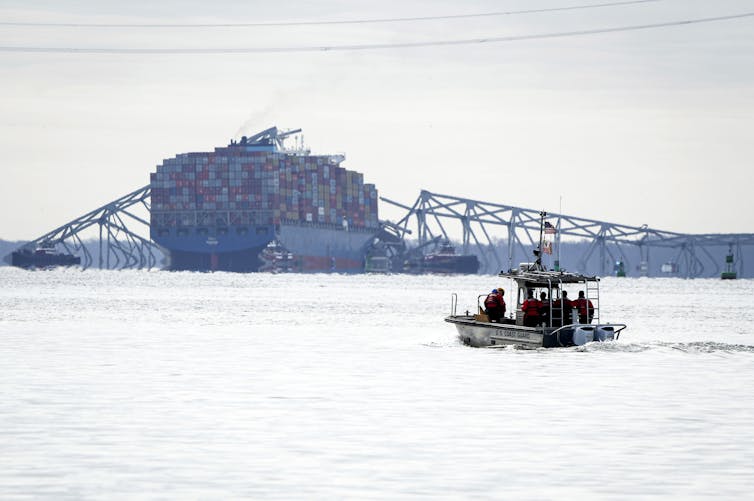
Second, and more generally, we must remain vigilant in managing our bridges. I’ve written previously about the current level of safety of Australian bridges, but also about how we can do better.
This tragic event only emphasises the need to spend more on maintaining our ageing infrastructure. This is the only way to ensure it remains safe and functional for the demands we put on it today.
- Engineering
- Infrastructure
- Urban infrastructure
- container ships
- Baltimore bridge collapse

School of Social Sciences – Public Policy and International Relations opportunities

School of Social Sciences – Human Geography opportunities

School of Social Sciences – Criminology opportunities

School of Social Sciences – Academic appointment opportunities

Lecturer (Hindi-Urdu)
You are using an outdated browser. Please upgrade your browser to improve your experience.
Apple AI research: ReALM is smaller, faster than GPT-4 when parsing contextual data
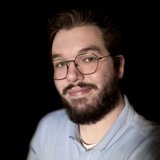
Apple is working to bring AI to Siri
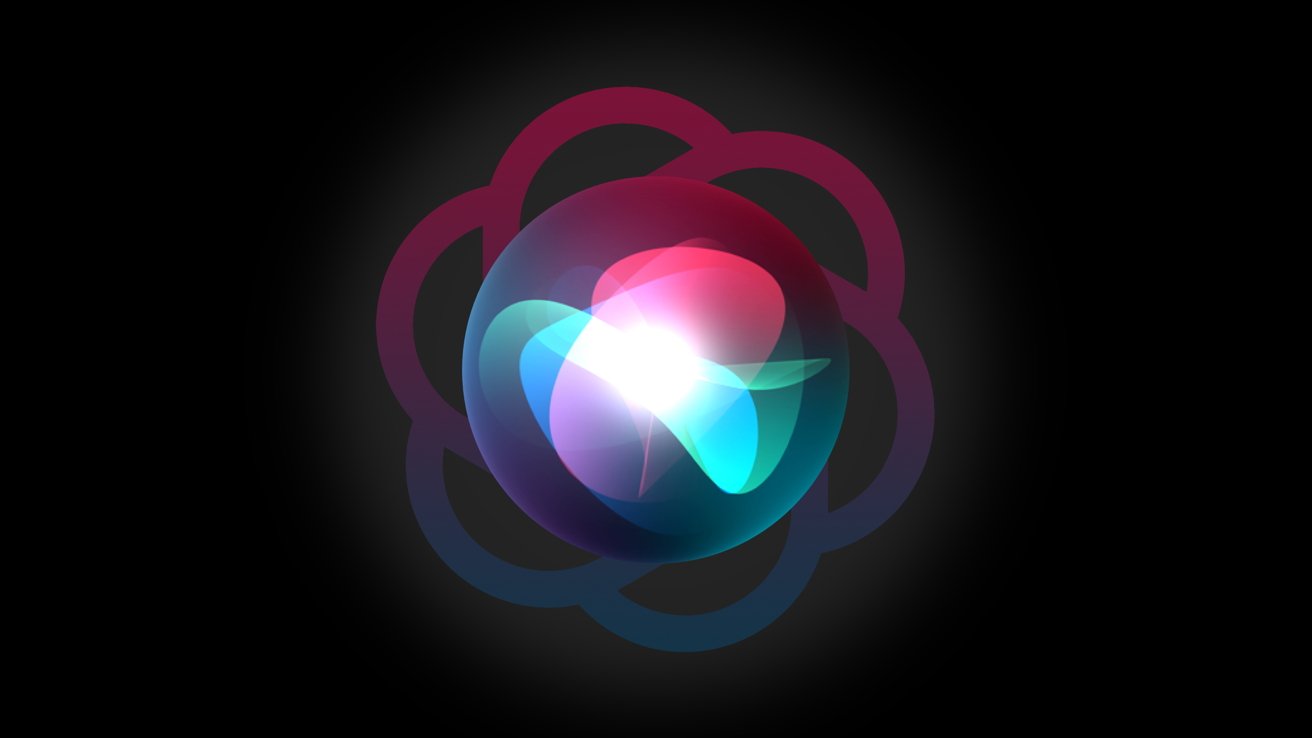
Artificial Intelligence research at Apple keeps being published as the company approaches a public launch of its AI initiatives in June during WWDC . There has been a variety of research published so far, including an image animation tool .
The latest paper was first shared by VentureBeat . The paper details something called ReALM — Reference Resolution As Language Modeling.
Having a computer program perform a task based on vague language inputs, like how a user might say "this" or "that," is called reference resolution. It's a complex issue to solve since computers can't interpret images the way humans can, but Apple may have found a streamlined resolution using LLMs.
When speaking to smart assistants like Siri , users might reference any number of contextual information to interact with, such as background tasks, on-display data, and other non-conversational entities. Traditional parsing methods rely on incredibly large models and reference materials like images, but Apple has streamlined the approach by converting everything to text.
Apple found that its smallest ReALM models performed similarly to GPT-4 with much fewer parameters, thus better suited for on-device use. Increasing the parameters used in ReALM made it substantially outperform GPT-4.
One reason for this performance boost is GPT-4's reliance on image parsing to understand on-screen information. Much of the image training data is built on natural imagery, not artificial code-based web pages filled with text, so direct OCR is less efficient.
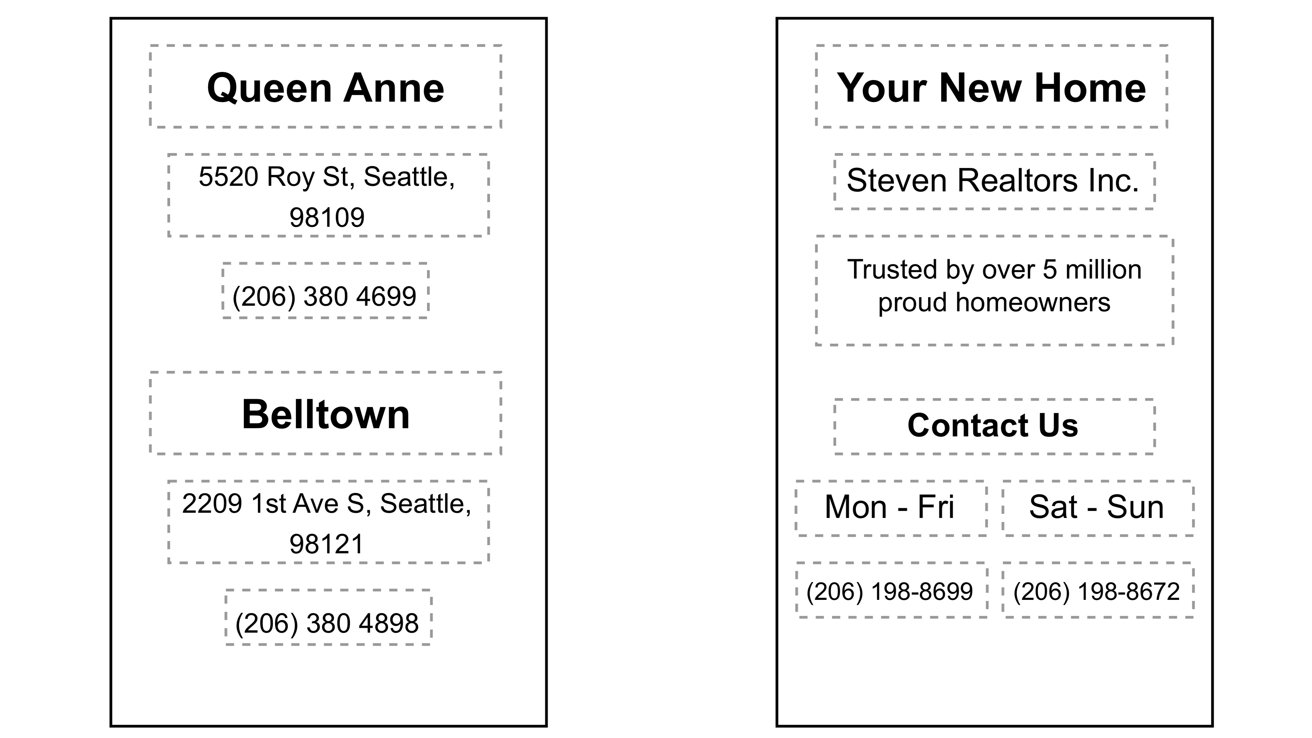
Converting an image into text allows ReALM to skip needing these advanced image recognition parameters, thus making it smaller and more efficient. Apple also avoids issues with hallucination by including the ability to constrain decoding or use simple post-processing.
For example, if you're scrolling a website and decide you'd like to call the business, simply saying "call the business" requires Siri to parse what you mean given the context. It would be able to "see" that there's a phone number on the page that is labeled as the business number and call it without further user prompt.
Apple is working to release a comprehensive AI strategy during WWDC 2024. Some rumors suggest the company will rely on smaller on-device models that preserve privacy and security, while licensing other company's LLMs for the more controversial off-device processing filled with ethical conundrums.
Top Stories
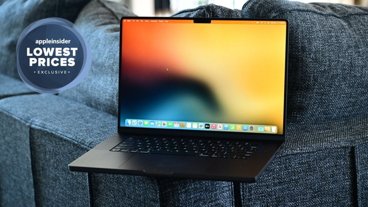
Save up to $350 on every Apple M3 MacBook Pro, plus get up to $80 off AppleCare
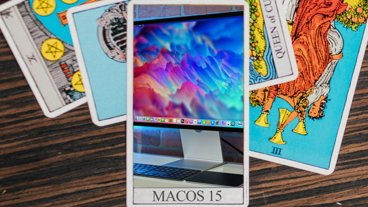
macOS 15 will get a big AI boost: what to expect at WWDC 2024
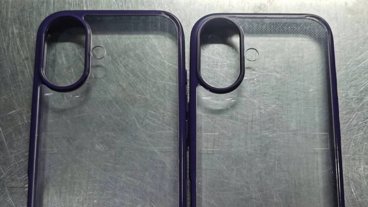
iPhone 16 case leak includes vertical camera bump at the back
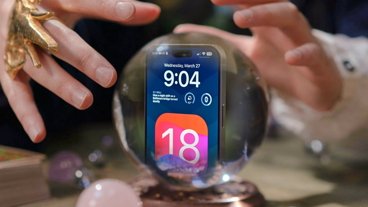
iOS 18 is coming soon with AI, a new interface, and accessibility: what to expect at WWDC 2024
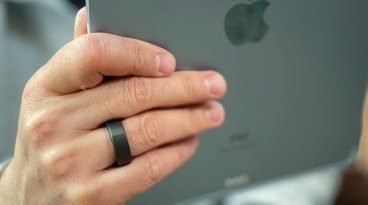
Apple Ring rumors & research - what you need to know about Apple's next wearable
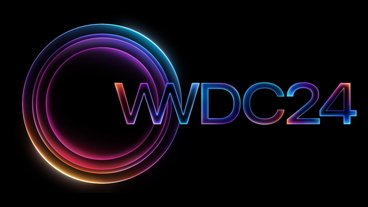
Apple AI, visionOS 2.0, iOS 18: What to expect from Apple during WWDC 2024 on June 10
Featured deals.
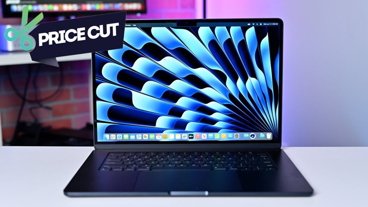
Blowout deals: Apple's MacBook Air up to $400 off, MacBook Pro starts at $899
Latest comparisons.
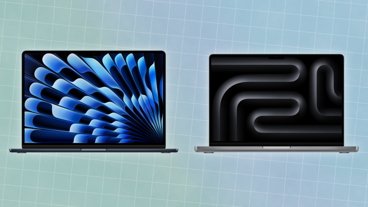
M3 15-inch MacBook Air vs M3 14-inch MacBook Pro — Ultimate buyer's guide
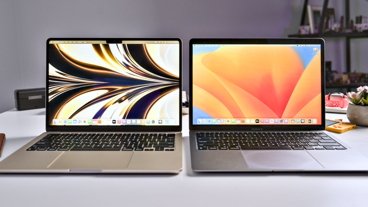
M3 MacBook Air vs M1 MacBook Air — Compared
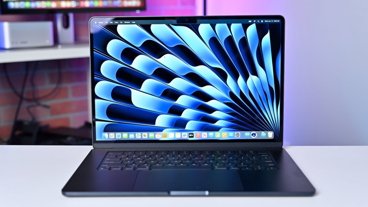
M3 MacBook Air vs M2 MacBook Air — Compared
Latest news.
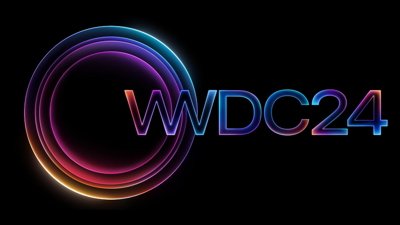
Apple will be holding WWDC 2024 from June 10, showing off its future operating system updates and maybe even new hardware. This is what has been rumored so far.
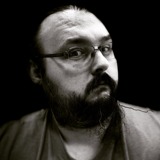
How to use Continuity Camera with Apple Music Sing on Apple TV 4K
You can use your iPhone or iPad to show yourself and friends on your Apple TV 4K performing karaoke with Apple Music Sing. Here's how.
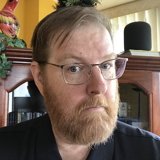
The Browser Company poaches Apple Safari design team member
The creators of Arc, a browser promising to browse for you with AI tools, have hired Charlie Deets, who was formerly one of two designers on the Apple Safari team.
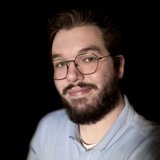
Apple Savings APY to decrease for first time on April 3
Apple Card customers taking advantage of the high-yield Apple Savings account will see their APY decrease for the first time from 4.5% to 4.4% on April 3.
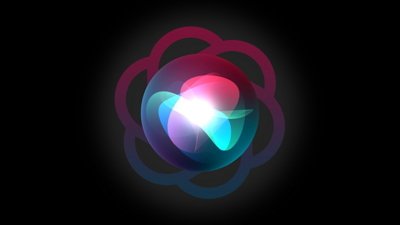
Apple AI research reveals a model that will make giving commands to Siri faster and more efficient by converting any given context into text, which is easier to parse by a Large Language Model.
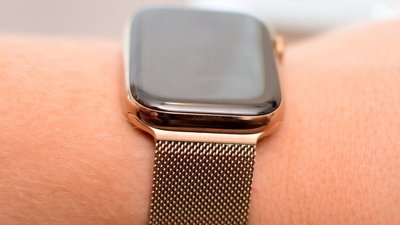
Apple staff offered Milanese Loop Apple Watch bands at 40% off
Apple has started to discount some of its Apple Watch bands for employees, but it is unclear if it is to prepare for changes with the next Apple Watch models.
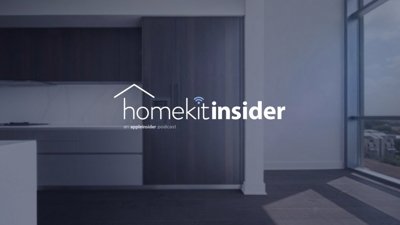
Migo Ascender stair-climbing vacuum, Qi2 gear, & TP-Link camera reviews on HomeKit Insider
On this episode of HomeKit Insider, Migo launches the Ascender stair-climbing robot, lots of Qi2 news, and HomeKit camera reviews.
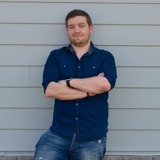
Blowout deals are in effect this April, with multiple MacBook Air configurations ranging from standard models to top-of-the-line specs up to $400 off. The M2 MacBook Pro also starts at $899 at Best Buy while supplies last.
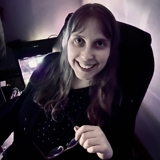
Apple has a new Swift tutorials webpage for budding developers
Apple has launched a new tutorial webpage featuring beginner resources for programming using Swift, Swift UI, and Xcode.
Latest Videos
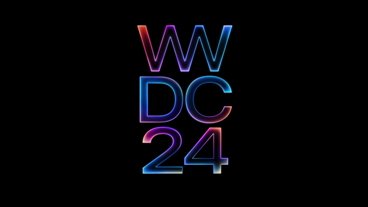
WWDC 2024 will show off Apple's AI efforts on June 10
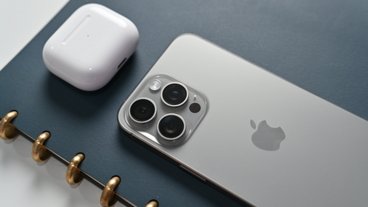
iPhone 15 Pro Max review six months later: Still an exciting upgrade
Latest reviews.
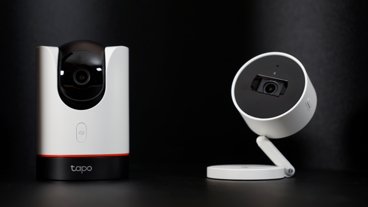
TP-Link Tapo Indoor cameras review: affordable HomeKit options with in-app AI tools
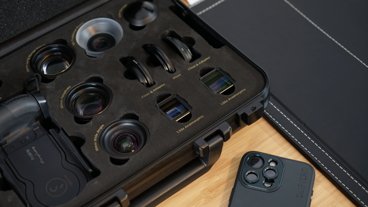
ShiftCam LensUltra Deluxe Kit review: Upgrade your iPhone photo shooting game
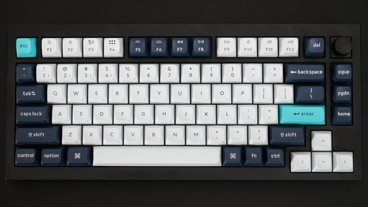
Keychron Q1 Max review: cushy, comfortable, costly

{{ title }}
{{ summary }}
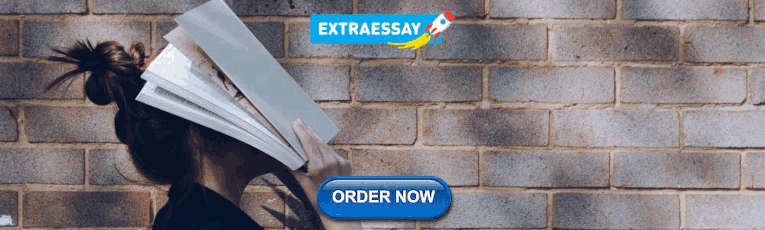
IMAGES
VIDEO
COMMENTS
Textual analysis is a broad term for various research methods used to describe, interpret and understand texts. All kinds of information can be gleaned from a text - from its literal meaning to the subtext, symbolism, assumptions, and values it reveals. The methods used to conduct textual analysis depend on the field and the aims of the ...
In all variants the research question plays the central role in this method: It provides the perspective for the textual work necessary at the beginning, that is, the intensive reading and study of the texts (Kuckartz 2016, p. 45). For qualitative methods, it is common for the individual analysis phases to be carried out on a circular basis.
Generally, textual analysis can be thought of as a three-step process that involves (i) collecting text data, (ii) preparing and cleaning text data, and (iii) analysing text data. During the collecting phase, the researcher collates the necessary text data on firms from one or more of a varied range of sources (e.g., corporate disclosures ...
Textual analysis is a research methodology that involves exploring written text as empirical data. Scholars explore both the content and structure of texts, and attempt to discern key themes and statistics emergent from them. This method of research is used in various academic disciplines, including cultural studies, literature, bilical studies ...
According to McKee (2003), textual analysis is an ongoing methodological process that involves gathering data, providing researchers with insights into the author's intended meaning within the ...
Textual analysis is a qualitative method used to examine content in media and popular culture, such as newspaper articles, television shows, websites, games, videos, and advertising. The method is linked closely to cultural studies. Based on semiotic and interpretive approaches, textual analysis is a type of qualitative analysis that focuses on ...
Textual Analysis. Textual analysis is the process of examining a text in order to understand its meaning. It can be used to analyze any type of text, including literature, poetry, speeches, and scientific papers. Textual analysis involves analyzing the structure, content, and style of a text. Textual analysis can be used to understand a text ...
'Methods' We Think and Work With: Methods as Explicit and Systematic Strategies to Solve Problems in Literary Studies 17 · Typologies of Methods and Approaches in Literary and Cultural Studies: A Schematic Overview 26 · Choosing and Using Methods in Literary Studies Wisely: On the Specificity of Scholarly Text Analysis and
Sentiment Analysis, also known as 'opinion mining', is the automated process of understanding the attributes of an opinion, that is, the emotions that underlie a text (e.g. positive, negative, and neutral). Sentiment analysis provides exciting opportunities in all kinds of fields.
Textual analysis is a way for researchers to gather information about how other human beings make sense of the world. It is a method-ology ± a data-gathering process ± for those researchers who want to understand the ways in which members of various cultures and subcultures make sense of who they are, and of how they ®t into the world in ...
Textual analysis is a method of examining and interpreting written, spoken, or visual texts to understand their underlying meanings, themes, and structures. It involves close scrutiny of language, style, and rhetorical devices to uncover the intended messages and implications within the text. This approach is commonly used in literary studies ...
Text analysis is a broad term that encompasses the examination and interpretation of textual data. It involves various techniques to understand, organize, and derive insights from text, including methods from linguistics, statistics, and machine learning. ... This video is an introduction to text mining and how it can be used in research. An ...
Qualitative researchers have developed a wide range of methods of analysis to make sense of textual data, one of the most common forms of data used in qualitative research (Attride-Stirling, 2001; Cho & Trent, 2006; Stenvoll & Svensson, 2011).As a result, qualitative text and discourse analysis (QTDA) has become a thriving methodological space characterized by the diversity of its approaches ...
Text and Textual Analysis. Jamie Baxter, in International Encyclopedia of Human Geography (Second Edition), 2020. Conclusion. Textual analysis is more than attaching themes to text; it is a mainstream form of qualitative research in Geography and the Social Sciences that provides deep, theoretically informed, conceptually organized, arguments for why the social world is organized in the way it is.
Textual analysis is a method of study utilized by researchers to examine messages as they appear through a variety of mediums. The data generated for textual analysis can come from documents, films, newspapers, paintings, web pages, and so forth. These forms of data serve as the "texts" under study and are used to assess the meanings ...
PDF | On Jan 1, 2015, N. Fairclough and others published Textual analysis | Find, read and cite all the research you need on ResearchGate
Textual Analysis - How to Engage in Textual Analysis. As a reader, a developing writer, and an informed student and citizen, you need to be able to locate, understand, and critically analyze others' purposes in communicating information. Being able to identify and articulate the meaning of other writers' arguments and theses enables you ...
`Alan McKee presents a student friendly introduction to the analysis of cultural texts. The book highlights the cultural differences in interpretation with an array of fascinating examples. Textual Analysis is written in an accessible style with several useful case studies.
Sentiment analysis is a method of analyzing text to determine whether the emotional tone or sentiment expressed in a piece of text is positive, negative, or neutral. Sentiment analysis is commonly used in businesses to gauge customer feedback, social media monitoring, and market research. Software for Sentiment Analysis.
Published by Alvin Nicolas at August 16th, 2021 , Revised On August 29, 2023. Textual analysis is the method of analysing and understanding the text. It includes the close reading of fictional texts, images symbols, and all forms of written literary texts. A researcher needs to look keenly at the text to identify the writer's context and its ...
10. Interviewing. 11. Creative Writing as a Research Method. 12. English Research Methods and the Digital Humanities. 9. Textual Analysis as a Research Method was published in Research Methods for English Studies on page 160.
Textual analysis plays various roles in accounting research: 52.7% of the textual analysis publications construct a variable directly from a document and use the variable as the dependent or explanatory variable for hypothesis testing, 29.9% extract targeted textual information (e.g., through keyword search or regular expressions) and construct ...
Research Letter. Competitor risk disclosure and firm M&A activities: evidence from textual analysis. Kezhi Liao a School of Management, Zhejiang University of Technology, ... The work was supported by the Key Projects of National Natural Science Foundation of Zhejiang Province [LQ20G020019].
Procedure. The procedure for this research is multi-stepped and is summarized in Fig. 1.First, we mapped retrospective qualitative data collected during the development of the SCI-HMT [] against the five domains of the CFIR in order to create a semi-structured interview guide (Step 1).Then, we used this interview guide to collect prospective data from health professionals and people with SCI ...
In this work, we discuss building performant Multimodal Large Language Models (MLLMs). In particular, we study the importance of various architecture components and data choices. Through careful and comprehensive ablations of the image encoder, the vision language connector, and various pre-training data choices, we identified several crucial design lessons. For example, we demonstrate that ...
Background Midwives encounter various difficulties while aiming to achieve excellence in providing maternity care to women with mobility disabilities. The study aimed to explore and describe midwives' experiences of caring for women with mobility disabilities during pregnancy, labour and puerperium in Eswatini. Methods A qualitative, exploratory, descriptive, contextual research design with ...
We work very hard to ensure bridges are safe, and overall the probability of being injured or worse in a bridge collapse remains even lower than the chance of being struck by lightning.
Apple AI research reveals a model that will make giving commands to Siri faster and more efficient by converting any given context into text, which is easier to parse by a Large Language Model.