Academia.edu no longer supports Internet Explorer.
To browse Academia.edu and the wider internet faster and more securely, please take a few seconds to upgrade your browser .
Enter the email address you signed up with and we'll email you a reset link.
- We're Hiring!
- Help Center
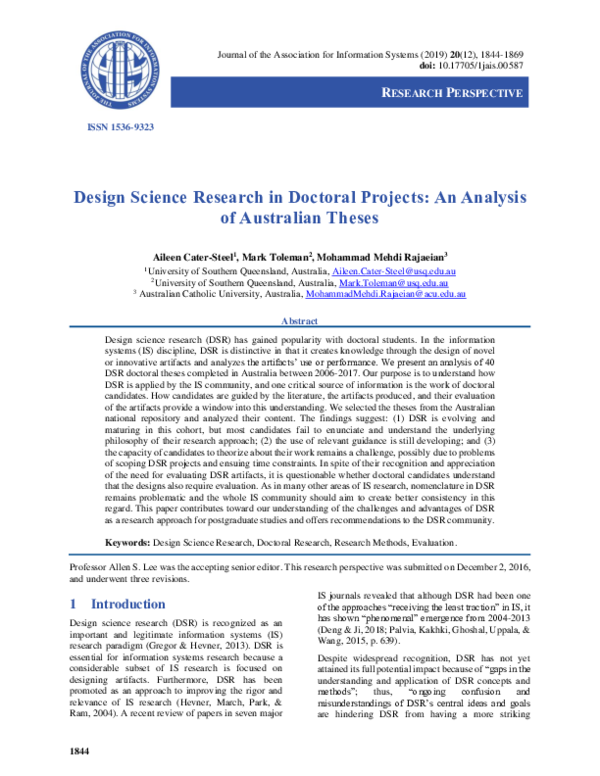
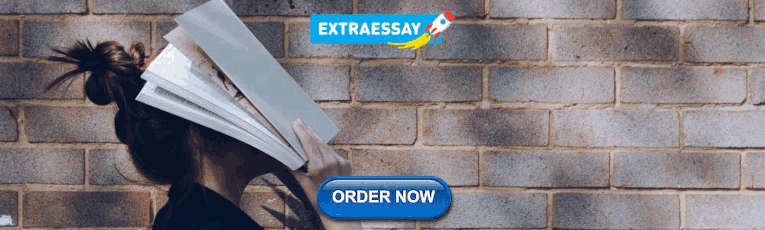
Design Science Research in Doctoral Projects: An Analysis of Australian Theses

Journal of the Association for Information Systems
Related Papers
ICT Education
Hanlie Smuts
Design science research (DSR) is well-known in different domains, including information systems (IS), for the construction of artefacts. One of the most challenging aspects of IS postgraduate studies (with DSR) is determining the structure of the study and its report, which should reflect all the components necessary to build a convincing argument in support of such a study’s claims or assertions. Analysing several postgraduate IS-DSR reports as examples, this paper presents a mapping between recommendable structures for research reports and the DSR process model of Vaishnavi and Kuechler, which several of our current postgraduate students have found helpful.
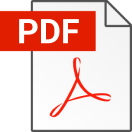
Management Information Systems Quarterly
Shirley Gregor
Informing Science: The International Journal of an Emerging Transdiscipline
Manuel Meireles
Aim/Purpose: To discuss the Design Science Research approach by comparing some of its canons with observed practices in projects in which it is applied, in order to understand and structure it better. Background: Recent criticisms of the application of the Design Science Research (DSR) approach have pointed out the need to make it more approachable and less confusing to overcome deficiencies such as the unrealistic evaluation. Methodology: We identified and analyzed 92 articles that presented artifacts developed from DSR projects and another 60 articles with preceding or subsequent actions associated with these 92 projects. We applied the content analysis technique to these 152 articles, enabling the preparation of network diagrams and an analysis of the longitudinal evolution of these projects in terms of activities performed and the types of artifacts involved. Contribution: The content analysis of these 152 articles enabled the preparation of network diagrams and an analysis of t...
Tuure Tuunanen , Kenneth Peffers , Johanna Bragge
Rahul Thakurta
Design science is an increasingly popular research paradigm in the information systems discipline. De- spite a recognition of the design science research par- adigm, questions are being raised about the nature of its existence and its contributions. Central to this ar- gument is the understanding of the relationship be- tween “theoretical research” and “design research” and the necessary implications for design. In this re- search, we contribute to this discourse by carrying out a structured literature review in order to appreciate the current state of the art in design science research. The results identify an incongruence between the methodological guidelines informing the design and how the design is carried out in practice. On the basis of our observations on the design process, the theoreti- cal foundations of design, and the design outcomes, we outline some research directions that we believe will contribute to methodically well-executed design sci- ence contributions in the f...
Proceedings of the 4th …
Olga Levina , Udo Bub
Discussions about the body of knowledge of information systems, including the research domain, relevant perspectives and methods have been going on for a long time. Many researchers vote for a combination of research perspectives and their respective research methodologies; rigour and relevance as requirements in design science are generally accepted. What has been lacking is a formalisation of a detailed research process for design science that takes into account all requirements. We have developed such a research process, building on top of existing processes and findings from design research. The process combines qualitative and quantitative research and references well-known research methods. Publication possibilities and self-contained work packages are recommended. Case studies using the process are presented and discussed.
Diane Strode , Susan Chard
Design science is a research paradigm where the development and evaluation of an artefact is a key contribution. Design science is used in many domains and this paper draws on those domains to formulate a generic structure for design science research suitable for small-scale postgraduate information technology research projects. The paper includes guidelines for writing proposals and a generic research report structure. The paper presents ethical issues to consider in design science research and contributes guidelines for assessment.
Design Science Research Cases
Jan vom Brocke
Design Science Research (DSR) is a problem-solving paradigm that seeks to enhance human knowledge via the creation of innovative artifacts. Simply stated, DSR seeks to enhance technology and science knowledge bases via the creation of innovative artifacts that solve problems and improve the environment in which they are instantiated. The results of DSR include both the newly designed artifacts and design knowledge (DK) that provides a fuller understanding via design theories of why the artifacts enhance (or, disrupt) the relevant application contexts. The goal of this introduction chapter is to provide a brief survey of DSR concepts for better understanding of the following chapters that present DSR case studies.
International Journal of Doctoral Studies
Seyum Getenet
Aim/Purpose: We show a new dimension to the process of using design-based research approach in doctoral dissertations. Background: Design-based research is a long-term and concentrated approach to educational inquiry. It is often a recommendation that doctoral students should not attempt to adopt this approach for their doctoral dissertations. In this paper, we document two doctoral dissertations that used a design-based research approach in two different contexts. Methodology : The study draws on a qualitative analysis of the methodological approaches of two doctoral dissertations through the lenses of Herrington, McKenney, Reeves and Oliver principles of design-based research approach. Contribution: The findings of this study add a new dimension to using design-based research approach in doctoral dissertations in shorter-term and less intensive contexts. Findings: The results of this study indicate that design-based research is not only an effective methodological approach in doct...
Communications in Computer and Information Science
Alta Van der Merwe
RELATED PAPERS
Journal of the Society of Dyers and Colourists
jiri militky
Journal of Food Quality
Leonardo Sabatino
Komputa : Jurnal Ilmiah Komputer dan Informatika
Ana Hadiana
Piero Del Soldato
Teresa Cristina Moraes Genro
Parasitology Research
Philippe Vignoles
Piyawat Wuttichaikitcharoen
Political Economy: Fiscal Policies & Behavior of Economic Agents eJournal
Andrew Morriss
James Fadokun
International Journal of Antimicrobial Agents
Samuel Kariuki
Journal of Leukocyte Biology
Michelle Azevedo
RESUMEN COTEC
DEVORA CEDEÑO
Titi Prihatin
Scientific Reports
Sandra Gomez
Anuario Filosófico
Lourdes Flamarique
Cognitive Social Science eJournal
Brett Freudenberg
Semina: Ciências Agrárias
camila soares batista
Jan Hendrickx
BOHR International Journal of Computer Science
Narjes alarsali
Roos Gerritsen
Ecehan Aras
Pedagogy, Culture and Society
Anna-Maija Pirttilä-Backman
Chemical Science
Alexander Hepp
Journal of Molecular Evolution
Bernhard Lieb
See More Documents Like This
RELATED TOPICS
- We're Hiring!
- Help Center
- Find new research papers in:
- Health Sciences
- Earth Sciences
- Cognitive Science
- Mathematics
- Computer Science
- Academia ©2024
Publications
Conversational Agents (CAs) have become a new paradigm for human-computer interaction. Despite the potential benefits, there are ethical challenges to the widespread use of these agents that may inhibit their use for individual and social goals. However, besides a multitude of behavioral and design-oriented studies on CAs, a distinct ethical perspective falls rather short in the current literature. In this paper, we present the first steps of our design science research project on principles for a value-sensitive design of CAs. Based on theoretical insights from 87 papers and eleven user interviews, we propose preliminary requirements and design principles for a value-sensitive design of CAs. Moreover, we evaluate the preliminary principles with an expert-based evaluation. The evaluation confirms that an ethical approach for design CAs might be promising for certain scenarios.
This essay derives a schema for specifying design principles for information technology-based artifacts in sociotechnical systems. Design principles are used to specify design knowledge in an accessible form, but there is wide variation and lack of precision across views on their formulation. This variation is a sign of important issues that should be addressed, including a lack of attention to human actors and levels of complexity as well as differing views on causality, on the nature of the mechanisms used to achieve goals, and on the need for justificatory knowledge. The new schema includes the well-recognized elements of design principles, including goals in a specific context and the mechanisms to achieve the goal. In addition, the schema allows: (i) consideration of the varying roles of the human actors involved and the utility of design principles; (ii) attending to the complexity of IT-based artifacts through decomposition;(iii) distinction of the types of causation (i.e., deterministic versus probabilistic); (iv) a variety of mechanisms in achieving aims; and (v) the optional definition of justificatory knowledge underlying the design principles. We illustrate the utility of the proposed schema by applying it to examples of published research.
Kuhns Thema ist der Prozeß, in dem wissenschaftliche Erkenntnisse erzielt werden. Fortschritt in der Wissenschaft - das ist seine These - vollzieht sich nicht durch kontinuierliche Veränderung, sondern durch revolutionäre Prozesse. Dabei beschreibt der Begriff der wissenschaftlichen Revolution den Vorgang, bei dem bestehende Erklärungsmodelle, an denen und mit denen die wissenschaftliche Welt bis dahin gearbeitet hat, abgelöst und durch andere ersetzt werden: es findet ein Paradigmenwechsel statt.
Sir Isaac Newton famously said, "If I have seen further it is by standing on the shoulders of giants." Research is a collaborative, evolutionary endeavor-and it is no different with design science research (DSR) which builds upon existing design knowledge and creates new design knowledge to pass on to future projects. However, despite the vast, growing body of DSR contributions, scant evidence of the accumulation and evolution of design knowledge is found in an organized DSR body of knowledge. Most contributions rather stand on their own feet than on the shoulders of giants, and this is limiting how far we can see; or in other words, the extent of the broader impacts we can make through DSR. In this editorial, we aim at providing guidance on how to position design knowledge contributions in wider problem and solution spaces. We propose (1) a model conceptualizing design knowledge as a resilient relationship between problem and solution spaces, (2) a model that demonstrates how individual DSR projects consume and produce design knowledge, (3) a map to position a design knowledge contribution in problem and solution spaces, and (4) principles on how to use this map in a DSR project. We show how fellow researchers, readers, editors, and reviewers, as well as the IS community as a whole, can make use of these proposals, while also illustrating future research opportunities.
We develop an empirically grounded understanding of how design knowledge accumulates over time. Drawing from theory on knowledge creation, we conceptualize accumulation along the goals and scope of knowledge in DSR as a distinct knowledge creation problem. Through two empirical studies, we theorize knowledge accumulation in DSR by unpacking (1) three knowledge creation mechanisms, and (2) explaining how their interplay forms different patterns of knowledge creation over time. We contribute a theoretical framework conducive to integrating knowledge produced through different methods by introducing the knowledge creation processes as a distinct unit of analysis in DSR and by pointing out that extant procedural models may need revision to account for the continuous knowledge creation occurring in potentially multiyear DSR projects.
The rising complexity of automotive software makes it increasingly difficult to develop the software with high quality in short time. Especially the late detection of early errors, such as requirement inconsistencies and ambiguities, often causes costly iterations. We address this problem with a new requirements specification and analysis technique based on executable scenarios and automated testing. The technique is based on the Scenario Modeling Language for Kotlin (SMLK), a Kotlin based framework that supports the modeling/programming of behavior as loosely coupled scenarios, which is close to how humans conceive and communicate behavioral requirements. Combined with JUnit, we propose the Test-Driven Scenario Specification (TDSS) process, which introduces agile practices into the early phases of development, significantly reducing the risk of requirement inconsistencies and ambiguities, and, thus, reducing development costs. We overview TDSS with the help of an example from the e-mobility domain, report on lessons learned, and outline open challenges.
More and more people use virtual assistants in their everyday life (e.g., on their mobile phones, in their homes, or in their cars). So-called vehicle assistance systems have evolved over the years and now perform various proactive tasks. However, we still lack concrete guidelines with all the specifics that one needs to consider to build virtual assistants that provide a convincing user experience (especially in vehicles). This research provides guidelines for designing virtual in- vehicle assistants. The developed guidelines offer a clear and structured overview of what designers have to consider while designing virtual in-vehicle assistants for a convincing user experience. Following design science research principles, we designed the guidelines based on the existing literature on the requirements of assistant systems and on the results from interviewing experts. In order to demonstrate the applicability of the guidelines, we developed a virtual reality prototype that considered the design guidelines. In a user experience test with 19 participants, we found that the prototype was easy to use, allowed good interaction, and increased the users’ overall comfort.
Digitalization triggers a shift in the compositions of skills and knowledge needed for students in their future work life. Hence, higher order thinking skills are becoming more important to solve future challenges. One subclass of these skills, which contributes significantly to communication, collaboration and problem-solving, is the skill of how to argue in a structured, reflective and well-formed way. However, educational organizations face difficulties in providing the boundary conditions necessary to develop this skill, due to increasing student numbers paired with financial constraints. In this short paper, we present the first steps of our design science research project on how to design an adaptive IT-tool that helps students develop their argumentation skill through formative feedback in large-scale lectures. Based on scientific learning theory and user interviews, we propose preliminary requirements and design principles for an adaptive argumentation learning tool. Furthermore, we present a first instantiation of those principles.
Innovation in the medical technology (med tech) industry has a major impact on well-being in society. Open innovation has the potential to accelerate the development of new or improved healthcare solutions. Building on work system theory (WST), this paper explores how a multi-sided open innovation platform can systematically be established in a German med tech industry cluster in situations where firms had no prior experience with this approach. We aim to uncover problems that may arise and identify opportunities for overcoming them. We performed an action research study in which we implemented and evaluated a multi-sided web-based open innovation platform in four real-world innovation challenges. Analyzing the four different challenges fostered a deeper understanding of the conceptual and organizational aspects of establishing the multi-sided open innovation platform as part of a larger work system. Reflecting on the findings, we developed five design principles that shall support the establishment of multi-sided open innovation platforms in other contexts. Thus, this paper contributes to both theory and practice.
One of the most critical tasks for startups is to validate their business model. Therefore, entrepreneurs try to collect information such as feedback from other actors to assess the validity of their assumptions and make decisions. However, previous work on decisional guidance for business model validation provides no solution for the highly uncertain and complex context of earlystage startups. The purpose of this paper is, thus, to develop design principles for a Hybrid Intelligence decision support system (HI-DSS) that combines the complementary capabilities of human and machine intelligence. We follow a design science research approach to design a prototype artifact and a set of design principles. Our study provides prescriptive knowledge for HI-DSS and contributes to previous work on decision support for business models, the applications of complementary strengths of humans and machines for making decisions, and support systems for extremely uncertain decision-making problems.
Posing research questions represents a fundamental step to guide and direct how researchers develop knowledge in research. In design science research (DSR), researchers need to pose research questions to define the scope and the modes of inquiry, characterize the artifacts, and communicate the contributions. Despite the importance of research questions, research provides few guidelines on how to construct suitable DSR research questions. We fill this gap by exploring ways of constructing DSR research questions and analyzing the research questions in a sample of 104 DSR publications. We found that about two-thirds of the analyzed DSR publications actually used research questions to link their problem statements to research approaches and that most questions focused on solving problems. Based on our analysis, we derive a typology of DSR question formulation to provide guidelines and patterns that help researchers formulate research questions when conducting their DSR projects.
Design science research (DSR) aims to deliver innovative solutions for real-world problems. DSR produces Information Systems (IS) artifacts and design knowledge describing means-end relationships between problem and solution spaces. A key success factor of any DSR research endeavor is an appropriate understanding and description of the underlying problem space. However, existing DSR literature lacks a solid conceptualization of the problem space in DSR. This paper addresses this gap and suggests a conceptualization of the problem space in DSR that builds on the four key concepts of stakeholders, needs, goals, and requirements. We showcase the application of our conceptualization in two published DSR projects. Our work contributes methodologically to the field of DSR as it helps DSR scholars to explore and describe the problem space in terms of a set of key concepts and their relationships.
To remain competitive, businesses need to develop innovative and profitable products, processes and services. The development of innovation relies on novel ideas, which can be generated during creative workshops. In this context the Design Thinking approach, a problem-solving methodology based on collaboration, user-centricity and creativity, may be used. However, guidance and moderation of this process require a vast amount of skills and knowledge. As technologies like artificial intelligence have the potential of making machines our collaboration partner in the future, creating virtual assistants adapting human behaviors is promising. To reduce cognitive dissonance and stress on both the moderators and participants, we investigate the potential of a virtual assistant to support moderation in a Design Thinking process to improve innovative output as well as perceived satisfaction. We therefore developed design guidelines for virtual assistants supporting creative workshops based on qualitative expert interviews and related literature following the Design Science Research Methodology.
With the rising interest in Design Science Research (DSR), it is crucial to engage in the ongoing debate on what constitutes an acceptable contribution for publishing DSR - the design artifact, the design theory, or both. In this editorial, we provide some constructive guidance across different positioning statements with actionable recommendations for DSR authors and reviewers. We expect this editorial to serve as a foundational step towards clarifying misconceptions about DSR contributions and to pave the way for the acceptance of more DSR papers to top IS journals.
This paper reports on the results of a design science research (DSR) study that develops design principles for information systems (IS) that support organisational sensemaking in environmental sustainability transformations. We identify initial design principles based on salient affordances required in organisational sensemaking and revise them through three rounds of developing, demonstrating and evaluating a prototypical implementation. Through our analysis, we learn how IS can support essential sensemaking practices in environmental sustainability transformations, including experiencing disruptive ambiguity through the provision of environmental data, noticing and bracketing, engaging in an open and inclusive communication and presuming potential alternative environmentally responsible actions. We make two key contributions: First, we provide a set of theory-inspired design principles for IS that support sensemaking in sustainability transformations, and revise them empirically using a DSR method. Second, we show how the concept of affordances can be used in DSR to investigate how IS can support organisational practices. While our findings are based on the investigation of the substantive context of environmental sustainability transformation, we suggest that they might be applicable in a broader set of contexts of organisational sensemaking and thus for a broader class of sensemaking support systems.
Design Science Research (DSR) is now an accepted research paradigm in the Information Systems (IS) field, aiming at developing purposeful IT artifacts and knowledge about the design of IT artifacts. A rich body of knowledge on approaches, methods, and frameworks supports researchers in conducting DSR projects. While methodological guidance is abundant, there is little support and guidance for documenting and effectively managing DSR processes. In this article, we present a set of design principles for tool support for DSR processes along with a prototypical implementation (MyDesignProcess.com). We argue that tool support for DSR should enable researchers and teams of researchers to structure, document, maintain, and present DSR, including the resulting design knowledge and artifacts. Such tool support can increase traceability, collaboration, and quality in DSR. We illustrate the use of our prototypical implementation by applying it to published cases, and we suggest guidelines for using tools to effectively manage design-oriented research.
In order to generate valuable innovations, it is important to come up with potential beneficial ideas. A well-known method for collective idea generation is Brainstorming and with Electronic Brainstorming, individuals can virtually brainstorm. However, an effective Brainstorming facilitation always needs a moderator. In our research, we designed and implemented a virtual moderator that can automatically facilitate a Brainstorming session. We used various artificial intelligence functions, like natural language processing, machine learning and reasoning and created a comprehensive Intelligent Moderator (IMO) for virtual Brainstorming.
This paper reports on the results of a study to investigate how scholars engage with and use the action design research (ADR) approach. ADR has been acknowledged as an important variant of the Design Science Research approach, and has been adopted by a number of scholars, as the methodological basis for doctoral dissertations as well as multidisciplinary research projects. With this use, the research community is learning about how to apply ADR’s central tenets in different contexts. In this paper, we draw on primary data from researchers who have recently engaged in or finished an ADR project to identify recurring problems and opportunities related to working in different ADR stages, balancing demands from practice and research, and addressing problem instance vs. class of problems. Our work contributes a greater understanding of how ADR projects are carried out in practice, how researchers use ADR, and pointers to possibilities for extending ADR.
In the IS discipline, the formulation of design principles is an important vehicle to convey design knowledge that contributes beyond instantiations applicable in a limited context of use. However, their formulation still varies in terms of orientation, clarity, and precision. In this paper, we focus on the design of artifacts that are oriented towards human use, and we identify and analyze three orientations in the formulation of such design principles in IS journals—action oriented, materiality oriented, and both action and materiality oriented. We propose an effective and actionable formulation of design principles that is both clear and precise.
This paper distinguishes and contrasts two design science research strategies in information systems. In the first strategy, a researcher constructs or builds an IT meta-artefact as a general solution concept to address a class of problem. In the second strategy, a researcher attempts to solve a client’s specific problem by building a concrete IT artefact in that specific context and distils from that experience prescriptive knowledge to be packaged into a general solution concept to address a class of problem. The two strategies are contrasted along 16 dimensions representing the context, outcomes, process and resource requirements.
Design research promotes understanding of advanced, cutting-edge information systems through the construction and evaluation of these systems and their components. Since this method of research can produce rigorous, meaningful results in the absence of a strong theory base, it excels in investigating new and even speculative technologies, offering the potential to advance accepted practice.
Unter „Mixed Methods“ wird üblicherweise die Kombination qualitativer und quantitativer Forschungsmethoden in einem Untersuchungsdesign verstanden. Es handelt sich um einen Begriff aus der anglo-amerikanischen Methodendebatte in den Sozial- und Erziehungswissenschaften, der seit dem Ende der 1990er-Jahre, konkret seit dem Erscheinen der Monographie „Mixed Methodology“ von Abbas Tashakkori und Charles Teddlie (1998) große Prominenz erlangt hat. Von den amerikanischen Erziehungswissenschaften ausgehend hat sich eine eigene Mixed Methods-Bewegung gebildet – mittlerweile existieren eine ganze Reihe von Lehrbüchern (etwa Creswell/Plano Clark 2007; Morse/Niehaus 2009; Kuckartz/Cresswell 2014), ein in zweiter Auflage erschienenes umfangreiches Handbuch (Tashakkori/Teddlie 2010), seit 2007 eine Zeitschrift mit Namen „Journal of Mixed Methods Research“ (JMMR) und eine internationale Fachgesellschaft unter dem Namen „Mixed Methods International Research Association“ (MMIRA).
Design science research (DSR) has staked its rightful ground as an important and legitimate Information Systems (IS) research paradigm. We contend that DSR has yet to attain its full potential impact on the development and use of information systems due to gaps in the understanding and application of DSR concepts and methods. This essay aims to help researchers (1) appreciate the levels of artifact abstractions that may be DSR contributions, (2) identify appropriate ways of consuming and producing knowledge when they are preparing journal articles or other scholarly works, (3) understand and position the knowledge contributions of their research projects, and (4) structure a DSR article so that it emphasizes significant contributions to the knowledge base. Our focal contribution is the DSR knowledge contribution framework with two dimensions based on the existing state of knowledge in both the problem and solution domains for the research opportunity under study. In addition, we propose a DSR communication schema with similarities to more conventional publication patterns, but which substitutes the description of the DSR artifact in place of a traditional results section. We evaluate the DSR contribution framework and the DSR communication schema via examinations of DSR exemplar publications.
Design research (DR) is an emergent research approach within information systems. There exist demands to clarify the meta-scientific foundations for this approach. Different responses to these demands are made. There exist attempts to position DR within interpretivism and critical realism. Some scholars have suggested pragmatism as an appropriate paradigm base for design research. This paper has taken pragmatism as a candidate paradigm and it has investigated and elaborated the epistemological foundations for DR. Different epistemic types of DR are identified using a pragmatist perspective. Design research is also related to four aspects/types of pragmatism: Local functional pragmatism (as the design of a useful artefact), general functional pragmatism (as creating design theories and methods aimed for general practice), referential pragmatism (focusing artefact affordances and actions) and methodological pragmatism (knowledge development through making).
One point of convergence in the many recent discussions on design science research in information systems (DSRIS) has been the desirability of a directive design theory (ISDT) as one of the outputs from a DSRIS project. However, the literature on theory development in DSRIS is very sparse. In this paper, we develop a framework to support theory development in DSRIS and explore its potential from multiple perspectives. The framework positions ISDT in a hierarchy of theories in IS design that includes a type of theory for describing how and why the design functions: Design-relevant explanatory/predictive theory (DREPT). DREPT formally captures the translation of general theory constructs from outside IS to the design realm. We introduce the framework from a knowledge representation perspective and then provide typological and epistemological perspectives. We begin by motivating the desirability of both directive-prescriptive theory (ISDT) and explanatory-predictive theory (DREPT) for IS design science research and practice. Since ISDT and DREPT are both, by definition, mid-range theories, we examine the notion of mid-range theory in other fields and then in the specific context of DSRIS. We position both types of theory in Gregor’s (2006) taxonomy of IS theory in our typological view of the framework. We then discuss design theory semantics from an epistemological view of the framework, relating it to an idealized design science research cycle. To demonstrate the potential of the framework for DSRIS, we use it to derive ISDT and DREPT from two published examples of DSRIS.
This paper explores which theorizing strategies can be employed in DSR to make a theoretical contribution by examining two illustrative case examples. First, we find that abduction, deduction, and induction all play a role in DSR. Second, we suggest that design theorists can choose among a range of theorizing strategies (i.e., inductive theorizing, deductive theorizing, and hybrid approaches) that differ in their degree to which they make use of abduction, deduction, and induction as well as their iterative sequencing over time in repeated theorizing cycles. Third, we reveal from the discussion of two prominent IS design theories that empirical and conceptual methods for theorizing play an important role in both the build and evaluate phases of the DSR cycle. Finally, we recommend theorists in future DSR projects that pursue the goal to develop design theory to think explicitly about their theorizing approach and select and use research methods accordingly.
Design Science Research for Information Systems (ISDSR) has received considerable attention recently. With the growing interest in ISDSR, calls continue to establish the rigor of artifact construction. In analogy to other scientific disciplines, the scientific foundation of artifact construction has been designated as IS Design Theory (ISDT) (Gregor 2006, p. 611). Although the ISDSR community has been discussing ISDTs since the early 1990s, no consensus on the definition or the componential structure of ISDTs has been reached yet. In this short article, we give an overview of the ongoing discussion on ISDTs. First, we introduce fundamental concepts of ISDT. Second, we give an overview on seminal contributions to the field of ISDTs in chronological order. Finally, we cluster the presented ISDT contributions into ISDT schools.
Prior research has identified the similarity of Action Research (AR) and Design Science Research (DSR). This paper analyses AR and DSR from several perspectives, including paradigmatic assumptions of ontology, epistemology, methodology, and ethics, their research interests, and activities. We identify that often AR does not share the paradigmatic assumptions and the research interests of DSR, that some activities in DSR are always mutually exclusive from AR, and that there may be no, little, or significant (but not total) overlaps between AR and DSR. Thus we judge that AR and DSR are decisively dissimilar. We further identify several key problems with combining AR and DSR based on the ethical requirement of researchers to identify and manage risks to research stakeholders. Management of such risks is done by careful disclosure, identifying research limitations or by choosing alternative methods than AR for accomplishing DSR.
The common understanding of design science research in information systems (DSRIS) continues to evolve. Only in the broadest terms has there been consensus: that DSRIS involves, in some way, learning through the act of building. However, what is to be built – the definition of the DSRIS artifact – and how it is to be built – the methodology of DSRIS – has drawn increasing discussion in recent years. The relationship of DSRIS to theory continues to make up a significant part of the discussion: how theory should inform DSRIS and whether or not DSRIS can or should be instrumental in developing and refining theory. In this paper, we present the exegesis of a DSRIS research project in which creating a (prescriptive) design theory through the process of developing and testing an information systems artifact is inextricably bound to the testing and refinement of its kernel theory.
Design work and design knowledge in Information Systems (IS) is important for both research and practice. Yet there has been comparatively little critical attention paid to the problem of specifying design theory so that it can be communicated, justified, and developed cumulatively. In this essay we focus on the structural components or anatomy of design theories in IS as a special class of theory. In doing so, we aim to extend the work of Walls, Widemeyer and El Sawy (1992) on the specification of information systems design theories (ISDT), drawing on other streams of thought on design research and theory to provide a basis for a more systematic and useable formulation of these theories. We identify eight separate components of design theories: (1) purpose and scope, (2) constructs, (3) principles of form and function, (4) artifact mutability, (5) testable propositions, (6) justificatory knowledge (kernel theories), (7) principles of implementation, and (8) an expository instantiation. This specification includes components missing in the Walls et al. adaptation of Dubin (1978) and Simon (1969) and also addresses explicitly problems associated with the role of instantiations and the specification of design theories for methodologies and interventions as well as for products and applications. The essay is significant as the unambiguous establishment of design knowledge as theory gives a sounder base for arguments for the rigor and legitimacy of IS as an applied discipline and for its continuing progress. A craft can proceed with the copying of one example of a design artifact by one artisan after another. A discipline cannot.
As a commentary to Juhani Iivari’s insightful essay, I briefly analyze design science research as an embodiment of three closely related cycles of activities. The Relevance Cycle inputs requirements from the contextual environment into the research and introduces the research artifacts into environmental field testing. The Rigor Cycle provides grounding theories and methods along with domain experience and expertise from the foundations knowledge base into the research and adds the new knowledge generated by the research to the growing knowledge base. The central Design Cycle supports a tighter loop of research activity for the construction and evaluation of design artifacts and processes. The recognition of these three cycles in a research project clearly positions and differentiates design science from other research paradigms. The commentary concludes with a claim to the pragmatic nature of design science.
The paper motivates, presents, demonstrates in use, and evaluates a methodology for conducting design science (DS) research in information systems (IS). DS is of importance in a discipline oriented to the creation of successful artifacts. Several researchers have pioneered DS research in IS, yet over the past 15 years, little DS research has been done within the discipline. The lack of a methodology to serve as a commonly accepted framework for DS research and of a template for its presentation may have contributed to its slow adoption. The design science research methodology (DSRM) presented here incorporates principles, practices, and procedures required to carry out such research and meets three objectives: it is consistent with prior literature, it provides a nominal process model for doing DS research, and it provides a mental model for presenting and evaluating DS research in IS. The DS process includes six steps: problem identification and motivation, definition of the objectives for a solution, design and development, demonstration, evaluation, and communication. We demonstrate and evaluate the methodology by presenting four case studies in terms of the DSRM, including cases that present the design of a database to support health assessment methods, a software reuse measure, an Internet video telephony application, and an IS planning method. The designed methodology effectively satisfies the three objectives and has the potential to help aid the acceptance of DS research in the IS discipline.
The aim of this research essay is to examine the structural nature of theory in Information Systems. Despite the importance of theory, questions relating to its form and structure are neglected in comparison with questions relating to epistemology. The essay addresses issues of causality, explanation, prediction, and generalization that underlie an understanding of theory. A taxonomy is proposed that classifies information systems theories with respect to the manner in which four central goals are addressed: analysis, explanation, prediction, and prescription. Five interrelated types of theory are distinguished: (1) theory for analyzing, (2) theory for explaining, (3) theory for predicting, (4) theory for explaining and predicting, and (5) theory for design and action. Examples illustrate the nature of each theory type. The applicability of the taxonomy is demonstrated by classifying a sample of journal articles. The paper contributes by showing that multiple views of theory exist and by exposing the assumptions underlying different viewpoints. In addition, it is suggested that the type of theory under development can influence the choice of an epistemological approach. Support is given for the legitimacy and value of each theory type. The building of integrated bodies of theory that encompass all theory types is advocated.
Within the information systems community there is growing interest in design theories. These theories are aimed to give knowledge support to design activities. Design theories are considered as theorized practical knowledge. This paper is an inquiry into the epistemology of design theories. It is an inquiry in how to justify such knowledge; the need to ground and how to ground a design theory. A distinction is made between empirical, theoretical and internal grounding. The empirical grounding has to do with the effectiveness of the application of knowledge. External theoretical grounding relates design theory to other theories. One part of this is the grounding of the design knowledge in general explanatory theories. Internal grounding means an investigation of internal warrants (e.g. as values and categories) and internal cohesion of the knowledge. Together, these different grounding processes form a coherent approach for the multi-grounding of design theory (MGDT). As illustrations some examples of design theories in IS are discussed. These are design theories concerning business interaction which are based on language action theories.
Two paradigms characterize much of the research in the Information Systems discipline: behavioral science and design science. The behavioral-science paradigm seeks to develop and verify theories that explain or predict human or organizational behavior. The design-science paradigm seeks to extend the boundaries of human and organizational capabilities by creating new and innovative artifacts. Both paradigms are foundational to the IS discipline, positioned as it is at the confluence of people, organizations, and technology. Our objective is to describe the performance of design-science research in Information Systems via a concise conceptual framework and clear guidelines for understanding, executing, and evaluating the research. In the design-science paradigm, knowledge and understanding of a problem domain and its solution are achieved in the building and application of the designed artifact. Three recent exemplars in the research literature are used to demonstrate the application of these guidelines. We conclude with an analysis of the challenges of performing high-quality design-science research in the context of the broader IS community.
Research in IT must address the design tasks faced by practitioners. Real problems must be properly conceptualized and represented, appropriate techniques for their solution must be constructed, and solutions must be implemented and evaluated using appropriate criteria. If significant progress is to be made, IT research must also develop an understanding of how and why IT systems work or do not work. Such an understanding must tie together natural laws governing IT systems with natural laws governing the environments in which they operate. This paper presents a two dimensional framework for research in information technology. The first dimension is based on broad types of design and natural science research activities: build, evaluate, theorize, and justify. The second dimension is based on broad types of outputs produced by design research: representational constructs, models, methods, and instantiations. We argue that both design science and natural science activities are needed to insure that IT research is both relevant and effective.
This paper defines an information system design theory (ISDT) to be a prescriptive theory which integrates normative and descriptive theories into design paths intended to produce more effective information systems. The nature of ISDTs is articulated using Dubin’s concept of theory building and Simon’s idea of a science of the artificial. An example of an ISDT is presented in the context of Executive Information Systems (EIS). Despite the increasing awareness of the potential of EIS for enhancing executive strategic decision-making effectiveness, there exists little theoretical work which directly guides EIS design. We contend that the underlying theoretical basis of EIS can be addressed through a design theory of vigilant information systems. Vigilance denotes the ability of an information system to help an executive remain alertly watchful for weak signals and discontinuities in the organizational environment relevant to emerging strategic threats and opportunities. Research on managerial information scanning and emerging issue tracking as well as theories of open loop control are synthesized to generate vigilant information system design theory propositions. Transformation of the propositions into testable empirical hypotheses is discussed.
In verschiedenen Forschungsprojekten haben wir mit dem Verfahren des offenen, leitfadenorientierten Expertlnneninterview gearbeitet und dabei die Erfahrung gemacht, daß wir methodisch auf einem wenig beackerten Terrain operieren mußten. Das gilt nahezu vollständig für Auswertungsprobleme. In der — spärlich vorhandenen — Literatur zu Expertlnneninterviews werden vorwiegend Fragen des Feldzugangs und der Gesprächsführung behandelt. Die Frage, wie “methodisch kontrolliertes Fremdverstehen” (vgl. Schütze u. a. 1973) im Rahmen von Expertlnneninterviews zu bewerkstelligen ist, bleibt völlig offen. Ziel dieses Artikels ist es, einige Fragen hinsichtlich der Methodik des Expertlnneninterviews zu behandeln. Das empirische Material, auf das wir uns beziehen, stammt aus Forschungsprojekten, die wir durchgeführt haben bzw. gegenwärtig bearbeitenl. Das Auswertungsverfahren, das wir vorstellen werden (s. Kap. 4), haben wir aus unserer eigenen Forschungspraxis entwickelt, die ihrerseits im Rekurs auf die Literatur zur qualitativen bzw. interpretativen Sozialforschung zustandegekommen ist.
This book is about options for inquiry: options among the paradigms—basic belief systems—that have emerged as successors to conventional positivism. Three options are explored in this book: postpositivism, on the shoulders of whose proponents the mantles of succession and of hegemony appear to have fallen, and two brash and sometimes contentious contendors, critical theory and constructivism. Although all three alternatives reject positivism, they make very different diagnoses of its problems and, therefore, offer very different remedies.
The authors critically review systems development in information systems (IS) research. Several classification schemes of research are described and systems development is identified as a developmental, engineering, and formulative type of research. A framework of research is proposed to explain the dual nature of systems development as a research methodology and a research domain in IS research. Progress in several disciplinary areas is reviewed to provide a basis to argue that systems development is a valid research methodology. A systems development research process is presented from a methodological perspective. Software engineering, the basic method is applying the systems development research methodology, is then discussed. A framework to classify IS research domain and various research methodologies in studying systems development is presented. It is suggested that systems development and empirical research methodologies are complementary to each other. It is further proposed that an integrated multidimensional and multimethodological approach will generate fruitful research results in IS research.
Disentangling Hype from Practicality: On Realistically Achieving Quantum Advantage
- Hacker News
- Download PDF
- Join the Discussion
- View in the ACM Digital Library
- Introduction
Key Insights
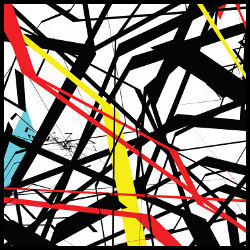
Operating on fundamentally different principles than conventional computers, quantum computers promise to solve a variety of important problems that seemed forever intractable on classical computers. Leveraging the quantum foundations of nature, the time to solve certain problems on quantum computers grows more slowly with the size of the problem than on classical computers—this is called quantum speedup. Going beyond quantum supremacy, 2 which was the demonstration of a quantum computer outperforming a classical one for an artificial problem, an important question is finding meaningful applications (of academic or commercial interest) that can realistically be solved faster on a quantum computer than on a classical one. We call this a practical quantum advantage, or quantum practicality for short.
Back to Top
- Most of today’s quantum algorithms may not achieve practical speedups. Material science and chemistry have a huge potential and we hope more practical algorithms will be invented based on our guidelines.
- Due to limitations of input and output bandwidth, quantum computers will be practical for “big compute” problems on small data, not big data problems.
- Quadratic speedups delivered by algorithms such as Grover’s search are insufficient for practical quantum advantage without significant improvements across the entire software/hardware stack.
There is a maze of hard problems that have been suggested to profit from quantum acceleration: from cryptanalysis, chemistry and materials science, to optimization, big data, machine learning, database search, drug design and protein folding, fluid dynamics and weather prediction. But which of these applications realistically offer a potential quantum advantage in practice? For this, we cannot only rely on asymptotic speedups but must consider the constants involved. Being optimistic in our outlook for quantum computers, we identify clear guidelines for quantum practicality and use them to classify which of the many proposed applications for quantum computing show promise and which ones would require significant algorithmic improvements to become practical and relevant.
To establish reliable guidelines, or lower bounds for the required speedup of a quantum computer, we err on the side of being optimistic for quantum and overly pessimistic for classical computing. Despite our overly optimistic assumptions, our analysis shows a wide range of often-cited applications is unlikely to result in a practical quantum advantage without significant algorithmic improvements. We compare the performance of only a single classical chip fabricated like the one used in the NVIDIA A100 GPU that fits around 54 billion transistors 15 with an optimistic assumption for a hypothetical quantum computer that may be available in the next decades with 10,000 error-corrected logical qubits, 10μs gate time for logical operations, the ability to simultaneously perform gate operations on all qubits and all-to-all connectivity for fault tolerant two-qubit gates. a
I/O bandwidth. We first consider the fundamental I/O bottleneck that limits quantum computers in their interaction with the classical world, which determines bounds for data input and output bandwidths. Scalable implementations of quantum random access memory (QRAM 8 , 9 ) demand a fault-tolerant error corrected implementation and the bandwidth is then fundamentally limited by the number of quantum gate operations or measurements that can be performed per unit time. We assume only a single gate operation per input bit. For our optimistic future quantum computer, the resulting rate is 10,000-times smaller than for an existing classical chip (see Table 1 ). We immediately see that any problem limited by accessing classical data, such as search problems in databases, will be solved faster by classical computers. Similarly, a potentially exponential quantum speedup in linear algebra problems 12 vanishes when the matrix must be loaded from classical data, or when the full solution vector should be read out. Generally, quantum computers will be practical for “big compute” problems on small data , not big data problems.

Crossover scale. With quantum speedup, asymptotically fewer operations will be needed on a quantum computer than on a classical computer. Due to the high operational complexity and slower gate operations, however, each operation on a quantum computer will be slower than a corresponding classical one. As sketched in the accompanying figure , classical computers will always be faster for small problems and quantum advantage is realized beyond a problem-dependent crossover scale where the gain due to quantum speedup overcomes the constant slowdown of the quantum computer. To have real practical impact, the crossover time must be short, not more than weeks. Constants matter in determining the utility for applications, as with any runtime estimate in computing.

Compute performance. To model performance, we employ the well-known work-depth model from classical parallel computing to determine upper bounds of classical silicon-based computations and an extension for quantum computations. In this model, the work is the total number of operations and applies to both classical and quantum executions. In Table 1 , we provide concrete examples using three types of operations: logical operations, 16-bit floating point, and 32-bit integer or fixed-point arithmetic operations for numerical modeling. For the quantum costs, we consider only the most expensive parts in our estimates, again benefiting quantum computers; for arithmetic, we count just the dominant cost of multiplications, assuming additions are free. Furthermore, for floating point multiplication, we consider only the cost of the multiplication of the mantissa (10 bits in fp16). We ignore all further overheads incurred by the quantum algorithm due to reversible computations, as well as the significant cost of mapping to a specific hardware architecture with limited qubit connectivity.
Crossover times for classical and quantum computation. To estimate lower bounds for the crossover times, we consider that while both classical and quantum computers must evaluate the same functions (usually called oracles) that describe a problem, quantum computers require fewer evaluations thereof due to quantum speedup. At the root of many quantum acceleration proposals lies a quadratic quantum speedup, including the well-known Grover algorithm. 10 , 11 For such an algorithm, a problem that needs X function calls on a quantum computer requires quadratically more, namely on the order of X 2 calls on a classical computer. To overcome the large constant performance difference between a quantum computer and a classical computer, which Table 1 shows to be more than a factor of 10 10 , many function calls X >> 10 10 is needed for the quantum speedup to deliver a practical advantage. In Table 2 , we estimate upper bounds for the complexity of the function that will lead to a cross-over time of 10 6 seconds, or approximately two weeks.

We see that with quadratic speedup even a single floating point or integer operation leads to crossover times of several months. Furthermore, at most 68 binary logical operations can be afforded to stay within our desired crossover time of two weeks, which is too low for any non-trivial application. Keeping in mind that these estimates are pessimistic for classical computation (a single of today’s classical chips) and overly optimistic for quantum computing (only considering the multiplication of the mantissa and assuming all-to-all qubit connectivity), we come to the clear conclusion that quadratic speedups are insufficient for practical quantum advantage. The numbers look better for cubic or quartic speedups where thousands or millions of operations may be feasible, and we conclude, similarly to Babbush et al., 3 that at least cubic or quartic speedups are required for a practical quantum advantage.
As a result of our overly optimistic assumptions in favor of quantum computing, these conclusions will remain valid even with significant advances in quantum technology of multiple orders of magnitude.
Practical and impractical applications. We can now use these considerations to discuss several classes of applications where our fundamental bounds draw a line for quantum practicality. The most likely problems to allow for a practical quantum advantage are those with exponential quantum speedup. This includes the simulation of quantum systems for problems in chemistry, materials science, and quantum physics, as well as cryptanalysis using Shor’s algorithm. 16 The solution of linear systems of equations for highly structured problems 12 also has an exponential speedup, but the I/O limitations discussed above will limit the practicality and undo this advantage if the matrix has to be loaded from memory instead of being computed based on limited data or knowledge of the full solution is required (as opposed to just some limited information obtained by sampling the solution).
Equally important, we identify likely dead ends in the maze of applications. A large range of problem areas with quadratic quantum speedups, such as many current machine learning training approaches, accelerating drug design and protein folding with Grover’s algorithm, speeding up Monte Carlo simulations through quantum walks, as well as more traditional scientific computing simulations including the solution of many non-linear systems of equations, such as fluid dynamics in the turbulent regime, weather, and climate simulations will not achieve quantum advantage with current quantum algorithms in the foreseeable future. We also conclude that the identified I/O limits constrain the performance of quantum computing for big data problems, unstructured linear systems, and database search based on Grover’s algorithm such that a speedup is unlikely in those cases. Furthermore, Aaronson et al. 1 show the achievable quantum speedup of unstructured black-box algorithms is limited to O ( N 4 ). This implies that any algorithm achieving higher speedup must exploit structure in the problem it solves.
These considerations help with separating hype from practicality in the search for quantum applications and can guide algorithmic developments. Specifically, our analysis shows it is necessary for the community to focus on super-quadratic speedups, ideally exponential speedups, and one needs to carefully consider I/O bottlenecks when deriving algorithms to exploit quantum computation best. Therefore, the most promising candidates for quantum practicality are small-data problems with exponential speedup. Specific examples where this is the case are quantum problems in chemistry and materials science, 5 which we identify as the most promising application. We recommend using precise requirements models 4 to get more reliable and realistic (less optimistic) estimates in cases where our rough guidelines indicate a potential practical quantum advantage.
Here, we provide more details for how we obtained the numbers mentioned earlier. We compare our quantum computer with a single microprocessor chip like the one used in the NVIDIA A100 GPU. 15 The A100 chip is around 850 mm 2 in size and manufactured in TSMC’s 7nm N7 silicon process. A100 shows that such a chip fits around 54.2 billion transistors and can operator at a cycle time of around 0.7ns.
Determining peak operation throughputs. In Table 1 , we provide concrete examples using three types of operations: logical operations, 16-bit floating point, and 32-bit integer arithmetic operations for numerical modeling. Other datatypes could be modeled using our methodology as well.
Classical NVIDIA A100. According to its datasheet, NVIDIA’s A100 GPU, a SIMT-style von Neumann load store architecture, delivers 312 tera-operations per second (Top/s) with half precision floating point (fp16) through tensor cores and 78Top/s through the normal processing pipeline. NVIDIA assumes a 50/50 mix of addition and multiplication operations and thus, we divide the number by two, yielding 195Top/s fp16 performance. The datasheet states 19.5Top/s for 32-bit integer operations, again assuming a 50/50 mix of addition and multiplication, leading to an effective 9.75Top/s. The binary tensor core performance is listed as 4,992Top/s with a limited set of instructions.
Classical special-purpose ASIC. Our main analysis assumes that we build a special-purpose ASIC using a similar technology. If we were to fill the equivalent chip-space of an A100 with a specialized circuit, we would use existing execution units, for which the size is typically measured in gate equivalents (GE). A 16-bit floating point unit (FPU) with addition and multiplication functions requires approximately 7kGE, a 32-bit integer unit requires 18kGE, 14 and we assume 50GE for a simple binary operation. All units include operand buffer registers and support a set of programmable instructions. We note that simple addition or multiplication circuits would be significantly cheaper. If we assume a transistor-to-gate ratio of 10 13 and that 50% of the total chip area is used for control logic of a dataflow ASIC with the required buffering, we can fit 54.2 B /(7 k • 10 • 2) = 387 k fp16 units. Similarly, we can fit 54.2 B (18 k • 10 • 2) = 151 k int32, or 54.2 B /(50 • 10 • 2) = 54.2M bin2 units on our hypothetical chip. Assuming a cycle time of 0.7ns, this leads to a total operation rate of 0.55 fp16, 0.22 int32, and 77.4 bin Pop/s for an application-specific ASIC with the A100’s technology and budget. The ASIC thus leads to a raw speedup between approximately 2x and 15x over a programmable circuit. Thus, on classical silicon, the performance ranges approximately between 10 13 and 10 16 op/s for binary, int32, and fp16 types.
Our analysis shows a wide range of often-cited applications is unlikely to result in a practical quantum advantage without significant algorithmic improvements.
Hypothetical future quantum computer. To determine the costs of N -bit multiplication on a quantum computer, we choose the controlled adder from Gidney 6 and implement the multiplication using N single-bit controlled adders, each requiring 2 N CCZ magic states. These states are produced in so called “magic state factories” that are implemented on the physical chip. While the resulting multiplier is entirely sequential, we found that this construction allows for more units to be placed on one chip than for a low-depth adder and/or for a tree-like reduction of partial products since the number of CCZ states is lower (and thus fewer magic state factories are required), and the number of work-qubits is lower. The resulting multiplier has a CCZ-depth and count of 2 N 2 using 5 N – 1 qubits (2 N input, 2 N – 1 output, N ancilla for the addition).
To compute the space overhead due to CCZ factories, we first use the analysis of Gidney and Fowler 7 to compute the number of physical qubits per factory when aiming for circuits (programs) using ≈ 10 8 CCZ magic states with physical gate errors of 10 -3 . We approximate the overhead in terms of logical qubits by dividing the physical space overhead by 2 d 2 , where we choose the error-correcting code distance d = 2 • 31 2 to be the same as the distance used for the second level of distillation. 7 Thus we divide Gidney and Fowler’s 147,904 physical qubits per factory (for details consult the anciliary spreadsheet (field B40) of Gidney and Fowler) by 2 d 2 = 2 • 31 2 and get an equivalent space of 77 logical qubits per factory.
For the multiplier of the 10-bit mantissa of an fp16 floating point number, we need 2 • 10 2 = 200 CCZ states and 5 • 10 = 50 qubits. Since each factory takes 5.5 cycles 7 and we can pipeline the production of CCZ states, we assume 5.5 factories per multiplication unit such that multipliers do not wait for magic state production on average. Thus, each multiplier requires 200 cycles and 5 N + 5.5 • 77 = 50 + 5.5 • 77 = 473.5 qubits. With a total of 10,000 logical qubits, we can implement 21 10-bit multipliers on our hypothetical quantum chip. With 10μs cycle time, the 200-cycle latency, we get the final rate of less than 10 5 cycle/ s / (200 cycle/op ) • 21= 10.5 kop/s. For int32 ( N =32), the calculation is equivalent. For binary, we assume two input and one output qubit for the (binary) adder (Toffoli gate) which does not need ancillas. The results are summarized in Table 1 .
A note on parallelism. We assumed massively parallel execution of the oracle on both the classical and quantum computer (that is, oracles with a depth of one). If the oracle does not admit such parallelization, for example, if depth = work in the worst-case scenario, then the comparison becomes more favorable towards the quantum computer. One could model this scenario by allowing the classical computer to only perform one operation per cycle. With a 2GHz clock frequency, this would mean a slowdown of about 100,000 times for fp16 on the GPU. In this extremely unrealistic algorithmic worst case, the oracle would still have to consist of only several thousands of fp16 operations with a quadratic speedup. However, we note that in practice, most oracles have low depth and parallelization across a single chip is achievable, which is what we assumed.
Determining maximum operation counts per oracle call. In Table 2 , we list the maximum number of operations of a certain type that can be run to achieve a quantum speedup within a runtime of 10 6 seconds (a little more than two weeks). The maximum number of classical operations that can be performed with a single classical chip in 10 6 seconds would be: 0.55 fp16, 0.22 int32, and 77.4 bin Zop. Similarly, assuming the rates from Table 1 , for a quantum chip: 7, 4, 2, and 350 Gop, respectively.
We now assume that all calculations are used in oracle calls on the quantum computer and we ignore all further costs on the quantum machine. We start by modeling algorithms that provide polynomial X k speedup, for small constants k. For example, for Grover’s algorithms, 11 k + 2. It is clear quantum computers are asymptotically faster (in the number of oracle queries) for any k >1. However, we are interested to find the oracle complexity (that is, the number of operations required to evaluate it) for which a quantum computer is faster than a classical computer within the time-window of 10 6 seconds.
Let the number of operations required to evaluate a single oracle call be M and let the number of required invocations be N. It takes a classical computer time T c = N k • M • t c , whereas a quantum computer solves the same problem in time T q = N k • M • t q where t c and t q denote the time to evaluate an operation on a classical and on a quantum computer, respectively. By demanding that the quantum computer should solve the problem faster than the classical computer and within 10 6 seconds, we find
which allows us to compute the maximal number of basic operations per oracle evaluation such that the quantum computer still achieves a practical speedup:
Acknowledgments. We thank L. Benini for helpful discussions about ASIC and processor design and related overheads and W. van Dam and anonymous reviewers for comments that improved an earlier draft.

Submit an Article to CACM
CACM welcomes unsolicited submissions on topics of relevance and value to the computing community.
You Just Read
Copyright held by authors. Publication rights licensed to ACM. Request permission to publish from [email protected]
The Digital Library is published by the Association for Computing Machinery. Copyright © 2023 ACM, Inc.
May 2023 Issue
Published: May 1, 2023
Vol. 66 No. 5
Pages: 82-87
Advertisement
Join the Discussion (0)
Become a member or sign in to post a comment, the latest from cacm.
Building Computing Systems for Embodied Artificial Intelligence
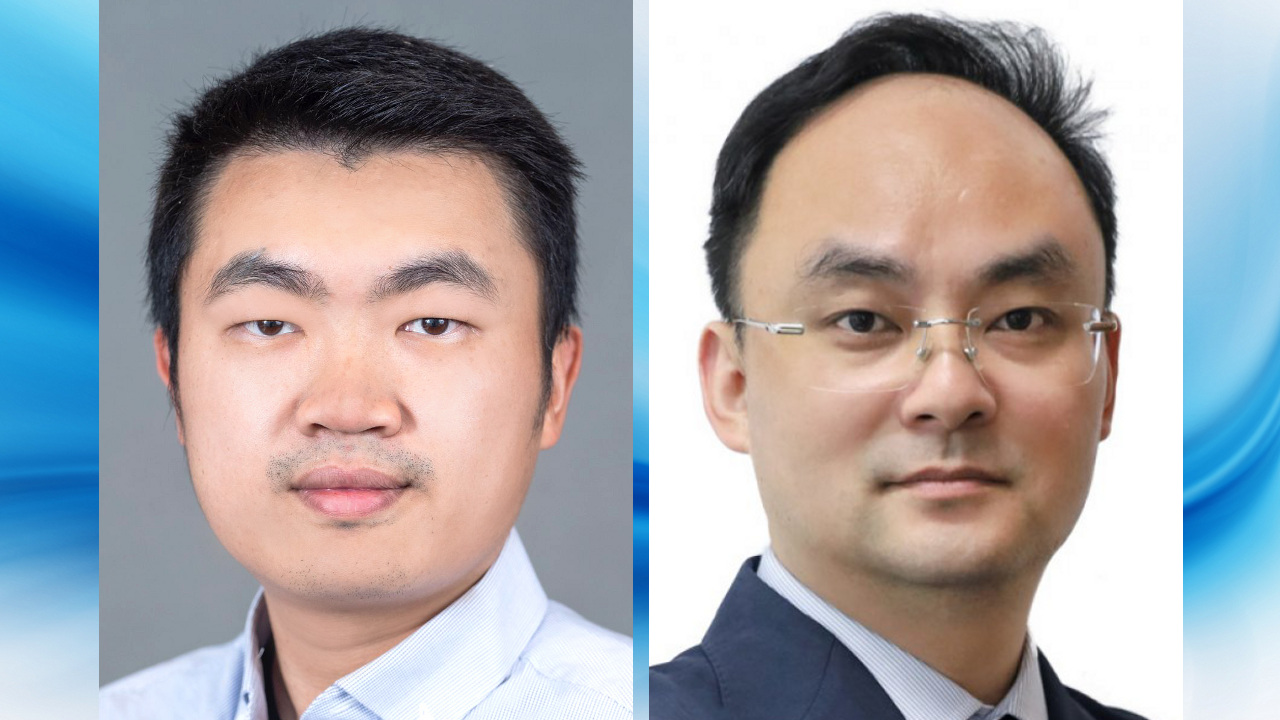
First Impressions: Designing Social Actions for Positive Social Change with Bard
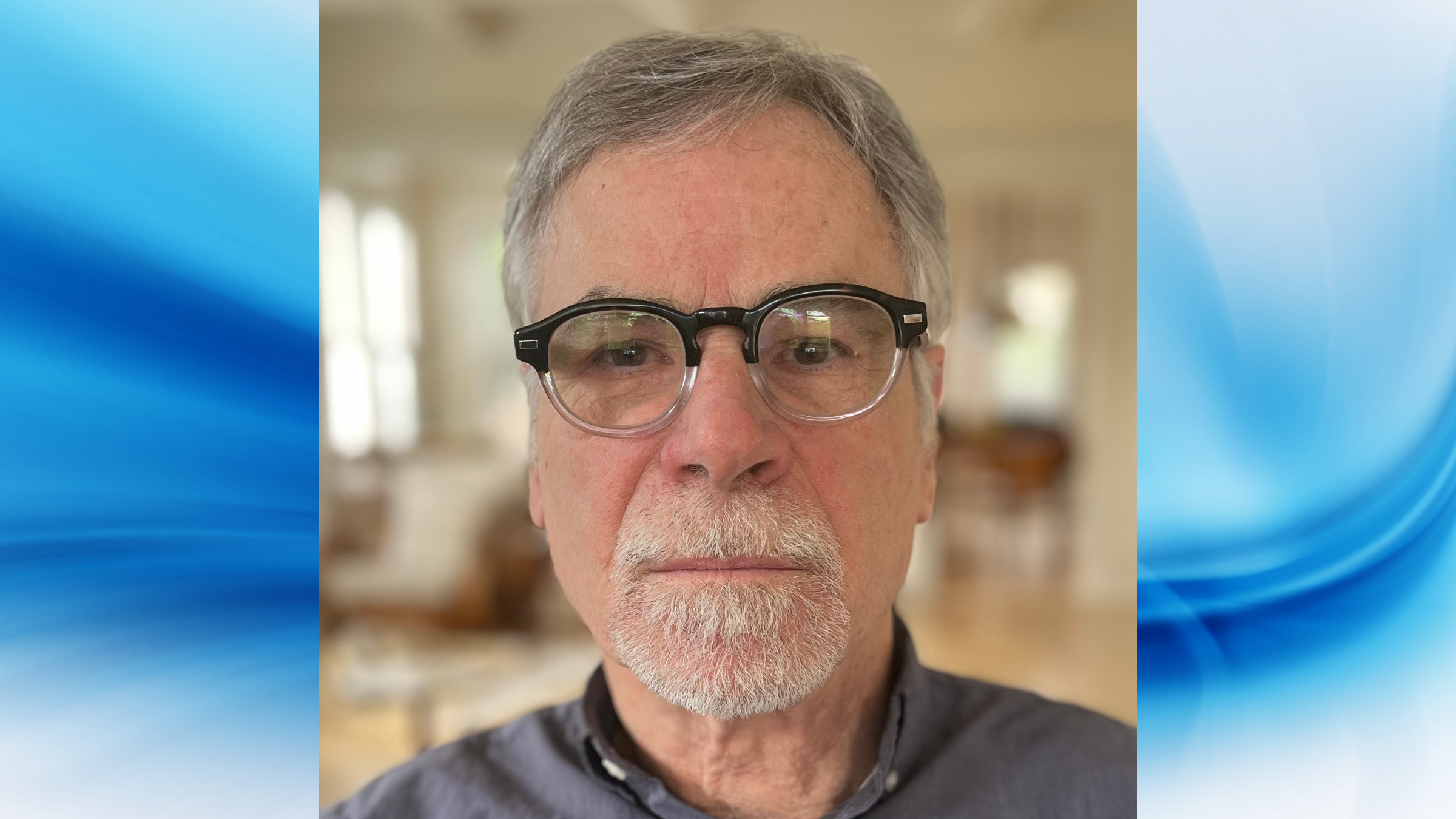
The Artist’s Role on a Science and Engineering Campus
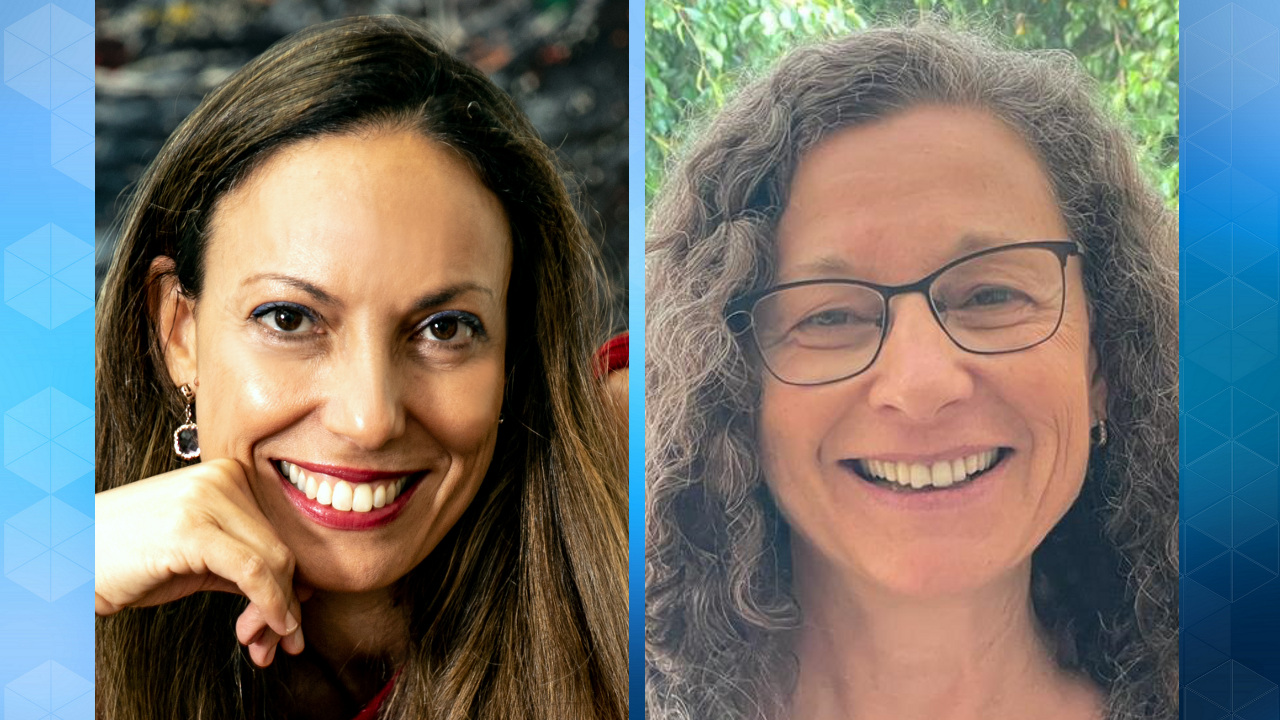
Shape the Future of Computing
ACM encourages its members to take a direct hand in shaping the future of the association. There are more ways than ever to get involved.
Communications of the ACM (CACM) is now a fully Open Access publication.
By opening CACM to the world, we hope to increase engagement among the broader computer science community and encourage non-members to discover the rich resources ACM has to offer.
Suggestions or feedback?
MIT News | Massachusetts Institute of Technology
- Machine learning
- Social justice
- Black holes
- Classes and programs
Departments
- Aeronautics and Astronautics
- Brain and Cognitive Sciences
- Architecture
- Political Science
- Mechanical Engineering
Centers, Labs, & Programs
- Abdul Latif Jameel Poverty Action Lab (J-PAL)
- Picower Institute for Learning and Memory
- Lincoln Laboratory
- School of Architecture + Planning
- School of Engineering
- School of Humanities, Arts, and Social Sciences
- Sloan School of Management
- School of Science
- MIT Schwarzman College of Computing
Tests show high-temperature superconducting magnets are ready for fusion
Press contact :, media download.
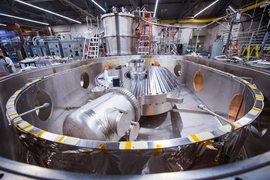
*Terms of Use:
Images for download on the MIT News office website are made available to non-commercial entities, press and the general public under a Creative Commons Attribution Non-Commercial No Derivatives license . You may not alter the images provided, other than to crop them to size. A credit line must be used when reproducing images; if one is not provided below, credit the images to "MIT."
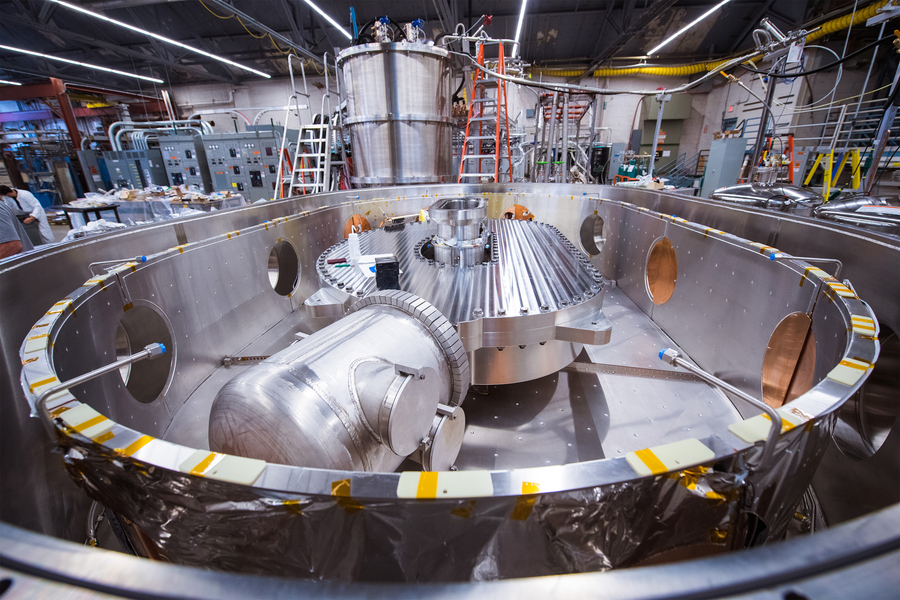
Previous image Next image
In the predawn hours of Sept. 5, 2021, engineers achieved a major milestone in the labs of MIT’s Plasma Science and Fusion Center (PSFC), when a new type of magnet, made from high-temperature superconducting material, achieved a world-record magnetic field strength of 20 tesla for a large-scale magnet. That’s the intensity needed to build a fusion power plant that is expected to produce a net output of power and potentially usher in an era of virtually limitless power production.
The test was immediately declared a success, having met all the criteria established for the design of the new fusion device, dubbed SPARC, for which the magnets are the key enabling technology. Champagne corks popped as the weary team of experimenters, who had labored long and hard to make the achievement possible, celebrated their accomplishment.
But that was far from the end of the process. Over the ensuing months, the team tore apart and inspected the components of the magnet, pored over and analyzed the data from hundreds of instruments that recorded details of the tests, and performed two additional test runs on the same magnet, ultimately pushing it to its breaking point in order to learn the details of any possible failure modes.
All of this work has now culminated in a detailed report by researchers at PSFC and MIT spinout company Commonwealth Fusion Systems (CFS), published in a collection of six peer-reviewed papers in a special edition of the March issue of IEEE Transactions on Applied Superconductivity . Together, the papers describe the design and fabrication of the magnet and the diagnostic equipment needed to evaluate its performance, as well as the lessons learned from the process. Overall, the team found, the predictions and computer modeling were spot-on, verifying that the magnet’s unique design elements could serve as the foundation for a fusion power plant.
Enabling practical fusion power
The successful test of the magnet, says Hitachi America Professor of Engineering Dennis Whyte, who recently stepped down as director of the PSFC, was “the most important thing, in my opinion, in the last 30 years of fusion research.”
Before the Sept. 5 demonstration, the best-available superconducting magnets were powerful enough to potentially achieve fusion energy — but only at sizes and costs that could never be practical or economically viable. Then, when the tests showed the practicality of such a strong magnet at a greatly reduced size, “overnight, it basically changed the cost per watt of a fusion reactor by a factor of almost 40 in one day,” Whyte says.
“Now fusion has a chance,” Whyte adds. Tokamaks, the most widely used design for experimental fusion devices, “have a chance, in my opinion, of being economical because you’ve got a quantum change in your ability, with the known confinement physics rules, about being able to greatly reduce the size and the cost of objects that would make fusion possible.”
The comprehensive data and analysis from the PSFC’s magnet test, as detailed in the six new papers, has demonstrated that plans for a new generation of fusion devices — the one designed by MIT and CFS, as well as similar designs by other commercial fusion companies — are built on a solid foundation in science.
The superconducting breakthrough
Fusion, the process of combining light atoms to form heavier ones, powers the sun and stars, but harnessing that process on Earth has proved to be a daunting challenge, with decades of hard work and many billions of dollars spent on experimental devices. The long-sought, but never yet achieved, goal is to build a fusion power plant that produces more energy than it consumes. Such a power plant could produce electricity without emitting greenhouse gases during operation, and generating very little radioactive waste. Fusion’s fuel, a form of hydrogen that can be derived from seawater, is virtually limitless.
But to make it work requires compressing the fuel at extraordinarily high temperatures and pressures, and since no known material could withstand such temperatures, the fuel must be held in place by extremely powerful magnetic fields. Producing such strong fields requires superconducting magnets, but all previous fusion magnets have been made with a superconducting material that requires frigid temperatures of about 4 degrees above absolute zero (4 kelvins, or -270 degrees Celsius). In the last few years, a newer material nicknamed REBCO, for rare-earth barium copper oxide, was added to fusion magnets, and allows them to operate at 20 kelvins, a temperature that despite being only 16 kelvins warmer, brings significant advantages in terms of material properties and practical engineering.
Taking advantage of this new higher-temperature superconducting material was not just a matter of substituting it in existing magnet designs. Instead, “it was a rework from the ground up of almost all the principles that you use to build superconducting magnets,” Whyte says. The new REBCO material is “extraordinarily different than the previous generation of superconductors. You’re not just going to adapt and replace, you’re actually going to innovate from the ground up.” The new papers in Transactions on Applied Superconductivity describe the details of that redesign process, now that patent protection is in place.
A key innovation: no insulation
One of the dramatic innovations, which had many others in the field skeptical of its chances of success, was the elimination of insulation around the thin, flat ribbons of superconducting tape that formed the magnet. Like virtually all electrical wires, conventional superconducting magnets are fully protected by insulating material to prevent short-circuits between the wires. But in the new magnet, the tape was left completely bare; the engineers relied on REBCO’s much greater conductivity to keep the current flowing through the material.
“When we started this project, in let’s say 2018, the technology of using high-temperature superconductors to build large-scale high-field magnets was in its infancy,” says Zach Hartwig, the Robert N. Noyce Career Development Professor in the Department of Nuclear Science and Engineering. Hartwig has a co-appointment at the PSFC and is the head of its engineering group, which led the magnet development project. “The state of the art was small benchtop experiments, not really representative of what it takes to build a full-size thing. Our magnet development project started at benchtop scale and ended up at full scale in a short amount of time,” he adds, noting that the team built a 20,000-pound magnet that produced a steady, even magnetic field of just over 20 tesla — far beyond any such field ever produced at large scale.
“The standard way to build these magnets is you would wind the conductor and you have insulation between the windings, and you need insulation to deal with the high voltages that are generated during off-normal events such as a shutdown.” Eliminating the layers of insulation, he says, “has the advantage of being a low-voltage system. It greatly simplifies the fabrication processes and schedule.” It also leaves more room for other elements, such as more cooling or more structure for strength.
The magnet assembly is a slightly smaller-scale version of the ones that will form the donut-shaped chamber of the SPARC fusion device now being built by CFS in Devens, Massachusetts. It consists of 16 plates, called pancakes, each bearing a spiral winding of the superconducting tape on one side and cooling channels for helium gas on the other.
But the no-insulation design was considered risky, and a lot was riding on the test program. “This was the first magnet at any sufficient scale that really probed what is involved in designing and building and testing a magnet with this so-called no-insulation no-twist technology,” Hartwig says. “It was very much a surprise to the community when we announced that it was a no-insulation coil.”
Pushing to the limit … and beyond
The initial test, described in previous papers, proved that the design and manufacturing process not only worked but was highly stable — something that some researchers had doubted. The next two test runs, also performed in late 2021, then pushed the device to the limit by deliberately creating unstable conditions, including a complete shutoff of incoming power that can lead to a catastrophic overheating. Known as quenching, this is considered a worst-case scenario for the operation of such magnets, with the potential to destroy the equipment.
Part of the mission of the test program, Hartwig says, was “to actually go off and intentionally quench a full-scale magnet, so that we can get the critical data at the right scale and the right conditions to advance the science, to validate the design codes, and then to take the magnet apart and see what went wrong, why did it go wrong, and how do we take the next iteration toward fixing that. … It was a very successful test.”
That final test, which ended with the melting of one corner of one of the 16 pancakes, produced a wealth of new information, Hartwig says. For one thing, they had been using several different computational models to design and predict the performance of various aspects of the magnet’s performance, and for the most part, the models agreed in their overall predictions and were well-validated by the series of tests and real-world measurements. But in predicting the effect of the quench, the model predictions diverged, so it was necessary to get the experimental data to evaluate the models’ validity.
“The highest-fidelity models that we had predicted almost exactly how the magnet would warm up, to what degree it would warm up as it started to quench, and where would the resulting damage to the magnet would be,” he says. As described in detail in one of the new reports, “That test actually told us exactly the physics that was going on, and it told us which models were useful going forward and which to leave by the wayside because they’re not right.”
Whyte says, “Basically we did the worst thing possible to a coil, on purpose, after we had tested all other aspects of the coil performance. And we found that most of the coil survived with no damage,” while one isolated area sustained some melting. “It’s like a few percent of the volume of the coil that got damaged.” And that led to revisions in the design that are expected to prevent such damage in the actual fusion device magnets, even under the most extreme conditions.
Hartwig emphasizes that a major reason the team was able to accomplish such a radical new record-setting magnet design, and get it right the very first time and on a breakneck schedule, was thanks to the deep level of knowledge, expertise, and equipment accumulated over decades of operation of the Alcator C-Mod tokamak, the Francis Bitter Magnet Laboratory, and other work carried out at PSFC. “This goes to the heart of the institutional capabilities of a place like this,” he says. “We had the capability, the infrastructure, and the space and the people to do these things under one roof.”
The collaboration with CFS was also key, he says, with MIT and CFS combining the most powerful aspects of an academic institution and private company to do things together that neither could have done on their own. “For example, one of the major contributions from CFS was leveraging the power of a private company to establish and scale up a supply chain at an unprecedented level and timeline for the most critical material in the project: 300 kilometers (186 miles) of high-temperature superconductor, which was procured with rigorous quality control in under a year, and integrated on schedule into the magnet.”
The integration of the two teams, those from MIT and those from CFS, also was crucial to the success, he says. “We thought of ourselves as one team, and that made it possible to do what we did.”
Share this news article on:
Related links.
- Dennis Whyte
- Zachary Hartwig
- Plasma Science and Fusion Center
- Department of Nuclear Science and Engineering
Related Topics
- Nuclear science and engineering
- Collaboration
- Nuclear power and reactors
- Renewable energy
- Sustainability
Related Articles
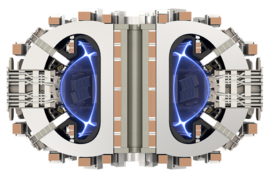
New study shows how universities are critical to emerging fusion industry
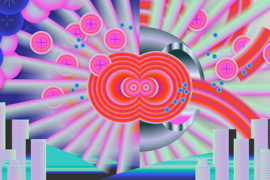
Fast-tracking fusion energy’s arrival with AI and accessibility
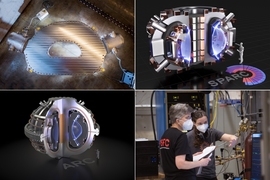
MIT expands research collaboration with Commonwealth Fusion Systems to build net energy fusion machine, SPARC
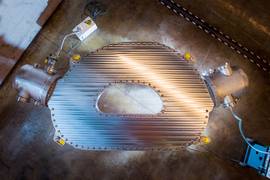
MIT-designed project achieves major advance toward fusion energy
Previous item Next item
More MIT News
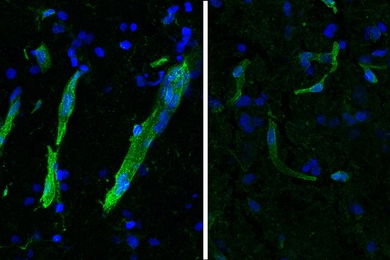
Study: Movement disorder ALS and cognitive disorder FTLD show strong molecular overlaps
Read full story →
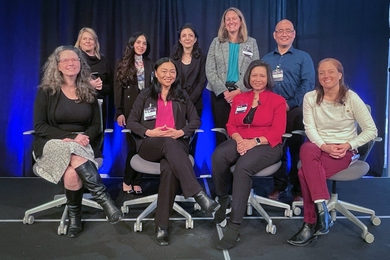
Students explore career opportunities in semiconductors
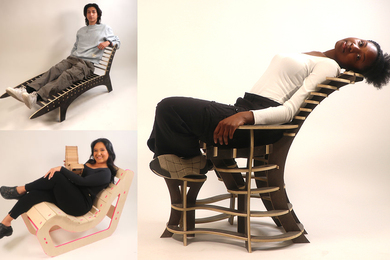
For MIT students, there is much to learn from crafting a chair
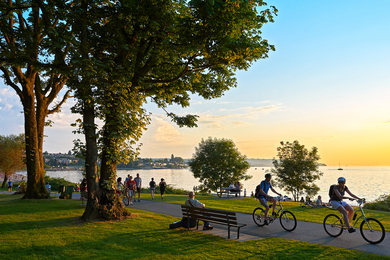
A new way to quantify climate change impacts: “Outdoor days”
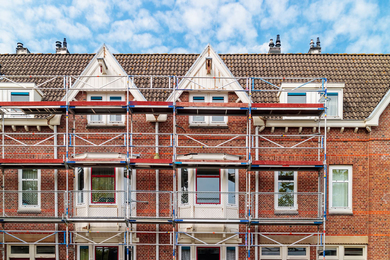
Think globally, rebuild locally
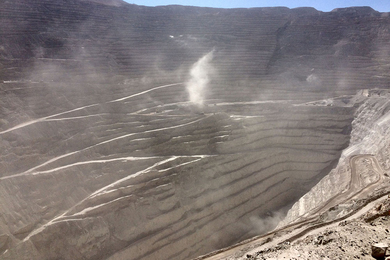
Understanding the impacts of mining on local environments and communities
- More news on MIT News homepage →
Massachusetts Institute of Technology 77 Massachusetts Avenue, Cambridge, MA, USA
- Map (opens in new window)
- Events (opens in new window)
- People (opens in new window)
- Careers (opens in new window)
- Accessibility
- Social Media Hub
- MIT on Facebook
- MIT on YouTube
- MIT on Instagram
How software engineering research aligns with design science: a review
- Open access
- Published: 18 April 2020
- Volume 25 , pages 2630–2660, ( 2020 )
Cite this article
You have full access to this open access article
- Emelie Engström 1 ,
- Margaret-Anne Storey 2 ,
- Per Runeson 1 ,
- Martin Höst 1 &
- Maria Teresa Baldassarre 3
9838 Accesses
24 Citations
13 Altmetric
Explore all metrics
Assessing and communicating software engineering research can be challenging. Design science is recognized as an appropriate research paradigm for applied research, but is rarely explicitly used as a way to present planned or achieved research contributions in software engineering. Applying the design science lens to software engineering research may improve the assessment and communication of research contributions.
The aim of this study is 1) to understand whether the design science lens helps summarize and assess software engineering research contributions, and 2) to characterize different types of design science contributions in the software engineering literature.
In previous research, we developed a visual abstract template, summarizing the core constructs of the design science paradigm. In this study, we use this template in a review of a set of 38 award winning software engineering publications to extract, analyze and characterize their design science contributions.
We identified five clusters of papers, classifying them according to their different types of design science contributions.
Conclusions
The design science lens helps emphasize the theoretical contribution of research output—in terms of technological rules—and reflect on the practical relevance, novelty and rigor of the rules proposed by the research.
Similar content being viewed by others
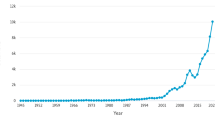
Literature reviews as independent studies: guidelines for academic practice
Sascha Kraus, Matthias Breier, … João J. Ferreira
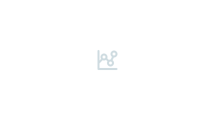
What is Qualitative in Research
Patrik Aspers & Ugo Corte
Design and Development Research
Avoid common mistakes on your manuscript.
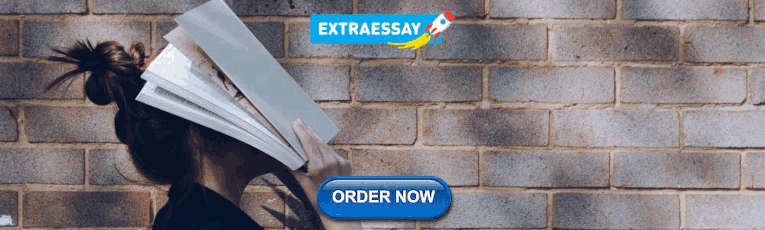
1 Introduction
Design science is a paradigm for conducting and communicating applied research such as software engineering. The goal of design science research is to produce prescriptive knowledge for professionals in a discipline and to share empirical insights gained from investigations of the prescriptions applied in context (van Aken 2004 ). Such knowledge is referred to as “design knowledge” as it helps practitioners design solutions to their problems. Similar to other design sciences, much software engineering research aims to design solutions to practical problems in a real-world context.
Design science is an established research paradigm in the fields of information systems (Hevner et al. 2004 ) and other engineering disciplines, such as mechanical, civil, architectural, and manufacturing engineering. Footnote 1 It is also increasingly used in computer science; for example, it is now accepted as the de facto paradigm for presenting design contributions from information visualization research (Sedlmair et al. 2012 ). Although Wierenga has promoted design science for capturing design knowledge in software engineering (Wieringa 2014 ), we seldom see it being referred to in our field (although there are some exceptions (Wohlin and Aurum 2015 )). We are puzzled by its low adoption as the use of this lens could increase the clarity of research contributions for both practitioners and researchers, as it has been shown to do in other fields (Shneiderman 2016 ).
The goal of our research is to investigate if and how a design science paradigm may be a viable way to assess and communicate research contributions in existing software engineering literature. To this end, we consider a set of software engineering research papers and view these contributions through a design science lens by using and improving a visual abstract template we previously developed to showcase design knowledge (Storey et al. 2017 ).
We inspected 38 ACM distinguished papers published at the International Conference on Software Engineering (ICSE) over a five-year period—publications considered by many in the community as well-known exemplars of fine software engineering research, and papers that are expected to broadly represent the diverse topics addressed by our research community. Although these papers set a high bar for framing their research contributions, we found that the design science lens improved our understanding of their contributions. Also, most of the papers described research contributions that are congruent with a design science paradigm, even though none of them explicitly used the term. Applying this lens helped us elucidate certain aspects of the contributions (such as relevance, novelty and rigor), which in some cases were obscured by the original framing of the paper. However, not all the papers we considered produced design knowledge, thus some research publications do not benefit from using this lens.
Our analysis from this exercise led to five clusters of papers based on the type of design knowledge reported. We compare the papers within each cluster and reflect on how the design knowledge is typically achieved and reported in these clusters of papers.
In the remainder of this paper, we first present background on design science and our conceptualization of it by means of a visual abstract template (Section 2 ). We then describe our methodology for generating visual abstracts for the cohort of ACM distinguished papers we studied (Section 3 ), and use the information highlighted by the abstracts to extract the design knowledge in each paper. Finally, we cluster the papers by the type of design knowledge produced (Section 4 ). We interpret and discuss the implications of our findings (Sections 5 and 6 ), outline the limitations of our study (Section 7 ), and discuss related work (Section 8 ) before concluding the paper (Section 9 ).
2 Background
Our conceptualization of design science in software engineering, which our analysis is based on, was formed from a thorough review of the literature and a series of internal group workshops on the topic. This work helped us develop a visual abstract template to use as a lens for communicating and assessing research contributions (Storey et al. 2017 ). In this section, we give a brief introduction to design science and the visual abstract template. We use the term design knowledge to refer to the knowledge produced in design science research.
2.1 Design Science
Design science is a common research paradigm used in many fields of information systems and other engineering disciplines. By research paradigm, we refer to van Aken’s definition: “the combination of research questions asked, the research methodologies allowed to answer them and the nature of the pursued research products” (van Aken 2005 ). The mission of design science is to solve real-world problems. Hence, design science researchers aim to develop general design knowledge in a specific field to help practitioners create solutions to their problems. In Fig. 1 , we illustrate the relationship between the problem domain and solution domain , as well as between theory and practice . The arrows in the figure represent different types of contributions of design science research, i.e., problem conceptualization, solution design, instantiation, abstraction, and validation.
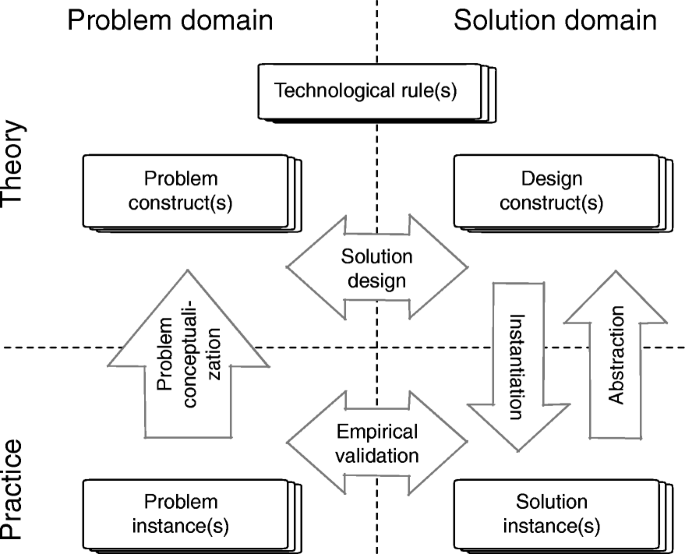
An illustration of the interplay between problem and solution as well as between theory and practice in design science research. The arrows illustrate the knowledge-creating activities, and the boxes represent the levels and types of knowledge that is created
Design knowledge is holistic and heuristic by its nature, and must be justified by in-context validations (Wieringa 2014 ; van Aken 2004 ). The term holistic is used by van Aken ( 2004 ) and refers to the “magic” aspect of design knowledge, implying that we never fully understand why a certain solution works in a specific context. There will always be hidden context factors that affect a problem-solution pair (Dybå et al. 2012 ). As a consequence, we can never prove the effect of a solution conclusively, and must rely on heuristic prescriptions. By evaluating multiple problem-solution pairs matching a given prescription, our understanding about that prescription increases. Design knowledge can be expressed in terms of technological rules (van Aken 2004 ), which are rules that capture general knowledge about the mappings between problems and proposed solutions .
Van Aken describes the typical design science research strategy to be the multiple case study (van Aken 2004 ), which can be compared with alpha and beta testing in clinical research, i.e., first case and succeeding cases. Rather than proving theory, design science research strives to refine theory, i.e., finding answers to questions about why, when, and where a solution may or may not work. Each new case adds insights that can refine the technological rule until saturation is achieved (van Aken 2004 ). Gregor and Hevner present a similar view of knowledge growth through multiple design cycles (Gregor and Hevner 2013 ). Wieringa and Moralı ( 2012 ) and Johannesson and Perjons ( 2014 ) discuss action research as one of several empirical methodologies that can be used to produce design knowledge. Sein et al. ( 2011 ) propose how design science can be adapted by action research to emphasise the construction of artifacts in design science. However, action research does not explicitly aim to develop knowledge that can be transferred to other contexts, but rather it tries to make a change in one specific local context.
2.2 A Design Science Visual Abstract Template
The visual abstract template we designed, shown in Fig. 2 , captures three main aspects of design science contributions: 1) the theory proposed or refined in terms of a technological rule; 2) the empirical contribution of the study in terms of one or more instances of a problem-solution pair and the corresponding design and validation cycles; and 3) support for the assessment of the value of the produced knowledge in terms of relevance, rigor, and novelty. While adhering to the design science paradigm puts the focus on how to produce and assess design knowledge (i.e., the technological rules), our visual abstract template is designed to help researchers effectively communicate as well as justify design knowledge. It also helps highlight which instantiations of the rule have been studied and how they were validated, how problem understanding was achieved, and what foundations for the proposed solution were considered. In the visual abstract template, the researcher is encouraged to reflect on how a study adds new knowledge to the general theory (i.e., the constructs of the technological rule) and to be aware of the relationship between the general rule and its instantiation (the studied problem-solution pair).
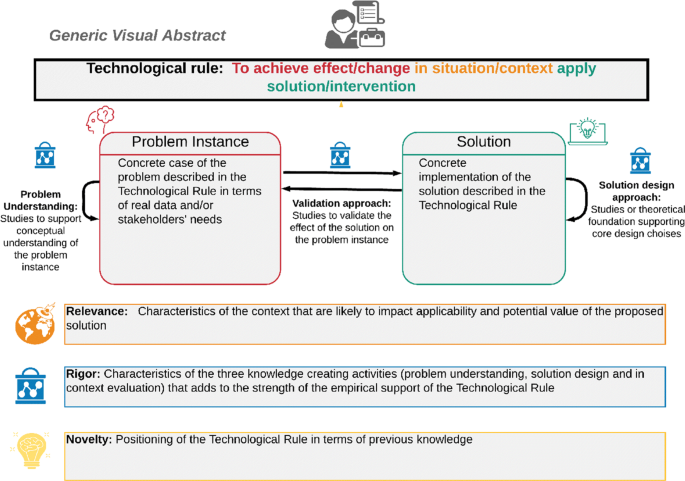
The visual abstract template (Storey et al. 2017 ) capturing 1) the theory proposed or refined in terms of a technological rule; 2) the empirical contribution of the study in terms of a problem-solution instance and the corresponding design and validation cycles; and 3) support for the assessment of the value of the produced knowledge in terms of relevance, rigor, and novelty
2.2.1 The Technological Rule
In line with van Aken ( 2004 ), our visual abstract template emphasizes technological rules (the top box in Fig. 2 ) as the main takeaway of design science within software engineering research. A technological rule can be expressed in the form: To achieve <Effect > in <Situation > apply <Intervention> . Here, a class of software engineering problems is generalized to a stakeholder’s desired effect of applying a potential intervention in a specified situation. Making this problem generalization explicit helps the researcher identify and communicate the different value-creating aspects of a research study or program. Refinements or evaluation of the technological rule may be derived from any one of the three processes of problem conceptualization , solution design , or empirical validation , applied in each instantiation.
Technological rules can be expressed at any convenient abstraction level and be hierarchically related to each other. However, technological rules expressed at a high abstraction level (e.g., “to produce software of high quality, apply good software engineering practices”) tend to be either too high-level or too bold (easy to debunk). On the other hand, an abstraction level that is too low level may lead to narrowly scoped and detailed rules that may lack relevance for most software engineers. It is important to explicitly formulate the technological rule when presenting design science research and to be consistent with it both when arguing for its relevance and novelty, as well as when presenting the empirical (or analytical) support for the claims.
2.2.2 The Problem-Solution Pair
The main body of the visual abstract template (the middle section in Fig. 2 ) focuses on the empirical contribution of one or more studies, and is composed of two boxes for the problem-solution instantiation of the technological rule and three corresponding descriptions of the knowledge-creating activities, problem conceptualization, solution design, and validation. This part of the VA helps to distinguish which empirical studies are done and how they contribute to insights about the problem, the solution, or solution evaluation.
2.2.3 The Assessment Criteria
The ultimate goal of design science research is to produce general design knowledge rather than to solve the problems of unique instances. Thus, the value of the research should be assessed with respect to the technological rule (i.e., the design knowledge) produced. The information in the three assessment boxes (the bottom of Fig. 2 ) aims to help the reader make an assessment that is relevant for their context. Hevner presents three research cycles in the conceptual model of design science, namely the relevance , rigor , and design cycles (Hevner et al. 2004 ). We propose that the contributions of design science research be assessed accordingly with respect to relevance, rigor , and novelty .
The relevance box aims to support answering the question To whom is this technological rule relevant? Relevance is a subjective concept and we are not striving to find a general definition. Instead we suggest that the basic information needed to assess relevance of a research contribution is the potential effect of the proposed intervention combined with the addressed context factors. The relevance of a research contribution could be viewed from two perspectives: the targeted practitioner’s perspective, and the research community’s perspective. From the individual practitioner’s point of view, the relevance of a research contribution relates to the prevalence and severity of the addressed problem and the applicability of the proposed intervention. This can be assessed by comparing their specific context with the one described in the research report. For the research community, a measure of relevance often relates to how common or severe the studied problem is. To enable both types of assessments, relevant context factors need to be reported.
The rigor box aims to support answering the question How mature is the technological rule? Rigor of a design science study refers to the strength of the added support for the technological rule and may be assessed with respect to all of the three knowledge-creating activities: problem conceptualization, solution design, and empirical validation. However, solution design is a creative process that does not necessarily add to the rigor of a study. One aspect of rigor in the design activity could be the extent to which the design is built on prior design knowledge. Also, the consideration of alternative solutions could be taken into account. The other two activities—problem conceptualization and empirical validation—are based on common empirical methods on which relevant validity criteria (e.g., construct validity) can be applied. Note that the template only captures the claims made in the paper, and the validity of the claims are assumed to be assessed in the peer review process.
The novelty box aims to capture the positioning of the technological rule in terms of previous knowledge, and it supports answering the question Are there other comparable rules (similar, more precise, or more general rules) that should also be considered when designing a similar solution in another context? Technological rules may be expressed at several abstraction levels; thus, it is always possible to identify a lower abstraction level where a research contribution may be novel, but doing so may be at the cost of more general relevance. For example, a technological rule that expresses the efficiency of a technique in general may be made more specialized if it instead expresses the efficiency in one specific type of project that has been studied. Then the relevance is less general, and the novelty may be increased since it is the first investigation at that level of detail. Similarly, rigor is increased since the claims are less bold.
To optimize rigor, novelty, and relevance of reported research, the researcher should strive to express the technological rule at the highest useful abstraction level, i.e., a level at which it is novel, the provided evidence gives strong support and it is not debunked by previous studies (or common sense). However, adding empirical support for existing but under-evaluated technological rules has value, making novelty less important than the rigor and relevance criteria. To this extent, replication of experiments has been discussed (Carver et al. 2014 ; Juristo and Gómez 2010 ; Shull et al. 2008 ) and is encouraged by the software engineering community. Footnote 2 The incremental adding of empirical support for a technological rule could be referred to as conceptual replication in which the same research question is evaluated by using a different study design, as discussed by Shull et al. ( 2008 ).
3 Methodology
The main goal of this work was to investigate how well software engineering (SE) research contributions are aligned with a design science paradigm. As part of this work, we aimed to answer the following research questions :
From a design science perspective, what types of contributions do we find in the SE community?
In papers that present design knowledge, how clearly are the theoretical contributions (i.e., the technological rules ) defined in these papers?
How are novelty , relevance and rigor discussed in papers with design knowledge contributions?
As mentioned above, our earlier research produced a visual abstract template for communicating design science research in SE (Storey et al. 2017 ). The principal steps of that study are presented in the left part of Fig. 3 . We started by reviewing the design science literature and extracting elements of the paradigm from different authors (van Aken 2004 ; Hevner et al. 2004 ; Wieringa 2014 ), from which we created our initial conceptualization of design science as a paradigm for SE. We then studied SE papers with design science contributions, and extracted information related to such contributions from the papers iteratively to identify elements of design science . This work resulted in the visual abstract template, with examples of SE research (Storey et al. 2017 ).
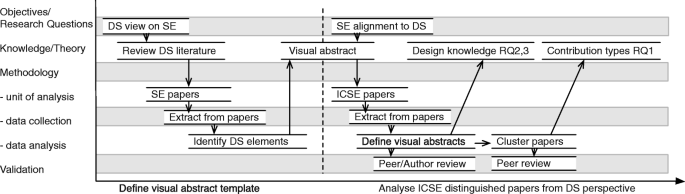
The approach followed to develop the initial version of the visual abstract (the left side) and the main steps of the research presented in this paper (the right side)
In this paper we describe how we use the visual abstract template to describe the research contributions in a particular set of papers from the main technical track at the ICSE conference: those that were selected as the top 10% of papers (i.e., papers designated the ACM SIGSOFT Distinguished Paper Award Footnote 3 ) across five years of the conference (2014–2018 inclusive) We chose ICSE because it is considered to be one of the top publishing venues in software engineering that covers a broad set of diverse topics, and we chose the “best” of those papers because we expected this cohort would represent exemplars of fine research. In total, we considered and applied the visual abstract template to describe the research contributions across 38 papers, which are listed separately at the end of the references for this paper.
The principal steps of this study are outlined in the right part of Fig. 3 and elaborated below. Each paper in the cohort of the ICSE distinguished papers from 2014–2018 was randomly assigned to two reviewers (among the authors of this paper). As the work was not about judging papers, but about understanding, we did not care for any conflicts of interest. The two reviewers independently extracted information from the papers to answer the set of design science questions listed in Table 1 . This set of questions were derived from the constituents of our conceptualization of design science as a paradigm.
The first author derived an initial list of questions, which were reviewed by the other authors with minor changes. Since the visual abstract also represents the constituents of design science, the questions map to each of the elements in the visual abstract template. Thus, we defined a visual abstract for each paper, which we iterated until we arrived at an agreement for a shared response to these questions, seeking additional input through peer reviews by the rest of the research groups of the authors or other experts for papers on topics unfamiliar to us.
The answers to the questions were captured in a spreadsheet to facilitate future analysis as well as ongoing review and internal auditing of our process. Our combined responses were then used to populate the visual abstract template for each paper, from which we analyzed the design knowledge contributions in SE (RQ 2 and 3). The collection of visual abstracts for all of the papers is available online at http://dsse.org , which constitutes our combined understanding of the analyzed software engineering research from a design science perspective.
As part of our analysis, we wanted to validate our interpretations with the original authors . To assess the value of such validation, we confirmed our interpretations of the 2014 and 2015 ICSE distinguished papers with the original authors. We heard back from half of the authors of this set of papers, who confirmed the accuracy of our responses (mentioning minor improvements only). We assessed this feedback as a preliminary validation of our process and did not see the need to repeat this step for the other papers—in each case, the abstracts for all papers we studied are available online Footnote 4 and the authors may comment publicly on our interpretations if they choose.
Once we finished creating all the visual abstracts, we clustered the papers (see the rightmost part of Fig. 3 ) to identify contribution types (RQ1). As we answered the questions in Table 1 , we presented our answers to other members in this paper’s author team for peer review , which in many cases led to refinements in our responses. We also printed the visual abstracts we created for each paper (in miniature), and working as a group in a face-to-face meeting, we sorted the visual abstracts into clusters to identify different types of design science contributions.
Following this face-to-face visual abstract clustering activity, we worked again in pairs to inspect each of the papers in the clusters to confirm our categorization. Then, we reviewed and confirmed the categorization of each paper as a group. During this confirmation process, we refined our categorization and collapsed two categories into one: we combined papers that were initially classified as exploratory with papers that we initially thought were design science contributions in terms of problem understanding, but on reflection these were better framed as explanatory papers as the investigated problems were not linked to a specific solution. We present the stable clusters that emerged from these activities in the following section of this paper.
4 Results from the Paper Cluster Analysis
Overall we identified five clusters, described in detail below, based on our analysis of how each paper contributed to the extracted technological rule. Note the rules are not extracted by the original authors of the papers but by us for the purpose of this particular review of the papers according to their design science contributions.
Problem-solution pair : this cluster represents papers that balance their focus on a problem instance and solution.
Solution validation : this cluster is characterized by papers that concentrate largely on the solution and its validation, rather than on problem conceptualization.
Solution design : papers in this cluster focus on the design of the solution more than on problem conceptualization or solution validation.
Descriptive : these papers address a general software engineering phenomenon rather than a specific instance of a problem-solution pair.
Meta : this cluster of papers may be any of the types above but are aimed at contributing research insights for researchers rather than for practitioners.
Figure 4 illustrates how the first four clusters (1–4) map to a design science view, including both the problem-solution dimension and the theory-practice one. Clusters 1–3 each represent different types of design science research contributions since the papers in these clusters consider explicit problem-solution pairs. Papers in the 4th cluster contribute explanatory knowledge and, although such knowledge may support software engineering solution design, they are better framed through an explanatory lens. Cluster 5 is not represented in this figure as these papers produce knowledge for software engineering researchers rather than for software engineering practitioners.
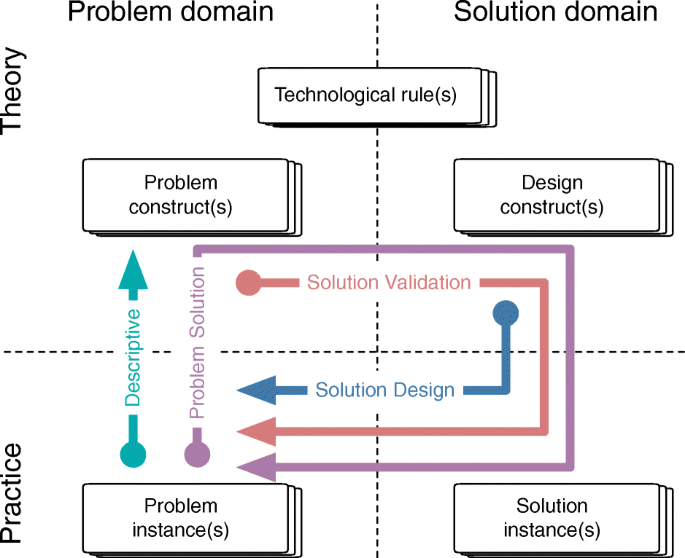
An illustration of how the identified clusters map to the problem/solution and the practice/theory axes respectively. The arrows show how typical studies in each cluster traverse the four quadrants (1. practical problem, 2. conceptual problem description, 3. general solution design, and 4. instantiated solution)
Figure 5 presents a visual representation of the main clusters that emerged from our analysis along with a listing of which papers (first author/year) belong to the different clusters. The two axes of this graph are defined as follows: the x-axis captures the solution contribution ranging from high-level recommendations to more concrete solutions that are designed and may be validated; and the y-axis indicates the problem understanding contribution, whereby the problem is already known or assumed, to where new insights are produced from the research.
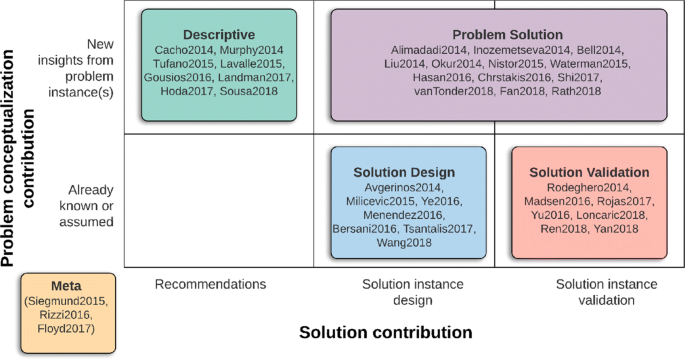
The main clusters that emerged from our analysis of the papers, showing the key design science contributions in terms of problem understanding insights and solution recommendations, design and/or validation
A more detailed and nuanced description for each cluster is provided below. For each cluster we refer to examples of papers from our analysis and include one visual abstract for each example to showcase the design knowledge that is or is not captured by each cluster.
4.1 Problem-Solution Pair
For the papers in this cluster, a problem instance is identified and investigated to gain a generalized problem formulation matching the proposed solution. A solution is proposed, designed and implemented, then validated rigorously in-context through empirical methods. It is the most populated cluster, indicating that many software engineering papers can be framed in accordance with a design science paradigm.
The technological rule is defined quite clearly in all of the papers belonging to this cluster and is in most cases a new proposal of either a tool or methodological approach necessary to solve the problem instance (see Fig. 6 ). Consequently, the relation between problem (e.g., difficulty of estimating energy consumption in Java Collection classes) and solution (e.g., use per-method energy profiles to chose among Java Collections and reduce/optimize energy consumption) is explicit.
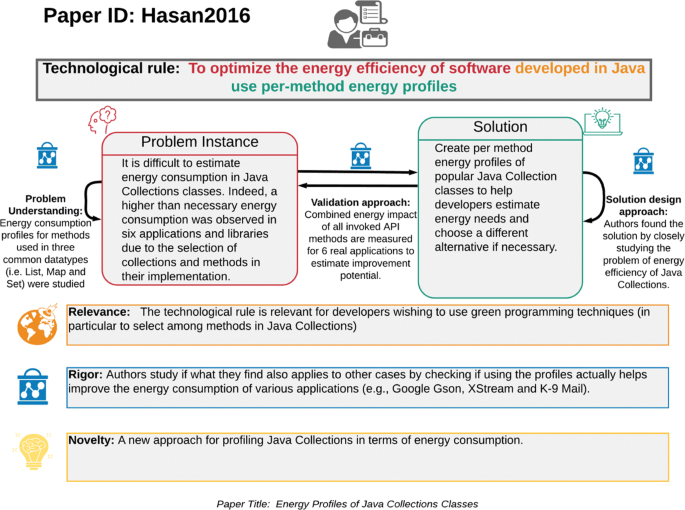
Visual abstract of a typical paper in the problem solution cluster, Hasan et al. ( 2016 )
Solutions are geared towards both practitioners and researchers, making it explicit and easy for a stakeholder to assess the relevance of the rule for their specific case. The solutions are mainly validated by conducting case studies on real projects (Nistor et al. 2015 ) or controlled experiments (Alimadadi et al. 2014 ; Bell and Kaiser 2014 ).
In some cases, alternative solutions are compared to the proposals made. For example, Rath et al. ( 2018 ) considered alternative information retrieval techniques and classifiers during the design of their solution, and used precision/recall values collected from all of the compared solutions to develop their classifier.
A representative example for this cluster is the paper by Hasan et al. ( 2016 ). The problem instance outlines how it is difficult to estimate energy consumption in Java Collections classes. As a solution, the authors created detailed profiles of commonly used API methods for three Java Collections datatypes, namely: List, Map, and Set, and validated them through a case study on Java libraries and applications. In this way, developers can use the information of the usage context of a data structure and the measured energy profiles to decide between alternative collection implementations and optimize their solutions. The visual abstract is shown in Fig. 6 . Other visual abstracts in this cluster (and other clusters) are available on our online website. Footnote 5
In summary, the problem-solution cluster papers can be seen as presenting complete design science contributions, considering both the general and specific aspects of a problem-solution pair investigated in context, with implications for researchers and practitioners.
4.2 Solution Validation
Papers in the solution validation cluster mainly focus on refining a previously proposed, but often implicit, technological rule. The problem is implicitly derived from a previous solution and its limitations rather than from an observed problem instance. Accordingly, in most cases, the problem is motivated by a general statement at an abstract level, making claims about “many bugs...” or “it is hard to...”. Some of the papers underpin these claims with references to empirical studies, either the authors’ own studies or from the literature, while others ground the motivation in what is assumed to be generally “known”.
As a typical example for this cluster, Loncaric et al. ( 2018 ) identify that others have tried to automate the synthesis of data structures and present a tool that embeds a new technique that overcomes the limitations of previous work. A proof of concept is demonstrated through four real cases. The corresponding visual abstract is presented in Fig. 7 .
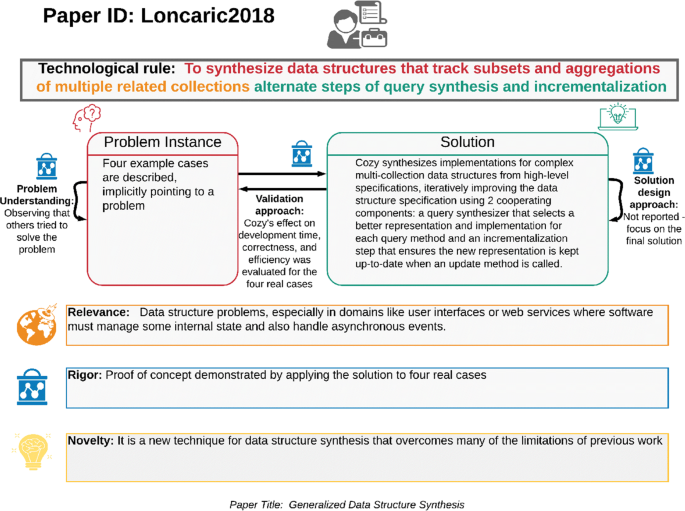
Visual abstract of a typical paper in the cluster of solution validation studies, Loncaric et al. ( 2018 )
Note that some of the papers in this cluster focus on understanding the problem with previous solutions, with the aim to improve the solution or come up with a new solution. For example, Rodeghero et al. ( 2014 ) attempt to improve code summarization techniques for program comprehension. They perform an extensive eye-tracking study to design a code summarization tool.
The technological rules are mostly implicit in these papers. As they are related to problems with existing solutions, rather than original problems in the SE domain, the presentation of the solutions are mostly related to previous solutions. A technological rule can sometimes be derived indirectly, through the aim of an earlier solution, but it is rarely defined explicitly.
The papers in this cluster discuss relevance to research explicitly, while the relevance to practice is mostly discussed indirectly, and at a high abstraction level. For example, Rojas et al. ( 2017 ) claim that writing good test cases and generating mutations is hard and boring, and thus they propose a gaming approach to make this more enjoyable and better. The validation is conducted, testing a specific code instance, while the original problem is rooted in high-level common sense knowledge. However, there are other papers in the cluster that back up the problem through evidence, such as a vulnerability database, used by Yan et al. ( 2018 ) to motivate addressing the vulnerability problem of Use-After-Free pointers.
In summary, the solution validation papers focus on refining an existing technological rule. The motivating problem is mostly expressed in terms of high-level knowledge, rather than specific instances, although some papers refer to empirical evidence for the existence and relevance of the problem. The more specific problem description is often related to problems with previous solutions. The papers clearly show a design science character, although they are at risk of solving academic problems, rather than practitioners’ problem instances.
4.3 Solution Design
The papers in this cluster present details of a new instantiation of a general solution. For example, Avgerinos et al. ( 2014 ) present a new way of testing with symbolic execution, see Fig. 8 . The presented approach finds more bugs than the previously available methods. However, the need for this tool was not explicitly stated and the authors perhaps assume the need is clear.
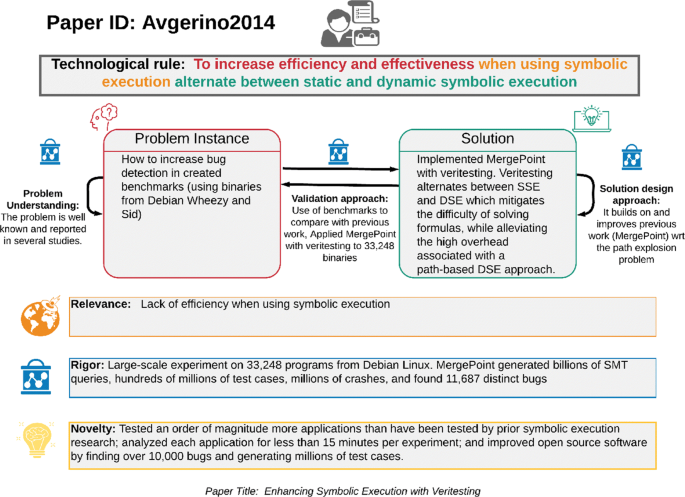
Visual abstract of a typical paper in the solution design cluster, Avgerinos et al. ( 2014 )
Similarly, Bersani et al. ( 2016 ) propose a new semantics for metric temporal logic (MTL) called Lazy Semantics for addressing memory scalability. The proposal builds on previous research and is focused on the solution, a new trace checking algorithm. A similar observation can be made for analysis and validation. For example, the analysis in Avgerinos et al. ( 2014 ) is conducted by using the proposed solution on a rather large code base and using well known metrics such as number of faults found, node coverage, and path coverage. Whereas in Bersani et al. ( 2016 ), the validation is carried out comparing the designed solution with other, point-based semantics.
For papers in this cluster, the problem is not explicitly formulated but it is more generally discussed in terms of, for example, decreasing the number of faults. The papers tend to describe the designed solutions in rather technical terms. This is also how the novelty typically is highlighted. Validations are often conducted by applying the proposed solution on a code base and analyzing metrics of, e.g., the number of faults found in testing, and no humans are directly involved as subjects in validations. Empirical data for the validations are either obtained by technically measuring e.g., execution time, or by using data already published in programmer-forums.
In summary, the solution design papers focus on low level technological rules. The motivating problem is in most cases technical details of a solution to a more general problem. While the validity of the general solution is implicit, the low level solution is often validated through controlled experiments or benchmarking in a laboratory setting. The papers clearly show a design science character, although at a low abstraction level.
4.4 Descriptive
The papers categorized in this cluster develop an understanding of a software engineering phenomenon that is currently not well understood. Such research studies may expose problems that need to be addressed, or they may reveal practices or tools that could benefit other challenging software engineering scenarios.
For example, Murphy-Hill et al. ( 2014 ) conducted a study of game developers and identify a number of recommendations for how game developers could be better supported through improved tools or practices, while Hoda and Noble ( 2017 ) carried out a grounded theory study to achieve an understanding of how teams transition to agile.
Concrete instances of software engineering phenomena have been studied in various ways. Gousios et al. ( 2016 ) surveyed 4,000 open source contributors to understand the pull-based code contribution process, Tufano et al. ( 2015 ) analyzed git commits from 200 open source repositories to investigate more about code smells, Cacho et al. ( 2014 ) studied changes to 119 versions of code extracted from 16 different projects to understand trade-offs between robustness and maintenance and Lavallée and Robillard ( 2015 ) reported on a 10 month observational study of one software development team to understand why “good developers write bad code”.
Figure 9 shows a typical example of a visual abstract from this cluster. The theoretical contributions of these studies are descriptive problem characterizations. In four out of eight papers, a list of recommendations is provided as well. Thus, it is in most cases possible to derive several technological rules from each such paper. However, these technological rules are not instantiated or evaluated further, and neither are they highlighted as the main contributions of the reported studies.
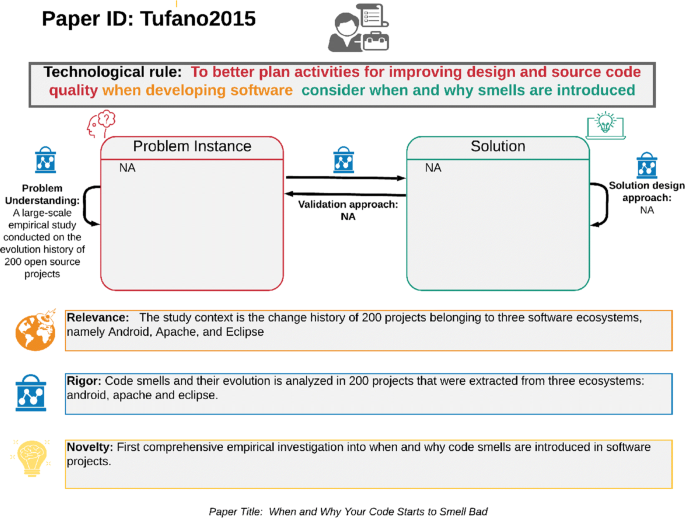
Visual abstract of a typical paper in the cluster of descriptive studies, Tufano et al. ( 2015 ). In this case the visual abstract template does not match the type of study, why some boxes are left empty (NA)
All papers in this cluster discuss relevance to practice: many explicitly discuss how common the phenomenon under study is (e.g., Gousios et al. 2016 show a diagram of the monthly growth of pull request usage on GitHub). Others implicitly highlight a knowledge gap assumed to be of importance (e.g., Lavallee et al. 2015 pinpoint the lack of knowledge about the impact of organizational factors on software quality). Novelty or positioning is, however, not described in terms of the problem or the solution but about aspects of the study as a whole. Gousios et al. ( 2016 ) add a novel perspective , the contributors’ code review, Lavallée and Robillard ( 2015 ) add more empirical data about organizational factors and software quality, and Tufano et al. ( 2015 ) claim to report the first empirical investigation of how code smells evolve over time.
In summary, although the descriptive papers may contribute to design knowledge, i.e., understanding of conceptual problems and initial recommendations, design knowledge in the form of technological rules are not directly described in the papers. The main contributions are discussed in more general terms such as descriptions of the phenomenon under study (defined in the titles) and general information about the study approach and the studied instances (which often appears in the abstracts). Potential problems and their solutions are described in the discussion sections of the papers. Their relevance to practice is in terms of the real-world problems or recommendations that other applications have that tend to be exposed by these kind of papers. Thus, such papers are typically reporting on exploratory research that may be quite high in novelty.
Three of the distinguished papers we reviewed do not aim to identify or solve software engineering problems in the real world. Rather, these studies aim at identifying or solving problems which software engineering researchers may experience. We therefore refer to them as Meta studies, i.e., addressing the meta level of software engineering research in contrast to the primary level of software engineering practice . Siegmund et al. ( 2015 ) conducted a study that reveals how the software engineering research community lacks a consensus on internal and external validity. Rizzi et al. ( 2016 ) advise researchers about how to improve the efficiency of tools that support large-scale trace checking. Finally, Floyd et al. ( 2017 ) propose how fMRI methods can help software engineering researchers gain more insights on how developers comprehend code, and in turn may improve comprehension activities. We show the visual abstract for the Floyd et al. ( 2017 ) paper in Fig. 10 .
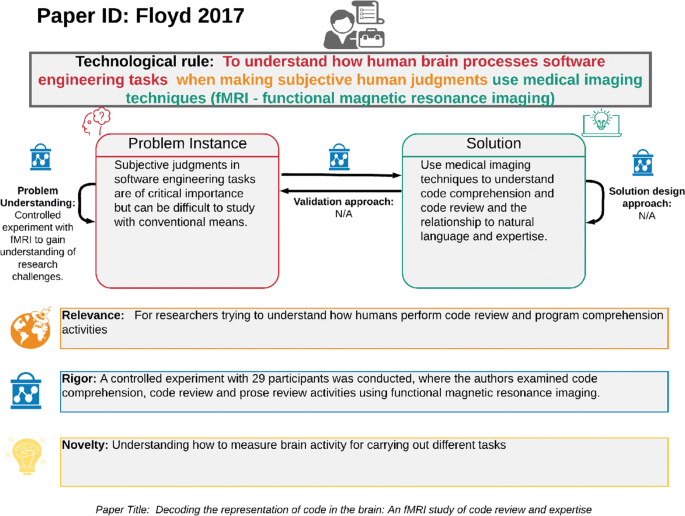
A typical example of a visual abstract in the Meta cluster, Floyd et al. ( 2017 )
Meta papers address software engineering research problems, and propose solutions for software engineering researchers. The design knowledge gained in these studies is primarily about the design of software engineering research , and the key stakeholders of the technological rule are rather the researchers rather than software engineers. Still, they fall under a design science paradigm and the Meta category of papers may show relevance to industry but in an indirect manner.
In summary, papers that we describe as Meta may fall under a design science research paradigm, leading to a technological rule with researchers rather than software engineers as the key stakeholders.
5 Discussion: Design Science Contributions in Software Engineering
The long term goal of much software engineering research is to provide useful recommendations on how to address real-world problems providing evidence for benefits and potential weaknesses of those recommendations. Our analysis of ICSE distinguished papers reveals empirical contributions (RQ1) related to problem conceptualization , solution design , solution instantiation , and empirical validation (see the path traversal in Fig. 4 ). In 13 of the 38 papers we analyzed, all four activities are explored in equal depth while others focus on one or two activities, as shown in the clusterings above in Section 4 . All of those activities generate knowledge corresponding to the elements of our visual abstract template (see Fig. 2 ). However, none of the papers are presented in terms of these elements and we had to spend significant effort using the questions in Table 1 to extract this knowledge in a systematic way. Extracting technological rules from most papers was also quite challenging. That said, applying the design science lens helped us notice and distinguish the different kinds of design contributions from the papers we analyzed, and guided our assessment of the papers in terms of research relevance, rigor and novelty. We discuss our experiences using the design science lens below and also the challenges we faced applying it to different types of papers. We also allude to experiences we have faced as researchers and reviewers of research papers below.
5.1 Problem Conceptualization and Descriptive Research in Software Engineering
We found a design science paradigm helped us distinguish descriptive research contributions from prescriptive research contributions in the papers we analyzed. Indeed eight of the papers we analyzed focused primarily on the understanding of software engineering problems or phenomenon that were not currently well understood. Descriptive papers are often labeled by the authors as “exploratory” research. Often these papers do not only describe or expose specific problems or phenomenon, but they may also describe why or how certain solutions or interventions are used, and conclude with directions for future research or with recommendations for practitioners to consider (e.g., to use a studied intervention in a new context).
We struggled at first to apply the design science lens to some of these descriptive papers as for most of them no explicit intervention or recommendations were described or given. Articulating clear technological rules was not possible as this research does not aim at producing design prescriptions (yet). However, on reflection we recognized that the design science lens helped us to recognize and appreciate the longer term goals behind this exploratory research that would later culminate in design knowledge. Sometimes we found that descriptive research is under appreciated over prescriptive solutions, but understanding problems clearly is also an important research contribution in a field like software engineering that changes rapidly. In fact, often researchers are “catching up” to what is happening in industry and to recognize new emerging problems that may arise in industrial settings as tools and practices evolve.
Another cluster of papers we identified, the 13 problem-solution pair papers, also contribute insights on problems experienced in software engineering projects. Many of the problem-solution papers derive problem insights from specific problem instances. This was the biggest cluster of papers. The design science lens helped us recognize and appreciate that these papers had contributions, not just on the solution design and validation side, but also contributed or confirmed insights on a studied problem. We have all had experiences when reviewing papers where a co-reviewer failed to recognize problem understanding contributions and argued that a given solution was either too trivial or poorly evaluated. As papers are written (and then typically read) in a linear fashion, losing track of the various contributions can happen. For us, laying out the contributions visually (and by answering the questions we explicitly posed in Table 1 ) helped us keep track of and appreciate contributions on both the problem and solution aspects.
5.2 Solution Design Contributions in Software Engineering Research
The other two main clusters of papers that are aimed at improving software engineering practice are the seven solution-design papers and seven solution-validation papers. These papers contribute design knowledge concerning an intervention and mostly rely on either previous research or accepted wisdom that the problem they address is in need of solving. For these papers, the first questions in Table 1 about the problem instance addressed and problem understanding approach did not always have an explicit answer in the paper. However, to conduct an empirical validation of the design, some kind of instantiation of the problem is required and we referred to these instances when extracting information about problem instance and problem understanding for our analysis. We found this to be an effective way to address distances between the abstraction level of the proposed technological rule and its empirical validation. Papers without specified problem instances are at risk of proposing solutions, which do not respond to real software engineering problems.
5.3 Identifying Technological Rules from Software Engineering Research
For most papers, we were able to extract technological rules from the presented research. However, none of the papers had any conclusion or recommendation in such a condensed form (see RQ2). In some cases, the abstracts and introduction sections were written clearly enough that we could identify the intended effect, the situation and the proposed solution intervention presented in the paper. Moreover, when research goals and questions were explicitly stated, technological rules were easier to formulate. Other papers required more detailed reading to extract the needed information. In some publication venues, structured abstracts are introduced as a means to achieve similar clarity and standardization (Budgen et al. 2008 ), but such abstracts are not typically used for ICSE. Introducing technological rules would, we believe, help in communicating the core of the contribution, both to peer academics and potentially also to industry. Development towards more explicit theory building in software engineering (Sjøberg et al. 2008 ; Stol and Fitzgerald 2015 ) may also pave the way for technological rules as a means to express theoretical contributions.
5.4 Assessing Design Knowledge Contributions: Rigor, Relevance and Novelty
Our analysis of rigor, relevance and novelty are based on questions 7-9 in Table 1 . Rigor can be considered in terms of the suitability of specific research methods used or in how a certain method is applied. Empirical research methods – quantitative as well as qualitative – fit well into both problem conceptualization and validation and we saw examples of very different methods being used. How rigor is ensured of course depends on the choice of method as we discuss above. We found that most authors discussed rigor —not surprising given that these papers were considered as the best papers from an already competitive publishing venue (see RQ3). Whether rigor was discussed for the steps of problem conceptualization, solution design and empirical validation, depended on the paper cluster. The solution validation and solution design papers tended to rigorously benchmark their solution against a code base or other artifacts to demonstrate the merits of the proposed approach. We found that validating the solutions in industrial contexts was not common in these two clusters of papers. Consequently we also found that relevance in terms of specific stakeholders was not discussed much in these papers (as compared to the descriptive or problem-solution clusters of papers).
How novelty was discussed by authors varied greatly depending on the paper cluster but also by the author. As the papers did not explicate the technological rules, none of them discussed their contribution in terms of technological rule novelty. Descriptive papers tended to focus on novelty of the described problem or phenomenon, problem-solution and solution-design papers focused on novelty of the solution, and solution-validation papers emphasized the solution (if refined or new) and insights from the solution validation.
6 Recommendations for Software Engineering Research
As researchers (and reviewers) ourselves we find that contributions from research papers are often not evident, and thus interested researchers and reviewers may miss the value in the papers. Furthermore, the technological rules, even for papers that aim at producing these rules, are not always easy to identify. To help in the design of research and perhaps also in the review of papers, we suggest using the design lens as follows:
Explicate design science constructs : We found design science constructs in most papers, but presenting each of the constructs explicitly, e.g., through the visual abstract (Storey et al. 2017 ), could help in communicating the research contributions to peer researchers and reviewers. Expressing the technological rules clearly and at a carefully selected level of abstraction, help in communicating the novelty of the contributions and may help in advancing the research in “standing on each others shoulders”.
Use real problem instances : Anchoring research in real problem instances could help to ensure the relevance of a solution. Without reference to an explicit problem instance, the research is at risk of losing the connection with the original question, as the details of a particular intervention are described or assessed by others.
Choose validation methods and context : Rigor in terms of method choice is an important consideration. The choice of methods and context for the validation may be different, depending on the intended scope of the theoretical contribution (i.e., the technological rule). If the scope is focused on fine tuning the design of an intervention, stakeholders may not need to be directly involved in the validation. If however the scope includes the perspective of stakeholders and their context, then methods and study contexts should reflect these perspectives.
Use the design science lens as a research guide : The visual abstract and its design science perspective may also be used to guide the design of studies and research programs, i.e., setting particular studies in a particular context. Similarly, a design science perspective can be used as an analysis tool in mapping studies (Petersen et al. 2008 ), to assess existing research and identify research gaps that can be explored in future research studies and lead to novel contributions.
Consider research design as a design science : The cluster of meta studies, which are primarily aimed for researchers as the stakeholders, indicate that the design science lens also fits for the design and conduct of studies that focus on understanding our research methodology and methods. Papers that address problems in conducting research and propose solutions to help achieve higher quality research contributions are important contributions for our community to reflect and grow in research maturity. Conducting and presenting these in the same way as studies in the software engineering domain adds to their credibility and emphasizes how they are relevant to our community. This paper is also an example of a meta study aimed at our research community members as stakeholders. We created a visual abstract for this paper as well, and it may be found with our online materials (at http://dsse.org ).
We hypothesize that following these recommendations, based on our in depth analysis of ICSE distinguished papers, would enable a more consistent assessment of rigor, relevance and novelty of the research contributions, and thus also help the peer review process for future conference and journal publications.
7 Limitations
In order to understand how design science can be a useful lens for describing software engineering research, we considered all papers that have received a distinguished paper award over a five year period within a major venue such as ICSE. We felt these may represent papers that our community considers relevant and fine exemplars of SE research. We acknowledge that we would likely see a different result for a different population of papers (e.g., all papers presented at ICSE or in other tracks or venues or journals). That said, we purposefully selected this sample of papers as an exploratory step.
Our view of design science may differ from other views that are reported in the literature. We developed it from examining several interpretations of design science as discussed in Storey et al. ( 2017 ) and in Section 2 . Our common view of design science in software engineering was developed over the course of two years spent reading and discussing many design science papers in related domains. Our interpretation was developed in an iterative manner. We have used our visual abstract template in several workshops (notably at ISERN 2017, Footnote 6 RET 2017 Footnote 7 and CBSoft 2019 Footnote 8 ) and received favorable feedback about the viable application of the template to software engineering papers that contain design knowledge. However, we recognize that applying the visual abstract to papers is not always straightforward and we found that even among our team, we would apply it differently and pull out different highlights from the papers we read. We found that the process of applying it is in the end more important than the actual text we put in the boxes as doing so helped us understand the main contributions in the papers we analyzed from a design science perspective.
We recognize that our interpretations of the research contributions from the papers we examined may not be entirely accurate or complete. For this reason we requested feedback from the authors of the papers from 2014 and 2015 to check that our view of the design knowledge in their papers was accurate based on our understanding of their work. Among the responses we received (7 of 14 of the paper authors responded), all but one agreed with our summaries presented through the visual abstracts, while the sole initial disagreement was due to misinterpretation of the visual abstract template. This feedback convinced us that we were proceeding in the right direction. Consequently, we decided to rely on our judgment alone for the remaining papers.
To do our own validation, we divided papers equally among us assigning two of us to each paper. We would independently answer the design science questions (as mentioned in Section 3 ), then refer back to the paper in cases of disagreement, and merge our responses until we reached full agreement. With cases of ongoing disagreement, we sought additional expert opinions. Finally, we reviewed all of the abstracts as a group to reconfirm our interpretations. These abstracts are available online and open for external audit by the paper authors or by others in the community.
To derive clusters of the papers, we followed a rigorous process. We met face to face in a several hour workshop and followed up in several sessions to derive the clusters and categorize and reconfirm the categorization of the papers. We recognize that how we clustered the papers is potentially subjective and others may feel papers belong in different clusters, and may also find different clusters. We have posted all of the visual abstracts and our cluster diagram online which links to all of the visual abstracts (see https://doi.org/10.5281/zenodo.3465675 ). We welcome comments on our clusters and the categorization of individual papers.
8 Related Work
In this paper, we introduced our conceptualization of design science and the visual abstract template which instantiates our conceptualization and was designed to support communication and dissemination of design knowledge. Furthermore, we reviewed a cohort of software engineering research papers through this lens to understand the design science contributions in the papers. In this section of the paper, we extend the scope of related work to include other conceptualizations of design science, as well as other reviews of design science research conducted in a related field.
Design science has been conceptualized by Wieringa in software engineering (Wieringa 2009 ) and by several researchers in other disciplines, such as information systems (Hevner et al. 2004 ; Gregor and Hevner 2013 ; Johannesson and Perjons 2014 ) and organization and management (van Aken 2005 ). Wieringa describes design science as an act of producing knowledge by designing useful things (Wieringa 2009 ) and makes a distinction between knowledge problems and practical problems. Similarly, Gregor and Hevner emphasize the dual focus on the artifact and its design (Gregor and Hevner 2013 ) in information systems, and argue for an iterative design process where evaluation of the artifact provides feedback to improve both the design process and the artifact.
In our paper, we do not distinguish between knowledge problems and solution problems within the design sciences, but stress that the researcher’s task is always to produce knowledge, which in turn can be used by practitioners for solving their problems. Such knowledge may be embedded in artifacts such as tools, models and techniques or distilled to simple technological rules. In accordance with van Aken ( 2005 ), we distinguish between the explanatory sciences and the design sciences as two different paradigms producing different types of theory (explanatory and prescriptive, respectively) with different validity criteria. This is similar to Wieringa’s distinction between knowledge problems and practical problems (Wieringa 2009 ). In our study, we identified one cluster of software engineering papers belonging to the explanatory sciences (descriptive) and three clusters of papers belonging to the design sciences (problem-solution, solution-design, and solution evaluation)
In the management domain, van Aken propose to distinguish management theory, that is prescriptive, from organizational theory, that is explanatory (van Aken 2005 ). A corresponding division of software engineering theory has not been proposed yet, although theory types are discussed by software engineering researchers (Sjøberg et al. 2008 ; Stol and Fitzgerald 2013 ).
In the literature, design science has been studied and is thereby conceptualized in a number literature studies, which are relevant for this study. In the area of information systems, several literature reviews were conducted of design science research. Indulska and Recker ( 2008 ) analyzed design science articles from 2005–07 from well-known information systems conferences. They identified 142 articles, which they divided into groups, such as methodology- and discussion-oriented papers and papers presenting implementations of the design science approach. They found an increasing number of design science papers over the studied years.
Deng et al. ( 2017 ) and Deng and Ji ( 2018 ) also published a systematic review of design science articles in information systems. They identified articles by searching in top information systems journals and conferences from the years 2001–15, filtering the results and applying snow-balling, resulting in a final review sample of 119 papers or books. In their review, they analyze the topic addressed, artifact type, and evaluation method used. In our review, we classified papers along another dimension, i.e., what types of software engineering design science contributions the papers present in terms of problem understanding, solution design and solution validation. To our knowledge no reviews of software engineering literature have been made from a design science perspective before.
Wieringa et al. ( 2011 ) analyzed reasons for the low use of theories in software engineering by studying a set of papers identified in Hannay et al. ( 2007 ). They compare identified theories in software engineering to general theories with respect to level of generalization, form of theory, and use of theory, and argue that the reasons for low use of theories have to do with idealizing assumptions, context of software engineering theories, and that statistical model building needs no theories.
Concerning research relevance, Beecham et al. communicated with a test group of practitioners (Beecham et al. 2014 ) and found that evidence based on experience was seen as most important, and if it was not available in their own organization, they would seek information from similar organizations in the world for insights on global software engineering. They compare typical sources for software engineering researchers and sources where practitioners seek information and found that the overlap is very small. Similar findings were obtained by Rainer et al. ( 2003 ) study based on focus groups with practitioners and a literature review. These observations point to the need for presenting research in a way that is useful for practitioners. This is also discussed by Grigoleit et al. ( 2015 ), who conclude that practitioners assess the usefulness of many artifacts as being too low. This is in line with our findings, where we put forward the design science lens as a means to better communicate prescriptive research contributions in software engineering. That said, we have not evaluated it with practitioners so far.
Another attempt to make evidence available to practitioners is presented by Cartaxo et al. ( 2016 ). They present the concept of “evidence briefings”, which is a way to summarize systematic literature reviews in a one-page format. They used accepted information design principles to design the structure of the one-page briefing. The format and content were positively validated by both practitioners and researchers. While evidence briefings may provide an effective way to synthesize evidence from several studies, our visual abstract template may provide a way to effectively summarize the contribution of one study or research program from a design science perspective.
9 Conclusions and Future Work
Design science, although suggested for some time as a useful research paradigm for software engineering research, is not commonly used as a way to frame software engineering research contributions. Yet our analysis of 38 ICSE distinguished papers indicates that the majority of these papers can be expressed in terms of a design science paradigm. Much software engineering research is solution oriented, providing design knowledge, although it is less clear which problems some papers aim to solve.
The technological rule, as a condensed summary of design knowledge, offers a means to communicate not just the solutions designed or validated, but also the problems addressed. We were able to derive technological rules from most papers, although they were not explicitly stated as such in these papers. In future work, we aim to investigate how technological rules could be linked across different research contributions that address the same underlying problem. A higher level technological rule could be decomposed into more narrow but related rules, thus bringing insights across multiple papers that are linked by context, intervention type and effect. Currently, we lack the machinery in our community to link papers at this theoretical level and the results in papers remain as silos and are often not even referenced in related work. The technological rule template could help fill this gap and help us to better understand what we know and what we don’t know yet about certain problems and challenges in software engineering.
Also as future work, we wish to investigate if the design science visual abstract (or some variant of it) could provide an efficient way to present software engineering research contributions to industry. We published our abstracts from this study online (at http://dsse.org ) but it remains to be seen if industry finds this format useful or not. We expect that extracting technological rules from a set of papers that address a common problem or topic is likely to be of more value to industry (but this was not a goal of this current work). In the meantime, we anticipate that our analysis of ICSE distinguished papers through the design science lens may help our community increase adoption of the design science lens, which we anticipate in turn will allow us to do a better job of communicating, understanding and building on each others’ work.
Furthermore, as a means for spreading the word of this research to the community, it is our intention to contact editors of important journals as well as program chairs of relevant conferences such as ICSE and promote the adoption of VAs for authors that submit a research paper.
http://springer.com/journal/163 Research in Engineering Design
See e.g. https://2018.fseconference.org/track/rosefest-2018
https://www.sigsoft.org/awards/distinguishedPaperAward.html
http://dsse.org
http://www.scs.ryerson.ca/eseiw2017/ISERN/index.html
https://dl.acm.org/citation.cfm?id=3149485.3149522
https://github.com/margaretstorey/cbsoft2019tutorial
Beecham S, O’Leary P, Baker S, Richardson I, Noll J (2014) Making software engineering research relevant. Computer 47(4):80–83. https://doi.org/10.1109/MC.2014.92
Article Google Scholar
Budgen D, Kitchenham BA, Charters SM, Turner M, Brereton P, Linkman SG (2008) Presenting software engineering results using structured abstracts: a randomised experiment. Empir Softw Eng 13 (4):435–468. https://doi.org/10.1007/s10664-008-9075-7
Cartaxo B, Pinto G, Vieira E, Soares S (2016) Evidence briefings: Towards a medium to transfer knowledge from systematic reviews to practitioners. In: Proceedings of the 10th ACM/IEEE International Symposium on Empirical Software Engineering and Measurement, ESEM ’16, pp 57:1–57:10
Carver JC, Juristo N, Baldassarre MT, Vegas S (2014) Replications of software engineering experiments. Empir Softw Eng 19 (2):267–276. https://doi.org/10.1007/s10664-013-9290-8
Deng Q, Ji S (2018) A review of design science research in information systems: Concept, process, outcome, and evaluation. Pacific Asia journal of the association for information systems, vol 10
Deng Q, Wang Y, Ji S (2017) Design science research in information systems: A systematic literature review 2001-2015. In: CONF-IRM 2017 Proceedings
Dybå T, Sjøberg D, Cruzes DS (2012) What works for whom, where, when, and why? On the role of context in empirical software engineering. In: Proceedings of the 2012 ACM-IEEE international symposium on empirical software engineering and measurement, pp 19–28. https://doi.org/10.1145/2372251.2372256
Gregor S, Hevner AR (2013) Positioning and presenting design science research for maximum impact. MIS Q 37(2):337–356
Grigoleit F, Vetro A, Diebold P, Fernandez DM, Bohm W (2015) In quest for proper mediums for technology transfer in software engineering. In: 2015 ACM/IEEE International Symposium on Empirical Software Engineering and Measurement (ESEM), pp 1–4. https://doi.org/10.1109/ESEM.2015.7321203
Hannay JE, Sjöberg DIK, Dybå T (2007) A systematic review of theory use in software engineering experiments. IEEE Trans Softw Eng 33(2):87–107
Hevner AR, March ST, Park J, Ram S (2004) Design science in information systems research. MIS Q 28(1):75–105
Indulska M, Recker JC (2008) Design science in IS research : a literature analysis. In: Gregor S, Ho S (eds) 4th Biennial ANU workshop on information systems foundations. ANU E Press, Canberra
Johannesson P, Perjons E (2014) An introduction to design science. Springer Publishing Company, Incorporated
Juristo N, Gómez OS (2010) Replication of software engineering experiments. In: Empirical software engineering and verification. Springer, pp 60–88
Petersen K, Feldt R, Mujtaba S, Mattsson M (2008) Systematic mapping studies in software engineering. In: Proceedings of the 12th International Conference on Evaluation and Assessment in Software Engineering, EASE’08. BCS Learning & Development Ltd., Swindon, pp 68–77
Rainer A, Hall T, Baddoo N (2003) Persuading developers to “buy into” software process improvement: a local opinion and empirical evidence. In: International Symposium on Empirical Software Engineering, ISESE, pp 326–335
Sedlmair M, Meyer M, Munzner T (2012) Design study methodology: Reflections from the trenches and the stacks. IEEE Trans Vis Comput Graph 18(12):2431–2440. https://doi.org/10.1109/TVCG.2012.213
Sein MK, Henfridsson O, Purao S, Rossi M, Lindgren R (2011) Action design research. MIS Q 35(1):37–56
Shneiderman B (2016) The new ABCs of research: achieving breakthrough collaborations, 1st edn. Oxford University Press, Inc., New York
Book Google Scholar
Shull FJ, Carver JC, Vegas S, Juristo N (2008) The role of replications in empirical software engineering. Empir Softw Eng 13(2):211–218. https://doi.org/10.1007/s10664-008-9060-1
Sjøberg DI, Dybå T, Anda BC, Hannay JE (2008) Building theories in software engineering. In: Guide to advanced empirical software engineering. Springer, pp 312–336
Stol KJ, Fitzgerald B (2013) Uncovering theories in software engineering. In: 2013 2nd SEMAT Workshop on a General Theory of Software Engineering (GTSE), pp 5–14. https://doi.org/10.1109/GTSE.2013.6613863
Stol KJ, Fitzgerald B (2015) Theory-oriented software engineering. Towards general theories of software engineering, vol 101, pp 79–98, DOI https://doi.org/10.1016/j.scico.2014.11.010
Storey MA, Engström E, Höst M, Runeson P, Bjarnason E (2017) Using a visual abstract as a lens for communicating and promoting design science research in software engineering. In: Empirical Software Engineering and Measurement (ESEM), pp 181–186. https://doi.org/10.1109/ESEM.2017.28
van Aken JE (2004) Management research based on the paradigm of the design sciences: the quest for field-tested and grounded technological rules: paradigm of the design sciences. J Manag Stud 41(2):219–246. https://doi.org/10.1111/j.1467-6486.2004.00430.x
van Aken JE (2005) Management research as a design science: articulating the research products of mode 2 knowledge production in management. Br J Manag 16 (1):19–36. https://doi.org/10.1111/j.1467-8551.2005.00437.x
Wieringa R (2009) Design science as nested problem solving. In: Proceedings of the 4th International Conference on Design Science Research in Information Systems and Technology, DESRIST ’09. ACM, New York, pp 8:1–8:12, DOI https://doi.org/10.1145/1555619.1555630 , (to appear in print)
Wieringa R, Daneva M, Condori-Fernandez N (2011) The structure of design theories, and an analysis of their use in software engineering experiments. In: 2011 International symposium on empirical software engineering and measurement, pp 295–304
Wieringa R, Moralı A (2012) Technical action research as a validation method in information systems design science. In: Peffers K, Rothenberger M, Kuechler B (eds) Design science research in information systems. Advances in theory and practice. Springer, Berlin, pp 220–238
Wieringa RJ (2014) Design science methodology for information systems and software engineering. Springer, Berlin
Wohlin C, Aurum A (2015) Towards a decision-making structure for selecting a research design in empirical software engineering. Empir Softw Eng 20(6):1427–1455. https://doi.org/10.1007/s10664-014-9319-7
References to ICSE distinguished papers
Alimadadi S, Sequeira S, Mesbah A, Pattabiraman K (2014) Understanding javascript event-based interactions. In: Proceedings of the 36th International Conference on Software Engineering, ICSE 2014. ACM, New York, pp 367–377, DOI https://doi.org/10.1145/2568225.2568268 , (to appear in print)
Avgerinos T, Rebert A, Cha SK, Brumley D (2014) Enhancing symbolic execution with veritesting. In: Proceedings of the 36th International Conference on Software Engineering, ICSE 2014. ACM, New York, pp 1083–1094, DOI https://doi.org/10.1145/2568225.2568293 , (to appear in print)
Bell J, Kaiser G (2014) Unit test virtualization with vmvm. In: Proceedings of the 36th International Conference on Software Engineering, ICSE 2014. ACM, New York, pp 550–561, DOI https://doi.org/10.1145/2568225.2568248 , (to appear in print)
Bersani MM, Bianculli D, Ghezzi C, Krstić S, Pietro PS (2016) Efficient large-scale trace checking using mapreduce. In: Proceedings of the 38th International Conference on Software Engineering, ICSE ’16. ACM, New York, pp 888–898, DOI https://doi.org/10.1145/2884781.2884832 , (to appear in print)
Cacho N, César T, Filipe T, Soares E, Cassio A, Souza R, Garcia I, Barbosa EA, Garcia A (2014) Trading robustness for maintainability: An empirical study of evolving c# programs. In: Proceedings of the 36th International Conference on Software Engineering, ICSE 2014. ACM, New York, pp 584–595, DOI https://doi.org/10.1145/2568225.2568308 , (to appear in print)
Christakis M, Müller P, Wüstholz V (2016) Guiding dynamic symbolic execution toward unverified program executions. In: Proceedings of the 38th International Conference on Software Engineering, ICSE ’16. ACM, New York, pp 144–155, DOI https://doi.org/10.1145/2884781.2884843 , (to appear in print)
Fan L, Su T, Chen S, Meng G, Liu Y, Xu L, Pu G, Su Z (2018) Large-scale analysis of framework-specific exceptions in android apps. In: Proceedings of the 40th International Conference on Software Engineering, ICSE ’18. ACM, New York, pp 408–419, DOI https://doi.org/10.1145/3180155.3180222 , (to appear in print)
Floyd B, Santander T, Weimer W (2017) Decoding the representation of code in the brain: An fmri study of code review and expertise. In: Proceedings of the 39th international conference on software engineering. IEEE Press, pp 175–186
Gousios G, Storey MA, Bacchelli A (2016) Work practices and challenges in pull-based development: The contributor’s perspective. In: Proceedings of the 38th International Conference on Software Engineering, ICSE ’16. ACM, New York, pp 285–296, DOI https://doi.org/10.1145/2884781.2884826 , (to appear in print)
Hasan S, King Z, Hafiz M, Sayagh M, Adams B, Hindle A (2016) Energy profiles of java collections classes. In: Proceedings of the 38th International Conference on Software Engineering, ICSE ’16. ACM, New York, pp 225–236, DOI https://doi.org/10.1145/2884781.2884869 , (to appear in print)
Hoda R, Noble J (2017) Becoming agile: a grounded theory of agile transitions in practice. In: Proceedings of the 39th International Conference on Software Engineering. IEEE Press, pp 141–151
Inozemtseva L, Holmes R (2014) Coverage is not strongly correlated with test suite effectiveness. In: Proceedings of the 36th International Conference on Software Engineering, ICSE 2014. ACM, New York, pp 435–445, DOI https://doi.org/10.1145/2568225.2568271 , (to appear in print)
Landman D, Serebrenik A, Vinju JJ (2017) Challenges for static analysis of java reflection: Literature review and empirical study. In: Proceedings of the 39th International Conference on Software Engineering, ICSE ’17. IEEE Press, Piscataway, pp 507–518, DOI https://doi.org/10.1109/ICSE.2017.53 , (to appear in print)
Lavallée M, Robillard PN (2015) Why good developers write bad code: An observational case study of the impacts of organizational factors on software quality. In: Proceedings of the 37th International Conference on Software Engineering - Volume 1, ICSE ’15. IEEE Press, Piscataway, pp 677–687
Liu Y, Xu C, Cheung SC (2014) Characterizing and detecting performance bugs for smartphone applications. In: Proceedings of the 36th International Conference on Software Engineering, ICSE 2014. ACM, New York, pp 1013–1024, DOI https://doi.org/10.1145/2568225.2568229 , (to appear in print)
Loncaric C, Ernst MD, Torlak E (2018) Generalized data structure synthesis. In: Chaudron M, Crnkovic I, Chechik M, Harman M (eds) Proceedings of the 40th international conference on software engineering, ICSE 2018, Gothenburg, Sweden, May 27 - June 03, 2018. ACM, pp 958–968. https://doi.org/10.1145/3180155.3180211
Madsen M, Tip F, Andreasen E, Sen K, Møller A (2016) Feedback-directed instrumentation for deployed javascript applications. In: Proceedings of the 38th International Conference on Software Engineering, ICSE 2016, Austin, TX, USA, May 14-22, 2016, pp 899–910. https://doi.org/10.1145/2884781.2884846
Menendez D, Nagarakatte S (2016) Termination-checking for llvm peephole optimizations. In: Proceedings of the 38th International Conference on Software Engineering, ICSE ’16. ACM, New York, pp 191–202, DOI https://doi.org/10.1145/2884781.2884809 , (to appear in print)
Milicevic A, Near JP, Kang E, Jackson D (2015) Alloy*: A general-purpose higher-order relational constraint solver. In: Proceedings of the 37th international conference on software engineering - Volume 1, ICSE ’15. IEEE Press, Piscataway, pp 609–619
Murphy-Hill E, Zimmermann T, Nagappan N (2014) Cowboys, ankle sprains, and keepers of quality: How is video game development different from software development?. In: Proceedings of the 36th International Conference on Software Engineering, ICSE 2014. ACM, New York, pp 1–11, DOI https://doi.org/10.1145/2568225.2568226 , (to appear in print)
Nistor A, Chang PC, Radoi C, Lu S (2015) Caramel: Detecting and fixing performance problems that have non-intrusive fixes. In: Proceedings of the 37th international conference on software engineering - Volume 1, ICSE ’15. IEEE Press, Piscataway, pp 902–912
Okur S, Hartveld DL, Dig D, Deursen AV (2014) A study and toolkit for asynchronous programming in c#. In: Proceedings of the 36th international conference on software engineering, ICSE 2014. ACM, New York, pp 1117–1127, DOI https://doi.org/10.1145/2568225.2568309 , (to appear in print)
Rath M, Rendall J, Guo JLC, Cleland-Huang J, Mäder P. (2018) Traceability in the wild: Automatically augmenting incomplete trace links. In: Proceedings of the 40th international conference on software engineering, ICSE ’18. ACM, New York, pp 834–845, DOI https://doi.org/10.1145/3180155.3180207 , (to appear in print)
Ren Z, Jiang H, Xuan J, Yang Z (2018) Automated localization for unreproducible builds. In: Chaudron M, Crnkovic I, Chechik M, Harman M (eds) Proceedings of the 40th International Conference on Software Engineering, ICSE 2018, Gothenburg, Sweden, May 27 - June 03, 2018. ACM, pp 71–81. https://doi.org/10.1145/3180155.3180224
Rizzi EF, Elbaum S, Dwyer MB (2016) On the techniques we create, the tools we build, and their misalignments: a study of klee. In: Proceedings of the 38th international conference on software engineering. ACM, pp 132–143
Rodeghero P, McMillan C, McBurney PW, Bosch N, D’Mello SK (2014) Improving automated source code summarization via an eye-tracking study of programmers. In: 36th International Conference on Software Engineering, ICSE ’14, Hyderabad, India - May 31 - June 07, 2014, pp 390–401. https://doi.org/10.1145/2568225.2568247
Rojas JM, White TD, Clegg BS, Fraser G (2017) Code defenders: crowdsourcing effective tests and subtle mutants with a mutation testing game. In: Proceedings of the 39th International Conference on Software Engineering, ICSE 2017, Buenos Aires, Argentina, May 20-28, 2017, pp 677–688. https://doi.org/10.1109/ICSE.2017.68
Shi A, Thummalapenta S, Lahiri SK, Bjorner N, Czerwonka J (2017) Optimizing test placement for module-level regression testing. In: Proceedings of the 39th International Conference on Software Engineering, ICSE ’17. IEEE Press, Piscataway, pp 689–699, DOI https://doi.org/10.1109/ICSE.2017.69 , (to appear in print)
Siegmund J, Siegmund N, Apel S (2015) Views on internal and external validity in empirical software engineering. In: Proceedings of the 37th international conference on software engineering-Volume 1. IEEE Press, pp 9–19
Sousa L, Oliveira A, Oizumi W, Barbosa S, Garcia A, Lee J, Kalinowski M, de Mello R, Fonseca B, Oliveira R, et al. (2018) Identifying design problems in the source code: a grounded theory. In: Proceedings of the 40th International Conference on Software Engineering. ACM, pp 921–931
van Tonder R, Goues CL (2018) Static automated program repair for heap properties. In: Proceedings of the 40th International Conference on Software Engineering, ICSE ’18. ACM, New York, pp 151–162, DOI https://doi.org/10.1145/3180155.3180250 , (to appear in print)
Tsantalis N, Mazinanian D, Rostami S (2017) Clone refactoring with lambda expressions. In: Proceedings of the 39th International Conference on Software Engineering, ICSE ’17. IEEE Press, Piscataway, pp 60–70, DOI https://doi.org/10.1109/ICSE.2017.14 , (to appear in print)
Tufano M, Palomba F, Bavota G, Oliveto R, Di Penta M, De Lucia A, Poshyvanyk D (2015) When and why your code starts to smell bad. In: Proceedings of the 37th International Conference on Software Engineering - Volume 1, ICSE ’15. IEEE Press, Piscataway, pp 403–414
Wang X, Sun J, Chen Z, Zhang P, Wang J, Lin Y (2018) Towards optimal concolic testing. In: Proceedings of the 40th International Conference on Software Engineering, ICSE ’18. ACM, New York, pp 291–302, DOI https://doi.org/10.1145/3180155.3180177 , (to appear in print)
Waterman M, Noble J, Allan G (2015) How much up-front?: A grounded theory of agile architecture. In: Proceedings of the 37th International Conference on Software Engineering - Volume 1, ICSE ’15. IEEE Press, Piscataway, pp 347–357
Yan H, Sui Y, Chen S, Xue J (2018) Spatio-temporal context reduction: a pointer-analysis-based static approach for detecting use-after-free vulnerabilities. In: Chaudron M, Crnkovic I, Chechik M, Harman M (eds) Proceedings of the 40th International Conference on Software Engineering, ICSE 2018, Gothenburg, Sweden, May 27 - June 03, 2018. ACM, pp 327–337. https://doi.org/10.1145/3180155.3180178
Ye X, Shen H, Ma X, Bunescu R, Liu C (2016) From word embeddings to document similarities for improved information retrieval in software engineering. In: Proceedings of the 38th International Conference on Software Engineering, ICSE ’16. ACM, New York, pp 404–415, DOI https://doi.org/10.1145/2884781.2884862 , (to appear in print)
Yu T, Qu X, Cohen MB (2016) Vdtest: an automated framework to support testing for virtual devices. In: Proceedings of the 38th International Conference on Software Engineering, ICSE 2016, Austin, TX, USA, May 14-22, 2016, pp 583–594. https://doi.org/10.1145/2884781.2884866
Download references
Acknowledgements
We would like to thank Cassandra Petrachenko for her careful edits of our paper. Daniela Soares Cruzes, Johan Linåker, Sergio Rico and Eirini Kalliamvakou gave us helpful comments on an earlier draft of this paper. We would also like to thank some of the authors of ICSE distinguished papers for giving us feedback, as well as participants at ISERN 2017, RET 2017, and CBSoft 2019 for trying out the visual abstract. Marco Gerosa also gave us valuable insights on the visual abstract and how we applied it. We thank the anonymous EMSE reviewers for helping us sharpening the contribution of this paper. The research was partially funded by the Faculty of Engineering at Lund University through the Lise Meitner guest professorship (Storey), the ELLIIT strategic research area (Engström), and the EASE industrial excellence center (Runeson).
Open access funding provided by Lund University.
Author information
Authors and affiliations.
Lund University, Lund, Sweden
Emelie Engström, Per Runeson & Martin Höst
University of Victoria, Victoria, BC, Canada
Margaret-Anne Storey
University of Bari, Bari, Italy
Maria Teresa Baldassarre
You can also search for this author in PubMed Google Scholar
Corresponding author
Correspondence to Emelie Engström .
Additional information
Communicated by: Sven Apel
Publisher’s note
Springer Nature remains neutral with regard to jurisdictional claims in published maps and institutional affiliations.
Rights and permissions
Open Access This article is licensed under a Creative Commons Attribution 4.0 International License, which permits use, sharing, adaptation, distribution and reproduction in any medium or format, as long as you give appropriate credit to the original author(s) and the source, provide a link to the Creative Commons licence, and indicate if changes were made. The images or other third party material in this article are included in the article's Creative Commons licence, unless indicated otherwise in a credit line to the material. If material is not included in the article's Creative Commons licence and your intended use is not permitted by statutory regulation or exceeds the permitted use, you will need to obtain permission directly from the copyright holder. To view a copy of this licence, visit http://creativecommons.org/licenses/by/4.0/ .
Reprints and permissions
About this article
Engström, E., Storey, MA., Runeson, P. et al. How software engineering research aligns with design science: a review. Empir Software Eng 25 , 2630–2660 (2020). https://doi.org/10.1007/s10664-020-09818-7
Download citation
Published : 18 April 2020
Issue Date : July 2020
DOI : https://doi.org/10.1007/s10664-020-09818-7
Share this article
Anyone you share the following link with will be able to read this content:
Sorry, a shareable link is not currently available for this article.
Provided by the Springer Nature SharedIt content-sharing initiative
- Design science
- Research review
- Empirical software engineering
- Find a journal
- Publish with us
- Track your research
Help | Advanced Search
Computer Science > Human-Computer Interaction
Title: a design space for intelligent and interactive writing assistants.
Abstract: In our era of rapid technological advancement, the research landscape for writing assistants has become increasingly fragmented across various research communities. We seek to address this challenge by proposing a design space as a structured way to examine and explore the multidimensional space of intelligent and interactive writing assistants. Through a large community collaboration, we explore five aspects of writing assistants: task, user, technology, interaction, and ecosystem. Within each aspect, we define dimensions (i.e., fundamental components of an aspect) and codes (i.e., potential options for each dimension) by systematically reviewing 115 papers. Our design space aims to offer researchers and designers a practical tool to navigate, comprehend, and compare the various possibilities of writing assistants, and aid in the envisioning and design of new writing assistants.
Submission history
Access paper:.
- Download PDF
- Other Formats

References & Citations
- Google Scholar
- Semantic Scholar
BibTeX formatted citation

Bibliographic and Citation Tools
Code, data and media associated with this article, recommenders and search tools.
- Institution
arXivLabs: experimental projects with community collaborators
arXivLabs is a framework that allows collaborators to develop and share new arXiv features directly on our website.
Both individuals and organizations that work with arXivLabs have embraced and accepted our values of openness, community, excellence, and user data privacy. arXiv is committed to these values and only works with partners that adhere to them.
Have an idea for a project that will add value for arXiv's community? Learn more about arXivLabs .
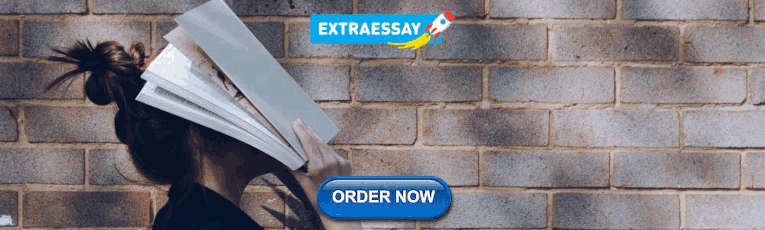
IMAGES
VIDEO
COMMENTS
Design Science Research (DSR) is a problem-solving paradigm that seeks to enhance human knowledge via the creation of innovative artifacts. ... and Indirect Effects of Design Artifacts.. Paper pre ...
Design science research is a qualitative research approach in which the object of study is the design process, i.e. it simultaneously generates knowledge about the method used to design an artefact and the design or the artefact itself. ... This paper uses techniques from design science research to analyse the method used when deriving the ...
Abstract. Design Science Research (DSR) is a problem-solving paradigm that seeks to enhance human knowledge via the creation of innovative artifacts. Simply stated, DSR seeks to enhance technology and science knowledge bases via the creation of innovative artifacts that solve problems and improve the environment in which they are instantiated ...
The Design Science journal is a designed product, maybe also a designed product-service system. After all the insights, experiences, data collections and scientific analyses have played their role, bringing a design into existence remains an act of faith. This journal is no exception. It is a collective act of faith by a large number of people ...
Presented research on Service Design Patterns with SDA is primarily informed and guided by a Design Science Research (DSR) approach oriented towards requirements discussed in (Hevner et al. 2004 ...
The proposed method framework for design science research includes five main activities that range from problem investigation and requirements definition, through artefact design and development, to demonstration and evaluation. A design science project needs to make use of rigorous research methods.
The integration of brain imaging tools and methods into Design Science research has opened novel avenues of assessing and understanding design cognition, i.e., the underlying, implicit thought processes involved in design and engineering (Hay et al., 2020).The advances in technology (e.g., portability of neuroimaging tools) and methodology (e.g., standardized analysis techniques) have made ...
Abstract. Design science is a research paradigm centered on the creation of innovative, first-of-a-kind information systems artifacts. Researchers in design science are still exploring developing ...
The Design Science Research (DSR) paradigm is highly relevant to the Information Systems (IS) discipline because DSR aims to improve the state of practice and contribute design knowledge through the systematic construction of useful artefacts. ... Secondly, we screened the identified set of papers and extracted 72 papers that used DSR as a ...
The design science research methodology (DSRM) emphasises the design and construction of applicable artefacts, such as systems, applications, methods, and others, ... Consequently, a research paper that seeks to develop an IS design theory (genre: ISDT) would not necessarily be expected to develop a fully operational information technology ...
2. Artifacts in design science research — the current status. A general understanding of artifact in Design Science Research is that it is an object made by humans with the intention that it is used to address a practical problem [8], [9].DSR arose in the 1990's within the field of Information Systems and although its idea of artifacts included physical artifacts like hammers or medical ...
Design science research has been defined as "research that invents a new purposeful artefact to address a generalised type of problem and evaluates its utility for solving problems of that type" (Venable and Baskerville, 2012, p. 142). Since the focus is on solutions, research in design science is oriented toward solving problems, while in ...
new methodology, a potential artifact in design research. Of the 15 articles, just two could arguably be considered DS research (see Appendix I for details). Given that many software engineering or computer science papers take such a design science approach (Morrison et al. 1995), we wonder why it shouldn't be happening in IS.
To conduct design science research (DSR) it is expected that some form of process model must be used, where each stage is explicitly outlined in the presentation of the research, with clear explanations. Since very few, if any, papers actually produces and presents DSR in such a manner, this provides an excellent opportunity to do so.
Design Science Research (DSR) is a popular new research approach and paradigm, for which a number of research methodologies have been developed. One of the challenges facing researchers wanting to apply this new approach is the choice of research methodology. In this paper we give an account of six
This paper provides an overview of DSR and tries to combine both, rigor and relevance, in a unified perception. Due to the significant increase of theoretical evaluation of software prototypes, design science research (DSR) as a new research direction has emerged in recent years with the aim to ensure for both, rigor and relevance in prototyping research projects.
Hevner's areas of research interest include design-science research, information-systems development, software engineering, distributed-database systems, healthcare systems and Internet of Things computing. He has published more than 200 research papers on these topics and has consulted for a number of Fortune 500 companies.
The authors design and demonstrate a process for carrying out design science (DS) research in information systems and demonstrate use of the process to conduct research in two case studies. Several IS researchers have pioneered the acceptance of DS research in IS, but in the last 15 years little DS research has been done within the discipline. The lack of a generally accepted process for DS ...
Design science research (DSR) is well-known in different domains, including information systems (IS), for the construction of artefacts. One of the most challenging aspects of IS postgraduate studies (with DSR) is determining the structure of the study and its report, which should reflect all the components necessary to build a convincing argument in support of such a study's claims or ...
In this paper, we present the first steps of our design science research project on principles for a value-sensitive design of CAs. Based on theoretical insights from 87 papers and eleven user interviews, we propose preliminary requirements and design principles for a value-sensitive design of CAs.
There is a maze of hard problems that have been suggested to profit from quantum acceleration: from cryptanalysis, chemistry and materials science, to optimization, big data, machine learning, database search, drug design and protein folding, fluid dynamics and weather prediction.
The comprehensive data and analysis from the PSFC's magnet test, as detailed in the six new papers, has demonstrated that plans for a new generation of fusion devices — the one designed by MIT and CFS, as well as similar designs by other commercial fusion companies — are built on a solid foundation in science. The superconducting breakthrough
The conclusion of a design science research paper is typically that an artifact is appropriate for solving a specific problem under specific conditions. Critical reflection of your design science research project is key. In design science research you will have to make even more decisions than in other research designs. Just look at all the ...
Various catalyst active site design strategies to increase activity and stability have recently been shown, and we refer to several review papers in this area for a more detailed summary ... R.A. acknowledges support from the National Science Foundation Graduate Research Fellowship under grant no. DGE 1256260. The authors acknowledge the ...
Leitfaden für die Nutzung von Design Science Research in Abschlussarbeiten. November 2020. Report number: IUBH Discussion Papers - IT & Engineering - No. 2/2020. Affiliation: IUBH ...
Design science is a paradigm for conducting and communicating applied research such as software engineering. The goal of design science research is to produce prescriptive knowledge for professionals in a discipline and to share empirical insights gained from investigations of the prescriptions applied in context (van Aken 2004).Such knowledge is referred to as "design knowledge" as it ...
Abstract: Prior to being transformed into useful items, plastic trash is first shredded into little pieces using a plastic shredder machine. The shredder machine that is currently on the market has a concept design that is quite similar. The performance of the shredding machine is mostly dependent on the shaft and blades. It was discovered that the geometry and orientation of the blades ...
In our era of rapid technological advancement, the research landscape for writing assistants has become increasingly fragmented across various research communities. We seek to address this challenge by proposing a design space as a structured way to examine and explore the multidimensional space of intelligent and interactive writing assistants. Through a large community collaboration, we ...