- Weight Loss
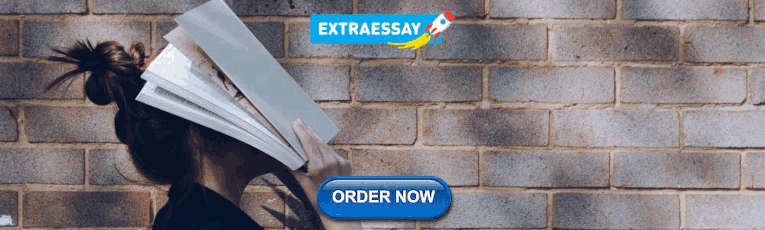
This Is How Much Weight People Have Lost — You Have to See This Visual!
Updated on 7/29/2017 at 7:45 PM
:upscale()/2017/07/25/925/n/1922729/e69571dd5977b45c062292.49362896_edit_img_cover_file_43771524_1500583793.jpg)
When you throw around a number, like "I've lost 20 pounds," or "I've dropped 50 pounds," it's hard to visualize exactly what that means. Check out these Instagram photos showing the equivalent of pounds lost compared to basic everyday objects. This is a great idea for staying motivated, to celebrate your victories, and to keep you inspired to reach your weight-loss goals.
Weight in Dog Food = 102 Pounds
Weight in dumbbells = 60 pounds, weight in cinder blocks = 90 pounds, weight in water jugs = 32 pounds, weight in a microwave = 36 pounds, weight in medicine balls = 94 pounds, weight in a tire = 21 pounds, weight in dog food = 60 pounds.
VisualBMI shows you what weight looks like on a human body. Using a large index of photos of men and women, you can get sense of what people look like at different weights or even the same weight. If you find this website useful, please share it or send a note.
Huge thanks to all those who share their stories and photos on reddit. This website would not be possible without them. These images are neither hosted by or affiliated with VisualBMI. These images were indexed from reddit posts that link to imgur. The subjects of these photos are not involved with VisualBMI in any way.
If this site was useful, bookmark and share with your friends.
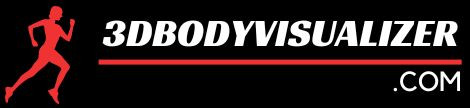
3D Body Visualizer
We know that no amount of reassurance can ever be enough for your body, weight, or height. This is why we brought you a solution enabling you to see yourself and judge your looks without overthinking or self-doubting. Whether you are a man or a woman, our tool can help you set personal goals, compare progress, and help you understand your fitness journey.
Through the power of visualization, our interactive 3D human modeling simulator allows you to create personalized virtual representations of yourself. You can fine-tune your height, weight, gender, and shape; your on-screen figure will update to represent the details you’ve entered.
Our website is the perfect tool for those looking to gain or lose weight. You can see how you appear in real life or experiment with different visual features, such as muscle definition or fat. Don’t want to become too muscular? See what you would look like after gaining more muscles. Don’t want to become too fat? Well, you can check that out too! Everything is baked right into our user-friendly interface.
How To Use 3D Visualizer
In our tool’s user-friendly interface, you will see different factors and measurements that you can modify about your 3D character. Above the character, you’ll see two options. The first one lets you modify the gender between Male, Female, and Trained Male. The second option lets you change the measuring units between CM/KG and In/LBS.
You can find the most important buttons at the bottom of the screen, where you will see sliders to input your weight and height. The sliders are handy because they allow you to gradually update both factors and see how your appearance is affected by the slow change. It is important to note that we have designed these factors to change with each other by default, as they do in real life. However, you can change this by fixing one of the factors in place.
Pressing the “Fixed” button on the right on either height or weight allows you to freeze it while you freely change the other. Now, changing one factor won’t affect the other.
How Accurate Is the Body Visualizer?
We aim to create the most accurate visualizer on the internet, enabling our users to see only the most accurate image of themselves. We have designed our 3D Visualization tool to give approximate visualization based on average body parameters.
How We Plan To Improve
We are always trying and planning to improve our Visualizer by adding more features. So far, we have successfully added some of our planned features, while others are still in the works, including leg length, hip length, chest length, waist length, breast size, and muscularity. The addition of more factors allows you to get a better representation of your body.
So, try out our 3D Body Visualizer and let us know if you found it useful!
3.1/5 - (294 votes)
15 thoughts on “3D Body Visualizer”
I love this new site. I like the way you show animated images instead of just static images. I assume this is a work in progress, so I have a few suggestions. I’d like to see in addition to the basic height and weight entries options for basic measurements such as chest (bust), waist and hips. Also, I’d like to see an option for including in the same image multiple subjects (for example, male and female together or up to five subjects in the same image.
I agree it sounds ike good suggestions
Nice good for visualising how I will look after weight loss
Can you add any more features? Like chest size, waist size, bicep size, etc.?
I agree with the previous comments; there needs to be measurements for various parts of the body. On top of that, I’d like to see more definition in the human anatomy. For example, if you were to scale down the weight to lower levels, you would see the ribs and hip bones become more visible. Or perhaps you could add a scale to increase muscle tone, as two people with a similar body mass would look fairly different from each other if one worked out regularly and one didn’t. Overall, I enjoy what you’re build here, but I feel like you could add a bit more realism and variation to the final product
the weight it not accurate at all. it looks 25 pounds heavier than it should be.
It would be good to put in some variables for skeletal structure, particularly thngs like leg length – the model looks nothing like me, if my legs were proportioned similarly I’d be 25-30 cm taller! As for shoulders/ribcage – some of us are almost as wide as we’re tall!
Its fun but add customization
I know its bot going to be accurate, but i dont use this for my own body. I make characters and i wanna know how they’d look, and it’s been good for me so far.
Great idea but what is wrong with your models anatomy, at least stop it looking like it is drunk and please try for a regular upright pose rather than hips thrust forward and shoulders so far back it looks painful.
would like to see some customization waist size, age etc.
Leave a Comment Cancel reply
Save my name, email, and website in this browser for the next time I comment.
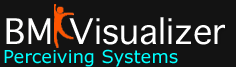
- CALCULATE BMI
- NON-WEBGL BMI
- Enable WebGL
Calculate your BMI and Visualize your Body Shape
- © 2013, Copyright Max Planck Gesellschaft
- Privacy Policy
- Terms of Use
- Open access
- Published: 18 April 2017
Do images of a personalised future body shape help with weight loss? A randomised controlled study
- Gemma Ossolinski 1 ,
- Moyez Jiwa 2 ,
- Alexandra McManus 3 &
- Richard Parsons 4
Trials volume 18 , Article number: 180 ( 2017 ) Cite this article
3170 Accesses
11 Citations
6 Altmetric
Metrics details
This randomised controlled study evaluated a computer-generated future self-image as a personalised, visual motivational tool for weight loss in adults.
One hundred and forty-five people (age 18–79 years) with a Body Mass Index (BMI) of at least 25 kg/m 2 were randomised to receive a hard copy future self-image at recruitment (early image) or after 8 weeks (delayed image). Participants received general healthy lifestyle information at recruitment and were weighed at 4-weekly intervals for 24 weeks. The image was created using an iPad app called ‘Future Me’. A second randomisation at 16 weeks allocated either an additional future self-image or no additional image.
Seventy-four participants were allocated to receive their image at commencement, and 71 to the delayed-image group. Regarding to weight loss, the delayed-image group did consistently better in all analyses. Twenty-four recruits were deemed non-starters, comprising 15 (21%) in the delayed-image group and 9 (12%) in the early-image group (χ 2 (1) = 2.1, p = 0.15). At 24 weeks there was a significant change in weight overall ( p < 0.0001), and a difference in rate of change between groups (delayed-image group: −0.60 kg, early-image group: −0.42 kg, p = 0.01). Men lost weight faster than women. The group into which participants were allocated at week 16 (second image or not) appeared not to influence the outcome ( p = 0.31). Analysis of all completers and withdrawals showed a strong trend over time ( p < 0.0001), and a difference in rate of change between groups (delayed-image: −0.50 kg, early-image: −0.27 kg, p = 0.0008).
One in five participants in the delayed-image group completing the 24-week intervention achieved a clinically significant weight loss, having received only future self-images and general lifestyle advice. Timing the provision of future self-images appears to be significant, and promising for future research to clarify their efficacy.
Trial Registration
Australian Clinical Trials Registry, identifier: ACTRN12613000883718 . Registered on 8 August 2013.
Peer Review reports
The prevalence of obesity in developed regions has risen at an alarming rate over the past 30 years, and continues to rise despite public awareness of the associated risk of chronic disease [ 1 ]. Outside of surgical intervention, weight loss for the individual relies on lifestyle change that promotes an overall reduction of dietary caloric intake and an increase in physical energy expenditure. Being aware of the need to lose weight is usually not enough to produce sustained lifestyle change. The individual requires the initial motivation to change unhealthy dietary habits and to revise entrenched sedentary behaviours, followed by continued effort to sustain the healthy lifestyle choices.
Health professionals are often counselled for weight loss, either in response to a direct request from an individual or because of an associated illness that requires medical attention. Weight-loss counselling in the general practice setting often takes place in time-constrained consultations [ 2 , 3 ]. In addition, a lack of patient motivation and failure to adhere to recommendations are often cited as a barrier to effective weight-loss intervention [ 4 ].
New technology, in the form of digital self-representations, known as Avatars, have been shown to improve health-related behaviours, including diet and exercise choices [ 5 , 6 ]. Written advice that is tailored and personalised for the individual has been shown to be more effective than traditional collective advice for diet and exercise behaviour change [ 7 , 8 ]. Empirical evidence from a pilot study published in 2015 suggests that self-representations in the form of computerised future self-images could enhance weight loss in women who are trying to lose weight [ 9 ]. This is consistent with the theory that a fundamental human need is to forge or maintain bonds; therefore, the prospect of a more appealing physical appearance may be a powerful motivator for behaviour change [ 10 ]. The aim of this study was to evaluate the effect of a personalised future self-image on weight change over a 6-month period, with a broader sample than the pilot study and to include both men and women of any age over 16 years.
A computerised application (app) prototype called ‘Future Me’ was developed previously by the research team. The app portrays the effect of lifestyle on future personal appearance using input calorie and exercise information to predict future Body Mass Index (BMI) [ 9 ]. The minimum viable product (MVP) features are shown in Fig. 1 below.
Features of the Future Me App (minimum viable product: MVP)
The trial was approved by the Curtin Human Research Ethics Committee (HR 112/2013). A sample size of 150 was determined to have the power to detect a 1-kg weight difference between groups, which was the mean weight difference seen at 8 weeks in the pilot study [ 9 ]. A total of 145 participants, slightly fewer than the target number, were recruited over 8 months from November 2013 to August 2014, when the recruitment phase period concluded. Eligibility criteria required participants to be at least 16 years old, with a BMI over 25.0 kg/m 2 and wanting to lose weight. Women who were pregnant or breastfeeding were ineligible. One participant was excluded due to recent thyroid surgery. During the study, one participant was withdrawn after becoming pregnant and another after opting for bariatric surgery. Participants were recruited through the Curtin University website, radio announcements, emails, and flyers. Recruitment took place at two general practices north and south of the Perth area, Curtin University, and two large employer groups located in the Perth metropolitan area.
At recruitment, participants completed a questionnaire outlining demographic details and an assessment of motivational state using the Prochaska Transtheoretical Model of Behaviour Change [ 11 ]. This model of behaviour change assesses an individual’s readiness to act on a new behaviour which can range from Pre-contemplation to Maintenance. In general the earlier the ‘stage of change’ for a behaviour the less readily an individual will act on a stimulus to change that behaviour.
Baseline height, weight, and waist circumference were measured. Then, all recruits were randomised, by selecting a concealed token produced beforehand using a random number generator, to receive a future self-image immediately (early-image group), or to receive the image after 8 weeks (delayed-image group). Based on the pilot study, 8 weeks was chosen as a reasonable amount of time for a difference in weight loss to emerge, and to retain sufficient numbers in the delayed-image group, whose members may be dissuaded by the need to return for weigh-ins after being denied the intervention at recruitment. To use the Future Me app, an iPad mini with the MVP installed was provided only during the meeting and operated by the participant with the researcher’s assistance. Each participant chose one preferred self-image from the set of five future time points at 4, 8, 12, 26, or 52 weeks. The current image (week 0) and the chosen future image were printed onto photo cards for the participant to keep.
Regardless of group allocation, all participants received 15 min of general lifestyle advice for weight loss. This advice included the following: to reduce overall calorie intake and/or increase physical activity in line with current guidelines [ 12 ]. To follow the National Health and Medical Research Council ‘Australian Guide to Healthy Eating’ chart [ 13 ]. Participants received a resource pamphlet listing several freely available online resources for weight management. It also listed a variety of professionals who could assist with weight loss, including accredited dieticians and accredited commercial weight-loss programmes (see Additional file 1 ).
Any weight-loss methods chosen were self-selected by the individual. Participants were asked to return once every 4 weeks for 24 weeks to record a weight measurement. The 4-week interval was chosen to minimise the impost on participants but to maintain close monitoring of their progress. Only weights recorded on the original set of scales or calibrated scales at alternative study locations were included in the final analysis. The researcher provided information on sources of advice at the time of weigh-ins, but did not provide any support in the intervening periods. At the 16-week visit participants were randomised again to either receive a second future self-image using their new weight parameters, or to continue with only the original future self-image. This step was designed to allow an estimate of the effect of repeated exposure to the image-creation process. Figure 2 summarises the study timeline.
Consolidated Standards of Reporting Trials (CONSORT) diagram for the study. The numbers of participants at each stage are shown in brackets
The status of the participants was defined in three groups. Participants were deemed to have engaged in the study if they returned for the first weigh-in after recruitment. Participants who did not return for this visit are termed non-starters and were excluded from the analysis. Participants were deemed to have completed the study if they recorded a weight at either week 20 or week 24. Participants who discontinued weight measurements between week 4 and week 20 are termed dropouts and are included in the intention-to-treat (ITT) analysis.
Missing data
It was common for participants to have an incomplete set of weight measurements. For completers, if a weight was recorded at 24 weeks, then any missing intermediate weight measures were substituted with a value obtained by linear interpolation from baseline to the 24-week observation. For example, if a subject was missing data at weeks 12 and 16, these values were estimated from the straight line joining values measured at baseline and at 24 weeks. In the three instances where a 20-week weight was available, but the final 24-week value was missing, the 24-week value was assumed to be the same as the 20-week value. For dropouts or withdrawals, a ‘last observation carried forward’ strategy was used to estimate all missing measurements, whereby each missing value was replaced by the weight at the previous time period.
Data analysis
Standard descriptive statistics (frequencies and percentages for categorical variables, means, and standard deviations for variables measured on a continuous scale) were used to summarise the profile of participants in the study. Comparisons between treatment groups for these variables were performed using the chi-square test or t test as appropriate, to check whether the groups were similar at baseline.
The primary outcome was weight loss over 16 weeks. A ‘per-protocol’ analysis was performed using participants whose weights were measured at weeks 8 and 16 (at least). Attendance at week 8 was required for participants in the delayed-image group to receive their image, and the week 16 measurement was required for the final measurement (following protocol). A separate ITT analysis was also performed up to week 16 (with missing data replaced as described above).
Secondary outcomes included weight loss and change in waist circumference over 24 weeks. These analyses were undertaken firstly using only ‘completers’ who attended at weeks 8 and 16 (per protocol), and repeated including withdrawals (ITT). Analyses on weight were performed using a random effects regression model. The independent variables included in the model were time and treatment (delayed versus early image), and their interaction. The purpose of the interaction term was to identify whether the rate of weight loss over time differed between treatments. Subsequently, the model was expanded to identify whether weight loss also depended on any of the other factors measured. These variables included: recruitment location; gender; age group (18–35, 36–55, 56–79 years); marital status; following a weight-loss programme at baseline; and motivational stage (Pre-contemplation, Contemplation, Action, Maintenance). Analysis of the data to 24 weeks included a term indicating the group to which participants were allocated at 16 weeks. A backwards elimination strategy was used to identify the best model including these other factors. This was done by including all independent variables in the model at first, and then dropping them, one at a time, until all variables remaining in the model were significantly associated with weight change. At that point, all the pairwise interaction terms were assessed for significance. Change in waist circumference was the length at week 24 minus the length at baseline, and analysed using a paired t test.
The variable for time was initially included as a categorical variable, so that no assumption was made concerning the linearity of the change in weight over time. However, change in weight was close to linear, and so the variable was subsequently treated as continuous. The changes in weight are expressed as a percentage of baseline weight per 4-week interval (approximately ‘per month’).
Statistical analyses were performed using the SAS version 9.2 software, and, following convention, a p value < 0.05 was taken to indicate a statistically significant association in all tests.
Group characteristics
One hundred and forty-five participants were recruited to the study, with 74 allocated to receive their image at commencement, and 71 to the delayed-image group. Baseline characteristics according to initial group allocation are presented in Table 1 . Twenty-four recruits were deemed non-starters, comprising 15 (21%) in the delayed-image group and 9 (10%) in the early-image group (χ 2 (1) = 2.1, p = 0.1465). Sixteen participants allocated to the early-image group, and 21 participants allocated to the delayed-image group withdrew, so that 84 (69.4%) of the 121 participants who engaged in the study, completed it. The completion rate was similar for early- and delayed-image groups (χ 2 (1) = 2.35; p = 0.13) and across different categories of baseline motivational status (χ 2 (2) =0.42; p = 0.8108).
Per-protocol analysis up to week 16
Of the 121 participants who engaged in the study, 55 attended at weeks 8 and 16 (the ‘per-protocol’ participants). Of these, 30 (46.2%) were in the early-image group and 25 (44.6%) were in the delayed-image group. The analysis showed a significant decline in weight over time ( p < 0.0001), and also a significant difference in rate of change between early and delayed images (delayed-image: −0.77%, early-image: −0.49%; p = 0.018).
ITT analysis up to week 16
When the same model was applied to the ITT dataset, there remained a significant change over time ( p < 0.0001), and a significant difference between groups (delayed-image: −0.50%, early-image: −0.30%, p = 0.007). This difference is smaller due to the missing value replacements that were performed in both groups.
Per-protocol analysis up to week 24
Similarly to the situation at 16 weeks, there appeared to be a very significant change in weight overall ( p < 0.0001), and a difference in rate of change between groups (delayed-image: −0.60%, early-image: −0.42%, p = 0.012). The group into which participants were allocated at week 16 (second image or not) appeared not to influence the outcome ( p = 0.3128).
ITT analysis up to week 24
Analysis of all completers and withdrawals showed a strong trend over time ( p < 0.0001), and a difference in rate of change between groups (delayed-image: −0.50%, early-image: −0.27%, p = 0.0008).
Table 2 shows the results of analysis of the ITT dataset to week 24 (all records except non-responders), when other variables were included as candidate-independent variables. The final model included only gender (men lost weight at a greater rate than women), and treatment group (greater weight loss for the delayed-image group). The figures in the table show the overall relative weight loss per 4 weeks (−0.7%), and the differences in weight loss between genders and treatment groups. For example, the weight loss for men in the delayed-image group is estimated to be −0.7%/4 weeks. For women in this group, it would be −0.35% (−0.7 + 0.35). For those in the early-image group, the corresponding figures would be −0.49% for men, and −0.14% for women.
Mean change in waist circumference over 24 weeks was –2.74 cm for the delayed-image group and –3.23 cm for the early-image group, which was not statistically significant ( p = 0.68). By week 24, 8 (12.3%) of the early-image and 12 (21.4%) of the late-image participants who were engaged in the study (ITT) achieved a weight loss of at least 5%. This difference was not statistically significant ( p = 0.1780). When based on ‘completers’, the percentages rise to 14.3% of the early-image group and 28.6% of the delayed-image group ( p = 0.1081).
This study has examined the rate of weight loss in adults who received a personalised image of predicted future body shape, with self-selected diet and exercise targets. On average, weight loss was modest over the 24-week period, but greater than has been recorded in other studies that have provided advice alone [ 14 ]. In this trial more than one fifth of completers in the delayed-image group lost 5% or more of their baseline weight.
A greater rate of weight loss was seen with the delayed-image group completers. This was an unexpected result. It was hypothesised that a longer period of exposure to the future self-image would result in more weight loss, favouring the early-image group. However, it may be that creation of the future self-image several weeks into a weight-loss attempt served as a greater trigger or additional reinforcement for further weight loss. An alternative explanation is that a greater number of participants in the delayed group were committed to weight-loss efforts. There was some evidence for this as a greater proportion of those in the delayed group were in the Contemplation phase of the change and a higher proportion were non-starters in this group. Failing to receive an early image may have prompted some recruits, those less committed to weight loss, to drop out early, leaving in comparison, relatively more committed recruits in the delayed-image group. We found, however, no significant interaction between initial motivational stage and percentage weight loss, and no significant effect of group allocation on continuation status, so this explanation is less plausible. Average age and baseline BMI were similar between the groups. Any apparent differences in male-to-female ratio and motivational stage would have in fact favoured weight loss in the early-image group.
Data from this study consistently demonstrated more rapid weight loss in men. Without information on individual dietary and physical activity records during the study it is not possible to confirm why this was the case, although the same pattern has been reported elsewhere [ 15 ]. Patterns of dietary change after weight-loss advice have been described in the medical literature for both men and women [ 16 , 17 ]. It may be that the male participants made more effective lifestyle changes. Weight loss for men and women can be expected to be similar when energy expenditure is also comparable [ 18 ]. The difference favouring more rapid weight loss in the delayed-image group was observed for men in the ITT analysis. The reason is unclear, but suggests that there may be a disparity between genders in how the image operates.
We found no significant difference between groups for weight loss at the 8-week weigh-in where the delayed group had not yet viewed the future self-image. Comparable results between groups at 8 weeks may be because it was not a long enough period of time to produce a divergence or, as already postulated, the effect of the future self-image was less significant at the outset of a weight-loss attempt.
Visual appearance is highly important for most people who want to lose weight [ 10 , 19 ]. The Future Me app allows the user to actually visualise the changes that they could achieve through lifestyle modification. Furthermore, the user is provided with an estimate of the effort in terms of diet and exercise, as well as the time required to achieve, the desired physical appearance. These factors are likely to help set realistic goals. The Future Me images can be created within minutes using only basic nutritional knowledge. These features make it an ideal tool for time-constrained, non-specialist clinical settings such as general practice.
The myriad health benefits achieved with weight loss for those in the overweight and obese range are well established. The use of a future self-image does not imply that the health message is any less important than physical appearance. Rather, the method acknowledges how important physical appearance is for many dieters, and allows a tangible visualisation of healthy lifestyle change.
Strengths and limitations
This study reports a randomised trial of an iPad app. The subjects were followed up for 24 weeks and the data are reported on an ITT basis. The team did not feel that any group could be randomised to have no access to the app because communication between participants may have led to disappointment and frustration for those participants who would have not received it. Nonetheless, we acknowledge that this reduces our capacity to assess the impact of the app against no intervention. Also, the attempt to test the dose effect of the app at 16 weeks was unsuccessful due to low attendance at the 16-week weigh-in where only 22 participants were allocated a second future self-image. In general, there was significant attrition during the study as appears to be case for many studies reporting weight-loss interventions. Sixteen percent of recruits were non-starters and the attrition rate for the remainder was 31%. This is on par with most weight-loss trials where attrition is commonly between 20 and 50%, and sometimes more [ 20 ].
A personalised future self-image may boost weight-loss efforts when implemented in the course of an ongoing weight-loss attempt. The Future Me app allows such images to be created in time-constrained clinical settings. Further research with this tool is needed to determine its overall efficacy and the optimal way to implement the app with existing weight management strategies.
Abbreviations
Application
Body Mass Index
Confidence interval
Minimum viable product
Mendis S. Global status report on non-communicable diseases. Switzerland: WHO Press, World Health Organisation; 2014. http://www.who.int/nmh/publications/ncd-status-report-2014/en/ . Accessed 12 Nov 2015.
Google Scholar
Forman-Hoffman V, Little A, Wahls T. Barriers to obesity management: a pilot study of primary care clinicians. BMC Fam Pract. 2006;7:35.
Article PubMed PubMed Central Google Scholar
Kolasa KM, Rickett K. Barriers to providing nutrition counseling cited by physicians: a survey of primary care practitioners. Nutr Clin Pract. 2010;25(5):502–9.
Article PubMed Google Scholar
Campbell K, Engel H, Timperio A, Cooper C, Crawford D. Obesity management: Australian general practitioners’ attitudes and practices. Obes Res. 2000;8(6):459–66.
Article CAS PubMed Google Scholar
Kim Y, Sundar SS. Visualizing ideal self vs. actual self through avatars: impact on preventive health outcomes. Comput Hum Behav. 2012;28(4):1356–64.
Article Google Scholar
Burford O, Jiwa M, Carter O, Parsons R, Hendrie D. Internet-based photoaging within Australian pharmacies to promote smoking cessation: randomized controlled trial. J Med Internet Res. 2013;15(3), e64.
Brug J, Steenhuis I, van Assema P, de Vries H. The impact of a computer-tailored nutrition intervention. Prev Med. 1996;25(3):236–42.
Neville L, O’Hara B, Milat A. Computer-tailored physical activity behavior change interventions targeting adults: a systematic review. Int J Behav Nutr Phys Act. 2009;6(1):30.
Jiwa M, Burford O, Parsons R. Preliminary findings of how visual demonstrations of changes to physical appearance may enhance weight loss attempts. Eur J Public Health. 2015;25(2):283–5.
Baumeister RF, Leary MR. The need to belong: desire for interpersonal attachments as a fundamental human motivation. Psychol Bull. 1995;117(3):497–529. doi: 10.1037/0033-2909.117.3.497 .
Prochaska JO, Velicer WF, Rossi JS, Goldstein MG, Marcus BH, Rakowski W, et al. Stages of change and decisional balance for 12 problem behaviors. Health Psychol. 1994;13(1):39–46.
Australian Government National Health and Medical Research Council. Summary guide for the management of overweight and obesity in primary care. Melbourne: National Health and Medical Research Council; 2013.
Australian Government National Health and Medical Research Council. Australian Guide to Healthy Eating. http://www.eatforhealth.gov.au/guidelines/australian-guide-healthy-eating . Accessed 12 Nov 2015.
Franz MJ, VanWormer JJ, Crain AL, Boucher JL, Histon T, Caplan W, et al. Weight-loss outcomes: a systematic review and meta-analysis of weight-loss clinical trials with a minimum 1-year follow-up. J Am Diet Assoc. 2007;107(10):1755–67.
Stubbs RJ, Pallister C, Whybrow S, Avery A, Lavin J. Weight outcomes audit for 34,271 adults referred to a primary care/commercial weight management partnership scheme. Obes Facts. 2011;4(2):113–20.
Collins CE, Morgan PJ, Warren JM, Lubans DR, Callister R. Men participating in a weight-loss intervention are able to implement key dietary messages, but not those relating to vegetables or alcohol: the Self-Help, Exercise and Diet using Internet Technology (SHED-IT) study. Public Health Nutr. 2011;14(1):168–75.
Howard BV, Manson JE, Stefanick ML, Beresford SA, Frank G, Jones B, et al. Low-fat dietary pattern and weight change over 7 years: the Women’s Health Initiative Dietary Modification Trial. JAMA. 2006;295(1):39–49.
Caudwell P, Gibbons C, Finlayson G, Näslund E, Blundell J. Exercise and weight loss: no sex differences in body weight response to exercise. Exerc Sport Sci Rev. 2014;42(3):92–101.
Robertson A, Mullan B, Todd J. A qualitative exploration of experiences of overweight young and older adults. An application of the integrated behaviour model. Appetite. 2014;75:157–64.
Moroshko I, Brennan L, O’Brien P. Predictors of dropout in weight loss interventions: a systematic review of the literature. Obes Rev. 2011;12(11):912–34.
Download references
Acknowledgements
We acknowledge the support of the Mandurah Medical Centre and the Peel Health Foundation.
The work has been supported by a grant from GP Synergy as part of general practice academic registrar training. Also partly funded by the Department of Medical Education, Curtin University.
Availability of data and materials
De-identified data for this study are available upon request from the authors.
Authors’ contributions
MJ, GO, and AM conceived the project and oversaw the design of the study. RP and GO conducted statistical analyses and analysed the data. GO coordinated the study. GO and RP oversaw the collection of data and implemented the study. All authors interpreted the data and results, prepared the report and the manuscript, and approved the final version.
Competing interests
MJ owns the intellectual property for the ‘Future me’ app.
Consent for publication
All authors consent to the publication of this paper.
Ethics approval and consent to participate
Curtin Human Research Ethics Committee (HR 112/2013). All participants provided informed written consent before participation.
Publisher’s Note
Springer Nature remains neutral with regard to jurisdictional claims in published maps and institutional affiliations.
Author information
Authors and affiliations.
Department of Medical Education, Curtin University, Perth, WA, 3845, Australia
Gemma Ossolinski
Melbourne Clinical School, School of Medicine Sydney, The University of Notre Dame Australia, Werribee, VIC, Australia
Faculty of Health Sciences, Curtin University, Perth, WA, Australia
Alexandra McManus
School of Occupational Therapy and Social Work and School of Pharmacy, Curtin University, Perth, WA, Australia
Richard Parsons
You can also search for this author in PubMed Google Scholar
Corresponding author
Correspondence to Gemma Ossolinski .
Additional file
Additional file 1:.
The Participant Resource Brochure. (PNG 340 kb)
Rights and permissions
Open Access This article is distributed under the terms of the Creative Commons Attribution 4.0 International License ( http://creativecommons.org/licenses/by/4.0/ ), which permits unrestricted use, distribution, and reproduction in any medium, provided you give appropriate credit to the original author(s) and the source, provide a link to the Creative Commons license, and indicate if changes were made. The Creative Commons Public Domain Dedication waiver ( http://creativecommons.org/publicdomain/zero/1.0/ ) applies to the data made available in this article, unless otherwise stated.
Reprints and permissions
About this article
Cite this article.
Ossolinski, G., Jiwa, M., McManus, A. et al. Do images of a personalised future body shape help with weight loss? A randomised controlled study. Trials 18 , 180 (2017). https://doi.org/10.1186/s13063-017-1907-6
Download citation
Received : 06 January 2016
Accepted : 16 March 2017
Published : 18 April 2017
DOI : https://doi.org/10.1186/s13063-017-1907-6
Share this article
Anyone you share the following link with will be able to read this content:
Sorry, a shareable link is not currently available for this article.
Provided by the Springer Nature SharedIt content-sharing initiative
- Weight loss
- Medical informatics applications
- Intervention study
- Health promotion
ISSN: 1745-6215
- Submission enquiries: Access here and click Contact Us
- General enquiries: [email protected]
- Search Please fill out this field.
- Newsletters
- Sweepstakes
- Special Diets
Food Serving Sizes: A Visual Guide
Find out how everyday objects can ease the guessing game of serving sizes and portion control.
Figuring Out Portion Sizes
What you eat is important, especially when it comes to making positive food choices, but how much you eat is the real brainteaser of healthy eating. When you look at the oversize food portions, ranging from the diameter of bagels to mounds of pasta, translating a serving size into portions is a big challenge in a more-is-better world.
Don't Miss: Desserts and Sweets for People with Diabetes
The first step is knowing the difference between a portion and a serving size. A serving size is a recommended standard measurement of food. A portion is how much food you eat, which could consist of multiple servings.
Visually comparing a serving size to an everyday object you have at home, such as a baseball or a shot glass, can be helpful in identifying what a serving size looks like without carting around a scale and measuring cups for every meal and snack. Here are some general guidelines for the number of daily servings from each food group*:
- Grains and starchy vegetables: 6-11 servings a day
- Nonstarchy vegetables: 3-5 servings a day
- Dairy: 2-4 servings a day
- Lean meats and meat substitutes: 4-6 ounces a day or 4-6 one-ounce servings a day
- Fruit: 2-3 servings a day
- Fats, oils, and sweets: Eat sparingly
*Check with your doctor or dietitian to determine the appropriate daily recommendations for you.
Whole Grain Bread
1 serving = 1 slice
A slice of bread is proportional to the size of one DVD disc.
1 serving = 1 teaspoon
One small pat of butter is equal to one serving size.
1 serving = 1/2 cup
A serving-size side of green peas is equal to half of a baseball.
Air-Popped or Light Microwave Popcorn
1 serving = 3 cups
Snack away on the healthier varieties of popcorn and enjoy a serving size of three baseballs.
Baked Potato or Sweet Potato
Choose a potato the size of a computer mouse.
Salad Greens
1 serving = 1 cup
When making your perfect salad, the serving of greens should be the size of one baseball.
Reduced-Fat Salad Dressing
1 serving = 1/4 cup
Top your salad with one golf-ball size serving of dressing.
Peanut Butter
1 serving = 1 tablespoon
You're doing great if your peanut butter serving fits into half of a 1-ounce shot glass.
1 serving = 1 ounce
A bagel the size of half of a baseball is equal to one serving.
1 serving = 1/3 cup cooked
A serving of pasta is roughly the same size as one tennis ball.
Olive oil is a great alternative to butter, but remember to keep the serving size similar to one pat of butter.
Canned Fruit
Canned fruit in light juices is equal to half of a baseball.
Baked French Fries
1 serving = 1 cup + 1 teaspoon of canola or olive oil
A serving of French fries looks like the equivalent of one baseball. Don't forget to account for the teaspoon of oil.
Shredded Cheese
1 serving = 2 tablespoons
Toss your salad or taco with a serving of shredded cheese equal to one 1-ounce shot glass.
Enjoy a serving of steamed broccoli that's the size of half of a baseball.
100-Percent Orange or Apple Juice
1 serving = 4 ounces or 1/2 cup
A fun-size juice box is the serving size you should aim for. Another way to think about it: an average woman's fist resting on its side.
1 serving = 1 medium apple
Pick an apple about the same size as one baseball.
1 serving = 3 ounces cooked
A serving of fish will have the thickness and length of a checkbook.
Reduced-Fat Mayonnaise
If you go for reduced-fat mayo, fill half of a 1-ounce shot glass for a serving.
Low-Fat Block Cheese
Keep your serving size of hard cheese to the equivalent of three dice.
Chicken, Beef, Pork, or Turkey
When cooking lean meat, choose a serving the size of one deck of cards.
Nonfat or Low-Fat Milk
A serving looks like the small 8-ounce carton of milk you loved in school.
1 serving size = 2 cookies
Cookies shouldn't be monster-size. Think Oreos for a good measure of comparison.
Scoop out the creamy dessert to equal half of a baseball.
If you must have your candy, a serving equals one 1-ounce shot glass.
Related Articles
A Systematic Review of Weight Perception in Virtual Reality: Techniques, Challenges, and Road Ahead
Ieee account.
- Change Username/Password
- Update Address
Purchase Details
- Payment Options
- Order History
- View Purchased Documents
Profile Information
- Communications Preferences
- Profession and Education
- Technical Interests
- US & Canada: +1 800 678 4333
- Worldwide: +1 732 981 0060
- Contact & Support
- About IEEE Xplore
- Accessibility
- Terms of Use
- Nondiscrimination Policy
- Privacy & Opting Out of Cookies
A not-for-profit organization, IEEE is the world's largest technical professional organization dedicated to advancing technology for the benefit of humanity. © Copyright 2024 IEEE - All rights reserved. Use of this web site signifies your agreement to the terms and conditions.
7 Fun Ways to Visually Track Weight Loss Progress
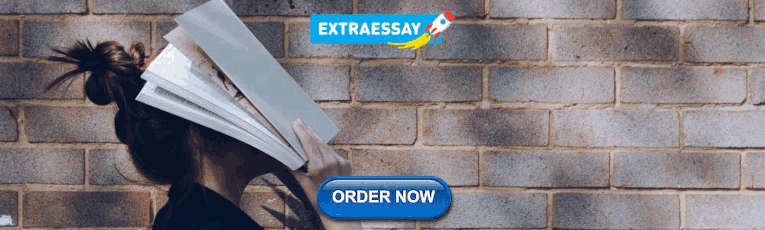
How to See What You Will Look Like After Weight Loss
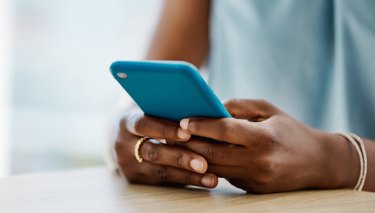
Ever wonder what you would look like if you lost weight? Well, thanks to technology, you can use a weight loss simulator to envision your appearance once you shed pounds.
Advertisement
Here's everything to know about what weight loss really looks like, plus weight loss visualizer apps to try.
Video of the Day
How Can You See What You Will Look Like After You’ve Lost Weight?
There's no way to know exactly what you will look like when you lose weight, as what weight loss really looks like (and how you carry weight in general) can depend on factors like your body type, genetics, age and gender, according to the National Institute of Diabetes and Digestive and Kidney Diseases .
Still, weight loss visualizers can come in handy if you're curious how you would look if you lost weight. Here's are some of the most popular weight loss picture apps to consider.
Visualize You App
Most people start out on their weight loss journey full of enthusiasm to shed some pounds and get fit. Unfortunately, if things don't progress the way they expected, many will lose the motivation to keep pushing forward, and eventually, throw in the towel. That's when using an app or weight loss simulator may come in handy.
There are several online virtual weight loss programs that allow you to see what you might look like after losing weight. One app, in particular, the Visualize You app, seems to be the leader in weight loss simulator programs.
According to their website , the Visualize You app transforms a current picture of you to a self-defined "goal weight." In other words, you can use your phone to transform a picture of yourself at 175 pounds to see what you might look like at 145 pounds.
While Visualize You uses your current height, weight, and picture, there is never a guarantee that you will look like the after photo. In other words, if you’re going to try a weight loss simulator or app, make sure you understand the limitations.
Other Weight Loss Simulators
In addition to the Visualize You app, there are a number of online simulators that can help you visualize what your body look likes after weight loss.
1. Change in Seconds
Change in Seconds is a virtual weight loss imager that uses your height, weight, body type (apple, hourglass, pear) and your goal weight. While the image is not of you, they have a virtual model that displays a current image of you and what you may look like at your goal weight.
2. Model My Diet
Model My Diet is another virtual body fat simulator program that uses your body type, current weight and goal weight to display a virtual image of you at your desired weight. One of the benefits of this site is they have a program for people assigned male at birth and people assigned female at birth.
Like the Visualize You app, these weight loss simulators have limitations, especially because the image you see is a model, not a photo of you.
That's why it's important to use these programs in moderation: If you feel that you're getting too focused on the "after" image they display, you may want to take a step back and spend your energy focusing on daily habits like moving more and eating nutritious foods that fuel your body.
Weight loss doesn't have to be about your physical appearance. Instead, celebrate non-scale victories like having more energy or accomplishing a fitness goal.
Tips for Losing Weight
The internet is full of quick weight loss tips and tricks that promise to deliver some impressive results in a short amount of time. Quite often, what these claims forget to mention is that any type of rapid weight loss that requires you to drastically change your lifestyle, is likely to be short-lived.
In fact, the Mayo Clinic recommends a weight loss of one to two pounds a week, as it is more likely to help you maintain your weight loss for the long term.
With that in mind, a good place to start is with your diet. Take inventory of how you eat , how much you eat and how often. Choose three days to log your meals and see if certain patterns emerge. Try to limit excess processed food and sugar, high-calorie drinks and foods that don't contain enough protein or fiber.
Per the American Academy of Dietetics , the best way to fill your dietary needs is to enjoy nutrient-rich foods from a variety of food groups. Choose from lean protein sources such as fish, whole-grains, low-fat dairy and plenty of fruits and vegetables.
The next step is to reduce the number of calories you eat in a day, according to the Mayo Clinic. Generally speaking, reducing your total calories by 250 to 500 each day can help you lose up to one pound a week.
Finally, including physical activity in your day can support your health and lead to greater weight loss. Aim to exercise five days a week with a combination of aerobic exercise and resistance training. By reducing your caloric intake and burning an extra 250 to 500 calories a day, you can lose up to two pounds a week, per the Mayo Clinic.
In addition to sweating it out on a cardio machine , weight training is also important for weight loss, according to the American Council on Exercise (ACE). The ACE recommends using an integrated approach that includes lifting weights at a moderate to heavy intensity, circuit training , supersets and lifting heavier weights.
FAQs About What You Would Look Like if You Lost Weight
You asked, we answered. Here are responses to common questions about weight loss:
1. How Does Weight Loss Change Your Appearance?
You can't target weight loss to one area of your body, so if you drop pounds, you're losing weight everywhere, according to the ACE . As a result, you'll likely notice your entire body slimming down as you shed fat.
However, exactly how much your size changes depends on how much weight you lose. Losing 5 pounds, for instance, may not have as big an effect on your appearance as losing 15 pounds. Similarly, how long it takes to notice weight loss depends on how much fat you shed relative to your initial weight.
And how does weight loss affect your face ? Similarly, your face will slim down as the rest of your body loses fat.
2. How Does Weight Loss Affect Your Skin?
If you lose a significant amount of weight (typically 100 pounds or more), you may have excess skin that is too stretched out to fit your new body size, according to the Cleveland Clinic . And this sagging skin may not have the elasticity to shrink, in which case you may require cosmetic procedures or surgeries to tighten or remove excess skin.
You may also notice some skin changes from more moderate weight loss. For instance, stretch marks that developed as you gained weight may become more visible as you shed fat.
Stretch marks typically appear as pink, red, black, blue or purple streaks on your body, per the Mayo Clinic , so if you notice differences in your skin color as your weight changes, this may be the reason why. Fortunately, they're harmless and may fade with time.
3. Why Doesn't It Look Like I've Lost Weight?
If the number on the scale is dropping but you aren't losing inches around your waist, there are a few potential explanations.
First, you may be losing visceral fat , the more dangerous type of fat that surrounds your internal organs and ups your risk for heart disease, diabetes and stroke, per the Centers for Disease Control and Prevention . Because it's deeper in your core, you may not notice a change in size right away.
Second, you may be losing muscle or water weight instead of fat. This is not ideal, and can happen if you lose weight too quickly, per the U.S. National Library of Medicine . To avoid this issue, stick to the expert-recommended weight-loss pace of 1 to 2 pounds a week.
4. What Does 20 Pounds of Weight Loss Look Like?
Remember, weight loss is relative. For example, 20 pounds of weight lost will look different on someone who's starting weight was 150 pounds versus 300 pounds.
Instead of getting hung up on the numbers, focus on the wins that don't relate to your physical appearance.
- The Mayo Clinic: "Fast Weight Loss: What's Wrong With It?"
- The American Academy of Dietetics: "Back to Basics for Healthy Weight Loss"
- Change in Seconds: "Virtual Weight Loss Simulator and Body Visualizer"
- Model My Diet: "Virtual Weight Loss Simulator and Motivation Tool"
- The American Council on Exercise: "Weight Lifting for Weight Loss"
- National Institute of Diabetes and Digestive and Kidney Diseases: "Factors Affecting Weight & Health"
- The American Council on Exercise: "Myths and Misconceptions: Spot Reduction and Feeling the Burn"
- Cleveland Clinic: "Excess Skin Removal"
- Mayo Clinic: "Stretch marks"
- Centers for Disease Control and Prevention: "Not Just Your Grandma’s Diabetes"
- Visualize You: "Visualize Your Weight"
- U.S. National Library of Medicine: "Diet for rapid weight loss"
Report an Issue
Screenshot loading...
- Supplements
- Weight Loss

Compare Your Weight Loss Results to Objects to See Progress
16 Dec 2018
We’ve all been disappointed by our weight loss results at some point in time. Maybe you’ve eaten super-healthily all week but the scales don’t reflect your efforts. Or you’ve hit the gym hard and done a few back-to-back classes but aren’t noticing any difference in your dress size.
This kind of situation can be really demotivating and can lead to a downward spiral if left unchecked. But there are lots of ways to switch up your mindset and turn your disappointment into meaningful progress. In this article, we look at one way to do this – using everyday household objects.
Using household objects as weight loss motivation
If you’re trying really hard to lose weight, it can be really disappointing when you don’t see results. Dieters can get deflated when they fail to see the weight loss they’ve achieved reflected in photos or better fitting clothes. This can be even tougher if you’re trying to lose a significant amount of weight.
We tend to lose weight equally from all parts of the body which means that it can take a while to notice results. Although the total amount lost might be a lot, it translates to a small amount from each part of the body, which means the changes are often less apparent than we’d like.
Another issue is that we’re simply less able to notice changes in ourselves. We see our reflection in the mirror every day so it’s hard to see small changes that happen gradually. It’s often the case that other people will notice our weight loss before we really see it for ourselves. This is because they see us less often, therefore, the changes are more noticeable to them. As a general rule of thumb, in:
- 1-2 weeks – you’ll start to feel better
- 4-6 weeks – other people will notice that you’ve lost weight
- 8-12 weeks – you’ll start to notice the weight loss for yourself
If you’re feeling disappointed with your progress, it can be helpful to look at it objectively. This is where household objects can be a useful and fun way to reflect on your progress. You might not feel like you’ve lost a significant amount of weight but when you see it’s the equivalent of a box of cereal or kitchen sink it’ll seem very different.
Weight loss comparison to objects
Let’s look at how different amounts of weight loss translate into various household items.
2lb – A large bag of sugar
2lb (1kg) of body fat takes up around 1000 cubic centimeters or just over 4 cups in volume. That’s quite a lot! Losing this amount of fat is the equivalent of a losing a large bag of sugar – and that’s no small feat! Carrying this seemingly harmless extra weight can make everything harder, from getting out of bed to walking down the street. So by losing it, you’ll start to notice everyday activities becoming that much easier.
8lb – Your head
Your head, complete with brain weighs around 8lb. Imagine how much lighter you’d feel without it on your shoulders! Granted, you kind of need your head, but if this weight loss is down to pure fat loss, you’ll certainly be feeling a lot lighter!
13lb – An obese cat
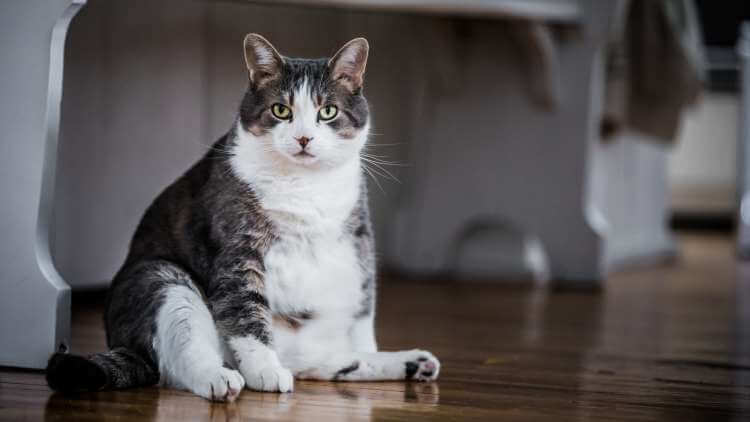
The average pet cat should weigh around 10lbs (depending on the breed) but an obese cat can weigh upwards of 13lbs. Although some people may find losing this weight noticeable, others may not – it’ll depend on your frame and starting weight.
But just imagine carrying an obese cat around in a backpack all day (with air holes of course!). Just getting up off the sofa with a Garfield-sized cat would be 10x harder, let alone trying to go for a run. So if you’ve lost 13+ pounds but don’t see it as a significant amount then think again. You’re freeing up your body for more active pursuits that’ll further contribute to your weight loss.
15lb – A vacuum cleaner
Vacuum cleaners weigh around 15lbs (7kg) on average, so losing this amount of weight is truly impressive. We all know how difficult it is to carry one up a flight of stairs! And if you’re not quite there yet, imagine how much easier everything will be once you have shed this weight.
55lb – A poodle
Although poodles come in lots of shapes and sizes, they weigh around 55lbs (25kg) on average. This is the equivalent of two obese cats or 25 bags of sugar! By losing this amount of weight you’ll not only decrease your waistline, but significantly reduce your risk of chronic diseases too.
175lb – A washing machine
A typical washing machine weighs around 175lbs (79kg) and takes up around 4 cubic feet. Taking your BMI from very obese down to a healthy range may involve losing this amount of weight for some people. It’ll take time but the health benefits will be well worth it (and we’re confident that you’ll definitely notice the difference!).
How much have you lost so far?
It’s easy to think 2lb is no great loss, but now you have some practical objects you can benchmark your weight loss against, you can really begin to appreciate how much you’ve achieved!
It’s also important to take stock of the other health benefits you’re benefiting from. Do you have more energy or find it easier to walk up flights of stairs? Has your skin become clearer or your brain less foggy? Perhaps you’re sleeping better as a result of consuming less sugar and becoming more active. Whatever stage you’re at in your weight loss journey, there will be side-benefits to your health that you might not have realized are connected. Be confident that you’re making progress and that every positive decision you make is getting you closer to your end goal.
Read: Top 10 dieting tips for those mega busy days
Still feeling frustrated by your weight loss progress?
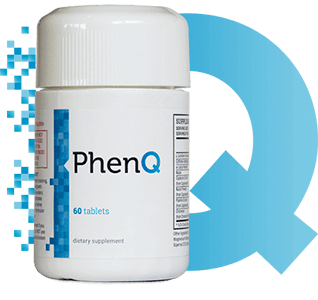
It also contains thermogenic ingredients that enable your body to burn fat faster and more efficiently. So, as well as decreasing your calorie intake, it’ll help you burn off stored body fat. You can learn more about how PhenQ can help here .
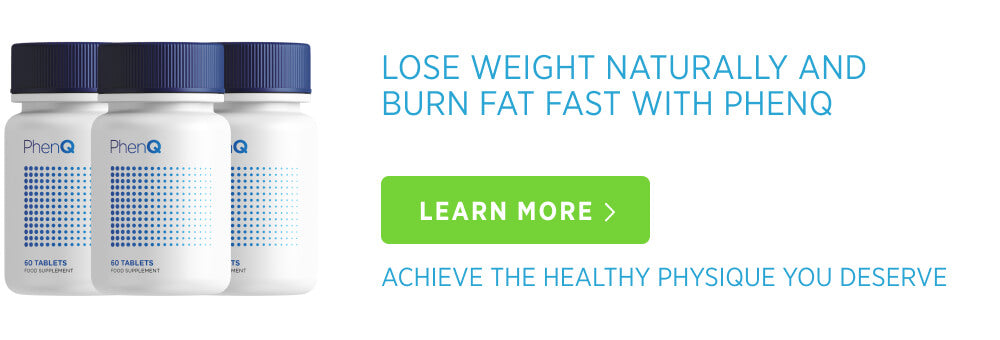

How Much Is A Pound Of Fat?
How much is a pound of fat? The skinny on fat The gross looking ball of yuck up above is 1 lb. of fat. Everyone wants to get rid of their fat, but the truth is, you could never get rid of all of it. Nor would you want to! In fact fat is so essential to our health that an average size 10, slim female carries approximately 100,000 calories of fat on her body. The average woman 5 ft. 4 in. weighs 166 lbs. Average fat% of 25-31% 46.5 lbs. of fat The average man 5 ft. 9 in. weighs 196 lbs. Average fat% of 18-24% 41.2 lbs. of fat To lose 1 lb. of fat, you need to burn 3,500 calories. Running (6mph) ~4h 17m (25.7 miles) Walking (3mph) ~13 hours (39 miles) Cycling (14-16mph) ~4h 20m (65 miles) Yoga ~17h 10m The foods below are approx. 3,500 calories. ~9 Subway Clubs (400 calories each) ~6.5 Big Macs (540 calories each) ~14 Snickers Bars (250 calories each) ~7 Large Fries (500 calories each) Did you know muscle and fat burn calories? 1 lb. muscle Burns 7-10 calories during the day 1lb. fat burns 2-3 calories during the day HOW MUCH IS A POUND OF FAT? The skinny on fat The gross looking ball of yuck up above is 1 lb. of fat. Everyone wants to get rid of their fat, but the truth is, you could never get rid of all of it. Nor would you want to! In fact fat is so essential to our health that an average size 10, slim female carries approximately 100,000 calories of fat on her body. The average woman* 5 ft. 4 in. weighs 166 lbs. Average fat% of 25-31% The average man* 5 ft. 9 in. weighs 196 lbs. Average fat% of 18-24% 46.5 Ibs. of fat 41.2 Ibs. of fat * In the U.S. Source: http://www.cdc.gov/nchs/fastats/bodymeas.htm To lose 1 lb. of fat, you need to burn 3,500 calories. Running (6mph) - 4h 17m (25.7 miles) Walking (3mph) 13 hours (39 miles) Cycling (14-16mph) - 4h 20m (65 miles) Yoga 17h 10m Calories burned based on the avg. weight for a U.S. male - 180 lbs. Source: http://www.myfitnesspal.com/exercise/lookup The foods below are approx. 3,500 calories. -9 Subway Clubs (400 calories each) -6.5 Big Macs (540 calories each) -7 Large Fries (500 calories each) -14 Snickers Bars SNICKERS (250 calories each) Source: http://www.myfitnesspal.com/food/search Did you know muscle and fat burn calories? 1 lb. muscle 1 lb. fat Burns 7-10 calories burns 2-3 calories during the day during the day Source: http://www.livestrong.com/article/438693-a-pound-of-fat-vs-a-pound-of-muscle/ This work is created under a DegreeSearch.org Creative Commons License BY NC ND
You may also like...

For hosted site:
For wordpress.com:
Thank you for visiting nature.com. You are using a browser version with limited support for CSS. To obtain the best experience, we recommend you use a more up to date browser (or turn off compatibility mode in Internet Explorer). In the meantime, to ensure continued support, we are displaying the site without styles and JavaScript.
- View all journals
- My Account Login
- Explore content
- About the journal
- Publish with us
- Sign up for alerts
- Open access
- Published: 05 June 2023
Multiple visual objects are represented differently in the human brain and convolutional neural networks
- Viola Mocz 1 ,
- Su Keun Jeong 3 ,
- Marvin Chun 1 , 2 &
- Yaoda Xu 1
Scientific Reports volume 13 , Article number: 9088 ( 2023 ) Cite this article
1697 Accesses
2 Citations
1 Altmetric
Metrics details
- Cognitive neuroscience
- Computational neuroscience
- Network models
- Neuroscience
- Object vision
- Visual system
Objects in the real world usually appear with other objects. To form object representations independent of whether or not other objects are encoded concurrently, in the primate brain, responses to an object pair are well approximated by the average responses to each constituent object shown alone. This is found at the single unit level in the slope of response amplitudes of macaque IT neurons to paired and single objects, and at the population level in fMRI voxel response patterns in human ventral object processing regions (e.g., LO). Here, we compare how the human brain and convolutional neural networks (CNNs) represent paired objects. In human LO, we show that averaging exists in both single fMRI voxels and voxel population responses. However, in the higher layers of five CNNs pretrained for object classification varying in architecture, depth and recurrent processing, slope distribution across units and, consequently, averaging at the population level both deviated significantly from the brain data. Object representations thus interact with each other in CNNs when objects are shown together and differ from when objects are shown individually. Such distortions could significantly limit CNNs’ ability to generalize object representations formed in different contexts.
Similar content being viewed by others
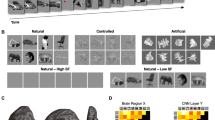
Limits to visual representational correspondence between convolutional neural networks and the human brain
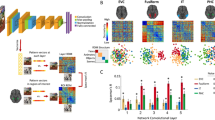
Emergence of Visual Center-Periphery Spatial Organization in Deep Convolutional Neural Networks
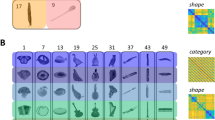
Orthogonal Representations of Object Shape and Category in Deep Convolutional Neural Networks and Human Visual Cortex
Introduction.
In everyday visual perception, objects are rarely encoded in isolation, but often with other objects appearing in the same scene. It is thus critical for primate vision to recover the identity of an object regardless of whether or not other objects are encoded concurrently. Both monkey neurophysiological and human fMRI studies have reported the existence of averaging for representing multiple objects at high-level vision. Specifically, in macaque inferotemporal (IT) cortex that is not category selective, neuronal response amplitude to a pair of unrelated objects can be approximated by the average response amplitude of each object shown alone 1 ; similarly, in human occipital-temporal cortex, fMRI response pattern to a pair of unrelated objects can be predicted by the average fMRI response pattern of each object shown alone 2 , 3 , 4 , 5 , 6 , 7 . The whole is thus equal to the average of parts at high-level primate vision (note that responses in category-selective regions may exhibit both the averaging and winner-take-all responses depending on the stimuli shown, see 5 , 6 , 8 ). Such a representational scheme can effectively avoid response saturation, especially for neurons responding vigorously to each constituent object, and prevent the loss of identity information when objects are encoded together 3 . This kind of tolerance to the encoding context, together with the ability of the primate high-level vision to extract object identity across changes in other non-identity features (such as viewpoint, position and size), has been argued as one of the hallmarks of primate high-level vision that allows us to rapidly recognize an object under different viewing conditions 9 , 10 , 11 .
In monkey neurophysiological studies, averaging was assessed at the single unit level by documenting the slope of single neuron response amplitudes to an object pair and the constituent objects. In fMRI studies, however, averaging was measured at the population level by correlating the voxel response patterns, and so it is not known whether averaging occurs at the individual voxel level. While averaging at the pattern level must stem from averaging at the individual voxel level, in a previous study, the slope of fMRI voxels to single and paired objects appeared to be substantially less than those found in macaque IT neurons. MacEvoy and Epstein 3 presented single or paired objects to human observers. Rather than calculating the slope of each fMRI voxel in the human lateral occipital cortex (LO, the homologous to macaque IT cortex) and plotting the slope distribution as was done in monkey neurophysiology, they plotted the median slopes within LO searchlight clusters and rank-ordered them according to the classification accuracy of the clusters. From this result, it appeared that the average slope across all voxels was substantially lower than 0.5 and lower than what was obtained from monkey neurophysiology. This suggests that averaging may not occur in individual fMRI voxels, or at the very least, it does not provide definitive evidence showing the presence of averaging across individual fMRI voxels in LO. Thus, the simple correspondence of averaging at the unit level and at the population level has not been established in fMRI. We have previously reported averaging in LO in fMRI response patterns 7 . The first goal of the present study is to reexamine this data and test if averaging is indeed present in individual fMRI voxels. If it does, we will further test if voxels showing better averaging in response amplitude may exhibit better response pattern averaging at the population level.
Convolutional neural networks (CNNs) are currently considered as one of the best models of primate vision, achieving human-level performance in object recognition tasks and showing correspondences in object representation with the primate ventral visual system 12 , 13 , 14 . Meanwhile, there still exist large discrepancies in visual representation and performance between the CNNs and the primate brain 15 , with CNNs only able to account for about 60% of the representational variance seen in primate high-level vision 14 , 16 , 17 , 18 , 19 . While CNNs are fully image computable and accessible, they are also “blackboxes”—extremely complex models with millions or even hundreds of millions of free parameters whose general operating principles at the algorithmic level 20 remain poorly understood 21 .
Because CNNs are trained with natural images containing a single target object appearing in a natural scene, it is unclear that objects are represented as distinctive units of visual processing and that an averaging relationship for representing multiple objects would automatically emerge. Moreover, neural averaging is similar to divisive normalization previously proposed to explain attentional effects in early visual areas 22 , 23 , 24 . Such a normalization process involves dividing the response of a neuron by a factor that includes a weighted sum of the activity of a pool of neurons through feedforward, lateral or feedback connections. Given that some of the well-known CNNs have no lateral or feedback connections, such as Alexnet 25 , VGG-16 26 , Googlenet 27 and Resnet-50 28 , these CNNs may not show response averaging for representing multiple objects. Nonetheless, by assessing the averaged slope across CNN unit responses, Jacob et al. 29 reported that higher layers of VGG16 exhibited averaging similar to that of macaque IT neurons. Because the slopes across all CNN units were averaged, this analysis does not tell us what the slope distribution across units is like and whether individual units indeed exhibit responses similar to those found in the primate brain. Meanwhile, averaging at the response pattern level has never been examined in CNNs. At the pattern level, if a large number of units fail to show averaging, even when the averaged slope from all the units is still pretty close to an averaging response, the pattern would deviate significantly from averaging. Thus, pattern analysis provides a more sensitive way of measuring averaging than the single unit slope analysis. Understanding the relationship between single and paired objects in CNNs is critical if we want to better understand the nature of visual object representations in CNNs and whether CNNs represent visual objects similarly as the primate brain. To do so, here we examined single unit and population responses from the higher layers of four CNNs pretrained for object categorization with varying architecture and depth. We additionally examined the higher layers of a CNN with recurrent processing to test whether averaging at both the single unit and population level may emerge when feedback connections are present in a network. As with the fMRI data, we also examined the relationship between unit and population responses by testing whether units showing better averaging in response amplitude would exhibit better pattern averaging at the population level.
In this study, we analyzed a previous fMRI data set 7 where participants viewed blocks of images showing either single object or object pairs selected from four different object categories (bicycle, couch, guitar, or shoe) and performed a 1-back repetition detection task (Fig. 1 a). We selected the 75 most reliable voxels from LO in our analysis to equate the number of voxels from each participant and to increase power 30 . However, all results remained virtually identical when we included all voxels from LO. This indicates the stability and robustness of the results which did not depend on including the most reliable voxels.
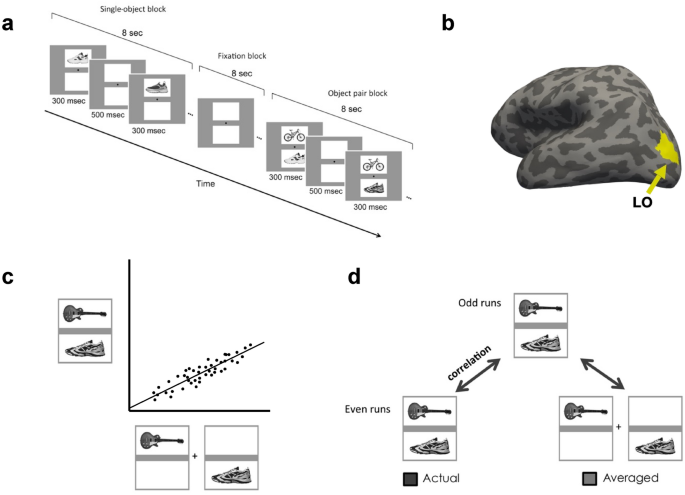
Experimental and analysis details. ( a ) Example trials of the main experiment. In single-object blocks, participants viewed a sequential presentation of single objects (different exemplars) from the same category at a fixed spatial location. In object pair blocks, they viewed a sequential presentation of object pairs from two different categories. The location of the objects from each of the two categories was fixed within a block. The task was to detect a 1-back repetition of the same object. In object pair blocks, the repetition could occur in either object category. The repetition occurred twice in each block. ( b ) Inflated brain surface from a representative participant showing LO. ( c ) A schematic illustration of the unit response analysis. We extracted, across all the included fMRI voxels/CNN units, the slope of the linear regression between an fMRI voxel/CNN unit’s response to a pair of objects and its summed response to the corresponding single objects shown in isolation. ( d ) A schematic illustration of the population response analysis. For LO, an object pair was correlated with itself across the odd and even halves of the runs (actual) and with the average of its constituent objects shown in isolation across the odd and even halves of the runs (average). For CNNs, because there is no noise, the object pair was simply correlated with the average of its constituent objects in isolation.
We also analyzed the higher layers from five CNNs pre-trained using ImageNet images 31 to perform object categorization. The CNNs examined included both shallower networks, Alexnet 25 and VGG-19 26 , and deeper networks, Googlenet 27 and Resnet-50 28 . We also included a recurrent network, Cornet-S, that has been shown to capture the recurrent processing in macaque IT cortex with a shallower structure and argued to be one of the current best models of the primate ventral visual system 18 , 32 . We further analyzed three versions of Resnet-50 trained on stylized versions of ImageNet images 33 to examine how reducing texture bias and increasing object shape processing in a CNN would impact its representation of multiple objects. For all CNNs, we created a comparable set of activation patterns as the brain data. In the fMRI experiment, objects were presented within a white rectangle on a gray background. It is possible that this gray background could affect object averaging as CNNs wouldn’t “filter out” the gray background as human participants would. We thus examined CNN responses to objects on both the gray and white backgrounds.
Evaluating the unit response to single and paired objects
In this analysis, we extracted, across all the included fMRI voxels/CNN units, the slope of the linear regression between an fMRI voxel/CNN unit’s response to a pair of objects and its summed response to the corresponding single objects shown in isolation. We averaged the slopes across all the human participants. The average slope should be 0.5 if the single fMRI voxel/CNN unit response to an object pair can be predicted by the average response of the corresponding objects shown alone. In a previous single-cell analysis of monkey IT, a slope of about 0.55 was reported 1 . We thus compared our slope results to both 0.5 and 0.55 as baselines. Following Jacob et al. 29 , we only selected CNN units that showed non-zero responses to objects at both locations.
The average slope for all participants when including the 75 most reliable voxels and all the voxels were 0.573 and 0.561, respectively (Fig. 3 a). These average slopes did not differ from the reported IT neuron slope of 0.55 ( t (9) = 0.866, p = 0.694, d = 0.273, for the 75 most reliable voxels; and t (9) = 0.406, p = 0.694, d = 0.128, for all the voxels; corrected for multiple comparisons using the Benjamini–Hochberg method for two comparisons, see 34 ). These slopes, however, did deviate from the perfect averaging slope of 0.5 ( t (9) = 2.741, p = 0.046, d = 0.867, for the 75 most reliable voxels; and t (9) = 2.22, p = 0.054, d = 0.701 for all the voxels; corrected). Inspection of the distribution of the voxel response aggregated across all participants revealed a distribution around the line of best fit, similar to a normal distribution and the distribution seen in the IT neuron responses (Fig. 2 a). Overall, we observed averaging in single fMRI voxels in human LO comparable to that of single neurons in macaque IT.
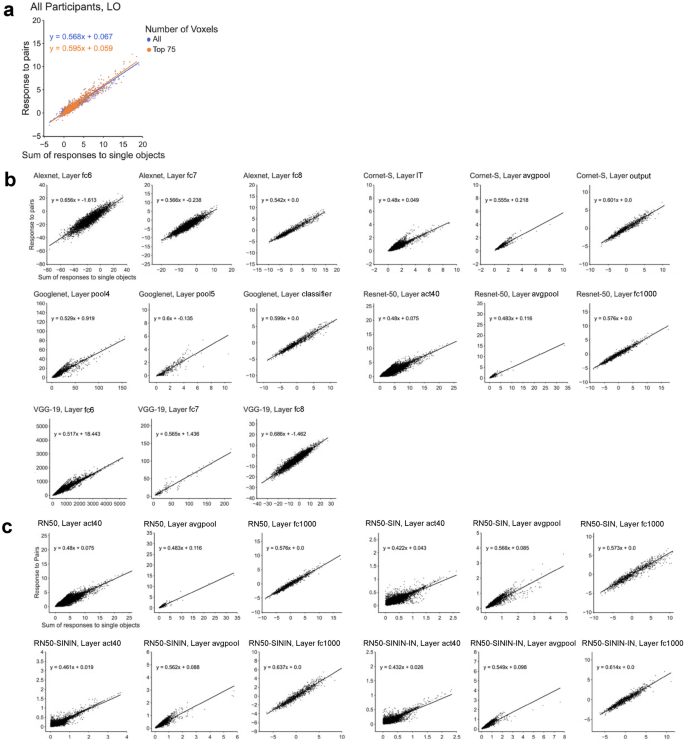
Single unit response amplitude distribution results. ( a ) Single voxel response to object pairs plotted against the sum of the individual object responses in LO for all of the participants for both the top 75 most reliable voxels and all the voxels. Units are in beta weights. ( b ) Responses of the five CNNs (pretrained on the original ImageNet images) to paired and single objects on gray background. ( c ) Responses of Resnet-50 to paired and single objects on gray background. Resnet-50 was pretrained either with the original ImageNet images (RN50-IN), the stylized ImageNet Images (RN50-SIN), both the original and the stylized ImageNet Images (RN50-SININ), or both sets of images, and then fine-tuned with the stylized ImageNet images (RN50-SININ-IN).
When the slopes from the sampled CNN layers were directly tested against the slope obtained from the human LO for the 75 most reliable voxels, 8 out of the 15 (53%) examined layers did not differ from LO for either the white or gray background images ( ts < 2.05, ps > 0.11; all others, ts > 2.82, ps < 0.06; see the asterisks marking the significance levels on Fig. 3 b; all pairwise comparisons reported here and below were corrected for multiple comparisons using the Benjamini–Hochberg method). When we further examined the layers that showed the greatest correspondence to LO as reported in a previous study 14 , 4 out of the 9 (44%) layers were not significantly different from LO (see the layers marked with bold font in Fig. 3 b). There was little effect of image background (i.e., whether the images appeared on the white or gray backgrounds), with only 3 out the 15 (20%) layers showing a discrepancy when comparing with LO (i.e., with performance on one background being similar and performance on the other background being different from that of LO). For the other 12 layers, performance (as compared to LO) did not differ across the two backgrounds. Overall, among the CNNs tested, Googlenet best resembled the human LO, with all of its sampled layers showing no significant difference from LO. Interestingly, the recurrent network examined, Cornet-S, did not seem to behave differently from the other networks.
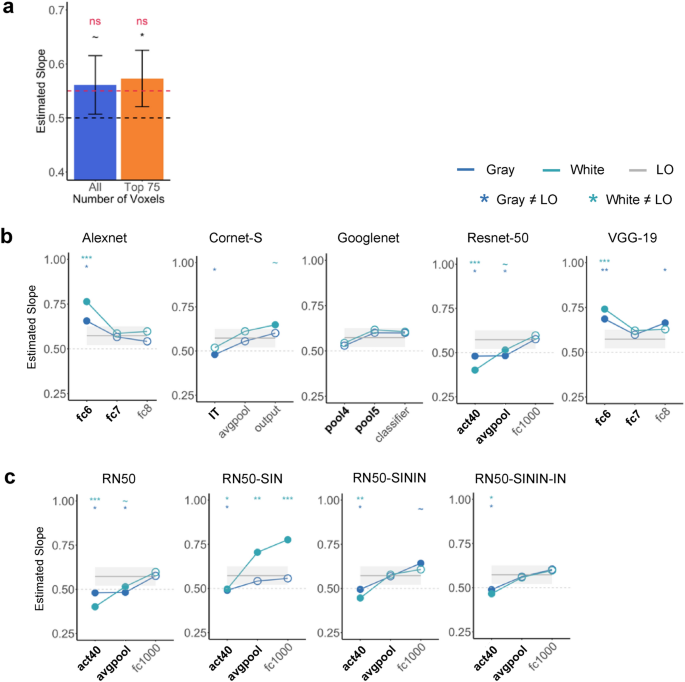
Single unit response summary results. ( a ) The averaged slope across all participants for both the top 75 most reliable LO voxels and for all the LO voxels. The average slope is compared to the slope of a perfect averaging (0.5) as well as the slope reported in single cells in macaque IT (0.55). ( b ) Comparing LO slope with those of 5 CNNs with the objects appearing on both the gray and white backgrounds. Bold layers were those that showed best correspondence with LO as shown by Xu and Vaziri-Pashkam 14 . For each layer, significant values for pairwise t tests against the slope of LO are marked with asterisks at the top of the plot. ( c ) Comparing LO slope with those of Resnet-50 under different training regimes. Resnet-50 was pretrained either with the original ImageNet images (RN50-IN), the stylized ImageNet Images (RN50-SIN), both the original and the stylized ImageNet Images (RN50-SININ), or both sets of images, and then fine-tuned with the stylized ImageNet images (RN50-SININ-IN). All t tests were corrected for multiple comparisons using the Benjamini–Hochberg method for 2 comparisons in LO, and for 6 comparisons (3 layers × 2 background colors) for each CNN. Error bars and ribbons represent the between-subjects 95% confidence interval of the mean. ~ 0.05 < p < 0.10, *p < 0.05, **p < 0.01, ***p < 0.001.
In Jacob et al. 29 , there was a noticeable improvement in unit averaging from lower to higher layers. Here we found that, except for Googlenet, the other CNNs all showed an improvement between the first two examined layers. Resnet-50 additionally showed an improvement between the last two examined higher layers.
Close inspection revealed that the distribution of the CNN unit responses to object pair and single objects varied greatly across the examined CNN layers, with some showing a distribution resembling that of a normal distribution and those of LO voxels and IT neurons, but others deviating greatly from such a distribution even when the average slope was close to 0.5 or 0.55 (Fig. 2 b). For example, although layer fc1000 in Resnet-50 and layer pool4 in Googlenet had slopes close to 0.5 and 0.55 (0.576 and 0.529, respectively), Resnet-50 had an approximately normal distribution while Googlenet does not. Additionally, although layer fc1000 in Resnet-50 and layer fc6 in Alexnet had approximately normal distributions, their slopes were very different from each other (0.576 and 0.656, respectively). This suggested that the representation of multiple objects in some higher CNN layers was different from that of the human LO and macaque IT.
Evaluating the pattern response to single and paired objects
In this analysis, we wanted to replicate the existence of averaging in fMRI response patterns as reported in Jeong and Xu 7 such that response patterns for an object pair may be predicted by the average pattern of the constituent objects shown in isolation. We also tested whether such averaging existed in CNN unit response patterns. To analyze the fMRI pattern responses, as in Jeong and Xu 7 , we conducted a split-half analysis to account for measurement noise (as correlation could never reach 1 even for the same condition across different halves of the data due to the presence of measurement noise in fMRI data). Specifically, we divided the runs into even and odd halves and averaged the response patterns for each condition within each half of the runs. We then correlated the response patterns of the same object pairs across even and odd halves (actual pair correlation) and correlated the response patterns of an object pair from one half and the average response pattern of the constituent single objects in the other half (average pair correlation). Finally, using the actual pair correlation as a baseline, we derived a noise-normalized correlation by dividing the average pair correlation by the actual pair correlation. If averaging existed in response patterns, then the response pattern of an object pair would be as similar to itself in the other half of the runs as it would be to the averaged response patterns of the single objects in the other half of the runs. We would then have a noise-normalized correlation no different from 1. For the CNNs, because there was no noise, we simply correlated the response pattern of a pair of objects with the average response pattern of the constituent single objects. We then compared this correlation to that of LO. We additionally assessed whether a weighted average of single objects would improve pattern averaging. We did so by systematically varying the contribution of the two single objects’ response patterns from 0 to 100%, with 10% increments in between.
In LO, the noise-normalized correlation did not differ from 1 for neither the Top 75 voxels ( t (9) = 1.588, p = 0.146, d = 0.502; one-tailed as only comparison in one direction was meaningful here) nor all voxels ( t (9) = 0.498, p = 0.315, d = 0.157; one-tailed) (corrected for two comparisons) (Fig. 4 a). Additional analyses showed that weighing the two constituent objects equally yielded the best averaging prediction (Fig. 5 c). These results replicated those of Jeong and Xu 7 and showed the existence of averaging in fMRI response patterns in human LO.
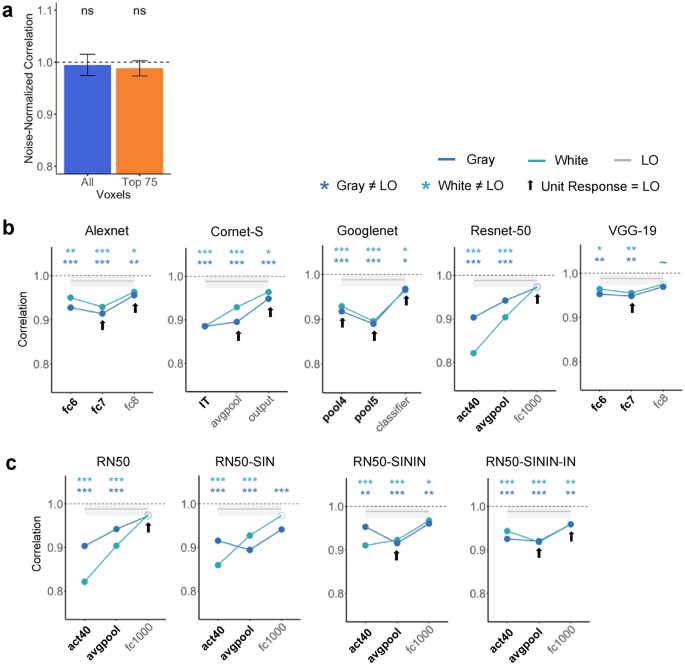
Pattern response results. ( a ) Noise-normalized correlation between the pattern response to an object pair and the average pattern response to the individual objects in LO for the top 75 most reliable voxels and all the voxels. A one-sided t-test was conducted comparing the correlations to 1. ( b ) Comparing pattern averaging in LO with those of five CNNs with the objects appearing on both the gray and white backgrounds. Bold layers were those that showed best correspondence with LO as shown by Xu and Vaziri-Pashkam 14 . For each layer, significant values for pairwise t tests against LO are marked with asterisks at the top of the plot. Layers showing comparable averaging in unit response amplitude to that of LO are marked with an arrow. ( c ) Comparing pattern averaging in LO with that of Resnet-50 under different training regimes. Resnet-50 was pretrained either with the original ImageNet images (RN50-IN), the stylized ImageNet Images (RN50-SIN), both the original and the stylized ImageNet Images (RN50-SININ), or both sets of images, and then fine-tuned with the stylized ImageNet images (RN50-SININ-IN). All t tests were corrected for multiple comparisons using the Benjamini–Hochberg method for 2 comparisons in LO, and for 6 comparisons (3 layers × 2 background colors) for each CNN. Error bars and ribbons represent the between-subjects 95% confidence interval of the mean. ~ 0.05 < p < 0.10, *p < 0.05, **p < 0.01, ***p < 0.001.
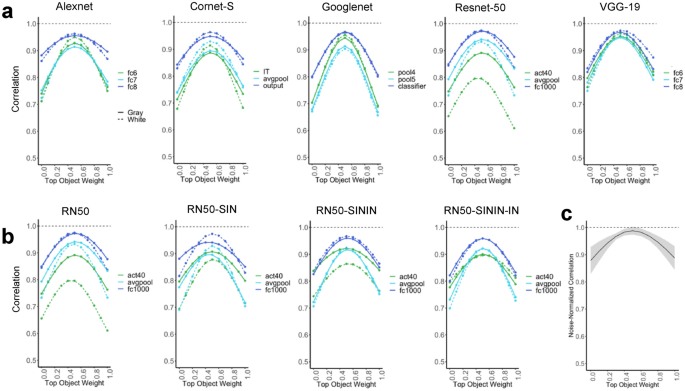
Pattern averaging results with a weighted average of the two single objects, where the weight of each object varies between 0 and 1 with increments of 0.1. ( a ) Weighted pattern averaging for the five CNNs trained on the original ImageNet images. ( b ) Weighted pattern averaging for Resnet-50. Resnet-50 was pretrained either with the original ImageNet images (RN50-IN), the stylized ImageNet Images (RN50-SIN), both the original and the stylized ImageNet Images (RN50-SININ), or both sets of images, and then fine-tuned with the stylized ImageNet images (RN50-SININ-IN). ( c ) Weighted pattern averaging for LO including all the voxels.
Overall, all sampled CNN layers showed a correlation in pattern response greater than 0.8. However, with the exception of the last sampled layer in Resnet-50, for all others, the correlation was significantly less than the noise-normalized correlation of the top 75 voxels in LO regardless of the background color (see the asterisks marking the significance levels in Fig. 4 b). This suggests that pattern averaging of single object responses could not fully predict that of the paired objects in most of the sampled CNN layers, different from the results obtained in human LO (Fig. 4 b). This was true even for layers showing strong averaging in single unit response amplitudes as shown in Fig. 3 b and marked on Fig. 4 b, indicating a discrepancy between unit and pattern responses in these CNN layers. This was also true for Cornet-S, the recurrent network that included feedback connections among the units to better model the primate visual system. Of all the CNN layers sampled, only the final layer of Resnet-50 exhibited pattern correlation and single unit responses matching those of LO (see Figs. 3 b, 4 b). That being said, both Cornet-S and Resnet-50 showed an improvement in averaging between the first two sampled layers, and all CNNs showed an improvement between the last two sampled layers, although such improvement was still not sufficient to reach the performance of LO. Additional analyses showed that, similar to LO, an equally weighted averaging of both constituent objects yielded the best pattern prediction (Fig. 5 a).
Evaluating the relationship between unit response and pattern response
In this analysis, we wanted to gain a better understanding of the relationship between unit and population responses and why there might be a discrepancy between unit and pattern responses in CNNs. We evaluated whether single units showing better averaging in response amplitude also show better averaging in response pattern. We divided LO voxels/CNN units into two groups by whether the slope in response amplitude averaging for the single voxel/CNN unit was near or far from 0.5, with the near condition defined as having a slope between 0.45 and 0.55 and the far condition defined as having a slope less than 0.45 or greater than 0.55. We then assessed averaging in response patterns in the near and far groups of voxels and units separately. We also noted the percentage of voxels/CNN units in the two groups.
We included all LO voxels in this analysis. Overall, approximately 75% of the total voxels across all of the participants had a slope near 0.5 (Fig. 6 a). Such a distribution is consistent with the voxel distribution seen in Fig. 6 a. While there was no difference between the noise-normalized pattern correlation and 1 for the near voxels ( t (9) = 1.942, p = 0.958, d = 0.614; corrected), the difference was marginally significant for the far voxels ( t (9) = 2.029, p = 0.073, d = 0.642; corrected), with a significant difference between the near and far voxels ( t (9) = 2.52, p = 0.033, d = 1.06). In human LO, voxels with a better averaging response in response amplitude thus also showed better averaging in response patterns.
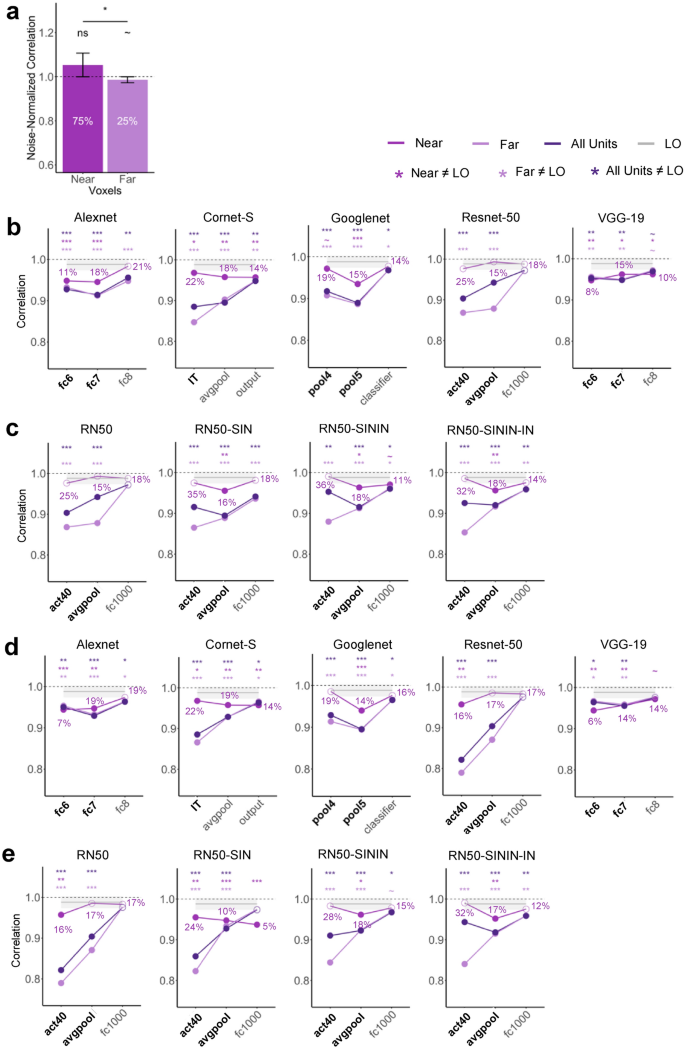
Relationship between unit and pattern responses. ( a ) Noise-normalized correlation between the pattern response to the pair and the average pattern response to the individual objects in LO for voxels that have slope near 0.5 (0.45 < slope < 0.55) and for voxels that have slope far from 0.5 (slope < 0.45 or slope > 0.55). The percentage of voxels considered to be near or far from slope 0.5 are marked. A one-sided t-test was conducted comparing the correlations to 1. ( b ) CNN pattern averaging results for images with gray background, separately for CNN units with slope near 0.5, units with slope far from 0.5, and all units, all compared with pattern averaging from LO. The percentages of units near slope 0.5 are marked. Bold layers were those that showed best correspondence with LO as shown by Xu and Vaziri-Pashkam 14 . For each layer, significant values for pairwise t tests against the slope of LO are marked with asterisks at the top of the plot. ( c ) For Resnet-50 under different training regimes, CNN pattern averaging results for images with gray background, separately for CNN units with slope near 0.5, units with slope far from 0.5, and all units, all compared with pattern averaging from LO. Resnet-50 was pretrained either with the original ImageNet images (RN50-IN), the stylized ImageNet Images (RN50-SIN), both the original and the stylized ImageNet Images (RN50-SININ), or both sets of images, and then fine-tuned with the stylized ImageNet images (RN50-SININ-IN). ( d ) CNN pattern averaging results for images with white background, separately for the three groups of units as in ( b ). ( e ) Resnet-50 pattern averaging results for images with white background, separately for the three groups of units as in c. All t tests were corrected for multiple comparisons using the Benjamini–Hochberg method for 2 comparisons in LO, and for 9 comparisons (3 layers × 3 groups of units) in each CNN. Error bars and ribbons represent the between-subjects 95% confidence interval of the mean. ~ 0.05 < p < 0.10, *p < 0.05, **p < 0.01, ***p < 0.001.
In CNNs, depending on the layer and network, only 5–35% of the total units had a slope near 0.5 (Fig. 6 b–e). This distribution was very different from that of LO where 75% of the voxels had a slope near 0.5. This confirmed the observation made earlier when we inspected the distribution of the unit responses (see Fig. 2 a,b) and once again showed that extracting the slope across all the units could significantly obscure differences in the overall unit response distributions. For the CNN units that were near 0.5, we did see improvement in pattern averaging, with 10/14 (71.4%) of the layers that did not previously show strong pattern averaging now showing increased averaging regardless of image background (see the asterisks marking the significance levels in Fig. 6 b,d). However, only 3/14 (21.4%) of these layers now showed averaging no different from LO. Thus, better averaging in unit response amplitudes improved but rarely led to full pattern averaging similar to that found in the human LO.
Evaluating the effect of shape training on unit and pattern normalization
Because CNNs trained on ImageNet images are biased toward texture rather than shape processing 33 , we evaluated whether CNNs biased towards shape processing may exhibit more LO like averaging in both response amplitude and response patterns. We tested 3 versions of Resnet-50 trained with stylized ImageNet images that emphasizes shape processing: Resnet-50 trained on Stylized ImageNet alone (RN50-SIN), Resnet-50 trained on both Original and Stylized ImageNet, and Resnet-50 trained on both Original and Stylized ImageNet (RN50-SININ), with fine-tuning on ImageNet (RN50-SININ-IN) 33 .
At the single unit level, when we compared the slopes of Resnet-50 trained on just the Original ImageNet images with those that were trained on Stylized ImageNet images, we saw the biggest improvement occurred in RN50-SININ-IN (Fig. 3 c). The original Resnet-50 was only comparable to LO in the final layer, but RN50-SININ-IN was comparable in the last two layers. Meanwhile, at the population level, such shape-training did not improve averaging in response patterns (Fig. 4 c). Thus, training Resnet-50 to emphasize shape rather than texture processing showed some improvement at the single unit level, but not at the population level.
Previous monkey neurophysiology, human fMRI and CNN studies have reported that neural/CNN responses to a pair of unrelated objects can be well predicted by the average responses to each constituent object shown in isolation, allowing primate vision to recover the identity of an object regardless of whether or not other objects are encoded concurrently. Such an averaging relationship has been found at the single unit level in the slope of response amplitudes of macaque IT neurons and CNN units to paired and single objects 1 , 29 , 35 and at the population level in response patterns of fMRI voxels in the human ventral object processing region 3 , 4 , 5 , 6 , 7 . Although it is assumed that averaging at the single unit and population levels must reflect the same underlying process, the only human fMRI study that has examined this appeared to indicate the absence of such averaging across all the LO voxels 7 . By evaluating the slope of single unit responses, object-categorization-trained CNN units are also shown to exhibit the same averaging responses as the macaque IT neurons 29 . However, averaging the slope across units ignores the overall response distribution, potentially obscuring important differences in how CNNs and the primate brain represent multiple objects. To address these questions, here we first tested whether averaging at the single unit level similar to that found in IT neurons may exist in single fMRI voxels. Conversely, we tested whether averaging in response patterns as observed in human fMRI studies may be present in CNN unit response patterns. We then examined whether voxels/units showing better averaging in response amplitude exhibit better response pattern averaging at the population level. To accomplish these goals, we analyzed fMRI responses from the human LO from an existing data set 7 . We also examined responses from five CNNs pretrained for object categorization, including Alexnet, Cornet-S, Googlenet, Resnet-50, and VGG-19.
In human LO, replicating Jeong and Xu 7 , we observed averaging in fMRI response pattern between paired and single objects. Unlike MacEvoy and Epstein 3 , we also observed averaging in the response amplitude of single fMRI voxels across LO similar to that reported in single neuron recordings in macaque IT 1 . Specifically, across all the included fMRI voxels, the slope of the linear regression between an fMRI voxel’s response to a pair of objects and its summed response to the single objects was indistinguishable from that of the IT neurons. The same results were obtained whether the top 75 most reliable voxels or all the voxels were included, demonstrating the robustness of the effect. Thus, by directly applying the method used in neurophysiology, we show that the same result reported in monkey neurophysiology studies is present in fMRI voxels even though fMRI is an indirect measure of neural activity with each voxel containing millions of neurons. Further analyses revealed that about 75% of LO voxels showed a slope close to 0.5 when the slope was measured within each voxel. As expected, voxels showing better averaging in response amplitude (i.e., with a slope close to 0.5) also exhibited better averaging in response patterns.
CNNs have been considered by some as the current best models of the primate ventral visual system 32 , 36 . Meanwhile, large discrepancies in object representation also exist between the primate brain and CNNs 14 , 15 , 19 , 33 , 37 . In a previous study, Jacob et al. 29 observed averaging in unit responses in the higher layers of VGG-16 pretrained for object categorization. Here in the higher layers of five CNNs with varying architecture, processing depth, and the presence/absence of recurrent processing, we observed in about half of the sample layers response amplitude averaging in the CNN units comparable to those found in the human LO (i.e., the slope across all the included units did not differ from that of LO). Except for Googlenet, which showed equally good averaging as the human LO in all the sampled layers, for the other four CNNs, there was a general increase in averaging from the lower to the higher sampled layers. Overall, these results replicated and extended the results of Jacob et al. 29 and showed that averaging, as assessed by the response amplitude slope across all the units, exists in CNN unit responses for representing a pair of objects at the higher levels of CNN visual processing.
Despite the prevalence of averaging in CNN single unit responses, except for the final layers of Resnet-50, no CNN higher layers showed averaging in response patterns comparable to that of the human LO regardless of differences in the exact architecture, depth, and presence/absence of recurrent processing. For example, the recurrent CNN we tested, Cornet-S, which was designed to closely model ventral visual processing, did not outperform the other CNNs. This was also true for layers showing good correspondence with LO in object representational structure as reported in a separate study (Ref. 14 ; note that the final sampled layer of Resnet-50, which showed the best pattern averaging as LO, did not show the best correspondence with LO in representational structure). There is thus a discrepancy between unit and pattern responses in CNNs. Further investigation revealed that, in many of the higher CNN layers sampled, the distribution of unit responses to paired and single objects did not resemble that of the human LO or macaque IT. In fact, when we divided up the units into two groups based on whether the response amplitude of a unit showed good or bad averaging (i.e., whether the slope was near or far from 0.5), we found that only a minority of the CNN units showed good averaging (5–35% depending on the layer) compared to the majority of the LO voxels that showed good averaging (75%). Thus, extracting the slope across all the units and comparing that with the brain obscured significant distributional differences between the brain and CNNs, leading to the impression that CNNs represent paired objects in a similar way as the primate brain, but in fact the majority do not. At the pattern level, when a large number of units failed to show averaging, even though the averaged slope from all the units would not be far from 0.5, the pattern would deviate significantly from averaging. In this regard, pattern analysis is a more sensitive way of measuring averaging than the single unit slope analysis. Like the LO voxels, CNN units with good averaging also tended to show better averaging in response patterns, but this rarely led to full pattern averaging similar to that found in the human LO.
In the present study, we also considered three factors that may impact the results. In the fMRI experiment, objects were presented within a white rectangle on a gray background. It is possible that this gray background could affect object averaging as CNNs wouldn’t “filter out” the gray background as human participants would. We thus examined CNN responses to objects on both the gray and white backgrounds, but found very little effect of background on CNN unit response amplitude or response pattern. In the main analyses, we only considered a simple average in which the response of each object contributed equally to the prediction of the response of the paired objects. In additional analyses, we also weighed the two objects differently but found that an equal weighting best predicted the response of object pairs from single objects in both the human LO and higher CNN layers. Lastly, to account for the texture bias observed in CNNs, we examined responses from Resnet-50 trained with the stylized versions of ImageNet images that emphasized shape processing 33 . While we observed some improvement in averaging in single unit responses, we saw no improvement in pattern averaging. Such a CNN training regime thus does not appear to make the CNN representation of the paired objects to be more brain-like.
Overall, we found that averaging existed in both single fMRI voxels and voxel population responses in the human LO, with better averaging at the single voxels leading to better averaging in fMRI response patterns. Although CNNs exhibited averaging at the unit level when the slope of response amplitudes for single and pair objects were considered across all the units, detailed investigation revealed that unit response distribution in most cases did not resemble those of the human LO or macaque IT. Consequently, averaging at the population level for the CNN units did not match that of LO. The whole is thus not equal to the average of its parts in CNNs.
Both monkey neurophysiology and human fMRI studies have shown that simple averaging no longer holds when two objects interact with each other, such as being connected 37 or forming familiar and meaningful pairs (e.g., a water jug and a cup 38 ). It would be interesting to include object pairs during CNN training and test whether the inclusion of these object pairs would further increase their interaction in CNN representation. Meanwhile, given that the specific object pairs we used here were unlikely to have appeared together in the CNN training data, our observed interactions in their representations when they were shown together still indicates that object representations formed in the current CNNs are likely very different from those in the primate brain.
Instead of examining CNNs trained for object classification, one could test averaging in CNNs trained for scene classification, as the training images in this case naturally contain multiple objects embedded in a co-occurring background. Consequently, averaging for single and paired objects may be present in such networks. In a human fMRI study, MacEvoy and Epstein 4 reported that while fMRI response patterns evoked by scenes in LO could be well predicted by the average patterns elicited by the signature objects in the scene, this was not the case in the parahippocampal place area, a brain region specialized for scene processing 39 . In other words, specialization in scene processing appears to result in an interaction, rather than the independent representations, of the objects in a scene. This predicts that scene-trained CNNs would not exhibit averaging for single and paired objects. However, we recently reported that object- and scene-trained CNNs represent the identities and the configuration of the objects in a scene in a similar way 40 , suggesting that scene-trained CNNs are not necessarily more sensitive to object configurations than object-trained CNNs. That said, it could still be useful for future studies to directly compare averaging in scene-trained CNNs and in object-trained CNNs.
It may be argued, however, that the pattern averaging results from CNNs still resemble those of the brain, even when there were significant differences. Given that the exact same visual features were present in an object pair and in single objects, a high correlation in CNN representations between the actual pair and the averaged pair was not surprising. However, while in the human brain, the paired object representation was equivalent to the average representation of the single objects shown alone, this was not the case in CNNs. Here, we are not interested in a “glass half empty vs half full” debate, but rather what the significant difference seen in CNNs would imply. As noted before, one of the hallmarks of primate high-level vision is its ability to extract object identity features among changes in non-identity features and form transformation-tolerant object representations 9 , 10 , 11 . This allows us to rapidly recognize an object under different viewing conditions. One aspect of this is clutter tolerance, the ability to form the same object representation regardless of whether it is shown alone or with another object 41 . Our results showed that in CNNs, object representations interacted with each other when objects were shown together and differed significantly from when objects were shown alone. In other words, CNNs distorted an object’s representation when it was shown with another object. Such a distortion would make object representations formed in CNNs context dependent and could well underlie the two major drawbacks current CNNs face: the requirement of large training data and the inability to generalize to new objects not included in training 15 . Our study thus identifies a potential limitation in the computational algorithms employed by current CNNs and suggests that CNNs do not form the same kind of transformation-tolerant visual object representations as the human brain. This echoes the finding from another recent study of ours which shows that, unlike human vision, CNNs do not maintain the object representational structure at higher levels of visual processing when objects undergo transformations involving changes such as position or size 42 .
In the primate brain, neural averaging has been linked to a normalization process that involves dividing the response of a neuron by a factor that includes a weighted sum of the activity of a pool of neurons through feedforward, lateral or feedback connections 22 , 23 , 24 . Future CNN architecture development may explicitly impose such a circuit property at higher levels of visual processing. It may also be possible to impose brain-like object averaging in CNN unit responses within the current architecture during training. Such manipulations may not only make CNNs exhibit brain-like averaging in object representations but may also help them overcome some of their current limitations, making CNNs both better models for the primate brain and better models for object recognition.
Materials and methods
In this study we reanalyzed data from an existing fMRI data set 7 where participants viewed both object pairs and their constituent single objects in a 1-back repetition detection task. We extracted fMRI responses from lateral occipital cortex (LO), a higher ventral visual object processing region homologous to the macaque IT. We also extracted CNN unit responses to the same images from five CNNs pre-trained on object recognition using ImageNet images 30 . We examined both shallower networks, including Alexnet 25 and VGG-19 26 , and deeper networks, including Googlenet 27 and Resnet-50 28 . We also included a recurrent network, Cornet-S, that has been shown to capture the recurrent processing in macaque IT cortex with a shallower structure, and is argued to be one of the current best models of the primate ventral visual system 18 , 32 . As an additional test, because CNNs trained on ImageNet images are biased toward texture rather than shape processing, we evaluated Resnet-50 trained with stylized ImageNet images that emphasized shape processing 33 . We examined single unit responses in human LO fMRI voxels and in CNN higher layer units. We also examined population responses in human LO fMRI voxel response patterns and in CNN unit response patterns. We then compared single unit and population responses both within human LO and CNNs and between the two systems.
The details of the fMRI experiment has been reported in a previous publication 6 . They are summarized here for the readers’ convenience.
Participants
Ten paid participants (eight women) took part in the study with informed consent. They all had normal or corrected-to-normal visual acuity, were right-handed, and between 18 and 35 years old (M = 29.33 years, SD = 3.08 years). One additional participant was tested but excluded from data analysis due to excessive head motion (> 3 mm). The study was approved by the institutional review board of Harvard University. All experiments were performed in accordance with relevant guidelines and regulations.
Experimental design and procedures
Main experiment.
Participants viewed blocks of images in the main experiment (Fig. 1 ). Each single-object block contained a sequential presentation of 10 images from the same object category either all above or all below the central fixation. Each object pair block contained a sequential presentation of two streams of 10 exemplars from two different categories with one always above and one always below the central fixation. Participants performed a 1-back repetition detection task and pressed a response key whenever they detected an immediate repetition of the exemplar. A repetition occurred twice in each block. In object pair blocks, the repetition occurred randomly in either the upper or lower location. The presentation order of the stimulus blocks and the presentation order of the exemplars within each block were randomly chosen. Four object categories (shoe, bike, guitar, and couch) with each containing 10 different exemplars were shown. All the exemplars from a given object category were shown in the same view and thus shared a similar outline. This allowed us to increase the difficulty of the 1-back task, thereby increasing participants’ attentional engagement on the task. This also resulted in objects from different categories to be more distinctive from each other. There were eight unique single-object blocks (4 object categories × 2 locations) and 12 unique object pair blocks (with all possible combinations of object categories and locations included).
Each exemplar image subtended approximately 5.5° × 2.8°. Two white square placeholders (7° × 4.7°), marking the two exemplar locations, were shown above and below the central fixation throughout a block of trials. The distance between the central fixation and the center of each placeholder was 3.2°. Each stimulus block lasted 8 s and contained 10 images, with each appearing 300 ms followed by a 500-ms blank interval. Fixation blocks, which lasted 8 s, were inserted at the beginning and end of the run and between each stimulus block. Each run contained 20 stimulus blocks, with each unique stimulus block appearing once, and 21 fixation blocks. Each participant was tested with 10 runs, each lasting 5 min 36 s. Participants’ eye movements during the main experiment were monitored with an EyeLink 1000 eye tracker to ensure proper central fixation.
LO localizer
Following Xu and Jeong 43 , to localize LO, participants viewed blocks of sequentially presented object and noise images (both subtended approximately 12° × 12°). Each object image contained four unique objects shown above, below, and to the left and right of the central fixation (the distance between the fixation and the center of each object was 4°). Gray-scaled photographs of everyday objects were used as the object stimuli. To prevent grouping between the objects, objects appeared on white placeholders (4.5° × 3.6°) that were visible throughout an object image block. Noise images were generated by phase-scrambling the object images used. Each block lasted 16 s and contained 20 images, with each image appearing for 500 ms followed by a 300-ms blank display. Participants were asked to detect the direction of a slight spatial jitter (either horizontal or vertical), which occurred randomly once in every 10 images. Eight object blocks and eight noise blocks were included in each run. Each participant was tested with two or three runs, each lasting 4 min 40 s.
MRI methods
fMRI data were acquired from a Siemens (Erlangen, Germany) Tim Trio 3-T scanner at the Harvard Center for Brain Science (Cambridge, MA). Participants viewed images back-projected onto a screen at the rear of the scanner bore through an angled mirror mounted on the head coil. All experiments were controlled by an Apple MacBook Pro laptop running MATLAB (The MathWorks Natick, MA) with Psychtoolbox extensions 44 . For the anatomical images, high-resolution T1-weighted images were acquired (repetition time = 2200 ms, echo time = 1.54 ms, flip angle = 7°, 144 slices, matrix size = 256 × 256, and voxel size = 1 × 1 × 1 mm). Functional data in the main experiment and in the LO localizer were acquired using the same gradient-echo echo-planar T2*-weighted sequence (repetition time = 2000 ms, echo time = 30 ms, flip angle = 90°, 31 slices, matrix size = 72 × 72, voxel size = 3 × 3 × 3 mm, 168 volumes for the main experiment and 140 volumes for the LO localizer).
Data analysis
Fmri data processing.
fMRI data were analyzed using FreeSurfer (surfer.nmr.mgh.harvard.edu), FsFast 45 , and in-house MATLAB and Python codes. FMRI data preprocessing included 3D motion correction, slice timing correction and linear and quadratic trend removal. No smoothing was applied to the data. The first two volumes of all functional runs were also discarded. All the analysis for the main experiment was performed in the volume. The ROIs were selected on the surface and then projected back to the volume for further analysis.
LO was defined separately in each participant as a cluster of continuous voxels in the lateral occipital cortex showing higher activations to the object images than to the noise images ( p < 0.001 uncorrected; Figure b. For two participants, the threshold of p < 0.001 resulted in too few voxels so the threshold was relaxed to p < 0.01.
For each participant, a general linear model with 20 factors (8 single-object conditions and 12 object-pair conditions) was applied to the fMRI data from the main experiment, and beta values were extracted from each stimulus block in each run and in each voxel of LO.
To equate the number of voxels across participants and to increase power, we selected 75 most reliable voxels in each ROI using reliability-based voxel selection 30 . This is based on the fact that, across the participants, the LO ROI ranged from 75 to 240 voxels before voxel selection. This method selects voxels whose response profiles are consistent across odd and even halves of the runs and works well when there are around 15 conditions. To implement this method, for each voxel, we calculated the split-half reliability by first averaging the runs within the odd and even halves and then correlating the resulting averaged responses for all conditions (20 in total) across the even and odd halves. We then selected the top 75 voxels with the highest correlations. The 75 voxels chosen had a moderate split-half reliability with an average of around r = 0.20 across the voxels and across the participants. We also analyze the data including all the voxels in LO and found virtually identical results.
CNN details
We tested 5 CNNs in our analyses (see Table 1 ). We examined both shallower networks, including Alexnet 25 and VGG-19 26 , and deeper networks, including Googlenet 27 and Resnet-50 28 . We also included a recurrent network, Cornet-S, that has been shown to capture the recurrent processing in macaque IT cortex with a shallower structure and argued to be one of the current best models of the primate ventral visual system 18 , 32 . All the CNNs used were pretrained with ImageNet images. As an additional test, because CNNs trained on ImageNet images are biased toward texture rather than shape processing, we evaluated 3 versions of Resnet-50 trained with stylized ImageNet images that emphasizes shape processing: Resnet-50 trained on Stylized ImageNet alone (RN50-SIN), Resnet-50 trained on both Original and Stylized ImageNet (RN50-SININ), and Resnet-50 trained on both Original and Stylized ImageNet, with fine-tuning on ImageNet (RN50-SININ-IN) 33 .
In previous studies 14 , 19 , 40 , 42 , 46 , 47 , we sampled 6 to 8 pooling and fully connected (FC) layers representative of all stages of visual processing in these same CNNs and compared them with the human ventral visual processing areas. Following Jacob et al. 29 , who have previously examined averaging in CNN single units, here we included only the 3 highest layers we sampled previously, as only these layers contained a large portion of units that respond to objects at both locations (see Table 1 for the specific CNN layers sampled). We included pooling layers here because they typically mark the end of processing for a block of layers when information is pooled to be passed on to the next block of layers. When there were no obvious pooling layers present, the last layer of a block was chosen. It has been shown previously that such a sampling procedure captures the evolution of the representation trajectory fairly well, if not fully, as adjacent layers exhibit identical or very similar representations 47 . In each CNN, the layer(s) showing stronger correlation with LO in their representational structure for real-world objects than other layers tested in the same CNN 14 were marked with bold font in all the results figures. For a given CNN layer, we extracted CNN unit responses to the 20 possible image conditions that the human participants saw in the scanner. These images all showed objects within a white rectangle on a gray background. As human participants could ignore the gray background and focus on the objects only but CNNs could not do so, we also extracted CNN unit responses to the same images on white backgrounds to assess the effect of background color on response averaging. Cornet-S and the three Stylized versions of Resnet-50 were implemented in Python. All other CNNs were implemented in Matlab. The output from all CNNs was analyzed and compared with brain responses using Python and R.
We followed the method of Zoccolan et al. 1 and Jacob et al. 29 to assess unit response to single and paired objects in single fMRI voxels in human LO and in CNN units (Fig. 1 c). In LO, for each participant, we first averaged the beta weights across all the runs for each condition in each voxel. We then extracted paired and single object responses from each of the 12 object pairs and averaged across all the pairs to generate an averaged paired and single object responses for that voxel. Finally, we included all the LO voxels for a given participant and extracted the slope of the linear regression between a voxel’s response to a pair of objects and its summed response to the corresponding single objects shown in isolation. The resulting slope was then averaged across the participants. The average slope should be 0.5 if the single voxel response to an object pair is equivalent to the average response of the corresponding objects shown alone. In a previous single-cell analysis of monkey IT, a slope of 0.55 was reported 1 . We thus compared our slope results to both 0.5 and 0.55 as baselines. For each CNN layer examined, we only included in the regression analysis units that were responsive to objects at both locations; in other words, units that showed a non-zero variance across the four single object conditions at each of the two locations. We then compared the slopes of the linear regressions of the CNN layers exacted using the same procedure as outlined above with that of human LO.
We followed the method of Jeong and Xu 7 to assess pattern response to single and paired objects in fMRI response patterns in human LO and in CNN unit response patterns (Fig. 1 d). In LO, to extract voxel response patterns, following established practice 48 , for each participant, we first z-normalized the beta values across all voxels for each condition in each run to remove amplitude differences between conditions and runs. We then divided the data into odd and even runs and averaged the runs within each half. We performed two correlations: correlating the voxel response pattern of the same object pair between odd and even runs (actual pair) and correlating the voxel response of an object pair and the average of its constituent objects shown alone between odd and even runs (averaged pair). To account for the fMRI measurement noise, we derived a noise-corrected correlation by dividing average pair correlation with actual pair correlation. If the noise-corrected correlation is no different from 1, it would indicate that the representation of an object pair is equivalent to the average representation of its constituent objects shown in isolation. This was done for each object pair and the results were averaged across all the object pairs for each participant. For CNNs, because there was no noise in the data, we simply calculated the response pattern correlation of an object pair and the average of its constituent objects. Similar to the CNN unit response analysis, we only included units that were responsive to objects at both locations. We then directly compared whether the correlations from the CNN layers were significantly different from that of the human LO.
To examine whether mechanisms other than a simple average or sum can predict the representation of an object pair, we additionally tested in both human LO and CNNs whether or not patterns generated by a weighted average model would better predict that of the actual object pair. To do so, we systematically varied the contribution of the two isolated objects’ patterns from 0 to 100%, with 10% increments in between. The resulting weighted average was then evaluated in the same way as before.
Here we examined whether fMRI voxels/CNN units with better averaging in response amplitude would exhibit better averaging in response pattern. To do so, we extracted the slope of the linear regression between an fMRI voxel/unit’s response to a pair of objects and its summed response to the corresponding single objects shown in isolation as before. We then sorted the voxels/CNN units into two groups based on whether their slopes were close to 0.5, defined as having a slope between 0.45 and 0.55 (referred to as the near condition), or whether they were far from 0.5, defined as having a slope less than 0.45 or greater than 0.55 (referred to as the far condition). Finally, we evaluated averaging in response patterns for the near and far voxels/units separately, and tested whether the near ones showed better pattern averaging than the far ones. We also noted the percentage of voxels/units that were either near or far.
Experimental design and statistical analyses
Ten human participants took part in the experiment. The factors described in the previous methods sections were evaluated at the group level using t-tests. One-tailed t tests were performed when the comparison in one direction was meaningful. We corrected for multiple comparisons in all post-hoc analyses using the Benjamini–Hochberg method 34 . For the fMRI analyses of LO, we corrected for two comparisons with a baseline level of normalization (0.5 or 0.55 for the unit response analysis and 1 for the population response analysis), accounting for including either all of the voxels or the top 75 most reliable voxels. For both the CNN unit and population analyses, for each CNN, we corrected for six comparisons with LO (three layers each and two background colors). When examining the relationship between CNN unit and population analyses, for each CNN, and for each background color, we corrected for nine comparisons (three layers and three groups of units included, i.e., near, far, and all units). We also calculated effect size using Cohen’s D 49 , 50 . All of the above statistical tests were conducted using R 51 .
Data availability
Data supporting the findings of this study are available at https://osf.io/nkdgf/ .
Zoccolan, D., Cox, D. D. & DiCarlo, J. J. Multiple object response normalization in monkey inferotemporal cortex. J. Neurosci. 25 , 8150–8164 (2005).
Article CAS PubMed PubMed Central Google Scholar
Bao, P. & Tsao, D. Y. Representation of multiple objects in macaque category-selective areas. Nat. Commun. 9 , 1774. https://doi.org/10.1038/s41467-018-04126-7 (2018).
Article ADS CAS PubMed PubMed Central Google Scholar
MacEvoy, S. P. & Epstein, R. A. Decoding the representation of multiple simultaneous objects in human occipitotemporal cortex. Curr. Biol. 19 , 943–947 (2009).
MacEvoy, S. P. & Epstein, R. A. Constructing scenes from objects in human occipitotemporal cortex. Nat. Neurosci. 14 , 1323–1329 (2011).
Reddy, L. & Kanwisher, N. Category selectivity in the ventral visual pathway confers robustness to clutter and diverted attention. Curr. Biol. 17 , 2067–2072 (2007).
Reddy, L., Kanwisher, N. G. & VanRullen, R. Attention and biased competition in multi-voxel object representations. Proc. Natl. Acad. Sci. U.S.A. 106 , 21447–21452 (2009).
Jeong, S. K. & Xu, Y. Task-context-dependent linear representation of multiple visual objects in human parietal cortex. J. Cogn. Neurosci. 29 , 1778–1789 (2017).
Article PubMed Google Scholar
Kliger, L. & Yovel, G. The functional organization of high-level visual cortex determines the representation of complex visual stimuli. J. Neurosci. 40 , 7545–7558 (2020).
DiCarlo, J. J. & Cox, D. D. Untangling invariant object recognition. Trends Cogn. Sci. 11 , 333–341 (2007).
DiCarlo, J. J., Zoccolan, D. & Rust, R. C. How does the brain solve visual object recognition? Neuron 73 , 415–434 (2012).
Tacchetti, A., Isik, L. & Poggio, T. A. Invariant recognition shapes neural representations of visual input. Annu. Rev. Vis. Sci. 4 , 403–422 (2018).
Khaligh-Razavi, S. M. & Kriegeskorte, N. Deep supervised, but not unsupervised, models may explain IT cortical representation. PLoS Comput. Biol. 10 , e1003915. https://doi.org/10.1371/journal.pcbi.1003915 (2014).
Cichy, R. M., Khosla, A., Pantazis, D., Torralba, A. & Oliva, A. Comparison of deep neural networks to spatio-temporal cortical dynamics of human visual object recognition reveals hierarchical correspondence. Sci. Rep. 6 , 27755. https://doi.org/10.1038/srep27755 (2016).
Xu, Y. & Vaziri-Pashkam, M. Limits to visual representational correspondence between convolutional neural networks and the human brain. Nat. Commun. 12 , 2065. https://doi.org/10.1038/s41467-021-22244-7 (2021).
Serre, T. Deep learning: The good, the bad, and the ugly. Annu. Rev. Vis. Sci. 5 , 399–426 (2019).
Yamins, D. L. K. et al. Performance-optimized hierarchical models predict neural responses in higher visual cortex. Proc. Natl. Acad. Sci. U.S.A. 111 , 8619–8624 (2014).
Bao, P., She, L., McGill, M. & Tsao, D. Y. A map of object space in primate inferotemporal cortex. Nature 583 , 103–108 (2020).
Kar, K., Kubilius, J., Schmidt, K., Issa, E. B. & DiCarlo, J. J. Evidence that recurrent circuits are critical to the ventral stream’s execution of core object recognition behavior. Nat. Neurosci. 22 , 974–983 (2019).
Xu, Y. & Vaziri-Pashkam, M. Examining the coding strength of object identity and nonidentity features in human occipito-temporal cortex and convolutional neural networks. J. Neurosci. 41 , 4234–4252 (2021).
Marr, D. Vision: A Computational Investigation into the Human Representation and Processing of Visual Information (W.H. Freeman, 1982).
Google Scholar
Kay, K. N. Principles for models of neural information processing. Neuroimage 180 , 101–109 (2018).
Carandini, M. & Heeger, D. J. Normalization as a canonical neural computation. Nat. Rev. Neurosci. 13 , 51–62 (2012).
Article CAS Google Scholar
Reynolds, J. H. & Heeger, D. J. The normalization model of attention. Neuron 61 , 168–185 (2009).
Heeger, D. J. Normalization of cell responses in cat striate cortex. Vis. Neurosci. 9 , 181–197 (1992).
Article CAS PubMed Google Scholar
Krizhevsky, A., Sutskever, I. & Hinton, G. E. ImageNet classification with deep convolutional neural networks. Adv. Neural Inf. Process. Syst. 25 , 1097–1105 (2012).
Simonyan, K. & Zisserman, A. Very deep convolutional networks for large-scale image recognition. Preprint at http://arxiv.org/abs/1409.1556 (2014).
Szegedy, C. et al . Going deeper with convolutions. In 2015 IEEE Conference on Computer Vision and Pattern Recognition (CVPR) 1–9 (2015).
He, K., Zhang, X., Ren, S. & Sun, J. Deep residual learning for image recognition. In 2016 IEEE Conference on Computer Vision and Pattern Recognition (CVPR) 770–778. https://doi.org/10.1109/CVPR.2016.90 (2016).
Jacob, G., Pramod, R. T., Katti, H. & Arun, S. P. Qualitative similarities and differences in visual object representations between brains and deep networks. Nat. Commun. 12 , 1872. https://doi.org/10.1038/s41467-021-22078-3 (2021).
Tarhan, L. & Konkle, T. Reliability-based voxel selection. Neuroimage 207 , 116350 (2019).
Deng, J., Dong, W., Socher, R., Li, L.-J., Li, K. & Fei-Fei, L. ImageNet: A large-scale hierarchical image database. In IEEE Conference on Computer Vision and Pattern Recognition 248–255. https://doi.org/10.1109/CVPR.2009.5206848 (2009).
Kubilius, J., Schrimpf, M. & Hong, H. Brain-like object recognition with high-performing shallow recurrent ANNs. In Thirty-Third Conference on Neural Information Processing Systems (Neural Information Processing Systems, 2019).
Geirhos, R. et al . ImageNet-trained CNNs are biased towards texture; increasing shape bias improves accuracy and robustness. Preprint at http://arxiv.org/abs/1811.12231 (2019).
Benjamini, Y. & Hochberg, Y. Controlling the false discovery rate: A practical and powerful approach to multiple testing. J. R. Stat. Soc. B Methods 57 , 289–300 (1995).
MathSciNet MATH Google Scholar
Reynolds, J. H., Chelazzi, L. & Desimone, R. Competitive mechanisms subserve attention in macaque areas V2 and V4. J. Neurosci. 19 , 1736–1753 (1999).
Cichy, R. M. & Kaiser, D. Deep neural networks as scientific models. Trends Cogn. Sci. 23 , 305–317 (2019).
Baker, C. I., Behrmann, M. & Olson, C. R. Impact of learning on representation of parts and wholes in monkey inferotemporal cortex. Nat. Neurosci. 5 , 1210–1216 (2002).
Baeck, A., Wagemans, J. & Op de Beeck, H. P. The distributed representation of random and meaningful object pairs in human occipitotemporal cortex: The weighted average as a general rule. Neuroimage 70 , 37–47 (2013).
Epstein, R. & Kanwisher, N. A cortical representation of the local visual environment. Nature 392 , 598–601 (1998).
Article ADS CAS PubMed Google Scholar
Tang, K., Chin, M., Chun, M. & Xu, Y. The contribution of object identity and configuration to scene representation in convolutional neural networks. PLoS ONE 17 , e0270667. https://doi.org/10.1371/journal.pone.0270667 (2022).
Rust, N. C. & DiCarlo, J. J. Selectivity and tolerance (“invariance”) both increase as visual information propagates from cortical area V4 to IT. J. Neurosci. 30 , 12978–12995 (2010).
Xu, Y. & Vaziri-Pashkam, M. Understanding transformation tolerant visual object representations in the human brain and convolutional neural networks. Neuroimage 263 , 119635 (2022).
Xu, Y. & Jeong, S. K. The contribution of human superior intra-parietal sulcus to visual short-term memory and perception. In Mechanisms of Sensory Working Memory: Attention and Performance XXV 1st edn (eds Jolicoeur, P. & Martinez-Trujillo, J.) 33–42 (Academic, 2015).
Chapter Google Scholar
Brainard, D. H. The psychophysics toolbox. Spat. Vis. 10 , 433–436 (1997).
Dale, A. M., Fischl, B. & Sereno, M. I. Cortical surface-based analysis: I. Segmentation and surface reconstruction. Neuroimage 9 , 179–194 (1999).
Mocz, V., Vaziri-Pashkam, M., Chun, M. & Xu, Y. Predicting identity-preserving object transformations in human posterior parietal cortex and convolutional neural networks. J. Cogn. Neurosci. 34 , 2406–2435 (2022).
Article PubMed PubMed Central Google Scholar
Taylor, J. & Xu, Y. Joint representation of color and shape in convolutional neural networks: A stimulus-rich network perspective. PLoS ONE 16 , e0253442. https://doi.org/10.1371/journal.pone.0253442 (2021).
Kamitani, Y. & Tong, F. Decoding the visual and subjective contents of the human brain. Nat. Neurosci. 8 , 679–685 (2005).
Cohen, J. Statistical Power Analysis for the Behavioral Sciences (Academic Press, 1969).
MATH Google Scholar
Cohen, J. Statistical Power Analysis for the Behavioral Sciences 2nd edn. (Erlbaum, 1988).
R Core Team. R: A Language and Environment for Statistical Computing . http://www.R-project.org/ (R Foundation for Statistical Computing, 2018).
Download references
Acknowledgements
The authors thank members of Visual Cognitive Neuroscience Lab, Turk-Browne Lab, Holmes Lab, Yale Cognitive and Neural Computation Lab, and Ilker Yildirim for their helpful feedback on this project. This research was supported by the National Institute of Health Grants 1R01EY030854 and 1R01EY022355 to Y.X.
Author information
Authors and affiliations.
Visual Cognitive Neuroscience Lab, Department of Psychology, Yale University, 2 Hillhouse Ave, New Haven, CT, 06520, USA
Viola Mocz, Marvin Chun & Yaoda Xu
Department of Neuroscience, Yale School of Medicine, New Haven, CT, 06520, USA
Marvin Chun
Department of Psychology, Chungbuk National University, Cheongju, South Korea
Su Keun Jeong
You can also search for this author in PubMed Google Scholar
Contributions
V.M., M.C. and Y.X. conceived the study; S.K.J. and Y.X. designed the fMRI experiments; S.K.J. implemented and conducted the experiments, and collected fMRI data; V.M. performed fMRI data preprocessing and beta weights extraction, and performed the normalization analysis; V.M. and Y.X. wrote the manuscript with comments from S.K.J. and M.C.
Corresponding author
Correspondence to Yaoda Xu .
Ethics declarations
Competing interests.
The authors declare no competing interests.
Additional information
Publisher's note.
Springer Nature remains neutral with regard to jurisdictional claims in published maps and institutional affiliations.
Rights and permissions
Open Access This article is licensed under a Creative Commons Attribution 4.0 International License, which permits use, sharing, adaptation, distribution and reproduction in any medium or format, as long as you give appropriate credit to the original author(s) and the source, provide a link to the Creative Commons licence, and indicate if changes were made. The images or other third party material in this article are included in the article's Creative Commons licence, unless indicated otherwise in a credit line to the material. If material is not included in the article's Creative Commons licence and your intended use is not permitted by statutory regulation or exceeds the permitted use, you will need to obtain permission directly from the copyright holder. To view a copy of this licence, visit http://creativecommons.org/licenses/by/4.0/ .
Reprints and permissions
About this article
Cite this article.
Mocz, V., Jeong, S.K., Chun, M. et al. Multiple visual objects are represented differently in the human brain and convolutional neural networks. Sci Rep 13 , 9088 (2023). https://doi.org/10.1038/s41598-023-36029-z
Download citation
Received : 23 January 2023
Accepted : 27 May 2023
Published : 05 June 2023
DOI : https://doi.org/10.1038/s41598-023-36029-z
Share this article
Anyone you share the following link with will be able to read this content:
Sorry, a shareable link is not currently available for this article.
Provided by the Springer Nature SharedIt content-sharing initiative
By submitting a comment you agree to abide by our Terms and Community Guidelines . If you find something abusive or that does not comply with our terms or guidelines please flag it as inappropriate.
Quick links
- Explore articles by subject
- Guide to authors
- Editorial policies
Sign up for the Nature Briefing newsletter — what matters in science, free to your inbox daily.

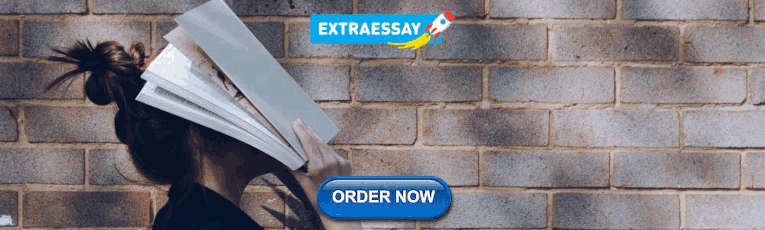
IMAGES
VIDEO
COMMENTS
Weight in Water Jugs = 32 Pounds. So at the store I had to know what 32lbs felt like. It's equal to 4 gallons. I.lost.the.weight.of.four.gallons. It is odd to think of weight in the form of an ...
40 pounds equals a five-gallon bottle of water or an average human leg. 44 pounds equals an elephant's heart. 50 pounds equals a small bale of hay. 55 pounds equals a 5,000-BTU air conditioner. 66 pounds equals the fats and oils an average American eats in a year. 70 pounds equals an Irish Setter.
About. VisualBMI shows you what weight looks like on a human body. Using a large index of photos of men and women, you can get sense of what people look like at different weights or even the same weight. If you find this website useful, please share it or send a note. Credits. Huge thanks to all those who share their stories and photos on reddit.
Through the power of visualization, our interactive 3D human modeling simulator allows you to create personalized virtual representations of yourself. You can fine-tune your height, weight, gender, and shape; your on-screen figure will update to represent the details you've entered. Our website is the perfect tool for those looking to gain or ...
Calculate your BMI and Visualize your Body Shape. Body Mass Index (BMI) is calculated using your height and weight and is approximately related to body fat percentage. Rotate the body to get a full 360o degree view. |. male.
Background This randomised controlled study evaluated a computer-generated future self-image as a personalised, visual motivational tool for weight loss in adults. Methods One hundred and forty-five people (age 18-79 years) with a Body Mass Index (BMI) of at least 25 kg/m2 were randomised to receive a hard copy future self-image at recruitment (early image) or after 8 weeks (delayed image ...
Here are some general guidelines for the number of daily servings from each food group*: Grains and starchy vegetables: 6-11 servings a day. Nonstarchy vegetables: 3-5 servings a day. Dairy: 2-4 servings a day. Lean meats and meat substitutes: 4-6 ounces a day or 4-6 one-ounce servings a day. Fruit: 2-3 servings a day.
The Body Visualizer Is A Fun 3D Body Shape Before & After Weight Loss Tool! It Helps You Visualize Your Ideal Weight & Monitor Your Progress. The Body Visualizer Game Uses Your Body Type, Height, Current Weight And Goal Weight To Display A Virtual Model Of You At Your Desired Weight. Most Individuals Initially Are Excited To Lose Weight.
Weight is perceived through the combination of multiple sensory systems, and a wide range of factors - including touch, visual, and force senses - can influence the perception of heaviness. There have been remarkable advancements in the development of haptic interfaces throughout the years. However, a number of challenges limit the progression to enable humans to sense the weight in ...
Charmed, I'm Sure. SparkPeople member TWEETYX2 wears a charm bracelet every day as a visual aid to remind herself, minute by minute, of her weight loss goals. Each charm represents a different source of motivation, such as a fork and spoon to remember to track her food intake, an apple as a symbol of healthy snacks, a crescent moon to remind ...
Virtual Models. Most virtual models offer some level of customization such as choosing hair and eye color, skin tone and even basic features such as face shape and body shape. This helps create a model that looks similar to your appearance. When you input your height, weight and measurements into the simulation, you create an on-screen 'before ...
Recent hierarchical convolutional neural networks (CNNs) have achieved human-like object categorization performance 1,2,3,4.It has additionally been shown that representations formed in lower and ...
Other Weight Loss Simulators. In addition to the Visualize You app, there are a number of online simulators that can help you visualize what your body look likes after weight loss. 1. Change in Seconds. Change in Seconds is a virtual weight loss imager that uses your height, weight, body type (apple, hourglass, pear) and your goal weight. While ...
A clustering around the center would indicate lots of "bullseyes", and lots of points on the outside indicate misses. But I also want to show that a bullseye is meaningless if the weight is 0, and a miss is huge if the weight is 100. Since it's a circular plot, I can use angle, distance, color, and dot size.
Weight loss comparison to objects. Let's look at how different amounts of weight loss translate into various household items. 2lb - A large bag of sugar. 2lb (1kg) of body fat takes up around 1000 cubic centimeters or just over 4 cups in volume. That's quite a lot! Losing this amount of fat is the equivalent of a losing a large bag of ...
Object identification is not simply object naming, it is recall of the constellation of properties and associations that distinguish this object from others. It is the process in which perception and memory become intertwined [4]. We might therefore view weight as being part of the identity of an object ('the heavy cylinder') rather than ...
Human behavioural studies have documented how object representations emerge through grouping, amodal completion, proto-objects and object files. By contrast, deep neural network models of visual ...
Read the weight loss comparison list below and share with OH members what your weight loss is equal to! 1 pound = a loaf of bread. 2 pounds = an IPad or the Imperial State Crown. 3 pounds = a can of Crisco vegetable oil. 4 pounds = a Reebok 4-Pound Ankle Wrap Weight set. 5 pounds = the world's largest gummy bear (Five-pound gummy bear is equal ...
Deep neural networks are widely considered as good models for biological vision. Here, we describe several qualitative similarities and differences in object representations between brains ...
Weight Loss Comparison Chart! I am much more of a visual person than a number person, so when I see weight loss progress, I always imagine the number as objects, rather than just a number. I put together this chart to show just what a few pounds really means. Hopefully it serves as a good motivational tool for those of you who are down on ...
Nor would you want to! In fact fat is so essential to our health that an average size 10, slim female carries approximately 100,000 calories of fat on her body. The average woman* 5 ft. 4 in. weighs 166 lbs. Average fat% of 25-31% The average man* 5 ft. 9 in. weighs 196 lbs. Average fat% of 18-24% 46.5 Ibs. of fat 41.2 Ibs. of fat * In the U.S ...
In two studies, the same participants prepared and then executed lifting actions with objects of varying weight. In the first study, we show that when lifting visually identical objects, where predicted weight is based solely on sensorimotor memory, weight is represented in object-sensitive OTC. In the second study, we show that when object ...
To form object representations independent of whether or not other objects are encoded concurrently, in the primate brain, responses to an object pair are well approximated by the average ...