Have a language expert improve your writing
Run a free plagiarism check in 10 minutes, generate accurate citations for free.
- Knowledge Base
Methodology
- Sampling Methods | Types, Techniques & Examples
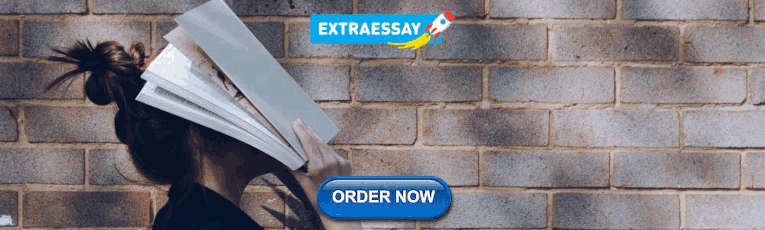
Sampling Methods | Types, Techniques & Examples
Published on September 19, 2019 by Shona McCombes . Revised on June 22, 2023.
When you conduct research about a group of people, it’s rarely possible to collect data from every person in that group. Instead, you select a sample . The sample is the group of individuals who will actually participate in the research.
To draw valid conclusions from your results, you have to carefully decide how you will select a sample that is representative of the group as a whole. This is called a sampling method . There are two primary types of sampling methods that you can use in your research:
- Probability sampling involves random selection, allowing you to make strong statistical inferences about the whole group.
- Non-probability sampling involves non-random selection based on convenience or other criteria, allowing you to easily collect data.
You should clearly explain how you selected your sample in the methodology section of your paper or thesis, as well as how you approached minimizing research bias in your work.
Table of contents
Population vs. sample, probability sampling methods, non-probability sampling methods, other interesting articles, frequently asked questions about sampling.
First, you need to understand the difference between a population and a sample , and identify the target population of your research.
- The population is the entire group that you want to draw conclusions about.
- The sample is the specific group of individuals that you will collect data from.
The population can be defined in terms of geographical location, age, income, or many other characteristics.
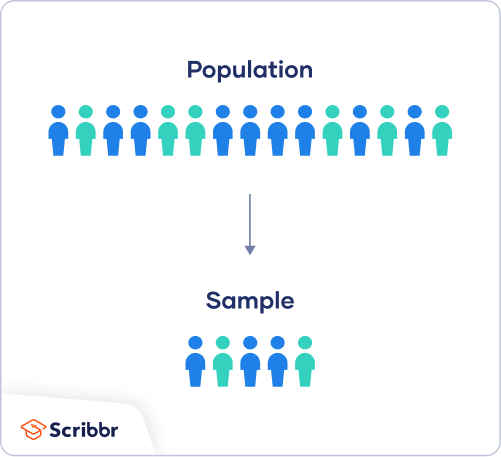
It is important to carefully define your target population according to the purpose and practicalities of your project.
If the population is very large, demographically mixed, and geographically dispersed, it might be difficult to gain access to a representative sample. A lack of a representative sample affects the validity of your results, and can lead to several research biases , particularly sampling bias .
Sampling frame
The sampling frame is the actual list of individuals that the sample will be drawn from. Ideally, it should include the entire target population (and nobody who is not part of that population).
Sample size
The number of individuals you should include in your sample depends on various factors, including the size and variability of the population and your research design. There are different sample size calculators and formulas depending on what you want to achieve with statistical analysis .
Prevent plagiarism. Run a free check.
Probability sampling means that every member of the population has a chance of being selected. It is mainly used in quantitative research . If you want to produce results that are representative of the whole population, probability sampling techniques are the most valid choice.
There are four main types of probability sample.
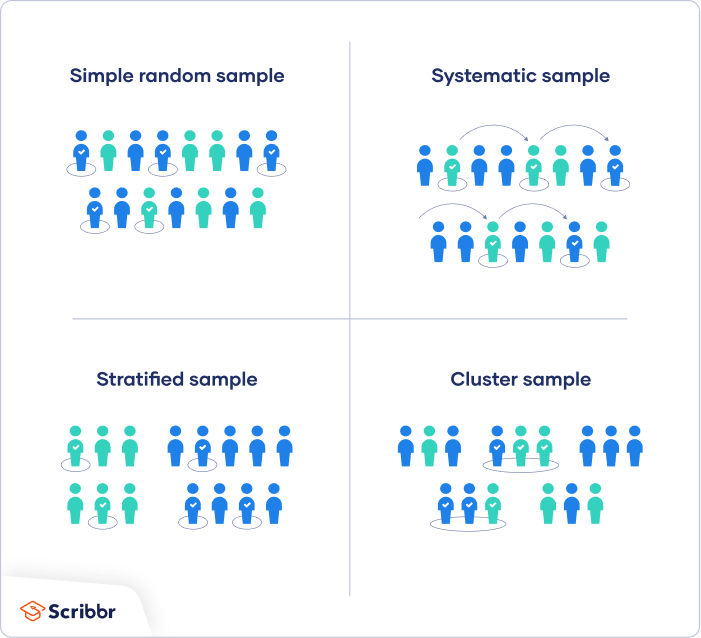
1. Simple random sampling
In a simple random sample, every member of the population has an equal chance of being selected. Your sampling frame should include the whole population.
To conduct this type of sampling, you can use tools like random number generators or other techniques that are based entirely on chance.
2. Systematic sampling
Systematic sampling is similar to simple random sampling, but it is usually slightly easier to conduct. Every member of the population is listed with a number, but instead of randomly generating numbers, individuals are chosen at regular intervals.
If you use this technique, it is important to make sure that there is no hidden pattern in the list that might skew the sample. For example, if the HR database groups employees by team, and team members are listed in order of seniority, there is a risk that your interval might skip over people in junior roles, resulting in a sample that is skewed towards senior employees.
3. Stratified sampling
Stratified sampling involves dividing the population into subpopulations that may differ in important ways. It allows you draw more precise conclusions by ensuring that every subgroup is properly represented in the sample.
To use this sampling method, you divide the population into subgroups (called strata) based on the relevant characteristic (e.g., gender identity, age range, income bracket, job role).
Based on the overall proportions of the population, you calculate how many people should be sampled from each subgroup. Then you use random or systematic sampling to select a sample from each subgroup.
4. Cluster sampling
Cluster sampling also involves dividing the population into subgroups, but each subgroup should have similar characteristics to the whole sample. Instead of sampling individuals from each subgroup, you randomly select entire subgroups.
If it is practically possible, you might include every individual from each sampled cluster. If the clusters themselves are large, you can also sample individuals from within each cluster using one of the techniques above. This is called multistage sampling .
This method is good for dealing with large and dispersed populations, but there is more risk of error in the sample, as there could be substantial differences between clusters. It’s difficult to guarantee that the sampled clusters are really representative of the whole population.
In a non-probability sample, individuals are selected based on non-random criteria, and not every individual has a chance of being included.
This type of sample is easier and cheaper to access, but it has a higher risk of sampling bias . That means the inferences you can make about the population are weaker than with probability samples, and your conclusions may be more limited. If you use a non-probability sample, you should still aim to make it as representative of the population as possible.
Non-probability sampling techniques are often used in exploratory and qualitative research . In these types of research, the aim is not to test a hypothesis about a broad population, but to develop an initial understanding of a small or under-researched population.
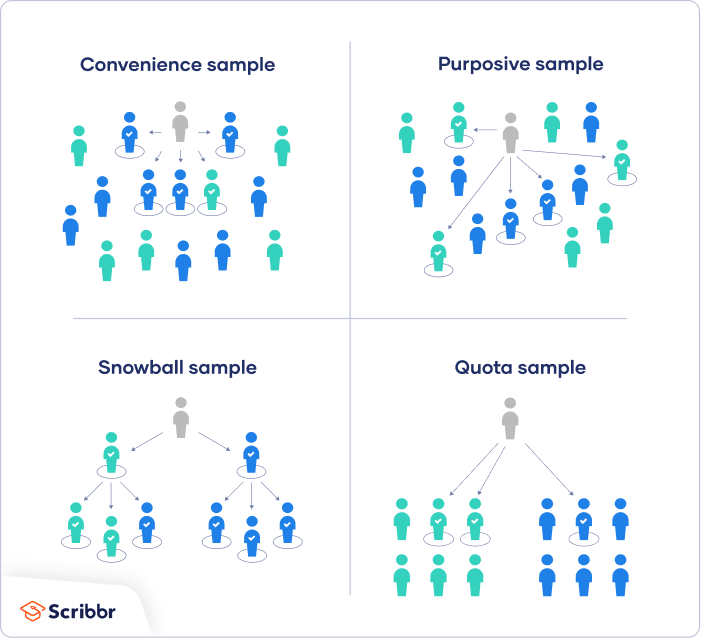
1. Convenience sampling
A convenience sample simply includes the individuals who happen to be most accessible to the researcher.
This is an easy and inexpensive way to gather initial data, but there is no way to tell if the sample is representative of the population, so it can’t produce generalizable results. Convenience samples are at risk for both sampling bias and selection bias .
2. Voluntary response sampling
Similar to a convenience sample, a voluntary response sample is mainly based on ease of access. Instead of the researcher choosing participants and directly contacting them, people volunteer themselves (e.g. by responding to a public online survey).
Voluntary response samples are always at least somewhat biased , as some people will inherently be more likely to volunteer than others, leading to self-selection bias .
3. Purposive sampling
This type of sampling, also known as judgement sampling, involves the researcher using their expertise to select a sample that is most useful to the purposes of the research.
It is often used in qualitative research , where the researcher wants to gain detailed knowledge about a specific phenomenon rather than make statistical inferences, or where the population is very small and specific. An effective purposive sample must have clear criteria and rationale for inclusion. Always make sure to describe your inclusion and exclusion criteria and beware of observer bias affecting your arguments.
4. Snowball sampling
If the population is hard to access, snowball sampling can be used to recruit participants via other participants. The number of people you have access to “snowballs” as you get in contact with more people. The downside here is also representativeness, as you have no way of knowing how representative your sample is due to the reliance on participants recruiting others. This can lead to sampling bias .
5. Quota sampling
Quota sampling relies on the non-random selection of a predetermined number or proportion of units. This is called a quota.
You first divide the population into mutually exclusive subgroups (called strata) and then recruit sample units until you reach your quota. These units share specific characteristics, determined by you prior to forming your strata. The aim of quota sampling is to control what or who makes up your sample.
If you want to know more about statistics , methodology , or research bias , make sure to check out some of our other articles with explanations and examples.
- Student’s t -distribution
- Normal distribution
- Null and Alternative Hypotheses
- Chi square tests
- Confidence interval
- Quartiles & Quantiles
- Cluster sampling
- Stratified sampling
- Data cleansing
- Reproducibility vs Replicability
- Peer review
- Prospective cohort study
Research bias
- Implicit bias
- Cognitive bias
- Placebo effect
- Hawthorne effect
- Hindsight bias
- Affect heuristic
- Social desirability bias
Receive feedback on language, structure, and formatting
Professional editors proofread and edit your paper by focusing on:
- Academic style
- Vague sentences
- Style consistency
See an example
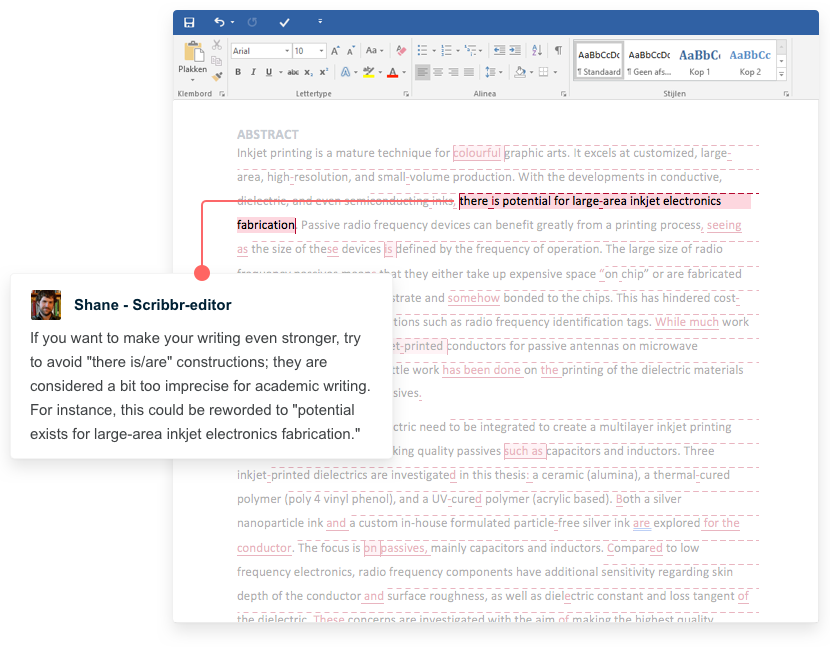
A sample is a subset of individuals from a larger population . Sampling means selecting the group that you will actually collect data from in your research. For example, if you are researching the opinions of students in your university, you could survey a sample of 100 students.
In statistics, sampling allows you to test a hypothesis about the characteristics of a population.
Samples are used to make inferences about populations . Samples are easier to collect data from because they are practical, cost-effective, convenient, and manageable.
Probability sampling means that every member of the target population has a known chance of being included in the sample.
Probability sampling methods include simple random sampling , systematic sampling , stratified sampling , and cluster sampling .
In non-probability sampling , the sample is selected based on non-random criteria, and not every member of the population has a chance of being included.
Common non-probability sampling methods include convenience sampling , voluntary response sampling, purposive sampling , snowball sampling, and quota sampling .
In multistage sampling , or multistage cluster sampling, you draw a sample from a population using smaller and smaller groups at each stage.
This method is often used to collect data from a large, geographically spread group of people in national surveys, for example. You take advantage of hierarchical groupings (e.g., from state to city to neighborhood) to create a sample that’s less expensive and time-consuming to collect data from.
Sampling bias occurs when some members of a population are systematically more likely to be selected in a sample than others.
Cite this Scribbr article
If you want to cite this source, you can copy and paste the citation or click the “Cite this Scribbr article” button to automatically add the citation to our free Citation Generator.
McCombes, S. (2023, June 22). Sampling Methods | Types, Techniques & Examples. Scribbr. Retrieved April 2, 2024, from https://www.scribbr.com/methodology/sampling-methods/
Is this article helpful?
Shona McCombes
Other students also liked, population vs. sample | definitions, differences & examples, simple random sampling | definition, steps & examples, sampling bias and how to avoid it | types & examples, "i thought ai proofreading was useless but..".
I've been using Scribbr for years now and I know it's a service that won't disappoint. It does a good job spotting mistakes”
Have a language expert improve your writing
Run a free plagiarism check in 10 minutes, automatically generate references for free.
- Knowledge Base
- Methodology
- Sampling Methods | Types, Techniques, & Examples
Sampling Methods | Types, Techniques, & Examples
Published on 3 May 2022 by Shona McCombes . Revised on 10 October 2022.
When you conduct research about a group of people, it’s rarely possible to collect data from every person in that group. Instead, you select a sample. The sample is the group of individuals who will actually participate in the research.
To draw valid conclusions from your results, you have to carefully decide how you will select a sample that is representative of the group as a whole. There are two types of sampling methods:
- Probability sampling involves random selection, allowing you to make strong statistical inferences about the whole group. It minimises the risk of selection bias .
- Non-probability sampling involves non-random selection based on convenience or other criteria, allowing you to easily collect data.
You should clearly explain how you selected your sample in the methodology section of your paper or thesis.
Table of contents
Population vs sample, probability sampling methods, non-probability sampling methods, frequently asked questions about sampling.
First, you need to understand the difference between a population and a sample , and identify the target population of your research.
- The population is the entire group that you want to draw conclusions about.
- The sample is the specific group of individuals that you will collect data from.
The population can be defined in terms of geographical location, age, income, and many other characteristics.
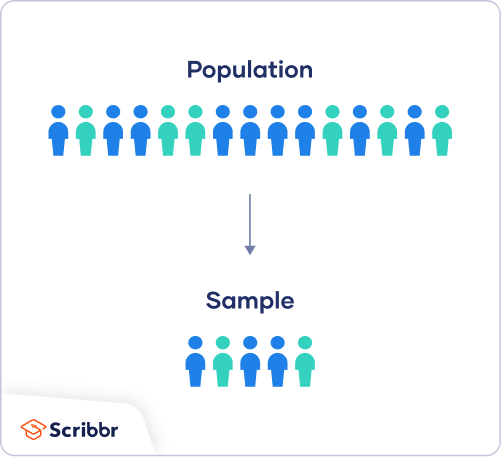
It is important to carefully define your target population according to the purpose and practicalities of your project.
If the population is very large, demographically mixed, and geographically dispersed, it might be difficult to gain access to a representative sample.
Sampling frame
The sampling frame is the actual list of individuals that the sample will be drawn from. Ideally, it should include the entire target population (and nobody who is not part of that population).
You are doing research on working conditions at Company X. Your population is all 1,000 employees of the company. Your sampling frame is the company’s HR database, which lists the names and contact details of every employee.
Sample size
The number of individuals you should include in your sample depends on various factors, including the size and variability of the population and your research design. There are different sample size calculators and formulas depending on what you want to achieve with statistical analysis .
Prevent plagiarism, run a free check.
Probability sampling means that every member of the population has a chance of being selected. It is mainly used in quantitative research . If you want to produce results that are representative of the whole population, probability sampling techniques are the most valid choice.
There are four main types of probability sample.
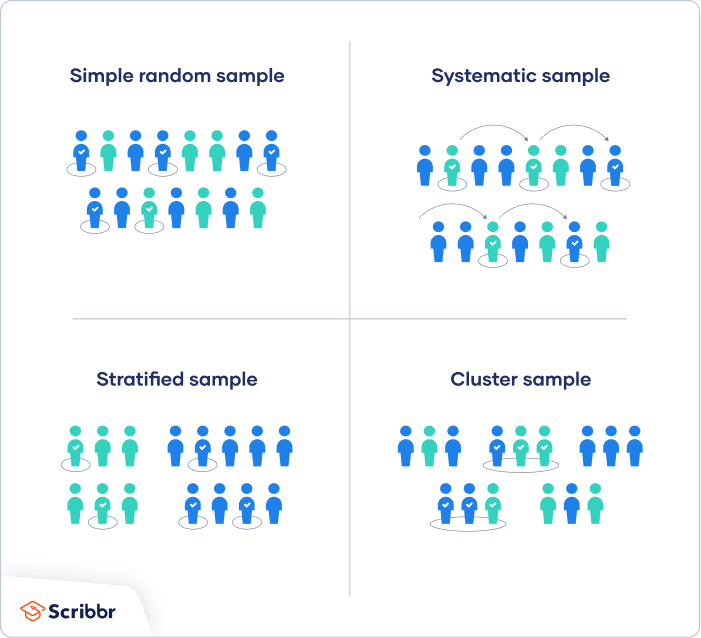
1. Simple random sampling
In a simple random sample , every member of the population has an equal chance of being selected. Your sampling frame should include the whole population.
To conduct this type of sampling, you can use tools like random number generators or other techniques that are based entirely on chance.
You want to select a simple random sample of 100 employees of Company X. You assign a number to every employee in the company database from 1 to 1000, and use a random number generator to select 100 numbers.
2. Systematic sampling
Systematic sampling is similar to simple random sampling, but it is usually slightly easier to conduct. Every member of the population is listed with a number, but instead of randomly generating numbers, individuals are chosen at regular intervals.
All employees of the company are listed in alphabetical order. From the first 10 numbers, you randomly select a starting point: number 6. From number 6 onwards, every 10th person on the list is selected (6, 16, 26, 36, and so on), and you end up with a sample of 100 people.
If you use this technique, it is important to make sure that there is no hidden pattern in the list that might skew the sample. For example, if the HR database groups employees by team, and team members are listed in order of seniority, there is a risk that your interval might skip over people in junior roles, resulting in a sample that is skewed towards senior employees.
3. Stratified sampling
Stratified sampling involves dividing the population into subpopulations that may differ in important ways. It allows you draw more precise conclusions by ensuring that every subgroup is properly represented in the sample.
To use this sampling method, you divide the population into subgroups (called strata) based on the relevant characteristic (e.g., gender, age range, income bracket, job role).
Based on the overall proportions of the population, you calculate how many people should be sampled from each subgroup. Then you use random or systematic sampling to select a sample from each subgroup.
The company has 800 female employees and 200 male employees. You want to ensure that the sample reflects the gender balance of the company, so you sort the population into two strata based on gender. Then you use random sampling on each group, selecting 80 women and 20 men, which gives you a representative sample of 100 people.
4. Cluster sampling
Cluster sampling also involves dividing the population into subgroups, but each subgroup should have similar characteristics to the whole sample. Instead of sampling individuals from each subgroup, you randomly select entire subgroups.
If it is practically possible, you might include every individual from each sampled cluster. If the clusters themselves are large, you can also sample individuals from within each cluster using one of the techniques above. This is called multistage sampling .
This method is good for dealing with large and dispersed populations, but there is more risk of error in the sample, as there could be substantial differences between clusters. It’s difficult to guarantee that the sampled clusters are really representative of the whole population.
The company has offices in 10 cities across the country (all with roughly the same number of employees in similar roles). You don’t have the capacity to travel to every office to collect your data, so you use random sampling to select 3 offices – these are your clusters.
In a non-probability sample , individuals are selected based on non-random criteria, and not every individual has a chance of being included.
This type of sample is easier and cheaper to access, but it has a higher risk of sampling bias . That means the inferences you can make about the population are weaker than with probability samples, and your conclusions may be more limited. If you use a non-probability sample, you should still aim to make it as representative of the population as possible.
Non-probability sampling techniques are often used in exploratory and qualitative research . In these types of research, the aim is not to test a hypothesis about a broad population, but to develop an initial understanding of a small or under-researched population.
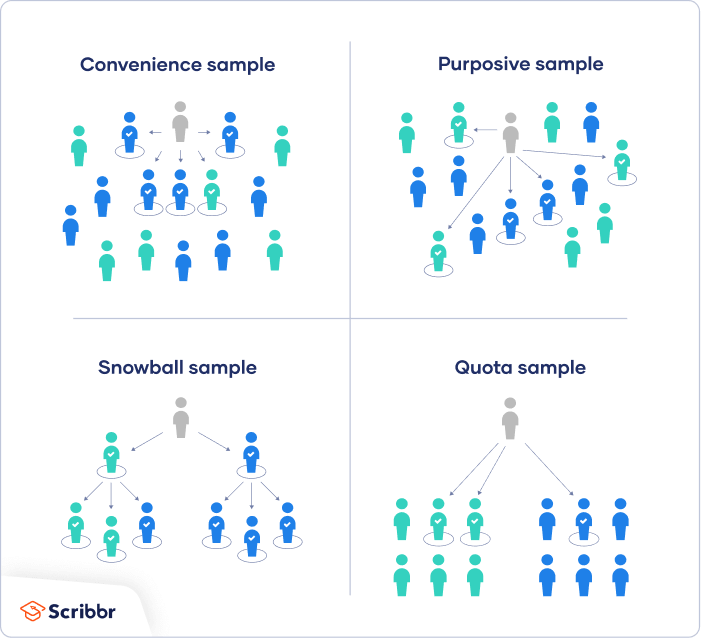
1. Convenience sampling
A convenience sample simply includes the individuals who happen to be most accessible to the researcher.
This is an easy and inexpensive way to gather initial data, but there is no way to tell if the sample is representative of the population, so it can’t produce generalisable results.
You are researching opinions about student support services in your university, so after each of your classes, you ask your fellow students to complete a survey on the topic. This is a convenient way to gather data, but as you only surveyed students taking the same classes as you at the same level, the sample is not representative of all the students at your university.
2. Voluntary response sampling
Similar to a convenience sample, a voluntary response sample is mainly based on ease of access. Instead of the researcher choosing participants and directly contacting them, people volunteer themselves (e.g., by responding to a public online survey).
Voluntary response samples are always at least somewhat biased, as some people will inherently be more likely to volunteer than others.
You send out the survey to all students at your university and many students decide to complete it. This can certainly give you some insight into the topic, but the people who responded are more likely to be those who have strong opinions about the student support services, so you can’t be sure that their opinions are representative of all students.
3. Purposive sampling
Purposive sampling , also known as judgement sampling, involves the researcher using their expertise to select a sample that is most useful to the purposes of the research.
It is often used in qualitative research , where the researcher wants to gain detailed knowledge about a specific phenomenon rather than make statistical inferences, or where the population is very small and specific. An effective purposive sample must have clear criteria and rationale for inclusion.
You want to know more about the opinions and experiences of students with a disability at your university, so you purposely select a number of students with different support needs in order to gather a varied range of data on their experiences with student services.
4. Snowball sampling
If the population is hard to access, snowball sampling can be used to recruit participants via other participants. The number of people you have access to ‘snowballs’ as you get in contact with more people.
You are researching experiences of homelessness in your city. Since there is no list of all homeless people in the city, probability sampling isn’t possible. You meet one person who agrees to participate in the research, and she puts you in contact with other homeless people she knows in the area.
A sample is a subset of individuals from a larger population. Sampling means selecting the group that you will actually collect data from in your research.
For example, if you are researching the opinions of students in your university, you could survey a sample of 100 students.
Statistical sampling allows you to test a hypothesis about the characteristics of a population. There are various sampling methods you can use to ensure that your sample is representative of the population as a whole.
Samples are used to make inferences about populations . Samples are easier to collect data from because they are practical, cost-effective, convenient, and manageable.
Probability sampling means that every member of the target population has a known chance of being included in the sample.
Probability sampling methods include simple random sampling , systematic sampling , stratified sampling , and cluster sampling .
In non-probability sampling , the sample is selected based on non-random criteria, and not every member of the population has a chance of being included.
Common non-probability sampling methods include convenience sampling , voluntary response sampling, purposive sampling , snowball sampling , and quota sampling .
Sampling bias occurs when some members of a population are systematically more likely to be selected in a sample than others.
Cite this Scribbr article
If you want to cite this source, you can copy and paste the citation or click the ‘Cite this Scribbr article’ button to automatically add the citation to our free Reference Generator.
McCombes, S. (2022, October 10). Sampling Methods | Types, Techniques, & Examples. Scribbr. Retrieved 2 April 2024, from https://www.scribbr.co.uk/research-methods/sampling/
Is this article helpful?
Shona McCombes
Other students also liked, what is quantitative research | definition & methods, a quick guide to experimental design | 5 steps & examples, controlled experiments | methods & examples of control.
Sampling Methods In Reseach: Types, Techniques, & Examples
Saul Mcleod, PhD
Editor-in-Chief for Simply Psychology
BSc (Hons) Psychology, MRes, PhD, University of Manchester
Saul Mcleod, PhD., is a qualified psychology teacher with over 18 years of experience in further and higher education. He has been published in peer-reviewed journals, including the Journal of Clinical Psychology.
Learn about our Editorial Process
Olivia Guy-Evans, MSc
Associate Editor for Simply Psychology
BSc (Hons) Psychology, MSc Psychology of Education
Olivia Guy-Evans is a writer and associate editor for Simply Psychology. She has previously worked in healthcare and educational sectors.
On This Page:
Sampling methods in psychology refer to strategies used to select a subset of individuals (a sample) from a larger population, to study and draw inferences about the entire population. Common methods include random sampling, stratified sampling, cluster sampling, and convenience sampling. Proper sampling ensures representative, generalizable, and valid research results.
- Sampling : the process of selecting a representative group from the population under study.
- Target population : the total group of individuals from which the sample might be drawn.
- Sample: a subset of individuals selected from a larger population for study or investigation. Those included in the sample are termed “participants.”
- Generalizability : the ability to apply research findings from a sample to the broader target population, contingent on the sample being representative of that population.
For instance, if the advert for volunteers is published in the New York Times, this limits how much the study’s findings can be generalized to the whole population, because NYT readers may not represent the entire population in certain respects (e.g., politically, socio-economically).
The Purpose of Sampling
We are interested in learning about large groups of people with something in common in psychological research. We call the group interested in studying our “target population.”
In some types of research, the target population might be as broad as all humans. Still, in other types of research, the target population might be a smaller group, such as teenagers, preschool children, or people who misuse drugs.
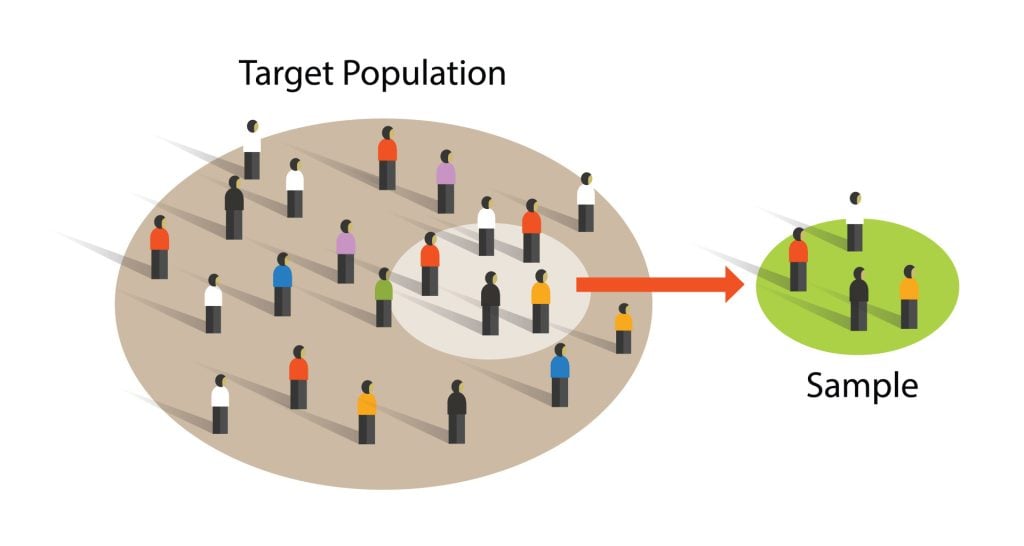
Studying every person in a target population is more or less impossible. Hence, psychologists select a sample or sub-group of the population that is likely to be representative of the target population we are interested in.
This is important because we want to generalize from the sample to the target population. The more representative the sample, the more confident the researcher can be that the results can be generalized to the target population.
One of the problems that can occur when selecting a sample from a target population is sampling bias. Sampling bias refers to situations where the sample does not reflect the characteristics of the target population.
Many psychology studies have a biased sample because they have used an opportunity sample that comprises university students as their participants (e.g., Asch ).
OK, so you’ve thought up this brilliant psychological study and designed it perfectly. But who will you try it out on, and how will you select your participants?
There are various sampling methods. The one chosen will depend on a number of factors (such as time, money, etc.).
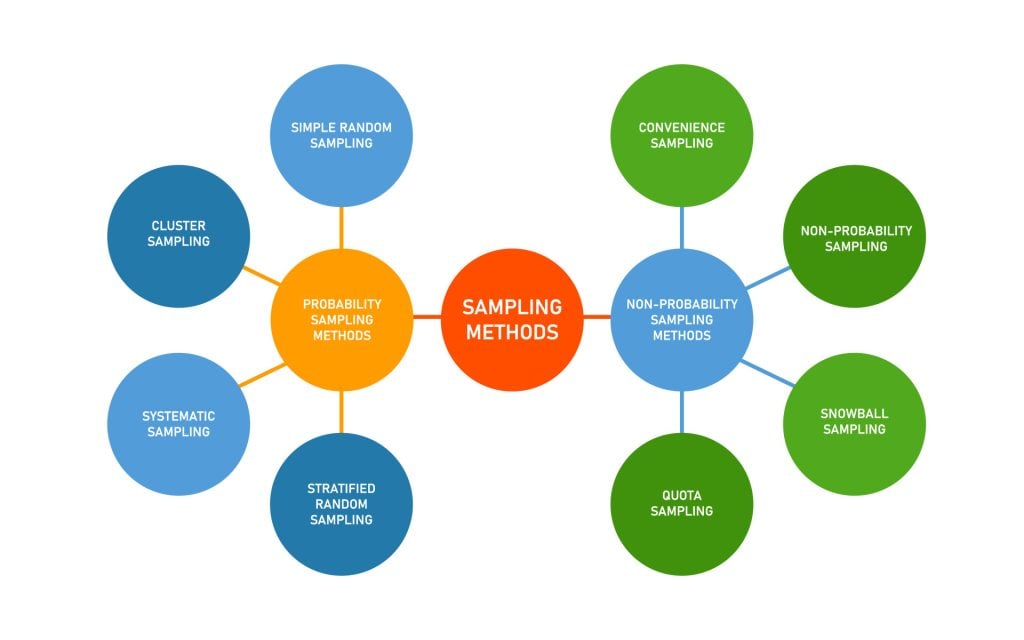
Random Sampling
Random sampling is a type of probability sampling where everyone in the entire target population has an equal chance of being selected.
This is similar to the national lottery. If the “population” is everyone who bought a lottery ticket, then everyone has an equal chance of winning the lottery (assuming they all have one ticket each).
Random samples require naming or numbering the target population and then using some raffle method to choose those to make up the sample. Random samples are the best method of selecting your sample from the population of interest.
- The advantages are that your sample should represent the target population and eliminate sampling bias.
- The disadvantage is that it is very difficult to achieve (i.e., time, effort, and money).
Stratified Sampling
During stratified sampling , the researcher identifies the different types of people that make up the target population and works out the proportions needed for the sample to be representative.
A list is made of each variable (e.g., IQ, gender, etc.) that might have an effect on the research. For example, if we are interested in the money spent on books by undergraduates, then the main subject studied may be an important variable.
For example, students studying English Literature may spend more money on books than engineering students, so if we use a large percentage of English students or engineering students, our results will not be accurate.
We have to determine the relative percentage of each group at a university, e.g., Engineering 10%, Social Sciences 15%, English 20%, Sciences 25%, Languages 10%, Law 5%, and Medicine 15%. The sample must then contain all these groups in the same proportion as the target population (university students).
- The disadvantage of stratified sampling is that gathering such a sample would be extremely time-consuming and difficult to do. This method is rarely used in Psychology.
- However, the advantage is that the sample should be highly representative of the target population, and therefore we can generalize from the results obtained.
Opportunity Sampling
Opportunity sampling is a method in which participants are chosen based on their ease of availability and proximity to the researcher, rather than using random or systematic criteria. It’s a type of convenience sampling .
An opportunity sample is obtained by asking members of the population of interest if they would participate in your research. An example would be selecting a sample of students from those coming out of the library.
- This is a quick and easy way of choosing participants (advantage)
- It may not provide a representative sample and could be biased (disadvantage).
Systematic Sampling
Systematic sampling is a method where every nth individual is selected from a list or sequence to form a sample, ensuring even and regular intervals between chosen subjects.
Participants are systematically selected (i.e., orderly/logical) from the target population, like every nth participant on a list of names.
To take a systematic sample, you list all the population members and then decide upon a sample you would like. By dividing the number of people in the population by the number of people you want in your sample, you get a number we will call n.
If you take every nth name, you will get a systematic sample of the correct size. If, for example, you wanted to sample 150 children from a school of 1,500, you would take every 10th name.
- The advantage of this method is that it should provide a representative sample.
Sample size
The sample size is a critical factor in determining the reliability and validity of a study’s findings. While increasing the sample size can enhance the generalizability of results, it’s also essential to balance practical considerations, such as resource constraints and diminishing returns from ever-larger samples.
Reliability and Validity
Reliability refers to the consistency and reproducibility of research findings across different occasions, researchers, or instruments. A small sample size may lead to inconsistent results due to increased susceptibility to random error or the influence of outliers. In contrast, a larger sample minimizes these errors, promoting more reliable results.
Validity pertains to the accuracy and truthfulness of research findings. For a study to be valid, it should accurately measure what it intends to do. A small, unrepresentative sample can compromise external validity, meaning the results don’t generalize well to the larger population. A larger sample captures more variability, ensuring that specific subgroups or anomalies don’t overly influence results.
Practical Considerations
Resource Constraints : Larger samples demand more time, money, and resources. Data collection becomes more extensive, data analysis more complex, and logistics more challenging.
Diminishing Returns : While increasing the sample size generally leads to improved accuracy and precision, there’s a point where adding more participants yields only marginal benefits. For instance, going from 50 to 500 participants might significantly boost a study’s robustness, but jumping from 10,000 to 10,500 might not offer a comparable advantage, especially considering the added costs.


Sampling Methods & Strategies 101
Everything you need to know (including examples)
By: Derek Jansen (MBA) | Expert Reviewed By: Kerryn Warren (PhD) | January 2023
If you’re new to research, sooner or later you’re bound to wander into the intimidating world of sampling methods and strategies. If you find yourself on this page, chances are you’re feeling a little overwhelmed or confused. Fear not – in this post we’ll unpack sampling in straightforward language , along with loads of examples .
Overview: Sampling Methods & Strategies
- What is sampling in a research context?
- The two overarching approaches
Simple random sampling
Stratified random sampling, cluster sampling, systematic sampling, purposive sampling, convenience sampling, snowball sampling.
- How to choose the right sampling method
What (exactly) is sampling?
At the simplest level, sampling (within a research context) is the process of selecting a subset of participants from a larger group . For example, if your research involved assessing US consumers’ perceptions about a particular brand of laundry detergent, you wouldn’t be able to collect data from every single person that uses laundry detergent (good luck with that!) – but you could potentially collect data from a smaller subset of this group.
In technical terms, the larger group is referred to as the population , and the subset (the group you’ll actually engage with in your research) is called the sample . Put another way, you can look at the population as a full cake and the sample as a single slice of that cake. In an ideal world, you’d want your sample to be perfectly representative of the population, as that would allow you to generalise your findings to the entire population. In other words, you’d want to cut a perfect cross-sectional slice of cake, such that the slice reflects every layer of the cake in perfect proportion.
Achieving a truly representative sample is, unfortunately, a little trickier than slicing a cake, as there are many practical challenges and obstacles to achieving this in a real-world setting. Thankfully though, you don’t always need to have a perfectly representative sample – it all depends on the specific research aims of each study – so don’t stress yourself out about that just yet!
With the concept of sampling broadly defined, let’s look at the different approaches to sampling to get a better understanding of what it all looks like in practice.
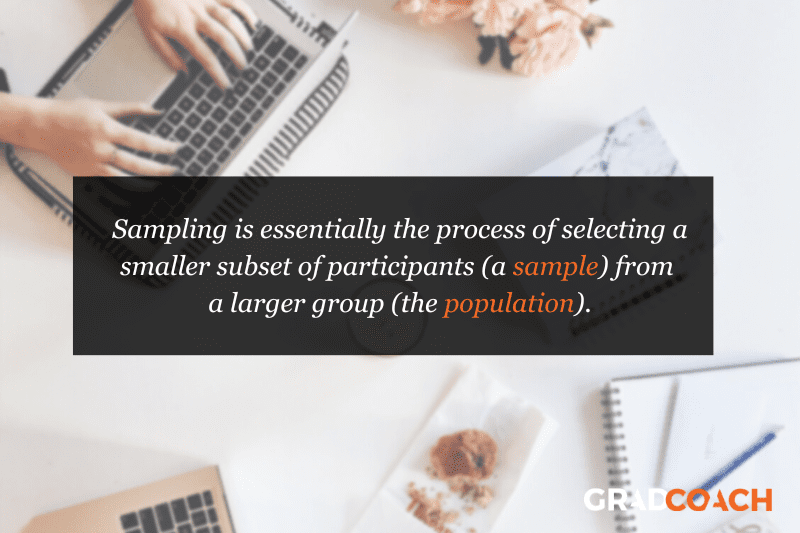
The two overarching sampling approaches
At the highest level, there are two approaches to sampling: probability sampling and non-probability sampling . Within each of these, there are a variety of sampling methods , which we’ll explore a little later.
Probability sampling involves selecting participants (or any unit of interest) on a statistically random basis , which is why it’s also called “random sampling”. In other words, the selection of each individual participant is based on a pre-determined process (not the discretion of the researcher). As a result, this approach achieves a random sample.
Probability-based sampling methods are most commonly used in quantitative research , especially when it’s important to achieve a representative sample that allows the researcher to generalise their findings.
Non-probability sampling , on the other hand, refers to sampling methods in which the selection of participants is not statistically random . In other words, the selection of individual participants is based on the discretion and judgment of the researcher, rather than on a pre-determined process.
Non-probability sampling methods are commonly used in qualitative research , where the richness and depth of the data are more important than the generalisability of the findings.
If that all sounds a little too conceptual and fluffy, don’t worry. Let’s take a look at some actual sampling methods to make it more tangible.
Need a helping hand?
Probability-based sampling methods
First, we’ll look at four common probability-based (random) sampling methods:
Importantly, this is not a comprehensive list of all the probability sampling methods – these are just four of the most common ones. So, if you’re interested in adopting a probability-based sampling approach, be sure to explore all the options.
Simple random sampling involves selecting participants in a completely random fashion , where each participant has an equal chance of being selected. Basically, this sampling method is the equivalent of pulling names out of a hat , except that you can do it digitally. For example, if you had a list of 500 people, you could use a random number generator to draw a list of 50 numbers (each number, reflecting a participant) and then use that dataset as your sample.
Thanks to its simplicity, simple random sampling is easy to implement , and as a consequence, is typically quite cheap and efficient . Given that the selection process is completely random, the results can be generalised fairly reliably. However, this also means it can hide the impact of large subgroups within the data, which can result in minority subgroups having little representation in the results – if any at all. To address this, one needs to take a slightly different approach, which we’ll look at next.
Stratified random sampling is similar to simple random sampling, but it kicks things up a notch. As the name suggests, stratified sampling involves selecting participants randomly , but from within certain pre-defined subgroups (i.e., strata) that share a common trait . For example, you might divide the population into strata based on gender, ethnicity, age range or level of education, and then select randomly from each group.
The benefit of this sampling method is that it gives you more control over the impact of large subgroups (strata) within the population. For example, if a population comprises 80% males and 20% females, you may want to “balance” this skew out by selecting a random sample from an equal number of males and females. This would, of course, reduce the representativeness of the sample, but it would allow you to identify differences between subgroups. So, depending on your research aims, the stratified approach could work well.
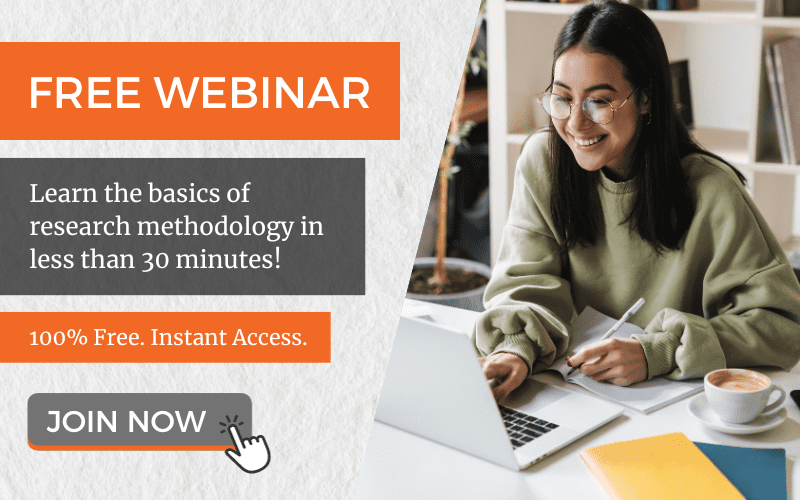
Next on the list is cluster sampling. As the name suggests, this sampling method involves sampling from naturally occurring, mutually exclusive clusters within a population – for example, area codes within a city or cities within a country. Once the clusters are defined, a set of clusters are randomly selected and then a set of participants are randomly selected from each cluster.
Now, you’re probably wondering, “how is cluster sampling different from stratified random sampling?”. Well, let’s look at the previous example where each cluster reflects an area code in a given city.
With cluster sampling, you would collect data from clusters of participants in a handful of area codes (let’s say 5 neighbourhoods). Conversely, with stratified random sampling, you would need to collect data from all over the city (i.e., many more neighbourhoods). You’d still achieve the same sample size either way (let’s say 200 people, for example), but with stratified sampling, you’d need to do a lot more running around, as participants would be scattered across a vast geographic area. As a result, cluster sampling is often the more practical and economical option.
If that all sounds a little mind-bending, you can use the following general rule of thumb. If a population is relatively homogeneous , cluster sampling will often be adequate. Conversely, if a population is quite heterogeneous (i.e., diverse), stratified sampling will generally be more appropriate.
The last probability sampling method we’ll look at is systematic sampling. This method simply involves selecting participants at a set interval , starting from a random point .
For example, if you have a list of students that reflects the population of a university, you could systematically sample that population by selecting participants at an interval of 8 . In other words, you would randomly select a starting point – let’s say student number 40 – followed by student 48, 56, 64, etc.
What’s important with systematic sampling is that the population list you select from needs to be randomly ordered . If there are underlying patterns in the list (for example, if the list is ordered by gender, IQ, age, etc.), this will result in a non-random sample, which would defeat the purpose of adopting this sampling method. Of course, you could safeguard against this by “shuffling” your population list using a random number generator or similar tool.
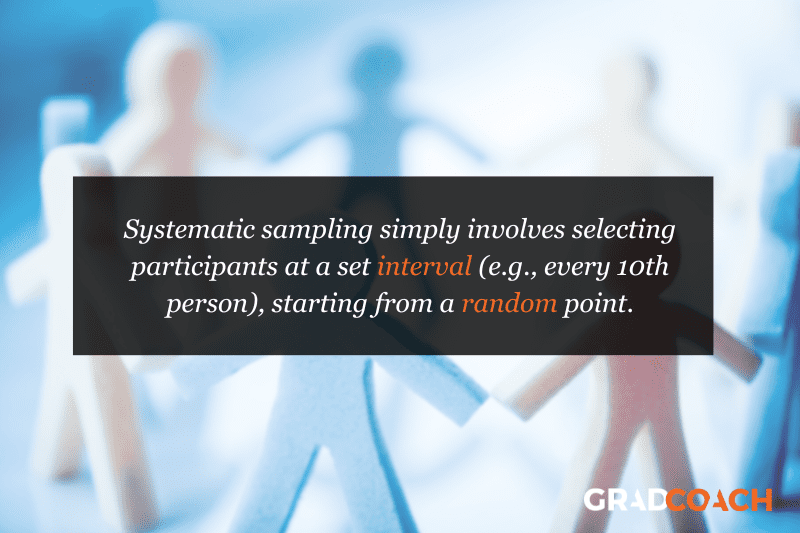
Non-probability-based sampling methods
Right, now that we’ve looked at a few probability-based sampling methods, let’s look at three non-probability methods :
Again, this is not an exhaustive list of all possible sampling methods, so be sure to explore further if you’re interested in adopting a non-probability sampling approach.
First up, we’ve got purposive sampling – also known as judgment , selective or subjective sampling. Again, the name provides some clues, as this method involves the researcher selecting participants using his or her own judgement , based on the purpose of the study (i.e., the research aims).
For example, suppose your research aims were to understand the perceptions of hyper-loyal customers of a particular retail store. In that case, you could use your judgement to engage with frequent shoppers, as well as rare or occasional shoppers, to understand what judgements drive the two behavioural extremes .
Purposive sampling is often used in studies where the aim is to gather information from a small population (especially rare or hard-to-find populations), as it allows the researcher to target specific individuals who have unique knowledge or experience . Naturally, this sampling method is quite prone to researcher bias and judgement error, and it’s unlikely to produce generalisable results, so it’s best suited to studies where the aim is to go deep rather than broad .
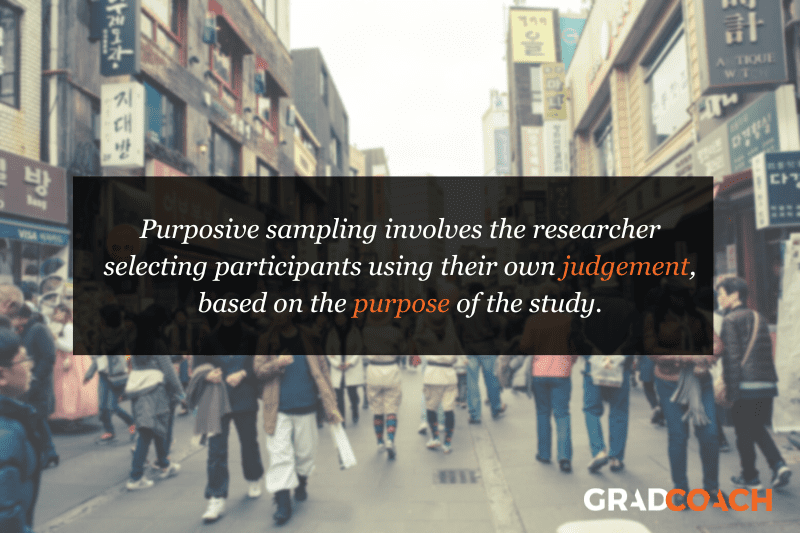
Next up, we have convenience sampling. As the name suggests, with this method, participants are selected based on their availability or accessibility . In other words, the sample is selected based on how convenient it is for the researcher to access it, as opposed to using a defined and objective process.
Naturally, convenience sampling provides a quick and easy way to gather data, as the sample is selected based on the individuals who are readily available or willing to participate. This makes it an attractive option if you’re particularly tight on resources and/or time. However, as you’d expect, this sampling method is unlikely to produce a representative sample and will of course be vulnerable to researcher bias , so it’s important to approach it with caution.
Last but not least, we have the snowball sampling method. This method relies on referrals from initial participants to recruit additional participants. In other words, the initial subjects form the first (small) snowball and each additional subject recruited through referral is added to the snowball, making it larger as it rolls along .
Snowball sampling is often used in research contexts where it’s difficult to identify and access a particular population. For example, people with a rare medical condition or members of an exclusive group. It can also be useful in cases where the research topic is sensitive or taboo and people are unlikely to open up unless they’re referred by someone they trust.
Simply put, snowball sampling is ideal for research that involves reaching hard-to-access populations . But, keep in mind that, once again, it’s a sampling method that’s highly prone to researcher bias and is unlikely to produce a representative sample. So, make sure that it aligns with your research aims and questions before adopting this method.
How to choose a sampling method
Now that we’ve looked at a few popular sampling methods (both probability and non-probability based), the obvious question is, “ how do I choose the right sampling method for my study?”. When selecting a sampling method for your research project, you’ll need to consider two important factors: your research aims and your resources .
As with all research design and methodology choices, your sampling approach needs to be guided by and aligned with your research aims, objectives and research questions – in other words, your golden thread. Specifically, you need to consider whether your research aims are primarily concerned with producing generalisable findings (in which case, you’ll likely opt for a probability-based sampling method) or with achieving rich , deep insights (in which case, a non-probability-based approach could be more practical). Typically, quantitative studies lean toward the former, while qualitative studies aim for the latter, so be sure to consider your broader methodology as well.
The second factor you need to consider is your resources and, more generally, the practical constraints at play. If, for example, you have easy, free access to a large sample at your workplace or university and a healthy budget to help you attract participants, that will open up multiple options in terms of sampling methods. Conversely, if you’re cash-strapped, short on time and don’t have unfettered access to your population of interest, you may be restricted to convenience or referral-based methods.
In short, be ready for trade-offs – you won’t always be able to utilise the “perfect” sampling method for your study, and that’s okay. Much like all the other methodological choices you’ll make as part of your study, you’ll often need to compromise and accept practical trade-offs when it comes to sampling. Don’t let this get you down though – as long as your sampling choice is well explained and justified, and the limitations of your approach are clearly articulated, you’ll be on the right track.
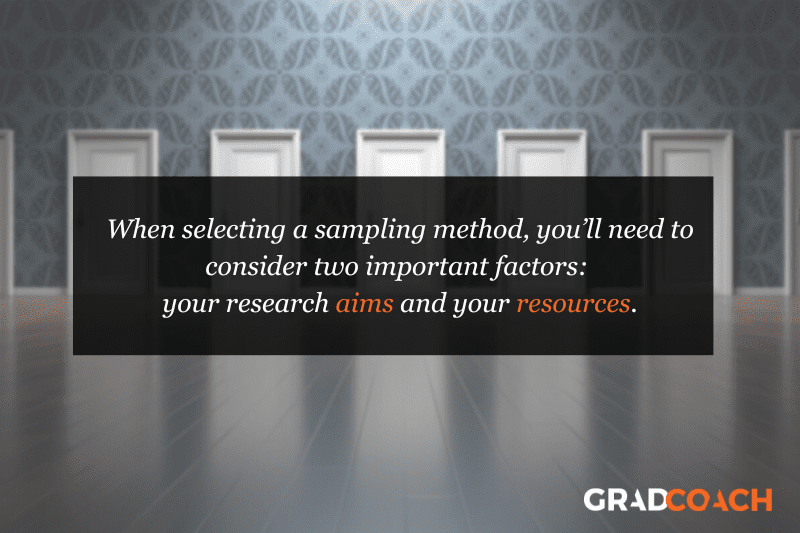
Let’s recap…
In this post, we’ve covered the basics of sampling within the context of a typical research project.
- Sampling refers to the process of defining a subgroup (sample) from the larger group of interest (population).
- The two overarching approaches to sampling are probability sampling (random) and non-probability sampling .
- Common probability-based sampling methods include simple random sampling, stratified random sampling, cluster sampling and systematic sampling.
- Common non-probability-based sampling methods include purposive sampling, convenience sampling and snowball sampling.
- When choosing a sampling method, you need to consider your research aims , objectives and questions, as well as your resources and other practical constraints .
If you’d like to see an example of a sampling strategy in action, be sure to check out our research methodology chapter sample .
Last but not least, if you need hands-on help with your sampling (or any other aspect of your research), take a look at our 1-on-1 coaching service , where we guide you through each step of the research process, at your own pace.
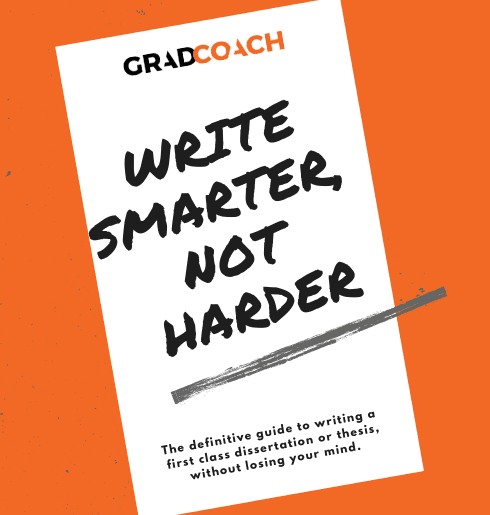
Psst… there’s more (for free)
This post is part of our dissertation mini-course, which covers everything you need to get started with your dissertation, thesis or research project.
You Might Also Like:
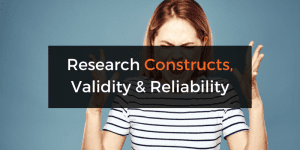
Excellent and helpful. Best site to get a full understanding of Research methodology. I’m nolonger as “clueless “..😉
Excellent and helpful for junior researcher!
Submit a Comment Cancel reply
Your email address will not be published. Required fields are marked *
Save my name, email, and website in this browser for the next time I comment.
- Print Friendly
- En español – ExME
- Em português – EME
What are sampling methods and how do you choose the best one?
Posted on 18th November 2020 by Mohamed Khalifa
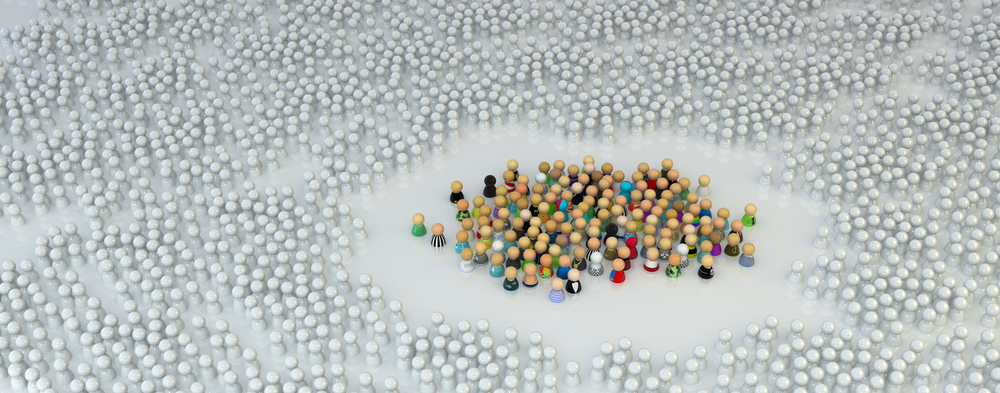
This tutorial will introduce sampling methods and potential sampling errors to avoid when conducting medical research.
Introduction to sampling methods
Examples of different sampling methods, choosing the best sampling method.
It is important to understand why we sample the population; for example, studies are built to investigate the relationships between risk factors and disease. In other words, we want to find out if this is a true association, while still aiming for the minimum risk for errors such as: chance, bias or confounding .
However, it would not be feasible to experiment on the whole population, we would need to take a good sample and aim to reduce the risk of having errors by proper sampling technique.
What is a sampling frame?
A sampling frame is a record of the target population containing all participants of interest. In other words, it is a list from which we can extract a sample.
What makes a good sample?
A good sample should be a representative subset of the population we are interested in studying, therefore, with each participant having equal chance of being randomly selected into the study.
We could choose a sampling method based on whether we want to account for sampling bias; a random sampling method is often preferred over a non-random method for this reason. Random sampling examples include: simple, systematic, stratified, and cluster sampling. Non-random sampling methods are liable to bias, and common examples include: convenience, purposive, snowballing, and quota sampling. For the purposes of this blog we will be focusing on random sampling methods .
Example: We want to conduct an experimental trial in a small population such as: employees in a company, or students in a college. We include everyone in a list and use a random number generator to select the participants
Advantages: Generalisable results possible, random sampling, the sampling frame is the whole population, every participant has an equal probability of being selected
Disadvantages: Less precise than stratified method, less representative than the systematic method
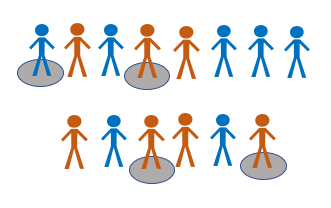
Example: Every nth patient entering the out-patient clinic is selected and included in our sample
Advantages: More feasible than simple or stratified methods, sampling frame is not always required
Disadvantages: Generalisability may decrease if baseline characteristics repeat across every nth participant
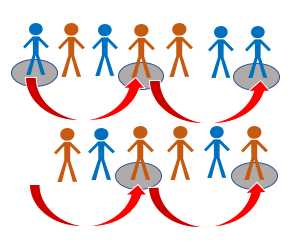
Example: We have a big population (a city) and we want to ensure representativeness of all groups with a pre-determined characteristic such as: age groups, ethnic origin, and gender
Advantages: Inclusive of strata (subgroups), reliable and generalisable results
Disadvantages: Does not work well with multiple variables
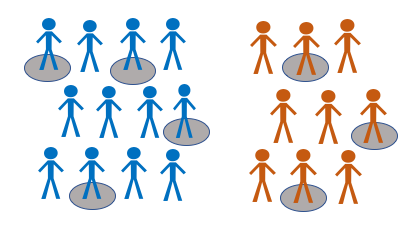
Example: 10 schools have the same number of students across the county. We can randomly select 3 out of 10 schools as our clusters
Advantages: Readily doable with most budgets, does not require a sampling frame
Disadvantages: Results may not be reliable nor generalisable
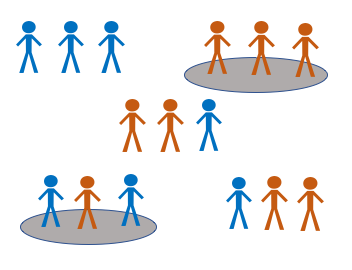
How can you identify sampling errors?
Non-random selection increases the probability of sampling (selection) bias if the sample does not represent the population we want to study. We could avoid this by random sampling and ensuring representativeness of our sample with regards to sample size.
An inadequate sample size decreases the confidence in our results as we may think there is no significant difference when actually there is. This type two error results from having a small sample size, or from participants dropping out of the sample.
In medical research of disease, if we select people with certain diseases while strictly excluding participants with other co-morbidities, we run the risk of diagnostic purity bias where important sub-groups of the population are not represented.
Furthermore, measurement bias may occur during re-collection of risk factors by participants (recall bias) or assessment of outcome where people who live longer are associated with treatment success, when in fact people who died were not included in the sample or data analysis (survivors bias).
By following the steps below we could choose the best sampling method for our study in an orderly fashion.
Research objectiveness
Firstly, a refined research question and goal would help us define our population of interest. If our calculated sample size is small then it would be easier to get a random sample. If, however, the sample size is large, then we should check if our budget and resources can handle a random sampling method.
Sampling frame availability
Secondly, we need to check for availability of a sampling frame (Simple), if not, could we make a list of our own (Stratified). If neither option is possible, we could still use other random sampling methods, for instance, systematic or cluster sampling.
Study design
Moreover, we could consider the prevalence of the topic (exposure or outcome) in the population, and what would be the suitable study design. In addition, checking if our target population is widely varied in its baseline characteristics. For example, a population with large ethnic subgroups could best be studied using a stratified sampling method.
Random sampling
Finally, the best sampling method is always the one that could best answer our research question while also allowing for others to make use of our results (generalisability of results). When we cannot afford a random sampling method, we can always choose from the non-random sampling methods.
To sum up, we now understand that choosing between random or non-random sampling methods is multifactorial. We might often be tempted to choose a convenience sample from the start, but that would not only decrease precision of our results, and would make us miss out on producing research that is more robust and reliable.
References (pdf)
Mohamed Khalifa
Leave a reply cancel reply.
Your email address will not be published. Required fields are marked *
Save my name, email, and website in this browser for the next time I comment.
No Comments on What are sampling methods and how do you choose the best one?
Thank you for this overview. A concise approach for research.
really helps! am an ecology student preparing to write my lab report for sampling.
I learned a lot to the given presentation.. It’s very comprehensive… Thanks for sharing…
Very informative and useful for my study. Thank you
Oversimplified info on sampling methods. Probabilistic of the sampling and sampling of samples by chance does rest solely on the random methods. Factors such as the random visits or presentation of the potential participants at clinics or sites could be sufficiently random in nature and should be used for the sake of efficiency and feasibility. Nevertheless, this approach has to be taken only after careful thoughts. Representativeness of the study samples have to be checked at the end or during reporting by comparing it to the published larger studies or register of some kind in/from the local population.
Thank you so much Mr.mohamed very useful and informative article
Subscribe to our newsletter
You will receive our monthly newsletter and free access to Trip Premium.
Related Articles
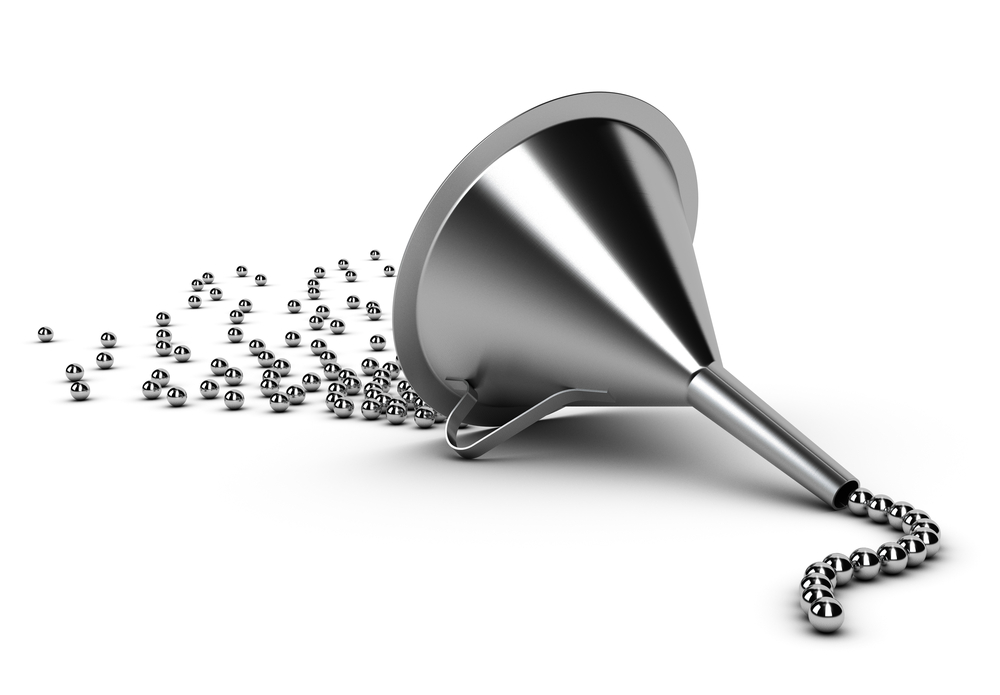
How to read a funnel plot
This blog introduces you to funnel plots, guiding you through how to read them and what may cause them to look asymmetrical.
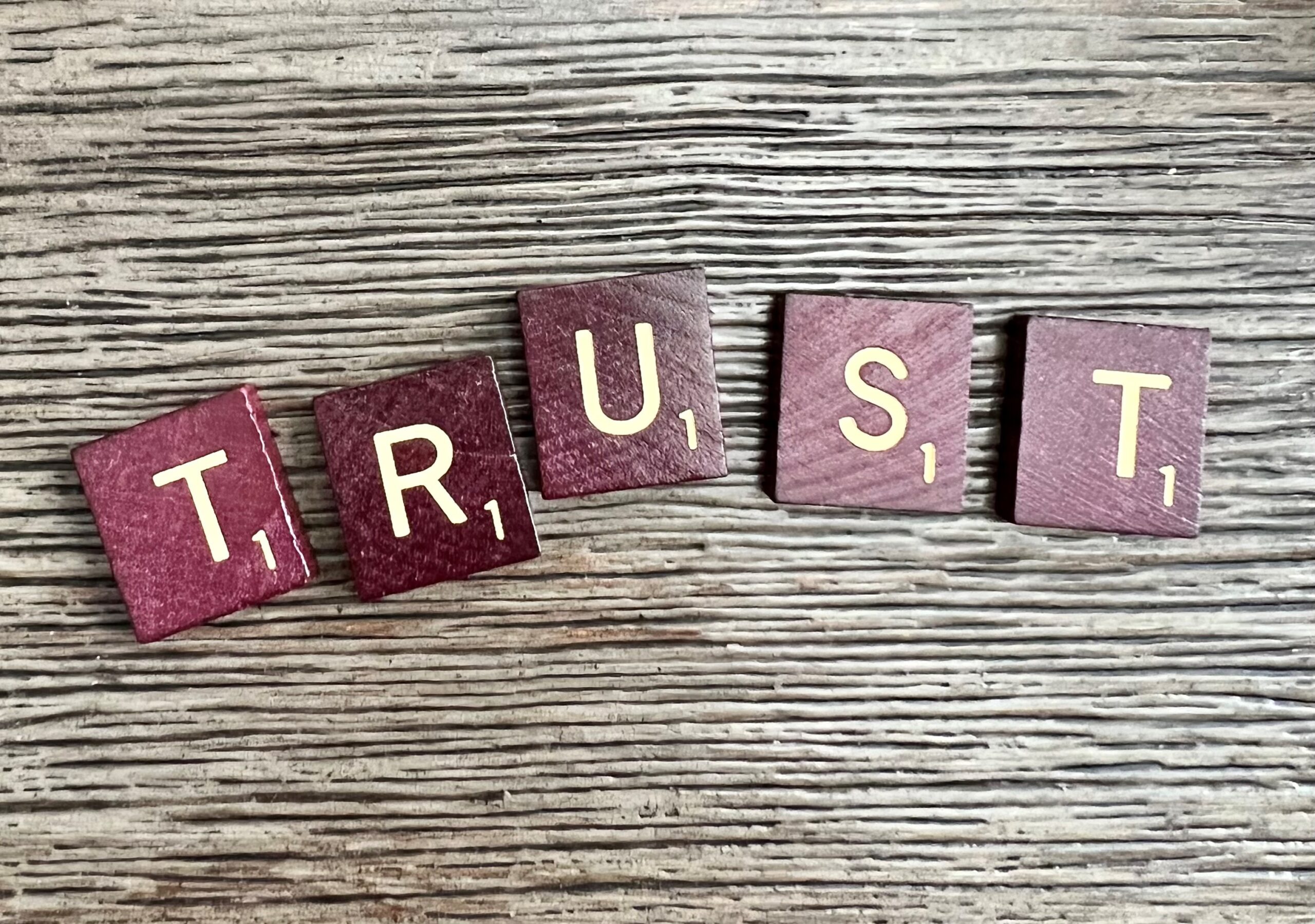
Internal and external validity: what are they and how do they differ?
Is this study valid? Can I trust this study’s methods and design? Can I apply the results of this study to other contexts? Learn more about internal and external validity in research to help you answer these questions when you next look at a paper.
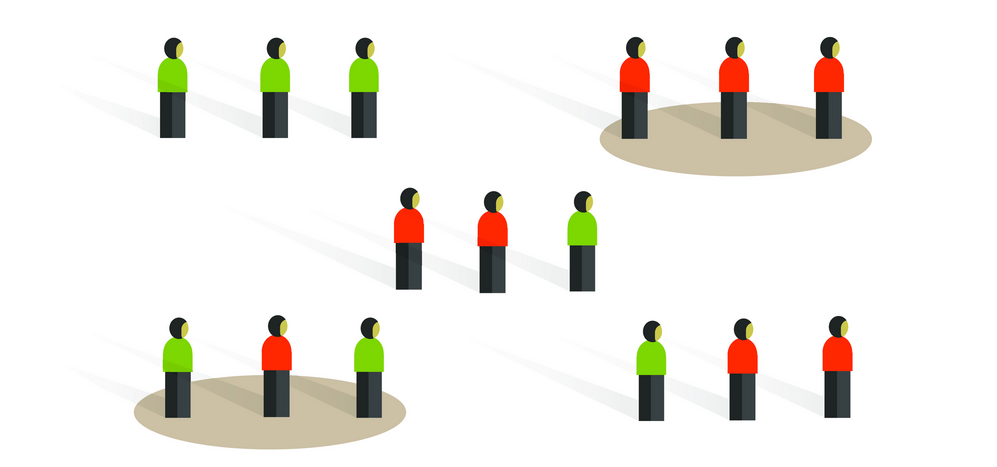
Cluster Randomized Trials: Concepts
This blog summarizes the concepts of cluster randomization, and the logistical and statistical considerations while designing a cluster randomized controlled trial.
Join thousands of product people at Insight Out Conf on April 11. Register free.
Insights hub solutions
Analyze data
Uncover deep customer insights with fast, powerful features, store insights, curate and manage insights in one searchable platform, scale research, unlock the potential of customer insights at enterprise scale.
Featured reads
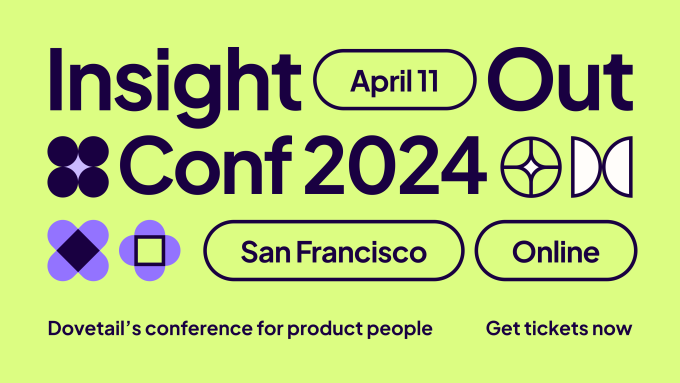
Inspiration
Three things to look forward to at Insight Out
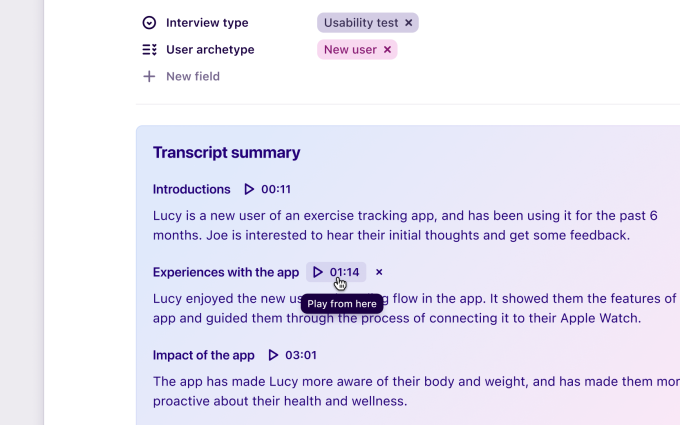
Tips and tricks
Make magic with your customer data in Dovetail
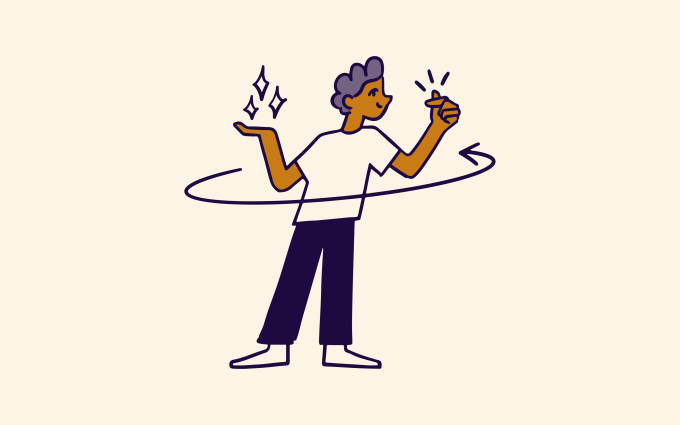
Four ways Dovetail helps Product Managers master continuous product discovery
Events and videos
© Dovetail Research Pty. Ltd.
An overview of sampling methods
Last updated
27 February 2023
Reviewed by
Cathy Heath
When researching perceptions or attributes of a product, service, or people, you have two options:
Survey every person in your chosen group (the target market, or population), collate your responses, and reach your conclusions.
Select a smaller group from within your target market and use their answers to represent everyone. This option is sampling .
Sampling saves you time and money. When you use the sampling method, the whole population being studied is called the sampling frame .
The sample you choose should represent your target market, or the sampling frame, well enough to do one of the following:
Generalize your findings across the sampling frame and use them as though you had surveyed everyone
Use the findings to decide on your next step, which might involve more in-depth sampling
Make research less tedious
Dovetail streamlines research to help you uncover and share actionable insights
How was sampling developed?
Valery Glivenko and Francesco Cantelli, two mathematicians studying probability theory in the early 1900s, devised the sampling method. Their research showed that a properly chosen sample of people would reflect the larger group’s status, opinions, decisions, and decision-making steps.
They proved you don't need to survey the entire target market, thereby saving the rest of us a lot of time and money.
- Why is sampling important?
We’ve already touched on the fact that sampling saves you time and money. When you get reliable results quickly, you can act on them sooner. And the money you save can pay for something else.
It’s often easier to survey a sample than a whole population. Sample inferences can be more reliable than those you get from a very large group because you can choose your samples carefully and scientifically.
Sampling is also useful because it is often impossible to survey the entire population. You probably have no choice but to collect only a sample in the first place.
Because you’re working with fewer people, you can collect richer data, which makes your research more accurate. You can:
Ask more questions
Go into more detail
Seek opinions instead of just collecting facts
Observe user behaviors
Double-check your findings if you need to
In short, sampling works! Let's take a look at the most common sampling methods.
- Types of sampling methods
There are two main sampling methods: probability sampling and non-probability sampling. These can be further refined, which we'll cover shortly. You can then decide which approach best suits your research project.
Probability sampling method
Probability sampling is used in quantitative research , so it provides data on the survey topic in terms of numbers. Probability relates to mathematics, hence the name ‘quantitative research’. Subjects are asked questions like:
How many boxes of candy do you buy at one time?
How often do you shop for candy?
How much would you pay for a box of candy?
This method is also called random sampling because everyone in the target market has an equal chance of being chosen for the survey. It is designed to reduce sampling error for the most important variables. You should, therefore, get results that fairly reflect the larger population.
Non-probability sampling method
In this method, not everyone has an equal chance of being part of the sample. It's usually easier (and cheaper) to select people for the sample group. You choose people who are more likely to be involved in or know more about the topic you’re researching.
Non-probability sampling is used for qualitative research. Qualitative data is generated by questions like:
Where do you usually shop for candy (supermarket, gas station, etc.?)
Which candy brand do you usually buy?
Why do you like that brand?
- Probability sampling methods
Here are five ways of doing probability sampling:
Simple random sampling (basic probability sampling)
Systematic sampling
Stratified sampling.
Cluster sampling
Multi-stage sampling
Simple random sampling.
There are three basic steps to simple random sampling:
Choose your sampling frame.
Decide on your sample size. Make sure it is large enough to give you reliable data.
Randomly choose your sample participants.
You could put all their names in a hat, shake the hat to mix the names, and pull out however many names you want in your sample (without looking!)
You could be more scientific by giving each participant a number and then using a random number generator program to choose the numbers.
Instead of choosing names or numbers, you decide beforehand on a selection method. For example, collect all the names in your sampling frame and start at, for example, the fifth person on the list, then choose every fourth name or every tenth name. Alternatively, you could choose everyone whose last name begins with randomly-selected initials, such as A, G, or W.
Choose your system of selecting names, and away you go.
This is a more sophisticated way to choose your sample. You break the sampling frame down into important subgroups or strata . Then, decide how many you want in your sample, and choose an equal number (or a proportionate number) from each subgroup.
For example, you want to survey how many people in a geographic area buy candy, so you compile a list of everyone in that area. You then break that list down into, for example, males and females, then into pre-teens, teenagers, young adults, senior citizens, etc. who are male or female.
So, if there are 1,000 young male adults and 2,000 young female adults in the whole sampling frame, you may want to choose 100 males and 200 females to keep the proportions balanced. You then choose the individual survey participants through the systematic sampling method.
Clustered sampling
This method is used when you want to subdivide a sample into smaller groups or clusters that are geographically or organizationally related.
Let’s say you’re doing quantitative research into candy sales. You could choose your sample participants from urban, suburban, or rural populations. This would give you three geographic clusters from which to select your participants.
This is a more refined way of doing cluster sampling. Let’s say you have your urban cluster, which is your primary sampling unit. You can subdivide this into a secondary sampling unit, say, participants who typically buy their candy in supermarkets. You could then further subdivide this group into your ultimate sampling unit. Finally, you select the actual survey participants from this unit.
- Uses of probability sampling
Probability sampling has three main advantages:
It helps minimizes the likelihood of sampling bias. How you choose your sample determines the quality of your results. Probability sampling gives you an unbiased, randomly selected sample of your target market.
It allows you to create representative samples and subgroups within a sample out of a large or diverse target market.
It lets you use sophisticated statistical methods to select as close to perfect samples as possible.
- Non-probability sampling methods
To recap, with non-probability sampling, you choose people for your sample in a non-random way, so not everyone in your sampling frame has an equal chance of being chosen. Your research findings, therefore, may not be as representative overall as probability sampling, but you may not want them to be.
Sampling bias is not a concern if all potential survey participants share similar traits. For example, you may want to specifically focus on young male adults who spend more than others on candy. In addition, it is usually a cheaper and quicker method because you don't have to work out a complex selection system that represents the entire population in that community.
Researchers do need to be mindful of carefully considering the strengths and limitations of each method before selecting a sampling technique.
Non-probability sampling is best for exploratory research , such as at the beginning of a research project.
There are five main types of non-probability sampling methods:
Convenience sampling
Purposive sampling, voluntary response sampling, snowball sampling, quota sampling.
The strategy of convenience sampling is to choose your sample quickly and efficiently, using the least effort, usually to save money.
Let's say you want to survey the opinions of 100 millennials about a particular topic. You could send out a questionnaire over the social media platforms millennials use. Ask respondents to confirm their birth year at the top of their response sheet and, when you have your 100 responses, begin your analysis. Or you could visit restaurants and bars where millennials spend their evenings and sign people up.
A drawback of convenience sampling is that it may not yield results that apply to a broader population.
This method relies on your judgment to choose the most likely sample to deliver the most useful results. You must know enough about the survey goals and the sampling frame to choose the most appropriate sample respondents.
Your knowledge and experience save you time because you know your ideal sample candidates, so you should get high-quality results.
This method is similar to convenience sampling, but it is based on potential sample members volunteering rather than you looking for people.
You make it known you want to do a survey on a particular topic for a particular reason and wait until enough people volunteer. Then you give them the questionnaire or arrange interviews to ask your questions directly.
Snowball sampling involves asking selected participants to refer others who may qualify for the survey. This method is best used when there is no sampling frame available. It is also useful when the researcher doesn’t know much about the target population.
Let's say you want to research a niche topic that involves people who may be difficult to locate. For our candy example, this could be young males who buy a lot of candy, go rock climbing during the day, and watch adventure movies at night. You ask each participant to name others they know who do the same things, so you can contact them. As you make contact with more people, your sample 'snowballs' until you have all the names you need.
This sampling method involves collecting the specific number of units (quotas) from your predetermined subpopulations. Quota sampling is a way of ensuring that your sample accurately represents the sampling frame.
- Uses of non-probability sampling
You can use non-probability sampling when you:
Want to do a quick test to see if a more detailed and sophisticated survey may be worthwhile
Want to explore an idea to see if it 'has legs'
Launch a pilot study
Do some initial qualitative research
Have little time or money available (half a loaf is better than no bread at all)
Want to see if the initial results will help you justify a longer, more detailed, and more expensive research project
- The main types of sampling bias, and how to avoid them
Sampling bias can fog or limit your research results. This will have an impact when you generalize your results across the whole target market. The two main causes of sampling bias are faulty research design and poor data collection or recording. They can affect probability and non-probability sampling.
Faulty research
If a surveyor chooses participants inappropriately, the results will not reflect the population as a whole.
A famous example is the 1948 presidential race. A telephone survey was conducted to see which candidate had more support. The problem with the research design was that, in 1948, most people with telephones were wealthy, and their opinions were very different from voters as a whole. The research implied Dewey would win, but it was Truman who became president.
Poor data collection or recording
This problem speaks for itself. The survey may be well structured, the sample groups appropriate, the questions clear and easy to understand, and the cluster sizes appropriate. But if surveyors check the wrong boxes when they get an answer or if the entire subgroup results are lost, the survey results will be biased.
How do you minimize bias in sampling?
To get results you can rely on, you must:
Know enough about your target market
Choose one or more sample surveys to cover the whole target market properly
Choose enough people in each sample so your results mirror your target market
Have content validity . This means the content of your questions must be direct and efficiently worded. If it isn’t, the viability of your survey could be questioned. That would also be a waste of time and money, so make the wording of your questions your top focus.
If using probability sampling, make sure your sampling frame includes everyone it should and that your random sampling selection process includes the right proportion of the subgroups
If using non-probability sampling, focus on fairness, equality, and completeness in identifying your samples and subgroups. Then balance those criteria against simple convenience or other relevant factors.
What are the five types of sampling bias?
Self-selection bias. If you mass-mail questionnaires to everyone in the sample, you’re more likely to get results from people with extrovert or activist personalities and not from introverts or pragmatists. So if your convenience sampling focuses on getting your quota responses quickly, it may be skewed.
Non-response bias. Unhappy customers, stressed-out employees, or other sub-groups may not want to cooperate or they may pull out early.
Undercoverage bias. If your survey is done, say, via email or social media platforms, it will miss people without internet access, such as those living in rural areas, the elderly, or lower-income groups.
Survivorship bias. Unsuccessful people are less likely to take part. Another example may be a researcher excluding results that don’t support the overall goal. If the CEO wants to tell the shareholders about a successful product or project at the AGM, some less positive survey results may go “missing” (to take an extreme example.) The result is that your data will reflect an overly optimistic representation of the truth.
Pre-screening bias. If the researcher, whose experience and knowledge are being used to pre-select respondents in a judgmental sampling, focuses more on convenience than judgment, the results may be compromised.
How do you minimize sampling bias?
Focus on the bullet points in the next section and:
Make survey questionnaires as direct, easy, short, and available as possible, so participants are more likely to complete them accurately and send them back
Follow up with the people who have been selected but have not returned their responses
Ignore any pressure that may produce bias
- How do you decide on the type of sampling to use?
Use the ideas you've gleaned from this article to give yourself a platform, then choose the best method to meet your goals while staying within your time and cost limits.
If it isn't obvious which method you should choose, use this strategy:
Clarify your research goals
Clarify how accurate your research results must be to reach your goals
Evaluate your goals against time and budget
List the two or three most obvious sampling methods that will work for you
Confirm the availability of your resources (researchers, computer time, etc.)
Compare each of the possible methods with your goals, accuracy, precision, resource, time, and cost constraints
Make your decision
- The takeaway
Effective market research is the basis of successful marketing, advertising, and future productivity. By selecting the most appropriate sampling methods, you will collect the most useful market data and make the most effective decisions.
Get started today
Go from raw data to valuable insights with a flexible research platform
Editor’s picks
Last updated: 21 December 2023
Last updated: 16 December 2023
Last updated: 6 October 2023
Last updated: 17 February 2024
Last updated: 5 March 2024
Last updated: 19 November 2023
Last updated: 15 February 2024
Last updated: 11 March 2024
Last updated: 12 December 2023
Last updated: 6 March 2024
Last updated: 10 April 2023
Last updated: 20 December 2023
Latest articles
Related topics, log in or sign up.
Get started for free
If you're seeing this message, it means we're having trouble loading external resources on our website.
If you're behind a web filter, please make sure that the domains *.kastatic.org and *.kasandbox.org are unblocked.
To log in and use all the features of Khan Academy, please enable JavaScript in your browser.
Statistics and probability
Course: statistics and probability > unit 6.
- Picking fairly
- Using probability to make fair decisions
- Techniques for generating a simple random sample
- Simple random samples
- Techniques for random sampling and avoiding bias
- Sampling methods
Sampling methods review
- Samples and surveys
What are sampling methods?
Bad ways to sample.
- (Choice A) Convenience sampling A Convenience sampling
- (Choice B) Voluntary response sampling B Voluntary response sampling
Good ways to sample
- (Choice A) Simple random sampling A Simple random sampling
- (Choice B) Stratified random sampling B Stratified random sampling
- (Choice C) Cluster random sampling C Cluster random sampling
- (Choice D) Systematic random sampling D Systematic random sampling
Want to join the conversation?
- Upvote Button navigates to signup page
- Downvote Button navigates to signup page
- Flag Button navigates to signup page

- Skip to main content
- Skip to primary sidebar
- Skip to footer
- QuestionPro

- Solutions Industries Gaming Automotive Sports and events Education Government Travel & Hospitality Financial Services Healthcare Cannabis Technology Use Case NPS+ Communities Audience Contactless surveys Mobile LivePolls Member Experience GDPR Positive People Science 360 Feedback Surveys
- Resources Blog eBooks Survey Templates Case Studies Training Help center

Home Market Research
Sampling Methods: Guide To All Types with Examples
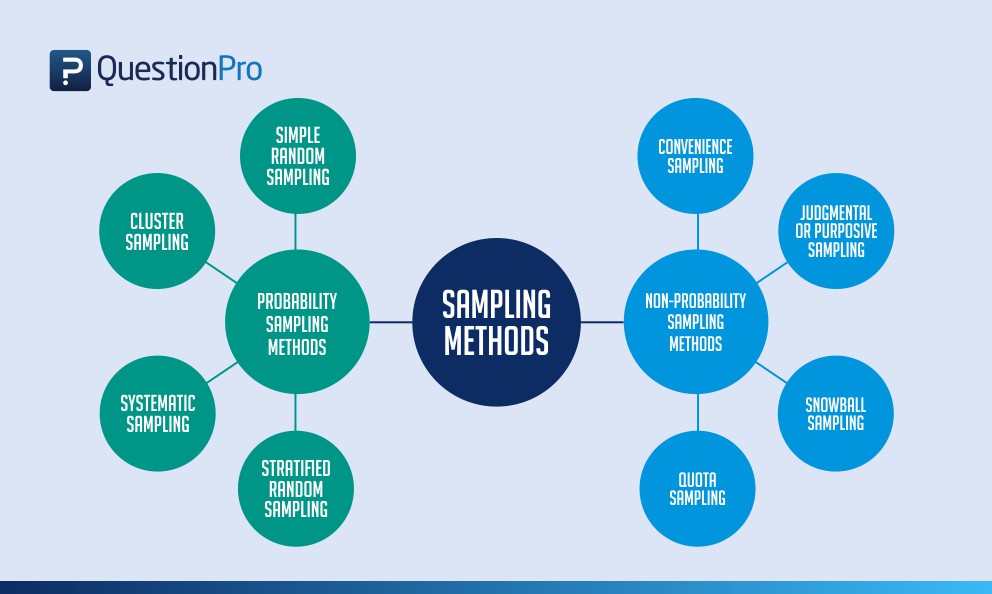
Sampling is an essential part of any research project. The right sampling method can make or break the validity of your research, and it’s essential to choose the right method for your specific question. In this article, we’ll take a closer look at some of the most popular sampling methods and provide real-world examples of how they can be used to gather accurate and reliable data.
LEARN ABOUT: Research Process Steps
From simple random sampling to complex stratified sampling , we’ll explore each method’s pros, cons, and best practices. So, whether you’re a seasoned researcher or just starting your journey, this article is a must-read for anyone looking to master sampling methods. Let’s get started!
Content Index
What is sampling?
Types of sampling: sampling methods, types of probability sampling with examples:, uses of probability sampling, types of non-probability sampling with examples, uses of non-probability sampling, how do you decide on the type of sampling to use, difference between probability sampling and non-probability sampling methods.
Sampling is a technique of selecting individual members or a subset of the population to make statistical inferences from them and estimate the characteristics of the whole population. Different sampling methods are widely used by researchers in market research so that they do not need to research the entire population to collect actionable insights.
It is also a time-convenient and cost-effective method and hence forms the basis of any research design . Sampling techniques can be used in research survey software for optimum derivation.
For example, suppose a drug manufacturer would like to research the adverse side effects of a drug on the country’s population. In that case, it is almost impossible to conduct a research study that involves everyone. In this case, the researcher decides on a sample of people from each demographic and then researches them, giving him/her indicative feedback on the drug’s behavior.
Learn more about Audience by QuestionPro
Sampling in market action research is of two types – probability sampling and non-probability sampling. Let’s take a closer look at these two methods of sampling.
- Probability sampling: Probability sampling is a sampling technique where a researcher selects a few criteria and chooses members of a population randomly. All the members have an equal opportunity to participate in the sample with this selection parameter.
- Non-probability sampling: In non-probability sampling, the researcher randomly chooses members for research. This sampling method is not a fixed or predefined selection process. This makes it difficult for all population elements to have equal opportunities to be included in a sample.
This blog discusses the various probability and non-probability sampling methods you can implement in any market research study.
LEARN ABOUT: Survey Sampling
Probability sampling is a technique in which researchers choose samples from a larger population based on the theory of probability. This sampling method considers every member of the population and forms samples based on a fixed process.
For example, in a population of 1000 members, every member will have a 1/1000 chance of being selected to be a part of a sample. Probability sampling eliminates sampling bias in the population and allows all members to be included in the sample.
There are four types of probability sampling techniques:
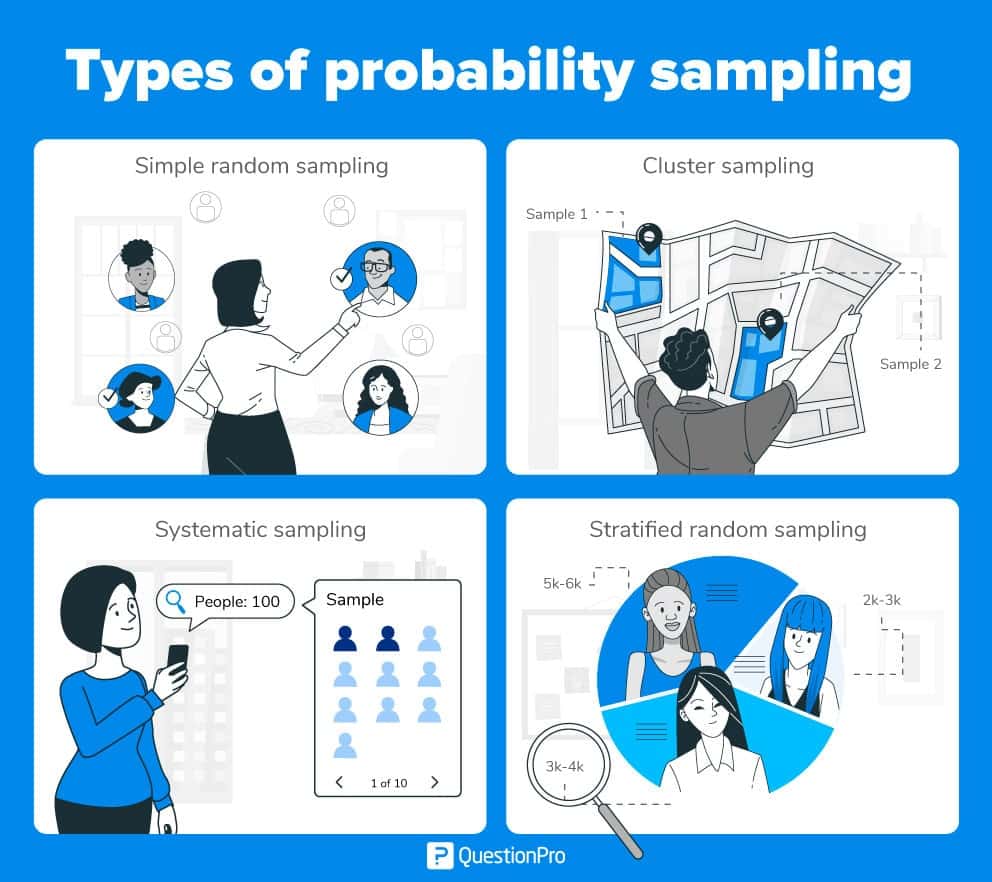
- Simple random sampling: One of the best probability sampling techniques that helps in saving time and resources is the Simple Random Sampling method. It is a reliable method of obtaining information where every single member of a population is chosen randomly, merely by chance. Each individual has the same probability of being chosen to be a part of a sample. For example, in an organization of 500 employees, if the HR team decides on conducting team-building activities, they would likely prefer picking chits out of a bowl. In this case, each of the 500 employees has an equal opportunity of being selected.
- Cluster sampling: Cluster sampling is a method where the researchers divide the entire population into sections or clusters representing a population. Clusters are identified and included in a sample based on demographic parameters like age, sex, location, etc. This makes it very simple for a survey creator to derive effective inferences from the feedback. For example, suppose the United States government wishes to evaluate the number of immigrants living in the Mainland US. In that case, they can divide it into clusters based on states such as California, Texas, Florida, Massachusetts, Colorado, Hawaii, etc. This way of conducting a survey will be more effective as the results will be organized into states and provide insightful immigration data.
- Systematic sampling: Researchers use the systematic sampling method to choose the sample members of a population at regular intervals. It requires selecting a starting point for the sample and sample size determination that can be repeated at regular intervals. This type of sampling method has a predefined range; hence, this sampling technique is the least time-consuming. For example, a researcher intends to collect a systematic sample of 500 people in a population of 5000. He/she numbers each element of the population from 1-5000 and will choose every 10th individual to be a part of the sample (Total population/ Sample Size = 5000/500 = 10).
- Stratified random sampling: Stratified random sampling is a method in which the researcher divides the population into smaller groups that don’t overlap but represent the entire population. While sampling, these groups can be organized, and then draw a sample from each group separately. For example, a researcher looking to analyze the characteristics of people belonging to different annual income divisions will create strata (groups) according to the annual family income. Eg – less than $20,000, $21,000 – $30,000, $31,000 to $40,000, $41,000 to $50,000, etc. By doing this, the researcher concludes the characteristics of people belonging to different income groups. Marketers can analyze which income groups to target and which ones to eliminate to create a roadmap that would bear fruitful results.
LEARN ABOUT: Purposive Sampling
There are multiple uses of probability sampling:
- Reduce Sample Bias: Using the probability sampling method, the research bias in the sample derived from a population is negligible to non-existent. The sample selection mainly depicts the researcher’s understanding and inference. Probability sampling leads to higher-quality data collection as the sample appropriately represents the population.
- Diverse Population: When the population is vast and diverse, it is essential to have adequate representation so that the data is not skewed toward one demographic . For example, suppose Square would like to understand the people that could make their point-of-sale devices. In that case, a survey conducted from a sample of people across the US from different industries and socio-economic backgrounds helps.
- Create an Accurate Sample: Probability sampling helps the researchers plan and create an accurate sample. This helps to obtain well-defined data.
The non-probability method is a sampling method that involves a collection of feedback based on a researcher or statistician’s sample selection capabilities and not on a fixed selection process. In most situations, the output of a survey conducted with a non-probable sample leads to skewed results, which may not represent the desired target population. But, there are situations, such as the preliminary stages of research or cost constraints for conducting research, where non-probability sampling will be much more useful than the other type.
Four types of non-probability sampling explain the purpose of this sampling method in a better manner:
- Convenience sampling: This method depends on the ease of access to subjects such as surveying customers at a mall or passers-by on a busy street. It is usually termed as convenience sampling because of the researcher’s ease of carrying it out and getting in touch with the subjects. Researchers have nearly no authority to select the sample elements, and it’s purely done based on proximity and not representativeness. This non-probability sampling method is used when there are time and cost limitations in collecting feedback. In situations with resource limitations, such as the initial stages of research, convenience sampling is used. For example, startups and NGOs usually conduct convenience sampling at a mall to distribute leaflets of upcoming events or promotion of a cause – they do that by standing at the mall entrance and giving out pamphlets randomly.
- Judgmental or purposive sampling: Judgmental or purposive samples are formed at the researcher’s discretion. Researchers purely consider the purpose of the study, along with the understanding of the target audience. For instance, when researchers want to understand the thought process of people interested in studying for their master’s degree. The selection criteria will be: “Are you interested in doing your masters in …?” and those who respond with a “No” are excluded from the sample.
- Snowball sampling: Snowball sampling is a sampling method that researchers apply when the subjects are difficult to trace. For example, surveying shelterless people or illegal immigrants will be extremely challenging. In such cases, using the snowball theory, researchers can track a few categories to interview and derive results. Researchers also implement this sampling method when the topic is highly sensitive and not openly discussed—for example, surveys to gather information about HIV Aids. Not many victims will readily respond to the questions. Still, researchers can contact people they might know or volunteers associated with the cause to get in touch with the victims and collect information.
- Quota sampling: In Quota sampling , members in this sampling technique selection happens based on a pre-set standard. In this case, as a sample is formed based on specific attributes, the created sample will have the same qualities found in the total population. It is a rapid method of collecting samples.
Non-probability sampling is used for the following:
- Create a hypothesis: Researchers use the non-probability sampling method to create an assumption when limited to no prior information is available. This method helps with the immediate return of data and builds a base for further research.
- Exploratory research: Researchers use this sampling technique widely when conducting qualitative research, pilot studies, or exploratory research .
- Budget and time constraints: The non-probability method when there are budget and time constraints, and some preliminary data must be collected. Since the survey design is not rigid, it is easier to pick respondents randomly and have them take the survey or questionnaire .
For any research, it is essential to choose a sampling method accurately to meet the goals of your study. The effectiveness of your sampling relies on various factors. Here are some steps expert researchers follow to decide the best sampling method.
- Jot down the research goals. Generally, it must be a combination of cost, precision, or accuracy.
- Identify the effective sampling techniques that might potentially achieve the research goals.
- Test each of these methods and examine whether they help achieve your goal.
- Select the method that works best for the research.
Unlock the power of accurate sampling!
We have looked at the different types of sampling methods above and their subtypes. To encapsulate the whole discussion, though, the significant differences between probability sampling methods and non-probability sampling methods are as below:
Now that we have learned how different sampling methods work and are widely used by researchers in market research so that they don’t need to research the entire population to collect actionable insights, let’s go over a tool that can help you manage these insights.
LEARN ABOUT: 12 Best Tools for Researchers
QuestionPro understands the need for an accurate, timely, and cost-effective method to select the proper sample; that’s why we bring QuestionPro Software, a set of tools that allow you to efficiently select your target audience , manage your insights in an organized, customizable repository and community management for post-survey feedback.
Don’t miss the chance to elevate the value of research.
FREE TRIAL LEARN MORE
MORE LIKE THIS
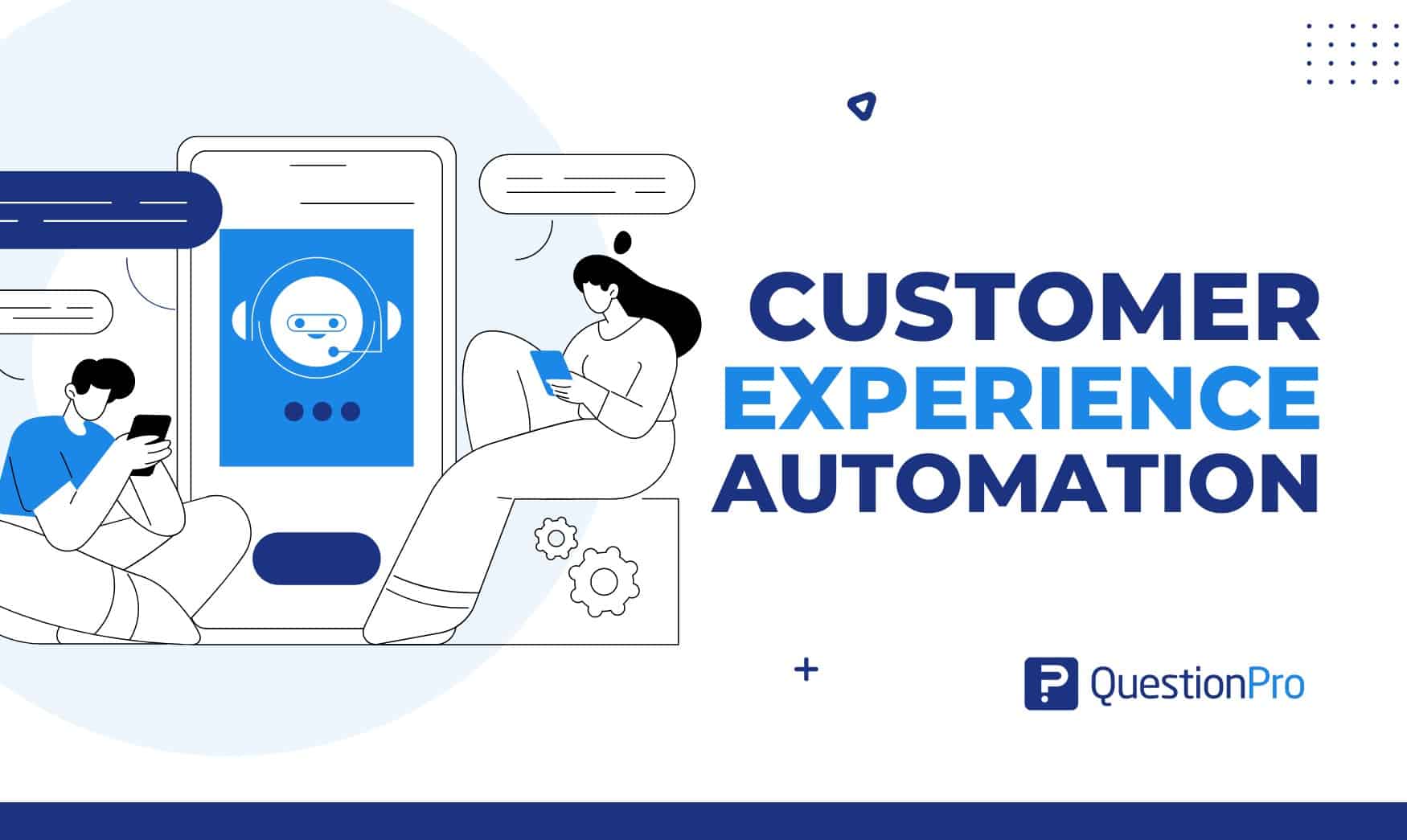
Customer Experience Automation: Benefits and Best Tools
Apr 1, 2024
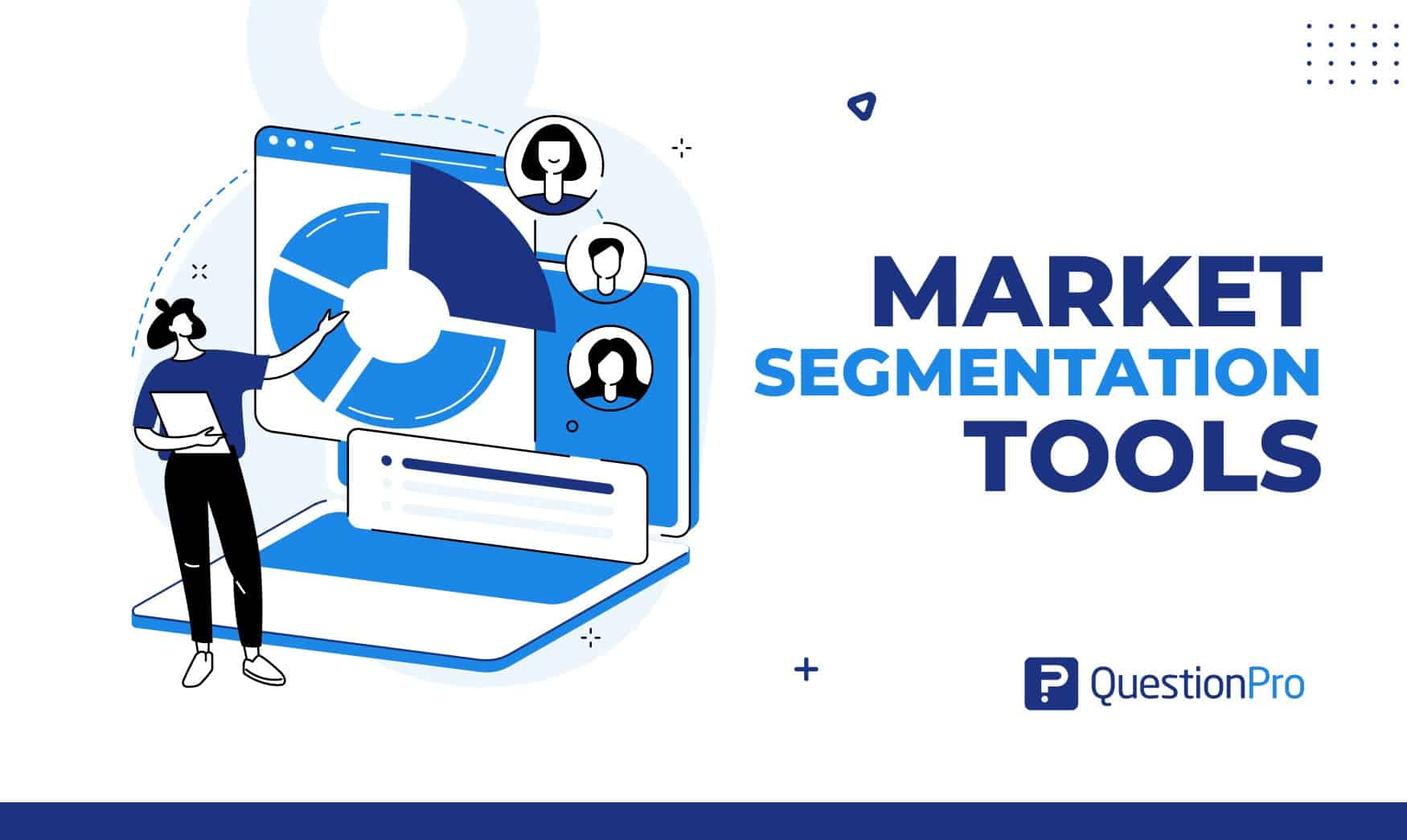
7 Best Market Segmentation Tools in 2024
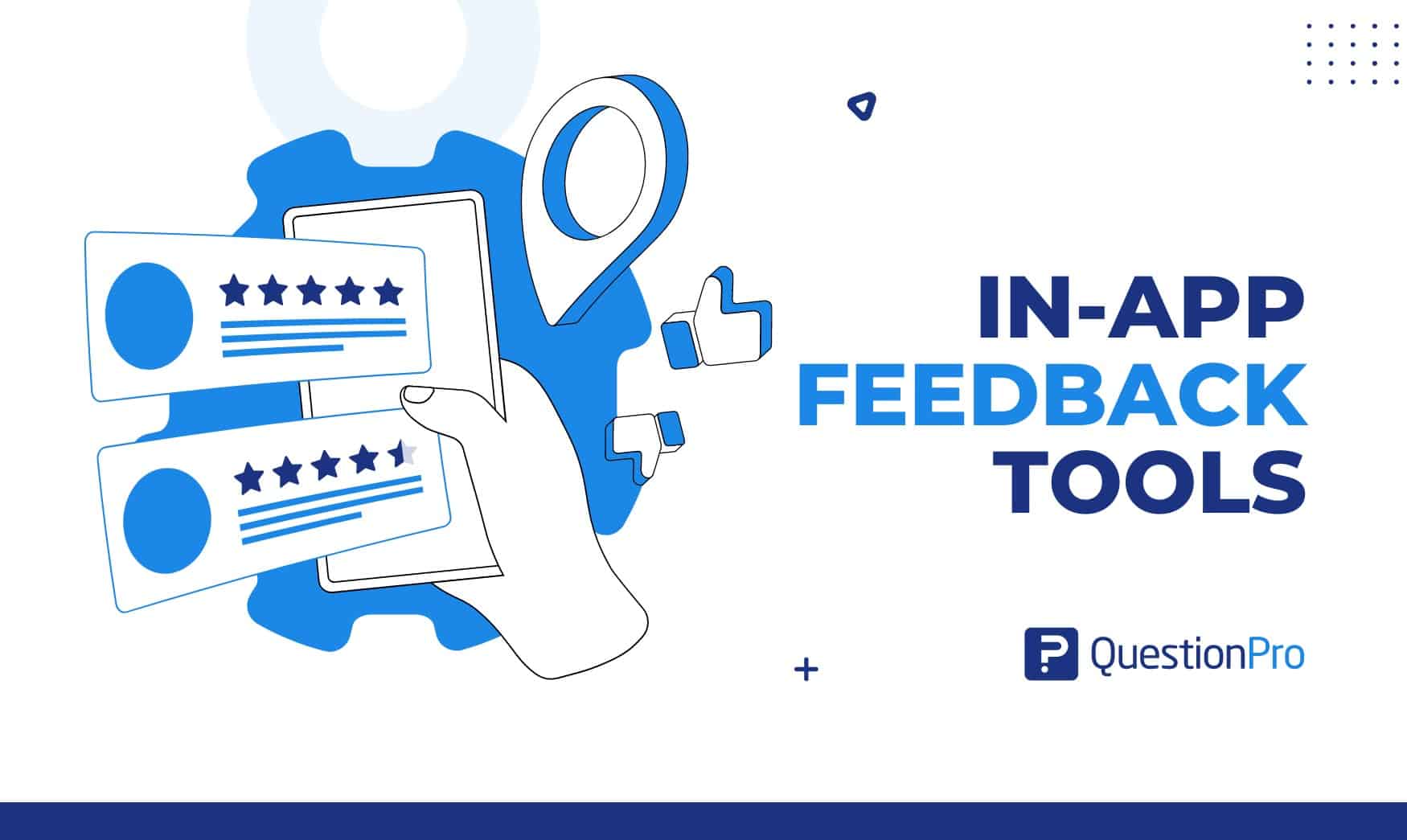
In-App Feedback Tools: How to Collect, Uses & 14 Best Tools
Mar 29, 2024
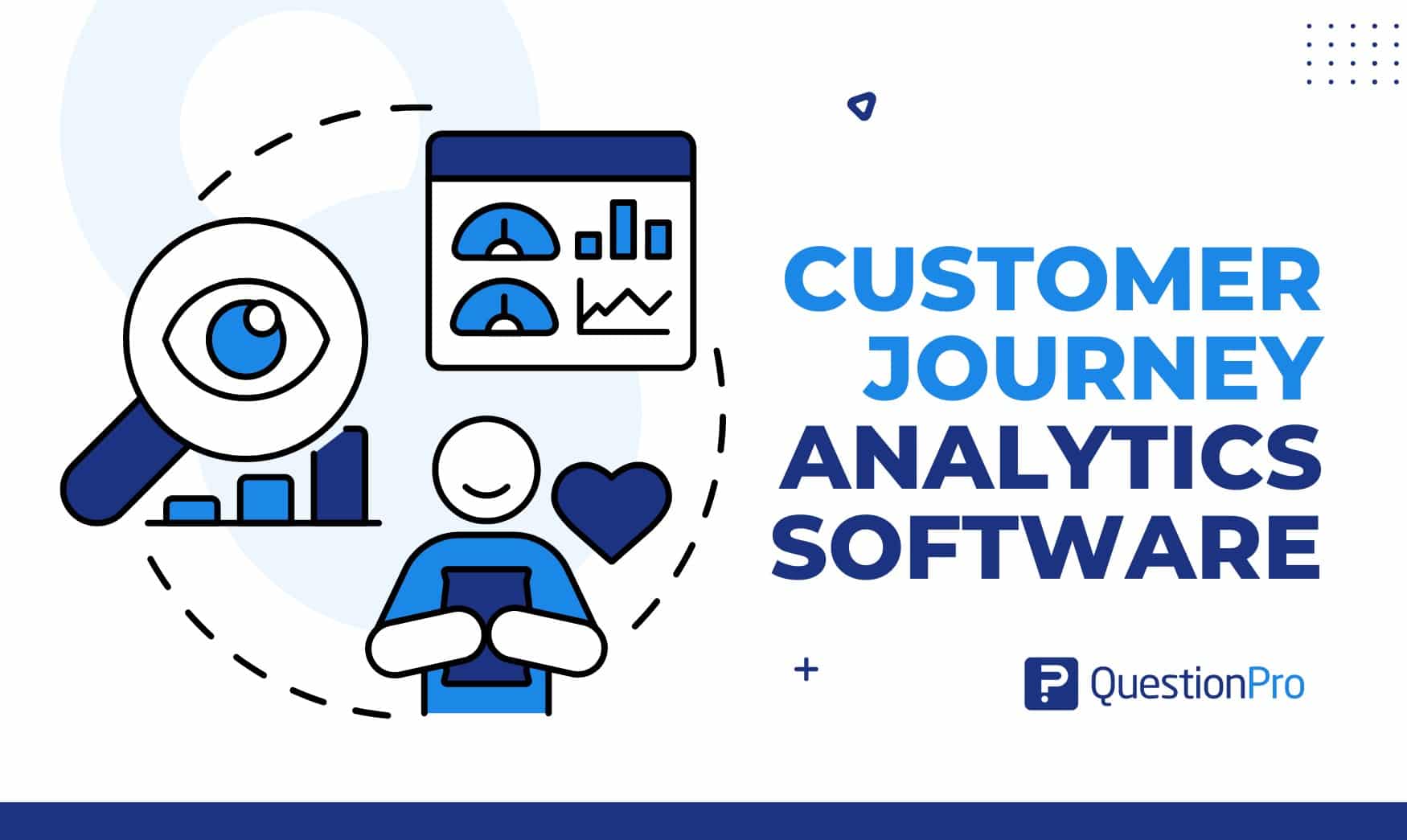
11 Best Customer Journey Analytics Software in 2024
Other categories.
- Academic Research
- Artificial Intelligence
- Assessments
- Brand Awareness
- Case Studies
- Communities
- Consumer Insights
- Customer effort score
- Customer Engagement
- Customer Experience
- Customer Loyalty
- Customer Research
- Customer Satisfaction
- Employee Benefits
- Employee Engagement
- Employee Retention
- Friday Five
- General Data Protection Regulation
- Insights Hub
- Life@QuestionPro
- Market Research
- Mobile diaries
- Mobile Surveys
- New Features
- Online Communities
- Question Types
- Questionnaire
- QuestionPro Products
- Release Notes
- Research Tools and Apps
- Revenue at Risk
- Survey Templates
- Training Tips
- Uncategorized
- Video Learning Series
- What’s Coming Up
- Workforce Intelligence
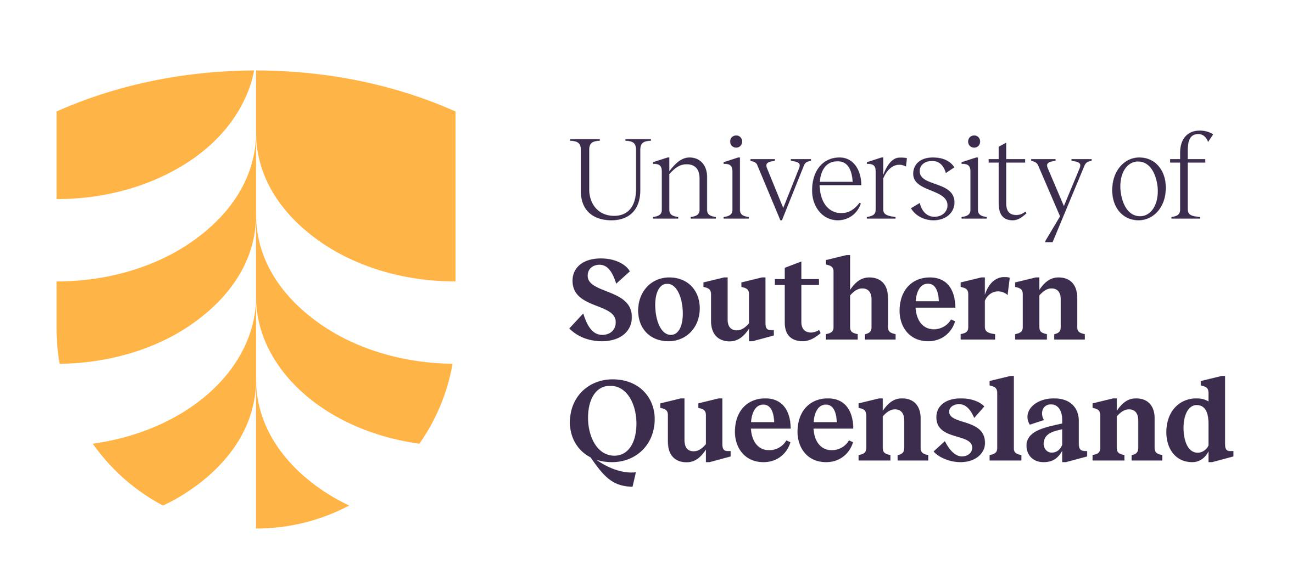
Want to create or adapt books like this? Learn more about how Pressbooks supports open publishing practices.
Sampling is the statistical process of selecting a subset—called a ‘sample’—of a population of interest for the purpose of making observations and statistical inferences about that population. Social science research is generally about inferring patterns of behaviours within specific populations. We cannot study entire populations because of feasibility and cost constraints, and hence, we must select a representative sample from the population of interest for observation and analysis. It is extremely important to choose a sample that is truly representative of the population so that the inferences derived from the sample can be generalised back to the population of interest. Improper and biased sampling is the primary reason for the often divergent and erroneous inferences reported in opinion polls and exit polls conducted by different polling groups such as CNN/Gallup Poll, ABC, and CBS, prior to every US Presidential election.
The sampling process
As Figure 8.1 shows, the sampling process comprises of several stages. The first stage is defining the target population. A population can be defined as all people or items ( unit of analysis ) with the characteristics that one wishes to study. The unit of analysis may be a person, group, organisation, country, object, or any other entity that you wish to draw scientific inferences about. Sometimes the population is obvious. For example, if a manufacturer wants to determine whether finished goods manufactured at a production line meet certain quality requirements or must be scrapped and reworked, then the population consists of the entire set of finished goods manufactured at that production facility. At other times, the target population may be a little harder to understand. If you wish to identify the primary drivers of academic learning among high school students, then what is your target population: high school students, their teachers, school principals, or parents? The right answer in this case is high school students, because you are interested in their performance, not the performance of their teachers, parents, or schools. Likewise, if you wish to analyse the behaviour of roulette wheels to identify biased wheels, your population of interest is not different observations from a single roulette wheel, but different roulette wheels (i.e., their behaviour over an infinite set of wheels).
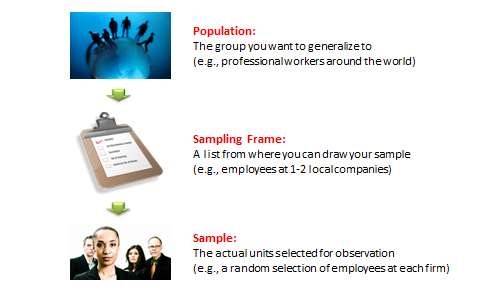
The second step in the sampling process is to choose a sampling frame . This is an accessible section of the target population—usually a list with contact information—from where a sample can be drawn. If your target population is professional employees at work, because you cannot access all professional employees around the world, a more realistic sampling frame will be employee lists of one or two local companies that are willing to participate in your study. If your target population is organisations, then the Fortune 500 list of firms or the Standard & Poor’s (S&P) list of firms registered with the New York Stock exchange may be acceptable sampling frames.
Note that sampling frames may not entirely be representative of the population at large, and if so, inferences derived by such a sample may not be generalisable to the population. For instance, if your target population is organisational employees at large (e.g., you wish to study employee self-esteem in this population) and your sampling frame is employees at automotive companies in the American Midwest, findings from such groups may not even be generalisable to the American workforce at large, let alone the global workplace. This is because the American auto industry has been under severe competitive pressures for the last 50 years and has seen numerous episodes of reorganisation and downsizing, possibly resulting in low employee morale and self-esteem. Furthermore, the majority of the American workforce is employed in service industries or in small businesses, and not in automotive industry. Hence, a sample of American auto industry employees is not particularly representative of the American workforce. Likewise, the Fortune 500 list includes the 500 largest American enterprises, which is not representative of all American firms, most of which are medium or small sized firms rather than large firms, and is therefore, a biased sampling frame. In contrast, the S&P list will allow you to select large, medium, and/or small companies, depending on whether you use the S&P LargeCap, MidCap, or SmallCap lists, but includes publicly traded firms (and not private firms) and is hence still biased. Also note that the population from which a sample is drawn may not necessarily be the same as the population about which we actually want information. For example, if a researcher wants to examine the success rate of a new ‘quit smoking’ program, then the target population is the universe of smokers who had access to this program, which may be an unknown population. Hence, the researcher may sample patients arriving at a local medical facility for smoking cessation treatment, some of whom may not have had exposure to this particular ‘quit smoking’ program, in which case, the sampling frame does not correspond to the population of interest.
The last step in sampling is choosing a sample from the sampling frame using a well-defined sampling technique. Sampling techniques can be grouped into two broad categories: probability (random) sampling and non-probability sampling. Probability sampling is ideal if generalisability of results is important for your study, but there may be unique circumstances where non-probability sampling can also be justified. These techniques are discussed in the next two sections.
Probability sampling
Probability sampling is a technique in which every unit in the population has a chance (non-zero probability) of being selected in the sample, and this chance can be accurately determined. Sample statistics thus produced, such as sample mean or standard deviation, are unbiased estimates of population parameters, as long as the sampled units are weighted according to their probability of selection. All probability sampling have two attributes in common: every unit in the population has a known non-zero probability of being sampled, and the sampling procedure involves random selection at some point. The different types of probability sampling techniques include:

Stratified sampling. In stratified sampling, the sampling frame is divided into homogeneous and non-overlapping subgroups (called ‘strata’), and a simple random sample is drawn within each subgroup. In the previous example of selecting 200 firms from a list of 1,000 firms, you can start by categorising the firms based on their size as large (more than 500 employees), medium (between 50 and 500 employees), and small (less than 50 employees). You can then randomly select 67 firms from each subgroup to make up your sample of 200 firms. However, since there are many more small firms in a sampling frame than large firms, having an equal number of small, medium, and large firms will make the sample less representative of the population (i.e., biased in favour of large firms that are fewer in number in the target population). This is called non-proportional stratified sampling because the proportion of the sample within each subgroup does not reflect the proportions in the sampling frame—or the population of interest—and the smaller subgroup (large-sized firms) is oversampled . An alternative technique will be to select subgroup samples in proportion to their size in the population. For instance, if there are 100 large firms, 300 mid-sized firms, and 600 small firms, you can sample 20 firms from the ‘large’ group, 60 from the ‘medium’ group and 120 from the ‘small’ group. In this case, the proportional distribution of firms in the population is retained in the sample, and hence this technique is called proportional stratified sampling. Note that the non-proportional approach is particularly effective in representing small subgroups, such as large-sized firms, and is not necessarily less representative of the population compared to the proportional approach, as long as the findings of the non-proportional approach are weighted in accordance to a subgroup’s proportion in the overall population.
Cluster sampling. If you have a population dispersed over a wide geographic region, it may not be feasible to conduct a simple random sampling of the entire population. In such case, it may be reasonable to divide the population into ‘clusters’—usually along geographic boundaries—randomly sample a few clusters, and measure all units within that cluster. For instance, if you wish to sample city governments in the state of New York, rather than travel all over the state to interview key city officials (as you may have to do with a simple random sample), you can cluster these governments based on their counties, randomly select a set of three counties, and then interview officials from every office in those counties. However, depending on between-cluster differences, the variability of sample estimates in a cluster sample will generally be higher than that of a simple random sample, and hence the results are less generalisable to the population than those obtained from simple random samples.
Matched-pairs sampling. Sometimes, researchers may want to compare two subgroups within one population based on a specific criterion. For instance, why are some firms consistently more profitable than other firms? To conduct such a study, you would have to categorise a sampling frame of firms into ‘high profitable’ firms and ‘low profitable firms’ based on gross margins, earnings per share, or some other measure of profitability. You would then select a simple random sample of firms in one subgroup, and match each firm in this group with a firm in the second subgroup, based on its size, industry segment, and/or other matching criteria. Now, you have two matched samples of high-profitability and low-profitability firms that you can study in greater detail. Matched-pairs sampling techniques are often an ideal way of understanding bipolar differences between different subgroups within a given population.
Multi-stage sampling. The probability sampling techniques described previously are all examples of single-stage sampling techniques. Depending on your sampling needs, you may combine these single-stage techniques to conduct multi-stage sampling. For instance, you can stratify a list of businesses based on firm size, and then conduct systematic sampling within each stratum. This is a two-stage combination of stratified and systematic sampling. Likewise, you can start with a cluster of school districts in the state of New York, and within each cluster, select a simple random sample of schools. Within each school, you can select a simple random sample of grade levels, and within each grade level, you can select a simple random sample of students for study. In this case, you have a four-stage sampling process consisting of cluster and simple random sampling.
Non-probability sampling
Non-probability sampling is a sampling technique in which some units of the population have zero chance of selection or where the probability of selection cannot be accurately determined. Typically, units are selected based on certain non-random criteria, such as quota or convenience. Because selection is non-random, non-probability sampling does not allow the estimation of sampling errors, and may be subjected to a sampling bias. Therefore, information from a sample cannot be generalised back to the population. Types of non-probability sampling techniques include:
Convenience sampling. Also called accidental or opportunity sampling, this is a technique in which a sample is drawn from that part of the population that is close to hand, readily available, or convenient. For instance, if you stand outside a shopping centre and hand out questionnaire surveys to people or interview them as they walk in, the sample of respondents you will obtain will be a convenience sample. This is a non-probability sample because you are systematically excluding all people who shop at other shopping centres. The opinions that you would get from your chosen sample may reflect the unique characteristics of this shopping centre such as the nature of its stores (e.g., high end-stores will attract a more affluent demographic), the demographic profile of its patrons, or its location (e.g., a shopping centre close to a university will attract primarily university students with unique purchasing habits), and therefore may not be representative of the opinions of the shopper population at large. Hence, the scientific generalisability of such observations will be very limited. Other examples of convenience sampling are sampling students registered in a certain class or sampling patients arriving at a certain medical clinic. This type of sampling is most useful for pilot testing, where the goal is instrument testing or measurement validation rather than obtaining generalisable inferences.
Quota sampling. In this technique, the population is segmented into mutually exclusive subgroups (just as in stratified sampling), and then a non-random set of observations is chosen from each subgroup to meet a predefined quota. In proportional quota sampling , the proportion of respondents in each subgroup should match that of the population. For instance, if the American population consists of 70 per cent Caucasians, 15 per cent Hispanic-Americans, and 13 per cent African-Americans, and you wish to understand their voting preferences in an sample of 98 people, you can stand outside a shopping centre and ask people their voting preferences. But you will have to stop asking Hispanic-looking people when you have 15 responses from that subgroup (or African-Americans when you have 13 responses) even as you continue sampling other ethnic groups, so that the ethnic composition of your sample matches that of the general American population.
Non-proportional quota sampling is less restrictive in that you do not have to achieve a proportional representation, but perhaps meet a minimum size in each subgroup. In this case, you may decide to have 50 respondents from each of the three ethnic subgroups (Caucasians, Hispanic-Americans, and African-Americans), and stop when your quota for each subgroup is reached. Neither type of quota sampling will be representative of the American population, since depending on whether your study was conducted in a shopping centre in New York or Kansas, your results may be entirely different. The non-proportional technique is even less representative of the population, but may be useful in that it allows capturing the opinions of small and under-represented groups through oversampling.
Expert sampling. This is a technique where respondents are chosen in a non-random manner based on their expertise on the phenomenon being studied. For instance, in order to understand the impacts of a new governmental policy such as the Sarbanes-Oxley Act, you can sample a group of corporate accountants who are familiar with this Act. The advantage of this approach is that since experts tend to be more familiar with the subject matter than non-experts, opinions from a sample of experts are more credible than a sample that includes both experts and non-experts, although the findings are still not generalisable to the overall population at large.
Snowball sampling. In snowball sampling, you start by identifying a few respondents that match the criteria for inclusion in your study, and then ask them to recommend others they know who also meet your selection criteria. For instance, if you wish to survey computer network administrators and you know of only one or two such people, you can start with them and ask them to recommend others who also work in network administration. Although this method hardly leads to representative samples, it may sometimes be the only way to reach hard-to-reach populations or when no sampling frame is available.
Statistics of sampling
In the preceding sections, we introduced terms such as population parameter, sample statistic, and sampling bias. In this section, we will try to understand what these terms mean and how they are related to each other.
When you measure a certain observation from a given unit, such as a person’s response to a Likert-scaled item, that observation is called a response (see Figure 8.2). In other words, a response is a measurement value provided by a sampled unit. Each respondent will give you different responses to different items in an instrument. Responses from different respondents to the same item or observation can be graphed into a frequency distribution based on their frequency of occurrences. For a large number of responses in a sample, this frequency distribution tends to resemble a bell-shaped curve called a normal distribution , which can be used to estimate overall characteristics of the entire sample, such as sample mean (average of all observations in a sample) or standard deviation (variability or spread of observations in a sample). These sample estimates are called sample statistics (a ‘statistic’ is a value that is estimated from observed data). Populations also have means and standard deviations that could be obtained if we could sample the entire population. However, since the entire population can never be sampled, population characteristics are always unknown, and are called population parameters (and not ‘statistic’ because they are not statistically estimated from data). Sample statistics may differ from population parameters if the sample is not perfectly representative of the population. The difference between the two is called sampling error . Theoretically, if we could gradually increase the sample size so that the sample approaches closer and closer to the population, then sampling error will decrease and a sample statistic will increasingly approximate the corresponding population parameter.
If a sample is truly representative of the population, then the estimated sample statistics should be identical to the corresponding theoretical population parameters. How do we know if the sample statistics are at least reasonably close to the population parameters? Here, we need to understand the concept of a sampling distribution . Imagine that you took three different random samples from a given population, as shown in Figure 8.3, and for each sample, you derived sample statistics such as sample mean and standard deviation. If each random sample was truly representative of the population, then your three sample means from the three random samples will be identical—and equal to the population parameter—and the variability in sample means will be zero. But this is extremely unlikely, given that each random sample will likely constitute a different subset of the population, and hence, their means may be slightly different from each other. However, you can take these three sample means and plot a frequency histogram of sample means. If the number of such samples increases from three to 10 to 100, the frequency histogram becomes a sampling distribution. Hence, a sampling distribution is a frequency distribution of a sample statistic (like sample mean) from a set of samples , while the commonly referenced frequency distribution is the distribution of a response (observation) from a single sample . Just like a frequency distribution, the sampling distribution will also tend to have more sample statistics clustered around the mean (which presumably is an estimate of a population parameter), with fewer values scattered around the mean. With an infinitely large number of samples, this distribution will approach a normal distribution. The variability or spread of a sample statistic in a sampling distribution (i.e., the standard deviation of a sampling statistic) is called its standard error . In contrast, the term standard deviation is reserved for variability of an observed response from a single sample.
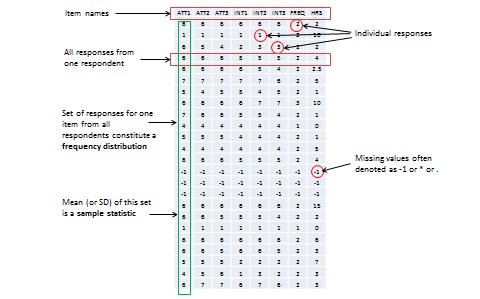
Social Science Research: Principles, Methods and Practices (Revised edition) Copyright © 2019 by Anol Bhattacherjee is licensed under a Creative Commons Attribution-NonCommercial-ShareAlike 4.0 International License , except where otherwise noted.
Share This Book

An official website of the United States government
The .gov means it’s official. Federal government websites often end in .gov or .mil. Before sharing sensitive information, make sure you’re on a federal government site.
The site is secure. The https:// ensures that you are connecting to the official website and that any information you provide is encrypted and transmitted securely.
- Publications
- Account settings
Preview improvements coming to the PMC website in October 2024. Learn More or Try it out now .
- Advanced Search
- Journal List
- Indian J Dermatol
- v.61(5); Sep-Oct 2016
Methodology Series Module 5: Sampling Strategies
Maninder singh setia.
Epidemiologist, MGM Institute of Health Sciences, Navi Mumbai, Maharashtra, India
Once the research question and the research design have been finalised, it is important to select the appropriate sample for the study. The method by which the researcher selects the sample is the ‘ Sampling Method’. There are essentially two types of sampling methods: 1) probability sampling – based on chance events (such as random numbers, flipping a coin etc.); and 2) non-probability sampling – based on researcher's choice, population that accessible & available. Some of the non-probability sampling methods are: purposive sampling, convenience sampling, or quota sampling. Random sampling method (such as simple random sample or stratified random sample) is a form of probability sampling. It is important to understand the different sampling methods used in clinical studies and mention this method clearly in the manuscript. The researcher should not misrepresent the sampling method in the manuscript (such as using the term ‘ random sample’ when the researcher has used convenience sample). The sampling method will depend on the research question. For instance, the researcher may want to understand an issue in greater detail for one particular population rather than worry about the ‘ generalizability’ of these results. In such a scenario, the researcher may want to use ‘ purposive sampling’ for the study.
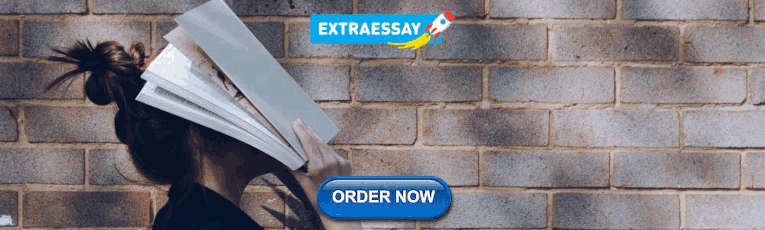
Introduction
The purpose of this section is to discuss various sampling methods used in research. After finalizing the research question and the research design, it is important to select the appropriate sample for the study. The method by which the researcher selects the sample is the “Sampling Method” [ Figure 1 ].

Flowchart from “Universe” to “Sampling Method”
Why do we need to sample?
Let us answer this research question: What is the prevalence of HIV in the adult Indian population?
The best response to this question will be obtained when we test every adult Indian for HIV. However, this is logistically difficult, time consuming, expensive, and difficult for a single researcher – do not forget about ethics of conducting such a study. The government usually conducts an exercise regularly to measure certain outcomes in the whole population – ”the census.” However, as researchers, we often have limited time and resources. Hence, we will have to select a few adult Indians who will consent to be a part of the study. We will test them for HIV and present out results (as our estimates of HIV prevalence). These selected individuals are called our “sample.” We hope that we have selected the appropriate sample that is required to answer our research question.
The researcher should clearly and explicitly mention the sampling method in the manuscript. The description of these helps the reviewers and readers assess the validity and generalizability of the results. Furthermore, the authors should also acknowledge the limitations of their sampling method and its effects on estimated obtained in the study.
Types of Methods
We will try to understand some of these sampling methods that are commonly used in clinical research. There are essentially two types of sampling methods: (1) Probability sampling – based on chance events (such as random numbers, flipping a coin, etc.) and (2) nonprobability sampling – based on researcher's choice, population that accessible and available.
What is a “convenience sample?”
Research question: How many patients with psoriasis also have high cholesterol levels (according to our definition)?
We plan to conduct the study in the outpatient department of our hospital.
This is a common scenario for clinical studies. The researcher recruits the participants who are easily accessible in a clinical setting – this type of sample is called a “convenience sample.” Furthermore, in such a clinic-based setting, the researcher will approach all the psoriasis patients that he/she comes across. They are informed about the study, and all those who consent to be the study are evaluated for eligibility. If they meet the inclusion criteria (and need not be excluded as per the criteria), they are recruited for the study. Thus, this will be “consecutive consenting sample.”
This method is relatively easy and is one of the common types of sampling methods used (particularly in postgraduate dissertations).
Since this is clinic-based sample, the estimates from such a study may not necessarily be generalizable to the larger population. To begin with, the patients who access healthcare potentially have a different “health-seeking behavior” compared with those who do not access health in these settings. Furthermore, many of the clinical cases in tertiary care centers may be severe, complicated, or recalcitrant. Thus, the estimates of biological parameters or outcomes may be different in these compared with the general population. The researcher should clearly discuss in the manuscript/report as to how the convenience sample may have biased the estimates (for example: Overestimated or underestimated the outcome in the population studied).
What is a “random sample?”
A “random sample” is a probability sample where every individual has an equal and independent probability of being selected in the sample.
Please note that “random sample” does not mean arbitrary sample. For example, if the researcher selects 10–12 individuals from the waiting area (without any structure), it is not a random sample. Randomization is a specific process, and only samples that are recruited using this process is a “random sample.”
What is a “simple random sample?”
Let us recruit a “simple random sample” in the above example. The center only allows a fixed number of patients every day. All the patients have to confirm the appointment a day in advance and should present in the clinic between 9 and 9:30 a.m. for the appointment. Thus, by 9:30 a.m., you will all have all the individuals who will be examined day.
We wish to select 50% of these patients for posttreatment survey.
- Make a list of all the patients present at 9:30 a.m.
- Give a number to each individual
- Use a “randomization method” to select five of these numbers. Although “random tables” have been used as a method of randomization, currently, many researchers use “computer-generated lists for random selection” of participants. Most of the statistical packages have programs for random selection of population. Please state the method that you have used for random selection in the manuscript
- Recruit the individuals whose numbers have been selected by the randomization method.
The process is described in Figure 2 .

Representation of Simple Random Sample
What is a major issue with this recruitment process?
As you may notice, “only males” have been recruited for the study. This scenario is possible in a simple random sample selection.
This is a limitation of this type of sampling method – population units which are smaller in number in the sampling frame may be underrepresented in this sample.
What is “stratified sample?”
In a stratified sample, the population is divided into two or more similar groups (based on demographic or clinical characteristics). The sample is recruited from each stratum. The researcher may use a simple random sample procedure within each stratum.
Let us address the limitation in the above example (selection of 50% of the participants for postprocedure survey).
- Divide the list into two strata: Males and females
- Use a “randomization method” to select three numbers among males and two numbers among females. As discussed earlier, the researcher may use random tables or computer generated random selection. Please state the method that you have used for random selection in the manuscript
The process is described in Figure 3 .

Representation of Stratified Random Sample
Thus, with this sampling method, we ensure that people from both sexes are included in the sample. This type of sampling method is used for sampling when we want to ensure that minority populations (in number) are adequately represented in the sample.
Kindly note that in this example, we sampled 50% of the population in each stratum. However, the researcher may oversample in one particular stratum and under-sample in the other. For instance, in this example, we may have taken three females and three males (if want to ensure equal representation of both). All this should be discussed explicitly in methods.
What is a “systematic sample?”
Sometimes, the researcher may decide to include study participants using a fixed pattern. For example, the researcher may recruit every second patient, or every patient whose registration ends with an even number or those who are admitted in certain days of the week (Tuesday/Thursday/Saturday). This type of sample is generally easy to implement. However, a lot of the recruitments are based on the researcher and may lead to selection bias. Furthermore, patients who come to the hospital may differ on different days of the week. For example, a higher proportion of working individuals may access the hospital on Saturdays.
This is not a “random sample.” Please do not write that “we selected the participants using a random sample method” if you have selected the sample systematically.
Another type of sampling discussed by some authors is “systematic random sample.” The steps for this method are:
- Make a list of all the potential recruits
- Using a random method (described earlier) to select a starting point (example number 4)
- Select this number and every fifth number from this starting point. Thus, the researcher will select number 9, 14, and so on.
Please note that the “skip” depends on the total number of potential participants and the total sample size. For instance, you have a total of fifty potential participants and you wish to recruit ten participants, do not skip to every 10 th patient.
Aday (1996) states that the skip depends on the total number of participants and the total sample size required.
- Fraction = total number of participants/total sample size
- In the above example, it will be 50/10 = 5
- Thus, using a random table or computer-generated random number selection, the researcher will select a random number from 1 to 5
- The number selected in two
- The researcher selects the second patient
- The next patient will be the fifth patient after patient number two – patient number 7
- The next patient will be patient number 12 and so on.
What is a “cluster sample?”
For some studies, the sample is selected from larger units or “clusters.” This type of method is generally used for “community-based studies.”
Research question: What is the prevalence of dermatological conditions in school children in city XXXXX?
In this study, we will select students from multiple schools. Thus, each school becomes one cluster. Each individual child in the school has much in common with other children in the same school compared with children from other schools (for example, they are more likely to have the same socioeconomic background). Thus, these children are recruited from the same cluster.
If the researcher uses “cluster sample,” he/she also performs “cluster analysis.” The statistical methods for these are different compared with nonclustered analysis (the methods we use commonly).
What is a “multistage sample?”
In many studies, we have to combine multiple methods for the appropriate and required sample.
Let us use a multistage sample to answer this research question.
Research question: What is the prevalence of dermatological conditions in school children in city XXXXX? (Assumption: The city is divided into four zones).
We have a list of all the schools in the city. How do we sample them?
Method 1: Select 10% of the schools using “simple random sample” method.
Question: What is the problem with this type of method?
Answer: As discussed earlier, it is possible that we may miss most of the schools from one particular zone.
However, we are interested to ensure that all zones are adequately represented in the sample.
- Stage 1: List all the schools in all zones
- Stage 2: Select 10% of schools from each zone using “random selection method” (first stratum)
- Stage 3: List all the students in Grade VIII, IX, and X(population of interest) in each school (second stratum)
- Stage 4: Create a separate list for males and females in each grade in each school (third stratum)
- Stage 5: Select 10% of males and females in each grade in each school.
Please note that this is just an example. You may have to change the proportion selected from each stratum based on the sample size and the total number of individuals in each stratum.
What are other types of sampling methods?
Although these are the common types of sampling methods that we use in clinical studies, we have also listed some other sampling methods in Table 1 .
Some other types of sampling methods

- It is important to understand the different sampling methods used in clinical studies. As stated earlier, please mention this method clearly in the manuscript
- Do not misrepresent the sampling method. For example, if you have not used “random method” for selection, do not state it in the manuscript
- Sometimes, the researcher may want to understand an issue in greater detail for one particular population rather than worry about the “generalizability” of these results. In such a scenario, the researcher may want to use ‘purposive sampling’.
Financial support and sponsorship
Conflicts of interest.
There are no conflicts of interest.
Bibliography
About The Author

Silvia Valcheva
Silvia Valcheva is a digital marketer with over a decade of experience creating content for the tech industry. She has a strong passion for writing about emerging software and technologies such as big data, AI (Artificial Intelligence), IoT (Internet of Things), process automation, etc.
thank you.. helped me a lot..

Glad to help!
Thankyou so much for this info. It helped me a lot
Leave a Reply Cancel Reply
This site uses Akismet to reduce spam. Learn how your comment data is processed .
- Math Article
Sampling Methods
We know that statistical research helps in drawing several conclusions based on the requirement of the experts. This uses the data collected for a specific purpose. We can collect the data using various sampling methods in statistics. However, the type of sampling method is chosen based on the objective of the statistical research. The statistical research is of two forms:
- In the first form, each domain is studied, and the result can be obtained by computing the sum of all units.
- In the second form, only a unit in the field of the survey is taken. It represents the domain. The result of these samples extends to the domain. This type of study is known as the sample survey.
In this article, let us discuss the different sampling methods in research such as probability sampling and non-probability sampling methods and various methods involved in those two approaches in detail.
What are the sampling methods or Sampling Techniques?
In Statistics , the sampling method or sampling technique is the process of studying the population by gathering information and analyzing that data. It is the basis of the data where the sample space is enormous .
There are several different sampling techniques available, and they can be subdivided into two groups. All these methods of sampling may involve specifically targeting hard or approach to reach groups.
Types of Sampling Method
In Statistics, there are different sampling techniques available to get relevant results from the population. The two different types of sampling methods are::
- Probability Sampling
- Non-probability Sampling
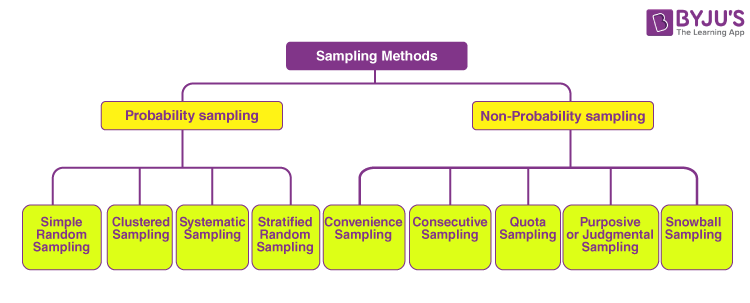
Also, read: Sample statistic
What is Probability Sampling?
The probability sampling method utilizes some form of random selection. In this method, all the eligible individuals have a chance of selecting the sample from the whole sample space. This method is more time consuming and expensive than the non-probability sampling method. The benefit of using probability sampling is that it guarantees the sample that should be the representative of the population.
Probability Sampling Types
Probability Sampling methods are further classified into different types, such as simple random sampling, systematic sampling, stratified sampling, and clustered sampling. Let us discuss the different types of probability sampling methods along with illustrative examples here in detail.
Simple Random Sampling
In simple random sampling technique, every item in the population has an equal and likely chance of being selected in the sample. Since the item selection entirely depends on the chance, this method is known as “ Method of chance Selection ”. As the sample size is large, and the item is chosen randomly, it is known as “ Representative Sampling ”.
Suppose we want to select a simple random sample of 200 students from a school. Here, we can assign a number to every student in the school database from 1 to 500 and use a random number generator to select a sample of 200 numbers.
Systematic Sampling
In the systematic sampling method, the items are selected from the target population by selecting the random selection point and selecting the other methods after a fixed sample interval. It is calculated by dividing the total population size by the desired population size.
Suppose the names of 300 students of a school are sorted in the reverse alphabetical order. To select a sample in a systematic sampling method, we have to choose some 15 students by randomly selecting a starting number, say 5. From number 5 onwards, will select every 15th person from the sorted list. Finally, we can end up with a sample of some students.
Stratified Sampling
In a stratified sampling method, the total population is divided into smaller groups to complete the sampling process. The small group is formed based on a few characteristics in the population. After separating the population into a smaller group, the statisticians randomly select the sample.
For example, there are three bags (A, B and C), each with different balls. Bag A has 50 balls, bag B has 100 balls, and bag C has 200 balls. We have to choose a sample of balls from each bag proportionally. Suppose 5 balls from bag A, 10 balls from bag B and 20 balls from bag C.
Clustered Sampling
In the clustered sampling method, the cluster or group of people are formed from the population set. The group has similar significatory characteristics. Also, they have an equal chance of being a part of the sample. This method uses simple random sampling for the cluster of population.
An educational institution has ten branches across the country with almost the number of students. If we want to collect some data regarding facilities and other things, we can’t travel to every unit to collect the required data. Hence, we can use random sampling to select three or four branches as clusters.
All these four methods can be understood in a better manner with the help of the figure given below. The figure contains various examples of how samples will be taken from the population using different techniques.
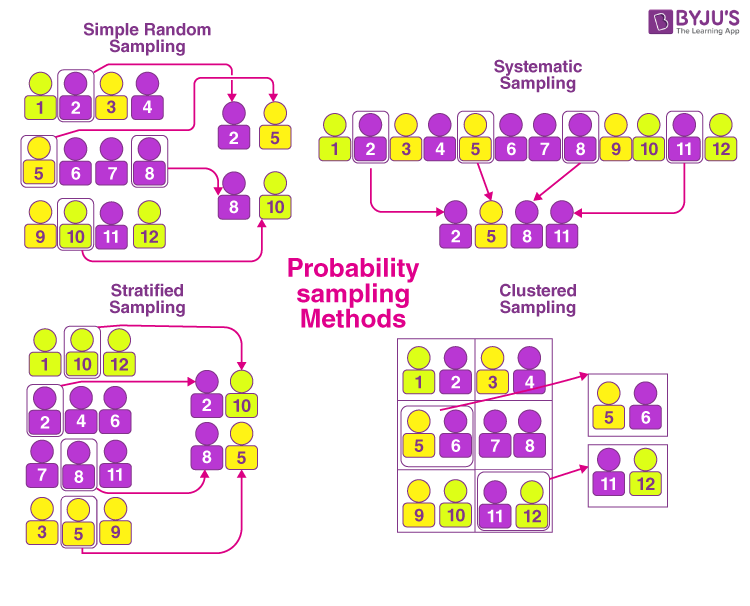
What is Non-Probability Sampling?
The non-probability sampling method is a technique in which the researcher selects the sample based on subjective judgment rather than the random selection. In this method, not all the members of the population have a chance to participate in the study.
Non-Probability Sampling Types
Non-probability Sampling methods are further classified into different types, such as convenience sampling, consecutive sampling, quota sampling, judgmental sampling, snowball sampling. Here, let us discuss all these types of non-probability sampling in detail.
Convenience Sampling
In a convenience sampling method, the samples are selected from the population directly because they are conveniently available for the researcher. The samples are easy to select, and the researcher did not choose the sample that outlines the entire population.
In researching customer support services in a particular region, we ask your few customers to complete a survey on the products after the purchase. This is a convenient way to collect data. Still, as we only surveyed customers taking the same product. At the same time, the sample is not representative of all the customers in that area.
Consecutive Sampling
Consecutive sampling is similar to convenience sampling with a slight variation. The researcher picks a single person or a group of people for sampling. Then the researcher researches for a period of time to analyze the result and move to another group if needed.
Quota Sampling
In the quota sampling method, the researcher forms a sample that involves the individuals to represent the population based on specific traits or qualities. The researcher chooses the sample subsets that bring the useful collection of data that generalizes the ent ire population.
Learn more about quota sampling here.
Purposive or Judgmental Sampling
In purposive sampling, the samples are selected only based on the researcher’s knowledge. As their knowledge is instrumental in creating the samples, there are the chances of obtaining highly accurate answers with a minimum marginal error. It is also known as judgmental sampling or authoritative sampling.
Snowball Sampling
Snowball sampl ing is also known as a chain- referral sampling technique. In this method, the samples have traits that are difficult to find. So, each identified member of a population is asked to find the other sampling units. Those sampling units also belong to the same targeted population.
Probability sampling vs Non-probability Sampling Methods
The below table shows a few differences between probability sampling methods and non-probability sampling methods.
Frequently Asked Questions on Sampling Methods
What are sampling methods in statistics, what are the methods of probability sampling, what are the non-probability sampling methods, what is an example of simple random sampling, how do you collect a convenience sample.
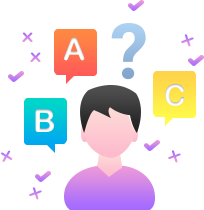
Put your understanding of this concept to test by answering a few MCQs. Click ‘Start Quiz’ to begin!
Select the correct answer and click on the “Finish” button Check your score and answers at the end of the quiz
Visit BYJU’S for all Maths related queries and study materials
Your result is as below
Request OTP on Voice Call

- Share Share
Register with BYJU'S & Download Free PDFs
Register with byju's & watch live videos.

- Privacy Policy
Buy Me a Coffee

Home » Research Methodology – Types, Examples and writing Guide
Research Methodology – Types, Examples and writing Guide
Table of Contents

Research Methodology
Definition:
Research Methodology refers to the systematic and scientific approach used to conduct research, investigate problems, and gather data and information for a specific purpose. It involves the techniques and procedures used to identify, collect , analyze , and interpret data to answer research questions or solve research problems . Moreover, They are philosophical and theoretical frameworks that guide the research process.
Structure of Research Methodology
Research methodology formats can vary depending on the specific requirements of the research project, but the following is a basic example of a structure for a research methodology section:
I. Introduction
- Provide an overview of the research problem and the need for a research methodology section
- Outline the main research questions and objectives
II. Research Design
- Explain the research design chosen and why it is appropriate for the research question(s) and objectives
- Discuss any alternative research designs considered and why they were not chosen
- Describe the research setting and participants (if applicable)
III. Data Collection Methods
- Describe the methods used to collect data (e.g., surveys, interviews, observations)
- Explain how the data collection methods were chosen and why they are appropriate for the research question(s) and objectives
- Detail any procedures or instruments used for data collection
IV. Data Analysis Methods
- Describe the methods used to analyze the data (e.g., statistical analysis, content analysis )
- Explain how the data analysis methods were chosen and why they are appropriate for the research question(s) and objectives
- Detail any procedures or software used for data analysis
V. Ethical Considerations
- Discuss any ethical issues that may arise from the research and how they were addressed
- Explain how informed consent was obtained (if applicable)
- Detail any measures taken to ensure confidentiality and anonymity
VI. Limitations
- Identify any potential limitations of the research methodology and how they may impact the results and conclusions
VII. Conclusion
- Summarize the key aspects of the research methodology section
- Explain how the research methodology addresses the research question(s) and objectives
Research Methodology Types
Types of Research Methodology are as follows:
Quantitative Research Methodology
This is a research methodology that involves the collection and analysis of numerical data using statistical methods. This type of research is often used to study cause-and-effect relationships and to make predictions.
Qualitative Research Methodology
This is a research methodology that involves the collection and analysis of non-numerical data such as words, images, and observations. This type of research is often used to explore complex phenomena, to gain an in-depth understanding of a particular topic, and to generate hypotheses.
Mixed-Methods Research Methodology
This is a research methodology that combines elements of both quantitative and qualitative research. This approach can be particularly useful for studies that aim to explore complex phenomena and to provide a more comprehensive understanding of a particular topic.
Case Study Research Methodology
This is a research methodology that involves in-depth examination of a single case or a small number of cases. Case studies are often used in psychology, sociology, and anthropology to gain a detailed understanding of a particular individual or group.
Action Research Methodology
This is a research methodology that involves a collaborative process between researchers and practitioners to identify and solve real-world problems. Action research is often used in education, healthcare, and social work.
Experimental Research Methodology
This is a research methodology that involves the manipulation of one or more independent variables to observe their effects on a dependent variable. Experimental research is often used to study cause-and-effect relationships and to make predictions.
Survey Research Methodology
This is a research methodology that involves the collection of data from a sample of individuals using questionnaires or interviews. Survey research is often used to study attitudes, opinions, and behaviors.
Grounded Theory Research Methodology
This is a research methodology that involves the development of theories based on the data collected during the research process. Grounded theory is often used in sociology and anthropology to generate theories about social phenomena.
Research Methodology Example
An Example of Research Methodology could be the following:
Research Methodology for Investigating the Effectiveness of Cognitive Behavioral Therapy in Reducing Symptoms of Depression in Adults
Introduction:
The aim of this research is to investigate the effectiveness of cognitive-behavioral therapy (CBT) in reducing symptoms of depression in adults. To achieve this objective, a randomized controlled trial (RCT) will be conducted using a mixed-methods approach.
Research Design:
The study will follow a pre-test and post-test design with two groups: an experimental group receiving CBT and a control group receiving no intervention. The study will also include a qualitative component, in which semi-structured interviews will be conducted with a subset of participants to explore their experiences of receiving CBT.
Participants:
Participants will be recruited from community mental health clinics in the local area. The sample will consist of 100 adults aged 18-65 years old who meet the diagnostic criteria for major depressive disorder. Participants will be randomly assigned to either the experimental group or the control group.
Intervention :
The experimental group will receive 12 weekly sessions of CBT, each lasting 60 minutes. The intervention will be delivered by licensed mental health professionals who have been trained in CBT. The control group will receive no intervention during the study period.
Data Collection:
Quantitative data will be collected through the use of standardized measures such as the Beck Depression Inventory-II (BDI-II) and the Generalized Anxiety Disorder-7 (GAD-7). Data will be collected at baseline, immediately after the intervention, and at a 3-month follow-up. Qualitative data will be collected through semi-structured interviews with a subset of participants from the experimental group. The interviews will be conducted at the end of the intervention period, and will explore participants’ experiences of receiving CBT.
Data Analysis:
Quantitative data will be analyzed using descriptive statistics, t-tests, and mixed-model analyses of variance (ANOVA) to assess the effectiveness of the intervention. Qualitative data will be analyzed using thematic analysis to identify common themes and patterns in participants’ experiences of receiving CBT.
Ethical Considerations:
This study will comply with ethical guidelines for research involving human subjects. Participants will provide informed consent before participating in the study, and their privacy and confidentiality will be protected throughout the study. Any adverse events or reactions will be reported and managed appropriately.
Data Management:
All data collected will be kept confidential and stored securely using password-protected databases. Identifying information will be removed from qualitative data transcripts to ensure participants’ anonymity.
Limitations:
One potential limitation of this study is that it only focuses on one type of psychotherapy, CBT, and may not generalize to other types of therapy or interventions. Another limitation is that the study will only include participants from community mental health clinics, which may not be representative of the general population.
Conclusion:
This research aims to investigate the effectiveness of CBT in reducing symptoms of depression in adults. By using a randomized controlled trial and a mixed-methods approach, the study will provide valuable insights into the mechanisms underlying the relationship between CBT and depression. The results of this study will have important implications for the development of effective treatments for depression in clinical settings.
How to Write Research Methodology
Writing a research methodology involves explaining the methods and techniques you used to conduct research, collect data, and analyze results. It’s an essential section of any research paper or thesis, as it helps readers understand the validity and reliability of your findings. Here are the steps to write a research methodology:
- Start by explaining your research question: Begin the methodology section by restating your research question and explaining why it’s important. This helps readers understand the purpose of your research and the rationale behind your methods.
- Describe your research design: Explain the overall approach you used to conduct research. This could be a qualitative or quantitative research design, experimental or non-experimental, case study or survey, etc. Discuss the advantages and limitations of the chosen design.
- Discuss your sample: Describe the participants or subjects you included in your study. Include details such as their demographics, sampling method, sample size, and any exclusion criteria used.
- Describe your data collection methods : Explain how you collected data from your participants. This could include surveys, interviews, observations, questionnaires, or experiments. Include details on how you obtained informed consent, how you administered the tools, and how you minimized the risk of bias.
- Explain your data analysis techniques: Describe the methods you used to analyze the data you collected. This could include statistical analysis, content analysis, thematic analysis, or discourse analysis. Explain how you dealt with missing data, outliers, and any other issues that arose during the analysis.
- Discuss the validity and reliability of your research : Explain how you ensured the validity and reliability of your study. This could include measures such as triangulation, member checking, peer review, or inter-coder reliability.
- Acknowledge any limitations of your research: Discuss any limitations of your study, including any potential threats to validity or generalizability. This helps readers understand the scope of your findings and how they might apply to other contexts.
- Provide a summary: End the methodology section by summarizing the methods and techniques you used to conduct your research. This provides a clear overview of your research methodology and helps readers understand the process you followed to arrive at your findings.
When to Write Research Methodology
Research methodology is typically written after the research proposal has been approved and before the actual research is conducted. It should be written prior to data collection and analysis, as it provides a clear roadmap for the research project.
The research methodology is an important section of any research paper or thesis, as it describes the methods and procedures that will be used to conduct the research. It should include details about the research design, data collection methods, data analysis techniques, and any ethical considerations.
The methodology should be written in a clear and concise manner, and it should be based on established research practices and standards. It is important to provide enough detail so that the reader can understand how the research was conducted and evaluate the validity of the results.
Applications of Research Methodology
Here are some of the applications of research methodology:
- To identify the research problem: Research methodology is used to identify the research problem, which is the first step in conducting any research.
- To design the research: Research methodology helps in designing the research by selecting the appropriate research method, research design, and sampling technique.
- To collect data: Research methodology provides a systematic approach to collect data from primary and secondary sources.
- To analyze data: Research methodology helps in analyzing the collected data using various statistical and non-statistical techniques.
- To test hypotheses: Research methodology provides a framework for testing hypotheses and drawing conclusions based on the analysis of data.
- To generalize findings: Research methodology helps in generalizing the findings of the research to the target population.
- To develop theories : Research methodology is used to develop new theories and modify existing theories based on the findings of the research.
- To evaluate programs and policies : Research methodology is used to evaluate the effectiveness of programs and policies by collecting data and analyzing it.
- To improve decision-making: Research methodology helps in making informed decisions by providing reliable and valid data.
Purpose of Research Methodology
Research methodology serves several important purposes, including:
- To guide the research process: Research methodology provides a systematic framework for conducting research. It helps researchers to plan their research, define their research questions, and select appropriate methods and techniques for collecting and analyzing data.
- To ensure research quality: Research methodology helps researchers to ensure that their research is rigorous, reliable, and valid. It provides guidelines for minimizing bias and error in data collection and analysis, and for ensuring that research findings are accurate and trustworthy.
- To replicate research: Research methodology provides a clear and detailed account of the research process, making it possible for other researchers to replicate the study and verify its findings.
- To advance knowledge: Research methodology enables researchers to generate new knowledge and to contribute to the body of knowledge in their field. It provides a means for testing hypotheses, exploring new ideas, and discovering new insights.
- To inform decision-making: Research methodology provides evidence-based information that can inform policy and decision-making in a variety of fields, including medicine, public health, education, and business.
Advantages of Research Methodology
Research methodology has several advantages that make it a valuable tool for conducting research in various fields. Here are some of the key advantages of research methodology:
- Systematic and structured approach : Research methodology provides a systematic and structured approach to conducting research, which ensures that the research is conducted in a rigorous and comprehensive manner.
- Objectivity : Research methodology aims to ensure objectivity in the research process, which means that the research findings are based on evidence and not influenced by personal bias or subjective opinions.
- Replicability : Research methodology ensures that research can be replicated by other researchers, which is essential for validating research findings and ensuring their accuracy.
- Reliability : Research methodology aims to ensure that the research findings are reliable, which means that they are consistent and can be depended upon.
- Validity : Research methodology ensures that the research findings are valid, which means that they accurately reflect the research question or hypothesis being tested.
- Efficiency : Research methodology provides a structured and efficient way of conducting research, which helps to save time and resources.
- Flexibility : Research methodology allows researchers to choose the most appropriate research methods and techniques based on the research question, data availability, and other relevant factors.
- Scope for innovation: Research methodology provides scope for innovation and creativity in designing research studies and developing new research techniques.
Research Methodology Vs Research Methods
About the author.
Muhammad Hassan
Researcher, Academic Writer, Web developer
You may also like

How to Cite Research Paper – All Formats and...

Data Collection – Methods Types and Examples

Delimitations in Research – Types, Examples and...

Research Paper Format – Types, Examples and...

Research Process – Steps, Examples and Tips

Research Design – Types, Methods and Examples

Comparison of three sequencing methods for identifying and quantifying antibiotic. resistance genes (ARGs) in sewage.
- Find this author on Google Scholar
- Find this author on PubMed
- Search for this author on this site
- For correspondence: [email protected]
- Info/History
- Supplementary material
- Preview PDF
Background: Globally, antimicrobial resistance (AMR) poses a critical threat, requiring robust surveillance methodologies to tackle the growing challenge of drug-resistant microbes. AMR is a huge challenge in India due to high disease burden, lack of etiology-based diagnostic tests and over the counter availability of antibiotics and inadequate treatment of wastewaters are important drivers of AMR in India. There is lack of effective surveillance platforms that monitor health-associated infections. This include developing an understanding on background levels of AMR in the environment and comparison of AMR monitoring methods. Objectives: This study evaluated the performance of three AMR sequencing methods, Illumina AmpliSeq AMR panel, QIAseq xHYB AMR panel and shotgun sequencing method for the detection of antimicrobial resistance genes (ARGs) in urban sewage. Our goal is to provide insights into the application and robustness of each sequencing method. Methods: We compare the prevalence, diversity, and composition of ARGs across sequencing method and by sample type (inlet vs outlet) in four sewage treatment plants (STP). Results: Regardless of the sequencing method used the dominant ARGs remained consistent, and their differential analysis showed consistent trends in detection of epidemiologically relevant ARGs. The cost-effectiveness analysis revealed comparable per-sample costs, with amplicon-based sequencing offering specificity for targeted genes, and shotgun sequencing uses a whole-genome sequencing approach that provides high-resolution taxonomic information for the characterisation of pathogens. This methodology can only detect ARGs that have been annotated in the reference database (e.g., CARD). Therefore, some novel types of ARGs present in the samples may be missed since the analysis is based on a similarity search. Differential abundance analysis to understand change in abundance in dominant ARGs from inlet to outlet of STP showed consistent trends across methods. However, with caution raised regarding potential artifacts introduced by enrichment steps in QIAseq xHYB AMR panel. Conclusion: The choice of panel used would be governed by the context of the study. Nonetheless, our exploratory study shows that the data gathered using different sequencing pipelines helps in quantifying the ARG burden in the environment. This information is crucial in understanding the spatio-temporal distribution of ARGs in different environment and could help in developing PCR-based approaches for targeted surveillance.
Competing Interest Statement
The authors have declared no competing interest.
Funding Statement
This work has been supported by the funding from Tata Trust to Tata Institute of Genetics and Society (TIGS) and Rockefeller foundation (grant number 2021 HTH 018).
Author Declarations
I confirm all relevant ethical guidelines have been followed, and any necessary IRB and/or ethics committee approvals have been obtained.
I confirm that all necessary patient/participant consent has been obtained and the appropriate institutional forms have been archived, and that any patient/participant/sample identifiers included were not known to anyone (e.g., hospital staff, patients or participants themselves) outside the research group so cannot be used to identify individuals.
I understand that all clinical trials and any other prospective interventional studies must be registered with an ICMJE-approved registry, such as ClinicalTrials.gov. I confirm that any such study reported in the manuscript has been registered and the trial registration ID is provided (note: if posting a prospective study registered retrospectively, please provide a statement in the trial ID field explaining why the study was not registered in advance).
I have followed all appropriate research reporting guidelines, such as any relevant EQUATOR Network research reporting checklist(s) and other pertinent material, if applicable.
Data Availability
All data produced in the present study are available upon reasonable request to the authors.
View the discussion thread.
Supplementary Material
Thank you for your interest in spreading the word about medRxiv.
NOTE: Your email address is requested solely to identify you as the sender of this article.

Citation Manager Formats
- EndNote (tagged)
- EndNote 8 (xml)
- RefWorks Tagged
- Ref Manager
- Tweet Widget
- Facebook Like
- Google Plus One
Subject Area
- Epidemiology
- Addiction Medicine (316)
- Allergy and Immunology (617)
- Anesthesia (159)
- Cardiovascular Medicine (2272)
- Dentistry and Oral Medicine (279)
- Dermatology (201)
- Emergency Medicine (369)
- Endocrinology (including Diabetes Mellitus and Metabolic Disease) (798)
- Epidemiology (11563)
- Forensic Medicine (10)
- Gastroenterology (676)
- Genetic and Genomic Medicine (3565)
- Geriatric Medicine (336)
- Health Economics (615)
- Health Informatics (2298)
- Health Policy (913)
- Health Systems and Quality Improvement (862)
- Hematology (334)
- HIV/AIDS (749)
- Infectious Diseases (except HIV/AIDS) (13139)
- Intensive Care and Critical Care Medicine (755)
- Medical Education (359)
- Medical Ethics (100)
- Nephrology (388)
- Neurology (3343)
- Nursing (191)
- Nutrition (506)
- Obstetrics and Gynecology (650)
- Occupational and Environmental Health (644)
- Oncology (1754)
- Ophthalmology (524)
- Orthopedics (208)
- Otolaryngology (284)
- Pain Medicine (223)
- Palliative Medicine (66)
- Pathology (437)
- Pediatrics (1000)
- Pharmacology and Therapeutics (421)
- Primary Care Research (403)
- Psychiatry and Clinical Psychology (3053)
- Public and Global Health (5982)
- Radiology and Imaging (1219)
- Rehabilitation Medicine and Physical Therapy (713)
- Respiratory Medicine (809)
- Rheumatology (367)
- Sexual and Reproductive Health (348)
- Sports Medicine (315)
- Surgery (386)
- Toxicology (50)
- Transplantation (170)
- Urology (142)
Click through the PLOS taxonomy to find articles in your field.
For more information about PLOS Subject Areas, click here .
Loading metrics
Open Access
Peer-reviewed
Research Article
Assessing current visual tooth wear age estimation methods for Rangifer tarandus using a known age sample from Canada
Roles Conceptualization, Data curation, Formal analysis, Investigation, Methodology, Validation, Visualization, Writing – original draft, Writing – review & editing
* E-mail: [email protected]
Affiliation Department of Anthropology, University of Saskatchewan, Saskatoon, Saskatchewan, Canada

Roles Conceptualization, Funding acquisition, Visualization, Writing – original draft, Writing – review & editing
Affiliations Department of Anthropology, University of Saskatchewan, Saskatoon, Saskatchewan, Canada, Department of Anthropology, University of Alberta, Edmonton, Alberta, Canada
Roles Data curation, Funding acquisition, Resources, Writing – review & editing
Affiliation Faculty of Veterinary Medicine, University of Calgary, Calgary, Alberta, Canada
Roles Data curation, Resources, Writing – review & editing
Affiliations Canadian Museum of Nature and Beaty Center for Species Discovery, Ottawa, Ontario, Canada, Biology Department, Laurentian University, Sudbury, Ontario, Canada
Roles Conceptualization, Data curation, Funding acquisition, Methodology, Project administration, Resources, Supervision, Visualization, Writing – original draft, Writing – review & editing
- Grace Kohut,
- Robert Losey,
- Susan Kutz,
- Kamal Khidas,
- Tatiana Nomokonova
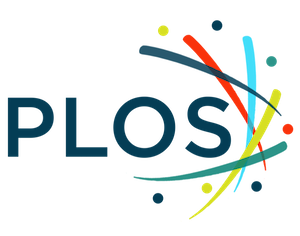
- Published: April 2, 2024
- https://doi.org/10.1371/journal.pone.0301408
- Reader Comments
Age estimation is crucial for investigating animal populations in the past and present. Visual examination of tooth wear and eruption is one of the most common ageing methods in zooarchaeology, wildlife management, palaeontology, and veterinary research. Such approaches are particularly advantageous because they are non-destructive, can be completed using photographs, and do not require specialized training. Several tooth wear and eruption methods have been developed for Rangifer tarandus , a widely distributed and long-utilized species in the North. This paper evaluates the practicality and effectiveness of three existing visual tooth wear and eruption methods for this species using a large known-age sample from several caribou populations in northern Canada (Bluenose East, Bluenose West, Dolphin-Union, Qamanirjuaq, and Beverly herds). These methods are evaluated based on: (1) the amount of error and bias between estimated and actual ages, (2) suitable and interpretable results, (3) user-friendly and unambiguous procedures, and (4) which teeth and visual features of those teeth are used to record wear and eruption status. This study finds that the three evaluated methods all have variable errors and biases, and two show extensive biases when applied to older individuals. Demographic data is simpler to generate and more flexible to report when methods allow age to be estimated as a continuous or discrete variable, rather than as age ranges. The dentition samples used by two of the previously developed methods impact their applicability to other populations of Rangifer . In one existing method, individuals were unavailable from some age ranges leaving gaps when assigning ages. For another Rangifer -ageing method, the population utilized was too distinct in morphology or diet to be used with the Canadian caribou analyzed here. Additional refinement of tooth wear and eruption ageing methods will benefit zooarchaeological research on reindeer and caribou remains.
Citation: Kohut G, Losey R, Kutz S, Khidas K, Nomokonova T (2024) Assessing current visual tooth wear age estimation methods for Rangifer tarandus using a known age sample from Canada. PLoS ONE 19(4): e0301408. https://doi.org/10.1371/journal.pone.0301408
Editor: Artak Heboyan, Yerevan State Medical University Named after Mkhitar Heratsi, ARMENIA
Received: December 23, 2023; Accepted: March 16, 2024; Published: April 2, 2024
Copyright: © 2024 Kohut et al. This is an open access article distributed under the terms of the Creative Commons Attribution License , which permits unrestricted use, distribution, and reproduction in any medium, provided the original author and source are credited.
Data Availability: All relevant data are within the manuscript and its Supporting Information files.
Funding: Funding for this project was provided by a grant from the Social Sciences and Humanities Research Council of Canada [#SSHRC IG435-2021-0014] to Tatiana Nomokonova. Funding for the caribou mandible collection and management was supported by grants from the NWT Cumulative Impacts Monitoring Program, Polar Knowledge Canada, Natural Sciences and Engineering Research Council, and Environment and Climate Change Canada to Susan Kutz. TN; #SSHRC IG435-2021-0014; Social Sciences and Humanities Research Council of Canada; https://www.sshrc-crsh.gc.ca/home-accueil-eng.aspx SK; NWT Cumulative Impacts Monitoring Program; https://www.gov.nt.ca/ecc/en/services/nwt-cumulative-impact-monitoring-program-nwt-cimp/about-us SK; Polar Knowledge Canada; https://www.canada.ca/en/polar-knowledge.html SK; Natural Sciences and Engineering Research Council; https://www.nserc-crsng.gc.ca/index_eng.asp SK; Environment and Climate Change Canada; https://www.canada.ca/en/environment-climate-change.html The sponsors or funders did not play any role in the study design, data collection and analysis, decision to publish, or preparation of the manuscript.
Competing interests: The authors have declared that no competing interests exist.
Introduction
Age estimation using animal dentition is an effective tool in zooarchaeology for investigating how humans interacted with fauna in the distant past [ 1 – 15 ]. For example, data from such estimations can be used to create age-based demographic profiles and survivorship curves that are informative about how people procured wild animals and managed domestic herds [ 10 , 12 , 16 – 19 ]. Ageing methods are especially valuable for key species such as caribou and reindeer ( Rangifer tarandus ). These animals are widespread across much of northern Eurasia and North America [ 20 – 23 ], and their remains are frequently found in abundance at archaeological sites beginning in the Late Pleistocene [e.g., 24 – 30 ]. Furthermore, reindeer and caribou, in both wild and domestic forms, continue to be essential to maintaining ways of life and well-being for many northern peoples [ 23 , 31 – 45 ].
Tooth wear age estimation is commonly used for analysing ungulate remains from archaeological sites. It is a non-destructive, inexpensive, and efficient technique that does not require specialized equipment or extensive experience, particularly when detailed methodological procedures are provided [ 15 ]. Animal teeth are subjected to wear on the occlusal surface and reduce in height over their lifetime [ 46 – 49 ]. Such wear progresses at a predictable rate, allowing the extent of wear to be used to estimate age at death, but always with some margin of error. For herbivores such as caribou and reindeer, the rate of wear is heavily influenced by the texture of their food, meaning that dietary differences (and any soil substrate ingested with food) lead to variation in wear patterns and rates [ 4 , 46 , 50 – 54 ]. Body size variation by sex and between populations also likely contributes to additional variation in these patterns. For example, the morphology of occlusal wear patterns of first and second molars have been used to differentiate between two subspecies of reindeer in Fennoscandia, mountain reindeer ( R . t . tarandus ) and forest reindeer ( R . t . fennicus ) [ 55 ]. Tooth wear methods are designed for specific species or populations due to these factors [ 1 ]. These approaches are usually paired with an examination of tooth eruption for individuals with deciduous (also known as primary) teeth in place or adult dentition still in the process of erupting [ 4 , 22 ].
Mandibular molars and premolars are most often used in zooarchaeological analysis because they remain in their alveoli more often than incisors and canines, and also in comparison to those in the fragile maxilla [ 6 , 10 , 14 , 56 ]. Methods using incisors and canines are often suitable in wildlife research and veterinary contexts because these teeth can be more easily observed in living animals [e.g., 57 – 59 ]. However, ruminant incisors and canines are smaller and morphologically simpler, and thus offer less precision for visual age estimation than post-canine teeth [ 1 , 52 ].
Several tooth wear ageing methods have been developed for Rangifer tarandus , including visual and crown height measurement approaches. Visual methods involve observing the severity of tooth wear on the occlusal surface in comparison to illustrated patterns of dentine and enamel wear or text descriptions of tooth wear characteristics. Three such methods available for this species were identified in the published literature: those by van den Berg, Loonen, and Çakırlar [ 60 ], Pasda [ 56 ], and Miller [ 61 ] which are described below. In such approaches, the analyst essentially matches observations on a tooth to the illustrations or descriptions and assigns a score representing either relative tooth wear severity or age group. Another approach for caribou and reindeer focuses on crown height measurements (often molars, but also premolars or incisors) [e.g., 52 , 61 – 65 ]. Measurements are considered more objective than visual assessment and the results of this approach are more easily analyzed statistically [ 13 , 66 ]. However, visual methods have some advantages over crown height approaches. Visual methods can be completed using photos rather than by directly accessing specimens, allowing for greater flexibility in data collection. Most importantly, these methods require no alteration to specimens other than cleaning of occlusal surfaces [ 60 ] whereas crown height cannot be measured for younger individuals without extracting teeth to reveal the full crown height [ 15 ]. Unless the alveolar bone is already fragmented, destructive procedures are necessary to extract post-canine teeth when the landmark being measured, usually the cemento-enamel junction or the bifurcation of the root, is below the alveolar bone. Chipped cusps or other damage also may prevent specimens from being properly measured, but this issue affects all tooth wear methodologies.
Visual approaches to scoring tooth wear can be effective and convenient tools for ageing reindeer and caribou remains, but their accuracy and user-friendliness commonly remain untested using other known age samples. This study evaluated published methods developed for Rangifer tarandus by van den Berg, Loonen, and Çakırlar [ 60 ], Pasda [ 56 ], and Miller [ 61 ] (referred to here as Methods A, B, and C, respectively) using a sample of known age caribou from Northern Canada. These methods were assessed in four ways. First, where possible, age estimations for each method were compared to known ages to assess error rates and biases. Second, we consider the format of the results from each method, including whether the estimated age was a continuous variable or an age range, and how easily the results could be interpreted. Third, the user-friendliness, clarity, and possible sources of subjectivity or misinterpretation in each method are discussed. Finally, we examine which teeth were chosen for analysis and the visual characteristics each method incorporates into its scoring models. Importantly, variations in how the three models were designed and differences in their intended uses make some numerical comparisons in the models’ performances impossible.
Materials and methods
The sample used in this study consists of left mandibles from 153 caribou, including 90 females and 63 males ranging in age between 3 months and 17 years ( Fig 1A ). Within this age range, only 15-year-old individuals were missing. An effort was made to maintain an even ratio of females to males for all age groups, but this was only possible for individuals up to eight years of age ( Fig 1B ). Male caribou and reindeer have lower life expectancies than females [ 22 , 61 ], and fewer older males make their way into collections. The oldest male individual available for this study was 11 years old, while females in the sample were up to 17 years of age. For both sexes, fewer caribou over age 10 were available compared to younger age classes.
- PPT PowerPoint slide
- PNG larger image
- TIFF original image
Age distribution of caribou mandible sample organized by (A) population and (B) sex.
https://doi.org/10.1371/journal.pone.0301408.g001
Individuals in this study with fully erupted adult dentition, as well as those originally used for Methods A [ 60 ], B [ 56 ], and C [ 61 ], were aged using cementum annulation. These cementum ages are referred to here as known ages. When not feasible to track individual animals from birth to death and collect their remains (e.g., virtually all wild ungulates), cementum annulation is frequently employed in age estimation. This method introduces some error but is nonetheless considered the most accurate skeletal ageing method currently available [ 4 , 51 , 67 – 69 ]. For example, using cementum annulation Veiberg and colleagues [ 69 ] found ages of semi-domestic reindeer ( R . t . tarandus ) in Norway were assigned correctly in 54% of cases, with 89% having age estimates within one year of true age. In another study, Svalbard reindeer ( R . t . platyrhynchus ) ages were correct in 71% of cases, with 94% aged to within one year of their known ages. Ages for individuals with erupting dentition can be estimated where the calving season is brief using the known date of death and the status of tooth eruption [ 61 ].
Rangifer tarandus in this study belong to several populations in Northern Canada ( Fig 2 ). Ninety-two (57 females, 35 males) barren-ground caribou ( R . t . groenlandicus ) were from the Qamanirjuaq and Beverly Herds. Whether these individuals belong to the Qamanirjuaq or Beverly herd is unknown. Since these herds share similar ranges which overlap during the winter months and are of the same subspecies [ 61 , 70 ], it was considered unnecessary to differentiate between the two. Their mandibles were collected by the Canadian Wildlife Services (CWS) between 1966 and 1968 and are curated at the Canadian Museum of Nature, Gatineau, QC, Canada. Adult ages were determined through cementum annulation by F. Miller and CWS personnel, and all or some were used in the original development of Method C [ 61 ]. The study sample also includes 45 (29 female, 16 male) caribou from the Dolphin-Union Herd ( R . t . groenlandicus x pearyi ), 10 (4 female, 6 male) from the Bluenose East Herd ( R . t . groenlandicus ), and 6 (all male) from the Bluenose West Herd ( R . t . groenlandicus ). Mandibles from these three herds were collected through hunter sampling programs by the Kutz Research Group, Faculty of Veterinary Medicine, University of Calgary, between 2008 and 2019. They are curated at the Zooarchaeology Lab, Department of Anthropology, University of Saskatchewan in Canada. For this latter group of specimens, adult ages were assessed through cementum annulation of incisors by Matson’s Laboratory (Manhattan, MT, USA). All mandibles in this sample are listed in the S1 Table .
Geographic distributions of caribou and reindeer populations discussed in this study: (A) Bluenose West and (B) Bluenose East caribou herds [ 71 , 72 ], (C) Dolphin-Union caribou [ 73 ], (D) Qamanirjuaq and Beverly caribou herds [ 61 , 70 , 74 ], (E) Sisimiut caribou [ 56 , 75 ], and (F) Svalbard (Nordenskiöld Land) reindeer [ 60 ]. Basemap made with Natural Earth.
https://doi.org/10.1371/journal.pone.0301408.g002
A total of 912 mandibular molars and premolars were present in the 153 mandible sample ( Table 1 , S1 Table ). This included 92 deciduous premolars, 383 permanent premolars, and 437 molars. Unerupted teeth (25 P 2 , 23 P 3 , 25 P 4 , 0 M 1 , 3 M 2 , and 16 M 3 ; 98 total) could not be scored for wear and were recorded as absent (not included in Table 1 counts). Seven additional teeth were missing due to irregular loss. One dP 2 (CMN-39169), P 2 , (CMN-39516), and two M 3 (CMN-39112 and DU-210) were lost at or around the time of death (alveoli are open without remodeling). One dP 2 (CMN-38606) was severely fractured with most of the cusp missing. One P 3 (BW-08-91) was lost antemortem (alveolus has remodelled) and the M 3 was missing from one mandible (CMN-39056) with bone deformation and resorption surrounding the M 3 alveolus.
https://doi.org/10.1371/journal.pone.0301408.t001
Tooth wear was observed in photos taken with a mirrorless camera from occlusal, lingual, and buccal views. All assessments were completed by the same person (G. Kohut). Teeth were evaluated blindly (without known age data) to reduce observer bias. Data were entered and processed with Microsoft Excel. Unless otherwise noted, ages of caribou and reindeer were considered in “birthday years” and rounded down to the nearest integer (i.e., a 3.8-year-old is a three-year-old, not a four-year-old).
Van den Berg et al.’s [ 60 ] method ( Table 2 ) was developed for Svalbard reindeer ( Fig 2 ) and is similar in design to Grant’s [ 5 ] widely used method for cattle, sheep/goats, and pigs. Svalbard reindeer ( R . t . platyrhynchus ) dentition from 151 known age individuals (292 mandibles) ranging from 0–15 years of age were used to create a series of black and white illustrations of the occlusal view of dentine and enamel for dP 4 , P 4 , M 1 , M 2 , and M 3 . Two versions are provided: the Svalbard or Absolute scheme and the uncalibrated or Relative scheme for other reindeer populations. The Svalbard version provides an age estimate in years and is meant for use solely with Svalbard reindeer. This population is genetically isolated and has adapted to the high-arctic Svalbard Archipelago with short limbs, small bodies, and small head sizes, differentiating them from most other subspecies in both morphology and diet [ 76 , 77 ]. The uncalibrated or Relative scheme is recommended by the authors for non-Svalbard populations, and this version provides an arbitrary score that ranks individuals relatively but does not provide an age estimate [ 60 ].
https://doi.org/10.1371/journal.pone.0301408.t002
To test these methodological schemes, age estimation for our study sample first involved the application of van den Berg, Loonen, and Çakırlar’s [ 60 ] uncalibrated or Relative teeth wear progression scheme. Each dP 4 or P 4 (whichever is present) and M 1 , M 2 , and M 3 were assigned a Tooth Wear Stage (TWS) letter based on the closest match between the occlusal pattern of dentine and enamel and the black and white illustrations. If a tooth was in the process of erupting, it was assigned a TWS code of “C, perforation in Crypt visible; V, tooth Visible in crypt; E, tooth eruption through bone; H, tooth almost halfway between bone and full height; [and] T, tooth (almost) at full height but unworn” [ 60 ]. Absent teeth or those that could not be assessed (e.g., broken crowns) were omitted. Teeth not visible because they had not yet erupted were scored as zero.
Because the Relative scheme does not produce age estimations, additional steps were taken. Specifically, the TWS scores were calibrated using the known age samples compiled for this study. Our approach in this step followed the calibration process utilized with the Svalbard Absolute scheme [ 60 ]. Namely, an age assessment was made for each tooth TWS by calculating the mean known age of all mandibles in the current study sample fit that a particular TWS. These calibrated scores were rounded to the nearest 0.5 to remain consistent with the original calibrated method and are referred to as the “calibrated version” of Method A. The mandible wear stage (MWS) was calculated as the mean TWS score for all teeth present in each mandible. Unerupted teeth were counted towards the total number of teeth while missing or damaged teeth were not. The estimated age in years equals the MWS. This process of testing Method A is not ideal, as the model was calibrated on the same specimens that were then aged, and the results compared to the known ages. This somewhat circular procedure should reduce errors in age assessment compared to the other models tested. At the same time, this step makes it problematic to directly compare these error rates with those of the other methods. Regardless, this procedure allowed us to assess the usability of the relative scheme in a similar way among all three methods.
The second assessment of Method A involved ageing our sample using the Absolute scheme as if the sample were composed of Svalbard reindeer. As mentioned, the use of the Absolute scheme with non-Svalbard reindeer was not recommended by the study authors for several valid reasons [ 60 ]. Our utilization of the Absolute scheme on non-Svalbard reindeer helps demonstrate this point, namely that it is problematic to apply an ageing method designed for a highly distinct population more broadly. Further, this step also allowed for an assessment of the practicality of employing the model realistically, albeit not on its intended target population.
Pasda’s [ 56 ] ageing method ( Table 2 ) was developed on Sisimiut caribou ( R . t . groenlandicus ) from Greenland ( Fig 2 ). The dentition of 63 reindeer of known age ranging from 0–14 years (0–177 months) was used to establish criteria for estimating age using mandibular molar and premolar wear patterns. These reindeer died of natural causes and their remains were collected after death. Cementum annulation examination was carried out by the study author [ 56 , 75 ]. Teeth were graded visually based on the severity of wear or tooth eruption and replacement. A series of representative photos of dentition from the occlusal and buccal views were also provided. Pasda cautions that “this classification was subjective and qualifies as a rough estimate” [ 56 , p. 33]. While Sisimiut caribou should be relatively comparable to the populations used in this study given that they belong to the same subspecies, Pasda [ 56 ] notes that reindeer in this region (Kangerlussuaq) are known for having accelerated tooth wear relative to more southern populations in Greenland, attributed to a courser diet.
Method B involved visually scoring the extent of wear of each tooth using the scoring criteria provided in Table 3 and then assigning age ranges using the descriptions available in the published text [ 56 , tbl. 8]. Ages in the current study were estimated following the published instructions, but some additional procedures also were implemented. First, following the original protocol, the premolars and molars in each mandible were assigned grades: no wear, slight wear, moderate wear, heavy wear, and very heavy wear following Table 3 [ 56 ]. Additionally, we recorded the status of eruption, if applicable, and whether premolars were deciduous or permanent.
https://doi.org/10.1371/journal.pone.0301408.t003
Second, using the teeth grades, individual mandibles were assigned to age ranges following the study protocol [ 56 , tbl. 8]. However, multiple age ranges were usually applicable to each mandible. A procedure for assigning these age categories was implemented: when two or more age ranges applied to one individual, the overlapping range was used as the final estimated age range. For example, if the M 1 and M 2 are both moderately worn, their age ranges in the original study protocol, 31–71 and 36–71 months respectively, were combined into an estimated age range of 36–71 months. For instances where two or more criteria applied to one mandible but have age ranges that do not overlap (for example, M 3 erupting at 13–18 months and M 1 moderately worn at 31–71 months), one of the ranges was disregarded. These choices were made without consulting the known age data. In cases where two or more age ranges overlap but one did not, the non-overlapping age range was omitted. If one of the non-overlapping criteria involved tooth eruption, that range was chosen since tooth eruption is more reliable for ageing than wear. Finally, if the above rules could not be used to choose between non-overlapping ranges, the criterion with a more clear and easily distinguished description of tooth wear was used, though these choices were admittedly subjective.
Miller’s [ 61 ] caribou ageing study ( Table 2 ) involved 999 known-age individuals between 0–17 years belonging to the Qamanirjuaq (published as Kaminuriak) and Beverly caribou herds ( R . t . groenlandicus ). These herds migrate in overlapping ranges in northern Canada ( Fig 2 ). A small portion of Miller’s caribou mandibles (n = 92) collected between 1966–1968 was also used in the present study. In Miller’s study, mandibular dentition was used to generate text descriptions of tooth wear and eruption for complete rows of teeth including molars, premolars, canines, and incisors, though canines and incisors are referenced less often [ 61 , p. 15–18]. Incisors and canines were not used in the present study as these teeth were not available for most specimens. Descriptions are available for 19 age categories, provided in months, ranging from birth to 10 years, including a 10+ years age category. Eleven of those age categories are within the first three years and are based on tooth eruption. Tooth eruption data is provided in a table [ 61 ] which reports the percent frequency of occurrence of whether each tooth type is unerupted, deciduous, partially, or fully erupted for ages between zero and 29 months.
Specific instructions for applying Miller’s data to estimate age were not provided, requiring that specific steps be outlined here. First, to establish an approximate age range, each mandible was compared to either the tooth eruption table [ 61 ] or reference photos, depending on whether tooth eruption was ongoing at death. Second, using the approximate age range as a starting point, each individual was assigned a more specific age estimate based on the tooth wear and eruption descriptions. For cases where one mandible fit the description for more than one age (which occurred frequently), the closest fitting description was chosen. Specifically, each individual was placed into age categories utilized by Miller [ 61 ], which were 1, 2, 3, 4–6, 6–9, and 10+ years. Miller [ 61 ] cautions that estimated ages are inaccurate from approximately four years and older, and these broader age categories are more appropriate. In our evaluation of method C, we excluded the 92 mandibles examined here used by Miller in the initial development of his method. Method C was thus applied only to the 61 individuals belonging to the Dolphin-Union, Bluenose East, and Bluenose West populations.
To present and interpret the study outcomes consistently, two known age category schemes were employed. First, the initial two years of life were divided into six-month intervals, followed by one-year intervals for ages two to 17. Second, individuals were divided into three age groups (0–2, 3–9, and 10+ years) to investigate trends according to general young, adult, and old adult life stage categories for reindeer and caribou [ 17 , 61 ]. The division between young and adult at three years was based on sexual maturity and adult tooth eruption completion, the latter occurring at ~29 months [ 61 , 78 – 81 ]. The beginning age for old adults is approximately when Rangifer become reproductively unviable (particularly when females stop producing calves) and body mass begins to decrease, though this varies by population [ 65 , 78 , 80 , 82 – 84 ]. Miller [ 61 ] also employs a broad 10+ year age category in his analyses, which we adopt here so that this age category can be reported consistently between Methods A, B, and C.
Assessing error is essential to understanding how accurately methods estimate age. Because the estimated age for Method A was a continuous variable, Method B provided a range, and Method C involved discrete or range values, direct statistical comparison between methods was impossible. Where estimated ages were a discrete value (Method A and Method C for 0–9 year olds), error was calculated as the difference between estimated age and known age for each individual. For methods that produce age ranges (Methods B and C), error was calculated as the difference between the known age and either the upper or lower limit of the estimated age. As age ranges were more likely to result in low or no error estimations (the known age falls within the estimated age range), greater accuracy was expected compared to methods where age is estimated as a continuous variable. The mean error ( ME ) was calculated for all three methods, here only to indicate whether ages were underestimated (negative value) or overestimated (positive value). The mean absolute error ( MAE ) was used to quantify the difference between estimated and known age. This approach prevented individual positive and negative errors from cancelling each other out. Given the differences in variable type for the three methods described above, the mean errors are not directly comparable.
Ages were estimated and the ME and MAE calculated for the mandibles in this sample following Methods A, B, and C. Estimated ages for each individual using Method A (calibrated, uncalibrated, and Svalbard versions), Method B, and Method C (discrete ages and age categories) are provided in S1 Table .
A calibrated version of Method A was successfully created based on the 153 caribou mandible sample in this study, with TWS values provided in Table 4 . Most TWSs could be assigned, but there were some gaps, particularly at younger ages such as dP 4 TWS a and b, P 4 TWS E, and older ages such as P4 TWS j and over, M 1 TWSs m and o, M 2 TWSs m, and M 3 TWS l and k. These gaps exist because no teeth matched the illustrated wear patterns. It is also notable that lengths of time between TWSs were not equal and some ages were less represented (e.g., 4, 6, and 8 years) in the calibrated TWS scheme. The P 4 TWS f score seemed out of order with a value of 16 falling between 10 and 12.
https://doi.org/10.1371/journal.pone.0301408.t004
As expected, the calibrated and Svalbard versions of Method A provided contrasting age estimation results, especially for older individuals. The calibrated version results ranged from 0.5 to 16.1 years while the Svalbard version ranged only between 0.0 and 11.5 years. Also, as anticipated, the calibrated version performed well for most ages. For adults between 3–9 years (known-age) the MAE is 0.99 years and for ages 10+ the MAE equalled 1.63 years ( Table 5 ). Based on ME and the scatter plot ( Fig 3A ), ages 2–6 tended to be overestimated while ages seven and over were more frequently underestimated.
https://doi.org/10.1371/journal.pone.0301408.g003
https://doi.org/10.1371/journal.pone.0301408.t005
The Svalbard version did not effectively model tooth wear for the barren-ground and Dolphin-Union caribou in this sample for most adult ages, as anticipated by the model’s designers [ 60 ]. The scatter diagram in Fig 3B shows a general trend of underestimating age after approximately four years old, with the oldest estimated age coming to 11.5 years (known to be 16 years old). The MAE remained low, less than one year, between 0–4 years old ( Table 5 ). After a known age of four years, the discrepancy between estimated and known age increased with a MAE of 1.5 years ( ME = 1.5 years) at six years old and MAE of 2.6 years ( ME = -2.6 years) at eight. For caribou 10 years and older, the MAE was 4.16 years ( ME = -4.16 years). Interestingly, the Svalbard version estimated the ages of younger individuals more accurately and with less bias than the calibrated version. For ages 0–2 years, the ME from the Svalbard version is only -0.03 compared to the calibrated ME of 0.38 years and the MAE from the Svalbard version was slightly lower (0.30 years) compared to the calibrated one (0.47 years). Based on visual inspection of the scatterplots ( Fig 3 ), the calibrated scheme for Method A yielded a similar degree of precision to the Svalbard and uncalibrated versions. The scatter plot data points were similarly diffuse for all three versions.
Method B estimated age categories for the sample of 153 mandibles were between three months and seven years ( Table 6 ). These ranges cover various lengths of time, some of which overlap with each other, and two were discrete values (0.5 years/six months and seven years/84 months). Sixty-nine (45.1%) mandibles were assigned into correct age categories while 35 (22.9%) were one year off, 14 (9.2%) were two years off, and 35 (22.9%) were off by three or more years. For young caribou 0–2 years old (known age) the MAE = 0.19 and the ME = -0.06 indicated low bias and error. The error was higher for adult animals three years and over, with a high degree of bias ( ME = -5.73, MAE = 5.73) ( Table 7 ). For older individuals, age was more noticeably underestimated (for 7–17 years known age: ME = -3.72, MAE = 3.72). This was largely because individuals with known ages of 8–17 years were placed in the 3.5–6 year, 5.5–6 year, and 7 year categories. Estimated age ranges were much closer to expected values for individuals seven years and younger ( ME = -0.29, MAE = 0.35) compared to ages eight years and over ( ME = -4.30, MAE = 4.30).
Highlighted cells indicate overlapping known and estimated age ranges.
https://doi.org/10.1371/journal.pone.0301408.t006
https://doi.org/10.1371/journal.pone.0301408.t007
The results of Method B, especially for older age categories, were affected by conflicting tooth wear criteria. For example, CMN-39516 was estimated to be 84 months (7 years) because it meets the criteria “premolars and M 3 moderately worn” (43–84 months) and “crowns of the molars and premolars show heavy, sometimes irregular wear” (>84 months) [ 56 ]. However, “M 1 shows excessive wear, only roots remaining in some cases” (>91 months) also applied to this specimen. The known age of this individual is 17 years. Because the two overlapping ranges were combined and kept (resulting in an estimated age range of 43–84 months) the higher range (>91 months) was omitted, contributing a high degree of error. Five other sets of conflicting ageing criteria were also found to be present in our sample, affecting the ageing of 32 specimens ( Table 8 ). Combined, these individuals show an ME = -1.8 years and MAE = 1.8 years.
https://doi.org/10.1371/journal.pone.0301408.t008
The estimated ages for the 61 mandibles evaluated by Method C ranged from three months to 10+ years. The estimated age values (before being placed into age categories) are visualized as a scatter plot in Fig 4 . Known and estimated age for individuals between 0–9 years followed a positively correlated linear relationship, with the data points becoming more diffuse after roughly four years of age. The results from Method C following the age categories recommended by Miller [ 61 ] are summarized in Table 9 . This comparison showed that 34 (55.7%) mandibles were categorized correctly while 18 (23.5%) were one year off, seven (11.5%) were two years off, and two (3.3%) were incorrect by three or more years. This pattern parallels that in the scatter plot generated for the estimated age values ( Fig 4 ). The overall ME of -0.17 suggested a small overall underestimation bias, though the ME is nearly always positive until five years (known age) and negative at six years and older ( Table 10 ).
Estimated age values in months provided in Miller [ 61 ] are used rather than years (e.g., 39 months/3.25 years instead of 3 years). The linear line of best fit applies only to individuals nine years and younger since older animals fall into a 10+ year category and cannot be modelled.
https://doi.org/10.1371/journal.pone.0301408.g004
https://doi.org/10.1371/journal.pone.0301408.t009
https://doi.org/10.1371/journal.pone.0301408.t010
As discussed, since the estimated age variables produced by each of these methods are different types, it is not possible to directly compare the results quantitatively. In general, all methods produced relatively small ME and MAE values for young Rangifer tarandus (known age = 0–2 years) compared to those for adults and old adults. Tooth eruption played the primary role in all three methods in ageing individuals of this age range. The timing of tooth eruption can be more precisely defined than the extent of tooth wear, and, at this early life stage, variation in the rate of wear is relatively minimal [ 46 , 60 , 61 ]. However, error approaching half a year, as seen in the calibrated version of Method A ( ME = 0.38, MAE = 0.47) for 0–2 year-olds ( Table 5 ), would have limited interpretive value for inferring the seasonality of death, a common but often potentially problematic zooarchaeological practice with juvenile ungulates [ 7 , 10 , 85 ].
All three methods presented some challenges when estimating adult ages. The results from applying the Svalbard version of Method A to the caribou in this sample fully support van den Berg et al.’s [ 60 ] argument that the Svalbard Absolute ageing scheme should not be used with other Rangifer populations. As mentioned, Svalbard reindeer are smaller, have a different morphology than barren-ground caribou, and live in a more severe, high-arctic habitat. The tendency of this method to underestimate ages of adults suggests that Svalbard reindeer teeth wear down much faster than in the known age sample compiled for this study. In contrast, while the calibrated version would almost certainly produce more accurate results for Rangifer populations similar to those included here, it has only been assessed using the same sample it was calibrated with, and not all illustrations have been assigned TWS values. This version of Method A should not be considered tested for accuracy and bias.
Method B began to underestimate adult age starting around seven years. Individuals three to six years old were estimated with relatively low error ( ME = -0.23; MAE = 0.24), but increased considerably for 7–9 year olds ( ME = -2.44; MAE = 2.44). Specifically, all but two 7–9 year old individuals (n = 34/36) were placed in the 3.5–6 year category ( Table 6 ). This pattern occurred primarily because no individuals were estimated to be older than seven years (84 months). It is uncertain if differences in the rate of tooth wear or dental morphology between the Canadian caribou in this sample and Sisimiut caribou from Greenland contributed to estimation error. Method B included several open-ended older individual age categories: >79 months, >67 months, >91 months, > 84–96 months, and > 173 months [ 56 ]. However, individuals matching these open-ended older age categories also matched other criteria that overlapped in age range. This bounds the upper limit of the estimated age for such individuals, often resulting in underestimation of their ages in our comparisons. The oldest age category in Method C was also an open-ended age range. It combined all older animals into a broad 10+ year category if “all molariform teeth were very worn: they were on a more or less even plane, and the buccal-lingual plane was nearly horizontal” and “the infundibulum between the anterior cusps of the M 1 was nearly obliterated or absent in some specimens” [ 61 , p. 18]. This approach results in fewer errors but also far less discrete age estimations for older individuals.
The ability to interpret age estimation results and assign meaningful age categories is crucial. Van den Berg and colleagues [ 60 ] did not propose age categories to use with their method. However, since the estimated age produced by Method A for the Svalbard or calibrated versions was a continuous variable, the results can be divided into any age category pattern desired. The uncalibrated version, which was designed for the relative ageing of assemblages, cannot be used in this manner because it is unknown what MWS values divide a sample into meaningful life stages. Relative age comparisons have interpretive value, but there are many cases where age estimates as continuous values, even for use within age categories, would also be useful.
The Method B age categories are very challenging to transform into a demographic profile because they overlap with each other, some representing very similar ranges, such as 12–18 months, 13–18 months, and 12–35 months. They also cover different range sizes; for example, nine individuals fell into the discrete 84 month (seven year) old category while 90 were assigned the 43–71 month (3.5–6 year) category. These results would require some reorganising before fitting into a histogram or other plot. The results from Method B are less flexible and more difficult to interpret than the other two methods because of these complicating factors.
Further, the age categories for Method B reflect the relatively limited Sisimiut caribou sample available. For example, no individuals with ages between 18–31 months were available [ 56 ], so criteria that involve later stages of tooth eruption (M 3 and premolars erupting, M 1 with slight wear, incomplete root development, and any molars or premolars showing no wear) end in the scheme at 18 months. In the Qamanirjuaq and Beverly caribou of the same subspecies ( R . t . groenlandicus ), the M 3 and permanent premolars continue to erupt until ~27 months [ 61 ]. Assuming that Sisimiut caribou tooth eruption extends sometime later than 18 months, estimated ages based on this method may be underestimated for individuals with erupting M 3 and premolars. Our results indicated that caribou with known ages of 18–29 months were underestimated with a ME of -0.35 years, which was notably high relative to the low degree of bias seen in 12–17 month olds ( ME = 0.01) and three year olds ( ME = -0.04) using this method.
Miller’s age categories for Method C could be used to reconstruct demographic profiles in wildlife management or zooarchaeological contexts [ 61 ]. By using increasingly broader age categories, Method C acknowledges that the accuracy of tooth wear ageing diminishes at older ages. However, this method would not adjust well if other age categories are desired, especially for individuals over 10 years old.
Assessing the clarity and user-friendliness of the instructions for each method is far more subjective than the assessments above but nonetheless warrants discussion. Assigning TWS scores according to Method A was intuitive and efficient to complete, in large part because the method relies on visual recognition of occlusal tooth wear patterns rather than reference to text descriptions. However, some teeth analyzed looked like they belonged between two TWSs or showed characteristics of more than one illustration, making selection of some TWS values ambiguous. Method B was also relatively quick to complete, as it involved assigning teeth to one of five tooth wear severity grades, all of which are textually defined and photographed. At the same time, potential for misinterpretation of these grades was repeatedly encountered during their assessment. This stems from some ambiguity in the definitions. For example, slight wear is defined as “polished surface and the dentine was exposed at the cusps”, moderate wear shows “dentine of teeth … showed greater and more even exposure”, and heavy wear is described as “teeth already irregularly worn” [ 56 , p. 33]. These definitions made it difficult to readily differentiate between wear categories. Method C was more time-consuming to implement and also used vague language that made choosing the most appropriate age category challenging. For example, age five is defined as having “slightly more wear on the posterior half of the P 2 , the anterior and posterior buccal cusps of M 1 and the distal cusp of M 3 ” [ 61 ]. Terms such as “slightly more” leave a great deal of room for subjectivity. Additionally, descriptors such as “very worn” [p. 18], “horizontally inclined buccal-lingual plane” [p. 17], and “extensive attrition” [p. 18] are used but not defined, again likely resulting in more subjective assignments.
Greater accuracy is expected where more teeth are included in analysis, as more wear data is available per individual. However, excluding some teeth enhances efficiency in method application because there are fewer features to observe per individual. Each of the three methods incorporates post-canine teeth differently. Method A does not involve dP 2 , P 2 , dP 3 , or P 3 . Criteria for Method B rely more on molars than premolars, though premolars may be relevant in assigning age criteria at any age [ 56 ]. Method C does not include M 2 in descriptions for ages five, six, and eight, and only generally describes wear for all molars together at ages three, four, and seven [ 61 ]. However, M 2 wear severity was still graded for all individuals.
All methods take quite different approaches to characterizing wear. As mentioned above, Method A [ 60 ] is focused on two-dimensional visual representation of enamel and dentine shapes, an approach that has proven useful in wear studies with other taxa [ 5 , 12 , 15 , 86 ]. Method B [ 56 ] uses one series of relative tooth wear grades (none, slight, moderate, heavy, and very heavy) for all tooth types. Method C [ 61 ] relies heavily on text description, highlighting whether lines of dentine are narrower or wider than adjacent enamel, the mesial half of the P 2 shows wear, particular cusps (especially on the M 3 ) show wear, the pointed appearance of premolar cusps, the angle of the buccal-lingual plane or flattening of the occlusal surface, and extreme wear on the M 1 . These traits, while reasonably extensive, largely ignore the shape and connections of dentine and enamel that can be easily recognized. In contrast, occlusal surface angles play prominent roles in Method C, but these are difficult to observe in photos and likely undiscernible if only occlusal views are available. For younger individuals, all three methods focus on tooth eruption sequences which, in agreement with the lower error found in this study, provide a relatively low error for age estimation.
Comparing known ages of caribou mandibles with estimated ages generated through three methods revealed the strengths and weaknesses of each approach. The two schemes of Method A, developed by van den Berg and colleagues [ 60 ], provide efficient and intuitive approaches. However, these two schemes do not allow for age to be estimated for the Canadian caribou populations represented in our sample. The Svalbard or Absolute scheme is intended only for Svalbard reindeer, a highly unique Rangifer population, and when used with this study’s sample (which is not recommended) underestimated the ages of most adults. The uncalibrated or Relative scheme used in Method A generates only relative age assessments. To be used to generate ‘absolute’ age estimates elsewhere will always require calibration using known-age specimens from relevant populations. This restrains its applicability to other Rangifer populations, virtually all of which have been used by people more extensively and for longer periods than those on the Svalbard Archipelago. At the same time, Method A is by far the most user-friendly approach tested in this study.
Method B, designed by Pasda [ 56 ], also provides an efficient process for visually grading tooth wear based on severity. However, this method results in age categories of varying lengths which overlap with one another, complicating interpretations of age-based data. This method also did not estimate any ages over seven years. Method C, by Miller [ 61 ], involved visually assessing mandibular tooth wear using text descriptions and reference photos to assign ages, which were then placed into recommended age categories. While these age categories were well-suited for tooth wear age estimation analysis, the procedure was less user-friendly than the other two methods and a relatively high number of individuals were incorrectly assigned. The strength of this study is its sample size, which dwarfs that of the other two studies.
Tooth wear age estimation is a valuable analytical tool for assessing the ages of Rangifer and other animals that were critical to past human populations. This study demonstrates a clear need for improved methodologies that are built from larger samples and that can be used on a greater breadth of Rangifer populations. Refined definitions of traits used in scoring wear also will be necessary, and ideally, both ‘absolute’ and relative age assessments will be possible. Such methodological developments would help strengthen archaeological interpretations of human involvement with Rangifer in the distant past, including as prey animals or domestic livestock. Just as importantly, a more widely applicable and reliable methodology of estimating age in Rangifer will also be of use in wildlife biology to characterize modern populations, which are under increasing threats.
Supporting information
S1 table. known and estimated ages of caribou mandibles..
https://doi.org/10.1371/journal.pone.0301408.s001
Acknowledgments
We would like to acknowledge partners of the Kutz Research Group, Faculty of Veterinary Medicine, University of Calgary for their contributions in collecting caribou mandible samples: Sahtu Renewable Resources Board, Government of the NWT, Government of Nunavut, Kugluktuk Angoniatti Association, Ekaluktutiak Hunters and Trappers Organization, and Canada North Outfitting. We would also like to thank Xavier Fernandez Aguilar, Fabien Mavrot, Angela Schneider, and James Wang from the Kutz Research Group, Faculty of Veterinary Medicine, University of Calgary for their roles in caribou mandible sample collection.
- View Article
- Google Scholar
- 4. Gifford-Gonzalez D. An introduction to zooarchaeology. Cham, Switzerland: Springer International Publishing AG; 2018. https://doi.org/10.1007/978-3-319-65682-3
- 5. Grant A. The use of tooth wear as a guide to the age of domestic ungulates. In: Wilson B, Grigson C, Payne S, editors. Ageing and sexing animal bones from archaeological sites. Oxford: British Archaeological Reports; 1982. pp. 91–108.
- 19. Russell N. Social zooarchaeology: Humans and animals in prehistory. Cambridge; New York: Cambridge University Press; 2012.
- 20. Holand Ø, Mizin I, Weladji RB. Rangifer tarandus tarandus (Linnaeus, 1758). In: Corlatti L, Zachos FE, editors. Terrestrial Cetartiodactyla. Cham: Springer International Publishing; 2022. pp. 247–276. https://doi.org/10.1007/978-3-030-24475-0_24
- 21. Salmi A-K. Introduction: Perspectives on the history and ethnoarchaeology of reindeer domestication and herding. In: Salmi A-K, editor. Domestication in Action. Cham: Springer International Publishing; 2022. pp. 3–33. https://doi.org/10.1007/978-3-030-98643-8_1
- 22. Spiess AE. Reindeer and caribou hunters: an archaeological study. New York: Academic Press; 1979.
- 36. Krupnik I. Arctic adaptations: native whalers and reindeer herders of northern Eurasia. Expanded English ed. Hanover NH: University Press of New England [for] Dartmouth College; 1993.
- PubMed/NCBI
- 42. Parlee B, Thorpe Natasha, McNabb T. Traditional knowledge: barren-ground caribou in the Northwest Territories. Edmonton, AB: University of Alberta; 2013 p. 95.
- 44. Turov MG. Khoziaistvo Evenkov Taezhnoi Zony Srednei Sibiri v Kontse XIX- NachaleXXv. Irkutsk: Izdatel’stvo Irkutskogo Gosudarstvennogo Universiteta; 1990. Russian.
- 46. Hillson S. Teeth Cambridge, UK; New York: Cambridge University Press; 2005.
- 51. Reitz EJ, Wing ES. Zooarchaeology. 2nd ed. Cambridge: Cambridge University Press; 2008.
- 61. Miller FL. Biology of the Kaminuriak population of barren-ground caribou Part 2: Dentition as an indicator of age and sex. Ottawa: Spalding Printing Co. Ltd.; 1974.
- 66. Gifford-Gonzalez D. and Refining the Quadratic Crown Height Method of Age Estimation. In: Stiner M, editor. Human Predators and Prey Mortality. Milton: Routledge; 1991. pp. 41–78.
- 70. BQCMB. Beverly and Qamamirjuaq Caribou Management Plan 2013–2022. Stonewall, Manitoba: BQCMB Secretariat; 2014.
- 73. Worthington L, Ganton A, Leclerc L-M, Davison T, Wilson J, Duclos I. Management Plan for the Dolphin and Union Caribou (Rangifer tarandus groenlandicus x pearyi) in the Northwest Territories and Nunavut. Ottawa: Environment and Climate Change Canada; 2018.
- 74. Parker GR. Biology of the Kaminuriak population of barren-ground caribou Part 1: Total numbers, mortality, recruitment, and seasonal distribution. Ottawa: Spalding Printing Co. Ltd.; 1972.
Thank you for visiting nature.com. You are using a browser version with limited support for CSS. To obtain the best experience, we recommend you use a more up to date browser (or turn off compatibility mode in Internet Explorer). In the meantime, to ensure continued support, we are displaying the site without styles and JavaScript.
- View all journals
- My Account Login
- Explore content
- About the journal
- Publish with us
- Sign up for alerts
- Open access
- Published: 02 April 2024
Efficient DNA-based data storage using shortmer combinatorial encoding
- Inbal Preuss 1 , 3 ,
- Michael Rosenberg 2 ,
- Zohar Yakhini 1 , 3 &
- Leon Anavy 1 , 3
Scientific Reports volume 14 , Article number: 7731 ( 2024 ) Cite this article
2 Altmetric
Metrics details
- Computational biology and bioinformatics
- Computational methods
- Computational science
- Computer science
- DNA and RNA
- DNA computing
- Information technology
Data storage in DNA has recently emerged as a promising archival solution, offering space-efficient and long-lasting digital storage solutions. Recent studies suggest leveraging the inherent redundancy of synthesis and sequencing technologies by using composite DNA alphabets. A major challenge of this approach involves the noisy inference process, obstructing large composite alphabets. This paper introduces a novel approach for DNA-based data storage, offering, in some implementations, a 6.5-fold increase in logical density over standard DNA-based storage systems, with near-zero reconstruction error. Combinatorial DNA encoding uses a set of clearly distinguishable DNA shortmers to construct large combinatorial alphabets, where each letter consists of a subset of shortmers. We formally define various combinatorial encoding schemes and investigate their theoretical properties. These include information density and reconstruction probabilities, as well as required synthesis and sequencing multiplicities. We then propose an end-to-end design for a combinatorial DNA-based data storage system, including encoding schemes, two-dimensional (2D) error correction codes, and reconstruction algorithms, under different error regimes. We performed simulations and show, for example, that the use of 2D Reed-Solomon error correction has significantly improved reconstruction rates. We validated our approach by constructing two combinatorial sequences using Gibson assembly, imitating a 4-cycle combinatorial synthesis process. We confirmed the successful reconstruction, and established the robustness of our approach for different error types. Subsampling experiments supported the important role of sampling rate and its effect on the overall performance. Our work demonstrates the potential of combinatorial shortmer encoding for DNA-based data storage and describes some theoretical research questions and technical challenges. Combining combinatorial principles with error-correcting strategies, and investing in the development of DNA synthesis technologies that efficiently support combinatorial synthesis, can pave the way to efficient, error-resilient DNA-based storage solutions.
Similar content being viewed by others
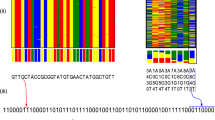
Data storage in DNA with fewer synthesis cycles using composite DNA letters
Leon Anavy, Inbal Vaknin, … Zohar Yakhini
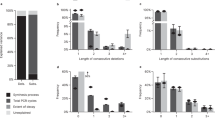
A digital twin for DNA data storage based on comprehensive quantification of errors and biases
Andreas L. Gimpel, Wendelin J. Stark, … Robert N. Grass
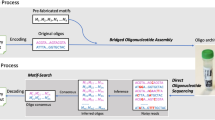
Scaling logical density of DNA storage with enzymatically-ligated composite motifs
Yiqing Yan, Nimesh Pinnamaneni, … Raja Appuswamy
Introduction
DNA is a promising media storage candidate for long-term data archiving, due to its high information density, long-term stability, and robustness. In recent years, several studies have demonstrated the use of synthetic DNA for storing digital information on a megabyte scale, exceeding the physical density of current magnetic tape-based systems by roughly six orders of magnitude 1 , 2 . Physical density is one of several quantitative metrics for evaluating the efficiency of DNA-based storage systems, measured by the data unit per gram of DNA. Another performance metric, which was introduced in 3 , is called logical density, refering to the amount of data encoded in each synthesis cycle. Since DNA synthesis is the main cost component in DNA-based storage systems, increasing the logical density is the main focus of this work.
Research efforts in the field of DNA-based storage systems have mainly focused on the application of various encoding schemes, while relying on standard DNA synthesis and sequencing technologies. These include the development of error-correcting codes for the unique information channel of DNA-based data storage 4 , 5 , 6 , 7 , 8 . Random access capabilities for reading specific information stored in DNA also require advanced coding schemes 9 , 10 , 11 . Yet, despite the enormous benefits potentially associated with capacity, robustness, and size, existing DNA-based storage technologies are characterized by inherent information redundancy. This is due to the nature of DNA synthesis and sequencing methodologies, which process multiple molecules that represent the same information bits in parallel. Recent studies suggest exploiting this redundancy to increase the logical density of the system, by extending the standard DNA alphabet using composite letters (also referred to as degenerate bases), and thereby encoding more than 2 bits per letter 12 , 13 . In this approach, a composite DNA letter uses all four DNA bases (A, C, G, and T), combined or mixed in a specified predetermined ratio \(\sigma =({\sigma }_{A},{\sigma }_{C},{\sigma }_{G},{\sigma }_{T})\) . A resolution parameter \(k={\sigma }_{A}+{\sigma }_{C}+{\sigma }_{G}+{\sigma }_{T}\) is defined, for controlling the alphabet size. The full composite alphabet of resolution \(k\) , denoted \({\Phi }_{k}\) , is the set of all composite letters, so that \({\Sigma }_{i\in (A,C,G,T\}}{\sigma }_{i}=k\) . Writing a composite letter is done by using a mixture of the DNA bases, determined by the letter’s ratio in the DNA synthesis cycle. Current synthesis technologies produce multiple copies, and by using the predetermined base mixture each copy will contain a different base, thus preserving the ratio of the bases at the sequence-population level.
While the use of numerical ratios supports higher logical density in composite synthesis, it also introduces challenges related to the synthesis and inference of exact ratios. Combinatorial approaches, which also consist of mixtures, address these challenges in a different way. Studies by Roquet et al. (2021) and Yan et al. (2023) contribute significantly to advancing DNA-based data storage technology. To encode and store data, Roquet et al. focus on a novel combinatorial assembly method for DNA. Yan et al. extend the frontiers of this technology by enhancing the logical density of DNA storage, using enzymatically-ligated composite motifs 13 , 14 .
In this paper, we present a novel approach for encoding information in DNA, using combinatorial encoding and shortmer DNA synthesis, leading to an efficient sequence design and improved DNA synthesis and readout interpretation. The method described herein leverages the advantages of combinatorial encoding schemes while relying on existing DNA chemical synthesis methods with some modifications. Using shortmer DNA synthesis also minimizes the effect of synthesis and sequencing errors. We formally define shortmer-based combinatorial encoding schemes, explore different designs, and analyze their performance. We use computer-based simulations of an end-to-end DNA-based data storage system built on combinatorial shortmer encodings, and study its performance. To demonstrate the potential of our suggested approach and experimentally test its validity, we performed an assembly-based molecular implementation of the proposed combinatorial encoding scheme and analyzed the resulting data. Finally, we discuss the potential of combinatorial encoding schemes and the future work required to enable these schemes in large-scale DNA-based data storage systems and other DNA data applications.
Design of shortmer combinatorial encoding for DNA storage
We suggest a novel method to extend the DNA alphabet while ensuring near-zero error rates.
Let \(\Omega\) be a set of DNA k-mers that will serve as building blocks for our encoding scheme. Denote the elements in \(\Omega\) as \({X}_{1},\dots ,{X}_{N}\) . Elements in \(\Omega\) are designed to be sufficiently different from each other, to minimize mix-up error probability. Formally, the set is designed to satisfy \(d\left({X}_{i},{X}_{j}\right)\ge d;\forall i\ne j\) , with the minimal Hamming distance \(d\) serving as a tunable parameter.
Other design criteria can be applied to the shortmers in \(\Omega\) , taking into consideration the properties of DNA synthesis, manipulation, and sequencing. These may include minimal Levenshtein distance, GC context, and avoiding long homopolymers. Clearly, any such filtering process will result in reduced alphabet size and reduced logical density.
Note that \(N=\left|\Omega \right|\le {4}^{k}\) . The elements in \(\Omega\) will be used as building blocks for combinatorial DNA synthesis in a method similar to the one used for composite DNA synthesis 3 . Examples of k-mer sets \(\Omega\) are presented in Supplementary Sect. 8.3 .
We define a combinatorial alphabet \(\Sigma\) over \(\Omega\) as follows. Each letter in the alphabet represents a non-empty subset of the elements in \(\Omega\) . Formally, a letter \(\sigma \in \Sigma\) , representing a subset \(S\subseteq \Omega /\varnothing\) , can be written as an N-dimensional binary vector where the indices for which \({\sigma }_{i}=1\) represents the k-mers from \(\Omega\) included in the subset S. We denote the k-mers in \(S\) as member k-mers of the letter \(\sigma\) . For example, \(\sigma =(\mathrm{0,1},\mathrm{0,1},\mathrm{1,0})\) represents \(S=\{{X}_{2},{X}_{4},{X}_{5}\}\) and \(\left|\Omega \right|=N=6\) . Figure 1 a,b illustrate an example of a combinatorial alphabet using \(N=16\) , in which every letter represents a subset of size 5 of \(\Omega\) . In Sect. “ Binary and binomial combinatorial alphabets ” includes a description of the construction of different combinatorial alphabets.
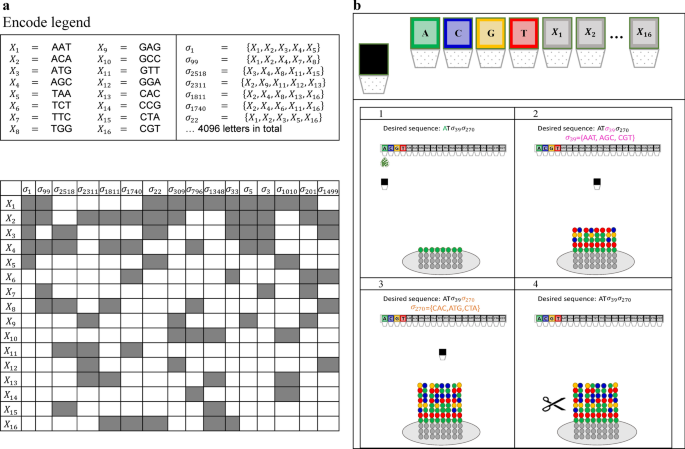
Our combinatorial encoding and synthesis approach. ( a ) Schematic view of a combinatorial alphabet (Encode legend). A set of 16 trimers, \({{\varvec{X}}}_{1},\dots ,{{\varvec{X}}}_{16}\) , is used to construct 4096 combinatorial letters, each representing a subset of 5 trimers as indicated on the right and depicted in the grayed-out cells of the table. ( b ) A suggested approach for combinatorial shortmer synthesis. A modified synthesizer would include designated containers for the 16-trimer building blocks and a mixing chamber. Standard DNA synthesis is used for the barcode sequence (1), while the combinatorial synthesis proceeds as follows: The trimers included in the synthesized combinatorial letter are injected into the mixing chamber and introduced into the elongating molecules (2). The process repeats for the next combinatorial letter (3), and finally, the resulting molecules are cleaved and collected (4).
To write a combinatorial letter \(\sigma\) in a specific position, a mixture of the member k-mers of \(\sigma\) is synthesized. To infer a combinatorial letter \(\sigma\) , a set of reads needs to be analyzed to determine which k-mers are observed in the analyzed position (See Sects. “ Binary and binomial combinatorial alphabets ” and “ Reconstruction probabilities for binomial encoding ” for more details). This set of k-mers observed in the sequencing readout and used for inferring \(\sigma\) is referred to as inferred member k-mers. While the synthesis output and the sequencing readout will include different counts for the member k-mers, the determination of the set of inferred k-mers will force binary assignment for each k-mer to fit into the design scheme of combinatorial encoding.
From a hardware/chemistry perspective, the combinatorial shortmer encoding scheme can potentially be based on using the standard phosphoramidite chemistry synthesis technology, with some alterations (See Fig. 1 b and Supplementary Sect. 8.1 ) 15 , 16 . First, DNA k-mers should be used as building blocks for the synthesis 17 . Such reagents are commercially available for DNA trimers and were used, for example, for the synthesis of codon optimization DNA libraries 18 , 19 . In addition, a mixing step should be added to each cycle of the DNA synthesis to allow mixing of the member k-mers prior to their introduction to the elongating molecules. Such systems are yet to be developed and current attempts for combinatorial DNA synthesis are based on enzymatic assembly of longer DNA fragments 13 , 14 .
Similar to composite DNA encoding, combinatorial encoding requires the barcoding of the sequences using unique barcodes composed of standard DNA barcodes. This design enables direct grouping of reads pertaining to the same combinatorial sequence. These groups of reads are the input for the process of reconstructing the combinatorial letters.
The extended combinatorial alphabets allow for a higher logical density of the DNA-based storage system, while the binary nature of the alphabet minimizes error rates.
Binary and binomial combinatorial alphabets
The main parameter that defines a combinatorial encoding scheme is the alphabet \(\Sigma\) . More specifically, it is the set of valid subsets of \(\Omega\) that can be used as letters. We define two general approaches for the construction of \(\Sigma\) . Namely, the binomial encoding and the full binary encoding .
In the binomial encoding scheme, only subsets of \(\Omega\) of size exactly \(K\) represent valid letters in \(\Sigma\) , so that every letter \(\sigma \in \Sigma\) consists of exactly \(K\) member k-mers. Therefore, all the letters in the alphabet have the same Hamming weight \(K\) . \(w\left(\sigma \right)=K, \forall \sigma \in \Sigma\) . This yields an effective alphabet of size \(\left|\Sigma \right|=\left(\begin{array}{c}N\\ K\end{array}\right)\) letters, where each combinatorial letter encodes \({{\text{log}}}_{2}\left(\left|\Sigma \right|\right)={{\text{log}}}_{2}\left(\begin{array}{c}N\\ K\end{array}\right)\) bits. An r-bit binary message requires \(\frac{r}{{{\text{log}}}_{2}\left(\begin{array}{c}N\\ K\end{array}\right)}\) synthesis cycles (and a DNA molecular segment with length \(\frac{kr}{{{\text{log}}}_{2}\left(\begin{array}{c}N\\ K\end{array}\right)}\) ). In practice, we would prefer working with alphabet sizes that are powers of two, where each letter will encode for \(\left\lfloor {\log_{2} \left( {\begin{array}{*{20}c} N \\ K \\ \end{array} } \right)} \right\rfloor\) bits. Note that this calculation ignores error correction redundancy, random access primers, and barcodes, which are all required for message reconstruction. See Supplementary Sect. 8.2 and Fig. 1 a, which illustrate a trimer-based binomial alphabet with \(N=16\) and \(K=5\) , resulting in an alphabet of size \(\left|\Sigma \right|=\left(\begin{array}{c}16 \\ 5 \end{array}\right)=\mathrm{4,368}\) that allows to encode \(\lfloor {log}_{2}(4368)\rfloor =12\) bits per letter or synthesis position.
In the full binary encoding scheme, all possible nonempty subsets of \(\Omega\) represent valid letters in the alphabet. This yields an effective alphabet of size \(\left|\Sigma \right|={2}^{N}-1\) letters, each encoding for \(\left\lfloor {\log_{2} \left( {\left| \Sigma \right|} \right)} \right\rfloor = N - 1\) bits.
From this point on, we focus on the binomial encoding.
Reconstruction probabilities for binomial encoding
In this section, the performance characteristics of binomial encoding are investigated. Specifically, we present a mathematical analysis of the probability of successfully reconstructing the intended message. In Sects. “ An end-to-end combinatorial shortmer storage system ” and " Experimental proof of concept ", results are presented from our simulations and a small-scale molecular implementation of the binomial encoding, respectively.
Reconstruction of a single combinatorial letter
Since every letter \(\sigma \in \Sigma\) consists exactly of the \(K\) member k-mers, the required number of reads for observing at least one read of each member k-mer in a single letter follows the coupon collector distribution 20 . The number of reads required to achieve this goal can be described as a random variable \(R={\sum }_{i=1}^{K}{R}_{i}\) , where \({R}_{1}=1\) and \({R}_{i}\sim Geom\left(\frac{K-i+1}{K}\right), i=2,\dots ,K\) . Hence, the expected number of required reads, is:
where \(Har(\cdot )\) is the harmonic number.
The expected number of reads required for reconstructing a single combinatorial letter thus remains reasonable for the relevant values of \(K\) . For example, when using a binomial encoding with \(K=5\) the expected number of reads required for reconstructing a single combinatorial letter is roughly \(11.5\) , which is very close to the experimental results presented in Sect. " Experimental proof of concept ".
By Chebyshev’s inequality (See Sect. " Reconstruction probability of a binomial encoding letter "), we can derive a (loose) upper bound on the probability of requiring more than \(E\left[R\right]+cK\) reads to observe at least one read of each member k-mer, where \(c>1\) is a parameter:
For example, when using a binomial encoding with \(K=5\) , the probability of requiring more than \(26.5\) reads (corresponding to \(c=3\) ) is bounded by \(0.18\) , which is consistent with the experimental result shown in Fig. 5 d.
Reconstruction of a combinatorial sequence
When we examine an entire \(K\) -subset binomial encoded combinatorial sequence of length \(l\) , we denote by \(R(l)\) the required number of reads to observe \(K\) distinct k-mers in every position. Assuming independence between different positions and not taking errors into account, we get the following relationship between \(c\) and any desired confidence level \(1-\delta\) (See Sect. " Reconstruction probability of a binomial encoding letter " for details):
And therefore:
The number of reads required to guarantee reconstruction of a binomial encoded message, at a \(1-\delta\) probability, with \(K=5,\) and \(l\) synthesized positions, is thus \(KHar\left(K\right)+cK\) when
Supplementary Table S2 shows several examples of this upper bound. As demonstrated in the simulations and the experimental results, this bound is not tight (See Sects. “ An end-to-end combinatorial shortmer storage system ” and " Experimental proof of concept ").
Note that with an online sequencing technology (such as nanopore sequencing) the sequencing reaction can be stopped after \(K\) distinct k-mers are confidently observed.
To take into account the probability of observing a k-mer that is not included in \(\Omega\) (e.g., due to synthesis or sequencing error), we can require that at least \(t>1\) reads of each of the \(K\) distinct k-mers will be observed. This is experimentally examined in Sect. " Experimental proof of concept ", while the formal derivation of the number of required reads is not as trivial, and will be addressed in future work.
The above analysis is based only on oligo recovery, which depends solely on the sampling rate, ignoring possible mix-up errors (i.e., incorrect k-mer readings). This assumption is based on the near-zero mix-up probability attained by the construction of \(\Omega\) , which maximizes the minimal Hamming distance between elements in \(\Omega\) . In Sect. " Experimental proof of concept ", this analysis is compared to experimental results obtained from using synthetic combinatorial DNA.
An end-to-end combinatorial shortmer storage system
We suggest a complete end-to-end workflow for DNA-based data storage with the combinatorial shortmer encoding presented in Fig. 2 . The workflow begins with encoding, followed by DNA synthesis, storage, and sequencing, and culminates in a final decoding step. A 2D Reed-Solomon (RS) error correction scheme, which corrects errors in the letter reconstruction (for example, due to synthesis, sequencing, and sampling errors) and any missing sequences (such as dropout errors), ensures the integrity of the system. Table 1 shows the encoding capacities of the proposed system, calculated on a 1 GB input file with standard encoding and three different binomial alphabets (See Supplementary Sect. 8.5 ). All calculations are based on error correction parameters similar to those previously described (See Sect. " Information capacities for selected encodings ") 3 , 4 , 21 , 22 . With these different alphabets, up to a 6.5-fold increase in information capacity is achieved per synthesis cycle, compared to standard DNA-based data storage. While different error correction codes can be used in this system, for our work we chose to implement a 2D RS.
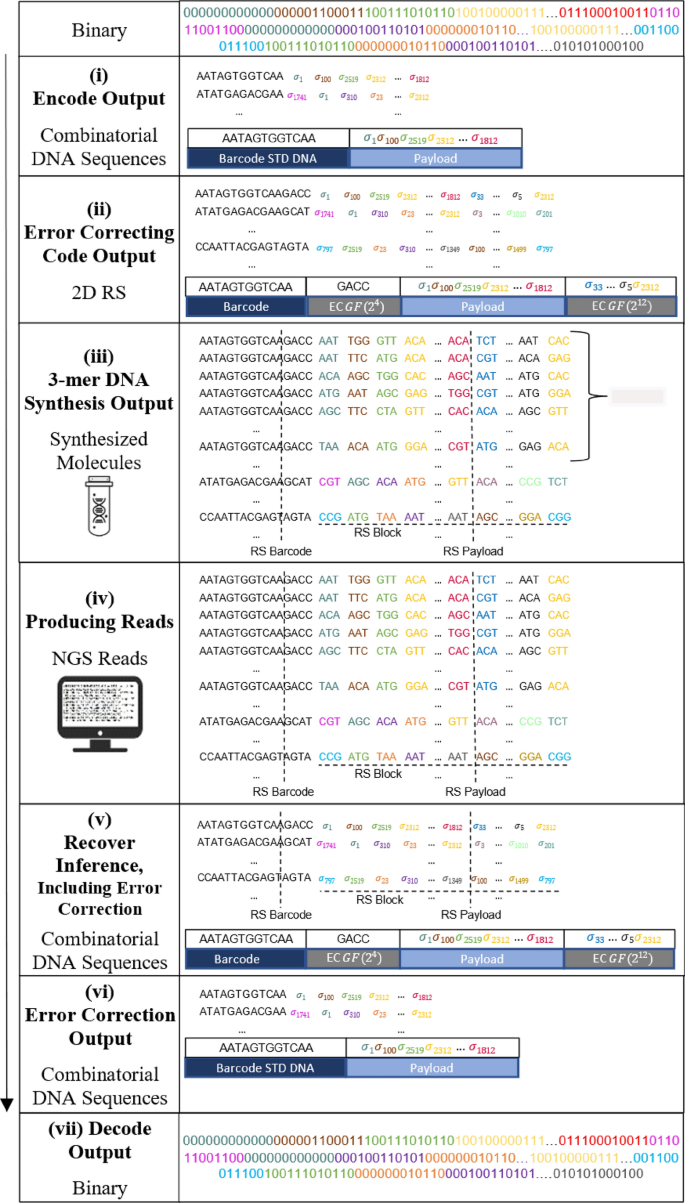
End-to-end workflow of a combinatorial DNA storage system. A binary message is broken into chunks, barcoded, and encoded into a combinatorial alphabet (i). RS encoding is added to each chunk and each column (ii). The combinatorial message is synthesized using combinatorial shormer synthesis (iii), and the DNA is sequenced (iv). Next, the combinatorial letters are reconstructed (v). Finally the message goes through 2D RS decoding (vi), followed by its translation back into the binary message (vii).
An example of the proposed approach, using a binomial alphabet with \(N=16\) and \(K=5\) and 2D RS, is detailed below. A binary message is encoded into a combinatorial message using the 4096-letter alphabet. Next, the message is broken into 120-letter chunks, and each chunk is barcoded. The 12nt barcodes are encoded using RS(6,8) over \(GF({2}^{4})\) , resulting in 16nt barcodes. Each chunk of 120 combinatorial letters is encoded using RS(120,134) over \(GF({2}^{12})\) . Every block of 42 sequences is then encoded using RS(42,48) over \(GF\left({2}^{12}\right)\) (See Sect. " An end-to-end combinatorial storage system " for details).
To better characterize the potential of this proposed system, we implemented an end-to-end simulation using the parameters mentioned above. We simulated the encoding and decoding of 10 KB messages with different binomial alphabets and error probabilities, and then measured the resulting reconstruction and decoding rates throughout the process. Figure 3 a depicts a schematic representation of our simulation workflow and indicates how the error rates are calculated (See Sect. " Reconstruction ").
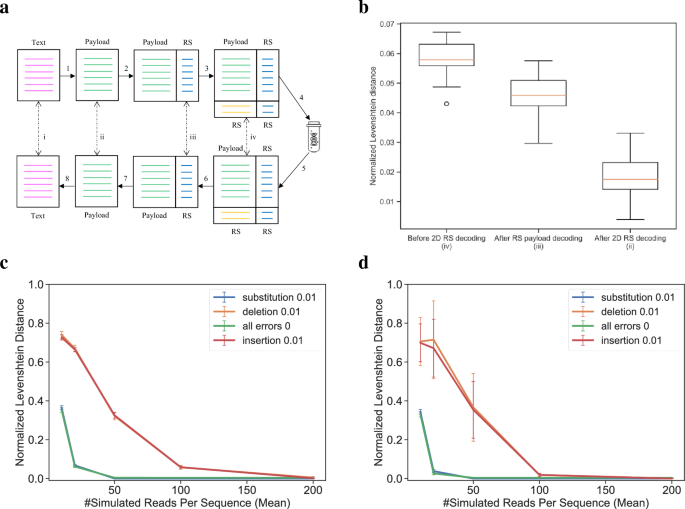
Simulation of an end-to-end combinatorial shortmer encoding. ( a ) A schematic view of the simulation workflow. A text message is translated into a combinatorial message (1), and encoded using RS error correction on the barcode and payload (2). Each block is encoded using outer RS error correction (3). DNA synthesis and sequencing are simulated under various error schemes, and the combinatorial letters are reconstructed (4–5). RS decoding is performed on each block (6) and on each sequence (7) before translation back to text (8). The Roman numerals (i-iv) represent the different error calculations. ( b ) Error rates in different stages of the decoding process. Boxplot of the normalized Levenshtein distance (See Sect. " Reconstruction ") for the different stages in a simulation (30 runs) of sampling 100 reads, with an insertion error rate of 0.01. The X-axis represents the stages of error correction (before 2D RS decoding (iv), after RS payload decoding (iii), and after 2D RS decoding (ii)). ( c , d ) Sampling rate effect on overall performance. Normalized Levenshtein distance as a function of sampling rate before RS decoding ( c ) and after 2d RS decoding (ii). Different lines represent different error types (substitution, deletion, and insertion) introduced at a rate of 0.01.
The results of the simulation runs are summarized in Fig. 3 b–d. Each run included 30 repeats with random input texts of 10 KB encoded using 98 combinatorial sequences, each composed of 134 combinatorial letters and 16nt barcode, as described above. Each run simulated the synthesis of 1000 molecules on average per combinatorial sequence and sampling of a subset of these molecules to be sequenced. The subset size was drawn randomly from \(N\left(\mu ,\sigma =100\right),\) where \(\mu\) is a parameter. Errors in predetermined rates were introduced during the simulation of both DNA synthesis and sequencing, as expected in actual usage 23 (See Sect. " Synthesis and sequencing simulation with errors " for details on the simulation runs). Reconstruction rates and Levenshtein distances are calculated throughout the simulation process, as described in Fig. 3 a.
Notably, the sampling rate is the dominant factor where even with zero synthesis and sequencing errors, low sampling rates yield such poor results (Fig. 3 c) that the RS error correction is unable to overcome (Fig. 3 d). The effect of substitution errors on the overall performance is smaller and they are also easier to detect and correct. This is because substitution errors occur at the nucleotide level rather than at the trimer level. The minimal Hamming distance \(d=2\) of the trimer set \(\Omega\) allows for the correction of single-base substitutions. The use of 2D RS error correction significantly improved reconstruction rates, as can be observed in Fig. 3 b.
To assess the effect of using the suggested approach on the cost of DNA-based data storage systems, we performed an analysis of the different cost components. In brief, we analyzed the effect on the number of synthesis cycles and the number of bases to sequence, taking into account the required sequencing depth to achieve a desired reconstruction probability (See Sect. " Cost analysis "). Figure 4 depicts the costs of storing 1 GB of information using different combinatorial alphabets. Clearly, combinatorial DNA encoding can potentially reduce DNA-based data storage costs as the alphabet size grows and each letter encodes more bits. This is especially relevant in comparison with the composite encoding scheme presented in 3 . While both methods increase the logical density by extending the alphabet using mixtures of DNA letters/k-mers and thus reducing the synthesis cost (See Fig. 4 a), a crucial difference lies in the effect on sequencing costs. Composite DNA uses mixed letters with varying proportions of the different letters, which makes reconstruction very challenging in larger alphabets and results in very high sequencing costs that undermine the reduced synthesis costs. On the other hand, combinatorial DNA encoding uses binary mixtures, which are much simpler to reconstruct, therefore maintaining the sequencing costs relatively constant as the alphabet grows (See Fig. 4 b). For assessing the sequencing costs, we used a coupon collector model presented in 24 to calculate the required sequencing depth that ensures a decoding probability with an error rate of less than \({10}^{-4}\) (See Supplementary Sect. 8.5 ). In comparison with the composite encoding scheme, our analysis demonstrates a required sequencing depth that grows moderately. Figure 4 c analyzes the normalized overall cost, based on different assumptions regarding the ratio between synthesis costs and sequencing costs, \({C}_{syn}:{C}_{seq}\) . With a cost ratio of 500:1, 1000:1, 2000:1, it is evident that synthesis costs outweigh the fluctuations in sequencing costs, indicating a monotonic reduction in overall costs. This is an improvement compared to the composite DNA approach presented in 3 , where costs are reduced only up to a certain alphabet size, and then increase again due to the increased sequencing cost. In combinatorial DNA encoding, costs continue to drop, while alphabet size increases.
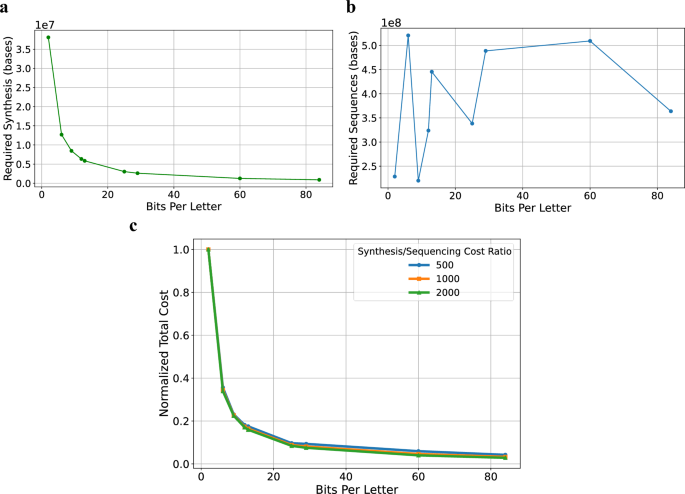
Cost analysis for a combinatorial DNA-based data storage system using different alphabets. ( a ) synthesis cost as a function of the alphabet size (presented as bit per letter, for simplicity). The cost is calculated as the number of synthesis cycles required for storing 1 GB of information. ( b ) Sequencing cost as a function of the alphabet size. Similarly to ( a ). ( c ) Normalized total cost as a function of the alphabet size for different synthesis-to-sequencing cost ratios. Costs are normalized by the total cost of a standard DNA-based system.
Experimental proof of concept
To assess and establish the potential of large combinatorial alphabets, we performed a small-scale experimental proof of concept study demonstrating the encoding and decoding of a 96-bit input message, which is equivalent to the text “DNA Storage!”. Since combinatorial DNA synthesis technology is not yet available, we demonstrated the combinatorial approach using Gibson assembly as an ad-hoc imitation for combinatorial synthesis. We constructed two combinatorial sequences, each containing a barcode and four payload cycles over a binomial alphabet with \(N=16\) and \(K=5\) . The assembly was performed using DNA fragments composed of a 20-mer information sequence and an overlap of 20 bp between adjacent fragments, as shown in Fig. 5 a. The assembled DNA was then stored and sequenced for analysis using Illumina Miseq (See Table 3 and Sect. " Cost analysis " for details about the sequencing procedures).
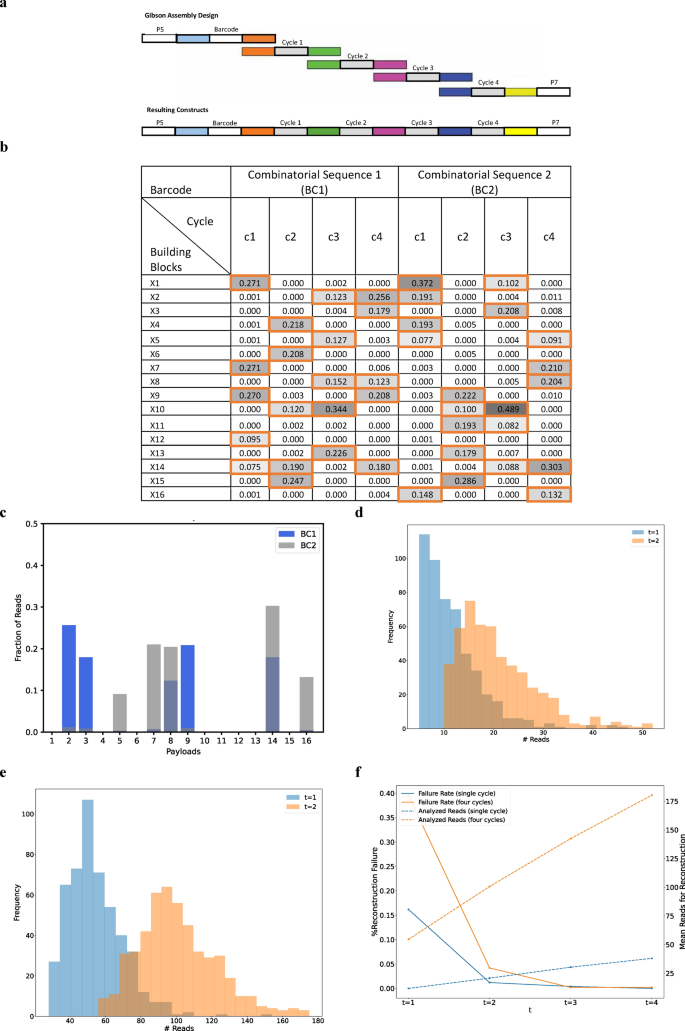
Experiment analysis. ( a ) A schematic view of the Gibson assembly. Each combinatorial sequence consists of a barcode segment and four payload segments (denoted as cycles 1–4). ( b ) Reconstruction results of the two combinatorial sequences. The color indicates read frequency, and the member k-mers are marked with orange boxes. ( c ) The distribution of reads over the 16 k-mers in an example combinatorial letter. Overlaid histograms represent the percentage of reads for each of the 16 k-mers for the same position in our two combinatorial sequences. This, in fact, is an enlarged view of the two c4 columns of panel b. ( d ) Required number of reads for reconstructing a single combinatorial letter. A histogram of the number of reads required to observe at least \(t=\mathrm{1,2}\) reads from \(K=5\) inferred k-mers. The results are based on resampling the reads 500 times, the data represents cycle 4. ( e ) Required number of reads for reconstructing a four-letter combinatorial sequence. Similar to d. ( f ), Reconstruction failure rate as a function of the required multiplicity \(t\) . Erroenous reconstruction rate shown for different values of required copies to observe each inferred k-mer ( \(t=\mathrm{1,2},\mathrm{3,4}\) ). The mean required number of reads for reconstruction is displayed using a secondary Y-axis in the dashed lines.
The sequencing output was then analyzed using the procedure described in Sect. “ Decoding and analysis ”. Both combinatorial sequences were successfully reconstructed from the sequencing reads, as presented in Fig. 5 b, and Supplementary Figs. S1, S2, and S3. The experiment also demonstrated the robustness of the binomial DNA encoding for synthesis and sequencing errors, as described in Fig. 5 c. We observed a minor leakage between the two synthesized sequences, which was overcome by the reconstruction pipeline (See Fig. 5 c, and Supplementary Figs. S1 , S2 , and S3 ). Note that there is a partial overlap between the member k-mers of the two sequences.
For comparison, a recent study by 14 encoded the 84-bit phrase “HelloWord” using a different encoding and synthesis approach. A comparison between the two experiments is shown in Table 2 . For example, while we used Gibson assembly as our synthesis method, they introduced a new method called Bridge Oligonucleotide Assembly. We encoded 12 bits per synthesis cycle and assembled four combinatorial fragments in each sequence, while 14 encoded 84 bits in a single combinatorial cycle. Our 96-bit were split and encoded using two combinatorial sequences, while they encoded the same 84-bits message, in its full format, on eight different sequences repeatedly. Our \({\text{N}}=16\) and 5 combinatorial factor, while their \(N=96\) and a higher 32 combinatorial factor.
To test the effect of random sampling on the reconstruction of combinatorial sequences, we performed a subsampling experiment with \(N=500\) repeats, presented in Fig. 5 d–f. We subsampled varying numbers of reads from the overall read pool and ran the reconstruction pipeline. Note that, as explained, the reconstruction of a single binomial position requires finding \(K=5\) inferred k-mers. That is, observing five unique k-mers at least \(t\) times. We tested the reconstruction performance using \(t=\mathrm{1,2},\mathrm{3,4}\) and recorded the effect on the successful reconstruction rate and required number of reads.
For \(t=1\) , reconstruction required analyzing 12.26 reads on average. These included 0.45 reads that contained an erroneous sequence that could not be mapped to a valid k-mer, and thus ignored. Note that the design of the set \(\Omega\) of valid k-mers allows us to ignore only the reads for which the Hamming distance for a valid k-mer exceeded a predefined threshold ( \(d=3\) ). If we ignored all the reads containing a sequence with non-zero Hamming distance to all k-mers, we would have skipped 2.26 extra reads, on average.
As expected, requiring \(t=2\) copies of each inferred k-mer resulted in an increase in the overall number of analyzed reads. Reconstruction of a single combinatorial letter required analyzing an average of 21.6 reads with 0.83 skipped and 3.99 non-zero Hamming distance reads. The complete distribution of the number of reads required for the reconstruction of a single position using \(t=\mathrm{1,2}\) is presented as a histogram in Fig. 5 d.
To reconstruct a complete combinatorial sequence of 4 positions, we required the condition to hold for all positions. For \(t=1\) , this entailed the analysis of 55.60 reads on average, out of which 1.04 reads were identified as erroneous and thus ignored, and with 7.36 non-zero Hamming distance reads. For \(t=2\) , an average of 102.66 reads were analyzed with 1.97 skipped and 13.24 non-zero Hamming distance reads. The complete distribution of the number of reads required for reconstructing a complete combinatorial sequence using \(t=\mathrm{1,2}\) is presented as a histogram in Fig. 5 e.
Note that these results correspond to the analysis presented in Sect. “ Reconstruction probabilities for binomial encoding ”, for the reconstruction of a single binomial position and a complete binomial sequence. Calculating the bound presented in Supplementary Table S2 , with \(K=5\) and \(l=4\) , yields a requirement of approximately 140 reads to obtain \(1-\delta =0.99\) probability of reconstruction. Clearly, this is well above the observed number of 55.60 reads. Note, as explained, the calculated bound is a loose bound.
The reconstruction procedure ends with a set of inferred k-mers that represent the inferred combinatorial letter. This set is not guaranteed to be correct, especially when using \(t=1\) , which means that noisy reads may result in an incorrect k-mer included in the inferred letter. Figure 5 f depicts the rate of incorrect reconstructions as a function of the number of required copies for each inferred k-mer ( \(t=\mathrm{1,2},\mathrm{3,4}\) ). Note that with \(t\ge 3\) results in 100% successful reconstruction. This, however, comes with a price, where more reads must be analyzed.
In this study, we introduced combinatorial shortmer encoding for DNA-based data storage, which extends the approach of composite DNA by resolving some of its sensitivity related issues. Combinatorial shortmer encoding allows for increased logical density while maintaining low error rates and high reconstruction rates. We explored two encoding schemes, binary and binomial, and evaluated some of their theoretical and practical characteristics. The inherent consistency of the binomial encoding scheme, where every letter in the sequence consists of exactly \(K\) distinct member k-mers, ensures uniformity in the encoded DNA sequences. This approach not only simplifies the reading process, but also allows for a more streamlined decoding. For instance, technologies like nanopore sequencing enable continuous sequencing until all k-mers at a given position are confidently observed.
Our suggested approach is designed to inherently overcome base substitution errors, which are the most common errors expected in every DNA-based data storage system that includes DNA sequencing. This is achieved by the selection of a set of \(N\) k-mer building blocks to be resilient to single-base substitutions. Other considerations may also be incorporated in the selection of the set of valid k-mers, taking into account any biological, chemical, or technological constraints. This represents an inherent tradeoff in DNA-based data storage between sequence constraints and information density. Insertion and deletion errors, which usually originate in the synthesis process, are more challenging to overcome. We introduced a 2D RS error correction scheme on the shortmer level, allowing for a successful message reconstruction even with error levels exceeding those expected in reality.
Our study highlights the significant effect of sampling rates on the overall performance of the system. The accuracy and completeness of sequence reconstruction require each of the sequences to be observed with a sufficiently high coverage. Our subsampling experiments underpin this observation, demonstrating the need for calibration of sampling rates to ensure the desired fidelity in DNA-based data storage. The crucial role of the sampling rate was also highlighted in 3 . However, while composite DNA uses mixed letter with varying proportions of the different letters, the combinatorial encoding, studied in this current work, uses binary mixtures and does not rely on proportions. This potentially allows scaling up the combinatorial encoding without a significant effects on the required sampling rates.
Combinatorial DNA coding can potentially reduce the overall costs of DNA-based data storage. Considering both sequencing costs, which fluctuate, and synthesis costs, which consistently drop, the increase in the alphabet size is accompanied by a decrease in overall cost. However, combinatorial DNA synthesis or assembly is still unavailable for large-scale commercial use. Thus, further development of combinatorial DNA synthesis technologies will continue to impose limitations and constraints on combinatorial encoding, and determine the overall costs.
While our proof-of-concept experiment showed success on a small scale, there are complexities to be addressed in considering large-scale applications. These include synthesis efficiency, error correction, and decoding efficiency. Nonetheless, the resilience of our binomial DNA encoding for both synthesis and sequencing errors highlights its practical potential and scalability. One specific aspect is the effect of combinatorial encoding on possible sequence-related constraints. While sequences with unwanted compositions (e.g., containing homopolymers) will unavoidably be part of the synthesized mixtures, the uniform sampling of the combinatorial shortmers in each position, together with the independence of the different positions, guarantees that only very few such sequences will be aythesized. In particular—these will not interfere with successful reconstruction. Another challenging aspect of scaling up combinatorial DNA systems is the need to use longer DNA k-mers to construct larger sets with the desired constraints. This may make the combinatorial synthesis impractical and will require balancing the increase in logical density with the technological complexity.
Several future research directions emerge from our study. First, it is important to develop error correction methods for better handling insertion and deletion errors. One approach for achieving this goal, is to adjust sampling rates: optimizing the sampling rate, especially in large-scale experiments, can lead to data retrieval at high accuracy. While our study highlighted the role of sampling rates in achieving desired outcomes, delving deeper into the underlying theory will lead to more improvements. For example, based on theoretical bounds of sampling rates, more concrete recommendations can be provided for real-world applications. The development of error correction codes, designed specifically to overcome the error types that characterize combinatorial encoding, is another important direction for future research. Most notably, transitioning from small-scale proof-of-concept experiments to larger-scale implementations is an important next step. Evaluating the scalability of our method across various scales and complexities will be enlightening, especially when considering synthesis efficiency and error rates. Finally, the consideration of advanced sequencing technologies could redefine the potential and efficacy of our proposed method, including its future practical implementation.
To sum up, combinatorial DNA synthesis and sequence design are important beyond the scope of DNA-based data storage. Generating combinatorial DNA libraries is an efficient tool in synthetic biology, better supporting large-scale experiments. DNA synthesis technologies that can incorporate a combinatorial synthesis of longer DNA fragments will enable the design and generation of more DNA libraries with applications in data storage and beyond.
Reconstruction probability of a binomial encoding letter
Let the number of reads required for reconstruction be a random variable \(R={\sum }_{i=1}^{K}{R}_{i}\) where \({R}_{1}=1\) and \({R}_{i}\sim Geom\left(\frac{K-i+1}{K}\right), i=2,\dots ,K\) . Hence, the expected number of required reads, is:
Using the independence of \({R}_{i}\) , the variance of \(R\) can be bound by (See 25 ):
By Chebyshev’s inequality, we get an upper bound (a loose bound) on the probability of requiring more than \(E\left[R\right]+cK\) reads to observe at least one read of each member k-mer:
Let \(c=b\frac{\pi }{\sqrt{6}}\) , or \(b=\frac{c\sqrt{6}}{\pi }\) , and we obtain:
Or specifically:
We now turn to address the reconstruction of an entire oligo of length \(l\) . Let \(R(l)\) be the random variable representing the number of reads required to have observed all the \(K\) member k-mers in every position. Setting any \(\delta >0\) , if we show that \(P\left(R\left(l\right)>m\right)\ge 1-\delta\) , then we know that by accumulating \(m\) reads the probability of correct full reconstruction is more than \(1-\delta\) . From Eq. ( 11 ), and assuming independence of the positions (in terms of observing all \(K\) member k-mers), we get Eq. ( 12 ):
From which we can extract \(c\) , so that:
Which yields:
This process allows us to evaluate the sequencing depth complexity. For example, consider \(l=100\) and \(\delta =0.01\) . We want to find \(c\) , so that using \(KHar\left(K\right)+cK\) reads will reconstruct the entire sequence with 0.99 probability. We therefore set:
And therefore, using 128 reads guarantees reconstruction with 0.99 probability.
An end-to-end combinatorial storage system
In Sect. “ An end-to-end combinatorial shortmer storage system ” we propose an end-to-end combinatorial storage system, as follows.
Combinatorial encoding and padding
A binary message is encoded using a large k-mer combinatorial alphabet (e.g., trimer-based alphabet of size \(\left|\Sigma \right|=4096\) letters, with \(N=\left|\Omega \right|=16\) ), resulting in \(r=12\) bits per combinatorial letter. The binary message is zero-padded to ensure its length is divisible by \(r\) prior to the combinatorial encoding. The complete message is broken into sequences of set length \(l=120\) , each sequence is then marked with a standard DNA barcode and translated using the table presented in the Encode legend (See Supplementary Sect. 8.2 ).
The length of the complete combinatorial sequence must be divisible by the payload size \(l\) and by the block size \(B\) . As described in Fig. 6 , this is ensured using another padding step, and the padding information is included in the final combinatorial sequence.
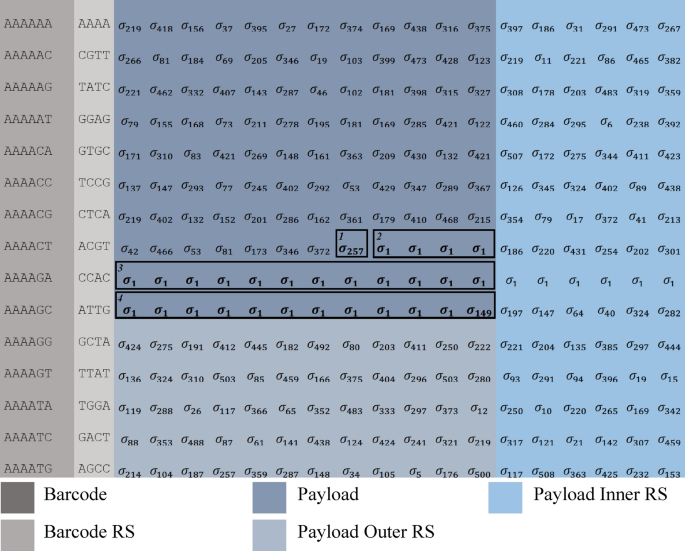
Example of message coding, including padding and RS error correction. Encoding of a ~ 0.1 KB message into a 512 letter binomial alphabet ( \(N=16, K=3)\) . First, bit padding is added, included here in the letter \(_{{\sigma_{257} }}^{1}\) . Next, block padding is added, included here in \(_{{\sigma_{1} }}^{2}\) and \(_{{\sigma_{1} }}^{3}\) . Padding information is included in the last sequence of all blocks. The last sequence holds the number of padding binary bits. In this example, \(_{{\sigma_{149} }}^{4}\) represents 148 bits of padding, composed of \(4+\left(4*9\right)+\left(12*9\right) bits\) , 4 bits from \(_{{\sigma_{257} }}^{1}\) , 4 letters from \(_{{\sigma_{1} }}^{2}\) and 12 letters from \(_{{\sigma_{1} }}^{3}\) .
Error correction codes
The 2D error correction scheme includes the use of three RS 26 encodings: on each barcode, on the payload part of each sequence, and an outer error correction code on each block of sequences.
Each barcode is encoded using a systematic RS(6,8) code over \(GF({2}^{4})\) , transforming the unique 12nt barcode into a 16nt sequence.
Each 120 combinatorial letter payload sequence is encoded using an RS(120,134) code over \(GF({2}^{12})\) , resulting in a sequence of length 134 combinatorial letters.
To protect against sequence dropouts, an outer error correction code is used on the columns of the matrix (See Fig. 6 ). Each block of \(B=42\) sequences, is encoded using a RS(42,48) RS code \(GF\left({2}^{12}\right)\) . This is applied in each column separately.
For simplicity, Fig. 6 demonstrates the encoding of ~ 0.1 KB using shorter messages with simpler error correction codes. The following parameters are used:
A barcode length of 6nt encoded using RS(3,5) code over \(GF\left({2}^{4}\right)\) to get 10nt.
A payload length of \(l=12\) encoded using RS(12,18) over \(GF\left({2}^{9}\right)\) for the \(\left(\begin{array}{c}16\\ 3\end{array}\right)\) binomial alphabet.
A 10-sequence block encoded, column-wise, using a (10,15) RS code over \(GF({2}^{9})\) .
The 824 bits are first padded to be \(828=92*9\) . The 92 combinatorial letter message is split into \(7\) sequences of 12 letters and an additional sequence of 8 letters. Finally, a complete block of 12 sequences (total of \(10*12=120\) letters) is created by padding with one additional sequence of 12 letters and including the padding information as the last sequence.
Synthesis and sequencing simulation with errors
Simulating the synthesis process. DNA molecules pertaining to the designed sequences are synthesized using combinatorial k-mer DNA synthesis (See Fig. 1 b). For each combinatorial sequence, we first determine the number of synthesized copies by sampling from \(X\sim N(\mu =1000, {\sigma }^{2}=100)\) . Let \(x\) be the number of copies for a specific sequence. Next, for every position in the sequence, we uniformly sample \(x\) independent k-mers from the set of member k-mers of the combinatorial letter in the specific position. We concatenate the sampled k-mers to the already existing \(x\) synthesized molecules.
Error simulation. Synthesis and sequencing errors are simulated as follows. Error probabilities for deletion, insertion, and substitution are given as parameters denoted as \({P}_{d}, {P}_{I},\) and \({P}_{s}\) respectively. Deletion and insertion errors are assumed to occur during k-mer synthesis and thus implemented on the k-mer level (i.e., an entire k-mer is deleted or inserted in a specific position during the synthesis simulation). Substitution errors are assumed to be sequencing errors and hence implemented on a single base level (i.e., a single letter is substituted, disregarding the position within the k-mer).
Mixing. Post synthesis, molecules undergo mixing to mirror genuine molecular combinations. This is achieved through a randomized data line shuffle using an SQLite database, enabling shuffle processes even for sizable input files 27 .
Reading and sampling. From the simulated synthesized molecule set, a subsample of predefined size \(S*number of synthesized seqeunces\) is drawn, simulating the sampling effect of the sequencing process.
Reconstruction
Barcode decoding The barcode sequence of each read is decoded using the RS(6,8) code.
Grouping by barcode The reads are then grouped by their barcode sequence to allow the reconstruction of the combinatorial sequences.
Filtering of read groups Barcodes (set of reads) with less than 10% of the sampling rate \(S\) reads are discarded.
Combinatorial reconstruction For each set of reads, every position is analyzed separately. The \(K\) most common k-mers are identified and used to determine the combinatorial letter \(\sigma\) in this position. Let \(\Delta\) be the difference between the length of the analyzed reads and the length of the designed sequence. \(\Delta =l-len(read)\) . Reads with \(\left|\Delta \right|>k-1\) are discarded from the analysis. Invalid k-mers (not in \(\Omega )\) are replaced by a dummy k-mer \({X}_{dummy}\) .
Missing barcodes Missing barcodes are replaced with dummy sequences to enable correct outer RS decoding.
Normalized Levenshtein distance Levenshtein distance between the observed sequence \(O\) and the expected sequence \(E\) is calculated 28 , 29 . Normalized Levenshtein distance is calculated by dividing the distance by the length of the expected sequence:
Cost analysis
Synthesis cost estimation was performed using the logical density calculation presented in Supplementary Sect. 8.5 and Supplementary Table S1 . To calculate the sequencing costs, we used the coupon collector model presented in 24 to assess the required sequencing depth given the combinatorial alphabet. Figure 4 b indicates the total number of reads required for reconstructing the sequences, calculated as the required sequencing depth multiplied by the number of sequences from Supplementary Sect. 8.5 and Supplementary Table S1 . The analysis was performed on the following set of combinatorial alphabets: Standard DNA, \(\left(\begin{array}{c}8\\ 4\end{array}\right), \left(\begin{array}{c}16\\ 3\end{array}\right), \left(\begin{array}{c}16\\ 5\end{array}\right), \left(\begin{array}{c}16\\ 7\end{array}\right), \left(\begin{array}{c}32\\ 10\end{array}\right), \left(\begin{array}{c}32\\ 16\end{array}\right), \left(\begin{array}{c}64\\ 32\end{array}\right), \left(\begin{array}{c}96\\ 32\end{array}\right)\) .
Proof of concept experiment
The proof-of-concept experiment was performed by imitating combinatorial synthesis using Gibson assembly of larger DNA fragments. Each DNA fragment was composed of a 20-mer information sequence and an overlap of 20 bp between adjacent fragments, as depicted in Fig. 5 a. Two combinatorial sequences were designed, each composed of a barcode fragment, 4 payload fragments, and Illumina Miseq P5 and P7 anchors at the ends. The information fragments included in each combinatorial position were chosen from a set of 16 sequences with sufficient pair-wise distance. The full list of DNA sequences and the design of combinatorial sequences is listed in Supplementary Sect. 8.6 .
DNA assembly and sequencing
Payload, barcode, and P7 anchor fragments with 20 bp overlaps for the purpose of Gibson assembly were produced by annealing complementary oligonucleotides manufactured by Integrated DNA Technologies (IDT). Oligos were dissolved in Duplex Buffer (100 mM Potassium Acetate; 30 mM HEPES, pH 7.5; available from IDT) to the final concentration of 100 micromolar. For annealing, 25 µl of each oligo in a pair were combined to the final concentration of 50 micromolars. The oligo mixes were incubated for 2 min at 94 0 C, and gradually cooled down to room temperature. The annealed payload oligos that belonged to the same cycle (5 oligos total) were mixed to the final concentration of 1 micromolar per oligo—a total of 5 micromolar, by adding 2 µl of each annealed oligo into the 90 µl of nuclease-free water—a final volume of 100 µl. Annealed barcode and P7 anchor oligos were also diluted to the final concentration of 5 micromolar in nuclease-free water, after thorough mixing by vortexing. The diluted oligos were stored at −20 °C.
Immediately prior to the Gibson assembly, payload oligo mixes, barcode, and P7 anchor oligos were further diluted 100-fold to the final working dilution of 0.05 pmol/microliter in nuclease-free water. Gibson reaction was assembled by adding 1 µl (0.05 pmol) of barcode, 4 × cycle mixes, and P7 anchor to the 4 µl of nuclease-free water and supplemented with 10 µl of NEBuilder HiFi DNA assembly master mix (New England Biolabs (NEB)) to the final volume of 20 µl, according to the manufacturer instructions. The reactions were incubated for 1 h at 50 °C, and purified with AmpPure Beads (Thermo Scientific) at 0.8X ratio (16 µl of beads per 20 µl Gibson reaction) to remove free oligos / incomplete assembly products. After adding beads and thorough mixing, the reactions were incubated for 10 min at room temperature and then placed on a magnet for 5 min at room temperature. After removing the sup, the beads were washed twice with 100 µl of 80% ethanol. The remaining washing solution was further removed by a 20 µl tip, and the beads dried for 3 min on the magnet with an open lid. After removing from the magnet, the beads were resuspended in 22 µl of IDTE buffer (IDT), incubated for 5 min at room temperature, and then placed back on the magnet.
20 µl of eluate were transferred into the separate 1.7 ml tube. 5 µl of the eluted DNA were used as a template for PCR amplification combined with 23 µl of nuclease-free water, 1 µl of 20 micromolar indexing primer 5, 1 µl of 20 micromolar indexing primer 7, and 10 µl of rhAMPseq master mix v8.1—a total of 40 µl. After initial denaturation of 3 min at 95 °C, the PCR reaction proceeded with 50 cycles of 15 s at 95 °C, 30 s at 60 °C, and 30 s at 72 °C, followed by final elongation of 1 min at 72 °C and hold at 4 °C. The PCR reactions were purified with Ampure beads at 0.8X ratio (32 µl beads per 40 µl of PCR reaction) as outlined above, and eluted in 22 µl IDTE buffer. The concentration and the average size of the eluted product were determined by Qubit High Sensitivity DNA kit and Agilent 2200 TapeStation system with D1000 high-sensitivity screen tape respectively. The eluted product was diluted to 4 nM concentration, and used as an input for denatured sequencing library preparation, per manufacturer instructions. The sequencing was performed on Illumina Miseq apparatus (V2 chemistry, 2 × 150 bp reads) using 6 picomolar denatured library supplemented with 40% PhiX sequencing control.
Decoding and analysis
This section outlines the key steps involved in our sequencing analysis pipeline, aimed at effectively processing and interpreting sequenced reads. The analysis pipeline gets the sequencing output file containing raw reads in “.fastq” format and a design file containing the combinatorial sequences.
Analysis steps:
Length filtering. We saved reads that were 220 bp in length, retaining only those corresponding to our designed read length.
Read retrieval. We carefully checked each read for the presence of BCs, universals, and payloads. To keep our data accurate, we discarded reads where the BCs, universals, or payloads had a Hamming distance of more than 3 errors.
Identifying inferred k-mers. For every BC and each cycle, we counted the \(K\) most common k-mers. We then compared these with the design file to quantify those matching (Fig. 5 b) (See Table 3 ).
Information capacities for selected encodings
Table 1 illustrates the logical densities derived from encoding a 1 GB binary message using oligonucleotides with a 12nt barcode and an additional 4nt for standard DNA RS error correction, and a 120 letters payload with 14 extra RS for the payload in combinatorial encoding schemes with parameters \(N\) and \(K\) .
The densities were calculated as follows:
Ethics declaration
No animal or human subjects were involved in the study.
Data availability
The raw data is available in ENA (European Nucleotide Archive). The datasets generated and/or analyzed during the current study are available in the ENA (European Nucleotide Archive) repository, Accession Number—ERR12364864.
Code Availability
Implementation of the algorithms and instructions on how to use them can be found in the GitHub repository in the following links: https://github.com/InbalPreuss/dna_storage_shortmer_simulation , https://github.com/InbalPreuss/dna_storage_experiment .
Church, G., Gao, Y. & Kosuri, S. Next-generation digital information storage in DNA. Science 337 , 1628 (2012).
Article ADS CAS PubMed Google Scholar
Goldman, N. et al. Towards practical, high-capacity, low-maintenance information storage in synthesized DNA. Nature 494 , 77–80 (2013).
Article ADS CAS PubMed PubMed Central Google Scholar
Anavy, L., Vaknin, I., Atar, O., Amit, R. & Yakhini, Z. Data storage in DNA with fewer synthesis cycles using composite DNA letters. Nat. Biotechnol. 37 , 1229–1236 (2019).
Article CAS PubMed Google Scholar
Erlich, Y. & Zielinski, D. DNA Fountain enables a robust and efficient storage architecture. Science 355 , 950–954 (2017).
Gabrys, R., Kiah, H., & Milenkovic, O. Asymmetric lee distance codes for DNA-based storage. In 2015 IEEE International Symposium on Information Theory (ISIT) (2015).
NallappaBhavithran, G., & Selvakumar, R. Indel Error Correction Codes for DNA Digital Data Storage and Retrieval. ArXiv abs/2302.1467 (2023).
Wang, C. et al. Mainstream encoding–decoding methods of DNA data. CCF Trans. High Perform. Comput. 4 , 23–22 (2022).
Article Google Scholar
Boruchvosky, A., Bar-Lev, D., & Yaakobi, E. DNA-Correcting Codes: End-to-end Correction in DNA Storage Systems. ArXiv, abs/2304.0391 (2023).
Bornholt, J. et al. Toward a DNA-based archival storage system. IEEE Micro 37 , 98–104 (2017).
Yazdi, S., Yuan, Y., Ma, J., Zhao, H. & Milenkovic, O. A rewritable, random-access DNA-based storage system. Sci. Rep. 5 , 1–10 (2015).
Google Scholar
Organick, L. et al. Random access in large-scale DNA data storage. Biotechnol. 36 , 242–248 (2018).
CAS Google Scholar
Choi, Y. et al. High information capacity DNA-based data storage with augmented encoding characters using degenerate bases. Sci. Rep. 9 , 6582 (2019).
Article ADS PubMed PubMed Central Google Scholar
Roquet, N., Bhatia, S., Flickinger, S., Mihm, S., Norsworthy, M., Leake, D., & Park, H. DNA-based data storage via combinatorial assembly. 20 April 2021 (online). https://doi.org/10.1101/2021.04.20.440194v1 .
Yan, Y., Pinnamaneni, N., Chalapati, S., Crosbie, C. & Appuswamy, R. Scaling logical density of DNA storage with enzymatically-ligated composite motifs. Sci. Rep. 13 , 15978 (2023).
LeProust, E. et al. Synthesis of high-quality libraries of long (105mer) oligonucleotides by a nover depurination controlled process. Nucl. Acids Res. 38 , 2522–2540 (2019).
Barrett, M. et al. Comparative genomic hybridization using oligonucleotide microarrays and total genomic DNA. Proc. Natl Acad. Sci. USA 101 , 17765–17770 (2004).
Eleuteri, A., Capaldi, D., Douglas, L. & Ravikumar, V. Oligodeoxyribonucleotide phosphorothioates: Substantial reduction of (N-1)-mer content through the use of trimeric phosphoramidite synthons. Nucleosides Nucleotides 3 , 475–483 (1999).
Yagodkin, A. et al. Improved synthesis of trinucleotide phosphoramidites and generation of randomized oligonucleotide libraries. Nucleosides Nucleotides Nucl. Acids 26 (5), 473–497 (2007).
Article CAS Google Scholar
Randolph, J., Yagodkin, A. & Mackie, H. Codon-based Mutagenesis. Nucl. Acids Symp. Ser. 52 , 479 (2008).
Ferrante, M., & Saltalamacchia, M. The Coupon Collector’s Problem , p 35 (2014).
Press, W. et al. HEDGES error-correcting code for DNA storage corrects indels and allows sequence constraints. Proc. Natl. Acad. Sci. 117 (31), 18489–18496 (2020).
Article ADS MathSciNet CAS PubMed PubMed Central Google Scholar
Haoling, Z., et al . SPIDER-WEB generates coding algorithms with superior error tolerance and real-time information retrieval capacity. arXiv preprint arXiv 2204.02855 (2022).
Sabary, O., Orlev, Y., Shafir, R. & Anavy, L. SOLQC: Synthetic oligo library quality control tool. Bioinformatics 2 , 740 (2020).
Preuss, I., Galili, B., Yakhini, Z., & Anavy, Z. Sequencing coverage analysis for combinatorial DNA-based storage systems . biorxiv (2024).
Ayoub, R. Euler and the zeta function. Am. Math. Mon. 81 , 1067–1086 (1974).
Article MathSciNet Google Scholar
Reed, I. & Solomon, G. Polynomial codes over certain finite fields. J. Soc. Ind. Appl. Math. 8 , 300–304 (1960).
Hipp, R. D. SQLite (2020) (Online). https://www.sqlite.org/index.html .
Levenshtein, V. Binary codes capable of correcting spurious insertions and deletions of ones. Problems Inf. Transm. 1 , 8–17 (1965).
Levenshtein, V. Binary codes capable of correcting deletion, insertions and reversals. Soviet Physics Doklady 10 (8), 707–710 (1966).
ADS MathSciNet Google Scholar
Download references
Acknowledgements
This project has received funding from the European Union’s Horizon Europe research and innovation programme under grant agreement No. 101115134 (DiDAX project). The authors of this paper thank the Yakhini research group for the fruitful discussions. The authors also thank Eland Nagar for his support and problem-solving approach regarding the experimental proof of concept.
European Union's Horizon Europe Research and Innovation Programme, 101115134.
Author information
Authors and affiliations.
School of Computer Science, Reichman University, 4610101, Herzliya, Israel
Inbal Preuss, Zohar Yakhini & Leon Anavy
Institute of Nanotechnology and Advanced Materials, The Mina and Everard Goodman Faculty of Life Sciences, Bar-Ilan University, 5290002, Ramat Gan, Israel
Michael Rosenberg
Faculty of Computer Science, Technion, 3200003, Haifa, Israel
You can also search for this author in PubMed Google Scholar
Contributions
I.P., Z.Y. and L.A conceived the idea, designed the experiments, interpreted and analyzed the data. I.P performed all computational work, including the simulations and the data analysis. M.R. was solely responsible for conducting the experimental work. All authors wrote the manuscript.
Corresponding author
Correspondence to Inbal Preuss .
Ethics declarations
Competing interests.
Zohar Yakhini, Leon Anavy have competing interests as defined by Nature Research. Z. Yakhini and L. Anavy are named as inventors on a patent related to the content of this paper: L. Anavy, Z. Yakhini, and R. Amit, "Molecular data storage systems and methods". United States of America Patent US20210141568A1, 2021.
Additional information
Publisher's note.
Springer Nature remains neutral with regard to jurisdictional claims in published maps and institutional affiliations.
Supplementary Information
Supplementary information 1., supplementary information 2..
Supplementary Video 1.
Supplementary Information 3.
Supplementary information 4., rights and permissions.
Open Access This article is licensed under a Creative Commons Attribution 4.0 International License, which permits use, sharing, adaptation, distribution and reproduction in any medium or format, as long as you give appropriate credit to the original author(s) and the source, provide a link to the Creative Commons licence, and indicate if changes were made. The images or other third party material in this article are included in the article's Creative Commons licence, unless indicated otherwise in a credit line to the material. If material is not included in the article's Creative Commons licence and your intended use is not permitted by statutory regulation or exceeds the permitted use, you will need to obtain permission directly from the copyright holder. To view a copy of this licence, visit http://creativecommons.org/licenses/by/4.0/ .
Reprints and permissions
About this article
Cite this article.
Preuss, I., Rosenberg, M., Yakhini, Z. et al. Efficient DNA-based data storage using shortmer combinatorial encoding. Sci Rep 14 , 7731 (2024). https://doi.org/10.1038/s41598-024-58386-z
Download citation
Received : 11 December 2023
Accepted : 28 March 2024
Published : 02 April 2024
DOI : https://doi.org/10.1038/s41598-024-58386-z
Share this article
Anyone you share the following link with will be able to read this content:
Sorry, a shareable link is not currently available for this article.
Provided by the Springer Nature SharedIt content-sharing initiative
By submitting a comment you agree to abide by our Terms and Community Guidelines . If you find something abusive or that does not comply with our terms or guidelines please flag it as inappropriate.
Quick links
- Explore articles by subject
- Guide to authors
- Editorial policies
Sign up for the Nature Briefing newsletter — what matters in science, free to your inbox daily.

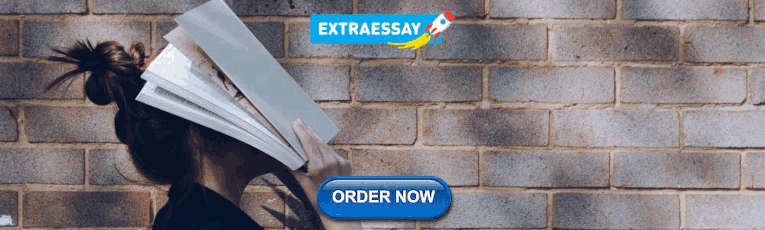
IMAGES
VIDEO
COMMENTS
Learn how to select a sample that is representative of the population and allows you to draw valid conclusions from your research. Find out the difference between probability and non-probability sampling methods, and see examples of each type with advantages and disadvantages.
This is often used to ensure that the sample is representative of the population as a whole. Cluster Sampling: In this method, the population is divided into clusters or groups, and then a random sample of clusters is selected. Then, all members of the selected clusters are included in the sample. Multi-Stage Sampling: This method combines two ...
Learn how to select a sample that is representative of the population and allows you to draw valid conclusions from your research. Find out the difference between probability and non-probability sampling methods, and the advantages and disadvantages of each. See examples of each type of sampling method with a table of contents.
Sampling methods in psychology refer to strategies used to select a subset of individuals (a sample) from a larger population, to study and draw inferences about the entire population. Common methods include random sampling, stratified sampling, cluster sampling, and convenience sampling. Proper sampling ensures representative, generalizable, and valid research results.
Simple random sampling. Simple random sampling involves selecting participants in a completely random fashion, where each participant has an equal chance of being selected.Basically, this sampling method is the equivalent of pulling names out of a hat, except that you can do it digitally.For example, if you had a list of 500 people, you could use a random number generator to draw a list of 50 ...
We could choose a sampling method based on whether we want to account for sampling bias; a random sampling method is often preferred over a non-random method for this reason. Random sampling examples include: simple, systematic, stratified, and cluster sampling. Non-random sampling methods are liable to bias, and common examples include ...
Understand sampling methods in research, from simple random sampling to stratified, systematic, and cluster sampling. Learn how these sampling techniques boost data accuracy and representation, ensuring robust, reliable results. Check this article to learn about the different sampling method techniques, types and examples.
A sample is the subset of the population that you actually measure, test, or evaluate and base your results. Sampling methods are how you obtain your sample. Before beginning your study, carefully define the population because your results apply to the target population. You can define your population as narrowly as necessary to meet the needs ...
Sampling types. There are two major categories of sampling methods ( figure 1 ): 1; probability sampling methods where all subjects in the target population have equal chances to be selected in the sample [ 1, 2] and 2; non-probability sampling methods where the sample population is selected in a non-systematic process that does not guarantee ...
7. Critical case sampling: This is another purposive sampling method. In critical case sampling, subjects are selected based on researchers' inferences that they might represent a broader trend. Sometimes critical case sampling leads to the discovery of many more subjects who share the same traits with the respondents.
Researchers do need to be mindful of carefully considering the strengths and limitations of each method before selecting a sampling technique. Non-probability sampling is best for exploratory research, such as at the beginning of a research project. There are five main types of non-probability sampling methods: Convenience sampling. Purposive ...
Cluster sampling- she puts 50 into random groups of 5 so we get 10 groups then randomly selects 5 of them and interviews everyone in those groups --> 25 people are asked. 2. Stratified sampling- she puts 50 into categories: high achieving smart kids, decently achieving kids, mediumly achieving kids, lower poorer achieving kids and clueless ...
Sampling in market action research is of two types - probability sampling and non-probability sampling. Let's take a closer look at these two methods of sampling. Probability sampling:Probability sampling is a sampling technique where a researcher selects a few criteria and chooses members of a population randomly.
Abstract. Knowledge of sampling methods is essential to design quality research. Critical questions are provided to help researchers choose a sampling method. This article reviews probability and non-probability sampling methods, lists and defines specific sampling techniques, and provides pros and cons for consideration.
Sampling is the statistical process of selecting a subset—called a 'sample'—of a population of interest for the purpose of making observations and statistical inferences about that population. Social science research is generally about inferring patterns of behaviours within specific populations. We cannot study entire populations because of feasibility and cost constraints, and hence ...
The method by which the researcher selects the sample is the ' Sampling Method'. There are essentially two types of sampling methods: 1) probability sampling - based on chance events (such as random numbers, flipping a coin etc.); and 2) non-probability sampling - based on researcher's choice, population that accessible & available.
in research including Probability sampling techniques, which include simple random sampling, systematic random sampling and strati ed. random sampling and Non-probability sampling, which include ...
collect data from all cases. Thus, there is a need to select a sample. The entire set of cases from. which researcher sample is drawn in called the population. Since, researchers neither have time ...
The main goal of any marketing or statistical research is to provide quality results that are a reliable basis for decision-making. That is why the different types of sampling methods and techniques have a crucial role in research methodology and statistics. Your sample is one of the key factors that determine if your findings are accurate.
Non-probability sampling method is a technique in which the researcher chooses samples based on subjective judgment, preferably random selection. These are also known as Random sampling methods. These are also called non-random sampling methods. These are used for research which is conclusive. These are used for research which is exploratory.
Qualitative Research Methodology. This is a research methodology that involves the collection and analysis of non-numerical data such as words, images, and observations. This type of research is often used to explore complex phenomena, to gain an in-depth understanding of a particular topic, and to generate hypotheses.
The research note was prepared based on experience in qualitative research sampling gained, ... Qualitative evaluation and research methods (2nd ed.), Sage, Newbury Park, California (1990) Google Scholar. Robinson, 2014. ... International Journal of Social Research Methodology, 21 (5) (2018), pp. 619-634. CrossRef View in Scopus Google Scholar.
1 Fixed effects model, which is often used in Econometrics (see, e.g., Wooldridge, Citation 2019) and which incorporates dummy variables representing clusters into the model and excludes level-2 variables, is frequently used as a viable strategy for dealing with small sample sizes at level-2 when the focus lies solely on the fixed effects at level-1 (e.g., McNeish & Kelley, Citation 2019).
Causal inference is an important method to learn the causal associations which are invariant across different environments, thus improving the generalisation ability of the model. However, existing methods usually require partitioning of the environment to learn invariant features, which mostly have imbalance problems due to the lack of ...
Sociocognitive research in management has long explored how actors can accrue assets in the form of audiences' positive evaluations and how these evaluations can be translated into tangible benefits (Fombrun & Shanley, 1990; Paruchuri, Pollock, & Kumar, 2019; Pfarrer et al., 2019; Podolny, 2005).This research has also examined how actors who possess high levels of these assets can affect ...
Background: Globally, antimicrobial resistance (AMR) poses a critical threat, requiring robust surveillance methodologies to tackle the growing challenge of drug-resistant microbes. AMR is a huge challenge in India due to high disease burden, lack of etiology-based diagnostic tests and over the counter availability of antibiotics and inadequate treatment of wastewaters are important drivers of ...
Age estimation is crucial for investigating animal populations in the past and present. Visual examination of tooth wear and eruption is one of the most common ageing methods in zooarchaeology, wildlife management, palaeontology, and veterinary research. Such approaches are particularly advantageous because they are non-destructive, can be completed using photographs, and do not require ...
The method described herein leverages the advantages of combinatorial encoding schemes while relying on existing DNA chemical synthesis methods with some modifications.