
- Table of Contents
- Random Entry
- Chronological
- Editorial Information
- About the SEP
- Editorial Board
- How to Cite the SEP
- Special Characters
- Advanced Tools
- Support the SEP
- PDFs for SEP Friends
- Make a Donation
- SEPIA for Libraries
- Entry Contents
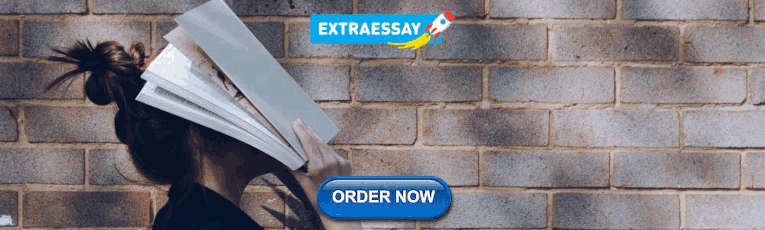
Bibliography
Academic tools.
- Friends PDF Preview
- Author and Citation Info
- Back to Top
The Uncertainty Principle
Quantum mechanics is generally regarded as the physical theory that is our best candidate for a fundamental and universal description of the physical world. The conceptual framework employed by this theory differs drastically from that of classical physics. Indeed, the transition from classical to quantum physics marks a genuine revolution in our understanding of the physical world.
One striking aspect of the difference between classical and quantum physics is that whereas classical mechanics presupposes that exact simultaneous values can be assigned to all physical quantities, quantum mechanics denies this possibility, the prime example being the position and momentum of a particle. According to quantum mechanics, the more precisely the position (momentum) of a particle is given, the less precisely can one say what its momentum (position) is. This is (a simplistic and preliminary formulation of) the quantum mechanical uncertainty principle for position and momentum. The uncertainty principle played an important role in many discussions on the philosophical implications of quantum mechanics, in particular in discussions on the consistency of the so-called Copenhagen interpretation, the interpretation endorsed by the founding fathers Heisenberg and Bohr.
This should not suggest that the uncertainty principle is the only aspect of the conceptual difference between classical and quantum physics: the implications of quantum mechanics for notions as (non)-locality, entanglement and identity play no less havoc with classical intuitions.
1. Introduction
2.1 heisenberg’s road to the uncertainty relations, 2.2 heisenberg’s argument, 2.3 the interpretation of heisenberg’s uncertainty relations, 2.4 uncertainty relations or uncertainty principle, 2.5 mathematical elaboration, 3.1 from wave-particle duality to complementarity, 3.2 bohr’s view on the uncertainty relations, 4. the minimal interpretation, 5.1 landau-pollak uncertainty relations, 5.2 entropic uncertainty relations, 6.1 the recent debate on error-disturbance relations, other internet resources, related entries.
The uncertainty principle is certainly one of the most famous aspects of quantum mechanics. It has often been regarded as the most distinctive feature in which quantum mechanics differs from classical theories of the physical world. Roughly speaking, the uncertainty principle (for position and momentum) states that one cannot assign exact simultaneous values to the position and momentum of a physical system. Rather, these quantities can only be determined with some characteristic “uncertainties” that cannot become arbitrarily small simultaneously. But what is the exact meaning of this principle, and indeed, is it really a principle of quantum mechanics? (In his original work, Heisenberg only speaks of uncertainty relations.) And, in particular, what does it mean to say that a quantity is determined only up to some uncertainty? These are the main questions we will explore in the following, focusing on the views of Heisenberg and Bohr.
The notion of “uncertainty” occurs in several different meanings in the physical literature. It may refer to a lack of knowledge of a quantity by an observer, or to the experimental inaccuracy with which a quantity is measured, or to some ambiguity in the definition of a quantity, or to a statistical spread in an ensemble of similarly prepared systems. Also, several different names are used for such uncertainties: inaccuracy, spread, imprecision, indefiniteness, indeterminateness, indeterminacy, latitude, etc. As we shall see, even Heisenberg and Bohr did not decide on a single terminology for quantum mechanical uncertainties. Forestalling a discussion about which name is the most appropriate one in quantum mechanics, we use the name “uncertainty principle” simply because it is the most common one in the literature.
2. Heisenberg
Heisenberg introduced his famous relations in an article of 1927, entitled Ueber den anschaulichen Inhalt der quantentheoretischen Kinematik und Mechanik . A (partial) translation of this title is: “On the anschaulich content of quantum theoretical kinematics and mechanics”. Here, the term anschaulich is particularly notable. Apparently, it is one of those German words that defy an unambiguous translation into other languages. Heisenberg’s title is translated as “ On the physical content …” by Wheeler and Zurek (1983). His collected works (Heisenberg 1984) translate it as “ On the perceptible content …”, while Cassidy’s biography of Heisenberg (Cassidy 1992), refers to the paper as “ On the perceptual content …”. Literally, the closest translation of the term anschaulich is “visualizable”. But, as in most languages, words that make reference to vision are not always intended literally. Seeing is widely used as a metaphor for understanding, especially for immediate understanding. Hence, anschaulich also means “intelligible” or “intuitive”. [ 1 ]
Why was this issue of the Anschaulichkeit of quantum mechanics such a prominent concern to Heisenberg? This question has already been considered by a number of commentators (Jammer 1974; Miller 1982; de Regt 1997; Beller 1999). For the answer, it turns out, we must go back a little in time. In 1925 Heisenberg had developed the first coherent mathematical formalism for quantum theory (Heisenberg 1925). His leading idea was that only those quantities that are in principle observable should play a role in the theory, and that all attempts to form a picture of what goes on inside the atom should be avoided. In atomic physics the observational data were obtained from spectroscopy and associated with atomic transitions. Thus, Heisenberg was led to consider the “transition quantities” as the basic ingredients of the theory. Max Born, later that year, realized that the transition quantities obeyed the rules of matrix calculus, a branch of mathematics that was not so well-known then as it is now. In a famous series of papers Heisenberg, Born and Jordan developed this idea into the matrix mechanics version of quantum theory.
Formally, matrix mechanics remains close to classical mechanics. The central idea is that all physical quantities must be represented by infinite self-adjoint matrices (later identified with operators on a Hilbert space). It is postulated that the matrices \(\bQ\) and \(\bP\) representing the canonical position and momentum variables of a particle satisfy the so-called canonical commutation rule
where \(\hslash = h/2\pi\), \(h\) denotes Planck’s constant, and boldface type is used to represent matrices (or operators). The new theory scored spectacular empirical success by encompassing nearly all spectroscopic data known at the time, especially after the concept of the electron spin was included in the theoretical framework.
It came as a big surprise, therefore, when one year later, Erwin Schrödinger presented an alternative theory, that became known as wave mechanics. Schrödinger assumed that an electron in an atom could be represented as an oscillating charge cloud, evolving continuously in space and time according to a wave equation. The discrete frequencies in the atomic spectra were not due to discontinuous transitions (quantum jumps) as in matrix mechanics, but to a resonance phenomenon. Schrödinger also showed that the two theories were equivalent. [ 2 ]
Even so, the two approaches differed greatly in interpretation and spirit. Whereas Heisenberg eschewed the use of visualizable pictures, and accepted discontinuous transitions as a primitive notion, Schrödinger claimed as an advantage of his theory that it was anschaulich . In Schrödinger’s vocabulary, this meant that the theory represented the observational data by means of continuously evolving causal processes in space and time. He considered this condition of Anschaulichkeit to be an essential requirement on any acceptable physical theory. Schrödinger was not alone in appreciating this aspect of his theory. Many other leading physicists were attracted to wave mechanics for the same reason. For a while, in 1926, before it emerged that wave mechanics had serious problems of its own, Schrödinger’s approach seemed to gather more support in the physics community than matrix mechanics.
Understandably, Heisenberg was unhappy about this development. In a letter of 8 June 1926 to Pauli he confessed that “The more I think about the physical part of Schrödinger’s theory, the more disgusting I find it”, and: “What Schrödinger writes about the Anschaulichkeit of his theory, … I consider Mist ” (Pauli 1979: 328). Again, this last German term is translated differently by various commentators: as “junk” (Miller 1982) “rubbish” (Beller 1999) “crap” (Cassidy 1992), “poppycock” (Bacciagaluppi & Valentini 2009) and perhaps more literally, as “bullshit” (Moore 1989; de Regt 1997). Nevertheless, in published writings, Heisenberg voiced a more balanced opinion. In a paper in Die Naturwissenschaften (1926) he summarized the peculiar situation that the simultaneous development of two competing theories had brought about. Although he argued that Schrödinger’s interpretation was untenable, he admitted that matrix mechanics did not provide the Anschaulichkeit which made wave mechanics so attractive. He concluded:
to obtain a contradiction-free anschaulich interpretation, we still lack some essential feature in our image of the structure of matter.
The purpose of his 1927 paper was to provide exactly this lacking feature.
Let us now look at the argument that led Heisenberg to his uncertainty relations. He started by redefining the notion of Anschaulichkeit . Whereas Schrödinger associated this term with the provision of a causal space-time picture of the phenomena, Heisenberg, by contrast, declared:
We believe we have gained anschaulich understanding of a physical theory, if in all simple cases, we can grasp the experimental consequences qualitatively and see that the theory does not lead to any contradictions. Heisenberg 1927: 172)
His goal was, of course, to show that, in this new sense of the word, matrix mechanics could lay the same claim to Anschaulichkeit as wave mechanics.
To do this, he adopted an operational assumption: terms like “the position of a particle” have meaning only if one specifies a suitable experiment by which “the position of a particle” can be measured. We will call this assumption the “measurement=meaning principle”. In general, there is no lack of such experiments, even in the domain of atomic physics. However, experiments are never completely accurate. We should be prepared to accept, therefore, that in general the meaning of these quantities is also determined only up to some characteristic inaccuracy.
As an example, he considered the measurement of the position of an electron by a microscope. The accuracy of such a measurement is limited by the wave length of the light illuminating the electron. Thus, it is possible, in principle, to make such a position measurement as accurate as one wishes, by using light of a very short wave length, e.g., \(\gamma\)-rays. But for \(\gamma\)-rays, the Compton effect cannot be ignored: the interaction of the electron and the illuminating light should then be considered as a collision of at least one photon with the electron. In such a collision, the electron suffers a recoil which disturbs its momentum. Moreover, the shorter the wave length, the larger is this change in momentum. Thus, at the moment when the position of the particle is accurately known, Heisenberg argued, its momentum cannot be accurately known:
At the instant of time when the position is determined, that is, at the instant when the photon is scattered by the electron, the electron undergoes a discontinuous change in momentum. This change is the greater the smaller the wavelength of the light employed, i.e., the more exact the determination of the position. At the instant at which the position of the electron is known, its momentum therefore can be known only up to magnitudes which correspond to that discontinuous change; thus, the more precisely the position is determined, the less precisely the momentum is known, and conversely. (Heisenberg 1927: 174–5)
This is the first formulation of the uncertainty principle. In its present form it is an epistemological principle, since it limits what we can know about the electron. From “elementary formulae of the Compton effect” Heisenberg estimated the “imprecisions” to be of the order
He continued: “In this circumstance we see the direct anschaulich content of the relation \(\boldsymbol{QP} - \boldsymbol{PQ} = i\hslash\).”
He went on to consider other experiments, designed to measure other physical quantities and obtained analogous relations for time and energy:
and action \(J\) and angle \(w\)
which he saw as corresponding to the “well-known” relations
However, these generalisations are not as straightforward as Heisenberg suggested. In particular, the status of the time variable in his several illustrations of relation (3) is not at all clear (Hilgevoord 2005; see also Section 2.5 ).
Heisenberg summarized his findings in a general conclusion: all concepts used in classical mechanics are also well-defined in the realm of atomic processes. But, as a pure fact of experience ( rein erfahrungsgemäß ), experiments that serve to provide such a definition for one quantity are subject to particular indeterminacies, obeying relations (2) – (4) which prohibit them from providing a simultaneous definition of two canonically conjugate quantities. Note that in this formulation the emphasis has slightly shifted: he now speaks of a limit on the definition of concepts, i.e., not merely on what we can know , but what we can meaningfully say about a particle. Of course, this stronger formulation follows by application of the above measurement=meaning principle: if there are, as Heisenberg claims, no experiments that allow a simultaneous precise measurement of two conjugate quantities, then these quantities are also not simultaneously well-defined.
Heisenberg’s paper has an interesting “Addition in proof” mentioning critical remarks by Bohr, who saw the paper only after it had been sent to the publisher. Among other things, Bohr pointed out that in the microscope experiment it is not the change of the momentum of the electron that is important, but rather the circumstance that this change cannot be precisely determined in the same experiment. An improved version of the argument, responding to this objection, is given in Heisenberg’s Chicago lectures of 1930.
Here (Heisenberg 1930: 16), it is assumed that the electron is illuminated by light of wavelength \(\lambda\) and that the scattered light enters a microscope with aperture angle \(\varepsilon\). According to the laws of classical optics, the accuracy of the microscope depends on both the wave length and the aperture angle; Abbe’s criterium for its “resolving power”, i.e., the size of the smallest discernable details, gives
On the other hand, the direction of a scattered photon, when it enters the microscope, is unknown within the angle \(\varepsilon\), rendering the momentum change of the electron uncertain by an amount
leading again to the result (2) .
Let us now analyse Heisenberg’s argument in more detail. Note that, even in this improved version, Heisenberg’s argument is incomplete. According to Heisenberg’s “measurement=meaning principle”, one must also specify, in the given context, what the meaning is of the phrase “momentum of the electron”, in order to make sense of the claim that this momentum is changed by the position measurement. A solution to this problem can again be found in the Chicago lectures (Heisenberg 1930: 15). Here, he assumes that initially the momentum of the electron is precisely known, e.g., it has been measured in a previous experiment with an inaccuracy \(\delta p_{i}\), which may be arbitrarily small. Then, its position is measured with inaccuracy \(\delta q\), and after this, its final momentum is measured with an inaccuracy \(\delta p_{f}\). All three measurements can be performed with arbitrary precision. Thus, the three quantities \(\delta p_{i}, \delta q\), and \(\delta p_{f}\) can be made as small as one wishes. If we assume further that the initial momentum has not changed until the position measurement, we can speak of a definite momentum until the time of the position measurement. Moreover we can give operational meaning to the idea that the momentum is changed during the position measurement: the outcome of the second momentum measurement (say \(p_{f}\) will generally differ from the initial value \(p_{i}\). In fact, one can also show that this change is discontinuous, by varying the time between the three measurements.
Let us try to see, adopting this more elaborate set-up, if we can complete Heisenberg’s argument. We have now been able to give empirical meaning to the “change of momentum” of the electron, \(p_{f} - p_{i}\). Heisenberg’s argument claims that the order of magnitude of this change is at least inversely proportional to the inaccuracy of the position measurement:
However, can we now draw the conclusion that the momentum is only imprecisely defined? Certainly not. Before the position measurement, its value was \(p_{i}\), after the measurement it is \(p_{f}\). One might, perhaps, claim that the value at the very instant of the position measurement is not yet defined, but we could simply settle this by a convention, e.g., we might assign the mean value \((p_{i} + p_{f})/2\) to the momentum at this instant. But then, the momentum is precisely determined at all instants, and Heisenberg’s formulation of the uncertainty principle no longer follows. The above attempt of completing Heisenberg’s argument thus overshoots its mark.
A solution to this problem can again be found in the Chicago Lectures. Heisenberg admits that position and momentum can be known exactly. He writes:
If the velocity of the electron is at first known, and the position then exactly measured, the position of the electron for times previous to the position measurement may be calculated. For these past times, \(\delta p\delta q\) is smaller than the usual bound. (Heisenberg 1930: 15)
Indeed, Heisenberg says: “the uncertainty relation does not hold for the past”.
Apparently, when Heisenberg refers to the uncertainty or imprecision of a quantity, he means that the value of this quantity cannot be given beforehand . In the sequence of measurements we have considered above, the uncertainty in the momentum after the measurement of position has occurred, refers to the idea that the value of the momentum is not fixed just before the final momentum measurement takes place. Once this measurement is performed, and reveals a value \(p_{f}\), the uncertainty relation no longer holds; these values then belong to the past. Clearly, then, Heisenberg is concerned with unpredictability : the point is not that the momentum of a particle changes, due to a position measurement, but rather that it changes by an unpredictable amount. It is, however always possible to measure, and hence define, the size of this change in a subsequent measurement of the final momentum with arbitrary precision.
Although Heisenberg admits that we can consistently attribute values of momentum and position to an electron in the past, he sees little merit in such talk. He points out that these values can never be used as initial conditions in a prediction about the future behavior of the electron, or subjected to experimental verification. Whether or not we grant them physical reality is, as he puts it, a matter of personal taste. Heisenberg’s own taste is, of course, to deny their physical reality. For example, he writes,
I believe that one can formulate the emergence of the classical “path” of a particle succinctly as follows: the “path” comes into being only because we observe it . (Heisenberg 1927: 185)
Apparently, in his view, a measurement does not only serve to give meaning to a quantity, it creates a particular value for this quantity. This may be called the “measurement=creation” principle. It is an ontological principle, for it states what is physically real.
This then leads to the following picture. First we measure the momentum of the electron very accurately. By “measurement= meaning”, this entails that the term “the momentum of the particle” is now well-defined. Moreover, by the “measurement=creation” principle, we may say that this momentum is physically real. Next, the position is measured with inaccuracy \(\delta q\). At this instant, the position of the particle becomes well-defined and, again, one can regard this as a physically real attribute of the particle. However, the momentum has now changed by an amount that is unpredictable by an order of magnitude \(\abs{p_{f} - p_{i}} \sim h/\delta q\). The meaning and validity of this claim can be verified by a subsequent momentum measurement.
The question is then what status we shall assign to the momentum of the electron just before its final measurement. Is it real? According to Heisenberg it is not. Before the final measurement, the best we can attribute to the electron is some unsharp, or fuzzy momentum. These terms are meant here in an ontological sense, characterizing a real attribute of the electron.
Heisenberg’s relations were soon considered to be a cornerstone of the Copenhagen interpretation of quantum mechanics. Just a few months later, Kennard (1927) already called them the “essential core” of the new theory. Taken together with Heisenberg’s contention that they provide the intuitive content of the theory and their prominent role in later discussions on the Copenhagen interpretation, a dominant view emerged in which the uncertainty relations were regarded as a fundamental principle of the theory.
The interpretation of these relations has often been debated. Do Heisenberg’s relations express restrictions on the experiments we can perform on quantum systems, and, therefore, restrictions on the information we can gather about such systems; or do they express restrictions on the meaning of the concepts we use to describe quantum systems? Or else, are they restrictions of an ontological nature, i.e., do they assert that a quantum system simply does not possess a definite value for its position and momentum at the same time? The difference between these interpretations is partly reflected in the various names by which the relations are known, e.g., as “inaccuracy relations”, or: “uncertainty”, “indeterminacy” or “unsharpness relations”. The debate between these views has been addressed by many authors, but it has never been settled completely. Let it suffice here to make only two general observations.
First, it is clear that in Heisenberg’s own view all the above questions stand or fall together. Indeed, we have seen that he adopted an operational “measurement=meaning” principle according to which the meaningfulness of a physical quantity was equivalent to the existence of an experiment purporting to measure that quantity. Similarly, his “measurement=creation” principle allowed him to attribute physical reality to such quantities. Hence, Heisenberg’s discussions moved rather freely and quickly from talk about experimental inaccuracies to epistemological or ontological issues and back again.
However, ontological questions seemed to be of somewhat less interest to him. For example, there is a passage (Heisenberg 1927: 197), where he discusses the idea that, behind our observational data, there might still exist a hidden reality in which quantum systems have definite values for position and momentum, unaffected by the uncertainty relations. He emphatically dismisses this conception as an unfruitful and meaningless speculation, because, as he says, the aim of physics is only to describe observable data. Similarly, in the Chicago Lectures, he warns against the fact that the human language permits the utterance of statements which have no empirical content, but nevertheless produce a picture in our imagination. He notes,
One should be especially careful in using the words “reality”, “actually”, etc., since these words very often lead to statements of the type just mentioned. (Heisenberg 1930: 11)
So, Heisenberg also endorsed an interpretation of his relations as rejecting a reality in which particles have simultaneous definite values for position and momentum.
The second observation is that although for Heisenberg experimental, informational, epistemological and ontological formulations of his relations were, so to say, just different sides of the same coin, this is not so for those who do not share his operational principles or his view on the task of physics. Alternative points of view, in which e.g., the ontological reading of the uncertainty relations is denied, are therefore still viable. The statement, often found in the literature of the thirties, that Heisenberg had proved the impossibility of associating a definite position and momentum to a particle is certainly wrong. But the precise meaning one can coherently attach to Heisenberg’s relations depends rather heavily on the interpretation one favors for quantum mechanics as a whole. And because no agreement has been reached on this latter issue, one cannot expect agreement on the meaning of the uncertainty relations either.
Let us now move to another question about Heisenberg’s relations: do they express a principle of quantum theory? Probably the first influential author to call these relations a “principle” was Eddington, who, in his Gifford Lectures of 1928 referred to them as the “Principle of Indeterminacy”. In the English literature the name uncertainty principle became most common. It is used both by Condon and Robertson in 1929, and also in the English version of Heisenberg’s Chicago Lectures (Heisenberg 1930), although, remarkably, nowhere in the original German version of the same book (see also Cassidy 1998). Indeed, Heisenberg never seems to have endorsed the name “principle” for his relations. His favourite terminology was “inaccuracy relations” ( Ungenauigkeitsrelationen ) or “indeterminacy relations” ( Unbestimmtheitsrelationen ). We know only one passage, in Heisenberg’s own Gifford lectures, delivered in 1955–56 (Heisenberg 1958: 43), where he mentioned that his relations “are usually called relations of uncertainty or principle of indeterminacy”. But this can well be read as his yielding to common practice rather than his own preference.
But does the relation (2) qualify as a principle of quantum mechanics? Several authors, foremost Karl Popper (1967), have contested this view. Popper argued that the uncertainty relations cannot be granted the status of a principle on the grounds that they are derivable from the theory, whereas one cannot obtain the theory from the uncertainty relations. (The argument being that one can never derive any equation, say, the Schrödinger equation, or the commutation relation (1) , from an inequality.)
Popper’s argument is, of course, correct but we think it misses the point. There are many statements in physical theories which are called principles even though they are in fact derivable from other statements in the theory in question. A more appropriate departing point for this issue is not the question of logical priority but rather Einstein’s distinction between “constructive theories” and “principle theories”.
Einstein proposed this famous classification in Einstein 1919. Constructive theories are theories which postulate the existence of simple entities behind the phenomena. They endeavour to reconstruct the phenomena by framing hypotheses about these entities. Principle theories, on the other hand, start from empirical principles, i.e., general statements of empirical regularities, employing no or only a bare minimum of theoretical terms. The purpose is to build up the theory from such principles. That is, one aims to show how these empirical principles provide sufficient conditions for the introduction of further theoretical concepts and structure.
The prime example of a theory of principle is thermodynamics. Here the role of the empirical principles is played by the statements of the impossibility of various kinds of perpetual motion machines. These are regarded as expressions of brute empirical fact, providing the appropriate conditions for the introduction of the concepts of energy and entropy and their properties. (There is a lot to be said about the tenability of this view, but that is not our topic here.)
Now obviously, once the formal thermodynamic theory is built, one can also derive the impossibility of the various kinds of perpetual motion. (They would violate the laws of energy conservation and entropy increase.) But this derivation should not misguide one into thinking that they were no principles of the theory after all. The point is just that empirical principles are statements that do not rely on the theoretical concepts (in this case entropy and energy) for their meaning. They are interpretable independently of these concepts and, further, their validity on the empirical level still provides the physical content of the theory.
A similar example is provided by special relativity, another theory of principle, which Einstein deliberately designed after the ideal of thermodynamics. Here, the empirical principles are the light postulate and the relativity principle. Again, once we have built up the modern theoretical formalism of the theory (Minkowski space-time), it is straightforward to prove the validity of these principles. But again this does not count as an argument for claiming that they were no principles after all. So the question whether the term “principle” is justified for Heisenberg’s relations, should, in our view, be understood as the question whether they are conceived of as empirical principles.
One can easily show that this idea was never far from Heisenberg’s intentions. We have already seen that Heisenberg presented the relations as the result of a “pure fact of experience”. A few months after his 1927 paper, he wrote a popular paper “ Über die Grundprincipien der Quantenmechanik ” (“On the fundamental principles of quantum mechanics”) where he made the point even more clearly. Here Heisenberg described his recent break-through in the interpretation of the theory as follows: “It seems to be a general law of nature that we cannot determine position and velocity simultaneously with arbitrary accuracy”. Now actually, and in spite of its title, the paper does not identify or discuss any “fundamental principle” of quantum mechanics. So, it must have seemed obvious to his readers that he intended to claim that the uncertainty relation was a fundamental principle, forced upon us as an empirical law of nature, rather than a result derived from the formalism of the theory.
This reading of Heisenberg’s intentions is corroborated by the fact that, even in his 1927 paper, applications of his relation frequently present the conclusion as a matter of principle. For example, he says “In a stationary state of an atom its phase is in principle indeterminate” (Heisenberg 1927: 177, [emphasis added]). Similarly, in a paper of 1928, he described the content of his relations as:
It has turned out that it is in principle impossible to know, to measure the position and velocity of a piece of matter with arbitrary accuracy. (Heisenberg 1984: 26, [emphasis added])
So, although Heisenberg did not originate the tradition of calling his relations a principle, it is not implausible to attribute the view to him that the uncertainty relations represent an empirical principle that could serve as a foundation of quantum mechanics. In fact, his 1927 paper expressed this desire explicitly:
Surely, one would like to be able to deduce the quantitative laws of quantum mechanics directly from their anschaulich foundations, that is, essentially, relation [ (2) ]. ( ibid : 196)
This is not to say that Heisenberg was successful in reaching this goal, or that he did not express other opinions on other occasions.
Let us conclude this section with three remarks. First, if the uncertainty relation is to serve as an empirical principle, one might well ask what its direct empirical support is. In Heisenberg’s analysis, no such support is mentioned. His arguments concerned thought experiments in which the validity of the theory, at least at a rudimentary level, is implicitly taken for granted. Jammer (1974: 82) conducted a literature search for high precision experiments that could seriously test the uncertainty relations and concluded they were still scarce in 1974. Real experimental support for the uncertainty relations in experiments in which the inaccuracies are close to the quantum limit have come about only more recently (see Kaiser, Werner, and George 1983; Uffink 1985; Nairz, Andt, and Zeilinger 2002).
A second point is the question whether the theoretical structure or the quantitative laws of quantum theory can indeed be derived on the basis of the uncertainty principle, as Heisenberg wished. Serious attempts to build up quantum theory as a full-fledged Theory of Principle on the basis of the uncertainty principle have never been carried out. Indeed, the most Heisenberg could and did claim in this respect was that the uncertainty relations created “room” (Heisenberg 1927: 180) or “freedom” (Heisenberg 1931: 43) for the introduction of some non-classical mode of description of experimental data, not that they uniquely lead to the formalism of quantum mechanics. A serious proposal to approach quantum mechanics as a theory of principle was provided more recently by Bub (2000) and Chiribella & Spekkens (2016). But, remarkably, this proposal does not use the uncertainty relation as one of its fundamental principles. Third, it is remarkable that in his later years Heisenberg put a somewhat different gloss on his relations. In his autobiography Der Teil und das Ganze of 1969 he described how he had found his relations inspired by a remark by Einstein that “it is the theory which decides what one can observe”—thus giving precedence to theory above experience, rather than the other way around. Some years later he even admitted that his famous discussions of thought experiments were actually trivial since
… if the process of observation itself is subject to the laws of quantum theory, it must be possible to represent its result in the mathematical scheme of this theory. (Heisenberg 1975: 6)
When Heisenberg introduced his relation, his argument was based only on qualitative examples. He did not provide a general, exact derivation of his relations. [ 3 ] Indeed, he did not even give a definition of the uncertainties \(\delta q\), etc., occurring in these relations. Of course, this was consistent with the announced goal of that paper, i.e., to provide some qualitative understanding of quantum mechanics for simple experiments.
The first mathematically exact formulation of the uncertainty relations is due to Kennard. He proved in 1927 the theorem that for all normalized state vectors \(\ket{\psi}\) the following inequality holds:
Here, \(\Delta_{\psi}\bP\) and \(\Delta_{\psi}\bQ\) are standard deviations of position and momentum in the state vector \(\ket{\psi}\), i.e.,
where \(\expval{\cdot}_{\psi} = \expvalexp{\cdot}{\psi}\) denotes the expectation value in state \(\ket{\psi}\). Equivalently we can use the wave function \(\psi(q)\) and its Fourier transform:
The inequality (9) was generalized by Robertson (1929) who proved that for all observables (self-adjoint operators) \(\bA\) and \(\bB\):
where \([\bA,\bB] := \bA\bB - \bB\bA\) denotes the commutator.
Since the above inequalities (9) and (12) have the virtue of being exact, in contrast to Heisenberg’s original semi-quantitative formulation, it is tempting to regard them as the exact counterpart of Heisenberg’s relations (2) – (4) . Indeed, such was Heisenberg’s own view. In his Chicago Lectures (Heisenberg 1930: 15–19), he presented Kennard’s derivation of relation (9) and claimed that “this proof does not differ at all in mathematical content” from his semi-quantitative argument, the only difference being that now “the proof is carried through exactly”.
But it may be useful to point out that both in status and intended role there is a difference between Kennard’s inequality and Heisenberg’s previous formulation (2) . The inequalities discussed here are not statements of empirical fact, but theorems of the quantum mechanical formalism. As such, they presuppose the validity of this formalism, and in particular the commutation relation (1) , rather than elucidating its intuitive content or to create “room” or “freedom” for the validity of this formalism. At best, one should see the above inequalities as showing that the formalism is consistent with Heisenberg’s empirical principle.
This situation is similar to that arising in other theories of principle where, as noted in Section 2.4 , one often finds that, next to an empirical principle, the formalism also provides a corresponding theorem. And similarly, this situation should not, by itself, cast doubt on the question whether Heisenberg’s relation can be regarded as a principle of quantum mechanics.
There is a second notable difference between (2) and (9) . Heisenberg did not give a general definition for the “uncertainties” \(\delta p\) and \(\delta q\). The most definite remark he made about them was that they could be taken as “something like the mean error”. In the discussions of thought experiments, he and Bohr would always quantify uncertainties on a case-to-case basis by choosing some parameters which happened to be relevant to the experiment at hand. By contrast, the inequalities (9) and (12) employ a single specific expression as a measure for “uncertainty”: the standard deviation. At the time, this choice was not unnatural, given that this expression is well-known and widely used in error theory and the description of statistical fluctuations. However, there was very little or no discussion of whether this choice was appropriate for a general formulation of the uncertainty relations. A standard deviation reflects the spread or expected fluctuations in a series of measurements of an observable in a given state. It is not at all easy to connect this idea with the concept of the “inaccuracy” of a measurement, such as the resolving power of a microscope. In fact, even though Heisenberg had taken Kennard’s inequality as the precise formulation of the uncertainty relation, he and Bohr never relied on standard deviations in their many discussions of thought experiments, and indeed, it has been shown (Uffink and Hilgevoord 1985; Hilgevoord and Uffink 1988) that these discussions cannot be framed in terms of standard deviations.
Another problem with the above elaboration is that the “well-known” relations (5) are actually false if energy \(\boldsymbol{E}\) and action \(\boldsymbol{J}\) are to be positive operators (Jordan 1927). In that case, self-adjoint operators \(\boldsymbol{t}\) and \(\boldsymbol{w}\) do not exist and inequalities analogous to (9) cannot be derived. Also, these inequalities do not hold for angle and angular momentum (Uffink 1990). These obstacles have led to a quite extensive literature on time-energy and angle-action uncertainty relations (Busch 1990; Hilgevoord 1996, 1998, 2005; Muga et al. 2002; Hilgevoord and Atkinson 2011; Pashby 2015).
In spite of the fact that Heisenberg’s and Bohr’s views on quantum mechanics are often lumped together as (part of) “the Copenhagen interpretation”, there is considerable difference between their views on the uncertainty relations.
Long before the development of modern quantum mechanics, Bohr had been particularly concerned with the problem of particle-wave duality, i.e., the problem that experimental evidence on the behaviour of both light and matter seemed to demand a wave picture in some cases, and a particle picture in others. Yet these pictures are mutually exclusive. Whereas a particle is always localized, the very definition of the notions of wavelength and frequency requires an extension in space and in time. Moreover, the classical particle picture is incompatible with the characteristic phenomenon of interference.
His long struggle with wave-particle duality had prepared him for a radical step when the dispute between matrix and wave mechanics broke out in 1926–27. For the main contestants, Heisenberg and Schrödinger, the issue at stake was which view could claim to provide a single coherent and universal framework for the description of the observational data. The choice was, essentially between a description in terms of continuously evolving waves, or else one of particles undergoing discontinuous quantum jumps. By contrast, Bohr insisted that elements from both views were equally valid and equally needed for an exhaustive description of the data. His way out of the contradiction was to renounce the idea that the pictures refer, in a literal one-to-one correspondence, to physical reality. Instead, the applicability of these pictures was to become dependent on the experimental context. This is the gist of the viewpoint he called “complementarity”.
Bohr first conceived the general outline of his complementarity argument in early 1927, during a skiing holiday in Norway, at the same time when Heisenberg wrote his uncertainty paper. When he returned to Copenhagen and found Heisenberg’s manuscript, they got into an intense discussion. On the one hand, Bohr was quite enthusiastic about Heisenberg’s ideas which seemed to fit wonderfully with his own thinking. Indeed, in his subsequent work, Bohr always presented the uncertainty relations as the symbolic expression of his complementarity viewpoint. On the other hand, he criticized Heisenberg severely for his suggestion that these relations were due to discontinuous changes occurring during a measurement process. Rather, Bohr argued, their proper derivation should start from the indispensability of both particle and wave concepts. He pointed out that the uncertainties in the experiment did not exclusively arise from the discontinuities but also from the fact that in the experiment we need to take into account both the particle theory and the wave theory. It is not so much the unknown disturbance which renders the momentum of the electron uncertain but rather the fact that the position and the momentum of the electron cannot be simultaneously defined in this experiment (see the “Addition in Proof” to Heisenberg’s paper).
We shall not go too deeply into the matter of Bohr’s interpretation of quantum mechanics since we are mostly interested in Bohr’s view on the uncertainty principle. For a more detailed discussion of the former we refer to Scheibe (1973), Folse (1985), Honner (1987) and Murdoch (1987). It may be useful, however, to sketch some of the main points. Central in Bohr’s considerations is the language we use in physics. No matter how abstract and subtle the concepts of modern physics may be, they are essentially an extension of our ordinary language and a means to communicate the results of our experiments. These results, obtained under well-defined experimental circumstances, are what Bohr calls the “phenomena”. A phenomenon is “the comprehension of the effects observed under given experimental conditions” (Bohr 1939: 24), it is the resultant of a physical object, a measuring apparatus and the interaction between them in a concrete experimental situation. The essential difference between classical and quantum physics is that in quantum physics the interaction between the object and the apparatus cannot be made arbitrarily small; the interaction must at least comprise one quantum. This is expressed by Bohr’s quantum postulate:
[… the] essence [of the formulation of the quantum theory] may be expressed in the so-called quantum postulate, which attributes to any atomic process an essential discontinuity or rather individuality, completely foreign to classical theories and symbolized by Planck’s quantum of action. (Bohr 1928: 580)
A phenomenon, therefore, is an indivisible whole and the result of a measurement cannot be considered as an autonomous manifestation of the object itself independently of the measurement context. The quantum postulate forces upon us a new way of describing physical phenomena:
In this situation, we are faced with the necessity of a radical revision of the foundation for the description and explanation of physical phenomena. Here, it must above all be recognized that, however far quantum effects transcend the scope of classical physical analysis, the account of the experimental arrangement and the record of the observations must always be expressed in common language supplemented with the terminology of classical physics. (Bohr 1948: 313)
This is what Scheibe (1973) has called the “buffer postulate” because it prevents the quantum from penetrating into the classical description: A phenomenon must always be described in classical terms; Planck’s constant does not occur in this description.
Together, the two postulates induce the following reasoning. In every phenomenon the interaction between the object and the apparatus comprises at least one quantum. But the description of the phenomenon must use classical notions in which the quantum of action does not occur. Hence, the interaction cannot be analysed in this description. On the other hand, the classical character of the description allows us to speak in terms of the object itself. Instead of saying: “the interaction between a particle and a photographic plate has resulted in a black spot in a certain place on the plate”, we are allowed to forgo mentioning the apparatus and say: “the particle has been found in this place”. The experimental context, rather than changing or disturbing pre-existing properties of the object, defines what can meaningfully be said about the object.
Because the interaction between object and apparatus is left out in our description of the phenomenon, we do not get the whole picture. Yet, any attempt to extend our description by performing the measurement of a different observable quantity of the object, or indeed, on the measurement apparatus, produces a new phenomenon and we are again confronted with the same situation. Because of the unanalyzable interaction in both measurements, the two descriptions cannot, generally, be united into a single picture. They are what Bohr calls complementary descriptions:
[the quantum of action]…forces us to adopt a new mode of description designated as complementary in the sense that any given application of classical concepts precludes the simultaneous use of other classical concepts which in a different connection are equally necessary for the elucidation of the phenomena. (Bohr 1929: 10)
The most important example of complementary descriptions is provided by the measurements of the position and momentum of an object. If one wants to measure the position of the object relative to a given spatial frame of reference, the measuring instrument must be rigidly fixed to the bodies which define the frame of reference. But this implies the impossibility of investigating the exchange of momentum between the object and the instrument and we are cut off from obtaining any information about the momentum of the object. If, on the other hand, one wants to measure the momentum of an object the measuring instrument must be able to move relative to the spatial reference frame. Bohr here assumes that a momentum measurement involves the registration of the recoil of some movable part of the instrument and the use of the law of momentum conservation. The looseness of the part of the instrument with which the object interacts entails that the instrument cannot serve to accurately determine the position of the object. Since a measuring instrument cannot be rigidly fixed to the spatial reference frame and, at the same time, be movable relative to it, the experiments which serve to precisely determine the position and the momentum of an object are mutually exclusive. Of course, in itself, this is not at all typical for quantum mechanics. But, because the interaction between object and instrument during the measurement can neither be neglected nor determined the two measurements cannot be combined. This means that in the description of the object one must choose between the assignment of a precise position or of a precise momentum.
Similar considerations hold with respect to the measurement of time and energy. Just as the spatial coordinate system must be fixed by means of solid bodies so must the time coordinate be fixed by means of unperturbed, synchronised clocks. But it is precisely this requirement which prevents one from taking into account of the exchange of energy with the instrument if this is to serve its purpose. Conversely, any conclusion about the object based on the conservation of energy prevents following its development in time.
The conclusion is that in quantum mechanics we are confronted with a complementarity between two descriptions which are united in the classical mode of description: the space-time description (or coordination) of a process and the description based on the applicability of the dynamical conservation laws. The quantum forces us to give up the classical mode of description (also called the “causal” mode of description by Bohr [ 4 ] : it is impossible to form a classical picture of what is going on when radiation interacts with matter as, e.g., in the Compton effect.
Any arrangement suited to study the exchange of energy and momentum between the electron and the photon must involve a latitude in the space-time description sufficient for the definition of wave-number and frequency which enter in the relation [\(E = h\nu\) and \(p = h\sigma\)]. Conversely, any attempt of locating the collision between the photon and the electron more accurately would, on account of the unavoidable interaction with the fixed scales and clocks defining the space-time reference frame, exclude all closer account as regards the balance of momentum and energy. (Bohr 1949: 210)
A causal description of the process cannot be attained; we have to content ourselves with complementary descriptions. “The viewpoint of complementarity may be regarded”, according to Bohr, “as a rational generalization of the very ideal of causality”.
In addition to complementary descriptions Bohr also talks about complementary phenomena and complementary quantities. Position and momentum, as well as time and energy, are complementary quantities. [ 5 ]
We have seen that Bohr’s approach to quantum theory puts heavy emphasis on the language used to communicate experimental observations, which, in his opinion, must always remain classical. By comparison, he seemed to put little value on arguments starting from the mathematical formalism of quantum theory. This informal approach is typical of all of Bohr’s discussions on the meaning of quantum mechanics. One might say that for Bohr the conceptual clarification of the situation has primary importance while the formalism is only a symbolic representation of this situation.
This is remarkable since, finally, it is the formalism which needs to be interpreted. This neglect of the formalism is one of the reasons why it is so difficult to get a clear understanding of Bohr’s interpretation of quantum mechanics and why it has aroused so much controversy. We close this section by citing from an article of 1948 to show how Bohr conceived the role of the formalism of quantum mechanics:
The entire formalism is to be considered as a tool for deriving predictions, of definite or statistical character, as regards information obtainable under experimental conditions described in classical terms and specified by means of parameters entering into the algebraic or differential equations of which the matrices or the wave-functions, respectively, are solutions. These symbols themselves, as is indicated already by the use of imaginary numbers, are not susceptible to pictorial interpretation; and even derived real functions like densities and currents are only to be regarded as expressing the probabilities for the occurrence of individual events observable under well-defined experimental conditions. (Bohr 1948: 314)
In his Como lecture, published in 1928, Bohr gave his own version of a derivation of the uncertainty relations between position and momentum and between time and energy. He started from the relations
which connect the notions of energy \(E\) and momentum \(p\) from the particle picture with those of frequency \(\nu\) and wavelength \(\lambda\) from the wave picture. He noticed that a wave packet of limited extension in space and time can only be built up by the superposition of a number of elementary waves with a large range of wave numbers and frequencies. Denoting the spatial and temporal extensions of the wave packet by \(\Delta x\) and \(\Delta t\), and the extensions in the wave number \(\sigma := 1/\lambda\) and frequency by \(\Delta \sigma\) and \(\Delta \nu\), it follows from Fourier analysis that in the most favorable case \(\Delta x \Delta \sigma \approx \Delta t \Delta \nu \approx 1\), and, using (13), one obtains the relations
Note that \(\Delta x, \Delta \sigma\), etc., are not standard deviations but unspecified measures of the size of a wave packet. (The original text has equality signs instead of approximate equality signs, but, since Bohr does not define the spreads exactly the use of approximate equality signs seems more in line with his intentions. Moreover, Bohr himself used approximate equality signs in later presentations.) These equations determine, according to Bohr:
the highest possible accuracy in the definition of the energy and momentum of the individuals associated with the wave field. (Bohr 1928: 571).
This circumstance may be regarded as a simple symbolic expression of the complementary nature of the space-time description and the claims of causality. ( ibid ). [ 6 ]
We note a few points about Bohr’s view on the uncertainty relations. First of all, Bohr does not refer to discontinuous changes in the relevant quantities during the measurement process. Rather, he emphasizes the possibility of defining these quantities. This view is markedly different from Heisenberg’s view. A draft version of the Como lecture is even more explicit on the difference between Bohr and Heisenberg:
These reciprocal uncertainty relations were given in a recent paper of Heisenberg as the expression of the statistical element which, due to the feature of discontinuity implied in the quantum postulate, characterizes any interpretation of observations by means of classical concepts. It must be remembered, however, that the uncertainty in question is not simply a consequence of a discontinuous change of energy and momentum say during an interaction between radiation and material particles employed in measuring the space-time coordinates of the individuals. According to the above considerations the question is rather that of the impossibility of defining rigorously such a change when the space-time coordination of the individuals is also considered. (Bohr 1985: 93)
Indeed, Bohr not only rejected Heisenberg’s argument that these relations are due to discontinuous disturbances implied by the act of measuring, but also his view that the measurement process creates a definite result:
The unaccustomed features of the situation with which we are confronted in quantum theory necessitate the greatest caution as regard all questions of terminology. Speaking, as it is often done of disturbing a phenomenon by observation, or even of creating physical attributes to objects by measuring processes is liable to be confusing, since all such sentences imply a departure from conventions of basic language which even though it can be practical for the sake of brevity, can never be unambiguous. (Bohr 1939: 24)
Nor did he approve of an epistemological formulation or one in terms of experimental inaccuracies:
[…] a sentence like “we cannot know both the momentum and the position of an atomic object” raises at once questions as to the physical reality of two such attributes of the object, which can be answered only by referring to the mutual exclusive conditions for an unambiguous use of space-time concepts, on the one hand, and dynamical conservation laws on the other hand. (Bohr 1948: 315; also Bohr 1949: 211) It would in particular not be out of place in this connection to warn against a misunderstanding likely to arise when one tries to express the content of Heisenberg’s well-known indeterminacy relation by such a statement as “the position and momentum of a particle cannot simultaneously be measured with arbitrary accuracy”. According to such a formulation it would appear as though we had to do with some arbitrary renunciation of the measurement of either the one or the other of two well-defined attributes of the object, which would not preclude the possibility of a future theory taking both attributes into account on the lines of the classical physics. (Bohr 1937: 292)
Instead, Bohr always stressed that the uncertainty relations are first and foremost an expression of complementarity. This may seem odd since complementarity is a dichotomic relation between two types of description whereas the uncertainty relations allow for intermediate situations between two extremes. They “express” the dichotomy in the sense that if we take the energy and momentum to be perfectly well-defined, symbolically \(\Delta E = \Delta p\) = 0, the position and time variables are completely undefined, \(\Delta x = \Delta t = \infty\), and vice versa. But they also allow intermediate situations in which the mentioned uncertainties are all non-zero and finite. This more positive aspect of the uncertainty relation is mentioned in the Como lecture:
At the same time, however, the general character of this relation makes it possible to a certain extent to reconcile the conservation laws with the space-time coordination of observations, the idea of a coincidence of well-defined events in space-time points being replaced by that of unsharply defined individuals within space-time regions. (Bohr 1928: 571)
However, Bohr never followed up on this suggestion that we might be able to strike a compromise between the two mutually exclusive modes of description in terms of unsharply defined quantities. Indeed, an attempt to do so, would take the formalism of quantum theory more seriously than the concepts of classical language, and this step Bohr refused to take. Instead, in his later writings he would be content with stating that the uncertainty relations simply defy an unambiguous interpretation in classical terms:
These so-called indeterminacy relations explicitly bear out the limitation of causal analysis, but it is important to recognize that no unambiguous interpretation of such a relation can be given in words suited to describe a situation in which physical attributes are objectified in a classical way. (Bohr 1948: 315)
Finally, on a more formal level, we note that Bohr’s derivation does not rely on the commutation relations (1) and (5) , but on Fourier analysis. These two approaches are equivalent as far as the relationship between position and momentum is concerned, but this is not so for time and energy since most physical systems do not have a time operator. Indeed, in his discussion with Einstein (Bohr 1949), Bohr considered time as a simple classical variable. This even holds for his famous discussion of the “clock-in-the-box” thought-experiment where the time, as defined by the clock in the box, is treated from the point of view of classical general relativity. Thus, in an approach based on commutation relations, the position-momentum and time-energy uncertainty relations are not on equal footing, which is contrary to Bohr’s approach in terms of Fourier analysis. For more details see (Hilgevoord 1996 and 1998).
In the previous two sections we have seen how both Heisenberg and Bohr attributed a far-reaching status to the uncertainty relations. They both argued that these relations place fundamental limits on the applicability of the usual classical concepts. Moreover, they both believed that these limitations were inevitable and forced upon us. However, we have also seen that they reached such conclusions by starting from radical and controversial assumptions. This entails, of course, that their radical conclusions remain unconvincing for those who reject these assumptions. Indeed, the operationalist-positivist viewpoint adopted by these authors has long since lost its appeal among philosophers of physics.
So the question may be asked what alternative views of the uncertainty relations are still viable. Of course, this problem is intimately connected with that of the interpretation of the wave function, and hence of quantum mechanics as a whole. Since there is no consensus about the latter, one cannot expect consensus about the interpretation of the uncertainty relations either. Here we only describe a point of view, which we call the “minimal interpretation”, that seems to be shared by both the adherents of the Copenhagen interpretation and of other views.
In quantum mechanics a system is supposed to be described by its wave function, also called its quantum state or state vector. Given the state vector \(\ket{\psi}\), one can derive probability distributions for all the physical quantities pertaining to the system, usually called its observables, such as its position, momentum, angular momentum, energy, etc. The operational meaning of these probability distributions is that they correspond to the distribution of the values obtained for these quantities in a long series of repetitions of the measurement. More precisely, one imagines a great number of copies of the system under consideration, all prepared in the same way. On each copy the momentum, say, is measured. Generally, the outcomes of these measurements differ and a distribution of outcomes is obtained. The theoretical momentum distribution derived from the quantum state is supposed to coincide with the hypothetical distribution of outcomes obtained in an infinite series of repetitions of the momentum measurement. The same holds, mutatis mutandis , for all the other physical quantities pertaining to the system. Note that no simultaneous measurements of two or more quantities are required in defining the operational meaning of the probability distributions.
The uncertainty relations discussed above can be considered as statements about the spreads of the probability distributions of the several physical quantities arising from the same state. For example, the uncertainty relation between the position and momentum of a system may be understood as the statement that the position and momentum distributions cannot both be arbitrarily narrow—in some sense of the word “narrow”—in any quantum state. Inequality (9) is an example of such a relation in which the standard deviation is employed as a measure of spread. From this characterization of uncertainty relations follows that a more detailed interpretation of the quantum state than the one given in the previous paragraph is not required to study uncertainty relations as such. In particular, a further ontological or linguistic interpretation of the notion of uncertainty, as limits on the applicability of our concepts given by Heisenberg or Bohr, need not be supposed.
Of course, this minimal interpretation leaves the question open whether it makes sense to attribute precise values of position and momentum to an individual system. Some interpretations of quantum mechanics, e.g., those of Heisenberg and Bohr, deny this; while others, e.g., the interpretation of de Broglie and Bohm insist that each individual system has a definite position and momentum (see the entry on Bohmian mechanics ). The only requirement is that, as an empirical fact, it is not possible to prepare pure ensembles in which all systems have the same values for these quantities, or ensembles in which the spreads are smaller than allowed by quantum theory. Although interpretations of quantum mechanics, in which each system has a definite value for its position and momentum are still viable, this is not to say that they are without strange features of their own; they do not imply a return to classical physics.
We end with a few remarks on this minimal interpretation. First, it may be noted that the minimal interpretation of the uncertainty relations is little more than filling in the empirical meaning of inequality (9) . As such, this view shares many of the limitations we have noted above about this inequality. Indeed, it is not straightforward to relate the spread in a statistical distribution of measurement results with the inaccuracy of this measurement, such as, e.g., the resolving power of a microscope, or of a disturbance of the system by the measurement. Moreover, the minimal interpretation does not address the question whether one can make simultaneous accurate measurements of position and momentum.
As a matter of fact, one can show that the standard formalism of quantum mechanics does not allow such simultaneous measurements. But this is not a consequence of relation (9) . Rather, it follows from the fact that this formalism simply does not contain any observable that would accomplish such a task. The extension of this formalism that allows observables to be represented by positive-operator-valued measures or POVM’s, does allow the formal introduction of observables describing joint measurements (see also section 6.1 ). But even here, for the case of position and momentum, one finds that such measurements have to be “unsharp”, which entails that they cannot be regarded as simultaneous accurate measurements.
If one feels that statements about inaccuracy of measurement, or the possibility of simultaneous measurements, belong to any satisfactory formulation of the uncertainty principle, one will need to look for other formulations of the uncertainty principle. Some candidates for such formulations will be discussed in Section 6 . First, however, we will look at formulations of the uncertainty principle that stay firmly within the minimal interpretation, and differ from (9) only by using measures of uncertainty other than the standard deviation.
5. Alternative measures of uncertainty
While the standard deviation is the most well-known quantitative measure for uncertainty or the spread in the probability distribution, it is not the only one, and indeed it has distinctive drawbacks that other such measures may lack. For example, in the definition of the standard deviations (11) one can see that that the probability density function \(\abs{\psi(q)}^2\) is weighed by a quadratic factor \(q^2\) that puts increasing emphasis on its tails. Therefore, the value of \(\Delta_\psi \bQ\) will depend predominantly at how this density behaves at the tails: if these falls off very quickly, e.g., like a Gaussian, it will be small, but if the tails drop off only slowly the standard deviation may be very large, even when most probability is concentrated in a small interval.
The upshot of this objection is that having a lower bound on the product of the standard deviations of position and momentum, as the Heisenberg-Kennard uncertainty relation (9) gives, does not by itself rule out a state where both the probability densities for position and momentum are extremely concentrated, in the sense of having more than \((1- \epsilon)\) of their probability concentrated in a a region of size smaller than \(\delta\), for any choice of \(\epsilon, \delta >0\). This means, in our view, that relation (9) actually fails to express what most physicists would take to be the very core idea of the uncertainty principle.
One way to deal with this objection is to consider alternative measures to quantify the spread or uncertainty associated with a probability density. Here we discuss two such proposals.
The most straightforward alternative is to pick some value \(\alpha\) close to one, say \(\alpha = 0.9\), and ask for the width of the smallest interval that supports the fraction \(\alpha\) of the total probability distribution in position and similarly for momentum:
In a previous work (Uffink and Hilgevoord 1985) we called such measures bulk widths , because they indicate how concentrated the ”bulk” (i.e., fraction \(\alpha\) or \(\beta\)) of the probability distribution is. Landau and Pollak (1961) obtained an uncertainty relation in terms of these bulk widths.
This Landau-Pollak inequality shows that if the choices of \(\alpha, \beta\) are not too low, there is a state-independent lower bound on the product of the bulk widths of the position and momentum distribution for any quantum state.
Note that bulk widths are not so sensitive to the behavior of the tails of the distributions and, therefore, the Landau-Pollak inequality is immune to the objection above.Thus, this inequality expresses constraints on quantum mechanical states not contained in relation (9) . Further, by the well-known Bienaymé-Chebyshev inequality, one has
so that inequality (16) implies (by choosing \(\alpha,\beta\) optimal) that \( \Delta_\psi \bQ \Delta_\psi \bP \geq 0.12 \hbar \). This, obviously, is not the best lower bound for the product of standard deviations, but the important point is here that the Landau-Pollak inequality (16) in terms of bulk widths implies the existence of a lower bound on the product of standard deviations, while conversely, the Heisenberg-Kennard equality (9) does not imply any bound on the product of bulk widths. A generalization of this approach to non-commuting observables in a finite-dimensional Hilbert space is discussed in Uffink 1990.
Another approach to express the uncertainty principle is to use entropic measures of uncertainty. The foremost example of these is the Shannon entropy , which for the position and momentum distribution of a given state vector \(\ket{\psi}\) may be defined as:
One can then show (see Beckner 1975; Białinicki-Birula and Micielski 1975) that
A nice feature of this entropic uncertainty relation is that it provides a strict improvement of the Heisenberg-Kennard relation. That is to say, one can show (independently of quantum theory) that for any probability density function \(p(x)\)
Applying this to the inequality (19) we get:
showing that the entropic uncertainty relation implies the Heisenberg-Kennard uncertainty relation. A drawback of this relation is that it does not completely evade the objection mentioned above, (i.e., these entropic measures of uncertainty can become as large as one pleases while \(1-\epsilon\) of the probability in the distribution is concentrated on a very small interval), but the examples needed to show this are admittedly more far-fetched.
For non-commuting observables in a \(n\)-dimensional Hilbert space, one can similarly define an entropic uncertainty in the probability distribution \(\abs{\braket{a_i}{\psi}}^2\) for a given state \(\ket{\psi}\) and a complete set of eigenstates \(\ket{a_i}\), \( (i= 1, \ldots n)\), of the observable \(\bA\):
and \(H(\bB,\psi)\) similarly in terms of the probability distribution \(\abs{\braket{b_j}{\psi}}^2\) for a complete set of eigenstates \(\ket{b_j}\), (\(j =1, \ldots, n\)) of observable \(\bB\). Then we obtain the uncertainty relation (Maassen and Uffink 1988):
which was further generalized and improved by (Frank and Lieb 2012). The most important advantage of these relations is that, in contrast to Robertson’s inequality (12) , the lower bound is a positive constant, independent of the state.
6. Uncertainty relations for inaccuracy and disturbance
Both the standard deviation and the alternative measures of uncertainty considered in the previous subsection (and many more that we have not mentioned!) are designed to indicate the width or spread of a single given probability distribution. Applied to quantum mechanics, where the probability distributions for position and momentum are obtained from a given quantum state vector, one can use them to formulate uncertainty relations that characterize the spread in those distribution for any given state. The resulting inequalities then express limitations on what state-preparations quantum mechanics allows. They are thus expressions of what may be called a preparation uncertainty principle :
In quantum mechanics, it is impossible to prepare any system in a state \(\ket{\psi}\) such that its position and momentum are both precisely predictable, in the sense of having both the expected spread in a measurement of position and the expected spread in a momentum measurement arbitrarily small.
The relations ( 9 , 16 , 19 ) all belong to this category; the mere difference being that they employ different measures of spread: viz. the standard deviation, the bulk width or the Shannon entropy.
Note that in this formulation, there is no reference to simultaneous or joint measurements, nor to any notion of accuracy like the resolving power of the measurement instrument, nor to the issue of how much the system in the state that is being measured is disturbed by this measurement. This section is devoted to attempts that go beyond the mold of this preparation uncertain principle.
We have seen that in 1927 Heisenberg argued that the measurement of (say) position must necessarily disturb the conjugate variable (i.e., momentum) by an amount that is inversely proportional to the inaccuracy of measurement of the former. We have also seen that this idea was not maintained in the Kennard’s uncertainty relation (9) , a relation that was embraced by Heisenberg (1930) and most textbooks.
A rather natural question thus arises whether there are further inequalities in quantum mechanics that would address Heisenberg’s original thinking more directly, i.e., that do deal with how much one variable is disturbed by the accurate measurement of another. That is, we will look at attempts that would establish a claim which may be called a measurement uncertainty principle .
In quantum mechanics, there is no measurement procedure by which one can accurately measure the position of a system without disturbing it momentum, in the sense that some measure of inaccuracy in position and some measure of the disturbance of momentum of the system by the measurement cannot both be arbitrarily small.
This formulation of the uncertainty principle has always remained controversial. Uncertainty relations that would express this alleged principle are often called “error-disturbance” relations or “noise-disturbance” relations We will look at two recent proposals to search for such relations: Ozawa (2003) and Busch, Lahti, and Werner (2013).
In Ozawa’s approach, we assume that a system \(\cal S\) of interest in state \(\ket{\psi}\) is coupled to a measurement device \(\cal M\) in state \(\ket{\chi}\), and their interaction is governed by a unitary operator \(U\). On the Hilbert space of the joint system the observable \(\bQ\) of the system \(\cal S\) we are interested in is represented by
The measurement interaction will allow us to perform an (inaccurate) measurement of this quantity by reading off a pointer observable \(\boldsymbol{Q'}\) of the measurement device after the interaction. Hence this inaccurate observable may be represented as
The measure of noise in the measurement of \(\bQ\) is then chosen as:
A comparison of the initial momentum \(\bP_{\rm in} = \bP \otimes \mathbb{1}\) and the final momentum after the measurement \(\bP_{\rm out} = U^\dagger (\bP \otimes \mathbb{1})U\) is made by choosing a measure of the disturbance of \(\bP\) by the measurement procedure:
Ozawa obtained an inequality involving those two measures, which, however, is more involved than previous uncertainty relations. For our purposes, however, the important point is that Ozawa showed that the product \(\epsilon_\psi (\bQ) \eta_\psi (\bP)\) has no positive lower bound. His conclusion from this was that Heisenberg’s noise-disturbance relation is violated.
Yet, whether Ozawa’s result indeed succeeds in formulating Heisenberg’s qualitative discussion of disturbance and accuracy in the microscope example has come under dispute. See Busch, Lahti and Werner (2013, and 2014 (Other Internet Resources)), and Ozawa (2013, Other Internet Resources).
An objection raised in this dispute is that a quantity like \(\expval{(\bQ'_{\rm out} - \bQ_{\rm in})^2}^{1/2}\) tells us very little about how good the observable \({\bQ'}_{\rm out}\) can stand in as an inaccurate measurement of \(\bQ_{\rm in}\). The main point to observe here is that these operators generally do not commute, and that measurements of \(\bQ'_{\rm out}\), of \(\bQ_{\rm in}\) and of their difference will require altogether three different measurement contexts. To require that \(\epsilon_\psi(\bQ)\) vanishes, for example, means only that the state prepared belongs to the linear subspace corresponding to the zero eigenvalue of the operator \(\bQ'_{\rm out} - {\bQ}_{\rm in}\), and therefore that \(\expval{\bQ'_{\rm out}}_\psi = \expval{\bQ_{\rm in}}_\psi\), but this does not preclude that the probability distribution of \(\bQ_{\rm out}\) in state \(\psi\) might be wildly different from that of \(\bQ_{\rm in}\). But then no one would think of \(\bQ_{\rm out}\) as an accurate measurement of \(\bQ_{\rm in}\) so that the definition of \(\epsilon_\psi(\bQ)\) does not express what it is supposed to express. A similar objection can also be raised against \(\eta_\psi (\bP)\).
Another observation is that Ozawa’s conclusion that there is no lower bound for his error-disturbance product for is not at all surprising. That is, even without probing the system by a measurement apparatus, one can show that such a lower bound does not exist. If the initial state of a system is prepared at time \(t=0\) as a Gaussian quasi-monochromatic wave packet with \(\expval{\bQ_0}_\psi =0\) and evolves freely, we can use a time-of-flight measurement to learn about its later position. Ehrenfest’s theorem tells us: \(\expval{\bQ_t}_\psi = \frac{t}{m} \expval{\bP}_\psi\).
Hence, as an approximative measurement of the position \(\bQ_t\), one could propose the observable \(\bQ'_t = \frac{t}{m}\bP\). It is known that under the stated conditions (and with \(m\) and \(t\) large) this approximation holds very well, i.e., we do not only have \(\expval{\bQ'_t -\bQ_t}_\psi =0\), but also \(\expval{(\bQ'_t -\bQ_t)^2} \approx 0\), as nearly as we please. But since \(\bQ'_t\) is just the momentum multiplied by a constant, its measurement will obviously not disturb the momentum of the system. In other words, for this example, one has \(\epsilon_\psi (\bQ)\) as small as we please with zero disturbance of the momentum. Therefore, any hopes that there could be a positive lower bound for the product \(\epsilon_\psi (\bQ) \eta_\psi (\bP)\) seem to be dashed, even with the simplest of measurement schemes, i.e. a free evolution.
Ozawa’s results do not show that Heisenberg’s analysis of the microscope argument was wrong. Rather, they throw doubt on the appropriateness of the definitions he used to formalize Heisenberg’s informal argument.
An entirely different analysis of the problem of substantiating a measurement uncertainty relation was offered by Busch, Lahti, and Werner (2013). These authors consider a measurement device \(\cal M\) that makes a joint unsharp measurement of both position and momentum. To describe such joint unsharp measurements, they employ the extended modern formalism that characterizes obervables not by self-adjoint operators but by positive-operator-valued measures (POVM’s). In the present case, this means that the measurement procedure is characterized by a collection of positive operators, \(M(p,q)\), where the pair \(p,q\) represent the outcome variables of the measurement, with
The two marginals of this POVM,
are also POVM’s in their own right and represent the unsharp position \(Q'\) and unsharp momentum \(P'\) observables respectively. (Note that these do not refer to a self-adjoint operator!)
For a system prepared in a state \(\ket{\psi}\), the joint probability density of obtaining outcomes \((p,q)\) in the joint unsharp measurement (28) is then
while the marginals of this joint probability distribution give the distributions for \(Q'\) and \(P'\).
Since a joint sharp measurement of position and momentum is impossible in quantum mechanics, these marginal distributions ( 31 ) obtained from \(M\) will differ from that of ideal measurements of \(\bQ\) and of \(\bP\) on the system of interest in state \(\ket{\psi}\). However, one can indicate how much these marginals deviate from separate exact position and momentum measurements on the state \(\ket{\psi}\) by a pairwise comparison of ( 31 ) to the exact distributions
In order to do so, BLW propose a distance function \(D\) between probability distributions, such that \(D(\mu, \mu')\) tells us how close the marginal position distribution \(\mu'(q)\) for the unsharp position \(Q'\) is to the exact distribution \(\mu(q)\) in a sharp position measurement, and likewise, \(D(\nu ,\nu')\) tells us how close the marginal momentum distribution \(\nu'(p)\) for \(P'\) is to the exact momentum distribution \(\nu(p)\).
The distance they chose is the Wasserstein-2 distance, a.k.a. (a variation on) the earth-movers distance.
Definition (Wasserstein-2 distance) Let \(\mu(x)\) and \(\mu'(y)\) be any two probability distributions on the real line, and \(\gamma(x,y)\) any joint probability distribution that has \(\mu'\) and \(\mu\) as its marginals. Then:
Applying this definition to the case at hand, i.e. pairwise to the quantum mechanical distributions \(\mu'(q)\) and \(\mu(q)\) and to \(\nu'(p)\) and \(\nu(p)\) in ( 31 ) and (32) , BLW’s final step is to take a supremum over all possible input states \(\ket{\psi}\) to obtain
From these definitions, they obtain
Arguing that \(\Delta(Q, Q')\) provides a sensible measure for the inaccuracy or noise about position, and \(\Delta(P, P')\) for the disturbance of momentum by any such joint unsharp measurement, the authors conclude, in contrast to Ozawa’s analysis, that an error-disturbance uncertainty relation does hold, which they take as “a remarkable vindication of Heisenberg’s intuitions” in the microscope thought experiment.
In comparison of the two, there are a few positive remarks to make about the Busch-Lahti-Werner (BLW) approach. First of all, by focusing on the distance (33) this approach is comparing entire probability distributions rather than just the expectations of operator differences. When this distance is very small, one is justified to conclude that the distribution has changed very little under the measurement procedure. This brings us closer to the conclusion that the error or disturbance introduced is small. Secondly, by introducing a supremum over all states to obtain \(\Delta( Q, Q')\), it follows that when this latter expression is small, the measured distribution \(\mu'\) differs only little from the exact distribution \(\mu\) whatever the state of the system is. As the authors argue, this means that \(\Delta(Q,Q')\) can be seen as a figure-of-merit of the measurement device alone, and in this sense analogous to the resolving power of a microscope.
But we also think there is an undesirable feature of the BLW approach. This is due to the supremum over states appearing twice , both in \(\Delta(Q,Q')\) and in \(\Delta(P,P')\). This feature, we argue, deprives their result from practical applicability.
To elucidate: In concrete applications, one would prepare a system in some state (not exactly known) and perform a given joint measurement \(M\) of \(Q'\) and \(P'\). If it is given that, say, \(\Delta(Q,Q')\) is very small, one can safely infer that \(Q\) has been measured with small inaccuracy, since this guarantees that the measured position distribution differs very little from what an exact position measurement would give, regardless of the state of the system. Now, one would like to be able to infer that in this case the disturbance of the momentum \(P\) from \(P'\) must be considerable for the state prepared . But the BLW only gives us:
and this does not imply anything for the state in question! Thus, the BLW uncertainty relation does not rule out that for some states it might be possible to perform a joint measurement in which both \(D(\mu, \mu')\) and \(D(\nu, \nu')\) are very small, and in this sense have negligibe error and disturbance. It seems premature to say that this vindicates Heisenberg’s intuitions.
Summing up, we emphasize that there is no contradiction between the BLW analysis and the Ozawa analysis: where Ozawa claims that the product of two quantities might for some states be less than the usual limit, BLW show that product of different quantities will satisfy this limit. The dispute is not about mathematically validity, but about how reasonable these quantities are to capture Heisenberg’s qualitative considerations. The present authors feel that, in this dispute, Ozawa’s analysis fail to be convincing. On the other hand, we also think that the BLW uncertainty relation is not satisfactory. Also, we would like to remark that both protagonists employ measures that are akin to standard deviations in being very sensitive to the tail behavior of probability distributions, and thus face a similar objection as raised in section 5 . The final word in this dispute on whether a measurement uncertainty principle holds has not been reached, in our view.
- Bacciagaluppi, G. and A. Valentini, 2009, Quantum Theory at the Crossroads; reconsidering the 1927 Solvay Conference , Cambridge: Cambridge University Press.
- Beller, M., 1999, Quantum Dialogue , Chicago: University of Chicago Press.
- Beckner, W., 1975, “Inequalities in Fourier analysis”, Annals of Mathematics , 102: 159–182.
- Białinicki-Birula, I. and J. Micielski, 1975, “Uncertainty relations for information entropy in wave mechanics”, Communications in Mathematical Physics , 44: 129–132.
- Bohr, N., 1928, “The Quantum postulate and the recent development of atomic theory”, Nature , (Supplement) 121: 580–590. Also in Bohr 1934, Wheeler and Zurek 1983, and Bohr 1985.
- –––, 1929, “Introductory survey”, in Bohr 1934: 1–24.
- –––, 1934, Atomic Theory and the Description of Nature , Cambridge: Cambridge University Press. Reissued in 1961. Appeared also as Volume I of The Philosophical Writings of Niels Bohr , Woodbridge, CT: Ox Bow Press, 1987.
- –––, 1937, “Causality and complementarity”, Philosophy of Science , 4: 289–298.
- –––, 1939, “The causality problem in atomic physics”, in New Theories in Physics , Paris: International Institute of Intellectual Co-operation. Also in Bohr 1996: 303–322.
- –––, 1948, “On the notions of causality and complementarity”, Dialectica , 2: 312–319. Also in Bohr 1996: 330–337.
- –––, 1949, “Discussion with Einstein on epistemological problems in atomic physics”, in Albert Einstein: philosopher-scientist. The library of living philosophers Vol. VII , P.A. Schilpp (ed.), La Salle: Open Court, pp. 201–241.
- –––, 1985, Collected Works , Volume 6, J. Kalckar (ed.) Amsterdam: North-Holland.
- –––, 1996, Collected Works , Volume 7, J. Kalckar (ed.) Amsterdam: North-Holland.
- Bub, J., 2000, “Quantum mechanics as a principle theory”, Studies in History and Philosophy of Modern Physics , 31B: 75–94.
- Busch, P., 1990, “ On the energy-time uncertainty relation”, Foundations of Physics , 20: 1–32, 33–43.
- Busch, P., P. Lahti, and R. Werner, 2013, “Proof of Heisenberg’s error-disturbance relation”, Physical Review Letters , 111, 160405. doi:10.1103/PhysRevLett.111.160405
- Cassidy, D.C., 1992, Uncertainty, the Life and Science of Werner Heisenberg , New York: Freeman.
- –––, 1998, “Answer to the question: When did the indeterminacy principle become the uncertainty principle?”, American Journal of Physics , 66: 278–279.
- Chiribella, G. and R.W. Spekkens, 2016, Quantum Theory, Informational Foundations and Foils , Dordrecht: Springer.
- Condon, E.U., 1929, “Remarks on uncertainty principles”, Science , 69: 573–574.
- Eddington, A., 1928, The Nature of the Physical World , Cambridge: Cambridge University Press.
- Einstein, A., 1919, “My Theory”, The Times (London), November 28, p. 13; reprinted as “What is the theory of relativity?”, in Ideas and Opinions , New York: Crown Publishers, 1954, pp. 227–232.
- Folse, H.J., 1985, The Philosophy of Niels Bohr , Amsterdam: Elsevier.
- Frank, R.L. and E.H. Lieb, 2012, “Entropy and the uncertainty principle”, Annales Henri Poincaré , 13: 1711–1717.
- Heisenberg, W., 1925, “Über quantentheoretische Umdeutung kinematischer und mechanischer Beziehungen”, Zeitschrift für Physik , 33: 879–893.
- –––, 1926, “Quantenmechanik”, Die Naturwissenschaften , 14: 899–894.
- –––, 1927, “Ueber den anschaulichen Inhalt der quantentheoretischen Kinematik and Mechanik”, Zeitschrift für Physik , 43: 172–198. English translation in Wheeler and Zurek 1983: 62–84.
- –––, 1927, “Ueber die Grundprincipien der ‘Quantenmechanik’ “ Forschungen und Fortschritte , 3: 83.
- –––, 1928, “Erkenntnistheoretische Probleme der modernen Physik”, in Heisenberg 1984: 22–28.
- –––, 1930, Die Physikalischen Prinzipien der Quantenmechanik , Leipzig: Hirzel. English translation The Physical Principles of Quantum Theory , Chicago: University of Chicago Press, 1930.
- –––, 1931, “Die Rolle der Unbestimmtheitsrelationen in der modernen Physik”, Monatshefte für Mathematik und Physik , 38: 365–372.
- –––, 1958, Physics and Philosophy , New York: Harper.
- –––, 1969, Der Teil und das Ganze , München : Piper.
- –––, 1975, “Bemerkungen über die Entstehung der Unbestimmtheitsrelation”, Physikalische Blätter , 31: 193–196. Translation in Price and Chissick, 1977.
- –––, 1984, Gesammelte Werke , Volume C1, W. Blum, H.-P. Dürr, and H. Rechenberg (eds), München: Piper.
- Hilgevoord, J., 1996, “The uncertainty principle for energy and time I”, American Journal of Physics , 64: 1451–1456.
- –––, 1998, “The uncertainty principle for energy and time II”, American Journal of Physics , 66: 396–402.
- –––, 2002, “Time in quantum mechanics”, American Journal of Physics , 70: 301–306.
- –––, 2005, “Time in quantum mechanics: a story of confusion. Studies in History and Philosophy of Modern Physics , 36: 29–60.
- Hilgevoord, J. and D. Atkinson, 2011, “Time in quantum mechanics”, in The Oxford Handbook of Philosophy of Time , C. Callender (ed.), Oxford: Oxford University Press, pp. 647–662.
- Hilgevoord, J. and J. Uffink, 1988, “The mathematical expression of the uncertainty principle”, in Microphysical Reality and Quantum Description , A. van der Merwe et al. (eds.), Dordrecht: Kluwer, pp. 91–114.
- –––, 1990, “A new view on the uncertainty principle”, in Sixty-Two years of Uncertainty, Historical and Physical Inquiries into the Foundations of Quantum Mechanics , A.E. Miller (ed.), New York: Plenum, pp. 121–139.
- –––, 1991, “Uncertainty in prediction and inference”, Foundations of Physics , 21: 323–341.
- Honner, J., 1987, The Description of Nature: Niels Bohr and The Philosophy of Quantum Physics , Oxford: Clarendon Press.
- Jammer, M., 1974, The Philosophy of Quantum Mechanics , New York: Wiley.
- Jordan, P., 1927, “Über eine neue Begründung der Quantenmechanik II”, Zeitschrift für Physik , 44: 1–25.
- Kaiser, H., S.A. Werner, and E.A. George, 1983, “Direct measurement of the longitudinal coherence length of a thermal neutron beam”, Physical Review Letters , 50: 560.
- Kennard, E.H., 1927, “Zur Quantenmechanik einfacher Bewegungstypen”, Zeitschrift für Physik , 44: 326–352.
- Landau, H.J. and H.O. Pollak, 1961, “Prolate spheroidal wave functions; Fourier analysis and uncertainty II”, Bell Systems Technical Journal , 40: 63–84.
- Maassen, H. and J. Uffink, 1988, “Generalized entropic uncertainty relations”, Physical Review Letters , 60: 1103–1106.
- Miller, A.I., 1982, “Redefining Anschaulichkeit”, in: A. Shimony and H.Feshbach (eds) Physics as Natural Philosophy , Cambridge, MA: MIT Press.
- Moore, W., 1989, Schrödinger, Life and Thought , Cambridge: Cambridge University Press, p. 221.
- Muga, J.G., R. Sala Mayato, and I.L. Egusquiza (eds.), 2002, Time in quantum mechanics , Berlin: Springer.
- Muller, F.A., 1997, “The equivalence myth of quantum mechanics”, Studies in History and Philosophy of Modern Physics , 28: 35–61, 219–247; ibid. 30(1999): 543–545.
- Murdoch, D., 1987, Niels Bohr’s Philosophy of Physics , Cambridge: Cambridge University Press.
- Nairz, O., M. Andt, and A. Zeilinger, 2002, “Experimental verification of the Heisenberg uncertainty principle for fullerene molecules”, Physical Review A , 65, 032109. doi:10.1103/PhysRevA.65.032109
- Ozawa, M., 2003, “Universally valid formulation of the Heisenberg uncertainty relation on noise and disturbance in measurement. Physical Review A , 67: 042105.
- Pashby, T., 2015, “Time and quantum theory: A history and a prospectus”, Studies in History and Philosophy of Modern Physics , 52: 24–38.
- Pauli, W., 1979, Wissentschaftlicher Briefwechsel mit Bohr, Einstein, Heisenberg u.a. , Volume 1 (1919–1929) A. Hermann, K. von Meyenn and V.F. Weiskopf (eds) Berlin: Springer.
- Popper, K., 1967, “Quantum mechanics without ‘the observer’”, in Quantum Theory and Reality , M. Bunge (ed.), Berlin: Springer.
- Price, W.C. and S.S. Chissick (eds), 1977, The Uncertainty Principle and the Foundations of Quantum Mechanics , New York: Wiley.
- Regt, H. de, 1997, “Erwin Schrödinger, Anschaulichkeit , and quantum theory”, Studies in History and Philosophy of Modern Physics , 28: 461–481.
- Robertson, H.P., 1929, “The uncertainty principle”, Physical Review , 34: 573–574; reprinted in Wheeler and Zurek 1983: 127–128.
- Scheibe, E., 1973, The Logical Analysis of Quantum Mechanics , Oxford: Pergamon Press.
- Uffink, J., 1985, “Verification of the uncertainty principle in neutron interferometry”, Physics Letters , 108 A: 59–62.
- –––, 1990, Measures of Uncertainty and the Uncertainty Principle , Ph.D. thesis, University of Utrecht, available online with online errata .
- –––, 1993, “The rate of evolution of a quantum state”, American Journal of Physics , 61: 935–936.
- –––, 1994, “The joint measurement problem”, International Journal of Theoretical Physics , 33: 199–212.
- Uffink, J. and J. Hilgevoord, 1985, “Uncertainty principle and uncertainty relations”, Foundations of Physics , 15: 925–944.
- von Neumann, J., 1932, Mathematische Grundlagen der Quantenmechanik , Berlin: J. Springer.
- Wheeler, J.A. and W.H. Zurek (eds), 1983, Quantum Theory and Measurement , Princeton, NJ: Princeton University Press.
How to cite this entry . Preview the PDF version of this entry at the Friends of the SEP Society . Look up topics and thinkers related to this entry at the Internet Philosophy Ontology Project (InPhO). Enhanced bibliography for this entry at PhilPapers , with links to its database.
- Busch, P., P. Lahti, and R. Werner, 2014, “ Measurement uncertainty: Reply to critics ,” February 13, arXiv:1402.3102 [quant-ph].
- Ozawa, M., 2013, “ Disproving Heisenberg’s error-disturbance relation ,” August 16, arXiv:1308.3540 [quant-ph].
- Exhibit on Heisenberg and the uncertainty principle , from the American Institute of Physics
- The Nobel prize site , containing a short biography of Heisenberg and his 1932 Nobel presentation speech
quantum mechanics | quantum mechanics: Bohmian mechanics | quantum mechanics: Copenhagen interpretation of
Copyright © 2016 by Jan Hilgevoord Jos Uffink < jbuffink @ umn . edu >
- Accessibility
Support SEP
Mirror sites.
View this site from another server:
- Info about mirror sites
The Stanford Encyclopedia of Philosophy is copyright © 2023 by The Metaphysics Research Lab , Department of Philosophy, Stanford University
Library of Congress Catalog Data: ISSN 1095-5054
Sense about Science
What does uncertainty mean for scientists.
Scientific researchers have to work out whether uncertainty can be calculated and how to do so, and then whether it matters and what can be done about it.
Uncertainty is a hard thing to discuss because it is inherently intangible. The difficulty expressing it is not exclusive to science. It became familiar after a rather baffling speech by US politician Donald Rumsfeld in 2002:
“Reports that say that something hasn’t happened are always interesting to me, because as we know, there are known knowns; there are things we know we know. We also know there are known unknowns; that is to say we know there are some things we do not know. But there are also unknown unknowns – the ones we don’t know we don’t know.”
Rumsfeld had a point. Some scientific uncertainty can be ‘known:’ in a sense, signposted. There is uncertainty about how much global temperatures will change if carbon dioxide emissions continue at current levels for the next 50 years. But researchers know what information they would need to work this out – it is a ‘known unknown.’ Knowing just what it is that we don’t yet know is very useful because it guides further research to find an answer.
But we also face ‘unknown unknowns’ – questions not yet thought of and information we do not realise is missing.
These concepts of uncertainty are like the difference between a Victorian map with central Africa marked ‘unexplored,’ which indicates ‘known unknown’ data, and medieval maps made before Europeans knew that the New World existed, which show sea where the land should be.
There are uncertainties we can work out and uncertainties we can’t:
“If I drop a tennis ball, what will happen? There is some uncertainty: I don’t know details about this particular ball. I haven’t done experiments to see whether the ball falls every time. But I still know, almost certainly, that the ball will fall. I know that this would be true if I was standing in Australia, and that the way it falls would be different if I was standing on the moon. There isn’t 100% certainty because the situation might be different from what we expect – if I were working with a magician I might be suspicious about the possibility of tricks; maybe magnets in the ball and the ceiling. This could be the case. But without evidence for it I would be foolish to bet against the ball falling. Indeed I’d bet a lot that the ball would fall. Now think of a different question. When I drop the tennis ball, where will it stop? This is difficult to tell. It might bounce off a chair, a table or someone’s foot. I can calculate some limits to give me a rough idea of where the ball might end up but there will remain substantial uncertainty. When we’re thinking about how the climate might change, the system is more complicated but the same thinking applies. There are many details about which we are extremely uncertain but there is very little uncertainty over the big picture of serious warming and significant disruption for human societies. The ball will fall we just don’t know where it will stop.” – David Stainforth Prof David Stainforth is a physicist with extensive experience of climate modelling. He co-founded and was chief scientist of the climateprediction.net project, the world’s largest climate modelling experiment.
The best approach to uncertainty is pragmatism. While it is important to be alert to the possibility of ‘unknown unknowns’ (questions not yet thought of and information we do not realise is missing) that possibility alone doesn’t point us towards better explanations.
On the other hand, if researchers are describing the uncertainty in how they understand a particular problem, it actually means quite a lot is known in this case about the strengths and weaknesses of the science. They may know enough about the uncertainty to be able to quantify it, e.g. ‘there is a 20% chance of rain.’ So, if we hear scientists talking about the uncertainty in their findings, in some detail, we should be more reassured rather than more worried!
There are some well-established principles in statistics for calculating and expressing uncertainty. They ask whether an observation or result is in the right ball-park, or how likely it is that an observation made in a piece of research is not simply a random event.
Confidence intervals tell us how reliable researchers think their observations are. Researchers gather data; if, for example, they want to find the average height of people in London, they measure the heights of a sample of Londoners and calculate the mean average. There is some chance that the mean average (the value) the researchers have calculated is not correct. The real average height of Londoners could be a bit higher or a bit lower.
The researchers need to express that uncertainty about the value as precisely as possible so that their result is useful to other researchers and everyone understands how likely it is to be right. Using the range of observed individual heights they can calculate the range (the confidence limits) that they would expect the mean average to fall within for at least 95 out of 100 repeats of the measurement exercise. This is a 95% confidence interval. If they wanted to know the limits of that range for a 99% confidence interval, i.e. where the mean average is likely to fall in 99 out of 100 repeats of the experiment, they would expect to give a bigger range.
This trade-off between precision and certainty is a fairly intuitive point. If you throw a ball at a target on the floor and are asked to draw a circle within which you are confident it will land, you would draw a bigger circle around the target if it had to land in the circle 99 times out of 100 than if it had to land in the circle 90 times out of 100.
When researchers are looking at cause-and-effect relationships, they usually calculate uncertainty by asking how likely it is that their observations represent a real effect, rather than simply happening by chance. This question is incorporated into the design of the study. Researchers start with a description of what they would expect to see if there was no effect (a ‘null hypothesis’); they then look at how close the match is between this pattern and their observations. Researchers can put a number on the extent to which the results and the hypothesis match (a ‘p-value’). This is essentially asking, ‘is our result different enough from a pattern of ‘no effect’ that there really looks to be something going on?’
Where uncertainty is known, it can be incorporated into the way that effects are predicted. For example, there is some uncertainty in the initial conditions that form the basis of a hurricane forecast. In the past, forecasters showed one predicted path of a hurricane, with caveats about the uncertainty of the initial conditions. Now they tend to use the uncertainty to vary the initial conditions they put into the forecast and show the resulting range of what the hurricane will do – an ensemble forecast – so we can see how many of the forecasts come up with a similar path.
Precise numbers should only be used where they are justified:
“In clinical medicine, doctors cannot predict exactly what will happen to anyone, and so may use a phrase such as ‘of 100 people like you, 96 will survive the operation’. Sometimes there is such limited evidence, say because a patient’s condition is completely novel, that no number can be attached with any confidence.” – David Spiegelhalter Sir David Spiegelhalter OBE FRS is a statistician and chair of the Winton Centre for Risk and Evidence Communication. “When the uncertainty makes the range of possibilities very broad, we should avoid trying to come up with a single, precise number because it creates a false impression of certainty – spurious precision.” – Michael Hanlon Michael Hanlon was a journalist and Science page editor at the Daily Telegraph.
Use separate measures for how good the evidence is and how confident the conclusion
Organisations have come up with new ways of expressing their confidence in the data. In medicine, the GRADE scale (a grading system for evidence and recommendations) is widely used, which takes into account the quality of the underlying evidence. And, in climate science, the most recent Intergovernmental Panel on Climate Change (IPCC) assessment used one verbal scale to express their confidence in the scientific understanding, and another to give the likelihood of something occurring (where ‘virtually certain’ corresponds to a level of more than a 99% likelihood of happening, for example).
Separate long-term effects from short-term effects
“Glaciologists use satellite data to assess changes in ice-sheet elevation and estimate their impact on sea level. We need to know whether these are long-term changes (for example produced by climate change) or just short-term fluctuations (for example produced by particularly heavy snowfall, or if a particularly warm summer makes the surface snow denser). We can understand the uncertainty in the satellite observations of long-term change better by defining the size of the effect that short-term fluctuations could have on the satellite.” – Elizabeth Morris Prof Elizabeth Morris OBE is a glaciologist and Emeritus Associate at the Scott Polar Research Institute at Cambridge University.
Sometimes it is a question of making more observations or designing experiments or computational models to improve the available information. This is not always as straightforward as it might seem, and it can take a while to identify the information that is missing.
“The early climate model simulations only included the effect of greenhouse gases. When run over the last 100 years or so for which we have instrumental measurements of surface temperature, these simulations were warm compared to observations. In time, the effect of other factors (industrial aerosols produced by burning fossil fuels, the effect of volcanic eruptions, possible variations in solar irradiance) were added. The agreement of scientists models with our observations improved, particularly due to the inclusion of the effect of industrial aerosols.” – John Mitchell Prof John Mitchell is a leading academic in communications systems engineering.
Sometimes it is only through technological developments that uncertainty can be reduced. This has happened in the search for exoplanets (planets that go round stars outside our solar system) – a step towards detecting whether there is life on other planets. These planets were only detected very recently when instruments and techniques became sufficiently accurate, but progress has been rapid. The first exoplanets were discovered in the 1990s, and thousands have now been identified. Their discovery is progressively reducing the degree of uncertainty we have about things like the existence of life on other planets, by improving our estimates of the number of planets outside the solar system, and of the fraction of these that may be able to support life.
But reducing uncertainty is often not possible or necessary and this is not a barrier to using scientific knowledge. The question is not ‘do we know everything?’ It is ‘do we know enough?’ or ‘how can we best make a decision using what we do know?’.
The question is not ‘do we know everything?’ It is ‘do we know enough?’ or ‘how can we best make a decision using what we do know?’
For example, Newton’s law of gravity is still sufficient to get us to the moon and back in spite of not being a complete model of the way gravity works. Or if, say, all values within a confidence interval point to the same clinical decision, further narrowing the uncertainty may be a waste of public funds. Researchers can incorporate uncertainty into calculations or work round it.
Quantify ‘routine’ uncertainty and incorporate it into calculations
Uncertainty is considered routine in a lot of cases – it can’t be eliminated but it’s not necessarily problematic (and a decision can be made ‘beyond reasonable doubt’). For example, there is a degree of imprecision in assessing the fetal age from ultrasound scans during pregnancy (which are used to estimate the due date). This arises from the natural variation of size-for-age and from differences in the skill, experience and equipment of the ultrasonographer. However, we can quantify this uncertainty and use it to explain to women that the calculated due date should be used as a guide, rather than an exact prediction of the actual due date.
Calculate risk, a more usable measure of uncertainty
When uncertainty relates to a real-life situation with important and perhaps detrimental consequences we can work out how the uncertainty affects the risk, which is more tangible.
There is some confusion between scientific and everyday uses of the words ‘uncertainty’ and ‘risk’. In everyday language, we might say that something that is uncertain is risky. But in scientific terms, risk broadly means uncertainty that can be quantified in relation to a particular hazard – and so for a given hazard, the risk is the chance of it happening. For example, research shows that oestrogen therapy (a form of HRT, to relieve the symptoms of menopause) appears to increase the risk of women suffering from heart disease (an associated hazard). Current evidence suggests the risk is small, but there is a lot of uncertainty around the estimate and so the real effect could be larger or smaller than estimated.
Risk is also a way of thinking about which uncertainties to worry about. For example, there is a greater risk of agricultural chemicals running off into waterways and damaging aquatic life when land is bare. We should therefore be more interested in reducing uncertainty about the hazard to aquatic life of those chemicals used on bare land. Risk is especially important when we want information that is useful for decision-making.
Mitigate the effects of uncertain events
Another response to uncertainty is mitigation. There is currently irresolvable uncertainty about earthquakes: researchers know where they are going to occur, but cannot predict when they will happen.
“In a scientific sense, earthquakes are unpredictable. But that does not mean that you can’t predict things about them.” – Peter Sammonds Prof Peter Sammonds is a leading academic in geophysics and climate risks.
Using historical data and geology, researchers can calculate how frequently a particular scale of earthquake is likely to occur in a specific location. A one-in-ten year calculation doesn’t say anything about which ten years in 100 will see an earthquake. But it means researchers can calculate what kind of reinforcement to buildings would be needed to make sure they could withstand this frequency of quakes. If a serious earthquake is a one-in-200 year event, a community might only put resources into reinforcing a building that is designed to last ten years if its function was sufficiently important – a school or hospital, for example.
This guide is based on the booklet, Making Sense of Uncertainty (2013)
Sense about Science 41 Portland Place, London W1B 1QH
T: + 44 7432 849 547 E: [email protected]

- Search Menu
- Browse content in Arts and Humanities
- Browse content in Archaeology
- Anglo-Saxon and Medieval Archaeology
- Archaeological Methodology and Techniques
- Archaeology by Region
- Archaeology of Religion
- Archaeology of Trade and Exchange
- Biblical Archaeology
- Contemporary and Public Archaeology
- Environmental Archaeology
- Historical Archaeology
- History and Theory of Archaeology
- Industrial Archaeology
- Landscape Archaeology
- Mortuary Archaeology
- Prehistoric Archaeology
- Underwater Archaeology
- Urban Archaeology
- Zooarchaeology
- Browse content in Architecture
- Architectural Structure and Design
- History of Architecture
- Residential and Domestic Buildings
- Theory of Architecture
- Browse content in Art
- Art Subjects and Themes
- History of Art
- Industrial and Commercial Art
- Theory of Art
- Biographical Studies
- Byzantine Studies
- Browse content in Classical Studies
- Classical Literature
- Classical Reception
- Classical History
- Classical Philosophy
- Classical Mythology
- Classical Art and Architecture
- Classical Oratory and Rhetoric
- Greek and Roman Archaeology
- Greek and Roman Epigraphy
- Greek and Roman Law
- Greek and Roman Papyrology
- Late Antiquity
- Religion in the Ancient World
- Digital Humanities
- Browse content in History
- Colonialism and Imperialism
- Diplomatic History
- Environmental History
- Genealogy, Heraldry, Names, and Honours
- Genocide and Ethnic Cleansing
- Historical Geography
- History by Period
- History of Agriculture
- History of Education
- History of Emotions
- History of Gender and Sexuality
- Industrial History
- Intellectual History
- International History
- Labour History
- Legal and Constitutional History
- Local and Family History
- Maritime History
- Military History
- National Liberation and Post-Colonialism
- Oral History
- Political History
- Public History
- Regional and National History
- Revolutions and Rebellions
- Slavery and Abolition of Slavery
- Social and Cultural History
- Theory, Methods, and Historiography
- Urban History
- World History
- Browse content in Language Teaching and Learning
- Language Learning (Specific Skills)
- Language Teaching Theory and Methods
- Browse content in Linguistics
- Applied Linguistics
- Cognitive Linguistics
- Computational Linguistics
- Forensic Linguistics
- Grammar, Syntax and Morphology
- Historical and Diachronic Linguistics
- History of English
- Language Variation
- Language Families
- Language Acquisition
- Language Evolution
- Language Reference
- Lexicography
- Linguistic Theories
- Linguistic Typology
- Linguistic Anthropology
- Phonetics and Phonology
- Psycholinguistics
- Sociolinguistics
- Translation and Interpretation
- Writing Systems
- Browse content in Literature
- Bibliography
- Children's Literature Studies
- Literary Studies (Modernism)
- Literary Studies (Asian)
- Literary Studies (European)
- Literary Studies (Eco-criticism)
- Literary Studies (Romanticism)
- Literary Studies (American)
- Literary Studies - World
- Literary Studies (1500 to 1800)
- Literary Studies (19th Century)
- Literary Studies (20th Century onwards)
- Literary Studies (African American Literature)
- Literary Studies (British and Irish)
- Literary Studies (Early and Medieval)
- Literary Studies (Fiction, Novelists, and Prose Writers)
- Literary Studies (Gender Studies)
- Literary Studies (Graphic Novels)
- Literary Studies (History of the Book)
- Literary Studies (Plays and Playwrights)
- Literary Studies (Poetry and Poets)
- Literary Studies (Postcolonial Literature)
- Literary Studies (Queer Studies)
- Literary Studies (Science Fiction)
- Literary Studies (Travel Literature)
- Literary Studies (War Literature)
- Literary Studies (Women's Writing)
- Literary Theory and Cultural Studies
- Mythology and Folklore
- Shakespeare Studies and Criticism
- Browse content in Media Studies
- Browse content in Music
- Applied Music
- Dance and Music
- Ethics in Music
- Ethnomusicology
- Gender and Sexuality in Music
- Medicine and Music
- Music Cultures
- Music and Culture
- Music and Religion
- Music and Media
- Music Education and Pedagogy
- Music Theory and Analysis
- Musical Scores, Lyrics, and Libretti
- Musical Structures, Styles, and Techniques
- Musicology and Music History
- Performance Practice and Studies
- Race and Ethnicity in Music
- Sound Studies
- Browse content in Performing Arts
- Browse content in Philosophy
- Aesthetics and Philosophy of Art
- Epistemology
- Feminist Philosophy
- History of Western Philosophy
- Metaphysics
- Moral Philosophy
- Non-Western Philosophy
- Philosophy of Action
- Philosophy of Law
- Philosophy of Religion
- Philosophy of Science
- Philosophy of Language
- Philosophy of Mind
- Philosophy of Perception
- Philosophy of Mathematics and Logic
- Practical Ethics
- Social and Political Philosophy
- Browse content in Religion
- Biblical Studies
- Christianity
- East Asian Religions
- History of Religion
- Judaism and Jewish Studies
- Qumran Studies
- Religion and Education
- Religion and Health
- Religion and Politics
- Religion and Science
- Religion and Law
- Religion and Art, Literature, and Music
- Religious Studies
- Browse content in Society and Culture
- Cookery, Food, and Drink
- Cultural Studies
- Customs and Traditions
- Ethical Issues and Debates
- Hobbies, Games, Arts and Crafts
- Lifestyle, Home, and Garden
- Natural world, Country Life, and Pets
- Popular Beliefs and Controversial Knowledge
- Sports and Outdoor Recreation
- Technology and Society
- Travel and Holiday
- Visual Culture
- Browse content in Law
- Arbitration
- Browse content in Company and Commercial Law
- Commercial Law
- Company Law
- Browse content in Comparative Law
- Systems of Law
- Competition Law
- Browse content in Constitutional and Administrative Law
- Government Powers
- Judicial Review
- Local Government Law
- Military and Defence Law
- Parliamentary and Legislative Practice
- Construction Law
- Contract Law
- Browse content in Criminal Law
- Criminal Procedure
- Criminal Evidence Law
- Sentencing and Punishment
- Employment and Labour Law
- Environment and Energy Law
- Browse content in Financial Law
- Banking Law
- Insolvency Law
- History of Law
- Human Rights and Immigration
- Intellectual Property Law
- Browse content in International Law
- Private International Law and Conflict of Laws
- Public International Law
- IT and Communications Law
- Jurisprudence and Philosophy of Law
- Law and Society
- Law and Politics
- Browse content in Legal System and Practice
- Courts and Procedure
- Legal Skills and Practice
- Primary Sources of Law
- Regulation of Legal Profession
- Medical and Healthcare Law
- Browse content in Policing
- Criminal Investigation and Detection
- Police and Security Services
- Police Procedure and Law
- Police Regional Planning
- Browse content in Property Law
- Personal Property Law
- Study and Revision
- Terrorism and National Security Law
- Browse content in Trusts Law
- Wills and Probate or Succession
- Browse content in Medicine and Health
- Browse content in Allied Health Professions
- Arts Therapies
- Clinical Science
- Dietetics and Nutrition
- Occupational Therapy
- Operating Department Practice
- Physiotherapy
- Radiography
- Speech and Language Therapy
- Browse content in Anaesthetics
- General Anaesthesia
- Neuroanaesthesia
- Browse content in Clinical Medicine
- Acute Medicine
- Cardiovascular Medicine
- Clinical Genetics
- Clinical Pharmacology and Therapeutics
- Dermatology
- Endocrinology and Diabetes
- Gastroenterology
- Genito-urinary Medicine
- Geriatric Medicine
- Infectious Diseases
- Medical Oncology
- Medical Toxicology
- Pain Medicine
- Palliative Medicine
- Rehabilitation Medicine
- Respiratory Medicine and Pulmonology
- Rheumatology
- Sleep Medicine
- Sports and Exercise Medicine
- Clinical Neuroscience
- Community Medical Services
- Critical Care
- Emergency Medicine
- Forensic Medicine
- Haematology
- History of Medicine
- Medical Ethics
- Browse content in Medical Dentistry
- Oral and Maxillofacial Surgery
- Paediatric Dentistry
- Restorative Dentistry and Orthodontics
- Surgical Dentistry
- Browse content in Medical Skills
- Clinical Skills
- Communication Skills
- Nursing Skills
- Surgical Skills
- Medical Statistics and Methodology
- Browse content in Neurology
- Clinical Neurophysiology
- Neuropathology
- Nursing Studies
- Browse content in Obstetrics and Gynaecology
- Gynaecology
- Occupational Medicine
- Ophthalmology
- Otolaryngology (ENT)
- Browse content in Paediatrics
- Neonatology
- Browse content in Pathology
- Chemical Pathology
- Clinical Cytogenetics and Molecular Genetics
- Histopathology
- Medical Microbiology and Virology
- Patient Education and Information
- Browse content in Pharmacology
- Psychopharmacology
- Browse content in Popular Health
- Caring for Others
- Complementary and Alternative Medicine
- Self-help and Personal Development
- Browse content in Preclinical Medicine
- Cell Biology
- Molecular Biology and Genetics
- Reproduction, Growth and Development
- Primary Care
- Professional Development in Medicine
- Browse content in Psychiatry
- Addiction Medicine
- Child and Adolescent Psychiatry
- Forensic Psychiatry
- Learning Disabilities
- Old Age Psychiatry
- Psychotherapy
- Browse content in Public Health and Epidemiology
- Epidemiology
- Public Health
- Browse content in Radiology
- Clinical Radiology
- Interventional Radiology
- Nuclear Medicine
- Radiation Oncology
- Reproductive Medicine
- Browse content in Surgery
- Cardiothoracic Surgery
- Gastro-intestinal and Colorectal Surgery
- General Surgery
- Neurosurgery
- Paediatric Surgery
- Peri-operative Care
- Plastic and Reconstructive Surgery
- Surgical Oncology
- Transplant Surgery
- Trauma and Orthopaedic Surgery
- Vascular Surgery
- Browse content in Science and Mathematics
- Browse content in Biological Sciences
- Aquatic Biology
- Biochemistry
- Bioinformatics and Computational Biology
- Developmental Biology
- Ecology and Conservation
- Evolutionary Biology
- Genetics and Genomics
- Microbiology
- Molecular and Cell Biology
- Natural History
- Plant Sciences and Forestry
- Research Methods in Life Sciences
- Structural Biology
- Systems Biology
- Zoology and Animal Sciences
- Browse content in Chemistry
- Analytical Chemistry
- Computational Chemistry
- Crystallography
- Environmental Chemistry
- Industrial Chemistry
- Inorganic Chemistry
- Materials Chemistry
- Medicinal Chemistry
- Mineralogy and Gems
- Organic Chemistry
- Physical Chemistry
- Polymer Chemistry
- Study and Communication Skills in Chemistry
- Theoretical Chemistry
- Browse content in Computer Science
- Artificial Intelligence
- Computer Architecture and Logic Design
- Game Studies
- Human-Computer Interaction
- Mathematical Theory of Computation
- Programming Languages
- Software Engineering
- Systems Analysis and Design
- Virtual Reality
- Browse content in Computing
- Business Applications
- Computer Games
- Computer Security
- Computer Networking and Communications
- Digital Lifestyle
- Graphical and Digital Media Applications
- Operating Systems
- Browse content in Earth Sciences and Geography
- Atmospheric Sciences
- Environmental Geography
- Geology and the Lithosphere
- Maps and Map-making
- Meteorology and Climatology
- Oceanography and Hydrology
- Palaeontology
- Physical Geography and Topography
- Regional Geography
- Soil Science
- Urban Geography
- Browse content in Engineering and Technology
- Agriculture and Farming
- Biological Engineering
- Civil Engineering, Surveying, and Building
- Electronics and Communications Engineering
- Energy Technology
- Engineering (General)
- Environmental Science, Engineering, and Technology
- History of Engineering and Technology
- Mechanical Engineering and Materials
- Technology of Industrial Chemistry
- Transport Technology and Trades
- Browse content in Environmental Science
- Applied Ecology (Environmental Science)
- Conservation of the Environment (Environmental Science)
- Environmental Sustainability
- Environmentalist Thought and Ideology (Environmental Science)
- Management of Land and Natural Resources (Environmental Science)
- Natural Disasters (Environmental Science)
- Nuclear Issues (Environmental Science)
- Pollution and Threats to the Environment (Environmental Science)
- Social Impact of Environmental Issues (Environmental Science)
- History of Science and Technology
- Browse content in Materials Science
- Ceramics and Glasses
- Composite Materials
- Metals, Alloying, and Corrosion
- Nanotechnology
- Browse content in Mathematics
- Applied Mathematics
- Biomathematics and Statistics
- History of Mathematics
- Mathematical Education
- Mathematical Finance
- Mathematical Analysis
- Numerical and Computational Mathematics
- Probability and Statistics
- Pure Mathematics
- Browse content in Neuroscience
- Cognition and Behavioural Neuroscience
- Development of the Nervous System
- Disorders of the Nervous System
- History of Neuroscience
- Invertebrate Neurobiology
- Molecular and Cellular Systems
- Neuroendocrinology and Autonomic Nervous System
- Neuroscientific Techniques
- Sensory and Motor Systems
- Browse content in Physics
- Astronomy and Astrophysics
- Atomic, Molecular, and Optical Physics
- Biological and Medical Physics
- Classical Mechanics
- Computational Physics
- Condensed Matter Physics
- Electromagnetism, Optics, and Acoustics
- History of Physics
- Mathematical and Statistical Physics
- Measurement Science
- Nuclear Physics
- Particles and Fields
- Plasma Physics
- Quantum Physics
- Relativity and Gravitation
- Semiconductor and Mesoscopic Physics
- Browse content in Psychology
- Affective Sciences
- Clinical Psychology
- Cognitive Neuroscience
- Cognitive Psychology
- Criminal and Forensic Psychology
- Developmental Psychology
- Educational Psychology
- Evolutionary Psychology
- Health Psychology
- History and Systems in Psychology
- Music Psychology
- Neuropsychology
- Organizational Psychology
- Psychological Assessment and Testing
- Psychology of Human-Technology Interaction
- Psychology Professional Development and Training
- Research Methods in Psychology
- Social Psychology
- Browse content in Social Sciences
- Browse content in Anthropology
- Anthropology of Religion
- Human Evolution
- Medical Anthropology
- Physical Anthropology
- Regional Anthropology
- Social and Cultural Anthropology
- Theory and Practice of Anthropology
- Browse content in Business and Management
- Business History
- Business Strategy
- Business Ethics
- Business and Government
- Business and Technology
- Business and the Environment
- Comparative Management
- Corporate Governance
- Corporate Social Responsibility
- Entrepreneurship
- Health Management
- Human Resource Management
- Industrial and Employment Relations
- Industry Studies
- Information and Communication Technologies
- International Business
- Knowledge Management
- Management and Management Techniques
- Operations Management
- Organizational Theory and Behaviour
- Pensions and Pension Management
- Public and Nonprofit Management
- Strategic Management
- Supply Chain Management
- Browse content in Criminology and Criminal Justice
- Criminal Justice
- Criminology
- Forms of Crime
- International and Comparative Criminology
- Youth Violence and Juvenile Justice
- Development Studies
- Browse content in Economics
- Agricultural, Environmental, and Natural Resource Economics
- Asian Economics
- Behavioural Finance
- Behavioural Economics and Neuroeconomics
- Econometrics and Mathematical Economics
- Economic Methodology
- Economic Systems
- Economic History
- Economic Development and Growth
- Financial Markets
- Financial Institutions and Services
- General Economics and Teaching
- Health, Education, and Welfare
- History of Economic Thought
- International Economics
- Labour and Demographic Economics
- Law and Economics
- Macroeconomics and Monetary Economics
- Microeconomics
- Public Economics
- Urban, Rural, and Regional Economics
- Welfare Economics
- Browse content in Education
- Adult Education and Continuous Learning
- Care and Counselling of Students
- Early Childhood and Elementary Education
- Educational Equipment and Technology
- Educational Strategies and Policy
- Higher and Further Education
- Organization and Management of Education
- Philosophy and Theory of Education
- Schools Studies
- Secondary Education
- Teaching of a Specific Subject
- Teaching of Specific Groups and Special Educational Needs
- Teaching Skills and Techniques
- Browse content in Environment
- Applied Ecology (Social Science)
- Climate Change
- Conservation of the Environment (Social Science)
- Environmentalist Thought and Ideology (Social Science)
- Natural Disasters (Environment)
- Social Impact of Environmental Issues (Social Science)
- Browse content in Human Geography
- Cultural Geography
- Economic Geography
- Political Geography
- Browse content in Interdisciplinary Studies
- Communication Studies
- Museums, Libraries, and Information Sciences
- Browse content in Politics
- African Politics
- Asian Politics
- Chinese Politics
- Comparative Politics
- Conflict Politics
- Elections and Electoral Studies
- Environmental Politics
- European Union
- Foreign Policy
- Gender and Politics
- Human Rights and Politics
- Indian Politics
- International Relations
- International Organization (Politics)
- International Political Economy
- Irish Politics
- Latin American Politics
- Middle Eastern Politics
- Political Theory
- Political Methodology
- Political Communication
- Political Philosophy
- Political Sociology
- Political Behaviour
- Political Economy
- Political Institutions
- Politics and Law
- Public Administration
- Public Policy
- Quantitative Political Methodology
- Regional Political Studies
- Russian Politics
- Security Studies
- State and Local Government
- UK Politics
- US Politics
- Browse content in Regional and Area Studies
- African Studies
- Asian Studies
- East Asian Studies
- Japanese Studies
- Latin American Studies
- Middle Eastern Studies
- Native American Studies
- Scottish Studies
- Browse content in Research and Information
- Research Methods
- Browse content in Social Work
- Addictions and Substance Misuse
- Adoption and Fostering
- Care of the Elderly
- Child and Adolescent Social Work
- Couple and Family Social Work
- Developmental and Physical Disabilities Social Work
- Direct Practice and Clinical Social Work
- Emergency Services
- Human Behaviour and the Social Environment
- International and Global Issues in Social Work
- Mental and Behavioural Health
- Social Justice and Human Rights
- Social Policy and Advocacy
- Social Work and Crime and Justice
- Social Work Macro Practice
- Social Work Practice Settings
- Social Work Research and Evidence-based Practice
- Welfare and Benefit Systems
- Browse content in Sociology
- Childhood Studies
- Community Development
- Comparative and Historical Sociology
- Economic Sociology
- Gender and Sexuality
- Gerontology and Ageing
- Health, Illness, and Medicine
- Marriage and the Family
- Migration Studies
- Occupations, Professions, and Work
- Organizations
- Population and Demography
- Race and Ethnicity
- Social Theory
- Social Movements and Social Change
- Social Research and Statistics
- Social Stratification, Inequality, and Mobility
- Sociology of Religion
- Sociology of Education
- Sport and Leisure
- Urban and Rural Studies
- Browse content in Warfare and Defence
- Defence Strategy, Planning, and Research
- Land Forces and Warfare
- Military Administration
- Military Life and Institutions
- Naval Forces and Warfare
- Other Warfare and Defence Issues
- Peace Studies and Conflict Resolution
- Weapons and Equipment

- < Previous chapter
15 How Uncertainty Makes Science Advance
- Published: October 2019
- Cite Icon Cite
- Permissions Icon Permissions
Rather than being a detriment to science, uncertainty actually helps it advance. And understanding the role that uncertainty plays in science is extremely helpful for avoiding four serious dangers. First, appreciating uncertainty in science helps one to avoid being dogmatically certain. Good science is always open to revision in the face of new evidence. Second, appreciating uncertainty in science allows one to avoid the mistaken view that all theories and ideas are equally plausible. Third, since uncertainty is an inherent feature of science, appreciating its role helps one better understand science. Fourth, with a proper appreciation of uncertainty in science, it is much harder for one to be deceived about things like the link between tobacco smoke and cancer or the reality of climate change. In the end, uncertainty in science helps us to make the best decisions we can while encouraging us to continually seek new discoveries.
Signed in as
Institutional accounts.
- Google Scholar Indexing
- GoogleCrawler [DO NOT DELETE]
Personal account
- Sign in with email/username & password
- Get email alerts
- Save searches
- Purchase content
- Activate your purchase/trial code
Institutional access
- Sign in with a library card Sign in with username/password Recommend to your librarian
- Institutional account management
- Get help with access
Access to content on Oxford Academic is often provided through institutional subscriptions and purchases. If you are a member of an institution with an active account, you may be able to access content in one of the following ways:
IP based access
Typically, access is provided across an institutional network to a range of IP addresses. This authentication occurs automatically, and it is not possible to sign out of an IP authenticated account.
Sign in through your institution
Choose this option to get remote access when outside your institution. Shibboleth/Open Athens technology is used to provide single sign-on between your institution’s website and Oxford Academic.
- Click Sign in through your institution.
- Select your institution from the list provided, which will take you to your institution's website to sign in.
- When on the institution site, please use the credentials provided by your institution. Do not use an Oxford Academic personal account.
- Following successful sign in, you will be returned to Oxford Academic.
If your institution is not listed or you cannot sign in to your institution’s website, please contact your librarian or administrator.
Sign in with a library card
Enter your library card number to sign in. If you cannot sign in, please contact your librarian.
Society Members
Society member access to a journal is achieved in one of the following ways:
Sign in through society site
Many societies offer single sign-on between the society website and Oxford Academic. If you see ‘Sign in through society site’ in the sign in pane within a journal:
- Click Sign in through society site.
- When on the society site, please use the credentials provided by that society. Do not use an Oxford Academic personal account.
If you do not have a society account or have forgotten your username or password, please contact your society.
Sign in using a personal account
Some societies use Oxford Academic personal accounts to provide access to their members. See below.
A personal account can be used to get email alerts, save searches, purchase content, and activate subscriptions.
Some societies use Oxford Academic personal accounts to provide access to their members.
Viewing your signed in accounts
Click the account icon in the top right to:
- View your signed in personal account and access account management features.
- View the institutional accounts that are providing access.
Signed in but can't access content
Oxford Academic is home to a wide variety of products. The institutional subscription may not cover the content that you are trying to access. If you believe you should have access to that content, please contact your librarian.
For librarians and administrators, your personal account also provides access to institutional account management. Here you will find options to view and activate subscriptions, manage institutional settings and access options, access usage statistics, and more.
Our books are available by subscription or purchase to libraries and institutions.
- About Oxford Academic
- Publish journals with us
- University press partners
- What we publish
- New features
- Open access
- Rights and permissions
- Accessibility
- Advertising
- Media enquiries
- Oxford University Press
- Oxford Languages
- University of Oxford
Oxford University Press is a department of the University of Oxford. It furthers the University's objective of excellence in research, scholarship, and education by publishing worldwide
- Copyright © 2024 Oxford University Press
- Cookie settings
- Cookie policy
- Privacy policy
- Legal notice
This Feature Is Available To Subscribers Only
Sign In or Create an Account
This PDF is available to Subscribers Only
For full access to this pdf, sign in to an existing account, or purchase an annual subscription.
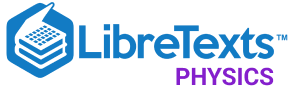
- school Campus Bookshelves
- menu_book Bookshelves
- perm_media Learning Objects
- login Login
- how_to_reg Request Instructor Account
- hub Instructor Commons
- Download Page (PDF)
- Download Full Book (PDF)
- Periodic Table
- Physics Constants
- Scientific Calculator
- Reference & Cite
- Tools expand_more
- Readability
selected template will load here
This action is not available.
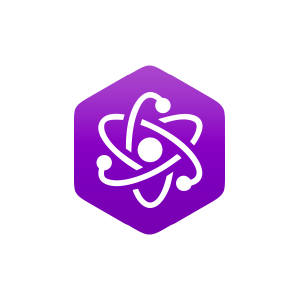
1: Uncertainty and Confirmation of Hypotheses
- Last updated
- Save as PDF
- Page ID 28040
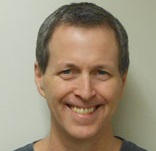
- Tom Weideman
- University of California, Davis
- 1.1: Background Material Our knowledge of position vectors allows us to define average position, and from there we define uncertainty in position.
- 1.2: Activities We practice taking and analyzing data, and learn an important nuance about hypotheses.
- IIT JEE Study Material
Heisenberg Uncertainty Principle
Heisenberg’s uncertainty principle states that it is impossible to measure or calculate exactly both the position and the momentum of an object. This principle is based on the wave-particle duality of matter. Although Heisenberg’s uncertainty principle can be ignored in the macroscopic world (the uncertainties in the position and velocity of objects with relatively large masses are negligible), it holds significant value in the quantum world. Since atoms and subatomic particles have very small masses, any increase in the accuracy of their positions will be accompanied by an increase in the uncertainty associated with their velocities.
Download Complete Chapter Notes of Structure of Atom Download Now
Table of Contents
- What Is Heisenberg’s Uncertainty Principle?
- Formula and Application
- Heisenberg Uncertainty Principle Explanation with Example
Heisenberg’s γ-ray Microscope
- Heisenberg’s Uncertainty Principle Equations
Solved Numerical Problems on Heisenberg’s Uncertainty Principle
In the field of quantum mechanics, Heisenberg’s uncertainty principle is a fundamental theory that explains why it is impossible to measure more than one quantum variable simultaneously. Another implication of the uncertainty principle is that it is impossible to accurately measure the energy of a system in some finite amount of time.
Heisenberg’s uncertainty principle imposes a restriction on the accuracy of simultaneous measurement of position and momentum. The more precise our measurement of position is, the less accurate will be our momentum measurement and vice-versa. The physical origin of Heisenberg’s uncertainty principle is with the quantum system. Determination of position by performing a measurement on the system disturbs it sufficiently to make the determination of q imprecise and vice-versa. We will learn about the principle in detail below.
What Is Heisenberg’s Uncertainty Principle?
Heisenberg’s uncertainty principle states that for particles exhibiting both particle and wave nature , it will not be possible to accurately determine both the position and velocity at the same time. The principle is named after German physicist Werner Heisenberg, who proposed the uncertainty principle in the year 1927. This principle was formulated when Heisenberg was trying to build an intuitive model of quantum physics. He discovered that there were certain fundamental factors that limited our actions in knowing certain quantities.
This principle basically highlights that simultaneous measurement of position and the velocity or momentum of microscopic matter waves will have an error such that the product of the error in measurement of position and momentum is equal or more than an integral multiple of a constant.
Origin of the Uncertainty Principle
One of the crucial points for the genesis of the uncertainty principle is solely due to the dual nature of a wave-particle. Every particle is said to have a wave nature, and the probability of finding particles is maximum, where the waveforms are the greatest. If the particle has greater undulation, the wavelength becomes more indistinct or vague. However, we are able to determine the momentum of the particle. From what we have learnt so far, we can say that the particles that have definite positions will have no fixed velocity. On the other hand, a particle with a well-defined wavelength will show a definitive or precise velocity. All in all, if we get an accurate reading of one quantity, it will only lead to large uncertainty in the measurement of the other.
Heisenberg Uncertainty Principle Formula and Application
If ∆x is the error in position measurement and ∆p is the error in the measurement of momentum, then
Since momentum, p = mv, Heisenberg’s uncertainty principle formula can be alternatively written as,
Where, ∆V is the error in the measurement of velocity, and assuming mass remains constant during the experiment,
Accurate measurement of position or momentum automatically indicates larger uncertainty (error) in the measurement of the other quantity.
Applying the Heisenberg principle to an electron in an orbit of an atom, with h = 6.626 ×10 -34 Js and m= 9.11 ×10 -31 Kg,
If the position of the electron is measured accurately to its size (10 -10 m), then the error in the measurement of its velocity will be equal to or larger than 10 6 m or 1000 km.
Heisenberg’s principle applies to only dual-natured microscopic particles and not to macroscopic particles whose wave nature is minimal.
Also Read: de Broglie Equation
Explaining Heisenberg Uncertainty Principle with an Example
Electromagnetic radiations and microscopic matter waves exhibit a dual nature of mass/momentum and wave character. The position and velocity/momentum of macroscopic matter waves can be determined accurately and simultaneously. For example, the location and speed of a moving car can be determined simultaneously with minimum error. But, in microscopic particles, it will not be possible to fix the position and measure the velocity/momentum of the particle simultaneously.
An electron in an atom has a mass of 9.91 × 10 -31 kg. Naked eyes cannot see such small particles. A powerful light may collide with the electron and illuminate it. Illumination helps in identifying and measuring the position of the electron. The collision of the powerful light source, while helping in identification, increases the momentum of the electron and makes it move away from the initial position. Thus, when fixing the position, the velocity/momentum of the particle would have changed from the original value. Hence, when the position is exact, the error occurs in measuring velocity or momentum. In the same way, the measurement of momentum, accurately, will change the position.
Hence, at any point in time, either position or momentum can only be measured accurately.
Simultaneous measurement of both of them will have an error in both position and momentum. Heisenberg quantified the error in the measurement of both position and momentum simultaneously.
A striking thought experiment illustrating the uncertainty principle is Bohr’s/Heisenberg’s Gamma-ray microscope. To observe a particle, say an electron, we shine it with the light ray of wavelength λ and collect the Compton scattered light in a microscope objective whose diameter subtends an angle θ with the electron, as shown in the figure below
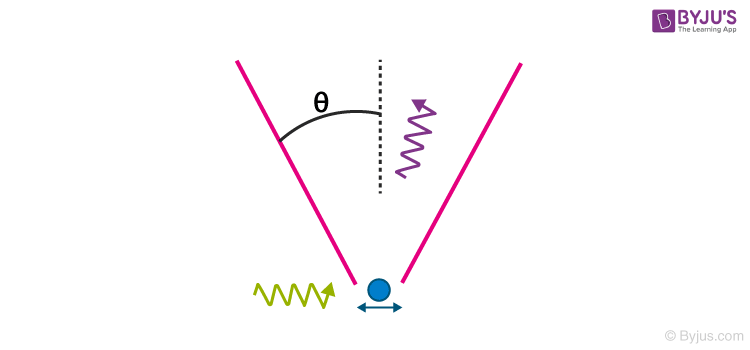
The precision with which the electron can be located, Delta x, is defined by the resolving power of the microscope,
It appears that by making λ small, we choose γ-ray, and by making sin θ large, Delta x can be made as small as desired. But, according to the uncertainty principle, we can do so only at the expense of our knowledge of the x-component of electron momentum.
In order to record the Compton scattered photon by the microscope, the photon must stay in the cone of angle θ, and hence its x-component of the momentum can vary within ±(h/λ) sin θ. This implies that the magnitude of the recoil momentum of the electron is uncertain by
The product of the uncertainty yields,
Is Heisenberg’s Uncertainty Principle Noticeable in All Matter Waves?
Heisenberg’s principle is applicable to all matter waves. The measurement error of any two conjugate properties, whose dimensions happen to be joule sec, like position-momentum, the time-energy will be guided by Heisenberg’s value.
But, it will be noticeable and of significance only for small particles like an electron with very low mass. A bigger particle with a heavy mass will show the error to be very small and negligible.
Heisenberg Uncertainty Principle Equations
Heisenberg’s uncertainty principle can be considered as a very precise mathematical statement that describes the nature of quantum systems. As such, we often consider two common equations related to the uncertainty principle, and they are as follows:
Equation 1: ∆X ⋅ ∆p ~ ħ
Equation 2: ∆E ⋅ ∆t ~ ħ
ħ = value of Planck’s constant divided by 2*pi ∆X = uncertainty in the position ∆p = uncertainty in momentum ∆E = uncertainty in the energy ∆t = uncertainty in time measurement
1. If the position of the electron is measured within an accuracy of + 0.002 nm, calculate the uncertainty in the momentum of the electron. Suppose the momentum of the electron is h / 4p m × 0.05 nm. Is there any problem in defining this value?
a) ∆x = 2×10 -12 m; \(\begin{array}{l}\Delta X \times \Delta mV \ge\frac{h}{4\pi } = \frac{6.626\times {{10}^{-34}}}{4\times 3.14}\end{array} \) \(\begin{array}{l}\because \Delta mV \ge \frac{h}{4\pi \Delta x} \ge \frac{6.626\times {{10}^{-34}}}{4\times 3.14\times 2\times {{10}^{-12}}}\,\end{array} \) = 2.64 × 10 -23 Kg m s -1
b) Momentum \(\begin{array}{l}mv = \frac{h\times 5\times {{10}^{-11}}}{4\times {{10}^{-12}}}=\frac{6.626\times {{10}^{-34}}\times 5\times {{10}^{-11}}}{4\times {{10}^{-12}}}\,\,\end{array} \) = 28 × 10 -33
Error in momentum measurement is 10 10 times larger than the actual momentum. The given momentum will not be acceptable.
2. Position of a chloride ion on a material can be determined to a maximum error of 1μm. If the mass of the chloride ion is 5.86 × 10 -26 kg, what will be the error in its velocity measurement?
∆x = 10 -6 m; \(\begin{array}{l}\Delta X \times \Delta mV \ge\frac{h}{4\pi }= \frac{6.626\times {{10}^{-34}}}{4\times 3.14}\end{array} \) = 5.28×10 -35 Js
3. The lifetime of an excited state of an atom is 3 × 10 -3 s. What is the minimum uncertainty in its energy in eV?
Time and energy are conjugate pairs with the Js unit. The product of measurement error is given by Heisenberg’s principle.
Assuming a maximum error in the measurement of lifetime equal to that of lifetime = 3 ×10 -3 s
⸪ 1 Joule = 6.242 × 10 18 ev,
= 1.1 × 10 -13
4. A wet ball weighing 10.1gm has a water of 0.1 g on it. The ball is moving with a constant velocity with an uncertainty of momentum of 10 -6 kg m/s. What will be the uncertainty in the measurement of the position of the ball, water and electron in the water molecule?
Velocity being constant, uncertainty in the measurement of the momentum is associated with the mass of the matter.
Uncertainty in the momentum of the dry ball = mass ×10 -6 = 10×10 -3 ×10 -6 Kg m s -1 .
Uncertainty in the momentum of the water = mass ×10 -6 = 0.1×10 -3 ×10 -6 Kg m s -1 .
Uncertainty in the momentum of the electron = mass ×10 -6 = 9×10 -31 ×10 -6 Kg m s -1 .
Uncertainty in position measurement is inversely proportional to the uncertainty in momentum.
= 10 8 : 10 10 : 1.1 × 10 36 or
= 1 : 10 2 : 10 28
5. Determine the minimum uncertainties in the positions of the electron if their speeds are known with a precision of 3 .0 × 10 −3 m/s.
Δ u = 3 .0 × 10 −3 m/s
Uncertained momentum Δ p = m Δ u
Uncertainty in position Δ x = ℏ / ( 2 Δ p )
For electron
Δ p = m Δ u
= ( 9.1 × 10 −3 1 kg × 3 .0 × 10 −3 )
Δ p = 2.73 × 10 -33 kg.m/s
Δx = 0.12 m
Frequently Asked Questions on Heisenberg’s Uncertainty Principle
Who was the first to come up with the idea of finding an electron in an orbital.
Heisenberg was the first one to think of or develop this idea.
Is it possible to determine that the electron is located at a certain exact location with 100% accuracy at a fixed time through measurement?
No, we cannot determine.
Which scientists proposed the idea of the uncertainty principle and the concept of the wave nature of matter?
It was Heisenberg and de Broglie.
What violates the Heisenberg uncertainty principle?
If an object travelling through spacetime can loop back in time in a certain way, then its trajectory can allow a pair of its components to be measured with perfect accuracy, violating Heisenberg’s uncertainty principle.
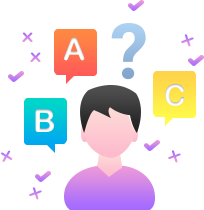
Put your understanding of this concept to test by answering a few MCQs. Click ‘Start Quiz’ to begin!
Select the correct answer and click on the “Finish” button Check your score and answers at the end of the quiz
Visit BYJU’S for all JEE related queries and study materials
Your result is as below
Request OTP on Voice Call
Leave a Comment Cancel reply
Your Mobile number and Email id will not be published. Required fields are marked *
Post My Comment

- Share Share
Register with Aakash BYJU'S & Download Free PDFs
Register with byju's & watch live videos.

An official website of the United States government
The .gov means it’s official. Federal government websites often end in .gov or .mil. Before sharing sensitive information, make sure you’re on a federal government site.
The site is secure. The https:// ensures that you are connecting to the official website and that any information you provide is encrypted and transmitted securely.
- Publications
- Account settings
Preview improvements coming to the PMC website in October 2024. Learn More or Try it out now .
- Advanced Search
- Journal List
- Front Psychol
The Relationship Between Uncertainty and Affect
Eric c. anderson.
1 Center for Outcomes Research and Evaluation, Maine Medical Center Research Institute, Portland, ME, United States
2 Department of Medicine, Tufts University Medical Center, Boston, MA, United States
R. Nicholas Carleton
3 Department of Psychology, University of Regina, Regina, SK, Canada
Michael Diefenbach
4 Departments of Medicine, Urology, and Psychiatry, Northwell Health, New York, NY, United States
Paul K. J. Han
Uncertainty and affect are fundamental and interrelated aspects of the human condition. Uncertainty is often associated with negative affect, but in some circumstances, it is associated with positive affect. In this article, we review different explanations for the varying relationship between uncertainty and affect. We identify “mental simulation” as a key process that links uncertainty to affective states. We suggest that people have a propensity to simulate negative outcomes, which result in a propensity toward negative affective responses to uncertainty. We also propose the existence of several important moderators of this process, including context and individual differences such as uncertainty tolerance, as well as emotion regulation strategies. Finally, we highlight important knowledge gaps and promising areas for future research, both empirical and conceptual, to further elucidate the relationship between uncertainty and affect.
Introduction
Uncertainty and affective feelings are both fundamental aspects of human life. People are uncertain about the weather, how long they will live, and how other human beings will act in a given situation. People experience affective feelings (e.g., anger, anxiety, and pleasure) related to traffic, medical diagnoses, and social interactions. Uncertainty and affect also appear to be closely linked to each other. People typically find uncertainty to be aversive ( Carleton, 2016b ) and are willing to pay to reduce uncertainty ( Lovallo and Kahneman, 2000 ); however, in some circumstances, people seem to find uncertainty attractive and seek out uncertainty-inducing activities—e.g., reading mystery novels ( Zillmann, 1996 ), watching sports ( Knobloch-Westerwick et al., 2009 ), or gambling. In fact, removing uncertainty from these activities seems to reduce enjoyment (e.g., movie or story spoilers). The reasons for these differences in people’s affective responses to uncertainty, however, are not well understood. Investigators from various psychological disciplines have offered some explanations; however, there is not a single, widely accepted, unifying theory accounting for the relationship between uncertainty and affect.
In this article, we explore this relationship further by reviewing important insights from existing theoretical accounts. Our objective is not to conduct a systematic review of all existing theories with potential relevance to this topic, and but rather to explore some of the more prominent theories that have focused explicitly on the experience of uncertainty, affect, and emotion. Our overarching aim is to synthesize common themes and ideas raised by these theories, and to identify potential mechanisms that might link uncertainty and affect. We acknowledge the existence of promising theoretical and empirical work in related fields—e.g., computational neuroscience—but leave the task of integrating this work for future analyses. We will show that existing theories of uncertainty, affect, and emotion suggest the importance of the psychological process of “mental simulation” as a key mediating factor in their relationship, and suggest potentially fruitful directions for future research to advance our understanding of these phenomena.
The Nature of Uncertainty
An essential initial task of any analysis such as this is to establish a useful working definition of the term “uncertainty.” Despite the large volume of scholarship on uncertainty by psychologists and other social scientists, this term has often been either not explicitly defined or else defined in varying and often inconsistent ways. The Merriam-Webster dictionary defines uncertainty as “the state of being uncertain” and uses a plethora of terms to describe what it means to be uncertain: indefinite, indeterminate, not certain to occur, problematical, not reliable, untrustworthy, not known beyond doubt, dubious, doubtful, not clearly identified or defined, not constant, variable, and fitful.
The Merriam-Webster dictionary definition highlights an important area of agreement on the nature of uncertainty—the notion that uncertainty is fundamentally a mental state, a subjective, cognitive experience of human beings rather than a feature of the objective, material world. The specific focus of this experience, furthermore, is ignorance—i.e., the lack of knowledge. Importantly, uncertainty is not equivalent to mere ignorance; rather, uncertainty is the conscious awareness, or subjective experience of ignorance. It is a higher-order metacognition representing a particular kind of explicit knowledge — an acknowledgment of what one does not know, but also that one does not know. Smithson (1989) has used the term “meta-ignorance” to describe this state of knowing that one is ignorant, but we believe the term “uncertainty” better distinguishes this higher-order state from plain, unconscious ignorance (the state of not knowing that one is ignorant).
We believe the distinction between uncertainty and ignorance is critical to the phenomenology of uncertainty. Unless a person has some awareness of their ignorance, it is unlikely to influence their thoughts, feelings, or actions. We acknowledge that there are varying levels of conscious awareness, however, and that the awareness of ignorance may occur at a preconscious or unconscious level. For example, perceptual awareness of numerous other kinds of stimuli exists without higher-level conscious awareness; individuals constantly register and form inferences from perceptual data through unconscious, automatic processes. Cognitive scientist Andy Clark has argued that perceptual uncertainty is largely reduced by unconscious automatic processes, and that human beings can be characterized as being engaged in a continuous act of “surfing uncertainty” ( Clark, 2015 ). In the same vein, dual-process theories of cognition distinguish unconscious, automatic “System 1” processes, from conscious, deliberate “System 2” processes, and a large body of empirical evidence has demonstrated that System 1 processes exert significant influence on judgment and decision making ( Gigerenzer and Goldstein, 1996 ; Greene et al., 2001 ; Masicampo and Baumeister, 2008 ; Kahneman, 2011 ; Rand et al., 2012 ).
One can thus argue that uncertainty can exist below full conscious awareness. However, the problem then becomes one of defining what full conscious awareness means, and what minimal level of consciousness of ignorance is necessary for uncertainty to exist as an experientially and psychologically consequential state. We cannot resolve this problem here, but simply contend that the conceptual boundaries separating conscious and unconscious awareness are fuzzy and debatable (see later section on “Conceptual Issues”), and exactly where the boundaries should be set depends on one’s objectives. Our primary objective is to understand the relationship between uncertainty and affect as conscious, consequential experiences—i.e., as states that are fully manifest in one’s awareness. This interest is largely driven by our practical concern with understanding of what uncertainties people identify as being problematic in their lives, how these uncertainties influence medical decision making and mental health ( Han et al., 2011 ), and what strategies people use to tolerate uncertainty ( Han, 2013 ; Hillen et al., 2017 ; Strout et al., 2018 ). For these reasons, we focus on uncertainties that lie squarely within people’s conscious awareness, although we acknowledge that some uncertainties do not.
Researchers have distinguished three different sources of uncertainty ( Han et al., 2011 ). The first source of uncertainty, probability (also commonly referred to as risk), arises from the randomness or indeterminacy of the future. The second source, ambiguity, arises from limitations in the reliability, credibility, or adequacy of probability (risk) information ( Ellsberg, 1961 ). The final source, complexity, arises from features of available information that make it difficult to comprehend, such as multiple possible causes or outcomes. Because our goal is to tie together broad themes relating uncertainty to affect, we do not highlight the considerable work which has focused on the individual sources of uncertainty ( Ellsberg, 1961 ; Tversky and Kahneman, 1981 ; Camerer and Weber, 1992 ; Rabin and Thaler, 2001 ; Siegrist et al., 2005 ; Zhang et al., 2014 ; Duttle and Inukai, 2015 ; Kovářík et al., 2016 ).
The Nature of Affect and Emotions
Another essential initial task of our analysis is to establish useful working definitions of “affect” and “emotions.” Both terms signify distinct mental states that are discrete and also distinguishable from moods. Moods, such as depression or mania, are thought to represent more diffuse states that are longer in duration and not necessarily caused by one particular stimulus or event. In this article, we will primarily use the term “affect” because it is inclusive enough to include the broad range of findings described here, but the more specific term “emotion” when discussing research that focuses on those discrete states.
Affect has often been used as an umbrella term that signifies feelings of pleasure or discomfort, arousal, stress, emotion, and mood. Used in a more specific way, however, affect is thought to represent a feature of mental states comprised of two dimensions: valence, which ranges from pleasant to unpleasant; and arousal, which ranges from activated to deactivated ( Russell and Barrett, 1999 ; Russell, 2003 ; Barrett, 2006 ). Thought theorists typically posit pleasant (positive affect) and unpleasant (negative affect) are poles on a single scale, others argue they are distinct processes that can be disassociated ( Cacioppo et al., 2012 ). At any given moment, a person’s affective state can be described in terms of some combination of valence and arousal, and these feelings are thought to be an important component of a unified conscious experience ( Wundt, 1998 ; Barrett and Bliss-Moreau, 2009 ).
Emotions, as distinguished from affect, are mental states whose essential nature has been an ongoing focus of lively debate ( Barrett et al., 2007a ). Discrete emotions are particular psychological states like disgust, guilt, or happiness. These emotional states have affective features: fear and anger are both typically unpleasant, high-arousal states, but the subjective experiences of fear and anger are not the same, and they have different causes and consequences. Importantly, the cause of an emotional response could be a physical stimulus, like seeing a bear on the trail ahead. Alternatively, however, the stimulus could also be a self-generated mental state, such as imagining being chased, or remembering being chased by a bear.
The Relationship Between Uncertainty and Affect: General Theories of Affect and Emotion
Having offered working definitions of both uncertainty and affect, we now turn to the central question of interest of the current analysis: the relationship between these two mental states. Foundational theories of affect and emotion offer some insights on the psychological mechanisms connecting uncertainty and affect.
The basic “Modal Model” of emotions ( Barrett et al., 2007b ; Gross, 2014 ) is a widely accepted theory that offers a simplified, but useful, commonsense starting point for thinking about the process by which emotions are generated. The “modal model” suggests that emotions are generated by (1) a situation, that is (2) attended to, and then (3) appraised, which creates (4) an emotional response ( Gross, 2014 ). Emotions are typically thought to represent a coordinated yet flexible multisystem response including changes to the autonomic nervous system, facial expressions, non-verbal behaviors, actions, and subjective feelings. These multisystem responses can then lead persons to change their focus of attention and to modify the situation, which creates a new cycle of emotion generation. For example, when Jill is walking alone at night (1), she might spot and attend to a shadowy alley (2). When approaching the alley, Jill might surveil the alley to look for evidence of threat. If a suspicious figure is spotted and appraised as a potential threat (3), Jill’s heart rate might quicken, muscles tense, and she might detour around the alley and have the subjective experience of feeling afraid (4). The action of detouring might lead to meeting an old friend, which would then trigger a new emotion generation process. On the other hand, the same situation might be experienced very differently if some contextual features are different. For instance, if Jill is looking for a secret nightclub (1), she might attend to an alley looking for signs of the club (2). Someone spotted in the alley might be appraised as a potential resource—someone who could give directions (3). This might lead to Jill’s muscles relaxing, approaching to ask for directions, and the subjective experience of feeling relief or excitement (4).
Appraisal theories of emotion are another important class of theories that specify a direct relationship between uncertainty and emotions (for a summary, see Moors et al., 2013 ). At their core, appraisal theories hold that emotions are adaptive processes that reflect appraisals of features of the environment that are significant for the organism’s survival and well-being. While appraisals can be conscious, rule-based processes, they are more often automatic, associations that match patterns in the environment to appraisals. Important appraisal variables include goal relevance, goal congruence, coping potential, and agency. Uncertainty vs. certainty about goals and outcomes is another appraisal dimension proposed by many appraisal theorists (see Moors et al., 2013 ). Therefore, the appraised uncertainty of a situation is fundamentally linked to the experience of different emotions. For example, the emotion “sadness” might be associated with certainty about a negative outcome. Consider a patient first learning she has been diagnosed with cancer. If she is convinced that treatment will not control her cancer (i.e., expresses high certainty about the lack of treatment efficacy), she might experience profound sadness. However, if she appraises the situation as less certain (e.g., the cancer might not progress or treatment might be effective), she might have a different emotional response. Thus, according to appraisal theories, the perceived uncertainty vs. certainty of a situation is a fundamental determinant of what specific emotion processes are elicited in that situation ( Moors et al., 2013 ).
One strength of appraisal theories is that they are compatible with people having different responses to the same situation. If two people differ in their appraisal of a situation’s certainty, goal congruence, controllability, or other appraisals, their corresponding emotional response will also differ. Appraisal theories also generally assume that the same stimuli will not always cause same emotions because the intervening appraisals might differ. However, the same appraisals should consistently cause the same emotions ( Moors et al., 2013 ).
The ability to imagine oneself in different situations, or simulate different perspectives, is thought to help people solve problems and make decisions (for discussion see, Taylor et al., 1998 ). Current theories link thoughts about the future (prospection), remembrances of the past (memory), and understandings of the viewpoint of others (theory of mind) to a common brain network, which includes the frontal and medial temporal-parietal lobes ( Buckner and Carroll, 2007 ). People use these cognitive processes to imagine possible future events and to imagine their resulting affective responses to create “affective forecasts” ( Wilson and Gilbert, 2005 ). There are biases associated with affective forecasts ( Gilbert et al., 1998 ), but there is also evidence that people use their affective forecasts to make decisions in many life domains (e.g., medical care; Ferrer et al., 2015 ). As we will discuss later, the capacity to simulate different outcomes might be a key link between uncertainty and affect.
Emotion Regulation
Affect and emotions can be controlled and regulated using various strategies. According to the process model (for review see, Gross, 2014 ), emotion regulation strategies can be usefully mapped onto different time points corresponding to different regulatory opportunities: situation selection, situation modification, attentional deployment, cognitive change, and response modulation (see Figure 1 ). For example, even before an emotion is generated, people can sometimes select the situation they are in (e.g., watching a comedy vs. horror movie). Next, people can modify the situation they are in (e.g., during a scary scene, turn the lights on, or mute the sound). Next, people can control what stimulus they attend to in the situation (e.g., closing eyes). People can also use cognitive processes to change how they think about the situation or their ability to meet the challenges posed by the situation (e.g., reappraising the stimulus as a movie—not as reality). Finally, people can attempt to modify their responses to the emotional episode (e.g., forcing a smile or taking deep breaths). These different regulation strategies align with the different components of emotion generation described above in the modal model of emotions.

The process model of emotion regulation, from Gross (2014) . Reprinted with permission of Guilford Press.
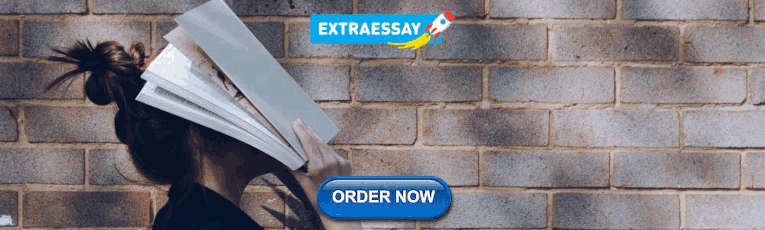
Affect and Emotion Drive Action
Most contemporary theories construe affect and emotions as adaptive processes that serve the key function of preparing the body for action. Specifically, affective responses prepare the body for possible actions using predictions about what physiological resources will be needed (i.e., allostasis; Sterling, 2012 ). This is important because in uncertain situations the affective response will drive physiological responses based on predictions. For instance, at the sight of a bear, the brain makes a quick prediction that fighting or fleeing might be needed, which triggers increased heart rate as the body prepares for action. This example points to a common theme: affect and emotions are theorized to represent fast and frugal ways of representing the world and making decisions quickly—often in uncertain, complex situations where conscious, deliberative reasoning is impractical (see the evaluative space model as one example; Cacioppo et al., 2012 ).
Several theories construe affect as a type of information processing and cognition ( Duncan and Barrett, 2007 ). For example, the affect-as-information theory ( Schwarz and Clore, 1983 ) suggests people can use their own transient affective states as information to make judgments. In similar fashion, the affect heuristic theory ( Slovic et al., 2007 ) suggests that mental representations of objects, events, and options are associated with affective tags. When making a decision, people consider the pool of affectively tagged information, which provides a quick gist impression of the options. Similarly, the somatic marker hypothesis ( Damasio, 1994 , 1996 ) suggests that changes in the visceral states of the body (heart rate, blood pressure, gut, and nervous system activity) act as affective signals that help guide people to adaptive action ( Reimann and Bechara, 2010 ).
Classic research has shown that affect can change perceptions of the probability, or risk, of uncertain events. For instance, reading a newspaper story about the tragic death of a young person leads participants to overestimate the probability of future negative events (e.g., floods, accidents, or diseases) by 74% ( Johnson and Tversky, 1983 ). Specific emotions can also influence explicit likelihood estimates in an emotion-congruent manner: participants induced to feel sadness perceived a greater likelihood of future sad events but not anger-inducing events ( DeSteno et al., 2000 ). Additionally, fearful people make pessimistic risk assessments, while angry people make optimistic risk assessments ( Lerner and Keltner, 2001 ). The risk-as-feelings theory suggests that people use feelings that contain information about risk when making decisions (as opposed to computing the normative “expected utility” of different options; Loewenstein et al., 2001 ). The authors suggest that uncertainty is represented within two systems: a cognitive system and an emotional system that can agree of conflict with each other (similar to other dual-process theories mentioned above).
In sum, contemporary theories suggest that affect and emotions typically serve an adaptive function of guiding attention, cognition, and action. When not beneficial, affect and emotions can be modified by different regulation strategies ( Gross, 2014 ). While there are a number of theories about the nature of affect and emotion, appraisal theories are particularly relevant for this discussion because they posit that emotions are directly shaped by the perceived certainty of a situation (in addition to other appraisal dimensions).
The Relationship Between Uncertainty and Affect: Specific Theoretical Accounts
Having established working definitions of uncertainty and affect and surveyed general theories of affect and emotion for key insights about the relationship between these phenomena, we now analyze theoretical accounts that more specifically and explicitly address the influence of uncertainty on affect. Most of these theories describe uncertainty as aversive, and most focus on the relationship between uncertainty and negative affect or emotional states. An exhaustive review of the psychological empirical literature is beyond the scope of the current analysis, and such reviews can be found elsewhere ( Carleton, 2016b ).
It is also important to note that although we focus here on the psychological literature, researchers in other domains have also studied affect and uncertainty using different methods and from different theoretical perspectives. For example, computational neuroscientists have used modeling techniques have been used to assess psychological, behavioral, and neuropsychological outcomes under conditions of reward and indeterminacy (e.g., Lowe and Ziemke, 2013 ; Lowe et al., 2017 ; Babayan et al., 2018 ; Starkweather et al., 2018 ). Like past work in other areas, this research has also tended to use variety definitions for affect and uncertainty. Furthermore, to our knowledge this work has not explicitly attempted to analyze the relationship between affect and uncertainty, although we believe it may offer useful insights for further empirical and theoretical work.
Behavioral Inhibition System Theory
The influential theory of behavioral inhibition was largely motivated by rodent learning studies and neurophysiological evidence, though it has been extended to anxiety disorders in humans ( Gray, 1976 ; Gray and McNaughton, 2000 ). This theory specifically posits that novel stimuli, unexpected events, or conflicts between competing behavioral options can all activate the BIS neurological system. The behavioral inhibition system (BIS) is responsible for effectively guiding behavior in these novel or unexpected situations. The BIS provides guidance by suppressing behavior, increasing attention to novel features, and heightening the organism’s arousal which allow the organism to act in an adaptive manner. At the neural level, BIS activation is identified as a 7.7-Hz hippocampal theta response, driven by activity in the septal area.
In Gray and McNaughton’s view, activation of the BIS is tightly coupled to anxiety. They state: “we identify anxiety with activity in the behavioral inhibition system” ( Gray and McNaughton, 2000 ; p. 84). That is, they stipulate that when the BIS activates, the animal or human experiences anxiety. According to this theory, indeterminacy related to novelty or unexpected events activate the behavioral inhibition system, which causes anxiety. The same indeterminacy also likely causes the subjective experience of uncertainty in humans, though it is less clear of what rodents experience. One interpretation of this account is that anxiety is the subjective experience of indeterminacy–just like uncertainty is the awareness of ignorance. The exact link between experienced uncertainty and anxiety is not spelled out, beyond that they are often generated by the very same situations.
One interesting implication of the BIS theory is that the conflict between two positive options would result in BIS activation (e.g., “Should I eat delicious food A or delicious food B”?; Iyengar and Lepper, 2000 ). Thus, uncertainty could arise in situations that are generally positive. However, the BIS model would still suggest that anxiety would be the affective state produced by multiple appealing options.
Uncertainty and Anticipation Model of Anxiety
Building on the BIS model outlined above, the Uncertainty and Anticipation Model of Anxiety (UAMA) theory of uncertainty and anxiety incorporates recent human neuroimaging research ( Grupe and Nitschke, 2013 ). The UAMA model proposes that clinical anxiety disorders are due to heightened expectancies about the probability and cost of future threats. UAMA attributes these effects to changes in five key psychological processes related to uncertainty: (1) changes in the calculation of expected value and aversive prediction error signaling; (2) hypervigilance and increased attention to possible threats; (3) deficient safety learning or an inability to regulate responding in safe situations; (4) subsequently increased cognitive and behavioral avoidance of situations or evidence that contradict negative predictions about the future (which allow the negative predictions to persist); and (5) exaggerated physiological and behavioral reactivity under uncertainty, leading to further avoidance of situations perceived as uncertain. UAMA focuses on clinical anxiety ( Grupe and Nitschke, 2013 ), but the psychological systems are present in healthy populations; therefore, this model could be useful in understanding the link between uncertainty and affect more broadly. However, the link between uncertainty and affect is not the sole focus of the model, and the authors do not explicitly address why uncertainty causes anxiety.
Entropy Model of Uncertainty
The Entropy Model of Uncertainty (EMU) model by Hirsh et al. (2012) utilizes the concept of entropy from thermodynamics and information theory to explain the nature and psychological effects of uncertainty. In this model, psychological entropy reflects the amount of uncertainty (i.e., entropy) in a system. This psychological entropy applies to uncertainty about either a perception (“what is that”?) or an action (“what is the right action”?). The authors proposes four tenets in the EMU model: (1) in general, uncertainty is a critical adaptive challenge for organisms, and thus managing uncertainty is important; (2) uncertainty creates conflicts between competing perceptual and behavioral affordances; (3) concrete goals and belief structures can reduce the experience of uncertainty by reducing the set of possible perceptions and actions; and (4) uncertainty is experienced subjectively as anxiety because uncertainty reflects the inability to perceive the world or know which action to take—two evolutionarily fundamental tasks. This theory, however, does not specify exactly why or how uncertainty is associated with anxiety (negative affect) beyond this evolutionary argument. It is descriptive rather than a causal theory that simply characterizes the association between uncertainty and anxiety.
Theory of Personal Uncertainty
Personal uncertainty has been described as the aversive feeling that is experienced when one is uncertain about oneself or one’s worldviews ( van den Bos, 2009 ). A central premise is that humans engage in a fundamental process of “sense-making” to understand their lives. Personal uncertainty challenges this “sense-making” process and the meaning people attribute to their lives. Personal uncertainty is experienced as negative and these negative feelings then motivate people to manage their uncertainty (for review see, van den Bos, 2009 ). One way to manage personal uncertainty is by adhering to cultural values and norms more strongly (e.g., belief in a just world; Lerner, 1980 ), a strategy that has been demonstrated in empirical studies ( van den Bos et al., 2005 ). After being primed to think about their own personal uncertainty, people become more rigid and closed-minded ( McGregor et al., 2001 ). Additionally, van den Bos (2009) argues that uncertainty may explain some of the effects traditionally attributed to terror management theory ( Greenberg et al., 1997 ). For instance, contemplating death might lead to uncertainty about what will happen after death. van den Bos (2009) contends that although mortality salience may account for various defensive reactions when people are confronted by the threat of mortality, these reactions are also driven by the personal uncertainty that the threat of mortality raises ( van den Bos, 2009 ).
The reactive approach motivation (RAM) theory ( McGregor et al., 2009 ) is compatible with the above work on personal uncertainty and BIS theory ( Gray and McNaughton, 2000 ). In addition to personal uncertainty, the model suggests that anxious uncertainty occurs when a person (or other animal) is caught between conflicting approach and avoidance motivations ( McGregor et al., 2010 ). For example, a hungry mouse which receives a shock when it approaches food is caught in a conflict between a motivation to approach the food and a motivation to avoid the shock. In this theory, “anxious uncertainty” is a term that directly connects affective responses (i.e., anxiety) to uncertainty. RAM theory additionally suggests that ideals function as abstract goals that can guide behavior when lower level goals or actions are blocked ( McGregor et al., 2009 ). Thus, a person can focus on ideals or worldview to help clarify what to do when experiencing anxious uncertainty.
Fear of the Unknown Theory
This theory proposes that fear of the unknown is a—and possibly the —fundamental fear of human beings ( Carleton, 2012 , 2016a ). A more complete discussion of the empirical background for this theory is available elsewhere ( Carleton, 2016a , b ), but to summarize, a large body of theoretical, logical, and experimental evidence supports the existence of a fundamental fear of the unknown that appears to be: (1) an emotion; (2) inherent; (3) logically evolutionarily supported; (4) continuously and normally distributed in the population; (5) a logical reduction of higher-order constructs; (6) logically non-derivative and irreducible; (7) able to account for variance in higher-order constructs; and (8) factorially distinct” ( Carleton, 2016b , p. 14). Carleton has further suggested that, using an iterative downward arrow approach, other fears are ultimately based on a person perceiving some piece of salient, key, or sufficient information, which ultimately causes an inherent, evolutionarily supported fear response. This suggestion was built upon a proposed contemporary definition for intolerance of uncertainty as, “an individual’s dispositional incapacity to endure the aversive response triggered by the perceived absence of salient, key, or sufficient information, and sustained by the associated perception of uncertainty” ( Carleton, 2016a , p. 31). This theoretical proposition and definition allows specific distinctions between stimuli (e.g., an unknown), automatic responses (e.g., a fear response first along the fast pathway and then along the slow pathway; Ledoux, 2000 ), and engagement with automatic responses (e.g., efforts to endure aversive elements of the automatic response). Carleton (2016a) has also suggested that efforts to predict and control events represent attempts to cope with fear of the unknown and intolerance of uncertainty, and that perceived successes at prediction and control facilitate perceptions of agency and self-efficacy, all of which can progressively reduce fear of the unknown. However, Carleton (2012) has cautioned that attempts to use prediction and control to minimize uncertainty may be less effective for reducing fear and anxiety than increasing one’s individual ability to tolerate uncertainty itself (i.e., to reduce the intensity of an individual’s fundamental fear response to unknowns).
Theoretical Gaps
We have briefly surveyed a number of psychological theories that have examined the relationship between uncertainty and affect. Some theories from the affective science literature have examined this relationship in a general, indirect manner, mentioning uncertainty incidentally or as an exemplar of stimuli that produce various affective or emotional states (e.g., appraisal theories, Moors et al., 2013 ). Other theories have examined the relationship more specifically and directly, focusing on uncertainty as a primary cause of affective reactions (e.g., van den Bos, 2009 ; Hirsh et al., 2012 ; Grupe and Nitschke, 2013 ; Carleton, 2016a ). These theories differ in how they describe this relationship and what psychological processes they focus on; however, what is more striking is their similarities. They all view uncertainty as a deficit in knowledge that is inherently aversive and results in negative affective states. In general, many theories suggest that animals and humans evolved to perceive or represent the world accurately and that feeling of uncertainty represents the failure of those systems. Uncertainty represents a possibly dangerous situation (“flying blind”) and that evolution has designed the brain to avoid that state at all costs.
In general, while the available literature offers useful insights, important knowledge gaps remain. In particular, none of the theories described here account for the possibility of uncertainty causing positive, rather than negative, affect. This is an important gap, however, given that emerging empirical evidence suggests that uncertainty can indeed be associated with positive affect, and also has more complex, indirect effects on affect. This evidence will now be briefly reviewed.
Uncertainty and Positive Affect
As described above, most past empirical and theoretical work has focused on negative or undesirable affective responses to uncertainty. Nevertheless, intuitively there are clearly life situations in which affective responses to uncertainty are positive. People watch television shows, movies, sporting events, gamble, and read mystery books in which the outcomes are uncertain—yet these activities are enjoyed. In fact, often these events would be less pleasant if the outcomes are made certain in advance (e.g., spoilers). Currently, a small set of studies provide initial empirical support for the notion that uncertainty can, at times, produce positive affect.
In one study ( Kurtz et al., 2007 ), participants were placed in conditions wherein they were uncertain about which of two gifts they would receive. Participants experiencing uncertainty maintained positive affect longer than those who knew which gift they would receive. Surprisingly, participants who were uncertain about which gift they would receive felt pleasant for a longer period of time than participants who knew they would receive both gifts. The same study also found evidence that participants did not accurately forecast how uncertainty would influence their feelings; specifically, participants predicted they would feel most pleasant if they received both gifts. When asked which situation they would prefer to be in, more participants chose the certain condition compared to the uncertain condition, even though participants in the uncertain condition actually felt pleasant longer. To explain these findings, the authors theorized that uncertainty about a pleasant event holds people’s attention, causing them to think more about the possibility of the event which extends their experience of positive affect. For example, uncertainty about which of two gifts might possibly be won leads people to imagine winning the first gift, and to further extend their processing of pleasant scenarios by then imagining winning the second gift. In this way, uncertainty may increase cognitive processing (i.e., two distinct simulations of winning, one for each gift), which then lengthens the experience of positive affect.
Uncertainty and the Intensification of Affect
Researchers have proposed and tested the “uncertainty intensification” hypothesis, wherein uncertainty during emotional events intensifies incidental affect ( Bar-Anan et al., 2009 ). According to this hypothesis, uncertainty causes negative affect to become more negative and positive affect to become more positive. This hypothesis was tested in four studies that used film clips to induce both positive and negative affect. To manipulate uncertainty, participants repeated phrases conveying certainty (“I see what’s happening”) or uncertainty (“I’m not sure what’s happening”). Participants in the uncertain condition reported more positive feelings toward positive films and more negative feelings toward negative films (as measured by an index of self-reported feelings). The authors also found that participants in the uncertain condition were more curious about the films and that curiosity mediated the relationship between uncertainty and affect. Curiosity, the authors argued, led to greater psychological engagement, which strengthened affective responses to the films and resulted in the intensification of affective states ( Bar-Anan et al., 2009 ).
Uncertainty and the Dampening of Affect
On the other hand, researchers have also found evidence that uncertainty can dampen or reduce the intensity of affective experiences ( van Dijk and Zeelenberg, 2006 ). In laboratory experiments, participants were asked to imagine themselves in scenarios in which they won various prizes: a CD, dinner for two, or one of the two prizes, but they were uncertain about which prize. Participants who were uncertain about which prize they won were found to experience less intense positive affect compared to participants in the other two conditions. In the second experiment using a similar design, participants were instructed to imagine they lost a lottery ticket that would have won different prizes: a CD, dinner for two, or one of the two prizes (but they were uncertain about which prize). Participants who were uncertain about which prize they lost experienced less intense negative affect associated with the loss. Thus, uncertainty about which prize was won or lost reduced the intensity of associated positive or negative affect—dampening the affective responses. The authors did not propose a specific mechanism for the effect, but raised the possibility that uncertainty might produce “mixed feelings that are difficult to integrate” ( van Dijk and Zeelenberg, 2006 , p. 175).
Moderators of the Effects of Uncertainty on Affect: Situational Characteristics
These findings by van Dijk and Zeelenberg (2006) seem to contradict findings from the study by Kurtz et al. (2007) , in which uncertainty about a prize led people to experience more positive affect. These seemingly discrepant results might be due to contextual factors, which may moderate the relationship between uncertainty and affect. One potential factor is ecological validity. In the Kurtz et al. (2007) study, the prizes were actually won (participants actually got to take them home), whereas in the van Dijk and Zeelenberg (2006) study, the prizes were hypothetical and participants were asked to imagine winning a prize based on a scenario. Whether the prizes were real or hypothetical may have moderated the influence of uncertainty on participants’ emotional experiences.
A moderating role of realism or ecological validity in people’s affective responses to uncertainty has been supported by both empirical evidence and theory. A study by Bar-Anan et al. (2009) found that people do not accurately forecast how uncertainty will influence their experiences of a future gain or loss. Their forecasts often depart from their experienced feelings (consistent with much work on affective forecasting; Wilson and Gilbert, 2005 ). Theories about negative affective responses to art posit that the perceived reality of a situation influences people’s responses to the situation. People enjoy negative affect in art when there is psychological distance between the event and perceiver, as in obviously contrived situations such as Greek tragedies or horror movies ( Menninghaus et al., 2017 ). Indeed, the authors argue people enjoy those forms of art precisely because they are not real. Uncertainty might function similarly: it is tolerable and even enjoyable in contrived or controlled settings where stakes are low, but intolerable and unpleasant in real-life situations with significant consequences.
Importantly, the studies described here manipulated uncertainty, but did not measure participants’ feelings of uncertainty. Accordingly, clear evidence is still lacking regarding the degree to which participants were truly uncertain in the sense of being consciously aware of their ignorance. The absence of such evidence thus allows for an alternative explanation for the discrepant results of past research—specifically, these discrepant results may result from variation in the levels of uncertainty manipulated in different studies. Lower levels of uncertainty might influence emotions in one direction (intensifying), while higher levels of uncertainty might influence emotions in the opposite direction (dampening). More research is needed to test these and other alternative explanations.
As mentioned previously, situational factors such as the realism of an uncertain stimulus may moderate the effects of uncertainty on affect. But these effects might also be moderated by numerous other situational factors and characteristics of individuals, which have not been fully characterized in past research. One potentially important moderator is the probability of a given outcome. It stands to reason that uncertain outcomes of lower probability, such as getting struck by lightning, would generate weaker affective responses than uncertain outcomes of higher probability, such as contracting the flu during a documented epidemic. Another potentially important situational moderator is the severity of a given outcome. It stands to reason that uncertainty outcomes of lower severity, such as contracting the flu, would generate weaker affective responses than uncertainty about developing cancer. However, we currently lack empirical evidence on these effects.
Characteristics of individuals may also moderate the effects of uncertainty on affect. These include sociodemographic characteristics (e.g., age, sex, and education), as well as health literacy and numeracy, and personality differences. Individuals’ life experiences (e.g., a person’s experience with cancer) will also likely shape responses. Empirical evidence on the moderating effects of these characteristics on the relationship between uncertainty and affect is also lacking, and further research is needed to explore these effects.
Moderators of the Effects of Uncertainty on Affect: Uncertainty Tolerance
A specific characteristic of individuals that has been better studied as a potential moderator of people’s responses to uncertainty is “uncertainty tolerance” (UT). This construct has been described by researchers using various overlapping, inversely-related, and logically equivalent terms (e.g., uncertainty tolerance, uncertainty intolerance, ambiguity tolerance, and ambiguity intolerance) and has also been defined in different ways, although existing definitions include several shared elements. In a recent review of the multi-disciplinary literature on UT ( Hillen et al., 2017 ), we assessed how researchers from various fields have conceptualized UT, either explicitly in published definitions of the construct, or implicitly in published measures used to assess UT. This analysis revealed that UT has been defined with respect to the presence or absence of a wide variety of cognitive, emotional, and behavioral responses to uncertainty—both negative and positive in valence, although mostly negative ( Figure 2 ). Building on previous work and definitions (see Carleton, 2016a ), we developed an integrative working definition of UT: “the set of negative and positive psychological responses—cognitive, emotional, and behavioral—provoked by the conscious awareness of ignorance about particular aspects of the world” (p. 70, Hillen et al., 2017 ).

Integrative model of uncertainty tolerance (from Hillen et al., 2017 ).
We argued that UT can be construed as either a state or a stable personality trait that predisposes individuals to specific psychological responses, but that the view of UT as a trait has dominated the literature ( Hillen et al., 2017 ). Available evidence suggests that UT differs among individuals, and UT is associated with other known personality traits including authoritarianism, dogmatism, and openness to experience. Empirical evidence also suggests that differences in UT are associated with various outcomes, including health-related outcomes ( Strout et al., 2018 ). It stands to reason that trait-level differences in UT may moderate the effects of uncertainty on affect.
Persons who report higher UT are more likely to report lower negative affect and higher life satisfaction ( Garrison et al., 2017 ), as well as higher self-esteem and creativity ( Pavlova, 2018 ). Higher UT also appears to be associated with greater risk-taking ( Kornilova et al., 2018 ), whereas lower UT and lower tolerance for ambiguity has been associated with behavioral outcomes indicative of risk aversion ( Tsui, 1993 ; Kornilova et al., 2018 ). UT appears positively related to adaptability and adaptive readiness ( Shamionov, 2017 ), and more specifically with the ability to cope constructively with chance events ( Kim et al., 2016 ). Persons with greater tolerance for ambiguity may also be more likely to engage in pro-social risk-taking behaviors ( Vives and Feldman Hall, 2018 ). That said, there does not appear to be evidence of a significant relationship between UT and cognitive control functions (e.g., conflict monitoring and attention allocation; Schroeder et al., 2018 ), suggesting tolerance for uncertainty may indeed be highly malleable ( Carleton, 2012 , 2016a ). Indeed, in a study of medical students, UT was increased as a function of education that specifically focused on increasing tolerance for ambiguity ( Taylor et al., 2018 ).
Reconciling Findings and Theories: The Central Role of Mental Simulation
As outlined in the previous sections, existing theories offer several useful insights about the relationship between uncertainty and affective and emotional states; however, more work is needed to fully describe the causal relationships between these phenomena. Existing theories do not account for why uncertainty is sometimes associated with positive affect. Rather, existing theories focus on downstream negative consequences of uncertainty and the psychological coping mechanisms people engage in to reduce negative affect (e.g., reaffirming goals and ideals; McGregor et al., 2009 ; van den Bos, 2009 ). The critical need moving forward is for an explanatory psychological theory that details the causal pathway from uncertainty to affective responses—both negative and positive. We believe that meeting this critical need will require much more research, both conceptual and empirical, and a synthesis of insights and evidence from multiple disciplinary perspectives. At the same time, we believe that existing insights and evidence make it possible to formulate a tentative, provisional account that can serve as a useful starting point for future work. In the next section, we will outline how mental simulation of possible future events might serve as the core psychological process linking uncertainty to affective and emotional states.
Humans constantly think about possible future events, imagining—or mentally simulating —alternative states of reality, such as what it will be like to gossip with friends, confront a co-worker, or commute home by a new route ( Seligman et al., 2013 ; Szpunar et al., 2014 ; Jing et al., 2016 ). This ability to simulate possible states of reality helps people plan for the future, test out alternative responses, and ultimately cope with stressful situations ( Taylor et al., 1998 ; Moulton and Kosslyn, 2009 ). In indeterminate situations, mental simulation of possible outcomes is a plausible adaptive strategy. For instance, if a medical test could show disease A or disease B, someone waiting anxiously for the results might first simulate what it would be like to have disease A, and then switch to simulating what it would be like to have disease B. This might help them plan for what would be needed in different possible situations. In this manner uncertainty might invite mental simulation.
We use the term “mental simulation” to cover a broad range of related topics: visual imagery, imagination, mind-wandering, daydreaming, default mode network activity—any kind of self-generated content that involves a simulation of the world. Simulation has emerged as a key, unifying process in cognitive science theories, such as grounded cognition —which posits that all mental functions are dependent on simulations (for review, see Barsalou, 2008 ). Mental simulations are thought to be linked to actions through imagining movements and motor imagery ( Jeannerod, 1994 ). Mental simulations can be effortful and goal oriented, as in mentally rotating objects to determine shape ( Shepard and Metzler, 1971 ). At other times, however, mental simulations can be automatically initiated, as in mind-wandering ( Smallwood and Schooler, 2015 ). Additionally, conscious awareness of mental simulation—in a broad sense covering the range of processes described above—might vary.
Several theories link mental simulation to affective processing. For instance, the somatic marker hypothesis suggests that simulation of bodily states (the reactivation of the “as-if body loop”) play a critical role in decision making and learning about rewards and punishment ( Damasio, 1996 ). Empirically, mental simulations can generate affective and emotional experiences ( Lang, 1977 , 1979 ). In fact, affective scientists use mental simulations to intentionally and specifically manipulate affect among participants in experimental studies (e.g., Wright and Mischel, 1982 ; Strack et al., 1985 ; Larsen and Ketelaar, 1991 ). By asking participants to mentally simulate sad or happy events from their own lives, researchers can reliably evoke these emotional states. These affective changes occur with corresponding low-level physiological changes, like fluctuations in heart rate, blood pressure, and electrodermal activity, which demonstrates that the physiological systems involved in affective states are also activated by mental simulations (for review, see Ji et al., 2016 ).
Thus, mental simulations might represent the critical mechanistic link between uncertainty and affective responses: uncertainty invites simulation of possible situations, and simulation, in turn, generates affective responses. For instance, if someone learns they might have cancer, they simulate what they think it would be like to have cancer (e.g., painful symptoms, treatment side-effects, hair loss, and death), which in turn generates negative affective responses.
The Content of Mental Simulations
If uncertainty induces mental simulations that evoke affective responses, then the nature of the affective responses (positive or negative valence) should depend on the content of the mental simulations. For instance, if uncertainty about playing the lottery induces mental simulations of a positive outcome such as buying a dream home or going on a vacation to Hawaii, then uncertainty will likely lead to pleasant affective feelings. However, if uncertainty about a medical test result induces mental simulations of a negative outcome such as receiving a phone call with a cancer diagnosis, then uncertainty will likely lead to negative affective feelings.
Therefore, the next important question is: What determines the contents of mental simulations? In psychology experiments, the content of mental simulations is often explicitly defined by researchers. For example, researchers instruct participants to simulate particular situations—e.g., remember a time they felt angry or happy. The content of simulation can also be shaped in more subtle ways as well, like describing a dilemma with a win or loss frame ( Kahneman and Tversky, 1979 ).
In everyday lives, however, little is known about what factors determine the content—and ultimately the affective valence—of mental simulations. We do know that minds are active even when people are resting and not given explicit instructions—that is, people’s minds wander (for review see Smallwood and Schooler, 2015 ). For instance, people spontaneously engage in a variety of mental simulations, including imagining future events, or thinking about an event from the perspectives of other people. This type of mind wandering is quite common ( Killingsworth and Gilbert, 2010 ) and is associated with brain activity in the default network that includes a medial temporal subsystem and medial prefrontal subsystem ( Grafton et al., 2007 ; Buckner et al., 2008 ; Christoff et al., 2009 ). Researchers measure activation in this region while participants are instructed to rest or focus on a fixation cross in neuroimaging studies. Understanding exactly why people spontaneously generate particular types of mental simulations in different life circumstances may help elucidate why uncertainty tends to provoke particular affective responses.
Bias Toward Negative Simulation
If mental simulation is a key process linking uncertainty to affect, then why is uncertainty typically experienced as a negative phenomenon? One possibility is that in situations with indeterminacy about whether a given outcome might be positive or negative, more weight is implicitly given to the potential negative outcome. An attentional bias toward the negative—toward prioritizing negative information—has been observed in many domains and may be a fundamental feature of the mind (for review, see Baumeister et al., 2001 ). For instance, even when confronted with conflicting visual information in binocular rivalry experiments, the visual system prioritizes faces associated with negative gossip over other faces ( Anderson et al., 2011 ). Additionally, negative beliefs have been shown to influence the perception of food, whereas positive beliefs do not ( Anderson and Barrett, 2016 ). This implicit, biased prioritization of negative stimuli may be an evolutionarily adaptive response, given that the cost of missing a negative threat may be much greater than the cost of missing a positive reward.
This bias toward negative as opposed to positive outcomes and information can be modeled using a framework that combines signal detection (decision making under uncertainty) and economic utility functions ( Lynn and Barrett, 2014 ). Signal detection theory posits that people operate in uncertain situations and must make decisions based on limited, conflicting, or noisy information (signals). For instance, a “signal” might consist of a co-worker’s ambiguous facial expression, which may or may not represent a social threat (e.g., “are they mad at me”?). The co-worker might truly be upset and in need of relationship repair, but may alternatively not be distressed and might be thinking of something else entirely, in which case behavior to repair the relationship might be unneeded or even costly.
Signal detection theory can be used to model the decision-making process as a function of the strength of the signal of potential threats, the perceived base rate of threats, and the perceived costs of missed detection (i.e., missing a threat) and false alarms (false-positive responses to non-existent threats). In situations in which the perceived frequency of threats or the cost of missing them is extremely high, it can be advantageous to liberally classify more signals as threats, by adopting a “zero-miss” strategy: treat any ambiguous stimuli as a threat. For instance, Quigley and Barrett (1999) give the following example of a child who grows up in an abusive household. When faced with uncertainty (i.e., “Is the caregiver angry?”), the child might use a zero-miss strategy that liberally classifies ambiguous expressions as threats. This strategy minimizes missed detections of threat, but simultaneously increases the number of false-positive responses to non-threatening caregiver expressions. This strategy would thus lead to frequent but ultimately unnecessary appeasement behaviors that may be costly from behavioral or social perspectives ( Quigley and Barrett, 1999 ; Lynn and Barrett, 2014 ).
The adaptive or evolutionary advantage of this tendency to adopt a zero-miss strategy—to categorize uncertain situations as negative by assuming the worst—may explain why individuals most often experience uncertainty as affectively negative (for discussion see Carleton, 2016a ). If one is confronted by a dark shadow, it may be more adaptive to act as if there is danger than to act as if there is not. This response may be either inherited or learned through experience, but in any case, simulation is the key link between uncertainty and affective responses. Uncertainty provides an opportunity to predict and simulate negative potential outcomes, and this simulation, in turn, generates negative affective responses.
This link between uncertainty and affect is likely moderated by various factors including trait-level characteristics of individuals. Dispositional optimism and pessimism are two such traits. It stands to reason that uncertainty would result in more aversive affective responses among people who are pessimistic—and thereby predisposed to simulate the worst possible outcomes, and less aversive responses among people who are optimistic—and predisposed to simulate more positive events. To our knowledge, however, little work has tested the factors that moderate and mediate the effects of uncertainty on affective outcomes.
This mental simulation account offers a novel way of understanding the relationship between uncertainty and affect by specifying mental simulation as the causal mechanism linking the two phenomena. Additionally, it suggests new research questions to be explored (see below for more details). This account may have some practical utility as well: if simulation is indeed the causal link between uncertainty and affect, changing what people simulate should change affective responses to uncertainty. More broadly, perspectives from emotion regulation ( Gross, 2014 ) offer theory-based guidance for possible strategies for coping with uncertainty. A more specific mechanistic understanding of the link between uncertainty and affect may help clinicians develop more effective interventions that target the causal pathways in people struggling to cope with uncertainty. We hope this account inspires new conceptual thinking, empirical studies, and practices to help people cope with uncertainty.
Effects of Uncertainty on Affect: Future Research Needs
Thus far, we have reviewed some empirical findings on the effects of uncertainty on affect, and suggested that mental simulation is a key process linking those phenomena. Uncertainty has been shown to cause both negative and positive affect and can both heighten and dampen existing affective feelings. We have argued that the mental simulation of potential future outcomes is a fundamental psychological process that may account for these effects; variability in affective responses to uncertainty may reflect variability in the content of people’s mental simulations. Clearly, more research is needed to fully specify and test this account against alternative theoretical models. Little is known about baseline individual differences in mental simulations in response to uncertainty. If simulation plays such a key role, it suggests the hypothesis that traits like optimism might moderate the content of simulations and thus affective responses. Moreover, it also suggests the hypothesis that influencing the content of mental simulations should change affective responses to uncertainty. This leads to the possibility that interventions that target simulation might help people regulate affective experience in the face of uncertainty.
Beyond testing hypotheses about the role of simulation in generating affective responses to uncertainty, we believe there are additional research questions that would be fruitful to explore further. We now outline several general questions that need to be answered to advance our understanding of the link between uncertainty and affect.
Empirical Issues: Mechanisms Linking Uncertainty to Affect
In general, more research is needed to test the different models to elucidate the mechanisms underlying the link between uncertainty and affect. In the next section, we outline suggestions for future work to explore factors that may mediate or moderate the relationship between uncertainty and affect.
Future research should explore what factors mediate the effects of uncertainty, and what elements of uncertainty represent the “active ingredient” responsible for its main effects. The experience of uncertainty is associated with a number of psychological phenomena: subjective perceptions of a lack of information, as well as other beliefs, attitudes, and judgments; affective feelings; and behavioral responses including information seeking and decision making (for discussion see Hillen et al., 2017 ). It is possible that any of these features might influence affect and other mental processes. For instance, uncertainty might be associated with attempts to gain more information, or sense making. These processes and not the feeling of uncertainty per se , might change affective experience ( Wilson et al., 2005 ). Future work is needed to tease apart these components of uncertainty to understand their causal significance.
More research is also needed to better understand the factors that moderate the effects of uncertainty on affect. Situational context is one potential moderator that needs to be explored in more detail. As described previously, in many situations uncertainty is experienced as unpleasant. However, in some contexts, people enjoy uncertainty (e.g., sports, gambling, movies). Some studies have found that uncertainty can intensify emotions ( Bar-Anan et al., 2009 ) while others found uncertainty dampens emotions ( van Dijk and Zeelenberg, 2006 ). Context appears to be a powerful moderator between uncertainty and affective experiences. For instance, whether people are uncertain about real events or imagined hypothetical scenarios might moderate affective reactions; uncertainty about imagined hypothetical scenarios might dampen affect ( van Dijk and Zeelenberg, 2006 ) while uncertainty about real events might intensify affect ( Bar-Anan et al., 2009 ).
Future work should also explore how different emotions are related to uncertainty. Past studies have typically explored how uncertainty influences affect and emotions broadly—e.g., intensifying or dampening all emotional and affective experiences ( van Dijk and Zeelenberg, 2006 ; Bar-Anan et al., 2009 ). It is quite possible, however, that uncertainty has differential effects on particular affective states or specific emotions (e.g., surprise, guilt, disgust). According to appraisal theories ( Moors et al., 2013 ), some emotions have uncertainty as a core appraisal dimension that triggers their activation (e.g., surprise, fear, anxiety—the emotions most commonly studied with uncertainty) while other emotions do not (e.g., guilt, shame, happiness). However, we are unaware of any empirical work has systematically studied the relationship between uncertainty and a broad range of particular affective and emotional states.
Additionally, future work should explore the breadth of variability in how uncertainty relates to affective states. In the evidence reviewed here, uncertainty intensified or dampened affect, but can uncertainty change the valence of an experience from positive to negative (or the other way around)? For instance, are there situations where low uncertainty leads people to experience a negative emotion (e.g., boredom), whereas the introduction of uncertainty into the situation might lead people experience a positive emotion (e.g., interest)? What factors moderate these effects?
Finally, more work should explore the range of individual differences in how uncertainty is experienced. Implicit in the very construct of “uncertainty tolerance” is the assumption that uncertainty is negative, a thing to be tolerated. But are there people at the other end of the spectrum who actually enjoy uncertainty? It is possible that thrill seekers, adventurers, and scientists are drawn to uncertainty? Further, what drives individual differences in uncertainty tolerance? Are they driven by life experience and/or genetic factors?
Methodological Issues: Measuring Uncertainty and Affect
An important limitation of past experiments examining the effects of uncertainty on affect is that participants’ experience of uncertainty is often experimentally manipulated but not measured. For instance, participants might be placed in the “uncertainty condition” where they are presumed to be uncertain about the outcome of a lottery. While this situation undoubtedly has elements of indeterminacy, the participants’ subjective experience of uncertainty is typically unmeasured (e.g., van Dijk and Zeelenberg, 2006 ; Kurtz et al., 2007 ; Bar-Anan et al., 2009 ). This is an important methodological deficiency given that people may not experience similar levels of uncertainty even when they are in similar situations. This limits our ability to draw firm inferences about the effects of uncertainty on affect. Thus, an important direction for future work is to improve the measurement of uncertainty itself.
A related limitation is the lack of specificity in measures of uncertainty, which have largely treated uncertainty as a monolithic phenomenon. Uncertainty, however, has numerous types that reflect the variety of sources and issues to which it applies ( Han et al., 2011 ). Sources include probability (risk), ambiguity, and complexity, while issues encompass a range of particular problems ranging from scientific to practical to personal in nature ( Han et al., 2011 ). An open empirical question is whether these different types of uncertainty have correspondingly different effects on affect—or lead to different specific emotions (e.g., anger, fear). More research is needed to develop reliable, valid measures that ascertain the variety of uncertainties that arise in life, and to examine their differential effects.
Additionally, there are many domains in which uncertainty is possible (e.g., financial, health, art) and it is not clear how similar or different the experience of uncertainty is in these different domains. This is particularly true for the construct of uncertainty tolerance. Little is known about whether uncertainty tolerance is domain-general or domain-specific.
Currently most measures of uncertainty rely on participant self-report of their experience of uncertainty. While useful, this approach requires introspection and accurate reporting and there may be situations in which these requirements produce inaccurate data. For instance, physicians may be motivated to present themselves as highly skilled and confident ( Katz, 2002 ), which might lead them to underreport their own experience of uncertainty. Other techniques for measuring uncertainty that do not rely on self-reports should be explored and validated. For instance, in cognitive psychology reaction time has been used as a measure of uncertainty or conflict between two options. Mouse-tracking has been used to study decisional conflict as people choose between two or more options, and mouse trajectories might be related to uncertainty ( Freeman and Ambady, 2010 ). Perceptual uncertainty has been linked to eye movements ( Brunyé and Gardony, 2017 ) so eye-tracking may be a promising measure of uncertainty in other domains. The Beads Task has been used to induce uncertainty, and has a behavioral measure that correlates with uncertainty tolerance ( Jacoby et al., 2014 ). Performance on this task might be used as an index of experienced uncertainty.
Finally, as noted in this review, there is evidence of a link between uncertainty and affect, and measures of affect might thus be used to draw inferences about experienced uncertainty. For instance, in contexts where affect has been shown to result from uncertainty (e.g., gambling), peripheral psychophysiology might yield information about experienced uncertainty. For example, in the Iowa Gambling task, participants choose between decks cards that could result in wins or losses ( Bechara et al., 2005 ). Participants reliably have skin conductance responses when they are uncertain about the outcome of their gamble ( Bechara et al., 2005 ). Researchers have traditionally used these skin conductance measures in this context to study affective responses to these gambles. However, those affective responses might be due in part to experienced uncertainty. Further work would be needed to explore the utility of these measures and experimental paradigms for studying uncertainty specifically.
Conceptual Issues: Uncertainty, Ignorance, and Consciousness
Uncertainty is ubiquitous, touching almost every aspect of our lives. As such, uncertainty has been studied from numerous disciplinary perspectives, including information theory, psychology, judgment and decision making, and economics, all of which have conceptualized uncertainty using different terminology and construed its nature and effects using different conceptual and methodological tools ( Smithson, 1989 ; Djulbegovic et al., 2011 ). The result has been a vast but often unconnected literature with both similar insights that have been expressed in different ways and different insights that have been expressed in similar ways. Theoretical and empirical findings from different fields have not been integrated in coherent fashion. Developing and advancing the science of uncertainty will require focused efforts to connect these findings.
Levels of Uncertainty and Consciousness
A particularly important and unresolved conceptual problem relates to whether uncertainty represents a conscious or unconscious state. In line with our previous work, here we have defined uncertainty as the conscious awareness of ignorance ( Han et al., 2011 ). However, we acknowledge that much cognition occurs unconsciously, automatically, and rapidly, and that no bright lines separate conscious vs . unconscious, deliberate vs . automatic, slow vs . fast cognitions. Consciousness is thus appropriately construed as a continuous rather than categorical phenomenon ( Pessoa et al., 2005 ; Rouder and Morey, 2009 ; Tamietto et al., 2015 ). Cognitions become more unconscious, automatic, and rapid as expertise is developed ( Logan, 1988 ), and the same may be true for mental simulations provoked by uncertainty.
Unconscious “uncertainty” has become a major theme in cognitive science, and recent work construes the brain as a “prediction machine” ( Friston, 2010 ; Clark, 2013 ). The perceptual system in particular has to make predictions based on noisy incoming signals of unknown significance. For instance, light striking the retina can be reflected off a number of sources, leading to perceptual uncertainty about exactly what objects are in the environment. According to predictive theories such as Clark’s, the brain perceives stimuli by matching incoming “bottom-up” sensory signals from the world with “top-down” predictions from the brain. When predictions are incorrect, neural error signals feedback to perceptual brain areas, enabling the brain to tune and calibrate future predictions. This feedback system, called predictive coding, enables the brain to reduce mismatches between the actual world and mental representations of it, fine-tuning an exquisite prediction machine to an ever-changing environment.
This emerging theoretical perspective construes responding to perceptual uncertainty as the fundamental task and challenge of the brain. The view of the mind as a prediction machine expands and refines our understanding of uncertainty as a “conscious” experience, but also calls into question the conceptual distinction between ignorance and uncertainty. If uncertainty can be unconscious as well as conscious, then the distinction between ignorance and uncertainty becomes obliterated.
We believe this conceptual equation goes too far—that there are qualitatively discrete levels of consciousness of ignorance, and defensible reasons for distinguishing between them. Even if unconscious uncertainty (what we call “ignorance”) is psychologically consequential at an automatic and perceptual or sensorimotor level, there still exists an important, categorically discrete realm of everyday human experience involving conscious deliberation and awareness of ignorance (what we call “uncertainty”), and this conscious awareness is also psychologically consequential. The vast body of research to date has shown that moving people from unconscious to conscious ignorance clearly has cognitive, emotional, and behavioral effects. The existence of these effects provides empirical justification for the distinction between conscious and unconscious ignorance, and we believe the respective terms “uncertainty” and “ignorance” provide a useful short-hand or linguistic representation of this distinction.
More research is needed to understand the affective and emotional effects of uncertainty existing at different levels of conscious awareness, ranging from complete unconsciousness (pure ignorance) to complete consciousness of ignorance (pure uncertainty). Additionally, more work is need to understand how automatic and rapid—vs. deliberate and slow—people’s cognitive and affective responses to uncertainty are in different life situations.
Applied Issues: Improving Affective Responses to Uncertainty
From a practical standpoint, people struggle with uncertainty in their lives. Uncertainty can lead to suboptimal decision making, negative affect, diminished well-being, and psychopathology ( Gray and McNaughton, 2000 ; Rabin and Thaler, 2001 ; McGregor et al., 2009 ; van den Bos, 2009 ; Hirsh et al., 2012 ; Grupe and Nitschke, 2013 ; Shihata et al., 2016 ; Carleton, 2016a ; Strout et al., 2018 ; Vives and Feldman Hall, 2018 ). Addressing these problems requires applied research aimed at improving people’s ability to regulate and cope with the negative emotional effects of uncertainty.
The ability to regulate affective/emotional responses has been increasingly recognized as an important factor in health psychology (for discussion, see DeSteno et al., 2013 ), and emotion regulation may play a critical role in people’s ability to cope with uncertainty. When someone experiences negative affect resulting from uncertainty, it should be possible to use various regulatory strategies to reduce the unpleasant experience. For instance, someone who has been diagnosed with early stage cancer might suffer from anxiety while waiting for further medical tests to be conducted. One strategy might be to re-appraise the situation: “Uncertainty about what type of cancer I have means there is a chance my cancer is benign–I’ll focus on that possibility.” They could also use distraction: “I will keep busy to try to not think about the fact that my cancer could progress.” These strategies might aim to both diminish negative feelings and enhance positive feelings.
In addition to strategies aimed at regulating one’s affective responses to uncertainty, people might also adopt strategies aimed at reducing uncertainty directly. These strategies can take many forms, from conducting another test to clarify a diagnosis to finding a specialist for a second opinion. However, these actions also raise the possibility of obtaining conflicting information—for example, differing opinions among experts or conflicting test results—which can generate greater uncertainty.
People may also cope with uncertainty in pathological ways ( Grupe and Nitschke, 2013 ; Carleton, 2016a ). For example, individuals with obsessive compulsive disorder might use repeated checking behaviors to manage their uncertainty ( Tolin et al., 2003 ). Similarly, individuals with generalized anxiety disorder might engage in repeated worrying to manage their perceptions of uncertainty ( Dugas et al., 1997 ; Davey and Wells, 2006 ). The full cause-effect pathway for the relationship between coping with uncertainty and pathology has not been definitively resolved ( Shihata et al., 2016 ); however, there is substantial evidence that increased uncertainty tolerance produces self-reported and behavioral reductions in pathology ( Hewitt et al., 2009 ; Barlow et al., 2011 ; Farchione et al., 2012 ; Mahoney and McEvoy, 2012 ; Boswell et al., 2013 ; Cuijpers et al., 2014 ; McEvoy and Erceg-Hurn, 2015 ). This suggests uncertainty tolerance and associated coping may be important etiological factors ( Boswell et al., 2013 ; Carleton, 2016a ). In any case, understanding the ways people regulate and ultimately cope with uncertainty is an important future research direction. Greater understanding of the diversity of regulatory and coping strategies and the outcomes of these strategies can then inform the development of interventions to help people cope more effectively with uncertainty in their lives.
Reversing the Causal Arrow: Can Affect Influence Uncertainty?
In this paper we have focused on uncertainty causing affective feelings, but causality in the opposite direction might be possible too. That is, can affective feeling influence the experience of uncertainty? Do people experiencing particular emotions have different experiences of uncertainty? We are not aware of any data or theories showing this directly, but as described earlier there is a vast literature describing how affect and emotions influence perceptions of risk ( Johnson and Tversky, 1983 ; DeSteno et al., 2000 ; Lerner and Keltner, 2001 ; Loewenstein et al., 2001 ) and decisions ( Schwarz and Clore, 1983 ; Damasio, 1994 , 1996 ; Slovic et al., 2007 ) in situations of complexity, ambiguity, and indeterminacy. Thus, affect and emotions seem to influence the mind in contexts where uncertainty is also present. More research is needed to directly test whether affect can directly influence uncertainty, and whether there is a bi-directional relationship between uncertainty and affect. Future theoretical work should attempt to integrate both causal directions into one theory: how uncertainty influences affect and how affect influences uncertainty.
Conclusions
The goal of this article was to briefly review and synthesize the literature on the relationship between uncertainty and affect. Although most empirical and theoretical research to date has focused on the negative effects of uncertainty on affect ( Gray and McNaughton, 2000 ; McGregor et al., 2009 ; van den Bos, 2009 ; Hirsh et al., 2012 ; Grupe and Nitschke, 2013 ; Carleton, 2016a ), there is some experimental evidence suggesting that uncertainty has positive effects ( Kurtz et al., 2007 ) and also intensifies ( Bar-Anan et al., 2009 ) or dampens affective feelings ( van Dijk and Zeelenberg, 2006 ). The obvious question is why uncertainty leads to these different outcomes and what factors moderate and mediate its effects? Existing theoretical frameworks do not focus explicitly on the relationship between uncertainty and affect, and are descriptive rather than explanatory. We suggest that uncertainty influences affective states by prompting the mental simulation of possible future outcomes. Additionally, people have a propensity to primarily simulate negative outcomes, which, in turn, tends to generate negative affect. We also propose the existence of several important moderators of this process, including context and other situation factors, as well as individual differences such as uncertainty tolerance. Our synthesis also highlights how negative responses to uncertainty may also be controlled by emotion regulation strategies. Finally, we offer hypotheses generated by our approach, highlight important knowledge gaps, and promising areas for future research, both empirical and conceptual, to improve our understanding of the relationship between uncertainty and affect.
Author Contributions
All authors listed have made a substantial, direct and intellectual contribution to the work, and approved it for publication.
Conflict of Interest
The authors declare that the research was conducted in the absence of any commercial or financial relationships that could be construed as a potential conflict of interest.
Acknowledgments
Special thanks to Deanna Williams for help in designing the figures.
- Anderson E. C., Barrett L. F. (2016). Affective beliefs influence the experience of eating meat . PLoS One 11 , 1–16. 10.1371/journal.pone.0160424 [ PMC free article ] [ PubMed ] [ CrossRef ] [ Google Scholar ]
- Anderson E. C., Siegel E. H., Bliss-Moreau E., Barrett L. F. (2011). The visual impact of gossip . Science 332 , 1446–1448. 10.1126/science.1201574, PMID: [ PMC free article ] [ PubMed ] [ CrossRef ] [ Google Scholar ]
- Babayan B. M., Uchida N., Gershman S. J. (2018). Belief state representation in the dopamine system . Nat. Commun. 9 :1891. 10.1038/s41467-018-04397-0 [ PMC free article ] [ PubMed ] [ CrossRef ] [ Google Scholar ]
- Bar-Anan Y., Wilson T. D., Gilbert D. T. (2009). The feeling of uncertainty intensifies affective reactions . Emotion 9 , 123–127. 10.1037/a0014607, PMID: [ PubMed ] [ CrossRef ] [ Google Scholar ]
- Barlow D. H., Ellard K. K., Fairholme C., Farchione T. J., Boisseau C., Allen L., et al. (2011). Unified protocol for the transdiagnostic treatment of emotional disorders . New York, NY, USA: Oxford University Press. [ Google Scholar ]
- Barrett L. F. (2006). Solving the emotion paradox: categorization and the experience of emotion . Personal. Soc. Psychol. Rev. 10 , 20–46. 10.1207/s15327957pspr1001_2 [ PubMed ] [ CrossRef ] [ Google Scholar ]
- Barrett L. F., Bliss-Moreau E. (2009). Affect as a psychological primitive . Adv. Exp. Soc. Psychol. 41 , 167–218. 10.1016/S0065-2601(08)00404-8, PMID: [ PMC free article ] [ PubMed ] [ CrossRef ] [ Google Scholar ]
- Barrett L. F., Lindquist K., Bliss-Moreau E., Duncan S., Gendron M., Mize J., et al. (2007a). Of mice and men: natural kinds of emotions in the mammalian brain? A response to Panksepp and izard . Perspect. Psychol. Sci. 2 , 297–312. Available at: http://pps.sagepub.com/content/2/3/297.short [ PMC free article ] [ PubMed ] [ Google Scholar ]
- Barrett L. F., Ochsner K. N., Gross J. J. (2007b). “ On the automaticity of emotion ” in Social psychology and the unconscious: The automaticity of higher mental processes . ed. Bargh J. A. (New York, NY: Psychology Press; ), Vol. 58 , 173–217. [ Google Scholar ]
- Barsalou L. W. (2008). Grounded cognition . Annu. Rev. Psychol. 59 , 617–645. 10.1146/annurev.psych.59.103006.093639, PMID: [ PubMed ] [ CrossRef ] [ Google Scholar ]
- Baumeister R. F., Bratslavsky E., Finkenauer C., Vohs K. D. (2001). Bad is stronger than good . Rev. Gen. Psychol. 5 , 323–370. 10.1037/1089-2680.5.4.323 [ CrossRef ] [ Google Scholar ]
- Bechara A., Damasio H., Tranel D., Damasio A. R. (2005). The Iowa gambling task and the somatic marker hypothesis: some questions and answers . Trends Cogn. Sci. 9 , 159–162. 10.1016/j.tics.2005.02.002, PMID: [ PubMed ] [ CrossRef ] [ Google Scholar ]
- Boswell J. F., Thompson-Hollands J., Farchione T. J., Barlow D. H. (2013). Intolerance of uncertainty: a common factor in the treatment of emotional disorders . J. Clin. Psychol. 69 , 630–645. 10.1002/jclp.21965, PMID: [ PMC free article ] [ PubMed ] [ CrossRef ] [ Google Scholar ]
- Brunyé T. T., Gardony A. L. (2017). Eye tracking measures of uncertainty during perceptual decision making . Int. J. Psychophysiol. 120 , 60–68. 10.1016/j.ijpsycho.2017.07.008 [ PubMed ] [ CrossRef ] [ Google Scholar ]
- Buckner R. L., Andrews-Hanna J. R., Schacter D. L. (2008). The brain’s default network: anatomy, function, and relevance to disease . Ann. N. Y. Acad. Sci. 1124 , 1–38. 10.1196/annals.1440.011, PMID: [ PubMed ] [ CrossRef ] [ Google Scholar ]
- Buckner R. L., Carroll D. C. (2007). Self-projection and the brain . Trends Cogn. Sci. 11 , 49–57. 10.1016/j.tics.2006.11.004, PMID: [ PubMed ] [ CrossRef ] [ Google Scholar ]
- Cacioppo J. T., Berntson G. G., Norris C. J., Gollan J. K. (2012). “ The evaluative space model ” in Handbook of Theories of Social Psychology . Sage Publications, Vol. 1 , 50–72. [ Google Scholar ]
- Camerer C., Weber M. (1992). Recent developments in Modeling preferences: uncertainty and ambiguity . J. Risk Uncertain. 5 , 325–370. 10.1007/BF00122575 [ CrossRef ] [ Google Scholar ]
- Carleton R. N. (2012). The intolerance of uncertainty construct in the context of anxiety disorders: theoretical and practical perspectives . Expert. Rev. Neurother. 12 , 937–947. 10.1586/ern.12.82 [ PubMed ] [ CrossRef ] [ Google Scholar ]
- Carleton R. N. (2016a). Fear of the unknown: one fear to rule them all? J. Anxiety Disord. 41 , 5–21. 10.1016/j.janxdis.2016.03.011 [ PubMed ] [ CrossRef ] [ Google Scholar ]
- Carleton R. N. (2016b). Into the unknown: a review and synthesis of contemporary models involving uncertainty . J. Anxiety Disord. 39 , 30–43. 10.1016/j.janxdis.2016.02.007 [ PubMed ] [ CrossRef ] [ Google Scholar ]
- Christoff K., Gordon A. M., Smallwood J., Smith R., Schooler J. W. (2009). Experience sampling during fMRI reveals default network and executive system contributions to mind wandering . PNAS 106 , 8719–8724. 10.1073/pnas.0900234106 [ PMC free article ] [ PubMed ] [ CrossRef ] [ Google Scholar ]
- Clark A. (2013). Whatever next? Predictive brains, situated agents, and the future of cognitive science . Behav. Brain Sci. 36 , 181–204. 10.1017/S0140525X12000477, PMID: [ PubMed ] [ CrossRef ] [ Google Scholar ]
- Clark A. (2015). Surfing uncertainty: Prediction, action, and the embodied mind . New York, NY: Oxford University Press. [ Google Scholar ]
- Cuijpers P., Sijbrandij M., Koole S., Huibers M., Berking M., Andersson G. (2014). Psychological treatment of generalized anxiety disorder: a meta-analysis . Clin. Psychol. Rev. 34 , 130–140. 10.1016/j.cpr.2014.01.002, PMID: [ PubMed ] [ CrossRef ] [ Google Scholar ]
- Damasio A. R. (1994). Descartes’ error: Emotion, reason, and the human brain . New York, NY: Grosset/Putnam. [ Google Scholar ]
- Damasio A. R. (1996). The somatic marker hypothesis and the possible functions of the prefrontal cortex . Philos. Trans. R Soc. Lond. Ser. B Biol. Sci. 351 , 1413–1420. [ PubMed ] [ Google Scholar ]
- Davey G. C. L., Wells A. (Eds.) (2006). Worry and its psychological disorders: Theory, assessment and treatment . Chichester, West Sussex: John Wiley & Sons. [ Google Scholar ]
- DeSteno D., Gross J. J., Kubzansky L. (2013). Affective science and health: the importance of emotion and emotion regulation . Health Psychol. 32 , 474–486. 10.1037/a0030259, PMID: [ PubMed ] [ CrossRef ] [ Google Scholar ]
- DeSteno D., Petty R. E., Wegener D. T., Rucker D. D. (2000). Beyond valence in the perception of likelihood: the role of emotion specificity . J. Pers. Soc. Psychol. 78 , 397–416. 10.1037/0022-3514.78.3.397 [ PubMed ] [ CrossRef ] [ Google Scholar ]
- Djulbegovic B., Hozo I., Greenland S. (2011). “ Uncertainty in clinical medicine ” in Philosophy of medicine . San Diego: North Holland. [ Google Scholar ]
- Dugas M. J., Freeston M. H., Ladouceur R. (1997). Intolerance of uncertainty and problem orientation in worry . Cogn. Ther. Res. 21 , 593–606. 10.1023/A:1021890322153 [ CrossRef ] [ Google Scholar ]
- Duncan S., Barrett L. F. (2007). Affect is a form of cognition: a neurobiological analysis . Cognit. Emot. 21 , 1184–1211. 10.1080/02699930701437931, PMID: [ PMC free article ] [ PubMed ] [ CrossRef ] [ Google Scholar ]
- Duttle K., Inukai K. (2015). Complexity aversion: influences of cognitive abilities, culture and system of thought . Econ. Bull. 35 , 846–855. 10.13140/RG.2.1.1604.8486 [ CrossRef ] [ Google Scholar ]
- Ellsberg D. (1961). Risk, ambiguity, and the savage axioms . Q. J. Econ. 75 , 643–669. [ Google Scholar ]
- Farchione T. J., Fairholme C. P., Ellard K. K., Boisseau C. L., Thompson-Hollands J., Carl J. R., et al.. (2012). Unified protocol for Transdiagnostic treatment of emotional disorders: a randomized controlled trial . Behav. Ther. 43 , 666–678. 10.1016/j.beth.2012.01.001, PMID: [ PMC free article ] [ PubMed ] [ CrossRef ] [ Google Scholar ]
- Ferrer R. A., Green P. a., Barrett L. F. (2015). Affective science perspectives on cancer control: strategically crafting a mutually beneficial research agenda . Perspect. Psychol. Sci. 10 , 328–345. 10.1177/1745691615576755, PMID: [ PMC free article ] [ PubMed ] [ CrossRef ] [ Google Scholar ]
- Freeman J. B., Ambady N. (2010). MouseTracker: software for studying real-time mental processing using a computer mouse-tracking method . Behav. Res. Methods 42 , 226–241. 10.3758/BRM.42.1.226, PMID: [ PubMed ] [ CrossRef ] [ Google Scholar ]
- Friston K. (2010). The free-energy principle: a unified brain theory? Nat. Rev. Neurosci. 11 , 127–138. 10.1038/nrn2787, PMID: [ PubMed ] [ CrossRef ] [ Google Scholar ]
- Garrison Y. L., Lee K. H., Ali S. R. (2017). Career identity and life satisfaction: the mediating role of tolerance for uncertainty and positive/negative affect . J. Career Dev. 44 , 516–529. 10.1177/0894845316668410 [ CrossRef ] [ Google Scholar ]
- Gigerenzer G., Goldstein D. G. (1996). Reasoning the fast and frugal way . Psychol. Rev. 103 , 650–669. 10.1037/0033-295X.103.4.650, PMID: [ PubMed ] [ CrossRef ] [ Google Scholar ]
- Gilbert D. T., Wilson T. D., Pinel E. C., Blumberg S. J., Wheatley T. P. (1998). Immune neglect: a source of durability bias in affective forecasting . J. Pers. Soc. Psychol. 75 , 617–638. 10.1037/0022-3514.75.3.617 [ PubMed ] [ CrossRef ] [ Google Scholar ]
- Grafton S. T., Van Horn J. D., Wegner D. M., Mason M. F., Macrae C. N., Norton M. I. (2007). Wandering minds: the default network and stimulus-independent thought . Science 315 , 393–395. 10.1007/BF02373035 [ PMC free article ] [ PubMed ] [ CrossRef ] [ Google Scholar ]
- Gray J. A. (1976). “ The behavioural inhibition system: a possible substrate for anxiety ” in Theoretical and experimental bases of behaviour modification . eds. Feldman M. P., Broadhurst A. M. (London: Wiley; ), 3–41. [ Google Scholar ]
- Gray J. A., McNaughton N. (2000). The neuropsychology of anxiety: An enquiry into the functions of the septo-hippocampal system. 2nd Edn . New York: Oxford University Press. [ Google Scholar ]
- Greenberg J., Solomon S., Pyszczynski T. (1997). “ Terror management theory of self-esteem and cultural worldviews: empirical assessments and conceptual refinements ” in Advances in experimental social psychology . ed. Zanna M. (New York: Academic Press; ), 61–139. [ Google Scholar ]
- Greene J. D., Sommerville R. B., Nystrom L. E., Darley J. M., Cohen J. D. (2001). An fMRI investigation of emotional engagement in moral judgment . Science 293 , 2105–2108. 10.1126/science.1062872, PMID: [ PubMed ] [ CrossRef ] [ Google Scholar ]
- Gross J. J. (2014). “ Emotion regulation: conceptual and empirical foundations ” in Handbook of Emotion Regulation . (New York: Guilford Press; ), 3–20. 10.1080/00140130600971135 [ CrossRef ] [ Google Scholar ]
- Grupe D. W., Nitschke J. B. (2013). Uncertainty and anticipation in anxiety: an integrated neurobiological and psychological perspective . Nat. Rev. Neurosci. 14 , 488–501. 10.1038/nrn3524, PMID: [ PMC free article ] [ PubMed ] [ CrossRef ] [ Google Scholar ]
- Han P. K. J. (2013). Conceptual, methodological, and ethical problems in communicating uncertainty in clinical evidence . Med. Care Res. Rev. 70 ( Suppl. 1 ), 14S–36S. 10.1177/1077558712459361 [ PMC free article ] [ PubMed ] [ CrossRef ] [ Google Scholar ]
- Han P. K. J., Klein W. M. P. P., Arora N. K. (2011). Varieties of uncertainty in health care: a conceptual taxonomy . Med. Decis. Mak. 31 , 828–838. 10.1177/0272989X11393976 [ PMC free article ] [ PubMed ] [ CrossRef ] [ Google Scholar ]
- Hewitt S. N., Egan S., Rees C. (2009). Preliminary investigation of intolerance of uncertainty treatment for anxiety disorders . Clin. Psychol. 13 , 52–58. 10.1080/13284200802702056 [ CrossRef ] [ Google Scholar ]
- Hillen M. A., Gutheil C. M., Strout T. D., Smets E. M. A., Han P. K. J. (2017). Tolerance of uncertainty: conceptual analysis, integrative model, and implications for healthcare . Soc. Sci. Med. 180 , 62–75. 10.1016/j.socscimed.2017.03.024, PMID: [ PubMed ] [ CrossRef ] [ Google Scholar ]
- Hirsh J. B., Mar R. A., Peterson J. B. (2012). Psychological entropy: a framework for understanding uncertainty-related anxiety . Psychol. Rev. 119 , 304–320. 10.1037/a0026767 [ PubMed ] [ CrossRef ] [ Google Scholar ]
- Iyengar S. S., Lepper M. R. (2000). When choice is demotivating: can one desire too much of a good thing? J. Pers. Soc. Psychol. 79 , 995–1006. 10.1037/0022-3514.79.6.995, PMID: [ PubMed ] [ CrossRef ] [ Google Scholar ]
- Jacoby R. J., Abramowitz J. S., Buck B. E., Fabricant L. E. (2014). How is the beads task related to intolerance of uncertainty in anxiety disorders? J. Anxiety Disord. 28 , 495–503. 10.1016/j.janxdis.2014.05.005, PMID: [ PubMed ] [ CrossRef ] [ Google Scholar ]
- Jeannerod M. (1994). The representing brain: neural correlates of motor intention and imagery . Behav. Brain Sci. 17 , 187–202. 10.1017/S0140525X00034026 [ CrossRef ] [ Google Scholar ]
- Ji J. L., Heyes S. B., Mac Leod C., Holmes E. A. (2016). Emotional mental imagery as simulation of reality: fear and beyond—a tribute to Peter Lang . Behav. Ther. 47 , 702–719. 10.1016/j.beth.2015.11.004, PMID: [ PMC free article ] [ PubMed ] [ CrossRef ] [ Google Scholar ]
- Jing H. G., Madore K. P., Schacter D. L. (2016). Worrying about the future: an episodic specificity induction impacts problem solving, reappraisal, and well-being . J. Exp. Psychol. Gen. 145 , 402–418. 10.1037/xge0000142 [ PMC free article ] [ PubMed ] [ CrossRef ] [ Google Scholar ]
- Johnson E. J., Tversky A. (1983). Affect, generalization, and the perception of risk . J. Pers. Soc. Psychol. 45 , 20–31. 10.1037/0022-3514.45.1.20 [ CrossRef ] [ Google Scholar ]
- Kahneman D. (2011). Thinking, fast and slow . New York, USA: Farrar, Straus and Giroux. [ Google Scholar ]
- Kahneman D., Tversky A. (1979). Prospect theory: an analysis of decision under risk . Econometrica 47 , 263–291. 10.2307/1914185 [ CrossRef ] [ Google Scholar ]
- Katz J. (2002). The silent world of doctor and patient . Baltimore and London: Johns Hopkins University Press. [ Google Scholar ]
- Killingsworth M. A., Gilbert D. T. (2010). A wandering mind is an unhappy mind . Science 330 :932. 10.1126/science.1192439, PMID: [ PubMed ] [ CrossRef ] [ Google Scholar ]
- Kim B., Rhee E., Ha G., Yang J., Lee S. M. (2016). Tolerance of uncertainty: links to happenstance, career decision self-efficacy, and career satisfaction . Career Dev. Q. 64 , 140–152. 10.1002/cdq.12047 [ CrossRef ] [ Google Scholar ]
- Knobloch-Westerwick S. S., David P., Eastin M. S., Tamborini R., Greenwood D. (2009). Sports spectators’ suspense: affect and uncertainty in sports entertainment . J. Commun. 59 , 750–767. 10.1111/j.1460-2466.2009.01456.x [ CrossRef ] [ Google Scholar ]
- Kornilova T. V., Chumakova M. A., Kornilov S. A. (2018). Tolerance and intolerance for uncertainty as predictors of decision making and risk acceptance in gaming strategies of the Iowa gambling task . Psychol. Russ. State Art 11 , 86–95. 10.11621/pir.2018.0306 [ CrossRef ] [ Google Scholar ]
- Kovářík J., Levin D., Wang T. (2016). Ellsberg paradox: ambiguity and complexity aversions compared . J. Risk Uncertain. 52 , 47–64. 10.1007/s11166-016-9232-0 [ CrossRef ] [ Google Scholar ]
- Kurtz J. L., Wilson T. D., Gilbert D. T. (2007). Quantity versus uncertainty: when winning one prize is better than winning two . J. Exp. Soc. Psychol. 43 , 979–985. 10.1016/j.jesp.2006.10.020 [ CrossRef ] [ Google Scholar ]
- Lang P. J. (1977). Imagery in therapy: an information processing analysis of fear . Behav. Ther. 8 , 862–886. 10.1016/S0005-7894(77)80157-3 [ PubMed ] [ CrossRef ] [ Google Scholar ]
- Lang P. J. (1979). A bio-informational theory of emotional imagery . Psychophysiology 16 , 495–512. 10.1111/j.1469-8986.1979.tb01511.x, PMID: [ PubMed ] [ CrossRef ] [ Google Scholar ]
- Larsen R. J., Ketelaar T. (1991). Personality and susceptibility to positive and negative emotional states . J. Pers. Soc. Psychol. 61 , 132–140. 10.1037//0022-3514.61.1.132 [ PubMed ] [ CrossRef ] [ Google Scholar ]
- Ledoux J. E. (2000). Emotion circuits in the brain . Annu. Rev. Neurosci. 23 , 155–184. 10.1146/annurev.neuro.23.1.155, PMID: [ PubMed ] [ CrossRef ] [ Google Scholar ]
- Lerner M. J. (1980). The belief in a just world . Boston, MA: Springer. [ Google Scholar ]
- Lerner J. S., Keltner D. (2001). Fear, anger, and risk . J. Pers. Soc. Psychol. 81 , 146–159. 10.1037/0022-3514.81.1.146, PMID: [ PubMed ] [ CrossRef ] [ Google Scholar ]
- Loewenstein G. F., Weber E. U., Hsee C. K., Welch N. (2001). Risk as feeling . Psychol. Bull. 127 , 267–286. 10.1037/0033-2909.127.2.267, PMID: [ PubMed ] [ CrossRef ] [ Google Scholar ]
- Logan G. (1988). Toward an instance theory of Automization . Psychol. Rev. 95 , 492–527. 10.1037/0033-295X.95.4.492 [ CrossRef ] [ Google Scholar ]
- Lovallo D., Kahneman D. (2000). Living with uncertainty: attractiveness and resolution timing . J. Behav. Decis. Mak. 13 , 179–190. 10.1002/(SICI)1099-0771(200004/06)13:2<179::AID-BDM332>3.0.CO;2-J [ CrossRef ] [ Google Scholar ]
- Lowe R., Almér A., Billing E., Sandamirskaya Y., Balkenius C. (2017). Affective–associative two-process theory: a neurocomputational account of partial reinforcement extinction effects . Biol. Cybern. 111 , 365–388. 10.1007/s00422-017-0730-1 [ PMC free article ] [ PubMed ] [ CrossRef ] [ Google Scholar ]
- Lowe R., Ziemke T. (2013). “ Exploring the relationship of reward and punishment in reinforcement learning ” in IEEE symposium on adaptive dynamic programming and reinforcement learning, ADPRL , 140–147.
- Lynn S. K., Barrett L. F. (2014). “Utilizing” signal detection theory . Psychol. Sci. 25 , 1663–1673. 10.1177/0956797614541991, PMID: [ PMC free article ] [ PubMed ] [ CrossRef ] [ Google Scholar ]
- Mahoney A. E. J., McEvoy P. M. (2012). Changes in intolerance of uncertainty during cognitive behavior group therapy for social phobia . J. Behav. Ther. Exp. Psychiatry 43 , 849–854. 10.1016/j.jbtep.2011.12.004, PMID: [ PubMed ] [ CrossRef ] [ Google Scholar ]
- Masicampo E., Baumeister R. F. (2008). Toward a physiology of dual-process reasoning and judgment . Psychol. Sci. 19 , 255–260. 10.1111/j.1467-9280.2008.02077.x, PMID: [ PubMed ] [ CrossRef ] [ Google Scholar ]
- McEvoy P. M., Erceg-Hurn D. M. (2015). The search for universal transdiagnostic and trans-therapy change processes: evidence for intolerance of uncertainty . J. Anxiety Disord. 41 , 96–107. 10.1016/j.janxdis.2016.02.002 [ PubMed ] [ CrossRef ] [ Google Scholar ]
- McGregor I., Nash K., Mann N., Phills C. E. (2010). Anxious uncertainty and reactive approach motivation (RAM) . J. Pers. Soc. Psychol. 99 , 133–147. 10.1037/a0019701 [ PubMed ] [ CrossRef ] [ Google Scholar ]
- McGregor I., Prentice M. S., Nash K. A. (2009). Personal uncertainty management by reactive approach motivation . Psychol. Inq. 20 , 225–229. 10.1080/10478400903333460 [ CrossRef ] [ Google Scholar ]
- McGregor I., Zanna M. P., Holmes J. G., Spencer S. J. (2001). Compensatory conviction in the face of personal uncertainty: going to extremes and being oneself . J. Pers. Soc. Psychol. 80 , 472–488. 10.1037/0022-3514.80.3.472 [ PubMed ] [ CrossRef ] [ Google Scholar ]
- Menninghaus W., Wagner V., Hanich J., Wassiliwizky E., Jacobsen T., Koelsch S. (2017). The distancing-embracing model of the enjoyment of negative emotions in art reception . Behav. Brain Sci. , 1–63. 10.1017/S0140525X17000309 [ PubMed ] [ CrossRef ] [ Google Scholar ]
- Moors A., Ellsworth P. C., Scherer K. R., Frijda N. H. (2013). Appraisal theories of emotion: state of the art and future development . Emot. Rev. 5 , 119–124. 10.1177/1754073912468165 [ CrossRef ] [ Google Scholar ]
- Moulton S. T., Kosslyn S. M. (2009). Imagining predictions: mental imagery as mental emulation . Philos. Trans. R. Soc. B. Biol. Sci. 364 , 1273–1280. 10.1098/rstb.2008.0314, PMID: [ PMC free article ] [ PubMed ] [ CrossRef ] [ Google Scholar ]
- Pavlova E. M. (2018). Model of connections between self-esteem of creativity and intelligence, tolerance of uncertainty and creativity . Psychol. J. High. School Econ. 15 , 69–78. 10.17323/1813-8918-2018-1-69-78 [ CrossRef ] [ Google Scholar ]
- Pessoa L., Japee S., Ungerleider L. G. (2005). Visual awareness and the detection of fearful faces . Emotion 5 , 243–247. 10.1037/1528-3542.5.2.243, PMID: [ PubMed ] [ CrossRef ] [ Google Scholar ]
- Quigley K. S., Barrett L. F. (1999). “ Emotional learning and mechanisms of intentional psychological change ” in Action and self-development: Theory and research through the life span . Thousand Oaks, CA: Sage, 435–464. Available at: http://resources.library.brandeis.edu/login?url=http://search.ebscohost.com/login.aspx?direct=true&db=psyh&AN=1999-04290-015&site=ehost-live&scope=site [ Google Scholar ]
- Rabin M., Thaler R. H. (2001). Anomalies: risk aversion . J. Econ. Perspect. 15 , 219–232. 10.1257/jep.15.1.219 [ CrossRef ] [ Google Scholar ]
- Rand D. G., Greene J. D., Nowak M. a. (2012). Spontaneous giving and calculated greed . Nature 489 , 427–430. 10.1038/nature11467, PMID: [ PubMed ] [ CrossRef ] [ Google Scholar ]
- Reimann M., Bechara A. (2010). The somatic marker framework as a neurological theory of decision-making: review, conceptual comparisons, and future neuroeconomics research . J. Econ. Psychol. 31 , 767–776. 10.1016/j.joep.2010.03.002 [ CrossRef ] [ Google Scholar ]
- Rouder J. N., Morey R. D. (2009). The nature of psychological thresholds . Psychol. Rev. 116 , 655–660. 10.1037/a0016413, PMID: [ PubMed ] [ CrossRef ] [ Google Scholar ]
- Russell J. A. (2003). Core affect and the psychological construction of emotion . Psychol. Rev. 110 , 145–172. 10.1037/0033-295X.110.1.145, PMID: [ PubMed ] [ CrossRef ] [ Google Scholar ]
- Russell J. A., Barrett L. F. (1999). Core affect, prototypical emotional episodes, and other things called emotion: dissecting the elephant . J. Pers. Soc. Psychol. 76 , 805–819. Available at: http://psycnet.apa.org/journals/psp/76/5/805/ [ PubMed ] [ Google Scholar ]
- Schroeder P. A., Dignath D., Janczyk M. (2018). Individual differences in uncertainty tolerance are not associated with cognitive control functions in the flanker task . Exp. Psychol. 65 , 245–256. 10.1027/1618-3169/a000408, PMID: [ PubMed ] [ CrossRef ] [ Google Scholar ]
- Schwarz N., Clore G. L. (1983). Mood, misattribution, and judgments of well-being: informative and directive functions of affective states . J. Pers. Soc. Psychol. 45 , 513–523. 10.1037/0022-3514.45.3.513 [ CrossRef ] [ Google Scholar ]
- Seligman M. E. P., Railton P., Baumeister R. F., Sripada C. (2013). Navigating into the future or driven by the past . Perspect. Psychol. Sci. 8 , 119–141. 10.1177/1745691612474317, PMID: [ PubMed ] [ CrossRef ] [ Google Scholar ]
- Shamionov R. M. (2017). Attitude to change and tolerance to uncertainty as predictors of adaptability and adaptive readiness . Russ. Psychol. J. 14 , 90–104. 10.21702/rpj.2017.2.5 [ CrossRef ] [ Google Scholar ]
- Shepard R. N., Metzler J. (1971). Mental rotation of three-dimensional objects abstract. The time required to recognize that two perspective drawings portray . Science 171 , 701–703. 10.1126/science.171.3972.701 [ PubMed ] [ CrossRef ] [ Google Scholar ]
- Shihata S., McEvoy P. M., Mullan B. A., Carleton R. N. (2016). Intolerance of uncertainty in emotional disorders: what uncertainties remain? J. Anxiety Disord. 41 , 115–124. 10.1016/j.janxdis.2016.05.001, PMID: [ PubMed ] [ CrossRef ] [ Google Scholar ]
- Siegrist M., Keller C., Kiers H. A. L. (2005). A new look at the psychometric paradigm of perception of hazards . Risk Anal. 25 , 211–222. 10.1111/j.0272-4332.2005.00580.x, PMID: [ PubMed ] [ CrossRef ] [ Google Scholar ]
- Slovic P., Finucane M. L., Peters E., MacGregor D. G. (2007). The affect heuristic . Eur. J. Oper. Res. 177 , 1333–1352. 10.1016/j.ejor.2005.04.006 [ CrossRef ] [ Google Scholar ]
- Smallwood J., Schooler J. W. (2015). The science of mind wandering: empirically navigating the stream of consciousness . Annu. Rev. Psychol. 66 , 487–518. 10.1146/annurev-psych-010814-015331, PMID: [ PubMed ] [ CrossRef ] [ Google Scholar ]
- Smithson M. (1989). Ignorance and uncertainty: Emerging paradigms . New York, NY, USA: Springer Verlag. [ Google Scholar ]
- Starkweather C. K., Gershman S. J., Uchida N. (2018). The medial prefrontal cortex shapes dopamine reward prediction errors under state uncertainty . Neuron 98 , 616–629.e6. 10.1016/j.neuron.2018.03.036 [ PMC free article ] [ PubMed ] [ CrossRef ] [ Google Scholar ]
- Sterling P. (2012). Allostasis: a model of predictive regulation . Physiol. Behav. 106 , 5–15. 10.1016/j.physbeh.2011.06.004, PMID: [ PubMed ] [ CrossRef ] [ Google Scholar ]
- Strack F., Schwarz N., Gschneidinger E. (1985). Happiness and reminiscing: the role of time perspective, affect, and mode of thinking . J. Pers. Soc. Psychol. 49 , 1460–1469. 10.1037/0022-3514.49.6.1460 [ CrossRef ] [ Google Scholar ]
- Strout T. D., Hillen M., Gutheil C., Anderson E. C., Hutchinson R., Ward H., et al.. (2018). Tolerance of uncertainty: a systematic review of health and healthcare-related outcomes . Patient Educ. Couns. 101 , 1518–1537. 10.1016/j.pec.2018.03.030, PMID: [ PubMed ] [ CrossRef ] [ Google Scholar ]
- Szpunar K. K., Spreng R. N., Schacter D. L. (2014). A taxonomy of prospection: introducing an organizational framework for future-oriented cognition . Proc. Natl. Acad. Sci. 111 , 18414–18421. 10.1073/pnas.1417144111, PMID: [ PMC free article ] [ PubMed ] [ CrossRef ] [ Google Scholar ]
- Tamietto M., Cauda F., Celeghin A., Diano M., Costa T., Cossa F. M., et al.. (2015). Once you feel it, you see it: insula and sensory-motor contribution to visual awareness for fearful bodies in parietal neglect . Cortex 62 , 56–72. 10.1016/j.cortex.2014.10.009, PMID: [ PubMed ] [ CrossRef ] [ Google Scholar ]
- Taylor S. E., Pham L. B., Rivkin I. D., Armor D. A. (1998). Harnessing the imagination: mental simulation, self-regulation, and coping . Am. Psychol. 53 , 429–439. 10.1037/0003-066X.53.4.429, PMID: [ PubMed ] [ CrossRef ] [ Google Scholar ]
- Taylor D., Picker B., Woolever D., Thayer E. K., Carney P. A., Galper A. B. (2018). A pilot study to address tolerance of uncertainty among family medicine residents . Fam. Med. 50 , 531–538. 10.22454/FamMed.2018.634768, PMID: [ PubMed ] [ CrossRef ] [ Google Scholar ]
- Tolin D. F., Abramowitz J. S., Brigidi B. D., Foa E. B. (2003). Intolerance of uncertainty in obsessive-compulsive disorder . J. Anxiety Disord. 17 , 233–242. 10.1016/S0887-6185(02)00182-2, PMID: [ PubMed ] [ CrossRef ] [ Google Scholar ]
- Tsui J. (1993). Tolerance for ambiguity, uncertainty audit qualifications and bankers’ perceptions . Psychol. Rep. 72 , 915–919. 10.2466/pr0.1993.72.3.915 [ CrossRef ] [ Google Scholar ]
- Tversky A., Kahneman D. (1981). The framing of decisions and the psychology of choice . Science 211 , 453–458. 10.1126/science.7455683, PMID: [ PubMed ] [ CrossRef ] [ Google Scholar ]
- van den Bos K. (2009). Making sense of life: the existential self trying to deal with personal uncertainty . Psychol. Inq. 20 , 197–217. 10.1080/10478400903333411 [ CrossRef ] [ Google Scholar ]
- van den Bos K., Poortvliet P. M., Maas M., Miedema J., van den Ham E. J. (2005). An enquiry concerning the principles of cultural norms and values: the impact of uncertainty and mortality salience on reactions to violations and bolstering of cultural worldviews . J. Exp. Soc. Psychol. 41 , 91–113. 10.1016/j.jesp.2004.06.001 [ CrossRef ] [ Google Scholar ]
- van Dijk E., Zeelenberg M. (2006). The dampening effect of uncertainty on positive and negative emotions . J. Behav. Decis. Mak. 176 , 171–176. 10.1002/bdm.504 [ CrossRef ] [ Google Scholar ]
- Vives M. L., Feldman Hall O. (2018). Tolerance to ambiguous uncertainty predicts prosocial behavior . Nat. Commun. 9 , 25–27. 10.1111/j.1474-919X.1989.tb02761.x [ PMC free article ] [ PubMed ] [ CrossRef ] [ Google Scholar ]
- Wilson T. D., Centerbar D. B., Kermer D. A., Gilbert D. T. (2005). The pleasures of uncertainty: prolonging positive moods in ways people do not anticipate . J. Pers. Soc. Psychol. 88 , 5–21. 10.1037/0022-3514.88.1.5, PMID: [ PubMed ] [ CrossRef ] [ Google Scholar ]
- Wilson T. D., Gilbert D. T. (2005). Affective forecasting: knowing what to want . Curr. Dir. Psychol. Sci. 14 , 131–134. 10.1111/j.0963-7214.2005.00355.x [ CrossRef ] [ Google Scholar ]
- Wright J., Mischel W. (1982). Influence of affect on cognitive social learning person variables . J. Pers. Soc. Psychol. 43 , 901–914. 10.1037/0022-3514.43.5.901 [ CrossRef ] [ Google Scholar ]
- Wundt W. (1998). Outlines of Psychology , C. H. Judd (trans.). Leipzig: Wilhelm Engelmann, translation of Grundriss der Psychologie, 1896. Available at: http://www.uni-leipzig.de/~psycho/wundt/opera/wundt/OLiPsych/OLiPsyIn.htm
- Zhang R., Brennan T. J., Lo A. W. (2014). The origin of risk aversion . Proc. Natl. Acad. Sci. 111 , 17777–17782. 10.1073/pnas.1406755111, PMID: [ PMC free article ] [ PubMed ] [ CrossRef ] [ Google Scholar ]
- Zillmann D. (1996) in LEA’s communication series. Suspense: Conceptualizations, theoretical analyses, and empirical explorations . eds. Vorderer P., Wulff H. J., Friedrichsen M. (Hillsdale, NJ, US: Lawrence Erlbaum Associates, Inc.). [ Google Scholar ]
Uncertain hypothesis test for uncertain differential equations
- Published: 03 June 2022
- Volume 22 , pages 195–211, ( 2023 )
Cite this article
- Tingqing Ye ORCID: orcid.org/0000-0001-8218-0305 1 &
- Baoding Liu 2
2886 Accesses
32 Citations
Explore all metrics
Uncertain hypothesis test is a statistical tool that uses uncertainty theory to determine whether some hypotheses are correct or not based on observed data. As an application of uncertain hypothesis test, this paper proposes a method to test whether an uncertain differential equation fits the observed data or not. In order to demonstrate the test method, some numerical examples are provided. Finally, both uncertain currency model and stochastic currency model are used to model US Dollar to Chinese Yuan (USD–CNY) exchange rates. As a result, it is shown that the uncertain currency model fits the exchange rates well, but the stochastic currency model does not.
Similar content being viewed by others
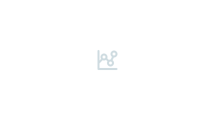
Parameter estimation of uncertain differential equations with estimating functions
Anshui Li & Yan Xia
Runge-Kutta Method for Solving Uncertain Differential Equations
Xiangfeng Yang & Yuanyuan Shen
Uncertain exponential currency model and currency option pricing
Xiaokang Li & Yuhong Sheng
Avoid common mistakes on your manuscript.
1 Introduction
Uncertainty theory, founded by Liu ( 2007 ) and perfected by Liu ( 2009 ), has been successfully applied to many fields, such as science, engineering, finance, environment, etc. Among the different applications, uncertain statistics was first introduced by Liu ( 2010 ) as a set of mathematical methods to collect, analyze and interpret data based on uncertainty theory. As a part of uncertain statistics, uncertain hypothesis test is a statistical tool based on uncertainty theory to determine whether some hypotheses are correct or not according to observed data. This work was initialized by Ye and Liu ( 2022 ). Following that, uncertain hypothesis test has been applied to other research areas of uncertain statistics, including uncertain regression analysis and uncertain time series analysis.
Uncertain regression analysis is used to explore the relationship between explanatory variables and response variables with uncertainty theory. Yao and Liu ( 2018 ) first proposed that the disturbance term of a regression model is not a random variable but an uncertain variable, which marks the beginning of the uncertain regression analysis. On this basis, Lio and Liu ( 2018 ) explored to estimate the uncertain disturbance term and obtained an interval estimation for predicting the response variables. In order to test whether an uncertain regression model is a good fit to observed data, Ye and Liu ( 2022 ) proposed a test method based on uncertain hypothesis test. As applications of uncertain regression analysis and uncertain hypothesis test, Liu ( 2021b ) used the uncertain growth model to study the number of COVID-19 infections in China, and Ye ( 2022a ) used the linear uncertain regression model to explore the relationship between labour income share and four influence factors, including trade openness, financial development, government intervention and industrial structure.
Uncertain time series analysis is used to predict future values via the previously observed values based on uncertainty theory. Yang and Liu ( 2019 ) first presented that the disturbance term of a time series model is an uncertain variable instead of a random variable, which marks the beginning of the uncertain time series analysis. In order to test whether an uncertain time series model is a good fit to observed data, Ye ( 2022b ) proposed a test method based on uncertain hypothesis test. As applications of uncertain time series analysis and uncertain hypothesis test, Ye and Yang ( 2021 ) studied the number of COVID-19 infections in China, Ye ( 2022c ) discussed the birth rates in China, and Ye ( 2022d ) investigated the grain yield in China.
As another part of uncertain statistics, uncertain differential equation (Liu 2008 ) is used to model the time evolution of a dynamic system. When using uncertain differential equation in practice, we first need to estimate unknown parameters in an uncertain differential equation to fit observed data as much as possible based on uncertainty theory. For that purpose, Yao and Liu ( 2020 ) first proposed the moment estimation based on the difference scheme of uncertain differential equation. Following that, Yang et al. ( 2020 ) presented the minimum cover estimation, Sheng et al. ( 2020 ) investigated the least squares estimation, and Liu and Liu ( 2022 ) introduced the maximum likelihood estimation. However, the above parameter estimation methods of uncertain differential equation based on difference scheme are not suitable for the case where the interval times between observations are not short enough. In order to deal with this problem, Liu and Liu ( 2022 ) presented the concept of residual to establish a connection between uncertain differential equation and observed data, and proposed a new method of parameter estimation in uncertain differential equation based on residuals. Up to now, uncertain differential equation has been widely applied in many fields such as finance (Liu 2013 ), chemical reaction (Tang and Yang 2021 ), electric circuit (Liu 2021a ), pharmacokinetics (Liu and Yang 2021 ), software reliability (Liu and Kang 2022 ), COVID-19 (Lio and Liu 2021 ), Alibaba stock (Liu and Liu 2022 ) and so on.
With the help of residuals, this paper employs uncertain hypothesis test to determine whether an uncertain differential equation is a good fit to the observed data. The rest of the paper is organized as follows. Section 2 introduces some basic knowledge about uncertain hypothesis test. After that, uncertain hypothesis test is used to determine whether a set of observed data follow a given linear uncertainty distribution in Sect. 3 . On this basis, Sect. 4 provides a method to test whether an uncertain differential equation fits the observed data, and gives two numerical examples to illustrate the test method. In Sect. 5 , uncertain currency model is applied to USD–CNY exchange rates. Then, some conclusions are made in Sect. 6 . Finally, as a comparison with uncertain currency model in Sect. 5 , stochastic currency model is also applied to USD–CNY exchange rates in the appendix, and the results show that the uncertain currency model fits the exchange rates well, but the stochastic currency model does not.
2 Preliminaries
This section will introduce some basic knowledge of uncertain hypothesis test. Let \(\xi \) be an uncertain variable with uncertainty distribution \(\Phi _{\theta }\) where \(\theta \) is an unknown parameter. Consider the following hypotheses:
where \(H_0\) is called a null hypothesis, and \(H_1\) is called an alternative hypothesis. Assume
are a set of observed data of the uncertain variable \(\xi \) . A rejection region for the null hypothesis \(H_0\) is a set \(W\subset \Re ^n\) . If the vector of observed data belongs to the rejection region W , i.e.,
then we reject \(H_0\) . Otherwise, we accept \(H_0\) . A core problem is how to choose a suitable rejection region W for the given hypothesis \(H_0\) .
Definition 1
(Ye and Liu 2022 ) Let \(\xi \) be a population with uncertainty distribution \(\Phi _{\theta }\) where \(\theta \) is an unknown parameter. A rejection region \(W\subset \Re ^n\) is said to be a test for the two-sided hypotheses \(H_0: \theta =\theta _0\) versus \(H_1: \theta \ne \theta _0\) at significance level \(\alpha \) if
for any \((z_1,z_2,\ldots ,z_n)\in W\) , there are at least \(\alpha \) of indexes i ’s with \(1\le i\le n\) such that

for some \(\theta \ne \theta _0\) and some \((z_1,z_2,\ldots ,z_n)\in W\) , there are more than \(1-\alpha \) of indexes i ’s with \(1\le i\le n\) and at least \(\alpha \) of indexes j ’s with \(1\le j\le n\) such that

In order to test whether a normal uncertainty distribution \(\mathcal {N}(e_0,\sigma _0)\) is a good fit to a set of observed data \(z_1,z_2,\ldots ,z_n\) , Ye and Liu ( 2022 ) proved by Definition 1 that a test at significance level \(\alpha \) is
where \(\Phi _0^{-1}\) is the inverse uncertainty distribution of \(\mathcal {N}(e_0,\sigma _0)\) , i.e.,
If the vector of observed data belongs to the test W , i.e.,
then the normal uncertainty distribution \(\mathcal {N}(e_0,\sigma _0)\) is not a good fit to the observed data. Otherwise, the normal uncertainty distribution \(\mathcal {N}(e_0,\sigma _0)\) is a good fit to the observed data.
3 Uncertain hypothesis test for linear uncertainty distribution
In this section, we would like to use the uncertain hypothesis test to determine whether a set of observed data follow a given linear uncertainty distribution.
Let \(\xi \) be an uncertain variable that follows a linear uncertainty distribution \(\mathcal {L}(a,b)\) with unknown parameters a and b . Then the test for the hypotheses
at significance level \(\alpha \) is
where \(\Phi _0^{-1}\) is the inverse uncertainty distribution of \(\mathcal {L}(a_0,b_0)\) , i.e.,
In order to prove that W is a test, we need to verify that W simultaneously meets Conditions (i) and (ii) in Definition 1 . For any \((z_1,z_2,\ldots ,z_n)\in W\) , it follows from the definition of W that there are at least \(\alpha \) of indexes i ’s with \(1\le i\le n\) such that

Thus W meets Condition (i) in Definition 1 .
In order to prove Condition (ii), we take
It is clear that \((z_1,z_2,\ldots ,z_n)\in W\) . Let \(\Phi _1\) denote the uncertainty distribution of \(\mathcal {L}(a_1,b_1)\) . On the one hand, we have

\(i=1,2,\ldots ,n\) . Thus

On the other hand, we have

\(i,j=1,2,\ldots ,n\) . Therefore W meets Condition (ii) in Definition 1 . \(\square \)
Let us use uncertain hypothesis test to determine whether the linear uncertainty distribution \(\mathcal {L}(0,1)\) fits the 30 observed data
plotted in Fig. 1 .
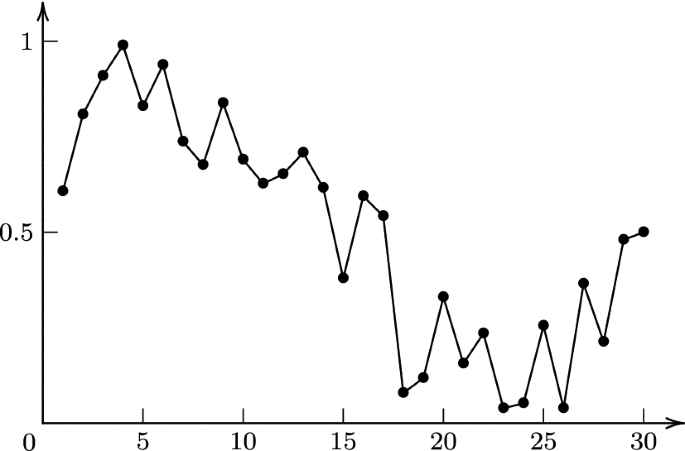
Observed data of the linear uncertainty distribution \(\mathcal {L}(0,1)\) in chronological order
Suppose \(\Phi ^{-1}\) is the inverse uncertainty distribution of \(\mathcal {L}(0,1)\) , i.e.,
Given a significance level \(\alpha =0.05\) , we obtain
It follows from \(\alpha \times 30=1.5\) and Theorem 1 that the test is
Since only the 4th observed datum \(0.989\notin [0.025,0.975]\) , the vector of observed data does not belong to W . Thus the linear uncertainty distribution \(\mathcal {L}(0,1)\) is a good fit to the observed data.
Let us use uncertain hypothesis test to determine whether the linear uncertainty distribution \(\mathcal {L}(5,6)\) fits the 30 observed data
plotted in Fig. 2 .
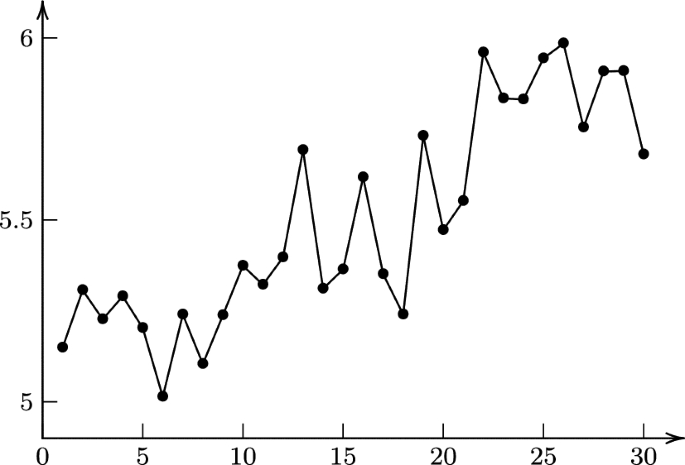
Observed data of the linear uncertainty distribution \(\mathcal {L}(5,6)\) in chronological order
Suppose \(\Phi ^{-1}\) is the inverse uncertainty distribution of \(\mathcal {L}(5,6)\) , i.e.,
Since the 6th and 26th observed data \(5.014,5.985\notin [5.025,5.975]\) , the vector of observed data belongs to W . Thus the linear uncertainty distribution \(\mathcal {L}(5,6)\) is not a good fit to the observed data.
4 Uncertain differential equation
In this section, we would like to employ the uncertain hypothesis test to determine whether an uncertain differential equation fits the observed data well. Consider an uncertain differential equation
where f and g are known continuous functions, and \(C_t\) is a Liu process. Assume
are observed values of some uncertain process \(X_t\) at times \(t_1,t_2,\ldots ,t_n\) with \(t_1<t_2<\cdots <t_n\) , respectively. For each index i ( \(2\le i\le n\) ), let \(X_{t_i}\) be the solution of the updated uncertain differential equation
at time \(t_i\) . Denote the uncertainty distribution of \(X_{t_i}\) by \(\Phi _{t_i}\) . It is clear that
is a linear uncertain variable \(\mathcal {L}(0,1)\) whose uncertainty distribution is
and inverse uncertainty distribution is
Then, Liu and Liu ( 2022 ) called
the i th residual of the uncertain differential equation ( 1 ) corresponding to the observed data ( 2 ) by substituting \(X_{t_i}\) with the observed value \(x_{t_i}\) in ( 4 ). Thus the residual \(\varepsilon _i\) may be regraded as a sample of the linear uncertainty distribution \(\mathcal {L}(0,1)\) .
If the uncertain differential equation ( 1 ) does fit the observed data ( 2 ) well, then the \(n-1\) residuals \(\varepsilon _2,\varepsilon _3,\ldots ,\varepsilon _n\) should follow the linear uncertainty distribution \(\mathcal {L}(0,1)\) , i.e.,
Thus, to test whether the uncertain differential equation ( 1 ) fits the observed data ( 2 ) well, we should test whether the linear uncertainty distribution \(\mathcal {L}(0,1)\) fits the residuals \(\varepsilon _2,\varepsilon _3,\ldots ,\varepsilon _n\) defined in ( 5 ), i.e.,
To do so, it follows from Theorem 1 that the test at a given significance level \(\alpha \) (e.g. 0.05) is
If the vector of the \(n-1\) residuals \(\varepsilon _2,\varepsilon _3,\ldots ,\varepsilon _n\) belongs to the test W , i.e.,
then the uncertain differential equation ( 1 ) is not a good fit to the observed data ( 2 ). If
then the uncertain differential equation ( 1 ) is a good fit to the observed data ( 2 ).
Let us employ the uncertain hypothesis test to determine whether the uncertain differential equation
fits the 30 observed data in Table 1 on the time horizon from 0 to 2.19.
In Table 1 , denote the observed values of \(X_t\) at times \(t_1,t_2,\ldots ,t_{30}\) by
respectively. For each index i ( \(2\le i\le 30\) ), we solve the updated uncertain differential equation
and obtain the uncertainty distribution of \(X_{t_i}\) as follows,
It follows from ( 5 ) that the i th residual is
See Fig. 3 . In order to test whether the uncertain differential equation ( 6 ) fits the observed data well, we should test whether the linear uncertainty distribution \(\mathcal {L}(0,1)\) fits the 29 residuals \(\varepsilon _2,\varepsilon _3,\ldots ,\varepsilon _{30}\) . Given a significance level \(\alpha =0.05\) , it follows from \(\alpha \times 29=1.45\) and Theorem 1 that the test is
Since only \(\varepsilon _{21}=0.983\notin [0.025,0.975]\) , we have \((\varepsilon _2,\varepsilon _3,\ldots ,\varepsilon _{30})\notin W\) . Thus the uncertain differential equation ( 6 ) is a good fit to the observed data.
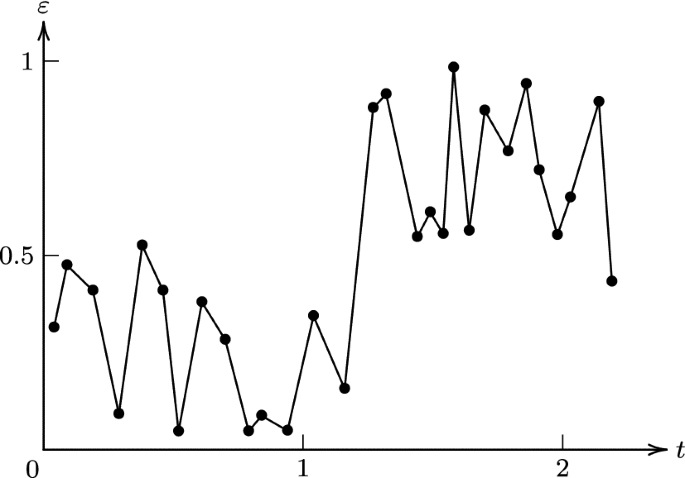
Residual plot of uncertain differential equation ( 6 )
fits the 30 observed data in Table 2 on the time horizon from 0 to 9.5.
In Table 2 , denote the observed values of \(X_t\) at times \(t_1,t_2,\ldots ,t_{30}\) by
respectively. For each i ( \(2\le i\le 30\) ), we can calculate the residual \(\varepsilon _i\) of the updated uncertain differential equation
with the help of an algorithm proposed by Liu and Liu ( 2022 ). See Fig. 4 . In order to test whether the uncertain differential equation ( 7 ) fits the observed data well, we should test whether the linear uncertainty distribution \(\mathcal {L}(0,1)\) fits the 29 residuals
Given a significance level \(\alpha =0.05\) , it follows from \(\alpha \times 29=1.45\) and Theorem 1 that the test is
Since only \(\varepsilon _{10}=0.987\notin [0.025,0.975]\) , we have \((\varepsilon _2,\varepsilon _3,\ldots ,\varepsilon _{30})\notin W\) . Thus the uncertain differential equation ( 7 ) is a good fit to the observed data.
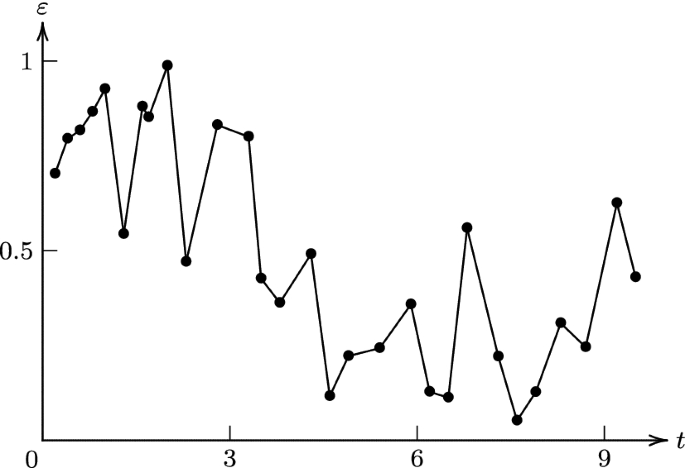
Residual plot of uncertain differential equation ( 7 )
5 Uncertain currency model
Table 3 shows US Dollar to Chinese Yuan (USD–CNY) exchange rates (weekly average) in Forex Capital Markets (FXCM) from October 2019 to June 2021, which are plotted in Fig. 5 .
Let \(i=1,2,\ldots ,91\) represent the weeks from October 1, 2019 to June 30, 2021, and denote the exchange rates by
Assume the exchange rate \(X_t\) follows the uncertain differential equation
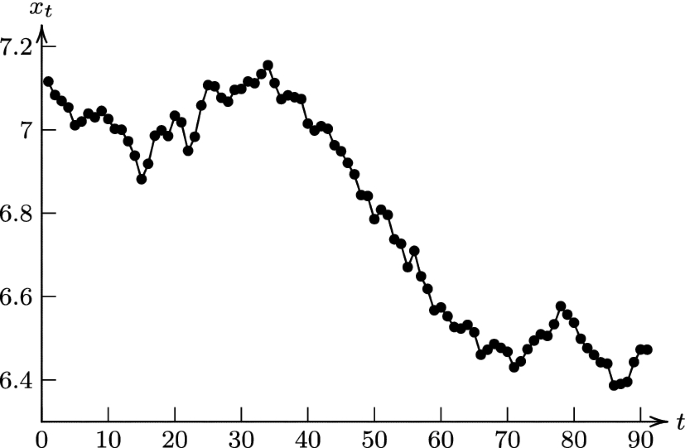
USD–CNY exchange rates (weekly average) from October 1, 2019 to June 30, 2021
where m , a and \(\sigma \) are unknown parameters to be estimated, and \(C_t\) is a Liu process. Then, Liu and Liu ( 2022 ) suggested that the moment estimate \((m, a,\sigma )\) is the solution of the system of equations,
\(i=2,3,\ldots ,91\) are residuals of the uncertain differential equation ( 8 ). Solving the above system of equations, we get
Thus we obtain an uncertain currency model
where \(X_t\) represents the exchange rate.
Finally, let us test whether the uncertain currency model ( 9 ) fits USD–CNY exchange rates. That is, we should test whether the linear uncertainty distribution \(\mathcal {L}(0,1)\) fits the 90 residuals
See Fig. 6 .
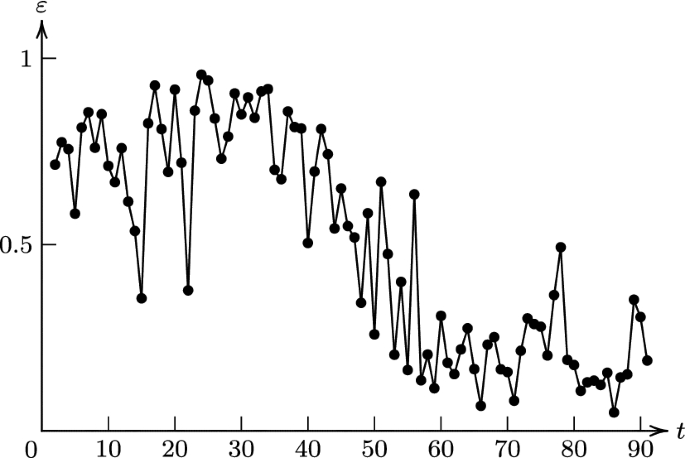
Residual plot of the uncertain currency model ( 9 ) corresponding to USD–CNY exchange rates
Given a significance level \(\alpha =0.05\) , it follows from \(\alpha \times 90=4.5\) and Theorem 1 that the test is
Since all residuals \(\varepsilon _i\) , \(i=2,3,\ldots ,91\) are between 0.025 and 0.975, we have
Thus the uncertain currency model ( 9 ) is a good fit to USD–CNY exchange rates.
6 Conclusion
In order to test whether an uncertain differential equation fits the observed data well, this paper presented the uncertain hypothesis test to determine whether the uncertain differential equation is a good fit to the observed data by testing whether the residuals of the uncertain differential equation follow the linear uncertainty distribution \(\mathcal {L}(0,1)\) . Then, some numerical examples were given to demonstrate the test method. Finally, both uncertain currency model and stochastic currency model were applied to USD–CNY exchange rates. The results showed that the uncertain currency model fits the exchange rates well, but the stochastic currency model does not.
Lio, W., & Liu, B. (2018). Residual and confidence interval for uncertain regression model with imprecise observations. Journal of Intelligent & Fuzzy Systems, 35 (2), 2573–2583.
Article Google Scholar
Lio, W., & Liu, B. (2021). Initial value estimation of uncertain differential equations and zero-day of COVID-19 spread in China. Fuzzy Optimization and Decision Making, 20 (2), 177–188.
Article MathSciNet MATH Google Scholar
Liu, B. (2007). Uncertainty Theory (2nd ed.). Springer.
Liu, B. (2008). Fuzzy process, hybrid process and uncertain process. Journal of Uncertain Systems, 2 (1), 3–16.
Google Scholar
Liu, B. (2009). Some research problems in uncertainty theory. Journal of Uncertain Systems, 3 (1), 3–10.
MathSciNet Google Scholar
Liu, B. (2010). Uncertainty theory: A branch of mathematics for modeling human uncertainty . Springer.
Liu, B. (2013). Toward uncertain finance theory. Journal of Uncertainty Analysis and Applications, 1 , 1.
Liu, Y. (2021a). Uncertain circuit equation. Journal of Uncertain Systems, 14 (3), 2150018.
Liu, Y., & Liu, B. (2022). Estimating unknown parameters in uncertain differential equation by maximum likelihood estimation. Soft Computing, 26 (6), 2773–2780.
Liu, Y., & Liu, B. (2022). Residual analysis and parameter estimation of uncertain differential equation. Fuzzy Optimization and Decision Making . https://doi.org/10.1007/s10700-021-09379-4 .
Liu, Z. (2021b). Uncertain growth model for the cumulative number of COVID-19 infections in China. Fuzzy Optimization and Decision Making, 20 (2), 229–242.
Liu, Z., & Yang, Y. (2021). Uncertain pharmacokinetic model based on uncertain differential equation. Applied Mathematics and Computation, 404 , 126118.
Liu, Z., & Kang, R. (2022). Software belief reliability growth model based on uncertain differential equation. IEEE Transactions on Reliability . https://doi.org/10.1109/TR.2022.3154770 .
Sheng, Y., Yao, K., & Chen, X. (2020). Least squares estimation in uncertain differential equations. IEEE Transactions on Fuzzy Systems, 28 (10), 2651–2655.
Tang, H., & Yang, X. (2021). Uncertain chemical reaction equation. Applied Mathematics and Computation, 411 , 126479.
Yang, X., & Liu, B. (2019). Uncertain time series analysis with imprecise observations. Fuzzy Optimization and Decision Making, 18 (3), 263–278.
Yang, X., Liu, Y., & Park, G. (2020). Parameter estimation of uncertain differential equation with application to financial market. Chaos, Solitons and Fractals, 139 , 110026.
Yao, K., & Liu, B. (2018). Uncertain regression analysis: An approach for imprecise observations. Soft Computing, 22 (17), 5579–5582.
Article MATH Google Scholar
Yao, K., & Liu, B. (2020). Parameter estimation in uncertain differential equations. Fuzzy Optimization and Decision Making, 19 (1), 1–12.
Ye, T., & Liu, B. (2022). Uncertain hypothesis test with application to uncertain regression analysis. Fuzzy Optimization and Decision Making, 21, 157–174.
Ye, T., & Yang, X. (2021). Analysis and prediction of confirmed COVID-19 cases in China with uncertain time series. Fuzzy Optimization and Decision Making, 20 (2), 209–228.
Article MathSciNet Google Scholar
Ye, T., & Zheng, H. (2022). Analysis of birth rates in China with uncertain time series model . Technical report.
Ye, T. (2022). Analysis of labour income share in China with uncertain regression model. Technical report.
Ye, T. (2022). Difference order selection in uncertain time series analysis. Technical Report.
Ye, T. (2022). Modeling grain yield in China with uncertain time series analysis. Technical report.
Download references
Acknowledgements
This work was supported by the National Natural Science Foundation of China Grant Nos.61873329 and 12026225.
Author information
Authors and affiliations.
School of Reliability and Systems Engineering, Beihang University, Beijing, 100191, China
Tingqing Ye
Department of Mathematical Sciences, Tsinghua University, Beijing, 100084, China
Baoding Liu
You can also search for this author in PubMed Google Scholar
Corresponding author
Correspondence to Baoding Liu .
Additional information
Publisher's note.
Springer Nature remains neutral with regard to jurisdictional claims in published maps and institutional affiliations.
Appendix: Stochastic currency model
Let us reconsider USD–CNY exchange rates (weekly average) from October 1, 2019 to June 30, 2021 in Example 5 . See Table 3 . Let \(i=1,2,\ldots ,91\) represent the weeks from October 1, 2019 to June 30, 2021, and denote the exchange rates by
Assume the exchange rate \(X_t\) follows the stochastic differential equation
where m , a and \(\sigma \) are unknown parameters to be estimated, and \(W_t\) is a Wiener process. For any fixed parameters \(m, a, \sigma \) and index i \((2\le i\le 91)\) , we solve the updated stochastic differential equation
and find that \(X_i\) is a normal random variable with expected value
and variance
Thus the probability distribution of the normal random variable \(X_i\) is
and \(\Phi _i(X_i)\) is always a uniform random variable \(\mathcal {U}(0,1)\) since
for any x with \(0<x<1\) . Substitute \(X_i\) with the corresponding observed value \(x_i\) , and write
Then \(\varepsilon _i(m,a,\sigma )\) is always a sample of uniform probability distribution \(\mathcal {U}(0,1)\) and called the i th residual of the stochastic differential equation ( 10 ). For each positive integer k , the k th sample moment of the 90 residuals \(\varepsilon _2(m,a,\sigma ),\varepsilon _3(m,a,\sigma ),\ldots ,\) \(\varepsilon _{91}(m,a,\sigma )\) is
and the k -th population moment of the uniform probability distribution \(\mathcal {U}(0,1)\) is
Since the number of unknown parameters in the stochastic differential equation ( 10 ) is 3, the moment estimate \((m,a,\sigma )\) is obtained by equating the first 3 sample moments to the corresponding first 3 population moments. In other words, the moment estimate \((m,a,\sigma )\) should solve the system of equations,
whose root is
Thus we obtain a stochastic currency model
where \(X_t\) represents exchange rate.
Does the stochastic currency model ( 11 ) fit USD–CNY exchange rates? In order to answer this question, let us consider the 90 residuals
See Fig. 7 . If the stochastic currency model ( 11 ) fits USD–CNY exchange rates well, then the residuals in Fig. 7 should be from the same population in the sense of probability theory. However, when we try to divide those residuals into two parts, i.e., the first half and the last half, and use two-sample Kolmogorov-Smirnov test to determine whether the residuals of these two parts are from the same population, we get the p -value of \(5\times 10^{-16}\) via the function “kstest2” in Matlab. This means the residuals neither come from the same population nor are white noise in the sense of probability theory, let alone follow the uniform probability distribution \(\mathcal {U}(0,1)\) . Thus the stochastic currency model ( 11 ) does not fit USD–CNY exchange rates. Furthermore, we cannot find a better stochastic differential equation to fit the USD–CNY exchange rates. This is the reason why we use uncertain differential equation to model currency exchange rate rather than stochastic differential equation.
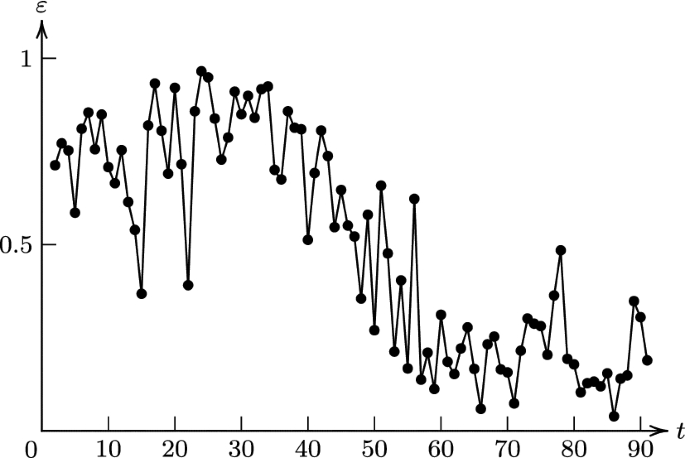
Residual plot of stochastic currency model ( 11 ) corresponding to USD–CNY exchange rates
Rights and permissions
Reprints and permissions
About this article
Ye, T., Liu, B. Uncertain hypothesis test for uncertain differential equations. Fuzzy Optim Decis Making 22 , 195–211 (2023). https://doi.org/10.1007/s10700-022-09389-w
Download citation
Accepted : 31 March 2022
Published : 03 June 2022
Issue Date : June 2023
DOI : https://doi.org/10.1007/s10700-022-09389-w
Share this article
Anyone you share the following link with will be able to read this content:
Sorry, a shareable link is not currently available for this article.
Provided by the Springer Nature SharedIt content-sharing initiative
- Uncertainty theory
- Uncertain statistics
- Uncertain hypothesis test
- Uncertain differential equation
- Uncertain currency model
- Find a journal
- Publish with us
- Track your research
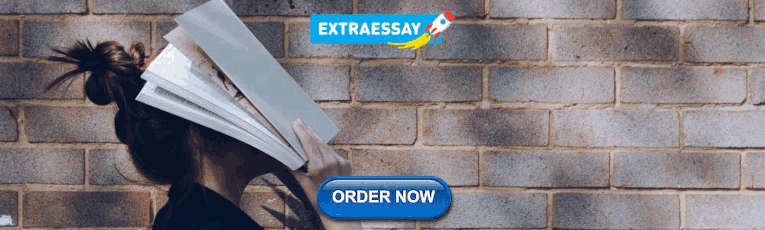
IMAGES
COMMENTS
The Uncertainty Principle. Quantum mechanics is generally regarded as the physical theory that is our best candidate for a fundamental and universal description of the physical world. The conceptual framework employed by this theory differs drastically from that of classical physics. Indeed, the transition from classical to quantum physics ...
Lab 1: Uncertainty and Confirmation of Hypotheses. An exploration of the basic tenets of experimental science using a specific example, with particular emphasis on uncertainty and the role it plays in confirming an hypothesis.
indicating that the uncertainty of this measurement is 0:2s or about 3%. Accuracy and Precision An important distinction in physics is the di erence between the accuracy and the precision of a measurement. Accuracy refers to the closeness of a measured value to a standard or known value. For example, if in lab you obtain a weight measurement
Direct uncertainty about a fact, number or scientific hypothesis is the type of uncertainty which can be the most precisely expressed and therefore lends itself to the widest possible range of forms of expression. In figure 2, we list these forms, in order of their decreasing precision (capability of expressing detail of magnitude).
What does uncertainty mean for scientists? There are different types of uncertainty that are an ordinary part of scientific research. These can be addressed or taken into account in several ways. Scientific researchers have to work out whether uncertainty can be calculated and how to do so, and then whether it matters and what can be done about it.
So 1300 could have two, three, or four significant figures. To avoid this ambiguity, we should write 1300 in scientific notation as 1.3 x 10 3, 1.30 x 10 3, or 1.300 x 10 3, depending on whether it has two, three, or four significant figures. Zeros are significant except when they serve only as placeholders.
Heisenberg's uncertainty principle is a key principle in quantum mechanics. Very roughly, it states that if we know everything about where a particle is located (the uncertainty of position is small), we know nothing about its momentum (the uncertainty of momentum is large), and vice versa. Versions of the uncertainty principle also exist for ...
This is in contrast to the definition of uncertainty often found in science, and discussed in our introduction (Section 1), where it is classified as arising due to the natural stochastic uncertainty (variability of the system, aleatoric), or the epistemic uncertainty (unknowns, lack of knowledge, imperfect knowledge) (see reviews in Refs.
First, appreciating uncertainty in science helps one to avoid being dogmatically certain. Good science is always open to revision in the face of new evidence. Second, appreciating uncertainty in science allows one to avoid the mistaken view that all theories and ideas are equally plausible. Third, since uncertainty is an inherent feature of ...
5 USES OF UNCERTAINTY ANALYSIS (II) • Provide the only known basis for deciding whether: - Data agrees with theory - Tests from different facilities (jet engine performance) agree - Hypothesis has been appropriately assessed (resolved) - Phenomena measured are real • Provide basis for defining whether a closure check has been achieved - Is continuity satisfied (does the same ...
Main elements of uncertainty analysis. Uncertainty analysis is the process of identifying and characterising uncertainty about questions of interest and/or quantities of interest in a scientific assessment. A question or quantity of interest may be the subject of the assessment as a whole, i.e. that which is required by the Terms of Reference ...
Social uncertainty creates a motivation for scientists to provide more evidence and improve the quality of evidence to resolve epistemic disagreement. Once social uncertainty is solved, only then can scientific knowledge be established. ... Therefore, this new definition of scientific literacy and goals pf learning science can reconceptualize ...
Uncertainty theory is a branch of mathematics based on normality, monotonicity, self-duality, countable subadditivity, and product measure axioms. [clarification needed] Mathematical measures of the likelihood of an event being true include probability theory, capacity, fuzzy logic, possibility, and credibility, as well as uncertainty.
The notion of uncertainty is of major importance in machine learning and constitutes a key element of machine learning methodology. In line with the statistical tradition, uncertainty has long been perceived as almost synonymous with standard probability and probabilistic predictions. Yet, due to the steadily increasing relevance of machine learning for practical applications and related ...
To summarize, to this day there remains a significant lack of agreement on what uncertainty is and how to model it in theory and in practice (Townsend et al., 2018).There are as many conceptualizations of uncertainty as treatments of it (Lipshitz & Strauss, 1997).Alternative theories use the same language of uncertainty, although different types of uncertainty are implicated, and starkly ...
Paternity uncertainty hypothesis is a construct in behavioral ecology and evolutionary psychology, used to explain differential investment in offspring between the sexes. The hypothesis states that due to the nature of internal fertilization, a male inseminator cannot be fully assured (consciously or otherwise) of the genetic identity of what ...
The uncertainty principle is alternatively expressed in terms of a particle's momentum and position. The momentum of a particle is equal to the product of its mass times its velocity. Thus, the product of the uncertainties in the momentum and the position of a particle equals h/(4π) or more.The principle applies to other related (conjugate) pairs of observables, such as energy and time: the ...
This paper introduces the Knightian Uncertainty Hypothesis (KUH), a new approach to macroeconomics and finance theory. KUH rests on a novel mathematical framework that characterizes both measurable and Knightian uncertainty about economic outcomes. Relying on this framework and John Muth's pathbreaking hypothesis, KUH represents participants'
1: Uncertainty and Confirmation of Hypotheses. Licensing. 1.1: Background Material. Tom Weideman. University of California, Davis. 1.1: Background Material. Our knowledge of position vectors allows us to define average position, and from there we define uncertainty in position. 1.2: Activities. We practice taking and analyzing data, and learn ...
Heisenberg's uncertainty principle states that it is impossible to measure or calculate exactly both the position and the momentum of an object. This principle is based on the wave-particle duality of matter. Although Heisenberg's uncertainty principle can be ignored in the macroscopic world (the uncertainties in the position and velocity of objects with relatively large masses are ...
This suggestion was built upon a proposed contemporary definition for intolerance of uncertainty as, "an individual's dispositional incapacity to endure the aversive response triggered by the perceived absence of ... According to this hypothesis, uncertainty causes negative affect to become more negative and positive affect to become more ...
This paper studies the effect of outcome uncertainty (OU) on fan attendance in sports. First, it develops a theoretical model of fan attendance in which fans derive utilities from match quality and team quality. The theoretical results suggest that empirical models should control for the effect of team quality ("standard model") to identify the effect of OU or include a cubic term of win ...
Uncertain hypothesis test is a statistical tool that uses uncertainty theory to determine whether some hypotheses are correct or not based on observed data. As an application of uncertain hypothesis test, this paper proposes a method to test whether an uncertain differential equation fits the observed data or not. In order to demonstrate the test method, some numerical examples are provided ...