To read this content please select one of the options below:
Please note you do not have access to teaching notes, validity and reliability tests in case study research: a literature review with “hands‐on” applications for each research phase.
Qualitative Market Research
ISSN : 1352-2752
Article publication date: 1 June 2003
Despite the advantages of the case study method, its reliability and validity remain in doubt. Tests to establish the validity and reliability of qualitative data are important to determine the stability and quality of the data obtained. However, there is no single, coherent set of validity and reliability tests for each research phase in case study research available in the literature. This article presents an argument for the case study method in marketing research, examining various criteria for judging the quality of the method and highlighting various techniques, which can be addressed to achieve objectivity, and rigorous and relevant information for planning to marketing actions. The purpose of this article is to invite further research by discussing the use of various scientific techniques for establishing the validity and reliability in case study research. The article provides guidelines for achieving high validity and reliability for each phase in case study research.
- Case studies
- Qualitative techniques
- Reliability
Riege, A.M. (2003), "Validity and reliability tests in case study research: a literature review with “hands‐on” applications for each research phase", Qualitative Market Research , Vol. 6 No. 2, pp. 75-86. https://doi.org/10.1108/13522750310470055
Copyright © 2003, MCB UP Limited
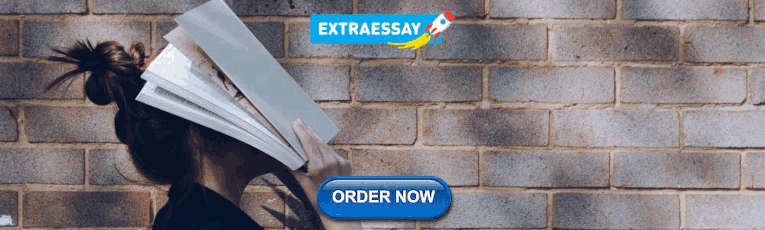
Related articles
We’re listening — tell us what you think, something didn’t work….
Report bugs here
All feedback is valuable
Please share your general feedback
Join us on our journey
Platform update page.
Visit emeraldpublishing.com/platformupdate to discover the latest news and updates
Questions & More Information
Answers to the most commonly asked questions here
Have a language expert improve your writing
Run a free plagiarism check in 10 minutes, generate accurate citations for free.
- Knowledge Base
Methodology
- The 4 Types of Validity in Research | Definitions & Examples
The 4 Types of Validity in Research | Definitions & Examples
Published on September 6, 2019 by Fiona Middleton . Revised on June 22, 2023.
Validity tells you how accurately a method measures something. If a method measures what it claims to measure, and the results closely correspond to real-world values, then it can be considered valid. There are four main types of validity:
- Construct validity : Does the test measure the concept that it’s intended to measure?
- Content validity : Is the test fully representative of what it aims to measure?
- Face validity : Does the content of the test appear to be suitable to its aims?
- Criterion validity : Do the results accurately measure the concrete outcome they are designed to measure?
In quantitative research , you have to consider the reliability and validity of your methods and measurements.
Note that this article deals with types of test validity, which determine the accuracy of the actual components of a measure. If you are doing experimental research, you also need to consider internal and external validity , which deal with the experimental design and the generalizability of results.
Table of contents
Construct validity, content validity, face validity, criterion validity, other interesting articles, frequently asked questions about types of validity.
Construct validity evaluates whether a measurement tool really represents the thing we are interested in measuring. It’s central to establishing the overall validity of a method.
What is a construct?
A construct refers to a concept or characteristic that can’t be directly observed, but can be measured by observing other indicators that are associated with it.
Constructs can be characteristics of individuals, such as intelligence, obesity, job satisfaction, or depression; they can also be broader concepts applied to organizations or social groups, such as gender equality, corporate social responsibility, or freedom of speech.
There is no objective, observable entity called “depression” that we can measure directly. But based on existing psychological research and theory, we can measure depression based on a collection of symptoms and indicators, such as low self-confidence and low energy levels.
What is construct validity?
Construct validity is about ensuring that the method of measurement matches the construct you want to measure. If you develop a questionnaire to diagnose depression, you need to know: does the questionnaire really measure the construct of depression? Or is it actually measuring the respondent’s mood, self-esteem, or some other construct?
To achieve construct validity, you have to ensure that your indicators and measurements are carefully developed based on relevant existing knowledge. The questionnaire must include only relevant questions that measure known indicators of depression.
The other types of validity described below can all be considered as forms of evidence for construct validity.
Receive feedback on language, structure, and formatting
Professional editors proofread and edit your paper by focusing on:
- Academic style
- Vague sentences
- Style consistency
See an example
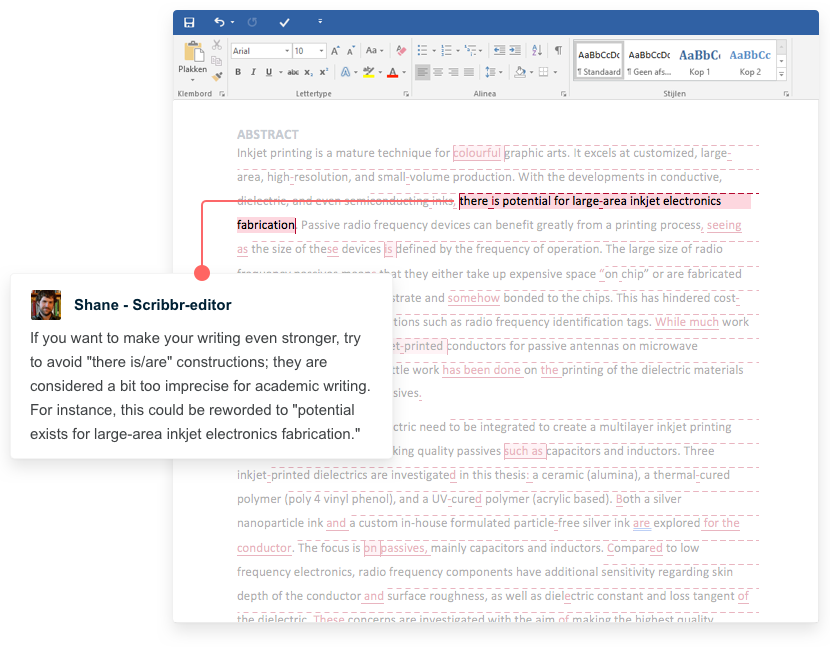
Content validity assesses whether a test is representative of all aspects of the construct.
To produce valid results, the content of a test, survey or measurement method must cover all relevant parts of the subject it aims to measure. If some aspects are missing from the measurement (or if irrelevant aspects are included), the validity is threatened and the research is likely suffering from omitted variable bias .
A mathematics teacher develops an end-of-semester algebra test for her class. The test should cover every form of algebra that was taught in the class. If some types of algebra are left out, then the results may not be an accurate indication of students’ understanding of the subject. Similarly, if she includes questions that are not related to algebra, the results are no longer a valid measure of algebra knowledge.
Face validity considers how suitable the content of a test seems to be on the surface. It’s similar to content validity, but face validity is a more informal and subjective assessment.
You create a survey to measure the regularity of people’s dietary habits. You review the survey items, which ask questions about every meal of the day and snacks eaten in between for every day of the week. On its surface, the survey seems like a good representation of what you want to test, so you consider it to have high face validity.
As face validity is a subjective measure, it’s often considered the weakest form of validity. However, it can be useful in the initial stages of developing a method.
Criterion validity evaluates how well a test can predict a concrete outcome, or how well the results of your test approximate the results of another test.
What is a criterion variable?
A criterion variable is an established and effective measurement that is widely considered valid, sometimes referred to as a “gold standard” measurement. Criterion variables can be very difficult to find.
What is criterion validity?
To evaluate criterion validity, you calculate the correlation between the results of your measurement and the results of the criterion measurement. If there is a high correlation, this gives a good indication that your test is measuring what it intends to measure.
A university professor creates a new test to measure applicants’ English writing ability. To assess how well the test really does measure students’ writing ability, she finds an existing test that is considered a valid measurement of English writing ability, and compares the results when the same group of students take both tests. If the outcomes are very similar, the new test has high criterion validity.
If you want to know more about statistics , methodology , or research bias , make sure to check out some of our other articles with explanations and examples.
- Normal distribution
- Degrees of freedom
- Null hypothesis
- Discourse analysis
- Control groups
- Mixed methods research
- Non-probability sampling
- Quantitative research
- Ecological validity
Research bias
- Rosenthal effect
- Implicit bias
- Cognitive bias
- Selection bias
- Negativity bias
- Status quo bias
Face validity and content validity are similar in that they both evaluate how suitable the content of a test is. The difference is that face validity is subjective, and assesses content at surface level.
When a test has strong face validity, anyone would agree that the test’s questions appear to measure what they are intended to measure.
For example, looking at a 4th grade math test consisting of problems in which students have to add and multiply, most people would agree that it has strong face validity (i.e., it looks like a math test).
On the other hand, content validity evaluates how well a test represents all the aspects of a topic. Assessing content validity is more systematic and relies on expert evaluation. of each question, analyzing whether each one covers the aspects that the test was designed to cover.
A 4th grade math test would have high content validity if it covered all the skills taught in that grade. Experts(in this case, math teachers), would have to evaluate the content validity by comparing the test to the learning objectives.
Criterion validity evaluates how well a test measures the outcome it was designed to measure. An outcome can be, for example, the onset of a disease.
Criterion validity consists of two subtypes depending on the time at which the two measures (the criterion and your test) are obtained:
- Concurrent validity is a validation strategy where the the scores of a test and the criterion are obtained at the same time .
- Predictive validity is a validation strategy where the criterion variables are measured after the scores of the test.
Convergent validity and discriminant validity are both subtypes of construct validity . Together, they help you evaluate whether a test measures the concept it was designed to measure.
- Convergent validity indicates whether a test that is designed to measure a particular construct correlates with other tests that assess the same or similar construct.
- Discriminant validity indicates whether two tests that should not be highly related to each other are indeed not related. This type of validity is also called divergent validity .
You need to assess both in order to demonstrate construct validity. Neither one alone is sufficient for establishing construct validity.
The purpose of theory-testing mode is to find evidence in order to disprove, refine, or support a theory. As such, generalizability is not the aim of theory-testing mode.
Due to this, the priority of researchers in theory-testing mode is to eliminate alternative causes for relationships between variables . In other words, they prioritize internal validity over external validity , including ecological validity .
It’s often best to ask a variety of people to review your measurements. You can ask experts, such as other researchers, or laypeople, such as potential participants, to judge the face validity of tests.
While experts have a deep understanding of research methods , the people you’re studying can provide you with valuable insights you may have missed otherwise.
Cite this Scribbr article
If you want to cite this source, you can copy and paste the citation or click the “Cite this Scribbr article” button to automatically add the citation to our free Citation Generator.
Middleton, F. (2023, June 22). The 4 Types of Validity in Research | Definitions & Examples. Scribbr. Retrieved April 2, 2024, from https://www.scribbr.com/methodology/types-of-validity/
Is this article helpful?
Fiona Middleton
Other students also liked, reliability vs. validity in research | difference, types and examples, construct validity | definition, types, & examples, external validity | definition, types, threats & examples, unlimited academic ai-proofreading.
✔ Document error-free in 5minutes ✔ Unlimited document corrections ✔ Specialized in correcting academic texts
Have a language expert improve your writing
Run a free plagiarism check in 10 minutes, automatically generate references for free.
- Knowledge Base
- Methodology
- Reliability vs Validity in Research | Differences, Types & Examples
Reliability vs Validity in Research | Differences, Types & Examples
Published on 3 May 2022 by Fiona Middleton . Revised on 10 October 2022.
Reliability and validity are concepts used to evaluate the quality of research. They indicate how well a method , technique, or test measures something. Reliability is about the consistency of a measure, and validity is about the accuracy of a measure.
It’s important to consider reliability and validity when you are creating your research design , planning your methods, and writing up your results, especially in quantitative research .
Table of contents
Understanding reliability vs validity, how are reliability and validity assessed, how to ensure validity and reliability in your research, where to write about reliability and validity in a thesis.
Reliability and validity are closely related, but they mean different things. A measurement can be reliable without being valid. However, if a measurement is valid, it is usually also reliable.
What is reliability?
Reliability refers to how consistently a method measures something. If the same result can be consistently achieved by using the same methods under the same circumstances, the measurement is considered reliable.
What is validity?
Validity refers to how accurately a method measures what it is intended to measure. If research has high validity, that means it produces results that correspond to real properties, characteristics, and variations in the physical or social world.
High reliability is one indicator that a measurement is valid. If a method is not reliable, it probably isn’t valid.
However, reliability on its own is not enough to ensure validity. Even if a test is reliable, it may not accurately reflect the real situation.
Validity is harder to assess than reliability, but it is even more important. To obtain useful results, the methods you use to collect your data must be valid: the research must be measuring what it claims to measure. This ensures that your discussion of the data and the conclusions you draw are also valid.
Prevent plagiarism, run a free check.
Reliability can be estimated by comparing different versions of the same measurement. Validity is harder to assess, but it can be estimated by comparing the results to other relevant data or theory. Methods of estimating reliability and validity are usually split up into different types.
Types of reliability
Different types of reliability can be estimated through various statistical methods.
Types of validity
The validity of a measurement can be estimated based on three main types of evidence. Each type can be evaluated through expert judgement or statistical methods.
To assess the validity of a cause-and-effect relationship, you also need to consider internal validity (the design of the experiment ) and external validity (the generalisability of the results).
The reliability and validity of your results depends on creating a strong research design , choosing appropriate methods and samples, and conducting the research carefully and consistently.
Ensuring validity
If you use scores or ratings to measure variations in something (such as psychological traits, levels of ability, or physical properties), it’s important that your results reflect the real variations as accurately as possible. Validity should be considered in the very earliest stages of your research, when you decide how you will collect your data .
- Choose appropriate methods of measurement
Ensure that your method and measurement technique are of high quality and targeted to measure exactly what you want to know. They should be thoroughly researched and based on existing knowledge.
For example, to collect data on a personality trait, you could use a standardised questionnaire that is considered reliable and valid. If you develop your own questionnaire, it should be based on established theory or the findings of previous studies, and the questions should be carefully and precisely worded.
- Use appropriate sampling methods to select your subjects
To produce valid generalisable results, clearly define the population you are researching (e.g., people from a specific age range, geographical location, or profession). Ensure that you have enough participants and that they are representative of the population.
Ensuring reliability
Reliability should be considered throughout the data collection process. When you use a tool or technique to collect data, it’s important that the results are precise, stable, and reproducible.
- Apply your methods consistently
Plan your method carefully to make sure you carry out the same steps in the same way for each measurement. This is especially important if multiple researchers are involved.
For example, if you are conducting interviews or observations, clearly define how specific behaviours or responses will be counted, and make sure questions are phrased the same way each time.
- Standardise the conditions of your research
When you collect your data, keep the circumstances as consistent as possible to reduce the influence of external factors that might create variation in the results.
For example, in an experimental setup, make sure all participants are given the same information and tested under the same conditions.
It’s appropriate to discuss reliability and validity in various sections of your thesis or dissertation or research paper. Showing that you have taken them into account in planning your research and interpreting the results makes your work more credible and trustworthy.
Cite this Scribbr article
If you want to cite this source, you can copy and paste the citation or click the ‘Cite this Scribbr article’ button to automatically add the citation to our free Reference Generator.
Middleton, F. (2022, October 10). Reliability vs Validity in Research | Differences, Types & Examples. Scribbr. Retrieved 2 April 2024, from https://www.scribbr.co.uk/research-methods/reliability-or-validity/
Is this article helpful?
Fiona Middleton
Other students also liked, the 4 types of validity | types, definitions & examples, a quick guide to experimental design | 5 steps & examples, sampling methods | types, techniques, & examples.
Academia.edu no longer supports Internet Explorer.
To browse Academia.edu and the wider internet faster and more securely, please take a few seconds to upgrade your browser .
Enter the email address you signed up with and we'll email you a reset link.
- We're Hiring!
- Help Center
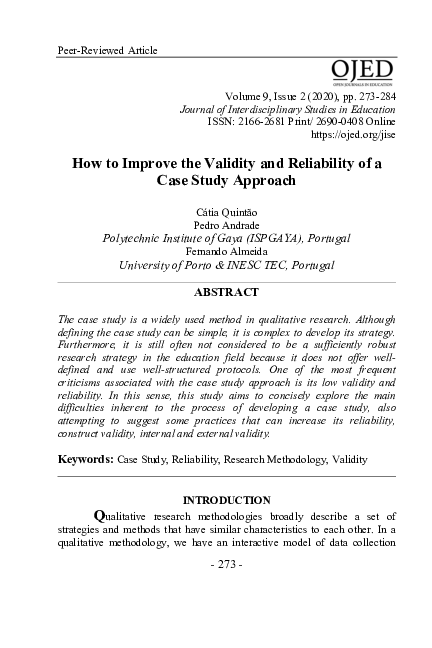
How to Improve the Validity and Reliability of a Case Study Approach

2020, Journal of Interdisciplinary Studies in Education
The case study is a widely used method in qualitative research. Although defining the case study can be simple, it is complex to develop its strategy. Furthermore, it is still often not considered to be a sufficiently robust research strategy in the education field because it does not offer well-defined and use well-structured protocols. One of the most frequent criticisms associated with the case study approach is its low validity and reliability. In this sense, this study aims to concisely explore the main difficulties inherent to the process of developing a case study, also attempting to suggest some practices that can increase its reliability, construct validity, internal and external validity. Qualitative research methodologies broadly describe a set of strategies and methods that have similar characteristics to each other. In a qualitative methodology, we have an interactive model of data collection
Related Papers
Anju Chhetri
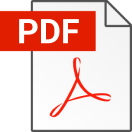
This article presents the case study as a type of qualitative research. Its aim is to give a detailed description of a case study-its definition, some classifications, and several advantages and disadvantages-in order to provide a better understanding of this widely used type of qualitative approac h. In comparison to other types of qualitative research, case studies have been little understood both from a methodological point of view, where disagreements exist about whether case studies should be considered a research method or a research type, and from a content point of view, where there are ambiguities regarding what should be considered a case or research subject. A great emphasis is placed on the disadvantages of case studies, where we try to refute some of the criticisms concerning case studies, particularly in comparison to quantitative research approaches.
A. Biba Rebolj
Dr. BABOUCARR NJIE
American Journal of Qualitative Research
Nikhil Chandra Shil, FCMA
Bedrettin Yazan
Case study methodology has long been a contested terrain in social sciences research which is characterized by varying, sometimes opposing, approaches espoused by many research methodologists. Despite being one of the most frequently used qualitative research methodologies in educational research, the methodologists do not have a full consensus on the design and implementation of case study, which hampers its full evolution. Focusing on the landmark works of three prominent methodologists, namely Robert Yin, Sharan Merriam, Robert Stake, I attempt to scrutinize the areas where their perspectives diverge, converge and complement one another in varying dimensions of case study research. I aim to help the emerging researchers in the field of education familiarize themselves with the diverse views regarding case study that lead to a vast array of techniques and strategies, out of which they can come up with a combined perspective which best serves their research purpose.
Thabit Alomari
Nina Oktavia
Case study is believed as the widely used kind of research to view phenomena, despite of some critics on it concerning mostly on its data reliability, validity and subjectivity. This article therefore discusses some aspects of case study which are considered important to be recognized by novice researchers, especially about the way how to design and how to make sure the quality and reliability of the case. In addition, the case studying educational research also becomes the focus to be discussed, completed with some examples, to be able to open our mind to the plenty opportunities for case study in education.
johnpatrick gonzales
Jesus eduardo Flores
Qualitative case study methodology provides tools for researchers to study complex phenomena within their contexts. When the approach is applied correctly, it becomes a valuable method for health science research to develop theory, evaluate programs, and develop interventions. The purpose of this paper is to guide the novice researcher in identifying the key elements for designing and implementing qualitative case study research projects. An overview of the types of case study designs is provided along with general recommendations for writing the research questions, developing propositions, determining the " case " under study, binding the case and a discussion of data sources and triangulation. To facilitate application of these principles, clear examples of research questions, study propositions and the different types of case study designs are provided. Key Words: Case Study and Qualitative Methods Introduction To graduate students and researchers unfamiliar with case study methodology, there is often misunderstanding about what a case study is and how it, as a form of qualitative research, can inform professional practice or evidence-informed decision making in both clinical and policy realms. In a graduate level introductory qualitative research methods course, we have listened to novice researchers describe their views of case studies and their perceptions of it as a method only to be used to study individuals or specific historical events, or as a teaching strategy to holistically understand exemplary " cases. " It has been a privilege to teach these students that rigorous qualitative case studies afford researchers opportunities to explore or describe a phenomenon in context using a variety of data sources. It allows the researcher to explore individuals or organizations, simple through complex interventions, relationships, communities, or programs (Yin, 2003) and supports the deconstruction and the subsequent reconstruction of various phenomena. This approach is valuable for health science research to develop theory, evaluate programs, and develop interventions because of its flexibility and rigor.
RELATED PAPERS
iJSRED Journal
Child's Nervous System
Tadanori Tomita
PROCEEDINGS OF THE EUROPEAN TRANSPORT CONFERENCE (ETC) 2007 HELD 17-19 OCTOBER 2007, LEIDEN, THE NETHERLANDS
Charlotte Marceau
Pesticide Science
Vincent Salgado
Journal of Special Education and Rehabilitation
Natasha Chichevska Jovanova
International microbiology : the official journal of the Spanish Society for Microbiology
Elisabetta Buommino
Sidney J A R D da Silva
Litterature Langages Et Arts Rencontres Et Creation 2007 Isbn 978 84 96826 15 1 Pag 73
Azucena Macho Vargas
Zeszyty Naukowe
Dominik Szczepański
Kahramanmaraş Sütçü İmam Üniversitesi Tarım ve Doğa Dergisi
Mustafa Kocakaya
imam santoso
Thomás Dorigon
Journal of Basic Design & Art
Stephanie Nuñez
Materials Research
Lucas Rocha
Synergismus Scyentifica Utfpr
Thiago Piva
Artefilosofia
Isabela Pinho
Jual Tongkat Komando Kabupaten Lumajang
Penjual Tongkat Komando
International Journal of Occupational Safety and Health
Sivabalan Kaniapan
Christian Gray
Pengaruh Budaya dan Etika Bisnis dalam Strategi Perusahaan di PT Untung Daya Perkasa Tambaknegara
MAN_ANISA LISDIYANTI
Didasc@lia: Didáctica y Educación
Hilário Freitas
Int. J. Integ. Biol
Savarimuthu Ignacimuthu
Daniel Siahaan
fawaz Akambi Aminou
Journal of Nano- and Electronic Physics
naoun mahieddine
See More Documents Like This
RELATED TOPICS
- We're Hiring!
- Help Center
- Find new research papers in:
- Health Sciences
- Earth Sciences
- Cognitive Science
- Mathematics
- Computer Science
- Academia ©2024
Log in using your username and password
- Search More Search for this keyword Advanced search
- Latest content
- Current issue
- Write for Us
- BMJ Journals More You are viewing from: Google Indexer
You are here
- Volume 18, Issue 3
- Validity and reliability in quantitative studies
- Article Text
- Article info
- Citation Tools
- Rapid Responses
- Article metrics

- Roberta Heale 1 ,
- Alison Twycross 2
- 1 School of Nursing, Laurentian University , Sudbury, Ontario , Canada
- 2 Faculty of Health and Social Care , London South Bank University , London , UK
- Correspondence to : Dr Roberta Heale, School of Nursing, Laurentian University, Ramsey Lake Road, Sudbury, Ontario, Canada P3E2C6; rheale{at}laurentian.ca
https://doi.org/10.1136/eb-2015-102129
Statistics from Altmetric.com
Request permissions.
If you wish to reuse any or all of this article please use the link below which will take you to the Copyright Clearance Center’s RightsLink service. You will be able to get a quick price and instant permission to reuse the content in many different ways.
Evidence-based practice includes, in part, implementation of the findings of well-conducted quality research studies. So being able to critique quantitative research is an important skill for nurses. Consideration must be given not only to the results of the study but also the rigour of the research. Rigour refers to the extent to which the researchers worked to enhance the quality of the studies. In quantitative research, this is achieved through measurement of the validity and reliability. 1
- View inline
Types of validity
The first category is content validity . This category looks at whether the instrument adequately covers all the content that it should with respect to the variable. In other words, does the instrument cover the entire domain related to the variable, or construct it was designed to measure? In an undergraduate nursing course with instruction about public health, an examination with content validity would cover all the content in the course with greater emphasis on the topics that had received greater coverage or more depth. A subset of content validity is face validity , where experts are asked their opinion about whether an instrument measures the concept intended.
Construct validity refers to whether you can draw inferences about test scores related to the concept being studied. For example, if a person has a high score on a survey that measures anxiety, does this person truly have a high degree of anxiety? In another example, a test of knowledge of medications that requires dosage calculations may instead be testing maths knowledge.
There are three types of evidence that can be used to demonstrate a research instrument has construct validity:
Homogeneity—meaning that the instrument measures one construct.
Convergence—this occurs when the instrument measures concepts similar to that of other instruments. Although if there are no similar instruments available this will not be possible to do.
Theory evidence—this is evident when behaviour is similar to theoretical propositions of the construct measured in the instrument. For example, when an instrument measures anxiety, one would expect to see that participants who score high on the instrument for anxiety also demonstrate symptoms of anxiety in their day-to-day lives. 2
The final measure of validity is criterion validity . A criterion is any other instrument that measures the same variable. Correlations can be conducted to determine the extent to which the different instruments measure the same variable. Criterion validity is measured in three ways:
Convergent validity—shows that an instrument is highly correlated with instruments measuring similar variables.
Divergent validity—shows that an instrument is poorly correlated to instruments that measure different variables. In this case, for example, there should be a low correlation between an instrument that measures motivation and one that measures self-efficacy.
Predictive validity—means that the instrument should have high correlations with future criterions. 2 For example, a score of high self-efficacy related to performing a task should predict the likelihood a participant completing the task.
Reliability
Reliability relates to the consistency of a measure. A participant completing an instrument meant to measure motivation should have approximately the same responses each time the test is completed. Although it is not possible to give an exact calculation of reliability, an estimate of reliability can be achieved through different measures. The three attributes of reliability are outlined in table 2 . How each attribute is tested for is described below.
Attributes of reliability
Homogeneity (internal consistency) is assessed using item-to-total correlation, split-half reliability, Kuder-Richardson coefficient and Cronbach's α. In split-half reliability, the results of a test, or instrument, are divided in half. Correlations are calculated comparing both halves. Strong correlations indicate high reliability, while weak correlations indicate the instrument may not be reliable. The Kuder-Richardson test is a more complicated version of the split-half test. In this process the average of all possible split half combinations is determined and a correlation between 0–1 is generated. This test is more accurate than the split-half test, but can only be completed on questions with two answers (eg, yes or no, 0 or 1). 3
Cronbach's α is the most commonly used test to determine the internal consistency of an instrument. In this test, the average of all correlations in every combination of split-halves is determined. Instruments with questions that have more than two responses can be used in this test. The Cronbach's α result is a number between 0 and 1. An acceptable reliability score is one that is 0.7 and higher. 1 , 3
Stability is tested using test–retest and parallel or alternate-form reliability testing. Test–retest reliability is assessed when an instrument is given to the same participants more than once under similar circumstances. A statistical comparison is made between participant's test scores for each of the times they have completed it. This provides an indication of the reliability of the instrument. Parallel-form reliability (or alternate-form reliability) is similar to test–retest reliability except that a different form of the original instrument is given to participants in subsequent tests. The domain, or concepts being tested are the same in both versions of the instrument but the wording of items is different. 2 For an instrument to demonstrate stability there should be a high correlation between the scores each time a participant completes the test. Generally speaking, a correlation coefficient of less than 0.3 signifies a weak correlation, 0.3–0.5 is moderate and greater than 0.5 is strong. 4
Equivalence is assessed through inter-rater reliability. This test includes a process for qualitatively determining the level of agreement between two or more observers. A good example of the process used in assessing inter-rater reliability is the scores of judges for a skating competition. The level of consistency across all judges in the scores given to skating participants is the measure of inter-rater reliability. An example in research is when researchers are asked to give a score for the relevancy of each item on an instrument. Consistency in their scores relates to the level of inter-rater reliability of the instrument.
Determining how rigorously the issues of reliability and validity have been addressed in a study is an essential component in the critique of research as well as influencing the decision about whether to implement of the study findings into nursing practice. In quantitative studies, rigour is determined through an evaluation of the validity and reliability of the tools or instruments utilised in the study. A good quality research study will provide evidence of how all these factors have been addressed. This will help you to assess the validity and reliability of the research and help you decide whether or not you should apply the findings in your area of clinical practice.
- Lobiondo-Wood G ,
- Shuttleworth M
- ↵ Laerd Statistics . Determining the correlation coefficient . 2013 . https://statistics.laerd.com/premium/pc/pearson-correlation-in-spss-8.php
Twitter Follow Roberta Heale at @robertaheale and Alison Twycross at @alitwy
Competing interests None declared.
Read the full text or download the PDF:
- Privacy Policy
Buy Me a Coffee

Home » Validity – Types, Examples and Guide
Validity – Types, Examples and Guide
Table of Contents

Definition:
Validity refers to the extent to which a concept, measure, or study accurately represents the intended meaning or reality it is intended to capture. It is a fundamental concept in research and assessment that assesses the soundness and appropriateness of the conclusions, inferences, or interpretations made based on the data or evidence collected.
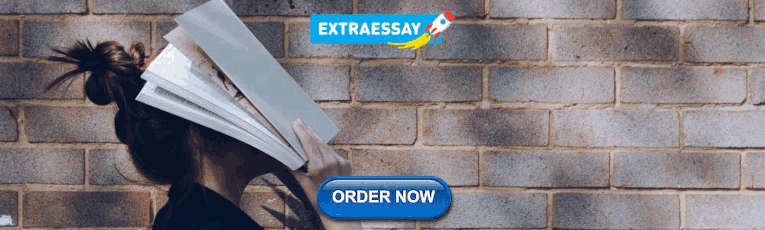
Research Validity
Research validity refers to the degree to which a study accurately measures or reflects what it claims to measure. In other words, research validity concerns whether the conclusions drawn from a study are based on accurate, reliable and relevant data.
Validity is a concept used in logic and research methodology to assess the strength of an argument or the quality of a research study. It refers to the extent to which a conclusion or result is supported by evidence and reasoning.
How to Ensure Validity in Research
Ensuring validity in research involves several steps and considerations throughout the research process. Here are some key strategies to help maintain research validity:
Clearly Define Research Objectives and Questions
Start by clearly defining your research objectives and formulating specific research questions. This helps focus your study and ensures that you are addressing relevant and meaningful research topics.
Use appropriate research design
Select a research design that aligns with your research objectives and questions. Different types of studies, such as experimental, observational, qualitative, or quantitative, have specific strengths and limitations. Choose the design that best suits your research goals.
Use reliable and valid measurement instruments
If you are measuring variables or constructs, ensure that the measurement instruments you use are reliable and valid. This involves using established and well-tested tools or developing your own instruments through rigorous validation processes.
Ensure a representative sample
When selecting participants or subjects for your study, aim for a sample that is representative of the population you want to generalize to. Consider factors such as age, gender, socioeconomic status, and other relevant demographics to ensure your findings can be generalized appropriately.
Address potential confounding factors
Identify potential confounding variables or biases that could impact your results. Implement strategies such as randomization, matching, or statistical control to minimize the influence of confounding factors and increase internal validity.
Minimize measurement and response biases
Be aware of measurement biases and response biases that can occur during data collection. Use standardized protocols, clear instructions, and trained data collectors to minimize these biases. Employ techniques like blinding or double-blinding in experimental studies to reduce bias.
Conduct appropriate statistical analyses
Ensure that the statistical analyses you employ are appropriate for your research design and data type. Select statistical tests that are relevant to your research questions and use robust analytical techniques to draw accurate conclusions from your data.
Consider external validity
While it may not always be possible to achieve high external validity, be mindful of the generalizability of your findings. Clearly describe your sample and study context to help readers understand the scope and limitations of your research.
Peer review and replication
Submit your research for peer review by experts in your field. Peer review helps identify potential flaws, biases, or methodological issues that can impact validity. Additionally, encourage replication studies by other researchers to validate your findings and enhance the overall reliability of the research.
Transparent reporting
Clearly and transparently report your research methods, procedures, data collection, and analysis techniques. Provide sufficient details for others to evaluate the validity of your study and replicate your work if needed.
Types of Validity
There are several types of validity that researchers consider when designing and evaluating studies. Here are some common types of validity:
Internal Validity
Internal validity relates to the degree to which a study accurately identifies causal relationships between variables. It addresses whether the observed effects can be attributed to the manipulated independent variable rather than confounding factors. Threats to internal validity include selection bias, history effects, maturation of participants, and instrumentation issues.
External Validity
External validity concerns the generalizability of research findings to the broader population or real-world settings. It assesses the extent to which the results can be applied to other individuals, contexts, or timeframes. Factors that can limit external validity include sample characteristics, research settings, and the specific conditions under which the study was conducted.
Construct Validity
Construct validity examines whether a study adequately measures the intended theoretical constructs or concepts. It focuses on the alignment between the operational definitions used in the study and the underlying theoretical constructs. Construct validity can be threatened by issues such as poor measurement tools, inadequate operational definitions, or a lack of clarity in the conceptual framework.
Content Validity
Content validity refers to the degree to which a measurement instrument or test adequately covers the entire range of the construct being measured. It assesses whether the items or questions included in the measurement tool represent the full scope of the construct. Content validity is often evaluated through expert judgment, reviewing the relevance and representativeness of the items.
Criterion Validity
Criterion validity determines the extent to which a measure or test is related to an external criterion or standard. It assesses whether the results obtained from a measurement instrument align with other established measures or outcomes. Criterion validity can be divided into two subtypes: concurrent validity, which examines the relationship between the measure and the criterion at the same time, and predictive validity, which investigates the measure’s ability to predict future outcomes.
Face Validity
Face validity refers to the degree to which a measurement or test appears, on the surface, to measure what it intends to measure. It is a subjective assessment based on whether the items seem relevant and appropriate to the construct being measured. Face validity is often used as an initial evaluation before conducting more rigorous validity assessments.
Importance of Validity
Validity is crucial in research for several reasons:
- Accurate Measurement: Validity ensures that the measurements or observations in a study accurately represent the intended constructs or variables. Without validity, researchers cannot be confident that their results truly reflect the phenomena they are studying. Validity allows researchers to draw accurate conclusions and make meaningful inferences based on their findings.
- Credibility and Trustworthiness: Validity enhances the credibility and trustworthiness of research. When a study demonstrates high validity, it indicates that the researchers have taken appropriate measures to ensure the accuracy and integrity of their work. This strengthens the confidence of other researchers, peers, and the wider scientific community in the study’s results and conclusions.
- Generalizability: Validity helps determine the extent to which research findings can be generalized beyond the specific sample and context of the study. By addressing external validity, researchers can assess whether their results can be applied to other populations, settings, or situations. This information is valuable for making informed decisions, implementing interventions, or developing policies based on research findings.
- Sound Decision-Making: Validity supports informed decision-making in various fields, such as medicine, psychology, education, and social sciences. When validity is established, policymakers, practitioners, and professionals can rely on research findings to guide their actions and interventions. Validity ensures that decisions are based on accurate and trustworthy information, which can lead to better outcomes and more effective practices.
- Avoiding Errors and Bias: Validity helps researchers identify and mitigate potential errors and biases in their studies. By addressing internal validity, researchers can minimize confounding factors and alternative explanations, ensuring that the observed effects are genuinely attributable to the manipulated variables. Validity assessments also highlight measurement errors or shortcomings, enabling researchers to improve their measurement tools and procedures.
- Progress of Scientific Knowledge: Validity is essential for the advancement of scientific knowledge. Valid research contributes to the accumulation of reliable and valid evidence, which forms the foundation for building theories, developing models, and refining existing knowledge. Validity allows researchers to build upon previous findings, replicate studies, and establish a cumulative body of knowledge in various disciplines. Without validity, the scientific community would struggle to make meaningful progress and establish a solid understanding of the phenomena under investigation.
- Ethical Considerations: Validity is closely linked to ethical considerations in research. Conducting valid research ensures that participants’ time, effort, and data are not wasted on flawed or invalid studies. It upholds the principle of respect for participants’ autonomy and promotes responsible research practices. Validity is also important when making claims or drawing conclusions that may have real-world implications, as misleading or invalid findings can have adverse effects on individuals, organizations, or society as a whole.
Examples of Validity
Here are some examples of validity in different contexts:
- Example 1: All men are mortal. John is a man. Therefore, John is mortal. This argument is logically valid because the conclusion follows logically from the premises.
- Example 2: If it is raining, then the ground is wet. The ground is wet. Therefore, it is raining. This argument is not logically valid because there could be other reasons for the ground being wet, such as watering the plants.
- Example 1: In a study examining the relationship between caffeine consumption and alertness, the researchers use established measures of both variables, ensuring that they are accurately capturing the concepts they intend to measure. This demonstrates construct validity.
- Example 2: A researcher develops a new questionnaire to measure anxiety levels. They administer the questionnaire to a group of participants and find that it correlates highly with other established anxiety measures. This indicates good construct validity for the new questionnaire.
- Example 1: A study on the effects of a particular teaching method is conducted in a controlled laboratory setting. The findings of the study may lack external validity because the conditions in the lab may not accurately reflect real-world classroom settings.
- Example 2: A research study on the effects of a new medication includes participants from diverse backgrounds and age groups, increasing the external validity of the findings to a broader population.
- Example 1: In an experiment, a researcher manipulates the independent variable (e.g., a new drug) and controls for other variables to ensure that any observed effects on the dependent variable (e.g., symptom reduction) are indeed due to the manipulation. This establishes internal validity.
- Example 2: A researcher conducts a study examining the relationship between exercise and mood by administering questionnaires to participants. However, the study lacks internal validity because it does not control for other potential factors that could influence mood, such as diet or stress levels.
- Example 1: A teacher develops a new test to assess students’ knowledge of a particular subject. The items on the test appear to be relevant to the topic at hand and align with what one would expect to find on such a test. This suggests face validity, as the test appears to measure what it intends to measure.
- Example 2: A company develops a new customer satisfaction survey. The questions included in the survey seem to address key aspects of the customer experience and capture the relevant information. This indicates face validity, as the survey seems appropriate for assessing customer satisfaction.
- Example 1: A team of experts reviews a comprehensive curriculum for a high school biology course. They evaluate the curriculum to ensure that it covers all the essential topics and concepts necessary for students to gain a thorough understanding of biology. This demonstrates content validity, as the curriculum is representative of the domain it intends to cover.
- Example 2: A researcher develops a questionnaire to assess career satisfaction. The questions in the questionnaire encompass various dimensions of job satisfaction, such as salary, work-life balance, and career growth. This indicates content validity, as the questionnaire adequately represents the different aspects of career satisfaction.
- Example 1: A company wants to evaluate the effectiveness of a new employee selection test. They administer the test to a group of job applicants and later assess the job performance of those who were hired. If there is a strong correlation between the test scores and subsequent job performance, it suggests criterion validity, indicating that the test is predictive of job success.
- Example 2: A researcher wants to determine if a new medical diagnostic tool accurately identifies a specific disease. They compare the results of the diagnostic tool with the gold standard diagnostic method and find a high level of agreement. This demonstrates criterion validity, indicating that the new tool is valid in accurately diagnosing the disease.
Where to Write About Validity in A Thesis
In a thesis, discussions related to validity are typically included in the methodology and results sections. Here are some specific places where you can address validity within your thesis:
Research Design and Methodology
In the methodology section, provide a clear and detailed description of the measures, instruments, or data collection methods used in your study. Discuss the steps taken to establish or assess the validity of these measures. Explain the rationale behind the selection of specific validity types relevant to your study, such as content validity, criterion validity, or construct validity. Discuss any modifications or adaptations made to existing measures and their potential impact on validity.
Measurement Procedures
In the methodology section, elaborate on the procedures implemented to ensure the validity of measurements. Describe how potential biases or confounding factors were addressed, controlled, or accounted for to enhance internal validity. Provide details on how you ensured that the measurement process accurately captures the intended constructs or variables of interest.
Data Collection
In the methodology section, discuss the steps taken to collect data and ensure data validity. Explain any measures implemented to minimize errors or biases during data collection, such as training of data collectors, standardized protocols, or quality control procedures. Address any potential limitations or threats to validity related to the data collection process.
Data Analysis and Results
In the results section, present the analysis and findings related to validity. Report any statistical tests, correlations, or other measures used to assess validity. Provide interpretations and explanations of the results obtained. Discuss the implications of the validity findings for the overall reliability and credibility of your study.
Limitations and Future Directions
In the discussion or conclusion section, reflect on the limitations of your study, including limitations related to validity. Acknowledge any potential threats or weaknesses to validity that you encountered during your research. Discuss how these limitations may have influenced the interpretation of your findings and suggest avenues for future research that could address these validity concerns.
Applications of Validity
Validity is applicable in various areas and contexts where research and measurement play a role. Here are some common applications of validity:
Psychological and Behavioral Research
Validity is crucial in psychology and behavioral research to ensure that measurement instruments accurately capture constructs such as personality traits, intelligence, attitudes, emotions, or psychological disorders. Validity assessments help researchers determine if their measures are truly measuring the intended psychological constructs and if the results can be generalized to broader populations or real-world settings.
Educational Assessment
Validity is essential in educational assessment to determine if tests, exams, or assessments accurately measure students’ knowledge, skills, or abilities. It ensures that the assessment aligns with the educational objectives and provides reliable information about student performance. Validity assessments help identify if the assessment is valid for all students, regardless of their demographic characteristics, language proficiency, or cultural background.
Program Evaluation
Validity plays a crucial role in program evaluation, where researchers assess the effectiveness and impact of interventions, policies, or programs. By establishing validity, evaluators can determine if the observed outcomes are genuinely attributable to the program being evaluated rather than extraneous factors. Validity assessments also help ensure that the evaluation findings are applicable to different populations, contexts, or timeframes.
Medical and Health Research
Validity is essential in medical and health research to ensure the accuracy and reliability of diagnostic tools, measurement instruments, and clinical assessments. Validity assessments help determine if a measurement accurately identifies the presence or absence of a medical condition, measures the effectiveness of a treatment, or predicts patient outcomes. Validity is crucial for establishing evidence-based medicine and informing medical decision-making.
Social Science Research
Validity is relevant in various social science disciplines, including sociology, anthropology, economics, and political science. Researchers use validity to ensure that their measures and methods accurately capture social phenomena, such as social attitudes, behaviors, social structures, or economic indicators. Validity assessments support the reliability and credibility of social science research findings.
Market Research and Surveys
Validity is important in market research and survey studies to ensure that the survey questions effectively measure consumer preferences, buying behaviors, or attitudes towards products or services. Validity assessments help researchers determine if the survey instrument is accurately capturing the desired information and if the results can be generalized to the target population.
Limitations of Validity
Here are some limitations of validity:
- Construct Validity: Limitations of construct validity include the potential for measurement error, inadequate operational definitions of constructs, or the failure to capture all aspects of a complex construct.
- Internal Validity: Limitations of internal validity may arise from confounding variables, selection bias, or the presence of extraneous factors that could influence the study outcomes, making it difficult to attribute causality accurately.
- External Validity: Limitations of external validity can occur when the study sample does not represent the broader population, when the research setting differs significantly from real-world conditions, or when the study lacks ecological validity, i.e., the findings do not reflect real-world complexities.
- Measurement Validity: Limitations of measurement validity can arise from measurement error, inadequately designed or flawed measurement scales, or limitations inherent in self-report measures, such as social desirability bias or recall bias.
- Statistical Conclusion Validity: Limitations in statistical conclusion validity can occur due to sampling errors, inadequate sample sizes, or improper statistical analysis techniques, leading to incorrect conclusions or generalizations.
- Temporal Validity: Limitations of temporal validity arise when the study results become outdated due to changes in the studied phenomena, interventions, or contextual factors.
- Researcher Bias: Researcher bias can affect the validity of a study. Biases can emerge through the researcher’s subjective interpretation, influence of personal beliefs, or preconceived notions, leading to unintentional distortion of findings or failure to consider alternative explanations.
- Ethical Validity: Limitations can arise if the study design or methods involve ethical concerns, such as the use of deceptive practices, inadequate informed consent, or potential harm to participants.
Also see Reliability Vs Validity
About the author
Muhammad Hassan
Researcher, Academic Writer, Web developer
You may also like

Alternate Forms Reliability – Methods, Examples...

Construct Validity – Types, Threats and Examples

Internal Validity – Threats, Examples and Guide

Reliability Vs Validity

Internal Consistency Reliability – Methods...

Split-Half Reliability – Methods, Examples and...

An official website of the United States government
The .gov means it’s official. Federal government websites often end in .gov or .mil. Before sharing sensitive information, make sure you’re on a federal government site.
The site is secure. The https:// ensures that you are connecting to the official website and that any information you provide is encrypted and transmitted securely.
- Publications
- Account settings
Preview improvements coming to the PMC website in October 2024. Learn More or Try it out now .
- Advanced Search
- Journal List
- J Bras Pneumol
- v.44(3); May-Jun 2018
Internal and external validity: can you apply research study results to your patients?
Cecilia maria patino.
1 . Methods in Epidemiologic, Clinical, and Operations Research-MECOR-program, American Thoracic Society/Asociación Latinoamericana del Tórax, Montevideo, Uruguay.
2 . Department of Preventive Medicine, Keck School of Medicine, University of Southern California, Los Angeles, CA, USA.
Juliana Carvalho Ferreira
3 . Divisão de Pneumologia, Instituto do Coração, Hospital das Clínicas, Faculdade de Medicina, Universidade de São Paulo, São Paulo (SP) Brasil.
CLINICAL SCENARIO
In a multicenter study in France, investigators conducted a randomized controlled trial to test the effect of prone vs. supine positioning ventilation on mortality among patients with early, severe ARDS. They showed that prolonged prone-positioning ventilation decreased 28-day mortality [hazard ratio (HR) = 0.39; 95% CI: 0.25-0.63]. 1
STUDY VALIDITY
The validity of a research study refers to how well the results among the study participants represent true findings among similar individuals outside the study. This concept of validity applies to all types of clinical studies, including those about prevalence, associations, interventions, and diagnosis. The validity of a research study includes two domains: internal and external validity.
Internal validity is defined as the extent to which the observed results represent the truth in the population we are studying and, thus, are not due to methodological errors. In our example, if the authors can support that the study has internal validity, they can conclude that prone positioning reduces mortality among patients with severe ARDS. The internal validity of a study can be threatened by many factors, including errors in measurement or in the selection of participants in the study, and researchers should think about and avoid these errors.
Once the internal validity of the study is established, the researcher can proceed to make a judgment regarding its external validity by asking whether the study results apply to similar patients in a different setting or not ( Figure 1 ). In the example, we would want to evaluate if the results of the clinical trial apply to ARDS patients in other ICUs. If the patients have early, severe ARDS, probably yes, but the study results may not apply to patients with mild ARDS . External validity refers to the extent to which the results of a study are generalizable to patients in our daily practice, especially for the population that the sample is thought to represent.

Lack of internal validity implies that the results of the study deviate from the truth, and, therefore, we cannot draw any conclusions; hence, if the results of a trial are not internally valid, external validity is irrelevant. 2 Lack of external validity implies that the results of the trial may not apply to patients who differ from the study population and, consequently, could lead to low adoption of the treatment tested in the trial by other clinicians.
INCREASING VALIDITY OF RESEARCH STUDIES
To increase internal validity, investigators should ensure careful study planning and adequate quality control and implementation strategies-including adequate recruitment strategies, data collection, data analysis, and sample size. External validity can be increased by using broad inclusion criteria that result in a study population that more closely resembles real-life patients, and, in the case of clinical trials, by choosing interventions that are feasible to apply. 2
- Introduction
- Conclusions
- Article Information
Screenshots of the smartphone cognitive tasks developed by Datacubed Health and included in the ALLFTD Mobile App. Details about the task design and instructions are included in the eMethods in Supplement 1. A, Flanker (Ducks in a Pond) is a task of cognitive control requiring participants to select the direction of the center duck. B, Go/no-go (Go Sushi Go!) requires participants to quickly tap on pieces of sushi (go) but not to tap when they see a fish skeleton (no-go). C, Card sort (Card Shuffle) is a task of cognitive flexibility requiring participants to learn rules that change during the task. D, The adaptative, associative memory task (Humi’s Bistro) requires participants to learn the food orders of several restaurant tables. E, Stroop (Color Clash) is a cognitive inhibition paradigm requiring participants to inhibit their tendency to read words and instead respond based on the color of the word. F, The 2-back task (Animal Parade) requires participants to determine whether animals on a parade float match the animals they saw 2 stimuli previously. G, Participants are asked to complete 3 testing sessions over 2 weeks. Shown in dark blue, they have 3 days to complete each testing session with a washout day between sessions on which no tests are available. Session 2 always begins on day 5 and session 3 on day 9. Screenshots are provided with permission from Datacubed Health.
Forest plots present internal consistency and test-retest reliability results in the discovery and validation cohorts, as well as an estimate in a combined sample of discovery and validation participants. ICC indicates interclass correlation coefficient.
A and B, Correlation matrices display associations of in-clinic criterion standard measures and ALLFTD mobile App (mApp) test scores in discovery and validation cohorts. Below the horizontal dashed lines, the associations among app tests and between app tests and demographic characteristics convergent clinical measures, divergent cognitive tests, and neuroimaging regions of interest can be viewed. Most app tests show strong correlations with each other and with age, convergent clinical measures, and brain volume. The measures show weaker correlations with divergent measures of visuospatial (Benson Figure Copy) and language (Multilingual Naming Test [MINT]) abilities. The strength of convergent correlations between app measures and outcomes is similar to the correlations between criterion standard neuropsychological scores and these outcomes, which can be viewed by looking across the rows above the horizontal black line. C and D, In the discovery and validation cohorts, receiver operating characteristics curves were calculated to determine how well a composite of app tests, the Uniform Data Set, version 3.0, Executive Functioning Composite (UDS3-EF), and the Montreal Cognitive Assessment (MoCA) discriminate individuals without symptoms (Clinical Dementia Rating Scale plus National Alzheimer’s Coordinating Center FTLD module sum of boxes [CDR plus NACC-FTLD-SB] score = 0) from individuals with the mildest symptoms of FTLD (CDR plus NACC-FTLD-SB score = 0.5). AUC indicates area under the curve; CVLT, California Verbal Learning Test.
eMethods. Instruments and Statistical Analysis
eResults. Participants
eTable 1. Participant Characteristics and Test Scores in Original and Validation Cohorts
eTable 2. Comparison of Diagnostic Accuracy for ALLFTD Mobile App Composite Score Across Cohorts
eTable 3. Number of Distractions Reported During the Remote Smartphone Testing Sessions
eTable 4. Qualitative Description of the Distractions Reported During Remote Testing Sessions
eFigure 1. Scatterplots of Test-Retest Reliability in a Mixed Sample of Adults Without Functional Impairment and Participants With FTLD
eFigure 2. Comparison of Test-Retest Reliability Estimates by Endorsement of Distractions
eFigure 3. Comparison of Test-Retest Reliability Estimates by Operating System
eFigure 4. Correlation Matrix in the Combined Cohort
eFigure 5. Neural Correlates of Smartphone Cognitive Test Performance
eReferences
Nonauthor Collaborators
Data Sharing Statement
See More About
Sign up for emails based on your interests, select your interests.
Customize your JAMA Network experience by selecting one or more topics from the list below.
- Academic Medicine
- Acid Base, Electrolytes, Fluids
- Allergy and Clinical Immunology
- American Indian or Alaska Natives
- Anesthesiology
- Anticoagulation
- Art and Images in Psychiatry
- Artificial Intelligence
- Assisted Reproduction
- Bleeding and Transfusion
- Caring for the Critically Ill Patient
- Challenges in Clinical Electrocardiography
- Climate and Health
- Climate Change
- Clinical Challenge
- Clinical Decision Support
- Clinical Implications of Basic Neuroscience
- Clinical Pharmacy and Pharmacology
- Complementary and Alternative Medicine
- Consensus Statements
- Coronavirus (COVID-19)
- Critical Care Medicine
- Cultural Competency
- Dental Medicine
- Dermatology
- Diabetes and Endocrinology
- Diagnostic Test Interpretation
- Drug Development
- Electronic Health Records
- Emergency Medicine
- End of Life, Hospice, Palliative Care
- Environmental Health
- Equity, Diversity, and Inclusion
- Facial Plastic Surgery
- Gastroenterology and Hepatology
- Genetics and Genomics
- Genomics and Precision Health
- Global Health
- Guide to Statistics and Methods
- Hair Disorders
- Health Care Delivery Models
- Health Care Economics, Insurance, Payment
- Health Care Quality
- Health Care Reform
- Health Care Safety
- Health Care Workforce
- Health Disparities
- Health Inequities
- Health Policy
- Health Systems Science
- History of Medicine
- Hypertension
- Images in Neurology
- Implementation Science
- Infectious Diseases
- Innovations in Health Care Delivery
- JAMA Infographic
- Law and Medicine
- Leading Change
- Less is More
- LGBTQIA Medicine
- Lifestyle Behaviors
- Medical Coding
- Medical Devices and Equipment
- Medical Education
- Medical Education and Training
- Medical Journals and Publishing
- Mobile Health and Telemedicine
- Narrative Medicine
- Neuroscience and Psychiatry
- Notable Notes
- Nutrition, Obesity, Exercise
- Obstetrics and Gynecology
- Occupational Health
- Ophthalmology
- Orthopedics
- Otolaryngology
- Pain Medicine
- Palliative Care
- Pathology and Laboratory Medicine
- Patient Care
- Patient Information
- Performance Improvement
- Performance Measures
- Perioperative Care and Consultation
- Pharmacoeconomics
- Pharmacoepidemiology
- Pharmacogenetics
- Pharmacy and Clinical Pharmacology
- Physical Medicine and Rehabilitation
- Physical Therapy
- Physician Leadership
- Population Health
- Primary Care
- Professional Well-being
- Professionalism
- Psychiatry and Behavioral Health
- Public Health
- Pulmonary Medicine
- Regulatory Agencies
- Reproductive Health
- Research, Methods, Statistics
- Resuscitation
- Rheumatology
- Risk Management
- Scientific Discovery and the Future of Medicine
- Shared Decision Making and Communication
- Sleep Medicine
- Sports Medicine
- Stem Cell Transplantation
- Substance Use and Addiction Medicine
- Surgical Innovation
- Surgical Pearls
- Teachable Moment
- Technology and Finance
- The Art of JAMA
- The Arts and Medicine
- The Rational Clinical Examination
- Tobacco and e-Cigarettes
- Translational Medicine
- Trauma and Injury
- Treatment Adherence
- Ultrasonography
- Users' Guide to the Medical Literature
- Vaccination
- Venous Thromboembolism
- Veterans Health
- Women's Health
- Workflow and Process
- Wound Care, Infection, Healing
Get the latest research based on your areas of interest.
Others also liked.
- Download PDF
- X Facebook More LinkedIn
Staffaroni AM , Clark AL , Taylor JC, et al. Reliability and Validity of Smartphone Cognitive Testing for Frontotemporal Lobar Degeneration. JAMA Netw Open. 2024;7(4):e244266. doi:10.1001/jamanetworkopen.2024.4266
Manage citations:
© 2024
- Permissions
Reliability and Validity of Smartphone Cognitive Testing for Frontotemporal Lobar Degeneration
- 1 Department of Neurology, Memory and Aging Center, Weill Institute for Neurosciences, University of California, San Francisco
- 2 Department of Neurology, Columbia University, New York, New York
- 3 Department of Neurology, Mayo Clinic, Rochester, Minnesota
- 4 Department of Quantitative Health Sciences, Division of Biomedical Statistics and Informatics, Mayo Clinic, Rochester, Minnesota
- 5 Department of Neurology, Case Western Reserve University, Cleveland, Ohio
- 6 Department of Neurosciences, University of California, San Diego, La Jolla
- 7 Department of Radiology, University of North Carolina, Chapel Hill
- 8 Department of Neurology, Indiana University, Indianapolis
- 9 Department of Neurology, Vanderbilt University, Nashville, Tennessee
- 10 Department of Neurology, University of Washington, Seattle
- 11 Department of Psychiatry and Psychology, Mayo Clinic, Rochester, Minnesota
- 12 Department of Neurology, Institute for Precision Health, University of California, Los Angeles
- 13 Department of Neurology, Knight Alzheimer Disease Research Center, Washington University, Saint Louis, Missouri
- 14 Department of Psychiatry, Knight Alzheimer Disease Research Center, Washington University, Saint Louis, Missouri
- 15 Department of Neuroscience, Mayo Clinic, Jacksonville, Florida
- 16 Department of Neurology, University of Pennsylvania Perelman School of Medicine, Philadelphia
- 17 Division of Neurology, University of British Columbia, Musqueam, Squamish & Tsleil-Waututh Traditional Territory, Vancouver, Canada
- 18 Department of Neurosciences, University of California, San Diego, La Jolla
- 19 Department of Neurology, Nantz National Alzheimer Center, Houston Methodist and Weill Cornell Medicine, Houston Methodist, Houston, Texas
- 20 Department of Neurology, UCLA (University of California, Los Angeles)
- 21 Department of Neurology, University of Colorado, Aurora
- 22 Department of Neurology, David Geffen School of Medicine, UCLA
- 23 Department of Neurology, University of Alabama, Birmingham
- 24 Tanz Centre for Research in Neurodegenerative Diseases, Division of Neurology, University of Toronto, Toronto, Ontario, Canada
- 25 Department of Neurology, Massachusetts General Hospital and Harvard Medical School, Boston
- 26 Department of Epidemiology and Biostatistics, University of California, San Francisco
- 27 Department of Psychological & Brain Sciences, Washington University, Saint Louis, Missouri
Question Can remote cognitive testing via smartphones yield reliable and valid data for frontotemporal lobar degeneration (FTLD)?
Findings In this cohort study of 360 patients, remotely deployed smartphone cognitive tests showed moderate to excellent reliability comparedwith criterion standard measures (in-person disease severity assessments and neuropsychological tests) and brain volumes. Smartphone tests accurately detected dementia and were more sensitive to the earliest stages of familial FTLD than standard neuropsychological tests.
Meaning These findings suggest that remotely deployed smartphone-based assessments may be reliable and valid tools for evaluating FTLD and may enhance early detection, supporting the inclusion of digital assessments in clinical trials for neurodegeneration.
Importance Frontotemporal lobar degeneration (FTLD) is relatively rare, behavioral and motor symptoms increase travel burden, and standard neuropsychological tests are not sensitive to early-stage disease. Remote smartphone-based cognitive assessments could mitigate these barriers to trial recruitment and success, but no such tools are validated for FTLD.
Objective To evaluate the reliability and validity of smartphone-based cognitive measures for remote FTLD evaluations.
Design, Setting, and Participants In this cohort study conducted from January 10, 2019, to July 31, 2023, controls and participants with FTLD performed smartphone application (app)–based executive functioning tasks and an associative memory task 3 times over 2 weeks. Observational research participants were enrolled through 18 centers of a North American FTLD research consortium (ALLFTD) and were asked to complete the tests remotely using their own smartphones. Of 1163 eligible individuals (enrolled in parent studies), 360 were enrolled in the present study; 364 refused and 439 were excluded. Participants were divided into discovery (n = 258) and validation (n = 102) cohorts. Among 329 participants with data available on disease stage, 195 were asymptomatic or had preclinical FTLD (59.3%), 66 had prodromal FTLD (20.1%), and 68 had symptomatic FTLD (20.7%) with a range of clinical syndromes.
Exposure Participants completed standard in-clinic measures and remotely administered ALLFTD mobile app (app) smartphone tests.
Main Outcomes and Measures Internal consistency, test-retest reliability, association of smartphone tests with criterion standard clinical measures, and diagnostic accuracy.
Results In the 360 participants (mean [SD] age, 54.0 [15.4] years; 209 [58.1%] women), smartphone tests showed moderate-to-excellent reliability (intraclass correlation coefficients, 0.77-0.95). Validity was supported by association of smartphones tests with disease severity ( r range, 0.38-0.59), criterion-standard neuropsychological tests ( r range, 0.40-0.66), and brain volume (standardized β range, 0.34-0.50). Smartphone tests accurately differentiated individuals with dementia from controls (area under the curve [AUC], 0.93 [95% CI, 0.90-0.96]) and were more sensitive to early symptoms (AUC, 0.82 [95% CI, 0.76-0.88]) than the Montreal Cognitive Assessment (AUC, 0.68 [95% CI, 0.59-0.78]) ( z of comparison, −2.49 [95% CI, −0.19 to −0.02]; P = .01). Reliability and validity findings were highly similar in the discovery and validation cohorts. Preclinical participants who carried pathogenic variants performed significantly worse than noncarrier family controls on 3 app tasks (eg, 2-back β = −0.49 [95% CI, −0.72 to −0.25]; P < .001) but not a composite of traditional neuropsychological measures (β = −0.14 [95% CI, −0.42 to 0.14]; P = .32).
Conclusions and Relevance The findings of this cohort study suggest that smartphones could offer a feasible, reliable, valid, and scalable solution for remote evaluations of FTLD and may improve early detection. Smartphone assessments should be considered as a complementary approach to traditional in-person trial designs. Future research should validate these results in diverse populations and evaluate the utility of these tests for longitudinal monitoring.
Frontotemporal lobar degeneration (FTLD) is a neurodegenerative pathology causing early-onset dementia syndromes with impaired behavior, cognition, language, and/or motor functioning. 1 Although over 30 FTLD trials are planned or in progress, there are several barriers to conducting FTLD trials. Clinical trials for neurodegenerative disease are expensive, 2 and frequent in-person trial visits are burdensome for patients, caregivers, and clinicians, 3 a concern magnified in FTLD by behavioral and motor impairments. Given the rarity and geographical dispersion of eligible participants, FTLD trials require global recruitment, 4 particularly for those that are far from expert FTLD clinical trial centers. Furthermore, criterion standard neuropsychological tests are not adequately sensitive until symptoms are already noticeable to families, limiting their usefulness as outcomes in early-stage FTLD treatment trials. 4
Reliable, valid, and scalable remote data collection methods may help surmount these barriers to FTLD clinical trials. Smartphones are garnering interest across neurological conditions as a method for administering remote cognitive and motor evaluations. Preliminary evidence supports the feasibility, reliability, and/or validity of unsupervised smartphone cognitive and motor testing in older adults at risk for Alzheimer disease, 5 - 8 Parkinson disease, 9 and Huntington disease. 10 The clinical heterogeneity of FTLD necessitates a uniquely comprehensive smartphone battery. In the ALLFTD Consortium (Advancing Research and Treatment in Frontotemporal Lobar Degeneration [ARTFLD] and Longitudinal Evaluation of Familial Frontotemporal Dementia Subjects [LEFFTDS]), the ALLFTD mobile Application (ALLFTD-mApp) was designed to remotely monitor cognitive, behavioral, language, and motor functioning in FTLD research. Taylor et al 11 recently reported that unsupervised ALLFTD-mApp data collection through a multicenter North American FTLD research network was feasible and acceptable to participants. Herein, we extend that work by investigating the reliability and validity of unsupervised remote smartphone tests of executive functioning and memory in a cohort with FTLD that has undergone extensive phenotyping.
Participants were enrolled from ongoing FTLD studies requiring in-person assessment, including participants from 18 centers from the ALLFTD study study 12 and University of California, San Francisco (UCSF) FTLD studies. To study the app in older individuals, a small group of older adults without functional impairment was recruited from the UCSF Brain Aging Network for Cognitive Health. All study procedures were approved by the UCSF or Johns Hopkins Central Institutional Review Board. All participants or legally authorized representatives provided written informed consent. The study followed the Strengthening the Reporting of Observational Studies in Epidemiology ( STROBE ) reporting guideline.
Inclusion criteria were age 18 years or older, having access to a smartphone, and reporting English as the primary language. Race and ethnicity were self reported by participants using options consistent with the National Alzheimer’s Coordinating Center (NACC) Uniform Data Set (UDS) and were collected to contextualize the generalizability of these results. Participants were asked to complete tests on their own smartphones. Informants were encouraged for all participants and required for those with symptomatic FTLD (Clinical Dementia Rating Scale plus NACC FTLD module [CDR plus NACC-FTLD] global score ≥1). Recruitment targeted individuals with CDR plus NACC-FTLD global scores less than 2, but sites had discretion to enroll more severely impaired participants. Exclusion criteria were consistent with the parent ALLFTD study. 12
Participants were enrolled in the ALLFTD-mApp study within 90 days of annual ALLFTD study visits (including neuropsychological and neuroimaging data collection). Site research coordinators (including J.C.T., A.B.W., S.D., and M.M.) assisted participants with app download, setup, and orientation and observed participants completing the first questionnaire. All cognitive tasks were self-administered without supervision (except pilot participants, discussed below) in a predefined order with minor adjustments throughout the study. Study partners of participants with symptomatic FTLD were asked to remain nearby during participation to help navigate the ALLFTD-mApp but were asked not to assist with testing.
The baseline participation window was divided into three 25- to 35-minute assessment sessions occurring over 11 days. All cognitive tests were repeated in every session to enhance task reliability 6 , 13 and enable assessment of test-retest reliability, except for card sort, which was administered once every 6 months due to expected practice effects. Adherence was defined as the percentage of all available tasks that were completed. Participants were asked to complete the triplicate of sessions every 6 months for the duration of the app study. Only the baseline triplicate was analyzed in this study.
Replicability was tested by dividing the sample into a discovery cohort (n = 258) comprising all participants enrolled until the initial data freeze (October 1, 2022) and a validation cohort (n = 102) comprising participants enrolled after October 1, 2022, and 18 pilot participants 11 who completed the first session in person with an examiner present during cognitive pretesting. Sensitivity analyses excluded this small pilot cohort.
ALLFTD investigators partnered with Datacubed Health 14 to develop the ALLFTD-mApp on Datacubed Health’s Linkt platform. The app includes cognitive, motor, and speech tasks. This study focuses on 6 cognitive tests developed by Datacubed Health 11 comprising an adaptive associative memory task (Humi’s Bistro) and gamified versions of classic executive functioning paradigms: flanker (Ducks in a Pond), Stroop (Color Clash), 2-back (Animal Parade), go/no-go (Go Sushi Go!), and card sort (Card Shuffle) ( Figure 1 and eMethods in Supplement 1 ). Most participants with symptomatic FTLD (49 [72.1%]) were not administered Stroop or 2-back, as pilot studies identified these as too difficult. 11 The app test results were summarized as a composite score (eMethods in Supplement 1 ). Participants completed surveys to assess technological familiarity (daily or less than daily use of a smartphone) and distractions (present or absent).
Criterion standard clinical data were collected during parent project visits. Syndromic diagnoses were made according to published criteria 15 - 19 based on multidisciplinary conferences that considered neurological history, neurological examination results, and collateral interview. 20
The CDR plus NACC-FTLD module is an 8-domain rating scale based on informant and participant report. 21 A global score was calculated to categorize disease severity as asymptomatic or preclinical if a pathogenic variant carrier (0), prodromal (0.5), or symptomatic (1.0-3.0). 22 A sum of the 8 domain box scores (CDR plus NACC-FTLD sum of boxes) was also calculated. 22
Participants completed the UDS Neuropsychological Battery, version 3.0 23 (eMethods in Supplement 1 ), which includes traditional neuropsychological measures and the Montreal Cognitive Assessment (MoCA), a global cognitive screen. Executive functioning and processing speed measures were summarized into a composite score (UDS3-EF). 24 Participants also completed a 9-item list-learning memory test (California Verbal Learning Test, 2nd edition, Short Form). 25 Most (339 [94.2%]) neuropsychological evaluations were conducted in person. In a subsample (n = 270), motor speed and dexterity were assessed using the Movement Disorder Society Uniform Parkinson Disease Rating Scale 26 Finger Tapping subscale (0 indicates no deficits [n = 240]).
We acquired T1-weighted brain magnetic resonance imaging for 199 participants. Details of image acquisition, harmonization, preprocessing, and processing are provided in eMethods in Supplement 1 and prior publications. 27 Briefly, SPM12 (Statistical Parametric Mapping) was used for segmentation 28 and Large Deformation Diffeomorphic Metric Mapping for generating group templates. 29 Gray matter volumes were calculated in template space by integrating voxels and dividing by total intracranial volume in 2 regions of interest (ROIs) 30 : a frontoparietal and subcortical ROI and a hippocampal ROI. Voxel-based morphometry was used to test unbiased voxel-wise associations of volume with smartphone tests (eMethods in Supplement 1 ). 31 , 32
Participants in the ALLFTD study underwent genetic testing 33 at the University of California, Los Angeles. DNA samples were screened using targeted sequencing of a custom panel of genes previously implicated in neurodegenerative diseases, including GRN ( 138945 ) and MAPT ( 157140 ). Hexanucleotide repeat expansions in C9orf72 ( 614260 ) were detected using both fluorescent and repeat-primed polymerase chain reaction analysis. 34
Statistical analyses were conducted using Stata, version 17.0 (StataCorp LLC), and R, version 4.4.2 (R Project for Statistical Computing). All tests were 2 sided, with a statistical significance threshold of P < .05.
Psychometric properties of the smartphone tests were explored using descriptive statistics. Comparisons between CDR plus NACC-FTLD groups (ie, asymptomatic or preclinical, prodromal, and symptomatic) for continuous variables, including demographic characteristics and cognitive task scores (first exposure to each measure), were analyzed by fitting linear regressions. We used χ 2 difference tests for frequency data (eg, sex and race and ethnicity).
Internal consistency, which measures reliability within a task, was estimated for participants’ first exposure to each test using Cronbach α (details in eMethods in Supplement 1 ). Test-retest reliability was estimated using intraclass correlation coefficients for participants who completed a task at least twice; all exposures were included. Reliability estimates are described as poor (<0.500), moderate (0.500-0.749), good (0.750-0.890), and excellent (≥0.900) 35 ; these are reporting rules of thumb, and clinical interpretation should consider raw estimates. We calculated 95% CIs via bootstrapping with 1000 samples.
Validity analyses used participants’ first exposure to each test. Linear regressions were fitted in participants without symptoms with age, sex, and educational level as independent variables to understand the unique contribution of each demographic factor to cognitive test scores. Correlations and linear regression between the app-based tasks and disease severity (CDR plus NACC-FTLD sum of boxes score), neuropsychological test scores, and gray matter ROIs were used to investigate construct validity in the full sample. Demographic characteristics were not entered as covariates because the primary goal was to assess associations between app-based measures and criterion standards, rather than understand the incremental predictive value of app measures. To address potential motor confounds, associations with disease severity were evaluated in a subsample without finger dexterity deficits on motor examination (using the Movement Disorder Society Uniform Parkinson Disease Rating Scale Finger Tapping subscale). To complement ROI-based neuroimaging analysis based on a priori hypotheses, we conducted voxel-based morphometry (eMethods in Supplement 1 ) to uncover other potential neural correlates of test performance. 31 , 32 Finally, we evaluated the association of the number of distractions and operating system with reliability and validity, controlling for age and disease severity, which are predictive factors associated with test performance in correlation analyses.
To evaluate the app’s ability to select participants with prodromal or symptomatic FTLD for trial enrollment, we tested discrimination of participants without symptoms from those with prodromal and symptomatic FTLD. To understand the app’s utility for screening early cognitive impairment, we fit receiver operating characteristics curves testing the predictive value of the app composite, UDS3-EF, and MoCA for differentiating participants without symptoms and those with preclinical FTLD from those with prodromal FTLD; areas under the curves (AUC) for the app and MoCA were compared using the DeLong test in participants with results for both predictive factors.
We compared app performance in preclinical participants who carried pathogenic variants with that in noncarrier controls using linear regression adjusted for age (a predictive factor in earlier models). For this analysis, we excluded those younger than 45 years to remove participants likely to be years from symptom onset based on natural history studies. 4 We analyzed memory performance in participants who carried MAPT pathogenic variants, as early executive deficits may be less prominent. 34 , 36
Of 1163 eligible participants, 360 were enrolled, 439 were excluded, and 364 refused to participate (additional details are provided in the eResults in Supplement 1 ). Participant characteristics are reported in Table 1 for the full sample. The discovery and validation cohorts did not significantly differ in terms of demographic characteristics, disease severity, or cognition (eTable 1 in Supplement 1 ). In the full sample, there were 209 women (58.1%) and 151 men (41.9%), and the mean (SD) age was 54.0 (15.4) years (range, 18-89 years). The mean (SD) educational level was 16.5 (2.3) years (range, 12-20 years). Among the 358 participants with racial and ethnic data available, 340 (95.0%) identified as White. For the 18 participants self-identifying as being of other race or ethnicity, the specific group was not provided to protect participant anonymity. Among the 329 participants with available CDR plus NACC-FTLD scores ( Table 1 ), 195 (59.3%) were asymptomatic or preclinical (Global Score, 0), 66 (20.1%) were prodromal (Global score, 0.5), and 68 (20.7%) were symptomatic (global score, 1.0 or 2.0). Of those with available genetic testing results (n = 222), 100 (45.0%) carried a pathogenic familial FTLD pathogenic variant, including 63 of 120 participants without symptoms and with available results. On average, participants completed 78% of available smartphone measures over a mean (SD) of 2.6 (0.6) sessions.
Descriptive statistics for each task are presented in Table 2 . Ceiling effects were not observed for any tests. A small percentage of participants were at the floor for flanker (19 [5.3%]), go/no-go (13 [4.0%]), and card sort (9 [3.3%]) scores. Floor effects were only observed in participants with prodromal or symptomatic FTLD.
Except for go/no-go, internal consistency estimates ranged from good to excellent (Cronbach α range, 0.84 [95% CI, 0.81-0.87] to 0.99 [95% CI, 0.99-0.99]), and test-retest reliabilities were moderate to excellent (interclass correlation coefficient [ICC] range, 0.77 [95% CI, 0.69-0.83] to 0.95 [95% CI, 0.93-0.96]), with slightly higher estimates in participants with prodromal or symptomatic FTLD ( Table 2 , Figure 2 , and eFigure 1 in Supplement 1 ). Go/no-go reliability was particularly poor in participants without symptoms (ICC, 0.10 [95% CI, −0.37 to 0.48]) and was removed from subsequent validation analyses except the correlation matrix ( Figure 3 A and B). The 95% CIs for reliability estimates overlapped in the discovery and validation cohorts ( Figure 2 ). Reliability estimates showed overlapping 95% CIs regardless of distractions (eFigure 2 in Supplement 1 ) or operating systems (eFigure 3 in Supplement 1 ), with a pattern of slightly lower reliability estimates when distractions were endorsed for all comparisons except Stroop (Cronbach α).
In 57 participants without symptoms who did not carry pathogenic variants, older age was associated with worse performance on all measures (β range, − 0.40 [95 CI, −0.68 to −0.13] to −0.78 [95 CI, −0.89 to −0.52]; P ≤ .03), except card sort (β = −0.22 [95% CI, −0.54 to 0.09]; P = .16) and go-no/go (β = −0.15 [95% CI, −0.44 to 0.14]; P = .31), though associations were in the expected direction. Associations with sex and educational level were not statistically significant.
Cognitive tests administered using the app showed evidence of convergent and divergent validity (eFigure 4 in Supplement 1 ), with very similar findings in discovery ( Figure 3 A) and validation cohorts ( Figure 3 B). App–based measures of executive functioning were generally correlated with criterion standard in-person measures of these domains and less with measures of other cognitive domains ( r range, 0.40-0.66). For example, the flanker task was associated with the UDS3-EF composite (β = 0.58 [95% CI, 0.48-0.68]; P < .001) and measures of visuoconstruction (β for Benson Figure Copy, 0.43 [95% CI, 0.32-0.54]; P = .01) and naming (β for Multilingual Naming Test, 0.25 [95% CI, 0.14-0.37]; P < .001). The app memory test was associated with criterion standard memory and executive functioning tests.
Worse performance on all app measures was associated with greater disease severity on CDR plus NACC-FTLD ( r range, 0.38-0.59) ( Table 1 , Figure 3 , and eFigure 4 in Supplement 1 ). The same pattern of results was observed after excluding those with finger dexterity issues. Except for go/no-go, performance of participants with prodromal FTLD was statistically significantly worse than that of participants without symptoms on all measures ( P < .001).
The AUC for the app composite to distinguish participants without symptoms from those with dementia was 0.93 (95% CI, 0.90-0.96). The app also accurately differentiated participants without symptoms from those with prodromal or symptomatic FTLD (AUC, 0.87 [95% CI, 0.84-0.92]). Compared with the MoCA (AUC, 0.68 [95% CI, 0.59-0.78), app composite performance (AUC, 0.82 [95% CI, 0.76-0.88]) more accurately differentiated participants without symptoms and with prodromal FTLD ( z of comparison, −2.49 [95% CI, −0.19 to −0.02]; P = .01), with similar accuracy to the UDS3-EF (AUC, 0.81 [95% CI, 0.73-0.88]); highly similar results (eTable 2 in Supplement 1 ) were observed in the discovery ( Figure 3 C) and validation ( Figure 3 D) cohorts.
In 56 participants without symptoms who were older than 45 years, those carrying GRN , C9orf72 , or another rare pathogenic variants performed significantly worse on 3 of 4 executive tests compared with noncarrier controls, including flanker (β = −0.26 [95% CI, −0.46 to −0.05]; P = .02), card sort (β = −0.28 [95% CI, −0.54 to −0.30]; P = .03), and 2-back (β = −0.49 [95% CI, −0.72 to −0.25]; P < .001). The estimated scores of participants who carried pathogenic variants were on average lower than those of carriers on a composite of criterion standard in-person tests, but the difference was not statistically significant (UDS3-EF β = −0.14 [95% CI, −0.42 to 0.14]; P = .32). Participants who carried preclinical MAPT pathogenic variants scored higher than noncarriers on the app Memory test, though the difference was not statistically significant (β = 0.21 [95% CI, −0.50 to 0.58]; P = .19).
In prespecified ROI analyses, worse app executive functioning scores were associated with lower frontoparietal and/or subcortical volume ( Figures 3 A and B) (β range, 0.34 [95% CI, 0.22-0.46] to 0.50 [95 CI, 0.40-0.60]; P < .001 for all) and worse memory scores with smaller hippocampal volume (β = 0.45 [95% CI, 0.34-0.56]; P < .001). Voxel-based morphometry (eFigure 5 in Supplement 1 ) suggested worse app performance was associated with widespread atrophy, particularly in frontotemporal cortices.
Only for card sort were distractions (eTables 3 and 4 in Supplement 1 ) associated with task performance; those experiencing distractions unexpectedly performed better (β = 0.16 [95% CI, 0.05-0.28]; P = .005). The iPhone operating system was associated with better performance on 2 speeded tasks: flanker (β = 0.16 [95% CI, 0.07-0.24]; P < .001) and go/no-go (β = 0.16 [95% CI, 0.06-0.26]; P = .002). In a sensitivity analysis, associations of all app tests with disease severity, UDS3-EF, and regional brain volumes remained after covarying for distractions and operating system, as did the models differentiating participants who carried preclinical pathogenic variants and noncarrier controls.
There is an urgent need to identify reliable and valid digital tools for remote neurobehavioral measurement in neurodegenerative diseases, including FTLD. Prior studies provided preliminary evidence that smartphones collect reliable and valid cognitive data in a variety of age-related and neurodegenerative illnesses. This is the first study, to our knowledge, to provide analogous support for the reliability and validity of remote cognitive testing via smartphones in FTLD and preliminary evidence that this approach improves early detection relative to traditional in-person measures.
Reliability, a prerequisite for a valid clinical trial end point, indicates measurements are consistent. In 2 cohorts, we found smartphone cognitive tests were reliable within a single administration (ie, internally consistent) and across repeated assessments (ie, test-retest reliability) with no apparent differences by operating system. For all measures except go/no-go, reliability estimates were moderate to excellent and on par with other remote digital assessments 5 , 6 , 10 , 37 , 38 and in-clinic criterion standards. 39 - 41 Go/no-go showed similar within- and between-person variability in participants without symptoms (ie, poor reliability), and participant feedback suggested instructions were confusing and the stimuli disappeared too quickly. Those endorsing distractions tended to have lower reliability, though 95% CIs largely overlapped; future research detailing the effect of the home environment on test performance is warranted.
Construct validity was supported by strong associations of smartphone tests with demographics, disease severity, neuroimaging, and criterion standard neuropsychological measures that replicated in a validation sample. These associations were similar to those observed among the criterion standard measures and similar to associations reported in other validation studies of smartphone cognitive tests. 5 , 6 , 10 Associations with disease severity were not explained by motor impairments. The iPhone operating system was associated with better performance on 2 time-based measures, consistent with prior findings. 6
A composite of brief smartphone tests was accurate in distinguishing dementia from cognitively unimpaired participants, screening out participants without symptoms, and detecting prodromal FTLD with greater sensitivity than the MoCA. Moreover, carriers of preclinical C9orf72 and GRN pathogenic variants performed significantly worse than noncarrier controls on 3 tests, whereas they did not significantly differ on criterion standard measures. These findings are consistent with previous studies showing digital executive functioning paradigms may be more sensitive to early FTLD than traditional measures. 42 , 43
This study has some limitations. Validation analyses focused on participants’ initial task exposure. Future studies will explore whether repeated measurements and more sophisticated approaches to composite building (current composite assumes equal weighting of tests) improve reliability and sensitivity, and a normative sample is being collected to better adjust for demographic effects on testing. 24 Longitudinal analyses will explore whether the floor effects in participants with symptomatic FTLD will affect the utility for monitoring. The generalizability of the findings is limited by the study cohort, which comprised participants who were college educated on average, mostly White, and primarily English speakers who owned smartphones and participated in the referring in-person research study. Equity in access to research is a priority in FTLD research 44 , 45 ; translations of the ALLFTD-mApp are in progress, cultural adaptations are being considered, and devices have been purchased for provisioning to improve the diversity of our sample.
The findings of this cohort study, coupled with prior reports indicating that smartphone testing is feasible and acceptable to patients with FTLD, 11 suggest that smartphones may complement traditional in-person research paradigms. More broadly, the scalability, ease of use, reliability, and validity of the ALLFTD-mApp suggest the feasibility and utility of remote digital assessments in dementia clinical trials. Future research should validate these results in diverse populations and evaluate the utility of these tests for longitudinal monitoring.
Accepted for Publication: February 2, 2024.
Published: April 1, 2024. doi:10.1001/jamanetworkopen.2024.4266
Open Access: This is an open access article distributed under the terms of the CC-BY License . © 2024 Staffaroni AM et al. JAMA Network Open .
Corresponding Author: Adam M. Staffaroni, PhD, Weill Institute for Neurosciences, Department of Neurology, Memory and Aging Center, University of California, San Francisco, 675 Nelson Rising Ln, Ste 190, San Francisco, CA 94158 ( [email protected] ).
Author Contributions: Dr Staffaroni had full access to all of the data in the study and takes responsibility for the integrity of the data and the accuracy of the data analysis.
Concept and design: Staffaroni, A. Clark, Taylor, Heuer, Wise, Forsberg, Miller, Hassenstab, Rosen, Boxer.
Acquisition, analysis, or interpretation of data: Staffaroni, A. Clark, Taylor, Heuer, Sanderson-Cimino, Wise, Dhanam, Cobigo, Wolf, Manoochehri, Mester, Rankin, Appleby, Bayram, Bozoki, D. Clark, Darby, Domoto-Reilly, Fields, Galasko, Geschwind, Ghoshal, Graff-Radford, Hsiung, Huey, Jones, Lapid, Litvan, Masdeu, Massimo, Mendez, Miyagawa, Pascual, Pressman, Ramanan, Ramos, Rascovsky, Roberson, Tartaglia, Wong, Kornak, Kremers, Kramer, Boeve, Boxer.
Drafting of the manuscript: Staffaroni, A. Clark, Taylor, Heuer, Wolf, Lapid.
Critical review of the manuscript for important intellectual content: Staffaroni, Taylor, Heuer, Sanderson-Cimino, Wise, Dhanam, Cobigo, Manoochehri, Forsberg, Mester, Rankin, Appleby, Bayram, Bozoki, D. Clark, Darby, Domoto-Reilly, Fields, Galasko, Geschwind, Ghoshal, Graff-Radford, Hsiung, Huey, Jones, Lapid, Litvan, Masdeu, Massimo, Mendez, Miyagawa, Pascual, Pressman, Ramanan, Ramos, Rascovsky, Roberson, Tartaglia, Wong, Miller, Kornak, Kremers, Hassenstab, Kramer, Boeve, Rosen, Boxer.
Statistical analysis: Staffaroni, A. Clark, Taylor, Heuer, Sanderson-Cimino, Cobigo, Kornak, Kremers.
Obtained funding: Staffaroni, Rosen, Boxer.
Administrative, technical, or material support: A. Clark, Taylor, Heuer, Wise, Dhanam, Wolf, Manoochehri, Forsberg, Darby, Domoto-Reilly, Ghoshal, Hsiung, Huey, Jones, Litvan, Massimo, Mendez, Miyagawa, Pascual, Pressman, Ramanan, Kramer, Boeve, Boxer.
Supervision: Geschwind, Miyagawa, Roberson, Kramer, Boxer.
Conflict of Interest Disclosures: Dr Staffaroni reported being a coinventor of 4 ALLFTD mobile application tasks (not analyzed in the present study) and receiving licensing fees from Datacubed Health; receiving research support from the National Institute on Aging (NIA) of the National Institutes of Health (NIH), Bluefield Project to Cure FTD, the Alzheimer’s Association, the Larry L. Hillblom Foundation, and the Rainwater Charitable Foundation; and consulting for Alector Inc, Eli Lilly and Company/Prevail Therapeutics, Passage Bio Inc, and Takeda Pharmaceutical Company. Dr Forsberg reported receiving research support from the NIH. Dr Rankin reported receiving research support from the NIH and the National Science Foundation and serving on the medical advisory board for Eli Lilly and Company. Dr Appleby reported receiving research support from the Centers for Disease Control and Prevention (CDC), the NIH, Ionis Pharmaceuticals Inc, Alector Inc, and the CJD Foundation and consulting for Acadia Pharmaceuticals Inc, Ionis Pharmaceuticals Inc, and Sangamo Therapeutics Inc. Dr Bayram reported receiving research support from the NIH. Dr Domoto-Reilly reported receiving research support from NIH and serving as an investigator for a clinical trial sponsored by Lawson Health Research Institute. Dr Bozoki reported receiving research funding from the NIH, Alector Inc, Cognition Therapeutics Inc, EIP Pharma, and Transposon Therapeutics Inc; consulting for Eisai and Creative Bio-Peptides Inc; and serving on the data safety monitoring board for AviadoBio. Dr Fields reported receiving research support from the NIH. Dr Galasko reported receiving research funding from the NIH; clinical trial funding from Alector Inc and Esai; consulting for Esai, General Electric Health Care, and Fujirebio; and serving on the data safety monitoring board of Cyclo Therapeutics Inc. Dr Geschwind reported consulting for Biogen Inc and receiving research support from Roche and Takeda Pharmaceutical Company for work in dementia. Dr Ghoshal reported participating in clinical trials of antidementia drugs sponsored by Bristol Myers Squibb, Eli Lilly and Company/Avid Radiopharmaceuticals, Janssen Immunotherapy, Novartis AG, Pfizer Inc, Wyeth Pharmaceuticals, SNIFF (The Study of Nasal Insulin to Fight Forgetfulness) study, and A4 (The Anti-Amyloid Treatment in Asymptomatic Alzheimer’s Disease) trial; receiving research support from Tau Consortium and the Association for Frontotemporal Dementia; and receiving funding from the NIH. Dr Graff-Radford reported receiving royalties from UpToDate; reported participating in multicenter therapy studies by sponsored by Biogen Inc, TauRx Therapeutics Ltd, AbbVie Inc, Novartis AG, and Eli Lilly and Company; and receiving research support from the NIH. Dr Grossman reported receiving grant support from the NIH, Avid Radiopharmaceuticals, and Piramal Pharma Ltd; participating in clinical trials sponsored by Biogen Inc, TauRx Therapeutics Ltd, and Alector Inc; consulting for Bracco and UCB; and serving on the editorial board of Neurology . Dr Hsiung reported receiving grant support from the Canadian Institutes of Health Research, the NIH, and the Alzheimer Society of British Columbia; participating in clinical trials sponsored by Anavax Life Sciences Corp, Biogen Inc, Cassava Sciences, Eli Lilly and Company, and Roche; and consulting for Biogen Inc, Novo Nordisk A/S, and Roche. Dr Huey reported receiving research support from the NIH. Dr Jones reported receiving research support from the NIH. Dr Litvan reported receiving research support from the NIH, the Michael J Fox Foundation, the Parkinson Foundation, the Lewy Body Association, CurePSP, Roche, AbbVie Inc, H Lundbeck A/S, Novartis AG, Transposon Therapeutics Inc, and UCB; serving as a member of the scientific advisory board for the Rossy PSP Program at the University of Toronto and for Amydis; and serving as chief editor of Frontiers in Neurology . Dr Masdeu reported consulting for and receiving research funding from Eli Lilly and Company; receiving personal fees from GE Healthcare; receiving grant funding and personal fees from Eli Lilly and Company; and receiving grant funding from Acadia Pharmaceutical Inc, Avanir Pharmaceuticals Inc, Biogen Inc, Eisai, Janssen Global Services LLC, the NIH, and Novartis AG outside the submitted work. Dr Mendez reported receiving research support from the NIH. Dr Miyagawa reported receiving research support from the Zander Family Foundation. Dr Pascual reported receiving research support from the NIH. Dr Pressman reported receiving research support from the NIH. Dr Ramos reported receiving research support from the NIH. Dr Roberson reported receiving research support from the NIA of the NIH, the Bluefield Project, and the Alzheimer’s Drug Discovery Foundation; serving on a data monitoring committee for Eli Lilly and Company; receiving licensing fees from Genentech Inc; and consulting for Applied Genetic Technologies Corp. Dr Tartaglia reported serving as an investigator for clinical trials sponsored by Biogen Inc, Avanex Corp, Green Valley, Roche/Genentech Inc, Bristol Myers Squibb, Eli Lilly and Company/Avid Radiopharmaceuticals, and Janssen Global Services LLC and receiving research support from the Canadian Institutes of Health Research (CIHR). Dr Wong reported receiving research support from the NIH. Dr Kornak reported providing expert witness testimony for Teva Pharmaceuticals Industries Ltd, Apotex Inc, and Puma Biotechnology and receiving research support from the NIH. Dr Kremers reported receiving research funding from NIH. Dr Kramer reported receiving research support from the NIH and royalties from Pearson Inc. Dr Boeve reported serving as an investigator for clinical trials sponsored by Alector Inc, Biogen Inc, and Transposon Therapeutics Inc; receiving royalties from Cambridge Medicine; serving on the Scientific Advisory Board of the Tau Consortium; and receiving research support from NIH, the Mayo Clinic Dorothy and Harry T. Mangurian Jr. Lewy Body Dementia Program, and the Little Family Foundation. Dr Rosen reported receiving research support from Biogen Inc, consulting for Wave Neuroscience and Ionis Pharmaceuticals, and receiving research support from the NIH. Dr Boxer reported being a coinventor of 4 of the ALLFTD mobile application tasks (not the focus of the present study) and previously receiving licensing fees; receiving research support from the NIH, the Tau Research Consortium, the Association for Frontotemporal Degeneration, Bluefield Project to Cure Frontotemporal Dementia, Corticobasal Degeneration Solutions, the Alzheimer’s Drug Discovery Foundation, and the Alzheimer’s Association; consulting for Aeovian Pharmaceuticals Inc, Applied Genetic Technologies Corp, Alector Inc, Arkuda Therapeutics, Arvinas Inc, AviadoBio, Boehringer Ingelheim, Denali Therapeutics Inc, GSK, Life Edit Therapeutics Inc, Humana Inc, Oligomerix, Oscotec Inc, Roche, Transposon Therapeutics Inc, TrueBinding Inc, and Wave Life Sciences; and receiving research support from Biogen Inc, Eisai, and Regeneron Pharmaceuticals Inc. No other disclosures were reported.
Funding/Support: This work was supported by grants AG063911, AG077557, AG62677, AG045390, NS092089, AG032306, AG016976, AG058233, AG038791, AG02350, AG019724, AG062422, NS050915, AG032289-11, AG077557, K23AG061253, and K24AG045333 from the NIH; the Association for Frontotemporal Degeneration; the Bluefield Project to Cure FTD; the Rainwater Charitable Foundation; and grant 2014-A-004-NET from the Larry L. Hillblom Foundation. Samples from the National Centralized Repository for Alzheimer’s Disease and Related Dementias, which receives government support under cooperative agreement grant U24 AG21886 from the NIA, were used in this study.
Role of the Funder/Sponsor: The funders had no role in the design and conduct of the study; collection, management, analysis, and interpretation of the data; preparation, review, or approval of the manuscript; and decision to submit the manuscript for publication.
Group Information: A complete list of the members of the ALLFTD Consortium appears in Supplement 2 .
Data Sharing Statement: See Supplement 3 .
Additional Contributions: We thank the participants and study partners for dedicating their time and effort, and for providing invaluable feedback as we learn how to incorporate digital technologies into FTLD research.
Additional Information: Dr Grossman passed away on April 4, 2023. We want to acknowledge his many contributions to this study, including data acquisition, and design and conduct of the study. He was an ALLFTD site principal investigator and contributed during the development of the ALLFTD mobile app.
- Register for email alerts with links to free full-text articles
- Access PDFs of free articles
- Manage your interests
- Save searches and receive search alerts
Advertisement
Supported by
Use of Abortion Pills Has Risen Significantly Post Roe, Research Shows

By Pam Belluck
Pam Belluck has been reporting about reproductive health for over a decade.
- Share full article
On the eve of oral arguments in a Supreme Court case that could affect future access to abortion pills, new research shows the fast-growing use of medication abortion nationally and the many ways women have obtained access to the method since Roe v. Wade was overturned in June 2022.
The Details

A study, published on Monday in the medical journal JAMA , found that the number of abortions using pills obtained outside the formal health system soared in the six months after the national right to abortion was overturned. Another report, published last week by the Guttmacher Institute , a research organization that supports abortion rights, found that medication abortions now account for nearly two-thirds of all abortions provided by the country’s formal health system, which includes clinics and telemedicine abortion services.
The JAMA study evaluated data from overseas telemedicine organizations, online vendors and networks of community volunteers that generally obtain pills from outside the United States. Before Roe was overturned, these avenues provided abortion pills to about 1,400 women per month, but in the six months afterward, the average jumped to 5,900 per month, the study reported.
Overall, the study found that while abortions in the formal health care system declined by about 32,000 from July through December 2022, much of that decline was offset by about 26,000 medication abortions from pills provided by sources outside the formal health system.
“We see what we see elsewhere in the world in the U.S. — that when anti-abortion laws go into effect, oftentimes outside of the formal health care setting is where people look, and the locus of care gets shifted,” said Dr. Abigail Aiken, who is an associate professor at the University of Texas at Austin and the lead author of the JAMA study.
The co-authors were a statistics professor at the university; the founder of Aid Access, a Europe-based organization that helped pioneer telemedicine abortion in the United States; and a leader of Plan C, an organization that provides consumers with information about medication abortion. Before publication, the study went through the rigorous peer review process required by a major medical journal.
The telemedicine organizations in the study evaluated prospective patients using written medical questionnaires, issued prescriptions from doctors who were typically in Europe and had pills shipped from pharmacies in India, generally charging about $100. Community networks typically asked for some information about the pregnancy and either delivered or mailed pills with detailed instructions, often for free.
Online vendors, which supplied a small percentage of the pills in the study and charged between $39 and $470, generally did not ask for women’s medical history and shipped the pills with the least detailed instructions. Vendors in the study were vetted by Plan C and found to be providing genuine abortion pills, Dr. Aiken said.
The Guttmacher report, focusing on the formal health care system, included data from clinics and telemedicine abortion services within the United States that provided abortion to patients who lived in or traveled to states with legal abortion between January and December 2023.
It found that pills accounted for 63 percent of those abortions, up from 53 percent in 2020. The total number of abortions in the report was over a million for the first time in more than a decade.
Why This Matters
Overall, the new reports suggest how rapidly the provision of abortion has adjusted amid post-Roe abortion bans in 14 states and tight restrictions in others.
The numbers may be an undercount and do not reflect the most recent shift: shield laws in six states allowing abortion providers to prescribe and mail pills to tens of thousands of women in states with bans without requiring them to travel. Since last summer, for example, Aid Access has stopped shipping medication from overseas and operating outside the formal health system; it is instead mailing pills to states with bans from within the United States with the protection of shield laws.
What’s Next
In the case that will be argued before the Supreme Court on Tuesday, the plaintiffs, who oppose abortion, are suing the Food and Drug Administration, seeking to block or drastically limit the availability of mifepristone, the first pill in the two-drug medication abortion regimen.
The JAMA study suggests that such a ruling could prompt more women to use avenues outside the formal American health care system, such as pills from other countries.
“There’s so many unknowns about what will happen with the decision,” Dr. Aiken said.
She added: “It’s possible that a decision by the Supreme Court in favor of the plaintiffs could have a knock-on effect where more people are looking to access outside the formal health care setting, either because they’re worried that access is going away or they’re having more trouble accessing the medications.”
Pam Belluck is a health and science reporter, covering a range of subjects, including reproductive health, long Covid, brain science, neurological disorders, mental health and genetics. More about Pam Belluck
- Global Elections
- TikTok Twitter Facebook
- About Careers
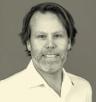
Despite the AI safety hype, a new study finds little research on the topic
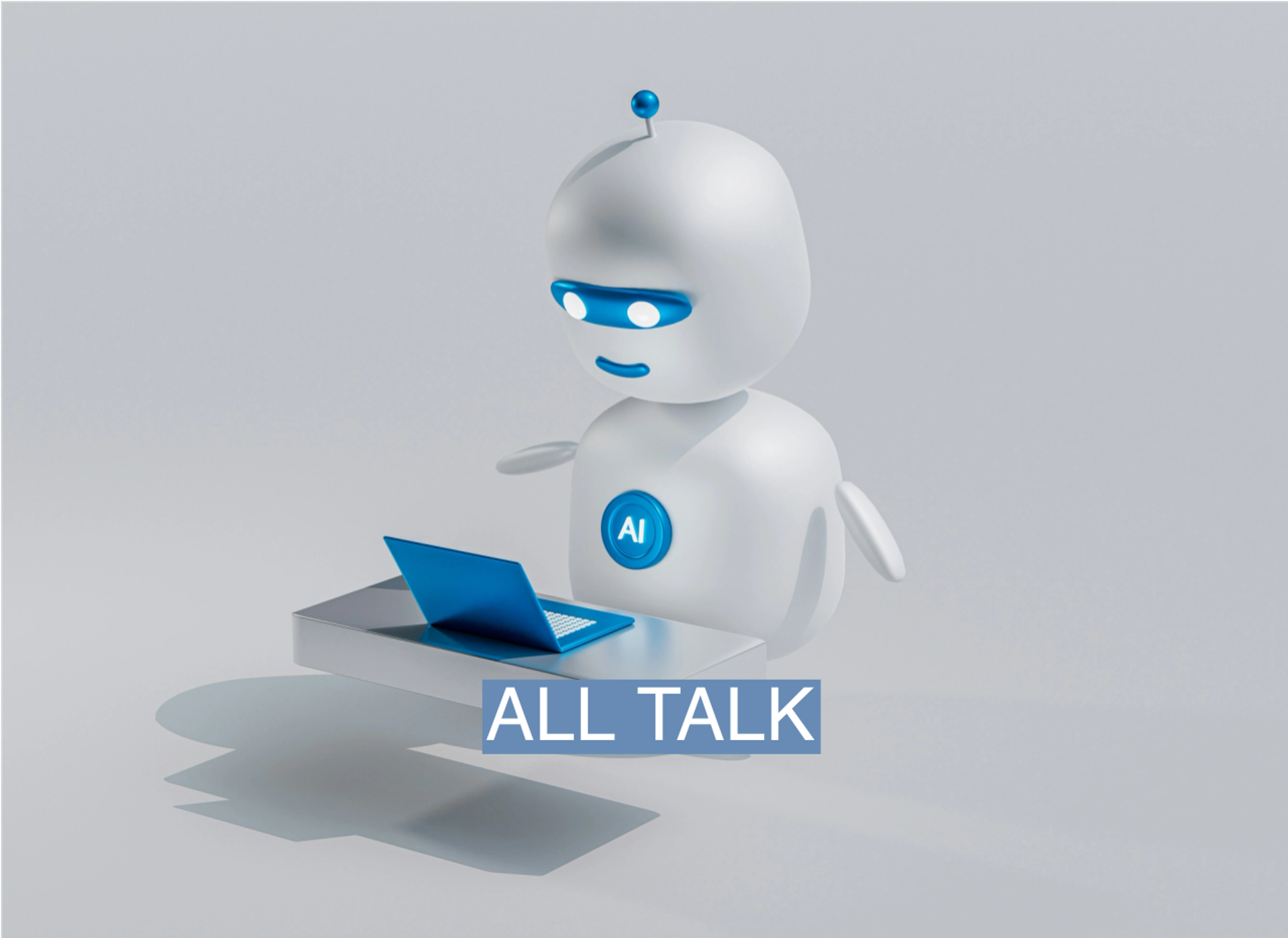
Sign up for Semafor Technology: What’s next in the new era of tech. Read it now .
In this article:

Reed’s view

Room for Disagreement

In public policy conversations about artificial intelligence, “safety research” is one of the biggest topics that has helped drive new regulations around the world.
But according to a new study, there appears to be more talk about safety than hard data.
AI safety accounts for only 2% of overall AI research, according to a new study conducted by Georgetown University’s Emerging Technology Observatory that was shared exclusively with Semafor.
Georgetown found that American scholarly institutions and companies are the biggest contributors to AI safety research, but it pales in comparison to the amount of overall studies into AI, raising questions about public and private sector priorities.
Of the 172,621 AI research papers published by American authors between 2017 and 2021, only 5% were on safety. For China, the difference was even starker, with only 1% of research published focusing on AI safety.
Nevertheless, studies on the topic are on the rise globally, with AI safety research papers more than quadrupling between 2017 and 2022.
Georgetown’s Emerging Technology Observatory is part of The Center for Security and Emerging Technology, which received over $100 million in funding from Open Philanthropy, the charity backed by Facebook co-founder Dustin Moskovitz, who is a major advocate of AI safety.
Moskovitz and the field of AI safety in general are tied to the Effective Altruism movement, which hopes to curb existential risks to humanity, such as runaway AI systems.
The topic of AI safety is a hot button issue in the tech industry and has spawned a counter movement, called Effective Accelerationism, which believes that focusing on the risks of technology does more harm than good by hindering critical progress.
Recently, the definition of AI safety has expanded to include more than just existential risks, such as bias in labor issues. That trend has drawn criticism from some in the AI safety field and praise from others.
The Georgetown researchers who conducted the study decided to include the broader definition of AI safety research, and not just existential risks.
The researchers relied on metadata from a database of 260 million scholarly articles that is maintained by the Emerging Technology Observatory and The Center for Security and Emerging Technology. It defined an AI safety article as one that “subject matter experts would consider highly relevant to the topic,” which requires some judgment calls on the part of the researchers.
As the researchers note, not all safety research comes in the form of a public research paper. Tech companies would argue that AI safety is built into the work they do. And the counterintuitive argument is that researchers have to build advanced AI to understand how to protect against it.
In a recent interview with Lex Fridman, OpenAI CEO Sam Altman said that at some point in the future, AI safety will be “mostly what we think about” at his firm. “More and more of the company thinks about those issues all the time,” he said. Still, OpenAI did not show up as a major contributor to AI research in the Georgetown study.
The Effective Accelerationist argument is that the risks of AI are overblown, and 30,000 AI safety papers over five years sounds significant, considering the nascent nature of this technology. How many papers on automobile safety were written before the Model T was invented and sold?
What makes less sense is proposing stringent AI regulations while not also advocating for a massive increase in grant money for AI research, including funding compute power needed for academics to study massive new AI models.
President Joe Biden’s executive order on AI does include provisions for AI safety research. The Commerce Department’s new AI Safety Institute is one example. And the National Artificial Intelligence Research Resource pilot program aims to add more compute power for researchers.
But these measures don’t even begin to keep up with the advances being made in industry.
Big technology companies are currently constructing supercomputers so enormous they would have been difficult to contemplate a few years ago. They will soon find out what happens when AI models are scaled to unfathomable levels, and they will likely keep those trade secrets close to the vest.
To get their hands on that kind of compute power, AI safety researchers will have to work for those companies.
As the CSET study points out, Google and Microsoft are some of the biggest contributors to published papers on AI safety research.
But much of that research came out of an era before ChatGPT. The consumer interest in generative AI has changed the commercial landscape and we’re now seeing fewer research papers come out of big technology companies, which are mostly keeping breakthroughs behind closed doors.
If elected officials really care about AI safety going forward, they would likely accomplish more by allocating taxpayer dollars to basic AI research than they would by passing a comprehensive AI bill when we know so little about how this technology will change society even five years from now.
One argument is that AI safety research is a futile endeavor, and the only way to ensure AI is safe is to pause its development. Tamlyn Hunt argued in this article in Scientific American: “Imagining we can understand AGI/ASI [Artificial General Intelligence and Artificial Super Intelligence], let alone control it, is like thinking a strand of a spider’s web could restrain Godzilla. Any solutions we can develop will be only probabilistic, not airtight. With AGI likely fooming into superintelligence essentially overnight, we can’t accept probabilistic solutions because AI will be so smart it will exploit any tiny hole, no matter how small. (Has the “foom” already happened? Suggestive reports about “Q*” in the wake of the bizarre drama at Open AI in November suggest that foom may be real already.)”
- AI is potentially so transformative — and destructive — that it is often compared to nuclear weapons. In that analogy, it would be as if the U.S. government allowed the private sector to be entirely responsible for creating the nuclear bomb. In this Salon article , Jacy Reese Anthis argues we need a Manhattan Project for AI.
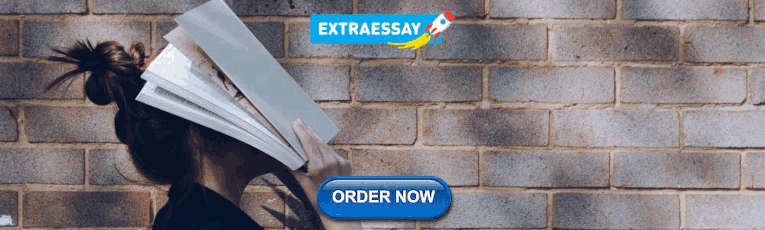
IMAGES
VIDEO
COMMENTS
Several methods can be employed in qualitative methodology, as indicated by Queirós et al. (2017): (i) observation; (ii) ethnography; (iii) field research; (iv) focus groups; or (v) case studies. The case study is a qualitative method that generally consists of a way to deepen an individual unit.
However, there is no single, coherent set of validity and reliability tests for each research phase in case study research available in the literature. This article presents an argument for the case study method in marketing research, examining various criteria for judging the quality of the method and highlighting various techniques, which can ...
validity and reliability of the case study evidence which are; (1) multiple sources of evidence; (2) create a case study database; and (3) maintain a chain of evidence. With regards to rigour and thoroughness in case study process, the elements of construct validity, internal validity, external validity and reliability is the strategy used to ...
In assessing validity of qualitative research, the challenge can start from the ontology and epistemology of the issue being studied, ... Most qualitative research studies, if not all, are meant to study a specific issue or phenomenon in a certain population or ethnic group, of a focused locality in a particular context, hence generalizability ...
The case study can be used for two main purposes: explorato ry and. descriptive (Yin, 2017). The exploratory study contributes to clarify a. situation where information is scarce. The level of ...
Reliability is about the consistency of a measure, and validity is about the accuracy of a measure.opt. It's important to consider reliability and validity when you are creating your research design, planning your methods, and writing up your results, especially in quantitative research. Failing to do so can lead to several types of research ...
Abstract. Despite the advantages of the case study method, its reliability and validity remain in doubt. Tests to establish the validity and reliability of qualitative data are important to determine the stability and quality of the data obtained. However, there is no single, coherent set of validity and reliability tests for each research ...
Validity and generalization continue to be challenging aspects in designing and conducting case study evaluations, especially when the number of cases being studied is highly limited (even limited to a single case). To address the challenge, this article highlights current knowledge regarding the use of: (1) rival explanations, triangulation ...
Focusing on the case study method helps to answer the identified research questions in an explanatory, descriptive way and also helps to construct validity and reliability which works as a medium ...
ABSTRACT. This chapter investigates in depth the issues of establishing reliability and validity in case study approaches to marketing research. IT will be particularly valuable to postgraduates in marketing research who are using the case study approach. It explores the difference between reliability (replicability of results) and validity ...
When a test has strong face validity, anyone would agree that the test's questions appear to measure what they are intended to measure. For example, looking at a 4th grade math test consisting of problems in which students have to add and multiply, most people would agree that it has strong face validity (i.e., it looks like a math test).
Revised on 10 October 2022. Reliability and validity are concepts used to evaluate the quality of research. They indicate how well a method, technique, or test measures something. Reliability is about the consistency of a measure, and validity is about the accuracy of a measure. It's important to consider reliability and validity when you are ...
This chapter thus calls into question some of the usual assumptions applied to case study research. Most case study researchers perceive only a distant and tenuous connection between their work and the laboratory experiment, with a manipulated treatment and randomized control. Type. Chapter. Information.
The theory contribution of case study research designs. Business Research, 10, 281-305. doi: 10.1007/s40685-017-0045-z Riege, A. (2003). Validity and reliability tests in case study research: a literature review with "hands‐on" applications for each research phase.
Central to all research is the goal of finding plausible and credible outcome explanations using the concepts of reliability and validity to attain rigor as "without rigor, research is worthless, becomes fiction, and loses its utility" (Morse et al. 2002:14).The validity of theory or findings (assuring that what is measured accurately reflects what is intended) and reliability (assuring ...
5.5 Conclusion. The energy and exactitude with which development researchers debate the veracity of claims about 'causality' and 'impact' (internal validity) has yet to inspire corresponding firepower in the domain of concerns about whether and how to 'replicate' and 'scale up' interventions (external validity).
Although the tests and measures used to establish the validity and reliability of quantitative research cannot be applied to qualitative research, there are ongoing debates about whether terms such as validity, reliability and generalisability are appropriate to evaluate qualitative research.2-4 In the broadest context these terms are applicable, with validity referring to the integrity and ...
Validity. Validity is defined as the extent to which a concept is accurately measured in a quantitative study. For example, a survey designed to explore depression but which actually measures anxiety would not be considered valid. The second measure of quality in a quantitative study is reliability, or the accuracy of an instrument.In other words, the extent to which a research instrument ...
A case study is one of the most commonly used methodologies of social research. This article attempts to look into the various dimensions of a case study research strategy, the different epistemological strands which determine the particular case study type and approach adopted in the field, discusses the factors which can enhance the effectiveness of a case study research, and the debate ...
This study aims to concisely explore the main difficulties inherent to the process of developing a case study, also attempting to suggest some practices that can increase its reliability, construct validity, internal and external validity. The case study is a widely used method in qualitative research. Although defining the case study can be simple, it is complex to develop its strategy.
Validity is important in market research and survey studies to ensure that the survey questions effectively measure consumer preferences, buying behaviors, or attitudes towards products or services. Validity assessments help researchers determine if the survey instrument is accurately capturing the desired information and if the results can be ...
The review suggests that there are inherent limitations in case study research, particularly related to validity and reliability which are difficult to eliminate in full. However, minimizing the ...
The validity of a research study refers to how well the results among the study participants represent true findings among similar individuals outside the study. This concept of validity applies to all types of clinical studies, including those about prevalence, associations, interventions, and diagnosis.
Observational research participants were enrolled through 18 centers of a North American FTLD research consortium (ALLFTD) and were asked to complete the tests remotely using their own smartphones. Of 1163 eligible individuals (enrolled in parent studies), 360 were enrolled in the present study; 364 refused and 439 were excluded.
From 2015-23, McKinsey & Company, a multinational strategy and management consulting firm, released four separate studies showing that DEI initiatives boost corporate earnings.
The tool was then applied to two real case studies in the agri-food sector, specifically analyzing an artichoke and olive oil producer, to assess its validity and effectiveness.FindingsThe study introduces the Circular Resource Box (CRB) as a key innovation in the C-VSM tool.
The findings from the National Institutes of Health are at odds with previous research that looked into the mysterious health incidents experienced by U.S. diplomats and spies.
The News. On the eve of oral arguments in a Supreme Court case that could affect future access to abortion pills, new research shows the fast-growing use of medication abortion nationally and the ...
Of the 172,621 AI research papers published by American authors between 2017 and 2021, only 5% were on safety. For China, the difference was even starker, with only 1% of research published focusing on AI safety. Nevertheless, studies on the topic are on the rise globally, with AI safety research papers more than quadrupling between 2017 and 2022.