- Open access
- Published: 07 February 2022
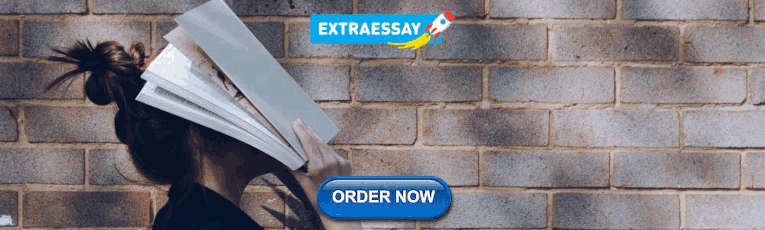
Cryptocurrency trading: a comprehensive survey
- Fan Fang 1 , 2 ,
- Carmine Ventre 1 ,
- Michail Basios 2 ,
- Leslie Kanthan 2 ,
- David Martinez-Rego 2 ,
- Fan Wu 2 &
- Lingbo Li ORCID: orcid.org/0000-0002-3073-1352 2
Financial Innovation volume 8 , Article number: 13 ( 2022 ) Cite this article
84k Accesses
149 Citations
19 Altmetric
Metrics details
In recent years, the tendency of the number of financial institutions to include cryptocurrencies in their portfolios has accelerated. Cryptocurrencies are the first pure digital assets to be included by asset managers. Although they have some commonalities with more traditional assets, they have their own separate nature and their behaviour as an asset is still in the process of being understood. It is therefore important to summarise existing research papers and results on cryptocurrency trading, including available trading platforms, trading signals, trading strategy research and risk management. This paper provides a comprehensive survey of cryptocurrency trading research, by covering 146 research papers on various aspects of cryptocurrency trading ( e . g ., cryptocurrency trading systems, bubble and extreme condition, prediction of volatility and return, crypto-assets portfolio construction and crypto-assets, technical trading and others). This paper also analyses datasets, research trends and distribution among research objects (contents/properties) and technologies, concluding with some promising opportunities that remain open in cryptocurrency trading.
Introduction
Cryptocurrencies have experienced broad market acceptance and fast development despite their recent conception. Many hedge funds and asset managers have begun to include cryptocurrency-related assets into their portfolios and trading strategies. The academic community has similarly spent considerable efforts in researching cryptocurrency trading. This paper seeks to provide a comprehensive survey of the research on cryptocurrency trading, by which we mean any study aimed at facilitating and building strategies to trade cryptocurrencies.
As an emerging market and research direction, cryptocurrencies and cryptocurrency trading have seen considerable progress and a notable upturn in interest and activity (Farell 2015 ). From Fig. 1 , we observe over 85% of papers have appeared since 2018, demonstrating the emergence of cryptocurrency trading as a new research area in financial trading. The sampling interval of this survey is from 2013 to June 2021.
The literature is organised according to six distinct aspects of cryptocurrency trading:
Cryptocurrency trading software systems (i.e., real-time trading systems, turtle trading systems, arbitrage trading systems);
Systematic trading including technical analysis, pairs trading and other systematic trading methods;
Emergent trading technologies including econometric methods, machine learning technology and other emergent trading methods;
Portfolio and cryptocurrency assets including research among cryptocurrency co-movements and crypto-asset portfolio research;
Market condition research including bubbles (Flood et al. 1986 ) or crash analysis and extreme conditions;
Other Miscellaneous cryptocurrency trading research.
In this survey we aim at compiling the most relevant research in these areas and extract a set of descriptive indicators that can give an idea of the level of maturity research in this area has achieved.
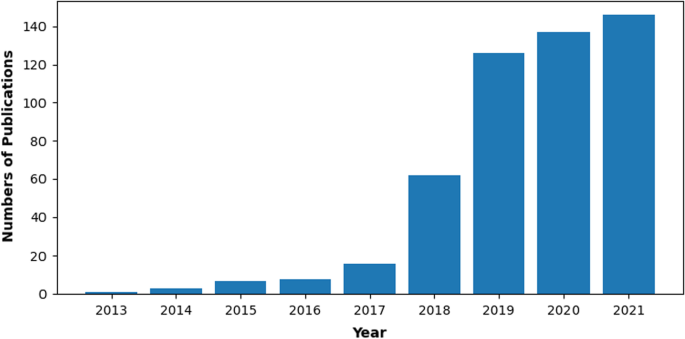
Cryptocurrency Trading Publications (cumulative) during 2013–2021(June 2021)
We also summarise research distribution (among research properties and categories/research technologies). The distribution among properties defines the classification of research objectives and content. The distribution among technologies defines the classification of methods or technological approaches to the study of cryptocurrency trading. Specifically, we subdivide research distribution among categories/technologies into statistical methods and machine learning technologies. Moreover, We identify datasets and opportunities (potential research directions) that have appeared in the cryptocurrency trading area. To ensure that our survey is self-contained, we aim to provide sufficient material to adequately guide financial trading researchers who are interested in cryptocurrency trading.
There has been related work that discussed or partially surveyed the literature related to cryptocurrency trading. Kyriazis ( 2019 ) investigated the efficiency and profitable trading opportunities in the cryptocurrency market. Ahamad et al. ( 2013 ) and Sharma et al. ( 2017 ) gave a brief survey on cryptocurrencies, merits of cryptocurrencies compared to fiat currencies and compared different cryptocurrencies that are proposed in the literature. Mukhopadhyay et al. ( 2016 ) gave a brief survey of cryptocurrency systems. Merediz-Solà and Bariviera ( 2019 ) performed a bibliometric analysis of bitcoin literature. The outcomes of this related work focused on specific area in cryptocurrency, including cryptocurrencies and cryptocurrency market introduction, cryptocurrency systems / platforms, bitcoin literature review, etc. To the best of our knowledge, no previous work has provided a comprehensive survey particularly focused on cryptocurrency trading.
In summary, the paper makes the following contributions:
Definition This paper defines cryptocurrency trading and categorises it into: cryptocurrency markets, cryptocurrency trading models, and cryptocurrency trading strategies. The core content of this survey is trading strategies for cryptocurrencies while we cover all aspects of it.
Multidisciplinary survey The paper provides a comprehensive survey of 146 cryptocurrency trading papers, across different academic disciplines such as finance and economics, artificial intelligence and computer science. Some papers may cover multiple aspects and will be surveyed for each category.
Analysis The paper analyses the research distribution, datasets and trends that characterise the cryptocurrency trading literature.
Horizons The paper identifies challenges, promising research directions in cryptocurrency trading, aimed to promote and facilitate further research.
Figure 2 depicts the paper structure, which is informed by the review schema adopted. More details about this can be found in " Paper collection and review schema " section.
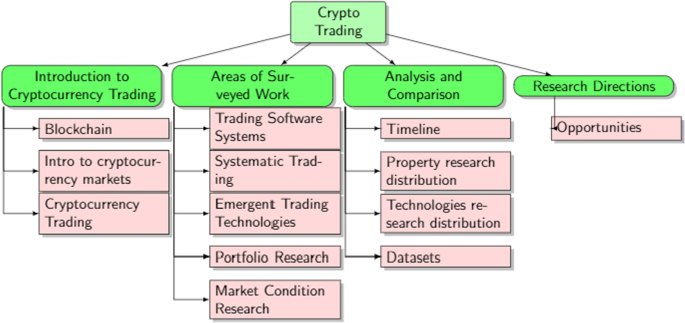
Tree structure of the contents in this paper
Cryptocurrency trading
This section provides an introduction to cryptocurrency trading. We will discuss Blockchain , as the enabling technology, cryptocurrency markets and cryptocurrency trading strategies .
Blockchain technology introduction
Blockchain is a digital ledger of economic transactions that can be used to record not just financial transactions, but any object with an intrinsic value (Tapscott and Tapscott 2016 ). In its simplest form, a Blockchain is a series of immutable data records with timestamps, which are managed by a cluster of machines that do not belong to any single entity. Each of these data block s is protected by cryptographic principle and bound to each other in a chain (cf. Fig. 3 for the workflow).
Cryptocurrencies like Bitcoin are conducted on a peer-to-peer network structure. Each peer has a complete history of all transactions, thus recording the balance of each account. For example, a transaction is a file that says “A pays X Bitcoins to B” that is signed by A using its private key. This is basic public-key cryptography, but also the building block on which cryptocurrencies are based. After being signed, the transaction is broadcast on the network. When a peer discovers a new transaction, it checks to make sure that the signature is valid (this is equivalent to using the signer’s public key, denoted as the algorithm in Fig. 3 ). If the verification is valid then the block is added to the chain; all other blocks added after it will “confirm” that transaction. For example, if a transaction is contained in block 502 and the length of the blockchain is 507 blocks, it means that the transaction has 5 confirmations (507–502) (Johar 2018 ).

Workflow of Blockchain transaction
From Blockchain to cryptocurrencies
Confirmation is a critical concept in cryptocurrencies; only miners can confirm transactions. Miners add blocks to the Blockchain; they retrieve transactions in the previous block and combine it with the hash of the preceding block to obtain its hash, and then store the derived hash into the current block. Miners in Blockchain accept transactions, mark them as legitimate and broadcast them across the network. After the miner confirms the transaction, each node must add it to its database. In layman terms, it has become part of the Blockchain and miners undertake this work to obtain cryptocurrency tokens, such as Bitcoin. In contrast to Blockchain, cryptocurrencies are related to the use of tokens based on distributed ledger technology. Any transaction involving purchase, sale, investment, etc. involves a Blockchain native token or sub-token. Blockchain is a platform that drives cryptocurrency and is a technology that acts as a distributed ledger for the network. The network creates a means of transaction and enables the transfer of value and information. Cryptocurrencies are the tokens used in these networks to send value and pay for these transactions. They can be thought of as tools on the Blockchain, and in some cases can also function as resources or utilities. In other instances, they are used to digitise the value of assets. In summary, cryptocurrencies are part of an ecosystem based on Blockchain technology.
Introduction of cryptocurrency market
What is cryptocurrency.
Cryptocurrency is a decentralised medium of exchange which uses cryptographic functions to conduct financial transactions (Doran 2014 ). Cryptocurrencies leverage the Blockchain technology to gain decentralisation, transparency, and immutability (Meunier 2018 ). In the above, we have discussed how Blockchain technology is implemented for cryptocurrencies.
In general, the security of cryptocurrencies is built on cryptography, neither by people nor on trust (Narayanan et al. 2016 ). For example, Bitcoin uses a method called “Elliptic Curve Cryptography” to ensure that transactions involving Bitcoin are secure (Wang et al. 2017 ). Elliptic curve cryptography is a type of public-key cryptography that relies on mathematics to ensure the security of transactions. When someone attempts to circumvent the aforesaid encryption scheme by brute force, it takes them one-tenth the age of the universe to find a value match when trying 250 billion possibilities every second (Grayblock 2018 ). Regarding its use as a currency, cryptocurrency has properties similar to fiat currencies. It has a controlled supply. Most cryptocurrencies limit the availability of their currency volumes. E.g. for Bitcoin, the supply will decrease over time and will reach its final quantity sometime around 2140. All cryptocurrencies control the supply of tokens through a timetable encoded in the Blockchain.
One of the most important features of cryptocurrencies is the exclusion of financial institution intermediaries (Harwick 2016 ). The absence of a “middleman” lowers transaction costs for traders. For comparison, if a bank’s database is hacked or damaged, the bank will rely entirely on its backup to recover any information that is lost or compromised. With cryptocurrencies, even if part of the network is compromised, the rest will continue to be able to verify transactions correctly. Cryptocurrencies also have the important feature of not being controlled by any central authority (Rose 2015 ): the decentralised nature of the Blockchain ensures cryptocurrencies are theoretically immune to government control and interference.
The pure digital asset is anything that exists in a digital format and carries with it the right to use it. Currently, digital assets include digital documents, motion picture and so on; the market for digital assets has in fact evolved since its inception in 2009, with the first digital asset “Bitcoin” (Kaal 2020 ). For this reason, we call the cryptocurrency the “first pure digital asset”.
As of December 20, 2019, there exist 4950 cryptocurrencies and 20,325 cryptocurrency markets; the market cap is around 190 billion dollars (CoinMaketCap 2019 ). Figure 4 shows historical data on global market capitalisation and 24-h trading volume (TradingView 2021 ). The blue line is the total cryptocurrency market capitalization and green/red histogram is the total cryptocurrency market volume. The total market cap is calculated by aggregating the dollar market cap of all cryptocurrencies. From the figure, we can observe how cryptocurrencies experience exponential growth in 2017 and a large bubble burst in early 2018. In the wake of the pandemic, cryptocurrencies raised dramatically in value in 2020. In 2021, the market value of cryptocurrencies has been very volatile but consistently at historically high levels.
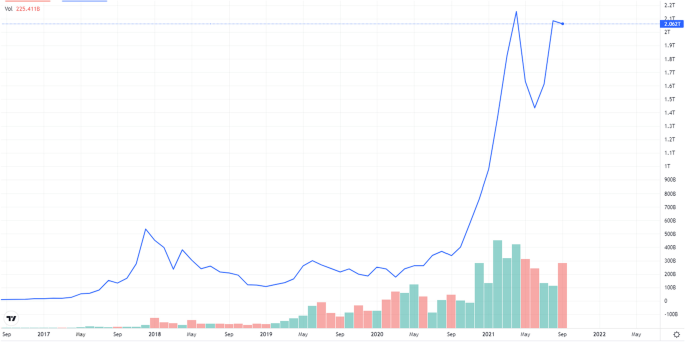
Total market capitalization and volume of cryptocurrency market, USD (TradingView 2021 )
There are three mainstream cryptocurrencies (Council 2021 ): Bitcoin (BTC), Ethereum (ETH), and Litecoin (LTC). Bitcoin was created in 2009 and garnered massive popularity. On October 31, 2008, an individual or group of individuals operating under the pseudonym Satoshi Nakamoto released the Bitcoin white paper and described it as: ”A pure peer-to-peer version of electronic cash that can be sent online for payment from one party to another without going through a counterparty, ie. a financial institution.” (Nakano et al. 2018 ) Launched by Vitalik Buterin in 2015, Ethereum is a special Blockchain with a special token called Ether (ETH symbol in exchanges). A very important feature of Ethereum is the ability to create new tokens on the Ethereum Blockchain. The Ethereum network went live on July 30, 2015, and pre-mined 72 million Ethereum. Litecoin is a peer-to-peer cryptocurrency created by Charlie Lee. It was created according to the Bitcoin protocol, but it uses a different hashing algorithm. Litecoin uses a memory-intensive proof-of-work algorithm, Scrypt.
Figure 5 shows percentages of total cryptocurrency market capitalisation; Bitcoin and Ethereum account for the majority of the total market capitalisation (data collected on 14 September 2021).
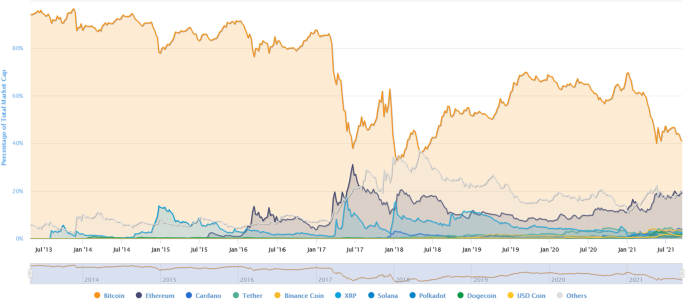
Percentage of Total Market Capitalisation (Coinmarketcap 2020 )
Cryptocurrency exchanges
A cryptocurrency exchange or digital currency exchange (DCE) is a business that allows customers to trade cryptocurrencies. Cryptocurrency exchanges can be market makers, usually using the bid-ask spread as a commission for services, or as a matching platform, by simply charging fees. A cryptocurrency exchange or digital currency exchange (DCE) is a place that allows customers to trade cryptocurrencies. Cryptocurrency exchanges can be market makers (usually using the bid-ask spread as a commission for services) or a matching platform (simply charging fees).
Table 1 shows the top or classical cryptocurrency exchanges according to the rank list, by volume, compiled on “nomics” website (Nomics 2020 ). Chicago Mercantile Exchange (CME), Chicago Board Options Exchange (CBOE) as well as BAKKT (backed by New York Stock Exchange) are regulated cryptocurrency exchanges. Fiat currency data also comes from “nomics” website (Nomics 2020 ). Regulatory authority and supported currencies of listed exchanges are collected from official websites or blogs.
First we give a definition of cryptocurrency trading .
Definition 1
Cryptocurrency trading is the act of buying and selling of cryptocurrencies with the intention of making a profit.
The definition of cryptocurrency trading can be broken down into three aspects: object, operation mode and trading strategy. The object of cryptocurrency trading is the asset being traded, which is “cryptocurrency”. The operation mode of cryptocurrency trading depends on the means of transaction in the cryptocurrency market, which can be classified into “trading of cryptocurrency Contract for Differences (CFD)” (The contract between the two parties, often referred to as the “buyer” and “seller”, stipulates that the buyer will pay the seller the difference between themselves when the position closes (Authority 2019 )) and “buying and selling cryptocurrencies via an exchange”. A trading strategy in cryptocurrency trading, formulated by an investor, is an algorithm that defines a set of predefined rules to buy and sell on cryptocurrency markets.
Advantages of trading cryptocurrency
The benefits of cryptocurrency trading include:
Drastic fluctuations The volatility of cryptocurrencies are often likely to attract speculative interest and investors. The rapid fluctuations of intraday prices can provide traders with great money-earning opportunities, but it also includes more risk.
24-h market The cryptocurrency market is available 24 h a day, 7 days a week because it is a decentralised market. Unlike buying and selling stocks and commodities, the cryptocurrency market is not traded physically from a single location. Cryptocurrency transactions can take place between individuals, in different venues across the world.
Near anonymity Buying goods and services using cryptocurrencies is done online and does not require to make one’s own identity public. With increasing concerns over identity theft and privacy, cryptocurrencies can thus provide users with some advantages regarding privacy.
Different exchanges have specific Know-Your-Customer (KYC) measures for identifying users or customers (Adeyanju 2019 ). The KYC undertook in the exchanges allows financial institutions to reduce the financial risk while maximising the wallet owner’s anonymity.
Peer-to-peer transactions One of the biggest benefits of cryptocurrencies is that they do not involve financial institution intermediaries. As mentioned above, this can reduce transaction costs. Moreover, this feature might appeal to users who distrust traditional systems.
Over-the-counter (OTC) cryptocurrency markets offer, in this context, peer-to-peer transactions on the Blockchain. The most famous cryptocurrency OTC market is “LocalBitcoin (Localbtc 2020 )”.
Programmable “smart” capabilities Some cryptocurrencies can bring other benefits to holders, including limited ownership and voting rights. Cryptocurrencies may also include a partial ownership interest in physical assets such as artwork or real estate.
Disadvantages of trading cryptocurrency
The disadvantages of cryptocurrency trading include:
Scalability problem Before the massive expansion of the technology infrastructure, the number of transactions and the speed of transactions cannot compete with traditional currency trading. Scalability issues led to a multi-day trading backlog in March 2020, affecting traders looking to move cryptocurrencies from their personal wallets to exchanges (Forbes 2021 ).
Cybersecurity issues As a digital technology, cryptocurrencies are subject to cyber security breaches and can fall into the hands of hackers. Recently, over $600 million of ethereum and other cryptocurrencies were stolen in August 2021 in blockchain-based platform Poly Network (Forbes 2021 ). Mitigating this situation requires ongoing maintenance of the security infrastructure and the use of enhanced cyber security measures that go beyond those used in traditional banking (Kou et al. 2021 ).
Regulations Authorities around the world face challenging questions about the nature and regulation of cryptocurrency as some parts of the system and its associated risks are largely unknown. There are currently three types of regulatory systems used to control digital currencies, they include: closed system for the Chinese market, open and liberal for the Swiss market,and open and strict system for the US market (UKTN 2021 ). At the same time, we notice that some countries such as India is not at par in using the cryptocurrency. As Buffett said, “It doesn’t make sense. This thing is not regulated. It’s not under control. It’s not under the supervision of \([\ldots ]\) United States Federal Reserve or any other central bank (Forbes 2017 ).”
Cryptocurrency trading strategy
Cryptocurrency trading strategy is the main focus of this survey. There are many trading strategies, which can be broadly divided into two main categories: technical and fundamental. Technical and fundamental trading are two main trading analysis thoughts when it comes to analyzing the financial markets. Most traders use these two analysis methods or both (Oberlechner 2001 ). From a survey on stock prediction, we in fact know that 66% of the relevant research work was based on technical analysis; while 23% and 11% were based on fundamental analysis and general analysis, respectively (Nti et al. 2020 ). Cryptocurrency trading can draw on the experience of stock market trading in most scenarios. So we divide trading strategies into two main categories: technical and fundamental trading.
They are similar in the sense that they both rely on quantifiable information that can be backtested against historical data to verify their performance. In recent years, a third kind of trading strategy, which we call programmatic trading, has received increasing attention. Such a trading strategy is similar to a technical trading strategy because it uses trading activity information on the exchange to make buying or selling decisions. programmatic traders build trading strategies with quantitative data, which is mainly derived from price, volume, technical indicators or ratios to take advantage of inefficiencies in the market and are executed automatically by trading software. Cryptocurrency market is different from traditional markets as there are more arbitrage opportunities, higher fluctuation and transparency. Due to these characteristics, most traders and analysts prefer using programmatic trading in cryptocurrency markets.
Cryptocurrency trading software system
Software trading systems allow international transactions, process customer accounts and information, and accept and execute transaction orders (Calo and Johnson 2002 ). A cryptocurrency trading system is a set of principles and procedures that are pre-programmed to allow trade between cryptocurrencies and between fiat currencies and cryptocurrencies. Cryptocurrency trading systems are built to overcome price manipulation, cybercriminal activities and transaction delays (Bauriya et al. 2019 ). When developing a cryptocurrency trading system, we must consider the capital market, base asset, investment plan and strategies (Molina 2019 ). Strategies are the most important part of an effective cryptocurrency trading system and they will be introduced below. There exist several cryptocurrency trading systems that are available commercially, for example, Capfolio, 3Commas, CCXT, Freqtrade and Ctubio. From these cryptocurrency trading systems, investors can obtain professional trading strategy support, fairness and transparency from the professional third-party consulting companies and fast customer services.
Systematic trading
Systematic trading is a way to define trading goals, risk controls and rules. In general, systematic trading includes high frequency trading and slower investment types like systematic trend tracking. In this survey, we divide systematic cryptocurrency trading into technical analysis, pairs trading and others. Technical analysis in cryptocurrency trading is the act of using historical patterns of transaction data to assist a trader in assessing current and projecting future market conditions for the purpose of making profitable trades. Price and volume charts summarise all trading activity made by market participants in an exchange and affect their decisions. Some experiments showed that the use of specific technical trading rules allows generating excess returns, which is useful to cryptocurrency traders and investors in making optimal trading and investment decisions (Gerritsen et al. 2019 ). Pairs trading is a systematic trading strategy that considers two similar assets with slightly different spreads. If the spread widens, short the high cryptocurrencies and buy the low cryptocurrencies. When the spread narrows again to a certain equilibrium value, a profit is generated (Elliott et al. 2005 ). Papers shown in this section involve the analysis and comparison of technical indicators, pairs and informed trading, amongst other strategies.
Tools for building automated trading systems
Tools for building automated trading systems in cryptocurrency market are those emergent trading strategies for cryptocurrency. These include strategies that are based on econometrics and machine learning technologies.
Econometrics on cryptocurrency
Econometric methods apply a combination of statistical and economic theories to estimate economic variables and predict their values (Vogelvang 2005 ). Statistical models use mathematical equations to encode information extracted from the data (Kaufman 2013 ). In some cases, statistical modeling techniques can quickly provide sufficiently accurate models (Ben-Akiva et al. 2002 ). Other methods might be used, such as sentiment-based prediction and long-and-short-term volatility classification based prediction (Chang et al. 2015 ). The prediction of volatility can be used to judge the price fluctuation of cryptocurrencies, which is also valuable for the pricing of cryptocurrency-related derivatives (Kat and Heynen 1994 ).
When studying cryptocurrency trading using econometrics, researchers apply statistical models on time-series data like generalised autoregressive conditional heteroskedasticity (GARCH) and BEKK (named after Baba, Engle, Kraft and Kroner, 1995 (Engle and Kroner 1995 )) models to evaluate the fluctuation of cryptocurrencies (Caporin and McAleer 2012 ). A linear statistical model is a method to evaluate the linear relationship between prices and an explanatory variable (Neter et al. 1996 ). When there exists more than one explanatory variable, we can model the linear relationship between explanatory (independent) and response (dependent) variables with multiple linear models. The common linear statistical model used in the time-series analysis is the autoregressive moving average (ARMA) model (Choi 2012 ).
Machine learning technology
Machine learning is an efficient tool for developing Bitcoin and other cryptocurrency trading strategies (McNally et al. 2018 ) because it can infer data relationships that are often not directly observable by humans. From the most basic perspective, Machine Learning relies on the definition of two main components: input features and objective function. The definition of Input Features (data sources) is where knowledge of fundamental and technical analysis comes into play. We may divide the input into several groups of features, for example, those based on Economic indicators (such as, gross domestic product indicator, interest rates, etc.), Social indicators (Google Trends, Twitter, etc.), Technical indicators (price, volume, etc.) and other Seasonal indicators (time of day, day of the week, etc.). The objective function defines the fitness criteria one uses to judge if the Machine Learning model has learnt the task at hand. Typical predictive models try to anticipate numeric (e.g., price) or categorical (e.g., trend) unseen outcomes. The machine learning model is trained by using historic input data (sometimes called in-sample) to generalise patterns therein to unseen (out-of-sample) data to (approximately) achieve the goal defined by the objective function. Clearly, in the case of trading, the goal is to infer trading signals from market indicators which help to anticipate asset future returns.
Generalisation error is a pervasive concern in the application of Machine Learning to real applications, and of utmost importance in Financial applications. We need to use statistical approaches, such as cross validation, to validate the model before we actually use it to make predictions. In machine learning, this is typically called “validation”. The process of using machine learning technology to predict cryptocurrency is shown in Fig. 6 .

Process of machine learning in predicting cryptocurrency
Depending on the formulation of the main learning loop, we can classify Machine Learning approaches into three categories: Supervised learning, Unsupervised learning and Reinforcement learning. We list a general comparison (IntelliPaat 2021 ) among these three machine learning methods in Table 2 . Supervised learning is used to derive a predictive function from labeled training data. Labeled training data means that each training instance includes inputs and expected outputs. Usually, these expected outputs are produced by a supervisor and represent the expected behaviour of the model. The most used labels in trading are derived from in sample future returns of assets. Unsupervised learning tries to infer structure from unlabeled training data and it can be used during exploratory data analysis to discover hidden patterns or to group data according to any pre-defined similarity metrics. Reinforcement learning utilises software agents trained to maximise a utility function, which defines their objective; this is flexible enough to allow agents to exchange short term returns for future ones. In the financial sector, some trading challenges can be expressed as a game in which an agent aims at maximising the return at the end of the period.
The use of machine learning in cryptocurrency trading research encompasses the connection between data sources’ understanding and machine learning model research. Further concrete examples are shown in a later section.
Portfolio research
Portfolio theory advocates diversification of investments to maximize returns for a given level of risk by allocating assets strategically. The celebrated mean-variance optimisation is a prominent example of this approach (Markowitz 1952 ). Generally, crypto asset denotes a digital asset (i.e., cryptocurrencies and derivatives). There are some common ways to build a diversified portfolio in crypto assets. The first method is to diversify across markets, which is to mix a wide variety of investments within a portfolio of the cryptocurrency market. The second method is to consider the industry sector, which is to avoid investing too much money in any one category. Diversified investment of portfolio in the cryptocurrency market includes portfolio across cryptocurrencies (Liu 2019 ) and portfolio across the global market including stocks and futures (Kajtazi and Moro 2019 ).
Market condition research
Market condition research appears especially important for cryptocurrencies. A financial bubble is a significant increase in the price of an asset without changes in its intrinsic value (Brunnermeier and Oehmke 2013 ; Kou et al. 2021 ). Many experts pinpoint a cryptocurrency bubble in 2017 when the prices of cryptocurrencies grew by 900 \(\%\) . In 2018, Bitcoin faced a collapse in its value. This significant fluctuation inspired researchers to study bubbles and extreme conditions in cryptocurrency trading. The cryptocurrency market has experienced a near continuous bull market since the fall of 2020, with the value of Bitcoin soaring from $10,645 on October 7, 2020 to an all-time high of $63,346 on April 15, 2021. This represents a gain of approximately +600% in just six months (Forbes 2021 ). Some experts believe that the extreme volatility of exchange rates means that cryptocurrency exposure should be kept at a low percentage of your portfolio. “I understand if you want to buy it because you believe the price will rise, but make sure it’s only a small part of your portfolio, maybe 1 or 2%!” says Thanos Papasavvas, founder of research group ABP Invest, who has a 20-year background in asset management (FT 2021 ). In any case, bubbles and crash analysis is an important researching area in cryptocurrency trading.
Paper collection and review schema
The section introduces the scope and approach of our paper collection, a basic analysis, and the structure of our survey.
Survey scope
We adopt a bottom-up approach to the research in cryptocurrency trading, starting from the systems up to risk management techniques. For the underlying trading system, the focus is on the optimisation of trading platforms structure and improvements of computer science technologies.
At a higher level, researchers focus on the design of models to predict return or volatility in cryptocurrency markets. These techniques become useful to the generation of trading signals. on the next level above predictive models, researchers discuss technical trading methods to trade in real cryptocurrency markets. Bubbles and extreme conditions are hot topics in cryptocurrency trading because, as discussed above, these markets have shown to be highly volatile (whilst volatility went down after crashes). Portfolio and cryptocurrency asset management are effective methods to control risk. We group these two areas in risk management research. Other papers included in this survey include topics like pricing rules, dynamic market analysis, regulatory implications, and so on. Table 3 shows the general scope of cryptocurrency trading included in this survey.
Since many trading strategies and methods in cryptocurrency trading are closely related to stock trading, some researchers migrate or use the research results for the latter to the former. When conducting this research, we only consider those papers whose research focuses on cryptocurrency markets or a comparison of trading in those and other financial markets.
Specifically, we apply the following criteria when collecting papers related to cryptocurrency trading:
The paper introduces or discusses the general idea of cryptocurrency trading or one of the related aspects of cryptocurrency trading.
The paper proposes an approach, study or framework that targets optimised efficiency or accuracy of cryptocurrency trading.
The paper compares different approaches or perspectives in trading cryptocurrency.
By “cryptocurrency trading” here, we mean one of the terms listed in Table 3 and discussed above.
Some researchers gave a brief survey of cryptocurrency (Ahamad et al. 2013 ; Sharma et al. 2017 ), cryptocurrency systems (Mukhopadhyay et al. 2016 ) and cryptocurrency trading opportunities (Kyriazis 2019 ). These surveys are rather limited in scope as compared to ours, which also includes a discussion on the latest papers in the area; we want to remark that this is a fast-moving research field.
Paper collection methodology
To collect the papers in different areas or platforms, we used keyword searches on Google Scholar and arXiv, two of the most popular scientific databases. We also choose other public repositories like SSRN but we find that almost all academic papers in these platforms can also be retrieved via Google Scholar; consequently, in our statistical analysis, we count those as Google Scholar hits. We choose arXiv as another source since it allows this survey to be contemporary with all the most recent findings in the area. The interested reader is warned that these papers have not undergone formal peer review. The keywords used for searching and collecting are listed below. [Crypto] means the cryptocurrency market, which is our research interest because methods might be different among different markets. We conducted 6 searches across the two repositories until July 1, 2021.
[Crypto] + Trading
[Crypto] + Trading system
[Crypto] + Prediction
[Crypto] + Trading strategy
[Crypto] + Risk Management
[Crypto] + Portfolio
To ensure high coverage, we adopted the so-called snowballing (Wohlin 2014 ) method on each paper found through these keywords. We checked papers added from snowballing methods that satisfy the criteria introduced above until we reached closure.
Collection results
Table 4 shows the details of the results from our paper collection. Keyword searches and snowballing resulted in 146 papers across the six research areas of interest in " Survey scope " section.
Figure 7 shows the distribution of papers published at different research sites. Among all the papers, 48.63% papers are published in Finance and Economics venues such as Journal of Financial Economics (JFE), Cambridge Centre for Alternative Finance (CCAF), Finance Research Letters, Centre for Economic Policy Research (CEPR), Finance Research Letters (FRL), Journal of Risk and Financial Management (JRFM) and some other high impact financial journals; 4.79% papers are published in Science venues such as Public Library Of Science one (PLOS one), Royal Society open science and SAGE; 14.38% papers are published in Intelligent Engineering and Data Mining venues such as Symposium Series on Computational Intelligence (SSCI), Intelligent Systems Conference (IntelliSys), Intelligent Data Engineering and Automated Learning (IDEAL) and International Conference on Data Mining (ICDM); 4.79% papers are published in Physics / Physicians venues (mostly in Physics venue) such as Physica A and Maths venue like Journal of Mathematics; 10.96% papers are published in AI and complex system venues such as Complexity and International Federation for Information Processing (IFIP); 15.07% papers are published in Others venues which contains independently published papers and dissertations; 1.37% papers are published on arXiv. The distribution of different venues shows that cryptocurrency trading is mostly published in Finance and Economics venues, but with a wide diversity otherwise.
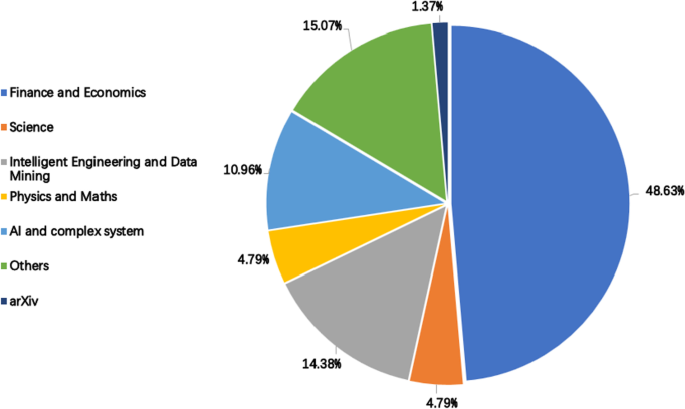
Publication venue distribution
Survey organisation
We discuss the contributions of the collected papers and a statistical analysis of these papers in the remainder of the paper, according to Table 5 .
The papers in our collection are organised and presented from six angles. We introduce the work about several different cryptocurrency trading software systems in " Cryptocurrency trading software systems " section. " Systematic trading " section introduces systematic trading applied to cryptocurrency trading. In " Emergent trading technologies " section, we introduce some emergent trading technologies including econometrics on cryptocurrencies, machine learning technologies and other emergent trading technologies in the cryptocurrency market. Section 8 introduces research on cryptocurrency pairs and related factors and crypto-asset portfolios research. In " Bubbles and crash analysis " and " Extreme condition " sections we discuss cryptocurrency market condition research, including bubbles, crash analysis, and extreme conditions. " Others related to cryptocurrency trading " section introduces other research included in cryptocurrency trading not covered above.
We would like to emphasize that the six headings above focus on a particular aspect of cryptocurrency trading; we give a complete organisation of the papers collected under each heading. This implies that those papers covering more than one aspect will be discussed in different sections, once from each angle.
We analyse and compare the number of research papers on different cryptocurrency trading properties and technologies in " Summary analysis of literature review " section, where we also summarise the datasets and the timeline of research in cryptocurrency trading.
We build upon this review to conclude in " Opportunities in cryptocurrency trading " section with some opportunities for future research.
Cryptocurrency trading Software Systems
Trading infrastructure systems.
Following the development of computer science and cryptocurrency trading, many cryptocurrency trading systems/bots have been developed. Table 6 compares the cryptocurrency trading systems existing in the market. The table is sorted based on URL types (GitHub or Official website) and GitHub stars (if appropriate).
Capfolio is a proprietary payable cryptocurrency trading system which is a professional analysis platform and has an advanced backtesting engine (Capfolio 2020 ). It supports five different cryptocurrency exchanges.
3 Commas is a proprietary payable cryptocurrency trading system platform that can take profit and stop-loss orders at the same time (3commas 2020 ). Twelve different cryptocurrency exchanges are compatible with this system.
CCXT is a cryptocurrency trading system with a unified API out of the box and optional normalized data and supports many Bitcoin / Ether / Altcoin exchange markets and merchant APIs. Any trader or developer can create a trading strategy based on this data and access public transactions through the APIs (Ccxt 2020 ). The CCXT library is used to connect and trade with cryptocurrency exchanges and payment processing services worldwide. It provides quick access to market data for storage, analysis, visualisation, indicator development, algorithmic trading, strategy backtesting, automated code generation and related software engineering. It is designed for coders, skilled traders, data scientists and financial analysts to build trading algorithms. Current CCXT features include:
Support for many cryptocurrency exchanges;
Fully implemented public and private APIs;
Optional normalized data for cross-exchange analysis and arbitrage;
Out-of-the-box unified API, very easy to integrate.
Blackbird Bitcoin Arbitrage is a C++ trading system that automatically executes long / short arbitrage between Bitcoin exchanges. It can generate market-neutral strategies that do not transfer funds between exchanges (Blackbird 2020 ). The motivation behind Blackbird is to naturally profit from these temporary price differences between different exchanges while being market neutral. Unlike other Bitcoin arbitrage systems, Blackbird does not sell but actually short sells Bitcoin on the short exchange. This feature offers two important advantages. Firstly, the strategy is always market agnostic: fluctuations (rising or falling) in the Bitcoin market will not affect the strategy returns. This eliminates the huge risks of this strategy. Secondly, this strategy does not require transferring funds (USD or BTC) between Bitcoin exchanges. Buy and sell transactions are conducted in parallel on two different exchanges. There is no need to deal with transmission delays.
StockSharp is an open-source trading platform for trading at any market of the world including 48 cryptocurrency exchanges (Stocksharp 2020 ). It has a free C# library and free trading charting application. Manual or automatic trading (algorithmic trading robot, regular or HFT) can be run on this platform. StockSharp consists of five components that offer different features:
S#.Designer - Free universal algorithm strategy app, easy to create strategies;
S#.Data - free software that can automatically load and store market data;
S#.Terminal - free trading chart application (trading terminal);
S#.Shell - ready-made graphics framework that can be changed according to needs and has a fully open source in C#;
S#.API - a free C# library for programmers using Visual Studio. Any trading strategies can be created in S#.API.
Freqtrade is a free and open-source cryptocurrency trading robot system written in Python. It is designed to support all major exchanges and is controlled by telegram. It contains backtesting, mapping and money management tools, and strategy optimization through machine learning (Fretrade 2020 ). Freqtrade has the following features:
Persistence: Persistence is achieved through SQLite technology;
Strategy optimization through machine learning: Use machine learning to optimize your trading strategy parameters with real trading data;
Marginal Position Size: Calculates winning rate, risk-return ratio, optimal stop loss and adjusts position size, and then trades positions for each specific market;
Telegram management: use telegram to manage the robot.
Dry run: Run the robot without spending money;
CryptoSignal is a professional technical analysis cryptocurrency trading system (Cryptosignal 2020 ). Investors can track over 500 coins of Bittrex, Bitfinex, GDAX, Gemini and more. Automated technical analysis includes momentum, RSI, Ichimoku Cloud, MACD, etc. The system gives alerts including Email, Slack, Telegram, etc. CryptoSignal has two primary features. First of all, it offers modular code for easy implementation of trading strategies; Secondly, it is easy to install with Docker.
Ctubio is a C++ based low latency (high frequency) cryptocurrency trading system (Ctubio 2020 ). This trading system can place or cancel orders through supported cryptocurrency exchanges in less than a few milliseconds. Moreover, it provides a charting system that can visualise the trading account status including trades completed, target position for fiat currency, etc.
Catalyst is an analysis and visualization of the cryptocurrency trading system (Catalyst 2020 ). It makes trading strategies easy to express and backtest them on historical data (daily and minute resolution), providing analysis and insights into the performance of specific strategies. Catalyst allows users to share and organise data and build profitable, data-driven investment strategies. Catalyst not only supports the trading execution but also offers historical price data of all crypto assets (from minute to daily resolution). Catalyst also has backtesting and real-time trading capabilities, which enables users to seamlessly transit between the two different trading modes. Lastly, Catalyst integrates statistics and machine learning libraries (such as matplotlib, scipy, statsmodels and sklearn) to support the development, analysis and visualization of the latest trading systems.
Golang Crypto Trading Bot is a Go based cryptocurrency trading system (Golang 2020 ). Users can test the strategy in sandbox environment simulation. If simulation mode is enabled, a fake balance for each coin must be specified for each exchange.
Real-time cryptocurrency trading systems
Bauriya et al. ( 2019 ) developed a real-time Cryptocurrency Trading System. A real-time cryptocurrency trading system is composed of clients, servers and databases. Traders use a web-application to login to the server to buy/sell crypto assets. The server collects cryptocurrency market data by creating a script that uses the Coinmarket API. Finally, the database collects balances, trades and order book information from the server. The authors tested the system with an experiment that demonstrates user-friendly and secure experiences for traders in the cryptocurrency exchange platform.
Turtle trading system in Cryptocurrency market
The original Turtle Trading system is a trend following trading system developed in the 1970s. The idea is to generate buy and sell signals on stock for short-term and long-term breakouts and its cut-loss condition which is measured by Average true range (ATR) (Kamrat et al. 2018 ). The trading system will adjust the size of assets based on their volatility. Essentially, if a turtle accumulates a position in a highly volatile market, it will be offset by a low volatility position. Extended Turtle Trading system is improved with smaller time interval spans and introduces a new rule by using exponential moving average (EMA). Three EMA values are used to trigger the “buy” signal: 30EMA (Fast), 60EMA (Slow), 100EMA (Long). The author of Kamrat et al. ( 2018 ) performed backtesting and comparing both trading systems (Original Turtle and Extended Turtle) on 8 prominent cryptocurrencies. Through the experiment, Original Turtle Trading System achieved an 18.59% average net profit margin (percentage of net profit over total revenue) and 35.94% average profitability (percentage of winning trades over total numbers of trades) in 87 trades through nearly one year. Extended Turtle Trading System achieved 114.41% average net profit margin and 52.75% average profitability in 41 trades through the same time interval. This research showed how Extended Turtle Trading System compared can improve over Original Turtle Trading System in trading cryptocurrencies.
Arbitrage trading systems for cryptocurrencies
Christian (Păuna 2018 ) introduced arbitrage trading systems for cryptocurrencies. Arbitrage trading aims to spot the differences in price that can occur when there are discrepancies in the levels of supply and demand across multiple exchanges. As a result, a trader could realise a quick and low-risk profit by buying from one exchange and selling at a higher price on a different exchange. Arbitrage trading signals are caught by automated trading software. The technical differences between data sources impose a server process to be organised for each data source. Relational databases and SQL are reliable solution due to the large amounts of relational data. The author used the system to catch arbitrage opportunities on 25 May 2018 among 787 cryptocurrencies on 7 different exchanges. The research paper (Păuna 2018 ) listed the best ten trading signals made by this system from 186 available found signals. The results showed that the system caught the trading signal of “BTG-BTC” to get a profit of up to 495.44% when arbitraging to buy in Cryptopia exchange and sell in Binance exchange. Another three well-traded arbitrage signals (profit expectation around 20% mentioned by the author) were found on 25 May 2018. Arbitrage Trading Software System introduced in that paper presented general principles and implementation of arbitrage trading system in the cryptocurrency market.
Characteristics of three cryptocurrency trading systems
Real-time trading systems use real-time functions to collect data and generate trading algorithms. Turtle trading system and arbitrage trading system have shown a sharp contrast in their profit and risk behaviour. Using Turtle trading system in cryptocurrency markets got high returns with high risk. Arbitrage trading system is inferior in terms of revenue but also has a lower risk. One feature that turtle trading system and arbitrage trading system have in common is they performed well in capturing alpha.
Technical analysis
Many researchers have focused on technical indicators (patterns) analysis for trading on cryptocurrency markets. Examples of studies with this approach include “Turtle Soup pattern strategy” (TradingstrategyGuides 2019 ), “Nem (XEM) strategy” (TradingstrategyGuides 2019 ), “Amazing Gann Box strategy” (TradingstrategyGuides 2019 ), “Busted Double Top Pattern strategy” (TradingstrategyGuides 2019 ), and “Bottom Rotation Trading strategy” (TradingstrategyGuides 2019 ). Table 7 shows the comparison among these five classical technical trading strategies using technical indicators. “Turtle soup pattern strategy” (TradingstrategyGuides 2019 ) used a 2-day breakout of price in predicting price trends of cryptocurrencies. This strategy is a kind of chart trading pattern. “Nem (XEM) strategy” combined Rate of Change (ROC) indicator and Relative Strength Index (RSI) in predicting price trends (TradingstrategyGuides 2019 ). “Amazing Gann Box” predicted exact points of increase and decrease in Gann Box which are used to catch explosive trends of cryptocurrency price (TradingstrategyGuides 2019 ). Technical analysis tools such as candlestick and box charts with Fibonacci Retracement based on golden ratio are used in this technical analysis. Fibonacci Retracement uses horizontal lines to indicate where possible support and resistance levels are in the market. “Busted Double Top Pattern” used a Bearish reversal trading pattern which generates a sell signal to predict price trends (TradingstrategyGuides 2019 ). “Bottom Rotation Trading” is a technical analysis method that picks the bottom before the reversal happens. This strategy used a price chart pattern and box chart as technical analysis tools.
Ha and Moon ( 2018 ) investigated using genetic programming (GP) to find attractive technical patterns in the cryptocurrency market. Over 12 technical indicators including Moving Average (MA) and Stochastic oscillator were used in experiments; adjusted gain, match count, relative market pressure and diversity measures have been used to quantify the attractiveness of technical patterns. With extended experiments, the GP system is shown to find successfully attractive technical patterns, which are useful for portfolio optimization. Hudson and Urquhart ( 2019 ) applied almost 15,000 to technical trading rules (classified into MA rules, filter rules, support resistance rules, oscillator rules and channel breakout rules). This comprehensive study found that technical trading rules provide investors with significant predictive power and profitability. Corbet et al. ( 2019 ) analysed various technical trading rules in the form of the moving average-oscillator and trading range break-out strategies to generate higher returns in cryptocurrency markets. By using one-minute dollar-denominated Bitcoin close-price data, the backtest showed variable-length moving average (VMA) rule performs best considering it generates the most useful signals in high frequency trading.
Grobys et al. ( 2020 ) examined a simple moving average trading strategy using daily price data for the 11 most traded cryptocurrencies over the period 2016-2018. The results showed that, excluding Bitcoin, technical trading rules produced an annualised excess return of 8.76% after controlling for average market returns. The analysis also suggests that cryptocurrency markets are inefficient. Al-Yahyaee et al. ( 2020 ) examined multiple fractals, long memory processes and efficiency assumptions of major cryptocurrencies using Hurst exponents, time-rolling MF-DFA and quantile regression methods. The results showed that all markets provide evidence of long-term memory properties and multiple fractals. Furthermore, the inefficiency of cryptocurrency markets is time-varying. The researchers concluded that high liquidity with low volatility facilitates arbitrage opportunities for active traders.
Pairs trading
Pairs trading is a trading strategy that attempts to exploit the mean-reversion between the prices of certain securities. Miroslav (Fil 2019 ) investigated the applicability of standard pairs trading approaches on cryptocurrency data with the benchmarks of Gatev et al. ( 2006 ). The pairs trading strategy is constructed in two steps. Firstly, suitable pairs with a stable long-run relationship are identified. Secondly, the long-run equilibrium is calculated and pairs trading strategy is defined by the spread based on the values. The research also extended intra-day pairs trading using high frequency data. Overall, the model was able to achieve a 3% monthly profit in Miroslav’s experiments (Fil 2019 ). Broek (van den Broek and Sharif 2018 ) applied pairs trading based on cointegration in cryptocurrency trading and 31 pairs were found to be significantly cointegrated (within sector and cross-sector). By selecting four pairs and testing over a 60-day trading period, the pairs trading strategy got its profitability from arbitrage opportunities, which rejected the Efficient-market hypothesis (EMH) for the cryptocurrency market. Lintilhac and Tourin ( 2017 ) proposed an optimal dynamic pair trading strategy model for a portfolio of assets. The experiment used stochastic control techniques to calculate optimal portfolio weights and correlated the results with several other strategies commonly used by practitioners including static dual-threshold strategies. Li and Tourin ( 2016 ) proposed a pairwise trading model incorporating time-varying volatility with constant elasticity of variance type. The experiment calculated the best pair strategy by using a finite difference method and estimated parameters by generalised moment method.
Other systematic trading methods in cryptocurrency trading mainly include informed trading. Using USD / BTC exchange rate trading data, Feng et al. ( 2018 ) found evidence of informed trading in the Bitcoin market in those quantiles of the order sizes of buyer-initiated (seller-initiated) orders are abnormally high before large positive (negative) events, compared to the quantiles of seller-initiated (buyer-initiated) orders; this study adopts a new indicator inspired by the volume imbalance indicator (Easley et al. 2008 ). The evidence of informed trading in the Bitcoin market suggests that investors profit on their private information when they get information before it is widely available.
Emergent trading technologies
Copula-quantile causality analysis and Granger-causality analysis are methods to investigate causality in cryptocurrency trading analysis. Bouri et al. ( 2019 ) applied a copula-quantile causality approach on volatility in the cryptocurrency market. The approach of the experiment extended the Copula-Granger-causality in distribution (CGCD) method of Lee and Yang ( 2014 ) in 2014. The experiment constructed two tests of CGCD using copula functions. The parametric test employed six parametric copula functions to discover dependency density between variables. The performance matrix of these functions varies with independent copula density. Three distribution regions are the focus of this research: left tail (1%, 5%, 10% quantile), central region (40%, 60% quantile and median) and right tail (90%, 95%, 99% quantile). The study provided significant evidence of Granger causality from trading volume to the returns of seven large cryptocurrencies on both left and right tails. Bouri et al. ( 2020 ) examined the causal linkages among the volatility of leading cryptocurrencies via the frequency-domain test of Bodart and Candelon ( 2009 ) and distinguished between temporary and permanent causation. The results showed that permanent shocks are more important in explaining Granger causality whereas transient shocks dominate the causality of smaller cryptocurrencies in the long term. Badenhorst et al. ( 2019 ) attempted to reveal whether spot and derivative market volumes affect Bitcoin price volatility with the Granger-causality method and ARCH (1,1). The result shows spot trading volumes have a significant positive effect on price volatility while the relationship between cryptocurrency volatility and the derivative market is uncertain. Bouri et al. ( 2020 ) used a dynamic equicorrelation (DECO) model and reported evidence that the average earnings equilibrium correlation changes over time between the 12 leading cryptocurrencies. The results showed increased cryptocurrency market consolidation despite significant price declined in 2018. Furthermore, measurement of trading volume and uncertainty are key determinants of integration.
Several econometrics methods in time-series research, such as GARCH and BEKK, have been used in the literature on cryptocurrency trading. Conrad et al. ( 2018 ) used the GARCH-MIDAS model to extract long and short-term volatility components of the Bitcoin market. The technical details of this model decomposed the conditional variance into the low-frequency and high-frequency components. The results identified that S&P 500 realized volatility has a negative and highly significant effect on long-term Bitcoin volatility and S&P 500 volatility risk premium has a significantly positive effect on long-term Bitcoin volatility. Ardia et al. ( 2019 ) used the Markov Switching GARCH (MSGARCH) model to test the existence of institutional changes in the GARCH volatility dynamics of Bitcoin’s logarithmic returns. Moreover, a Bayesian method was used for estimating model parameters and calculating VaR prediction. The results showed that MSGARCH models clearly outperform single-regime GARCH for Value-at-Risk forecasting. Troster et al. ( 2019 ) performed general GARCH and GAS (Generalized Auto-regressive Score) analysis to model and predict Bitcoin’s returns and risks. The experiment found that the GAS model with heavy-tailed distribution can provide the best out-of-sample prediction and goodness-of-fit attributes for Bitcoin’s return and risk modeling. The results also illustrated the importance of modeling excess kurtosis for Bitcoin returns.
Charles and Darné ( 2019 ) studied four cryptocurrency markets including Bitcoin, Dash, Litecoin and Ripple. Results showed cryptocurrency returns are strongly characterised by the presence of jumps as well as structural breaks except the Dash market. Four GARCH-type models (i.e., GARCH, APARCH, IGARCH and FIGARCH) and three return types with structural breaks (original returns, jump-filtered returns, and jump-filtered returns) are considered. The research indicated the importance of jumps in cryptocurrency volatility and structural breakthroughs. Malladi and Dheeriya ( 2021 ) examined the time series analysis of Bitcoin and Ripple’s returns and volatility to examine the dependence of their prices in part on global equity indices, gold prices and fear indicators such as volatility indices and US economic policy uncertainty indices. Autoregressive-moving-average model with exogenous inputs model (ARMAX), GARCH, VAR and Granger causality tests are used in the experiments. The results showed that there is no causal relationship between global stock market and gold returns on bitcoin returns, but a causal relationship between ripple returns on bitcoin prices is found.
Some researchers focused on long memory methods for volatility in cryptocurrency markets. Long memory methods focused on long-range dependence and significant long-term correlations among fluctuations on markets. Chaim and Laurini ( 2019 ) estimated a multivariate stochastic volatility model with discontinuous jumps in cryptocurrency markets. The results showed that permanent volatility appears to be driven by major market developments and popular interest levels. Caporale et al. ( 2018 ) examined persistence in the cryptocurrency market by Rescaled range (R/S) analysis and fractional integration. The results of the study indicated that the market is persistent (there is a positive correlation between its past and future values) and that its level changes over time. Khuntia and Pattanayak ( 2018 ) applied the adaptive market hypothesis (AMH) in the predictability of Bitcoin evolving returns. The consistent test of (Domínguez and Lobato 2003 ), generalized spectral (GS) of (Escanciano and Velasco 2006 ) are applied in capturing time-varying linear and nonlinear dependence in bitcoin returns. The results verified Evolving Efficiency in Bitcoin price changes and evidence of dynamic efficiency in line with AMH’s claims. Gradojevic and Tsiakas ( 2021 ) examined volatility cascades across multiple trading ranges in the cryptocurrency market. Using a wavelet Hidden Markov Tree model, authors estimated the transition probability of propagating high or low volatility at one time scale (range) to high or low volatility at the next time scale. The results showed that the volatility cascade tends to be symmetrical when moving from long to short term. In contrast, when moving from short to long term, the volatility cascade is very asymmetric.
Nikolova et al. ( 2020 ) provided a new method to calculate the probability of volatility clusters, especially for cryptocurrencies (high volatility of their exchange rates). The authors used the FD4 method to calculate the Hurst index of a volatility series and describe explicit criteria for determining the existence of fixed size volatility clusters by calculation. The results showed that the volatility of cryptocurrencies changes more rapidly than that of traditional assets, and much more rapidly than that of Bitcoin/USD, Ethereum/USD, and Ripple/USD pairs. Ma et al. ( 2020 ) investigated whether a new Markov Regime Transformation Mixed Data Sampling (MRS-MIADS) model can improve the prediction accuracy of Bitcoin’s Realised Variance (RV). The results showed that the proposed new MRS-MIDAS model exhibits statistically significant improvements in predicting the RV of Bitcoin. At the same time, the occurrence of jumps significantly increases the persistence of high volatility and switches between high and low volatility.
Katsiampa et al. ( 2018 ) applied three pair-wise bivariate BEKK models to examine the conditional volatility dynamics along with interlinkages and conditional correlations between three pairs of cryptocurrencies in 2018. More specifically, the BEKK-MGARCH methodology also captured cross-market effects of shocks and volatility, which are also known as shock transmission effects and volatility spillover effects. The experiment found evidence of bi-directional shock transmission effects between Bitcoin and both Ether and Litcoin. In particular, bi-directional shock spillover effects are identified between three pairs (Bitcoin, Ether and Litcoin) and time-varying conditional correlations exist with positive correlations mostly prevailing. In 2019, Katsiampa ( 2019 ) further researched an asymmetric diagonal BEKK model to examine conditional variances of five cryptocurrencies that are significantly affected by both previous squared errors and past conditional volatility. The experiment tested the null hypothesis of the unit root against the stationarity hypothesis. Once stationarity is ensured, ARCH LM is tested for ARCH effects to examine the requirement of volatility modeling in return series. Moreover, volatility co-movements among cryptocurrency pairs are also tested by the multivariate GARCH model. The results confirmed the non-normality and heteroskedasticity of price returns in cryptocurrency markets. The finding also identified the effects of cryptocurrencies’ volatility dynamics due to major news.
Hultman ( 2018 ) set out to examine GARCH (1,1), bivariate-BEKK (1,1) and a standard stochastic model to forecast the volatility of Bitcoin. A rolling window approach is used in these experiments. Mean absolute error (MAE), Mean squared error (MSE) and Root-mean-square deviation (RMSE) are three loss criteria adopted to evaluate the degree of error between predicted and true values. The result shows the following rank of loss functions: GARCH (1,1) > bivariate-BEKK (1,1) > Standard stochastic for all the three different loss criteria; in other words, GARCH(1,1) appeared best in predicting the volatility of Bitcoin. Wavelet time-scale persistence analysis is also applied in the prediction and research of volatility in cryptocurrency markets (Omane-Adjepong et al. 2019 ). The results showed that information efficiency (efficiency) and volatility persistence in the cryptocurrency market are highly sensitive to time scales, measures of returns and volatility, and institutional changes. Omane-Adjepong et al. ( 2019 ) connected with similar research by Corbet et al. ( 2018 ) and showed that GARCH is quicker than BEKK to absorb new information regarding the data.
Zhang and Li ( 2020 ) examined how to price exceptional volatility in a cross-section of cryptocurrency returns. Using portfolio-level analysis and Fama-MacBeth regression analysis, the authors demonstrated that idiosyncratic volatility is positively correlated with expected returns on cryptocurrencies.
As we have previously stated, Machine learning technology constructs computer algorithms that automatically improve themselves by finding patterns in existing data without explicit instructions (Holmes et al. 1994 ). The rapid development of machine learning in recent years has promoted its application to cryptocurrency trading, especially in the prediction of cryptocurrency returns. Some ML algorithms solve both classification and regression problems from a methodological point of view. For clearer classification, we focus on the application of these ML algorithms in cryptocurrency trading. For example, Decision Tree (DT) can solve both classification and regression problems. But in cryptocurrency trading, researchers focus more on using DT in solving classification problems. Here we classify DT as “Classification Algorithms”.
Common machine learning technology in this survey
Several machine learning technologies are applied in cryptocurrency trading. We distinguish these by the objective set to the algorithm: classification, clustering, regression, reinforcement learning. We have separated a section specifically on deep learning due to its intrinsic variation of techniques and wide adoption.
Classification algorithms Classification in machine learning has the objective of categorising incoming objects into different categories as needed, where we can assign labels to each category (e.g., up and down). Naive Bayes (NB) (Rish et al. 2001 ), Support Vector Machine (SVM) (Wang 2005 ), K-Nearest Neighbours (KNN) (Wang 2005 ), Decision Tree (DT) (Friedl and Brodley 1997 ), Random Forest (RF) (Liaw and Wiener 2002 ) and Gradient Boosting (GB) (Friedman et al. 2001 ) algorithms habe been used in cryptocurrency trading based on papers we collected. NB is a probabilistic classifier based on Bayes’ theorem with strong (naive) conditional independence assumptions between features (Rish et al. 2001 ). SVM is a supervised learning model that aims at achieving high margin classifiers connecting to learning bounds theory (Zemmal et al. 2016 ). SVMs assign new examples to one category or another, making it a non-probabilistic binary linear classifier (Wang 2005 ), although some corrections can make a probabilistic interpretation of their output (Keerthi et al. 2001 ). KNN is a memory-based or lazy learning algorithm, where the function is only approximated locally, and all calculations are being postponed to inference time (Wang 2005 ). DT is a decision support tool algorithm that uses a tree-like decision graph or model to segment input patterns into regions to then assign an associated label to each region (Friedl and Brodley 1997 ; Fang et al. 2020 ). RF is an ensemble learning method. The algorithm operates by constructing a large number of decision trees during training and outputting the average consensus as predicted class in the case of classification or mean prediction value in the case of regression (Liaw and Wiener 2002 ). GB produces a prediction model in the form of an ensemble of weak prediction models (Friedman et al. 2001 ).
Clustering algorithms Clustering is a machine learning technique that involves grouping data points in a way that each group shows some regularity (Jianliang et al. 2009 ). K-Means is a vector quantization used for clustering analysis in data mining. K-means stores the k -centroids used to define the clusters; a point is considered to be in a particular cluster if it is closer to the cluster’s centroid than any other centroid (Wagstaff et al. 2001 ). K-Means is one of the most used clustering algorithms used in cryptocurrency trading according to the papers we collected. Clustering algorithms have been successfully applied in many financial applications, such as fraud detection, rejection inference and credit assessment. Automated detection clusters are critical as they help to understand sub-patterns of data that can be used to infer user behaviour and identify potential risks (Li et al. 2021 ; Kou et al. 2014 ).
Regression algorithms We have defined regression as any statistical technique that aims at estimating a continuous value (Kutner et al. 2005 ). Linear Regression (LR) and Scatterplot Smoothing are common techniques used in solving regression problems in cryptocurrency trading. LR is a linear method used to model the relationship between a scalar response (or dependent variable) and one or more explanatory variables (or independent variables) (Kutner et al. 2005 ). Scatterplot Smoothing is a technology to fit functions through scatter plots to best represent relationships between variables (Friedman and Tibshirani 1984 ).
Deep Learning algorithms Deep learning is a modern take on artificial neural networks (ANNs) (Zhang et al. 2019 ), made possible by the advances in computational power. An ANN is a computational system inspired by the natural neural networks that make up the animal’s brain. The system “learns” to perform tasks including the prediction by considering examples. Deep learning’s superior accuracy comes from high computational complexity cost. Deep learning algorithms are currently the basis for many modern artificial intelligence applications (Sze et al. 2017 ). Convolutional neural networks (CNNs) (Lawrence et al. 1997 ), Recurrent neural networks (RNNs) (Mikolov et al. 2011 ), Gated recurrent units (GRUs) (Chung et al. 2014 ), Multilayer perceptron (MLP) and Long short-term memory (LSTM) (Cheng et al. 2016 ) networks are the most common deep learning technologies used in cryptocurrency trading. A CNN is a specific type of neural network layer commonly used for supervised learning. CNNs have found their best success in image processing and natural language processing problems. An attempt to use CNNs in cryptocurrency can be shown in (Kalchbrenner et al. 2014 ). An RNN is a type of artificial neural network in which connections between nodes form a directed graph with possible loops. This structure of RNNs makes them suitable for processing time-series data (Mikolov et al. 2011 ) due to the introduction of memory in the recurrent connections. They face nevertheless for the vanishing gradients problem (Pascanu et al. 2013 ) and so different variations have been recently proposed. LSTM (Cheng et al. 2016 ) is a particular RNN architecture widely used. LSTMs have shown to be superior to nongated RNNs on financial time-series problems because they have the ability to selectively remember patterns for a long time. A GRU (Chung et al. 2014 ) is another gated version of the standard RNN which has been used in crypto trading (Dutta et al. 2020 ). Another deep learning technology used in cryptocurrency trading is Seq2seq, which is a specific implementation of the Encoder-Decoder architecture (Xu et al. 2017 ). Seq2seq was first aimed at solving natural language processing problems but has been also applied it in cryptocurrency trend predictions in Sriram et al. ( 2017 ).
Reinforcement learning algorithms Reinforcement learning (RL) is an area of machine learning leveraging the idea that software agents act in the environment to maximize a cumulative reward (Sutton and Barto 1998 ). Deep Q-Learning (DQN) (Gu et al. 2016 ) and Deep Boltzmann Machine (DBM) (Salakhutdinov and Hinton 2009 ) are common technologies used in cryptocurrency trading using RL. Deep Q learning uses neural networks to approximate Q-value functions. A state is given as input, and Q values for all possible actions are generated as outputs (Gu et al. 2016 ). DBM is a type of binary paired Markov random field (undirected probability graphical model) with multiple layers of hidden random variables (Salakhutdinov and Hinton 2009 ). It is a network of randomly coupled random binary units.
Research on machine learning models
In the development of machine learning trading signals, technical indicators have usually been used as input features. Nakano et al. ( 2018 ) explored Bitcoin intraday technical trading based on ANNs for return prediction. The experiment obtained medium frequency price and volume data (time interval of data is 15min) of Bitcoin from a cryptocurrency exchange. An ANN predicts the price trends (up and down) in the next period from the input data. Data is preprocessed to construct a training dataset that contains a matrix of technical patterns including EMA, Emerging Markets Small Cap (EMSD), relative strength index (RSI), etc. Their numerical experiments contain different research aspects including base ANN research, effects of different layers, effects of different activation functions, different outputs, different inputs and effects of additional technical indicators. The results have shown that the use of various technical indicators possibly prevents over-fitting in the classification of non-stationary financial time-series data, which enhances trading performance compared to the primitive technical trading strategy. (Buy-and-Hold is the benchmark strategy in this experiment.)
Some classification and regression machine learning models are applied in cryptocurrency trading by predicting price trends. Most researchers have focused on the comparison of different classification and regression machine learning methods. Sun et al. ( 2019 ) used random forests (RFs) with factors in Alpha01 (Kakushadze 2016 ) (capturing features from the history of the cryptocurrency market) to build a prediction model. The experiment collected data from API in cryptocurrency exchanges and selected 5-min frequency data for backtesting. The results showed that the performances are proportional to the amount of data (more data, more accurate) and the factors used in the RF model appear to have different importance. For example, “Alpha024” and “Alpha032” features appeared as the most important in the model adopted. (The alpha features come from paper “101 Formulaic Alphas” (Kakushadze 2016 ).) Vo and Yost-Bremm ( 2018 ) applied RFs in High-Frequency cryptocurrency Trading (HFT) and compared it with deep learning models. Minute-level data is collected when utilising a forward fill imputation method to replace the NULL value (i.e., a missing value). Different periods and RF trees are tested in the experiments. The authors also compared F-1 precision and recall metrics between RF and Deep Learning (DL). The results showed that RF is effective despite multicollinearity occurring in ML features, the lack of model identification also potentially leading to model identification issues; this research also attempted to create an HFT strategy for Bitcoin using RF.
Slepaczuk and Zenkova ( 2018 ) investigated the profitability of an algorithmic trading strategy based on training an SVM model to identify cryptocurrencies with high or low predicted returns. The results showed that the performance of the SVM strategy was the fourth being better only than S&P B&H strategy, which simply buys-and-hold the S&P index. (There are other 4 benchmark strategies in this research.) The authors observed that SVM needs a large number of parameters and so is very prone to overfitting, which caused its bad performance. Barnwal et al. ( 2019 ) used generative and discriminative classifiers to create a stacking model, particularly 3 generative and 6 discriminative classifiers combined by a one-layer Neural Network, to predict the direction of cryptocurrency price. A discriminative classifier directly models the relationship between unknown and known data, while generative classifiers model the prediction indirectly through the data generation distribution (Ng and Jordan 2002 ). Technical indicators including trend, momentum, volume and volatility, are collected as features of the model. The authors discussed how different classifiers and features affect the prediction. Attanasio et al. ( 2019 ) compared a variety of classification algorithms including SVM, NB and RF in predicting next-day price trends of a given cryptocurrency. The results showed that due to the heterogeneity and volatility of cryptocurrencies’ financial instruments, forecasting models based on a series of forecasts appeared better than a single classification technology in trading cryptocurrencies. Madan et al. ( 2015 ) modeled the Bitcoin price prediction problem as a binomial classification task, experimenting with a custom algorithm that leverages both random forests and generalized linear models. Daily data, 10-min data and 10-s data are used in the experiments. The experiments showed that 10-min data gave a better sensitivity and specificity ratio than 10-second data (10-second prediction achieved around 10% accuracy). Considering predictive trading, 10-min data helped show clearer trends in the experiment compared to 10-second backtesting. Similarly, Virk ( 2017 ) compared RF, SVM, GB and LR to predict the price of Bitcoin. The results showed that SVM achieved the highest accuracy of 62.31% and precision value 0.77 among binomial classification machine learning algorithms.
Different deep learning models have been used in finding patterns of price movements in cryptocurrency markets. Zhengyang et al. ( 2019 ) implemented two machine learning models, fully-connected ANN and LSTM to predict cryptocurrency price dynamics. The results showed that ANN, in general, outperforms LSTM although theoretically, LSTM is more suitable than ANN in terms of modeling time series dynamics; the performance measures considered are MAE and RMSE in joint prediction (five cryptocurrencies daily prices prediction). The findings show that the future state of a time series for cryptocurrencies is highly dependent on its historic evolution. Kwon et al. ( 2019 ) used an LSTM model, with a three-dimensional price tensor representing the past price changes of cryptocurrencies as input. This model outperforms the GB model in terms of F1-score. Specifically, it has a performance improvement of about \(7\%\) over the GB model in 10-min price prediction. In particular, the experiments showed that LSTM is more suitable when classifying cryptocurrency data with high volatility.
Alessandretti et al. ( 2018 ) tested Gradient boosting decision trees (including single regression and XGBoost-augmented regression) and the LSTM model on forecasting daily cryptocurrency prices. They found methods based on gradient boosting decision trees worked best when predictions were based on short-term windows of 5/10 days while LSTM worked best when predictions were based on 50 days of data. The relative importance of the features in both models are compared and an optimised portfolio composition (based on geometric mean return and Sharpe ratio) is discussed in this paper. Phaladisailoed and Numnonda ( 2018 ) chose regression models (Theil-Sen Regression and Huber Regression) and deep learning-based models (LSTM and GRU) to compare the performance of predicting the rise and fall of Bitcoin price. In terms of two common measure metrics, MSE and R-Square ( \(\hbox {R}^2\) ), GRU shows the best accuracy.
Researchers have also focused on comparing classical statistical models and machine/deep learning models. Rane and Dhage ( 2019 ) described classical time series prediction methods and machine learning algorithms used for predicting Bitcoin price. Statistical models such as Autoregressive Integrated Moving Average models (ARIMA), Binomial Generalized Linear Model and GARCH are compared with machine learning models such as SVM, LSTM and Non-linear Auto-Regressive with Exogenous Input Model (NARX). The observation and results showed that the NARX model is the best model with nearly 52% predicting accuracy based on 10 seconds interval. Rebane et al. ( 2018 ) compared traditional models like ARIMA with a modern popular model like seq2seq in predicting cryptocurrency returns. The result showed that the seq2seq model exhibited demonstrable improvement over the ARIMA model for Bitcoin-USD prediction but the seq2seq model showed very poor performance in extreme cases. The authors proposed performing additional investigations, such as the use of LSTM instead of GRU units to improve the performance. Similar models were also compared by Stuerner ( 2019 ) who explored the superiority of automated investment approach in trend following and technical analysis in cryptocurrency trading. Persson et al. ( 2018 ) explored the vector autoregressive model (VAR model), a more complex RNN, and a hybrid of the two in residual recurrent neural networks (R2N2) in predicting cryptocurrency returns. The RNN with ten hidden layers is optimised for the setting and the neural network augmented by VAR allows the network to be shallower, quicker and to have a better prediction than an RNN. RNN, VAR and R2N2 models are compared. The results showed that the VAR model has phenomenal test period performance and thus props up the R2N2 model, while the RNN performs poorly. This research is an attempt at optimisation of model design and applying to the prediction on cryptocurrency returns.
Deep neural network
Deep Neural Network architectures play important roles in forecasting. Researchers had applied many advanced deep neural network models in cryptocurrency trading like stacking (CNN + RNN) and Autoencoder-Decoder. In this subsection, we describe the cutting edge Deep Neural Network researches in cryptocurrency trading. Recent studies show the productivity of using models based on such architectures for modeling and forecasting financial time series, including cryptocurrencies. Livieris et al. ( 2020 ) proposed model called CNN-LSTM for accurate prediction of gold prices and movements. The first component of the model consists of a convolutional layer and a pooling layer, where complex mathematical operations are performed to develop the features of the input data. The second component uses the generated LSTM and the features of the dense layer. The results show that due to the sensitivity of the various hyperparameters of the proposed CNN-LSTM and its high complexity, additional optimisation configurations and major feature engineering have the potential to further improve the predictive power. More Intelligent Evolutionary Optimisation (IEO) for hyperparameter optimisation is core problem when tuning the overall optimization process of machine learning models (Huan et al. 2020 ). Lu et al. ( 2020 ) proposed a CNN-LSTM based method for stock price prediction. In In terms of MAE, RMSE and \(R^2\) metrics, the experimental results showed that CNN-LSTM has the highest prediction accuracy and the best performance compared with MLP, CNN, RNN, LSTM, and CNN-RNN.
Fang et al. ( 2021 ) applied an autoencoder-augmented LSTM structure in predicting the mid-price of 8 cryptocurrency pairs. Level-2 limit order book live data is collected and the experiment achieved 78% accuracy of price movements prediction in high frequency trading (tick level). This research improved and verified the view of Sirignano and Cont ( 2019 ) that universal models have better performance than currency-pair specific models for cryptocurrency markets. Moreover, “Walkthrough” (i.e., retrain the original deep learning model itself when it appears to no longer be valid) is proposed as a method to optimise the training of a deep learning model and shown to significantly improve the prediction accuracy. Yao et al. ( 2018 ) proposed a new method for predicting cryptocurrency prices based on deep learning techniques such as RNN and LSTM, taking into account various factors such as market capitalization, trading volume, circulating supply and maximum supply. The experimental results showed that the model performs well for a certain size of dataset. Livieris et al. ( 2020 ) combined three of the most widely used integration learning strategies: integrated averaging, bagging and stacking, with advanced deep learning models for predicting hourly prices of major cryptocurrencies. The proposed integrated model is evaluated using a state-of-the-art deep learning model as a component learner, which consists of a combination of LSTM, bidirectional LSTM and convolutional layers. The authors’ detailed experimental analysis shows that integrated learning and deep learning can effectively reinforce each other to develop robust, stable and reliable predictive models. Kumar and Rath ( 2020 ) analyzed how deep learning techniques such as MLP and LSTM can help predict the price trend of Ethereum. By applying day/hour/minute historical data, the LSTM model is more robust and accurate to long-term dependencies than the MLP while LSTM outperformed the MLP marginally but not very significantly.
Sentiment analysis
Sentiment analysis, a popular research topic in the age of social media, has also been adopted to improve predictions for cryptocurrency trading. This data source typically has to be combined with Machine Learning for the generation of trading signals.
Lamon et al. ( 2017 ) used daily news and social media data labeled on actual price changes, rather than on positive and negative sentiment. By this approach, the prediction on price is replaced with positive and negative sentiment. The experiment acquired cryptocurrency-related news article headlines from the website like “cryptocoinsnews” and twitter API. Weights are taken in positive and negative words in the cryptocurrency market. Authors compared Logistic Regression (LR), Linear Support Vector Machine (LSVM) and NB as classifiers and concluded that LR is the best classifier in daily price prediction with 43.9% of price increases correctly predicted and 61.9% of price decreases correctly forecasted. Smuts ( 2019 ) conducted a similar binary sentiment-based price prediction method with an LSTM model using Google Trends and Telegram sentiment. In detail, the sentiment was extracted from Telegram by using a novel measure called VADER (Hutto and Gilbert 2014 ). The backtesting reached 76% accuracy on the test set during the first half of 2018 in predicting hourly prices.
Nasir et al. ( 2019 ) researched the relationship between cryptocurrency returns and search engines. The experiment employed a rich set of established empirical approaches including VAR framework, copulas approach and non-parametric drawings of time series. The results found that Google searches exert significant influence on Bitcoin returns, especially in the short-term intervals. Kristoufek ( 2013 ) discussed positive and negative feedback on Google trends or daily views on Wikipedia. The author mentioned different methods including Cointegration, Vector autoregression and Vector error-correction model to find causal relationships between prices and searched terms in the cryptocurrency market. The results indicated that search trends and cryptocurrency prices are connected. There is also a clear asymmetry between the effects of increased interest in currencies above or below their trend values from the experiment. Kim et al. ( 2016 ) analysed user comments and replies in online communities and their connection with cryptocurrency volatility. After crawling comments and replies in online communities, authors tagged the extent of positive and negative topics. Then the relationship between price and the number of transactions of cryptocurrency is tested according to comments and replies to selected data. At last, a prediction model using machine learning based on selected data is created to predict fluctuations in the cryptocurrency market. The results show the amount of accumulated data and animated community activities exerted a direct effect on fluctuation in the price and volume of a cryptocurrency.
Phillips and Gorse ( 2018 ) applied dynamic topic modeling and Hawkes model to decipher relationships between topics and cryptocurrency price movements. The authors used Latent Dirichlet allocation (LDA) model for topic modeling, which assumes each document contains multiple topics to different extents. The experiment showed that particular topics tend to precede certain types of price movements in the cryptocurrency market and the authors proposed the relationships could be built into real-time cryptocurrency trading. Li et al. ( 2019 ) analysed Twitter sentiment and trading volume and an Extreme Gradient Boosting Regression Tree Model in the prediction of ZClassic (ZCL) cryptocurrency market. Sentiment analysis using natural language processing from the Python package “Textblob” assigns impactful words a polarity value. Values of weighted and unweighted sentiment indices are calculated on an hourly basis by summing weights of coinciding tweets, which makes us compare this index to ZCL price data. The model achieved a Pearson correlation of 0.806 when applied to test data, yielding a statistical significance at the \(p < 0.0001\) level. Flori ( 2019 ) relied on a Bayesian framework that combines market-neutral information with subjective beliefs to construct diversified investment strategies in the Bitcoin market. The result shows that news and media attention seem to contribute to influence the demand for Bitcoin and enlarge the perimeter of the potential investors, probably stimulating price euphoria and upwards-downwards market dynamics. The authors’ research highlighted the importance of news in guiding portfolio re-balancing. Bouri and Gupta ( 2019 ) compared the ability of newspaper-based metrics and internet search-based uncertainty metrics in predicting bitcoin returns. The predictive power of Internet-based economic uncertainty-related query indices is statistically stronger than that of newspapers in predicting bitcoin returns.
Similarly, Colianni et al. ( 2015 ), Garcia and Schweitzer ( 2015 ), Zamuda et al. ( 2019 ) et al. used sentiment analysis technology applying it in the cryptocurrency trading area and had similar results. Colianni et al. ( 2015 ) cleaned data and applied supervised machine learning algorithms such as logistic regression, Naive Bayes and support vector machines, etc. on Twitter Sentiment Analysis for cryptocurrency trading. Garcia and Schweitzer ( 2015 ) applied multidimensional analysis and impulse analysis in social signals of sentiment effects and algorithmic trading of Bitcoin. The results verified the long-standing assumption that transaction-based social media sentiment has the potential to generate a positive return on investment. Zamuda et al. ( 2019 ) adopted new sentiment analysis indicators and used multi-target portfolio selection to avoid risks in cryptocurrency trading. The perspective is rationalized based on the elastic demand for computing resources of the cloud infrastructure. A general model evaluating the influence between user’s network Action-Reaction-Influence-Model (ARIM) is mentioned in this research. Bartolucci et al. ( 2019 ) researched cryptocurrency prices with the “Butterfly effect”, which means “issues” of the open-source project provides insights to improve prediction of cryptocurrency prices. Sentiment, politeness, emotions analysis of GitHub comments are applied in Ethereum and Bitcoin markets. The results showed that these metrics have predictive power on cryptocurrency prices.
Reinforcement learning
Deep reinforcement algorithms bypass prediction and go straight to market management actions to achieve high cumulated profit (Henderson et al. 2018 ; Liu et al. 2021 ). Bu and Cho ( 2018 ) proposed a combination of double Q-network and unsupervised pre-training using DBM to generate and enhance the optimal Q-function in cryptocurrency trading. The trading model contains agents in series in the form of two neural networks, unsupervised learning modules and environments. The input market state connects an encoding network which includes spectral feature extraction (convolution-pooling module) and temporal feature extraction (LSTM module). A double-Q network follows the encoding network and actions are generated from this network. Compared to existing deep learning models (LSTM, CNN, MLP, etc.), this model achieved the highest profit even facing an extreme market situation (recorded 24% of the profit while cryptocurrency market price drops by \(-64\%\) ). Juchli ( 2018 ) applied two implementations of reinforcement learning agents, a Q-Learning agent, which serves as the learner when no market variables are provided, and a DQN agent which was developed to handle the features previously mentioned. The DQN agent was backtested under the application of two different neural network architectures. The results showed that the DQN-CNN agent (convolutional neural network) is superior to the DQN-MLP agent (multilayer perceptron) in backtesting prediction. Lucarelli and Borrotti ( 2019 ) focused on improving automated cryptocurrency trading with a deep reinforcement learning approach. Double and Dueling double deep Q-learning networks are compared for 4 years. By setting rewards functions as Sharpe ratio and profit, the double Q-learning method demonstrated to be the most profitable approach in trading cryptocurrency. Sattarov et al. ( 2020 ) applied deep reinforcement learning and used historical data from BTC, LTC and ETH to observe historical price movements and acted on real-time prices. The model proposed by the authors helped traders to correctly choose one of the following three actions: buy, sell and hold stocks and get advice on the correct option. Experiments applying BTC via deep reinforcement learning showed that investors made a net profit of 14.4% in one month. Similarly, tests on LTC and ETH ended with 74% and 41% profits respectively. Koker and Koutmos ( 2020 ) pointed out direct reinforcement (DR) based model for active trading. Within the model, the authors attempt to estimate the parameters of the non-linear autoregressive model to achieve maximum risk-adjusted returns. Traders can take long or short positions in each of our sampled cryptocurrency markets, establish or hold them at the end of time interval t , and re-evaluate at the end of \(t+1\) . The results provide some preliminary evidence that cryptocurrency prices may not follow a purely random wandering process.
Atsalakis et al. ( 2019 ) proposes a computational intelligence technique that uses a hybrid Neuro-Fuzzy controller, namely PATSOS, to forecast the direction in the change of the daily price of Bitcoin. The proposed methodology outperforms two other computational intelligence models, the first being developed with a simpler neuro-fuzzy approach, and the second being developed with artificial neural networks. According to the signals of the proposed model, the investment return obtained through trading simulation is 71.21% higher than the investment return obtained through a simple buy and hold strategy. This application is proposed for the first time in the forecasting of Bitcoin price movements. Topological data analysis is applied to forecasting price trends of cryptocurrency markets in Kim et al. ( 2018 ). The approach is to harness topological features of attractors of dynamical systems for arbitrary temporal data. The results showed that the method can effectively separate important topological patterns and sampling noise (like bid-ask bounces, discreteness of price changes, differences in trade sizes or informational content of price changes, etc.) by providing theoretical results. Kurbucz ( 2019 ) designed a complex method consisting of single-hidden layer feedforward neural networks (SLFNs) to (1) determine the predictive power of the most frequent edges of the transaction network (a public ledger that records all Bitcoin transactions) on the future price of Bitcoin; and, (2) to provide an efficient technique for applying this untapped dataset in day trading. The research found a significantly high accuracy (60.05%) for the price movement classifications base on information that can be obtained using a small subset of edges (approximately 0.45% of all unique edges). It is worth noting that, Kondor et al. ( 2014 ), Kondor et al. ( 2014 ) firstly published some papers giving analysis on transaction networks on cryptocurrency markets and applied related research in identifying Bitcoin users (Juhász et al. 2018 ).
Abay et al. ( 2019 ) attempted to understand the network dynamics behind the Blockchain graphs using topological features. The results showed that standard graph features such as the degree distribution of transaction graphs may not be sufficient to capture network dynamics and their potential impact on Bitcoin price fluctuations. Omane-Adjepong et al. ( 2019 ) applied wavelet time-scale persistence in analysing returns and volatility in cryptocurrency markets. The experiment examined the long-memory and market efficiency characteristics in cryptocurrency markets using daily data for more than two years. The authors employed a log-periodogram regression method in researching stationarity in the cryptocurrency market and used ARFIMA-FIGARCH class of models in examining long-memory behaviour of cryptocurrencies across time and scale. In general, experiments indicated that heterogeneous memory behaviour existed in eight cryptocurrency markets using daily data over the full-time period and across scales (August 25, 2015 to March 13, 2018).
Portfolio, cryptocurrency assets and market condition research
Research among cryptocurrency pairs and related factors.
Ji et al. ( 2019 ) examined connectedness via return and volatility spillovers across six large cryptocurrencies (collected from coinmarketcap lists from August 7 2015 to February 22 2018) and found Litecoin and Bitcoin to have the most effect on other cryptocurrencies. The authors followed methods of Diebold and Yılmaz ( 2014 ) and built positive/negative returns and volatility connectedness networks. Furthermore, the regression model is used to identify drivers of various cryptocurrency integration levels. Further analysis revealed that the relationship between each cryptocurrency in terms of return and volatility is not necessarily due to its market size. Omane-Adjepong and Alagidede ( 2019 ) explored market coherence and volatility causal linkages of seven leading cryptocurrencies. Wavelet-based methods are used to examine market connectedness. Parametric and nonparametric tests are employed to investigate directions of volatility spillovers of the assets. Experiments revealed from diversification benefits to linkages of connectedness and volatility in cryptocurrency markets. Bouri et al. ( 2020 ) found the presence of jumps was detected in a series of 12 cryptocurrency returns, and significant jumping activity was found in all cases. More results underscore the importance of the jump in trading volume for the formation of cryptocurrency leapfrogging. Drożdż et al. ( 2020 ) examined the correlation of daily exchange rate fluctuations within a basket of the 100 highest market capitalization cryptocurrencies for the period October 1, 2015 to March 31, 2019. The corresponding dynamics mainly involve one of the leading eigenvalues of the correlation matrix, while the others are mainly consistent with the eigenvalues of the Wishart random matrix. The study shows that Bitcoin (BTC) was dominant during the period under consideration, signalling exchange rate dynamics at least as influential as the US dollar (USD).
Some researchers explored the relationship between cryptocurrency and different factors, including futures, gold, etc. Hale et al. ( 2018 ) suggested that Bitcoin prices rise and fall rapidly after CME issues futures consistent with pricing dynamics. Specifically, the authors pointed out that the rapid rise and subsequent decline in prices after the introduction of futures is consistent with trading behaviour in the cryptocurrency market. Kristjanpoller et al. ( 2020 ) focused on the asymmetric interrelationships between major currencies and cryptocurrencies. The results of multiple fractal asymmetric de-trending cross-correlation analysis show evidence of significant persistence and asymmetric multiplicity in the cross-correlation between most cryptocurrency pairs and ETF pairs. Bai and Robinson ( 2019 ) studied a trading algorithm for foreign exchange on a cryptocurrency Market using the Automated Triangular Arbitrage method. Implementing a pricing strategy, implementing trading algorithms and developing a given trading simulation are three problems solved by this research. Kang et al. ( 2019 ) examined the hedging and diversification properties of gold futures versus Bitcoin prices by using dynamic conditional correlations (DCCs) and wavelet coherence. DCC-GARCH model (Engle 2002 ) is used to estimate the time-varying correlation between Bitcoin and gold futures by modeling the variance and the co-variance but also this two flexibility. Wavelet coherence method focused more on co-movement between Bitcoin and gold futures. From experiments, the wavelet coherence results indicated volatility persistence, causality and phase difference between Bitcoin and gold. Qiao et al. ( 2020 ) used wavelet coherence and relevance networks to investigate synergistic motion between Bitcoin and other cryptocurrencies. The authors then tested the hedging effect of bitcoin on others at different time frequencies by risk reduction and downside risk reduction. The empirical results provide evidence of linkage and hedging effects. Bitcoin’s returns and volatility are ahead of other cryptocurrencies at low frequencies from the analysis, and in the long run, Bitcoin has a more pronounced hedging effect on other cryptocurrencies. Dyhrberg ( 2016 ) applied the GARCH model and the exponential GARCH model in analysing similarities between Bitcoin, gold and the US dollar. The experiments showed that Bitcoin, gold and the US dollar have similarities with the variables of the GARCH model, have similar hedging capabilities and react symmetrically to good and bad news. The authors observed that Bitcoin can combine some advantages of commodities and currencies in financial markets to be a tool for portfolio management.
Baur et al. ( 2018 ) extended the research of Dyhrberg et al.; the same data and sample periods are tested (Dyhrberg 2016 ) with GARCH and EGARCH-(1,1) models but the experiments reached different conclusions. Baur et al. found that Bitcoin has unique risk-return characteristics compared with other assets. They noticed that Bitcoin excess returns and volatility resemble a rather highly speculative asset with respect to gold or the US dollar. Bouri et al. ( 2017 ) studied the relationship between Bitcoin and energy commodities by applying DCCs and GARCH (1,1) models. In particular, the results showed that Bitcoin is a strong hedge and safe haven for energy commodities. Kakushadze ( 2018 ) proposed factor models for the cross-section of daily cryptoasset returns and provided source code for data downloads, computing risk factors and backtesting for all cryptocurrencies and a host of various other digital assets. The results showed that cross-sectional statistical arbitrage trading may be possible for cryptoassets subject to efficient executions and shorting. Beneki et al. ( 2019 ) tested hedging abilities between Bitcoin and Ethereum by a multivariate BEKK-GARCH methodology and impulse response analysis within VAR model. The results indicated a volatility transaction from Ethereum to Bitcoin, which implied possible profitable trading strategies on the cryptocurrency derivatives market. Caporale and Plastun ( 2019 ) examined the week effect in cryptocurrency markets and explored the feasibility of this indicator in trading practice. Student t -test, ANOVA, Kruskal-Wallis and Mann-Whitney tests were carried out for cryptocurrency data in order to compare time periods that may be characterised by anomalies with other time periods. When an anomaly is detected, an algorithm was established to exploit profit opportunities (MetaTrader terminal in MQL4 is mentioned in this research). The results showed evidence of anomaly (abnormal positive returns on Mondays) in the Bitcoin market by backtesting in 2013-2016.
A number of special research methods have proven to be relevant to cryptocurrency pairs, which is reflected in cryptocurrency trading. Delfabbro et al. ( 2021 ) pointed out that cryprocurrency trading have similarities to gambling. Decisions are often based on limited information, short-term profit motives, and highly volatile and uncertain outcomes. The authors examined whether gambling and problem gambling are reliable predictors of reported cryptocurrency trading strength. Results showed that problem gambling scores (PGSI) and engaging in stock trading were significantly correlated with measures of cryptocurrency trading intensity based on time spent per day, number of trades and level of expenditure. In further research, Delfabbro et al. ( 2021 ) reviewed the specific structural features of cryptocurrency trading and its potential to give rise to excessive or harmful behaviour, including over-spending and compulsive checking. There are some similarities noted between online sports betting and day trading, but there are also some important differences. These include the 24/7 nature of trading, the global nature of the market and the powerful role of social media, social influences and non-balance sheet related events as determinants of price movement. Cheng and Yen ( 2020 ) investigated whether the economic policy uncertainty (EPU) index provided by Baker et al. ( 2016 ) can predict the returns of cryptocurrencies. The results suggest that China’s EPU Index can predict monthly returns for Bitcoin, whereas the EPU Index for the US or other Asian countries has no predictive power. In addition, China’s ban on cryptocurrency trading only affects bitcoin returns among major cryptocurrencies. Leirvik ( 2021 ) analysed the relationship between the particular volatility of market liquidity and the returns of the five largest cryptocurrencies by market capitalisation. The results showed that in general there is a positive correlation between the volatility of liquidity and the returns of large-cap cryptocurrencies. For the most liquid and popular cryptocurrencies, this effect does not exist: Bitcoin. Moreover, the liquidity of cryptocurrencies increases over time, but varies greatly over time.
Crypto-asset portfolio research
Some researchers applied portfolio theory for crypto assets. Corbet et al. ( 2019 ) gave a systematic analysis of cryptocurrencies as financial assets. Brauneis and Mestel ( 2019 ) applied the Markowitz mean-variance framework in order to assess the risk-return benefits of cryptocurrency portfolios. In an out-of-sample analysis accounting for transaction cost, they found that combining cryptocurrencies enriches the set of ‘low’-risk cryptocurrency investment opportunities. In terms of the Sharpe ratio and certainty equivalent returns, the 1/ N -portfolio (i.e., “naive” strategies, such as equally dividing amongst asset classes) outperformed single cryptocurrencies and more than 75% in terms of the Sharpe ratio and certainty equivalent returns of mean-variance optimal portfolios. Castro et al. ( 2019 ) developed a portfolio optimisation model based on the Omega measure which is more comprehensive than the Markowitz model and applied this to four crypto-asset investment portfolios by means of a numerical application. Experiments showed crypto-assets improves the return of the portfolios, but on the other hand, also increase the risk exposure.
Bedi and Nashier ( 2020 ) examined diversification capabilities of Bitcoin for a global portfolio spread across six asset classes from the standpoint of investors dealing in five major fiat currencies, namely US Dollar, Great Britain Pound, Euro, Japanese Yen and Chinese Yuan. They employed modified Conditional Value-at-Risk and standard deviation as measures of risk to perform portfolio optimisations across three asset allocation strategies and provided insights into the sharp disparity in Bitcoin trading volumes across national currencies from a portfolio theory perspective. Similar research has been done by Antipova ( 2019 ), which explored the possibility of establishing and optimizing a global portfolio by diversifying investments using one or more cryptocurrencies, and assessing returns to investors in terms of risks and returns. Fantazzini and Zimin ( 2020 ) proposed a set of models that can be used to estimate the market risk for a portfolio of crypto-currencies, and simultaneously estimate their credit risk using the Zero Price Probability (ZPP) model. The results revealed the superiority of the t-copula/skewed-t GARCH model for market risk, and the ZPP-based models for credit risk. Ji et al. ( 2019 ) examined the common dynamics of bitcoin exchanges. Using a connectivity metric based on the actual daily volatility of the bitcoin price, they found that Coinbase is undoubtedly the market leader, while Binance performance is surprisingly weak. The results also suggested that safer asset extraction is more important for volatility linkages between Bitcoin exchanges relative to trading volumes. Fasanya et al. ( 2020 ) quantified returns and volatility transmission between cryptocurrency portfolios by using a spillover approach and rolling sample analysis. The results showed that there is a significant difference between the behaviour of cryptocurrency portfolio returns and the volatility spillover index over time. Given the spillover index, the authors found evidence of interdependence between cryptocurrency portfolios, with the spillover index showing an increased degree of integration between cryptocurrency portfolios.
Trucíos et al. ( 247 ) proposed a methodology based on vine copulas and robust volatility models to estimate the Value-at-Risk (VaR) and Expected Shortfall (ES) of cryptocurrency portfolios. The proposed algorithm displayed good performance in estimating both VaR and ES. Hrytsiuk et al. ( 2019 ) showed that the cryptocurrency returns can be described by the Cauchy distribution and obtained the analytical expressions for VaR risk measures and performed calculations accordingly. As a result of the optimisation, the sets of optimal cryptocurrency portfolios were built in their experiments.
Jiang and Liang ( 2017 ) proposed a two-hidden-layer CNN that takes the historical price of a group of cryptocurrency assets as an input and outputs the weight of the group of cryptocurrency assets. This research focused on portfolio research in cryptocurrency assets using emerging technologies like CNN. Training is conducted in an intensive manner to maximise cumulative returns, which is considered a reward function of the CNN network. The performance of the CNN strategy is compared with the three benchmarks and the other three portfolio management algorithms (buy and hold strategy, Uniform Constant Rebalanced Portfolio and Universal Portfolio with Online Newton Step and Passive Aggressive Mean Reversion); the results are positive in that the model is only second to the Passive Aggressive Mean Reversion algorithm (PAMR). Estalayo et al. ( 2019 ) reported initial findings around the combination of DL models and Multi-Objective Evolutionary Algorithms (MOEAs) for allocating cryptocurrency portfolios. Technical rationale and details were given on the design of a stacked DL recurrent neural network, and how its predictive power can be exploited for yielding accurate ex-ante estimates of the return and risk of the portfolio. Results obtained for a set of experiments carried out with real cryptocurrency data have verified the superior performance of their designed deep learning model with respect to other regression techniques.
Bubbles and Crash Analysis
Bubbles and crash analysis is an important researching area in cryptocurrency trading. Phillips and Yu proposed a methodology to test for the presence of cryptocurrency bubble (Cheung et al. 2015 ), which is extended by Corbet et al. ( 2018 ). The method is based on supremum Augmented Dickey-Fuller (SADF) to test for the bubble through the inclusion of a sequence of forwarding recursive right-tailed ADF unit root tests. An extended methodology generalised SADF (GSAFD), is also tested for bubbles within cryptocurrency data. The research concluded that there is no clear evidence of a persistent bubble in cryptocurrency markets including Bitcoin or Ethereum. Bouri et al. ( 2019 ) date-stamped price explosiveness in seven large cryptocurrencies and revealed evidence of multiple periods of explosivity in all cases. GSADF is used to identify multiple explosiveness periods and logistic regression is employed to uncover evidence of co-explosivity across cryptocurrencies. The results showed that the likelihood of explosive periods in one cryptocurrency generally depends on the presence of explosivity in other cryptocurrencies and points toward a contemporaneous co-explosivity that does not necessarily depend on the size of each cryptocurrency.
Extended research by Phillips et al. ( 2015a , 2015b ) (who applied a recursive augmented Dickey-Fuller algorithm, which is called PSY test) and Enoksen and Landsnes ( 2019 ) studied possible predictors of bubble periods of certain cryptocurrencies. The evaluation includes multiple bubble periods in all cryptocurrencies. The result shows that higher volatility and trading volume is positively associated with the presence of bubbles across cryptocurrencies. In terms of bubble prediction, the authors found the probit model to perform better than the linear models.
Phillips and Gorse ( 2017 ) used Hidden Markov Model (HMM) and Superiority and Inferiority Ranking (SIR) method to identify bubble-like behaviour in cryptocurrency time series. Considering HMM and SIR method, an epidemic detection mechanism is used in social media to predict cryptocurrency price bubbles, which classify bubbles through epidemic and non-epidemic labels. Experiments have demonstrated a strong relationship between Reddit usage and cryptocurrency prices. This work also provides some empirical evidence that bubbles mirror the social epidemic-like spread of an investment idea. Caporale and Plastun ( 2018 ) examined the price overreactions in the case of cryptocurrency trading. Some parametric and non-parametric tests confirmed the presence of price patterns after overreactions, which identified that the next-day price changes in both directions are bigger than after “normal” days. The results also showed that the overreaction detected in the cryptocurrency market would not give available profit opportunities (possibly due to transaction costs) that cannot be considered as evidence of the EMH. Chaim and Laurini ( 2018 ) analysed the high unconditional volatility of cryptocurrency from a standard log-normal stochastic volatility model to discontinuous jumps of volatility and returns. The experiment indicated the importance of incorporating permanent jumps to volatility in cryptocurrency markets.
Cross et al. ( 2021 ) investigated the existence and nature of the interdependence of bitcoin, ethereum, litecoin and ripple during the cryptocurrency bubble of 2017-18. A generalized time-varying asset pricing model approach is proposed. The results showed that the negative news impact of the boom period in 2017 for LiteCoin and Ripple, which incurred a risk premium for investors, could explain the returns of cryptocurrencies during the 2018 crash.
Extreme condition
Differently from traditional fiat currencies, cryptocurrencies are risky and exhibit heavier tail behaviour. Katsiampa et al. ( 2018 ) found extreme dependence between returns and trading volumes. Evidence of asymmetric return-volume relationship in the cryptocurrency market was also found by the experiment, as a result of discrepancies in the correlation between positive and negative return exceedances across all the cryptocurrencies.
There has been a price crash in late 2017 to early 2018 in cryptocurrency (Yaya et al. 2018 ). Yaya et al. ( 2018 ) researched the persistence and dependence of Bitcoin on other popular alternative coins before and after the 2017/18 crash in cryptocurrency markets. The result showed that higher persistence of shocks is expected after the crash due to speculations in the mind of cryptocurrency traders, and more evidence of non-mean reversions, implying chances of further price fall in cryptocurrencies.
Manahov ( 2021 ) obtained millisecond data for major cryptocurrencies as well as the cryptocurrency indices Cryptocurrency IndeX (CRIX) and Cryptocurrencies Index 30 (CCI30) to investigate the relationship between cryptocurrency liquidity, herding behaviour and profitability during extreme price movements (EPM). Millisecond data was obtained for major cryptocurrencies as well as the cryptocurrency indices CRIX and CCI30 to investigate the relationship between cryptocurrency liquidity, herding behaviour and profitability during EPM. The experiments demonstrate that cryptocurrency traders (CTs) can promote EPM and demand liquidity even during periods of maximum EPM. The authors’ robustness checks suggest that herding behaviour follows a dynamic pattern with decreasing magnitude over time. Shahzad et al. ( 2021 ) investigated the interdependence of median-based and tail-based returns between cryptocurrencies under normal and extreme market conditions. The experiment used daily data and combines LASSO techniques with quantile regression within a network analysis framework. The main results showed that the interdependence of the tails is higher than the median, especially in the right tail. Fluctuations in market, size and momentum drive return connectivity and clustering coefficients under both normal and extreme market conditions. Chan et al. ( 2022 ) examined the extreme dependence and correlation between high-frequency cryptocurrency (Bitcoin and Ethereum, relative to the euro and the US dollar) returns and trading volumes in the extreme tails associated with booms and busts in cryptocurrency markets. Experiments with extreme value theory methods highlight how these results can help traders and practitioners who rely on technical indicators in their trading strategies - especially in times of extreme market volatility or irrational market booms.
Others related to Cryptocurrency Trading
Some other research papers related to cryptocurrency trading treat distributed in market behaviour, regulatory mechanisms and benchmarks.
Krafft et al. ( 2018 ) and Yang et al. ( 2018 ) analysed market dynamics and behavioural anomalies respectively to understand effects of market behaviour in the cryptocurrency market. Krafft et al. discussed potential ultimate causes, potential behavioural mechanisms and potential moderating contextual factors to enumerate possible influence of GUI and API on cryptocurrency markets. Then they highlighted the potential social and economic impact of human-computer interaction in digital agency design. Yang, on the other hand, applied behavioural theories of asset pricing anomalies in testing 20 market anomalies using cryptocurrency trading data. The results showed that anomaly research focused more on the role of speculators, which gave a new idea to research the momentum and reversal in the cryptocurrency market. Marchesi ( 2016 ) implemented a mechanism to form a Bitcoin price and specific behaviour for each type of trader including the initial wealth distribution following Pareto’s law, order-based transaction and price settlement mechanism. Specifically, the model reproduced the unit root attributes of the price series, the fat tail phenomenon, the volatility clustering of price returns, the generation of Bitcoins, hashing power and power consumption.
Leclair ( 2018 ) and Vidal-Tomás et al. ( 2019 ) analysed the existence of herding in the cryptocurrency market. Leclair applied herding methods of Hwang and Salmon ( 2004 ) in estimating the market herd dynamics in the CAPM framework. Vidal-Thomás et al. analyse the existence of herds in the cryptocurrency market by returning the cross-sectional standard (absolute) deviations. Both their findings showed significant evidence of market herding in the cryptocurrency market. Makarov and Schoar ( 2020 ) studied price impact and arbitrage dynamics in the cryptocurrency market and found that 85% of the variations in bitcoin returns and the idiosyncratic components of order flow (Liu et al. 2021 ) play an important role in explaining the size of the arbitrage spreads between exchanges. King and Koutmos ( 2021 ) examined the extent to which herding and feedback trading behaviour drive the price dynamics of nine major cryptocurrencies. The study documented heterogeneity in the types of feedback trading strategies used by investors in different markets and evidence of herding or “trend chasing” behaviour in some cryptocurrency markets.
In November 2019, Griffin et al. put forward a paper on the thesis of unsupported digital money inflating cryptocurrency prices (Griffin and Shams 2019 ), which caused a great stir in the academic circle and public opinion. Using algorithms to analyse Blockchain data, they found that purchases with Tether are timed following market downturns and result in sizeable increases in Bitcoin prices. By mapping the blockchains of Bitcoin and Tether, they were able to establish that one large player on Bitfinex uses Tether to purchase large amounts of Bitcoin when prices are falling and following the prod of Tether.
More researches involved benchmark and development in cryptocurrency market (Hileman and Rauchs 2017 ; Zhou and Kalev 2019 ), regulatory framework analysis (Shanaev et al. 2020 ; Feinstein and Werbach 2021 ), data mining technology in cryptocurrency trading (Patil et al. 2018 ), application of efficient market hypothesis in the cryptocurrency market (Sigaki et al. 2019 ), Decentralized Exchanges (DEXs) and artificial financial markets for studying a cryptocurrency market (Cocco et al. 2017 ). Hileman and Rauchs ( 2017 ) segmented the cryptocurrency industry into four key sectors: exchanges, wallets, payments and mining. They gave a benchmarking study of individuals, data, regulation, compliance practices, costs of firms and a global map of mining in the cryptocurrency market in 2017. Zhou and Kalev ( 2019 ) discussed the status and future of computer trading in the largest group of Asia-Pacific economies and then considered algorithmic and high frequency trading in cryptocurrency markets as well. Shanaev et al. ( 2020 ) used data on 120 regulatory events to study the implications of cryptocurrency regulation and the results showed that stricter regulation of cryptocurrency is not desirable. Feinstein and Werbach ( 2021 ) collected raw data on global cryptocurrency regulations and used them to empirically test the trading activity of many exchanges against key regulatory announcements. No systematic evidence has been found that regulatory measures cause traders to flee or enter the affected regional jurisdictions according to authors’ analysis. Patil et al. ( 2018 ) used the average absolute error calculated between the actual and predicted values of the market sentiment of different cryptocurrencies on that day as a method for quantifying the uncertainty. They used the comparison of uncertainty quantification methods and opinion mining to analyse current market conditions. Sigaki et al. ( 2019 ) used permutation entropy and statistical complexity on the sliding time window returned by the price log to quantify the dynamic efficiency of more than four hundred cryptocurrencies. As a result, the cryptocurrency market showed significant compliance with efficient market assumptions. Aspris et al. ( 2021 ) surveyed the rapid rise of DEXs, including automated market makers. The study demonstrated the significant differences in the listing and trading characteristics of these tokens compared to their centralised equivalents. Cocco et al. ( 2017 ) described an agent-based artificial cryptocurrency market in which heterogeneous agents buy or sell cryptocurrencies. The proposed simulator is able to reproduce some real statistical properties of price returns observed in the Bitcoin real market. Marko (Ogorevc 2019 ) considered the future use of cryptocurrencies as money based on the long-term value of cryptocurrencies. Gandal and Halaburda ( 2014 ) analysed the influence of network effect on the competition of new cryptocurrency markets. Bariviera and Merediz-Sola ( 2020 ) gave a survey based on hybrid analysis, which proposed a methodological hybrid method for a comprehensive literature review and provided the latest technology in the cryptocurrency economics literature.
There also exists some research and papers introducing the basic process and rules of cryptocurrency trading including findings of Hansel ( 2018 ), Kate ( 2018 ), Garza ( 2019 ), Ward ( 2018 ) and Fantazzini ( 2019 ). Hansel ( 2018 ) introduced the basics of cryptocurrency, Bitcoin and Blockchain, ways to identify the profitable trends in the market, ways to use Altcoin trading platforms such as GDAX and Coinbase, methods of using a crypto wallet to store and protect the coins in their book. Kate ( 2018 ) set six steps to show how to start an investment without any technical skills in the cryptocurrency market. This book is an entry-level trading manual for starters learning cryptocurrency trading. Garza ( 2019 ) simulated an automatic cryptocurrency trading system, which helps investors limit systemic risks and improve market returns. This paper is an example to start designing an automatic cryptocurrency trading system. Ward ( 2018 ) discussed algorithmic cryptocurrency trading using several general algorithms, and modifications thereof including adjusting the parameters used in each strategy, as well as mixing multiple strategies or dynamically changing between strategies. This paper is an example to start algorithmic trading in cryptocurrency market. Fantazzini ( 2019 ) introduced the R packages Bitcoin-Finance and bubble, including financial analysis of cryptocurrency markets including Bitcoin.
A community resource, that is, a platform for scholarly communication, about cryptocurrencies and Blockchains is “Blockchain research network”, see (research network 2020 ).
Summary Analysis of Literature Review
This section analyses the timeline, the research distribution among technology and methods, the research distribution among properties. It also summarises the datasets that have been used in cryptocurrency trading research.
Figure 8 shows several major events in cryptocurrency trading. The timeline contains milestone events in cryptocurrency trading and important scientific breakthroughs in this area.
As early as 2009, Satoshi Nakamoto proposed and invented the first decentralised cryptocurrency, Nakamoto ( 2009 ). It is considered to be the start of cryptocurrency. In 2010, the first cryptocurrency exchange was founded, which means cryptocurrency would not be an OTC market but traded on exchanges based on an auction market system.
In 2013, Kristoufek ( 2013 ) concluded that there is a strong correlation between Bitcoin price and the frequency of “Bitcoin” search queries in Google Trends and Wikipedia. In 2014, Lee and Yang ( 2014 ) firstly proposed to check causality from copula-based causality in the quantile method from trading volumes of seven major cryptocurrencies to returns and volatility.
In 2015, Cheah and Fry ( 2015 ) discussed the bubble and speculation of Bitcoin and cryptocurrencies. In 2016, Dyhrberg explored Bitcoin volatility using GARCH models combined with gold and US dollars (Dyhrberg 2016 ).
From late 2016 to 2017, machine learning and deep learning technology were applied in the prediction of cryptocurrency return. In 2016, McNally ( 2016 ) predicted Bitcoin price using the LSTM algorithm. Bell ( 2016 ); Żbikowski ( 2016 ) applied SVM algorithm to predict trends of cryptocurrency price. In 2017, Jiang and Liang ( 2017 ) used double Q-network and pre-trained it using DBM for the prediction of cryptocurrencies portfolio weights.
From 2019 to 2020, several research directions including cross asset portfolios (Bedi and Nashier 2020 ; Castro et al. 2019 ; Brauneis and Mestel 2019 ), transaction network applications (Kurbucz 2019 ; Bouri et al. 2019 ), machine learning optimisation (Rane and Dhage 2019 ; Atsalakis et al. 2019 ; Zhengyang et al. 2019 ) have been considered in the cryptocurrency trading area.
In 2021, more regulation issues were put out the stage. On 18 May 2021, China banned financial institutions and payment companies from providing services related to cryptocurrency transactions, which led to a sharp drop in the price of bitcoin (Reuters 2021 ). In June 2021, El Salvador becomes the first country to accept Bitcoin as legal tender (MercoPress 2021 ).
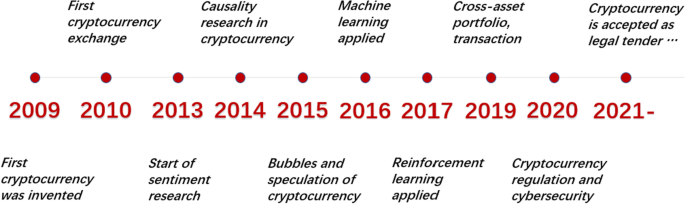
Timeline of cryptocurrency trading research
Research Distribution among Properties
We counted the number of papers covering different aspects of cryptocurrency trading. Figure 9 shows the result. The attributes in the legend are ranked according to the number of papers that specifically test the attribute.
Over one-third (37.67%) of the papers research prediction of returns. Another one-third of papers focus on researching bubbles and extreme conditions and the relationship between pairs and portfolios in cryptocurrency trading. The remaining researching topics (prediction of volatility, trading system, technical trading and others) have roughly one-third share.
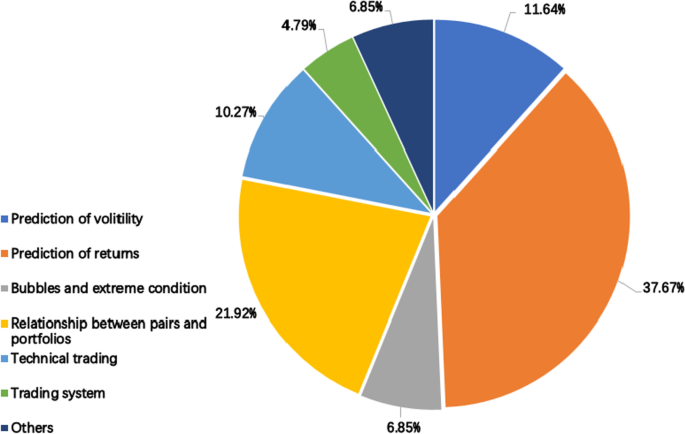
Research distribution among cryptocurrency trading properties
Research Distribution among Categories and Technologies
This section introduces and compares categories and technologies in cryptocurrency trading. When papers cover multiple technologies or compare different methods, we draw statistics from different technical perspectives.
Among all the 146 papers, 102 papers (69.86%) cover statistical methods and machine learning categories. These papers basically research technical-level cryptocurrency trading including mathematical modeling and statistics. Other papers related to trading systems on pure technical indicators and introducing the industry and its history are not included in this analysis. Among all 102 papers, 88 papers (86.28%) present statistical methods and technologies in cryptocurrency trading research and 13.72% papers research machine learning applied to cryptocurrency trading (cf. Fig. 10 ). It is interesting to mention that, there are 17 papers (16.67%) applying and comparing more than one technique in cryptocurrency trading. More specifically, Bach and Kasper ( 2018 ), Alessandretti et al. ( 2018 ), Vo and Yost-Bremm ( 2018 ), Phaladisailoed and Numnonda ( 2018 ), Siaminos ( 2019 ), Rane and Dhage ( 2019 ) used both statistical methods and machine learning methods in cryptocurrency trading.
Table 8 shows the results of search hits in all trading areas (not limited to cryptocurrencies). From the table, we can see that most research findings focused on statistical methods in trading, which means most of the research on traditional markets still focused on using statistical methods for trading. But we observed that machine learning in trading had a higher degree of attention. It might because the traditional technical and fundamental have been arbitraged, so the market has moved in recent years to find new anomalies to exploit. Meanwhile, the results also showed there exist many opportunities for research in the widely studied areas of machine learning applied to trade in cryptocurrency markets (cf. " Opportunities in cryptocurrency trading " section).
Research Distribution among Statistical methods
As from Fig. 10 , we further classified the papers using statistical methods into 6 categories: (i) basic regression methods; (ii) linear classifiers and clustering; (iii) time-series analysis; (iv) decision trees and probabilistic classifiers; (v) modern portfolios theory; and, (vi) Others.
Basic regression methods include regression methods (Linear Regression), function estimation and CGCD method. Linear Classifiers and Clustering include SVM and KNN algorithm. Time-series analysis include GARCH model, BEKK model, ARIMA model, Wavelet time-scale method. Decision Trees and probabilistic classifiers include Boosting Tree, RF model. Modern portfolio theory include Value-at-Risk (VaR) theory, expected-shortfall (ES), Markowitz mean-variance framework. Others include industry, market data and research analysis in cryptocurrency market.
The figure shows that basic Regression methods and time-series analysis are the most commonly used methods in this area.
Research Distribution among Machine Learning Categories
Papers using machine learning account for 13.7 (c.f Fig. 10 ) of the total. We further classified these papers into three categories: (vii) ANNs, (viii) LSTM/RNN/GRUs, and (ix) DL/RL.
The figure also shows that methods based on LSTM, RNN and GRU are the most popular in this subfield.
ANNs contains papers researching ANN applications in cryptocurrency trading such as back propagation (BP) NN. LSTM/RNN/GRUs include papers using neural networks that exploit the temporal structure of the data, a technology especially suitable for time series prediction and financial trading. DL/RL includes papers using Multilayer Neural Networks and Reinforcement Learning. The difference between ANN and DL is that generally, DL refers to an ANN with multiple hidden layers while ANN refers to simple structure neural network contained input layer, hidden layer (one or multiple), and an output layer.
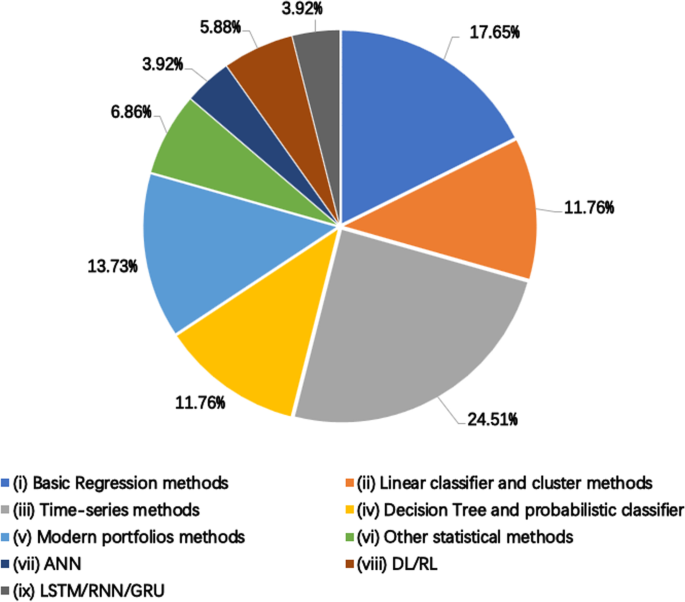
Research distribution among cryptocurrency trading technologies and methods
Datasets used in Cryptocurrency Trading
Tables 9 – 11 show the details for some representative datasets used in cryptocurrency trading research. Table 9 shows the market datasets. They mostly include price, trading volume, order-level information, collected from cryptocurrency exchanges. Table 10 shows the sentiment-based data. Most of the datasets in this table contain market data and media/Internet data with emotional or statistical labels. Table 11 gives two examples of datasets used in the collected papers that are not covered in the first two tables.
The column “Currency” shows the types of cryptocurrencies included; this shows that Bitcoin is the most commonly used currency for cryptocurrency researches. The column “Description” shows a general description and types of datasets. The column “Data Resolution” means latency of the data (e.g., used in the backtest) – this is useful to distinguish between high-frequency trading and low-frequency trading. The column “Time range” shows the time span of datasets used in experiments; this is convenient to distinguish between the current performance in a specific time interval and the long-term effect. We also present how the dataset has been used (i.e., the task), cf. column “Usage”. “Data Sources” gives details on where the data is retrieved from, including cryptocurrency exchanges, aggregated cryptocurrency index and user forums (for sentiment analysis).
Alexander and Dakos ( 2020 ) made an investigation of cryptocurrency data as well. They summarised data collected from 152 published and SSRN discussion papers about cryptocurrencies and analysed their data quality. They found that less than half the cryptocurrency papers published since January 2017 employ correct data.
Opportunities in cryptocurrency trading
This section discusses potential opportunities for future research in cryptocurrency trading.
Sentiment-based research As discussed above, there is a substantial body of work, which uses natural language processing technology, for sentiment analysis with the ultimate goal of using news and media contents to improve the performance of cryptocurrency trading strategies.
Possible research directions may lie in a larger volume of media input (e.g., adding video sources) in sentiment analysis; updating baseline natural language processing model to perform more robust text preprocessing; applying neural networks in label training; extending samples in terms of holding period; transaction-fees; opinion dynamics (Zha et al. 2020 ) and, user reputation research.
Long-and-short term trading research There are significant differences between long and short time horizons in cryptocurrency trading. In long-term trading, investors might obtain greater profits but have more possibilities to control risk when managing a position for weeks or months. It is mandatory to control for risk on long term strategies due to the increase in the holding period, directly proportional to the risk incurred by the trader. On the other hand, the longer the horizon, the higher the risk and the most important the risk control. The shorter the horizon, the higher the cost and the lower the risk, so cost takes over the design of a strategy. In short-term trading, automated algorithmic trading can be applied when holding periods are less than a week. Researchers can differentiate between long-term and short-term trading in cryptocurrency trading by applying wavelet technology analysing bubble regimes (Phillips and Gorse 2018 ) and considering price explosiveness (Bouri et al. 2019 ) hypotheses for short-term and long-term research.
The existing work is mainly about showing the differences between long and short-term cryptocurrency trading. Long-term trading means less time would cost in trend tracing and simple technical indicators in market analysis. Short-term trading can limit overall risk because small positions are used in every transaction. But market noise (interference) and short transaction time might cause some stress in short term trading. It might also be interesting to explore the extraction of trading signals, time series research, application to portfolio management, the relationship between a huge market crash and small price drop, derivative pricing in cryptocurrency market, etc.
Correlation between cryptocurrency and others By the effects of monetary policy and business cycles that are not controlled by the central bank, cryptocurrency is always negatively correlated with overall financial market trends. There have been some studies discussing correlations between cryptocurrencies and other financial markets (Kang et al. 2019 ; Castro et al. 2019 ), which can be used to predict the direction of the cryptocurrency market.
Considering the characteristics of cryptocurrency, the correlation between cryptocurrency and other assets still requires further research. Possible breakthroughs might be achieved with principal component analysis, the relationship between cryptocurrency and other currencies in extreme conditions (i.e., financial collapse).
Bubbles and crash research . To discuss the high volatility and return of cryptocurrencies, current research has focussed on bubbles of cryptocurrency markets (Cheung et al. 2015 ), correlation between cryptocurrency bubbles and indicators like volatility index (VIX) (Enoksen and Landsnes 2019 ) (which is a “panic index” to measure the implied volatility of S&P500 Index Options), spillover effects in cryptocurrency market (Luu Duc Huynh 2019 ).
Additional research for bubbles and crashes in cryptocurrency trading could include a connection between the process of bubble generation and financial collapse and conducting a coherent analysis (coherent process analysis from the formation of bubbles to aftermath analysis of bubble burst), analysis of bubble theory by Microeconomics, trying other physical or industrial models in analysing bubbles in cryptocurrency market (i.e., Omori law (Weber et al. 2007 )), discussing the supply and demand relationship of cryptocurrency in bubble analysis (like using supply and demand graph to simulate the generation of bubbles and simulate the bubble burst).
Game theory and agent-based analysis Applying game theory or agent-based modelling in trading is a hot research direction in the traditional financial market. It might also be interesting to apply this method to trading in cryptocurrency markets.
Public nature of Blockchain technology Investigations on the connections between the formation of a given currency’s transaction network and its price has increased rapidly in recent years; the growing attention on user identification (Juhász et al. 2018 ) also strongly supports this direction. With an in-depth understanding of these networks, we may identify new features in price prediction and may be closer to understanding financial bubbles in cryptocurrency trading.
Balance between the opening of trading research literature and the fading of alphas Mclean et al. McLean and Pontiff ( 2016 ) pointed out that investors learn about mispricing in stock markets from academic publications. Similarly, cryptocurrency market predictability could also be affected by research papers in the area. A possible attempt is to try new pricing methods applying real-time market changes. Considering the proportion of informed traders increasing in the cryptocurrency market in the pricing process is another breaking point (looking for a balance between alpha trading and trading research literature).
Conclusions
We provided a comprehensive overview and analysis of the research work on cryptocurrency trading. This survey presented a nomenclature of the definitions and current state of the art. The paper provides a comprehensive survey of 146 cryptocurrency trading papers and analyses the research distribution that characterise the cryptocurrency trading literature. Research distribution among properties and categories/technologies are analysed in this survey respectively. We further summarised the datasets used for experiments and analysed the research trends and opportunities in cryptocurrency trading. Future research directions and opportunities are discussed in " Opportunities in cryptocurrency trading " section.
We expect this survey to be beneficial to academics (e.g., finance researchers) and quantitative traders alike. The survey represents a quick way to get familiar with the literature on cryptocurrency trading and can motivate more researchers to contribute to the pressing problems in the area, for example along the lines we have identified.
Availability of data and materials
The datasets used and/or analyzed during the current study are available from the corresponding author upon request. Some datasets generated and/or analyzed during the current study are available in Google Scholar, arXiv and SSRN.
3commas: 3Commas Smart Trading terminal and auto trading bots. https://3commas.io/ . [Online, Accessed 26 Jan 2020] (2020)
Abay NC, Akcora CG, Gel YR, Kantarcioglu M, Islambekov UD, Tian Y, Thuraisingham B (2019) Chainnet: Learning on blockchain graphs with topological features. In: 2019 IEEE International Conference on Data Mining (ICDM), pp 946–951. IEEE
Adeyanju, C.: What crypto exchanges do to comply with KYC, AML and CFT regulations. https://cointelegraph.com/news/what-crypto-exchanges-do-to-comply-with-kyc-aml-and-cft-regulations . [Online, Accessed January 11, 2020] (2019)
Ahamad S, Nair M, Varghese B (2013) A survey on crypto currencies. In: 4th International Conference on Advances in Computer Science, AETACS, pp. 42–48. Citeseer
Alessandretti L, ElBahrawy A, Aiello LM, Baronchelli A (2018) Anticipating cryptocurrency prices using machine learning. Complexity
Alexander C, Dakos M (2020) A critical investigation of cryptocurrency data and analysis. Quantitative Finance 20(2):173–188
Google Scholar
Al-Yahyaee KH, Mensi W, Ko H-U, Yoon S-M, Kang SH (2020) Why cryptocurrency markets are inefficient: The impact of liquidity and volatility. The North American Journal of Economics and Finance 52:101168
Antipova V (2019) Building and testing global investment portfolios using alternative asset classes. Master’s thesis, Vytautas Magnus University, Lithuania
Ardia D, Bluteau K, Rüede M (2019) Regime changes in bitcoin garch volatility dynamics. Financ Res Lett 29:266–271
Aspris A, Foley S, Svec J, Wang L (2021) Decentralized exchanges: The “wild west’’ of cryptocurrency trading. Int Rev Financ Anal 77:101845
Atsalakis GS, Atsalaki IG, Pasiouras F, Zopounidis C (2019) Bitcoin price forecasting with neuro-fuzzy techniques. Eur J Oper Res 276(2):770–780
Attanasio G, Cagliero L, Garza P, Baralis E (2019) Quantitative cryptocurrency trading: exploring the use of machine learning techniques. In: Proceedings of the 5th Workshop on Data Science for Macro-modeling with Financial and Economic Datasets, p. 1. ACM
Authority, F.C.: Contract for differences. https://www.fca.org.uk/firms/contracts-for-difference . [Online, Accessed January 29, 2020] (2019)
Bach WG, Kasper L (2018) On machine learning based cryptocurrency trading. Master’s thesis, Aalborg University, Denmark
Badenhorst JJ et al (2019) Effect of bitcoin spot and derivative trading volumes on price volatility. PhD thesis, University of Pretoria
Bai S, Robinson F (2019) Automated triangular arbitrage:: A trading algorithm for foreign exchange on a cryptocurrency market. Bachelor’s thesis, KTH Royal Institute of Technology, Sweden
Baker SR, Bloom N, Davis SJ (2016) Measuring economic policy uncertainty. Q J Econ 131(4):1593–1636
BAKKT: BAKKT markets. https://www.bakkt.com/index . [Online, Accessed February 11, 2020] (2020)
BAKKT: BAKKT terms of use. https://www.bakkt.com/terms-of-use . [Online, Accessed 11 Feb 2020] (2020)
Bariviera AF, Merediz-Sola I (2020) Where do we stand in cryptocurrencies economic research? a survey based on hybrid analysis. arXiv preprint arXiv:2003.09723
Barnwal A, Bharti H, Ali A, Singh V (2019) Stacking with neural network for cryptocurrency investment. arXiv preprint arXiv:1902.07855
Bartolucci S, Destefanis G, Ortu M, Uras N, Marchesi M, Tonelli R (2019) The butterfly affect: impact of development practices on cryptocurrency prices
Baur DG, Dimpfl T, Kuck K (2018) Bitcoin, gold and the us dollar-a replication and extension. Financ Res Lett 25:103–110
Bauriya A, Tikone A, Nandgaonkar P, Sakure KS (2019) Real-time cryptocurrency trading system. Int Res J Eng Technol (IRJET) 06
Bedi P, Nashier T (2020) On the investment credentials of bitcoin: a cross-currency perspective. Res Int Bus Financ 51:101087
Bell T (2016) Bitcoin trading agents. University of Southampton
Ben-Akiva M, McFadden D, Train K, Walker J, Bhat C, Bierlaire M, Bolduc D, Boersch-Supan A, Brownstone D, Bunch DS et al (2002) Hybrid choice models: progress and challenges. Mark Lett 13(3):163–175
Beneki C, Koulis A, Kyriazis NA, Papadamou S (2019) Investigating volatility transmission and hedging properties between bitcoin and ethereum. Res Int Bus Financ 48:219–227
Binance: Binance Partners with Coinfirm to Protect the Global Cryptocurrency Economy and Ensure Compliance with FATF AML Rules. https://www.binance.com/en/blog/386484403820867584/Binance-Partners-with-Coinfirm-to-Protect-the-Global-Cryptocurrency-Economy-and-Ensure-Compliance-with-FATF-AML-Rules . [Online, Accessed February 11, 2020] (2020)
Binance: Binance Review 2020: Pros, Cons, Fees, Features, and Safety. https://insidebitcoins.com/cryptocurrency-exchanges/binance-review/ . [Online, Accessed 11 Feb 2020] (2020)
Bitfinex: Bitfinex markets. https://www.bitfinex.com/ . [Online, Accessed 11 Feb 2020] (2020)
Bitfinex: Bitfinex terms of service. https://www.bitfinex.com/legal/terms . [Online, Accessed 11 Feb 2020] (2020)
Bitfinex: New York Court Rules That State Attorney Has Jurisdiction Over Bitfinex. https://cointelegraph.com/news/new-york-court-rules-that-state-attorney-has-jurisdiction-over-bitfinex . [Online, Accessed February 11, 2020] (2020)
Bitmex: Beginner’s Guide to BitMEX: Complete Review. https://blockonomi.com/bitmex-review/ . [Online, Accessed 11 Feb 2020] (2020)
Bitmex: Bitmex. https://www.bitmex.com/register . [Online, Accessed 11 Feb 2020] (2020)
Bitstamp: Bitstamp Review 2020. https://www.fxempire.com/crypto/exchange/bitstamp/review . [Online, Accessed 11 Feb 2020] (2020)
Bitstamp: Bitstamp who we are. https://www.bitstamp.net/about-us/ . [Online, Accessed 11 Feb 2020] (2020)
Bitstamp: Terms of Use. https://www.bitstamp.net/terms-of-use/sa . [Online, Accessed February 11, 2020] (2020)
Blackbird: Blackbird Bitcoin Arbitrage: a long/short market-neutral strategy. https://github.com/butor/blackbird . [Online, Accessed 26 Jan 2020] (2020)
Bloomberg: Coinbase Inc. https://www.bloomberg.com/profile/company/0776164D:US . [Online, Accessed 11 Feb 2020] (2020)
Bodart V, Candelon B (2009) Evidence of interdependence and contagion using a frequency domain framework. Emerg Mark Rev 10(2):140–150
Bouri E, Jalkh N, Molnár P, Roubaud D (2017) Bitcoin for energy commodities before and after the December 2013 crash: diversifier, hedge or safe haven? Appl Econ 49(50):5063–5073
Bouri E, Lau CKM, Lucey B, Roubaud D (2019) Trading volume and the predictability of return and volatility in the cryptocurrency market. Financ Res Lett 29:340–346
Bouri E, Shahzad SJH, Roubaud D (2019) Co-explosivity in the cryptocurrency market. Financ Res Lett 29:178–183
Bouri E, Lucey B, Roubaud D (2020) The volatility surprise of leading cryptocurrencies: Transitory and permanent linkages. Financ Res Lett 33:101188
Bouri E, Roubaud D, Shahzad SJH (2020) Do bitcoin and other cryptocurrencies jump together? Q Rev Econ Finance 76:396–409
Bouri E, Gupta R (2019) Predicting bitcoin returns: Comparing the roles of newspaper-and internet search-based measures of uncertainty. Finance Res Lett 101398
Bouri E, Vo XV, Saeed T (2020) Return equicorrelation in the cryptocurrency market: analysis and determinants. Finance Research Letters, 101497
Brauneis A, Mestel R (2019) Cryptocurrency-portfolios in a mean-variance framework. Financ Res Lett 28:259–264
Brunnermeier MK, Oehmke M (2013) Bubbles, financial crises, and systemic risk. In: Handbook of the Economics of Finance vol. 2, pp 1221–1288. Elsevier
Bu S-J, Cho S-B (2018) Learning optimal q-function using deep boltzmann machine for reliable trading of cryptocurrency. In: International Conference on Intelligent Data Engineering and Automated Learning, pp 468–480. Springer
Calo B, Johnson W (2002) Global trading system. Google Patents. US Patent App. 09/769,036
Capfolio: Capfolio cryptocurrency trading platform. https://www.capfol.io/ . [Online, Accessed 26 Jan 2020] (2020)
Caporale GM, Plastun A (2018) Price overreactions in the cryptocurrency market. CESifo Working Paper 6861, Munich. http://hdl.handle.net/10419/174984
Caporale GM, Plastun A (2019) The day of the week effect in the cryptocurrency market. Finance Res Lett 31
Caporale GM, Gil-Alana L, Plastun A (2018) Persistence in the cryptocurrency market. Res Int Bus Financ 46:141–148
Caporin M, McAleer M (2012) Do we really need both bekk and dcc? a tale of two multivariate garch models. J Econ Surv 26(4):736–751
Castro JG, Tito EAH, Brandão LET, Gomes LL (2019) Crypto-assets portfolio optimization under the omega measure. Eng Econom 1–21
Catalyst: An Algorithmic Trading Library for Crypto-Assets in Python. https://github.com/enigmampc/catalyst . [Online, Accessed 26 Jan 2020] (2020)
CBOE: CBOE history. http://www.cboe.com/aboutcboe/history . [Online, Accessed 11 Feb 2020] (2020)
CBOE: CBOE products. https://www.cboe.com . [Online, Accessed 11 Feb 2020] (2020)
CBOE: CFE Regulation. https://www.cboe.com/aboutcboe/legal-regulatory/departmental-overviews/cfe-regulation . [Online, Accessed February 11, 2020] (2020)
Ccxt: CCXT - CryptoCurrency eXchange Trading Library. https://github.com/ccxt/ccxt . [Online, Accessed 26 Jan 2020] (2020)
Chaim P, Laurini MP (2018) Volatility and return jumps in bitcoin. Econ Lett 173:158–163
Chaim P, Laurini MP (2019) Nonlinear dependence in cryptocurrency markets. N Am J Econ Finance 48:32–47
Chan S, Chu J, Zhang Y, Nadarajah S (2022) An extreme value analysis of the tail relationships between returns and volumes for high frequency cryptocurrencies. Res Int Bus Financ 59:101541
Chang C-C, Hsieh P-F, Wang Y-H (2015) Sophistication, sentiment, and misreaction. J Financ Quant Anal 50(4):903–928
Charles A, Darné O et al (2019) Volatility estimation for cryptocurrencies: further evidence with jumps and structural breaks. Econ Bull 39(2):954–968
Cheah E-T, Fry J (2015) Speculative bubbles in bitcoin markets? an empirical investigation into the fundamental value of bitcoin. Econ Lett 130:32–36
Cheng H-P, Yen K-C (2020) The relationship between the economic policy uncertainty and the cryptocurrency market. Financ Res Lett 35:101308
Cheng J, Dong L, Lapata M (2016) Long short-term memory-networks for machine reading. arXiv preprint arXiv:1601.06733
Cheung A, Roca E, Su J-J (2015) Crypto-currency bubbles: an application of the phillips–shi–yu (2013) methodology on mt. gox bitcoin prices. Appl Econ 47(23):2348–2358
Choi B (2012) ARMA model identification. Springer, New York
Chung J, Gulcehre C, Cho K, Bengio Y (2014) Empirical evaluation of gated recurrent neural networks on sequence modeling. arXiv preprint arXiv:1412.3555
CME: CME Cryptocurrency products. https://www.cmegroup.com/trading/cryptocurrency-indices.html . [Online, Accessed 11 Feb 2020] (2020)
CME: CME Group Rules and Regulation Overview. https://www.cmegroup.com/education/courses/market-regulation/overview/cme-group-rules-and-regulation-overview.html . [Online, Accessed February 11, 2020] (2020)
CME: CME groups overview. https://www.cmegroup.com/company/history/ . [Online, Accessed 11 Feb 2020] (2020)
Cocco L, Concas G, Marchesi M (2017) Using an artificial financial market for studying a cryptocurrency market. J Econ Interac Coord 12(2):345–365
Cocco L, Marchesi M (2016) Modeling and simulation of the economics of mining in the bitcoin market. PloS one 11(10)
Coinbase: Coinbase Supported cryptocurrencies. https://help.coinbase.com/en/coinbase/getting-started/general-crypto-education/supported-cryptocurrencies.html . [Online, Accessed 11 Feb 2020] (2020)
Coinbase: Our path to listing SEC-regulated crypto securities. https://blog.coinbase.com/our-path-to-listing-sec-regulated-crypto-securities-a1724e13bb5a . [Online, Accessed February 11, 2020] (2020)
CoinMaketCap: Top 100 cryptocurrencies by market capitalization (2019). Accessed 20 Dec 2019
Coinmarketcap: Percentage of Total Market Capitalization. https://coinmarketcap.com/charts/#dominance-percentage . [Online, Accessed January 11, 2020] (2020)
Colianni S, Rosales S, Signorotti M (2015) Algorithmic trading of cryptocurrency based on twitter sentiment analysis. CS229 Project, 1–5
Conrad C, Custovic A, Ghysels E (2018) Long-and short-term cryptocurrency volatility components: A garch-midas analysis. J Risk Financ Manag 11(2):23
Corbet S, Meegan A, Larkin C, Lucey B, Yarovaya L (2018) Exploring the dynamic relationships between cryptocurrencies and other financial assets. Econ Lett 165:28–34
Corbet S, Lucey B, Yarovaya L (2018) Datestamping the bitcoin and ethereum bubbles. Financ Res Lett 26:81–88
Corbet S, Eraslan V, Lucey B, Sensoy A (2019) The effectiveness of technical trading rules in cryptocurrency markets. Financ Res Lett 31:32–37
Corbet S, Lucey B, Urquhart A, Yarovaya L (2019) Cryptocurrencies as a financial asset: a systematic analysis. Int Rev Financ Anal 62:182–199
Council FB (2021) The Main Roadblocks To Crypto Moving Mainstream. https://www.forbes.com/sites/forbesbusinesscouncil/2021/06/23/the-main-roadblocks-to-crypto-moving-mainstream/?sh=2e629de922b9 . [Online, Accessed: June 23, 2021]
Cross JL, Hou C, Trinh K (2021) Returns, volatility and the cryptocurrency bubble of 2017-18. Econ Model 105643
Cryptosignal: Automated Crypto Trading and Technical Analysis (TA) Bot. https://github.com/CryptoSignal/crypto-signal . [Online, Accessed 26 Jan 2020] (2020)
Ctubio: Ctubio - Cryptocurrency trading bot. https://github.com/ctubio/Krypto-trading-bot . [Online, Accessed 26 Jan 2020] (2020)
Delfabbro P, King D, Williams J, Georgiou N (2021) Cryptocurrency trading, gambling and problem gambling. Addict Behav 122:107021
Delfabbro P, King DL, Williams J (2021) The psychology of cryptocurrency trading: Risk and protective factors. J Behav Addict
Diebold FX, Yılmaz K (2014) On the network topology of variance decompositions: measuring the connectedness of financial firms. J Econom 182(1):119–134
Domínguez MA, Lobato IN (2003) Testing the martingale difference hypothesis. Econom Rev 22(4):351–377
Doran MD (2014) A forensic look at bitcoin cryptocurrency. PhD thesis, Utica College
Drożdż S, Minati L, Oświecimka P, Stanuszek M, Watorek M (2020) Competition of noise and collectivity in global cryptocurrency trading: route to a self-contained market. Chaos: Interdiscip J Nonlinear Sci 30(2):023122
Dutta A, Kumar S, Basu M (2020) A gated recurrent unit approach to bitcoin price prediction. J Risk Financ Manag 13(2):23
Dyhrberg AH (2016) Bitcoin, gold and the dollar-a garch volatility analysis. Financ Res Lett 16:85–92
Easley D, Engle RF, O’Hara M, Wu L (2008) Time-varying arrival rates of informed and uninformed trades. J Financ Econom 6(2):171–207
Elliott RJ, Van Der Hoek* J, Malcolm WP (2005) Pairs trading. Quant Finance 5(3):271–276
Engle R (2002) Dynamic conditional correlation: a simple class of multivariate generalized autoregressive conditional heteroskedasticity models. J Bus Econ Stat 20(3):339–350
Engle RF, Kroner KF (1995) Multivariate simultaneous generalized arch. Econom Theor 11(1):122–150
Enoksen FA, Landsnes CJ (2019) What can predict bubbles in cryptocurrency prices? Master’s thesis, University of Stavanger, Norway
Escanciano JC, Velasco C (2006) Generalized spectral tests for the martingale difference hypothesis. J Econom 134(1):151–185
Estalayo I, Del Ser J, Osaba E, Bilbao MN, Muhammad K, Gálvez A, Iglesias A (2019) Return, diversification and risk in cryptocurrency portfolios using deep recurrent neural networks and multi-objective evolutionary algorithms. In: 2019 IEEE Congress on Evolutionary Computation (CEC), pp 755–761. IEEE
Fang F, Chung W, Ventre C, Basios M, Kanthan L, Li L, Wu F (2021) Ascertaining price formation in cryptocurrency markets with machine learning. Eur J Finance 1–23
Fang F, Ventre C, Li L, Kanthan L, Wu F, Basios M (2020) Better model selection with a new definition of feature importance. arXiv preprint arXiv:2009.07708
Fantazzini D (2019) Quantitative finance with r and cryptocurrencies. Amazon KDP, ISBN-13, 978–1090685315
Fantazzini D, Zimin S (2020) A multivariate approach for the simultaneous modelling of market risk and credit risk for cryptocurrencies. J Ind Bus Econ 47(1):19–69
Farell R (2015) An analysis of the cryptocurrency industry
Fasanya IO, Oyewole O, Odudu T (2020) Returns and volatility spillovers among cryptocurrency portfolios. Int J Manag Finance
Feinstein BD, Werbach K (2021) The impact of cryptocurrency regulation on trading markets. Journal of Financial Regulation 7(1):48–99
Feng W, Wang Y, Zhang Z (2018) Informed trading in the bitcoin market. Financ Res Lett 26:63–70
Fil M (2019) Pairs trading in cryptocurrency markets. Univerzita Karlova, Fakulta sociálních věd
Flood R, Hodrick RJ, Kaplan P (1986) An evaluation of recent evidence on stock market bubbles. National Bureau of Economic Research Cambridge Mass, USA
Flori A (2019) News and subjective beliefs: a bayesian approach to bitcoin investments. Res Int Bus Financ 50:336–356
Forbes: Here’s What Caused Bitcoin’s ‘Extreme’ Price Plunge. https://www.forbes.com/sites/billybambrough/2020/03/19/major-bitcoin-exchange-bitmex-has-a-serious-problem/?sh=1be57a0d4f7d . [Online, Accessed: March 19, 2020] (2021)
Forbes: Is The Crypto Market Maturing? An Analysis For Entrepreneurs. https://www.forbes.com/sites/theyec/2021/06/01/is-the-crypto-market-maturing-an-analysis-for-entrepreneurs/?sh=1170160bba22 . [Online, Accessed: June 1, 2021] (2021)
Forbes: More Than \$600 Million Stolen In Ethereum And Other Cryptocurrencies-Marking One Of Crypto’s Biggest Hacks Ever. https://www.forbes.com/sites/jonathanponciano/2021/08/10/more-than-600-million-stolen-in-ethereum-and-other-cryptocurrencies-marking-one-of-cryptos-biggest-hacks-ever/?sh=502ce7387f62 . [Online, Accessed: August 10, 2021] (2021)
Forbes: Why Buffett Sees Bitcoin Bubble. https://www.forbes.com/sites/johnwasik/2017/11/06/why-buffett-sees-bitcoin-bubble/?sh=196c2a8062a8 . [Online, Accessed: November 6, 2017] (2017)
Fretrade: Freqtrade. https://github.com/freqtrade/freqtrade . [Online, Accessed 26 Jan 2020] (2020)
Friedl MA, Brodley CE (1997) Decision tree classification of land cover from remotely sensed data. Remote Sens Environ 61(3):399–409
Friedman JH (2001) Greedy function approximation: a gradient boosting machine. Annals of statistics, 1189–1232
Friedman J, Tibshirani R (1984) The monotone smoothing of scatterplots. Technometrics 26(3):243–250
FT: Bitcoin: too good to miss or a bubble ready to burst? https://www.ft.com/crypto/ . [Online, Accessed: November 9, 2021] (2021)
Gandal N, Halaburda H (2014) Competition in the cryptocurrency market. CEPR Discussion Paper No. DP10157
Garcia D, Schweitzer F (2015) Social signals and algorithmic trading of bitcoin. R Soc Open Sci 2(9):150288
Garza P (2019) Formal automatic trading in the cryptocurrency era. PhD thesis, Politecnico di Torino
Gatev E, Goetzmann WN, Rouwenhorst KG (2006) Pairs trading: performance of a relative-value arbitrage rule. Rev Financ Stud 19(3):797–827
Gerritsen DF, Bouri E, Ramezanifar E, Roubaud D (2019) The profitability of technical trading rules in the bitcoin market. Finance Res Lett
Golang: A golang implementation of a console-based trading bot for cryptocurrency exchanges. https://github.com/saniales/golang-crypto-trading-bot . [Online, Accessed 26 Jan 2020] (2020)
Gradojevic N, Tsiakas I (2021) Volatility cascades in cryptocurrency trading. J Empir Financ 62:252–265
Grayblock: Elliptic-Curve Cryptography. https://medium.com/coinmonks/elliptic-curve-cryptography-6de8fc748b8b . [Online, Accessed December 29, 2019] (2018)
Griffin JM, Shams A (2019) Is bitcoin really un-tethered? Available at SSRN 3195066
Grobys K, Ahmed S, Sapkota N (2020) Technical trading rules in the cryptocurrency market. Financ Res Lett 32:101396
Gu S, Lillicrap T, Sutskever I, Levine S (2016) Continuous deep q-learning with model-based acceleration. In: International Conference on Machine Learning, pp 2829–2838
Guo T, Bifet A, Antulov-Fantulin N (2018) Bitcoin volatility forecasting with a glimpse into buy and sell orders. In: 2018 IEEE International Conference on Data Mining (ICDM), pp. 989–994. IEEE
Ha S, Moon B-R (2018) Finding attractive technical patterns in cryptocurrency markets. Memetic Comput 10(3):301–306
Hale G, Krishnamurthy A, Kudlyak M, Shultz P et al (2018) How futures trading changed bitcoin prices. FRBSF Econ Lett 12
Hansel D (2018) Cryptocurrency trading: How to make money by trading bitcoin and other cryptocurrency (volume 2)
Harwick C (2016) Cryptocurrency and the problem of intermediation. Independ Rev 20(4):569–588
Henderson P, Islam R, Bachman P, Pineau J, Precup D, Meger D (2018) Deep reinforcement learning that matters. In: Thirty-Second AAAI Conference on Artificial Intelligence
Hileman G, Rauchs M (2017) Global cryptocurrency benchmarking study. Cambridge Centre for Alternative Finance 33
Holmes G, Donkin A, Witten IH (1994) Weka: A machine learning workbench. In: Proceedings of ANZIIS’94-Australian New Zealand Intelligent Information Systems Conference, pp 357–361. IEEE
Hrytsiuk P, Babych T, Bachyshyna L (2019) Cryptocurrency portfolio optimization using value-at-risk measure. In: Strategies, Models and Technologies of Economic Systems Management (SMTESM 2019). Atlantis Press
Huan Y, Wu F, Basios M, Kanthan L, Li L, Xu B (2020) Ieo: Intelligent evolutionary optimisation for hyperparameter tuning. arXiv preprint arXiv:2009.06390
Hudson R, Urquhart A (2019) Technical analysis and cryptocurrencies. Available at SSRN 3387950
Hultman H (2018) Volatility forecasting an empirical study on bitcoin using garch and stochastic volatility models. Master’s thesis, Lund University, Sweden
Hutto CJ, Gilbert E (2014) Vader: A parsimonious rule-based model for sentiment analysis of social media text. In: Eighth International AAAI Conference on Weblogs and Social Media
Hwang S, Salmon M (2004) Market stress and herding. J Empir Financ 11(4):585–616
IntelliPaat: Supervised Learning vs Unsupervised Learning vs Reinforcement Learning. https://intellipaat.com/blog/supervised-learning-vs-unsupervised-learning-vs-reinforcement-learning/ . [Online, Accessed: September 14, 2021] (2021)
Ji Q, Bouri E, Lau CKM, Roubaud D (2019) Dynamic connectedness and integration in cryptocurrency markets. Int Rev Financ Anal 63:257–272
Jiang Z, Liang J (2017) Cryptocurrency portfolio management with deep reinforcement learning. In: 2017 Intelligent Systems Conference (IntelliSys), pp 905–913. IEEE
Jianliang M, Haikun S, Ling B (2009) The application on intrusion detection based on k-means cluster algorithm. In: 2009 International Forum on Information Technology and Applications, vol 1, pp 150–152. IEEE
Ji Q, Bouri E, Kristoufek L, Lucey B (2019) Realised volatility connectedness among bitcoin exchange markets. Finance Res Lett 101391
Johar S (2018) How do blockchain mining and transactions work explained in 7 simple steps. https://blog.goodaudience.com/how-a-miner-adds-transactions-to-the-blockchain-in-seven-steps-856053271476 . [Online, Accessed 26 Jan 2020]
Juchli M (2018) Limit order placement optimization with deep reinforcement learning: Learning from patterns in cryptocurrency market data. Master’s thesis, TU Delft Electrical Engineering, Netherlands
Juhász PL, Stéger J, Kondor D, Vattay G (2018) A bayesian approach to identify bitcoin users. PloS one 13(12)
Kaal WA (2020) Digital asset market evolution. J Corp Law 20–02
Kajtazi A, Moro A (2019) The role of bitcoin in well diversified portfolios: a comparative global study. Int Rev Financ Anal 61:143–157
Kakushadze Z (2018) Cryptoasset factor models. Algorithmic Finance (Preprint), 1–18
Kakushadze Z (2016) 101 formulaic alphas. Wilmott 2016(84):72–81
Kalchbrenner N, Grefenstette E, Blunsom P (2014) A convolutional neural network for modelling sentences. arXiv preprint arXiv:1404.2188
Kamrat S, Suesangiamsakul N, Marukatat R (2018) Technical analysis for cryptocurrency trading on mobile phones. In: 2018 3rd Technology Innovation Management and Engineering Science International Conference (TIMES-iCON), pp. 1–4 . IEEE
Kang SH, McIver RP, Hernandez JA (2019) Co-movements between bitcoin and gold: A wavelet coherence analysis. Physica A: Stat Mech Appl 120888
Kang K, Choo J, Kim Y (2019) Whose opinion matters? analyzing relationships between bitcoin prices and user groups in online community. Social Science Computer Review, 0894439319840716
Kat HM, Heynen RC (1994) Volatility prediction: A comparison of the stochastic volatility, garch (1, 1) and egarch (1, 1) models. J Derivat 2(2)
Kate C (2018) Cryptocurrency trading for beginners: 6-steps action plan to your first investment
Katsiampa P (2019) An empirical investigation of volatility dynamics in the cryptocurrency market. Res Int Bus Finance
Katsiampa P, Corbet S, Lucey BM (2018) Volatility spillover effects in leading cryptocurrencies: A bekk-mgarch analysis. Available at SSRN 3232912
Katsiampa P, Gkillas K, Longin F (2018) Cryptocurrency market activity during extremely volatile periods. https://doi.org/10.2139/ssrn.3220781
Kaufman PJ (2013) Trading Systems and Methods,+ Website, vol 591. Wiley, Hoboken
Keerthi SS, Shevade SK, Bhattacharyya C, Murthy KRK (2001) Improvements to Platt’s smo algorithm for svm classifier design. Neural Comput 13(3):637–649
Khuntia S, Pattanayak J (2018) Adaptive market hypothesis and evolving predictability of bitcoin. Econ Lett 167:26–28
Kim YB, Kim JG, Kim W, Im JH, Kim TH, Kang SJ, Kim CH (2016) Predicting fluctuations in cryptocurrency transactions based on user comments and replies. PLoS ONE 11(8):0161197
Kim K, Kim J, Rinaldo A (2018) Time series featurization via topological data analysis: an application to cryptocurrency trend forecasting. arXiv preprint arXiv:1812.02987
King T, Koutmos D (2021) Herding and feedback trading in cryptocurrency markets. Ann Oper Res 300(1):79–96
Koker TE, Koutmos D (2020) Cryptocurrency trading using machine learning. J Risk Financ Manag 13(8):178
Kondor D, Csabai I, Szüle J, Pósfai M, Vattay G (2014) Inferring the interplay between network structure and market effects in bitcoin. New J Phys 16(12):125003
Kondor D, Csabai I, Szule J, Psfai M, Vattay G (2014) Inferring the interplay between network structure and market effects in bitcoin. New J Phys 16(12):125003. https://doi.org/10.1088/1367-2630/16/12/125003
Article Google Scholar
Kondor D, Pósfai M, Csabai I, Vattay G (2014) Do the rich get richer? An empirical analysis of the bitcoin transaction network. PloS one 9(2):
Kou G, Peng Y, Wang G (2014) Evaluation of clustering algorithms for financial risk analysis using mcdm methods. Inf Sci 275:1–12
Kou G, Akdeniz ÖO, Dinçer H, Yüksel S (2021) Fintech investments in European banks: a hybrid it2 fuzzy multidimensional decision-making approach. Financ Innov 7(1):1–28
Kou G, Xu Y, Peng Y, Shen F, Chen Y, Chang K, Kou S (2021) Bankruptcy prediction for smes using transactional data and two-stage multiobjective feature selection. Decis Support Syst 140:113429
Krafft PM, Della Penna N, Pentland AS (2018) An experimental study of cryptocurrency market dynamics. In: Proceedings of the 2018 CHI Conference on Human Factors in Computing Systems, p. 605. ACM
Kristjanpoller W, Bouri E, Takaishi T (2020) Cryptocurrencies and equity funds: Evidence from an asymmetric multifractal analysis. Physica A 545:123711
Kristoufek L (2013) Bitcoin meets google trends and wikipedia: Quantifying the relationship between phenomena of the internet era. Sci Rep 3:3415
Kumar D, Rath S (2020) Predicting the trends of price for ethereum using deep learning techniques. In: Artificial Intelligence and Evolutionary Computations in Engineering Systems, pp 103–114. Springer
Kurbucz MT (2019) Predicting the price of bitcoin by the most frequent edges of its transaction network. Econ Lett 184:108655
Kutner MH, Nachtsheim CJ, Neter J, Li W et al (2005) Applied linear statistical models, vol 5. McGraw-Hill, New York
Kwon D-H, Kim J-B, Heo J-S, Kim C-M, Han Y-H (2019) Time series classification of cryptocurrency price trend based on a recurrent lstm neural network. J Inform Process Syst 15(3)
Kyriazis NA (2019) A survey on efficiency and profitable trading opportunities in cryptocurrency markets. J Risk Financ Manag 12(2):67
Lamon C, Nielsen E, Redondo E (2017) Cryptocurrency price prediction using news and social media sentiment. SMU Data Sci Rev 1(3):1–22
Lawrence S, Giles CL, Tsoi AC, Back AD (1997) Face recognition: a convolutional neural-network approach. IEEE Trans Neural Networks 8(1):98–113
Leclair EM (2018) Herding in the cryptocurrency market. Econ 5029 final research, Carleton University, Canada
Lee T-H, Yang W (2014) Granger-causality in quantiles between financial markets: Using copula approach. Int Rev Financ Anal 33:70–78
Leirvik T (2021) Cryptocurrency returns and the volatility of liquidity. Finance Res Lett 102031
Li TN, Tourin A (2016) Optimal pairs trading with time-varying volatility. Int J Financ Eng 3(03):1650023
Li TR, Chamrajnagar A, Fong X, Rizik N, Fu F (2019) Sentiment-based prediction of alternative cryptocurrency price fluctuations using gradient boosting tree model. Front Phys 7:98
Liaw A, Wiener M et al (2002) Classification and regression by randomforest. R news 2(3):18–22
Li T, Kou G, Peng Y, Philip SY (2021) An integrated cluster detection, optimization, and interpretation approach for financial data. IEEE Trans Cybern
Lintilhac PS, Tourin A (2017) Model-based pairs trading in the bitcoin markets. Quant Finance 17(5):703–716
Liu W (2019) Portfolio diversification across cryptocurrencies. Financ Res Lett 29:200–205
Liu B, Polukarov M, Ventre C, Li L, Kanthan L (2021) Agent-based markets: Equilibrium strategies and robustness. In: Proceedings of the 2nd ACM International Conference on AI in Finance
Liu B, Polukarov M, Ventre C, Li L, Kanthan L (2021) Call markets with adaptive clearing intervals. In: Proceedings of the 20th International Conference on Autonomous Agents and MultiAgent Systems, pp. 1587–1589
Livieris IE, Pintelas E, Pintelas P (2020) A cnn-lstm model for gold price time-series forecasting. Neural Comput Appl 32(23):17351–17360
Livieris IE, Pintelas E, Stavroyiannis S, Pintelas P (2020) Ensemble deep learning models for forecasting cryptocurrency time-series. Algorithms 13(5):121
Localbtc: Localbitcoins purchasing online. https://localbitcoins.com . [Online, Accessed: January 11, 2020] (2020)
Lucarelli G, Borrotti M (2019) A deep reinforcement learning approach for automated cryptocurrency trading. In: IFIP International Conference on Artificial Intelligence Applications and Innovations, pp 247–258. Springer
Lu W, Li J, Li Y, Sun A, Wang J (2020) A cnn-lstm-based model to forecast stock prices. Complexity 2020
Luu Duc Huynh T (2019) Spillover risks on cryptocurrency markets: A look from var-svar granger causality and student copulas. Journal of Risk and Financial Management 12(2):52
Ma F, Liang C, Ma Y, Wahab M (2020) Cryptocurrency volatility forecasting: a Markov regime-switching midas approach. J Forecast 39(8):1277–1290
Madan I, Saluja S, Zhao A (2015) Automated bitcoin trading via machine learning algorithms. http://cs229.stanford.edu/proj2014/Isaac%20Madan
Makarov I, Schoar A (2020) Trading and arbitrage in cryptocurrency markets. J Financ Econ 135(2):293–319
Malladi RK, Dheeriya PL (2021) Time series analysis of cryptocurrency returns and volatilities. J Econ Finance 45(1):75–94
Maltatoday: Why world leader crypto exchange Binance moved to Malta. https://www.maltatoday.com.mt/business/business_news/93170/why_world_leader_crypto_exchange_binance_moved_to_malta#.XlKZ8Gj7Q2x . [Online, Accessed 11 Feb 2020] (2020)
Manahov V (2021) Cryptocurrency liquidity during extreme price movements: is there a problem with virtual money? Quant Finance 21(2):341–360
Markowitz H (1952) Portfolio selection. J Financ 7(1):77–91
McLean RD, Pontiff J (2016) Does academic research destroy stock return predictability? J Financ 71(1):5–32
McNally S (2016) Predicting the price of bitcoin using machine learning. PhD thesis, Dublin, National College of Ireland
McNally S, Roche J, Caton S (2018) Predicting the price of bitcoin using machine learning. In: 2018 26th Euromicro International Conference on Parallel, Distributed and Network-based Processing (PDP), pp 339–343. IEEE
MercoPress: Bitcoin legal tender in El Salvador, first country ever. https://en.mercopress.com/2021/06/10/bitcoin-legal-tender-in-el-salvador-first-country-ever . [Online, Accessed: June 10, 2021] (2021)
Merediz-Solà I, Bariviera AF (2019) A bibliometric analysis of bitcoin scientific production. Res Int Bus Financ 50:294–305
Meunier S (2018) Blockchain 101: What is blockchain and how does this revolutionary technology work? In: Transforming Climate Finance and Green Investment with Blockchains. Elsevier, pp 23–34
Mikolov T, Kombrink S, Burget L, Černockỳ J, Khudanpur S (2011) Extensions of recurrent neural network language model. In: 2011 IEEE International Conference on Acoustics, Speech and Signal Processing (ICASSP), pp 5528–5531. IEEE
MIT: Bitcoin network dataset. https://senseable2015-6.mit.edu/bitcoin . [Online, Accessed January 11, 2020] (2015)
Molina J (2019) Develop your Crypto-Trading System Using Plain Logic, Part 1. https://medium.com/swlh/develop-your-crypto-trading-system-using-plain-logic-part-1-caac02f0a37d . Accessed 20 Sep 2019
Mukhopadhyay U, Skjellum A, Hambolu O, Oakley J, Yu L, Brooks R (2016) A brief survey of cryptocurrency systems. In: 2016 14th Annual Conference on Privacy, Security and Trust (PST), pp. 745–752. IEEE
Nakamoto S (2009) Bitcoin open source implementation of p2p currency. P2P foundation 18
Nakano M, Takahashi A, Takahashi S (2018) Bitcoin technical trading with artificial neural network. Physica A 510:587–609
Narayanan A, Bonneau J, Felten E, Miller A, Goldfeder S (2016) Bitcoin and cryptocurrency technologies: a comprehensive introduction. Princeton University Press, Princeton
Nasir MA, Huynh TLD, Nguyen SP, Duong D (2019) Forecasting cryptocurrency returns and volume using search engines. Financ Innov 5(1):2
Neter J, Kutner MH, Nachtsheim CJ, Wasserman W (1996) Applied Linear Statistical Models vol. 4. Irwin Chicago
Ng AY, Jordan MI (2002) On discriminative vs. generative classifiers: A comparison of logistic regression and naive bayes. In: Advances in Neural Information Processing Systems, pp. 841–848
Nikolova V, Trinidad Segovia JE, Fernández-Martínez M, Sánchez-Granero MA (2020) A novel methodology to calculate the probability of volatility clusters in financial series: An application to cryptocurrency markets. Mathematics 8(8):1216
Nomics: Top Cryptocurrency Exchanges List. https://nomics.com/exchanges . [Online, Accessed: January 11, 2020] (2020)
Nti IK, Adekoya AF, Weyori BA (2020) A systematic review of fundamental and technical analysis of stock market predictions. Artif Intell Rev 53(4):3007–3057
Oberlechner T (2001) Importance of technical and fundamental analysis in the European foreign exchange market. Int J Finance Econ 6(1):81–93
Ogorevc M (2019) Cryptocurrency as money: A trading strategy solution. Available at SSRN 3436041
Omane-Adjepong M, Alagidede IP (2019) Multiresolution analysis and spillovers of major cryptocurrency markets. Res Int Bus Financ 49:191–206
Omane-Adjepong M, Alagidede P, Akosah NK (2019) Wavelet time-scale persistence analysis of cryptocurrency market returns and volatility. Physica A 514:105–120
Pascanu R, Mikolov T, Bengio Y (2013) On the difficulty of training recurrent neural networks. In: International Conference on Machine Learning, pp 1310–1318
Patil AP, Akarsh T, Parkavi A (2018) A study of opinion mining and data mining techniques to analyse the cryptocurrency market. In: 2018 3rd International Conference on Computational Systems and Information Technology for Sustainable Solutions (CSITSS), pp. 198–203. IEEE
Păuna C (2018) Arbitrage trading systems for cryptocurrencies. Design principles and server architecture. Informatica Economica 22(2):35–42
Persson S, Slottje A, Shaw I (2018) Hybrid autoregressive-recurrent neural network architecture for algorithmic trading of cryptocurrencies. Cs230 deep learning thesis, Stanford University
Phaladisailoed T, Numnonda T (2018) Machine learning models comparison for bitcoin price prediction. In: 2018 10th International Conference on Information Technology and Electrical Engineering (ICITEE), pp 506–511. IEEE
Phillips RC, Gorse D (2017) Predicting cryptocurrency price bubbles using social media data and epidemic modelling. In: 2017 IEEE Symposium Series on Computational Intelligence (SSCI), pp 1–7. IEEE
Phillips RC, Gorse D (2018) Mutual-excitation of cryptocurrency market returns and social media topics. In: Proceedings of the 4th International Conference on Frontiers of Educational Technologies, pp 80–86. ACM
Phillips RC, Gorse D (2018) Cryptocurrency price drivers: Wavelet coherence analysis revisited. PLoS ONE 13(4):0195200
Phillips PC, Shi S, Yu J (2015) Testing for multiple bubbles: Historical episodes of exuberance and collapse in the s&p 500. Int Econ Rev 56(4):1043–1078
Phillips PC, Shi S, Yu J (2015) Testing for multiple bubbles: limit theory of real-time detectors. Int Econ Rev 56(4):1079–1134
Poloniex (2020) Poloniex markets. https://poloniex.com/ . [Online, Accessed 11 Feb 2020]
Qiao X, Zhu H, Hau L (2020) Time-frequency co-movement of cryptocurrency return and volatility: evidence from wavelet coherence analysis. Int Rev Financ Anal 71:101541
Rane PV, Dhage SN (2019) Systematic erudition of bitcoin price prediction using machine learning techniques. In: 2019 5th International Conference on Advanced Computing & Communication Systems (ICACCS), pp 594–598. IEEE
Rebane J, Karlsson I, Denic S, Papapetrou P (2018) Seq2seq rnns and arima models for cryptocurrency prediction: a comparative study. SIGKDD Fintech 18
Research Network B (2020) Platform for scholarly communication about cryptocurrencies and blockchains. https://www.blockchainresearchnetwork.org/ . [Online, Accessed: April 17, 2020]
Reuters: China bans financial, payment institutions from cryptocurrency business. https://www.reuters.com/technology/chinese-financial-payment-bodies-barred-cryptocurrency-business-2021-05-18/ . [Online, Accessed 18 May 2021] (2021)
Rish I et al (2001) An empirical study of the naive bayes classifier. In: IJCAI 2001 Workshop on Empirical Methods in Artificial Intelligence, vol. 3, pp 41–46
Rose C (2015) The evolution of digital currencies: Bitcoin, a cryptocurrency causing a monetary revolution. Int Bus Econ Res J (IBER) 14(4):617–622
Salakhutdinov R, Hinton G (2009) Deep boltzmann machines. In: Artificial Intelligence and Statistics, pp 448–455
Sattarov O, Muminov A, Lee CW, Kang HK, Oh R, Ahn J, Oh HJ, Jeon HS (2020) Recommending cryptocurrency trading points with deep reinforcement learning approach. Appl Sci 10(4):1506
Shahzad SJH, Bouri E, Ahmad T, Naeem MA (2021) Extreme tail network analysis of cryptocurrencies and trading strategies. Finance Res Lett 102106
Shanaev S, Sharma S, Ghimire B, Shuraeva A (2020) Taming the blockchain beast? regulatory implications for the cryptocurrency market. Res Int Bus Financ 51:101080
Sharma S, Krishma N, Raina E (2017) Survey paper on cryptocurrency. International Journal of Scientific Research in Computer Science, Engineering and Information Technology 2(3):307–310
Siaminos G (2019) Predicting the value of cryptocurrencies using machine learning time series analysis time series analysis time
Sigaki HY, Perc M, Ribeiro HV (2019) Clustering patterns in efficiency and the coming-of-age of the cryptocurrency market. Sci Rep 9(1):1440
Sirignano J, Cont R (2019) Universal features of price formation in financial markets: perspectives from deep learning. Quant Finance 19(9):1449–1459
Slepaczuk R, Zenkova M (2018) Robustness of support vector machines in algorithmic trading on cryptocurrency market. Cent Eur Econ J 5:186–205
Smuts N (2019) What drives cryptocurrency prices? An investigation of google trends and telegram sentiment. ACM SIGMETRICS Perform Evalu Rev 46(3):131–134
Sriram A, Jun H, Satheesh S, Coates A (2017) Cold fusion: Training seq2seq models together with language models. arXiv preprint arXiv:1708.06426
Stocksharp: StockSharp - trading platform. https://github.com/StockSharp/StockSharp . [Online, Accessed 26 Jan 2020] (2020)
Stuerner P (2019) Algorithmic cryptocurrency trading. PhD thesis, Ulm University
Sun J, Zhou Y, Lin J (2019) Using machine learning for cryptocurrency trading. In: 2019 IEEE International Conference on Industrial Cyber Physical Systems (ICPS), pp 647–652. IEEE
Sutton RS, Barto AG et al (1998) Introduction to reinforcement learning, vol 135. MIT Press, Cambridge
Sze V, Chen Y-H, Yang T-J, Emer JS (2017) Efficient processing of deep neural networks: a tutorial and survey. Proc IEEE 105(12):2295–2329
Tapscott D, Tapscott A (2016) Blockchain Revolution: How the Technology Behind Bitcoin Is Changing Money, Business, and the World. Penguin
TradingstrategyGuides: EOS Cryptocurrency Trading Strategy–Turtle Soup Pattern. https://tradingstrategyguides.com/eos-cryptocurrency-trading-strategy/ . [Online, Accessed January 29, 2020] (2019)
TradingstrategyGuides: Free OMNI Cryptocurrency Strategy–Amazing Gann Box. https://tradingstrategyguides.com/free-omni-cryptocurrency-strategy/ . [Online, Accessed January 29, 2020] (2019)
TradingstrategyGuides: IOTA Cryptocurrency Strategy–Busted Double Top Pattern. https://tradingstrategyguides.com/iota-cryptocurrency-strategy/ . [Online, Accessed January 29, 2020] (2019)
TradingstrategyGuides: Nem (XEM) Cryptocurrency Strategy–Momentum Pinball Setup. https://tradingstrategyguides.com/nem-xem-cryptocurrency-strategy/ . [Online, Accessed January 29, 2020] (2019)
TradingstrategyGuides: Tether Trading Strategy–Bottom Rotation Trading. https://tradingstrategyguides.com/tether-trading-strategy/ . [Online, Accessed January 29, 2020] (2019)
TradingView: Total Crypto Market Capitalization and Volume. https://www.tradingview.com/markets/cryptocurrencies/global-charts/ . [Online, Accessed September 10, 2021] (2021)
Troster V, Tiwari AK, Shahbaz M, Macedo DN (2019) Bitcoin returns and risk: A general garch and gas analysis. Financ Res Lett 30:187–193
Trucíos C, Tiwari AK, Alqahtani F (2019) Value-at-risk and expected shortfall in cryptocurrencies’ portfolio: A vine copula-based approach. Available at SSRN 3441892
UKTN: Bitcoin and the Challenges for Financial Regulation. https://www.uktech.news . [Online, Accessed: February 24, 2021] (2021)
van den Broek L, Sharif Z (2018) Cointegration-based pairs trading framework with application to the cryptocurrency market
Vidal-Tomás D, Ibáñez AM, Farinós JE (2019) Herding in the cryptocurrency market: Cssd and csad approaches. Financ Res Lett 30:181–186
Virk DS (2017) Prediction of bitcoin price using data mining. Master’s thesis, National College of Ireland
Vogelvang B (2005) Econometrics: theory and applications with eviews. Pearson Education, London
Vo A, Yost-Bremm C (2018) A high-frequency algorithmic trading strategy for cryptocurrency. J Comput Inform Syst 1–14
Wagstaff K, Cardie C, Rogers S, Schrödl S et al (2001) Constrained k-means clustering with background knowledge. In: Icml, vol 1, pp 577–584
Wang L (2005) Support vector machines: theory and applications, vol 177. Springer, New York
Wang H, He D, Ji Y (2017) Designated-verifier proof of assets for bitcoin exchange using elliptic curve cryptography. Future Generat Comput Syst
Ward M (2018) Algorithmic trading for cryptocurrencies. Departmental honors thesis, UtahState University, United States
Weber P, Wang F, Vodenska-Chitkushev I, Havlin S, Stanley HE (2007) Relation between volatility correlations in financial markets and omori processes occurring on all scales. Phys Rev E 76(1):016109
Wohlin C (2014) Guidelines for snowballing in systematic literature studies and a replication in software engineering. In: Proceedings of the 18th International Conference on Evaluation and Assessment in Software Engineering, pp 1–10
Xu Z, Wang S, Zhu F, Huang J (2017) Seq2seq fingerprint: An unsupervised deep molecular embedding for drug discovery. In: Proceedings of the 8th ACM International Conference on Bioinformatics, Computational Biology, and Health Informatics, pp 285–294
Yang H (2018) Behavioral anomalies in cryptocurrency markets. Available at SSRN 3174421. https://doi.org/10.2139/ssrn.3174421
Yao Y, Yi J, Zhai S, Lin Y, Kim T, Zhang G, Lee LY (2018) Predictive analysis of cryptocurrency price using deep learning. Int J Eng Technol 7(3.27):258–264
Yaya OS, Ogbonna EA, Olubusoye OE (2018) How persistent and dependent are pricing of bitcoin to other cryptocurrencies before and after 2017/18 crash?
Zamuda A, Crescimanna V, Burguillo JC, Dias JM, Wegrzyn-Wolska K, Rached I, Gonzlez H, Senkerik R, Pop C, Cioara T et al (2019) Forecasting cryptocurrency value by sentiment analysis: An hpc-oriented survey of the state-of-the-art in the cloud era. In: High-Performance Modelling and Simulation for Big Data Applications, pp 325–349. Springer
Żbikowski K (2016) Application of machine learning algorithms for bitcoin automated trading. In: Machine Intelligence and Big Data in Industry, pp 161–168. Springer
Zemmal N, Azizi N, Dey N, Sellami M (2016) Adaptive semi supervised support vector machine semi supervised learning with features cooperation for breast cancer classification. J Med Imaging Health Inform 6(1):53–62
Zha Q, Kou G, Zhang H, Liang H, Chen X, Li C-C, Dong Y (2020) Opinion dynamics in finance and business: a literature review and research opportunities. Financial Innovation 6(1):1–22
Zhang W, Li Y (2020) Is idiosyncratic volatility priced in cryptocurrency markets? Res Int Bus Financ 54:101252
Zhang S, Yao L, Sun A, Tay Y (2019) Deep learning based recommender system: a survey and new perspectives. ACM Comput Surv 52(1). https://doi.org/10.1145/3285029
Zhengyang W, Xingzhou L, Jinjin R, Jiaqing K (2019) Prediction of cryptocurrency price dynamics with multiple machine learning techniques. In: Proceedings of the 2019 4th International Conference on Machine Learning Technologies, pp 15–19. ACM
Zhou H, Kalev PS (2019) Algorithmic and high frequency trading in asia-pacific, now and the future. Pac Basin Financ J 53:186–207
Download references
This research received no specific grant from any funding agency in the public, commercial, or not-forprofit sectors.
Author information
Authors and affiliations.
D epartment of Informatics, Faculty of Natural, Mathematical & Engineering Science, King’s College London, Strand, London, WC2R 2LS, UK
Fan Fang & Carmine Ventre
Turing Intelligence, Technology Limited, London, UK
Fan Fang, Michail Basios, Leslie Kanthan, David Martinez-Rego, Fan Wu & Lingbo Li
You can also search for this author in PubMed Google Scholar
Contributions
FF carried out the research papers’ collection and analysis, and drafted the manuscript. CV suggested some analysis of the literature and contributed to writing and organizing the manuscript. MB, HK, LK, DMR, FW conceived of the study, especially in connection with the “future research opportunities” and helped draft the manuscript. LL had the idea for the topic of this paper and participated in the design of the study. All authors read and approved the final manuscript.
Corresponding authors
Correspondence to Fan Fang or Lingbo Li .
Ethics declarations
Competing interests.
The authors declare that they have no competing interests.
Additional information
Publisher's note.
Springer Nature remains neutral with regard to jurisdictional claims in published maps and institutional affiliations.
Rights and permissions
Open Access This article is licensed under a Creative Commons Attribution 4.0 International License, which permits use, sharing, adaptation, distribution and reproduction in any medium or format, as long as you give appropriate credit to the original author(s) and the source, provide a link to the Creative Commons licence, and indicate if changes were made. The images or other third party material in this article are included in the article's Creative Commons licence, unless indicated otherwise in a credit line to the material. If material is not included in the article's Creative Commons licence and your intended use is not permitted by statutory regulation or exceeds the permitted use, you will need to obtain permission directly from the copyright holder. To view a copy of this licence, visit http://creativecommons.org/licenses/by/4.0/ .
Reprints and permissions
About this article
Cite this article.
Fang, F., Ventre, C., Basios, M. et al. Cryptocurrency trading: a comprehensive survey. Financ Innov 8 , 13 (2022). https://doi.org/10.1186/s40854-021-00321-6
Download citation
Received : 14 January 2021
Accepted : 02 December 2021
Published : 07 February 2022
DOI : https://doi.org/10.1186/s40854-021-00321-6
Share this article
Anyone you share the following link with will be able to read this content:
Sorry, a shareable link is not currently available for this article.
Provided by the Springer Nature SharedIt content-sharing initiative
- Cryptocurrency
- Machine learning
- Econometrics
Thank you for visiting nature.com. You are using a browser version with limited support for CSS. To obtain the best experience, we recommend you use a more up to date browser (or turn off compatibility mode in Internet Explorer). In the meantime, to ensure continued support, we are displaying the site without styles and JavaScript.
- View all journals
- My Account Login
- Explore content
- About the journal
- Publish with us
- Sign up for alerts
- Open access
- Published: 02 January 2024
Cryptocurrency awareness, acceptance, and adoption: the role of trust as a cornerstone
- Muhammad Farrukh Shahzad ORCID: orcid.org/0000-0002-6578-4139 1 ,
- Shuo Xu 1 ,
- Weng Marc Lim 2 , 3 , 4 ,
- Muhammad Faisal Hasnain 5 &
- Shahneela Nusrat 6
Humanities and Social Sciences Communications volume 11 , Article number: 4 ( 2024 ) Cite this article
4428 Accesses
2 Citations
2 Altmetric
Metrics details
- Business and management
- Science, technology and society
Cryptocurrencies—i.e., digital or virtual currencies secured by cryptography based on blockchain technology, such as Bitcoin and Ethereum—have brought transformative changes to the global economic landscape. These innovative transaction methods have rapidly made their mark in the financial sector, reshaping the dynamics of the global economy. However, there remains a notable hesitation in its widespread acceptance and adoption, largely due to misconceptions and lack of proper guidance about its use. Such gaps in understanding create an opportunity to address these concerns. Using the technology acceptance model (TAM), this study develops a parsimonious model to explain the awareness, acceptance, and adoption of cryptocurrency. The model was assessed using partial least squares structural equation modeling (PLS-SEM) with a sample of 332 participants aged 18 to 40 years. The findings suggest that cryptocurrency awareness plays a direct, positive, and significant role in shaping cryptocurrency adoption and that this positive relationship is mediated by factors that exemplify cryptocurrency acceptance, namely the ease of use and usefulness of cryptocurrency. The results also reveal that trust is a significant factor that strengthens these direct and mediating relationships. These insights emphasize the necessity of fostering an informed understanding of cryptocurrencies to accelerate their broader adoption in the financial ecosystem. By addressing the misconceptions and reinforcing factors like ease of use, usefulness, and trust, policymakers and financial institutions can better position themselves to integrate and promote cryptocurrency in mainstream financial systems.
Similar content being viewed by others
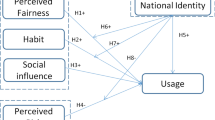
Extending UTAUT with national identity and fairness to understand user adoption of DCEP in China
Bingyan Wu, Xiaoqing An, … Ho Young Shin
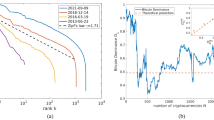
Modeling innovation in the cryptocurrency ecosystem
Giordano De Marzo, Francesco Pandolfelli & Vito D. P. Servedio
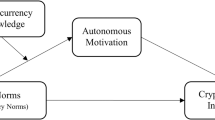
Group norms and policy norms trigger different autonomous motivations for Chinese investors in cryptocurrency investment
Yongzhi Gong, Xiaofei Tang & En-Chung Chang
Introduction
Cryptocurrency has heralded a transformative shift in the global financial sector, offering a reimagined concept of money (Johar et al., 2021 ; Kakinaka and Umeno, 2022 ). Underpinned by blockchain and cryptography, cryptocurrency represents a novel class of digital or virtual currency. At its core, a blockchain serves as a decentralized ledger, chronicling every transaction across a distributed network of computers, assuring both transparency and permanence, whereas cryptography ensures that transactions are safeguarded against tampering and that participants’ identities remain confidential (Kumar et al., 2023 ; Sahoo et al., 2022 ). Popular examples of cryptocurrencies include Bitcoin and Ethereum. Unlike traditional currencies like the dollar or euro issued by central banks, cryptocurrencies function outside established financial systems. They are not tied to tangible assets like gold or governed by central financial institutions. Instead, they are created through a computational process called mining, which involves using computational power to solve complex mathematical problems, leading to the creation and verification of new transactions on a cryptocurrency network. While digital forms of money like Apple Pay or PayPal credits exist in the digital or online realm, they operate within the confines of traditional banking systems and lack the decentralized essence of blockchain (Jalan et al., 2023 ). On the other hand, cryptocurrencies, thanks to their inherent decentralized, transparent, and immutable nature, stand distinct and have ignited profound discussions in modern financial and technological arenas.
As per recent estimates, the aggregate value of transactions in digital payments is projected to reach US$9.46 trillion in 2023, with an expected annual increase of more than 11.8%, resulting in a total projection of US$14.78 trillion by 2027 (Siska, 2023 ). As the internet’s ubiquity grew, so did the shift from conventional cash payments to online transaction methods, reshaping global monetary systems (Sukumaran et al., 2022 ). Digital advancements and technological accessibility have also spurred an uptick in consumers engaging in online transactions via cryptocurrency (Kumar et al., 2021 ; Shin and Rice, 2022 ). For many, the allure of cryptocurrency lies in its borderless nature, simplicity, and speed (Galariotis and Karagiannis, 2020 ; Zafar et al., 2021 ). Indeed, cryptocurrency has reshaped the global financial infrastructure, compelling institutions to innovate in the digital transaction space (Khan et al., 2020 ). This momentum has spurred monetary systems to evolve, adapting and instituting policies that align with technological progress (Hasan et al., 2022 ).
A comprehensive understanding of cryptocurrencies is crucial in the current digital age (Uematsu and Tanaka, 2019 ). The growing popularity of cryptocurrencies is evident, especially as they herald transformative changes in global money markets (Tandon et al., 2021 ). Yet, adoption is tempered by challenges. While technology awareness can enhance acceptance, issues like limited technological know-how and understanding of online trading, legislative constraints, and security concerns act as deterrents (Albayati et al., 2020 ; Li et al., 2023 ; Rejeb et al., 2023 ). Moreover, while studies suggest that cryptocurrencies could promote financial inclusivity for underrepresented demographics, concerns remain about unequal wealth distribution among cryptocurrency holders (Abdul-Rahim et al., 2022 ; Allen et al., 2022 ). Recognizing the intricacies of cryptocurrency is crucial in today’s digital age, ensuring individuals stay abreast of technological advances (Yayla et al., 2023 ).
The present study argues that understanding cryptocurrency awareness, acceptance, and adoption is imperative in today’s evolving digital financial landscape. As cryptocurrency positions itself at the forefront of financial innovation, comprehending the factors that drive or deter its acceptance can offer valuable insights into shaping future economic policies, strategies, and infrastructures. Awareness speaks to the extent of knowledge and understanding individuals have about cryptocurrency; acceptance gauges their openness to adopting it as a part of their financial behaviors; and adoption reflects the incorporation of cryptocurrency into their monetary transactions. Moreover, the role of trust as a moderator is especially crucial. Trust, or the lack thereof, can significantly influence an individual’s decision-making process concerning cryptocurrency. In an environment where transactions are decentralized and often lack traditional oversight, trust can be the linchpin that determines whether an individual engages with or shies away from cryptocurrency. By examining the moderating role of trust, we can better understand the psychological factors that play a pivotal role in cryptocurrency’s broader acceptance and usage in society.
Given the above, the goal of this study is to examine the relationships between cryptocurrency awareness, acceptance, and adoption, as well as the moderating role of trust in these relationships. To do so, this study adopts the technology acceptance model (TAM) as a theoretical lens, wherein the technology in point is cryptocurrency. Essentially, TAM assumes that the acceptance of a technology could be understood by its ease of use and usefulness, which in turn shapes the adoption of that technology (Davis, 1989 ). Extending this theory, this study introduces (i) the concept of awareness, which is posited to exert a positive influence on consumers’ perception of the ease of use and usefulness of the technology on the basis that consumers are in a better position to form an opinion about a technology when they are knowledgeable about that technology, and (ii) the notion of trust, which is proposed to strengthen the relationship between awareness, acceptance, and adoption on the basis that trust acts as an enabler, mitigating potential fears and uncertainties associated with the adoption of new technologies, particularly in financial transactions where credibility and security and are paramount.
This study contributes to both theory and practice in several significant ways. Theoretically, by adopting and extending TAM to the realm of cryptocurrency, we strive to not only affirm the theoretical generalizability of the theory but also illuminate how awareness and trust play pivotal roles in the acceptance and adoption of such innovative financial technologies, which answered the call by past scholars such as (Farrukh et al., 2023 ; Kumar et al., 2021 ). By integrating the concept of awareness into TAM, this study posits that a heightened level of understanding can bolster consumers’ perceptions regarding the ease of use and usefulness of cryptocurrencies. In doing so, this research will enrich TAM by accounting for the influence of awareness in the adoption of emerging technologies. Furthermore, the introduction of trust as a moderating variable elucidates how confidence can amplify or mitigate the relationships between awareness, acceptance, and adoption of cryptocurrency. This presents a nuanced understanding of the psychological facets that underpin the decision-making processes surrounding technology adoption in financial contexts. The use of TAM as a foundational theoretical lens and the inclusion of new variables like awareness and trust into the model is in line with the recommendation of Lim ( 2018 ) for contributions amounting to theoretical generalizability and extension in relation to the theory. Practically, the findings of this study can guide policymakers, financial institutions, and tech developers in recognizing the crucial factors that drive or inhibit the mass adoption of cryptocurrency. By discerning the importance of awareness and trust, stakeholders can develop targeted educational campaigns and security measures, respectively, to bolster the public’s confidence and understanding of cryptocurrencies. In essence, this research offers strategic insights to industries and governments, enabling them to tailor their initiatives in promoting a more inclusive, transparent, and trustworthy digital financial landscape.
Theoretical background: technology acceptance model (TAM)
TAM is pivotal in predicting and explaining technology adoption behaviors. At its core, TAM posits two central constructs that determine one’s intention to accept and adopt a new technology: perceived ease of use (PEOU) and perceived usefulness (PU) (Davis, 1989 ). PEOU refers to the degree to which one believes that using a particular technology would be free from effort (Sagheer et al., 2022 ). Essentially, the simpler and more straightforward a technology is perceived, the more likely it is to be adopted. This is founded on the principle that individuals are naturally inclined to choose technologies that do not require significant effort or time (Siagian et al., 2022 ). Second, PU is centered on one’s belief that utilizing a particular technology will enhance their performance (Fagan et al., 2012 ). It captures the value proposition of the technology: if people deem a technology as beneficial and enhancing their productivity or efficiency, they are more inclined to use it (Lu et al., 2022 ). Through PEOU and PU, one’s intention toward technology adoption is kindled (Davis, 1989 ).
TAM is a robust theoretical framework that aids consumers in making informed decisions regarding the embrace of technological advancements (Lim, 2018 ). Our study underscores the relevance of TAM’s acceptance elements, specifically PEOU and PU, in gauging consumers’ intention towards adopting cryptocurrency. Evidently, past scholars have consistently utilized TAM as a cornerstone theory in assessing individual behavior concerning the uptake of novel technologies (Kumar et al., 2021 ; Sagheer et al., 2022 ; Shahzad et al., 2018 ; Yuen et al., 2021 ).
In the ever-evolving landscape of cryptocurrency, there remains a noticeable dearth of scholarly attention on its utilization as a medium of exchange (Khan et al., 2020 ). Addressing this gap, our study endeavors to craft a theoretical framework, firmly anchored in TAM, to shed light on the determinants of cryptocurrency adoption. It is imperative to acknowledge that intentions stand as potent precursors of actions, with these intentions being invariably shaped by an individual’s attitudes and perceptions toward a specific endeavor (Davis, 1989 ; Lim, 2018 ).
Conceptual background: cryptocurrency
Cryptocurrency, fundamentally a digital or virtual form of currency utilizing cryptography for security, made its debut in 2008 through a white paper published by an individual or group under the pseudonym Satoshi Nakamoto; this innovation was later introduced in 2009 (Tauni et al., 2015 ). These digital assets offer decentralized control as opposed to centralized banking systems, promoting financial autonomy and inclusivity.
As a testament to its transformative potential, recent research highlights its increasing influence in various sectors of life, from remittances to cross-border trade (Parate et al., 2023 ). Given its rising economic significance, numerous countries are gradually recognizing and shifting towards cryptocurrency. With its decentralized nature, individuals find appeal in the autonomy, privacy, and potential returns it promises, away from traditional banking systems (Bibi, 2023 ).
Cryptocurrency can be broken down into two components: ‘crypto’ and ‘currency’. While ‘crypto’ refers to cryptography that secures user information and transactions, ‘currency’ is simply a medium of exchange. Globally, cryptocurrency has initiated significant changes in the financial sector, especially in developing countries. Noteworthily, a distinct characteristic of certain cryptocurrencies is their limited supply, which, for some, offers a potential hedge against inflation. This contrasts with traditional fiat currencies, which can be influenced by central bank policies (Allen et al., 2022 ). With more individuals adopting cryptocurrency, there is potential for a shift in the demand for traditional banking services, subsequently influencing interest rates and banks’ profitability (Tandon et al., 2021 ). Put simply, cryptocurrency represents a digital equivalent of cash that promises quicker, more reliable, and cost-effective transactions than its government-issued counterparts (Entrialgo, 2017 ). Its popularity has surged in recent years, as evidenced by its prominence in trading indices (Kher et al., 2021 ). Hence, this study zeroes in on the individual intention to adopt cryptocurrency.
Yet, the path to widespread adoption of cryptocurrency is not without hurdles. Among the challenges, environmental concerns related to the energy-intensive mining processes of certain cryptocurrencies have sparked debates on their sustainability (Rejeb et al., 2023 ). With regulatory landscapes still maturing, many investors approach this technology with caution due to potential risks (Shahzad et al., 2018 ). Common barriers include the nascent stage of cryptocurrency development, restricted access to advanced technology, and marketplace challenges such as unstructured trading environments, undefined financial regulations, and heightened security risks (Aboalsamh et al., 2023 ). Addressing these concerns, this study seeks to provide insights into the potential digital evolution awaiting individuals. The significance of cryptocurrencies as a potential mainstay in global finance is undeniable (Granić and Marangunić, 2019 ).
Leading financial markets, including the U.S., are actively researching and considering integrating cryptocurrency into their financial ecosystems (Hasgül et al., 2023 ). The horizon seems promising, with evolving technological solutions aiming to address current challenges and projections hinting at an even more integrated role of cryptocurrencies in global finance.
Taking Pakistan as a case study, its largely cash-driven economy juxtaposes with its youthful population and growing internet accessibility—factors ripe for digital payment adoption. In this regard, cryptocurrency’s potential as a trusted financial instrument in developing countries like Pakistan is substantial. Historical data indicates a rising preference for virtual currencies over traditional payment methods in regions like this (Arpaci et al., 2023 ). In an exemplary move, El Salvador passed the Bitcoin law in June 2021, granting it legal tender status alongside the U.S. dollar. This step, intended to streamline daily transactions and reduce remittance costs, has been met with diverse reactions globally (Gaikwad and Mavale, 2021 ). Its long-term impact, especially in the domain of remittances, remains under scrutiny (Howson and de Vries, 2022 ).
Hypotheses development
Cryptocurrency awareness and adoption.
Awareness plays a pivotal role in the acceptance and adoption of new technologies. Tracing back to diffusion innovation theory, awareness is considered the initial phase, crucial for the success of subsequent adoption stages (Lu et al., 2022 ). Building on this foundational understanding, studies examining technology implementation have reaffirmed the positive association between awareness levels and attitudes toward novel technologies (Arpaci et al., 2023 ).
An individual’s awareness encapsulates their comprehension of its advantages, potential drawbacks, and practical methods for its utilization (Zou et al., 2023 ). Consequently, the depth of a person’s awareness often sways their perceptions and, more critically, their readiness to adopt. A compelling parallel can be found in behavioral intention, a metric that gauges the likelihood of users engaging in a specific action. This metric plays a decisive role in both the determination to integrate cryptocurrency into one’s financial portfolio (Li, 2023 ). Historically, a robust behavioral intention has been a harbinger of successful technology adoption, diminishing the risk of committing to unsuitable or inferior innovations (Mizanur and Sloan, 2017 ; Siagian et al., 2022 ).
Recent empirical evidence corroborates this relationship between awareness and adoption. For instance, (Kakinaka and Umeno, 2022 ) highlighted that heightened awareness invariably bolstered positive intentions toward technology adoption. This intrinsic connection between cognitive awareness and behavioral outcomes serves as a cornerstone of our investigation. Intriguingly, the association between self-conceptualized technology awareness and behavior is not merely anecdotal but is underpinned by empirical studies. For example, (Gupta and Arora, 2020 ) underscored how nuanced technology awareness could shape individuals’ propensity to assimilate cryptocurrency into their financial activities.
Given the increasing emphasis on behavioral intention as a reliable predictor of technology adoption (Nadeem et al., 2021 ) our research delves into the interplay between technology awareness and the predisposition toward digital assets like cryptocurrency. In light of the aforementioned discussion and supporting evidence, we propose the following hypothesis:
H1 . Cryptocurrency awareness exerts a positive influence on cryptocurrency adoption.
The mediating role of cryptocurrency ease of use
Ease of use stands as a pivotal factor in determining the adoption of new technologies (Lim, 2018 ). The more intuitive and straightforward a technology appears, the higher the likelihood of its widespread acceptance (Sagheer et al., 2022 ). As a technology’s usability becomes evident, individuals are more inclined to integrate it into their routine. This notion is central to TAM, which has been widely acknowledged in the tech domain (Sudzina et al., 2023 ).
The emphasis on ease of use stems from a need to create technologies that are accessible, responsive, and adaptable. When people find a technology to match these criteria, they are more inclined to adopt that technology. It is about meeting the needs of consumers without inundating them with unnecessary complexity (Nadeem et al., 2021 ). While over-simplification might occasionally deter usage, efficient tools that streamline processes generally receive consumers’ approval (Wibasuri, 2022 ).
Historical evidence suggests a strong association between ease of use and behavioral intentions toward the adoption of novel technologies. For instance, Albayati et al. ( 2020 ) and Treiblmaier and Sillaber ( 2021 ) explored this connection, though only Shahzad et al. ( 2018 ) provided particularly insightful findings. Current research builds on this foundation, investigating the belief that user-friendliness and efficiency in cryptocurrencies can enhance user experience and, consequently, adoption rates. Furthermore, this study aligns with past research (Siagian et al., 2022 ), emphasizing the connection between technology awareness and the intention to adopt the technology. It recognizes the vital role of ease of use as a bridge between these elements.
To this end, numerous studies have already highlighted the profound impact of ease of use on shaping intentions towards new technological integrations (Biswas et al., 2021 ; Chen and Aklikokou, 2020 ). With this context in mind, our research specifically delves into how bolstering ease of use can pave the way for broader cryptocurrency adoption. Based on the synthesis of the above discussions, we put forth:
H2 . Cryptocurrency ease of use significantly mediates the relationship between cryptocurrency awareness and adoption.
The mediating role of cryptocurrency usefulness
Usefulness reflects the consumers’ belief that embracing a novel technology will enhance their performance (Davis, 1989 ; Lim, 2018 ). Prior research underscores that consumers are more likely to embrace cryptocurrency if they deem it beneficial (Kim et al., 2021 ). Historically, usefulness has been a cornerstone determinant of TAM, underscoring its centrality in assessing technological innovations (Fagan et al., 2012 ; Salas, 2020 ). Its role in information systems, such as facilitating the ease of adoption of cryptocurrency, is indeed substantial (Albayati et al., 2020 ).
As digital platforms burgeon, consumers increasingly derive their understanding of a technology’s usefulness from these platforms. This sentiment aligns with our study’s emphasis: online platforms serve as a conduit through which people gauge the usefulness of cryptocurrency. The decision to engage with or refrain from technology often hinges on its utility (Theiri et al., 2022 ). Past research has ventured into examining the role of usefulness in influencing behavioral intentions, but the findings have been mixed, prompting further inquiry (Basuki et al., 2022 ). For instance, consumers frequently endorse applications that are skillful, user-friendly, and competent, as these qualities often correlate with the usefulness of the tool (Granić and Marangunić, 2019 ). Yet, factors such as changing regulatory landscapes or environmental uncertainties can modulate consumers’ perceptions of a technology’s usefulness (Stocklmayer and Gilbert, 2002 ). Some prior studies, interestingly, have highlighted that consumers’ inclinations were swayed more by entertainment values than sheer usefulness, illuminating the multifaceted nature of consumer engagement (Abdul-Rahim et al., 2022 ).
Literature has consistently underscored the symbiotic relationship between awareness of technology and its perceived usefulness in shaping behavioral intentions. Notably, Namahoot and Rattanawiboonsom ( 2022 ) contended that consumers found electronic payments, including those using cryptocurrency, useful. This assertion is buttressed by other studies that emphasize the direct impact of technological awareness on behavioral intention through the prism of usefulness (Almajali et al., 2022 ; Sagheer et al., 2022 ). Noteworthily, a survey by Chen and Aklikokou ( 2020 ) illuminated the mediating role of usefulness in amplifying adoption behavior. Delving deeper into this interplay Schaupp and Festa ( 2018 ) pondered the ease with which newcomers could adopt cryptocurrency, positing that those acquainted with digital currencies are naturally more receptive, given the usefulness they derive from it. This is further validated by research in China (Shahzad et al., 2018 ), which determined that usefulness was a positive determinant for cryptocurrency adoption. In light of the aforementioned insights and findings, our hypothesis is articulated as:
H3 . Cryptocurrency usefulness significantly mediates the relationship between cryptocurrency awareness and adoption.
The moderating role of cryptocurrency trust
Trust in the context of innovative technologies refers to a consumer’s comfort, confidence, and assurance in its utilization (Quan et al., 2023 ). It is a cornerstone of acceptance and adoption, determining whether individuals feel secure in embracing new innovations (Akther and Nur, 2022 ). Over time, the evolution of social relationships has underscored the importance of consistent trust when adopting new technologies (Matemba and Li, 2018 ). Studies, such as those by Hasan et al. ( 2022 ), emphasized that heightened trust typically amplifies the adoption rate of technologies, especially those in their nascent stages. By fostering trust in cryptocurrency, the present study seeks to pave the way for its broader acceptance and adoption.
In digital commerce, where intangibility is a given, trust is paramount. Such trust encourages users to engage safely, reducing uncertainties and reservations (Shin and Rice, 2022 ). Mutual trust between users and providers is essential. Trust solidifies user-provider relationships and ensures sustained confidence in new ventures (Sukumaran et al., 2022 ). Essentially, trustworthy entities reduce risks, propelling individuals to explore and adopt. Research has shown that those with a positive inclination towards digital technologies display a stronger affinity for acceptance, contingent upon factors like trust and associated security measures (Völter et al., 2021 ). This is corroborated by Utz et al. ( 2022 ) who posited that trust fortifies individuals’ intention to use blockchain, a technology in which cryptocurrency operates upon.
Moreover, trust safeguards consumers’ financial and personal information, symbolizing a heightened level of confidence in cryptocurrency adoption (Tan and Saraniemi, 2022 ). A significant observation from past research is that when user trust wanes, their risk aversion decreases, and they become more susceptible to pitfalls (Sukumaran et al., 2022 ). A pivotal point raised by researchers, such as McCloskey ( 2006 ), is that trust plays a determinative role in influencing a user’s perception of how easy and useful a technology is. Trust, therefore, emerges as a linchpin that shapes user behavior, especially concerning ease of use and usefulness of technology (Albayati et al., 2020 ; Deebak et al., 2022 ).
In the midst of the prevailing discourse on technology adoption, trust emerges as a crucial determinant. The current study delves into the moderating role of trust, examining its influence on the relationship between cryptocurrency awareness, acceptance (ease of use, usefulness), and adoption. Our study seeks to clarify the nuances of this relationship, thereby enriching understanding and advancing the field. Specifically, trust in cryptocurrency is rooted in blockchain-based consensus protocols, such as proof of stake. This foundational trust enhances people’s confidence to adopt cryptocurrency, with TAM predictors serving as key indicators of this trust chain. In light of these considerations, the following hypotheses are proposed:
H4a . Cryptocurrency trust significantly moderates the relationship between cryptocurrency awareness, ease of use, and adoption.
H4b . Cryptocurrency trust significantly moderates the relationship between cryptocurrency awareness, ease of use, and adoption.
The conceptual framework, which depicts the hypothesized relationships, is presented in Fig. 1 . Essentially, cryptocurrency awareness is expected to shape cryptocurrency adoption positively, with the ease of use and usefulness of cryptocurrency mediating this relationship, and the trust in cryptocurrency strengthening these mediating relationships.
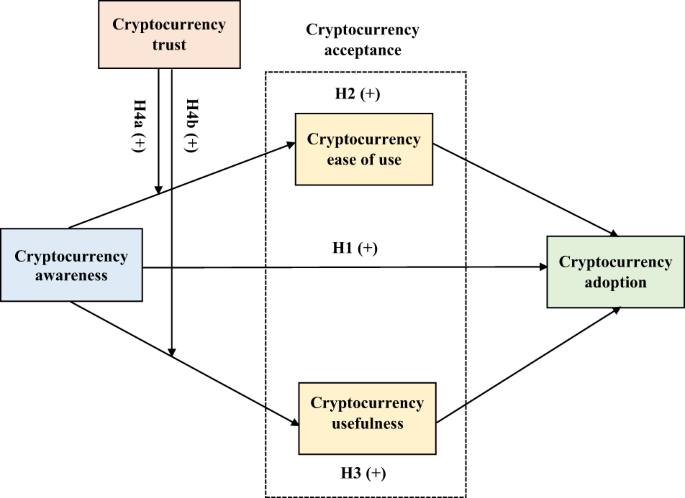
The cryptocurrency awareness, acceptance, and adoption model.
Instrumentation
The study utilized a survey-based approach to gauge respondents’ awareness, acceptance, and adoption of cryptocurrency. Drawing from existing literature, a questionnaire was designed, ensuring that it is tailored to fit the scope and focus of this investigation (Shahzad et al., 2022 ). The items in the questionnaire were measured using a five-point Likert scale, with the following constructs:
Cryptocurrency awareness
This construct deals with an individual’s understanding of cryptocurrency. For this study, it was operationalized with eight items adopted from Sagheer et al. ( 2022 ).
Cryptocurrency ease of use
Addressing an individual’s perception regarding the simplicity of embracing cryptocurrency, six items from Chen and Aklikokou ( 2020 ) were used.
Cryptocurrency usefulness
Signifying an individual’s belief in how the adoption of cryptocurrency can augment their efficiency, this was gauged through six items, with references from Albayati et al. ( 2020 ).
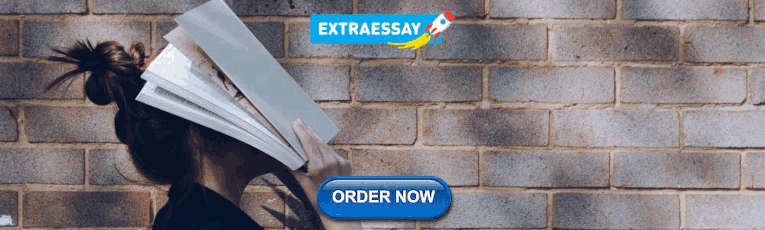
Cryptocurrency trust
Critical to the acceptance process, trust helps potential adopters believe in the credibility and reliability of cryptocurrency. Trust was evaluated through five items based on the work of Shahzad et al. ( 2018 ).
Cryptocurrency adoption
Representing an individual’s projected likelihood of adopting cryptocurrency, this was defined through five items, also taken from Shahzad et al. ( 2018 ).
While the emerging acceptance of cryptocurrency can be witnessed across various age groups, this study specifically targeted individuals aged 18 to 40 years in Lahore, Pakistan. The choice of this sampling area, with its blend of institutions and vibrant economic activities, ensured the representation of various sub-groups including university students, government/private employees, and business owners (Shahzad et al., 2021 ).
The data collection process initiated with screening questions, determining the familiarity with digital tools and internet use, as these were pre-requisites of actual or potential adoption of cryptocurrency. This phase ensured that only the relevant participants, who met the established criteria, were considered.
A total of 551 questionnaires were distributed both physically and online to individuals who passed the screening criteria and voluntarily consented to participate in the cryptocurrency survey. After accounting for missing values, outliers, and other discrepancies, 332 responses were deemed suitable for further analysis, yielding a usable response rate of 60.2%. This number aligns with Roscoe et al. ( 1975 ), who recommend a sample size ranging between 30 and 500 for empirical investigations. Among these respondents, 100% were familiar with digital tools, and 39% reported spending over seven hours daily on the internet, indicating a tech-savvy sample. Participants were assured of the confidentiality of their responses to ensure candidness in their answers.
Profile of participants
The sample for the study comprised a diverse group of participants spanning various demographics. Table 1 presents a comprehensive profile of the participants based on gender, age, education, and occupation.
Among the 332 respondents, the gender distribution was fairly balanced. Women represented a slight majority, accounting for 54% ( n : 178) of the sample, while men constituted 46% ( n : 154).
The age distribution revealed that a significant majority of the participants were relatively young. Those in the age group of 18 to 30 years formed the largest chunk, representing 71% ( n : 235) of the total sample. Respondents aged between 31 to 40 years constituted 29% ( n : 97).
Delving into educational qualifications, a significant portion of the participants held a bachelor’s degree, representing 45% ( n : 150) of the sample. Those with a master’s degree formed 35% ( n : 115) of the respondents. Participants with a diploma accounted for 16% ( n : 52), and a very small fraction held a doctoral degree, constituting 2% ( n : 8) of the sample. Other educational qualifications, which could include certifications from online courses, were held by 2% ( n : 7) of the participants.
In terms of the professional backgrounds of the participants, a predominant 66% ( n : 218) were students, which aligns with the age distribution. Business owners and private sector employees were equally represented at 13% each ( n : 45 and n : 44, respectively). Government employees made up a smaller portion of the sample at 8% ( n : 25).
Measurement model
To establish the appropriateness and accuracy of our measurement model, we employed the partial least squares structural equation modeling (PLS-SEM) algorithm, a method extensively applied in related research. The assessment focused on three critical aspects: reliability, convergent validity, and discriminant validity, each of which contributes significantly to the robustness of the model and its resultant interpretations (Farrukh et al., 2023 ). Table 2 delineates the main statistical indices—i.e., Cronbach’s alpha ( α ), composite reliability (CR), and average variance extracted (AVE)—associated with each construct of our measurement model.
For internal consistency, an evaluation criterion based on Cronbach’s alpha was utilized. Introduced by Lee Cronbach in 1951, this metric determines how closely related a set of items are to each other within a construct (Sarstedt et al., 2014 ). A value closer to 1 indicates a higher consistency among the items, with values above 0.7 being regarded as indicative of good internal reliability (Voorhees et al., 2016 ). As observed in Table 2 , all constructs exceeded this threshold, signaling a high degree of internal consistency. To supplement the insights gleaned from Cronbach’s alpha and address its limitations, composite reliability was employed. This metric evaluates the internal consistency of the variables based on outer loading values (Martins et al., 2023 ). Akin to our findings for Cronbach’s alpha, the CR values for all constructs, as depicted in Table 2 , comfortably exceeded the 0.7 benchmark.
Convergent validity, which underscores the degree to which items of a construct are positively correlated, was assessed using AVE. In line with the criteria proposed by Sarstedt et al. ( 2014 ), AVE values above 0.5 denote satisfactory convergent validity. As evidenced in Table 2 , all constructs not only met but often exceeded this criterion, pointing to strong convergent validity.
Discriminant validity measures the extent to which a construct is truly distinct from others in the model (Martins et al., 2023 ; Shahzad, Xu, Khan, et al., 2023 ; Shahzad, Xu, Rehman, et al., 2023 ). This distinctiveness ensures that the constructs do not overlap, adding credence to the model’s unique explanations for the observed phenomena (Martins et al., 2023 ). We employed the Fornell-Larcker ( 1981 ) criterion for this purpose. Essentially, for robust discriminant validity, the square root of the AVE for a given construct should exceed its highest correlation with any other construct (Fornell and Larcker, 1981 ). Table 3 provides a matrix depicting these values. The diagonal, representing the square root of AVE values, is consistently larger than the off-diagonal values in their respective rows and columns, which are the correlations between constructs.
Structural model
The structural model’s examination offers insights into the relationships between constructs and evaluates the hypothesized pathways (Farrukh et al., 2023 ). Using bootstrapping in PLS-SEM, we deciphered the direct, mediating, and moderating effects of the constructs concerning cryptocurrency adoption. Table 4 provides a summary of these findings.
Main effects
The hypothesis positing a relationship between cryptocurrency awareness and cryptocurrency adoption (H 1 ) displayed a β -value of 0.192, significant at the 0.05 level. This means that as cryptocurrency awareness increases, there is a corresponding increase in the likelihood of cryptocurrency adoption. This supports the notion that more knowledgeable or informed individuals about cryptocurrencies are more inclined to adopt them. The fact that H 1 is supported highlights the importance of educating and raising awareness among potential users to promote cryptocurrency adoption.
Other observations indicate that enhanced cryptocurrency awareness bolsters the perception of its ease of use ( β : 0.470, p < 0.01) and its overall usefulness ( β : 0.288, p < 0.001), and that the ease of use ( β : 0.220, p < 0.01) and usefulness ( β : 0.134, p < 0.05) of cryptocurrency are significant determinants of its adoption. Noteworthily, a heightened trust in cryptocurrency significantly augments its ease of use ( β : 0.224, p < 0.01) and usefulness ( β : 0.220, p < 0.01). Additionally, the R 2 values depict the proportion of variance in the dependent variable predicted by the independent variable(s). The current model elucidates 51.5% of the variance in cryptocurrency ease of use, 14.8% in cryptocurrency usefulness, and 18.9% in cryptocurrency adoption.
Mediation effects
The hypothesis suggesting that cryptocurrency awareness indirectly informs cryptocurrency adoption via its ease of use (H 2 ) is significant, with a β -value of 0.103 ( p < 0.01). As such, H 2 is supported. Similarly, the pathway from cryptocurrency awareness via its usefulness to its adoption (H 3 ) is corroborated with a significant β -value of 0.038 ( p < 0.05). As a result, H 3 is supported.
Noteworthily, mediating effects reveal the mechanism or process through which an independent variable influences a dependent variable. The positive β -value of 0.103 for H 2 indicates that an increase in cryptocurrency awareness boosts the perception of its ease of use, which subsequently amplifies the rate of cryptocurrency adoption. This finding emphasizes the importance of not only making potential users aware of cryptocurrencies but also ensuring that they find the technology easy to use. The easier a potential user perceives the use of cryptocurrencies, the higher the likelihood they will adopt it—especially when they are well-informed or aware. Similarly, the positive β -value of 0.038 for H 3 suggests that an enhanced awareness of cryptocurrency leads to an increased perception of its usefulness, consequently fostering its adoption. This points towards the value of positioning cryptocurrencies as not just a novel technology, but as a tool that has tangible benefits and applications in everyday life. An informed individual who perceives cryptocurrencies as beneficial or useful is more likely to embrace them.
Moderation effects
The hypothesis indicating that cryptocurrency trust moderates the pathway between cryptocurrency awareness, its ease of use, and subsequent adoption (H4a) is supported by a significant β -value of 0.164 ( p < 0.05). Trust also appears to play a moderating role between cryptocurrency awareness and its perceived usefulness, affecting its adoption (H4b). This is evidenced by a significant β -value of 0.117 ( p < 0.05), thus supporting H4b.
The positive β -values for the moderating effect of cryptocurrency trust on the relationships in H 4a and H 4b underscore the enhancing role trust plays in these relationships. For H 4a , with a β -value of 0.164, this suggests that as trust in cryptocurrency increases, the positive relationship between cryptocurrency awareness and its ease of use in predicting cryptocurrency adoption becomes even stronger. This can be interpreted as trust amplifying the effect of awareness on adoption through ease of use. It implies that when individuals have higher trust in cryptocurrency, the awareness they have about it becomes more effective in shaping their perceptions of its ease of use, subsequently leading to higher adoption rates. Similarly, for H 4b , the positive β -value of 0.117 implies that increased trust in cryptocurrency augments the relationship between cryptocurrency awareness and its usefulness in determining adoption. This indicates that the beneficial effect of being aware of cryptocurrency on its usefulness becomes more pronounced when individuals trust cryptocurrency. This elevated perception of usefulness, in turn, makes it more likely for them to adopt cryptocurrency.
In the digital age, with technological advancements creating ripples throughout every industry, the finance sector has also seen significant shifts. Cryptocurrency, a nascent financial instrument, has brought about a paradigm shift in the way we perceive and handle monetary transactions. These digital assets, from their inception, have not only prompted discussions around technological intricacies but have also spurred debates in relation to awareness, usability, and trust. Our study aims to add to this discourse by illuminating cryptocurrency awareness, acceptance, and adoption.
On cryptocurrency awareness as the main predictor
The current research confirms that a clear understanding or awareness of cryptocurrency plays a pivotal role in its adoption. This aligns with the intuitive idea that when people understand something, they are more likely to engage with it. This finding resonates with Gong et al. ( 2023 ) assertion that a person’s ability to recognize and comprehend the efficacy of a technological innovation, in this case, cryptocurrency, is crucial for its acceptance in the market. Essentially, the more informed an individual is about cryptocurrency, the more likely they are to venture into its use without apprehension.
On cryptocurrency, ease of use, and usefulness as mediators
The present study also illuminates the vital roles that cryptocurrency ease of use and usefulness play in the adoption process. These factors essentially dictate the user’s experience and the perceived tangible benefits of engaging with cryptocurrency.
On the one hand, cryptocurrency ease of use emphasizes the importance of user-friendly experiences in technology adoption, a sentiment resonant with Chen and Aklikokou ( 2020 ) research. If potential users perceive a technology as complicated or inaccessible, they are less likely to engage with it, even if they are aware of its existence.
On the other hand, cryptocurrency’s usefulness underscores the tangible benefits users derive from the technology. This aligns with Theiri et al. ( 2022 ) assertion that the perceived usefulness of technological innovation can heavily impact one’s intent to engage with it. For cryptocurrencies to become mainstream, platforms need to clearly elucidate not just the ‘how-to’ but also the ‘why’ of their technology.
On cryptocurrency trust as a moderator
The discussion on cryptocurrency adoption is incomplete without addressing cryptocurrency trust. Trust emerges as a significant moderator, bridging the gap between awareness and adoption. The research aligns with Zafar et al. ( 2021 ) stance that trust can significantly reduce the ambiguity and potential perceived risks associated with adopting new technologies. In the world of digital transactions, where tangible cash does not exchange hands, cryptocurrency trust becomes a linchpin ensuring the smooth transition from traditional to digital currencies.
Theoretical implications
The evolution of technology, especially in the realm of financial systems, necessitates a continuous reassessment and refinement of existing theoretical and conceptual frameworks. Cryptocurrencies, with their transformative potential and inherent complexities, present an opportune context for examining the adaptability and relevance of established theories. Against this backdrop, our exploration into the application and extension of TAM for understanding cryptocurrency adoption stands as a testimony to the model’s versatility and the necessity for its evolution in line with contemporary technological challenges. Delving deeper into the findings, several pivotal theoretical implications emerge, which are expounded upon below.
Extending TAM to cryptocurrencies
Since its inception, TAM has served as a linchpin in understanding technology acceptance across various domains (Lim, 2018 ). In expanding TAM’s purview to include cryptocurrencies, this study embarks on uncharted theoretical territory. The implications of this extension are manifold. Firstly, it attests to the dynamism and versatility of TAM as a model, proving its applicability even in contexts as nascent and volatile as cryptocurrency. Secondly, it allows for a structured analysis of an area that, despite its growing relevance, remains under-theorized. By providing a structured lens, the extended TAM offers a blueprint for future researchers to further investigate and understand the nuances of cryptocurrency adoption.
Incorporation of awareness in TAM
Awareness, as highlighted by this study, is not merely an ancillary factor but a core determinant in the adoption process, especially for emergent technologies like cryptocurrency. While traditional TAM constructs acknowledge the importance of prior experience (Shahzad et al., 2018 ), the explicit introduction of awareness propels it to the forefront of technology acceptance discussions. This enhancement suggests that for newer technologies, a foundational level of knowledge or awareness might be a pre-requisite before users can even begin to gauge the ease of use or usefulness. This research’s emphasis on awareness invites future theoretical explorations on how awareness is cultivated, its potential barriers, and its progressive influence on user acceptance as technologies evolve.
Introduction of trust in TAM
Trust, particularly in financial domains, is a multifaceted construct that encompasses cognitive, emotional, and behavioral dimensions. By weaving trust into the fabric of TAM as a moderating entity, this study illuminates the complexities between logic-driven usability concerns and emotionally charged trust issues. Recognizing trust as a moderator showcases the intricate balance consumers must strike when considering the adoption of financial technologies: while they may perceive cryptocurrencies as easy to use or useful, trust or lack thereof can significantly skew these perceptions and consequent behaviors. This enriched understanding of trust’s moderating role invites deeper dives into the nuances of trust—how it is established, maintained, and potentially restored—in the context of the technology adoption of cryptocurrency.
Responding to scholarly calls
Academic discourse thrives on the interconnectedness of scholarly pursuits. By heeding the calls of Kumar et al. ( 2021 ) and others, this study stands as a testament to the collaborative nature of academic advancement. Addressing gaps identified by predecessors not only strengthens the study’s relevance but also positions it as a responsive piece of scholarly work. It also sets the stage for potential reciprocal advancements, as future researchers might build upon these findings, leading to an iterative and collaborative expansion of knowledge in the domain, in this case, cryptocurrency.
Theoretical generalizability and extension
As Lim ( 2018 ) emphasized, the true strength of a theory lies not just in its foundational tenets but also in its adaptability to new contexts and challenges. By demonstrating TAM’s applicability in the cryptocurrency arena and further enhancing it with new constructs, this study magnifies the theory’s robustness. This act of both generalizing and extending underscores the importance of continually revisiting and refining theoretical models, ensuring they remain relevant and reflective of evolving technological landscapes.
Practical implications
The findings of this research, while deeply embedded in academic exploration, carry profound implications for real-world applications, especially for policymakers, financial institutions, and technology developers. As the digital world becomes increasingly intertwined with daily life, understanding the determinants that shape the adoption of emerging financial technologies such as cryptocurrencies is of paramount importance.
Nurturing cryptocurrency awareness
Our study emphasizes the centrality of awareness in the adoption process. For policymakers and institutions keen on promoting cryptocurrencies, it becomes imperative to first ensure that the general populace has a foundational understanding of what these digital currencies entail. This does not just mean superficial knowledge but a deeper comprehension of how cryptocurrencies work, their benefits, potential risks, and the broader implications for the financial landscape. Financial institutions, educational institutions, and governments can collaborate to create awareness campaigns, host workshops, or develop educational modules tailored to different demographic groups. Such initiatives can demystify the concept of cryptocurrencies and potentially accelerate their mainstream acceptance.
Strengthening trust in cryptocurrency
The inherent nature of cryptocurrency, with its foundation in cryptographic algorithms and blockchain technology, already provides a level of security and transparency that is unparalleled in many traditional financial systems. Blockchain’s decentralized and immutable ledger ensures that transactions are transparent and resistant to tampering. However, trust in cryptocurrency goes beyond the mere technological facets.
To begin, while blockchain and cryptography form the core of the technology, the platforms, wallets, exchanges, and interfaces that users interact with are not infallible. These can be susceptible to hacking, mismanagement, or fraudulent schemes, as has been evidenced by numerous high-profile cryptocurrency thefts and exchange failures. To address these concerns, tech developers and financial institutions should continuously invest in enhancing the security of these peripheral platforms. This can include multi-factor authentication, cold storage solutions, and regular security audits.
Next, for many potential adopters, the complex technicalities of blockchain and cryptography can be overwhelming. They might not fully grasp the intricacies of how these technologies bolster security, leading to hesitations in adoption. To mitigate this, there is a need for transparent communication that demystifies these concepts for the average user. Educational initiatives that simplify and explain the technological underpinnings can foster trust among users who might be on the fence due to a lack of understanding.
Lastly, the regulatory landscape plays a pivotal role in shaping trust. In the absence of clear regulatory guidelines, the cryptocurrency market can become a wild west of sorts, with potential risks for investors and users. Policymakers can help cultivate trust by introducing balanced regulations that protect users without stifling innovation. This could involve setting standards for cryptocurrency exchanges, mandating transparency in initial coin offerings (ICOs), or establishing a legal framework for the resolution of disputes in the crypto domain.
Tailoring education and outreach initiatives
The interplay between awareness, ease of use, and usefulness suggests that a one-size-fits-all approach to cryptocurrency education might be suboptimal. Different demographic groups might have varied levels of technological proficiency and financial literacy. Policymakers and institutions should consider these nuances when designing outreach programs. For instance, younger demographics might be more receptive to digital workshops or mobile apps that educate about cryptocurrencies, while older groups might benefit from traditional seminars or literature.
Informed policy making
Our research equips policymakers with insights into the multifaceted factors that influence cryptocurrency adoption. With a nuanced understanding of the role of awareness and trust, governments can make informed decisions when crafting policies around cryptocurrency regulation. For example, by recognizing the importance of trust, policymakers might emphasize transparency and accountability in cryptocurrency transactions, mandating periodic audits or setting up regulatory bodies specifically for monitoring cryptocurrency-related activities.
In a rapidly evolving digital landscape, the role of cryptocurrencies has emerged as a significant paradigm shift in our understanding of finance and monetary transactions. This study, through its adoption and extension of TAM to the realm of cryptocurrency, has cast light upon the intricate dynamics underpinning the acceptance and adoption of such innovative financial technologies.
The findings suggest that awareness is a foundational element in the acceptance process. A heightened level of understanding substantially bolsters consumers’ perceptions regarding the ease of use and usefulness of cryptocurrencies. Noteworthily, the established constructs of ease of use and usefulness in TAM remain significant predictors for cryptocurrency adoption, emphasizing the robustness of the model even in novel contexts. More importantly, trust acts as a pivotal moderating variable. While the inherent cryptographic nature and blockchain foundations of cryptocurrency provide a baseline of trust, it is the additional psychological facets of confidence and reliability that influence adoption.
Theoretically, our findings serve as a testament to the generalizability and versatility of TAM, affirming its applicability even in complex, relatively nascent domains like cryptocurrency. By integrating the pivotal variables of awareness and trust into the model, this research has not only enriched TAM but has also provided a nuanced understanding of how individuals perceive, trust, and ultimately decide to use or abstain from using cryptocurrencies. Our study underscores that while technological advancements like cryptography and blockchain lay the groundwork, the human factors of awareness and trust become instrumental in the decision-making processes surrounding technology adoption in financial contexts.
Practically, our findings carry profound implications for policymakers, financial institutions, and tech developers. The recognition that awareness and trust are cardinal in driving cryptocurrency adoption means stakeholders have a roadmap for fostering a more inclusive and transparent digital financial ecosystem. Whether it is through comprehensive educational campaigns, fortified security measures, or sensible regulation, the path to bolstering public confidence and understanding in cryptocurrencies has been delineated.
As with all explorations, our study opens the doors to numerous avenues for future research. Questions regarding the long-term sustainability of cryptocurrencies, the evolving regulatory landscape, or the potential integration of other behavioral and societal factors into the adoption model all warrant further investigation. Additionally, as the world of cryptocurrency continues to evolve with the introduction of new technologies and platforms, continuous assessment of user acceptance and trust will be paramount.
Despite the important contributions this study offers, several limitations exist that provide avenues for future research endeavors to delve deeper into the underlying problem. One salient limitation is the focus on a demographic predominantly consisting of graduates, whose perspectives and lifestyles might differ considerably from those who are less educated. Their propensity to be more liberal, technologically advanced, and quicker to engage with novel concepts might influence their receptiveness to cryptocurrencies. Future studies could benefit from exploring different demographic variables and diverse population samples.
Another constraint is the geographical scope of the study. The data were predominantly drawn from respondents in Lahore, Pakistan. Expanding this to other prominent cities like Faisalabad, Multan, Islamabad, and Karachi could present a more comprehensive picture of cryptocurrency acceptance across different urban contexts in the country. Moreover, an international comparison of cryptocurrency adaptability, contrasting Pakistan with both developed and developing nations, might provide intriguing insights.
Furthermore, while this research has prioritized awareness as a central variable, there are other pertinent variables left uncharted. Aspects such as government support, societal influences, and even technological design could be examined within the framework of TAM in subsequent studies.
Moreover, it is important to note the specificity of the variables explored. This research primarily centered on adoption-centric variables of cryptocurrencies. Expansive variables, such as the long-term sustainability of cryptocurrencies and facets like Bitcoin mining, warrant examination in future research endeavors.
Last but not least, a procedural limitation arises from the methodology employed: data collection at a single point in time using an adapted questionnaire. It would be invaluable for future researchers to embrace a longitudinal research approach, which would lend robustness and ensure the consistency and validity of findings over time.
In closing, this study underscores the transformative potential of cryptocurrencies in reshaping our financial future. However, for this potential to be fully realized, understanding and addressing the intricacies of user awareness, acceptance, and adoption, as delineated in this research, will be pivotal. We remain hopeful that our findings will serve as a cornerstone for both academic and practical endeavors in the realm of cryptocurrency, propelling us towards a more inclusive, transparent, and trustworthy digital financial landscape.
Data availability
The data used in this study can be made available by the corresponding author(s) upon reasonable request.
Abdul-Rahim R, Bohari SA, Aman A, Awang Z (2022) Benefit–risk perceptions of fintech adoption for sustainability from bank consumers’ perspective: the moderating role of fear of COVID-19. Sustainability (Switzerland), 14(14). https://doi.org/10.3390/su14148357
Aboalsamh HM, Khrais LT, Albahussain SA (2023) Pioneering perception of green fintech in promoting sustainable digital services application within smart cities. Sustainability (Switz) 15(14):1–13. https://doi.org/10.3390/su151411440
Article Google Scholar
Akther T, Nur T (2022) A model of factors influencing COVID-19 vaccine acceptance: a synthesis of the theory of reasoned action, conspiracy theory belief, awareness, perceived usefulness, and perceived ease of use. PLoS ONE 17(Jan):1–20. https://doi.org/10.1371/journal.pone.0261869
Article CAS Google Scholar
Albayati H, Kim SK, Rho JJ (2020) Accepting financial transactions using blockchain technology and cryptocurrency: a customer perspective approach. Technol Soc 62:101320. https://doi.org/10.1016/j.techsoc.2020.101320
Allen F, Gu X, Jagtiani J (2022) Fintech, cryptocurrencies, and CBDC: financial structural transformation in China. J Int Money Financ 124:102625. https://doi.org/10.1016/j.jimonfin.2022.102625
Almajali DA, Masa’Deh R, Dahalin ZMD (2022) Factors influencing the adoption of Cryptocurrency in Jordan: an application of the extended TRA model. Cogent Soc Sci 8(1). https://doi.org/10.1080/23311886.2022.2103901
Arpaci I, Bahari M (2023) A complementary SEM and deep ANN approach to predict the adoption of cryptocurrencies from the perspective of cybersecurity. Elsevier. https://doi.org/10.1016/j.chb.2023.107678
Basuki R, Tarigan ZJH, Siagian H, Limanta LS, Setiawan D, Mochtar J (2022) The effects of perceived ease of use, usefulness, enjoyment and intention to use online platforms on behavioral intention in online movie watching during the pandemic era. Int J Data Netw Sci 6(1):253–262. https://doi.org/10.5267/J.IJDNS.2021.9.003
Bibi S (2023) Money in the time of crypto. Res Int Bus Financ 65:101964. https://doi.org/10.1016/j.ribaf.2023.101964
Biswas A, Bhattacharya D, Kumar KA (2021) DeepFake detection using 3D-Xception net with discrete Fourier transformation. J Inf Syst Telecommun 9(35):161–168. https://doi.org/10.52547/jist.9.35.161
Chen L, Aklikokou AK (2020) Determinants of E-government Adoption: testing the mediating effects of perceived usefulness and perceived ease of use. Int J Public Adm 43(10):850–865. https://doi.org/10.1080/01900692.2019.1660989
Davis FD (1989) Perceived usefulness, perceived ease of use, and user acceptance of information technology. MIS Q: Manag Inf Syst 13(3):319–339. https://doi.org/10.2307/249008
Deebak BD, Memon FH, Dev K, Khowaja SA, Wang W, Qureshi NMF (2022) TAB-SAPP: a trust-aware blockchain-based seamless authentication for massive IoT-enabled industrial applications. IEEE Trans Ind Inform 19(1):243–250. https://doi.org/10.1109/TII.2022.3159164
Entrialgo M (2017) Are the intentions to entrepreneurship of men and women shaped differently? The impact of entrepreneurial role-model exposure and entrepreneurship education. Entrepreneurship Res J 1–14. https://doi.org/10.1515/erj-2017-0013
Fagan M, Kilmon C, Pandey V (2012) Exploring the adoption of a virtual reality simulation: The role of perceived ease of use, perceived usefulness and personal innovativeness. Campus-Wide Inf Syst 29(2):117–127. https://doi.org/10.1108/10650741211212368
Farrukh M, Xu S, Baheer R, Ahmad W (2023) Unveiling the role of supply chain parameters approved by blockchain technology towards firm performance through trust: the moderating role of government support. Heliyon 9(11):e21831. https://doi.org/10.1016/j.heliyon.2023.e21831
Farrukh M, Xu S, Naveed W, Nusrat S (2023) Investigating the impact of artificial intelligence on human resource functions in the health sector of China: a mediated moderation model. Heliyon 9(11):e21818. https://doi.org/10.1016/j.heliyon.2023.e21818
Fornell C, Larcker DF (1981) Evaluating structural equation models with unobservable variables and measurement error. J Mark Res 18(1):39. https://doi.org/10.2307/3151312
Gaikwad A, Mavale S (2021) The impact of cryptocurrency adoption as a legal tender in El salvador. Int J Eng Manag Res 11(6):112–115. https://doi.org/10.31033/ijemr.11.6.16
Galariotis E, Karagiannis K (2020) Cultural dimensions, economic policy uncertainty, and momentum investing: international evidence. Eur J Financ 0(0):1–18. https://doi.org/10.1080/1351847X.2020.1782959
Gong Y, Tang X, Chang EC (2023) Group norms and policy norms trigger different autonomous motivations for Chinese investors in cryptocurrency investment. Humanit Soc Sci Commun 10(1):1–10. https://doi.org/10.1057/s41599-023-01870-0
Granić A, Marangunić N (2019) Technology acceptance model in educational context: a systematic literature review. Br J Educ Technol 50(5):2572–2593. https://doi.org/10.1111/bjet.12864
Gupta K, Arora N (2020) Investigating consumer intention to accept mobile payment systems through unified theory of acceptance model: an Indian perspective. South Asian J Bus Stud 9(1):88–114. https://doi.org/10.1108/SAJBS-03-2019-0037
Hasan R, Miah MD, Hassan MK (2022) The nexus between environmental and financial performance: Evidence from gulf cooperative council banks. Bus Strategy Environ 31(7):2882–2907. https://doi.org/10.1002/bse.3053
Hasan SZ, Ayub H, Ellahi A, Saleem M (2022) A moderated mediation model of factors influencing intention to adopt cryptocurrency among university students. Hum Behav Emerg Technol 2022:1–14. https://doi.org/10.1155/2022/9718920
Hasgül E, Karataş M, Pak Güre MD, Duyan V (2023) A perspective from Turkey on construction of the new digital world: analysis of emotions and future expectations regarding Metaverse on Twitter. Humanit Soc Sci Commun 10(1):1–8. https://doi.org/10.1057/s41599-023-01958-7
Howson P, de Vries A (2022) Preying on the poor? Opportunities and challenges for tackling the social and environmental threats of cryptocurrencies for vulnerable and low-income communities. Energy Res Soc Sci 84(Aug):102394. https://doi.org/10.1016/j.erss.2021.102394
Jalan A, Matkovskyy R, Urquhart A, Yarovaya L (2023) The role of interpersonal trust in cryptocurrency adoption. J Int Financ Mark, Inst Money 83(Dec):101715. https://doi.org/10.1016/j.intfin.2022.101715
Johar S, Ahmad N, Asher W, Cruickshank H, Durrani A (2021) Research and applied perspective to blockchain technology: a comprehensive survey. Appl Sci 11(14):6252. https://doi.org/10.3390/app11146252
Kakinaka S, Umeno K (2022) Cryptocurrency market efficiency in short- and long-term horizons during COVID-19: An asymmetric multifractal analysis approach. Financ Res Lett 46(PA):102319. https://doi.org/10.1016/j.frl.2021.102319
Khan MZ, Ali Y, Sultan HBin, Hasan M, Baloch S (2020) Future of currency: a comparison between traditional, digital fiat and cryptocurrency exchange mediums. Int J Blockchain Cryptocurrencies 1(2):206. https://doi.org/10.1504/ijbc.2020.109003
Kher R, Terjesen S, Liu C (2021) Blockchain, Bitcoin, and ICOs: a review and research agenda. Small Bus Econ 56(4):1699–1720. https://doi.org/10.1007/s11187-019-00286-y
Kim J, Merrill K, Collins C (2021) AI as a friend or assistant: the mediating role of perceived usefulness in social AI vs. functional AI. Telemat Inform 64(Aug):101694. https://doi.org/10.1016/j.tele.2021.101694
Kumar S, Lim WM, Pandey N, Westland JC (2021) 20 years of electronic commerce research. In: Electronic Commerce Research (vol. 21, Issue 1). Springer, USA
Kumar S, Lim WM, Sivarajah U, Kaur J (2023) Artificial Intelligence and Blockchain Integration in Business: Trends from A Bibliometric-content Analysis. Inf Syst Front 871–896. https://doi.org/10.1007/s10796-022-10279-0
Li C, Khaliq N, Chinove L, Khaliq U, Popp J, Oláh J (2023) Cryptocurrency acceptance model to analyze consumers’ usage intention: evidence from Pakistan. SAGE Open 13(1):1–19. https://doi.org/10.1177/21582440231156360
Li J (2023) Predicting the demand for central bank digital currency: a structural analysis with survey data. J Monetary Econ 134:73–85. https://doi.org/10.1016/j.jmoneco.2022.11.007
Lim WM (2018). Dialectic antidotes to critics of the technology acceptance model: conceptual, methodological, and replication treatments for behavioural modelling in technology-mediated environments. 22, 1–11. https://doi.org/10.3127/ajis.v22i0.1651
Lu A, Deng R, Huang Y, Song T, Shen Y, Fan Z, Zhang J (2022) The roles of mobile app perceived usefulness and perceived ease of use in app-based Chinese and English learning flow and satisfaction. Educ Inf Technol 27(7):10349–10370. https://doi.org/10.1007/s10639-022-11036-1
Martins JM, Shahzad MF, Javed I (2023) Assessing the impact of workplace harassment on turnover intention. Evid Bank Ind 7(5):1699–1722. https://doi.org/10.28991/ESJ-2023-07-05-016
Martins JM, Muhammad FS, Shuo X (2023) Examining the factors influencing entrepreneurial intention to initiate new ventures: Focusing on knowledge of entrepreneurial skills, ability to take risk and entrepreneurial innovativeness in open innovation business model. Res Sq 1125–1146. https://doi.org/10.21203/rs.3.rs-2664778/v1
Martins JM, Shahzad MF, Xu S (2023) Factors influencing entrepreneurial intention to initiate new ventures: evidence from university students. J Innov Entrep. https://doi.org/10.1186/s13731-023-00333-9
Matemba ED, Li G (2018) Technology in society consumers ’ willingness to adopt and use WeChat wallet: an empirical study in South Africa. Technol Soc 53:55–68. https://doi.org/10.1016/j.techsoc.2017.12.001
McCloskey DW (2006) The importance of ease of use, usefulness, and trust to online consumers: An examination of the technology acceptance model with older customers. J Organ End Use Comput (JOEUC) 18(3):47–65. https://doi.org/10.4018/joeuc.2006070103
Mizanur RM, Sloan TR (2017) User adoption of mobile commerce in Bangladesh: Integrating perceived risk, perceived cost and personal awareness with TAM. Int Technol Manag Rev 103–124. https://doi.org/10.2991/itmr.2017.6.3.4
Nadeem MA, Liu Z, Pitafi AH, Younis A, Xu Y (2021) Investigating the adoption factors of cryptocurrencies—a case of Bitcoin: empirical evidence from China. SAGE Open 11(1). https://doi.org/10.1177/2158244021998704
Namahoot KS, Rattanawiboonsom V (2022) Integration of TAM model of consumers’ intention to adopt cryptocurrency platform in Thailand: the mediating role of attitude and perceived risk. Hum Behav Emerg Technol 2022. https://doi.org/10.1155/2022/9642998
Parate S, Josyula HP, Reddi LT (2023) Digital identity verification: transforming KYC processes in banking through advanced technology and enhanced security measures. Int Res J Mod Eng Technol Sci 09:128–137. https://doi.org/10.56726/irjmets44476
Quan W, Moon H, Kim S (Sam), Han H(2023) Mobile, traditional, and cryptocurrency payments influence consumer trust, attitude, and destination choice: Chinese versus Koreans Int J Hospit Manag 108(Oct):103363. https://doi.org/10.1016/j.ijhm.2022.103363
Rejeb A, Rejeb K, Alnabulsi K, Zailani S (2023) Tracing knowledge diffusion trajectories in scholarly Bitcoin research: co-word and main path analyses. J Risk Financ Manag 16(8). https://doi.org/10.3390/jrfm16080355
Roscoe AM, Lang D, Sheth JN (1975) Follow-up methods, questionnaire length, and market differences in mail surveys. J Mark 39(2):20. https://doi.org/10.2307/1250111
Sagheer N, Khan KI, Fahd S, Mahmood S, Rashid T, Jamil H (2022). Factors affecting adaptability of cryptocurrency: an application of technology acceptance model. Front Psychol 13. https://doi.org/10.3389/fpsyg.2022.903473
Sahoo S, Kumar S, Sivarajah U, Lim WM, Westland JC, Kumar A (2022). Blockchain for sustainable supply chain management: trends and ways forward. In: Electronic Commerce Research (Issue 0123456789). Springer, USA
Salas A (2020) Literature review of faculty-perceived usefulness of instructional technology in classroom dynamics. Contemp Educ Technol 7(2):174–186. https://doi.org/10.30935/cedtech/6170
Sarstedt M, Ringle CM, Hair JF (2014) PLS-SEM: looking back and moving forward. Long Range Plan 47(3):132–137. https://doi.org/10.1016/j.lrp.2014.02.008
Schaupp LC, Festa M (2018) Cryptocurrency adoption and the road to regulation. Proceedings of the 19th Annual International Conference on Digital Government Research: Governance in the Data Age, 1–9. https://doi.org/10.1145/3209281.3209336
Shahzad F, Shahzad MF, Dilanchiev A, Irfan M (2022) Modeling the influence of paternalistic leadership and personality characteristics on alienation and organizational culture in the aviation industry of Pakistan: the mediating role of cohesiveness. Sustainability (Switzerland), 14(22). https://doi.org/10.3390/su142215473
Shahzad F, Xiu GY, Wang J, Shahbaz M (2018) An empirical investigation on the adoption of cryptocurrencies among the people of mainland China. Technol Soc 55(Jan):33–40. https://doi.org/10.1016/j.techsoc.2018.05.006
Shahzad MF, Khan KI, Saleem S, Rashid T (2021) What factors affect the entrepreneurial intention to start-ups? The role of entrepreneurial skills, propensity to take risks, and innovativeness in open business models. https://doi.org/10.3390/joitmc7030173
Shahzad MF, Xu S, Khan KI, Hasnain MF (2023) Effect of social influence, environmental awareness, and safety affordance on actual use of 5G technologies among Chinese students. Sci Rep 0123456789, 1–16. https://doi.org/10.1038/s41598-023-50078-4
Shahzad MF, Xu S, Rehman O, Javed I (2023) Impact of gamification on green consumption behavior integrating technological awareness, motivation, enjoyment and virtual CSR. Sci Rep 1–18. https://doi.org/10.1038/s41598-023-48835-6
Shin D, Rice J (2022) Cryptocurrency: a panacea for economic growth and sustainability? A critical review of crypto innovation. Telemat Inform 71(Apr):101830. https://doi.org/10.1016/j.tele.2022.101830
Siagian H, Tarigan ZJH, Basana SR, Basuki R (2022) The effect of perceived security, perceived ease of use, and perceived usefulness on consumer behavioral intention through trust in digital payment platform. Int J Data Netw Sci 6(3):861–874. https://doi.org/10.5267/j.ijdns.2022.2.010
Siska E (2023) Digital bank financial soundness analysis at PT Bank Jago Tbk. CAMEL Framew Approach 3(3):527–532. https://doi.org/10.47065/arbitrase.v3i3.700
Stocklmayer S, Gilbert JK (2002) New experiences and old knowledge: Towards a model for the personal awareness of science and technology. Int J Sci Educ 24(8):835–858. https://doi.org/10.1080/09500690210126775
Sudzina F, Dobes M, Pavlicek A (2023) Towards the psychological profile of cryptocurrency early adopters: Overconfidence and self-control as predictors of cryptocurrency use. Curr Psychol 42(11):8713–8717. https://doi.org/10.1007/s12144-021-02225-1
Sukumaran S, Bee TS, Wasiuzzaman S (2022) Cryptocurrency as an investment: the Malaysian Context. Risks 10(4). https://doi.org/10.3390/risks10040086
Tan TM, Saraniemi S (2022) Trust in blockchain-enabled exchanges: future directions in blockchain marketing. J Acad Market Sci 0123456789. https://doi.org/10.1007/s11747-022-00889-0
Tandon C, Revankar S, Parihar SS (2021) How can we predict the impact of the social media messages on the value of cryptocurrency? Insights from big data analytics. Int J Inf Manag Data Insights 1(2):100035. https://doi.org/10.1016/j.jjimei.2021.100035
Tauni MZ, Fang HX, Rao ZuR, Yousaf S (2015) The influence of Investor personality traits on information acquisition and trading behavior: evidence from Chinese futures exchange. Personal Individ Differ 87(Aug):248–255. https://doi.org/10.1016/j.paid.2015.08.026
Theiri S, Nekhili R, Sultan J (2022) Cryptocurrency liquidity during the Russia–Ukraine war: the case of Bitcoin and Ethereum. J Risk Financ 24(1):59–71. https://doi.org/10.1108/JRF-05-2022-0103
Treiblmaier H, Sillaber C (2021) The impact of blockchain on e-commerce: a framework for salient research topics. Electron Commer Res Appl 48(Apr):101054. https://doi.org/10.1016/j.elerap.2021.101054
Uematsu Y, Tanaka S (2019) High‐dimensional macroeconomic forecasting and variable selection via penalized regression. Econ J 22(1):34–56. https://doi.org/10.1111/ectj.12117
Article MathSciNet Google Scholar
Utz M, Johanning S, Roth T, Bruckner T, Strüker J (2022) From ambivalence to trust: using blockchain in customer loyalty programs. Int J Inf Manag 68(Mar):102496. https://doi.org/10.1016/j.ijinfomgt.2022.102496
Völter F, Urbach N, Padget J (2021) Trusting the trust machine: evaluating trust signals of blockchain applications. Int J Inf Manag 68(Sept). https://doi.org/10.1016/j.ijinfomgt.2021.102429
Voorhees CM, Brady MK, Calantone R, Ramirez E (2016) Discriminant validity testing in marketing: an analysis, causes for concern, and proposed remedies. J Acad Mark Sci 44(1):119–134. https://doi.org/10.1007/s11747-015-0455-4
Wibasuri A (2022) Exploring the impact of relevant factors on the acceptance of cryptocurrency mobile apps: an extended technology acceptance model (TAM-3). 6(June). https://doi.org/10.29099/ijair.v6i1.1.971
Yayla A, Dincelli E, Parameswaran S (2023) A mining town in a digital land: browser-based cryptocurrency mining as an alternative to online advertising. Inf Syst Front 0123456789. https://doi.org/10.1007/s10796-023-10386-6
Yuen KF, Cai L, Qi G, Wang X (2021) Factors influencing autonomous vehicle adoption: an application of the technology acceptance model and innovation diffusion theory. Technol Anal Strateg Manag 33(5):505–519. https://doi.org/10.1080/09537325.2020.1826423
Zafar S, Riaz S, Mahmood W (2021). Conducting the cashless revolution in Pakistan using enterprise integration. August, 12–25. https://doi.org/10.5815/ijeme.2021.04.02
Zou Z, Liu X, Wang M, Yang X (2023) Insight into digital finance and fintech: a bibliometric and content analysis. Technol Soc 73(Oct):102221. https://doi.org/10.1016/j.techsoc.2023.102221
Download references
Acknowledgements
This work received financial support from the National Natural Science Foundation of China under grant numbers 72074014 and 72174016.
Author information
Authors and affiliations.
College of Economics and Management, Beijing University of Technology, Beijing, China
Muhammad Farrukh Shahzad & Shuo Xu
Sunway Business School, Sunway University, Sunway, Selangor, Malaysia
Weng Marc Lim
School of Business, Law and Entrepreneurship, Swinburne University of Technology, Hawthorn, VIC, Australia
Faculty of Business, Design and Arts, Swinburne University of Technology, Kuching, Sarawak, Malaysia
Department of Chemistry, University of Agriculture, Faisalabad, 38000, Pakistan
Muhammad Faisal Hasnain
College of Environment and Life Science, Beijing University of Technology, 100124, Beijing, People’s Republic of China
Shahneela Nusrat
You can also search for this author in PubMed Google Scholar
Contributions
Conceptualization of study, data analysis, and original draft preparation, MFS; Preparation of data file, interpretation, results, and discussion, SN and MFH; Supervision, reviewing, and editing, WML; Project administration, and resource management, SX and MFS; Funding, SX. All authors have read and agreed to the published version of the manuscript.
Corresponding author
Correspondence to Shuo Xu .
Ethics declarations
Competing interests.
The authors declare no competing interests.
Ethical approval
The Ethics Committee of Beijing University of Technology, China, approved this study under B20221105W. This study was conducted according to the 1964 Helsinki Declaration and Guidelines.
Informed consent
Informed implied consent was obtained from all participants involved in the study. In alignment with national regulations and institutional guidelines, this research did not necessitate written informed consent. Instead, participants were required to complete an online informed consent process. During this process, they were clearly informed about the following key aspects: (i) confidentiality, wherein personal information provided by the participants would remain confidential and would not be published or disclosed, and (ii) use of data, wherein the data collected from the participants would be exclusively used for academic research purposes and not for any commercial activities. To proceed with their participation in the study, participants had to explicitly acknowledge their understanding and agreement by clicking the “agree and continue” button. This action served as their consent to participate and allowed them to access and complete the questionnaires.
Additional information
Publisher’s note Springer Nature remains neutral with regard to jurisdictional claims in published maps and institutional affiliations.
Supplementary information
Data set, 332 responses, rights and permissions.
Open Access This article is licensed under a Creative Commons Attribution 4.0 International License, which permits use, sharing, adaptation, distribution and reproduction in any medium or format, as long as you give appropriate credit to the original author(s) and the source, provide a link to the Creative Commons license, and indicate if changes were made. The images or other third party material in this article are included in the article’s Creative Commons license, unless indicated otherwise in a credit line to the material. If material is not included in the article’s Creative Commons license and your intended use is not permitted by statutory regulation or exceeds the permitted use, you will need to obtain permission directly from the copyright holder. To view a copy of this license, visit http://creativecommons.org/licenses/by/4.0/ .
Reprints and permissions
About this article
Cite this article.
Shahzad, M.F., Xu, S., Lim, W.M. et al. Cryptocurrency awareness, acceptance, and adoption: the role of trust as a cornerstone. Humanit Soc Sci Commun 11 , 4 (2024). https://doi.org/10.1057/s41599-023-02528-7
Download citation
Received : 20 May 2023
Accepted : 08 December 2023
Published : 02 January 2024
DOI : https://doi.org/10.1057/s41599-023-02528-7
Share this article
Anyone you share the following link with will be able to read this content:
Sorry, a shareable link is not currently available for this article.
Provided by the Springer Nature SharedIt content-sharing initiative
Quick links
- Explore articles by subject
- Guide to authors
- Editorial policies

Cryptocurrencies: applications and investment opportunities
Journal of Capital Markets Studies
ISSN : 2514-4774
Article publication date: 4 October 2019
Issue publication date: 5 November 2019
This study investigates the role of cryptocurrencies in enhancing the performance of portfolios constructed from traditional asset classes. Using a long sample period covering not only the large value increases but also the dramatic declines during the beginning of 2018, the purpose of this paper is to provide a more complete analysis of the dynamic nature of cryptocurrencies as individual investment opportunities, and as components of optimal portfolios.
Design/methodology/approach
The mean-variance optimization technique of Merton (1990) is applied to develop the risk and return characteristics of the efficient portfolios, along with the optimal weights of the asset class components in the portfolios.
The authors provide evidence that as a single investment, the best cryptocurrency is Ripple, followed by Bitcoin and Litecoin. Furthermore, cryptocurrencies have a useful role in the optimal portfolio construction and in investments, in addition to their original purposes for which they were created. Bitcoin is the best cryptocurrency enhancing the characteristics of the optimal portfolio. Ripple and Litecoin follow in terms of their usefulness in an optimal portfolio as single cryptocurrencies. Including all these cryptocurrencies in a portfolio generates the best (most optimal) results. Contributions of the cryptocurrencies to the optimal portfolio evolve over time. Therefore, the results and conclusions of this study have no guarantee for continuation in an exact manner in the future. However, the increasing popularity and the unique characteristics of cryptocurrencies will assist their future presence in investment portfolios.
Originality/value
This is one of the first studies that examine the role of popular cryptocurrencies in enhancing a portfolio composed of traditional asset classes. The sample period is the largest that has been used in this strand of the literature, and allows to compare optimal portfolios in early/recent subsamples, and during the pre-/post-cryptocurrency crisis periods.
- Portfolio optimization
- Cryptocurrencies
Inci, A.C. and Lagasse, R. (2019), "Cryptocurrencies: applications and investment opportunities", Journal of Capital Markets Studies , Vol. 3 No. 2, pp. 98-112. https://doi.org/10.1108/JCMS-05-2019-0032
Emerald Publishing Limited
Copyright © 2019, A. Can Inci and Rachel Lagasse
Published in Journal of Capital Markets Studies . Published by Emerald Publishing Limited. This article is published under the Creative Commons Attribution (CC BY 4.0) licence. Anyone may reproduce, distribute, translate and create derivative works of this article (for both commercial and non-commercial purposes), subject to full attribution to the original publication and authors. The full terms of this licence may be seen at http://creativecommons.org/licences/by/4.0/legalcode
1. Introduction
Cryptocurrencies are a basic topic of understanding to some, and a complete mystery to many. Throughout the past few years, these digital currencies have become the next big thing for select investors, but a topic of complete confusion and question for others. What are cryptocurrencies, and why have they become such a large topic for discussion as of recently? In the last quarter of 2017, this question was asked widely, as Bitcoin surged in value more than 2,500 percent from mid-December 2016 to mid-December 2017. The interest on cryptocurrencies increased even more after the great cryptocurrency crash in the beginning of 2018. In the course of one day, Bitcoin lost about 15 percent of its value, dropping from $13,500 to $11,500 in a matter of hours ( Burgess, 2018 ). Although Bitcoin is the most well-known digital currency, it is just one of the more than 1,500 cryptocurrencies. Other popular cryptocurrencies with the largest market capitalizations are Ethereum, Ripple and Litecoin. Cryptocurrencies operate differently and are distinguished from one another mainly due to their values, transaction speeds, usages and volatility characteristics.
Although cryptocurrencies were not originally created as investment assets, many investors use them as such. Due to the fact that cryptocurrencies are still relatively new in the time frame of the financial securities world, there has not been extensive research done on the effects of including them in a portfolio. This study aims to find diversification benefits of cryptocurrencies, and how they contribute to an optimal investment portfolio.
Our sample period covers most of the 2010s. We examine the most popular cryptocurrencies: Bitcoin, Ripple and Litecoin. We document that as an independent single investment, Ripple has the highest return, followed by Litecoin and Bitcoin. However, volatility of Ripple is also the highest, followed by those of Litecoin and Bitcoin. Combining risk and return together, the coefficient of variations reveal that the best cryptocurrency is Ripple, followed by Bitcoin, and then by Litecoin. All these cryptocurrencies have been better for investment purposes than the traditional asset classes in the 2010s.
We also examine the role of cryptocurrencies in optimal portfolios. We document that adding a cryptocurrency has always helped the optimal portfolio to achieve a better return and risk combination. Bitcoin has been the most useful cryptocurrency in this regard, followed by Ripple and Litecoin. We report that the contribution of the cryptocurrencies to an optimal portfolio is dynamic and, therefore, evolves over time. The cryptocurrency crash clearly has led to reduction in the attractiveness of cryptocurrencies as investment alternatives but the dynamic characteristics of cryptocurrencies warrant their future demand in investment portfolios. We conclude in our study that popular cryptocurrencies have had a useful role in portfolio construction and in investments, in addition to their original purposes for which they were created.
We would also like to point out that even though the investment portfolio benefits of cryptocurrencies are clear in the 2000s, the future is always unpredictable and the past results and conclusions need not necessarily extend into the future. From a qualitative perspective, the popularity of and familiarity with cryptocurrencies increase continually. The variety of these investment tools is also expanding. The blockchain technology with which most cryptocurrencies have strong links is getting more and more into the mainstream of institutional and corporate operations. With all these continual developments, it is a safe conjecture that cryptocurrencies will have an important role in investment portfolios. However, the return and covariance characteristics observed and reported in our paper are historical; with no guarantee for continuation in an exact manner into the future.
The rest of the paper is organized as follows. Section 2 presents the literature review with an overview of cryptocurrencies, along with their popularity, problems and their roles in investment portfolios. Section 3 is about research methodology. Section 4 presents the data. Section 5 covers the results and the discussion. Section 6 concludes the paper.
2. Literature review
2.1 cryptocurrencies overview.
A cryptocurrency can be defined as “cash for the internet.” It is a piece of digital information that one can hold onto, and it has value as long as no one else has access to the information and keys that provide the value. The entity who holds the information at that point of time is also the holder of the value of that cryptocurrency.
The creation of cryptocurrencies is based on the need for internet cash combined with the desire for anonymity in internet transactions. As explained in Geiregat (2018) , scientists and activists concerned with privacy and personal liberty started the experimentation and eventually the invention of the cryptocurrency: an unregulated, decentralized, completely anonymous system of transactions directly from user to user (peer-to-peer) without a bank account or credit card.
Cryptocurrencies have three core characteristics: decentralized, unregulated and anonymous. In his manifesto, Nakamoto (2008) highlighted the problems of lack of privacy and safety in transactions, and proposed a well-thought out solution: the blockchain. Decentralization of cryptocurrencies originates from the nature of the blockchain technology. Being a distributed ledger, operated within peer-to-peer networks, the data and information contained on the blockchain platform are available to and reside in thousands of computers all over the world, so that any user, miner or bystander has access to the data. This makes the system highly unlikely to be hacked compared to a centralized organization or system, such as a bank, where there is one central repository of information susceptible to breaches in security. Biais et al. (2019) model the blockchain protocol as a stochastic game and analyze the equilibrium strategies of rational, strategic miners by mining the longest chain as a perfect Markov equilibrium in line with Nakamoto (2008) . The blockchain protocol, however, is also a coordination game, with multiple equilibria, hence, the decentralization characteristic.
Blockchain technology and its solicitation through cryptocurrencies provide decentralized consensus and potentially enlarge the contracting space through smart contracts. At the same time, generating decentralized consensus entails distributing information that necessarily alters the informational environment. Cong and He (2019) analyze how decentralization relates to consensus quality and how the quintessential features of blockchain remold the landscape of competition. Smart contracts can mitigate informational asymmetry and improve welfare and consumer surplus through enhanced entry and competition. In general, blockchains sustain market equilibria with a wider range of economic outcomes.
The second key characteristic of the cryptocurrency is the lack of regulation. No government or organization has any control or say over any cryptocurrency, which makes them attractive for many reasons. Because there is no government monitoring, transactions are not subject to sales tax. Moreover, since no one is regulating transactions on a federal or other level, no intermediary such as lawyers, banks or payment providers is needed in the user-to-user system. These aspects of cryptocurrencies open them to potential issues with fraud and to interference by governments in the future.
The third key characteristic of cryptocurrencies is that they are anonymous and untraceable. When a transaction occurs on the blockchain network, each user involved in that transaction has a specific personal key, similar to a user name. Once that transaction is completed and verified, those personal keys are also completed and can never be used again. Every time a user engages in a transaction, a unique and untraceable personal key is generated. Moreover, there is no need for the user to engage a bank account or a credit card for the transaction, keeping the anonymity. This key anonymity characteristic also opens the door to more potential problems for cryptocurrencies.
2.2 Issues and problems of cryptocurrencies
Cryptocurrencies have been among the largest unregulated markets in the world. Foley et al. (2019) document that roughly one fourth of Bitcoin users may have been involved in illegal activity. Their study estimates that about $76bn of illegal activity each year may involve Bitcoin. They also document that the illegal share of Bitcoin activity has declined with mainstream interest in Bitcoin and with the emergence of more opaque cryptocurrencies. Practical problems related to investing in cryptocurrencies include illiquidity, theft, fraud, ransom attacks/hacking and potential constrictive government regulation. Since cryptocurrencies are unregulated, decentralized, untraceable and anonymous, there are no protections, liability clauses or insurers.
Although the lack of regulation is an important problem for the lack of protection from theft and ransom attacks, increased levels of regulation could pose an even bigger problem for these digital coins. Government regulation could disrupt the true nature of cryptocurrencies that makes them attractive to users, could lead to drastic declines in their value, and could cause significant illiquidity, making them unattractive to investors. Overall, the potential for regulation is a major threat to cryptocurrencies for the near future.
2.3 Popular cryptocurrencies
The top four cryptocurrencies in terms of market capitalization are Bitcoin, Ethereum, Ripple and Litecoin. Bitcoin holds the largest market capitalization allocating half of the cryptocurrency market. Ethereum and Ripple also hold significant spots covering 10 percent of the market capitalization each. Litecoin is on the rise with a smaller holding of the market capitalization of roughly 3 percent. These four cryptocurrencies constitute three fourths of the entire market. As reported in Radovanov et al. (2018) , although correlations vary between these cryptocurrencies, they are fundamentally different from each other because of their unit values, market capitalizations and user applications.
Bitcoin has been the most talked about, popular and sophisticated cryptocurrency ( Velde, 2013 ). Launched in 2009, the value has grown tenfold from 2013 to 2018. Figure 1 depicts the Bitcoin price vs the Dow Jones Industrial Average in 2010s. We can see the exponential increase in the Bitcoin value and the dramatic decline during the cryptocurrency crash of January 2018. In the Nakamoto (2008) manifesto outlining the idea of Bitcoin and blockchain, a low-cost secure payment system has been proposed that does not involve a central authority or trusted third party. As explained further in Velde (2013) , Bitcoin is not a claim to a physical object or to a currency; rather, it aims to be a currency itself to replace the usual physical object of a currency with a computer file. Bitcoin and other cryptocurrencies use the blockchain network but they differ in from each other terms in terms of the difficulty in mining the specific currency. Bitcoin has a specific hash rate of verifying transactions, a certain number of miners and a goal of six blocks to be created per hour (for an average transaction speed of ten minutes), making it organically fit the blockchain network. Overall, Bitcoin has been primarily utilized as a means to transfer funds within the blockchain environment, but also as a speculative investment opportunity given that the cryptocurrency derives its value from exchange.
Second in the market capitalization in the cryptocurrency market is Ethereum, created in 2014. Ether, the digital coin of Ethereum, is rival to Bitcoin due to the promise of the technology it is built in. Instead of being used as a digital currency, or an alternative to fiat money, Ethereum has been built for smart contracts and for decentralized applications. Smart contracts are agreements in the blockchain network that function like software programs that can bind obligations based on predetermined conditions. Ethereum’s built-in programming language allows anyone to build augmented applications. The Ethereum network has the potential to host numerous functions such as social networks, public utility applications, crowd-sourced prediction markets and investment companies. Ethereum’s ability to create new social structures within a completely virtual network sets it apart from Bitcoin and other cryptocurrencies.
The third cryptocurrency in market capitalization is Ripple, created in 2013 with the primary purpose of helping banks transfer cash faster and cheaper, especially internationally. Traditional methods of cross-border payments are slow, of low value, of high volume and have high transaction costs. Ripple is primarily meant to be used as a currency translation tool, and to be a catalyst, setting it apart from other cryptocurrencies.
The fourth popular cryptocurrency is Litecoin, created in 2011, mainly as an alternative and as an improvement to Bitcoin with faster settlement times for transactions and with lower fees. Litecoin is used for the same purpose like Bitcoin as a method of payment and as an exchange of funds but with better performance for micropayments.
2.4 Cryptocurrencies in investment portfolios
There are studies that look into the effects of using Bitcoin to diversify an investment portfolio, such as Wu and Pandey (2014) , Klabbers (2017) and Andrianto and Diputra (2017) . These studies primarily focus only on Bitcoin. Using dated and limited sample periods from 2010 to 2013 in Wu and Pandey (2014) , from 2013 to 2016 in Andrianto and Diputra (2017) and from 2010 to 2016 in Klabbers (2017) , the usefulness of Bitcoin as an investment asset in enhancing the efficiency of an investment portfolio were documented.
Lack of sufficient information and the novelty of cryptocurrencies lead to discomfort in the opinions of different investors and financial experts in using Bitcoin and other cryptocurrencies in an investment portfolio ( Burgess, 2018 ). Burgess (2018) documents one expert recommending allocations of no more than 2 percent of a portfolio to Bitcoin due to high-volatility, and not recommending for anyone nearing retirement. Investors generally consider cryptocurrencies as high risk investments and tend to keep some in the portfolio only as an insurance policy: in order to settle ransomware attacks with these cryptocurrencies.
The academic studies mentioned above demonstrate the usefulness of Bitcoin in portfolio efficiency; however, the attitude of investors in general has been lukewarm. To reconcile these contrasting views, we use a more recent and longer sample period ranging from August 2013 through January 2019. Furthermore, we use multiple cryptocurrencies and broad traditional asset classes. We document the dynamic nature of the contribution of cryptocurrencies in a portfolio. We demonstrate that all cryptocurrencies contribute to an efficient portfolio, regardless of the type of cryptocurrency. Our results emphasize the evolving dynamics of return-risk characteristics, while Bitcoin and Ripple held larger positions in optimal portfolios, Litecoin has taken over in recent years, especially after the cryptocurrency crash of January 2018.
Briere et al. (2015) analyze investments in Bitcoin by US investors within a diversified portfolio of both traditional and alternative assets. Over the period of the analysis, Bitcoin had an exceptionally high average return and volatility, and correlation with other assets was low, proving that it had high diversification benefits. However, the conclusion made clear that these results should be looked at cautiously, as the data in this study reflected the early-stage behavior of Bitcoin and might not appropriately represent the performance of the portfolio in the long or even medium run. Overall, there have been some studies conducted on the effect of Bitcoin in enhancing portfolio diversification. But these studies focus only on Bitcoin and not on a combination of other cryptocurrencies; are mostly outdated; and use short sample periods with a short-term outlook, not taking into account the long-term volatility effects nor the possibility of increased regulation.
Along the lines of portfolio diversification with more than one cryptocurrency, Brauneis and Mestel (2019) focus on a portfolio made up of only cryptocurrencies and they present evidence of substantial risk reduction. In an attempt to address and quantify the portfolio effects in the cryptocurrency investment universe, they rely on the traditional mean-variance framework as proposed by Markowitz (1952) . There is a considerable body of literature that proposes alternatives to the mean-variance optimization when returns are not normal. However, seminal papers such as Levy and Markowitz (1979) and Kroll et al. (1984) demonstrate the equivalence of the mean-variance approach to expected utility maximization under non-normality. In this study, we follow the same principles laid out in Brauneis and Mestel (2019) and provide preliminary evidence on the portfolio effects of multiple cryptocurrency investments. However, our portfolio structure, unlike theirs, is more flexible and general: we examine the most important cryptocurrencies, but we include them in a portfolio of traditional asset classes: equity, bond, real estate and volatility, mainly considering a US based investor (equity is represented by the US stock market), who is somewhat sophisticated (with a derivatives proxy based on volatility index). We examine this overall portfolio through the mean-variance framework.
Anyfantaki et al. (2018) examine the role of cryptocurrencies in a traditional portfolio mainly from a theoretical perspective. Their empirical analysis covers a two-year period from mid-2015 to mid-2017, which is significantly shorter than ours, but provides similar evidence of diversification benefits of cryptocurrencies both in- and out-of-sample. Klein et al. (2018) compare Bitcoin to gold and examine the role of each financial security in a traditional portfolio. They find that Bitcoin exhibits very different properties with respect to gold in that, while gold is useful during down times, Bitcoin is hardly helpful during those same periods.
Limited number of previous studies finds that in the short-term investment horizon, cryptocurrencies may offer diversification benefits. For example, Corbet et al. (2018) look into investing in Bitcoin, Ripple and Litecoin, along with other traditional and alternative financial assets, and document Bitcoin’s impact on the other cryptocurrencies. Smaller currencies like Ripple and Litecoin, although have no effect on the value of Bitcoin, are highly interconnected amongst themselves and influence one another’s values. Results from the Corbet et al. (2018) study show that cryptocurrencies are “isolated from other markets,” and general market conditions are not highly influential on cryptocurrencies. Therefore, cryptocurrencies do present diversification benefits as they are disconnected from the general financial markets. However, the cryptocurrency market contains its own idiosyncratic risks that are not easy to hedge against. The Corbet et al. (2018) study mainly explores the time series relationships and correlations amongst cryptocurrencies and between cryptocurrency – regular asset pairs. In our study, we focus on the optimal portfolio characteristics with cryptocurrencies as part of portfolios.
Elendner et al. (2018) provide a qualitative description of cryptocurrency portfolios. They examine numerous portfolios of cryptocurrencies, as well as the cryptocurrency index, CRIX and evaluate the performance of these portfolios. They also consider equally weighted and value-weighted broad portfolios made up of cryptocurrencies and traditional assets. They provide evidence of diversification benefits of cryptocurrencies because of their limited co-movements with traditional financial securities. However, the optimal portfolio construction is not part of the Elendner et al. (2018) study. Focusing on ten major cryptocurrencies, Liu (2019) examines the role of diversification amongst cryptocurrencies. Evaluation of the out-of-sample performance of this cryptocurrency-only investment spectrum shows that portfolio diversification across different cryptocurrencies is beneficial, implying that each cryptocurrency has unique characteristics. Borri (2019) examines contagion and flight-to-quality characteristics of cryptocurrencies amongst themselves focusing on the tail-risk events and provides evidence that cryptocurrency portfolios offer better risk-adjusted and conditional returns than individual cryptocurrencies. On the other hand, Bouri et al. (2019) find that common characteristics between cryptocurrencies increase during uncertain periods. In a more rigorous approach by Härdle and Trimborn (2015) , the relationship between cryptocurrencies and the cryptocurrency index – CRIX is examined at a theoretical level.
3. Research methodology
This paper aims to answer should, and if yes how, cryptocurrencies can be used to diversify an investment portfolio. Additionally, we ask how cryptocurrencies correlate with each other and with other asset classes, and how cryptocurrencies rank as individual investments, taking both risk and return properties into account.
In the portfolio optimization framework, we follow the mean-variance optimization technique outlined in Kroll et al. (1984) and Merton (1990) . The Kroll et al. (1984) study compares the mean-variance optimization technique with direct utility maximization with infinite number of alternate probability distributions for the expected utility maximizer. They conclude under standard portfolio constraint sets faced by a wide variety of professional and individual investors that the mean-variance portfolio estimation is a good procedure to determine the optimal portfolio for investment purposes.
We follow the portfolio optimization methods based on the Merton proposition involving envelope portfolios ( Merton, 1990 ). This method finds the optimal weights of each asset class on the tangent portfolio of the efficient frontier. Using the average daily returns and the variance-covariance characteristics of the financial assets through linear algebra and matrix operations, the optimal weights are assigned to each asset in the portfolio to establish the most efficient portfolio possible.
The main conjecture of this study is that cryptocurrencies, when considered as financial securities, would provide enhancements to portfolio with a higher return and/or lower risk because of their unique and different characteristics compared to traditional financial assets. We also conjecture that amongst themselves, cryptocurrencies have been quite different; therefore, all three that we examine in this paper would have contributions to the optimal portfolio.
Daily price data for seven different asset classes are used in the analyses. We consider cryptocurrencies as financial securities for investment portfolios. For the three cryptocurrencies, the data are obtained from Coin Metrics. The price data for the other four asset classes are collected from FactSet. We focus on Bitcoin, Ripple and Litecoin because they have been in existence the longest. Our sample period with daily frequency is from August 5, 2013 through January 17, 2019, covering the majority of 2010s.
The seven asset classes chosen for the optimization are three cryptocurrencies: Bitcoin (BTC), Ripple (XRP), Litecoin (LTC); equity represented by the Dow Jones Industrial Average; real estate represented by the Vanguard Real Estate Index; fixed income securities represented by the Vanguard Total Bond Market Index; and a derivatives proxy utilized by sophisticated investors represented by the CBOE Volatility Index. It should be noted that Ethereum was not used in the study since, although it is a popular cryptocurrency, it came into existence quite recently in 2014.
Once the price data on all the seven asset classes were collected from the two different sources, they were compiled into one cohesive data frame and incomplete information due to differences in the dates of the data for the cryptocurrencies was eliminated. Daily returns are calculated using: (1) r ( t ) = ln ( P ( t ) ) − ln ( P ( t − 1 ) ) , where ln(P(t) and ln(P(t−1) are the natural logarithms of the closing prices on day t and day t− 1.
4.1 The portfolio optimization technique
The particular portfolio optimization analysis involves linear algebra and matrix operations. The weights of the optimal portfolio are obtained in accordance with the Merton (1990) proposition, which states that all envelope portfolios in the financial securities universe solve the following equation: (2) x = S − 1 { E ( R ) − c } Sum ( S − 1 { E ( R ) − c } ) , where x is the vector of optimal weights of the components of the portfolio (with these weights adding up to 1), S is variance-covariance matrix, E(R) is the expected return vector composed of anticipated daily returns of the components of the portfolio, and c is an arbitrary constant. Any constant c leads to an envelope frontier portfolio. The denominator of the right hand side of Equation (2) normalizes the numerator and makes sure that the optimal weights add up to 1.
The Merton (1990) proposition gives a specific method of finding the tangency portfolio, given c . In that specific optimization, the constant c is economically interpreted as the risk-free rate of return, r f . The variance-covariance matrix, S , represents the risk characteristics of the investment portfolio. It is defined as a diagonal and symmetric matrix, where the diagonal elements are variances of the individual financial securities, and the off-diagonal elements are the covariances between financial security pairs. Hence, the formal name of the S is the variance-covariance matrix.
creation of the return vector where each element is the average of the daily returns of the financial securities in the portfolio;
creation of the variance-covariance matrix, S , using the entire data set of the returns of all the investments in the portfolio; and
determination of the optimal weights vector, x , following the Merton (1990) proposition summarized in Equation (2) .
Once the initial optimization was completed using all of the data collected, multiple other robustness analyses were conducted with different portfolios through the inclusion and exclusion of asset classes. Further tests were also conducted by covering different sample periods. These tests and their results are discussed in the following section.
5. Results and discussion
The portfolio with the traditional asset classes in equity, fixed income, real estate, along with the volatility index representing derivatives market for sophisticated investors is further enhanced with the cryptocurrencies, Bitcoin, Ripple and Litecoin. Table I presents the summary statistics of the return characteristics of these financial securities for the sample period covering August 2013–January 2019.
We see that the cryptocurrencies generate the highest daily average raw returns during the period of investigation. Ripple has the highest return, followed by Litecoin and Bitcoin. Volatility index average return is also high (in fact, higher than the Bitcoin return). Thus, we can say that during most of the 2010s, traditional asset classes have generated lower returns than the popular cryptocurrencies. Examination of the volatilities of these investments also shows, however, that the cryptocurrencies have higher volatilities compared to those of other investment alternatives.
In order to take into account the volatility of the assets as well as the returns, we focus on CV – the coefficient of variation, which is defined as the ratio of standard deviation of daily returns to the average of the returns. The lower the CV, the better the investment, taking both risk and return into account. As we can see from Table I , the popular cryptocurrencies have the lowest CVs compared to the other asset classes during the 2010s, indicating their superiority as investment alternatives. The best cryptocurrency in this regards is Ripple, followed by Bitcoin and then by Litecoin. The remaining asset classes in terms of attractiveness are US equities, volatility index, bonds and real estate.
Before moving on with the construction of the optimal portfolio, it is useful to get an overall view of the correlations between the financial securities during the 2010s. Table II presents the correlation matrix, where the Pearson correlation coefficient for every asset pair is listed. As one can observe from the correlation matrix, there are indeed benefits to forming portfolios during the investigation period. The low correlations within the asset pairs, the negative correlation values and the evidence of no relationship between equity returns and cryptocurrency returns are all indications of the advantages and the rational necessity of forming portfolios of these securities during the majority of the 2010s period. Volatility-based investment opportunities such as VIX based financial products naturally have high negative correlations with equity markets. As for cryptocurrency correlations between themselves, the highest positive correlations have been between Bitcoin and Litecoin.
The results from the correlation table enable the construction of portfolios enhancing return and reducing risk, potentially at the same time. According to this conjecture, the CV of such portfolios should be lower than any of the individual asset classes examined in this paper during the same sample period. To explore this conjecture, we follow the Merton (1990) approach and construct the optimal portfolio using these seven (three popular cryptocurrencies, three traditional investments and one derivatives proxy) investment alternatives. The optimal portfolio would be an efficient portfolio with as much profit and as little risk as possible. By taking into account the risk free rate during the sample period of the portfolio creation, the optimal portfolio would also be the tangent portfolio residing on a straight line starting from the risk free rate and is tangent to the efficient frontier according to portfolio theory. Following the steps of the Merton’s proposition for the mean-variance optimization, the results are provided in Table III .
In Panel A of Table III , we see the critical pieces of information for the optimal portfolio. The daily return of the optimal portfolio is 5.1 basis points. The volatility is 32.37 basis points, which is lower than all the individual investment alternatives except for fixed income. Most importantly, the coefficient of variation is 6.35, and this is much smaller and therefore superior to the individual assets (the lowest CV of the individual investments is 15.2 for Ripple). Panel B provides the optimal weights of the portfolio as percentages. The optimal portfolio for the 2013–2019 sample period of the study for the 2010s primarily includes stocks and bonds. Bitcoin and Ripple do have a part in the optimal portfolio with 2.6 and 1.1 percent weights, respectively. Litecoin has a short position with a small negative weight. Given that Bitcoin and Ripple enhance the optimal portfolio in the 2010s period with their positive weights, and Litecoin with a nonzero negative weight, we can conclude that the popular cryptocurrencies have had a role in portfolio construction and in investments, in addition to their original purposes for which they were created.
Finally, Panel C of Table III provides the variance-covariance matrix, which summarizes the risk features of the components of the optimal portfolio. The diagonal elements of the matrix are the variances of the individual components, while the off-diagonal elements the covariances between investment pairs.
We also conducted some bootstrap analysis following the recommendation of an anonymous referee to determine the weights in an optimal portfolio for a fixed time horizon of 500 business days. We selected individual days’ returns randomly with replacement for the original sample for each portfolio component. By selecting the daily returns randomly, the cross-sectional covariance structure would be preserved, but we get a better picture of a possible return distribution. Our bootstrapping process has indeed emphasized the importance of the cryptocurrencies: the optimal weights are 5.5 percent for Bitcoin, 3.3 percent for Ripple and 3.1 percent for Litecoin, making up about 12 percent of the portfolio during our sample period according to the bootstrapping procedure.
The optimal portfolio in Table III contained all three popular cryptocurrencies investigated in this study. While in the Table I discussion we have concluded that as a single investment, Ripple is the best cryptocurrency with its smallest CV, followed by Bitcoin and Litecoin, we now investigate how each cryptocurrency individually and separately contributes to the optimal portfolio. In Table IV , we create optimal portfolios with a single cryptocurrency as the fifth portfolio component in addition to the equity, fixed income, real estate and volatility index components. First, the optimal portfolio without any cryptocurrency is presented in Panel A. In addition to the weights of the portfolio components, the return and the standard deviation are provided. Summarizing all, the CV of this portfolio is 7.34.
Panel B depicts the results of the portfolio augmented with Bitcoin. The weight of Bitcoin in this optimal portfolio is 3.02 percent and this positive weight helps the optimal portfolio achieve a lower CV of 6.60. Panels C and D are for portfolios augmented with Ripple (1.5 percent weight) and Litecoin (1.3 percent weight). These portfolios have CVs of 6.68 and 6.93, respectively. Overall, we can conclude that adding a cryptocurrency has consistently helped the optimal portfolio to achieve a better return and risk combination summarized with a lower CV; Bitcoin has been the best cryptocurrency enhancing the characteristics of the portfolio because the optimal portfolio with Bitcoin has the lowest CV during the sample period; Litecoin has been the least useful cryptocurrency: the CV of the optimal portfolio is lower than the CV of the original no cryptocurrency portfolio, but higher than the CVs of the portfolios with the other cryptocurrencies.
Are the portfolio contributions of cryptocurrencies static or dynamic over time? To investigate this issue, we split the entire sample into two subsamples: the early subsample from August 4, 2013 through April 26, 2016 and the recent subsample from April 27, 2016 through January 17, 2019. We construct the optimal portfolio using the cryptocurrencies and the traditional assets for each subsample separately. The results reported in Table V provide some interesting observations. The early subsample results in Panel A depict the attractiveness of all the cryptocurrencies. All three cryptocurrencies have positive weights in the optimal portfolio. Bitcoin has the largest weight, followed by Ripple and Litecoin. In the recent subsample, the contributions of the cryptocurrencies become more selective. Bitcoin weight increases, Ripple weight stays positive, while Litecoin weight is negative in the optimal portfolio in Panel B. We see that the contributions of the cryptocurrencies to an optimal portfolio evolve over time, indicating the highly dynamic nature of these investments.
For further verification of the dynamic evolution of optimal weights and cryptocurrency, we split our sample period into three sub-samples (early: August 2013–May 2015, middle: June 2015–March 2017, recent: April 2017–January 2019) and executed the optimization process to get the optimal weights, return, risk and CV. The Bitcoin weights change as 0.9, 5.9 and 6.5 percent for early, middle and recent subsamples (for Ripple the portfolio weight evolves as 0.6, −1.2 and 1.2 percent; and for Litecoin as 0.5, 0.3 and 0.4 percent). Moreover, using the optimal portfolio weights of the previous sub-sample for the next sub-sample generates a portfolio return of 3.3 bp for the middle sub-sample (compared to the optimal 5.2 bp) and 5.2 bp for the recent sub-sample (compared to the optimal 15.6 bp). All these out-of-sample analyses indicate the evolving and dynamic nature of the markets where the conclusions of this study provide a guideline into the future, but no guarantees.
For robustness, we explore two different avenues. In the optimal portfolio construction, it is natural for some securities to have negative weights, i.e., they would be short-sold. The short positions for these inferior securities would enable the portfolio to place heavier weight on more attractive securities. While short selling is an organic part of free and efficient markets, it is prohibited by regulators in some markets due to ethical (benefiting from the decline of security prices may be frowned upon), psychological (leading to herding behaviors of excessive selling), or other reasons. In markets where short selling is not allowed, securities cannot have negative weights in portfolios. As the first robustness investigation, we explore optimal portfolios with this extra constraint of no short selling. The results are presented in Table VI , Panel A. Litecoin and Real Estate do not have any contribution to the portfolio and have zero weights, but Bitcoin and Ripple do have positive contributions to the optimal portfolio during the sample period. As expected, with the extra no-short restriction, the CV of the portfolio is worse than that of the portfolio reported in Table III .
Second, we examine the dynamic behavior of the optimal portfolio before and after the cryptocurrency crash. Throughout the beginning of 2018, from January to the mid of February, all cryptocurrencies lost significant value. This 2018 cryptocurrency crash, also known as the Bitcoin crash or the great crypto crash, was the sell-off of many cryptocurrencies during the month from January 6, 2018 to February 6, 2018, during when the Bitcoin value fell by about 65 percent. The capitalization of the cryptocurrency market lost more than $300bn in the first quarter of 2018.
Panel B of Table VI first reports the features of the optimal portfolio constructed during pre-crash period from August 2013 through December 2017. Consistent with Panel A of Table V , all the cryptocurrencies with their positive weights contribute to the formation of the optimal portfolio. The period after the Bitcoin crash period of 2018, March 2018 through January 2019, has been of poor performance for equity markets and real estate markets. This is reflected to the negative weights of equity and real estate in the post-crisis portfolio. The largest weight goes to bonds. As for cryptocurrencies, both Bitcoin and Ripple have large negative weights, while Litecoin has a positive weight in the portfolio – while the then popular Bitcoin and Ripple lose confidence, the demand for the upcoming Litecoin increases. The results in Panel B again indicate the dynamic and evolving characteristics of the cryptocurrency market. The crash has clearly led to reduction in the attractiveness of cryptocurrencies as investment alternatives; however, the dynamic characteristics of cryptocurrencies warrant their future demand in investment portfolios.
6. Conclusion
In this study, we investigate popular cryptocurrencies as individual investment opportunities and as components of the construction of an optimal portfolio. Our sample period covers most of the 2010s. We examine three popular cryptocurrencies: Bitcoin, Ripple and Litecoin. As an independent single investment, Ripple has had the highest return, followed by Litecoin and Bitcoin during the sample period. However, volatility of Ripple is also the highest, followed by those of Litecoin and Bitcoin. Combining risk and return together, coefficient of variations reveal that the best cryptocurrency has been Ripple, followed by Bitcoin and then by Litecoin. All these cryptocurrencies have been better than the traditional asset classes in the 2010s for investment purposes.
We examine the role of cryptocurrencies in an optimal portfolio. We document that adding a cryptocurrency has consistently helped the optimal portfolio achieve a better return-and-risk combination. Bitcoin has been the best cryptocurrency in this regard, followed by Ripple and Litecoin. We report that the contribution of the cryptocurrencies to an optimal portfolio is dynamic and, therefore, evolves over time: when we split our entire sample into two subsamples, we find that all three cryptocurrencies have a positive role in the optimal portfolio in the early subsample. In the recent subsample, the role of the cryptocurrencies is not unidirectional. The Bitcoin crash has led to reduction in the attractiveness of cryptocurrencies as investment alternatives but their dynamic characteristics warrant their future demand for investment portfolios. We conclude in our study that popular cryptocurrencies have had an important role in portfolio construction and in investments, in addition to their original purposes for which they have been created.
As for the potential issues in the data analysis and for future avenues for research, we note that cryptocurrencies are still relatively new. One of the most popular cryptocurrencies, Ethereum, could not be included in our investigation because of the lack of statistically sufficient data. Our sample period covered the great cryptocurrency crash, illustrating the high volatility of these assets. As more data become available, as cryptocurrencies become more stable, and as familiarity with cryptocurrencies increases, the long-term effects of these assets in the formation of the optimal portfolio will become more informative.
Dow Jones industrial average index and the Bitcoin price in US dollars
Summary statistics
Notes: Various robustness test results are reported. Panel A is about markets and time periods where short-selling may not be possible. The optimal portfolio characteristics with different cryptocurrencies are provided in this panel where short-selling is prohibited. Panel B is about the Bitcoin and cryptocurrency market crash in the beginning of 2018 from January to February. Before crash optimal portfolio weights for the subsample from August 2013 through December 2017 are followed by after crash optimal portfolio weights for the subsample from January 2018 through January 2019. Optimal portfolio weights are given as percentages. BTC is Bitcoin, XRP is Ripple, LTC is Litecoin, DJIA is Dow Jones Industrial Average Index, Real Estate is the Vanguard Real Estate Index and Bonds is the Vanguard Total Bond Market Index
Andrianto , Y. and Diputra , Y. ( 2017 ), “ The effect of cryptocurrency on investment portfolio effectiveness ”, Journal of Finance and Accounting , Vol. 5 No. 6 , pp. 229 - 238 .
Anyfantaki , S. , Arvanitis , S. and Topaloglou , N. ( 2018 ), “ Diversification, integration and cryptocurrency market ”, working paper, Bank of Greece, Athens, March 1 .
Biais , B. , Bisière , C. , Bouvard , M. and Casamatta , C. ( 2019 ), “ The blockchain folk theorem ”, Review of Financial Studies , Vol. 32 No. 5 , pp. 1662 - 1715 .
Borri , N. ( 2019 ), “ Conditional tail-risk in cryptocurrency markets ”, Journal of Empirical Finance , Vol. 50 No. C , pp. 1 - 19 .
Bouri , E. , Gupta , R. and Roubaud , D. ( 2019 ), “ Herding behaviour in cryptocurrencies ”, Finance Research Letters , Vol. 29 No. C , pp. 216 - 221 .
Brauneis , A. and Mestel , R. ( 2019 ), “ Cryptocurrency-portfolios in a mean-variance framework ”, Finance Research Letters , Vol. 28 No. C , pp. 259 - 265 .
Briere , M. , Oosterlinck , K. and Szafarz , A. ( 2015 ), “ Virtual currency, tangible return: portfolio diversification with Bitcoin ”, Journal of Asset Management , Vol. 16 No. 6 , pp. 365 - 373 .
Burgess , R. ( 2018 ), “ Ups, downs of investing in Bitcoin ”, available at: www.theindianalawyer.com/articles/45940-the-ups-and-downs-of-investing-in-bitcoin (accessed December 15, 2018 ).
Cong , L.W. and He , Z. ( 2019 ), “ Blockchain disruption and smart contracts ”, Review of Financial Studies , Vol. 32 No. 5 , pp. 1754 - 1797 .
Corbet , S. , Meegan , A. , Larkin , C. , Lucey , B. and Yarovaya , L. ( 2018 ), “ Exploring the dynamic relationships between cryptocurrencies and other financial assets ”, Economic Letters , Vol. 165 No. C , pp. 28 - 34 .
Elendner , H. , Trimborn , S. , Ong , B. and Lee , T.M. ( 2018 ), “ The cross-section of crypto-currencies as financial assets: investing in crypto-currencies beyond Bitcoin ”, in Chuen , D.L.K. and Deng , R. (Eds), Handbook of Blockchain, Digital Finance, and Inclusion , Academic Press , Cambridge, MA and Elsevier , Amsterdam , pp. 145 - 173 .
Foley , S. , Karlsen , J.R. and Putniņš , T.J. ( 2019 ), “ Sex, drugs, and Bitcoin: how much illegal activity is financed through cryptocurrencies? ”, Review of Financial Studies , Vol. 32 No. 5 , pp. 1798 - 1853 .
Geiregat , S. ( 2018 ), “ Cryptocurrencies are (smart) contracts ”, Computer Law & Security Review , Vol. 34 No. 5 , pp. 1144 - 1149 .
Härdle , W.K. and Trimborn , S. ( 2015 ), “ CRIX or evaluating blockchain based currencies ”, Working Paper No. 2015-048, SFB 649 Economic Risk, Berlin, October 15 .
Klabbers , S. ( 2017 ), “ Bitcoin as an investment asset ”, working paper, Radboud Universiteit Nijmegen, Nijmegen, June 2 .
Klein , T. , Thu , H.P. and Walther , T. ( 2018 ), “ Bitcoin is not the new gold – a comparison of volatility, correlation, and portfolio performance ”, International Review of Financial Analysis , Vol. 59 No. C , pp. 105 - 116 .
Kroll , Y. , Levy , H. and Markowitz , H.M. ( 1984 ), “ Mean-variance versus direct utility maximization ”, Journal of Finance , Vol. 39 No. 1 , pp. 47 - 61 .
Levy , H. and Markowitz , H.M. ( 1979 ), “ Approximating expected utility by a function of mean and variance ”, American Economic Review , Vol. 69 No. 3 , pp. 308 - 317 .
Liu , W. ( 2019 ), “ Portfolio diversification across cryptocurrencies ”, Finance Research Letters , Vol. 29 No. C , pp. 200 - 205 .
Markowitz , H. ( 1952 ), “ Portfolio selection ”, Journal of Finance , Vol. 7 No. 1 , pp. 77 - 91 .
Merton , R.C. ( 1990 ), Continuous-Time Finance , Blackwell , Oxford .
Nakamoto , S. ( 2008 ), “ Bitcoin – a peer-to-peer electronic cash system ”, available at https://bitcoin.org/bitcoin.pdf (accessed October 15, 2018 ).
Radovanov , B. , Marcikić , A. and Gvozdenović , N. ( 2018 ), “ A time series analysis of four major cryptocurrencies ”, Facta Universitatis – Economics and Organization , Vol. 15 No. 3 , pp. 271 - 278 .
Velde , F. ( 2013 ), “ Bitcoin: a primer ”, Chicago Fed Letter No. 317, Chicago .
Wu , C.Y. and Pandey , V.K. ( 2014 ), “ The value of Bitcoin in enhancing the efficiency of an investor’s portfolio ”, Journal of Financial Planning , Vol. 27 No. 9 , pp. 44 - 52 .
Corresponding author
Related articles, we’re listening — tell us what you think, something didn’t work….
Report bugs here
All feedback is valuable
Please share your general feedback
Join us on our journey
Platform update page.
Visit emeraldpublishing.com/platformupdate to discover the latest news and updates
Questions & More Information
Answers to the most commonly asked questions here
A place next to Satoshi: foundations of blockchain and cryptocurrency research in business and economics
- Open access
- Published: 15 May 2020
- Volume 124 , pages 1305–1333, ( 2020 )
Cite this article
You have full access to this open access article
- Lennart Ante ORCID: orcid.org/0000-0002-5088-7127 1 , 2
7750 Accesses
26 Citations
2 Altmetric
Explore all metrics
Blockchain technology has become an ubiquitous phenomenon. While the topic originated in computer science, the business and economics literature was comparatively slow to pick up on it. To better understand the academic basis, current developments and future research avenues of the discourse, 9672 cited references of 467 blockchain and cryptocurrency articles from the fields of business and economics are gathered from the Web of Science Core Collection and are analyzed. Five major strands of research are identified through factor analysis. They are reviewed and their interrelation is mapped using social network analysis. Research on (I) market efficiency and economics and (II) asset pricing and valuation is relatively mature and focuses on cryptocurrencies, while research on (III) the principles and applications of blockchain technology, (IV) transactions and anonymity and (V) monetary theory and policy lacks maturity. Potential paths for future research are pointed out and in conclusion, it is assessed that this young field of research still leaves plenty of room for manoeuvre. A scientific place next to Nakamoto (2008) is still available for existing, emerging and new research streams.
Similar content being viewed by others
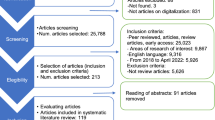
The role of digitalization in business and management: a systematic literature review
Esther Calderon-Monge & Domingo Ribeiro-Soriano
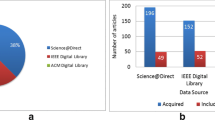
Blockchain smart contracts: Applications, challenges, and future trends
Shafaq Naheed Khan, Faiza Loukil, … Anoud Bani-Hani
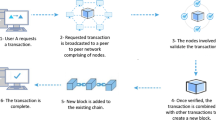
Blockchain Use Cases in the Sports Industry: A Systematic Review
Ahmed-Sami Berkani, Hamouma Moumen, … Ahcène Bounceur
Avoid common mistakes on your manuscript.
Introduction
Over the last few years, blockchain technology has shown the potential to affect nearly all industries and sectors. While Bitcoin or, more generally, cryptocurrency was only its first application, the underlying blockchain technology offers much wider scope. The special feature of blockchain technology is that it is not an actual invention but ‘merely’ an intelligent concatenation of existing mechanisms, which include technical mechanisms but also economic incentive models. A wide range of possible applications and their (potential) consequences remain to be researched. While such a technical topic was predictably first embraced by computer scientists and engineers, the business, economics and finance literature has since discovered blockchain for itself and has been producing a steady flow of both empirical and theoretical contributions for several years now.
The concept of blockchain and Bitcoin is attributed to Nakamoto ( 2008 ), who suggested the use of a currency or digital cash as the first application of the underlying technology to solve the double-spending problem. Since then, the number of technology applications has soared. The literature has expanded to cover the transformation of financial technologies (e.g. Ashta and Biot-Paquerot 2018 ; Chen et al. 2017 ; Gomber et al. 2018 ; Kim and Sarin 2019 ; Yang and Li 2018 ) and potential areas of application such as clearing and settlement (e.g. Caytas 2016 ; Chiu and Koeppl 2019 ; Fiedler et al. 2018 ; Tsai et al. 2018 ), banking and trade finance (e.g. Cocco et al. 2017 ; Guo and Liang 2016 ) or crowd-financing through initial coin offerings (ICOs) (e.g. Adhami et al. 2018 ; Ante et al. 2018 ; Fisch 2019 ). For the health care sector, blockchain is being analyzed as a mechanism to secure and to automate data flows (e.g. Esposito et al. 2018 ; Liang et al. 2018 ; Yue et al. 2016 ). Other applications include supply chain management (e.g. Apte and Petrovsky 2016 ; Francisco and Swanson 2018 ), digital identity and personal data (e.g. Dunphy and Petitcolas 2018 ; Zyskind et al. 2015 ), energy markets (e.g. Andoni et al. 2019 ; Oh et al. 2017 ), government and voting (e.g. Atzori 2017 ; Ølnes et al. 2017 ), law and contracts (e.g. Governatori et al. 2018 ; O’Shields 2017 ), or the sharing economy (e.g. Pazaitis et al. 2017 ; Sun et al. 2016 ). Research also deals with digital assets such as Bitcoin and their properties (e.g. anonymity and irreversibility) and markets (e.g. Chaum 2012 ; Urquhart 2016 ). The potential for additional research is as widely scattered as the emerging literature. It is therefore worthwhile to identify, summarize and review the literature, to identify potential avenues of research and to understand more precisely the theories and thoughts that underlie the current state of research. While the research on the solution of the double-spending problem and the secure digital transfer of value via the Internet has a longer tradition, academic research on Bitcoin or blockchain unequivocally begins in 2008. This also raises the question of which research streams formed during this period and which prior literature they are based on. Did the emerging streams of research rely on specific literature that already dealt with the blockchain phenomenon or rather on ‘established’ scientific literature and theories? What is the mixture of these two types of sources for each new stream of research? Knowing the relevant contributions, thoughts, theories, authors and journals will help researchers to optimally prepare, supplement or conceive projects and to identify promising new avenues of research in this comparatively new field, where scholars enjoy great liberty to pursue their personal interest when selecting a research topic.
A systematic analysis of the field of blockchain research is a necessary first step to obtain a fundamental overview and to identify lines of research that may have been neglected or that may hold specific potential. This is done by co-citation analysis in the form of exploratory factor analysis to prevent subjectivity. In addition, it is identified, using social network analysis, how research directions change over time and how they are related to each other. After considering the research streams and the actual articles, a number of gaps in the existing research and, by implication, promising avenues for future research are pointed out.
Note that the term cryptocurrency refers not only to currencies but also to so-called tokens, which can be used for financing purposes or supply chain transparency, for example. The analysis explicitly includes cryptocurrency, not least because the terms blockchain and Bitcoin were used somewhat interchangeably until recently, with early research in this area referring to Bitcoin throughout.
The paper proceeds as follows: Related work is described in the “ Related work ” Section. The “ Methodology ” Section presents the methodology in terms of data collection and analysis. The “ Findings on research streams ” Section contains the results, providing an initial overview and then going into detail regarding the development of the five identified research streams. “ Social network analysis ” Section specifically presents the results of the social network analysis. The “ Discussion ” Section discusses the results, including limitations and future research avenues. Finally, the “ Conclusion ” Section contains a brief conclusion.
Related work
The summary, processing and analysis of existing research represents an important task for the further development of scientific discourses. For this purpose, different methodologies are available, such as structured literature reviews or bibliometric studies. Literature reviews are often subject to author bias, while bibliometric studies use quantitative approaches to investigate relationships between relevant characteristics, such as authors, publications, citations or keywords (Tranfield et al. 2003 ; Zupic and Čater 2015 ).
A number of literature reviews of the blockchain and cryptocurrency literature focused, for example, on individual application areas of blockchains such as the Internet of Things (Conoscenti et al. 2017 ; Khan and Salah 2018 ), the sharing economy (Hawlitschek et al. 2018 ), service systems (Seebacher and Schüritz 2017 ), big data challenges (Karafiloski and Mishev 2017 ), cyber security (Taylor et al. 2019 ) or e-government (Batubara et al. 2018 ). Yli-Huumo et al. ( 2016 ) present a very helpful, though no longer quite up to date, systematic mapping analysis of the state of blockchain research and Corbet et al. ( 2019 ) systematically analyzed cryptocurrencies as financial assets based on 32 empirical publications on the topic. They identified the five thematic areas (1) bubble dynamics, (2) regulation, (3) cybercrime, (4) diversification and (5) efficiency and outlined ten different gaps in the literature (see Table 5 in their study for an overview).
Currently, there are various bibliometric studies on the topics blockchain, cryptocurrency, Bitcoin and/or smart contracts. Liu ( 2016 ) collected 253 Bitcoin-related publications from Scopus and applied co-word analysis, finding three groups based on similarities that relate to (1) technological, (2) financial, and (3) legal/regulatory questions. Holub and Johnson ( 2018 ) collected Bitcoin-related literature from 22 databases and, after refinement, mapped 1206 papers based on categories and disciplines. They showed that 29.9% of publications belonged to technology-related categories, while 24.9% were economics-related and only half of the papers in their sample have been published in journals. The study of Merediz-Solá and Bariviera ( 2019 ) used 1162 publications extracted from Web of Science to study research on Bitcoin (excluding blockchain applications). The authors provided an overview of the research environment, analyzed the degree of concentration of variables such as authorship and visualized connections of words in titles and abstracts or journals.
Zeng et al. ( 2018 ) based their analysis on 473 blockchain-related papers extracted from EI Compendex (EI) and 497 from the China National Knowledge Infrastructure (CNKI)—both engineering-focused databases. The authors analyzed the publications using social network analysis and textual analysis and identified that the topic blockchain has become more important than Bitcoin since 2017. This shift of relevance from Bitcoin to blockchain has also been found in another bibliometric study on the topic that is based on 995 papers from Web of Science (Dabbagh et al. 2019 ). Miau and Yang ( 2018 ) extracted 801 articles from Scopus that related to various blockchain and cryptocurrency search terms. They used Lotkas’s law and the K–S test to derive author productivity and identified distinct phases in the research development, beginning with Bitcoin and cryptocurrencies in 2008/09, followed by rapid literature growth in 2014/15 and, most recently, focusing on blockchain technology and smart contracts after 2016. Firdaus et al. ( 2019 ) analyzed the topic blockchain based on 1119 publications between 2013 and 2018 extracted from Scopus. Here, the analysis methods comprised descriptive evaluations, such as rankings and author dominance, and visualizations. The authors state to refer to literature from the year 2013 onwards, as the blockchain was introduced in that year. In fact, blockchain technology was introduced by Nakamoto ( 2008 ). Klarin ( 2020 ) based his analysis on 174 publications extracted from Scopus, identified the four thematic clusters (1) Bitcoin and cryptocurrencies, (2) blockchain adoption, (3) cryptocurrency and blockchain environment, and (4) business model innovations via co-citation analysis and outlined a detailed overview of future research directions for each cluster. One single bibliometric study focused explicitly on the concept of smart contracts, scripts that are anchored on blockchains. Over 1700 documents extracted from Scopus were analyzed descriptively and trends were identified via word cloud and clustering using the software VOSviewer. Six clusters were identified that deal with (1) smart cards, (2) contracts, (3) smart power grids, (4) blockchain, (5) economics and (6) energy. The authors conclude that there are two trends for research on smart contracts: e-commerce and smart power grids (Salmerón-Manzano and Manzano-Agugliaro 2019 ).
While research in the areas of cryptocurrency and blockchain has already been examined in the context of various bibliometric publications, the focus of the investigations has been, at least to a large extent, on performance analysis, i.e. the evaluation of scientific performance of authors or institutions (Zupic and Čater 2015 ). The approach of uncovering the intellectual structures and dynamics of research fields (science mapping) via co-citation analysis was only done by few studies using the VOSviewer software (e.g. Klarin 2020 ; Miau and Yang 2018 ). The aim of this work is to uncover the intellectual structure of blockchain and cryptocurrency literature in business and economics by empirically analyzing the research field via co-citation analysis using exploratory factor analysis, which has been widely used to identify research streams in bibliometric data (e.g. Kuntner and Teichert 2016 ; Nerur et al. 2008 ; Wörfel 2019 ; Zuschke 2019 )—but not yet for blockchain-related topics. This allows to reduce complexity for researchers by providing an objective overview and review of the most important research streams and their publications in the field of blockchain and cryptocurrency. Furthermore, this study differs from existing studies in that it explicitly focuses on the business and economics literature.
Methodology
The data were collected from the Web of Science Core Collection in July 2019. To capture all publications on blockchain and cryptocurrencies, the search term TS = (blockchain* OR distributed ledger* OR cryptocurrenc* OR bitcoin*) is used. Only peer-reviewed articles in the fields of business, finance and economics published after 2007 were considered. The extracted sample comprised 494 articles. (Without the discipline constraint, 2132 articles were obtained, with computer science and engineering accounting for most of the additional papers.) 27 articles proved to be unrelated to blockchain and cryptocurrency, so the final record contains 467 articles, with references to 9672 sources. These sources then naturally also refer to dates prior to 2008 and only a part of them are peer-reviewed publications. The final list of the 467 articles can be retrieved under https://doi.org/10.17632/x5cstsscmz.1 .
This paper relies on co-citation analysis. Co-citation occurs when two articles simultaneously feature in the bibliography of a third article, which means that the first two articles must precede the article that cites them. This temporal relationship can help to identify the academic basis of research results. A higher co-citation frequency is typically interpreted to indicate greater relevance of an article within a given research stream (Small 1973 , 1977 ). For the analysis, the extracted references were compiled in a square similarity matrix, whose diagonal values are replaced by the mean value of the respective column (McCain 1990 ; Small 1973 ). For this purpose, the data extracted from Web of Science is processed using the Bibexcel toolbox for bibliometricians (Persson et al. 2009 ), which allows easy subsequent use for factor analysis using SPSS and social network analysis using UCINET (Borgatti et al. 2002 ). A cutoff value of three co-citations was selected for the purpose of co-citation matrix creation, which resulted in a sample consisting of the 202 publications with three or more co-citations. Bibliometric data is usually highly skewed, which means that a comparatively small but highly important part of the literature contains most of the achievements in a particular field (Chen and Leimkuhler 1986 ). A bibliometric analysis therefore need not examine all articles cited in a discipline; it suffices to analyze the most widely-cited ones.
The analysis of the co-citation data relies on exploratory factor analysis. Factor analysis is a static method that allows to extract underlying structures from a data set by identifying relationships between variables. It provides a suitable method of analysis when there are no hypotheses regarding the structure of a data set prior to an investigation. Since a symmetrical co-citation matrix contains the number of co-citations for each article, the articles can be divided into contiguous groups or factors by means of factor analysis. If we assume that a multitude of similar sources for research work implies similar ideas and mindsets, these factors represent scientific discourses or streams of research. Within the framework of factor analysis, a correlation matrix is first generated from a data matrix, which then becomes a factor matrix. The research streams are distinguished from factors that are not considered further on the basis of a sharp decline in the eigenvalues associated with the factors, assessment of the generated scree plot and the respective percentage of variance explained. In addition, further factors in the analysis output include so few articles that they cannot be described as an individual strand of research. Factors are initially identified though principle component analysis, which extracts the highest variance of the data set and assigns the first factor to it. This process is then continued until a final factor with the proportionally smallest variance has been determined. Factors are then 'rotated' to distribute the extent of variability more uniformly between factors. Factors loadings are thus redefined so that they are relatively high (− 1 or 1) or low (0). This allows for a better differentiation between factors and the analysis derived from it. Factor loadings are generated via Varimax rotation with Kaiser normalization, as communalities between items are high (Kaiser 1959 ). They represent numerical values that indicate the strength and orientation of a factor to a measured variable—in this case a measure of the thematic fit of a particular publication to a research stream. A factor loading above 0.4 indicates that an article is part of a factor (i.e. scientific discourse), while a value above 0.7 indicates high relevance (McCain 1990 ). Besides factor loadings, factor scores are calculated using the regression method, in which the effects of each observation on factors are predicted. The identified values represent the relevance or spacing of an observation with respect to a factor (DiStefano et al. 2009 ; Gorsuch 1988 ). Transferred to bibliometric analysis factor scores indicate the contribution that an article makes to a research stream (Nerur et al. 2008 ). An article with a low factor loading and a high factor score does not fit well into a stream, but is nevertheless important to it.
Social network analysis is then used to uncover relationships and dependencies between publications and research streams. The relationships among individual articles are visualized in a two-dimensional plane using the UCINET NetDraw algorithm. This is done on the basis of intellectual proximity, which is determined by the number of co-citations (Biehl et al. 2006 ). A final set of coordinates is determined by arranging Euclidean distances in relation to the geodesic distance between nodes, in this case publications (Carrington et al. 2005 ). A map of the research clusters is thus obtained that reveals the relationships among them and indicates the relevance of individual articles by the size of the nodes.
Findings on research streams
Table 1 provides an overview of the identified streams of research and further statistics. It is shown how publications are distributed over the five factors determined through factor analysis. Only the highest factor loading is considered, i.e. if an observation has factor loadings above 0.4 for several factors, only the highest one is assigned. Through factor analysis, five different discourses are identified, which together account for 63.08% of the total variance in the sample. In this context, variance signals the relevance of a research stream for the entire research environment (Nerur et al. 2008 ). Specifically, principal component analysis and the varimax rotation method with Kaiser normalization are used. The rotation converged after 29 iterations. A Kaiser–Meyer–Olkin (KMO) measure of 0.851 and a highly significant Bartlett test statistic ( p < 0.001) are obtained. These test statistics apply to each of Tables 1 , 2 , 3 , 4 , 5 , 6 and 7 and are therefore not repeated. Factor analysis thus proves to be a suitable method of analysis.
The first and largest strand of research deals with the market economics and efficiency of cryptocurrencies. It accounts for 36.65% of the variance in the sample and a percentage of 35.6% of all examined publications can be assigned to the stream, of which more than half have factor loadings above 0.7 and thus represent publications of high thematic fit. The second stream (12.15% of the variance) concerns asset valuation and price formation and consists of 26.4% of all articles (9.2% with factor loadings above 0.7). The third stream accounts for 5.52% of the variance and deals with the principles and applications of blockchain. A share of 12.1% of papers can be assigned to the stream, while 5.7% of the sample represent important publications. The fourth stream (5.04%) covers special properties of cryptocurrency transactions, such as anonymity or irreversibility, and their regulatory implications. It covers 8.6% of all publications analyzed in the factor analysis and has the smallest share of high-fit publications (3.4%). Lastly, the fifth stream accounts for 3.73% of the variance and deals with monetary theory and policy, such as the suitability of Bitcoin as a currency. It represents a share of 7.5% of all papers examined, of which 75% are highly relevant publications for the stream.
The Bitcoin whitepaper (Nakamoto 2008 ) is the scientific basis of blockchain and cryptocurrency research. Its factor loading and factor score with respect to each of the five discourses are shown in Table 1 . Based on the factor loading, the whitepaper is most closely affiliated with stream II (0.487), with stream I in second place (0.456). Based on the factor score, the whitepaper is highly relevant to streams III (9.751) and IV (3.167). Its relevance is somewhat lower for research on asset valuation and price formation, and monetary theory and policy. The relevance of Nakamoto ( 2008 ) is lowest with respect to stream I.
Figure 1 shows histograms of the distribution of factor loadings and factor scores for all five identified research streams. It can be seen that the distribution of factor loadings in streams I and II varies comparatively strongly, while a trend can be observed in the three following streams. There, the largest part of all loadings is found between − 0.1 and 0.1, which shows that factors are composed of only a few specific publications and that the majority of the remaining ones are hardly thematically related. Stream IV represents a special feature, which is expressed by the fact that the highest identified factor loadings is 0.78. In terms of factor scores, stream III with has an extremely high factor score of 9.75.
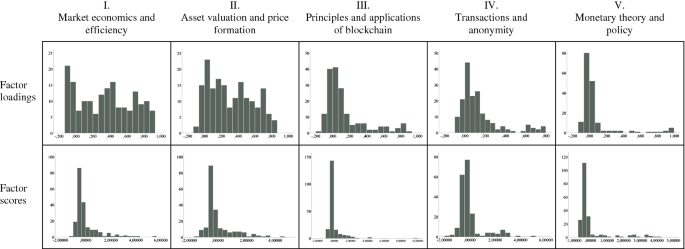
Distribution of factor loadings and factor scores across research streams. Frequencies are displayed on the y-axis, factor loadings and factor scores on the x-axis respectively
Table 2 shows the correlation coefficients among the five factors or research streams, as obtained via oblique rotation. Factors one and five exhibit negative coefficients throughout. The strongest positive correlation exists between streams II and IV, while the strongest negative correlation applies to the relationships between streams I and III, and II and V, respectively. This suggests that streams I and III as well as streams II and IV have little in common, while streams II and V have high similarity.
Figure 2 shows the relative development of the research streams over time based on the number of publications. For this purpose, every publication with more than three co-citations was assigned to one of the five streams or ‘other’, and the relative size of the six categories was graphed for each year or period.
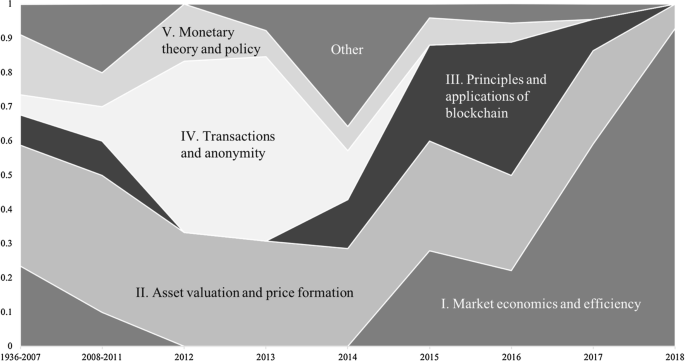
Relative development of research stream publications over time
Note that the years before the publication of the Bitcoin whitepaper were grouped into a single period, as were the years 2008 to 2011, because of the very low number of publications prior to 2012. Research stream I has been gaining ground steadily since 2014 and by 2018 accounted for 92.3% of the research on blockchain and cryptocurrencies examined here. The streams on ‘transactions and anonymity’ and ‘monetary theory and policy’ hit their peak importance in the period 2012 to 2014, while ‘blockchain basics’ peaked in 2015/16. Stream II accounted for a consistently large share.
The following subsections describe each of the research streams in detail before their interrelations are analyzed via social network analysis.
Market economics and efficiency
The first research stream comprises 33 articles, explains 36.65% of the variance and deals with the economics and efficiency of cryptocurrency markets, with most studies focusing on Bitcoin. The overall aim of the discourse is to promote the understanding of cryptocurrency markets. The ten most relevant publications within the stream and their factor loadings and scores are shown in Table 3 . The distribution of publications across journals is presented below the table. Economics Letters has the highest number of publications in this field, followed by Finance Research Letters. This stream of research is heavily based on theories of market efficiency, so it comes as no surprise that besides cryptocurrency-specific articles, basic literature on capital market efficiency (Fama 1970 ) or portfolio theory (Markowitz 1952 ) also shows up in this stream. Many of the publications in this stream are scientific letters and research notes, which build upon or reply to each other. The vast majority of the publications date from 2017/18.
The study by Donier and Bouchaud ( 2015 ) deals with the efficiency of the Bitcoin market and tests various liquidity measures as early warning signs of a bitcoin crash. Urquhart ( 2016 ) has the highest factor score (5.565), which suggests the greatest relevance for this research stream. The author uses a number of tests to identify autocorrelation for Bitcoin prices and finds that it persists over the entire observation period (Aug 2010 to Jul 2016). When the period is divided into two phases, however, it turns out that the first phase is responsible for the autocorrelation effect. Nadarajah and Chu ( 2017 ) examine the same data set again with the help of power transformations of daily returns and find no such effect. Fry and Cheah ( 2016 ) point out the speculative component of cryptocurrency markets and model financial bubbles and crashes. The authors analyze these markets by means of econophysics models and find evidence of spillover effects from Ripple to Bitcoin. Bouri et al. ( 2017 ) investigate the volatility of Bitcoin returns before and after the market crash in 2013 due to the collapse of the Mt.Gox exchange and find serial correlation. Corbet, Lucey, et al. ( 2018a , b ) analyze potential price bubbles in Bitcoin and Ethereum and conclude that there are periods with clear bubble behaviour—with Bitcoin being in a bubble phase when the paper was published. Kristoufek ( 2018 ) shows that in cool-down periods directly after bubbles or price surges, the Bitcoin market becomes efficient, while it remained inefficient for most of the period 2010 to 2017.
The Bitcoin market exhibits time frames that are efficient and ones that are driven by anti-persistence (Alvarez-Ramirez et al. 2018 ). The paper by Bariviera ( 2017 ) has the second highest factor loading (0.899) in the research stream and furthermore has a high factor score of 3.352. The study analyzes daily Bitcoin price data from 2011 to 2017 using two variations of the Hurst exponent, a metric that characterizes the scaling behavior of the cumulative deviations from the mean of a time series (Hurst 1951 ). The results show that daily returns behave consistently during the first part of the observation period, while the dimension of informativity increases from 2014. The volatility of the Bitcoin price exhibits long memory for the full observation period. Comparing the explanation of volatility by different GARCH models, Katsiampa ( 2017 ) shows that both short-run and long-run components of conditional variance are important for a suitable model. The investigation of nonlinear patterns of volatility in Bitcoin markets suggests long-term memory and provides strong evidence against the efficient market hypothesis (Lahmiri et al. 2018 ). Cheah et al. ( 2018 ) find long memory and cointegration in Bitcoin markets, with a negative correlation between cointegration and uncertainty.
The study with the highest factor loading, Tiwari et al. ( 2018 ), is an extension to Urquhart ( 2016 ), Nadarajah and Chu ( 2017 ) and Bariviera ( 2017 ), and confirms the earlier result that the Bitcoin market is informationally efficient. While most previous studies only considered Bitcoin, Brauneis and Mestel ( 2018 ) expand this focus by testing the efficiency of nine additional cryptocurrencies and conclude that higher liquidity leads to reduced efficiency. Similarly, Wei ( 2018a , b ) examines the liquidity of 456 cryptocurrencies and shows that greater market capitalization reduces the predictability of market behavior. The author concludes that liquidity is a significant factor for the market efficiency of cryptocurrencies. Corbet et al. ( 2018a , b ) find that across different cryptocurrencies, investors with a short investment horizon may gain diversification benefits. By some degree of contrast, empirical findings by Ciaian et al. ( 2018 ) suggest that Bitcoin and Altcoin (non-Bitcoin cryptocurrencies; in this case, 16 of them) markets are interdependent.
Phillip et al. ( 2018 ) analyze 224 different cryptocurrencies and identify unique properties, like stochastic volatility, Student-t error distributions and leverage effects. Studying the tail behavior of the five largest cryptocurrencies and using value-at-risk and expected shortfall as risk metrics, Gkillas and Katsiampa ( 2018 ) find that Bitcoin Cash is the riskiest asset, while Bitcoin and Litecoin have the lowest risk.
Urquhart ( 2017 ) studies price clustering of Bitcoin, finding that prices are clustered at round digits. In addition, price and trading volume have a significantly positive relationship with price clustering around round digits, which confirms Harris' ( 1991 ) negotiation hypothesis. Urquhart ( 2018 ) examines the relationship between Bitcoin fundamentals and the attention of investors. Realized volatility and trading volume are found to drive the next-day attention for bitcoin. The study has a relatively low factor score (1.137) compared to the other publications in the top ten, which suggests a lower overall relevance for the discourse.
Other studies in this research stream address the question whether Bitcoin is a medium of exchange or an asset. Dyhrberg ( 2016a ), Bariviera et al. ( 2017 ) and Baur et al. ( 2018a , b ) compare currency dynamics to Bitcoin, while Baur et al. ( 2018a , b ) find that Bitcoin is not correlated with traditional asset classes (stocks, bonds and commodities)—regardless of market timing. The authors analyze transaction data and suggest that Bitcoin is used as a speculative investment. In comparison to foreign exchange markets, Bitcoin has narrower bid-ask spreads, resulting in favorable exchange rates due to its simpler infrastructure (Kim 2017 ).
Asset valuation and pricing
The second research stream accounts for 12.15% of the variance. This stream is somewhat similar to the first one, not least with regard to its set of contributors. In comparison to stream I, however, the publications are older, and several articles do not explicitly refer to cryptocurrencies but merely provide a basis for such studies. The overall theme of the stream can be summarized as asset valuation and price formation. The discourse tackles the question of how cryptocurrency prices can be explained and determined. The ten most important publications of the stream based on factor loadings are presented in Table 4 . There is no trend regarding the outlets of publication, as all twelve papers were published in different journals.
The article with the highest factor loading, Engle ( 2002 ), is a methodology paper that introduced a new form of multivariate GARCH models – dynamic conditional correlation models—as an estimation technique. This illustrates the relevance of GARCH models in the analysis of crypto markets and the large number of formal and theoretical models in this research stream. Another major methodological paper, Glosten et al. ( 1993 ), ranks 9th in the stream with a factor loading of 0.735. Using a GARCH-M model, the study finds support for negative relationships between conditional expected monthly returns and the conditional variance of monthly stock returns: Positive expected returns are associated with a downward revision of conditional volatility, and vice versa. Baur and Lucey ( 2010 ) analyze the suitability of gold as a hedge against stocks and bonds. The study is an empirical and theoretical basis for similar research (e.g. Bouri et al. 2017 ; Dyhrberg 2016b ) that is later conducted on cryptocurrencies but which is assigned to research stream I.
Yermack ( 2015 ) investigates whether Bitcoin is a real currency. The author notes that currencies are a medium of exchange, a store of value and a unit of account, but Bitcoin did not fulfil these functions at the time (2013). Its daily trading statistics are uncorrelated with established currencies or gold. Against this background and Bitcoin’s various risks, such as hacks, theft or lack of access to the financial system, the author considers Bitcoin as a speculative investment rather than a currency. By contrast, Bouoiyour et al. ( 2016 ) classify Bitcoin as driven by long-term fundamentals (above one year). This is the result of a study using Empirical Mode Decomposition (EMD), where a data set is divided into several smaller independent data sets.
Using ARDL bounds testing, Bouoiyour and Selmi ( 2015 ) investigate the main determinants of the Bitcoin price. They find Bitcoin to be subject to extensive speculation and to be suitable to some extent as a basis for transactions of value on the Internet. The authors conclude that Bitcoin is a highly speculative asset, which prevents its wider use. Kristoufek ( 2015 ) analyses determinants of the Bitcoin price and effects of the Chinese market using a continuous wavelet framework and finds that fundamental values such as trading volume, ‘money’ supply and price level play a long-term role. A rising Bitcoin price attracts new miners. However, this effect declines over time as technology and mining processes become more professional. The price of Bitcoin depends on investor interest, which is particularly evident during price spikes. Another study of Bitcoin price formation finds that market forces and the attractiveness for investors have significant effects on Bitcoin prices, but the effects vary over time (Ciaian et al. 2016 ). Hayes ( 2017 ) investigates the explanatory factors for value formation in a cross-section of 66 cryptocurrencies, finding three primary factors: the degree of competition in mining, the technical complexity of the mining algorithm and the rate of inflation.
Principles and applications of blockchain
The third research stream comprises 10 articles, which together account for 5.52% of the variance. The factor loadings and factor scores of these articles are shown in Table 5 . The table additionally contains the Bitcoin whitepaper (Nakamoto 2008 ), which with a factor score of 9.751 is exceedingly relevant to the discourse. In principle, the articles deal with economic theory and economic and technical fundamentals of blockchain technology. Additionally, potential application areas or specific cryptocurrencies are introduced and discussed.
The third research stream consists of publications that explain blockchains by (1) providing general introductions and highlighting the potential of the technology (Iansiti and Lakhani 2017 ; Swan 2015 ; Tapscott and Tapscott 2018 ; The Economist 2015 ), (2) introducing specific cryptocurrencies or blockchains, like Bitcoin (Nakamoto 2008 ) or Ethereum (Wood 2014 ), and (3) describing specific applications (of blockchain) on a conceptual, technical or economic level—especially the concept of smart contracts, i.e. the decentralized execution of computer protocols (Szabo 1997 ; Wright and De Filippi 2015 ). Economic theories and mechanisms (Coase 1937 ; Jensen and Meckling 1976 ; Lamport et al. 1982 ) form an important theoretical basis for the discourse.
Coase ( 1937 ) explains why individuals seek to form partnerships and companies, rather than entering into bilateral contracts on the open market. Transaction costs—those associated with searching, information, negotiation or confidentiality—mean that some services cost more than they are worth. Coase’ theory of the firm states that enterprises are formed specifically to reduce such costs. Blockchain being a technology for peer-to-peer transactions of value, the relevance of the theory becomes immediately clear. Jensen and Meckling ( 1976 ) provide an extension with their theory of corporate ownership based on property rights theory, agency theory and finance theory. The authors show that while a 100% owner of a company makes decisions to maximize his own benefit, as soon as external capital flows into the company, the ensuing incentive conflict gives rise to agency costs. The owner no longer bears the full costs of the non-pecuniary benefits he grants himself. This results in agency costs, which materialize as monitoring, economic bonding or residual loss. The third major non-blockchain paper in this research stream is Lamport et al. ( 1982 ), who deal with the Byzantine Generals Problem, a theoretical situation where a group of generals can only communicate through messengers. However, some generals may be traitors who spread false information for confusion. The aim is to ascertain which information can be trusted. The solution requires a two-thirds majority of honest generals. The study provides a theoretical basis for consensus mechanisms and an alternative to the proof-of-work mechanism that Bitcoin uses. The extended mechanism of Practical Byzantine Fault Tolerance (PBFT) (Castro and Liskov 1999 ) is used by various blockchain infrastructures today.
Nakamoto ( 2008 ) provided the theory underlying blockchain technology and Bitcoin. The article is frequently cited and highly relevant in the field of blockchain-specific literature, as reflected by its extremely high factor score of 9.951. The author combined various existing technologies, such as time-stamping (Bayer et al. 1993 ; Haber and Stornetta 1990 , 1997 ; Massias et al. 1999 ) and announcements (Dai 1998 ) of transactions, proof-of-work (Back 2002 ), merkle trees (Merkle 1980 ) and economic incentive systems in a single mechanism to solve the double-spending problem and thus created the basis for today’s concept of blockchain technology.
Following Buterin ( 2013 ), Wood ( 2014 ) introduced Ethereum, to date the second largest public blockchain infrastructure. Ethereum’s main goal is to enable smart contracts, autonomous decentralized computer code that is executed on the blockchain. The blockchain uses proof-of-work, which was first introduced by Dwork and Naor ( 1992 ), before Back ( 2002 ) and Vishnumurthy et al. ( 2003 ) applied it to secure a currency. Besides Bitcoin, the Ethereum whitepaper mentions prior cryptocurrency implementations that were discussed in the academic literature, including Litecoin, Primecoin and Namecoin (Aron 2012 ; Sprankel 2013 ). Szabo ( 1997 ) provides a formal introduction to smart contracts; Miller ( 1997 ) made additional early contributions to the field. Wright and De Filippi ( 2015 ) take up the concept of smart contracts and show how self-executing digital contracts or smart property can be implemented with the help of blockchain. The authors also describe how this can in theory lead to decentralized autonomous organizations (DAOs), which could fundamentally change the nature of today’s central authorities and entities.
Transactions and anonymity
The fourth research stream consists of 10 articles and explains 5.04% of the variance. These ten publications are listed in Table 6 along with 3 additional papers with high factor scores. This research stream primarily deals with the fundamental properties of cryptocurrency, in particular transactions, irreversibility and (pseudo-)anonymity, and their consequences. This includes for example the possibility of secure online peer-to-peer payment or the risks associated with digital currency, such as money laundering. Potential solutions and regulatory approaches are discussed. Most of the publications are from the period 2012 to 2014 and cover either legal or technical aspects. No two papers were published in the same outlet, as shown below the table.
One article in the research stream is from pre-Bitcoin times: Dai ( 1998 ), referenced in Nakamoto ( 2008 ), which in turn has a high factor score of 3.167 and is therefore a formative source for this research stream, to which however it cannot be assigned due to the comparatively low factor loading of 0.238. Dai ( 1998 ) describes the design of a potential digital currency called b-money, which already exhibits many similarities to Bitcoin. Like the Bitcoin whitepaper, the paper by Eyal and Sirer ( 2014 ), which analyzes mining and potential vulnerabilities, also has a high factor score (3.138) but does not quite fit into the stream based on its factor loading. Böhme et al. ( 2015 ) provide an overview of Bitcoin’s economic, technological and governance aspects. Both studies thus provide basic background information on the Bitcoin network.
Bryans ( 2014 ) approaches money laundering and Bitcoin from a legal perspective, concluding that Bitcoin is a disruptive financial technology which poses major difficulties for established entities in the area of payment processing. New legislation will have difficulties covering digital currency, as its technological development is too far-reaching. The author points out in particular that the lack of oversight is no reason to prohibit Bitcoin and similar currencies. Regulation should only intervene once the law can effectively punish offenders. This is particularly the case for cryptocurrency exchanges, which represent the interface between fiat and digital currencies, as decentralized technology itself cannot be regulated. Stokes ( 2012 ) also deals with money laundering and digital currency, examining the cases of Bitcoin and the Linden dollar, a digital currency in the online multi-player game Second Life.
Maurer et al. ( 2013 ) investigate the semiotics of Bitcoin with a focus on its property as an irreversible payment system. They argue that Bitcoin may be an appropriate method for enthusiasts looking for a payment system that ensures privacy and freedom from government or corporate interference. The promise and potential of Bitcoin derives not from the capability of government agencies or from mutual trust but from a cryptographic protocol. To this end, the authors suggest that practical materialism is based on concerns about privacy and values. Bitcoin is a promise that no other currency offers in this form: its value is secured by an algorithm and a peer-to-peer network. Ober et al. ( 2013 ), a publication from computer science, discuss Bitcoin and its facilitation of anonymous transactions between users in a network. They demonstrate that the Bitcoin network is a valuable source for empirical research on network structure and dynamics and the associated challenges of anonymity, as well as on payment systems. The authors identify dynamic effects that both enhance and inhibit anonymity. Another study from computer science, Reid and Harrigan ( 2013 ), analyzes the Bitcoin network and the degree to which the transactions are anonymous. The authors conclude that all historical transactions are publicly accessible and can be viewed by users by means of appropriate tools. Accounts can be linked and bitcoins can be ‘colored’ to trace the transaction history. Bitcoin is therefore not a completely anonymous network on the technical level. Similarly, other studies in the stream are written by reputable scientists from the field of cryptography and (also) analyze the nature of Bitcoin transactions and their degree of anonymity (e.g. Chaum 2012 ; Meiklejohn et al. 2013 ; Ron and Shamir 2014 ).
Monetary theory and policy
The fifth research stream explains 3.73% of the variance and consists of 10 articles plus three additional ones with high factor scores (cf. Table 7 ). The basic theme of the discourse is monetary theory, economics and policy. The network of authors is comparatively small. George Selgin and Randall Wright authored three publications, and William J. Luther contributed to four.
King ( 2016 ) provides an overview of the institutions of monetary policy but notes that his article raises more questions than it answers. The paper presents externalities in the theory of money and discusses the role of institutions. Finally, three case studies are presented, which serve to emphasize the importance of credible and stable institutions.
Selgin ( 2002 ) is the study with the highest factor loading (0.945) in the research stream. The article explores the implications of adaptive learning for monetary evolution using a search-theoretic framework. The author affirms the statement of Grossman ( 1991 ) that reconciliation of a theory with facts in monetary economics poses a significant problem, and introduces a formal monetary economics model that allows adaptive learning in a search-theoretic framework. Other studies in the research stream also deal with search-theoretic models of money (Kiyotaki and Wright 2016 ; Li and Wright 1998 ). The article with the second highest factor loading (0.943) is also by Selgin ( 1994 ) and discusses whether, given monetary nationalism, nations have an incentive to establish their own currencies, which have no relation to an exchange rate of established money, through market interventions. Salter and Luther ( 2014 ) also discuss the role of governments in monetary policy and introduce a general theory of the appearance and perpetuation of money.
Besides economic analyses, the discourse also includes legal policy recommendations for dealing with digital currencies. White ( 2015 ) provides an overview of the market for digital currencies and explains that the market is still developing and full of surprises. He suggests that regulation should be very cautious, as interference in entrepreneurial discovery prevents potential breakthroughs that society does not yet know it misses (Kirzner 1985 ). Selgin ( 2015 ) discusses synthetic commodity money. Bitcoin is cited as an example and its underlying properties such as mining and emission are discussed against the background of monetary theory. The study has the highest factor score (4.228) and is therefore a major influence on the discourse. Similarly, Hendrickson et al. ( 2016 ), the study with the second highest factor score (3.804), explicitly analyze Bitcoin as a currency and, among other things, build an equilibrium model in which Bitcoin coexists with a government-issued currency.
- Social network analysis
Having identified the most relevant research streams on blockchain and cryptocurrency in the areas of business and economics, the question arises how they are related. Social network analysis can serve to visualize the connections within and between the research streams. The results are shown in Fig. 3 . The frequency of citation, a metric for the relevance of a publication, is represented by the size of the nodes. Each node is colored according to the research stream it is associated with, i.e. with respect to which it has a factor score of 0.4 or more. If a paper exceeds that value with respect to several factors, it is assigned where the factor score is greatest. The nodes (publications) are linked by a line in case of five or more co-citations.
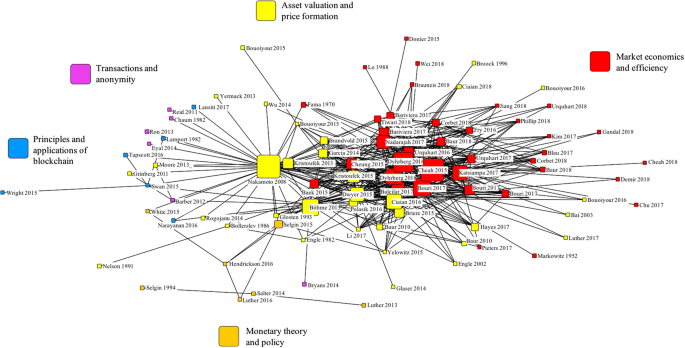
Visualization of research stream interrelations. The size of a node represents the absolute number of citations, lines between articles represent five co-citations between articles. For readability, only relationships ≥ 5 and only first authors are shown. Color represents research stream affiliation of nodes. Created with UCINET software
The social network analysis yields conclusions about the state of research and the maturity of the streams. Streams III (transactions and anonymity) and IV (principles and applications of blockchain) use Nakamoto ( 2008 ) as their ‘original source’, as the discourses do not yet have their own intellectual midpoints, as evidenced by the very high factor scores of the Bitcoin whitepaper in the two streams. The whitepaper is best assigned to the second research stream (cf. Table 1 ). This stream has already developed considerably further, having produced several other prominent publications (e.g. Böhme et al. 2015 ; Ciaian et al. 2016 ; Dwyer 2015 ; Kristoufek 2013 , 2015 ).
The most developed stream is No. I, market economics and efficiency. Its relationship to other streams is mostly limited to No. II, asset valuation and pricing. These two streams share a number of authors: Baur and Lucey ( 2010 ) and Baur and McDermott ( 2010 ) belong to stream II, while Baur et al. ( 2018a , b ) belong to stream I. The field of monetary theory and implications is characterized by Selgin ( 2015 ), which is closely related to the research stream on asset valuation and price formation, and thus to the Bitcoin whitepaper. Many of the other publications clearly distance themselves thematically from the other research areas, which is also expressed, for example, by the very high factor loadings of the individual publications (see Table 7 ).
Blockchain and its manifestation cryptocurrency are basic technologies that entail a multitude of effects and vast potential for business models, corporate decisions and the economy. Researchers in this field have already generated substantial insights. Through factor analysis, five prominent research streams have been identified that are characterized by different studies. Since the technology has only existed since 2008, various other research streams can be expected to emerge in the future. Unsurprisingly, the results show Nakamoto ( 2008 ) to be a common core of several research streams. Nevertheless, it is already apparent that the progress of research is creating its own basis. Figure 3 , for example, shows that among others Cheah and Fry ( 2015 ) and Dyhrberg ( 2016b ) are located in the middle of the stream.
Various methods and economic theories are identified as formative literature. For example, GARCH models in stream II and economic theory in stream IV seem to be of high relevance. A partial absence, or rather wide spread across different methods and theories is observed, which can be an indication that the scientific discourse is still so young that researchers can apply a variety of methods. It is possible to refer to different theories. This may consolidate in the future if the streams continue to grow or split up into subcategories.
The first stream deals with the efficiency and economics of cryptocurrency markets and has already created its own scientific basis (cf. Figure 3 ). Fundamental theoretical contributions such as Fama ( 1970 ) or Markowitz ( 1952 ) are referenced but are not overly relevant for the discourse. This own basis largely comprises findings from the years 2015 to 2017, which could suggest that the field is developing rapidly (cf. Figure 2 ). Stream I is also characterized by a small but highly active group of authors, many of whom joined the field only recently. Logically, this results in numerous co-citations among these authors.
The second stream is best described as research on asset valuation and price formation. It is clearly related to the first stream, which is to be expected given the similarities between (financial) market behavior and price development in these markets. In Fig. 3 , we see that stream II is concentrated at the center of the network and closely connected to stream I. In addition, a number of stream II publications are located in the outer parts of the network around streams I, III and IV. Only stream V has no articles close to its own network that can be assigned to the discourse of asset pricing. Questions about the prices or trading of cryptocurrency seem to be of universal relevance, which is why they interact with all other streams. Here again the question arises whether research stream II will split up in the near future. This seems likely, given the diversity of the articles. However, it should be pointed out that Nakamoto ( 2008 ), for example, only fits well with stream II. The whitepaper’s factor loading of 0.487 with respect to stream II is also similar to what it is with respect to the first stream (0.456).
In the emerging field of blockchain principles and applications, original fundamental research is only just beginning to replace the Bitcoin whitepaper, which continues to be of great importance. At present, the outstanding articles of the stream consist of economic and technical fundamentals as well as introductory literature (in the form of books). Blockchain and cryptocurrencies are constantly changing, and the existing basic literature (e.g. Swan 2015 ; Tapscott and Tapscott 2018 ) may soon become outdated, if that has not already happened. The stream may split into various more specialised streams in the future, including application-specific streams.
While more developed discourses will have peer-reviewed articles as their formative works, this is not the case for research on blockchain and its application, nor for transactions and anonymity. Most publications in stream IV date from 2013/14, which may mean that most relevant findings were published then and scientific progress has slowed down. However, it is much more likely that this branch of research has been neglected (by peer-reviewed research). This does not necessarily apply to other scientific fields like computer science or engineering that have not been examined here. In this respect, there is an opportunity to gain a foothold within the stream and to shape its further development, for example through the systematic processing, review, presentation or transfer of the technical literature.
Research on monetary theory is a special field that differs strongly from the other discourses (see Fig. 3 ). The small number of authors indicates that the stream remains specialized. However, Selgin ( 2015 ) constitutes a meaningful connection to other discourses, while having great influence on the stream itself. The inflow of further researchers dealing with monetary theory and cryptocurrency may yield additional connections to other streams – a worthwhile opportunity for future research.
Limitations
This study is subject to some limitations, of which only the most relevant ones can be mentioned here. In line with common practice in the literature, the data were collected exclusively from the Web of Science. While many comparable databases exist, which might have yielded additional papers, they were not used for technical reasons (ease of data export). Furthermore, research on blockchain and cryptocurrencies is also published outside of peer-reviewed journals (cf. e.g. the seminal whitepapers). Therefore, it is likely that the search of the Web of Science will have missed some important contributions. Finally, the choice of search terms of course shaped the results and is open to debate.
Future research avenues
Figure 3 shows that streams one and two are already very dense and have an established scientific basis. Looking at the other streams, especially III and IV, plenty of space next to Nakamoto ( 2008 ) seems available. It seems plausible that the scientific basis for these streams has not yet been established, which can be interpreted as an opportunity for researchers to fill this space. The blockchain ecosystem is evolving rapidly.
Figure 2 shows very clearly that research on market economics and efficiency has increased dramatically in relation to the other areas since 2014. However, this is probêably due not only to the relevance of the topic but also to comparatively swift channels of publication (e.g. via research letters or notes). Research in the other streams should in principle be similarly far-reaching, which indicates potential research gaps.
Three of the five streams appear to still be developing, which suggests a wealth of starting points in these areas. While this article has focused on the sources underlying the 467 scientific publications, trends for future research can also be derived from this core sample of papers. Several research clusters within this set could develop into research streams in their own right. So far, there are no dedicated streams on application areas, management or entrepreneurship. If and when such streams arise, this will be a good opportunity for enterprising researchers.
The literature suggests that blockchain and cryptocurrency will have a big impact on financial services (Fanning and Centers 2016 ). Therefore, a separate stream may well form around potential applications in the financial sector, that bases on research stream III. Various applications are already being discussed in the literature, such as accounting, auditing, assurance or governance (Dai and Vasarhelyi 2017 ; Schmitz and Leoni 2019 ; Shermin 2017 ; Tan and Low 2019 ). Research on entrepreneurial finance like blockchain-based financing in the form of ICOs (Adhami et al. 2018 ; Ante et al. 2018 ; Drobetz et al. 2019 ; Fisch 2019 ) or individual investors in cryptocurrencies (Ante et al. 2020 ) is getting well underway. This research often refers to signaling theory (Spence 1973 ) and builds on the literature on crowdfunding (e.g. Agrawal et al. 2015 ; Ahlers et al. 2015 ; Mollick 2014 ), initial public offerings (e.g. Carter and Manaster 1990 ; Certo et al. 2001 ; Colombo et al. 2019 ) and venture capital (e.g. Ahlstrom and Bruton 2006 ). Within the framework of blockchain-based financing, future research potential abounds. On the one hand, the market for decentralized finance (DeFi) is constantly producing new mechanisms, such as initial exchange offerings (IEOs), initial dex offerings (IDOs) or security token offerings (STOs) (Ante and Fiedler 2019 ). On the other hand, the existing literature on financing, in particular crowdfunding and IPOs, forms a basis for various evaluation options and methods that can still be carried out for blockchain research. Niche aspects of financial markets, such as cross-listings (Ante and Meyer 2019 ; Meyer and Ante 2020 ), price manipulation (Gandal et al. 2018 ), money laundering (Ante 2018 ) or informed trading (Ante 2019 ; Feng et al. 2018 ), are only just starting to be addressed by the cryptocurrency literature.
Energy markets or smart grids and blockchain technology have been a big topic in engineering and computer science but are only now being researched in business science and economics. Green and Newman ( 2017 ) explain the potential of the blockchain in the energy sector, and Oh et al. ( 2017 ) show what blockchain technology can do for energy trading. Andoni et al. ( 2019 ) provide a general introduction to the wide-ranging potential and points to explore around blockchain and energy, including for example metering and billing, cryptocurrency-usage, decentralized (wholesale) trading, green certificates and grid management. A separate research stream will likely form around the field of energy, smart grids and decentralized markets. Other sectors on which scientists have recently published initial findings and which warrant significantly more research include the sharing economy (Pazaitis et al. 2017 ), the real estate economy (Veuger 2012 ), blockchain-based ownership structures (McConaghy et al. 2017 ), digital identity (Wolfond 2017 ), healthcare (Engelhardt 2017 ) and supply chain and provenance (Kim and Laskowski 2018 ).
Current developments around Facebook’s crypto currency project Libra (Libra Association 2019 ) or the potential introduction of central bank digital currency (CBDC) by China (Reuters 2019 ) and other countries are likely to create much greater attention for research stream V. Economists have already begun to comment on Libra (e.g. Cecchetti and Schienholtz 2019 ; Eichengreen 2019 ), though not in peer-reviewed journals. The concept of so-called stable coins—blockchain tokens whose value is backed by fiat currencies or other assets such as gold—will for example find its way into monetary policy research and other research streams (e.g. Wei 2018b ). Here, a differentiation can be made between decentralized protocols (e.g. makerdao.com) and stable coins issued by companies (e.g. tether.to). Against this background, a potential merging of research stream V with the other areas presents clear opportunities for researchers.
This study has analysed the scientific basis of the existing literature in the field of blockchain technology and cryptocurrency using quantitative methodology, which enables a neutral and objective evaluation. Five research streams with the greatest thematic relevance were identified—two of which are already mature while three are still emerging—and their underlying content and approaches were presented. The results provide a basis for all researchers working within one of the five research streams. The identification of core publications in each sphere may serve as a starting point for new research endeavours. The interrelations between the discourses show that this young field of research still leaves a lot of room for manoeuvre, including fundamental original work. In other words, there is still ample space for scientific discovery next to Nakamoto ( 2008 ).
Adhami, S., Giudici, G., & Martinazzi, S. (2018). Why do businesses go crypto? An empirical analysis of initial coin offerings. Journal of Economics and Business, 100 , 64–75. https://doi.org/10.1016/j.jeconbus.2018.04.001 .
Article Google Scholar
Agrawal, A., Catalini, C., & Goldfarb, A. (2015). Crowdfunding: geography, social networks, and the timing of investment decisions. Journal of Economics and Management Strategy, 24 (2), 253–274. https://doi.org/10.1111/jems.12093 .
Ahlers, G. K., Cumming, D., Günther, C., & Schweizer, D. (2015). Signaling in equity crowdfunding. Entrepreneurship Theory and Practice, 39 (4), 955–980. https://doi.org/10.1111/etap.12157 .
Ahlstrom, D., & Bruton, G. D. (2006). Venture capital in emerging economies: networks and institutional change. Entrepreneurship Theory and Practice, 30 (2), 299–320. https://doi.org/10.1111/j.1540-6520.2006.00122.x .
Aiyagari, S. R., & Wallace, N. (1997). Government transaction policy, the medium of exchange, and welfare. Journal of Economic Theory, 74 (1), 1–18. https://doi.org/10.1006/jeth.1996.2250 .
Article MathSciNet MATH Google Scholar
Alvarez-Ramirez, J., Rodriguez, E., & Ibarra-Valdez, C. (2018). Long-range correlations and asymmetry in the Bitcoin market. Physica A: Statistical Mechanics and its Applications, 492 , 948–955. https://doi.org/10.1016/j.physa.2017.11.025 .
Andoni, M., Robu, V., Flynn, D., Abram, S., Geach, D., Jenkins, D., et al. (2019). Blockchain technology in the energy sector: A systematic review of challenges and opportunities. Renewable and Sustainable Energy Reviews, 100 , 143–174. https://doi.org/10.1016/j.rser.2018.10.014 .
Ante, L. (2018). Cryptocurrency, Blockchain and Crime. In K. McCarthy (Ed.), The Money Laundering Market: Regulating the Criminal Economy (pp. 171–198). Agenda Publishing. doi:10.2307/j.ctv5cg8z1.10
Ante, L. (2019). Market reaction to exchange listings of cryptocurrencies. . https://doi.org/10.13140/RG.2.2.19924.76161 .
Ante, L., & Fiedler, I. (2019). Cheap signals in security token offerings (STOs). . https://doi.org/10.2139/ssrn.3356303 .
Ante, L., Fiedler, I., von Meduna, M., & Steinmetz, F. (2020). Returns from investing in cryptocurrency: evidence from german individual investors. . https://doi.org/10.2139/ssrn.3540876 .
Ante, L., & Meyer, A. (2019). Cross-listings of blockchain-based tokens issued through initial coin offerings: do liquidity and specific cryptocurrency exchanges matter? doi:10.13140/RG.2.2.27494.37442
Ante, L., Sandner, P., & Fiedler, I. (2018). Blockchain-based ICOs: pure hype or the dawn of a new era of startup financing? Journal of Risk and Financial Management, 11 (4), 80. https://doi.org/10.3390/jrfm11040080 .
Apte, S., & Petrovsky, N. (2016). Will blockchain technology revolutionize excipient supply chain management? Journal of Excipients and Food Chemicals, 7 (3), 76–78.
Google Scholar
Aron, J. (2012). BitCoin software finds new life. New Scientist, 213 (2847), 20.
Ashta, A., & Biot-Paquerot, G. (2018). FinTech evolution: Strategic value management issues in a fast changing industry. Strategic Change, 27 (4), 301–311. https://doi.org/10.1002/jsc.2203 .
Atzori, M. (2017). Blockchain technology and decentralized governance: is the state still necessary? Journal of Governance and Regulation, 6 (1), 1–37. https://doi.org/10.22495/jgr_v6_i1_p5 .
Back, A. (2002). Hashcash - a denial of service counter-measure . www.hashcash.org/hashcash.pdf . Accessed 1 September 2019
Bariviera, A. F. (2017). The inefficiency of Bitcoin revisited: A dynamic approach. Economics Letters, 161 , 1–4. https://doi.org/10.1016/j.econlet.2017.09.013 .
Article MathSciNet Google Scholar
Bariviera, A. F., Basgall, M. J., Hasperué, W., & Naiouf, M. (2017). Some stylized facts of the Bitcoin market. Physica A: Statistical Mechanics and its Applications, 484 , 82–90. https://doi.org/10.1016/j.physa.2017.04.159 .
Batubara, F. R., Ubacht, J., & Janssen, M. (2018). Challenges of Blockchain Technology Adoption for e-Government: A Systematic Literature Review. In Proceedings of the 19th Annual International Conference on Digital Government Research: Governance in the Data Age . ACM.
Baur, D. G., Dimpfl, T., & Kuck, K. (2018a). Bitcoin, gold and the US dollar—A replication and extension. Finance Research Letters, 25 , 103–110. https://doi.org/10.1016/j.frl.2017.10.012 .
Baur, D. G., Hong, K. H., & Lee, A. D. (2018b). Bitcoin: Medium of exchange or speculative assets? Journal of International Financial Markets, Institutions and Money, 54 , 177–189. https://doi.org/10.1016/j.intfin.2017.12.004 .
Baur, D. G., & Lucey, B. M. (2010). Is gold a hedge or a safe haven? An analysis of stocks, bonds and gold. Financial Review, 45 (2), 217–229. https://doi.org/10.1111/j.1540-6288.2010.00244.x .
Baur, D. G., & McDermott, T. K. (2010). Is gold a safe haven? International evidence. Journal of Banking and Finance, 34 (8), 1886–1898. https://doi.org/10.1016/j.jbankfin.2009.12.008 .
Bayer, D., Haber, S., & Stornetta, S. W. (1993). Improving the efficiency and reliability of digital time-stamping. Sequences, II , 329–334. https://doi.org/10.1007/978-1-4613-9323-8_24 .
Article MATH Google Scholar
Biehl, M., Kim, H., & Wade, M. (2006). Relationships among the academic business disciplines: A multi-method citation analysis. Omega, 34 (4), 359–371. https://doi.org/10.1016/j.omega.2004.12.002 .
Böhme, R., Christin, N., Edelman, B., & Moore, T. (2015). Bitcoin: economics, technology, and governance. Journal of Economic Perspectives, 29 (2), 213–238. https://doi.org/10.1257/jep.29.2.213 .
Borgatti, S. P., Everett, M. G., & Freeman, L. C. (2002). Ucinet for Windows: Software for social network analysis. Harvard MA: analytical technologies , 6 .
Bouoiyour, J., Aviral, R., Tiwari, K., & Olayeni, O. R. (2016). What drives Bitcoin price? Economics Bulletin, 36 (2), 843–850.
Bouoiyour, J., & Selmi, R. (2015). What does Bitcoin look like? In Handbook of digital currency (pp. 31–43).
Bouri, E., Molnár, P., Azzi, G., Roubaud, D., & Hagfors, L. I. (2017). On the hedge and safe haven properties of Bitcoin: Is it really more than a diversifier? Finance Research Letters, 20 , 192–198. https://doi.org/10.1016/j.frl.2016.09.025 .
Brauneis, A., & Mestel, R. (2018). Price discovery of cryptocurrencies: Bitcoin and beyond. Economics Letters, 165 , 58–61. https://doi.org/10.1016/j.econlet.2018.02.001 .
Bryans, D. (2014). Bitcoin and money laundering: mining for an effective solution. Indiana Law Journal, 89 (1), 441–472.
Buterin, V. (2013). Ethereum White Paper - A Next Generation Smart Contract & Decentralized Application Platform . https://blockchainlab.com/pdf/Ethereum_white_paper-a_next_generation_smart_contract_and_decentralized_application_platform-vitalik-buterin.pdf
Carrington, P. J., Scott, J., & Wassermann, S. (2005). Models and methods in social network analysis . New York, NY: Cambridge University Press.
Book Google Scholar
Carter, R., & Manaster, S. (1990). Initial public offerings and underwriter reputation. The Journal of Finance, 45 (4), 1045–1067. https://doi.org/10.1111/j.1540-6261.1990.tb02426.x .
Castro, M., & Liskov, B. (1999). Practical byzantine fault tolerance. OSDI, 99 , 173–186.
Caytas, J. D. (2016). Developing blockchain real-time clearing and settlement in the EU, U.S., and globally .
Cecchetti, S., & Schienholtz, K. (2019). Libra: A dramatic call to regulatory action. VOX CEPR Policy Portal . Retrieved 31 Aug 2019 from: https://voxeu.org/article/libra-dramatic-call-regulatory-action .
Certo, S. T., Daily, C. M., & Dalton, D. R. (2001). Signaling firm value through board structure: an investigation of initial public offerings. Entrepreneurship Theory and Practice, 26 (2), 33–50. https://doi.org/10.1177/104225870102600202 .
Chaum, D. (2012). Advances in Cryptology: proceedings of Crypto . Springer Science & Business Media.
Cheah, E. T., & Fry, J. (2015). Speculative bubbles in Bitcoin markets? An empirical investigation into the fundamental value of Bitcoin. Economics Letters, 130 , 32–36.
Cheah, E. T., Mishra, T., Parhi, M., & Zhang, Z. (2018). Long memory interdependency and inefficiency in Bitcoin markets. Economics Letters , 167 , 18–25. https://doi.org/10.1016/j.econlet.2018.02.010 .
Chen, Y., & Leimkuhler, F. F. (1986). A relationship between Lotka’s Law, Bradford’s Law, and Zipf’s Law. Journal of the American Society for Information Science, 37 (5), 307–314.
Chen, Z., Li, Y., Wu, Y., & Luo, J. (2017). The transition from traditional banking to mobile internet finance: an organizational innovation perspective – a comparative study of Citibank and ICBC. Financial Innovation, 3 (1), 1–6. https://doi.org/10.1186/s40854-017-0062-0 .
Chiu, J., & Koeppl, T. V. (2019). Blockchain-based settlement for asset trading. The Review of Financial Studies, 32 (5), 1716–1753. https://doi.org/10.1093/rfs/hhy122 .
Ciaian, P., Rajcaniova, M., & Kancs, D. (2016). The economics of BitCoin price formation. Applied Economics, 48 (19), 1799–1815. https://doi.org/10.1080/00036846.2015.1109038 .
Ciaian, P., Rajcaniova, M., & Kancs, D. (2018). Virtual relationships: Short- and long-run evidence from BitCoin and altcoin markets. Journal of International Financial Markets, Institutions and Money, 52 , 173–195. https://doi.org/10.1016/j.intfin.2017.11.001 .
Coase, R. H. (1937). The nature of the firm. Econometrica, 4 (16), 386–405. https://doi.org/10.1093/oxfordjournals.jleo.a036946 .
Cocco, L., Pinna, A., & Marchesi, M. (2017). Banking on blockchain: Costs savings thanks to the blockchain technology. Future Internet, 9 (3), 1–20. https://doi.org/10.3390/fi9030025 .
Colombo, M. G., Meoli, M., & Vismara, S. (2019). Signaling in science-based IPOs: The combined effect of affiliation with prestigious universities, underwriters, and venture capitalists. Journal of Business Venturing, 34 (1), 141–177.
Conoscenti, M., Vetro, A., & De Martin, J. C. (2017). Blockchain for the Internet of Things: A systematic literature review. In Proceedings of IEEE/ACS International Conference on Computer Systems and Applications, AICCSA (pp. 1–6). IEEE. doi:10.1109/AICCSA.2016.7945805
Corbet, S., Lucey, B., Urquhart, A., & Yarovaya, L. (2019). Cryptocurrencies as a financial asset: A systematic analysis. International Review of Financial Analysis, 62 , 182–199. https://doi.org/10.1016/j.irfa.2018.09.003 .
Corbet, S., Lucey, B., & Yarovaya, L. (2018a). Datestamping the Bitcoin and Ethereum bubbles. Finance Research Letters, 26 , 81–88. https://doi.org/10.1016/j.frl.2017.12.006 .
Corbet, S., Meegan, A., Larkin, C., Lucey, B., & Yarovaya, L. (2018b). Exploring the dynamic relationships between cryptocurrencies and other financial assets. Economics Letters, 165 , 28–34. https://doi.org/10.1016/j.econlet.2018.01.004 .
Dabbagh, M., Sookhak, M., & Safa, N. S. (2019). The evolution of blockchain: A bibliometric study. IEEE Access, 7 , 19212–19221. https://doi.org/10.1109/ACCESS.2019.2895646 .
Dai, J., & Vasarhelyi, M. A. (2017). Toward blockchain-based accounting and assurance. Journal of Information Systems . https://doi.org/10.2308/isys-51804 .
Dai, W. (1998). b-money. Retrieved 9 Sep 2019 from: www.weidai.com/bmoney.txt .
DiStefano, C., Zhu, M., & Mîndrilǎ, D. (2009). Understanding and using factor scores: Considerations for the applied researcher. Practical Assessment, Research and Evaluation, 14 (1), 20.
Donier, J., & Bouchaud, J. P. (2015). Why do markets crash? Bitcoin data offers unprecedented insights. PLoS ONE, 10 (10), 23–25. https://doi.org/10.1371/journal.pone.0139356 .
Drobetz, W., Momtaz, P. P., & Schröder, H. (2019). Investor sentiment and initial coin offerings. The Journal of Alternative Investments, 21 (4), 41–55. https://doi.org/10.3905/jai.2019.1.069 .
Dunphy, P., & Petitcolas, F. A. P. (2018). A first look at identity management schemes on the blockchain. IEEE Security and Privacy, 16 (4), 20–29. https://doi.org/10.1109/MSP.2018.3111247 .
Dwork, C., & Naor, M. (1992). Pricing via processing or combatting junk mail. Annual International Cryptology Conference (pp. 139–147). Berlin, Heidelberg: Springer.
Dwyer, G. P. (2015). The economics of Bitcoin and similar private digital currencies. Journal of Financial Stability, 17 , 81–91. https://doi.org/10.1016/j.jfs.2014.11.006 .
Dyhrberg, A. H. (2016a). Bitcoin, gold and the dollar – A GARCH volatility analysis. Finance Research Letters, 16 , 85–92. https://doi.org/10.1016/j.frl.2015.10.008 .
Dyhrberg, A. H. (2016b). Hedging capabilities of bitcoin. Is it the virtual gold? Finance Research Letters, 16 , 139–144. https://doi.org/10.1016/j.frl.2015.10.025 .
Eichengreen, B. (2019). Libra: The known unknown and unknown unkowns. VOX CEPR Policy Portal . Retrieved 8 Sep 2019 from: https://voxeu.org/article/libra-known-unknowns-and-unknown-unknowns .
Engelhardt, M. A. (2017). Hitching healthcare to the chain: an introduction to blockchain technology in the healthcare sector. Technology Innovation Management Review, 7 (10), 22–34. https://doi.org/10.22215/timreview/1111 .
Engle, R. (2002). Dynamic conditional correlation: A simple class of multivariate generalized autoregressive conditional heteroskedasticity models. Journal of Business and Economic Statistics, 20 (3), 339–350. https://doi.org/10.1198/073500102288618487 .
Esposito, C., De Santis, A., Tortora, G., Chang, H., & Choo, K. K. R. (2018). Blockchain: A panacea for healthcare cloud-based data security and privacy? IEEE Cloud Computing, 5 (1), 31–37. https://doi.org/10.1109/MCC.2018.011791712 .
Eyal, I., & Sirer, E. G. (2014). Majority Is Not Enough: Bitcoin mining is vulnerable. In Eighteenth International Conference on Financial Cryptography and Data Security .
Fama, E. F. (1970). Efficient capital markets: A review of theory and empirical work. The Journal of Finance, 25 (2), 383–417. https://doi.org/10.2307/2325486 .
Fanning, K., & Centers, D. P. (2016). Blockchain and its coming impact on financial services. Journal of Corporate Accounting and Finance, 27 (5), 53–57. https://doi.org/10.1002/jcaf .
Feng, W., Wang, Y., & Zhang, Z. (2018). Informed trading in the Bitcoin market. Finance Research Letters, 26 , 63–70. https://doi.org/10.1016/j.frl.2017.11.009 .
Fiedler, I., Ante, L., Steinmetz, F., & Häseler, S. (2018). Distributed Ledger Technology: A Possible Way Forward for Securities Clearing. Binary District . Retrieved 8 Sep 2019 from: https://journal.binarydistrict.com/distributed-ledger-technology-a-possible-way-forward-for-securities-clearing/ .
Firdaus, A., Razak, M. F. A., Feizollah, A., Hashem, I. A. T., Hazim, M., & Anuar, N. B. (2019). The rise of “blockchain”: bibliometric analysis of blockchain study. Scientometrics, 120 (3), 1289–1331. https://doi.org/10.1007/s11192-019-03170-4 .
Fisch, C. (2019). Initial coin offerings (ICOs) to finance new ventures. Journal of Business Venturing, 34 (1), 1–22. https://doi.org/10.1016/j.jbusvent.2018.09.007 .
Francisco, K., & Swanson, D. (2018). The supply chain has no clothes: technology adoption of blockchain for supply chain transparency. Logistics, 2 (1), 2. https://doi.org/10.3390/logistics2010002 .
Fry, J., & Cheah, E. T. (2016). Negative bubbles and shocks in cryptocurrency markets. International Review of Financial Analysis, 47 , 343–352. https://doi.org/10.1016/j.irfa.2016.02.008 .
Gandal, N., Hamrick, J. T., Moore, T., & Oberman, T. (2018). Price manipulation in the Bitcoin ecosystem. Journal of Monetary Economics, 95 , 86–96. https://doi.org/10.1016/j.jmoneco.2017.12.004 .
Gkillas, K., & Katsiampa, P. (2018). An application of extreme value theory to cryptocurrencies. Economics Letters, 164 , 109–111. https://doi.org/10.1016/j.econlet.2018.01.020 .
Glosten, L. R., Jagannathan, R., & Runkle, D. E. (1993). On the relation between the expected value and the volatility of the nominal excess return on stocks. The Journal of Finance, 48 (5), 1779–1801.
Gomber, P., Kauffman, R. J., Parker, C., & Weber, B. W. (2018). On the fintech revolution: interpreting the forces of innovation, disruption, and transformation in financial services. Journal of Management Information Systems, 35 (1), 220–265. https://doi.org/10.1080/07421222.2018.1440766 .
Gorsuch, R. L. (1988). Exploratory Factor Analysis. In J. R. Nesselroade & R. B. Cattell (Eds.), Handbook of Multivariate Experimental Psychology (pp. 231–258). Boston, MA: Springer, US. https://doi.org/10.1007/978-1-4613-0893-5_6 .
Chapter Google Scholar
Governatori, G., Idelberger, F., Milosevic, Z., Riveret, R., Sartor, G., & Xu, X. (2018). On legal contracts, imperative and declarative smart contracts, and blockchain systems. Artificial Intelligence and Law, 26 (4), 377–408.
Green, J., & Newman, P. (2017). Citizen utilities: The emerging power paradigm. Energy Policy, 105 (March), 283–293. https://doi.org/10.1016/j.enpol.2017.02.004 .
Grossman, H. (1991). Monetary Economics – A Review Essay . National Bureau of Economic Research.
Guo, Y., & Liang, C. (2016). Blockchain application and outlook in the banking industry. Financial Innovation, 2 (1), 24. https://doi.org/10.1186/s40854-016-0034-9 .
Haber, S., & Stornetta, S. W. (1990). How to time-stamp a digital document. Conference on the Theory and Application of Cryptography (pp. 437–455). Berlin, Heidelberg: Springer.
Haber, S., & Stornetta, S. W. (1997). Secure names for bit-strings. Proceedings of the ACM Conference on Computer and Communications Security , 28–35.
Harris, L. (1991). Stock price clustering and discreteness. Review of Financial Studies, 4 , 389–415.
Hawlitschek, F., Notheisen, B., & Teubner, T. (2018). The limits of trust-free systems: A literature review on blockchain technology and trust in the sharing economy. Electronic Commerce Research and Applications, 29 , 50–63. https://doi.org/10.1016/j.elerap.2018.03.005 .
Hayes, A. S. (2017). Cryptocurrency value formation: An empirical study leading to a cost of production model for valuing bitcoin. Telematics and Informatics, 34 (7), 1308–1321. https://doi.org/10.1016/j.tele.2016.05.005 .
Hendrickson, J. R., Hogan, T. L., & Luther, W. J. (2016). The political economy of bitcoin. Economic Inquiry, 54 (2), 925–939. https://doi.org/10.1111/ecin.12291 .
Holub, M., & Johnson, J. (2018). Bitcoin research across disciplines. Information Society, 34 (2), 114–126. https://doi.org/10.1080/01972243.2017.1414094 .
Hurst, H. E. (1951). Long-term storage capacity of reservoirs. Transactions of the American Society of Civil Engineers, 116 , 770–808.
Iansiti, M., & Lakhani, H. (2017). The truth about blockchain. Harvard Business Review, 95 (1), 118–127.
Jensen, M. C., & Meckling, W. H. (1976). Theory of the firm managerial behaviour, ageny costs and ownership structure. Journal of Financial Economics, 3 , 305–360.
Kaiser, H. F. (1959). Computer program for varimax rotation in factor analysis. Educational and Psychological Measurement, 19 (3), 413–420. https://doi.org/10.1177/001316445901900314 .
Karafiloski, E., & Mishev, A. (2017). Blockchain solutions for big data challenges: A literature review. In 17th IEEE International Conference on Smart Technologies, EUROCON 2017 - Conference Proceedings (pp. 763–768). doi:10.1109/EUROCON.2017.8011213
Katsiampa, P. (2017). Volatility estimation for Bitcoin: A comparison of GARCH models. Economics Letters, 158 , 3–6. https://doi.org/10.1016/j.econlet.2017.06.023 .
Khan, M. A., & Salah, K. (2018). IoT security: Review, blockchain solutions, and open challenges. Future Generation Computer Systems, 82 , 395–411. https://doi.org/10.1016/j.future.2017.11.022 .
Kim, H. M., & Laskowski, M. (2018). Toward an ontology-driven blockchain design for supply-chain provenance. Intelligent Systems in Accounting, Finance and Management, 25 (1), 18–27. https://doi.org/10.1002/isaf.1424 .
Kim, S., & Sarin, A. (2019). Distributed ledger and blockchain technology: framework and use cases. Journal of Investment Management . https://doi.org/10.2139/ssrn.3373347 .
Kim, T. (2017). On the transaction cost of Bitcoin. Finance Research Letters, 23 , 300–305. https://doi.org/10.1016/j.frl.2017.07.014 .
King, M. (2016). The institutions of monetary policy. The American Economic Review, 94 (2), 1–13. https://doi.org/10.1257/0002828041301957 .
Kirzner, I. M. (1985). The Perils of Regulation: A Market-Process Approach. In Discovery and the Capitalist Process . Chicago: University of Chicago Press.
Kiyotaki, B. N., & Wright, R. (2016). A search-theoretic approach to monetary economics. The American Economic Review, 83 (1), 63–77.
Klarin, A. (2020). The decade-long cryptocurrencies and the blockchain rollercoaster: Mapping the intellectual structure and charting future directions. Research in International Business and Finance, 51 , 101067. https://doi.org/10.1016/j.ribaf.2019.101067 .
Kristoufek, L. (2013). BitCoin meets Google Trends and Wikipedia: Quantifying the relationship between phenomena of the Internet era. Scientific Reports, 3 , 1–7. https://doi.org/10.1038/srep03415 .
Kristoufek, L. (2015). What are the main drivers of the bitcoin price? Evidence from wavelet coherence analysis. PLoS ONE, 10 (4), 1–15. https://doi.org/10.1371/journal.pone.0123923 .
Kristoufek, L. (2018). On Bitcoin markets (in)efficiency and its evolution. Physica A: Statistical Mechanics and its Applications, 503 , 257–262. https://doi.org/10.1016/j.physa.2018.02.161 .
Krugman, P. (2013). Bitcoin Is Evil. The New York Times . Retrieved 4 Sep 2019 from: https//krugman.blogs.nytimes.com/2013/12/28/bitcoin-is-evil/.
Kuntner, T., & Teichert, T. (2016). The scope of price promotion research: An informetric study. Journal of Business Research, 69 (8), 2687–2696. https://doi.org/10.1016/j.jbusres.2015.11.004 .
Lagos, R., & Wright, R. (2005). A unified framework for monetary theory and policy analysis. Journal of Political Economy, 113 (3), 463–484. https://doi.org/10.1086/429804 .
Lahmiri, S., Bekiros, S., & Salvi, A. (2018). Long-range memory, distributional variation and randomness of bitcoin volatility. Chaos, Solitons and Fractals, 107 , 43–48. https://doi.org/10.1016/j.chaos.2017.12.018 .
Lamport, L., Shostak, R., & Pease, M. (1982). The Byzantine generals problem. ACM Transactions on Programming Languages and Systems (TOPLAS), 4 (3), 382–401.
Li, Y., & Wright, R. (1998). Government transaction policy, media of exchange, and prices. Journal of Economic Theory, 81 (2), 290–313. https://doi.org/10.1006/jeth.1997.2363 .
Liang, X., Zhao, J., Shetty, S., Liu, J., & Li, D. (2018). Integrating blockchain for data sharing and collaboration in mobile healthcare applications. IEEE International Symposium on Personal, Indoor and Mobile Radio Communications, PIMRC , 2017 - October , 1–5. doi:10.1109/PIMRC.2017.8292361
Libra Association. (2019). An Introduction to Libra. Retrieved 15 Sep 2019 from: https://libra.org/en-US/wp-content/uploads/sites/23/2019/06/LibraWhitePaper_en_US.pdf .
Liu, J. (2016). Bitcoin Literature: a Co-Word Analysis. In 6th Economics & Finance Conference, OECD: Paris (pp. 262–272). doi:10.20472/efc.2016.006.013
Luther, W. J. (2013). Friedman versus Hayek on private outside monies: New evidence for the debate. Economic Affairs, 33 (1), 127–135. https://doi.org/10.1111/ecaf.12001 .
Luther, W. J. (2016). Bitcoin and the future of digital payments. The Independent Review, 20 (3), 397–404.
Markowitz, H. (1952). Portfolio selection. The Journal of Finance, 7 (1), 77–91.
Massias, H., Avila, X. S., & Quisquater, J. J. (1999). Design of a secure timestamping service with minimal trust requirement. In The 20th Symposium on Information Theory in the Benelux .
Maurer, B., Nelms, T. C., & Swartz, L. (2013). When perhaps the real problem is money itself!: The practical materiality of Bitcoin. Social Semiotics, 23 (2), 261–277. https://doi.org/10.1080/10350330.2013.777594 .
McCain, K. W. (1990). Mapping authors in intellectual space: A technical overview. Journal of the American Society for Information Science, 41 (6), 433–443. https://doi.org/10.1002/(SICI)1097-4571(199009)41:6%3c433:AID-ASI11%3e3.0.CO;2-Q .
McConaghy, M., McMullen, G., Parry, G., McConaghy, T., & Holtzman, D. (2017). Visibility and digital art: Blockchain as an ownership layer on the Internet. Strategic Change, 26 (5), 461–470. https://doi.org/10.1002/jsc.2146 .
Meiklejohn, S., Pomarole, M., Jordan, G., Levchenko, K., McCoy, D., Voelker, G. M., & Savage, S. (2013). A fistful of Bitcoins: Characterizing payments among men with no names. In Proceedings of the 2013 conference on Internet measurement conference. ACM (pp. 127–140). doi:10.1145/2896384
Merediz-Solá, I., & Bariviera, A. F. (2019). A bibliometric analysis of bitcoin scientific production. Research in International Business and Finance, 50 (June), 294–305. https://doi.org/10.1016/j.ribaf.2019.06.008 .
Merkle, R. C. (1980). Protocols for public key cryptosystems. Proceedings - IEEE Symposium on Security and Privacy, 122–134 , https://doi.org/10.1109/SP.1980.10006 .
Meyer, A., & Ante, L. (2020). Effects of initial coin offering characteristics on cross-listing returns. . https://doi.org/10.13140/RG.2.2.10972.33920 .
Miau, S., & Yang, J. M. (2018). Bibliometrics-based evaluation of the Blockchain research trend: 2008–March 2017. Technology Analysis and Strategic Management, 30 (9), 1029–1045. https://doi.org/10.1080/09537325.2018.1434138 .
Miller, M. (1997). The Future of Law. In paper delivered at the Extro (p. 3).
Mollick, E. R. (2014). The dynamics of crowdfunding: An exploratory study. Journal of Business Venturing, 29 (1), 1–16. https://doi.org/10.1016/j.jbusvent.2013.06.005 .
Nadarajah, S., & Chu, J. (2017). On the inefficiency of Bitcoin. Economics Letters, 150 , 6–9. https://doi.org/10.1016/j.econlet.2016.10.033 .
Nakamoto, S. (2008). Bitcoin: A Peer-to Peer Electronic Cash System. Retrieved 12 June 2019 from: https://bitcoin.org/bitcoin.pdf .
Nerur, S. P., Rasheed, A. A., & Natarajan, V. (2008). The intellectual structure of the strategic management field: An author co-citation analysis. Strategic Management Journal, 29 (3), 319–336. https://doi.org/10.1002/smj.659 .
O’Shields, R. (2017). Smart contracts: Legal agreements for the blockchain. North Carolina Banking Institute, 21 (1), 177–194.
Ober, M., Katzenbeisser, S., & Hamacher, K. (2013). Structure and anonymity of the bitcoin transaction graph. Future Internet, 5 (2), 237–250. https://doi.org/10.3390/fi5020237 .
Oh, S.-C., Kim, M.-S., Park, Y., Roh, G.-T., & Lee, C.-W. (2017). Implementation of blockchain-based energy trading system. Asia Pacific Journal of Innovation and Entrepreneurship, 11 (3), 322–334. https://doi.org/10.1108/apjie-12-2017-037 .
Ølnes, S., Ubacht, J., & Janssen, M. (2017). Blockchain in government: Benefits and implications of distributed ledger technology for information sharing. Government Information Quarterly, 34 (3), 355–364. https://doi.org/10.1016/j.giq.2017.09.007 .
Pazaitis, A., De Filippi, P., & Kostakis, V. (2017). Blockchain and value systems in the sharing economy: The illustrative case of Backfeed. Technological Forecasting and Social Change, 125 , 105–115. https://doi.org/10.1016/j.techfore.2017.05.025 .
Persson, O., Danell, R., & Schneider, J. W. (2009). How to use Bibexcel for various types of bibliometric analysis. Celebrating scholarly communication studies: A Festschrift for Olle Persson at his 60th Birthday , 9–24. https://lup.lub.lu.se/record/1458990/file/1458992.pdf
Phillip, A., Chan, J., & Peiris, S. (2018). A new look at cryptocurrencies. Economics Letters, 163 , 6–9. https://doi.org/10.1016/j.econlet.2017.11.020 .
Reid, F., & Harrigan, M. (2013). An analysis of anonymity in the bitcoin system. Security and Privacy in Social Networks, 197–223 , https://doi.org/10.1007/978-1-4614-4139-7_10 .
Reuters. (2019). China says new digital currency will be similar to Facebook’s Libra. Reuters . Retrieved 4 Sep 2019 from: www.reuters.com/article/us-china-cryptocurrency-cenbank/china-says-new-digital-currency-will-be-similar-to-facebooks-libra .
Rogojanu, A., & Badea, L. (2014). The issue of competing currencies: Case study—Bitcoin. Theoretical and Applied Economics, 21 (1), 103–114.
Ron, D., & Shamir, A. (2014). Quantitative Analysis of the Full Bitcoin Transaction Graph. In International Conference on Financial Cryptography and Data Security . Springer: Berlin, Heidelberg. doi:10.1007/978–3–642-39884-1
Salmerón-Manzano, E., & Manzano-Agugliaro, F. (2019). The role of smart contracts in sustainability: Worldwide research trends. Sustainability, 11 (11), 3049. https://doi.org/10.3390/su11113049 .
Salter, A. W., & Luther, W. J. (2014). Synthesizing state and spontaneous order theories of money. In Entangled Political Economy (pp. 161–178). Emerald Group Publishing Limited.
Schmitz, J., & Leoni, G. (2019). Accounting and auditing at the time of blockchain technology: A research agenda. Australian Accounting Review, 29 (2), 331–342. https://doi.org/10.1111/auar.12286 .
Seebacher, S., & Schüritz, R. (2017). Blockchain technology as an enabler of service systems: A structured literature review. International Conference on Exploring Services Science (pp. 12–23). Cham: Springer. https://doi.org/10.1007/978-3-319-56925-3_2 .
Selgin, G. (1994). On ensuring the acceptability of a new fiat money. Journal of Money, Credit and Banking, 26 (4), 808–826. https://doi.org/10.2307/2077948 .
Selgin, G. (2002). Adaptive learning and the transition to fiat money. The Economic Journal, 113 (484), 147–165. https://doi.org/10.1111/1468-0297.00094 .
Selgin, G. (2015). Synthetic commodity money. Journal of Financial Stability, 17 , 92–99. https://doi.org/10.1016/j.jfs.2014.07.002 .
Shermin, V. (2017). Disrupting governance with blockchains and smart contracts. Strategic Change, 26 (5), 499–509. https://doi.org/10.1002/jsc.2150 .
Small, H. G. (1973). Co-citation in the scientific literature: A new measure of the relationship between two documents. Journal of the American Society for Information Science, 24 , 265–269. https://doi.org/10.1002/asi.4630240406 .
Small, H. G. (1977). A co-citation model of a scientific specialty: A longitudinal study of collagen research. Social Studies of Science, 7 (2), 139–166. https://doi.org/10.1177/030631277700700202 .
Spence, M. (1973). Job market signaling. The Quarterly Journal of Economics, 87 (3), 355–374. https://doi.org/10.1055/s-2004-820924 .
Sprankel, S. (2013). Technical Basis of Digital Currencies . www.coderblog.de/wp-content/uploads/technical-basis-of-digital-currencies.pdf
Stokes, R. (2012). Virtual money laundering: The case of Bitcoin and the Linden dollar. Information and Communications Technology Law, 21 (3), 221–236. https://doi.org/10.1080/13600834.2012.744225 .
Sun, J., Yan, J., & Zhang, K. Z. K. (2016). Blockchain-based sharing services: What blockchain technology can contribute to smart cities. Financial Innovation, 2 (1), 1–9. https://doi.org/10.1186/s40854-016-0040-y .
Swan, M. (2015). Blockchain: Blueprint for a new economy . O’Reilly Media, Inc.
Szabo, N. (1997). Formalizing and Securing Relationships on Public Networks. First Monday , 2 (9). doi:10.5210/fm.v2i9.548
Tan, B. S., & Low, K. Y. (2019). Blockchain as the database engine in the accounting system. Australian Accounting Review, 29 (2), 312–318. https://doi.org/10.1111/auar.12278 .
Tapscott, D., & Tapscott, A. (2018). Blockchain revolution: How the technology behind bitcoin and other cryptocurrencies is changing the world . Portfolio.
Taylor, P. J., Dargahi, T., Dehghantanha, A., Parizi, R. M., & Choo, K. K. R. (2019). A systematic literature review of blockchain cyber security. Digital Communications and Networks , (June 2018). doi:10.1016/j.dcan.2019.01.005
The Economist. (2015). The Trust Machine. www.economist.com/leaders/2015/10/31/the-trust-machine
Tiwari, A. K., Jana, R. K., Das, D., & Roubaud, D. (2018). Informational efficiency of Bitcoin – An extension. Economics Letters, 163 , 106–109. https://doi.org/10.1016/j.econlet.2017.12.006 .
Tranfield, D., Denyer, D., & Smart, P. (2003). Towards a methodology for developing evidence-informed management knowledge by means of systematic review. British Journal of Management, 14 (3), 207–222. https://doi.org/10.1111/1467-8551.00375 .
Tsai, W. T., Deng, E., Ding, X., & Li, J. (2018). Application of blockchain to trade clearing. In 2018 IEEE 18th International Conference on Software Quality, Reliability, and Security Companion (pp. 154–163). IEEE. doi:10.1109/QRS-C.2018.00039
Urquhart, A. (2016). The inefficiency of Bitcoin. Economics Letters, 148 , 80–82.
Urquhart, A. (2017). Price clustering in Bitcoin. Economics Letters, 159 , 145–148. https://doi.org/10.1016/j.econlet.2017.07.035 .
Urquhart, A. (2018). What causes the attention of Bitcoin? Economics Letters, 166 , 40–44. https://doi.org/10.1016/j.econlet.2018.02.017 .
Veuger, J. (2012). Trust in a viable real estate economy with disruption and blockchain. Facilities, 36 (1/2), 103–120.
Vishnumurthy, V., Chandrakumar, S., & Sirer, E. G. (2003). Karma: A secure economic framework for peer-to-peer resource sharing. Workshop on Economics of Peer-to-peer Systems , 35 (6).
Wei, W. C. (2018a). Liquidity and market efficiency in cryptocurrencies. Economics Letters, 168 , 21–24. https://doi.org/10.1016/j.econlet.2018.04.003 .
Wei, W. C. (2018b). The impact of Tether grants on Bitcoin. Economics Letters, 171 , 19–22. https://doi.org/10.1016/j.econlet.2018.07.001 .
White, L. H. (2015). The market for cryptocurrencies. Cato Journal, 35 (2), 383–402.
Wolfond, G. (2017). A Blockchain ecosystem for digital identity: improving service delivery in Canada’s public and private sectors. Technology Innovation Management Review, 7 (10), 35–40. https://doi.org/10.22215/timreview/1112 .
Wood, G. (2014). Ethereum: a secure decentralised generalised transaction ledger . https://ethereum.github.io/yellowpaper/paper.pdf
Wörfel, P. (2019). Unravelling the intellectual discourse of implicit consumer cognition : A bibliometric review. Journal of Retailing and Consumer Services , (September), 101960. https://doi.org/10.1016/j.jretconser.2019.101960
Wright, A., & De Filippi, P. (2015). Decentralized blockchain technology and the rise of lex cryptographia. . https://doi.org/10.2139/ssrn.2580664 .
Yang, D., & Li, M. (2018). Evolutionary approaches and the construction of technology-driven regulations. Emerging Markets Finance and Trade, 54 (14), 3256–3271. https://doi.org/10.1080/1540496X.2018.1496422 .
Yermack, D. (2015). Is Bitcoin a real currency? Handbook of Digital Currency . https://doi.org/10.2139/ssrn.2361599 .
Yli-Huumo, J., Ko, D., Choi, S., Park, S., & Smolander, K. (2016). Where is current research on Blockchain technology? – A systematic review. PLoS ONE, 11 (10), 1–27. https://doi.org/10.1371/journal.pone.0163477 .
Yonghong, J., He, N., & Weihua, R. (2018). Time-varying long-term memory in Bitcoin market. Finance Research Letters, 25 , 280–284. https://doi.org/10.1016/j.frl.2017.12.009 .
Yue, X., Wang, H., Jin, D., Li, M., & Jiang, W. (2016). Healthcare data gateways: found healthcare intelligence on blockchain with novel privacy risk control. Journal of Medical Systems, 40 (10), 218.
Zeng, S., Ni, X., Yuan, Y., & Wang, F. Y. (2018). A Bibliometric Analysis of Blockchain Research. In IEEE Intelligent Vehicles Symposium, Proceedings (pp. 102–107). IEEE. doi:10.1109/IVS.2018.8500606
Zupic, I., & Čater, T. (2015). Bibliometric methods in management and organization. Organizational Research Methods, 18 (3), 429–472. https://doi.org/10.1177/1094428114562629 .
Zuschke, N. (2019). An analysis of process-tracing research on consumer decision-making. Journal of Business Research, 1–16 , https://doi.org/10.1016/j.jbusres.2019.01.028 .
Zyskind, G., Nathan, O., & Pentland, A. S. (2015). Decentralizing privacy: Using blockchain to protect personal data. In Proceedings – 2015 IEEE Security and Privacy Workshops, SPW 2015 (pp. 180–184). doi:10.1109/SPW.2015.27
Download references
Acknowledgements
Open Access funding provided by Projekt DEAL.
Author information
Authors and affiliations.
Blockchain Research Lab, Colonnaden 72, Hamburg, 20354, Germany
Lennart Ante
Faculty of Business, Economics & Social Sciences, University of Hamburg, Von-Melle-Park 5, 20146, Hamburg, Germany
You can also search for this author in PubMed Google Scholar
Corresponding author
Correspondence to Lennart Ante .
Ethics declarations
Conflict of interest.
The authors declare that they have no conflict of interest.
Rights and permissions
Open Access This article is licensed under a Creative Commons Attribution 4.0 International License, which permits use, sharing, adaptation, distribution and reproduction in any medium or format, as long as you give appropriate credit to the original author(s) and the source, provide a link to the Creative Commons licence, and indicate if changes were made. The images or other third party material in this article are included in the article's Creative Commons licence, unless indicated otherwise in a credit line to the material. If material is not included in the article's Creative Commons licence and your intended use is not permitted by statutory regulation or exceeds the permitted use, you will need to obtain permission directly from the copyright holder. To view a copy of this licence, visit http://creativecommons.org/licenses/by/4.0/ .
Reprints and permissions
About this article
Ante, L. A place next to Satoshi: foundations of blockchain and cryptocurrency research in business and economics. Scientometrics 124 , 1305–1333 (2020). https://doi.org/10.1007/s11192-020-03492-8
Download citation
Received : 02 December 2019
Published : 15 May 2020
Issue Date : August 2020
DOI : https://doi.org/10.1007/s11192-020-03492-8
Share this article
Anyone you share the following link with will be able to read this content:
Sorry, a shareable link is not currently available for this article.
Provided by the Springer Nature SharedIt content-sharing initiative
- Distributed ledger
- Informetric analysis
- Bibliometric analysis
- Find a journal
- Publish with us
- Track your research
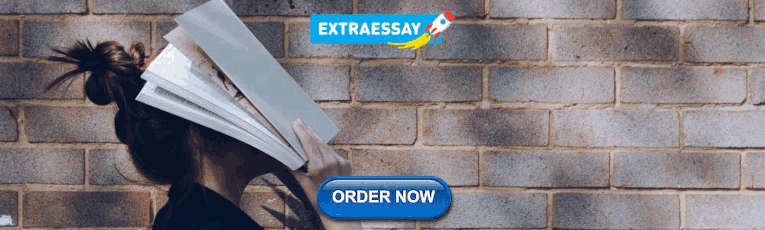
IMAGES
VIDEO
COMMENTS
This paper provides a snapshot of the current state of cryptocurrency research based on the papers presented at the Cryptocurrency Research Conference 2020 and submitted to this Special Issue, highlighting key themes, challenges, and opportunities for future investigation. 2. The cryptocurrency research conference
Research Significance and Objectives This research was intended to assess the sustainability of cryptocurrency as an asset and define if a shift to clean energy development will occur fast enough to keep up with cryptocurrency's exponential growth. I evaluated the potential evolution of
Abstract. Cryptocurrency, an encrypted, peer- to -peer network for facil itating digital barter, is a technology. developed eight years ago. Bitcoin, the first and most popular cryptocurrency, is ...
In this paper, we investigate the role of cryptocurrencies in modern finance. We apply a narrative literature review method to synthesize prior research and draw insights into the opportunities ...
This paper provides a comprehensive survey of cryptocurrency trading research, by covering 146 research papers on various aspects of cryptocurrency trading (e.g., cryptocurrency trading systems, bubble and extreme condition, prediction of volatility and return, crypto-assets portfolio construction and crypto-assets, technical trading and others).
The papers in this special issue focus on the emerging phenomenon of cryptocurrencies. Cryptocurrencies are digital financial assets, for which ownership and transfers of ownership are guaranteed by a cryptographic decentralized technology. The rise of cryptocurrencies' value on the market and the growing popularity around the world open a number of challenges and concerns for business and ...
Table 2 identifies the key characteristics of the fifty-two quantitative investigations of cryptocurrency markets. The research papers are sub-categorised in the same manner with data reported to include the dependent variable of the investigated methodology and indeed the type of methodology used, the rationale behind its use, the frequency and source the data used, the selected control ...
Only 2 included papers (4%) were available before 2014, with only 1 (2%) and 3 (6%) more being published in 2015 and 2016, respectively. Of the papers included in this literature review, 30% were released in 2018, 38% in 2019, and 4% in 2020. These statistics thus provide evidence of the strong development of cryptocurrency research in recent ...
The purpose of this paper is to identify research that has been carried out about cryptocurrency regulation contributions and the current challenges that need to be addressed in future studies. The methodology used to conduct this research and report the findings was systematic mapping. We use this methodology to search, identify, and select all relevant primary studies on cryptocurrency ...
The motivation of proposing and editing the Special Issue "Blockchain and cryptocurrencies" came from the inspirational invited and contributed talks at the 43rd annual A.M.A.S.E.S. conference held in Perugia in September 2019. All the papers have gone through the journal regular refereeing process under the same standards set by the journal, and nine contributions were finally accepted ...
Cryptocurrency, fundamentally a digital or virtual form of currency utilizing cryptography for security, made its debut in 2008 through a white paper published by an individual or group under the ...
According to Exton and Doidge (2018), the share of respondents owning cryptocurrencies ranges from 5% in Belgium to 12% in Romania and 18% in Turkey. Deutsche Postbank (2018) estimates the share of German citizens owning cryptocurrency at 3%, and at 4% among digital natives. Global Web Index (2018) conducted a worldwide survey among 111,899 ...
[Context] The goal of security is to protect digital assets, devices, and services from being disrupted, exploited or stolen by unauthorized users. It is also about having reliable information available at the right time. [Motivation] Since the inception in 2009 of the first cryptocurrency, few studies have been undertaken to analyze and review the state-of-the-art research and current ...
Abstract. Cryptocurrencies have taken center stage in the financial sector of the globe. A cryptocurrency is a form of digital and virtual currency that is secured and encrypted by blockchain and ...
This paper explores the state of the art in the cryptocurrency literature, with a special emphasis on the links between financial dimensions and ESG features. The study uses bibliometric analysis to illustrate the history of cryptocurrency publication activity, focusing on the most popular subjects and research trends. Between 2014 and 2021, 1442 papers on cryptocurrencies were published in ...
Bitcoin is the best cryptocurrency enhancing the characteristics of the optimal portfolio. Ripple and Litecoin follow in terms of their usefulness in an optimal portfolio as single cryptocurrencies. ... Research methodology. This paper aims to answer should, and if yes how, cryptocurrencies can be used to diversify an investment portfolio ...
The Cryptocurrency Special Topic Hub presents interdisciplinary scholarship covering all aspects of cryptocurrencies, from the technical platforms, solutions, and tools used, to user identity, authentication, and the encryption empowering it, to the regulatory policies it influences. It is important to note that many of these papers have not ...
Blockchain technology has become an ubiquitous phenomenon. While the topic originated in computer science, the business and economics literature was comparatively slow to pick up on it. To better understand the academic basis, current developments and future research avenues of the discourse, 9672 cited references of 467 blockchain and cryptocurrency articles from the fields of business and ...
Benu Chatterjee. Abstract: The research paper investigates the profound impact that cryptocurrencies have exerted on traditional financial systems since the emergence of Bitcoin in 2009. The rapid growth of cryptocurrency market and its increasing integration in global economics have raised significant questions about the future coexistence and ...
Asia, we establish 10 facts about crypto currency. exchanges and cryptocurrency buying and. selling. 1) Individuals keep cryptocurrency. Worakamol Wisets ri et al. 2696. portfolios of a sm all ...
Write a first draft: Begin by summarising the main objectives, methods, results, and conclusions of the study in your own words. Focus on capturing the essence of the research paper while omitting unnecessary details. Expand: Expand on your initial draft by providing more context, explanation, and interpretation of the key points.
The objective of all digital payment methods is to offer consumers an alternative mode of paying physical cash [1]. Cryptocurrency is a type of digital currency where transactions are verified and records are kept by a decentralized system employing encryption ... The authors received no financial support for the research, authorship and/or ...
The main objective of this paper is to evaluate whether the Indian users are ready to adopt cryptocurrency as future currency and to analyze the probability of legalization of cryptocurrency in India.