
- Research Guides
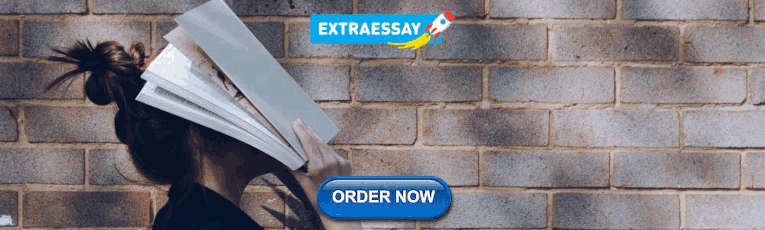
6 Stages of Research
- 1: Task Definition
- 2: Information Seeking
- 3: Location & Access
- 4: Use of Information
- 5: Synthesis
- 6: Evaluation
Ask a Librarian

Browse our FAQ, Chat Live with a Librarian, Call, Text, Email, or Make an Appointment.
We are here to help!
Ask the Right Questions
The scope of an investigation determines how large or small your investigation will be. Determining the scope of an investigation is the critical first step in the research process because you will know how far and how deep to look for answers. This lesson will teach you how to develop a research question as a way to determine the scope of an investigation.

Click the image to open the tutorial in a new window.
Keyword(s): 5W Criteria, Ask the Right Questions, Guided Inquiry, Information Literacy, Library, New Literacies Alliance, Research as Inquiry, Research Question
Purpose of this guide
The purpose of this guide is to walk you through the 6 stages of writing an effective research paper. By breaking the process down into these 6 stages, your paper will be better and you will get more out of the research experience.
The 6 stages are:
- Task Definition (developing a topic)
- Information Seeking (coming up with a research plan)
- Location & Access (finding good sources)
- Use of Information (Reading, taking notes, and generally making the writing process easier)
- Synthesis (coming up with your own ideas and presenting them well)
- Evaluation (reflection)
This research guide is based on the Big6 Information Literacy model from https://thebig6.org/
Task Definition
The purpose of task definition is to help you develop an effective topic for your paper. .
Developing a topic is often one of the hardest and most important steps in writing a paper or doing a research project. But here are some tips:
- A research topic is a question, not a statement. You shouldn't already know the answer when you start researching.
- Research something you actually care about or find interesting. It turns the research process from a chore into something enjoyable and whoever reads your work can tell the difference.
- Read the assignment before and after you think you have come up with your topic to make sure you are answering the prompt.
Steps to Developing a Topic
- Assignment Requirements
- General Idea
- Background Research
- Ask Questions
- Topic Question
Read your assignment and note any requirements.
- Is there a required page length?
- How many sources do you need?
- Does the paper have to be in a specific format like APA?
- Are there any listed goals for the topic, such as synthesizing different opinions, or applying a theory to a real-life example?
Formulate a general idea.
- Look at your syllabus or course schedule for broad topic ideas.
- Think about reading assignments or class lectures that you found interesting.
- Talk with your professor or a librarian.
- Check out social media and see what has been trending that is related to your course.
- Think about ideas from popular videos, TV shows, and movies.
- Read The New York Times (FHSU students have free access through the Library)
- Watch NBC Learn (FHSU students have free access through the Library)
- Search your library for relevant journals and publications related to your course and browse them for ideas
- Browse online discussion forums, news, and blogs for professional organizations for hot topics
Do some background research on your general idea.
- You have access to reference materials through the Library for background research.
- See what your course notes and textbook say about the subject.
- Google it.
Reference e-books on a wide range of topics. Sources include dictionaries, encyclopedias, key concepts, key thinkers, handbooks, atlases, and more. Search by keyword or browse titles by topic.
Over 1200 cross-searchable reference e-books on a wide variety of subjects.
Mind map it.
A mind map is an effective way of organizing your thoughts and generating new questions as you learn about your topic.
- Video on how to do a mind map.
- Coggle Free mind mapping software that is great for beginners and easy to use.
- MindMup Mindmup is a free, easy to use online software that allows you to publish and share your mind maps with others.
Ask Questions to focus on what interests you.
Who? What? When? Where? Why?
We can focus our ideas by brainstorming what interests us when asking who, what, when where, and why:

Research Question: Does flexible seating in an elementary classroom improve student focus?
Write out your topic question & reread the assignment criteria.
- Can you answer your question well in the number of pages required?
- Does your topic still meet the requirements of the paper? Ex: is the question still about the sociology of gender studies and women?
- Is the topic too narrow to find research?
Developing a Topic Tutorial
The following tutorial from Forsyth Library will walk you through the process of defining your topic.
- Next: 2: Information Seeking >>
- Last Updated: Mar 29, 2024 11:34 AM
- URL: https://fhsuguides.fhsu.edu/6stages
- Search Search Please fill out this field.
- What Is R&D?
- Understanding R&D
- Types of R&D
- Pros and Cons
- Considerations
- R&D vs. Applied Research
- Who Spends the Most?
The Bottom Line
- Business Essentials
Research and Development (R&D) Definition, Types, and Importance
:max_bytes(150000):strip_icc():format(webp)/wk_headshot_aug_2018_02__william_kenton-5bfc261446e0fb005118afc9.jpg)
Investopedia / Ellen Lindner
What Is Research and Development (R&D)?
The term research and development (R&D) is used to describe a series of activities that companies undertake to innovate and introduce new products and services. R&D is often the first stage in the development process. Companies require knowledge, talent, and investment in order to further their R&D needs and goals. The purpose of research and development is generally to take new products and services to market and add to the company's bottom line .
Key Takeaways
- Research and development represents the activities companies undertake to innovate and introduce new products and services or to improve their existing offerings.
- R&D allows a company to stay ahead of its competition by catering to new wants or needs in the market.
- Companies in different sectors and industries conduct R&D—pharmaceuticals, semiconductors, and technology companies generally spend the most.
- R&D is often a broad approach to exploratory advancement, while applied research is more geared towards researching a more narrow scope.
- The accounting for treatment for R&D costs can materially impact a company's income statement and balance sheet.
Understanding Research and Development (R&D)
The concept of research and development is widely linked to innovation both in the corporate and government sectors. R&D allows a company to stay ahead of its competition. Without an R&D program, a company may not survive on its own and may have to rely on other ways to innovate such as engaging in mergers and acquisitions (M&A) or partnerships. Through R&D, companies can design new products and improve their existing offerings.
R&D is distinct from most operational activities performed by a corporation. The research and/or development is typically not performed with the expectation of immediate profit. Instead, it is expected to contribute to the long-term profitability of a company. R&D may often allow companies to secure intellectual property, including patents , copyrights, and trademarks as discoveries are made and products created.
Companies that set up and employ departments dedicated entirely to R&D commit substantial capital to the effort. They must estimate the risk-adjusted return on their R&D expenditures, which inevitably involves risk of capital. That's because there is no immediate payoff, and the return on investment (ROI) is uncertain. As more money is invested in R&D, the level of capital risk increases. Other companies may choose to outsource their R&D for a variety of reasons including size and cost.
Companies across all sectors and industries undergo R&D activities. Corporations experience growth through these improvements and the development of new goods and services. Pharmaceuticals, semiconductors , and software/technology companies tend to spend the most on R&D. In Europe, R&D is known as research and technical or technological development.
Many small and mid-sized businesses may choose to outsource their R&D efforts because they don't have the right staff in-house to meet their needs.
Types of R&D
There are several different types of R&D that exist in the corporate world and within government. The type used depends entirely on the entity undertaking it and the results can differ.
Basic Research
There are business incubators and accelerators, where corporations invest in startups and provide funding assistance and guidance to entrepreneurs in the hope that innovations will result that they can use to their benefit.
M&As and partnerships are also forms of R&D as companies join forces to take advantage of other companies' institutional knowledge and talent.
Applied Research
One R&D model is a department staffed primarily by engineers who develop new products —a task that typically involves extensive research. There is no specific goal or application in mind with this model. Instead, the research is done for the sake of research.
Development Research
This model involves a department composed of industrial scientists or researchers, all of who are tasked with applied research in technical, scientific, or industrial fields. This model facilitates the development of future products or the improvement of current products and/or operating procedures.
$42.7 billion of research and development costs later, Amazon was granted 2,244 new patents in 2020. Their patents included advancements in artificial intelligence, machine learning, and cloud computing.
Advantages and Disadvantages of R&D
There are several key benefits to research and development. It facilitates innovation, allowing companies to improve existing products and services or by letting them develop new ones to bring to the market.
Because R&D also is a key component of innovation, it requires a greater degree of skill from employees who take part. This allows companies to expand their talent pool, which often comes with special skill sets.
The advantages go beyond corporations. Consumers stand to benefit from R&D because it gives them better, high-quality products and services as well as a wider range of options. Corporations can, therefore, rely on consumers to remain loyal to their brands. It also helps drive productivity and economic growth.
Disadvantages
One of the major drawbacks to R&D is the cost. First, there is the financial expense as it requires a significant investment of cash upfront. This can include setting up a separate R&D department, hiring talent, and product and service testing, among others.
Innovation doesn't happen overnight so there is also a time factor to consider. This means that it takes a lot of time to bring products and services to market from conception to production to delivery.
Because it does take time to go from concept to product, companies stand the risk of being at the mercy of changing market trends . So what they thought may be a great seller at one time may reach the market too late and not fly off the shelves once it's ready.
Facilitates innovation
Improved or new products and services
Expands knowledge and talent pool
Increased consumer choice and brand loyalty
Economic driver
Financial investment
Shifting market trends
R&D Accounting
R&D may be beneficial to a company's bottom line, but it is considered an expense . After all, companies spend substantial amounts on research and trying to develop new products and services. As such, these expenses are often reported for accounting purposes on the income statement and do not carry long-term value.
There are certain situations where R&D costs are capitalized and reported on the balance sheet. Some examples include but are not limited to:
- Materials, fixed assets, or other assets have alternative future uses with an estimable value and useful life.
- Software that can be converted or applied elsewhere in the company to have a useful life beyond a specific single R&D project.
- Indirect costs or overhead expenses allocated between projects.
- R&D purchased from a third party that is accompanied by intangible value. That intangible asset may be recorded as a separate balance sheet asset.
R&D Considerations
Before taking on the task of research and development, it's important for companies and governments to consider some of the key factors associated with it. Some of the most notable considerations are:
- Objectives and Outcome: One of the most important factors to consider is the intended goals of the R&D project. Is it to innovate and fill a need for certain products that aren't being sold? Or is it to make improvements on existing ones? Whatever the reason, it's always important to note that there should be some flexibility as things can change over time.
- Timing: R&D requires a lot of time. This involves reviewing the market to see where there may be a lack of certain products and services or finding ways to improve on those that are already on the shelves.
- Cost: R&D costs a great deal of money, especially when it comes to the upfront costs. And there may be higher costs associated with the conception and production of new products rather than updating existing ones.
- Risks: As with any venture, R&D does come with risks. R&D doesn't come with any guarantees, no matter the time and money that goes into it. This means that companies and governments may sacrifice their ROI if the end product isn't successful.
Research and Development vs. Applied Research
Basic research is aimed at a fuller, more complete understanding of the fundamental aspects of a concept or phenomenon. This understanding is generally the first step in R&D. These activities provide a basis of information without directed applications toward products, policies, or operational processes .
Applied research entails the activities used to gain knowledge with a specific goal in mind. The activities may be to determine and develop new products, policies, or operational processes. While basic research is time-consuming, applied research is painstaking and more costly because of its detailed and complex nature.
Who Spends the Most on R&D?
Companies spend billions of dollars on R&D to produce the newest, most sought-after products. According to public company filings, these companies incurred the highest research and development spending in 2020:
- Amazon: $42.7 billion
- Alphabet.: $27.6 billion
- Huawei: $22.0 billion
- Microsoft: $19.3 billion
- Apple: $18.8 billion
- Samsung: $18.8 billion
- Facebook: $18.5 billion
What Types of Activities Can Be Found in Research and Development?
Research and development activities focus on the innovation of new products or services in a company. Among the primary purposes of R&D activities is for a company to remain competitive as it produces products that advance and elevate its current product line. Since R&D typically operates on a longer-term horizon, its activities are not anticipated to generate immediate returns. However, in time, R&D projects may lead to patents, trademarks, or breakthrough discoveries with lasting benefits to the company.
What Is an Example of Research and Development?
Alphabet allocated over $16 billion annually to R&D in 2018. Under its R&D arm X, the moonshot factory, it has developed Waymo self-driving cars. Meanwhile, Amazon has spent even more on R&D projects, with key developments in cloud computing and its cashier-less store Amazon Go. At the same time, R&D can take the approach of a merger & acquisition, where a company will leverage the talent and intel of another company to create a competitive edge. The same can be said with company investment in accelerators and incubators, whose developments it could later leverage.
Why Is Research and Development Important?
Given the rapid rate of technological advancement, R&D is important for companies to stay competitive. Specifically, R&D allows companies to create products that are difficult for their competitors to replicate. Meanwhile, R&D efforts can lead to improved productivity that helps increase margins, further creating an edge in outpacing competitors. From a broader perspective, R&D can allow a company to stay ahead of the curve, anticipating customer demands or trends.
There are many things companies can do in order to advance in their industries and the overall market. Research and development is just one way they can set themselves apart from their competition. It opens up the potential for innovation and increasing sales. But it does come with some drawbacks—the most obvious being the financial cost and the time it takes to innovate.
NASDAQ. " Which Companies Spend the Most in Research and Development (R&D)? "
Strategy+Business. " WHAT THE TOP INNOVATORS GET RIGHT ."
:max_bytes(150000):strip_icc():format(webp)/research_pharma-5bfc322b46e0fb0051bf11a0.jpg)
- Terms of Service
- Editorial Policy
- Privacy Policy
- Your Privacy Choices
Ask a question
Start a discussion.
- Jira Jira Software
- Jira Service Desk Jira Service Management
- Jira Work Management
- Confluence Confluence
- Trello Trello
Community resources
- Announcements
- Technical support
- Documentation
Atlassian Community Events
- Atlassian University
- groups-icon Welcome Center
- groups-icon Featured Groups
- groups-icon Product Groups
- groups-icon Regional Groups
- groups-icon Industry Groups
- groups-icon Community Groups
- Learning Paths
- Certifications
- Courses by Product
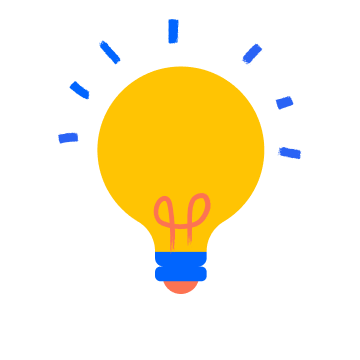
Get product advice from experts
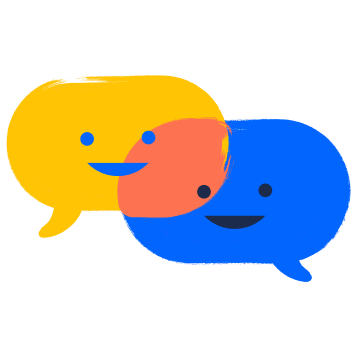
Join a community group
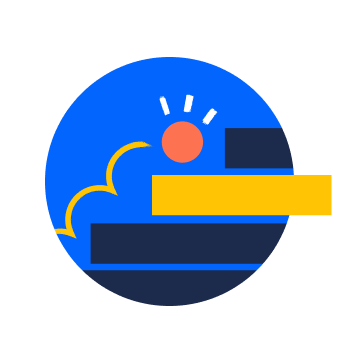
Advance your career with learning paths
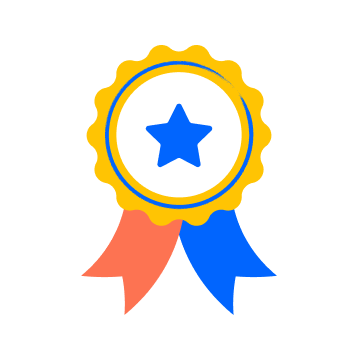
Earn badges and rewards
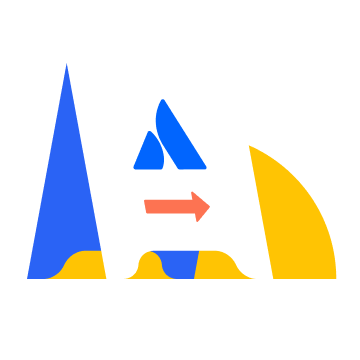
Connect and share ideas at events
- Featured Groups
- App Central
What is the Research and Development (R&D) Process?
Was this helpful?
Iryna Komarnitska_SaaSJet_
About this author
Product Marketer
7 accepted answers
226 total posts
- +31 more...
- atlassian-marketplace
- Community Guidelines
- Privacy policy
- Notice at Collection
- Terms of use
- © 2024 Atlassian
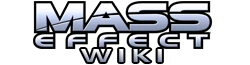
Task: Research Center Development
- Edit source
- View history
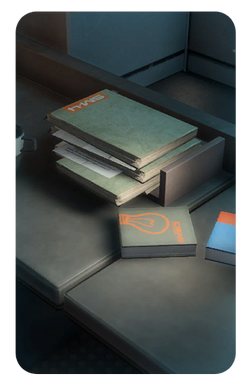
You have reactivated a Research Center on Eos . Test its capabilities by gathering the resources to craft a weapon.
- 1 Acquisition
- 2.1 Collect area resources and craft a weapon
Acquisition [ ]

Walkthrough [ ]
Collect area resources and craft a weapon [ ].
The earliest you can complete this task is just after acquisition provided you have accumulated enough resources from previous missions (if you have been rigorously sending out APEX teams after completing First Strike , even better).
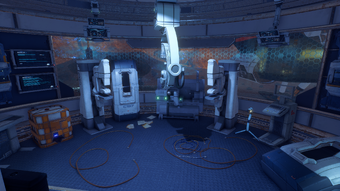
If not, there are many resources spread around Site 1: Promise, which you can collect to be able to build the weapon. The building itself has a couple of lootable crates just beside the research center to get you started. When you've gathered enough resources, simply use the research center and craft almost any weapon in the Development menu.
Note: It's not actually required to use the Eos research center to craft the weapon. Doing it on the Tempest or anywhere else suitable will also complete the task.
Rewards [ ]
- Mass Effect
- 3 Romance (Mass Effect: Andromeda)
Log in using your username and password
- Search More Search for this keyword Advanced search
- Latest content
- Current issue
- For authors
- New editors
- BMJ Journals More You are viewing from: Google Indexer
You are here
- Volume 34, Issue 1
- Stages in the development of a research project: putting the idea together
- Article Text
- Article info
- Citation Tools
- Rapid Responses
- Article metrics

- Roger G Eston ,
- Ann V Rowlands
- School of Sport, Health and Exercise Sciences, University of Wales, Bangor LL57 2EN, Wales, United Kingdom
- Correspondence to: Dr R G Eston.
https://doi.org/10.1136/bjsm.34.1.59
Statistics from Altmetric.com
Request permissions.
If you wish to reuse any or all of this article please use the link below which will take you to the Copyright Clearance Center’s RightsLink service. You will be able to get a quick price and instant permission to reuse the content in many different ways.
The purpose of this paper is to provide an overview of the process and stages involved in developing a research idea from its inception to realisation. It is not designed to be an all encompassing summary of the research process. It fprovides a brief guide to the most common sequence of stages involved in the development of a research idea into a viable research proposal. Useful references for further reading on important issues that are beyond the scope of this article are also provided.
Familiarity with the topic
Reviewing the literature.
Most literature searches begin with one of the many electronic databases available, using the author's name or a combination of carefully selected keywords. Individual databases are limited as to which journals are listed. Within sports and exercise science, Sports Discus and Medline are widely used. To avoid missing pertinent articles, it is suggested that a number of electronic databases are searched. Medline covers biomedically orientated journals, Sports Discus covers sport and exercise orientated sources, and the Science Citation Index covers scientific articles in general. In addition, the National Sports Medicine Institute has recently released the Sports Medicine and Related Topics (SMART) database of journal articles, which covers all aspects of sports and exercise science and medicine from 1985. Addresses for these databases on the internet are: for Medline, http://www.ncbi.nlm.nih.gov/PubMed/medline.html; for the Science Citation Index (United Kingdom higher education institutions only), http://www.bids.ac.uk/; for SMART, http://smart.nsmi.org.uk .
Most universities offering courses in sports and exercise science courses have access to Sports Discus available on a centrally accessed CD-ROM. Further relevant papers may be obtained by studying their reference lists. Papers that provide a critical review of the topic are also very useful. Many journals such as Sports Medicine specialise in reviews, although these can also be found in other mainstream journals such as the British Journal of Sports Medicine, Medicine and Science in Sports and Exercise , and Journal of Sports Sciences . If the library does not hold a particular journal, it should be possible to obtain issues through the university's interlibrary loan service, although this is expensive and may limit the number of papers obtained.
When writing a review paper or conducting a more sophisticated empirically based review, such as a meta-analysis, it is important to endeavour to obtain all papers asking a given research question, 2 whether they are published or not. Although this may not be practically possible, the researcher should be careful not to select only those papers that are easiest to obtain as this may bias the review. Hence, further steps should be taken to obtain more elusive papers and/or obtain a random selection of relevant papers. Rosenthal 3 has written a comprehensive paper on bibliographic retrieval for researchers wishing to conduct a meta-analysis.
The research hypothesis and rationale
The typical empirical journal article and research proposal commences with a brief literature review to provide the background and rationale for the research. Questions that remain unanswered, or findings that need clarifying, are often highlighted here. This leads into explicit statements about the importance and necessity of the planned research.
Once the research problem has been stated, the hypothesis is normally presented. 4 However, often, hypotheses are not stated in research papers, primarily because of authors' assumptions that the reader can implicitly determine the hypotheses being tested from the description of the purpose or statement of the problem, which is most commonly stated at the end of the introduction. 1
The expected results form the research hypothesis. For example, it may be hypothesised that the mean cholesterol levels of trained men are lower than those of sedentary men. This is a research hypothesis, as it states what the results are expected to be. Conversely, the null hypothesis often states what the researcher does not expect to be the case. Its purpose is for use in the statistical test of reliability of results. It usually, although not always, 1 states that there are no differences between treatments, or that there is no relation between variables. 4 For example, the null hypothesis for the above study may state that there is no difference between the mean cholesterol levels of trained and sedentary men. If the null hypothesis were true, any observed differences would be due to chance alone, and the statistically non-significant differences that existed in the sample would not be inferred to exist in the population. Huck and Cormier 1 (chapters 7 and 8) give a detailed description of the different forms of hypotheses and the stages of hypothesis testing.
The design of the study
A study should be designed to answer the research question being asked. A thorough evaluation of the literature can help the researcher avoid repeating design mistakes that have been made in the past. Theoretically, research studies should become better and better with time as past mistakes are rectified and studies become more and more robust. However, this is generally not the case as in reality each study is a new and novel endeavour. 5
TYPES OF STUDY
As in medical research, broadly speaking the types of study used in sports science research can be split into two groups: observational and experimental. 5
Observational design
In observational studies, the participants receive no treatment or experimental manipulation. As the name suggests, the variables of interest are recorded from the participant with no attempt to influence the variables in any way. This is a descriptive study. In observational studies, the researcher analyses the data with the aim of determining differences or relations between variables and reasons why they do or do not exist. In experimental studies, the effect of treatment or manipulation of the independent variable is examined. 5 Examples of observational studies include those that have recorded skinfold levels and other estimates of body fat, 6– 9 or the measurement of body fat and physical activity in children to assess whether there is a relation between the two. 10, 11 In the latter examples, if the participants had received an aerobic training programme to assess the effects on body fat, the independent variable in the study would have been directly manipulated and the study would be experimental in nature. 12
Experimental design
If the study has an experimental design—that is, one of the independent variables is to be manipulated, it is important to be sure that any observed changes in the dependent variable—for example, power output—are due to the experimental treatment—for example, creatine ingestion—and not due to chance, growth, learning, or other extraneous factors. For example, in a study to investigate the effects of creatine supplementation on maximal anaerobic capacity—for example, that of Worth et al 13 —a control group was necessary to separate the treatment effect from any other causes that may have improved performance. A placebo group was also included in the above study to determine whether any improvement in performance was due to the creatine supplementation or to a psychological effect. 4
There are situations in which the inclusion of a placebo is not possible. For example, in studies in which the treatment is obvious to the participant (and the investigator). An example of this is a study to examine the effects of cryotherapy on exercise induced muscle damage and the soreness that accompanies it—for example, the study of Eston and Peters. 14 In this study, the control group had no treatment for the symptoms of delayed onset muscle soreness, while the treatment group received cryotherapy by immersing the damaged arm in cool water for a limited period of time on several occasions after the eccentric exercise bout. It was not possible to have a placebo group in this study because it would be very clear to the participants what treatment they were receiving.
Repeated measures and independent groups design
Experimental studies can be conducted using separate groups for treatment, control, and placebo conditions (independent groups design) or by using the same group for all conditions (repeated measures design). The option chosen depends on the design of the experiment. There are advantages and disadvantages to each method.
REPEATED MEASURES DESIGN
In a repeated measures design, the same group is tested under all conditions. The experiment is more powerful, as the within group variability due to individual differences is removed 15 and thus the number of participants (n) in each condition can be smaller than if separate groups are required for each condition. However, the commitment required from each participant is greater. In addition, there may need to be a large gap between conditions because there may be long lasting effects that may remain during the subsequent condition. For example, if the treatment is a drug, it may remain in the participant's system after the drug course has finished. It is important that the drug is completely flushed out from the system or it may affect the results from the control or placebo condition. An example of this type of study is that of Head et al . 16 In this study, all participants received two types of β-blockers and a placebo for five days in a double blind randomised cross over design. A minimum of two days was allowed for wash out.
For many studies a repeated measures design is the best tool for tackling the research question. In a study of this nature, in which the same group of participants are exposed to several conditions, it is essential that the order in which they are exposed to the conditions is randomised. 4 This helps control for any learning effect or acclimatisation related to the testing procedure. For example, when the effects of practice in using ratings of perceived exertion (RPE) to regulate exercise intensity were assessed, healthy 17 and blind participants 18 performed bouts of exercise at randomised RPEs.
INDEPENDENT GROUPS DESIGN
If two or more independent groups are used in a study, the groups should be similar except for the factor that is being investigated. For example, if the treatment group is comprised of young men, the control group should also consist of young men, not older men or young women. Ideally the participants should only differ with respect to the variable of interest. The method of allocating participants to groups must not be affected by the characteristics of the participants, therefore each participant should have an equal chance of being in any group. Bland 5 (chapter 2) describes various methods of randomly allocating participants to groups. There are numerous examples of random assignment of participants to independent groups—for example, Doyle and Parfitt 19 and Ehrlich and Haber. 20
The equivalence of the groups with respect to various measures can be checked before treatment by simple independent groups t tests or, in the case of more than two groups, by a one way analysis of variance. Provided that there is sufficient power to detect differences that are meaningful, these tests can provide an assurance of the equivalence of the groups. Alternatively, but less commonly, the investigators may adjust the scores after treatment on the basis of differences in the groups' scores before the test by using analysis of covariance procedures—for example, the study of Eston et al . 21 In this study, scores on muscle strength after treatment were adjusted for each group using the score obtained before the test as the covariate. 22 This reduced the possibility of the scores obtained after treatment being influenced by initial group differences.
When independent groups are used, the commitment required from the participant is less. Normally, he/she will experience the procedure only once. The time taken is therefore less, as all groups may be studied simultaneously. However, the design is less powerful, as the within group variability is greater because of individual differences between groups. 15 This implies that more participants per group are necessary (in comparison with a repeated measures design) for the design to have sufficient power.
MIXED MODEL DESIGN
Perhaps the most commonly used experimental design in sports and exercise science research is the mixed model analysis of variance. This contains at least one repeated measures factor and one independent groups factor. A typical example of this would be an experimental study that compares effects before and after treatment. For example, the effects of aerobics training on peak oxygen uptake and submaximal heart rate measures in girls, 23 or the effects of a prophylactic anti-inflammatory drug on muscle soreness after strenuous eccentric exercise—for example, the study of Semark et al . 24 In both of these studies, the participants were randomly assigned to an experimental group and a control group. In the latter study, the control group received a placebo. There are many other examples of the mixed model type of study.
Blind/double blind studies
In a single blind study, participants do not know whether they are receiving the placebo or the experimental treatment. A double blind study is when the tester also does not know what treatment the participant is receiving. This strengthens the design as it also reduces the tester's potential influence on the participants' results. Hence, neither the participant's nor the tester's expectations of the effects of the treatment should affect the outcome of the study. This is obviously important in studies to determine the effects of orally administered substances on performance, such as in the study by Head et al , 16 which assessed the effects of two different types of β-blocker on exercise metabolism, or in studies to assess the effects of oral creatine supplementation on anaerobic capacity. 13
Power of the study
There is increasing criticism about the lack of statistical power of papers published in sports and exercise science and psychology journals. 25 – 27 Statistical power refers to the probability of rejecting the null hypothesis—that is, the probability that the study will lead to significant results. 26 If the null hypothesis is false but not rejected, a type 2 error is incurred. Cohen 26 suggested that a power of 0.80 is adequate when an alpha is set at 0.05—that is, the risk of type 1 error, which is rejection of the null hypothesis when it is true, is 0.05. This means that the risk of a type 2 error is 0.20.
An important consideration in relation to the statistical power of the study is the magnitude of the relation or treatment effect. This is known as the effect size. When calculated a priori, this quantifies the degree to which the investigator believes the null hypothesis to be false. 26 Each statistical test has an effect size index, which ranges from zero upwards and is scale free. 26 For example, the effect size index for a correlation is simply r ; no conversion is necessary. For assessment of the difference between two sample means, Cohen's d , Hedges g , or Glass's Δ can be used. These divide the difference between two means by a standard deviation (see Rosenthal, 28 p 35). Formulae are available for converting other test statistics—for example, t test, one way analysis of variance, and χ 2 results—into effect size indexes (see Rosenthal, 28 p 19).
To evaluate an effect size, some idea of its scale is needed. 26 Effect sizes are often described as small, medium, and large. Correlations ( r ) equalling 0.1, 0.3, and 0.5 and Cohen's d equalling 0.2, 0.5, and 0.8 equate to small, medium, and large effect sizes respectively. A table detailing the magnitude of other effect size indexes equal to small, medium, and large effect sizes is presented in Cohen. 26 The smaller the expected effect size, the larger the sample size necessary if the study is to have sufficient power to detect that effect size.
An example of a study in which the effect size may be medium, could be one to assess the effects of habitual physical activity on body fat in children—for example, that of Rowlands et al . 10 In this study, there was a moderate correlation between habitual physical activity and body fat, corresponding to a medium effect size. A large effect size may be expected in a study to assess the effects of a very low energy diet on body fat in overweight women; an example is the study of Eston et al . 29 In this study, a greatly reduced energy intake (daily intake 1695 kJ a day for six weeks) resulted in a substantial decrease in total body mass and percentage body fat.
The effect size should be estimated during the design stage of a study. This allows the determination of the sample size required to give adequate power for a given alpha. Hence, the study can be designed to ensure it has sufficient power to detect the effect of interest—that is, minimising type 2 error. A simple table detailing sample sizes necessary to detect small, medium, and large effect sizes, with a power of 0.80 and an alpha of 0.05, is presented in Cohen. 26 This table covers eight statistical tests including the difference between independent means, product-moment correlation, χ 2 , and one way analysis of variance. More detailed descriptions of power analysis and methods for determining the sample size necessary in more complex tests can be found in the texts by Cohen 30 and Stevens. 15 Power calculations can also be carried out on interactive sites on the internet—for example, http://members.aol.com/johnp71/javastat.html#Power .
When empirical data are available, this can sometimes be used to estimate the effect size for a study. However, for some research questions it is difficult to find enough information to estimate the expected effect size. Here, the expected effect size may be difficult to calculate because of the limited number of studies that provide empirical information on the topic, or there may be insufficient detail provided in the results of the relevant studies. To enable comparison of effect sizes from studies that differ in sample size, it is recommended that, in addition to reporting the test statistic and p value, the appropriate effect size index is also reported.
A review of 108 articles published in the Australian Journal of Science and Medicine in Sport (AJMS; now The Journal of Science and Medicine in Sport ) in 1996 or 1997 showed that the median power to detect small, medium, and large effect sizes was 0.10, 0.46, and 0.84 respectively. 27 No study had adequate power to detect a small effect size, 38% had adequate power to detect a medium effect size, and 75% had adequate power to detect a large effect size. It is clear that, as recently as two to three years ago, the power of studies was often not being considered at the design stage of a study, if at all.
Ethical considerations
A further consideration in the design of a study involves the ethics of the testing procedures. Some journals will not accept papers unless the study has had ethics approval from a recognised ethics committee. The ethical implications of the study are dependent on the procedures to be undertaken and the nature of the participants. For example, the British Association of Sport and Exercise Sciences (BASES) recommend that ethical clearance should be obtained before imposing unusual or severe psychological or physiological stress, administering any ergogenic aid, working with clients with disabilities, or using biopsy or venepuncture techniques. 31 The above list is not complete, and where there is any doubt cases should be looked at individually. Certain procedures that may be approved for adult participants may not be approved for children. Children are recognised as a vulnerable group with a limited comprehension capacity. 32 Consequently, they are unable legally to give consent. However, it is generally accepted that parents/guardians can give parental permission, and children who are old enough can choose whether or not to participate. Rowland 32 (chapter 5) presents a thorough discussion on the ethical aspects of research with children.
Whether the participants are children or not, the relevance of each of the measures or treatments should be considered during the design stage of the research. There should be a clear and justifiable rationale for the necessity of invasive procedures, particularly if there are valid alternative and non-invasive measures available. The frequency of the invasive procedures and the effect this has on the participants should be considered.
A further ethical consideration involves the denial of potentially beneficial treatment. For example, in an experimental design there may be one group of participants who receive the treatment, one group who receive the placebo, and one group who receive the control treatment. Those who receive the placebo will, by definition, think they are receiving the treatment. In this case, both the placebo and control group have been denied the treatment. It is important to consider the ethics of denying these groups the treatment, particularly if the treatment is expected to be beneficial. This does not arise in a repeated measures design as all participants are exposed to all treatments.
A possible solution is to offer all groups the treatment after the study. This would not be possible with some studies—for example, when the purpose of the treatment is to reduce the symptoms of delayed onset muscle soreness, because the symptoms would have dissipated by the end of the study. However, if the effects of an ergogenic aid were studied, the participants may volunteer because they believe they will have the opportunity to benefit from the ergogenic aid. In this situation it would be possible to offer the aid to all participants after the study. Ethically, this may be preferable to withholding the treatment from two thirds of the volunteers. It may also prevent participants from withdrawing from a study which they consider is providing little or no benefit to them.
This issue becomes increasingly important if the treatment is for a medical condition or for rehabilitation. This is a common scenario in clinical trials. It has been argued that withholding a potentially beneficial treatment from patients is ethically justified, as any biologically active treatment is also potentially harmful. Hence, the benefits need to be conclusively demonstrated in properly controlled trials before general administration. 5 The ethics of withholding treatment clearly depend on the type of treatment and participants involved.
Before a study is embarked on, it is necessary to ensure that the study is viable. This involves making a realistic assessment of the costs, time, and availability of the participants. If there is an application for funding, these details have to be approved by the host institution and the funding body. Costs will be related to the sample size, duration of the study, equipment needed, consumables, research assistants or other staff, travel, conference presentations, and institutional overheads.
tk;3The facilities available for conducting the research also need to be considered. If the study is laboratory based, it may be necessary to book laboratory time relatively early, as many people typically share laboratory facilities. This can only be achieved if a reasonably accurate estimate of the laboratory time needed to conduct the research is known. A pilot study can help answer these questions, identify problems, and prevent or limit methodological faults in the main study. Piloting procedures are an essential part of preparing a study. 4
Most studies within sports science and sports medicine require human participants. It is important to consider how these participants will be obtained and how representative of the relevant population they are. It may be necessary to advertise or send letters to request participation. If this is so, consideration should be given as to where to advertise, or where to obtain addresses of potential participants. For example, an advertisement in a leisure centre is likely to attract a different type of participant from a similar advertisement in a doctors surgery or outpatients clinic. The methods of obtaining participants will be largely determined by the population the sample is supposed to represent. For some studies, it is acceptable to use the most convenient sample of sports and exercise science undergraduates, but this is not appropriate for all proposed research.
Expected outcomes
During the planning stages of the study, the potential benefits should be considered. The expected outcomes are strongly linked with the literature review, hypothesis, and rationale. A useful exercise is to plot a graph of the expected outcomes for each group. This also helps to identify the most appropriate statistical analysis of the prospective data. An assessment of the expected outcomes and the potential value of these outcomes will help show whether or not the study is worth while.
We have considered some of the most important factors involved in designing a viable study that will adequately address the research question. Although we do not profess to be experts in all aspects of the above, we have learned through experience that attention to many of the above points will help to avoid frustration during the experimental process and when the study is presented for external review and subsequent presentation and publication. Good luck in your research.
- ↵ Huck SW, Cormier WH. Reading statistics and research . New York: Harper Collins College Publishers, 1996.
- ↵ Rosenthal R. Writing meta-analytic reviews. Psychol Bull 1995 ; 118 : 183 –92. OpenUrl CrossRef Web of Science
- ↵ Rosenthal MC. Bibliographic retrieval for the social and behavioural scientist. Research in Higher Education 1985 ; 22 : 315 –33.
- ↵ Thomas JR, Nelson JK. Research methods in physical activity . Champaign, IL: Human Kinetics, 1990.
- ↵ Bland M. An introduction to medical statistics , 2nd ed. Oxford: Oxford Medical Publications, Oxford University Press, 1996.
- ↵ Durnin JVGA, Womersley J. Body fat assessed from total body density and its estimation from skinfold thickness: measurements on 481 men and women aged from 16 to 72 years. Br J Nutr 1974 ; 32 : 77 –97. OpenUrl CrossRef PubMed Web of Science
- Jackson AS, Pollock ML. Generalized equations for predicting body density of men. Br J Nutr 1978 ; 40 : 497 –504. OpenUrl CrossRef PubMed Web of Science
- Eston, RG, Fu F. Fung L. Validity of conventional anthropometric techniques for estimating body composition in Chinese adults. Br J Sports Med 1995 ; 29 : 52 –6. OpenUrl Abstract / FREE Full Text
- ↵ Eston RG, Evans R, Fu F. Estimation of body composition in Chinese and British males by ultrasonic assessment of segmental adipose tissue volume. Br J Sports Med 1994 ; 28 : 9 –13. OpenUrl Abstract / FREE Full Text
- ↵ Rowlands AV, Eston RG, Ingledew DK. The relationship between activity levels, body fat and aerobic fitness in 8–10 year old children. J Appl Physiol 1999 ; 86 : 1428 –35. OpenUrl Abstract / FREE Full Text
- ↵ Taylor W, Baranowski T. Physical activity, cardiovascular fitness and adiposity in children. Res Q Exerc Sport 1991 ; 62 : 157 –63. OpenUrl PubMed Web of Science
- ↵ Epstein LH, Wing RR, Koeske R, et al . Comparison of lifestyle change and programmed aerobic exercise on weight and fitness changes in obese children. Behavioural Therapy 1982 ; 13 : 651 –65. OpenUrl CrossRef Web of Science
- ↵ Worth SJ, Eston RG, Lemmey AB. Effects of oral creatine supplementation on anaerobic capacity in young trained men and women. J Sports Sci 1999 ; 17 : 565 –6P. OpenUrl
- ↵ Eston RG, Peters D. Effects of cold water immersion on the symptoms of exercise-induced muscle damage. J Sports Sci 1999 ; 17 : 231 –8. OpenUrl CrossRef PubMed Web of Science
- ↵ Stevens J. Applied multivariate statistics for the social sciences , 3rd ed. Mahwah, NJ: Lawrence Erlbaum Associates, 1996.
- ↵ Head A, Maxwell S, Kendall MJ. Exercise metabolism in healthy volunteers taking celipropol, atenelol and placebo. Br J Sports Med 1997 ; 31 : 120 – 5. OpenUrl Abstract / FREE Full Text
- ↵ Eston RG, Williams JG. Reliability of ratings of perceived effort for regulation of exercise intensity. Br J Sports Med 1988 ; 22 : 153 –5. OpenUrl Abstract / FREE Full Text
- ↵ Buckley JP, Eston RG, Sims JW, et al . Reliability of regulating exercise intensity using a braille ratings of perceived exertion scale with blind subjects. Med Sci Sports Exerc 1999 ; 31 : S113 . OpenUrl
- ↵ Doyle J, Parfitt G. The effect of induced mood states on performance profile areas of perceived need. J Sports Sci 1999 ; 17 : 115 –27. OpenUrl PubMed Web of Science
- ↵ Erhlich D, Haber P. Influence of acupuncture on physical performance capacity and haemodynamic parameters. Int J Sports Med 1992 ; 13 : 486 –91. OpenUrl CrossRef PubMed Web of Science
- ↵ Eston RG, Finney S, Baltzopoulos V, et al . Muscle soreness and strength loss changes after downhill running following a prior bout of isokinetic eccentric exercise. J Sports Sci 1996 ; 14 : 291 –9. OpenUrl CrossRef PubMed
- ↵ Vincent WJ. Statistics in kinesiology . Champaign, IL: Human Kinetics, 1995:189.
- ↵ Welsman JR, Armstrong N, Withers S. Responses of young girls to two modes of aerobic training. Br J Sports Med 1997 ; 31 : 139 –42. OpenUrl Abstract / FREE Full Text
- ↵ Semark A, Noakes TD, St Clair Gibson A, et al . The effect of a prophylactic dose of flurbiprofen on muscle soreness and sprinting performance in trained subjects. J Sports Sci 1999 ; 17 : 197 –203. OpenUrl CrossRef PubMed Web of Science
- ↵ Christensen JE, Christensen CE. Statistical power analysis of health, physical education and recreation research. Res Q 1977 ; 48 : 204 –8. OpenUrl PubMed Web of Science
- ↵ Cohen J. A power primer. Psychol Bull 1992 ; 112 : 155 –9. OpenUrl CrossRef PubMed Web of Science
- ↵ Speed HD, Anderson MB. Power, effect size and the misinterpretation of statistical inference in exercise science. 1998 Australian Conference of Science and Medicine in Sport . Bruce, Australia: Sports Medicine Australia, 1998:215P.
- ↵ Rosenthal R. Meta-analytic procedures for social research (revised edition). Newbury Park, CA: Sage, 1991.
- ↵ Eston RG, Shephard S, Kreitzman S, et al . Effect of very low calorie diet on body composition and exercise response in sedentary women. Eur J Appl Physiol 1992 ; 65 : 452 –8. OpenUrl
- ↵ Cohen J. Statistical power analysis for the behavioural sciences , 2nd ed. Mahwah, NJ: Hillsdale, Lawrence Erlbaum Associates, 1988.
- ↵ BASES, British Association of Sport and Exercise Sciences. Code of conduct . Headingley, Leeds: BASES, 1995.
- ↵ Rowland TW. Developmental exercise physiology . Champaign, IL: Human Kinetics, 1996.
Read the full text or download the PDF:
- Research and Development (R&D) | Overview & Process
Featured in:
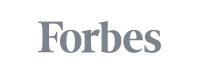
Companies often spend resources on certain investigative undertakings in an effort to make discoveries that can help develop new products or way of doing things or work towards enhancing pre-existing products or processes. These activities come under the Research and Development (R&D) umbrella.
R&D is an important means for achieving future growth and maintaining a relevant product in the market . There is a misconception that R&D is the domain of high tech technology firms or the big pharmaceutical companies. In fact, most established consumer goods companies dedicate a significant part of their resources towards developing new versions of products or improving existing designs . However, where most other firms may only spend less than 5 percent of their revenue on research, industries such as pharmaceutical, software or high technology products need to spend significantly given the nature of their products.
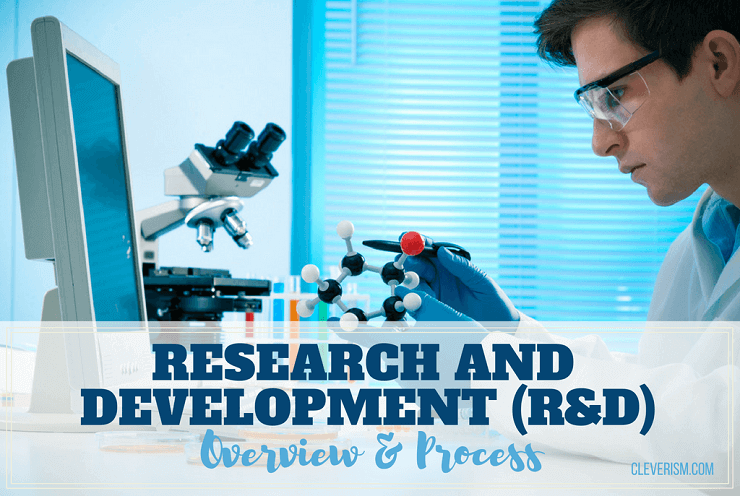
© Shutterstock.com | Alexander Raths
In this article, we look at 1) types of R&D , 2) understanding similar terminology , 3) making the R&D decision , 4) basic R&D process , 5) creating an effective R&D process , 6) advantages of R&D , and 7) R&D challenges .
TYPES OF R&D
A US government agency, the National Science Foundation defines three types of R&D .
Basic Research
When research aims to understand a subject matter more completely and build on the body of knowledge relating to it, then it falls in the basic research category. This research does not have much practical or commercial application. The findings of such research may often be of potential interest to a company
Applied Research
Applied research has more specific and directed objectives. This type of research aims to determine methods to address a specific customer/industry need or requirement. These investigations are all focused on specific commercial objectives regarding products or processes.
Development
Development is when findings of a research are utilized for the production of specific products including materials, systems and methods. Design and development of prototypes and processes are also part of this area. A vital differentiation at this point is between development and engineering or manufacturing. Development is research that generates requisite knowledge and designs for production and converts these into prototypes. Engineering is utilization of these plans and research to produce commercial products.
UNDERSTANDING SIMILAR TERMINOLOGY
There are a number of terms that are often used interchangeably. Thought there is often overlap in all of these processes, there still remains a considerable difference in what they represent. This is why it is important to understand these differences.
The creation of new body of knowledge about existing products or processes, or the creation of an entirely new product is called R&D. This is systematic creative work, and the resulting new knowledge is then used to formulate new materials or entire new products as well as to alter and improve existing ones
Innovation includes either of two events or a combination of both of them. These are either the exploitation of a new market opportunity or the development and subsequent marketing of a technical invention. A technical invention with no demand will not be an innovation.
New Product Development
This is a management or business term where there is some change in the appearance, materials or marketing of a product but no new invention. It is basically the conversion of a market need or opportunity into a new product or a product upgrade
When an idea is turned into information which can lead to a new product then it is called design. This term is interpreted differently from country to country and varies between analytical marketing approaches to a more creative process.
Product Design
Misleadingly thought of as the superficial appearance of a product, product design actually encompasses a lot more. It is a cross functional process that includes market research, technical research, design of a concept, prototype creation, final product creation and launch . Usually, this is the refinement of an existing product rather than a new product.
MAKING THE R&D DECISION
Investment in R&D can be extensive and a long term commitment. Often, the required knowledge already exists and can be acquired for a price. Before committing to investment in R&D, a company needs to analyze whether it makes more sense to produce their own knowledge base or acquire existing work. The influence of the following factors can help make this decision.
Proprietariness
If the nature of the research is such that it can be protected through patents or non-disclosure agreements , then this research becomes the sole property of the company undertaking it and becomes much more valuable. Patents can allow a company several years of a head start to maximize profits and cement its position in the market. This sort of situation justifies the cost of the R&D process. On the other hand, if the research cannot be protected, then it may be easily copied by a competitor with little or no monetary expense. In this case, it may be a good idea to acquire research.
Setting up a R&D wing only makes sense if the market growth rate is slow or relatively moderate. In a fast paced environment, competitors may rush ahead before research has been completed, making the entire process useless.
Because of its nature, R&D is not always a guaranteed success commercially. In this regard, it may be desirable to acquire the required research to convert it into necessary marketable products. There is significantly less risk in acquisition as there may be an opportunity to test the technology out before formally purchasing anything.
Considering the long term potential success of a product, acquiring technology is less risky but more costly than generating own research. This is because license fees or royalties may need to be paid and there may even be an arrangement that requires payments tied to sales figures and may continue for as long as the license period. There is also the danger of geographical limitations or other restrictive caveats. In addition, if the technology changes mid license, all the investment will become a sunk cost. Setting up R&D has its own costs associated with it. There needs to be massive initial investment that leads to negative cash flow for a long time. But it does protect the company from the rest of the limitations of acquiring research.
All these aspects need to be carefully assessed and a pros vs. cons assessment needs to be conducted before the make or buy decision is finalized.
BASIC R&D PROCESS
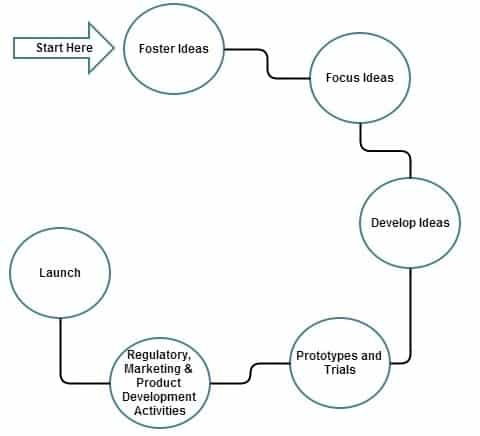
Foster Ideas
At this point the research team may sit down to brainstorm. The discussion may start with an understanding and itemization of the issues faced in their particular industry and then narrowed down to important or core areas of opportunity or concern.
Focus Ideas
The initial pool of ideas is vast and may be generic. The team will then sift through these and locate ideas with potential or those that do not have insurmountable limitations. At this point the team may look into existing products and assess how original a new idea is and how well it can be developed.
Develop Ideas
Once an idea has been thoroughly researched, it may be combined with a market survey to assess market readiness. Ideas with true potential are once again narrowed down and the process of turning research into a marketable commodity begins.
Prototypes and Trials
Researchers may work closely with product developers to understand and agree on how an idea may be turned into a practical product. As the process iterates, the prototype complexity may start to increase and issues such as mass production and sales tactics may begin to enter the process.
Regulatory, Marketing & Product Development Activities
As the product takes shape, the process that began with R&D divides into relevant areas necessary to bring the research product to the market. Regulatory aspects are assessed and work begins to meet all the criteria for approvals and launch. The marketing function begins developing strategies and preparing their materials while sales, pricing and distribution are also planned for.
The product that started as a research question will now be ready for its biggest test, the introduction to the market. The evaluation of the product continues at this stage and beyond, eventually leading to possible re-designs if needed. At any point in this process the idea may be abandoned. Its feasibility may be questioned or the research may not reveal what the business hoped for. It is therefore important to analyze each idea critically at every stage and not become emotionally invested in anything.
CREATING AN EFFECTIVE R&D PROCESS
A formal R&D function adds great value to any organization. It can significantly contribute towards organizational growth and sustained market share. However, all business may not have the necessary resources to set up such a function. In such cases, or in organizations where a formal R&D function is not really required, it is a good idea to foster an R&D mindset . When all employees are encouraged to think creatively and with a research oriented thought process, they all feel invested in the business and there will be the possibility of innovation and unique ideas and solutions. This mindset can be slowly inculcated within the company by following the steps mentioned below.
Assess Customer Needs
It is a good idea to regularly scan and assess the market and identify whether the company’s offering is doing well or if it is in trouble. If it is successful, encourage employees to identify reasons for success so that these can then be used as benchmarks or best practices. If the product is not doing well, then encourage teams to research reasons why. Perhaps a competitor is offering a better solution or perhaps the product cannot meet the customer’s needs effectively.
Identify Objectives
Allow your employees to see clearly what the business objectives are. The end goal for a commercial enterprise is to enhance profits. If this is the case, then all research the employees engage in should focus on reaching this goal while fulfilling a customer need.
Define and Design Processes
A definite project management process helps keep formal and informal research programs on schedule. Realistic goals and targets help focus the process and ensures that relevant and realistic timelines are decided upon.
Create a Team
A team may need to be created if a specific project is on the agenda. This team should be cross functional and will be able to work towards a specific goal in a systematic manner. If the surrounding organizational environment also has a research mindset then they will be better prepared and suited to assist the core team when ever needed.
Whenever needed, it may be a good idea to outsource research projects. Universities and specific research organizations can help achieve research objectives that may not be manageable within a limited organizational budget.
ADVANTAGES OF R&D
Though setting up an R&D function is not an easy task by any means, it has its unique advantages for the organization. These include the following.
Research and Development expenses are often tax deductible. This depends on the country of operations of course but a significant write-off can be a great way to offset large initial investments. But it is important to understand what kind of research activities are deductible and which ones are not. Generally, things like market research or an assessment of historical information are not deductible.
A company can use research to identify leaner and more cost effective means of manufacturing. This reduction in cost can either help provide a more reasonably priced product to the customer or increase the profit margin.
When an investor sets out to put their resources into any company, they tend to prefer those who can become market leaders and innovate constantly. An effective R&D function goes a long way in helping to achieve these objectives for a company. Investors see this as a proactive approach to business and they may end up financing the costs associated with maintaining this R&D function.
Recruitment
Top talent is also attracted to innovative companies doing exciting things. With a successful Research and Development function, qualified candidates will be excited to join the company.
Through R&D based developments, companies can acquire patents for their products. These can help them gain market advantage and cement their position in the industry. This one time product development can lead to long term profits.
R&D CHALLENGES
R&D also has many challenges associated with it. These may include the following.
Initial setup costs as well as continued investment are necessary to keep research work cutting edge and relevant. Not all companies may find it feasible to continue this expenditure.
Increased Timescales
Once a commitment to R&D is made, it may take many years for the actual product to reach the market and a number of years will be filled with no return on continued heavy investment.
Uncertain Results
Not all research that is undertaken yields results. Many ideas and solutions are scrapped midway and work has to start from the beginning.
Market Conditions
There is always the danger that a significant new invention or innovation will render years of research obsolete and create setbacks in the industry with competitors becoming front runners for the customer’s business.
It is important for any business to understand the advantages and disadvantages of engaging in Research and Development activities. Once these are studied, then the step can be taken towards becoming and R&D organization.
In the meanwhile, it is good practice to inculcate a research mind set and research oriented thinking within all employees, no matter what their functional area of expertise. This will help bring about new ideas, new solutions and an innovative way of approaching all business problems, whether small or large.
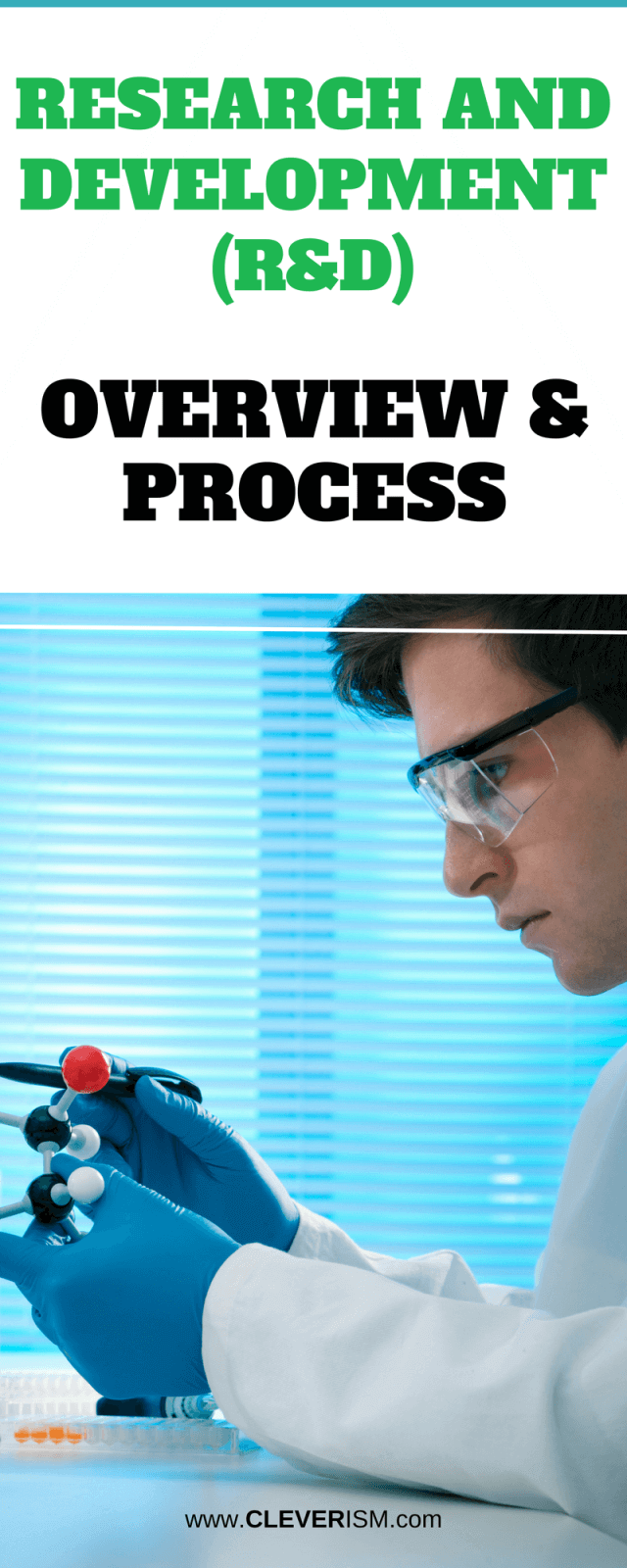
Comments are closed.
Related posts
How to Design and Execute a Backlinking Strategy that Works
SEO professionals have many strategies available to them to increase the visibility of their …
Blumberg Capital | Interview with its Founder & Managing Partner – David Blumberg
In San Francisco, we talked with venture capitalist and the founder of Blumberg Capital, David …
Raising Capital for an Under-Performing Business
When starting a new business, you must have done your homework: how to take care of the logistics, …
408,000 + job opportunities

Not yet a member? Sign Up
join cleverism
Find your dream job. Get on promotion fasstrack and increase tour lifetime salary.
Post your jobs & get access to millions of ambitious, well-educated talents that are going the extra mile.
First name*
Company name*
Company Website*
E-mail (work)*
Login or Register
Password reset instructions will be sent to your E-mail.

An official website of the United States government
The .gov means it’s official. Federal government websites often end in .gov or .mil. Before sharing sensitive information, make sure you’re on a federal government site.
The site is secure. The https:// ensures that you are connecting to the official website and that any information you provide is encrypted and transmitted securely.
- Publications
- Account settings
Preview improvements coming to the PMC website in October 2024. Learn More or Try it out now .
- Advanced Search
- Journal List
- NASA Author Manuscripts

Taking Engagement to Task: The Nature and Functioning of Task Engagement Across Transitions
Daniel w. newton.
1 University of Missouri
Jeffery A. LePine
2 Arizona State University
Ji Koung Kim
3 Texas A&M University
Ned Wellman
John t. bush.
Author Note
Engagement is widely viewed as a motivational state that captures the degree that individuals apply their physical, cognitive, and emotional energies to their jobs, and that positively impacts job performance. However, this job-level view overlooks the possibility that engagement may vary across the different tasks that comprise a job, and that engagement in one task may influence engagement and performance in a subsequent task. In this article, we develop and test hypotheses that emerge from a task-level view of engagement and the general notion that there is “residual engagement” from a task that carries forward to a subsequent task. We propose that although task engagement (engagement in a specific task that comprises a broader role) positively spills over to influence task engagement and performance in a subsequent task, in part due to the transmission of positive affect, task engagement simultaneously engenders attention residue that impedes subsequent task engagement and performance. These predictions were supported in a study of 477 task transitions made by 20 crew members aboard NASA’s Human Exploration Research Analog (Study 1) and in a laboratory study of 346 participants who transitioned between a firefighting task and an assembly task (Study 2). Our investigation explains how engagement flows across tasks, illuminates a negative implication of engagement that has been masked by the predominant job-level perspective, and identifies completeness as a task attribute that reduces this negative consequence of engagement.
“I think that’s when mistakes happen is because you’re not fully engaged, and … you move from one thing to the next, to the next. It’s hard to keep your head in one game after the other after the other.” (International Space Station astronaut)
The extent to which members of organizations engage with their work is a critical determinant of their effectiveness (e.g., Morgan, 2017 ; Zenger & Folkman, 2017 ). Defined as the investment of one’s physical, cognitive, and emotional energies into the various activities that constitute one’s work role ( Kahn, 1990 ), engagement is beneficial to both individuals and organizations. For individuals, engagement is a positive motivational force that transmits the effects of a variety of individual and contextual factors to important job-relevant behaviors ( Rich, LePine, & Crawford, 2010 ; Salanova & Schaufeli, 2008 ; Schaufeli & Bakker, 2004 ). Indeed, individuals who invest more of their personal energies at work are more satisfied with their jobs and are viewed as performing their jobs more effectively ( Christian, Garza, & Slaughter, 2011 ). For organizations, employee engagement is positively associated with indicators of organization effectiveness such as customer loyalty, sales, and profitability ( Harter, Schmidt, Killham, & Agrawal, 2009 ). As such, scholars have proposed that an engaged workforce is a source of competitive advantage that allows organizations to gain an upper hand over their rivals ( Gruman & Saks, 2011 ; Macey, Schneider, Barbera, & Young, 2009 ).
Engagement researchers tend to presume that individuals’ engagement is relatively consistent across the tasks that comprise their jobs. As such, theories of engagement emphasize the investment of energies in individuals’ overall jobs, and the most frequently-used measures of engagement (e.g., Rich et al., 2010 ; Schaufeli, Salanova, González-Romá, & Bakker, 2002 ) capture how individuals attach themselves to their overall jobs or how they tend to immerse themselves in all activities that define their work roles ( Byrne, Peters, & Weston, 2016 ). Indeed, Rich and colleagues’ (2010) elaboration of Kahn’s (1990) engagement concept used the term “job engagement” to describe the construct, to make the job-level focus explicit. Although this job-level perspective of engagement has clearly taken root in the literature and yielded valuable insights, it is limited in its ability to fully capture the nature and implications of engagement in organizations today.
Many jobs today are multifaceted in that they comprise a number of very different tasks ( Cohen, 2013 ; Grant & Parker, 2009 ; Hasan, Ferguson, & Koning, 2015 ). As illustrated by our opening quote, the need to switch rapidly between work tasks may be relevant to engagement, and yet it is unclear how engagement functions in contexts with these temporal rhythms or time sensitivity. Although Kahn (1990) mentioned that engagement might vary throughout the course of the workday—a claim that has been supported indirectly by evidence that engagement fluctuates on a daily basis ( Fletcher, Bailey, & Gilman, 2018 ; Xanthopoulou, Bakker, Demerouti, & Schaufeli, 2009 )—prior theorizing has not considered how engagement in the specific tasks that comprise one’s job could ebb or flow as individuals transition from one task to the next. Moreover, little is known about the extent to which the spillover of engagement across task transitions affects performance in subsequent tasks. Thus, a task-focused perspective on engagement may result in a more complete and relevant theoretical explanation of the phenomenon, and lead to practical insights related to issues such as task sequencing that could benefit employees who work in multifaceted jobs.
The objective of this manuscript is to expand our understanding of engagement by advancing a task-level view of the concept and its functioning. Specifically, we develop and test a model that explains how engagement in one task carries over to influence engagement in a subsequent task, and how this dynamic transfer of engagement influences performance at the task-level. Expanding on Kahn’s (1990) work, we propose that engagement in a task has both positive and negative consequences for engagement in a subsequent task. We propose a positive emotional pathway in which engagement in a task produces positive affect, which spills over to influence engagement in a subsequent task. We further propose a negative cognitive pathway in which engagement in a task causes individuals to experience attention residue —ruminative thinking about a prior task while engaged in a subsequent task ( Leroy, 2009 ; Leroy & Glomb, 2018 ; Leroy & Schmidt, 2016 )—which hinders engagement and performance in the subsequent task. We augment our theorizing with qualitative data provided by astronauts involved in missions aboard the International Space Station and crew members confined in an analog environment on the ground, who reported on their experiences transitioning between tasks.
We formally test our hypotheses in two studies. In Study 1, a field study with a sample of 477 task transitions experienced by 20 crew members in The National Aeronautics and Space Administration’s (NASA) Human Exploration Research Analog, we examine whether engagement in a prior task has a positive indirect effect on performance in a subsequent task through engagement in the subsequent task, and whether this indirect relationship may be partially offset by a negative indirect effect through attention residue. We then test whether activated positive affect accounts for the positive spillover of engagement, and whether task completion is a boundary condition that mitigates the effects of attention residue in a laboratory study of 346 participants transitioning between a group and individual task (Study 2).
The studies reported herein make several important theoretical contributions. First, we advance engagement theory by responding to calls to explore the nature and functioning of engagement at the task-level (e.g., Bakker, 2014 ; Sonnentag, 2011 ). Broadly, we advance the idea that engagement varies at the task-level, and that this “task engagement” flows across transitions to new tasks. More specifically, we develop and test hypotheses regarding how engagement experienced in a task may be related to performance in a subsequent task because it influences engagement in the subsequent task. In this respect, we show how individuals’ energies may flow from one task to another, and also how this flow can be disrupted. Second, and relatedly, we illuminate both positive and negative consequences of task engagement. Although job-level engagement has been shown to improve job performance ( Christian et al., 2011 ), we theorize and find that task-level engagement carries cognitive costs that can impair subsequent task performance. In doing so, we respond to calls to explore how disconnected cognitive energies may influence employee motivation (e.g., Randall, Oswald, & Beier, 2014 ). Third, our research provides an integrative account of the mechanisms responsible for the conveyance of personal energies during transitions to new tasks. On the one hand, we identify positive affect as a mechanism that is partially responsible for the beneficial spillover of task engagement. On the other hand, we confirm that attention residue is responsible for the negative impact of prior task engagement on subsequent task performance.
From a practical standpoint, our findings illustrate the potential benefits of understanding how task engagement influences subsequent task performance. Many individuals begin their workday with relatively unengaging tasks (e.g., checking email) that can have negative implications on their well-being (e.g., Kushlev & Dunn, 2015 ). Our results highlight the importance of individuals’ engaging in their work early in the day to reap the positive benefits from “residual engagement” on subsequent engagement and performance. Of course, residual engagement also involves attention residue, which limits these benefits and, consistent with Leroy’s (2009) work, can be managed by facilitating completion in a prior task in order to reduce the effect of task engagement on attention residue in contexts where time urgency exists. Our discussion centers on the application of these ideas to practices such as task scheduling, which could be enhanced to accentuate the positive spillover of engagement and avoid the dampening effect of attention residue on engagement and performance in subsequent tasks.
Theory and Hypotheses
Kahn (1990) characterized engagement as a holistic concept describing how individuals invest their personal energies in their work. Specifically, Kahn theorized that engagement occurs as individuals harness their physical, cognitive, and emotional energies to their work role performances. Individuals invest their physical energy when they apply bodily effort and intensity to their work. They invest their cognitive energy when they mentally focus their attention on their work role requirements. And finally, individuals invest their emotional energy when they care and are enthusiastic about their work. Meta-analyses have shown that individuals’ engagement in their jobs is positively associated with outcomes such as task and citizenship performance ( Christian et al., 2011 ).
Task-level Engagement
Empirical work has begun to examine how engagement might vary within one’s job. For example, Xanthopoulou et al. (2009) found that fast food workers’ daily engagement varied as a function of their perceived job and personal resources. As another example, Fletcher et al. (2018) found that daily engagement varied as a function of the meaningfulness of daily job tasks and whether resources were sufficient. Although these studies did not examine engagement at the task-level or across task transitions, they do provide evidence that engagement is not always stable for a given job as has been assumed by most prior research. In this manuscript, we further advance the idea that engagement varies within one’s job by offering a task-level view of engagement, which we believe is essential to understanding employee functioning and effectiveness in multifaceted jobs (e.g., Cohen, 2013 ; Sonnentag, 2012 ). To this end, we define task engagement as the degree to which individuals invest their physical, cognitive, and emotional energies into a specific task that composes part of their work role.
The notion of a within-job perspective of engagement and its value was alluded to by Kahn (1990 , p. 719) who wrote that “like using the zoom lens of a camera: a distant stationary image is brought close and revealed as a series of innumerable leaps of engagement and falls of engagement.” Although he stopped short of advancing a task-level engagement concept, Kahn acknowledged that “people are constantly bringing in and leaving out various depths of their selves during the course of their work days” (pp. 692–693). Kahn and others (e.g., Fletcher et al., 2018 ) have also argued that engagement may fluctuate as a function of factors that vary at the task-level. For example, Kreiner, Hollensbe, and Sheep (2006) noted that professional clergy might be engaged in meaningful tasks connected to caring for their flock, but less so for more mundane, functional tasks such as balancing the church budget.
Our task-level view of engagement proposes that individuals may apply different levels of their full selves to different tasks throughout the workday, and that this is reflected by the allocation of emotional, cognitive, and physical energies that vary across the tasks that are performed. Given that scholars have already acknowledged that the process of attaching and detaching one’s self from specific tasks may be influenced, in part, by differences in the characteristics of the particular tasks for which one is responsible ( Saks & Gruman, 2014 ), and because our research centers on the question of how this task-level view informs our understanding of effectiveness in jobs where these tasks are connected to each other, we focus on how the attachment and detachment of one’s self to a task influences the attachment of one’s self through a transition to a subsequent task.
Insights from NASA crew members
We developed the theoretical model we report below a priori based on the literatures on engagement ( Kahn, 1990 ; Rich et al., 2010 ; Shin & Grant, 2018 ), role transitions ( Culbertson, Mills, & Fullagar, 2012 ; Rodríguez-Muñoz, Sanz-Vergel, Demerouti, & Bakker, 2014 ; Rothbard, 2001 ), and attention residue ( Leroy, 2009 ; Leroy & Glomb, 2018 ; Leroy & Schmidt, 2016 ). However, to bring our theorizing to life and better situate it within our research context, we supplement the following section with illustrative quotes from a sample of 30 NASA crew members. The crew members consisted of astronauts conducting missions aboard the International Space Station (ISS) and astronaut-like crew members, who are often aspiring astronauts, in a confined ground-based analog environment (Human Exploration Research Analog; HERA) designed to simulate conditions in space. These environments exert significant time pressure on crew members, as their workday consisted primarily of highly divergent tasks and structured transitions between those tasks. We collected the quotes by applying the critical incident technique ( Flanagan, 1954 ) in 25 surveys administered during ISS missions, in which we asked crew members to recall a recent transition between two tasks and then describe the nature of the tasks, assess how they transitioned, and report any challenges they experienced transitioning. Additionally, within 10 days of the completion of the ISS and HERA missions, we conducted eight 30-minute semi-structured interviews. Given the limited number of data points, we did not achieve theoretical saturation ( O’Reilly, Paper, & Marx, 2012 ). However, we believe the quotes add dynamism and precision to our theorizing by illustrating the flow of engagement across task transitions in our research context and by highlighting the importance of the mechanisms and boundary condition in our conceptual model (i.e., positive affect, attention residue, and task 1 completion).
Spillover of Task Engagement
In many jobs, individuals are responsible for a series of different tasks or performance episodes ( Cohen, 2013 ; Ilgen & Hollenbeck, 1991 ). Our task-centric view of engagement recognizes that engagement may vary across different tasks, and that one’s level of engagement in a task is likely to predict task performance (e.g., Christian et al., 2011 ). However, we also propose that when individuals stop working on a task, the energies applied to that particular task may remain activated through the transition to a new task to influence performance in the subsequent task (e.g., D’Mello & Graesser, 2011 ). In short, an individual’s task engagement, or their allocation of personal energies in a task, may endure and impact their performance on a subsequent task. This concept, which we refer to as residual engagement , is supported by research which has established that motivationally relevant experiences in a task can spill over and influence performance in a subsequent task (e.g., Schmidt & DeShon, 2009 , 2010 ). Moreover, researchers have provided glimpses into how and why these effects might occur. For example, individuals’ physical activity at work has bearing on subsequent productivity ( Coulson, McKenna, & Field, 2008 ), individuals continue to think about a task even after transitioning to something else ( Levinson, Smallwood, & Davidson, 2012 ), and the duration of an emotional experience may last well beyond a trigger event ( Rothbard, 2001 ; Verduyn, Van Mechelen, & Tuerlinckx, 2011 ). Next, we develop hypotheses regarding mechanisms that could explain how, why, and under what conditions engagement in one task might influence performance in a subsequent task.
Positive affect
Researchers have theorized that engagement engenders and activates positive affect ( Bakker & Bal, 2010 ; Kahn, 1990 ; Rothbard, 2001 ), defined as the degree to which one experiences a positive mood and feelings ( Watson & Clark, 1997 ; Watson, Clark, & Tellegen, 1988 ). When individuals allocate and apply their physical, cognitive and emotional energies to a task, they come to appraise that task as being more personally important, which in turn activates positive emotional energy and fosters an inherently satisfying emotional state. For instance, Kahn (1990 , p. 701) described an engaged architect who subsequently expressed the “joy of creating designs both aesthetical and functional.” Consistent with Kahn’s description, numerous researchers have demonstrated that engagement activates positive feelings that make individuals feel happy, alert, and inspired (e.g., Culbertson et al., 2012 ; Rothbard, 2001 ; Salanova, Llorens, & Schaufeli, 2011 ). This line of thinking is also consistent with scholarly work theorizing that positive affect is an outcome of motivation (e.g., George & Brief 1996 ; Naylor, Pritchard, & Ilgen, 1980 ).
When a discrete event elicits an emotion, that emotion is relatively persistent and individuals continue to experience the emotion as it lingers ( Verduyn et al., 2011 ). In this vein, positive affect does not immediately dissipate once activated, but can influence individuals’ subsequent activities (e.g., Bledow, Schmitt, Frese, & Kühnel, 2011 ). Specifically, researchers have theorized that the presence of positive affect may facilitate an individual’s entry into a subsequent task ( Richardson & Taylor, 2012 ). Indeed, as Erez and Isen (2002) demonstrated, affect engendered in one context carries over to other contexts, such that individuals approach subsequent work with more motivation. Similarly, others have concluded that being engaged in a task may create an afterglow that influences subsequent tasks ( Isen & Reeve, 2005 ; Shin & Grant, 2018 ). Taken together, we argue that task engagement engenders the flow of continued positive thoughts and feelings ( Isen, Shalker, Clark, & Karp, 1978 ), which in turn produces an expanded reservoir of available energies ( Elsbach & Hargadon, 2006 ; Fredrickson, 2001 , 2004 ; Salanova, Bakker, & Llorens, 2006 ). Individuals can then draw upon and apply these enhanced personal energies to subsequent tasks and successfully accomplish the requirements of those tasks. Thus, a high level of engagement in one task can lead to positive “gain cycles and spirals” ( Salanova et al., 2011 ), such that the positive and invigorating effects of engagement influence subsequent task performance because they create positive emotions and feelings that carry over to influence subsequent task engagement (e.g., Erez & Isen, 2002 ; Fisher, 2003 ).
The positive emotional spillover we have discussed is illustrated in insights we obtained from NASA crew members. For example, one HERA crew member described how positive affect experienced when engaged in a task lingered throughout subsequent task work:
“There was one instance that I can remember that was MMSEV [multi-mission space exploration vehicle] transitioning to something else. I remember thinking—because I was like, ‘Oh my God, I actually do feel affected’ because I was so happy from the previous activity. And that was really kind of like my attitude carried over and that was about it. If I’m emotionally invested for whatever reason in something, that would have a lasting effect afterwards.”
Another HERA crew member noted:
“If anything spilled over for me it would be Robot and in terms of how I felt about Robot, I’ve said this a couple times: it was about 90 percent exhilaration and happiness and about 10 percent pure hate.”
In summary, we argue that task engagement may enhance performance in a subsequent task because it engenders positive affect, which in turn, promotes engagement in the subsequent task (e.g., Beal, Weiss, Barros, & MacDermid, 2005 ; Rich et al., 2010 ).
Hypothesis 1: Task 1 engagement is positively associated with task 2 performance. Hypothesis 2: Task 1 engagement has a positive indirect effect on task 2 performance through task 2 engagement. Hypothesis 3: Task 1 engagement has a positive indirect effect on task 2 performance through positive affect and task 2 engagement.
Attention Residue
Although the relationships we hypothesized in the previous section have not been directly articulated previously, they reflect the predominant view that engagement is inherently good and can result in a positive upward spiral ( Salanova et al., 2011 ). That is, engagement engenders positive feelings, which beget greater engagement. In this section, we identify a second mechanism through which task engagement may impact subsequent task engagement and performance. Importantly, this second mechanism serves to offset or mitigate the first, and thus, explains why the upward spiral of engagement is not limitless, or even assured. In essence, we argue that while task engagement, in general, may positively spill over from one task to another because it engenders positive affect, there may be negative cognitive consequences of task engagement that partially negate its benefits ( George, 2011 ; Sonnentag, 2011 ).
As Kahn (1990) suggested, highly engaged employees may at times experience reduced availability of energies and engagement in subsequent work. Individuals who experience high task engagement are intensely involved and absorbed in their task activities, and when confronted with an altogether new task may have difficulty letting go because it requires the decoupling of the self with personally meaningful efforts that are intrinsically satisfying. Engagement in a task reflects thinking deeply about the task, and when asked to transition to a new task, it may be difficult to switch this thinking off and redirect it to the new task. The implications of this process are that engagement in a task may inhibit the transfer of cognitive energies to a new task, thereby limiting the level of engagement in the new task. This argument is aligned with research suggesting that it is difficult for people to switch cognitive gears at work (e.g., Ancona & Chong, 1996 ; Freeman & Muraven, 2010 ; Louis & Sutton, 1991 ). This line of reasoning is also consistent with experiences relayed by participants in our interviews. For example, one ISS astronaut noted that after one particular task transition, “I kept thinking that I should have known better how to hook up the CMRS [Crew Medical Restraint System] and realized that I hadn’t done it in a very long time.” Similarly, a HERA crew member noted how they ruminated on engaging tasks after transitioning:
“Unpacking what went right and wrong on a mission and how we could do better in the next MMSEV, for example, or same thing with Robot, just could I have done that faster, could we have had better strategy? So it’s kind of still on my mind as I transition.”
The idea that individuals may not completely refocus their cognitive energies after a transition to a new task has been explored by Leroy and colleagues in their research on attention residue, which refers to persisting thoughts about a previous task after starting a new one ( Leroy, 2009 ; Leroy & Glomb, 2018 ; Leroy & Schmidt, 2016 ). Consistent with Leroy and colleagues’ findings, as well as our prior arguments, we argue that task engagement engenders attention residue, which in turn inhibits engagement in the subsequent task and hinders performance (e.g., Leroy, 2009 ; Leroy & Schmidt, 2016 ). This reasoning aligns with a long-standing notion that applying cognitive energy to a specific task soaks up cognitive resources and thereby limits the amount of cognitive energy individuals can allocate to other tasks to benefit task performances ( Harrison & Wagner, 2016 ; James, 1890 ; Kanfer & Ackerman, 1989 ). Indeed, research on attention residue and task transitions has shown that transitioning from an engaging task can create interference that leads to reduced performance in terms of slower reaction times and elevated error rates (e.g., Cellier & Eyrolle, 1992 ; Freeman & Muraven, 2010 ; Kiesel et al., 2010 ; Kühnel, Sonnentag, & Westman, 2009 ; Leroy, 2009 ; Leroy & Schmidt, 2016 ).
Hypothesis 4: Task 1 engagement is positively associated with attention residue. Hypothesis 5: Task 1 engagement has a negative indirect effect on task 2 performance through attention residue and task 2 engagement.
Task Completion
Thus far, we have argued that there are two offsetting paths through which task engagement influences subsequent task engagement and performance. On the one hand, engagement instills positive affect, which in turn fosters engagement and performance in the subsequent task. On the other hand, engagement engenders attention residue, which in turn inhibits engagement and performance in the subsequent task. With this framework in mind, one key to understanding how to manage engagement and performance in multifaceted jobs lies in identifying factors that could serve to mitigate the negative pathway attributed to attention residue. Here we argue that task completion, or the degree to which one perceives that a task has reached closure ( Leroy, 2009 ; Webster & Kruglanski, 1994 ), is likely to limit the attention residue individuals experience when they transition out of an engaging task.
We propose that task 1 engagement may produce the most attention residue when individuals perceive that they have left a task incomplete. Individuals who are engaged in a task couple their full selves to the task and are motivated to invest their energies to fully satisfy necessary task requirements ( Diefendorff & Chandler, 2011 ; Kahn, 1990 ; Lewin, 1935 ). A requirement to transition from an incomplete engaging task may result in a sharp tension and an accompanying thought process, which may amplify the effects of engagement in a task on attention residue and subsequent task engagement and performance ( Leroy, 2009 ; Zeigarnik, 1967 ). An individual in this dissatisfying situation may be left with a range of thoughts regarding implications of not finishing the engaging task and how best to catch up at some point in the future. As the level of attention residue from the first task increases, it is likely to inhibit the development of engagement in the subsequent task. The significance and implications of transitioning from an engaging but incomplete task were noted by several of the crew members in our sample. For example, one ISS astronaut noted after a task transition:
“I kept thinking about the first task because it had not been completed yet. But this second event is always time critical so you have to break off in the middle of the procedure to make the second event. This makes returning to the first event very hard. Especially because now you are behind the timeline.”
Similarly, a HERA crew member indicated:
“I would say if there was something at the end of the task, let’s say it was kind of unclear whether or not the task’s completed in its entirety, or if for some reason we had unclear instructions or something to that effect where it left it kind of open ended, then sometimes I might think back about, oh you know, we need to check on that.”
As is evident in these quotes, an engaging but incomplete task causes individuals to expend cognitive energy thinking about how they might pick up the incomplete task at a later time, making it difficult for them to engage with a subsequent task. These effects may be exacerbated by the time pressure individuals experience.
In contrast, we propose that task 1 engagement is less likely to result in attention residue when individuals perceive they have completed the task. As individuals fulfill requirements of an engaging task, they may perceive a sense of closure with the task, and consequently, are less likely to ruminate about the task, or plan new or different strategies for task completion. Instead, their personal resources are likely to be more available to invest in other tasks which comprise their broader work roles. This argument is consistent with Leroy’s (2009) work, which has shown that task completion reduces attention residue in contexts when individuals experience time pressure. Taken together, we hypothesize that:
Hypothesis 6: Task 1 completion moderates the positive relationship between task 1 engagement and attention residue such that the relationship is stronger when task 1 is incomplete and weaker when task 1 is complete. Hypothesis 7: Task 1 completion moderates the negative indirect relationship between task 1 engagement and task 2 performance through attention residue and task 2 engagement such that the indirect effect is stronger when task 1 is incomplete and weaker when task 1 is complete.
Overview of Studies
We tested our conceptual model across two studies. With Study 1, our goal was to establish whether the positive spillover of task engagement on subsequent task engagement and performance is offset by attention residue. In this study, we collected 477 task-transition-task episodes across five missions in a confined NASA isolation field environment and formally tested Hypotheses 1, 2, 4, and 5. With Study 2, we tested our entire conceptual model (Hypotheses 1–7) and accounted for some of the methodological limitations of the data gathered in Study 1. The second study involved 346 participants who participated in a laboratory study involving a firefighting simulation and a transition to an assembly task. Study 1 received Institutional Review Board approval under protocol #2668 [ Residual Engagement – Human Exploration Research Analog (HERA) ] from Arizona State University and protocol #1772 ( Understanding and Preventing Crew Member Task Entrainment ) from NASA. Study 2 received Institutional Review Board approval under protocol #2374 ( Residual Engagement Lab Experiment ) from Arizona State University.
Sample and procedure
We assessed the offsetting effect of attention residue on task engagement spillover by collecting data from NASA’s HERA isolation facility (the Human Exploration Research Analog) where crews of four lived together and performed tasks in a confined space-like environment without leaving for 30–45 days. Participants included 20 crew members from five missions. This NASA context provided an appropriate environment to test our hypotheses because crew members’ work schedule and time is highly programmed and regimented. Specifically, the crew members’ workday consists of the performance of a wide array of tasks and transitions between the tasks. Prior to each mission, NASA compiles a daily “playbook” or schedule for each crew member. The playbook is available to view throughout the “habitat” and creates time pressure for crew members by serving as a constant reminder to complete tasks in the allotted time period before beginning another task.
The focus of this study was task-transition-task episodes in which crew members worked on one task and then transitioned directly to a different task. We worked closely with NASA subject matter experts to identify episodes that could potentially vary in terms of the level of crew member engagement. For example, crew members performed simulated moon walks, rover landing tasks, emergency simulations, public outreach events, asteroid sampling analysis, seed growth projects, brine shrimp analysis, and general maintenance tasks to maintain the habitat. In order to make a conservative test of our hypotheses, we coordinated with NASA subject matter experts to balance task-transition-task episodes, such that crew members switched between engaging tasks, mundane tasks, or a combination of the two. In short, the types and combinations of tasks produced an ideal setting to examine the dynamic nature of task engagement that may exist in many types of jobs and ensured adequate variance on our independent variable—task 1 engagement. Moreover, some of the tasks were performed by crew members working alone (e.g., seed growth, system maintenance), whereas other tasks were performed with others (e.g., simulated moon walks, rover landing tasks). Crew members completed an average of 25 tasks per day, with the average task duration lasting approximately 30 minutes.
During the five missions (four of which consisted of 22 training days and 8 “rest” days, and one of which consisted of 32 training days and 13 “rest” days), we captured 477 task-transition-task episodes that crew members naturally performed during their work day. We also worked with NASA subject matter experts to select the episodes where (1) crew members transitioned immediately from one task to another (without a break period in between), and where (2) the first and second tasks were different enough to constitute a transition between different tasks (rather than two periods of performing the same task). Upon completion of a daily task 1-transition-task 2 episode, we administered a brief survey in which crew members rated their engagement in the first and second tasks, their attention residue in the initial task during the second task, and task performance. Crew members never reported on more than one task-transition-task episode per work day to protect against contamination with other transition episodes on the same day. Although we acknowledge limitations of the retrospective design (discussed later), we had to balance these concerns with constraints of the NASA mission and the necessity to study naturally occurring transitions. Administering surveys in the middle of a task-transition-task episode would have potentially disrupted the very flows of engagement we were attempting to study.
Task engagement
We measured crew members’ engagement in both task 1 and task 2. To fit our surveys in the time window allowed by NASA, we used 9 items (3 items each for physical, cognitive, and emotional engagement) that Crawford, LePine, and Buckman (2013) adapted from the Rich et al. (2010) job engagement scale. We further adapted the items by changing the referent experience from “job” to “task.” Sample items include “I exerted my full effort in the first task,” “I felt energetic working on the first task,” and “I concentrated completely on the first task.” Crew members rated their level of agreement on a 5-point Likert scale (1 = “ strongly disagree ” to 5 = “ strongly agree ”). Coefficient alpha was .96 for engagement in task 1, and .96 for engagement in task 2.
Attention residue
Leroy and Glomb (2018) recently validated a nine-item measure of attention residue. However, the limited time window allowed by NASA necessitated a shortened measure. Consequently, we developed and administered a three-item measure of attention residue. Prefaced by the phrase “while performing the second task,” our scale consists of the following items: “My mind kept on drifting back to the first task,” “I kept on thinking about the first task,” and “I thought about how to do the first task better.” Participants rated their level of agreement on a 5-point Likert scale (1 = “ strongly disagree ” to 5 = “ strongly agree ”). Coefficient alpha for this scale was .95. To examine the validity of our attention residue scale, we recruited 114 full-time Mturk workers [33% were female, 65% were Caucasian, average age was 36.0 ( SD = 11.8), and average years of work experience was 13.7 ( SD = 9.5)] and asked them to respond to our three-item measure and Leroy and Glomb’s nine-item measure. In a principal components factor analysis with varimax rotation conducted in SPSS v. 25, we found that two factors emerged with eigenvalues greater than 1.0 and which explained 75.6% of the variance. The first factor included all three of our items and six of Leroy and Glomb’s items. The second factor consisted solely of the three reverse coded items in Leroy and Glomb’s scale. Each item factor loading was statistically significant with values greater than .67, and no cross-loadings above .25. The correlation between Leroy and Glomb’s scale and our measure was .79; however, the correlation increased to .89 when removing the three reverse coded items. These findings provide us reasonable assurance that the two scales tap the same underlying construct.
Task 2 performance
We assessed participants’ performance in the second task using a 3-item scale developed by Aubé and Rousseau (2005) . Sample items for this scale include “Attained the assigned performance goals on the second task” and “Produced quality work on the second task.” Although we acknowledge the limitations of self-report performance ratings, in this context participants understood that the attainment of goals and the quality of outputs can be verified by the parties involved in the mission. All items were on a 5-point Likert scale ranging from 1 (“ strongly disagree” ) to 5 (“ strongly agree ”). Reliability of this scale was .82.
We controlled for crew members’ perceptions of performance on the first task using the same measure as task 2 performance. Controlling for task 1 performance is important because the extent to which individuals believe that they performed well (or poorly) on a task could relate to their task engagement and attention residue after transitioning to a different task. In addition, social bonds may develop among members of a group involved in a group task (e.g., Hollenbeck, Ellis, Humphrey, Garza, & Ilgen, 2011 ; Kelly & McGrath, 1985 ); thus, individuals who transition from a group-based task to an individual-based task may differ in their psychological experience, such as the proclivity to experience attention residue and engagement. To account for this possibility, we included three dummy variables to control for the different types of transitions. We chose individual task to individual task for the comparison group, and thus, the three transition dummy variables represented (a) individual task to crew task, (b) crew task to individual task, and (c) crew task to crew task. Finally, we included two temporal controls: the duration of the first task and the time of day a task-transition-task episode was completed (dummy coded as morning or afternoon). We reasoned that the time spent on a task could potentially introduce mind wandering ( Randall et al., 2014 ), and crew members could be more fatigued with tasks performed later in the day ( Hülsheger, 2016 ).
Confirmatory factor analysis
Descriptive statistics, correlations, and reliabilities for our Study 1 variables are presented in Table 1 . In order to verify the factor structure of our measurement model and further establish discriminant validity of our attention residue scale, we conducted a confirmatory factor analysis (CFA) using Mplus 7.4 ( Muthén & Muthén, 2015 ). We specified a CFA model with three latent factors (task engagement, attention residue, and task 2 performance), using individual items as indicators. Task engagement was modeled as a higher-order factor consisting of three lower-order dimensions: physical, cognitive, and emotional engagement ( Rich et al., 2010 ). In addition, as our model includes the same latent factor (engagement) measured across two tasks, we modeled task 1 engagement and excluded task 2 engagement in our CFA model. Results with task 2 engagement included and task 1 engagement excluded did not significantly alter the results of the CFA. Overall, our hypothesized three-factor model showed good fit to the data: χ 2 (84) = 185.98, p < .001; CFI = .99; RMSEA = .05; SRMR = .02. In addition, all factor loadings were statistically significant, the average variance explained was greater than .50 for each factor ( Fornell & Larcker, 1981 ), and the three-factor model fit the data better than alternative models that included one or two latent factors.
Descriptive Statistics, Correlations, and Reliabilities (Study 1)
Note . N = 477. Transition Type Dummy Coded (i.e., Individual to Team Transition, Team to Individual Transition, and Team to Team Transition); Time of Day Dummy Coded (1 = afternoon; 0 = morning).
Hypotheses testing
Although the primary focus of our theoretical model is on the flow of task engagement across task transitions, the hierarchical structure of our data (477 task-transition-task episodes nested within 20 individuals) necessitated that we control for the nesting of task transition episodes within crew members. Following guidelines provided by Raudenbush and Bryk (2002) , we first assessed whether sufficient level-2 variance (between-individuals) was present in our data. Specifically, we tested three null models for each of our dependent or endogenous variables of interest (attention residue, task 2 engagement, and task 2 performance). Results indicated that significant variance at level-2 (individual-level of analysis) existed for attention residue (τ 2 = .30, p = .003, ICC(1) = .31), task 2 engagement (τ 2 = .17, p < .001, ICC(1) = .22), and task 2 performance (τ 2 = .06, p = .003, ICC(1) = .13). We should note that although the 20 crew members that comprise our sample were nested in 5 crews, we did not account for crew-level nesting effects for two reasons. First, our three endogenous variables of interest had trivial levels of variance at the crew-level: task-2 engagement (τ 2 = .03, ICC(1) = .04), attention residue (τ 2 = .07, ICC(1) = .08), and task performance (τ 2 = .02, ICC(1) = .05). Second, the small number of crews did not afford us with sufficient statistical power to specify a three-level structural model. Accordingly, we specified a model in Mplus that accounted for nesting effects at the individual-level of analysis (level-2). As depicted in Figure 1 , our hypothesized path model suggests good fit to the data: χ 2 (6) = 5.48, p = .48; CFI = 1.00; RMSEA = .00; SRMR = .02 (for within-level of analysis) and SRMR = .00 (for between-level of analysis).

Structural Equation Model (Study 1). N = 477. Unstandardized path coefficients are presented; standard errors are in parenthesis. All paths with a solid line are significant at p < .05.
Results from our analysis do not support Hypothesis 1. That is, we did not find a positive relationship between task 1 engagement and task 2 performance ( b = −.07, p = .08). In contrast, Hypothesis 4, which predicts that task 1 engagement is positively related to attention residue, is supported ( b = .24, p < .001).
Hypothesis 2 predicts a positive indirect relationship between task 1 engagement and task 2 performance through task 2 engagement, and Hypothesis 5 predicts a negative indirect relationship between task 1 engagement and task 2 performance through attention residue and task 2 engagement. Because indirect effects represent the product of multiple path coefficients, and therefore are not normally distributed, we estimated the sampling distribution of the first (task 1 engagement → task 2 engagement) and second (task 2 engagement → task 2 performance) stage coefficients using a Monte Carlo simulation in R with 20,000 iterations (e.g., Bauer, Preacher, & Gil, 2006 ; MacKinnon, Fairchild, & Fritz, 2007 ; MacKinnon, Lockwood, Hoffman, West, & Sheets, 2002 ; Preacher, Zyphur, & Zhang, 2010 ) to test Hypothesis 2. We used this same Monte Carlo approach to estimate the sampling distribution of the first (task 1 engagement → attention residue), second (attention residue → task 2 engagement), and third (task 2 engagement → task 2 performance) stage coefficients to test Hypotheses 5. This approach provides bias-corrected confidence intervals for assessing the statistical significance of the indirect effects depicted in Figure 1 .
As shown in Table 2 , task 1 engagement has a positive indirect effect on task 2 performance through task 2 engagement ( indirect effect = .08, 95% CI = .052, .119), supporting Hypothesis 2. In other words, engagement in a task fosters engagement in a subsequent task, which, in turn, is positively associated with performance in the subsequent task. In support of Hypothesis 5, task 1 engagement has a negative indirect effect on task 2 performance through attention residue and task 2 engagement ( indirect effect = −.01, 95% CI = −.016, −.003). Stated more plainly, engagement in a task engenders attention residue, which negatively impacts subsequent task performance through subsequent task engagement.
Indirect Effects from Task 1 Engagement to Task 2 Performance (Study 1)
Note . N = 477. Unstandardized indirect effects, standard errors, and Monte Carlo bootstrapped (20,000) confidence intervals are reported.
We did not find support for our hypothesis that task 1 engagement and task 2 performance are positively related. However, the lack of a positive direct effect in our model, and the weak and non-significant zero-order relationship depicted in Table 1 are understandable in light of our hypothesis regarding the mitigating effects of attention residue. That is, the lack of an apparent relationship between task 1 engagement and task 2 performance may be explained by an indirect effect of attention residue that partially offsets the positive spillover of task 1 engagement to task 2 performance through task 2 engagement. In other words, it is not that task engagement is irrelevant to performance in a subsequent task; rather, task engagement has mixed implications with respect to its impact on performance in subsequent tasks.
Of course, Study 1 is subject to limitations. First, constraints imposed by the research setting limited our ability to temporally separate the measurement of our study variables and, therefore, our ability to draw causal inferences. Second, task performance was self-reported, which increases the risk of bias ( Podsakoff, MacKenzie, Lee, & Podsakoff, 2003 ). However, we were able to access a limited number of objective ratings of crew member performance (the number of objectives met during multi-mission space exploration flights). The correlation between self and objective performance ratings in 54 of the tasks was .49 ( p < .001), which is consistent with findings of prior research regarding the relationship between subjective and objective metrics of performance ( Bommer, Johnson, Rich, Podsakoff, & MacKenzie, 1995 ). Given that we controlled for self-rated prior performance, we are optimistic regarding the validity of the self-ratings in our study. Nevertheless, a study with non-same source ratings of performance would be valuable. Perhaps more importantly, although we found support for the idea that engagement in the first task fosters engagement in the second task, we did not fully explore why engagement in the two tasks were related, nor did we explore factors that might influence the strength of the explanatory pathways. In particular, a second study examining our hypotheses concerning the mediating role of positive affect and the moderating role of task completion could provide further insight into how and under what conditions task engagement might influence engagement and performance in a subsequent task, and how performance in multifaceted work could also be enhanced.
Study 2 was designed to build upon Study 1 in several ways. First, whereas Study 1 only tested a subset of our hypotheses, Study 2 tests our entire conceptual model. Second, although Study 1 occurred in a context where task transitions are frequent and critical to mission success, aspects of the design increased the likelihood of measurement bias and presented threats to internal validity. Thus, in Study 2 we used a laboratory study to increase the degree of control over the tasks and reduce potential concerns regarding common method variance and retrospective accounts of beliefs and feelings regarding prior tasks.
Participants
We conducted a laboratory study in which 364 undergraduate students at a large United States university participated in exchange for course credit. We excluded 18 participants who either expressed suspicions regarding the nature of our study or responded carelessly to the survey as identified by an even-odd consistency measure ( Meade & Craig, 2012 ). Our final sample was comprised of 346 participants. Participants’ average age was 22.81 years ( SD = 3.41) and 61.8% were male. 46.5% of participants were Caucasian, 29.2% were Asian, and 13.6% were Hispanic. 54.0% of participants reported that they were currently employed, with an average work experience of 4.06 years ( SD = 3.53).
Each session was comprised of a group of four participants. Each group was randomly assigned to either the task 1 incomplete condition or the task 1 complete condition. Upon arriving at our lab, participants completed a survey of individual differences. Based on results of Study 1 which indicated that the type of transition (e.g., crew to individual, individual to crew, individual to individual, crew to crew) explained little variance in our outcomes, we controlled for type of transition by design. That is, we structured the study such that all participants first performed a group task that was followed by an individual task. In introducing the study, the experimenter told participants that they would take part in a series of tasks—a group firefighting task and an independent assembly task of the firefighting truck—that were part of their larger firefighting job. We allotted 20 minutes to each task so that participants would sense a degree of urgency, consistent with our previous study.
Upon completion of the initial survey, the experimenter introduced C3Fire ( Johansson, Trnka, Granlund, & Götmar, 2010 ), a firefighting simulation, and informed the participants they would work on this computer simulation as a group for 20 minutes. We chose a firefighting task because it is meaningful and consequential to participants, and thus likely to engender task engagement that could spill over into a second task. Although there were elements of the task that necessitated some degree of interdependence and communication among participants, we programed the simulation in a way that emphasized distinct individual roles and the performance of those individual roles. Thus, the task was designed to promote sufficient individual-level variance to maintain the individual as the primary unit of theory and analysis. The experimenter conducted a brief training session (approximately 10 minutes) during which he explained the objective of the firefighting simulation and participants’ individual roles.
Participants in the C3Fire simulation extinguish as many forest wildfires as possible while also containing the fire and protecting landmarks such as houses, schools, and hospitals. Participants were randomly assigned to one of the following four roles within the simulation: fire chief, fire fighter, fire scout, and water carrier. The fire chief was responsible for coordinating crew actions and movements, and was the only member that could see the location of other crew members and the location of all active fires. The firefighter’s task was to put out and contain the fires. Although the firefighter could quickly extinguish fires, movement across terrain to other fires was slow. The fire scout, however, could move quickly across the terrain. Consequently, the fire scout’s duties were to respond to, contain, and extinguish distant fires. To facilitate quick movement, the scout’s compliment of equipment was small, and thus, was limited in firefighting capabilities. Finally, the water carrier was tasked with transporting water to other crew members so they could perform their task responsibilities. Members had distinct duties and communicated with each other during the simulation, but we programmed the task so that members spent the majority of their time performing their individual roles, which were quite similar in the level of complexity and required effort.
We manipulated task 1 completion after participants had reached 20 minutes of activity on the firefighting simulation. At that time, the experimenter told participants in the task 1 complete condition that the firefighting task simulation is complete. In the task 1 incomplete condition the experimenter told participants that they had not completed the firefighting task simulation, and that they would need to continue to fight remaining fires later in the session. Although participants did not receive individual performance feedback, participants in both conditions were shown the wildfire map of their simulation results so that they could assess their group’s performance in the task. Participants then answered a brief survey asking about their level of engagement and positive affect during the simulation.
Following this survey, participants were informed that “many jobs contain maintenance tasks—wherein equipment and materials must be maintained in order to retain their useful life. During the course of your firefighting work, you have driven over rough, burned out terrain—and the top portion of your fire truck has been damaged. Consequently, if you want to fight future fires, you must now maintain and repair your truck.” Participants were told that to simulate this aspect of the firefighting job, they would need to assemble a Lego fire truck. Participants were given a complete set of Legos and were told they could take 20 minutes to complete the task of building the truck according to the instructions, which we supplied (“truck maintenance task”). We chose a Lego task because it is similar to some of the assembly tasks performed by the crew members in Study 1 and provides a clear metric of performance. When participants completed the Lego truck, they informed the experimenter, who recorded how long it took the participant to finish the task (some participants finished the task early). After the 20 minutes were complete, participants who had not yet finished the truck maintenance task were asked to stop working.
Participants were then given a survey in which they rated their attention residue from task 1 (firefighting simulation) during task 2 (truck maintenance task) and also their engagement in task 2. During this time, the experimenter assessed the number of Lego pieces each participant had accurately and inaccurately assembled. Finally, participants provided demographic information, indicated whether they had suspicions about the study’s goals, and provided general open-ended comments about their reactions to the study. Upon completion of the final survey, participants were debriefed and dismissed. In all, each study lasted approximately 90 minutes.
We used the long form of Rich et al.’s (2010) engagement scale, which included the 9 items from Study 1, to assess participants’ engagement in the firefighting and truck maintenance tasks (e.g., Crane, Crawford, Buckman, & LePine, 2017 ). Example items include “I worked with high intensity on the (firefighting simulation/truck maintenance task),” “My heart was in the (firefighting simulation/truck maintenance task)”, “I paid a lot of attention to the (firefighting simulation/truck maintenance task).” Participants rated their level of agreement on a 5-point Likert scale (1 = “ strongly disagree ” to 5 = “ strongly agree” ). Reliability of this measure was .94 for engagement in task 1, and .95 for engagement in task 2. 1
We assessed participants’ attention residue with the three-item measure described in Study 1, and adapted it to pertain to the lab tasks. Participants rated their level of agreement on a 5-point Likert scale (1 = “ strongly disagree ” to 5 = “ strongly agree” ). Coefficient alpha was .79.
We measured participants’ positive affect during the firefighting simulation using 10 items from the PANAS-X ( Watson & Clark, 1994 ). Participants were instructed to indicate the extent to which they felt “interested,” “excited,” “strong,” “enthusiastic,” “proud,” “alert,” “inspired,” “determined,” “attentive,” and “active” during the firefighting simulation on a 5-point Likert scale (1 = “ very slightly or not at all ” to 5 = “ extremely” ). Coefficient alpha for this scale was .89.
We operationalized participants’ performance in the truck maintenance task by first calculating the number of Lego blocks they accurately assembled per minute. For instance, if a participant correctly put together a total of 60 blocks in 10 minutes, he/she received a score of 6. The truck maintenance task had a total of 77 Lego pieces, and participants’ average score was 4.68 ( SD = 1.65). In order to assist in the interpretability of indirect effects in our model, we standardized participants’ scores by conducting a z-score linear transformation. The mean score was 0 after transformation, with a standard deviation of 1.00.
Control variables
Although we randomly assigned participants to conditions, we measured rather than manipulated many of the variables in our model. Therefore, we included several statistical control variables to rule out alternative explanations and more accurately capture the relationships between our focal variables ( Bernerth & Aguinis, 2016 ). This approach is commonly adopted in studies with similar research designs (e.g., Chen & Mathieu, 2008 ; Wagner, Barnes, Lin, & Ferris, 2012 ). First, individuals with high levels of general mental ability (GMA) may have more cognitive energies at their disposal to invest in their tasks and may be better able to focus on current tasks (e.g., Kanfer & Ackerman, 1989 ; Randall et al., 2014 ). As such, we controlled for participants’ general mental ability by administering a six-minute general aptitude test we developed for settings where time constraints and participant fatigue is a concern. 2 We also controlled for participants’ trait positive affect ( Watson & Clark, 1994 ) as prior research indicates that the extent to which individuals experience positive affect in certain situations (i.e., state affect) is largely influenced by individuals’ general mood (i.e., trait affect; Nemanick & Munz, 1997 ; Watson, 1988 ). Furthermore, individuals’ dispositional willingness to try something different ( McCrae & Costa, 1997 ) may influence how effectively they transition between tasks and perform those tasks ( Dane, 2018 ), so we used 10 items from the international personality item pool to control for participants’ openness to experience ( Goldberg et al., 2006 ). Additionally, because individuals performed the first task in teams with specific roles where social bonds could develop ( Hollenbeck et al., 2011 ), we controlled for team membership as well as team role. Consistent with Study 1, we reasoned that participants’ performance on the first task could influence the degree to which they ruminated or experienced positive emotions with the first task that carried over to the second task. Consequently, we controlled for participants’ objective performance and perceptions of their performance on the firefighting task. Finally, we controlled for participants’ demographics (i.e., age and gender), as certain characteristics may potentially impact their familiarity with and performance on our experimental tasks (e.g., Blakemore & Centers, 2005 ).
Manipulation check
Descriptive statistics, correlations, and reliabilities for Study 2 are presented in Table 3 . As part of the survey participants completed when they rated their engagement and positive affect in the firefighting simulation, we also asked participants to rate the extent to which they had fully completed the firefighting simulation on a 5-point Likert scale (1 = “ strongly disagree ” to 5 = “ strongly agree ”). The mean of this manipulation check item was significantly higher for the task 1 complete condition ( M = 4.05, SD = 1.05) than for the task 1 incomplete condition ( M = 3.17, SD = 1.26; t (344) = 7.03, p < .001), suggesting that our manipulation worked as intended.
Descriptive Statistics, Correlations, and Reliabilities (Study 2)
Note . N = 332. Task 1 Completion coded 0 = Incomplete Condition; 1 = Complete Condition. Gender coded 1 = Male; 2 = Female. Team Role coded 1 = Fire Chief; 0 = Other Role.
As our sample for Study 2 had a nested structure (individual participants nested in teams), we first assessed whether significant between-team variance was present in our data. Consistent with Study 1, we conducted our Study 2 analysis in Mplus 7.4 ( Muthén & Muthén, 2015 ). Moreover, following the same procedure as in Study 1, we tested null models for the endogenous variables in our path model ( Raudenbush & Bryk, 2002 ). However, results indicated that significant between-team (level-2) variance does not exist for our endogenous variables of interest: task 2 engagement (τ 2 = .01, p = .78, ICC(1) = .02), attention residue (τ 2 = .00, p = .93, ICC(1) = .03), positive affect (τ 2 = .06, p = .12, ICC(1) = .11), and task 2 performance (τ 2 = .04, p = .40, ICC(1) = .05). As such, we specified our path model at the individual level of analysis.
As shown in Figure 2 , our hypothesized model provided good fit to the data: χ 2 (9) = 10.79, p = .29; CFI = 1.00; RMSEA = .03; SRMR = .02. In addition, our model replicated the results of Study 1 (Hypotheses 1, 2, 4, and 5). Hypothesis 1, which predicted a positive relationship between task 1 engagement and task 2 performance, was not supported ( b = −.04, p = .71). However, consistent with our predictions for Hypothesis 4, task 1 engagement had a positive relationship with attention residue during the second task ( b = .17, p = .03). To test the indirect effects of task 1 engagement on task 2 performance (i.e., Hypotheses 2, 3, and 5), we utilized the same approach as in Study 1. Specifically, we estimated the sampling distributions of the first, second, and third stage coefficients using a Monte Carlo simulation with 20,000 iterations, then calculated the boundaries of a bias-corrected 95% confidence interval ( Preacher et al., 2010 ). As reported in Table 4 , task 1 engagement had a positive indirect effect on task 2 performance via task 2 engagement ( indirect effect = .17, 95% CI = .071, .288), providing support for Hypothesis 2. In support of Hypotheses 3, task 1 engagement had a positive indirect effect on task 2 performance through positive affect and task 2 engagement ( indirect effect = .06, 95% CI = .006, .127). Finally, task 1 engagement had a negative indirect effect on task 2 performance via attention residue and task 2 engagement ( indirect effect = −.02, 95% CI = −.051, −.001), providing further support for Hypotheses 5.

Structural Equation Model (Study 2). N = 332. Unstandardized path coefficients are presented; standard errors are in parenthesis. All paths with a solid line are significant at p < .05.
Indirect Effects of Task 1 Engagement on Task 2 Performance (Study 2)
Note . N = 332. Unstandardized indirect effects, standard errors, and Monte Carlo bootstrapped (20,000) confidence intervals are reported.
Hypothesis 6 predicts that task completion moderates the positive relationship between task 1 engagement and attention residue such that this relationship is stronger when task 1 is incomplete and weaker when task 1 is complete. As shown in Figure 2 , the interaction of task 1 engagement and task 1 completion was significantly associated with attention residue ( b = −.31, p = .04). To assess the nature of the interaction, we conducted a simple slopes analysis ( Aiken & West, 1991 ) and plotted the interaction at “incomplete” and “complete” conditions of the moderator, as shown in Figure 3 . Providing further support for Hypothesis 6, the positive relationship between task 1 engagement and attention residue was stronger in the task 1 incomplete condition ( simple slope = .32, p = .01) than in the task 1 complete condition ( simple slope = .02, p = .86).

Task 1 Engagement and Task 1 Completion Predicting Attention Residue (Study 2)
Hypothesis 7 predicts that task 1 completion moderates the negative indirect effect from task 1 engagement to task 2 performance through attention residue and task 2 engagement such that the negative indirect effect is stronger when task 1 is incomplete and weaker when task 1 is complete. To test for conditional indirect effects (i.e., moderated mediation), we utilized the same approach as our analysis of indirect effects outlined above, but with the simple slopes at the complete and incomplete conditions replacing the coefficient for the first path of the indirect effect (task 1 engagement → attention residue; Preacher, Rucker, & Hayes, 2007 ). Results show that the indirect effect is negative when task 1 is incomplete ( indirect effect = −.04, 95% CI = .074, −.009), but non-significant when task 1 is complete ( indirect effect = −.00, 95% CI = −.026, .020). As an indication of moderated mediation and supporting Hypothesis 7, the conditional indirect effects are significantly different (Δ indirect effect = .04, 95% CI = .007, .086).
Study 2 results provide a more complete depiction of the dynamic effects of engagement in one task on engagement and performance in a subsequent task. We again did not find support for the direct relationship between task 1 engagement and task 2 performance. However, consistent with Study 1, our results suggest that this is a function of two offsetting pathways. That is, there is a positive spillover pathway, attributable to positive affect, which is partially offset by a negative pathway through attention residue. We also found that the debilitating effects of attention residue on subsequent engagement and performance are mitigated when a prior engaging task is viewed as being more complete. Thus, our findings illuminate the potential of managing attention residue so that the enhancing effects of task engagement on subsequent task engagement and performance can flow throughout a workday.
Overall Discussion
Across two studies, we develop and test a task-level view of engagement that explains how and under what conditions engagement in one task may spill over and be related to engagement and, by extension, performance in a subsequent task. In both studies, we found that task engagement positively cascades to a subsequent task when individuals transition from one task to another. However, we also found that task engagement can lead to attention residue that reduces the positive effect of task engagement on subsequent task engagement and performance. In Study 2, we specifically examine and find support for positive affect as the mechanism partially responsible for the positive spillover of engagement. Furthermore, task completion mitigates the effects of task 1 engagement on attention residue.
Theoretical Implications
The research reported in this article has several important implications. First, our view of engagement at the task-level provides a more nuanced and dynamic explanation of how engagement operates. By focusing our theoretical attention at the task-level, we were able to examine how engagement in a specific task influences the extent to which individuals invest their energies in a subsequent task. Our results underscore the critical role that task transitions play in understanding task engagement and task performance, primarily due to the residual engagement or spillover effect of engagement that we observed across transitions. This spillover of engagement further illuminates “the momentary ebbs and flows” of engagement over the course of daily performance episodes ( Kahn, 1990 , p. 693). In this respect, we not only respond to calls to explore engagement in specific tasks ( Bakker, 2014 ; Sonnentag, 2011 ), but also consider the dynamic nature of engagement across task transitions in a way that is more reflective of today’s multifaceted work environment.
Second, in contrast to the predominant view that engagement at the job level is largely beneficial in terms of general work performance (e.g., Christian et al., 2011 ), we find that viewing engagement at the task-level uncovers both positive and negative performance implications. Importantly, we find that the emotional and cognitive components of engagement may have differential effects at the task-level. Although the emotional component of engagement positively spills over to facilitate subsequent task engagement and performance, the cognitive component hinders subsequent task performance by producing attention residue. Our findings—that aspects of engagement may have some drawbacks when viewing how engagement unfolds from one task to the next—respond to scholars who have speculated that engagement may have previously unexplored costs ( George, 2011 ; Sonnentag, 2011 ). Moreover, attention residue provides an explanation for why there may be limits to engaged employees’ energies (e.g., Kim, Park, & Headrick, 2018 ) and what could otherwise be an upward spiral of engagement from one task to another. Indeed, to the extent that engagement in a task is viewed as something that is unequivocally positive with regard to the next task, the upward bounds could have no limits. Thus, the inclusion of attention residue to our understanding of the functioning of task engagement has ecological value and addresses an obvious logical gap with current theory. At the very least, our work presents a more balanced view of engagement that illuminates the performance implications of its bright and dark sides.
Third and relatedly, we provide a more fine-grained perspective of the psychological mechanisms that facilitate or hinder the transfer of individuals’ personal energies and produce these positive and negative effects across task transitions. By unpacking the dynamic nature of task engagement, we “specify more precisely the processes underlying micro role transitions” ( Ashforth, Kreiner, & Fugate, 2000 , p. 486) that occur between the tasks that comprise a work role. On the one hand, our results show that when individuals invest their energies in a task, their efforts generate positive affect which in turn facilitates increased engagement and performance in subsequent tasks. On the other hand, investing in one’s work can also produce attention residue. In contrast to positive affect, attention residue restricts subsequent task engagement and performance as individuals’ focus remains tethered to a previous task. Thus, due to positive affect and attention residue, task engagement appears to have mixed implications for individuals as the mechanisms differentially impact subsequent task engagement and subsequent task performance. By increasing our understanding of how positive affect and attention residue operate in tandem, we provide a view of the task transition phenomenon that is more cohesive. In sum, we provide greater theoretical insight regarding engagement in boundary-crossing activities at the task-level as well as the internal mechanisms that span multiple boundaries.
Finally, our research extends Leroy’s (2009) research on the workplace implications of attention residue. We replicate Leroy’s findings that experiencing attention residue from a prior task can impede performance on a subsequent task. However, we extend prior work by identifying task engagement as an attribute of task work that can intensify the experience of attention residue. These findings are very significant given that “very little is known about predictors of performance variability within persons who are still participating in the workplace” ( Sonnentag & Frese, 2012 , p. 569), particularly with respect to how engagement dynamically impacts performance. Our finding that the association between task 1 engagement and attention residue is weaker when individuals perceive that the prior task is completed is also consistent with Leroy’s (2009) finding that task completion reduces attention residue under conditions of high time pressure. However, the present research is the first to establish that the effects of completion are strong enough to offset the negative cognitive effects of high levels of task 1 engagement. These findings suggest that the beneficial effects of task completion in mitigating attention residue may be even stronger than has been suggested by previous research.
Limitations and Future Research
Although our studies have several theoretical implications, there are also limitations. First, we considered task engagement and its effects over the course of only one task transition episode, and we were unable to capture the far-reaching impact of positive affect and attention residue over multiple transitions. To provide a richer understanding of task engagement, future research could examine these effects over many performance episodes and transitions. This examination could lend additional insight into how individuals invest energies in specific tasks and even how they manage a state of flow over many episodes (e.g., Csikszentmihalyi, 1997 ). Relatedly, in Study 2 we manipulated a boundary condition rather than the independent variable which may reduce our ability to make casual claims. Although we considered manipulating task 1 engagement, we realized that doing so would actually manipulate antecedents of engagement such as task meaningfulness or one’s willingness to invest in the task ( Kahn, 1990 ). Given our Study 1 findings, we took as given that task 1 engagement would be related to task 2 engagement, and instead sought to parse out the mechanisms and boundary conditions that influenced this relationship. Thus, we control for alternative explanations and replicate our findings across two studies which gives us confidence in our theoretical model. In short, we see value in potential studies that track the ebbs and flows of task engagement over many transitions.
Second, our examination of positive affect may neglect other emotions, such as negative affect, that individuals experience across a task transition. Although our focus on positive affect is consistent with Kahn’s (1990) theorizing and empirical measures ( Rich et al., 2010 ), considering the role of negative affect calls to mind research on affect and multiple goal pursuit (e.g., Carver, 2003 ; Carver & Scheier, 1990 ). This research has proposed and found that experiencing negative affect while working on a task can cause individuals to invest more heavily in that task, because it provides a signal that additional resources are needed to complete the task effectively. This research also articulates how positive affect experienced in a task may cause individuals to invest fewer resources because it signals successful task accomplishment. Carver and colleagues’ perspective contrasts with our engagement-based arguments and findings that positive affect helps sustain engagement from task to task. Although our intention was not to compare the multiple goal pursuit perspective with Kahn’s (1990) work, an integration of these perspectives could be a worthwhile endeavor to develop a more nuanced theory of performance in multiple tasks. Such a theory could potentially propose circumstances that influence whether positive affect and negative affect lead to engagement or disengagement by virtue of spillover and goal-related discrepancy cognitions.
Third, there may be value in further examining task engagement and attention residue. Task similarity, or the degree to which a task requires comparable sets of personal energies, is one potential condition. Our study participants may have stayed absorbed in a previous task after switching due to similar energies being required and perceived similarity in our tasks (e.g., Ashforth et al., 2000 ). However, if two tasks are highly similar, attention residue might facilitate rather than hinder a task transition because individuals are in sync with the mode of the first task and would need few cognitive resources to adjust. An interdependence perspective may be one valuable lens to view task similarity (e.g., Bush, LePine, & Newton, 2018 ) to directly determine when switching between individual and team tasks is most beneficial or detrimental.
Considering effective task transitions also evokes task planning ( Earley, Wojnaroski, & Prest, 1987 ) and prospective task engagement wherein individuals look forward to an upcoming task. Although task anticipation may aid in preparing for and performing a future task, it may also occupy individuals’ attention and take their focus away from preceding tasks ( Leroy & Glomb, 2018 ) in a way that impairs previous task performance. In other words, we believe that an examination of how individuals anticipate upcoming tasks to the potential detriment of present tasks could be particularly important to explore. In probing these potential relationships, scholars should be aware that our measure of attention residue is narrower in scope than Leroy and Glomb’s (2018) measure, particularly if their task context involves a wider array of cognitive residue than our study contexts. In short, testing some of the boundary conditions that alter the nature of the relationships implied in our work could be fruitful avenues of inquiry.
Finally, because we focused exclusively on engagement at the task-level, we are unable to explore the implications of task engagement for individuals’ overall job engagement. Although our approach is consistent with the conventional view that tasks are nested within jobs ( Cohen, 2013 ; Ilgen & Hollenbeck, 1991 ; Pearlman, 1980 ), our research also raises the question of how engagement at the two levels is connected. This relationship might take several possible forms. For example, job engagement may reflect the average of individuals’ engagement across all of their tasks. Alternatively, job engagement may be more a function of individuals’ core tasks, the tasks that require the most time, or the tasks that are the most memorable. It may also be that job engagement is a function of the engagement experienced in the task immediately preceding the measurement of job engagement. This latter possibility is consistent with the notion of residual engagement, and has implications regarding when job engagement should be measured. Subsequent research that explores these and related possibilities by bridging the task-level and job-level aspects of engagement would further advance engagement theory.
Practical Implications
Our work has several implications for practice. Organizational leaders desire an engaged workforce (e.g., Morgan, 2017 ; Saks & Gruman, 2014 ), and our empirical findings offer some ideas as to how organizations may address employee task engagement. Individuals in organizations would do well to take part in intentional task planning that accentuates the positive effects of task engagement through positive affect and mitigates the negative effects of task engagement through attention residue. For example, many employees begin their workday by focusing their energies on tasks that are often less than engaging (e.g., checking email), and beginning with a less engaging task may not lead employees to start their day “off on the right foot.” Instead, our findings suggest that when individuals invest their energies in an engaging task then they not only experience positive feelings, but are also more engaged in a subsequent task and perform that subsequent task more effectively. Thus, organizations may find value in encouraging employees to deliberately plan and prioritize their workdays so that they begin with an engaging task and thereby reap the positive—and potentially multiplicative—benefits of task engagement in their subsequent task activities ( Salanova et al., 2011 ). Of course, our findings point to an important caveat in scheduling engaging tasks early in the day. Specifically, it may be important to select tasks where some degree of completeness can be reached prior to a task transition. Not being able to finish an engaging task elevates attention residue that dampens the positive effects from the engaging task. In some jobs—such as those involving project work—engaging tasks are often on-going, which makes “completing” the task difficult. In these cases, it may be possible to segment the work into smaller defined chunks (i.e., sets of related activities with defined objective) and setting aside time to achieve them so that a sense of closure can be reached and the positive effects of task engagement can be most pronounced.
Individuals who desire to perform effectively may need to balance how they allocate their energies across the many tasks that are required in their job. It may be helpful for individuals to identify an optimal number of tasks that they can transition between before the availability of their cognitive energies is spent (e.g., Kahn, 1990 ). Organizational leaders could be aware of the demands they put on their employees, and could help structure or support task activities in a way that maximizes the positive transfer of engagement across tasks. For example, organizations may find value in a mental thought exercise that enables employees to close the door mentally on a prior engaging task even if it is incomplete. As another example, instead of interrupting employees and immediately demanding their attention, managers could allow employees to wrap up a current task so that employees can be fully attentive to a manager’s request. Finally, managers may desire to temper their performance expectations on highly engaging tasks that occur back-to-back, or may find it valuable to help employees in these instances so that subsequent engagement and performance do not suffer.
We offer a task-level view of engagement to understand how the motivation pinned to the various tasks that comprise a multifaceted job are connected to each other and to performance. More plainly, we explain how task engagement influences performance in a subsequent task, through engagement in the subsequent task. We show that task engagement is associated with positive affect, and in turn, engagement and performance in a subsequent task. We also show that task engagement is associated with attention residue, which in turn, impedes subsequent task engagement and performance. Finally, we show that the negative effect of attention residue is ameliorated when a task is viewed as complete. In articulating the positive and negative pathways through which task engagement operates, we present a balanced view of engagement. Our moderator suggests how the dark side of engagement in multifaceted work can be managed.
Acknowledgments
This research is supported by The National Aeronautics and Space Administration (NASA), grant NNX15AK77G awarded to the first, second, and fourth authors.
Earlier versions of this article were presented at the annual meeting of the Academy of Management, the Association for Psychological Science annual convention, the Interdisciplinary Network of Group Research conference, and NASA’s Human Research Program Investigators’ Workshop.
1 Given the importance of distinguishing task engagement from job engagement for our theoretical arguments, we took an additional step to ensure that participants were rating their levels of engagement in each task . Specifically, we examined participants’ open-ended comments regarding the experiment, and counted the number of times participants mentioned the terms “task” and “job.” The word job came up in 7 participant responses (out of 346), and the term was used generically to refer to performance or how team members filled different roles in the firefighting task (e.g., “Overall our team did a really good job” and “Everyone should do their own job well in a group”). In contrast, the word task came up in 45 participant responses and was used most often in reference to the two experimental activities and switching between them (e.g., “completing a task and then working on a different task” or “switching from multiple tasks”). Combined with our experimental protocol that explicitly described the firefighting simulation and Lego truck assembly as different tasks that comprise the firefighter job, we are confident that our items accurately captured participants’ engagement at the task-level.
2 Our test is similar to the Wonderlic with respect to the types of items. We administered the test we developed, along with the Wonderlic, to 73 undergraduate students [81% were male, 60% were Caucasian, and their average age was 22.6 ( SD = 2.72)]. We alternated between administering our GMA test first and the Wonderlic first, and found that scores on our GMA test are correlated with scores on the Wonderlic at .74, which gives us assurance that our test is tapping participants’ GMA the same way the Wonderlic is. The magnitude of the correlation is about what we expect given research on alternative test forms reliability (e.g., Coyle, 2006 ). It is important to note that this correlation is commensurate or stronger than the correlation between scores on the Wonderlic and scores on other standardized tests that have been used as an indicator of GMA (e.g., Coyle, 2006 ; Coyle & Pillow, 2008 ; Frederick, 2005 ).
- Aiken LS, & West SG (1991). Multiple regression: Testing and interpreting interactions . Newbury Park, CA: Sage Publications. [ Google Scholar ]
- Ancona D, & Chong CL (1996). Entrainment: Pace, cycle, and rhythm in organizational behavior . Research in Organizational Behavior , 18 , 251–284. [ Google Scholar ]
- Ashforth BE, Kreiner GE, & Fugate M (2000). All in a day’s work: Boundaries and micro role transitions . Academy of Management Review , 25 ( 3 ), 472–491. doi: 10.5465/AMR.2000.3363315 [ CrossRef ] [ Google Scholar ]
- Aubé C, & Rousseau V (2005). Team goal commitment and team effectiveness: The role of task interdependence and supportive behaviors . Group Dynamics: Theory, Research, and Practice , 9 ( 3 ), 189–204. doi: 10.1037/1089-2699.9.3.189 [ CrossRef ] [ Google Scholar ]
- Bakker AB (2014). Daily fluctuations in work engagement . European Psychologist , 19 , 227–236. doi: 10.1027/1016-9040/a000160 [ CrossRef ] [ Google Scholar ]
- Bakker AB, & Bal MP (2010). Weekly work engagement and performance: A study among starting teachers . Journal of Occupational and Organizational Psychology , 83 ( 1 ), 189–206. doi: 10.1348/096317909X402596 [ CrossRef ] [ Google Scholar ]
- Bauer DJ, Preacher KJ, & Gil KM (2006). Conceptualizing and testing random indirect effects and moderated mediation in multilevel models: New procedures and recommendations . Psychological Methods , 11 ( 2 ), 142–163. doi: 10.1037/1082-989X.11.2.142 [ PubMed ] [ CrossRef ] [ Google Scholar ]
- Beal DJ, Weiss HM, Barros E, & MacDermid SM (2005). An episodic process model of affective influences on performance . Journal of Applied Psychology , 90 ( 6 ), 1054–1068. doi: 10.1037/0021-9010.90.6.1054 [ PubMed ] [ CrossRef ] [ Google Scholar ]
- Bernerth JB, & Aguinis H (2016). A critical review and best-practice recommendations for control variable usage . Personnel Psychology , 69 ( 1 ), 229–283. doi: 10.1111/peps.12103 [ CrossRef ] [ Google Scholar ]
- Blakemore JEO, & Centers RE (2005). Characteristics of boys’ and girls’ toys . Sex Roles , 53 ( 9–10 ), 619–633. doi: 10.1007/s11199-005-7729-0 [ CrossRef ] [ Google Scholar ]
- Bledow R, Schmitt A, Frese M, & Kühnel J (2011). The affective shift model of work engagement . Journal of Applied Psychology , 96 ( 6 ), 1246–1257. doi: 10.1037/a0024532 [ PubMed ] [ CrossRef ] [ Google Scholar ]
- Bommer WH, Johnson JL, Rich GA, Podsakoff PM, & MacKenzie SB (1995). On the interchangeability of objective and subjective measures of employee performance: A meta-analysis . Personnel Psychology , 48 ( 3 ), 587–605. doi: 10.1111/j.17446570.1995.tb01772.x [ CrossRef ] [ Google Scholar ]
- Bush JT, LePine JA, & Newton DW (2018). Teams in transition: An integrative review and synthesis of research on team task transitions and propositions for future research . Human Resource Management Review , 28 ( 4 ), 423–433. doi: 10.1016/j.hrmr.2017.06.005 [ CrossRef ] [ Google Scholar ]
- Byrne ZS, Peters JM, & Weston JW (2016). The struggle with employee engagement: Measures and construct clarification using five samples . Journal of Applied Psychology , 101 ( 9 ), 1201–1227. doi: 10.1037/apl0000124 [ PubMed ] [ CrossRef ] [ Google Scholar ]
- Carver CS (2003). Pleasure as a sign you can attend to something else: Placing positive feelings within a general model of affect . Cognition & Emotion , 17 ( 2 ), 241–261. doi: 10.1080/02699930244000291 [ PubMed ] [ CrossRef ] [ Google Scholar ]
- Carver CS, & Scheier MF (1990). Origins and functions of positive and negative affect: A control-process view . Psychological Review , 97 ( 1 ), 19–35. doi: 10.1037/0033-295X.97.1.19 [ CrossRef ] [ Google Scholar ]
- Cellier J-M, & Eyrolle H (1992). Interference between switched tasks . Ergonomics , 35 ( 1 ), 25–36. doi: 10.1080/00140139208967795 [ CrossRef ] [ Google Scholar ]
- Chen G, & Mathieu JE (2008). Goal orientation dispositions and performance trajectories: The roles of supplementary and complementary situational inducements . Organizational Behavior and Human Decision Processes , 106 ( 1 ), 21–38. doi: 10.1016/j.obhdp.2007.11.001 [ CrossRef ] [ Google Scholar ]
- Christian MS, Garza AS, & Slaughter JE (2011). Work engagement: A quantitative review and test of its relations with task and contextual performance . Personnel Psychology , 64 ( 1 ), 89–136. doi: 10.1111/j.1744-6570.2010.01203.x [ CrossRef ] [ Google Scholar ]
- Cohen LE (2013). Assembling jobs: A model of how tasks are bundled into and across jobs . Organization Science , 24 ( 2 ), 432–454. doi: 10.1287/orsc.1110.0737 [ CrossRef ] [ Google Scholar ]
- Coulson JC, McKenna J, & Field M (2008). Exercising at work and self-reported work performance . International Journal of Workplace Health Management , 1 ( 3 ), 176–197. doi: 10.1108/17538350810926534 [ CrossRef ] [ Google Scholar ]
- Coyle TR (2006). Test–retest changes on scholastic aptitude tests are not related to g . Intelligence , 34 ( 1 ), 15–27. doi: 10.1016/j.intell.2005.04.001 [ CrossRef ] [ Google Scholar ]
- Coyle TR, & Pillow DR (2008). SAT and ACT predict college GPA after removing g . Intelligence , 36 ( 6 ), 719–729. doi: 10.1016/j.intell.2008.05.001 [ CrossRef ] [ Google Scholar ]
- Crane BD, Crawford ER, Buckman BR, & LePine JA (2017). More than words: The influence of leaders’ questions on follower role identities and engagement .
- Crawford ER, LePine JA, & Buckman BR (2013). Job engagement scale short form .
- Csikszentmihalyi M (1997). Finding flow: The psychology of engagement with everyday life . New York: Basic Books. [ Google Scholar ]
- Culbertson SS, Mills MJ, & Fullagar CJ (2012). Work engagement and work-family facilitation: Making homes happier through positive affective spillover . Human Relations , 65 ( 9 ), 1155–1177. doi: 10.1177/0018726712440295 [ CrossRef ] [ Google Scholar ]
- Dane E (2018). Where is my mind? Theorizing mind wandering and its performance-related consequences in organizations . Academy of Management Review , 43 ( 2 ), 179–197. doi: 10.5465/amr.2015.0196 [ CrossRef ] [ Google Scholar ]
- Diefendorff JM, & Chandler MM (2011). Motivating employees. In Zedeck S (Ed.), APA handbook of industrial and organizational psychology (Vol. 3 , pp. 65–135). Washington, DC: American Psychological Association. [ Google Scholar ]
- D’Mello S, & Graesser A (2011). The half-life of cognitive-affective states during complex learning . Cognition and Emotion , 25 ( 7 ), 1299–1308. doi: 10.1080/02699931.2011.613668 [ PubMed ] [ CrossRef ] [ Google Scholar ]
- Earley PC, Wojnaroski P, & Prest W (1987). Task planning and energy expended: Exploration of how goals influence performance . Journal of Applied Psychology , 72 ( 1 ), 107–114. doi: 10.1037/0021-9010.72.1.107 [ CrossRef ] [ Google Scholar ]
- Elsbach KD, & Hargadon AB (2006). Enhancing creativity through “mindless” work: A framework of workday design . Organization Science , 17 ( 4 ), 470–483. doi: 10.1287/orsc.1060.0193 [ CrossRef ] [ Google Scholar ]
- Erez A, & Isen AM (2002). The influence of positive affect on the components of expectancy motivation . Journal of Applied Psychology , 87 ( 6 ), 1055–1067. doi: 10.1037/0021-9010.87.6.1055 [ PubMed ] [ CrossRef ] [ Google Scholar ]
- Fisher CD (2003). Why do lay people believe that satisfaction and performance are correlated? Possible sources of a commonsense theory . Journal of Organizational Behavior , 24 ( 6 ), 753–777. doi: 10.1002/job.219 [ CrossRef ] [ Google Scholar ]
- Flanagan JC (1954). The critical incident technique . Psychological Bulletin , 51 ( 4 ), 327–358. doi: 10.1037/h0061470 [ PubMed ] [ CrossRef ] [ Google Scholar ]
- Fletcher L, Bailey C, & Gilman MW (2018). Fluctuating levels of personal role engagement within the working day: A multilevel study . Human Resource Management Journal , 28 ( 1 ), 128–147. doi: 10.1111/1748-8583.12168 [ CrossRef ] [ Google Scholar ]
- Fornell C, & Larcker DF (1981). Evaluating structural equation models with unobservable variables and measurement error . Journal of Marketing Research , 18 ( 1 ), 39–50. doi: 10.2307/3150980 [ CrossRef ] [ Google Scholar ]
- Frederick S (2005). Cognitive reflection and decision making . Journal of Economic Perspectives , 19 ( 4 ), 25–42. doi: 10.1257/089533005775196732 [ CrossRef ] [ Google Scholar ]
- Fredrickson BL (2001). The role of positive emotions in positive psychology: The broaden-and-build theory of positive emotions . American Psychologist , 56 ( 3 ), 218–226. [ PMC free article ] [ PubMed ] [ Google Scholar ]
- Fredrickson BL (2004). The broaden-and-build theory of positive emotions . Philosophical Transactions of the Royal Society B: Biological Sciences , 359 ( 1449 ), 1367–1377. [ PMC free article ] [ PubMed ] [ Google Scholar ]
- Freeman N, & Muraven M (2010). Don’t interrupt me! Task interruption depletes the self’s limited resources . Motivation and Emotion , 34 ( 3 ), 230–241. doi: 10.1007/s11031-010-9169-6 [ CrossRef ] [ Google Scholar ]
- George JM (2011). The wider context, costs, and benefits of work engagement . European Journal of Work and Organizational Psychology , 20 ( 1 ), 53–59. doi: 10.1080/1359432X.2010.509924 [ CrossRef ] [ Google Scholar ]
- George JM, & Brief AP (1996). Motivational agendas in the workplace: The effects of feelings on focus of attention and work motivation. In Staw B & Cummings LL (Eds.), Research in Organizational Behavior: An annual series of analytical essays and critical reviews (pp. 75–109). Greenwich, CT: JAI Press. [ Google Scholar ]
- Goldberg LR, Johnson JA, Eber HW, Hogan R, Ashton MC, Cloninger CR, & Gough HG (2006). The international personality item pool and the future of public-domain personality measures . Journal of Research in Personality , 40 ( 1 ), 84–96. doi: 10.1016/j.jrp.2005.08.007 [ CrossRef ] [ Google Scholar ]
- Grant AM, & Parker SK (2009). Redesigning work design theories: The rise of relational and proactive perspectives . The Academy of Management Annals , 3 ( 1 ), 317–375. doi: 10.1080/19416520903047327 [ CrossRef ] [ Google Scholar ]
- Gruman JA, & Saks AM (2011). Performance management and employee engagement . Human Resource Management Review , 21 ( 2 ), 123–136. doi: 10.1016/j.hrmr.2010.09.004 [ CrossRef ] [ Google Scholar ]
- Harrison S, & Wagner DT (2016). Spilling outside the box: The effects of individuals’ creative behaviors at work on time spent with their spouses at home . Academy of Management Journal , 59 ( 3 ), 841–859. doi: 10.5465/amj.2013.0560 [ CrossRef ] [ Google Scholar ]
- Harter J, Schmidt F, Killham E, & Agrawal S (2009). Q12® MetaAnalysis: The relationship between engagement at work and organizational outcomes . Gallup Organization. [ Google Scholar ]
- Hasan S, Ferguson J-P, & Koning R (2015). The lives and deaths of jobs: Technical interdependence and survival in a job structure . Organization Science , 26 ( 6 ), 1665–1681. doi: 10.1287/orsc.2015.1014 [ CrossRef ] [ Google Scholar ]
- Hollenbeck JR, Ellis AP, Humphrey SE, Garza AS, & Ilgen DR (2011). Asymmetry in structural adaptation: The differential impact of centralizing versus decentralizing team decision-making structures . Organizational Behavior and Human Decision Processes , 114 ( 1 ), 64–74. doi: 10.1016/j.obhdp.2010.08.003 [ CrossRef ] [ Google Scholar ]
- Hülsheger UR (2016). From dawn till dusk: Shedding light on the recovery process by investigating daily change patterns in fatigue . Journal of Applied Psychology , 101 ( 6 ), 905–914. doi: 10.1037/apl0000104 [ PubMed ] [ CrossRef ] [ Google Scholar ]
- Ilgen DR, & Hollenbeck JR (1991). The structures of work: job design and roles Handbook of industrial and organizational psychology (Vol. 2 , pp. 165–207). Palo Alto, CA: Consulting Psychologists Press. [ Google Scholar ]
- Isen AM, & Reeve J (2005). The influence of positive affect on intrinsic and extrinsic motivation: Facilitating enjoyment of play, responsible work behavior, and self-control . Motivation and Emotion , 29 ( 4 ), 297–325. doi: 10.1007/s11031-006-9019-8 [ CrossRef ] [ Google Scholar ]
- Isen AM, Shalker TE, Clark M, & Karp L (1978). Affect, accessibility of material in memory, and behavior: A cognitive loop? Journal of Personality and Social Psychology , 36 ( 1 ), 1–12. doi: 10.1037/0022-3514.36.1.1 [ PubMed ] [ CrossRef ] [ Google Scholar ]
- James W (1890). The Principles of Psychology . New York: Dover. [ Google Scholar ]
- Johansson BJ, Trnka J, Granlund R, & Götmar A (2010). The effect of a geographical information system on performance and communication of a command and control organization . Intl. Journal of Human–Computer Interaction , 26 ( 2–3 ), 228–246. doi: 10.1080/10447310903498981 [ CrossRef ] [ Google Scholar ]
- Kahn WA (1990). Psychological conditions of personal engagement and disengagement at work . Academy of Management Journal , 33 ( 4 ), 692–724. doi: 10.2307/256287 [ CrossRef ] [ Google Scholar ]
- Kanfer R, & Ackerman PL (1989). Motivation and cognitive abilities: An integrative/aptitude-treatment interaction approach to skill acquisition . Journal of Applied Psychology , 74 ( 4 ), 657–690. doi: 10.1037/0021-9010.74.4.657 [ CrossRef ] [ Google Scholar ]
- Kelly JR, & McGrath JE (1985). Effects of time limits and task types on task-performance and interaction of 4-person groups . Journal of Personality and Social Psychology , 49 ( 2 ), 395–407. doi: 10.1037/0022-3514.49.2.395 [ CrossRef ] [ Google Scholar ]
- Kiesel A, Steinhauser M, Wendt M, Falkenstein M, Jost K, Philipp AM, & Koch I (2010). Control and interference in task switching: A review . Psychological Bulletin , 136 ( 5 ), 849–874. doi: 10.1037/a0019842 [ PubMed ] [ CrossRef ] [ Google Scholar ]
- Kim S, Park Y, & Headrick L (2018). Daily micro-breaks and job performance: General work engagement as a cross-level moderator . Journal of Applied Psychology , 103 ( 7 ), 772–786. doi: 10.1037/apl0000308 [ PubMed ] [ CrossRef ] [ Google Scholar ]
- Kreiner GE, Hollensbe EC, & Sheep ML (2006). Where is the” me” among the” we”? Identity work and the search for optimal balance . Academy of Management Journal , 49 ( 5 ), 1031–1057. doi: 10.5465/amj.2006.22798186 [ CrossRef ] [ Google Scholar ]
- Kühnel J, Sonnentag S, & Westman M (2009). Does work engagement increase after a short respite? The role of job involvement as a double-edged sword . Journal of Occupational and Organizational Psychology , 82 ( 3 ), 575–594. doi: 10.1348/096317908X349362 [ CrossRef ] [ Google Scholar ]
- Kushlev K, & Dunn EW (2015). Checking email less frequently reduces stress . Computers in Human Behavior , 43 , 220–228. doi: 10.1016/j.chb.2014.11.005 [ CrossRef ] [ Google Scholar ]
- Leroy S (2009). Why is it so hard to do my work? The challenge of attention residue when switching between work tasks . Organizational Behavior and Human Decision Processes , 109 ( 2 ), 168–181. doi: 10.1016/j.obhdp.2009.04.002 [ CrossRef ] [ Google Scholar ]
- Leroy S, & Glomb TM (2018). Tasks interrupted: How anticipating time pressure on resumption of an interrupted task causes attention residue and low performance on interrupting tasks and how a “ready-to-resume” plan mitigates the effects . Organization Science , 29 ( 3 ), 380–397. doi: 10.1287/orsc.2017.1184 [ CrossRef ] [ Google Scholar ]
- Leroy S, & Schmidt AM (2016). The effect of regulatory focus on attention residue and performance during interruptions . Organizational Behavior and Human Decision Processes , 137 , 218–235. doi: 10.1016/j.obhdp.2016.07.006 [ CrossRef ] [ Google Scholar ]
- Levinson DB, Smallwood J, & Davidson RJ (2012). The persistence of thought: Evidence for a role of working memory in the maintenance of task-unrelated thinking . Psychological Science , 23 ( 4 ), 375–380. doi: 10.1177/0956797611431465 [ PMC free article ] [ PubMed ] [ CrossRef ] [ Google Scholar ]
- Lewin K (1935). Dynamic theory of personality . New York: McGraw-Hill. [ Google Scholar ]
- Louis MR, & Sutton RI (1991). Switching cognitive gears: From habits of mind to active thinking . Human Relations , 44 ( 1 ), 55–76. doi: 10.1177/001872679104400104 [ CrossRef ] [ Google Scholar ]
- Macey WH, Schneider B, Barbera KM, & Young SA (2009). Employee engagement: Tools for analysis, practice, and competitive advantage . Chichester, United Kingdom: John Wiley & Sons. [ Google Scholar ]
- MacKinnon DP, Fairchild AJ, & Fritz MS (2007). Mediation analysis . Annual Review of Psychology , 58 , 593–614. doi: 10.1146/annurev.psych.58.110405.085542 [ PMC free article ] [ PubMed ] [ CrossRef ] [ Google Scholar ]
- MacKinnon DP, Lockwood CM, Hoffman JM, West SG, & Sheets V (2002). A comparison of methods to test mediation and other intervening variable effects . Psychological Methods , 7 ( 1 ), 83–104. doi: 10.1037/1082-989x.7.1.83 [ PMC free article ] [ PubMed ] [ CrossRef ] [ Google Scholar ]
- McCrae RR, & Costa PT Jr (1997). Conceptions and correlates of openness to experience. In Hogan R, Johnson J & Briggs S (Eds.), Handbook of Personality Psychology (pp. 825–847). San Diego, CA: Academic Press. [ Google Scholar ]
- Meade AW, & Craig SB (2012). Identifying careless responses in survey data . Psychological Methods , 17 ( 3 ), 437–455. doi: 10.1037/a0028085 [ PubMed ] [ CrossRef ] [ Google Scholar ]
- Morgan J (2017). Why the millions we spend on employee engagement buy us so little . Harvard Business Review. Retrieved from https://hbr.org [ Google Scholar ]
- Muthén LK, & Muthén BO (2015). Mplus 7.4 . Los Angeles, CA: Muthén & Muthén. [ Google Scholar ]
- Naylor JC, Pritchard RD, & Ilgen DR (1980). A theory of behavior in organizations . New York: Academic Press. [ Google Scholar ]
- Nemanick R, & Munz DC (1997). Extraversion and neuroticism, trait mood, and state affect: A hierarchical relationship? Journal of Social Behavior and Personality , 12 ( 4 ), 1079–1092. [ Google Scholar ]
- O’Reilly K, Paper D, & Marx S (2012). Demystifying grounded theory for business research . Organizational Research Methods , 15 ( 2 ), 247–262. doi: 10.1177/1094428111434559 [ CrossRef ] [ Google Scholar ]
- Pearlman K (1980). Job families: A review and discussion of their implications for personnel selection . Psychological Bulletin , 87 ( 1 ), 1–28. doi: 10.1037/0033-2909.87.1.1 [ CrossRef ] [ Google Scholar ]
- Podsakoff PM, MacKenzie SB, Lee JY, & Podsakoff NP (2003). Common method biases in behavioral research: A critical review of the literature and recommended remedies . Journal of Applied Psychology , 88 ( 5 ), 879–903. doi: 10.1037/0021-9101.88.5.879 [ PubMed ] [ CrossRef ] [ Google Scholar ]
- Preacher KJ, Rucker DD, & Hayes AF (2007). Addressing moderated mediation hypotheses: Theory, methods, and prescriptions . Multivariate Behavioral Research , 42 ( 1 ), 185–227. doi: 10.1080/00273170701341316 [ PubMed ] [ CrossRef ] [ Google Scholar ]
- Preacher KJ, Zyphur MJ, & Zhang Z (2010). A general multilevel SEM framework for assessing multilevel mediation . Psychological Methods , 15 ( 3 ), 209–233. doi: 10.1037/a0020141 [ PubMed ] [ CrossRef ] [ Google Scholar ]
- Randall JG, Oswald FL, & Beier ME (2014). Mind-wandering, cognition, and performance: A theory-driven meta-analysis of attention regulation . Psychological Bulletin , 140 ( 6 ), 1411–1431. doi: 10.1037/a0037428 [ PubMed ] [ CrossRef ] [ Google Scholar ]
- Raudenbush SW, & Bryk AS (2002). Hierarchical linear models: Applications and data analysis methods (2 ed.). Thousand Oaks, CA: Sage Publications. [ Google Scholar ]
- Rich BL, LePine JA, & Crawford ER (2010). Job engagement: Antecedents and effects on job performance . Academy of Management Journal , 53 ( 3 ), 617–635. doi: 10.5465/AMJ.2010.51468988 [ CrossRef ] [ Google Scholar ]
- Richardson HA, & Taylor SG (2012). Understanding input events: A model of employees’ responses to requests for their input . Academy of Management Review , 37 ( 3 ), 471–491. doi: 10.5465/amr.2010.0327 [ CrossRef ] [ Google Scholar ]
- Rodríguez-Muñoz A, Sanz-Vergel AI, Demerouti E, & Bakker AB (2014). Engaged at work and happy at home: A spillover–crossover model . Journal of Happiness Studies , 15 ( 2 ), 271–283. doi: 10.1007/s10902-013-9421-3 [ CrossRef ] [ Google Scholar ]
- Rothbard NP (2001). Enriching or depleting? The dynamics of engagement in work and family roles . Administrative Science Quarterly , 46 ( 4 ), 655–684. doi: 10.2307/3094827 [ CrossRef ] [ Google Scholar ]
- Saks AM, & Gruman JA (2014). What do we really know about employee engagement? Human Resource Development Quarterly , 25 ( 2 ), 155–182. doi: 10.1002/hrdq.21187 [ CrossRef ] [ Google Scholar ]
- Salanova M, Bakker AB, & Llorens S (2006). Flow at work: Evidence for an upward spiral of personal and organizational resources . Journal of Happiness Studies , 7 ( 1 ), 1–22. doi: 10.1007/s10902-005-8854-8 [ CrossRef ] [ Google Scholar ]
- Salanova M, Llorens S, & Schaufeli WB (2011). “Yes, I can, I feel good, and I just do it!” On gain cycles and spirals of efficacy beliefs, affect, and engagement . Applied Psychology , 60 ( 2 ), 255–285. doi: 10.1111/j.1464-0597.2010.00435.x [ CrossRef ] [ Google Scholar ]
- Salanova M, & Schaufeli WB (2008). A cross-national study of work engagement as a mediator between job resources and proactive behaviour . The International Journal of Human Resource Management , 19 ( 1 ), 116–131. doi: 10.1080/09585190701763982 [ CrossRef ] [ Google Scholar ]
- Schaufeli WB, & Bakker AB (2004). Job demands, job resources, and their relationship with burnout and engagement: A multi-sample study . Journal of Organizational Behavior , 25 ( 3 ), 293–315. doi: 10.1002/job.248 [ CrossRef ] [ Google Scholar ]
- Schaufeli WB, Salanova M, González-Romá V, & Bakker AB (2002). The measurement of engagement and burnout: A two sample confirmatory factor analytic approach . Journal of Happiness Studies , 3 ( 1 ), 71–92. doi: 10.1023/A:1015630930326 [ CrossRef ] [ Google Scholar ]
- Schmidt AM, & DeShon RP (2009). Prior performance and goal progress as moderators of the relationship between self-efficacy and performance . Human Performance , 22 ( 3 ), 191–203. doi: 10.1080/08959280902970377 [ CrossRef ] [ Google Scholar ]
- Schmidt AM, & DeShon RP (2010). The moderating effects of performance ambiguity on the relationship between self-efficacy and performance . Journal of Applied Psychology , 95 ( 3 ), 572–581. doi: 10.1037/a0018289 [ PubMed ] [ CrossRef ] [ Google Scholar ]
- Shin J, & Grant AM (2018). Bored by interest: Intrinsic motivation in one task can reduce performance on other tasks . Academy of Management Journal . doi: 10.5465/amj.2017.0735 [ CrossRef ] [ Google Scholar ]
- Sonnentag S (2011). Research on work engagement is well and alive . European Journal of Work and Organizational Psychology , 20 ( 1 ), 29–38. doi: 10.1080/1359432X.2010.510639 [ CrossRef ] [ Google Scholar ]
- Sonnentag S (2012). Time in organizational research: Catching up on a long neglected topic in order to improve theory . Organizational Psychology Review , 2 ( 4 ), 361–368. doi: 10.1177/2041386612442079 [ CrossRef ] [ Google Scholar ]
- Sonnentag S, & Frese M (2012). Dynamic performance. In Kozlowski SW (Ed.), The Oxford handbook of organizational psychology (Vol. 1 , pp. 548–575). Oxford: Oxford UP. [ Google Scholar ]
- Verduyn P, Van Mechelen I, & Tuerlinckx F (2011). The relation between event processing and the duration of emotional experience . Emotion , 11 ( 1 ), 20–28. doi: 10.1037/a0021239 [ PubMed ] [ CrossRef ] [ Google Scholar ]
- Wagner DT, Barnes CM, Lim VK, & Ferris DL (2012). Lost sleep and cyberloafing: Evidence from the laboratory and a daylight saving time quasi-experiment . Journal of Applied Psychology , 97 ( 5 ), 1068–1076. doi: 10.1037/a0027557 [ PubMed ] [ CrossRef ] [ Google Scholar ]
- Watson D (1988). Intraindividual and interindividual analyses of positive and negative affect: Their relation to health complaints, perceived stress, and daily activities . Journal of Personality and Social Psychology , 54 ( 6 ), 1020–1030. doi: 10.1037/0022-3514.54.6.1020 [ PubMed ] [ CrossRef ] [ Google Scholar ]
- Watson D, & Clark LA (1994). Manual for the positive and negative affect schedule (expanded form) . University of Iowa. Iowa City, IA. [ Google Scholar ]
- Watson D, & Clark LA (1997). Measurement and mismeasurement of mood: Recurrent and emergent issues . Journal of Personality Assessment , 68 ( 2 ), 267–296. doi: 10.1207/s15327752jpa6802_4 [ PubMed ] [ CrossRef ] [ Google Scholar ]
- Watson D, Clark LA, & Tellegen A (1988). Development and validation of brief measures of positive and negative affect: The PANAS scales . Journal of Personality and Social Psychology , 54 ( 6 ), 1063–1070. [ PubMed ] [ Google Scholar ]
- Webster DM, & Kruglanski AW (1994). Individual differences in need for cognitive closure . Journal of Personality and Social Psychology , 67 ( 6 ), 1049–1062. doi: 10.1037/0022-3514.67.6.1049 [ PubMed ] [ CrossRef ] [ Google Scholar ]
- Xanthopoulou D, Bakker AB, Demerouti E, & Schaufeli WB (2009). Work engagement and financial returns: A diary study on the role of job and personal resources . Journal of Occupational and Organizational Psychology , 82 ( 1 ), 183–200. doi: 10.1348/096317908X285633 [ CrossRef ] [ Google Scholar ]
- Zeigarnik B (1967). On finished and unfinished tasks. In Ellis WD (Ed.), A source book of Gestalt psychology (Vol. 1 , pp. 300–314). New York: Humanities Press. [ Google Scholar ]
- Zenger J, & Folkman J (2017). How managers drive results and employee engagement at the same time . Harvard Business Review. Retrieved from https://hbr.org [ Google Scholar ]
Join thousands of product people at Insight Out Conf on April 11. Register free.
Insights hub solutions
Analyze data
Uncover deep customer insights with fast, powerful features, store insights, curate and manage insights in one searchable platform, scale research, unlock the potential of customer insights at enterprise scale.
Featured reads
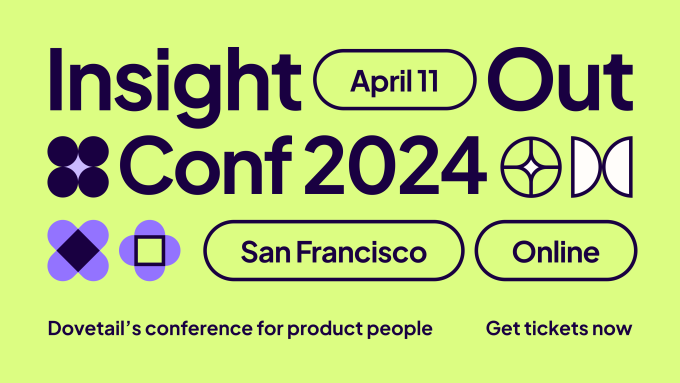
Inspiration
Three things to look forward to at Insight Out
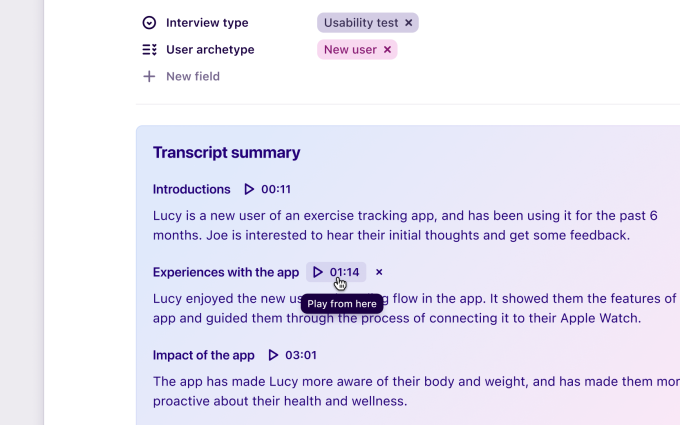
Tips and tricks
Make magic with your customer data in Dovetail
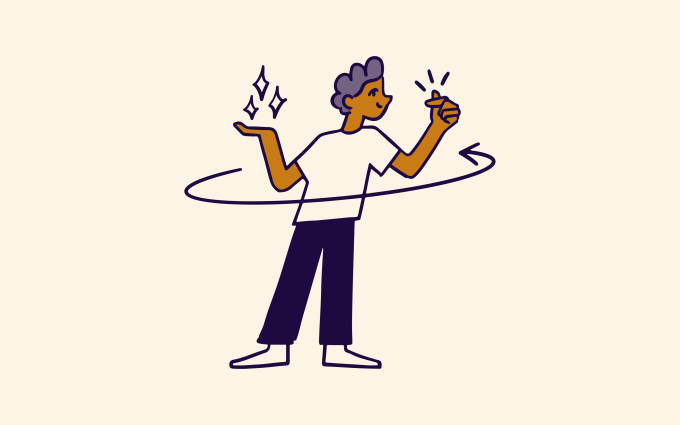
Four ways Dovetail helps Product Managers master continuous product discovery
Events and videos
© Dovetail Research Pty. Ltd.
How to write a research plan: Step-by-step guide
Last updated
30 January 2024
Reviewed by
Today’s businesses and institutions rely on data and analytics to inform their product and service decisions. These metrics influence how organizations stay competitive and inspire innovation. However, gathering data and insights requires carefully constructed research, and every research project needs a roadmap. This is where a research plan comes into play.
There’s general research planning; then there’s an official, well-executed research plan. Whatever data-driven research project you’re gearing up for, the research plan will be your framework for execution. The plan should also be detailed and thorough, with a diligent set of criteria to formulate your research efforts. Not including these key elements in your plan can be just as harmful as having no plan at all.
Read this step-by-step guide for writing a detailed research plan that can apply to any project, whether it’s scientific, educational, or business-related.
- What is a research plan?
A research plan is a documented overview of a project in its entirety, from end to end. It details the research efforts, participants, and methods needed, along with any anticipated results. It also outlines the project’s goals and mission, creating layers of steps to achieve those goals within a specified timeline.
Without a research plan, you and your team are flying blind, potentially wasting time and resources to pursue research without structured guidance.
The principal investigator, or PI, is responsible for facilitating the research oversight. They will create the research plan and inform team members and stakeholders of every detail relating to the project. The PI will also use the research plan to inform decision-making throughout the project.
- Why do you need a research plan?
Create a research plan before starting any official research to maximize every effort in pursuing and collecting the research data. Crucially, the plan will model the activities needed at each phase of the research project.
Like any roadmap, a research plan serves as a valuable tool providing direction for those involved in the project—both internally and externally. It will keep you and your immediate team organized and task-focused while also providing necessary definitions and timelines so you can execute your project initiatives with full understanding and transparency.
External stakeholders appreciate a working research plan because it’s a great communication tool, documenting progress and changing dynamics as they arise. Any participants of your planned research sessions will be informed about the purpose of your study, while the exercises will be based on the key messaging outlined in the official plan.
Here are some of the benefits of creating a research plan document for every project:
Project organization and structure
Well-informed participants
All stakeholders and teams align in support of the project
Clearly defined project definitions and purposes
Distractions are eliminated, prioritizing task focus
Timely management of individual task schedules and roles
Costly reworks are avoided
- What should a research plan include?
The different aspects of your research plan will depend on the nature of the project. However, most official research plan documents will include the core elements below. Each aims to define the problem statement, devising an official plan for seeking a solution.
Specific project goals and individual objectives
Ideal strategies or methods for reaching those goals
Required resources
Descriptions of the target audience, sample sizes, demographics, and scopes
Key performance indicators (KPIs)
Project background
Research and testing support
Preliminary studies and progress reporting mechanisms
Cost estimates and change order processes
Depending on the research project’s size and scope, your research plan could be brief—perhaps only a few pages of documented plans. Alternatively, it could be a fully comprehensive report. Either way, it’s an essential first step in dictating your project’s facilitation in the most efficient and effective way.
- How to write a research plan for your project
When you start writing your research plan, aim to be detailed about each step, requirement, and idea. The more time you spend curating your research plan, the more precise your research execution efforts will be.
Account for every potential scenario, and be sure to address each and every aspect of the research.
Consider following this flow to develop a great research plan for your project:
Define your project’s purpose
Start by defining your project’s purpose. Identify what your project aims to accomplish and what you are researching. Remember to use clear language.
Thinking about the project’s purpose will help you set realistic goals and inform how you divide tasks and assign responsibilities. These individual tasks will be your stepping stones to reach your overarching goal.
Additionally, you’ll want to identify the specific problem, the usability metrics needed, and the intended solutions.
Know the following three things about your project’s purpose before you outline anything else:
What you’re doing
Why you’re doing it
What you expect from it
Identify individual objectives
With your overarching project objectives in place, you can identify any individual goals or steps needed to reach those objectives. Break them down into phases or steps. You can work backward from the project goal and identify every process required to facilitate it.
Be mindful to identify each unique task so that you can assign responsibilities to various team members. At this point in your research plan development, you’ll also want to assign priority to those smaller, more manageable steps and phases that require more immediate or dedicated attention.
Select research methods
Research methods might include any of the following:
User interviews: this is a qualitative research method where researchers engage with participants in one-on-one or group conversations. The aim is to gather insights into their experiences, preferences, and opinions to uncover patterns, trends, and data.
Field studies: this approach allows for a contextual understanding of behaviors, interactions, and processes in real-world settings. It involves the researcher immersing themselves in the field, conducting observations, interviews, or experiments to gather in-depth insights.
Card sorting: participants categorize information by sorting content cards into groups based on their perceived similarities. You might use this process to gain insights into participants’ mental models and preferences when navigating or organizing information on websites, apps, or other systems.
Focus groups: use organized discussions among select groups of participants to provide relevant views and experiences about a particular topic.
Diary studies: ask participants to record their experiences, thoughts, and activities in a diary over a specified period. This method provides a deeper understanding of user experiences, uncovers patterns, and identifies areas for improvement.
Five-second testing: participants are shown a design, such as a web page or interface, for just five seconds. They then answer questions about their initial impressions and recall, allowing you to evaluate the design’s effectiveness.
Surveys: get feedback from participant groups with structured surveys. You can use online forms, telephone interviews, or paper questionnaires to reveal trends, patterns, and correlations.
Tree testing: tree testing involves researching web assets through the lens of findability and navigability. Participants are given a textual representation of the site’s hierarchy (the “tree”) and asked to locate specific information or complete tasks by selecting paths.
Usability testing: ask participants to interact with a product, website, or application to evaluate its ease of use. This method enables you to uncover areas for improvement in digital key feature functionality by observing participants using the product.
Live website testing: research and collect analytics that outlines the design, usability, and performance efficiencies of a website in real time.
There are no limits to the number of research methods you could use within your project. Just make sure your research methods help you determine the following:
What do you plan to do with the research findings?
What decisions will this research inform? How can your stakeholders leverage the research data and results?
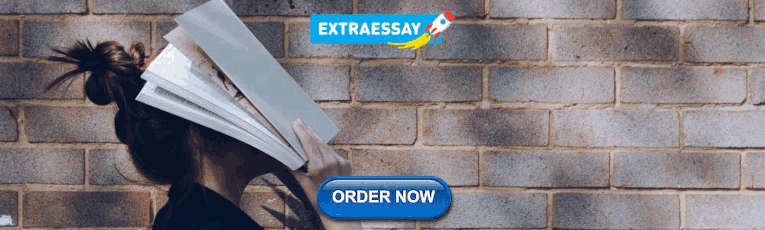
Recruit participants and allocate tasks
Next, identify the participants needed to complete the research and the resources required to complete the tasks. Different people will be proficient at different tasks, and having a task allocation plan will allow everything to run smoothly.
Prepare a thorough project summary
Every well-designed research plan will feature a project summary. This official summary will guide your research alongside its communications or messaging. You’ll use the summary while recruiting participants and during stakeholder meetings. It can also be useful when conducting field studies.
Ensure this summary includes all the elements of your research project. Separate the steps into an easily explainable piece of text that includes the following:
An introduction: the message you’ll deliver to participants about the interview, pre-planned questioning, and testing tasks.
Interview questions: prepare questions you intend to ask participants as part of your research study, guiding the sessions from start to finish.
An exit message: draft messaging your teams will use to conclude testing or survey sessions. These should include the next steps and express gratitude for the participant’s time.
Create a realistic timeline
While your project might already have a deadline or a results timeline in place, you’ll need to consider the time needed to execute it effectively.
Realistically outline the time needed to properly execute each supporting phase of research and implementation. And, as you evaluate the necessary schedules, be sure to include additional time for achieving each milestone in case any changes or unexpected delays arise.
For this part of your research plan, you might find it helpful to create visuals to ensure your research team and stakeholders fully understand the information.
Determine how to present your results
A research plan must also describe how you intend to present your results. Depending on the nature of your project and its goals, you might dedicate one team member (the PI) or assume responsibility for communicating the findings yourself.
In this part of the research plan, you’ll articulate how you’ll share the results. Detail any materials you’ll use, such as:
Presentations and slides
A project report booklet
A project findings pamphlet
Documents with key takeaways and statistics
Graphic visuals to support your findings
- Format your research plan
As you create your research plan, you can enjoy a little creative freedom. A plan can assume many forms, so format it how you see fit. Determine the best layout based on your specific project, intended communications, and the preferences of your teams and stakeholders.
Find format inspiration among the following layouts:
Written outlines
Narrative storytelling
Visual mapping
Graphic timelines
Remember, the research plan format you choose will be subject to change and adaptation as your research and findings unfold. However, your final format should ideally outline questions, problems, opportunities, and expectations.
- Research plan example
Imagine you’ve been tasked with finding out how to get more customers to order takeout from an online food delivery platform. The goal is to improve satisfaction and retain existing customers. You set out to discover why more people aren’t ordering and what it is they do want to order or experience.
You identify the need for a research project that helps you understand what drives customer loyalty. But before you jump in and start calling past customers, you need to develop a research plan—the roadmap that provides focus, clarity, and realistic details to the project.
Here’s an example outline of a research plan you might put together:
Project title
Project members involved in the research plan
Purpose of the project (provide a summary of the research plan’s intent)
Objective 1 (provide a short description for each objective)
Objective 2
Objective 3
Proposed timeline
Audience (detail the group you want to research, such as customers or non-customers)
Budget (how much you think it might cost to do the research)
Risk factors/contingencies (any potential risk factors that may impact the project’s success)
Remember, your research plan doesn’t have to reinvent the wheel—it just needs to fit your project’s unique needs and aims.
Customizing a research plan template
Some companies offer research plan templates to help get you started. However, it may make more sense to develop your own customized plan template. Be sure to include the core elements of a great research plan with your template layout, including the following:
Introductions to participants and stakeholders
Background problems and needs statement
Significance, ethics, and purpose
Research methods, questions, and designs
Preliminary beliefs and expectations
Implications and intended outcomes
Realistic timelines for each phase
Conclusion and presentations
How many pages should a research plan be?
Generally, a research plan can vary in length between 500 to 1,500 words. This is roughly three pages of content. More substantial projects will be 2,000 to 3,500 words, taking up four to seven pages of planning documents.
What is the difference between a research plan and a research proposal?
A research plan is a roadmap to success for research teams. A research proposal, on the other hand, is a dissertation aimed at convincing or earning the support of others. Both are relevant in creating a guide to follow to complete a project goal.
What are the seven steps to developing a research plan?
While each research project is different, it’s best to follow these seven general steps to create your research plan:
Defining the problem
Identifying goals
Choosing research methods
Recruiting participants
Preparing the brief or summary
Establishing task timelines
Defining how you will present the findings
Get started today
Go from raw data to valuable insights with a flexible research platform
Editor’s picks
Last updated: 21 December 2023
Last updated: 16 December 2023
Last updated: 17 February 2024
Last updated: 19 November 2023
Last updated: 5 March 2024
Last updated: 15 February 2024
Last updated: 11 March 2024
Last updated: 12 December 2023
Last updated: 6 March 2024
Last updated: 10 April 2023
Last updated: 20 December 2023
Latest articles
Related topics, log in or sign up.
Get started for free

Want to create or adapt books like this? Learn more about how Pressbooks supports open publishing practices.
11.2 Steps in Developing a Research Proposal
Learning objectives.
- Identify the steps in developing a research proposal.
- Choose a topic and formulate a research question and working thesis.
- Develop a research proposal.
Writing a good research paper takes time, thought, and effort. Although this assignment is challenging, it is manageable. Focusing on one step at a time will help you develop a thoughtful, informative, well-supported research paper.
Your first step is to choose a topic and then to develop research questions, a working thesis, and a written research proposal. Set aside adequate time for this part of the process. Fully exploring ideas will help you build a solid foundation for your paper.
Choosing a Topic
When you choose a topic for a research paper, you are making a major commitment. Your choice will help determine whether you enjoy the lengthy process of research and writing—and whether your final paper fulfills the assignment requirements. If you choose your topic hastily, you may later find it difficult to work with your topic. By taking your time and choosing carefully, you can ensure that this assignment is not only challenging but also rewarding.
Writers understand the importance of choosing a topic that fulfills the assignment requirements and fits the assignment’s purpose and audience. (For more information about purpose and audience, see Chapter 6 “Writing Paragraphs: Separating Ideas and Shaping Content” .) Choosing a topic that interests you is also crucial. You instructor may provide a list of suggested topics or ask that you develop a topic on your own. In either case, try to identify topics that genuinely interest you.
After identifying potential topic ideas, you will need to evaluate your ideas and choose one topic to pursue. Will you be able to find enough information about the topic? Can you develop a paper about this topic that presents and supports your original ideas? Is the topic too broad or too narrow for the scope of the assignment? If so, can you modify it so it is more manageable? You will ask these questions during this preliminary phase of the research process.
Identifying Potential Topics
Sometimes, your instructor may provide a list of suggested topics. If so, you may benefit from identifying several possibilities before committing to one idea. It is important to know how to narrow down your ideas into a concise, manageable thesis. You may also use the list as a starting point to help you identify additional, related topics. Discussing your ideas with your instructor will help ensure that you choose a manageable topic that fits the requirements of the assignment.
In this chapter, you will follow a writer named Jorge, who is studying health care administration, as he prepares a research paper. You will also plan, research, and draft your own research paper.
Jorge was assigned to write a research paper on health and the media for an introductory course in health care. Although a general topic was selected for the students, Jorge had to decide which specific issues interested him. He brainstormed a list of possibilities.
If you are writing a research paper for a specialized course, look back through your notes and course activities. Identify reading assignments and class discussions that especially engaged you. Doing so can help you identify topics to pursue.
- Health Maintenance Organizations (HMOs) in the news
- Sexual education programs
- Hollywood and eating disorders
- Americans’ access to public health information
- Media portrayal of health care reform bill
- Depictions of drugs on television
- The effect of the Internet on mental health
- Popularized diets (such as low-carbohydrate diets)
- Fear of pandemics (bird flu, HINI, SARS)
- Electronic entertainment and obesity
- Advertisements for prescription drugs
- Public education and disease prevention
Set a timer for five minutes. Use brainstorming or idea mapping to create a list of topics you would be interested in researching for a paper about the influence of the Internet on social networking. Do you closely follow the media coverage of a particular website, such as Twitter? Would you like to learn more about a certain industry, such as online dating? Which social networking sites do you and your friends use? List as many ideas related to this topic as you can.
Narrowing Your Topic
Once you have a list of potential topics, you will need to choose one as the focus of your essay. You will also need to narrow your topic. Most writers find that the topics they listed during brainstorming or idea mapping are broad—too broad for the scope of the assignment. Working with an overly broad topic, such as sexual education programs or popularized diets, can be frustrating and overwhelming. Each topic has so many facets that it would be impossible to cover them all in a college research paper. However, more specific choices, such as the pros and cons of sexual education in kids’ television programs or the physical effects of the South Beach diet, are specific enough to write about without being too narrow to sustain an entire research paper.
A good research paper provides focused, in-depth information and analysis. If your topic is too broad, you will find it difficult to do more than skim the surface when you research it and write about it. Narrowing your focus is essential to making your topic manageable. To narrow your focus, explore your topic in writing, conduct preliminary research, and discuss both the topic and the research with others.
Exploring Your Topic in Writing
“How am I supposed to narrow my topic when I haven’t even begun researching yet?” In fact, you may already know more than you realize. Review your list and identify your top two or three topics. Set aside some time to explore each one through freewriting. (For more information about freewriting, see Chapter 8 “The Writing Process: How Do I Begin?” .) Simply taking the time to focus on your topic may yield fresh angles.
Jorge knew that he was especially interested in the topic of diet fads, but he also knew that it was much too broad for his assignment. He used freewriting to explore his thoughts so he could narrow his topic. Read Jorge’s ideas.
Conducting Preliminary Research
Another way writers may focus a topic is to conduct preliminary research . Like freewriting, exploratory reading can help you identify interesting angles. Surfing the web and browsing through newspaper and magazine articles are good ways to start. Find out what people are saying about your topic on blogs and online discussion groups. Discussing your topic with others can also inspire you. Talk about your ideas with your classmates, your friends, or your instructor.
Jorge’s freewriting exercise helped him realize that the assigned topic of health and the media intersected with a few of his interests—diet, nutrition, and obesity. Preliminary online research and discussions with his classmates strengthened his impression that many people are confused or misled by media coverage of these subjects.
Jorge decided to focus his paper on a topic that had garnered a great deal of media attention—low-carbohydrate diets. He wanted to find out whether low-carbohydrate diets were as effective as their proponents claimed.
Writing at Work
At work, you may need to research a topic quickly to find general information. This information can be useful in understanding trends in a given industry or generating competition. For example, a company may research a competitor’s prices and use the information when pricing their own product. You may find it useful to skim a variety of reliable sources and take notes on your findings.
The reliability of online sources varies greatly. In this exploratory phase of your research, you do not need to evaluate sources as closely as you will later. However, use common sense as you refine your paper topic. If you read a fascinating blog comment that gives you a new idea for your paper, be sure to check out other, more reliable sources as well to make sure the idea is worth pursuing.
Review the list of topics you created in Note 11.18 “Exercise 1” and identify two or three topics you would like to explore further. For each of these topics, spend five to ten minutes writing about the topic without stopping. Then review your writing to identify possible areas of focus.
Set aside time to conduct preliminary research about your potential topics. Then choose a topic to pursue for your research paper.
Collaboration
Please share your topic list with a classmate. Select one or two topics on his or her list that you would like to learn more about and return it to him or her. Discuss why you found the topics interesting, and learn which of your topics your classmate selected and why.
A Plan for Research
Your freewriting and preliminary research have helped you choose a focused, manageable topic for your research paper. To work with your topic successfully, you will need to determine what exactly you want to learn about it—and later, what you want to say about it. Before you begin conducting in-depth research, you will further define your focus by developing a research question , a working thesis, and a research proposal.
Formulating a Research Question
In forming a research question, you are setting a goal for your research. Your main research question should be substantial enough to form the guiding principle of your paper—but focused enough to guide your research. A strong research question requires you not only to find information but also to put together different pieces of information, interpret and analyze them, and figure out what you think. As you consider potential research questions, ask yourself whether they would be too hard or too easy to answer.
To determine your research question, review the freewriting you completed earlier. Skim through books, articles, and websites and list the questions you have. (You may wish to use the 5WH strategy to help you formulate questions. See Chapter 8 “The Writing Process: How Do I Begin?” for more information about 5WH questions.) Include simple, factual questions and more complex questions that would require analysis and interpretation. Determine your main question—the primary focus of your paper—and several subquestions that you will need to research to answer your main question.
Here are the research questions Jorge will use to focus his research. Notice that his main research question has no obvious, straightforward answer. Jorge will need to research his subquestions, which address narrower topics, to answer his main question.
Using the topic you selected in Note 11.24 “Exercise 2” , write your main research question and at least four to five subquestions. Check that your main research question is appropriately complex for your assignment.
Constructing a Working ThesIs
A working thesis concisely states a writer’s initial answer to the main research question. It does not merely state a fact or present a subjective opinion. Instead, it expresses a debatable idea or claim that you hope to prove through additional research. Your working thesis is called a working thesis for a reason—it is subject to change. As you learn more about your topic, you may change your thinking in light of your research findings. Let your working thesis serve as a guide to your research, but do not be afraid to modify it based on what you learn.
Jorge began his research with a strong point of view based on his preliminary writing and research. Read his working thesis statement, which presents the point he will argue. Notice how it states Jorge’s tentative answer to his research question.
One way to determine your working thesis is to consider how you would complete sentences such as I believe or My opinion is . However, keep in mind that academic writing generally does not use first-person pronouns. These statements are useful starting points, but formal research papers use an objective voice.
Write a working thesis statement that presents your preliminary answer to the research question you wrote in Note 11.27 “Exercise 3” . Check that your working thesis statement presents an idea or claim that could be supported or refuted by evidence from research.
Creating a Research Proposal
A research proposal is a brief document—no more than one typed page—that summarizes the preliminary work you have completed. Your purpose in writing it is to formalize your plan for research and present it to your instructor for feedback. In your research proposal, you will present your main research question, related subquestions, and working thesis. You will also briefly discuss the value of researching this topic and indicate how you plan to gather information.
When Jorge began drafting his research proposal, he realized that he had already created most of the pieces he needed. However, he knew he also had to explain how his research would be relevant to other future health care professionals. In addition, he wanted to form a general plan for doing the research and identifying potentially useful sources. Read Jorge’s research proposal.
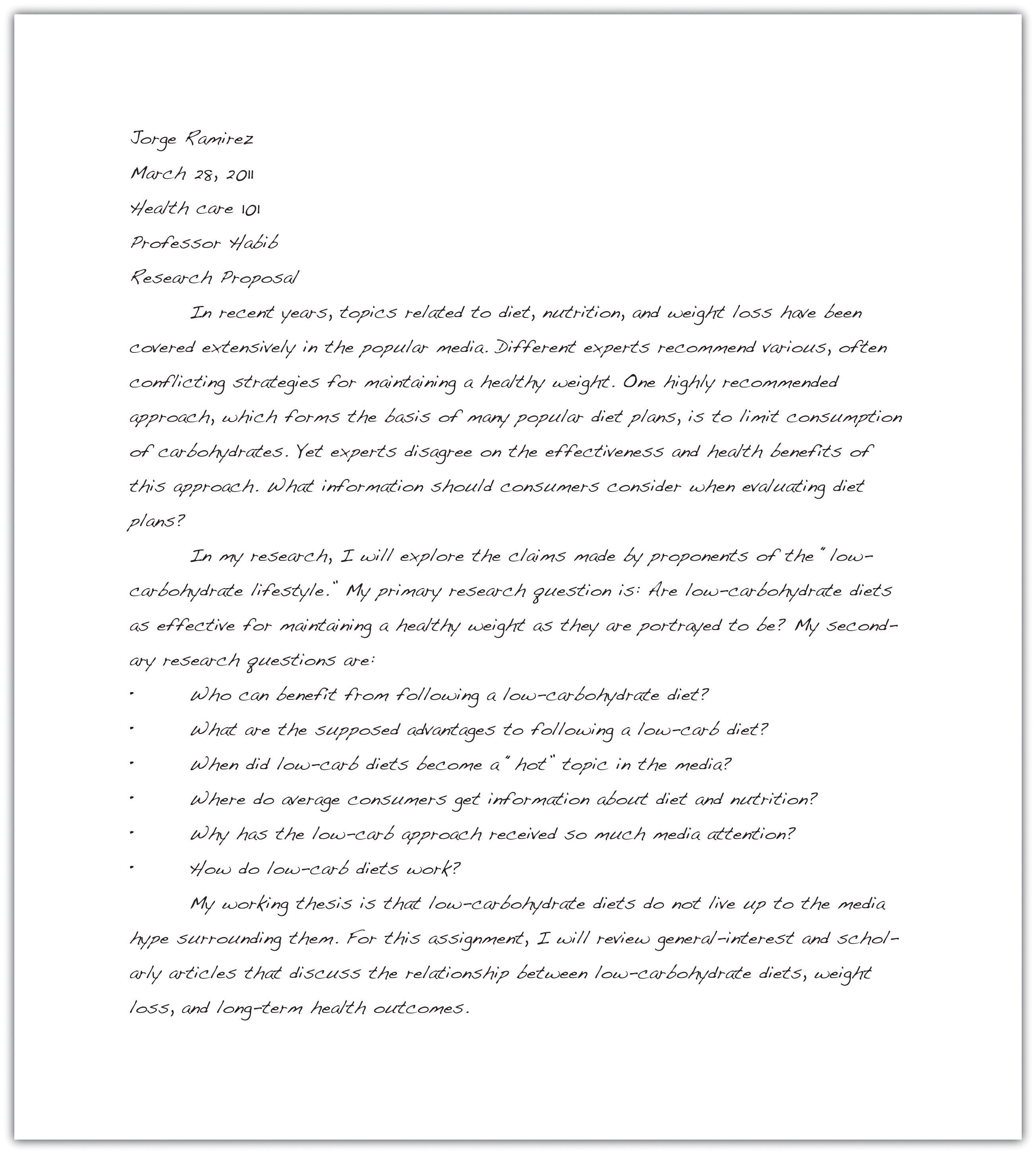
Before you begin a new project at work, you may have to develop a project summary document that states the purpose of the project, explains why it would be a wise use of company resources, and briefly outlines the steps involved in completing the project. This type of document is similar to a research proposal. Both documents define and limit a project, explain its value, discuss how to proceed, and identify what resources you will use.
Writing Your Own Research Proposal
Now you may write your own research proposal, if you have not done so already. Follow the guidelines provided in this lesson.
Key Takeaways
- Developing a research proposal involves the following preliminary steps: identifying potential ideas, choosing ideas to explore further, choosing and narrowing a topic, formulating a research question, and developing a working thesis.
- A good topic for a research paper interests the writer and fulfills the requirements of the assignment.
- Defining and narrowing a topic helps writers conduct focused, in-depth research.
- Writers conduct preliminary research to identify possible topics and research questions and to develop a working thesis.
- A good research question interests readers, is neither too broad nor too narrow, and has no obvious answer.
- A good working thesis expresses a debatable idea or claim that can be supported with evidence from research.
- Writers create a research proposal to present their topic, main research question, subquestions, and working thesis to an instructor for approval or feedback.
Writing for Success Copyright © 2015 by University of Minnesota is licensed under a Creative Commons Attribution-NonCommercial-ShareAlike 4.0 International License , except where otherwise noted.
- WordPress.org
- Documentation
- Learn WordPress
Product Development Use Cases
Risks with product development, what product development engineers do, what are product development strategies, is product development part of r&d, why product development is necessary, is product development agile, product development key skills, stages to product development.
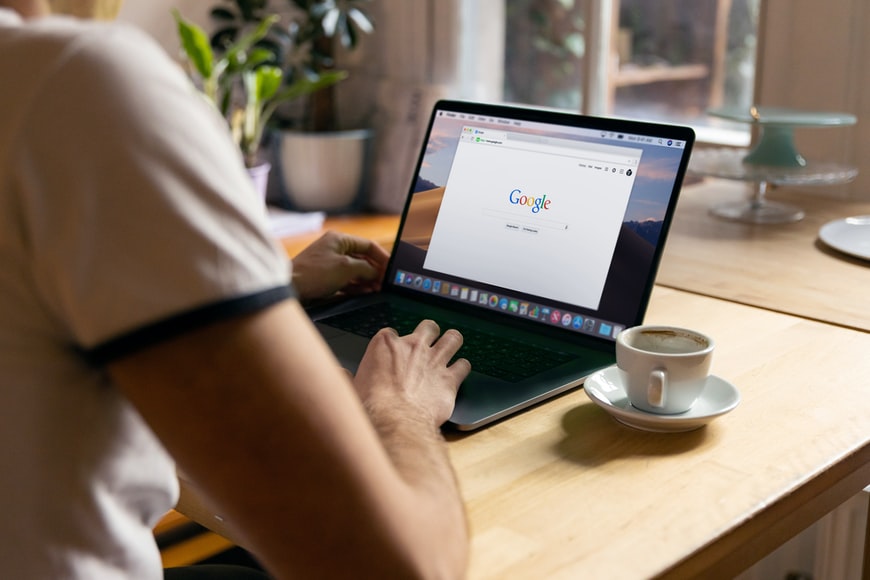
Research and Development Duties and Responsibilities
What are the research and development duties and responsibilities? Read on to learn more.
What Is Research and Development?
Research and development (R&D) is the process of designing, conducting, and documenting experiments to make scientific and technological advances .
The purpose of research and development is to increase knowledge, solve problems, and develop new technologies. And that will lead to new products, services, or ways of doing things.
The term research and development is often used interchangeably with the term applied research.
Applied research goes beyond basic research to focus on producing practical results that can be used by industry or society.
Research and development can happen in private industry, universities, medical centers, or even government agencies.
Most companies employ their own research teams to conduct R&D activities.
Research and development specialists are responsible for leading research efforts.
They are typically multidisciplinary in their approach. Also, they are working closely with teams of engineers, scientists, and other professionals to achieve research goals .
Research and development specialists are expected to have an excellent knowledge of their organization’s products or services.
They must have a strong understanding of the organization’s customers’ needs. They should also know how the product or service can meet those needs.
Research and development specialists may also be responsible for ensuring that the organization meets regulatory requirements for conducting clinical trials or product testing.
They often work in teams with marketing specialists to design new products or develop new uses for existing products.
Research and Development Responsibilities
Research and development specialists share the following responsibilities:
- Develop a comprehensive plan, including what experiments will be conducted, how many subjects will be involved in the trial, and an outline of the tests to be used.
- Collecting data as needed from experiments previously conducted by other doctors, medical researchers, or engineers .
- Conducting research tests and experiments to gather data on a particular project or product.
- Analyzing the data produced by the experiment and making any necessary adjustments to the study’s design or results.
- Creating reports detailing test results and any conclusions drawn from those results.
Research and development specialists may prepare these reports for internal use within their own company or agency, as well as for publication in scientific journals, trade publications, or technical manuals.
Developing new technologies or processes that can be used during clinical trials for drugs or medical devices.
Research and development specialists may also work with doctors to conduct clinical outcome studies to determine whether a drug is effective at treating certain diseases or conditions.
Specialists may also evaluate whether existing drugs can be used to treat other conditions that were not originally intended by drug manufacturers.
The Bottom Line
People who work as research and development specialists typically enjoy working with their hands and must be highly detail-oriented.
They must also be able to work as part of a team, as well as independently, and communicate effectively with doctors and other research staff.
Research and development specialists may need to travel to conduct experiments or visit client facilities to learn more about their needs, which could require them to spend long hours on the road.
Leave a Reply Cancel reply
Your email address will not be published. Required fields are marked *
Save my name, email, and website in this browser for the next time I comment.
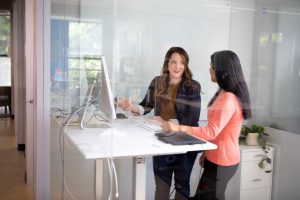
More Stories
Today, there are many reasons why product development is necessary. This is why companies need to do this process smartly....
Product Development KPI Metrics
Product development KPI metrics are important for companies to have. But what kinds of metrics should these be? And how...
Need for Product Development
Today, the need for product development is rising over time. That is why companies need to be more innovative with...
Product Development Function
What is the most important product development function? What are some of the other functions of this process? Let us...
Product Development vs Product Design
Today, we will talk about product development vs product design. What differs each process? And how can you implement each...
Product Development Project
What is a product development project? What are the things you need to do to make this project successful? If...
Recent Post
Top category, vp research and development.
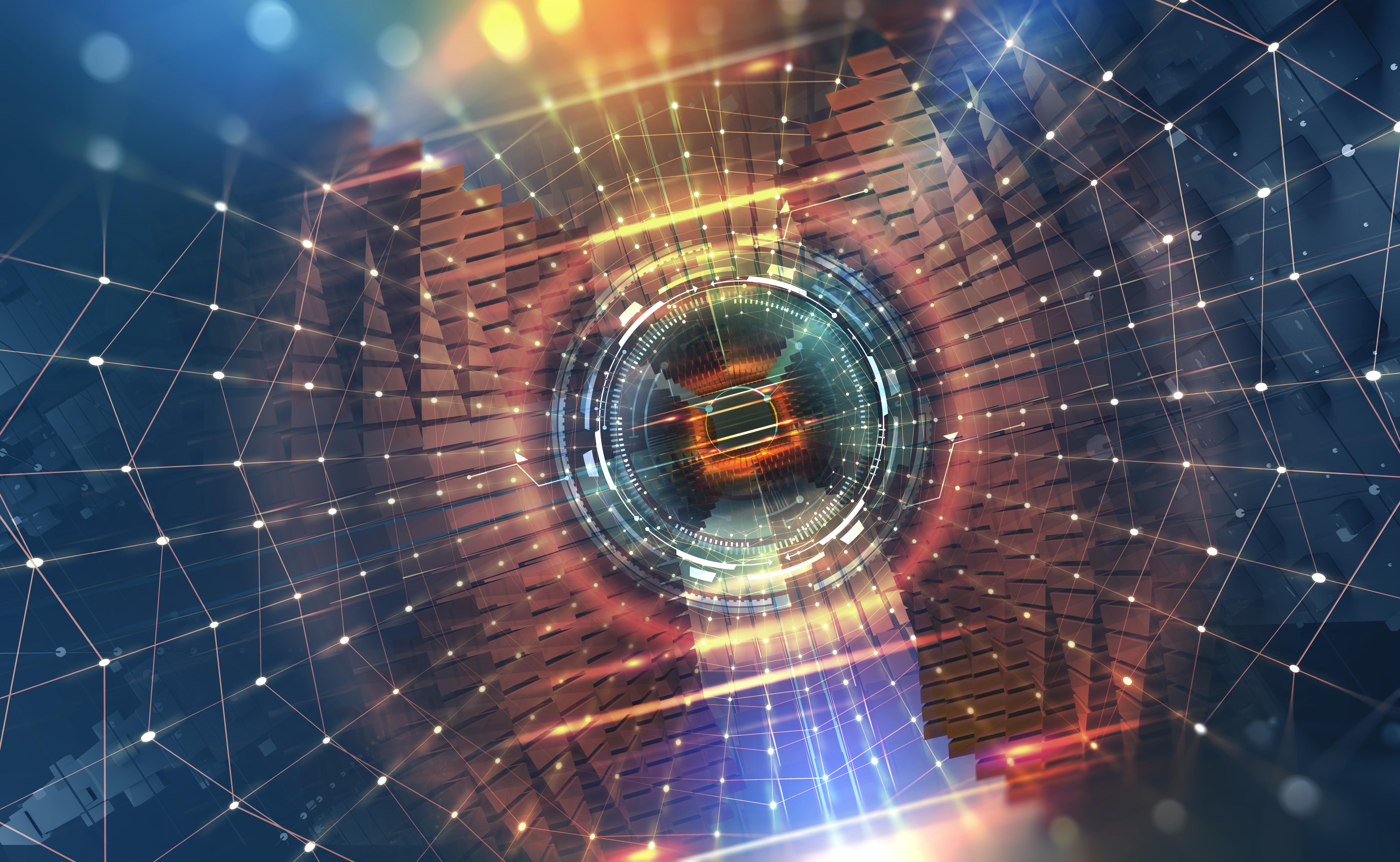
An Agile Framework for Research and Development
A quick search of "Agile for research and development" makes two things clear:
- Research and development (R&D) processes need agility beyond the software development space.
- Companies struggle to match agile frameworks to their R&D needs.
As LMI generated an operating model to develop prototypes around innovative and crowdsourced ideas in short timelines (30–45 days), the difficulty became apparent. While LMI has mature teams practicing scrum, Kanban, extreme programming (XP), and the Scaled Agile Framework (SAFe), the compressed timelines and enhanced uncertainty around scope and technology presented unique challenges requiring a new approach. This method (shown in Figure 1) expressly supports the development of rapid prototypes where scope and technology are initially undefined. Why does R&D need something different?
- The open-ended definition of “done” for exploratory efforts clashes with sprint planning (scrum).
- Reaching an endpoint in a timebox is a challenge for Kanban.
- Shortened timelines devalue estimation while augmenting demand for shorter feedback loops.
- Abridged timelines mean that overhead with iteration management needs to be kept tight.
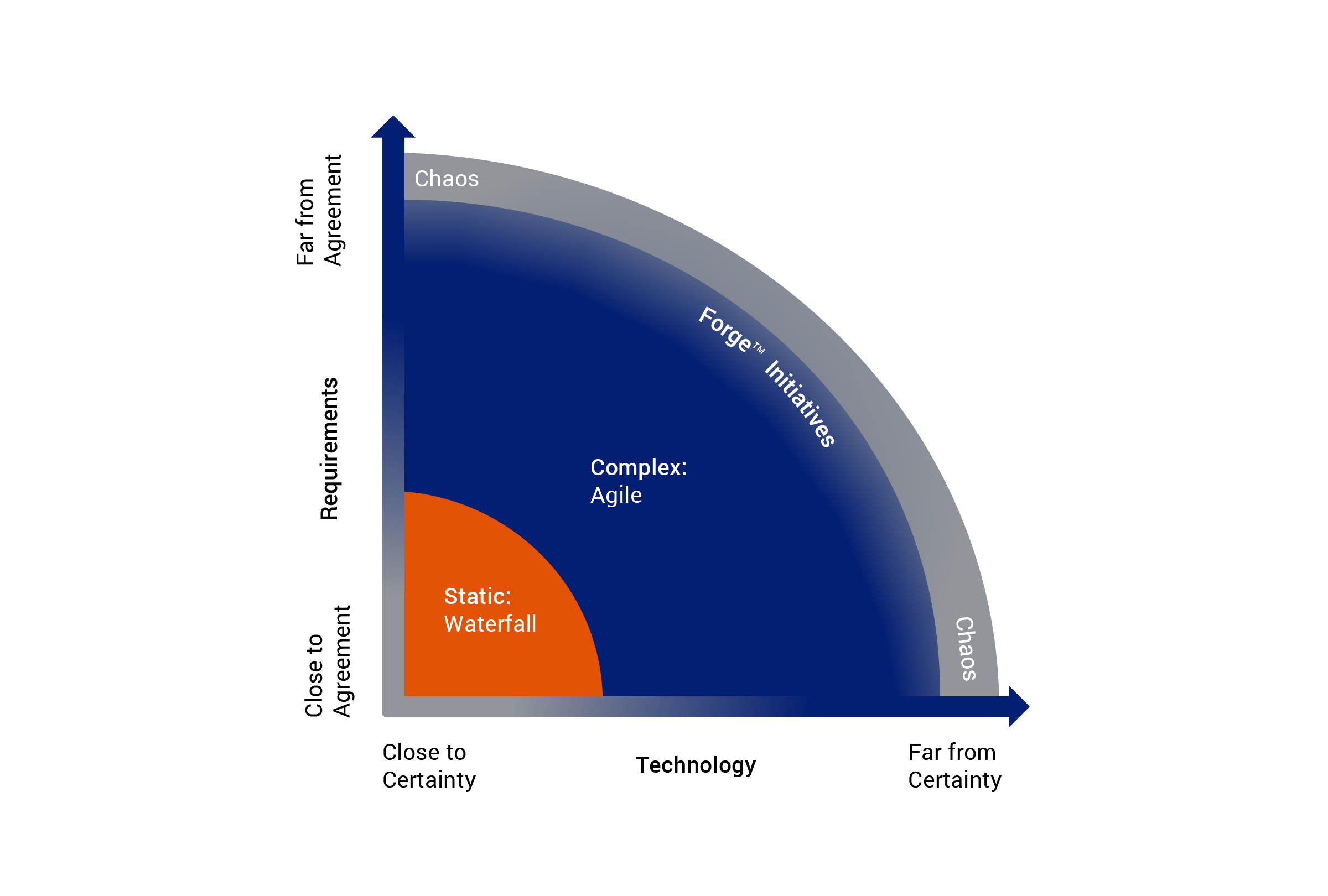
Focusing on the Right Things
To support these efforts LMI developed Ranger™ – a deconstructed agile framework . Framework development must start with goals and principles. Inculcating a team with unified purpose creates alignment and offers team members a beacon when facing an uncertain situation. Standards and principles drive our practices and tools. With each prototype kickoff, we reintroduce our mission and core values.
Our mission is to prove the concept. We aim to establish or demonstrate feasibility, not implement the concept perfectly. We do this through the following core values:
- Creating and preserving momentum
- Practicing ruthless prioritization and ignoring sunk costs
- Emphasizing positioning over planning.
With our mission in mind, the team decided which practices and roles to introduce, alter, and keep from various agile frameworks.
Designing Agile Practices Around Core Values
Ranger™ has similarities to scrum, Kanban, and the dynamic systems development method. However, significant changes and small adjustments make this process unique.
The Significant Changes
Most agile practices use consistent iteration lengths of 2–4 weeks. When working on a 30- or 45-day prototype, this period represents an unacceptable timeline for feedback. Compressed project lengths make capacity measures, like story points, relatively useless (it takes about three iterations to calculate velocity). Ranger™ solves this issue by creating variable-length iterations, called orbits . An orbit lasts 2–5 business days and is goal-focused. The team agrees to the length at each orbit start, based on the goal. Setting a short orbit helps the team right-size goals while variable orbit sizes enable the team to swarm around the true objective without adding filler to round out capacity. When reflecting on our core values, varying length iterations consistently helped our teams create momentum, prioritize ruthlessly, and emphasize positioning.
The other significant adjustment was around roles. Prototype idea generators and subject matter experts often have other commitments preventing them from working as a product owner. We formalized the roles of innovators and sponsors for unique contributions to the prototype vision.
- The innovator has the idea or vision of what a fully developed product can be.
- Market and service line sponsors have insights into proposals and clients that could benefit from the prototype outcome in the near term.
- The product owner defines an achievable prototype scope that marries these insights.
Splitting these responsibilities across technical, business, and functional experts generates a healthy friction. Introducing and sharing multiple vantage points promotes broader education around the problem and how the prototype fits into that narrative.
The Small Adjustments
Although, like with other agile frameworks, teams perform daily stand-ups, iteration reviews, and practice continuous improvement through retrospectives, these ceremonies underwent minor adjustments to account for our context. For example, we have a single formal retrospective at about the 1/3 mark of the prototype and a post-mortem retrospective at its conclusion. This timeline ensures improvement and reflection while preserving momentum and reducing overhead. Figure 2 shows how these ceremonies fit in the framework.
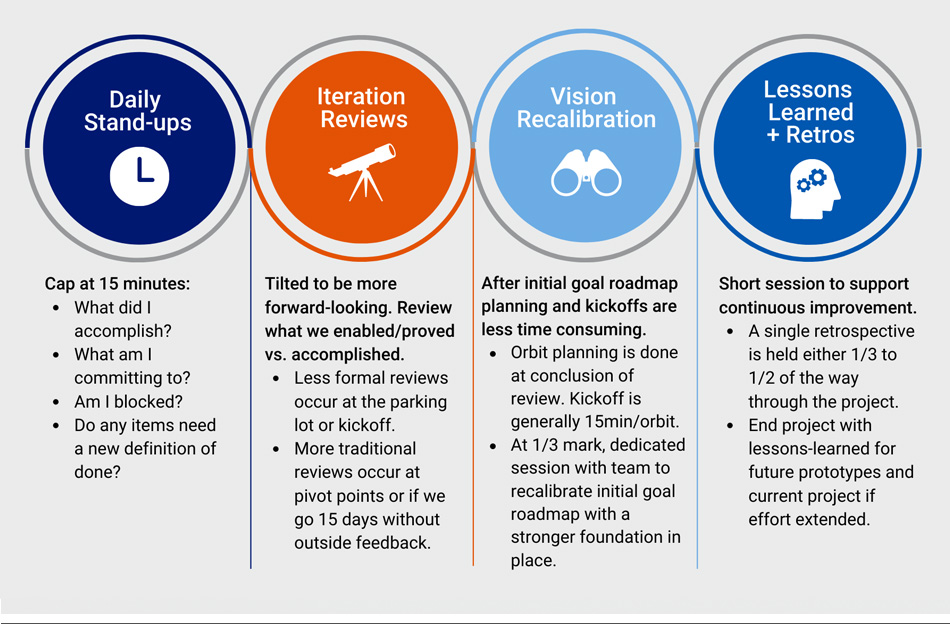
The Results
In one year, 16 prototypes reached completion using the Ranger™ framework. While not every prototype receives further funding for product development, each furnishes enough information for an educated decision regarding further investment. All prototypes have achieved that objective, with a representative proof of concept delivered on time to support decision-making.
Several of the most successful prototypes had significant pivots or concluded with a scope outside of the initial plan, outlining the need for constant positioning based on discoveries and overall agility. Understanding a problem and proving the capacity to solve it in these timeframes has implications for the government’s acquisition and proposal process and can increase confidence in how the government approaches complex problems.
While existing methods might not fit perfectly in every context, adaptations and custom frameworks can bring agility to non-traditional use cases.
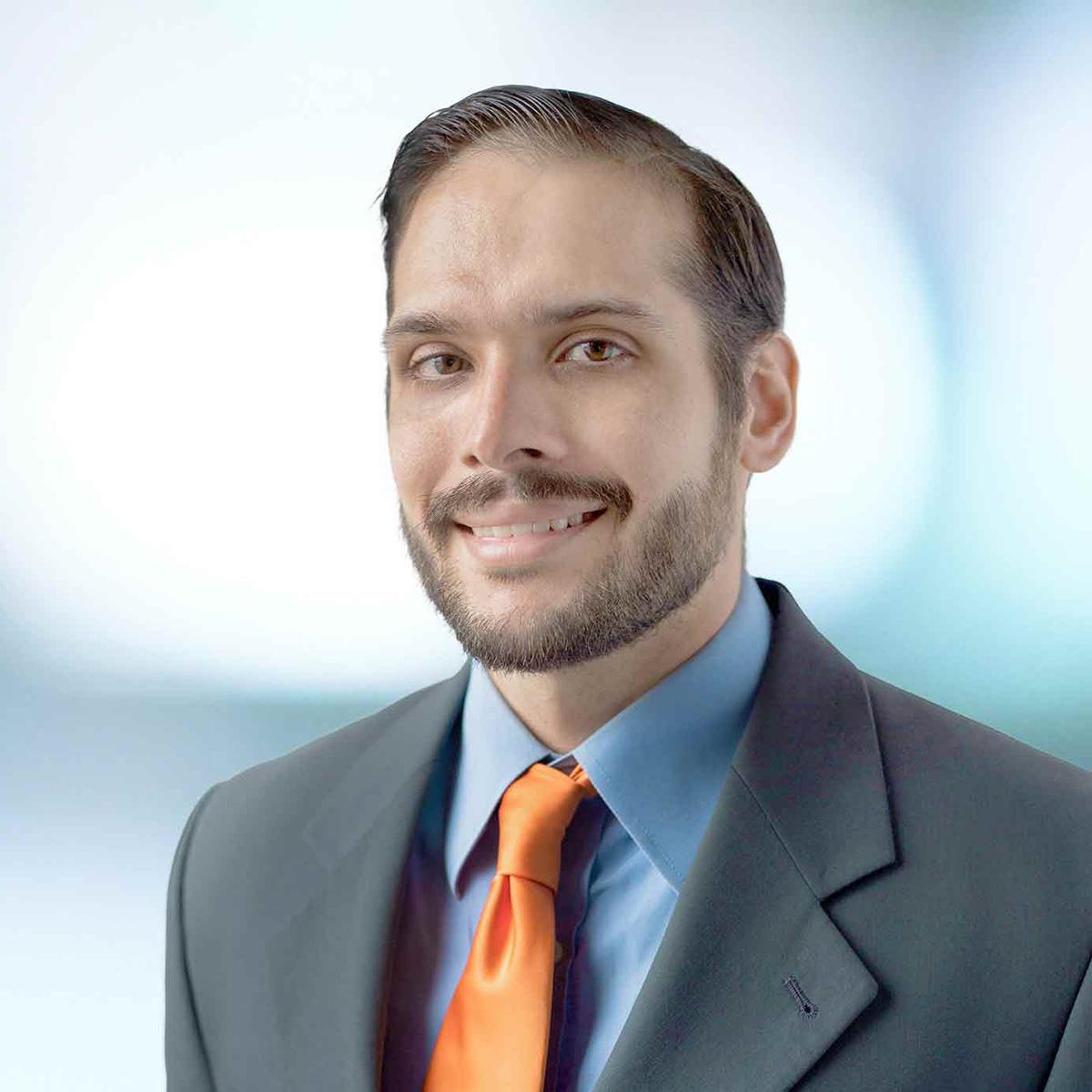
Joseph Mariña
Joseph Mariña has experience as a scrum master and agile coach across many agile frameworks. He has more than ten years of experience working on an array of technical solutions.
Related Content

How We Innovate
Operating in an innovation ecosystem, we combine market-leading technical resources, strategic partnerships, and agile development capabilities to accelerate mission success.
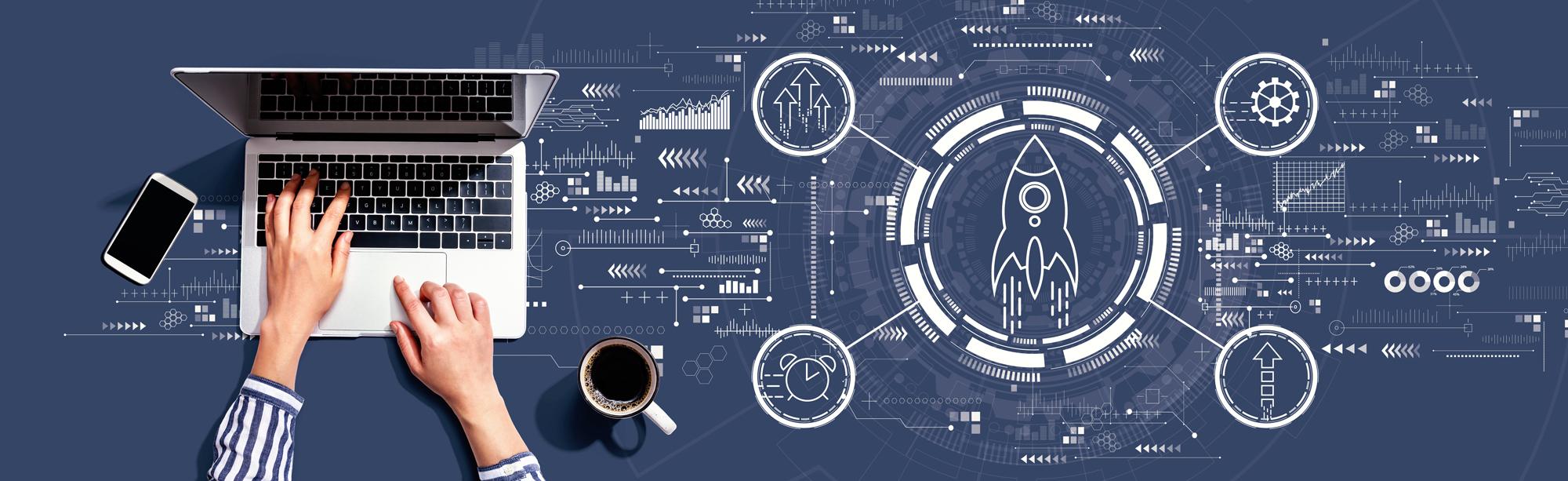
Ranger™ Framework: Exploring with Ranger™
Ranger™ is a deconstructed agile framework designed for the development of quick (~45 business day) prototypes and research projects. It best serves efforts around feasibility rather than perfect implementation.

Enterprise Agility
Employing an Agile mindset to accelerate organizational transformation, strengthen programs, and meet dynamic business needs.
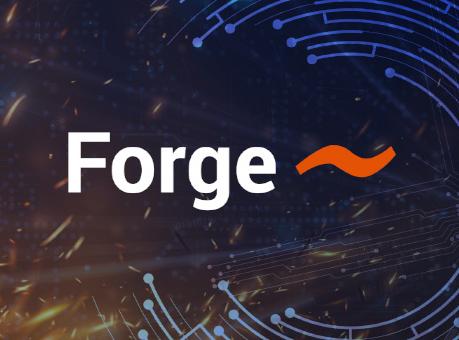
Forge™ Technology Studio
Leveraging agile methods and human-centered design, the Forge™ empowers us to deliver technology solutions rapidly.
Research and Development Tasks in Teacher Education: Institutional Framing and Student Experiences
- First Online: 01 March 2018
Cite this chapter
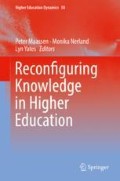
- Crina Damşa 6
Part of the book series: Higher Education Dynamics ((HEDY,volume 50))
847 Accesses
2 Citations
Research and development tasks have been proposed as a learning solution in teacher education, where dynamic subject-matter knowledge and professional practices, and increasingly complex teaching environments generate emerging challenges for teacher education institutions and students. While there is an awareness that research is a complex practice, students are, however, expected to handle research activities as a natural part of the curriculum. This empirical study examined how teacher education students worked on research and development tasks linked to their school internships. The findings show that research and development tasks, viewed from an institutional perspective, bring together knowledge, activities, and experiences that allow students to construct their own repertoire of knowledgeable and teaching practices. But they also generate challenges, related to time management, to finding a balance between the needs of the teaching practice and the expectations of the teacher education programme, or to integrating different types of knowledge. The study raises awareness of how these tasks can be used to expose students to the realities of the teaching practice but also of the need for guidance and support required when such tasks are a part of the curriculum.
This is a preview of subscription content, log in via an institution to check access.
Access this chapter
- Available as PDF
- Read on any device
- Instant download
- Own it forever
- Available as EPUB and PDF
- Compact, lightweight edition
- Dispatched in 3 to 5 business days
- Free shipping worldwide - see info
- Durable hardcover edition
Tax calculation will be finalised at checkout
Purchases are for personal use only
Institutional subscriptions
A definition and extensive discussion on the notion of research-based education can be found in Afdal and Damşa, Chap. 8 , this volume.
For reasons of confidentiality, the male participant will be referred to as ‘she’/‘her’ throughout the remainder of the text.
The CASS-Query platform (Contextual Activity Sampling System; Salo et al. 2014 ) is developed and hosted at the Metropolia University of Applied Sciences in Finland.
This aspect is further addressed in the following sub-section ‘The student perspective’.
The CASS platform failed to collect the survey answers for the last 2 weeks before the assignment deadline. The information regarding the activities was instead collected in the final interview.
Aditomo, A., Goodyear, P., Bliuc, A.-M., & Ellis, R. (2013). Inquiry-based learning in higher education: Principal forms, educational objectives, and disciplinary variations. Studies in Higher Education, 38 (9), 1239–1258. https://doi.org/10.1080/03075079.2011.616584 .
Article Google Scholar
Ashwin, P., Abbas, A., & McLean, M. (2016). How does completing a dissertation transform undergraduate students’ understandings of disciplinary knowledge? Assessment & Evaluation in Higher Education, 42 (4), 517–530.
Bakken, J., & Sollid, H. (2014). Forskende lærerstudenter. In A. B. Reinertsen, B. Groven, K. A. Knutas, & A. Holm (Eds.), FoU i praksis 2013. Konferanse om praksisrettet FoU i lærerutdanning (pp. 12–18). Oslo: Akademika forlag.
Google Scholar
Byman, R., Krokfors, L., Toom, A., Maaranen, K., Jyrhämä, R., Kynäslahti, H., & Kansanen, P. (2009). Educating inquiry-oriented teachers: Students’ attitudes and experiences towards research-based teacher education. Educational Research and Evaluation, 15 (1), 79–92.
Cochran-Smith, M., & Villegas, A. M. (2015). Framing teacher preparation research: An overview of the field, Part 1. Journal of Teacher Education, 66 (1), 7–20. https://doi.org/10.1177/0022487114549072 .
Dunn, M., Harrison, L. J., & Coombe, K. (2008). In good hands: Preparing research-skilled graduates for the early childhood profession. Teaching and Teacher Education, 24 (3), 703–714. https://doi.org/10.1016/j.tate.2007.09.002 .
Jyrhämä, R., Kynäslahti, H., Krokfors, L., Byman, R., Maaranen, K., Toom, A., & Kansanen, P. (2008). The appreciation and realisation of research-based teacher education: Finnish students’ experiences of teacher education. European Journal of Teacher Education, 31 (1), 1–16.
Klette, K., & Carlsten, T. C. (2012). Knowledge in teacher learning: New professional challenges. In K. Jensen, L. C. Lahn, & M. Nerland (Eds.), Professional learning in the knowledge society (pp. 27–48). Rotterdam: Sense Publishers.
Markauskaite, L., & Goodyear, P. (2014). Professional work and knowledge. In S. Billett, C. Harteis, & H. Gruber (Eds.), International handbook of research in professional and practice-based learning (pp. 79–106). Dordrecht: Springer.
Munthe, E., & Rogne, M. (2015). Research-based teacher education. Teaching and Teacher Education, 46 , 17–24. https://doi.org/10.1016/j.tate.2014.10.006 .
Nicolini, D., Mengis, J., & Swan, J. (2012). Understanding the role of objects in cross-disciplinary collaboration. Organization Science, 23 (3), 612–629.
Rasmussen, I., & Damşa, C. I. (2016). Heterochrony through moment-to-moment interaction: A micro-analytical exploration of learning as sense making with multiple resources. International Journal of Educational Research, 72 , 149–161. https://doi.org/10.1016/j.ijer.2016.04.003 .
Säljö, R. (2010). Digital tools and challenges to institutional traditions of learning: Technologies, social memory and the performative nature of learning. Journal of Computer Assisted Learning, 26 , 53–64. https://doi.org/10.1111/j.1365-2729.2009.00341.x .
Salo, K., Shakya, U., & Damena, M. (2014). Device agnostic CASS client. In A. Marcus (Ed.), Design, user experience, and usability: User experience design for diverse interaction platforms and environments (pp. 334–345). Dordrecht: Springer.
Smith, K. (2015). The role of research in teacher education. Research in Teacher Education, 5 (2), 43–46.
Smith, K., & Sela, O. (2005). Action research as a bridge between pre-service teacher education and in-service professional development. European Journal of Teacher Education, 28 (3), 293–311.
Toom, A., Kynäaslahti, H., Krokfors, L., Jyrhämä, R., Byman, R., Stenberg, K., Maaranen, K., & Kansanen, P. (2010). Experiences of a research-based approach to teacher education: Suggestions for future policies. European Journal of Education, 45 (2), 331–344.
Trent, J. (2010). Teacher education as identity construction: Insights from action research. Journal of Education for Teaching, 36 (2), 153–168. https://doi.org/10.1080/02607471003651672 .
Van der Linden, W., Bakx, A., Ros, A., Beijaard, D., & Vermeulen, M. (2012). Student teachers’ development of a positive attitude towards research and research knowledge and skills. European Journal of Teacher Education, 35 (4), 401–419. https://doi.org/10.1080/02619768.2011.643401 .
White, S., Hepple, E., Tangen, D., Comelli, M., Alwi, A., & Abu Hassan Shaari, Z. (2016). An introduction to education research methods: Exploring the learning journey of pre-service teachers in a transnational programme. Asia-Pacific Journal of Teacher Education, 44 (1), 35–48. https://doi.org/10.1080/1359866X.2015.1021294 .
Download references
Author information
Authors and affiliations.
Department of Education, Faculty of Educational Sciences, University of Oslo, Oslo, Norway
Crina Damşa
You can also search for this author in PubMed Google Scholar
Corresponding author
Correspondence to Crina Damşa .
Editor information
Editors and affiliations.
Department of Education Faculty of Educational Sciences, University of Oslo, Oslo, Norway
Peter Maassen
Monika Nerland
Melbourne Graduate School of Education, University of Melbourne, Parkville, Australia
Rights and permissions
Reprints and permissions
Copyright information
© 2018 Springer International Publishing AG
About this chapter
Damşa, C. (2018). Research and Development Tasks in Teacher Education: Institutional Framing and Student Experiences. In: Maassen, P., Nerland, M., Yates, L. (eds) Reconfiguring Knowledge in Higher Education. Higher Education Dynamics, vol 50. Springer, Cham. https://doi.org/10.1007/978-3-319-72832-2_9
Download citation
DOI : https://doi.org/10.1007/978-3-319-72832-2_9
Published : 01 March 2018
Publisher Name : Springer, Cham
Print ISBN : 978-3-319-72831-5
Online ISBN : 978-3-319-72832-2
eBook Packages : Education Education (R0)
Share this chapter
Anyone you share the following link with will be able to read this content:
Sorry, a shareable link is not currently available for this article.
Provided by the Springer Nature SharedIt content-sharing initiative
- Publish with us
Policies and ethics
- Find a journal
- Track your research
Resume Builder
- Resume Experts
- Search Jobs
- Search for Talent
- Employer Branding
- Outplacement
Research & Development Job Description
Research & development duties & responsibilities.
To write an effective research & development job description, begin by listing detailed duties, responsibilities and expectations. We have included research & development job description templates that you can modify and use.
Sample responsibilities for this position include:
Research & Development Qualifications
Qualifications for a job description may include education, certification, and experience.
Licensing or Certifications for Research & Development
List any licenses or certifications required by the position: PMP, CPA, MBA, PMI, R.C, CMA, CMM, CPIM, ISO, ASQ
Education for Research & Development
Typically a job would require a certain level of education.
Employers hiring for the research & development job most commonly would prefer for their future employee to have a relevant degree such as Bachelor's and Master's Degree in Science, Engineering, Education, Chemistry, Writing, Technical, Chemical Engineering, Business, Communication, Journalism
Skills for Research & Development
Desired skills for research & development include:
Desired experience for research & development includes:
Research & Development Examples
- Microsoft Word (.docx) .DOCX
- PDF Document (.pdf) .PDF
- Image File (.png) .PNG
- Seeks opportunities for professional development that will enhance job performance
- Manage and maintain all project development tools
- Provide high-quality ideas and contributions to solve difficult analytical development problems as assigned
- Provide analytical development expertise and act as a technical consultant to both internal and external customers
- Prepare presentations and present data and analytical development services to internal and external customers
- Develop and determine research project strategies
- Conduct investigative research using local, state, national sources, and a variety of print and electronic sources to identify potential donors and provide in-depth personal and financial information on prospects
- Supervise professional individual contributors
- Operating lab equipment and continuing to learn to use new equipment as it arrives
- Opening up PDR standards
- Associate or Fellow of the Casualty Actuarial Society (ACAS or FCAS) or equivalent international designation (preferred)
- 6+ years of experience in General Insurance
- Experience working with Geographic Information Systems and spatial analysis (preferred)
- Bachelor's degree in a relevant scientific discipline, such as biology, biochemistry or bioengineering required
- Minimum of 7 years of experience in academic or industry setting is required
- Matching approved formula numbers to samples received
- Design and implement scientific studies to support new technology development, enhancement of existing processes (includes data collection and statistical analysis)
- Updating charts with new standard numbers, fragrance information,and percentages
- Setting up meetings on Outlook with fragrance houses for stability review (booking rooms, adjusting times / schedules, and recapping meetings)
- Retrieving data from PLM system and organizing the data and presenting to the Program Manager for efficient formula release
- Cross-referencing and confirming critical data numbers across multiple charts
- Provide technical leadership and support to insure effective and efficient manufacturing of products
- Plans and prepares comprehensive and/or complex in-depth research services for fundraising purposes requiring a high degree of sensitivity
- Develops and maintains electronic information systems and procedures to facilitate operations
- Accountable for total program scope
- Direct the work of technical and support personnel
- Strong knowledge of theoretical and practical sensory and consumer research skills and well known in the discipline as the subject matter expert
- Ability to use computers and related software (Sensory and Statistical software), including word processing and spreadsheets for data analysis and monitoring (Microsoft Word, Excel, PowerPoint, Access)
- Must be proficient in Word and Excel spreadsheet design
- Welding (Mild and Stainless) & fabrication an advantage
- Prototype installation of engines into machines
- Lifting up to 50 lbs on regular basis
- Emerging Technologies - Ensure that we stay on the forefront with the way entertain and tell our stories
- 5+ years working at a film or television studio, agency or other related entertainment company in the Research & Strategy or comparable department
- Highly organized, data driven, analytical, proficient in creating and presenting reports and findings to executive management
- Having working knowledge of available market research tools and 3rd party companies
- Promotes collaboration with fundraising staff by participating in strategy meetings to share pertinent information already obtained, determine research needs, and ensure research efforts provide useful information that supports fundraising efforts
- Reviews print and on-line media to identify new major (high potential) donor prospects and to locate timely information about alumni, higher education, business transactions and market trends
- Researches, synthesizes, and analyzes biographic and financial information on specific individuals, foundations and corporations for major gift fundraising needs, includes analyzing, prioritizing and disseminating results of database screenings
- Utilizes intuitive and creative approaches in all aspects of research
- Participates in annual Research and Prospect Management planning sessions with fundraising units to help formulate a strategic operating plan for each fiscal year
- Updates donor database (Ellucian Advance) with information on donors and alumni
- Proficiency in Microsoft Office Applications including Word and Excel skills is required
- Sensory training and test execution for a food or beverage company/supplier
- Driving a company or rental vehicle for up to 8 hours as needed
- Strong written and verbal communication skills with the ability to work independently within a team framework, managing multiple priorities
- Proficiency in computing skills, including Microsoft Office, data analysis and graphing programs
- Experience with graphics programs such as Adobe Illustrator, Photoshop or InDesign is a plus
- Participates in research orientation training for new Advancement employees to educate and increase awareness of services and information available through their own efforts, and information that can be obtained through research staff, materials and databases
- Participates in special projects as requested by director
- Ability to deal with confidential material and uphold high ethical standards
- Experience with research tools available (e.g., online, printed, ) preferred
- Support the Associate Director of Administration (AD) in running the UACC, including assistance with hiring staff, coordinating and planning retreats, organizing and compiling the P30 Cancer Center Support Grant (CCSG) and other multi-investigator grants organizing aspects of the associated site visits
- Develops new products from concept to successful scale up and production, meeting all agreed upon standards, and keeping the projects on schedule and under budget, so far as this is possible
- Modifies existing products
- Uses expertise to help troubleshoot problems with existing products or ingredients
- Creates QA documentation for new products, including formulations, bills of materiel, ingredient statements, raw material specifications, finished product specifications, quality indexes, Helps QA to update and maintain documentation for existing products
- May conduct weekly Benchmarking review
- Experience with database querying languages is also a plus
- Ability to analyze and synthesize financial information
- Expert knowledge of internet navigation tools and research tools available (e.g., on-line, printed)
- Knowledge of Ellucian Advance (donor database) preferred
- Ability to manage and prioritize multiple tasks and projects simultaneously, and to be cognizant of and address details
- Expert ability to analyze issues and solve problems
- Prepares written and oral reports for presentation to the supervisor(s) and development group
- May conduct quarterly Bill of Materials (BOM) audits
- May have the responsibility of doing consumer testing
- May have the responsibility of maintaining records for the organization of samples tested (The Sample Test Reports)
- Expectation to work on multiple projects at one time
- Maintain GMP regulations and safe work conditions within all facilities being worked in
- Enabling the execution of and delivering value of their contract and alliances
- Effectively interact with other departments & organizations
- Identify and implement new and improved technologies as used by competitors, suppliers, customers
- Maintains the professional and ethical standards set forth for the field by the Association of Professional Researchers for Advancement (APRA)
- Solid knowledge of Securities and Exchange Commission documents and financial terminology
- Personal computer proficiency required, especially as related to the software referenced under “software utilized” (see below)
- BS in Meat/Food Science or related field plus a minimum of 7 years relevant work experience highly desirable
- MS in Meat /Food Science or related field plus a minimum of 5 years relevant work experience highly desirable
- Master's degree and five years of experience in an academic or other research environment are required or a PhD or terminal degree and three years of experience in an academic or other research environment are preferred
- Degree in social or natural science, engineering or related field is preferred
Related Job Descriptions
Create a Resume in Minutes with Professional Resume Templates
I am an Employer
I am a candidate.

- SUGGESTED TOPICS
- The Magazine
- Newsletters
- Managing Yourself
- Managing Teams
- Work-life Balance
- The Big Idea
- Data & Visuals
- Reading Lists
- Case Selections
- HBR Learning
- Topic Feeds
- Account Settings
- Email Preferences
Research: How Different Fields Are Using GenAI to Redefine Roles
- Maryam Alavi
Examples from customer support, management consulting, professional writing, legal analysis, and software and technology.
The interactive, conversational, analytical, and generative features of GenAI offer support for creativity, problem-solving, and processing and digestion of large bodies of information. Therefore, these features can act as cognitive resources for knowledge workers. Moreover, the capabilities of GenAI can mitigate various hindrances to effective performance that knowledge workers may encounter in their jobs, including time pressure, gaps in knowledge and skills, and negative feelings (such as boredom stemming from repetitive tasks or frustration arising from interactions with dissatisfied customers). Empirical research and field observations have already begun to reveal the value of GenAI capabilities and their potential for job crafting.
There is an expectation that implementing new and emerging Generative AI (GenAI) tools enhances the effectiveness and competitiveness of organizations. This belief is evidenced by current and planned investments in GenAI tools, especially by firms in knowledge-intensive industries such as finance, healthcare, and entertainment, among others. According to forecasts, enterprise spending on GenAI will increase by two-fold in 2024 and grow to $151.1 billion by 2027 .
- Maryam Alavi is the Elizabeth D. & Thomas M. Holder Chair & Professor of IT Management, Scheller College of Business, Georgia Institute of Technology .
Partner Center
MIT Technology Review
- Newsletters
An OpenAI spinoff has built an AI model that helps robots learn tasks like humans
But can it graduate from the lab to the warehouse floor?
- James O'Donnell archive page
In the summer of 2021, OpenAI quietly shuttered its robotics team, announcing that progress was being stifled by a lack of data necessary to train robots in how to move and reason using artificial intelligence.
Now three of OpenAI’s early research scientists say the startup they spun off in 2017, called Covariant, has solved that problem and unveiled a system that combines the reasoning skills of large language models with the physical dexterity of an advanced robot.
The new model, called RFM-1, was trained on years of data collected from Covariant’s small fleet of item-picking robots that customers like Crate & Barrel and Bonprix use in warehouses around the world, as well as words and videos from the internet. In the coming months, the model will be released to Covariant customers. The company hopes the system will become more capable and efficient as it’s deployed in the real world.
So what can it do? In a demonstration I attended last week, Covariant cofounders Peter Chen and Pieter Abbeel showed me how users can prompt the model using five different types of input: text, images, video, robot instructions, and measurements.
For example, show it an image of a bin filled with sports equipment, and tell it to pick up the pack of tennis balls. The robot can then grab the item, generate an image of what the bin will look like after the tennis balls are gone, or create a video showing a bird’s-eye view of how the robot will look doing the task.
If the model predicts it won’t be able to properly grasp the item, it might even type back, “I can’t get a good grip. Do you have any tips?” A response could advise it to use a specific number of the suction cups on its arms to give it better a grasp—eight versus six, for example.
This represents a leap forward, Chen told me, in robots that can adapt to their environment using training data rather than the complex, task-specific code that powered the previous generation of industrial robots. It’s also a step toward worksites where managers can issue instructions in human language without concern for the limitations of human labor. (“Pack 600 meal-prep kits for red pepper pasta using the following recipe. Take no breaks!”)
Lerrel Pinto, a researcher who runs the general-purpose robotics and AI lab at New York University and has no ties to Covariant, says that even though roboticists have built basic multimodal robots before and used them in lab settings, deploying one at scale that’s able to communicate in this many modes marks an impressive feat for the company.
To outpace its competitors, Covariant will have to get its hands on enough data for the robot to become useful in the wild, Pinto told me. Warehouse floors and loading docks are where it will be put to the test, constantly interacting with new instructions, people, objects, and environments.
“The groups which are going to train good models are going to be the ones that have either access to already large amounts of robot data or capabilities to generate those data,” he says.
Covariant says the model has a “human-like” ability to reason, but it has its limitations. During the demonstration, in which I could see a live feed of a Covariant robot as well as a chat window to communicate with it, Chen invited me to prompt the model with anything I wanted. When I asked the robot to “return the banana to Tote Two,” it struggled with retracing its steps, leading it to pick up a sponge, then an apple, then a host of other items before it finally accomplished the banana task.
“It doesn’t understand the new concept,” Chen said by way of explanation, “but it’s a good example—it might not work well yet in the places where you don’t have good training data.”
The company’s new model embodies a paradigm shift rippling through the robotics world. Rather than teaching a robot how the world works manually, through instructions like physics equations and code, researchers are teaching it in the same way humans learn: through millions of observations.
The result “really can act as a very effective flexible brain to solve arbitrary robot tasks,” Chen said.
The playing field of companies using AI to power more nimble robotic systems is likely to grow crowded this year. Earlier this month, the humanoid-robotics startup Figure AI announced it would be partnering with OpenAI and raised $675 million from tech giants like Nvidia and Microsoft. Marc Raibert, the founder of Boston Dynamics, recently started an initiative to better integrate AI into robotics.
This means that advancements in machine learning will likely start translating to advancements in robotics. However, some issues remain unresolved. If large language models continue to be trained on millions of words without compensating the authors of those words, perhaps it will be expected that robotics models will also be trained on videos without paying their creators. And if language models hallucinate and perpetuate biases, what equivalents will surface in robotics?
In the meantime, Covariant will push forward, keen on having RFM-1 continually learn and refine. Eventually, the researchers aim to have the robot train on videos that the model itself creates—the type of meta-learning that not only makes my head spin but also sparks concern about what will happen if errors made by the model compound themselves. But with such a hunger for more training data, researchers see it almost as inevitable.
Artificial intelligence
Large language models can do jaw-dropping things. but nobody knows exactly why..
And that's a problem. Figuring it out is one of the biggest scientific puzzles of our time and a crucial step towards controlling more powerful future models.
- Will Douglas Heaven archive page
OpenAI teases an amazing new generative video model called Sora
The firm is sharing Sora with a small group of safety testers but the rest of us will have to wait to learn more.
Google’s Gemini is now in everything. Here’s how you can try it out.
Gmail, Docs, and more will now come with Gemini baked in. But Europeans will have to wait before they can download the app.
Google DeepMind’s new generative model makes Super Mario–like games from scratch
Genie learns how to control games by watching hours and hours of video. It could help train next-gen robots too.
Stay connected
Get the latest updates from mit technology review.
Discover special offers, top stories, upcoming events, and more.
Thank you for submitting your email!
It looks like something went wrong.
We’re having trouble saving your preferences. Try refreshing this page and updating them one more time. If you continue to get this message, reach out to us at [email protected] with a list of newsletters you’d like to receive.
Havighurst’s Developmental Task Theory

What are the most important tasks in your life right now? I’m not talking about little things like brushing your teeth or paying a bill. I’m talking about the biggest goals in your overall development. According to the Havighurst's Developmental Task Theory, your age plays a big role in this answer.
Who Was Robert J. Havighurst?
Havighurst was an American psychologist who developed his theory on Developmental Tasks between 1948 and 1953. His work came after the work of Sigmund Freud and Jean Piaget, but before Erik Erikson. He is also known for popularizing the phrase “teachable moments” in his 1952 book Human Development and Education.
In his book, he writes:
“A developmental task is a task which is learned at a specific point and which makes achievement of succeeding tasks possible. When the timing is right, the ability to learn a particular task will be possible. This is referred to as a 'teachable moment.' It is important to keep in mind that unless the time is right, learning will not occur. Hence, it is important to repeat important points whenever possible so that when a student's teachable moment occurs, s/he can benefit from the knowledge."
What Is Developmental Task Theory?
Havighurst’s Theory defined developmental stages and tasks pertaining to each stage. This mirrors the work of Piaget and Erikson, who also identified "stages" of development and what each child achieved at different ages. Within each stage is a list of tasks that the individual feels that they must complete.
What Are the Stages in Havighurst's Developmental Task Theory?
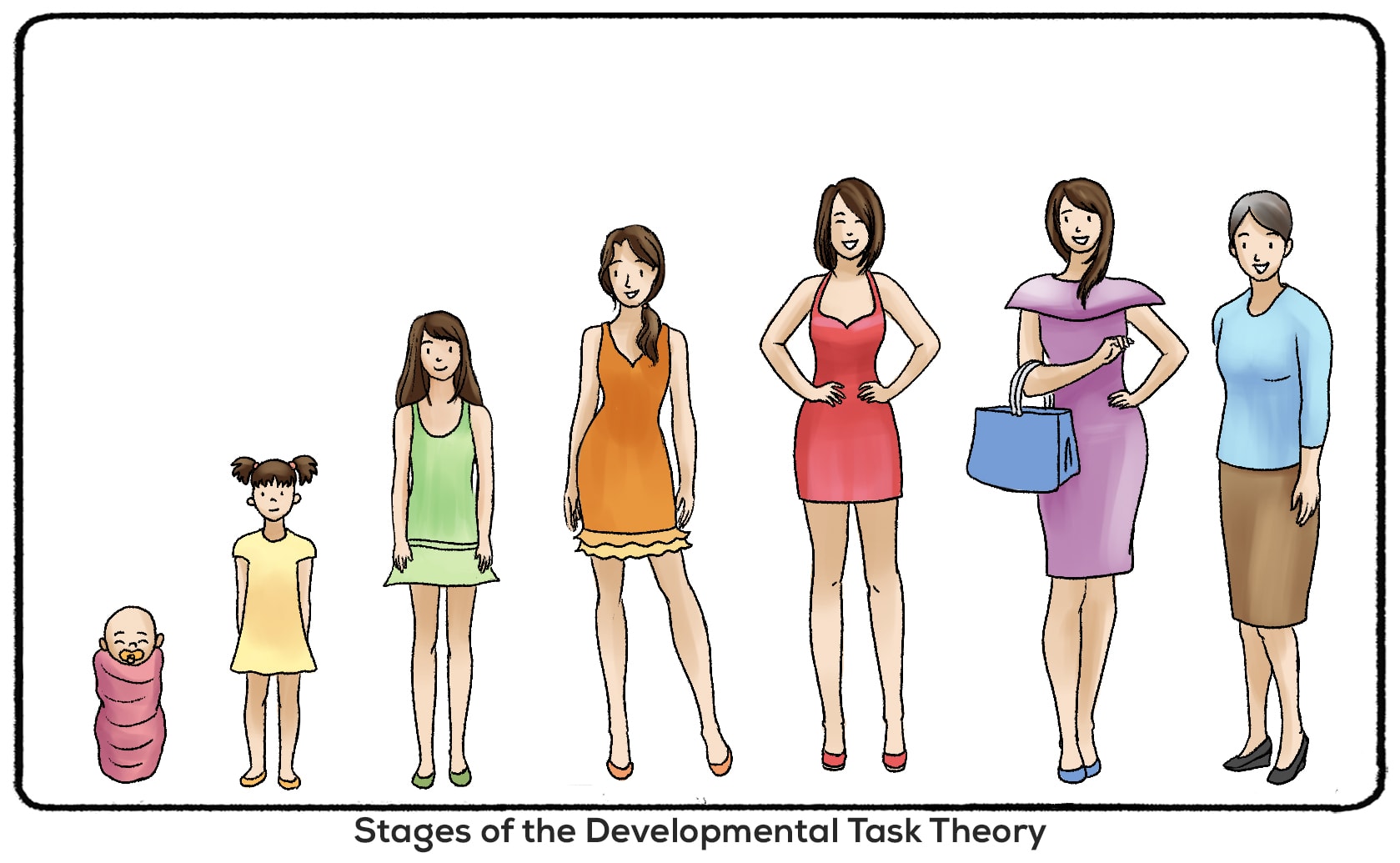
The stages in Havighurst's Theory include:
- Infancy and early childhood (0-6 years old)
- Later childhood (6–13 years old)
- Adolescence (13–18 years old)
- Early Adulthood (19–30 years old)
- Middle Age (30–60 years old)
- Later maturity (60 years old and over)
If the child completes the "correct" tasks in the "correct" time frame, they will feel happy and accepted by society. Failing to complete these tasks will make the individual unhappy and out of place in society.
While societal rules and customs certainly influence the tasks within each stage of development, other factors are also at play here. Havighurst also defined a list of what influences the tasks an individual strives to complete at each stage of development:
Social Influences (Pressures of Society)
These are the rules of society and other cultural ideas that influence an individual’s developmental tasks. Havighurst lists “Achieving a masculine or feminine social role” multiple times as a developmental task. That is going to look different in every culture (and will look depending on the individual’s age.) In cultures where masculine and feminine roles are not strictly defined, it may not be considered a task at all. As time progresses and societies change their ideas of gender, these tasks may look different or become less important to complete.
Psychological Influences (Personal Values)
These tasks do not just come from external forces. An individual’s personality and interests will also influence the tasks required to develop successfully. Someone who is more motivated by money and riches may face different developmental tasks than someone who puts more value into personal relationships or dedicating themselves to a noble cause. Psychological influences may also lead an individual to prioritize some developmental tasks over others.
Biological Influences (Physical Maturation)
Biology is also at play here. Certain tasks are reserved for childhood or adulthood simply because the body can or cannot take on those tasks. On one end of a person’s life is infancy and early adulthood, where developmental tasks include “learning to walk.” On the other end is later maturity, where developmental tasks include “adjusting to decreasing physical strength and health.”
If someone is seriously injured or develops a debilitating condition later in life, their developmental tasks may change.
Examples of Developmental Tasks
All of these tasks are subject to change due to biological, psychological, and social influences. But Havighurst provided an example list of tasks that go with each stage of life. I’m just going to include a handful of tasks for each stage, although Havighurst listed many more in his work.
Developmental Tasks in the stage of Infancy and Early Childhood (0-6 years old) include:
- Learning to walk
- Learning to talk
- Toilet training
- Learning the foundations of reading
Developmental Tasks in the stage of Middle Childhood (6-12 years old) include :
- Learning physical skills necessary for ordinary games
- Learning to get along and play with children of the same age
- Achieving personal independence
- Recognizing what society deems as masculine or feminine social roles
Developmental Tasks in the stage of Adolescence (13-18 years old) include:
- Accepting one’s physical body as it goes through changes
- Preparing for partnership and family life
- Preparing for an economic career
- Acquiring a set of values and an ethical system as a guide to behavior; developing an ideology
Developmental Tasks in the stage of Early Adulthood (19-30 years old) include:
- Finding a partner (and learning to cohabitate with them)
- Achieving a preferred masculine or feminine social role
- Managing a home and starting a family
- Beginning a career
- Taking on civic responsibility

Developmental Tasks in the stage of Middle Age (31-60 years old) include:
- Achieving adult civic and social responsibility
- Assisting teenage children to become responsible and happy adults
- Developing adult leisure-time activities
- Accepting and adjusting to the physiologic changes or middle age
Developmental Tasks in the stage of Later Maturity (61-death) include:
- Adjusting to decreasing physical strength and health
- Adjusting to retirement
- Meeting social and civil obligations
Again, these tasks may look different for every individual. Civic and social responsibility, for example, may look different for every person, or may not be a priority at the age that Havighurst proposes. These tasks may serve as a guideline or a jumping-off point if you are thinking about your larger goals, but remember that they are influenced by different factors, including personal values.
Havighurst's Developmental Task Theory vs. Piaget's Stages of Cognitive Development
One of the biggest differences between Havighurst’s Developmental Task Model and Piaget’s Stages of Cognitive Development is the length at which these theories cover. Havighurst’s theory covers the life of the person and Piaget’s theory covers infancy through the age of 12.
Another difference is that Havighurst’s developmental tasks vary so widely based on societal and internal pressures. Various tasks when it comes to making friends, learning to read at the appropriate time, or feeling the pressure to adapt to society’s masculine and feminine roles may all be considered developmental tasks under Havighurst.
But Piaget’s theory is more universal. He looks at skills that largely do not include interactions with others (although the child’s grasp of abstract concepts like empathy affects their relationships.) Conservation, object permanence, and problem-solving are all important cognitive developments no matter what society or the individual dictates.
Jean Piaget's stages of development focus on cognitive development and reaching a child's full potential of intelligence. These stages align with Havighurst’s only if society and individuals prioritize intelligence from an early age.
Comparing Havighurst vs. Piaget (Examples)
One of the example tasks used in Havighurst’s Middle Childhood stage (ages 6-12) is “learning to get along and play with children of the same age.” When speaking in broad terms of application, this is identical to cognitive developments achieved within Jean Piaget’s Concrete Operational Stage (ages 6-12.) But Piaget’s theory breaks down certain skills and abilities that allow a child to get along with children of their own age. Empathy is developed during this stage. With empathy, children are more likely to include each other, anticipate the child’s needs, and create a positive environment and relationship.
Also during the Middle Childhood stage is the example task of “recognizing what society deems as masculine or feminine social roles.” Whether a child is born male or female does not impact how they will develop under Piaget’s theory. Later research based on his theory also fails to see a difference in the development of skills like conservation. Recognizing what society has deemed as “masculine” and “feminine” tasks, however, does start around this age , at least in American society. This task may not be as relevant to children who grow up in a country like Sweden, where those roles are not as important.
Havighurst's Developmental Task Theory vs. Erikson's Stages of Psychosocial Development
Erikson’s Stages of Psychosocial Development contain eight stages, compared to Havighurst’s six. Erikson’s theory speaks to the feelings a person has about themselves and their place in the world, rather than their ability to complete tasks dictated by societal norms. Both last for the entire length of a person’s life.
Remember, Havighurst created his developmental stages before Erikson. The two theories have a few things in common, but the “achievements” of each stage of life are different.
Erikson's stages of development focus on how people face certain crises. These crises are at the core of every person’s development: identity vs. confusion, intimacy vs. isolation, etc. Going through these crises, a person learns how to interact with themselves and the world around them.
Comparing Havighurst vs. Erikson (Examples)
Resolving these crises looks different than simply completing a task or checking items off their “bucket list”. Take, for example, the crisis of autonomy vs. shame. This stage takes place when a child is between the ages of 18 months and three years. During this point, the child is moving through the “Infancy and Early Childhood” stage of Havighurst’s Developmental Task Theory, which lasts from birth to six years old.
Erikson suggested that during this stage, a child aims to develop personal autonomy. They do this when they are confident in making decisions for themselves about their needs. This could look like going to the toilet on their own, a task that is outlined in Havighurst’s Developmental Task Theory. Or, it could look like choosing what they wear during the day.
Another example is Erikson’s intimacy vs. isolation, which takes place around the ages of 20 and 40. During these years, an adult is experiencing what Havighurst identifies as the Early Adulthood and Middle Age stages. The intimacy vs. isolation stage is all about the journey to finding love, intimacy, and close relationships (romantic or otherwise). Does this mean getting married? Co-habitating with a partner? Making the choice between love and career? When it comes to Havighurst’s Developmental Task Theory, the answer all depends on the pressures of society and personal values. To Erikson, this stage is simply about finding intimacy and avoiding isolation. Whether this is resolved successfully also depends on whether the person feels comfortable in their own identity.
Havighurst's Developmental Task Theory vs. Freud's Stages of Psychosexual Development
Similar to Havighurst vs. Piaget, Freud’s stages of psychosexual development primarily focus on childhood, although the “genital stage” in Freud’s theory lasts from puberty until death. Like Piaget, the challenges and resolutions in Freud’s theory are universal…although there are fundamental differences that male and female children face.
Comparing Havighurst vs. Freud (Examples)
Like Havighurst (and arguably, Erikson), toilet training is a fundamental achievement in Freud’s theory. Freud’s anal stage takes place between the years of 1 and 3. What matters in Freud’s theory is not so much that toilet training is achieved, but how the relationship between parents and child changes during this stage. Traumatic memories during the toilet training process may lead some children to having an anal-retentive personality that lasts into adulthood.
All of these theories of child development look are slightly different, but still have similarities and suggest similar methods for helping a child grow. Which theories had you heard of before reading this blog post? Which stand out to you? Let us know!
Related posts:
- Piaget's Theory of Moral Development (4 Stages + Examples)
- Concrete Operational Stage (3rd Cognitive Development)
Developmental Psychology
- The Psychology of Long Distance Relationships
- Beck’s Depression Inventory (BDI Test)
Reference this article:
About The Author
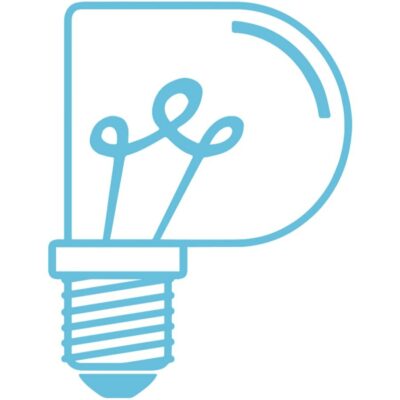
Developmental Psychology:
Erikson's Psychosocial Stages
Trust vs Mistrust
Autonomy vs Shame
Initiative vs Guilt
Industry vs inferiority
Identity vs Confusion
Intimacy vs Isolation
Generativity vs Stagnation
Integrity vs Despair
Attachment Styles
Avoidant Attachment
Anxious Attachment
Secure Attachment
Lawrence Kohlberg's Stages of Moral Development
Piaget's Cognitive Development
Sensorimotor Stage
Object Permanence
Preoperational Stage
Concrete Operational Stage
Formal Operational Stage
Unconditional Positive Regard
Birth Order
Zone of Proximal Development

PracticalPie.com is a participant in the Amazon Associates Program. As an Amazon Associate we earn from qualifying purchases.
Follow Us On:
Youtube Facebook Instagram X/Twitter
Psychology Resources
Developmental
Personality
Relationships
Psychologists
Serial Killers
Psychology Tests
Personality Quiz
Memory Test
Depression test
Type A/B Personality Test
© PracticalPsychology. All rights reserved
Privacy Policy | Terms of Use

Driving Simulation and AI Deepen Insights into Impulsivity
- Artificial Intelligence (AI)
- Decision Making
- Personality Traits
- Psychological Science
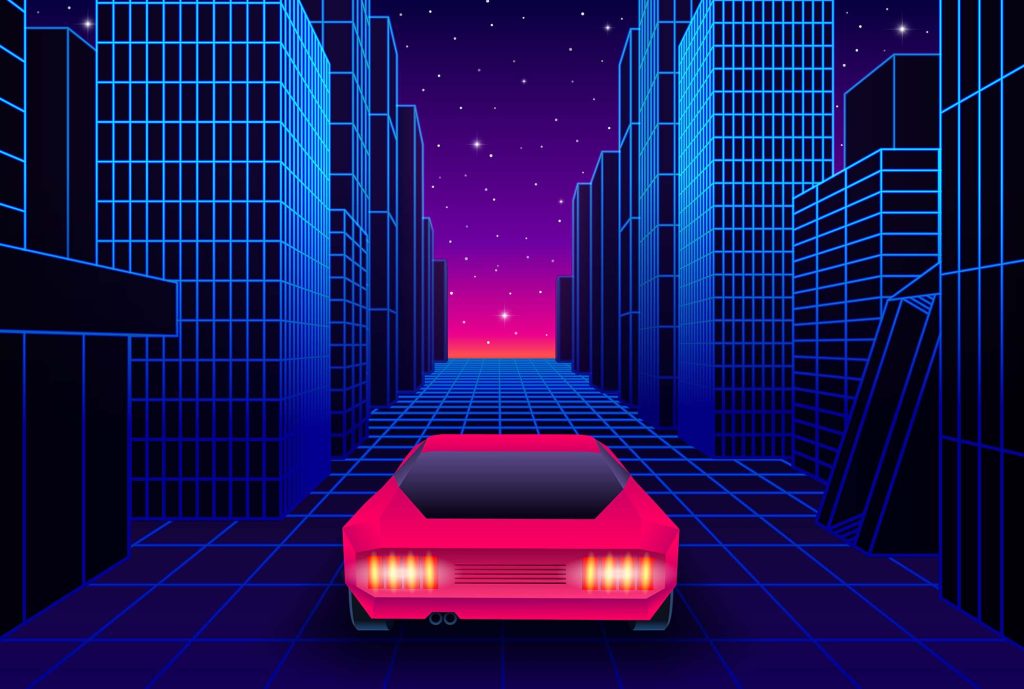
Psychological research often relies on participants to report or reflect on their own behavior , but these perceptions don’t always align with how they act in the real world or even during experiments in a laboratory. Lab experiments sometimes have participants engage in tasks that don’t capture the full range of behaviors people display in their day-to-day lives, but pairing realistic tasks with machine learning could help researchers more accurately assess individuals’ personality traits , wrote San Ho Lee and colleagues (Seoul National University) in a Psychological Science article .
The study employed an inverse reinforcement learning (ILR) algorithm capable of inferring the reward function that underlies observed behaviors.
“The combination of real-time tasks and deep IRL offers a promising novel approach to improving the assessment of psychological constructs underlying human behaviors and decision-making,” Lee and coauthors Myeong Seop Song, Min-hwan Oh, and Woo-Young Ahn wrote.
The researchers put their IRL algorithm to the test through a study of 47 students at Seoul National University. In addition to completing the Barrat Impulsiveness Scale (BIS), which measures participants’ perceptions of their own motor, planning, and attentional abilities, the students completed a series of three tasks designed to assess impulsivity:
- a simulated driving task in which the participants’ goal was to drive as fast as possible using arrows on a keyboard, without crashing into another car,
- a delay-discounting task in which participants chose between different rewards offered at various points in the future, and
- a go/no-go task in which participants needed to press or not press a key on a keyboard in response to text that appeared on screen.
In line with previous research, Lee and colleagues found a gap between participants’ BIS scores and their performance on the delay-discounting and go/no-go tasks. However, participants’ BIS score significantly correlated with their overall performance on the driving task.
“The results support our hypothesis that a real-time task in a realistic environment would better reflect impulsivity than traditional trial-based tasks,” Lee and colleagues explained. “Behavioral task measures can represent individual traits measured with a self-report questionnaire if the task offers a wide range of states in which participants can exhibit diverse behaviors as they do in real-world situations.”
The researchers also found that a deep neural network (DNN) model—trained by the IRL algorithm with data about participants’ driving task performance—was able to identify new indicators of impulsivity that corresponded with the participants’ BIS scores. DNNs can identify complex relationships between actions and rewards that may not be apparent to human observers, the researchers explained.
In this case, the algorithm compared participants’ actual actions (moving up, no action, moving down, acceleration, and deceleration) with the hypothetical actions generated by an artificial participant operating according to the DNN model. The closer the artificial participants’ actions matched those of the real participants, the more accurate the algorithms’ DNN model appeared to be. The model’s average accuracy was found to be 64%, much higher than the chance rate of 20%.
Related content we think you’ll enjoy
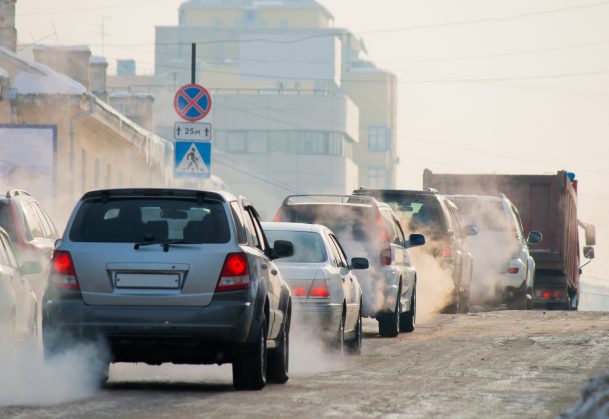
Driving and Cars
How do motorists develop the higher-order cognitive processes required to navigate safely? Research explores this and more.
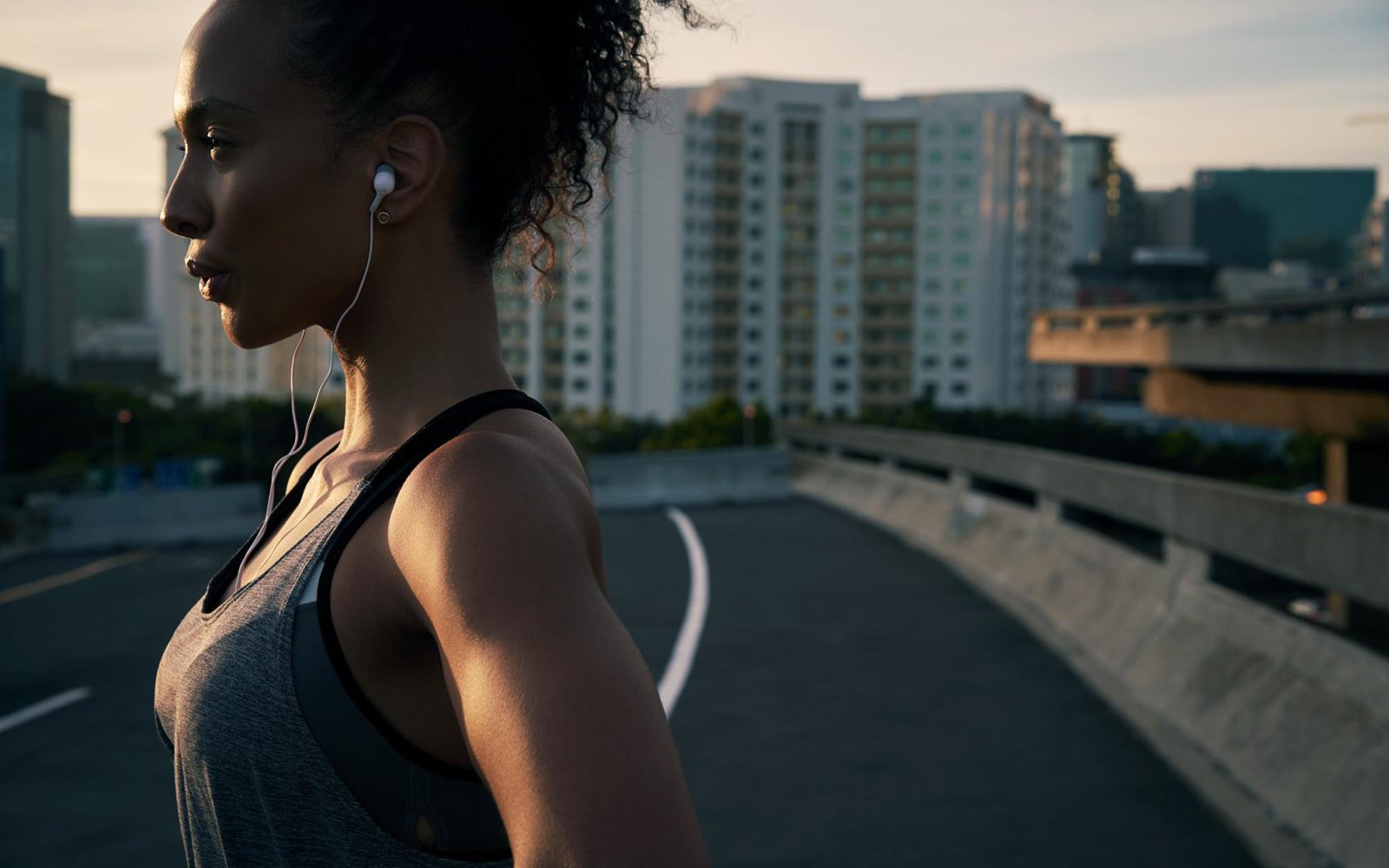
Teaching: Adolescent Self-Control / Loyalty Benefits and Backfires
Lesson plans about self-control in adolescents and how loyalty can lead us to act ethically or unethically.
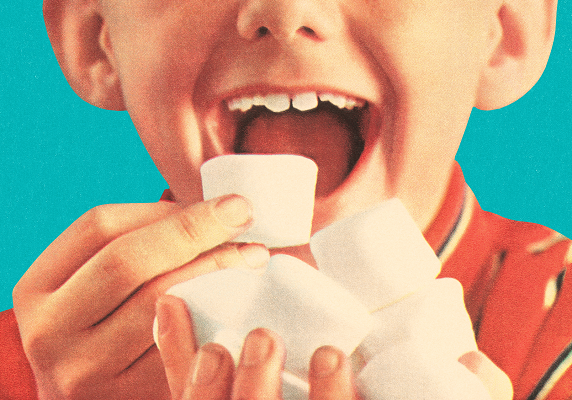
Children Will Wait to Impress Others—Another Twist on the Classic Marshmallow Test
When it comes to self-control, young children are better able to resist temptation and wait for greater rewards if they take into consideration the opinions of others.
The researchers said they also found an important difference between human and artificial participants: humans chose to take no action more often than artificial participants, possibly because the algorithms do not fully account for the physical cost of actions.
“Although the IRL agents learn from human demonstrations that reflect constraints on human behaviors, they might not replicate infrequent inaction because of fatigue or inattention in situations in which the participant typically took action,” Lee and colleagues wrote.
Nonetheless, the DNN model trained by the IRL algorithm was able to identify that participants were motivated by at least two features during the driving task: the speed of their own car and their distance from the car ahead of them in the same lane. Although most participants were motivated to drive at low to moderate speed while maintaining a close to moderate distance from the car ahead of them, participants with higher BIS scores, and thus higher impulsivity, were found to drive faster and closer to the car ahead of them.
More impulsive participants were also less likely to decelerate before crashing and more likely to change lane immediately before passing a car, rather than switching lanes at a greater distance.
“We found stronger indicators of impulsivity from IRL rewards than from summary statistics (e.g., mean speed, number of crashes). This suggests that IRL offers more than just a descriptive analysis because the reward functions can provide insights into participants’ characteristics that may not be apparent in their behaviors,” Lee and colleagues wrote.
In future work, the researchers plan to investigate how people with mental health conditions associated with heightened impulsivity perform on the highway task, as well as the neural correlates of IRL reward functions. Incorporating more realistic laboratory tasks into neuropsychological assessment could help improve their validity by measuring patients’ behaviors under more naturalistic conditions, Lee and colleagues wrote.
Feedback on this article? Email [email protected] or login to comment .
Reference
Lee, S. H., Song, M. S., Oh, M., & Ahn, Y. (2024). Bridging the gap between self-report and behavioral laboratory measures: A real-time driving task with inverse reinforcement learning. Psychological Science. https://doi.org/10.1177/09567976241228503
APS regularly opens certain online articles for discussion on our website. Effective February 2021, you must be a logged-in APS member to post comments. By posting a comment, you agree to our Community Guidelines and the display of your profile information, including your name and affiliation. Any opinions, findings, conclusions, or recommendations present in article comments are those of the writers and do not necessarily reflect the views of APS or the article’s author. For more information, please see our Community Guidelines .
Please login with your APS account to comment.

Cattell Fund Projects Include Research on Children’s Executive Function, Empathy Choice, and More
The James McKeen Cattell Fund has recognized APS Fellow Stephanie M. Carlson, C. Daryl Cameron, Robert Hampton, and Kevin Holmes as recipients of its Sabbatical Fund Fellowship for 2023–2024.

Embracing Discomfort Can Open Our Minds to New Ideas
When trying something new, discomfort might feel like a sign we’re in over our heads. Embracing these feelings as a part of learning could help motivate personal growth.
Privacy Overview
Vanderbilt to establish a college dedicated to computing, AI and data science
Media inquiries.
- 615-322-6397 Email
Latest Stories
- Vanderbilt University Statement – March 28, 2024
- Lorrie Moore wins prestigious National Book Critics Circle Award, continues to gather accolades for new novel
- Teams to create one-stop resource for human pancreatic data to foster diabetes research
Mar 25, 2024, 11:20 AM
Vanderbilt has begun work to establish a transformative college dedicated to computer science, AI, data science and related fields, university leaders announced today. In addition to meeting the growing demand for degrees in technological fields and advancing research in rapidly evolving, computing-related disciplines, the new, interdisciplinary college will collaborate with all of Vanderbilt’s schools and colleges to advance breakthrough discoveries and strengthen computing education through a “computing for all” approach.
The College of Connected Computing will be led by a new dean, who will report to Provost and Vice Chancellor for Academic Affairs C. Cybele Raver and to School of Engineering Dean Krishnendu “Krish” Roy. The search for the college’s dean is scheduled to begin in late August, and recruiting of faculty will begin in the coming months. It will be the first new college at Vanderbilt since the university and the Blair School of Music merged in 1981.
“Of all the factors shaping society, few are more influential than the rapid emergence of advanced computing, AI and data science,” Chancellor Daniel Diermeier said. “To continue to carry out our mission, prepare all our students for their careers and advance research across the university, Vanderbilt must contribute even more to the study, understanding and innovative application of these fast-changing disciplines. Our aim is to make Vanderbilt a global leader in these fields, ensuring our continued academic excellence and capacity for world-changing innovation.”
“Our new college will enable us to build upon our strong programs and catapult Vanderbilt to the forefront of breakthrough discovery and innovation—in key areas of computer science and also in a wide range of other disciplines that capitalize on advanced computational methods. In launching this new college, we will provide students with highest-caliber educational opportunities at the intersection of these pathbreaking fields,” Raver said. “The creation of this college represents a tremendous win and will be transformative for our entire university community.”
Raver noted the ways that Vanderbilt is forging a bold and distinct strategic path to address burgeoning research and educational opportunities, including increasing demand for expertise in computing-related fields. Moreover, she said, the global interest in AI “aligns perfectly” with Vanderbilt’s leading work in that field. She said a dedicated college will enable Vanderbilt to keep making groundbreaking discoveries at the intersections of computing and other disciplines and will more effectively leverage advanced computing to address some of society’s most pressing challenges.
“The establishment of this interdisciplinary, ‘cross-cutting’ college is a watershed moment—not only for the School of Engineering, but also for the entire university,” Roy said. “The future of education, research and thinking in all disciplines is now inherently tied to, and will be greatly influenced by, the knowledge and power of computing. The idea of ‘computing for all’ is fundamental to the future of learning.”
Many of the specific details about the college—including its departments, degree programs and research infrastructure—will be informed by the recommendations of a task force on connected computing composed of faculty from across the university. In addition, Vice Provost for Research and Innovation Padma Raghavan will launch a Computing Catalyst working group that will engage faculty and staff leaders in computing from across campus and solicit their input on strategically expanding the university’s computing resources. “The decision to establish this new college is rooted in conversations with faculty,” Raver said. “We are continuing that faculty engagement with this working group, and we’re fortunate to have the advice of some of the best minds in these fields as we embark on this exciting journey.”
The members of the Connected Computing Task Force include:
Krishnendu Roy , Chair Bruce and Bridgitt Evans Dean of Engineering University Distinguished Professor of Biomedical Engineering; Pathology, Microbiology and Immunology; and Chemical and Biomolecular Engineering
Douglas Adams Vice Dean of the School of Engineering Daniel F. Flowers Chair Distinguished Professor of Civil and Environmental Engineering Professor of Mechanical Engineering Faculty Affiliate, VINSE
Hiba Baroud Associate Chair and Associate Professor of Civil and Environmental Engineering James and Alice B. Clark Foundation Faculty Fellow Associate Professor of Computer Science Faculty Affiliate, VECTOR , Data Science Institute
Gautam Biswas Cornelius Vanderbilt Professor of Computer Science and Computer Engineering Professor of Engineering Management Senior Research Scientist, ISIS Faculty Affiliate, Data Science Institute
Erin Calipari Associate Professor of Pharmacology Associate Professor of Molecular Physiology & Biophysics Associate Professor of Psychiatry & Behavioral Sciences Director, Vanderbilt Center for Addiction Research Faculty Affiliate, Vanderbilt Brain Institute
Laurie Cutting Patricia and Rodes Hart Professor and Professor of Special Education Professor of Psychology Professor of Pediatrics Professor of Electrical and Computer Engineering Professor of Radiology & Radiological Sciences Associate Provost in the Office of the Vice Provost of Research and Innovation Associate Director of the Vanderbilt Kennedy Center Faculty Affiliate, Vanderbilt Brain Institute
Benoit Dawant Cornelius Vanderbilt Professor of Electrical Engineering Incoming Chair of the Department of Electrical and Computer Engineering Director and Steering Committee Chair, Vanderbilt Institute for Surgery & Engineering Professor of Biomedical Engineering Professor of Computer Science
Abhishek Dubey Associate Professor of Computer Science Associate Professor of Electrical and Computer Engineering Director, SCOPE lab at ISIS Faculty Affiliate, Institute for Software Integrated Systems and Data Science Institute
Bennett Landman Stevenson Professor of Electrical and Computer Engineering and Chair of the Department of Electrical and Computer Engineering Professor of Biomedical Engineering Professor of Computer Science Professor of Neurology Associate Professor of Biomedical Informatics Associate Professor of Psychiatry and Behavioral Sciences Associate Professor of Radiology and Radiological Sciences Faculty Affiliate, Vanderbilt Institute for Surgery and Engineering (VISE) , Vanderbilt Brain Institute , Vanderbilt Kennedy Center , Vanderbilt University Institute of Image Science (VUIIS) , Data Science Institute
Michael Matheny Professor of Biomedical Informatics Professor of Biostatistics Professor of Medicine Director, Center for Improving the Public’s Health Through Informatics
Sandeep Neema Professor of Computer Science Professor of Electrical and Computer Engineering Chair of the Executive Council, Institute for Software Integrated Systems
Ipek Oguz Assistant Professor of Computer Science Assistant Professor of Biomedical Engineering Assistant Professor of Electrical & Computer Engineering Faculty Affiliate, Vanderbilt Institute for Surgery and Engineering (VISE)
J.B. Ruhl David Daniels Allen Distinguished Chair of Law Director, Program in Law and Innovation Co-Director, Energy, Environment and Land Use Program Faculty Affiliate, Data Science Institute
Jesse Spencer-Smith Professor of the Practice of Computer Science Adjunct Professor of Psychology Interim Director and Chief Data Scientist, Data Science Institute
Jonathan Sprinkle Professor of Computer Science Professor of Electrical & Computer Engineering Professor of Civil & Environmental Engineering Faculty Affiliate, Institute for Software Integrated Systems
Yuankai “Kenny” Tao Associate Professor of Biomedical Engineering Associate Professor of Ophthalmology & Visual Sciences SPIE Faculty Fellow in Engineering Faculty Affiliate, Vanderbilt Institute for Surgery & Engineering
Holly Tucker Mellon Foundation Chair in the Humanities Professor of French Director, Robert Penn Warren Center for the Humanities
Kalman Varga Vice Chair of the Department of Physics & Astronomy Professor of Physics Director, Minor in Scientific Computing Faculty Affiliate, VINSE
Steven Wernke Chair of the Department of Anthropology Associate Professor of Anthropology Director, Vanderbilt Initiative for Interdisciplinary Geospatial Research Faculty Affiliate, Data Science Institute
Jules White Professor of Computer Science Associate Professor of Biomedical Informatics Senior Advisor to the Chancellor for Generative AI in Education and Enterprise Solutions Faculty Affiliate, Institute for Software Integrated Systems , Data Science Institute
Dan Work Director of Graduate Studies in Civil Engineering Professor of Civil & Environmental Engineering Professor of Computer Science Faculty Affiliate, VECTOR , Institute for Software Integrated Systems , Data Science Institute
Tracey George ex officio Vice Provost for Faculty Affairs and Professional Education Charles B. Cox III and Lucy D. Cox Family Chair in Law and Liberty Professor of Law
Tiffiny Tung Ex officio Vice Provost for Undergraduate Education Gertrude Conaway Vanderbilt Chair in the Social and Natural Sciences Professor of Anthropology
Members of the Vanderbilt community can learn more about this initiative and share feedback with the faculty working group by visiting vanderbilt.edu/about/computingtaskforce .
Keep Reading
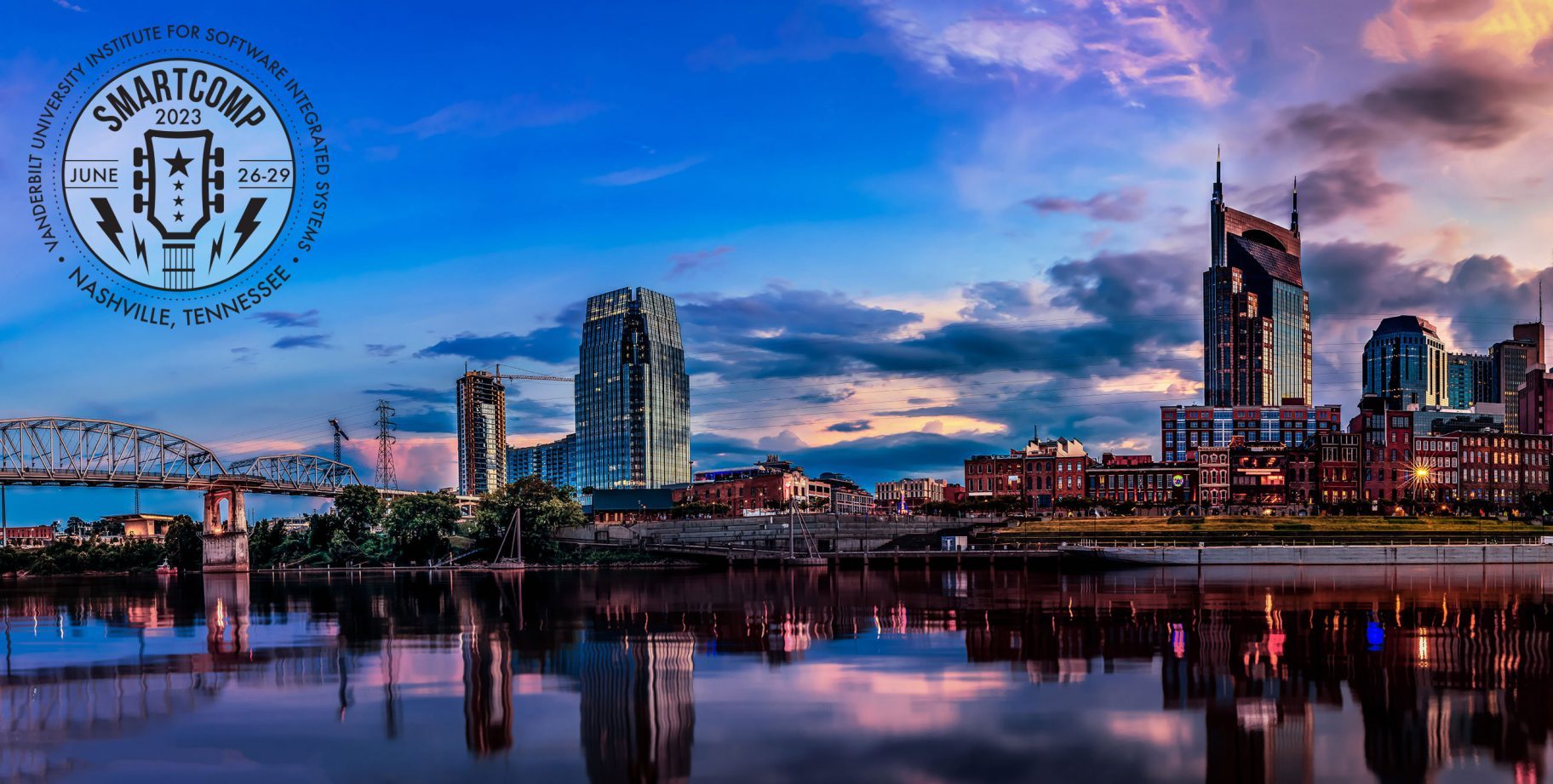
Vanderbilt hosts SmartComp 2023, prestigious IEEE international conference on smart computing
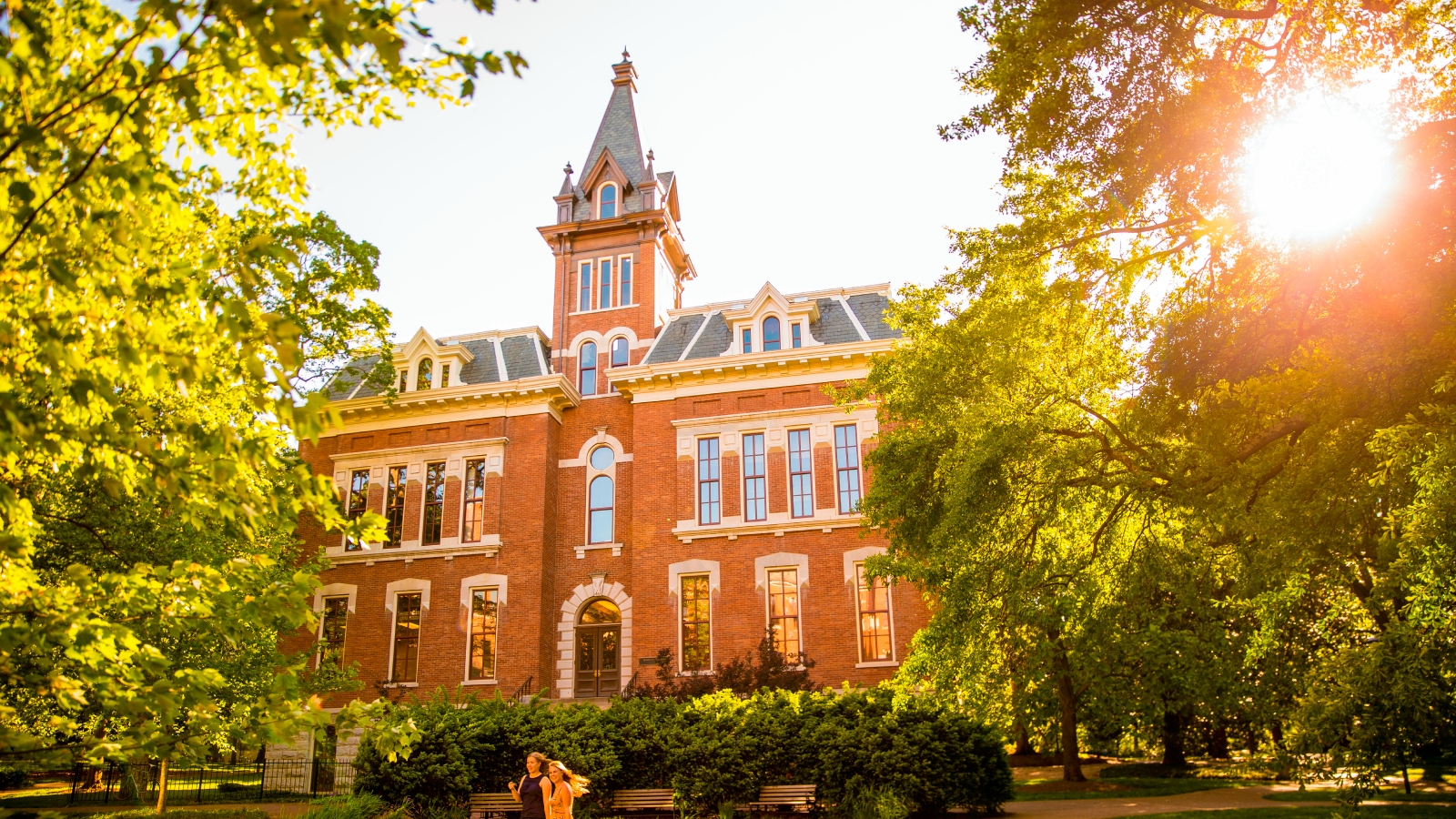
‘U.S. News’ graduate school rankings: Here is how Vanderbilt graduate and professional programs placed
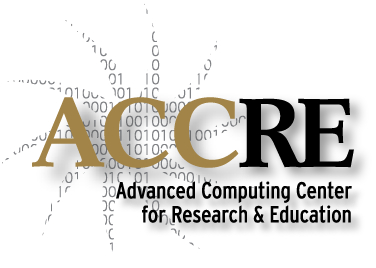
Vanderbilt announces search for new ACCRE executive director
Explore story topics.
- Engineering and Technology
- myVU Latest Headlines
- C. Cybele Raver
- College of Connected Computing
- Connected Computing Task Force
- Daniel Diermeier
- feature myvu
- featured myvu
- Office of the Chancellor
- Office of the Provost
- School of Engineering
- vuhome-highlight
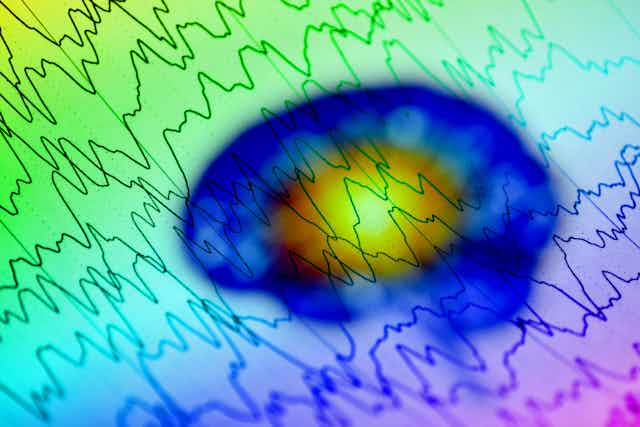
‘Noisy’ autistic brains seem better at certain tasks. Here’s why neuroaffirmative research matters
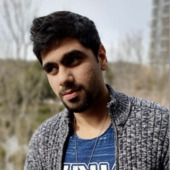
PhD candidiate, University of Canberra
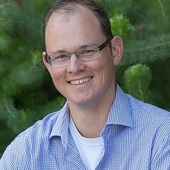
Associate professor, University of Canberra
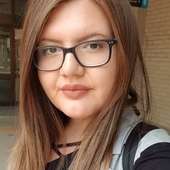
Honours Graduate Student, University of Canberra
Disclosure statement
Jeroen van Boxtel receives funding from Australian Government through an Australian Research Council Discovery Project (project number DP220100406), the ACT government.
Jovana Acevska and Pratik Raul do not work for, consult, own shares in or receive funding from any company or organisation that would benefit from this article, and have disclosed no relevant affiliations beyond their academic appointment.
University of Canberra provides funding as a member of The Conversation AU.
View all partners
Autism is a neurodevelopmental difference associated with specific experiences and characteristics.
For decades, autism research has focused on behavioural, cognitive, social and communication difficulties. These studies highlighted how autistic people face issues with everyday tasks that allistic (meaning non-autistic) people do not. Some difficulties may include recognising emotions or social cues.
But some research, including our own study, has explored specific advantages in autism. Studies have shown that in some cognitive tasks, autistic people perform better than allistic people. Autistic people may have greater success in identifying a simple shape embedded within a more complex design , arranging blocks of different shapes and colours , or spotting an object within a cluttered visual environment (similar to Where’s Wally?). Such enhanced performance has been recorded in babies as young as nine months who show emerging signs of autism.
How and why do autistic individuals do so well on these tasks? The answer may be surprising: more “neural noise”.
Read more: From deficits to a spectrum, thinking around autism has changed. Now there are calls for a 'profound autism' diagnosis
What is neural noise?
Generally, when you think of noise, you probably think of auditory noise, the ups and downs in the amplitude of sound frequencies we hear.
A similar thing happens in the brain with random fluctuations in neural activity. This is called neural noise.
This noise is always present, and comes on top of any brain activity caused by things we see, hear, smell and touch. This means that in the brain, an identical stimulus that is presented multiple times won’t cause exactly the same activity. Sometimes the brain is more active, sometimes less. In fact, even the response to a single stimulus or event will fluctuate continuously.
Neural noise in autism
There are many sources of neural noise in the brain. These include how the neurons become excited and calm again, changes in attention and arousal levels, and biochemical processes at the cellular level, among others. An allistic brain has mechanisms to manage and use this noise . For instance, cells in the hippocampus (the brain’s memory system) can make use of neural noise to enhance memory encoding and recall.
Evidence for high neural noise in autism can be seen in electroencephalography (EEG) recordings , where increased levels of neural fluctuations were observed in autistic children. This means their neural activity is less predictable, showing a wider range of activity (higher ups and downs) in response to the same stimulus.
In simple terms, if we imagine the EEG responses like a sound wave, we would expect to see small ups and downs (amplitude) in allistic brains each time they encounter a stimulus. But autistic brains seem to show bigger ups and downs, demonstrating greater amplitude of neural noise.
Many studies have linked this noisy autistic brain with cognitive, social and behavioural difficulties .
Read more: Most adults with autism can recognise facial emotions, almost as well as those without the condition
But could noise be a bonus?
The diagnosis of autism has a long clinical history . A shift from the medical to a more social model has also seen advocacy for it to be reframed as a difference, rather than a disorder or deficit. This change has also entered autism research. Neuroaffirming research can examine the uniqueness and strengths of neurodivergence.
Psychology and perception researcher David Simmons and colleagues at the University of Glasgow were the first to suggest that while high neural noise is generally a disadvantage in autism, it can sometimes provide benefits due to a phenomenon called stochastic resonance . This is where optimal amounts of noise can enhance performance . In line with this theory, high neural noise in the autistic brain might enhance performance for some cognitive tasks.
Our 2023 research explores this idea . We recruited participants from the general population and investigated their performance on letter-detection tasks. At the same time, we measured their level of autistic traits.
We performed two letter-detection experiments (one in a lab and one online) where participants had to identify a letter when displayed among background visual static of various intensities.
By using the static, we added additional visual noise to the neural noise already present in our participants’ brains. We hypothesised the visual noise would push participants with low internal brain noise (or low autistic traits) to perform better (as suggested by previous research on stochastic resonance). The more interesting prediction was that noise would not help individuals who already had a lot of brain noise (that is, those with high autistic traits), because their own neural noise already ensured optimal performance.
Indeed, one of our experiments showed people with high neural noise (high autistic traits) did not benefit from additional noise. Moreover, they showed superior performance (greater accuracy) relative to people with low neural noise when the added visual static was low. This suggests their own neural noise already caused a natural stochastic resonance effect, resulting in better performance.
It is important to note we did not include clinically diagnosed autistic participants, but overall, we showed the theory of enhanced performance due to stochastic resonance in autism has merits.
Read more: Autism is still underdiagnosed in girls and women. That can compound the challenges they face
Why this is important?
Autistic people face ignorance, prejudice and discrimination that can harm wellbeing . Poor mental and physical health, reduced social connections and increased “camouflaging” of autistic traits are some of the negative impacts that autistic people face.
So, research underlining and investigating the strengths inherent in autism can help reduce stigma, allow autistic people to be themselves and acknowledge autistic people do not require “fixing”.
The autistic brain is different. It comes with limitations, but it also has its strengths.
- Autism research
- Disability coverage

Biocloud Project Manager - Australian Biocommons

Director, Defence and Security

Opportunities with the new CIEHF

School of Social Sciences – Public Policy and International Relations opportunities

Deputy Editor - Technology
- See us on facebook
- See us on twitter
- See us on youtube
- See us on linkedin
- See us on instagram
Generative AI develops potential new drugs for antibiotic-resistant bacteria
Stanford Medicine researchers devise a new artificial intelligence model, SyntheMol, which creates recipes for chemists to synthesize the drugs in the lab.
March 28, 2024 - By Rachel Tompa
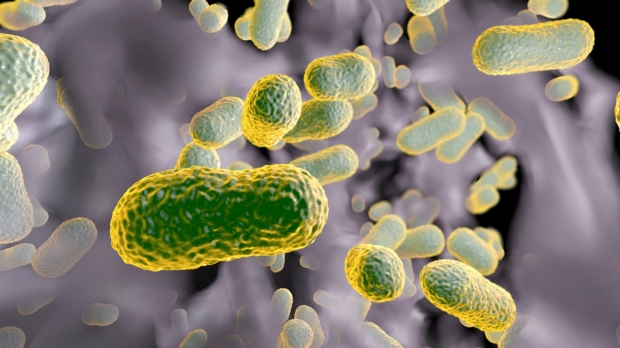
Acinetobacter baumannii infection is a leading cause of death related to antibiotic resistance. Stanford Medicine researchers employed artificial intelligence to provide recipes for drugs that can treat it. Kateryna Kon /Shutterstock.com
With nearly 5 million deaths linked to antibiotic resistance globally every year, new ways to combat resistant bacterial strains are urgently needed.
Researchers at Stanford Medicine and McMaster University are tackling this problem with generative artificial intelligence. A new model, dubbed SyntheMol (for synthesizing molecules), created structures and chemical recipes for six novel drugs aimed at killing resistant strains of Acinetobacter baumannii, one of the leading pathogens responsible for antibacterial resistance-related deaths.
The researchers described their model and experimental validation of these new compounds in a study published March 22 in the journal Nature Machine Intelligence .
“There’s a huge public health need to develop new antibiotics quickly,” said James Zou , PhD, an associate professor of biomedical data science and co-senior author on the study. “Our hypothesis was that there are a lot of potential molecules out there that could be effective drugs, but we haven’t made or tested them yet. That’s why we wanted to use AI to design entirely new molecules that have never been seen in nature.”
Before the advent of generative AI, the same type of artificial intelligence technology that underlies large language models like ChatGPT, researchers had taken different computational approaches to antibiotic development. They used algorithms to scroll through existing drug libraries, identifying those compounds most likely to act against a given pathogen. This technique, which sifted through 100 million known compounds , yielded results but just scratched the surface in finding all the chemical compounds that could have antibacterial properties.
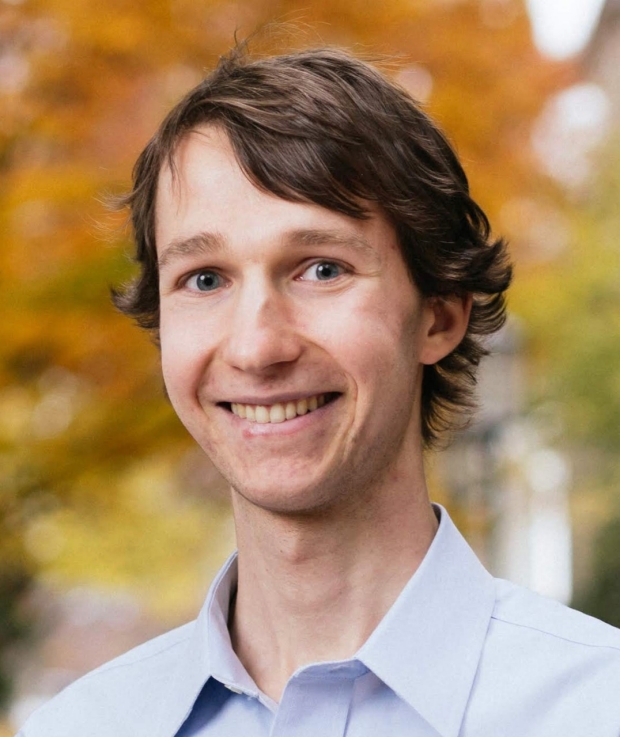
Kyle Swanson
“Chemical space is gigantic,” said Kyle Swanson , a Stanford computational science doctoral student and co-lead author on the study. “People have estimated that there are close to 10 60 possible drug-like molecules. So, 100 million is nowhere close to covering that entire space.”
Hallucinating for drug development
Generative AI’s tendency to “hallucinate,” or make up responses out of whole cloth, could be a boon when it comes to drug discovery, but previous attempts to generate new drugs with this kind of AI resulted in compounds that would be impossible to make in the real world, Swanson said. The researchers needed to put guardrails around SyntheMol’s activity — namely, to ensure that any molecules the model dreamed up could be synthesized in a lab.
“We’ve approached this problem by trying to bridge that gap between computational work and wet lab validation,” Swanson said.
The model was trained to construct potential drugs using a library of more than 130,000 molecular building blocks and a set of validated chemical reactions. It generated not only the final compound but also the steps it took with those building blocks, giving the researchers a set of recipes to produce the drugs.
The researchers also trained the model on existing data of different chemicals’ antibacterial activity against A. baumannii . With these guidelines and its building block starting set, SyntheMol generated around 25,000 possible antibiotics and the recipes to make them in less than nine hours. To prevent the bacteria from quickly developing resistance to the new compounds, researchers then filtered the generated compounds to only those that were dissimilar from existing compounds.
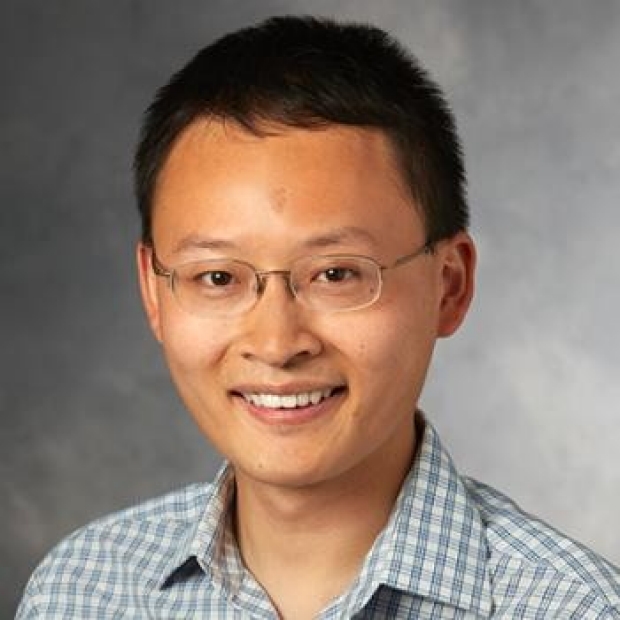
“Now we have not just entirely new molecules but also explicit instructions for how to make those molecules,” Zou said.
A new chemical space
The researchers chose the 70 compounds with the highest potential to kill the bacterium and worked with the Ukrainian chemical company Enamine to synthesize them. The company was able to efficiently generate 58 of these compounds, six of which killed a resistant strain of A. baumannii when researchers tested them in the lab. These new compounds also showed antibacterial activity against other kinds of infectious bacteria prone to antibiotic resistance, including E. coli, Klebsiella pneumoniae and MRSA.
The scientists were able to further test two of the six compounds for toxicity in mice, as the other four didn’t dissolve in water. The two they tested seemed safe; the next step is to test the drugs in mice infected with A. baumannii to see if they work in a living body, Zou said.
The six compounds are vastly different from each other and from existing antibiotics. The researchers don’t know how their antibacterial properties work at the molecular level, but exploring those details could yield general principles relevant to other antibiotic development.
“This AI is really designing and teaching us about this entirely new part of the chemical space that humans just haven’t explored before,” Zou said.
Zou and Swanson are also refining SyntheMol and broadening its reach. They’re collaborating with other research groups to use the model for drug discovery for heart disease and to create new fluorescent molecules for laboratory research.
The study was funded by the Weston Family Foundation, the David Braley Centre for Antibiotic Discovery, the Canadian Institutes of Health Research, M. and M. Heersink, the Chan-Zuckerberg Biohub, and the Knight-Hennessy scholarship.
For more news about responsible AI in health and medicine, sign up for the RAISE Health newsletter.
Register for the RAISE Health Symposium on May 14.
- Rachel Tompa Rachel Tompa is a freelance science writer.
About Stanford Medicine
Stanford Medicine is an integrated academic health system comprising the Stanford School of Medicine and adult and pediatric health care delivery systems. Together, they harness the full potential of biomedicine through collaborative research, education and clinical care for patients. For more information, please visit med.stanford.edu .
Artificial intelligence
Exploring ways AI is applied to health care
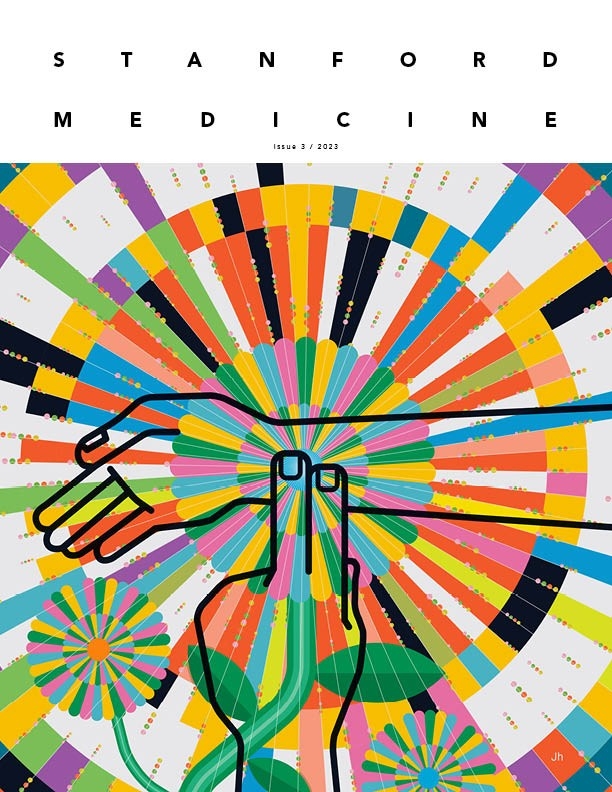
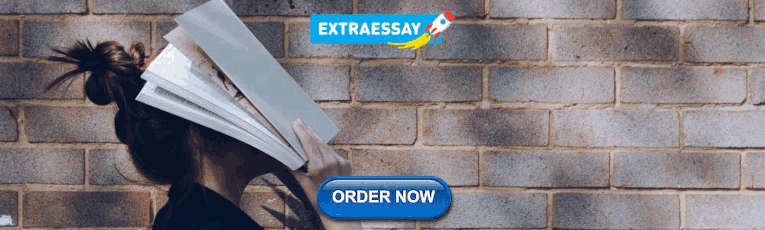
IMAGES
VIDEO
COMMENTS
The Guide walks the reader through the three stages of research strategy development: inform, create, and implement. 5.1.1 Inform. When creating a research strategy, there are three different stages. First, it is important to begin by collecting information to inform the research approach and strategy.
The 6 stages are: Task Definition (developing a topic) Information Seeking (coming up with a research plan) Location & Access (finding good sources) Use of Information (Reading, taking notes, and generally making the writing process easier) Synthesis (coming up with your own ideas and presenting them well)
The concept of research is as old as science; the concept of the intimate relationship between research and subsequent development, however, was not generally recognized until the 1950s. Research and development is the beginning of most systems of industrial production. The innovations that result in new products and new processes usually have ...
Research And Development - R&D: Research and development (R&D) refers to the investigative activities a business conducts to improve existing products and procedures or to lead to the development ...
Definition of R&D. Research and development (R&D) is essential for a business's growth. R&D is about creating new knowledge. Companies do R&D to develop new product or service ideas, improve current ones, or add new features. The main goal of R&D is to create products that enhance the company's lineup. R&D doesn't usually give instant results.
The management of research and development activities. Most research and development projects are examples of a project, or one-shot, production system.Here, as opposed to the ongoing activity found in batch or continuous systems, resources are brought together for a period of time, focused on a particular task, such as the development of a new product, and then disbanded and reassigned.
You have reactivated a Research Center on Eos. Test its capabilities by gathering the resources to craft a weapon. The small northeastern building beside the ND1 Nomad's crate can be opened once Pathfinder Ryder has restored power in Site 1: Promise. Interact with the Terminal (marked with a ) inside and acknowledge the "ACTIVATE: Power Relay" to start this mission. The earliest you can ...
The purpose of this paper is to provide an overview of the process and stages involved in developing a research idea from its inception to realisation. It is not designed to be an all encompassing summary of the research process. It fprovides a brief guide to the most common sequence of stages involved in the development of a research idea into a viable research proposal. Useful references for ...
Definition. Research and development (R&D) is a broad category describing the entity of basic research, applied research, and development activities. In general research and development means systematic activities in order to increase knowledge and use of this knowledge when developing new products, processes, or services.
Research and development ( R&D or R+D; also known in Europe as research and technological development or RTD) [1] is the set of innovative activities undertaken by corporations or governments in developing new services or products, and improving existing ones. [2] [3] Research and development constitutes the first stage of development of a ...
Companies often spend resources on certain investigative undertakings in an effort to make discoveries that can help develop new products or way of doing things or work towards enhancing pre-existing products or processes. These activities come under the Research and Development (R&D) umbrella. R&D is an important means for achieving future growth and maintaining a relevant product in the market.
As the level of attention residue from the first task increases, it is likely to inhibit the development of engagement in the subsequent task. The significance and implications of transitioning from an engaging but incomplete task were noted by several of the crew members in our sample. ... An integrative review and synthesis of research on ...
Research and development includes the many tasks a company undertakes in order to invent and introduce new products and services for consumers or clients. Most of these tasks involve tasks to better understand which specific products and services are most desired, useful and marketable to their clients or consumer base.
Start by defining your project's purpose. Identify what your project aims to accomplish and what you are researching. Remember to use clear language. Thinking about the project's purpose will help you set realistic goals and inform how you divide tasks and assign responsibilities.
Research development is a relatively new field and more and more institutions are establishing research development offices or expanding existing offices (Kuo, 2016 p. 2). Those involved currently self-identify as research development professionals or move into roles that have already been defined as being part of the field.
Key Takeaways. Developing a research proposal involves the following preliminary steps: identifying potential ideas, choosing ideas to explore further, choosing and narrowing a topic, formulating a research question, and developing a working thesis. A good topic for a research paper interests the writer and fulfills the requirements of the ...
Research and development (R&D) is the process of designing, conducting, and documenting experiments to make scientific and technological advances . The purpose of research and development is to increase knowledge, solve problems, and develop new technologies. And that will lead to new products, services, or ways of doing things.
Research and development (R&D) processes need agility beyond the software development space. Companies struggle to match agile frameworks to their R&D needs. As LMI generated an operating model to develop prototypes around innovative and crowdsourced ideas in short timelines (30-45 days), the difficulty became apparent. While LMI has mature ...
Research and development tasks have been proposed as a learning solution in teacher education, where dynamic subject-matter knowledge and professional practices, and increasingly complex teaching environments generate emerging challenges for teacher education institutions and students. While there is an awareness that research is a complex ...
Responsibilities for research & development. Design and implement scientific studies to support new technology development, enhancement of existing processes (includes data collection and statistical analysis) Updating charts with new standard numbers, fragrance information,and percentages.
Abstract. The term "developmental-task" was introduced by Robert Havighurst in the 1950's. According to R. Harvighurst, the term refers to tasks which arise in a social context during an ...
The interactive, conversational, analytical, and generative features of GenAI offer support for creativity, problem-solving, and processing and digestion of large bodies of information. Therefore ...
In this research memorandum, one of a series of eight such reports, we describe the development process by which we produced a series of performance tasks designed for preservice elementary teachers for formative assessment use in the context of teacher education programs. Each performance task provides an opportunity for preservice elementary
Now three of OpenAI's early research scientists say the startup they spun off in 2017, called Covariant, has solved that problem and unveiled a system that combines the reasoning skills of large ...
Havighurst was an American psychologist who developed his theory on Developmental Tasks between 1948 and 1953. His work came after the work of Sigmund Freud and Jean Piaget, but before Erik Erikson. He is also known for popularizing the phrase "teachable moments" in his 1952 book Human Development and Education.
Psychological research often relies on participants to report or reflect on their own behavior, but these perceptions don't always align with how they act in the real world or even during experiments in a laboratory.Lab experiments sometimes have participants engage in tasks that don't capture the full range of behaviors people display in their day-to-day lives, but pairing realistic tasks ...
Many of the specific details about the college—including its departments, degree programs and research infrastructure—will be informed by the recommendations of a task force on connected ...
For decades, autism research has focused on behavioural, cognitive, social and communication difficulties. These studies highlighted how autistic people face issues with everyday tasks that ...
The GARDEM contract vehicle provides research, development, prototyping, integration, testing, demonstration, deployment, and maintenance of innovative technologies and concepts on a continuous timeline through the contract life cycle. ... Parsons has won multiple task orders on the contract since it was first awarded in 2019. To learn more ...
Hallucinating for drug development Generative AI's tendency to "hallucinate," or make up responses out of whole cloth, could be a boon when it comes to drug discovery, but previous attempts to generate new drugs with this kind of AI resulted in compounds that would be impossible to make in the real world, Swanson said.