
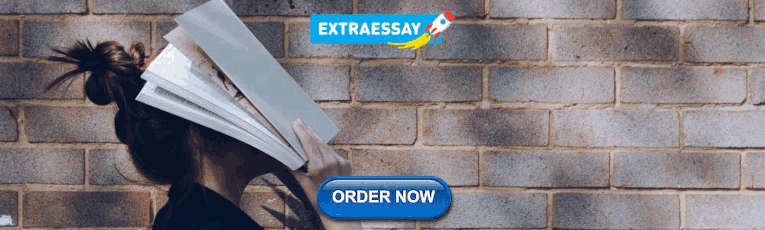
Conceptual and Theoretical Frameworks for Thesis Studies: What you must know
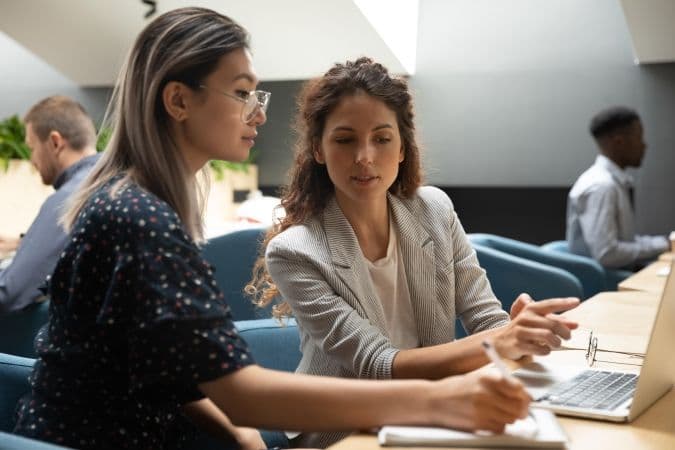
A theoretical framework is a conceptual model that provides a systematic and structured way of thinking about a research problem or question. It helps to identify key variables and the relationships between them and to guide the selection and interpretation of data. Theoretical frameworks draw on existing theories and research and can be used to develop new hypotheses or test existing ones. They provide a foundation for research design, data collection, and analysis and can help to ensure that research is relevant, rigorous, and coherent. Theoretical frameworks are common in many disciplines, including social sciences, natural sciences, and humanities, and are essential for building knowledge and advancing understanding in a field.
This article explains the importance of frameworks in a thesis study and the differences between conceptual frameworks and theoretical frameworks. It provides guidelines on how to write a thesis framework, definitions of variable types, and examples of framework types.
What is a research framework and why do I need one?
When planning your thesis study, you need to justify your research and explain its design to your readers. This is called the research framework.
When planning your thesis study, you need to justify your research and explain its design to your readers. This is called the research framework. Think of it as the foundation of a building. A good building needs a strong foundation. Similarly, your research needs to be supported by reviewing and explaining the existing knowledge in the field, describing how your research study will fit within or contribute to the existing literature (e.g., it could challenge or test an existing theory or address a knowledge gap), and informing the reader how your study design aligns with your thesis question or hypothesis.
Important components of the framework are a literature review of recent studies associated with your thesis topic as well as theories/models used in your field of research. The literature review acts as a filtering tool to select appropriate thesis questions and guide data collection, analysis, and interpretation of your findings. Think broadly! Apart from reviewing relevant published papers in your field of research, also explore theories that you have come across in your undergraduate courses, other published thesis studies, encyclopedias, and handbooks.
There are two types of research frameworks: theoretical and conceptual .
What is a conceptual framework?
A conceptual framework is a written or visual representation that explains the study variables and their relationships with each other. The starting point is a literature review of existing studies and theories about your topic.
Steps to develop a conceptual framework
- Clarify your study topic by identifying and defining key concepts in your thesis problem statement and thesis question. Essentially, your thesis should address a knowledge gap.
- Perform a literature review to provide a background to interpret and explain the study findings. Also, draw on empirical knowledge that you have gained from personal experience.
- Identify crucial variables from the literature review and your empirical knowledge, classify them as dependent or independent variables, and define them.
- Brainstorm all the possible factors that could affect each dependent variable.
- Propose relationships among the variables and determine any associations that exist between all variables.
- Use a flowchart or tree diagram to present your conceptual framework.
Types of variables
When developing a conceptual framework, you will need to identify the following:
- Independent variables
- Dependent variables
- Moderating variables
- Mediating variables
- Control variables
First, identify the independent (cause) and dependent (effect) variables in your study. Then, identify variables that influence this relationship, such as moderating variables, mediating variables, and control variables. A moderating variable changes the relationship between independent and dependent variables when its value increases or decreases. A mediating variable links independent and dependent variables to better explain the relationship between them. A control variable could potentially impact the cause-and-effect relationship but is kept constant throughout the study so that its effects on the findings/outcomes can be ruled out.
Example of a conceptual framework
You want to investigate the hours spent exercising (cause) on childhood obesity (effect).

Now, you need to consider moderating variables that affect the cause-and-effect relationship. In our example, the amount of junk food eaten would affect the level of obesity.
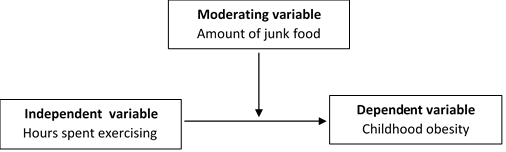
Next, you need to consider mediating variables. In our example, the maximum heart rate during exercise would affect the child’s weight.
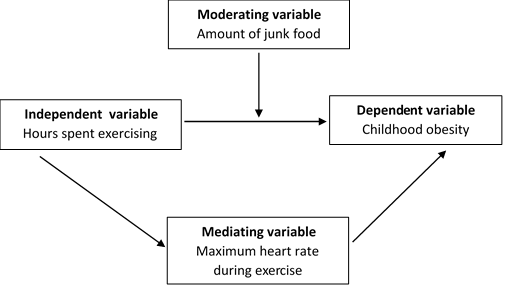
Finally, you need to consider control variables. In this example, because we do not want to investigate the role of age in obesity, we can use this as a control variable. Thus, the study subjects would be children of a specific age (e.g., aged 6–10 years).
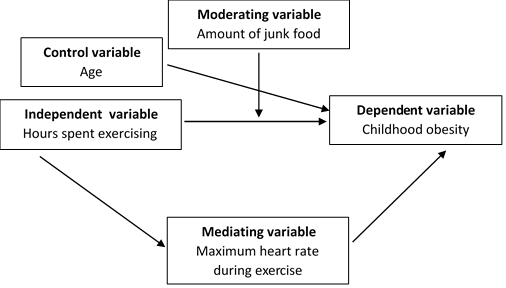
What is a theoretical framework?
A theoretical framework provides a general framework for data analysis. It defines the concepts used and explains existing theories and models in your field of research.
A theoretical framework provides a general framework for data analysis. It defines the concepts used and explains existing theories and models in your field of research. It also explains any assumptions that were used to inform your approach and your choice of specific rationales. Theoretical frameworks are often used in the fields of social sciences.
Purpose of a theoretical framework
- Test and challenge existing theories
- Establish orderly connections between observations and facts
- Predict and control situations
- Develop hypotheses
Steps to develop a theoretical framework
- Identify and define key concepts in your thesis problem statement and thesis question.
- Explain and evaluate existing theories by writing a literature review that describes the concepts, models, and theories that support your study.
- Choose the theory that best explains the relationships between the key variables in your study.
- Explain how your research study fills a knowledge gap or fits into existing studies (e.g., testing if an established theory applies to your thesis context).
- Discuss the relevance of any theoretical assumptions and limitations.
A thesis topic can be approached from a variety of angles, depending on the theories used.
- In psychology, a behavioral approach would use different methods and assumptions compared with a cognitive approach when treating anxiety.
- In literature, a book could be analyzed using different literary theories, such as Marxism or poststructuralism.
Structuring a theoretical framework
The structure of a theoretical framework is fluid, and there are no specific rules that need to be followed, as long as it is clearly and logically presented.
The theoretical framework is a natural extension of your literature review. The literature review should identify gaps in the field of your research, and reviewing existing theories will help to determine how these can be addressed. The structure of a theoretical framework is fluid, and there are no specific rules that need to be followed, as long as it is clearly and logically presented. The theoretical framework is sometimes integrated into the literature review chapter of a thesis, but it can also be included as a separate chapter, depending on the complexity of the theories.
Example of a theoretical framework
The sales staff at Company X are unmotivated and struggling to meet their monthly targets. Some members of the management team believe that this could be achieved by implementing a comprehensive product-training program, but others believe that introducing a sales commission structure will help.
Company X is not achieving their monthly sales targets
To increase monthly sales.
Research question:
How can Company X motivate their sales team to achieve its monthly sales targets?
Sub-questions:
- Why do the sales staff feel unmotivated?
- What is the relationship between motivation and monetary rewards?
- Do the sales staff feel that they have sufficient product knowledge?
Theoretical framework:
A literature search will need to be performed to understand the background of the many different theories of motivation in psychology. For example, Maslow’s Hierarchy of Needs (basic human needs—physiological, safety, love/belonging, esteem, and self-actualization—have to be fulfilled before one can live up to their true potential), Vroom’s Theory of Expectancy (people decide upon their actions based on the outcomes they expect), and Locke’s Goal-Setting Theory (goals are a key driver of one’s behavior). These theories would need to be investigated to determine which would be the best approach to increase the motivation of the sales staff in Company X so that the monthly sales targets are met.
A robust conceptual or theoretical framework is crucial when writing a thesis/dissertation. It defines your research gap, identifies your approach, and guides the interpretation of your results.
A thesis is the most important document you will write during your academic studies. For professional thesis editing and thesis proofreading services, check out Enago's Thesis Editing service s for more information.
Editor’s pick
Get free updates.
Subscribe to our newsletter for regular insights from the research and publishing industry!
What type of framework is used in the Humanities and Social Sciences (HSS) domain? +
Theoretical frameworks are typically used in the HSS domain, while conceptual frameworks are used in the Sciences domain.
What is the difference between mediating versus moderating variables? +
The difference between mediators and moderators can be confusing. A moderating variable is unaffected by the independent variable and can increase or decrease the strength of the relationship between the independent and dependent variables. A mediating variable is affected by the independent variable and can explain the relationship between the independent and dependent variables. T he statistical correlation between the independent and dependent variables is higher when the mediating variable is excluded.
What software should I use to present my conceptual framework? +
The software program Creately provides some useful templates that can help you get started. Other recommended programs are SmartDraw , Inkscape , and diagrams.net .
- How It Works
- PhD thesis writing
- Master thesis writing
- Bachelor thesis writing
- Dissertation writing service
- Dissertation abstract writing
- Thesis proposal writing
- Thesis editing service
- Thesis proofreading service
- Thesis formatting service
- Coursework writing service
- Research paper writing service
- Architecture thesis writing
- Computer science thesis writing
- Engineering thesis writing
- History thesis writing
- MBA thesis writing
- Nursing dissertation writing
- Psychology dissertation writing
- Sociology thesis writing
- Statistics dissertation writing
- Buy dissertation online
- Write my dissertation
- Cheap thesis
- Cheap dissertation
- Custom dissertation
- Dissertation help
- Pay for thesis
- Pay for dissertation
- Senior thesis
- Write my thesis
Theoretical Framework: Research Writing Guide
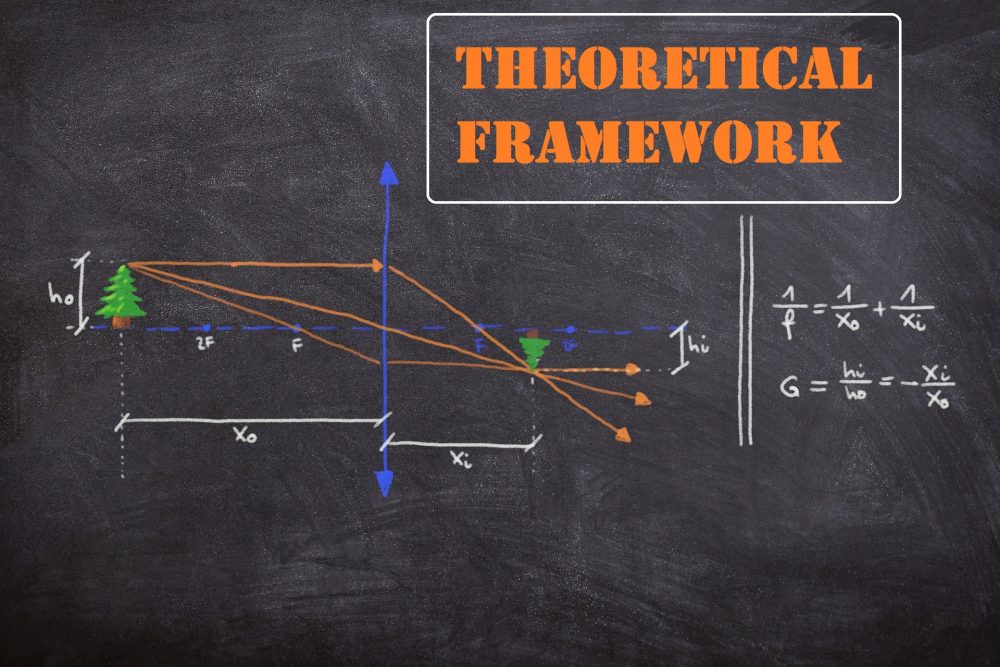
In a thesis or dissertation, a theoretical framework is a section where the writer evaluates or discusses the most relevant theories to their study.
The purpose of this section is to:
- Define the key concepts
- Combine and evaluate relevant models and theories
- Explain expectations and assumptions that guide the project
The proper presentation of this information frames the research while justifying the approach taken by the writer. This section does this by showing the established ideas on which you ground your work.
Essentially, this section of a dissertation a foundation that supports the analysis that follows. It also allows the author to convincingly interpret their results and state or explain their relevance in a larger context.
When properly written, this section works like the software or buildings that provide critical support to the other aspects of the study. Writing a strong framework with a strong theoretical basis enhances investigations that lead to the achievement of specific study goals.
A well-written framework reduces a dreadful research topic into two basic concepts. These are:
- The study problem
- The rationale behind its investigation
When writing the framework section, focus on creating a piece that connects you with the existing knowledge via the guidance of relevant theories. Also, provide the basis of your hypothesis and your chosen research methods. A professional dissertation writer will help, if you’re in trouble.
What Is a Theoretical Framework?
- The Length of a Theoretical Framework
Theoretical or Conceptual Framework?
Types of theoretical framework, how to write a theoretical framework.
- Summary of a Theoretical Framework Sample
Just like the name suggests, this part of a dissertation or thesis is about theories. Researchers develop theories to draw connections, explain phenomena, and make predictions.
The simplest theoretical framework definition describes it as a collection of theories or interrelated concepts. It comprises concepts and their definitions, as well as, a reference to existing theory and scholarly literature that will be used in a particular study. Your content in this section of a thesis or dissertation must show your understanding of concepts and theories relevant to your research topic. It must also relate them to the considered broader field of knowledge.
- Some students confuse conceptual vs. theoretical framework. In some cases, learners use these terms interchangeably. But though these terms help readers understand the research problem while guiding the collection, as well as, analysis of information, they are different.
- According to the above definition of theoretical framework, it comprises concepts or theories relevant to a study. It highlights how the author will understand and investigate the research problem.
- On the other hand, a conceptual framework can include several formal theories partly or entirely, and other empirical findings and concepts from the field’s literature. The main difference between theoretical and conceptual framework is that the latter demonstrates the relationships among ideas and their relationship with the study.
- A conceptual framework is commonly used in qualitative research. Although some researchers use a theoretical framework in qualitative research, it is common in quantitative research. A conceptual framework is commonly used in qualitative research, especially in behavioral and social science studies.
The Length of a Theoretical Framework
The complexity and length of this section depend on the topic and study field. Some fields and topics have an obvious and well-established theoretical basis. Others need a more detailed justification and explanation.
Maybe you already know that you will apply a specific theory or several theories to your specific context. For instance, you may intend to use the social impact theory when conducting your market research. In that case, the main task is to discuss the main aspects of this theory and then convince the readers that it offers a solid basis that will enable you to answer the research question. It’s also crucial that you evaluate more theories, as long as, they are relevant to your study. Also, tell your readers why you’ve chosen that specific approach.
In some cases, authors draw on different theories and then combine ideas. This approach can lead to strong research. However, it may require more work because you have to implement the theories in your work.
Most theoretical framework examples range between three and five pages. However, no rules govern the length of this section of a dissertation. Nevertheless, try to keep yours within the range of 3-5 pages. This length is adequate for providing all the relevant information your reader wants to know about your chosen theories and assumptions.
Perhaps, you are torn between a theoretical and conceptual framework. Well, the best approach for deciding what to use in your paper is determining the kind of study you want to conduct. If you must use a theoretical framework in qualitative research, determine the theories you intend to use.
That’s because most types of theoretical framework in qualitative research are found in studies based on existing theories. For instance, you can use this framework when your study is based on motivation theory.
On the other hand, a conceptual framework is ideal for something you will develop based on a theory. Thus, you can use some or all concepts of this theory. Thus, you develop a conceptual framework to solve a problem for which you’re doing the study to find a solution.
At this point, you’re no longer asking, ‘what is theoretical framework?’ But, you most likely want to know the types of frameworks that you can consider for your research. Well, this framework provides a lens or a perspective via which you will examine your topic. And this perspective can be from any study field depending on your academic paper.
For instance, a nursing student can use a theoretical framework in nursing research as long as it defines the concepts while explaining the phenomena in question. However, learners can consider other categories and types of theoretical framework in research.
They include:
- Dynamic and sustainability framework
- Implementation results framework
- Theoretical domains validation framework
- Consolidated implementation research and theoretical domains framework
- Active research implementation framework
- Evaluation framework
The internet has many resources with examples of theoretical framework in qualitative research and quantitative research. Check them out before you use any framework in your research to know what it entails.
This article has already answered the question, ‘what is theoretical framework in research?’ It has also highlighted the types of this framework. But, how do you complete your theoretical framework research work?
Here is a guide for creating this framework for your research:
- Identify the main concepts : Start by picking the main terms of your research problem or research questions. Some concepts can have several definitions. Your framework should define what each concept means clearly. For instance, if concepts like “customer satisfaction” and “customer loyalty” are central to your study, define them and discuss theories that explain their relationship.
- Explain and evaluate relevant theories : Engage in an extensive literature review to find out the definition of the connections between theories and concepts by other researchers. As you compose your framework, focus on critically evaluating different approaches and comparing them. Establish the most appropriate definitions for your research after discussing different theories and models. Mention all important concepts that are connected to the theories that you discuss in your framework. Explain why you choose a well-established theory for your study and what makes it the most suitable for that purpose. If unsure about the best way to do this, check a theoretical framework example online first.
- Demonstrate how your study fits in : In addition to discussing theories by other people, your framework should demonstrate how your project will implement these ideas. That means you have to test whether your chosen theory holds in your specific context. Also, use this theory to interpret the findings of your study. It’s also crucial to challenge or critique the theory. What’s more, combine various theories in a unique or new way. If possible and relevant, use your framework to come up with your research hypothesis.
- Structure your framework : When writing a dissertation or a thesis paper, you can integrate your framework in the literature review chapter. However, you can have it as a separate section or chapter of your paper. If you will be dealing with several complex theories in your paper, have a separate chapter or section for the framework. Nevertheless, you don’t have to follow specific, fixed rules when it comes to structuring the research theoretical framework section. But, your framework should have a logical, clear structure. For instance, you can draw on your study problems or questions and then structure every section around a major concept or question.
These tips should guide you in writing a framework with the theories or concepts you intend to use in your thesis or dissertation. However, you can apply them differently depending on the nature of your study. For instance, a business paper framework may not be the same as a nursing theoretical framework because these are different study fields. However, the concept of creating this framework is the same.
Summary of a Theoretical Framework Sample
For some researchers, an ideal approach is to define theoretical framework. However, some researchers assume the reader already knows what this framework is all about. As such, they go straight to the details. Below is a summary of a theoretical framework in research example.
Company Y wants to resolve the problem of having many customers buy its products online without returning for subsequent purchases. As such, the company management is looking for ways to enhance customer loyalty, hoping that better customer satisfaction will lead to the achievement of this goal.
In your research, you have developed a problem statement, research question, and research question as follows:
- Research problem : Most online buyers do not come back for subsequent purchases.
- Objective : To boost customer loyalty hoping to increase revenue through online sales
- Research questions : How can company Y improve the satisfaction of online customers to enhance customer loyalty?
Your framework should focus on answering these questions:
- Is there a relationship between customer satisfaction and customer loyalty?
- How loyal and satisfied are the online customers of company Y currently?
- What are some of the factors affecting the loyalty and satisfaction of the online customers of company Y?
Customer satisfaction and customer loyalty are major concepts that play a role in such a research paper. Therefore, they should be investigated and measured using theories or concepts that should be featured in the framework.
The information contained in this framework could be different from that of a theoretical framework nursing educators expect. That’s because this framework is meant for a business-oriented research paper. Nevertheless, the approach for writing both frameworks is the same.
The framework section of a thesis or dissertation paper clarifies implicit theories or concepts in a clearly defined manner. It also shows how they connect to the current research and why they are suitable for it. Your academic supervisor will most likely check this section first. Therefore, understanding its purpose and how to write it properly is very important.
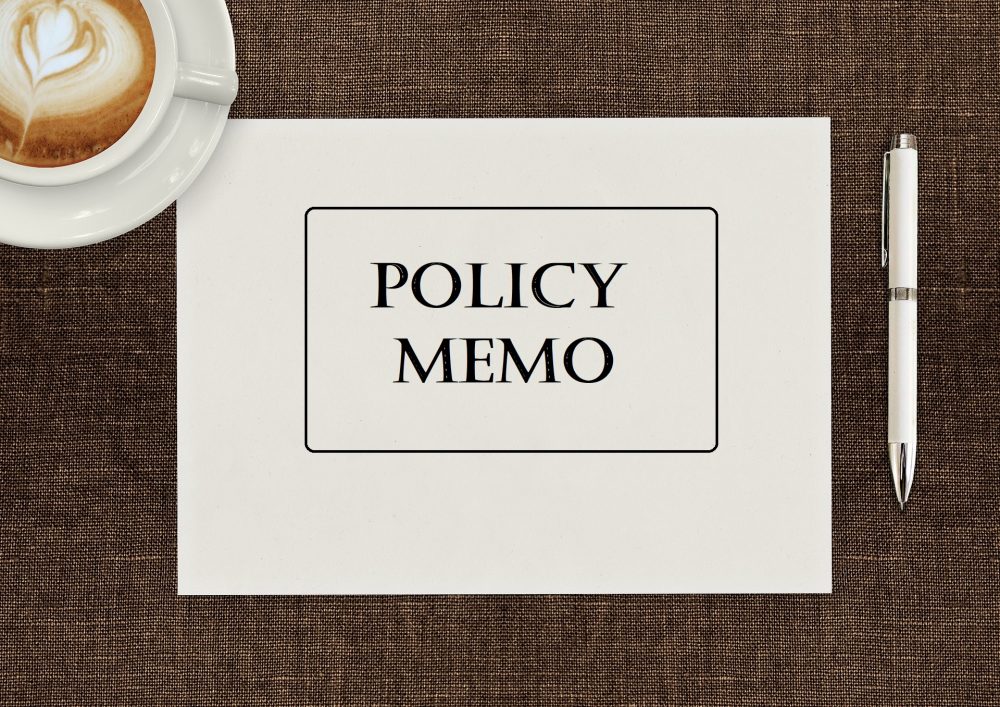
Leave a Reply Cancel reply
Your email address will not be published. Required fields are marked *
Comment * Error message
Name * Error message
Email * Error message
Save my name, email, and website in this browser for the next time I comment.
As Putin continues killing civilians, bombing kindergartens, and threatening WWIII, Ukraine fights for the world's peaceful future.
Ukraine Live Updates
- Privacy Policy
Buy Me a Coffee

Home » Theoretical Framework – Types, Examples and Writing Guide
Theoretical Framework – Types, Examples and Writing Guide
Table of Contents

Theoretical Framework
Definition:
Theoretical framework refers to a set of concepts, theories, ideas , and assumptions that serve as a foundation for understanding a particular phenomenon or problem. It provides a conceptual framework that helps researchers to design and conduct their research, as well as to analyze and interpret their findings.
In research, a theoretical framework explains the relationship between various variables, identifies gaps in existing knowledge, and guides the development of research questions, hypotheses, and methodologies. It also helps to contextualize the research within a broader theoretical perspective, and can be used to guide the interpretation of results and the formulation of recommendations.
Types of Theoretical Framework
Types of Types of Theoretical Framework are as follows:
Conceptual Framework
This type of framework defines the key concepts and relationships between them. It helps to provide a theoretical foundation for a study or research project .
Deductive Framework
This type of framework starts with a general theory or hypothesis and then uses data to test and refine it. It is often used in quantitative research .
Inductive Framework
This type of framework starts with data and then develops a theory or hypothesis based on the patterns and themes that emerge from the data. It is often used in qualitative research .
Empirical Framework
This type of framework focuses on the collection and analysis of empirical data, such as surveys or experiments. It is often used in scientific research .
Normative Framework
This type of framework defines a set of norms or values that guide behavior or decision-making. It is often used in ethics and social sciences.
Explanatory Framework
This type of framework seeks to explain the underlying mechanisms or causes of a particular phenomenon or behavior. It is often used in psychology and social sciences.
Components of Theoretical Framework
The components of a theoretical framework include:
- Concepts : The basic building blocks of a theoretical framework. Concepts are abstract ideas or generalizations that represent objects, events, or phenomena.
- Variables : These are measurable and observable aspects of a concept. In a research context, variables can be manipulated or measured to test hypotheses.
- Assumptions : These are beliefs or statements that are taken for granted and are not tested in a study. They provide a starting point for developing hypotheses.
- Propositions : These are statements that explain the relationships between concepts and variables in a theoretical framework.
- Hypotheses : These are testable predictions that are derived from the theoretical framework. Hypotheses are used to guide data collection and analysis.
- Constructs : These are abstract concepts that cannot be directly measured but are inferred from observable variables. Constructs provide a way to understand complex phenomena.
- Models : These are simplified representations of reality that are used to explain, predict, or control a phenomenon.
How to Write Theoretical Framework
A theoretical framework is an essential part of any research study or paper, as it helps to provide a theoretical basis for the research and guide the analysis and interpretation of the data. Here are some steps to help you write a theoretical framework:
- Identify the key concepts and variables : Start by identifying the main concepts and variables that your research is exploring. These could include things like motivation, behavior, attitudes, or any other relevant concepts.
- Review relevant literature: Conduct a thorough review of the existing literature in your field to identify key theories and ideas that relate to your research. This will help you to understand the existing knowledge and theories that are relevant to your research and provide a basis for your theoretical framework.
- Develop a conceptual framework : Based on your literature review, develop a conceptual framework that outlines the key concepts and their relationships. This framework should provide a clear and concise overview of the theoretical perspective that underpins your research.
- Identify hypotheses and research questions: Based on your conceptual framework, identify the hypotheses and research questions that you want to test or explore in your research.
- Test your theoretical framework: Once you have developed your theoretical framework, test it by applying it to your research data. This will help you to identify any gaps or weaknesses in your framework and refine it as necessary.
- Write up your theoretical framework: Finally, write up your theoretical framework in a clear and concise manner, using appropriate terminology and referencing the relevant literature to support your arguments.
Theoretical Framework Examples
Here are some examples of theoretical frameworks:
- Social Learning Theory : This framework, developed by Albert Bandura, suggests that people learn from their environment, including the behaviors of others, and that behavior is influenced by both external and internal factors.
- Maslow’s Hierarchy of Needs : Abraham Maslow proposed that human needs are arranged in a hierarchy, with basic physiological needs at the bottom, followed by safety, love and belonging, esteem, and self-actualization at the top. This framework has been used in various fields, including psychology and education.
- Ecological Systems Theory : This framework, developed by Urie Bronfenbrenner, suggests that a person’s development is influenced by the interaction between the individual and the various environments in which they live, such as family, school, and community.
- Feminist Theory: This framework examines how gender and power intersect to influence social, cultural, and political issues. It emphasizes the importance of understanding and challenging systems of oppression.
- Cognitive Behavioral Theory: This framework suggests that our thoughts, beliefs, and attitudes influence our behavior, and that changing our thought patterns can lead to changes in behavior and emotional responses.
- Attachment Theory: This framework examines the ways in which early relationships with caregivers shape our later relationships and attachment styles.
- Critical Race Theory : This framework examines how race intersects with other forms of social stratification and oppression to perpetuate inequality and discrimination.
When to Have A Theoretical Framework
Following are some situations When to Have A Theoretical Framework:
- A theoretical framework should be developed when conducting research in any discipline, as it provides a foundation for understanding the research problem and guiding the research process.
- A theoretical framework is essential when conducting research on complex phenomena, as it helps to organize and structure the research questions, hypotheses, and findings.
- A theoretical framework should be developed when the research problem requires a deeper understanding of the underlying concepts and principles that govern the phenomenon being studied.
- A theoretical framework is particularly important when conducting research in social sciences, as it helps to explain the relationships between variables and provides a framework for testing hypotheses.
- A theoretical framework should be developed when conducting research in applied fields, such as engineering or medicine, as it helps to provide a theoretical basis for the development of new technologies or treatments.
- A theoretical framework should be developed when conducting research that seeks to address a specific gap in knowledge, as it helps to define the problem and identify potential solutions.
- A theoretical framework is also important when conducting research that involves the analysis of existing theories or concepts, as it helps to provide a framework for comparing and contrasting different theories and concepts.
- A theoretical framework should be developed when conducting research that seeks to make predictions or develop generalizations about a particular phenomenon, as it helps to provide a basis for evaluating the accuracy of these predictions or generalizations.
- Finally, a theoretical framework should be developed when conducting research that seeks to make a contribution to the field, as it helps to situate the research within the broader context of the discipline and identify its significance.
Purpose of Theoretical Framework
The purposes of a theoretical framework include:
- Providing a conceptual framework for the study: A theoretical framework helps researchers to define and clarify the concepts and variables of interest in their research. It enables researchers to develop a clear and concise definition of the problem, which in turn helps to guide the research process.
- Guiding the research design: A theoretical framework can guide the selection of research methods, data collection techniques, and data analysis procedures. By outlining the key concepts and assumptions underlying the research questions, the theoretical framework can help researchers to identify the most appropriate research design for their study.
- Supporting the interpretation of research findings: A theoretical framework provides a framework for interpreting the research findings by helping researchers to make connections between their findings and existing theory. It enables researchers to identify the implications of their findings for theory development and to assess the generalizability of their findings.
- Enhancing the credibility of the research: A well-developed theoretical framework can enhance the credibility of the research by providing a strong theoretical foundation for the study. It demonstrates that the research is based on a solid understanding of the relevant theory and that the research questions are grounded in a clear conceptual framework.
- Facilitating communication and collaboration: A theoretical framework provides a common language and conceptual framework for researchers, enabling them to communicate and collaborate more effectively. It helps to ensure that everyone involved in the research is working towards the same goals and is using the same concepts and definitions.
Characteristics of Theoretical Framework
Some of the characteristics of a theoretical framework include:
- Conceptual clarity: The concepts used in the theoretical framework should be clearly defined and understood by all stakeholders.
- Logical coherence : The framework should be internally consistent, with each concept and assumption logically connected to the others.
- Empirical relevance: The framework should be based on empirical evidence and research findings.
- Parsimony : The framework should be as simple as possible, without sacrificing its ability to explain the phenomenon in question.
- Flexibility : The framework should be adaptable to new findings and insights.
- Testability : The framework should be testable through research, with clear hypotheses that can be falsified or supported by data.
- Applicability : The framework should be useful for practical applications, such as designing interventions or policies.
Advantages of Theoretical Framework
Here are some of the advantages of having a theoretical framework:
- Provides a clear direction : A theoretical framework helps researchers to identify the key concepts and variables they need to study and the relationships between them. This provides a clear direction for the research and helps researchers to focus their efforts and resources.
- Increases the validity of the research: A theoretical framework helps to ensure that the research is based on sound theoretical principles and concepts. This increases the validity of the research by ensuring that it is grounded in established knowledge and is not based on arbitrary assumptions.
- Enables comparisons between studies : A theoretical framework provides a common language and set of concepts that researchers can use to compare and contrast their findings. This helps to build a cumulative body of knowledge and allows researchers to identify patterns and trends across different studies.
- Helps to generate hypotheses: A theoretical framework provides a basis for generating hypotheses about the relationships between different concepts and variables. This can help to guide the research process and identify areas that require further investigation.
- Facilitates communication: A theoretical framework provides a common language and set of concepts that researchers can use to communicate their findings to other researchers and to the wider community. This makes it easier for others to understand the research and its implications.
About the author
Muhammad Hassan
Researcher, Academic Writer, Web developer
You may also like

How to Cite Research Paper – All Formats and...

Data Collection – Methods Types and Examples

Delimitations in Research – Types, Examples and...

Research Paper Format – Types, Examples and...

Research Process – Steps, Examples and Tips

Research Design – Types, Methods and Examples
- Staff Directory
- Library Policies
- Hege Research Award
- Quaker Archives
- Art Gallery
- Student Support
- Teaching & Learning
- Reserving spaces
- Technology Lending
- Interlibrary Loan
- Course Reserves
- Copyright & Fair Use
- Poster Printing
- Virtual Reference
- Research Guides
- Off-campus access
- Digital Scholarship
- Guilford Sources
- Open Educational Resources
- Quaker Collections
- Digital Collections
- College Archives
- Underground Railroad
- Universities Studying Slavery
- Images & Exhibitions
Service Alert

Hege Library & Learning Technologies
Guide for Thesis Research
- Introduction to the Thesis Process
- Project Planning
- Literature Review
- Theoretical Frameworks
- Research Methodology
- GC Honors Program Theses
- Thesis Submission Instructions This link opens in a new window
- Accessing Guilford Theses from 1898 to 2020 This link opens in a new window
Some Articles About Theory
The following are articles that may help you understand the importance of theory as a fundamental aspect of academic research.
- It's Just a Theory
- Literature Reviews, Conceptual Frameworks, and Theoretical Frameworks: Terms, Functions, and Distinctions
- Use of Theoretical Frameworks in Research
Why is theory important?

Theories reflect previous study and analysis that has been conducted in your field. They propose explanations for phenomena that occur in an area of study. Over time, theories are reexamined, refined, and sometimes discarded in favor of new ones, always with the purpose of providing ever more accurate explanations for the dynamics that operate in our world.
The following quote, taken from John Kuada's book Research Methodology: A Project Guide for University Students , helps to explain the importance of theory when developing a research project:
“Theory provides the language, the concepts, and assumptions that help researchers to make sense of the phenomenon that they seek to investigate. It enables researchers to connect the issues they are investigating to the existing body of knowledge in the area” (Kuada, 2012, p. 64).
A theory can help researchers make predictions about the phenomena they are setting out to study. They can be informative in terms of determining what variables should be observed, as well as how data should be collected, analyzed, and interpreted on the way to presenting and justifying conclusions.
As a researcher working on a project, it is essential that you be aware of theories that have gained prominence in your field. Think of scholarship as an ongoing conversation. As people publish ideas and develop theories, they help shape that conversation. When you do research and present your findings and ideas, you are joining in on those discussions. You become a contributor. Therefore, it is good to have a sense of what has been said before.
Identify major theories in your field. Be conscious of the fundamental concepts that have guided scholars in your area, and be aware of emerging perspectives and trends. Try to identify a theoretical base from which you can develop your arguments. This will greatly strengthen your positions when the time comes to present your thesis.
Resources About Theory and Theoretical Frameworks
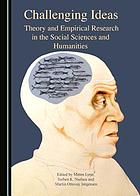
- << Previous: Literature Review
- Next: Research Methodology >>
- Last Updated: Jan 23, 2024 4:31 PM
- URL: https://library.guilford.edu/thesis-guide
- Translators
- Graphic Designers
- Editing Services
- Academic Editing Services
- Admissions Editing Services
- Admissions Essay Editing Services
- AI Content Editing Services
- APA Style Editing Services
- Application Essay Editing Services
- Book Editing Services
- Business Editing Services
- Capstone Paper Editing Services
- Children's Book Editing Services
- College Application Editing Services
- College Essay Editing Services
- Copy Editing Services
- Developmental Editing Services
- Dissertation Editing Services
- eBook Editing Services
- English Editing Services
- Horror Story Editing Services
- Legal Editing Services
- Line Editing Services
- Manuscript Editing Services
- MLA Style Editing Services
- Novel Editing Services
- Paper Editing Services
- Personal Statement Editing Services
- Research Paper Editing Services
- Résumé Editing Services
- Scientific Editing Services
- Short Story Editing Services
- Statement of Purpose Editing Services
- Substantive Editing Services
- Thesis Editing Services
Proofreading
- Proofreading Services
- Admissions Essay Proofreading Services
- Children's Book Proofreading Services
- Legal Proofreading Services
- Novel Proofreading Services
- Personal Statement Proofreading Services
- Research Proposal Proofreading Services
- Statement of Purpose Proofreading Services
Translation
- Translation Services
Graphic Design
- Graphic Design Services
- Dungeons & Dragons Design Services
- Sticker Design Services
- Writing Services
Please enter the email address you used for your account. Your sign in information will be sent to your email address after it has been verified.
6 Steps to Mastering the Theoretical Framework of a Dissertation
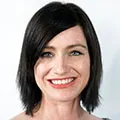
As the pivotal section of your dissertation, the theoretical framework will be the lens through which your readers should evaluate your research. It's also a necessary part of your writing and research processes from which every written section will be built.
In their journal article titled Understanding, selecting, and integrating a theoretical framework in dissertation research: Creating the blueprint for your "house" , authors Cynthia Grant and Azadeh Osanloo write:
The theoretical framework is one of the most important aspects in the research process, yet is often misunderstood by doctoral candidates as they prepare their dissertation research study. The importance of theory-driven thinking and acting is emphasized in relation to the selection of a topic, the development of research questions, the conceptualization of the literature review, the design approach, and the analysis plan for the dissertation study. Using a metaphor of the "blueprint" of a house, this article explains the application of a theoretical framework in a dissertation. Administrative Issues Journal
They continue in their paper to discuss how architects and contractors understand that prior to building a house, there must be a blueprint created. This blueprint will then serve as a guide for everyone involved in the construction of the home, including those building the foundation, installing the plumbing and electrical systems, etc. They then state, We believe the blueprint is an appropriate analogy of the theoretical framework of the dissertation.
As with drawing and creating any blueprint, it is often the most difficult part of the building process. Many potential conflicts must be considered and mitigated, and much thought must be put into how the foundation will support the rest of the home. Without proper consideration on the front end, the entire structure could be at risk.
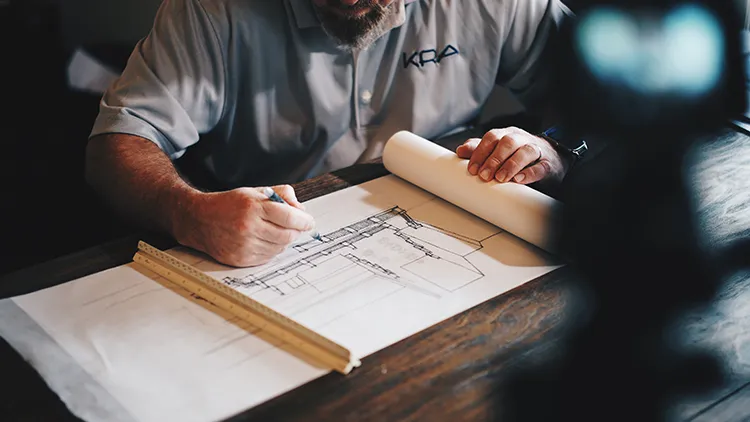
With this in mind, I'm going to discuss six steps to mastering the theoretical framework section—the "blueprint" for your dissertation. If you follow these steps and complete the checklist included, your blueprint is guaranteed to be a solid one.
Complete your review of literature first
In order to identify the scope of your theoretical framework, you'll need to address research that has already been completed by others, as well as gaps in the research. Understanding this, it's clear why you'll need to complete your review of literature before you can adequately write a theoretical framework for your dissertation or thesis.
Simply put, before conducting any extensive research on a topic or hypothesis, you need to understand where the gaps are and how they can be filled. As will be mentioned in a later step, it's important to note within your theoretical framework if you have closed any gaps in the literature through your research. It's also important to know the research that has laid a foundation for the current knowledge, including any theories, assumptions, or studies that have been done that you can draw on for your own. Without performing this necessary step, you're likely to produce research that is redundant, and therefore not likely to be published.
Understand the purpose of a theoretical framework
When you present a research problem, an important step in doing so is to provide context and background to that specific problem. This allows your reader to understand both the scope and the purpose of your research, while giving you a direction in your writing. Just as a blueprint for a home needs to provide needed context to all of the builders and professionals involved in the building process, so does the theoretical framework of your dissertation.
So, in building your theoretical framework, there are several details that need to be considered and explained, including:
- The definition of any concepts or theories you're building on or exploring (this is especially important if it is a theory that is taken from another discipline or is relatively new).
- The context in which this concept has been explored in the past.
- The important literature that has already been published on the concept or theory, including citations.
- The context in which you plan to explore the concept or theory. You can briefly mention your intended methods used, along with methods that have been used in the past—but keep in mind that there will be a separate section of your dissertation to present these in detail.
- Any gaps that you hope to fill in the research
- Any limitations encountered by past researchers and any that you encountered in your own exploration of the topic.
- Basically, your theoretical framework helps to give your reader a general understanding of the research problem, how it has already been explored, and where your research falls in the scope of it. In such, be sure to keep it written in present tense, since it is research that is presently being done. When you refer to past research by others, you can do so in past tense, but anything related to your own research should be written in the present.
Use your theoretical framework to justify your research
In your literature review, you'll focus on finding research that has been conducted that is pertinent to your own study. This could be literature that establishes theories connected with your research, or provides pertinent analytic models. You will then mention these theories or models in your own theoretical framework and justify why they are the basis of—or relevant to—your research.
Basically, think of your theoretical framework as a quick, powerful way to justify to your reader why this research is important. If you are expanding upon past research by other scholars, your theoretical framework should mention the foundation they've laid and why it is important to build on that, or how it needs to be applied to a more modern concept. If there are gaps in the research on certain topics or theories, and your research fills these gaps, mention that in your theoretical framework, as well. It is your opportunity to justify the work you've done in a scientific context—both to your dissertation committee and to any publications interested in publishing your work.
Keep it within three to five pages
While there are usually no hard and fast rules related to the length of your theoretical framework, it is most common to keep it within three to five pages. This length should be enough to provide all of the relevant information to your reader without going into depth about the theories or assumptions mentioned. If you find yourself needing many more pages to write your theoretical framework, it is likely that you've failed to provide a succinct explanation for a theory, concept, or past study. Remember—you'll have ample opportunity throughout the course of writing your dissertation to expand and expound on these concepts, past studies, methods, and hypotheses. Your theoretical framework is not the place for these details.
If you've written an abstract, consider your theoretical framework to be somewhat of an extended abstract. It should offer a glimpse of the entirety of your research without going into a detailed explanation of the methods or background of it. In many cases, chiseling the theoretical framework down to the three to five-page length is a process of determining whether detail is needed in establishing understanding for your reader.
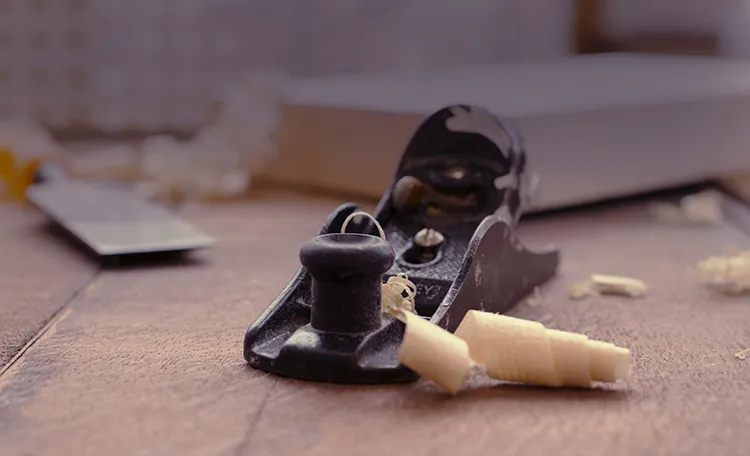
Use models and other graphics
Since your theoretical framework should clarify complicated theories or assumptions related to your research, it's often a good idea to include models and other helpful graphics to achieve this aim. If space is an issue, most formats allow you to include these illustrations or models in the appendix of your paper and refer to them within the main text.
Use a checklist after completing your first draft
You should consider the following questions as you draft your theoretical framework and check them off as a checklist after completing your first draft:
- Have the main theories and models related to your research been presented and briefly explained? In other words, does it offer an explicit statement of assumptions and/or theories that allows the reader to make a critical evaluation of them?
- Have you correctly cited the main scientific articles on the subject?
- Does it tell the reader about current knowledge related to the assumptions/theories and any gaps in that knowledge?
- Does it offer information related to notable connections between concepts?
- Does it include a relevant theory that forms the basis of your hypotheses and methods?
- Does it answer the question of "why" your research is valid and important? In other words, does it provide scientific justification for your research?
- If your research fills a gap in the literature, does your theoretical framework state this explicitly?
- Does it include the constructs and variables (both independent and dependent) that are relevant to your study?
- Does it state assumptions and propositions that are relevant to your research (along with the guiding theories related to these)?
- Does it "frame" your entire research, giving it direction and a backbone to support your hypotheses?
- Are your research questions answered?
- Is it logical?
- Is it free of grammar, punctuation, spelling, and syntax errors?
A final note
In conclusion, I would like to leave you with a quote from Grant and Osanloo:
The importance of utilizing a theoretical framework in a dissertation study cannot be stressed enough. The theoretical framework is the foundation from which all knowledge is constructed (metaphorically and literally) for a research study. It serves as the structure and support for the rationale for the study, the problem statement, the purpose, the significance, and the research questions. The theoretical framework provides a grounding base, or an anchor, for the literature review, and most importantly, the methods and analysis. Administrative Issues Journal
Related Posts
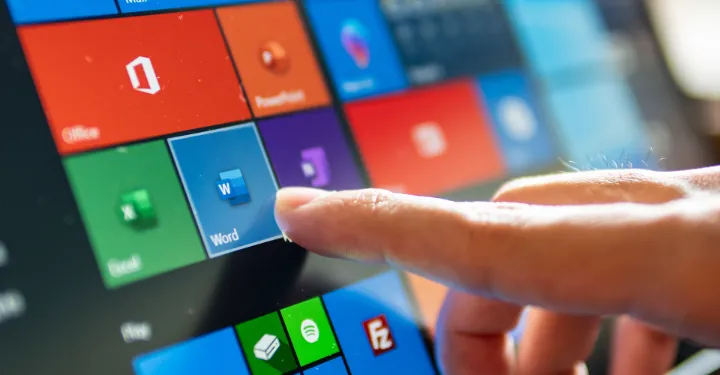
How To Use Microsoft Word References Tool For Smarter Academic Writing
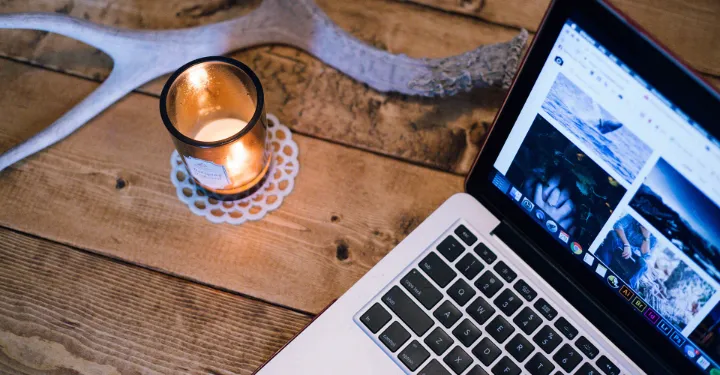
How to Cite a Website
- Academic Writing Advice
- All Blog Posts
- Writing Advice
- Admissions Writing Advice
- Book Writing Advice
- Short Story Advice
- Employment Writing Advice
- Business Writing Advice
- Web Content Advice
- Article Writing Advice
- Magazine Writing Advice
- Grammar Advice
- Dialect Advice
- Editing Advice
- Freelance Advice
- Legal Writing Advice
- Poetry Advice
- Graphic Design Advice
- Logo Design Advice
- Translation Advice
- Blog Reviews
- Short Story Award Winners
- Scholarship Winners
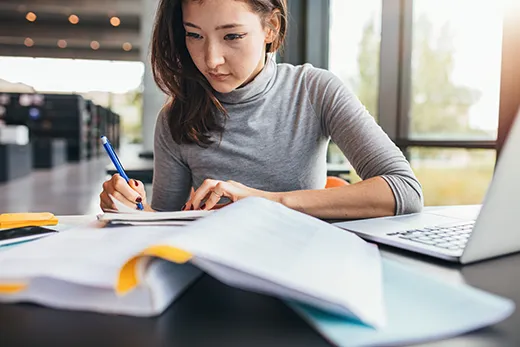
Need an academic editor before submitting your work?

Theoretical vs Conceptual Framework
What they are & how they’re different (with examples)
By: Derek Jansen (MBA) | Reviewed By: Eunice Rautenbach (DTech) | March 2023
If you’re new to academic research, sooner or later you’re bound to run into the terms theoretical framework and conceptual framework . These are closely related but distinctly different things (despite some people using them interchangeably) and it’s important to understand what each means. In this post, we’ll unpack both theoretical and conceptual frameworks in plain language along with practical examples , so that you can approach your research with confidence.
Overview: Theoretical vs Conceptual
What is a theoretical framework, example of a theoretical framework, what is a conceptual framework, example of a conceptual framework.
- Theoretical vs conceptual: which one should I use?
A theoretical framework (also sometimes referred to as a foundation of theory) is essentially a set of concepts, definitions, and propositions that together form a structured, comprehensive view of a specific phenomenon.
In other words, a theoretical framework is a collection of existing theories, models and frameworks that provides a foundation of core knowledge – a “lay of the land”, so to speak, from which you can build a research study. For this reason, it’s usually presented fairly early within the literature review section of a dissertation, thesis or research paper .
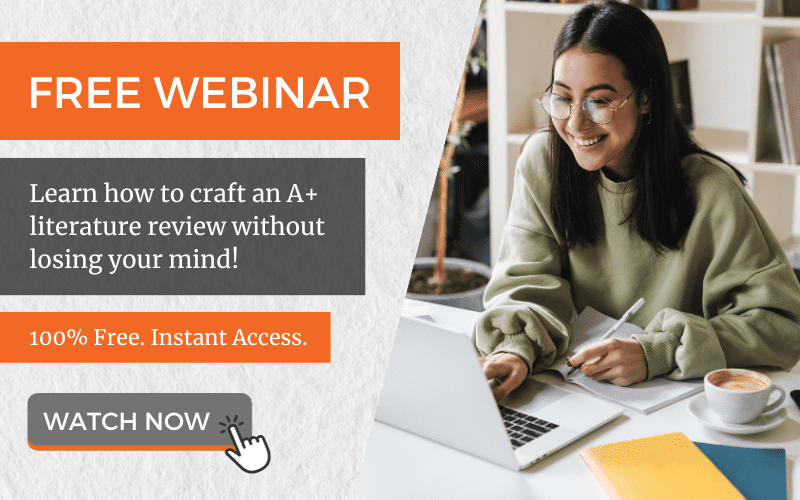
Let’s look at an example to make the theoretical framework a little more tangible.
If your research aims involve understanding what factors contributed toward people trusting investment brokers, you’d need to first lay down some theory so that it’s crystal clear what exactly you mean by this. For example, you would need to define what you mean by “trust”, as there are many potential definitions of this concept. The same would be true for any other constructs or variables of interest.
You’d also need to identify what existing theories have to say in relation to your research aim. In this case, you could discuss some of the key literature in relation to organisational trust. A quick search on Google Scholar using some well-considered keywords generally provides a good starting point.
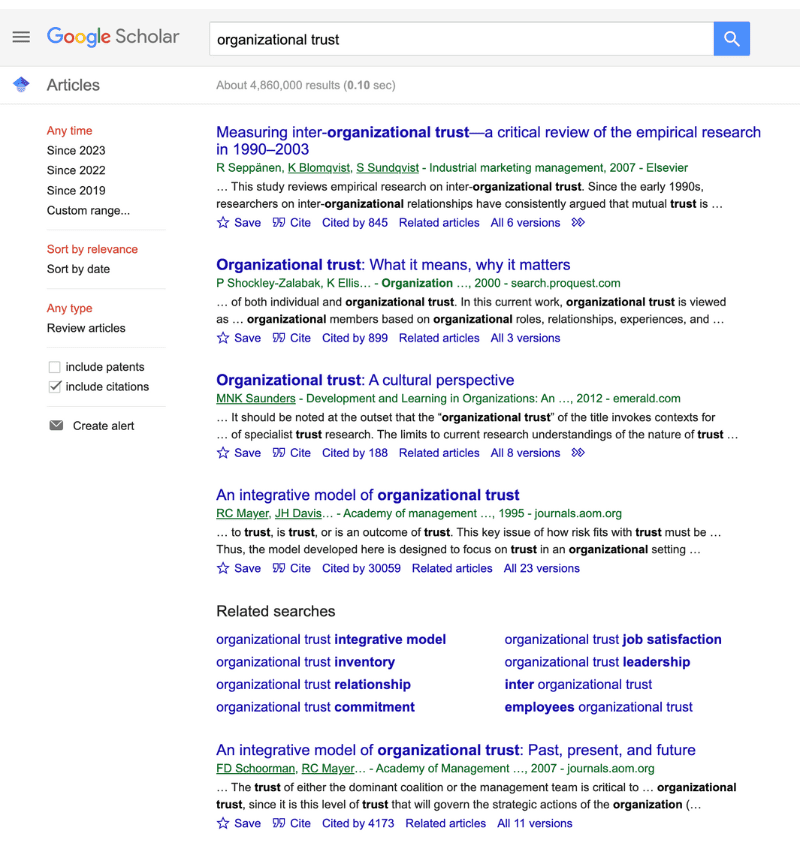
Typically, you’ll present your theoretical framework in written form , although sometimes it will make sense to utilise some visuals to show how different theories relate to each other. Your theoretical framework may revolve around just one major theory , or it could comprise a collection of different interrelated theories and models. In some cases, there will be a lot to cover and in some cases, not. Regardless of size, the theoretical framework is a critical ingredient in any study.
Simply put, the theoretical framework is the core foundation of theory that you’ll build your research upon. As we’ve mentioned many times on the blog, good research is developed by standing on the shoulders of giants . It’s extremely unlikely that your research topic will be completely novel and that there’ll be absolutely no existing theory that relates to it. If that’s the case, the most likely explanation is that you just haven’t reviewed enough literature yet! So, make sure that you take the time to review and digest the seminal sources.
Need a helping hand?
A conceptual framework is typically a visual representation (although it can also be written out) of the expected relationships and connections between various concepts, constructs or variables. In other words, a conceptual framework visualises how the researcher views and organises the various concepts and variables within their study. This is typically based on aspects drawn from the theoretical framework, so there is a relationship between the two.
Quite commonly, conceptual frameworks are used to visualise the potential causal relationships and pathways that the researcher expects to find, based on their understanding of both the theoretical literature and the existing empirical research . Therefore, the conceptual framework is often used to develop research questions and hypotheses .
Let’s look at an example of a conceptual framework to make it a little more tangible. You’ll notice that in this specific conceptual framework, the hypotheses are integrated into the visual, helping to connect the rest of the document to the framework.
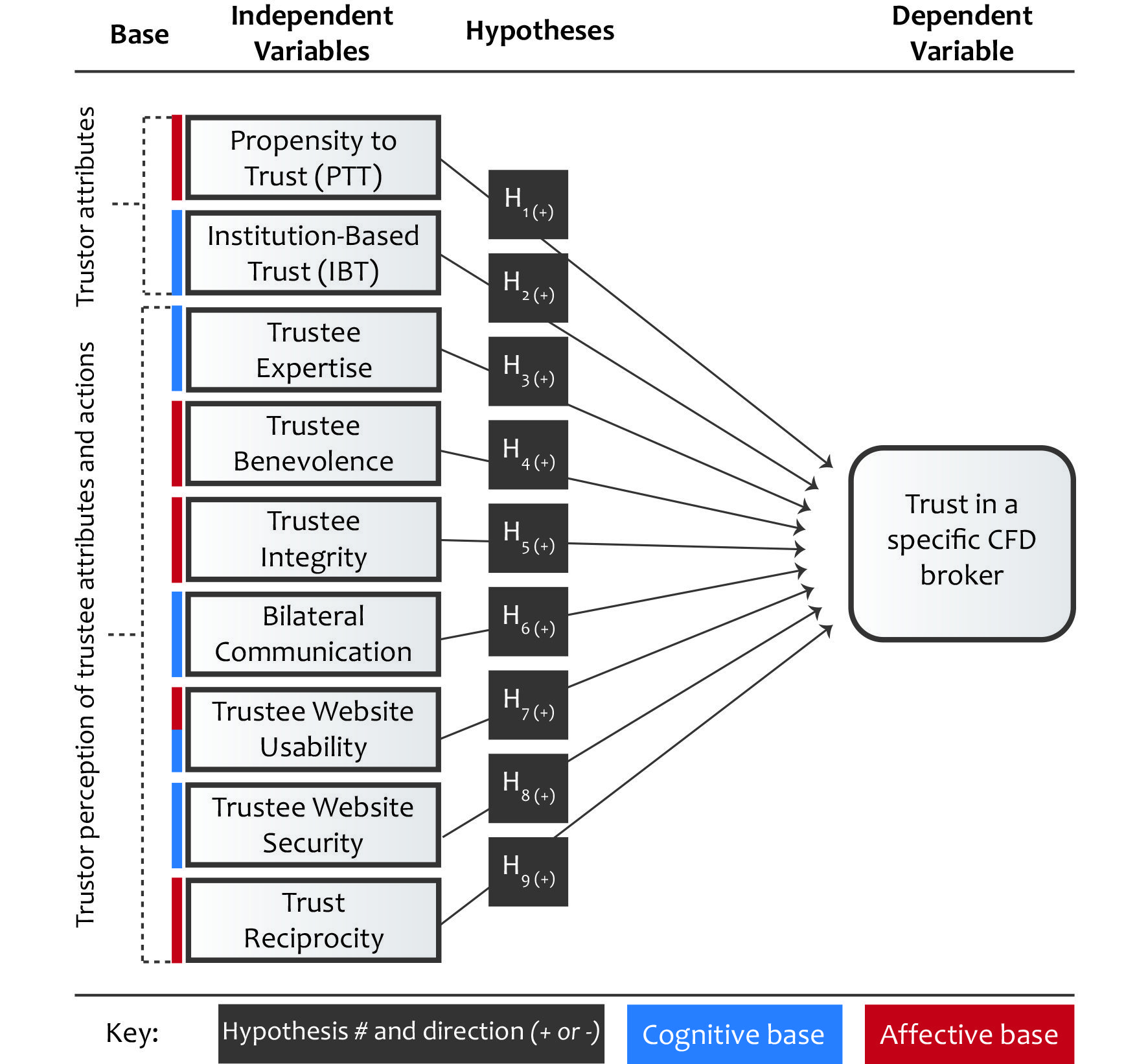
As you can see, conceptual frameworks often make use of different shapes , lines and arrows to visualise the connections and relationships between different components and/or variables. Ultimately, the conceptual framework provides an opportunity for you to make explicit your understanding of how everything is connected . So, be sure to make use of all the visual aids you can – clean design, well-considered colours and concise text are your friends.
Theoretical framework vs conceptual framework
As you can see, the theoretical framework and the conceptual framework are closely related concepts, but they differ in terms of focus and purpose. The theoretical framework is used to lay down a foundation of theory on which your study will be built, whereas the conceptual framework visualises what you anticipate the relationships between concepts, constructs and variables may be, based on your understanding of the existing literature and the specific context and focus of your research. In other words, they’re different tools for different jobs , but they’re neighbours in the toolbox.
Naturally, the theoretical framework and the conceptual framework are not mutually exclusive . In fact, it’s quite likely that you’ll include both in your dissertation or thesis, especially if your research aims involve investigating relationships between variables. Of course, every research project is different and universities differ in terms of their expectations for dissertations and theses, so it’s always a good idea to have a look at past projects to get a feel for what the norms and expectations are at your specific institution.
Want to learn more about research terminology, methods and techniques? Be sure to check out the rest of the Grad Coach blog . Alternatively, if you’re looking for hands-on help, have a look at our private coaching service , where we hold your hand through the research process, step by step.
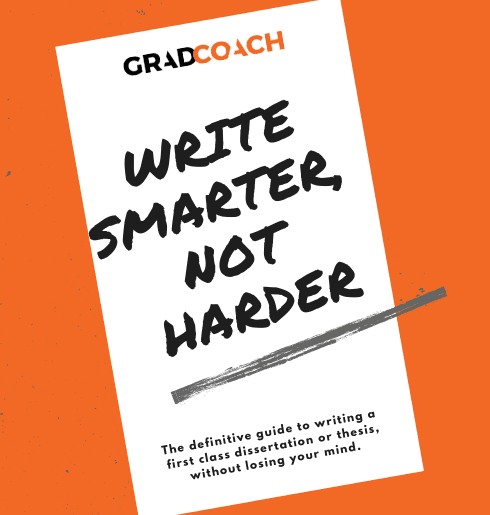
Psst… there’s more (for free)
This post is part of our dissertation mini-course, which covers everything you need to get started with your dissertation, thesis or research project.
17 Comments
Thank you for giving a valuable lesson
good thanks!
VERY INSIGHTFUL
thanks for given very interested understand about both theoritical and conceptual framework
I am researching teacher beliefs about inclusive education but not using a theoretical framework just conceptual frame using teacher beliefs, inclusive education and inclusive practices as my concepts
good, fantastic
great! thanks for the clarification. I am planning to use both for my implementation evaluation of EmONC service at primary health care facility level. its theoretical foundation rooted from the principles of implementation science.
This is a good one…now have a better understanding of Theoretical and Conceptual frameworks. Highly grateful
Very educating and fantastic,good to be part of you guys,I appreciate your enlightened concern.
Thanks for shedding light on these two t opics. Much clearer in my head now.
Simple and clear!
The differences between the two topics was well explained, thank you very much!
Thank you great insight
Superb. Thank you so much.
Hello Gradcoach! I’m excited with your fantastic educational videos which mainly focused on all over research process. I’m a student, I kindly ask and need your support. So, if it’s possible please send me the PDF format of all topic provided here, I put my email below, thank you!
I am really grateful I found this website. This is very helpful for an MPA student like myself.
I’m clear with these two terminologies now. Useful information. I appreciate it. Thank you
Submit a Comment Cancel reply
Your email address will not be published. Required fields are marked *
Save my name, email, and website in this browser for the next time I comment.
- Print Friendly
- Link to facebook
- Link to linkedin
- Link to twitter
- Link to youtube
- Writing Tips
How to Make a Conceptual Framework
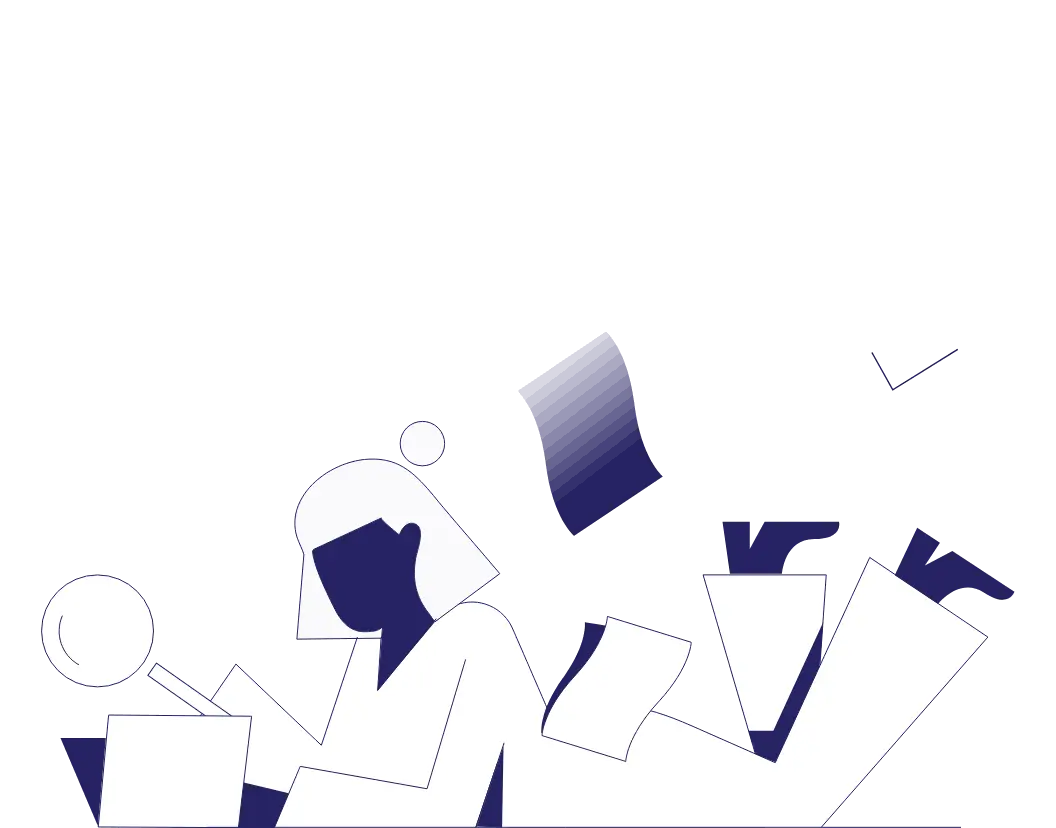
- 6-minute read
- 2nd January 2022
What is a conceptual framework? And why is it important?
A conceptual framework illustrates the relationship between the variables of a research question. It’s an outline of what you’d expect to find in a research project.
Conceptual frameworks should be constructed before data collection and are vital because they map out the actions needed in the study. This should be the first step of an undergraduate or graduate research project.
What Is In a Conceptual Framework?
In a conceptual framework, you’ll find a visual representation of the key concepts and relationships that are central to a research study or project . This can be in form of a diagram, flow chart, or any other visual representation. Overall, a conceptual framework serves as a guide for understanding the problem being studied and the methods being used to investigate it.
Steps to Developing the Perfect Conceptual Framework
- Pick a question
- Conduct a literature review
- Identify your variables
- Create your conceptual framework
1. Pick a Question
You should already have some idea of the broad area of your research project. Try to narrow down your research field to a manageable topic in terms of time and resources. From there, you need to formulate your research question. A research question answers the researcher’s query: “What do I want to know about my topic?” Research questions should be focused, concise, arguable and, ideally, should address a topic of importance within your field of research.
An example of a simple research question is: “What is the relationship between sunny days and ice cream sales?”
2. Conduct a Literature Review
A literature review is an analysis of the scholarly publications on a chosen topic. To undertake a literature review, search for articles with the same theme as your research question. Choose updated and relevant articles to analyze and use peer-reviewed and well-respected journals whenever possible.
For the above example, the literature review would investigate publications that discuss how ice cream sales are affected by the weather. The literature review should reveal the variables involved and any current hypotheses about this relationship.
3. Identify Your Variables
There are two key variables in every experiment: independent and dependent variables.
Independent Variables
The independent variable (otherwise known as the predictor or explanatory variable) is the expected cause of the experiment: what the scientist changes or changes on its own. In our example, the independent variable would be “the number of sunny days.”
Dependent Variables
The dependent variable (otherwise known as the response or outcome variable) is the expected effect of the experiment: what is being studied or measured. In our example, the dependent variable would be “the quantity of ice cream sold.”
Next, there are control variables.
Control Variables
A control variable is a variable that may impact the dependent variable but whose effects are not going to be measured in the research project. In our example, a control variable could be “the socioeconomic status of participants.” Control variables should be kept constant to isolate the effects of the other variables in the experiment.
Finally, there are intervening and extraneous variables.
Find this useful?
Subscribe to our newsletter and get writing tips from our editors straight to your inbox.
Intervening Variables
Intervening variables link the independent and dependent variables and clarify their connection. In our example, an intervening variable could be “temperature.”
Extraneous Variables
Extraneous variables are any variables that are not being investigated but could impact the outcomes of the study. Some instances of extraneous variables for our example would be “the average price of ice cream” or “the number of varieties of ice cream available.” If you control an extraneous variable, it becomes a control variable.
4. Create Your Conceptual Framework
Having picked your research question, undertaken a literature review, and identified the relevant variables, it’s now time to construct your conceptual framework. Conceptual frameworks are clear and often visual representations of the relationships between variables.
We’ll start with the basics: the independent and dependent variables.
Our hypothesis is that the quantity of ice cream sold directly depends on the number of sunny days; hence, there is a cause-and-effect relationship between the independent variable (the number of sunny days) and the dependent and independent variable (the quantity of ice cream sold).
Next, introduce a control variable. Remember, this is anything that might directly affect the dependent variable but is not being measured in the experiment:
Finally, introduce the intervening and extraneous variables.
The intervening variable (temperature) clarifies the relationship between the independent variable (the number of sunny days) and the dependent variable (the quantity of ice cream sold). Extraneous variables, such as the average price of ice cream, are variables that are not controlled and can potentially impact the dependent variable.
Are Conceptual Frameworks and Research Paradigms the Same?
In simple terms, the research paradigm is what informs your conceptual framework. In defining our research paradigm we ask the big questions—Is there an objective truth and how can we understand it? If we decide the answer is yes, we may be working with a positivist research paradigm and will choose to build a conceptual framework that displays the relationship between fixed variables. If not, we may be working with a constructivist research paradigm, and thus our conceptual framework will be more of a loose amalgamation of ideas, theories, and themes (a qualitative study). If this is confusing–don’t worry! We have an excellent blog post explaining research paradigms in more detail.
Where is the Conceptual Framework Located in a Thesis?
This will depend on your discipline, research type, and school’s guidelines, but most papers will include a section presenting the conceptual framework in the introduction, literature review, or opening chapter. It’s best to present your conceptual framework after presenting your research question, but before outlining your methodology.
Can a Conceptual Framework be Used in a Qualitative Study?
Yes. Despite being less clear-cut than a quantitative study, all studies should present some form of a conceptual framework. Let’s say you were doing a study on care home practices and happiness, and you came across a “happiness model” constructed by a relevant theorist in your literature review. Your conceptual framework could be an outline or a visual depiction of how you will use this model to collect and interpret qualitative data for your own study (such as interview responses). Check out this useful resource showing other examples of conceptual frameworks for qualitative studies .
Expert Proofreading for Researchers
Whether you’re a seasoned academic or not, you will want your research paper to be error-free and fluently written. That’s where proofreading comes in. Our editors are on hand 24 hours a day to ensure your writing is concise, clear, and precise. Submit a free sample of your writing today to try our services.
Share this article:
Post A New Comment
Got content that needs a quick turnaround? Let us polish your work. Explore our editorial business services.
3-minute read
What Is a Content Editor?
Are you interested in learning more about the role of a content editor and the...
4-minute read
The Benefits of Using an Online Proofreading Service
Proofreading is important to ensure your writing is clear and concise for your readers. Whether...
2-minute read
6 Online AI Presentation Maker Tools
Creating presentations can be time-consuming and frustrating. Trying to construct a visually appealing and informative...
What Is Market Research?
No matter your industry, conducting market research helps you keep up to date with shifting...
8 Press Release Distribution Services for Your Business
In a world where you need to stand out, press releases are key to being...
How to Get a Patent
In the United States, the US Patent and Trademarks Office issues patents. In the United...
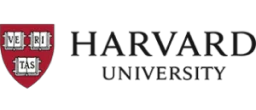
Make sure your writing is the best it can be with our expert English proofreading and editing.
- Resources Home 🏠
- Try SciSpace Copilot
- Search research papers
- Add Copilot Extension
- Try AI Detector
- Try Paraphraser
- Try Citation Generator
- April Papers
- June Papers
- July Papers

What is a thesis | A Complete Guide with Examples

Table of Contents
A thesis is a comprehensive academic paper based on your original research that presents new findings, arguments, and ideas of your study. It’s typically submitted at the end of your master’s degree or as a capstone of your bachelor’s degree.
However, writing a thesis can be laborious, especially for beginners. From the initial challenge of pinpointing a compelling research topic to organizing and presenting findings, the process is filled with potential pitfalls.
Therefore, to help you, this guide talks about what is a thesis. Additionally, it offers revelations and methodologies to transform it from an overwhelming task to a manageable and rewarding academic milestone.
What is a thesis?
A thesis is an in-depth research study that identifies a particular topic of inquiry and presents a clear argument or perspective about that topic using evidence and logic.
Writing a thesis showcases your ability of critical thinking, gathering evidence, and making a compelling argument. Integral to these competencies is thorough research, which not only fortifies your propositions but also confers credibility to your entire study.
Furthermore, there's another phenomenon you might often confuse with the thesis: the ' working thesis .' However, they aren't similar and shouldn't be used interchangeably.
A working thesis, often referred to as a preliminary or tentative thesis, is an initial version of your thesis statement. It serves as a draft or a starting point that guides your research in its early stages.
As you research more and gather more evidence, your initial thesis (aka working thesis) might change. It's like a starting point that can be adjusted as you learn more. It's normal for your main topic to change a few times before you finalize it.
While a thesis identifies and provides an overarching argument, the key to clearly communicating the central point of that argument lies in writing a strong thesis statement.
What is a thesis statement?
A strong thesis statement (aka thesis sentence) is a concise summary of the main argument or claim of the paper. It serves as a critical anchor in any academic work, succinctly encapsulating the primary argument or main idea of the entire paper.
Typically found within the introductory section, a strong thesis statement acts as a roadmap of your thesis, directing readers through your arguments and findings. By delineating the core focus of your investigation, it offers readers an immediate understanding of the context and the gravity of your study.
Furthermore, an effectively crafted thesis statement can set forth the boundaries of your research, helping readers anticipate the specific areas of inquiry you are addressing.
Different types of thesis statements
A good thesis statement is clear, specific, and arguable. Therefore, it is necessary for you to choose the right type of thesis statement for your academic papers.
Thesis statements can be classified based on their purpose and structure. Here are the primary types of thesis statements:
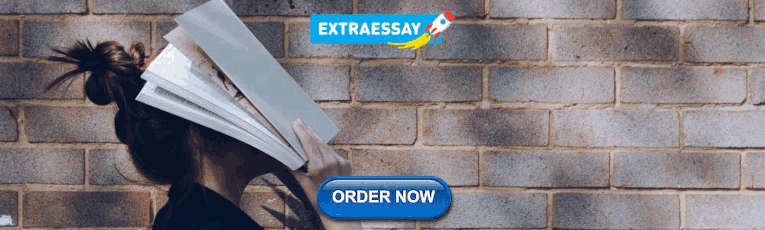
Argumentative (or Persuasive) thesis statement
Purpose : To convince the reader of a particular stance or point of view by presenting evidence and formulating a compelling argument.
Example : Reducing plastic use in daily life is essential for environmental health.
Analytical thesis statement
Purpose : To break down an idea or issue into its components and evaluate it.
Example : By examining the long-term effects, social implications, and economic impact of climate change, it becomes evident that immediate global action is necessary.
Expository (or Descriptive) thesis statement
Purpose : To explain a topic or subject to the reader.
Example : The Great Depression, spanning the 1930s, was a severe worldwide economic downturn triggered by a stock market crash, bank failures, and reduced consumer spending.
Cause and effect thesis statement
Purpose : To demonstrate a cause and its resulting effect.
Example : Overuse of smartphones can lead to impaired sleep patterns, reduced face-to-face social interactions, and increased levels of anxiety.
Compare and contrast thesis statement
Purpose : To highlight similarities and differences between two subjects.
Example : "While both novels '1984' and 'Brave New World' delve into dystopian futures, they differ in their portrayal of individual freedom, societal control, and the role of technology."
When you write a thesis statement , it's important to ensure clarity and precision, so the reader immediately understands the central focus of your work.
What is the difference between a thesis and a thesis statement?
While both terms are frequently used interchangeably, they have distinct meanings.
A thesis refers to the entire research document, encompassing all its chapters and sections. In contrast, a thesis statement is a brief assertion that encapsulates the central argument of the research.
Here’s an in-depth differentiation table of a thesis and a thesis statement.
Now, to craft a compelling thesis, it's crucial to adhere to a specific structure. Let’s break down these essential components that make up a thesis structure
15 components of a thesis structure
Navigating a thesis can be daunting. However, understanding its structure can make the process more manageable.
Here are the key components or different sections of a thesis structure:
Your thesis begins with the title page. It's not just a formality but the gateway to your research.

Here, you'll prominently display the necessary information about you (the author) and your institutional details.
- Title of your thesis
- Your full name
- Your department
- Your institution and degree program
- Your submission date
- Your Supervisor's name (in some cases)
- Your Department or faculty (in some cases)
- Your University's logo (in some cases)
- Your Student ID (in some cases)
In a concise manner, you'll have to summarize the critical aspects of your research in typically no more than 200-300 words.

This includes the problem statement, methodology, key findings, and conclusions. For many, the abstract will determine if they delve deeper into your work, so ensure it's clear and compelling.
Acknowledgments
Research is rarely a solitary endeavor. In the acknowledgments section, you have the chance to express gratitude to those who've supported your journey.

This might include advisors, peers, institutions, or even personal sources of inspiration and support. It's a personal touch, reflecting the humanity behind the academic rigor.
Table of contents
A roadmap for your readers, the table of contents lists the chapters, sections, and subsections of your thesis.
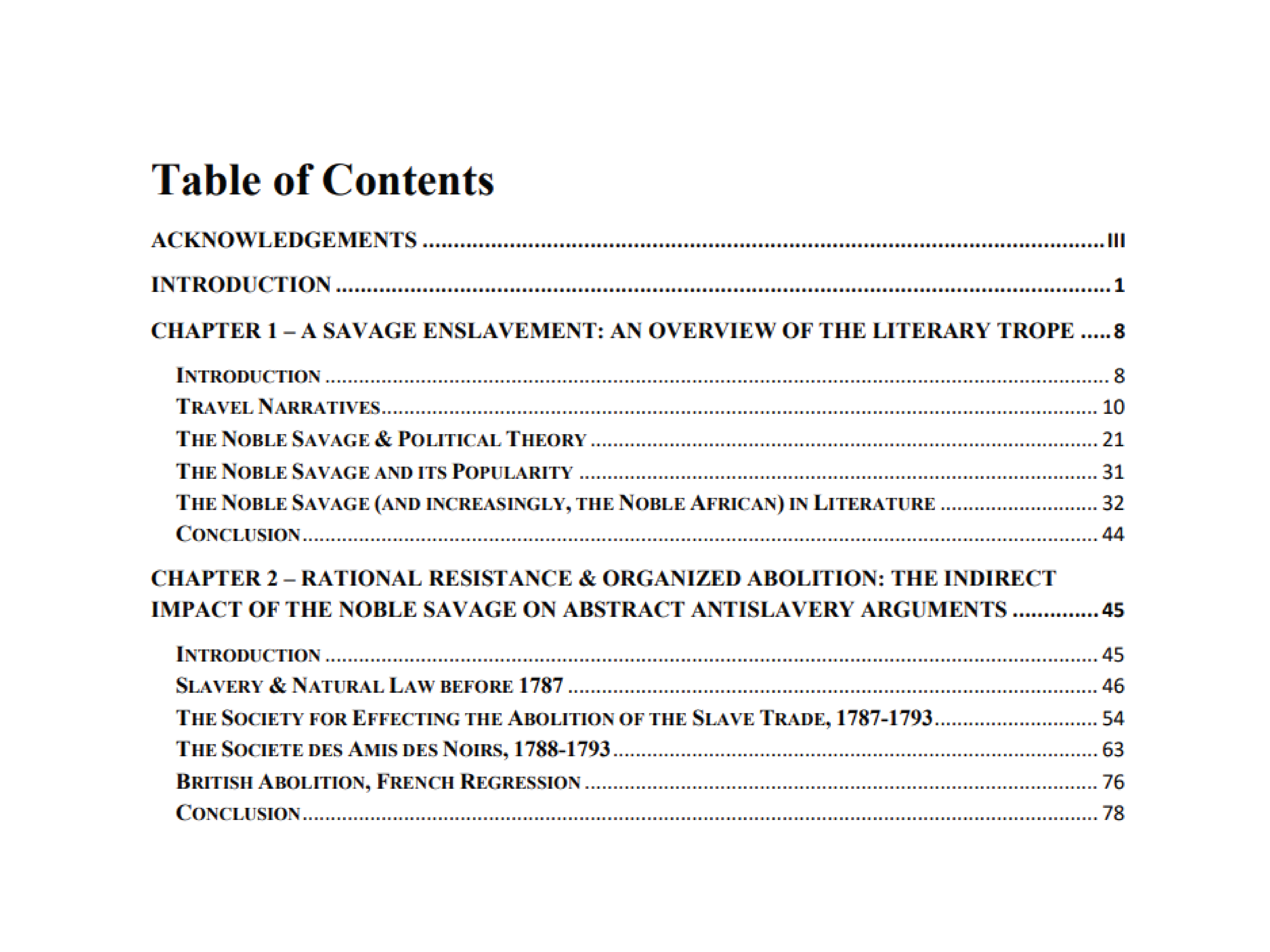
By providing page numbers, you allow readers to navigate your work easily, jumping to sections that pique their interest.
List of figures and tables
Research often involves data, and presenting this data visually can enhance understanding. This section provides an organized listing of all figures and tables in your thesis.

It's a visual index, ensuring that readers can quickly locate and reference your graphical data.
Introduction
Here's where you introduce your research topic, articulate the research question or objective, and outline the significance of your study.

- Present the research topic : Clearly articulate the central theme or subject of your research.
- Background information : Ground your research topic, providing any necessary context or background information your readers might need to understand the significance of your study.
- Define the scope : Clearly delineate the boundaries of your research, indicating what will and won't be covered.
- Literature review : Introduce any relevant existing research on your topic, situating your work within the broader academic conversation and highlighting where your research fits in.
- State the research Question(s) or objective(s) : Clearly articulate the primary questions or objectives your research aims to address.
- Outline the study's structure : Give a brief overview of how the subsequent sections of your work will unfold, guiding your readers through the journey ahead.
The introduction should captivate your readers, making them eager to delve deeper into your research journey.
Literature review section
Your study correlates with existing research. Therefore, in the literature review section, you'll engage in a dialogue with existing knowledge, highlighting relevant studies, theories, and findings.
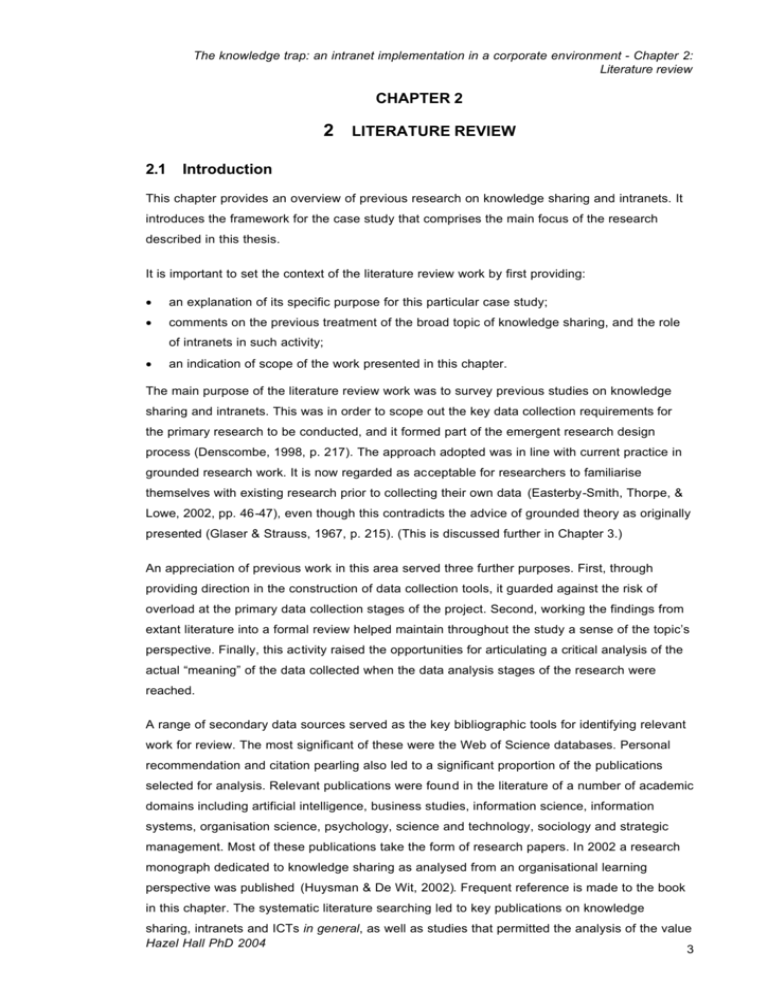
It's here that you identify gaps in the current knowledge, positioning your research as a bridge to new insights.
To streamline this process, consider leveraging AI tools. For example, the SciSpace literature review tool enables you to efficiently explore and delve into research papers, simplifying your literature review journey.
Methodology
In the research methodology section, you’ll detail the tools, techniques, and processes you employed to gather and analyze data. This section will inform the readers about how you approached your research questions and ensures the reproducibility of your study.
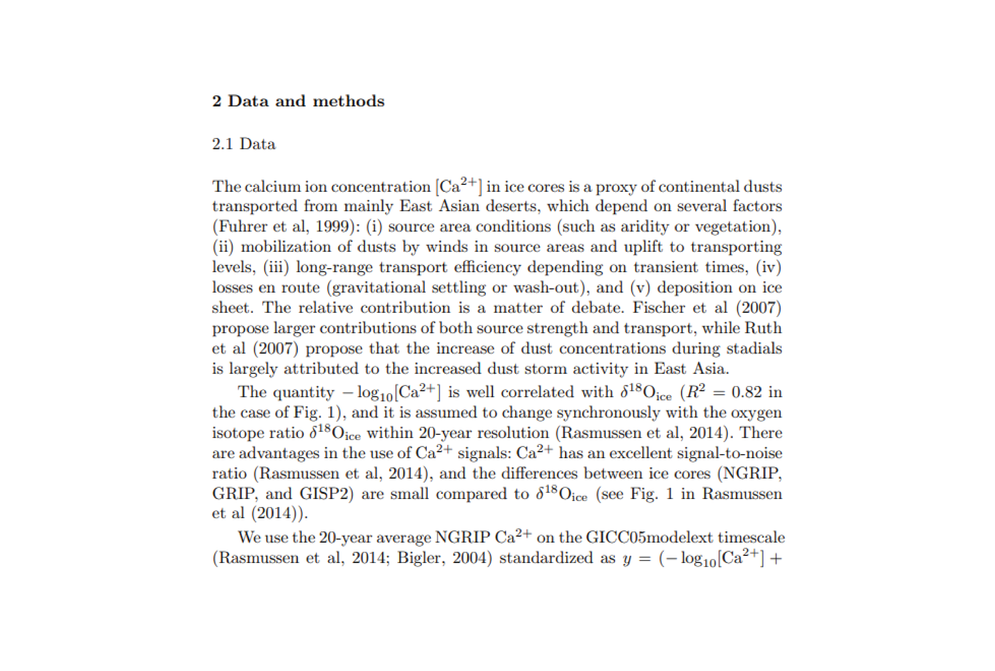
Here's a breakdown of what it should encompass:
- Research Design : Describe the overall structure and approach of your research. Are you conducting a qualitative study with in-depth interviews? Or is it a quantitative study using statistical analysis? Perhaps it's a mixed-methods approach?
- Data Collection : Detail the methods you used to gather data. This could include surveys, experiments, observations, interviews, archival research, etc. Mention where you sourced your data, the duration of data collection, and any tools or instruments used.
- Sampling : If applicable, explain how you selected participants or data sources for your study. Discuss the size of your sample and the rationale behind choosing it.
- Data Analysis : Describe the techniques and tools you used to process and analyze the data. This could range from statistical tests in quantitative research to thematic analysis in qualitative research.
- Validity and Reliability : Address the steps you took to ensure the validity and reliability of your findings to ensure that your results are both accurate and consistent.
- Ethical Considerations : Highlight any ethical issues related to your research and the measures you took to address them, including — informed consent, confidentiality, and data storage and protection measures.
Moreover, different research questions necessitate different types of methodologies. For instance:
- Experimental methodology : Often used in sciences, this involves a controlled experiment to discern causality.
- Qualitative methodology : Employed when exploring patterns or phenomena without numerical data. Methods can include interviews, focus groups, or content analysis.
- Quantitative methodology : Concerned with measurable data and often involves statistical analysis. Surveys and structured observations are common tools here.
- Mixed methods : As the name implies, this combines both qualitative and quantitative methodologies.
The Methodology section isn’t just about detailing the methods but also justifying why they were chosen. The appropriateness of the methods in addressing your research question can significantly impact the credibility of your findings.
Results (or Findings)
This section presents the outcomes of your research. It's crucial to note that the nature of your results may vary; they could be quantitative, qualitative, or a mix of both.

Quantitative results often present statistical data, showcasing measurable outcomes, and they benefit from tables, graphs, and figures to depict these data points.
Qualitative results , on the other hand, might delve into patterns, themes, or narratives derived from non-numerical data, such as interviews or observations.
Regardless of the nature of your results, clarity is essential. This section is purely about presenting the data without offering interpretations — that comes later in the discussion.
In the discussion section, the raw data transforms into valuable insights.
Start by revisiting your research question and contrast it with the findings. How do your results expand, constrict, or challenge current academic conversations?
Dive into the intricacies of the data, guiding the reader through its implications. Detail potential limitations transparently, signaling your awareness of the research's boundaries. This is where your academic voice should be resonant and confident.
Practical implications (Recommendation) section
Based on the insights derived from your research, this section provides actionable suggestions or proposed solutions.
Whether aimed at industry professionals or the general public, recommendations translate your academic findings into potential real-world actions. They help readers understand the practical implications of your work and how it can be applied to effect change or improvement in a given field.
When crafting recommendations, it's essential to ensure they're feasible and rooted in the evidence provided by your research. They shouldn't merely be aspirational but should offer a clear path forward, grounded in your findings.
The conclusion provides closure to your research narrative.
It's not merely a recap but a synthesis of your main findings and their broader implications. Reconnect with the research questions or hypotheses posited at the beginning, offering clear answers based on your findings.

Reflect on the broader contributions of your study, considering its impact on the academic community and potential real-world applications.
Lastly, the conclusion should leave your readers with a clear understanding of the value and impact of your study.
References (or Bibliography)
Every theory you've expounded upon, every data point you've cited, and every methodological precedent you've followed finds its acknowledgment here.

In references, it's crucial to ensure meticulous consistency in formatting, mirroring the specific guidelines of the chosen citation style .
Proper referencing helps to avoid plagiarism , gives credit to original ideas, and allows readers to explore topics of interest. Moreover, it situates your work within the continuum of academic knowledge.
To properly cite the sources used in the study, you can rely on online citation generator tools to generate accurate citations!
Here’s more on how you can cite your sources.
Often, the depth of research produces a wealth of material that, while crucial, can make the core content of the thesis cumbersome. The appendix is where you mention extra information that supports your research but isn't central to the main text.

Whether it's raw datasets, detailed procedural methodologies, extended case studies, or any other ancillary material, the appendices ensure that these elements are archived for reference without breaking the main narrative's flow.
For thorough researchers and readers keen on meticulous details, the appendices provide a treasure trove of insights.
Glossary (optional)
In academics, specialized terminologies, and jargon are inevitable. However, not every reader is versed in every term.
The glossary, while optional, is a critical tool for accessibility. It's a bridge ensuring that even readers from outside the discipline can access, understand, and appreciate your work.

By defining complex terms and providing context, you're inviting a wider audience to engage with your research, enhancing its reach and impact.
Remember, while these components provide a structured framework, the essence of your thesis lies in the originality of your ideas, the rigor of your research, and the clarity of your presentation.
As you craft each section, keep your readers in mind, ensuring that your passion and dedication shine through every page.
Thesis examples
To further elucidate the concept of a thesis, here are illustrative examples from various fields:
Example 1 (History): Abolition, Africans, and Abstraction: the Influence of the ‘Noble Savage’ on British and French Antislavery Thought, 1787-1807 by Suchait Kahlon.
Example 2 (Climate Dynamics): Influence of external forcings on abrupt millennial-scale climate changes: a statistical modelling study by Takahito Mitsui · Michel Crucifix
Checklist for your thesis evaluation
Evaluating your thesis ensures that your research meets the standards of academia. Here's an elaborate checklist to guide you through this critical process.
Content and structure
- Is the thesis statement clear, concise, and debatable?
- Does the introduction provide sufficient background and context?
- Is the literature review comprehensive, relevant, and well-organized?
- Does the methodology section clearly describe and justify the research methods?
- Are the results/findings presented clearly and logically?
- Does the discussion interpret the results in light of the research question and existing literature?
- Is the conclusion summarizing the research and suggesting future directions or implications?
Clarity and coherence
- Is the writing clear and free of jargon?
- Are ideas and sections logically connected and flowing?
- Is there a clear narrative or argument throughout the thesis?
Research quality
- Is the research question significant and relevant?
- Are the research methods appropriate for the question?
- Is the sample size (if applicable) adequate?
- Are the data analysis techniques appropriate and correctly applied?
- Are potential biases or limitations addressed?
Originality and significance
- Does the thesis contribute new knowledge or insights to the field?
- Is the research grounded in existing literature while offering fresh perspectives?
Formatting and presentation
- Is the thesis formatted according to institutional guidelines?
- Are figures, tables, and charts clear, labeled, and referenced in the text?
- Is the bibliography or reference list complete and consistently formatted?
- Are appendices relevant and appropriately referenced in the main text?
Grammar and language
- Is the thesis free of grammatical and spelling errors?
- Is the language professional, consistent, and appropriate for an academic audience?
- Are quotations and paraphrased material correctly cited?
Feedback and revision
- Have you sought feedback from peers, advisors, or experts in the field?
- Have you addressed the feedback and made the necessary revisions?
Overall assessment
- Does the thesis as a whole feel cohesive and comprehensive?
- Would the thesis be understandable and valuable to someone in your field?
Ensure to use this checklist to leave no ground for doubt or missed information in your thesis.
After writing your thesis, the next step is to discuss and defend your findings verbally in front of a knowledgeable panel. You’ve to be well prepared as your professors may grade your presentation abilities.
Preparing your thesis defense
A thesis defense, also known as "defending the thesis," is the culmination of a scholar's research journey. It's the final frontier, where you’ll present their findings and face scrutiny from a panel of experts.
Typically, the defense involves a public presentation where you’ll have to outline your study, followed by a question-and-answer session with a committee of experts. This committee assesses the validity, originality, and significance of the research.
The defense serves as a rite of passage for scholars. It's an opportunity to showcase expertise, address criticisms, and refine arguments. A successful defense not only validates the research but also establishes your authority as a researcher in your field.
Here’s how you can effectively prepare for your thesis defense .
Now, having touched upon the process of defending a thesis, it's worth noting that scholarly work can take various forms, depending on academic and regional practices.
One such form, often paralleled with the thesis, is the 'dissertation.' But what differentiates the two?
Dissertation vs. Thesis
Often used interchangeably in casual discourse, they refer to distinct research projects undertaken at different levels of higher education.
To the uninitiated, understanding their meaning might be elusive. So, let's demystify these terms and delve into their core differences.
Here's a table differentiating between the two.
Wrapping up
From understanding the foundational concept of a thesis to navigating its various components, differentiating it from a dissertation, and recognizing the importance of proper citation — this guide covers it all.
As scholars and readers, understanding these nuances not only aids in academic pursuits but also fosters a deeper appreciation for the relentless quest for knowledge that drives academia.
It’s important to remember that every thesis is a testament to curiosity, dedication, and the indomitable spirit of discovery.
Good luck with your thesis writing!
Frequently Asked Questions
A thesis typically ranges between 40-80 pages, but its length can vary based on the research topic, institution guidelines, and level of study.
A PhD thesis usually spans 200-300 pages, though this can vary based on the discipline, complexity of the research, and institutional requirements.
To identify a thesis topic, consider current trends in your field, gaps in existing literature, personal interests, and discussions with advisors or mentors. Additionally, reviewing related journals and conference proceedings can provide insights into potential areas of exploration.
The conceptual framework is often situated in the literature review or theoretical framework section of a thesis. It helps set the stage by providing the context, defining key concepts, and explaining the relationships between variables.
A thesis statement should be concise, clear, and specific. It should state the main argument or point of your research. Start by pinpointing the central question or issue your research addresses, then condense that into a single statement, ensuring it reflects the essence of your paper.
You might also like

AI for Meta Analysis — A Comprehensive Guide
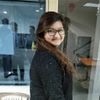
Cybersecurity in Higher Education: Safeguarding Students and Faculty Data

How To Write An Argumentative Essay
Understanding and solving intractable resource governance problems.
- In the Press
- Conferences and Talks
- Exploring models of electronic wastes governance in the United States and Mexico: Recycling, risk and environmental justice
- The Collaborative Resource Governance Lab (CoReGovLab)
- Water Conflicts in Mexico: A Multi-Method Approach
- Past projects
- Publications and scholarly output
- Research Interests
- Higher education and academia
- Public administration, public policy and public management research
- Research-oriented blog posts
- Stuff about research methods
- Research trajectory
- Publications
- Developing a Writing Practice
- Outlining Papers
- Publishing strategies
- Writing a book manuscript
- Writing a research paper, book chapter or dissertation/thesis chapter
- Everything Notebook
- Literature Reviews
- Note-Taking Techniques
- Organization and Time Management
- Planning Methods and Approaches
- Qualitative Methods, Qualitative Research, Qualitative Analysis
- Reading Notes of Books
- Reading Strategies
- Teaching Public Policy, Public Administration and Public Management
- My Reading Notes of Books on How to Write a Doctoral Dissertation/How to Conduct PhD Research
- Writing a Thesis (Undergraduate or Masters) or a Dissertation (PhD)
- Reading strategies for undergraduates
- Social Media in Academia
- Resources for Job Seekers in the Academic Market
- Writing Groups and Retreats
- Regional Development (Fall 2015)
- State and Local Government (Fall 2015)
- Public Policy Analysis (Fall 2016)
- Regional Development (Fall 2016)
- Public Policy Analysis (Fall 2018)
- Public Policy Analysis (Fall 2019)
- Public Policy Analysis (Spring 2016)
- POLI 351 Environmental Policy and Politics (Summer Session 2011)
- POLI 352 Comparative Politics of Public Policy (Term 2)
- POLI 375A Global Environmental Politics (Term 2)
- POLI 350A Public Policy (Term 2)
- POLI 351 Environmental Policy and Politics (Term 1)
- POLI 332 Latin American Environmental Politics (Term 2, Spring 2012)
- POLI 350A Public Policy (Term 1, Sep-Dec 2011)
- POLI 375A Global Environmental Politics (Term 1, Sep-Dec 2011)
Writing theoretical frameworks, analytical frameworks and conceptual frameworks
Three of the most challenging concepts for me to explain are the interrelated ideas of a theoretical framework, a conceptual framework, and an analytical framework. All three of these tend to be used interchangeably. While I find these concepts somewhat fuzzy and I struggle sometimes to explain the differences between them and clarify their usage for my students (and clearly I am not alone in this challenge), this blog post is an attempt to help discern these analytical categories more clearly.
A lot of people (my own students included) have asked me if the theoretical framework is their literature review. That’s actually not the case. A theoretical framework , the way I define it, is comprised of the different theories and theoretical constructs that help explain a phenomenon. A theoretical framework sets out the various expectations that a theory posits and how they would apply to a specific case under analysis, and how one would use theory to explain a particular phenomenon. I like how theoretical frameworks are defined in this blog post . Dr. Cyrus Samii offers an explanation of what a good theoretical framework does for students .
For example, you can use framing theory to help you explain how different actors perceive the world. Your theoretical framework may be based on theories of framing, but it can also include others. For example, in this paper, Zeitoun and Allan explain their theoretical framework, aptly named hydro-hegemony . In doing so, Zeitoun and Allan explain the role of each theoretical construct (Power, Hydro-Hegemony, Political Economy) and how they apply to transboundary water conflict. Another good example of a theoretical framework is that posited by Dr. Michael J. Bloomfield in his book Dirty Gold, as I mention in this tweet:
In Chapter 2, @mj_bloomfield nicely sets his theoretical framework borrowing from sociology, IR, and business-strategy scholarship pic.twitter.com/jTGF4PPymn — Dr Raul Pacheco-Vega (@raulpacheco) December 24, 2017
An analytical framework is, the way I see it, a model that helps explain how a certain type of analysis will be conducted. For example, in this paper, Franks and Cleaver develop an analytical framework that includes scholarship on poverty measurement to help us understand how water governance and poverty are interrelated . Other authors describe an analytical framework as a “conceptual framework that helps analyse particular phenomena”, as posited here , ungated version can be read here .
I think it’s easy to conflate analytical frameworks with theoretical and conceptual ones because of the way in which concepts, theories and ideas are harnessed to explain a phenomenon. But I believe the most important element of an analytical framework is instrumental : their purpose is to help undertake analyses. You use elements of an analytical framework to deconstruct a specific concept/set of concepts/phenomenon. For example, in this paper , Bodde et al develop an analytical framework to characterise sources of uncertainties in strategic environmental assessments.
A robust conceptual framework describes the different concepts one would need to know to understand a particular phenomenon, without pretending to create causal links across variables and outcomes. In my view, theoretical frameworks set expectations, because theories are constructs that help explain relationships between variables and specific outcomes and responses. Conceptual frameworks, the way I see them, are like lenses through which you can see a particular phenomenon.
A conceptual framework should serve to help illuminate and clarify fuzzy ideas, and fill lacunae. Viewed this way, a conceptual framework offers insight that would not be otherwise be gained without a more profound understanding of the concepts explained in the framework. For example, in this article, Beck offers social movement theory as a conceptual framework that can help understand terrorism . As I explained in my metaphor above, social movement theory is the lens through which you see terrorism, and you get a clearer understanding of how it operates precisely because you used this particular theory.
Dan Kaminsky offered a really interesting explanation connecting these topics to time, read his tweet below.
I think this maps to time. Theoretical frameworks talk about how we got here. Conceptual frameworks discuss what we have. Analytical frameworks discuss where we can go with this. See also legislative/executive/judicial. — Dan Kaminsky (@dakami) September 28, 2018
One of my CIDE students, Andres Ruiz, reminded me of this article on conceptual frameworks in the International Journal of Qualitative Methods. I’ll also be adding resources as I get them via Twitter or email. Hopefully this blog post will help clarify this idea!
You can share this blog post on the following social networks by clicking on their icon.
Posted in academia .
Tagged with analytical framework , conceptual framework , theoretical framework .
By Raul Pacheco-Vega – September 28, 2018
7 Responses
Stay in touch with the conversation, subscribe to the RSS feed for comments on this post .
Thanks, this had some useful clarifications for me!
I GOT CONFUSED AGAIN!
No need to be confused!
Thanks for the Clarification, Dr Raul. My cluttered mind is largely cleared, now.
Thanks,very helpful
I too was/am confused but this helps 🙂
Thank you very much, Dr.
Leave a Reply Cancel Some HTML is OK
Name (required)
Email (required, but never shared)
or, reply to this post via trackback .
About Raul Pacheco-Vega, PhD
Find me online.
My Research Output
- Google Scholar Profile
- Academia.Edu
- ResearchGate
My Social Networks
- Polycentricity Network
Recent Posts
- “State-Sponsored Activism: Bureaucrats and Social Movements in Brazil” – Jessica Rich – my reading notes
- Reading Like a Writer – Francine Prose – my reading notes
- Using the Pacheco-Vega workflows and frameworks to write and/or revise a scholarly book
- On framing, the value of narrative and storytelling in scholarly research, and the importance of asking the “what is this a story of” question
- The Abstract Decomposition Matrix Technique to find a gap in the literature
Follow me on Twitter:
Proudly powered by WordPress and Carrington .
Carrington Theme by Crowd Favorite
While Sandel argues that pursuing perfection through genetic engineering would decrease our sense of humility, he claims that the sense of solidarity we would lose is also important.
This thesis summarizes several points in Sandel’s argument, but it does not make a claim about how we should understand his argument. A reader who read Sandel’s argument would not also need to read an essay based on this descriptive thesis.
Broad thesis (arguable, but difficult to support with evidence)
Michael Sandel’s arguments about genetic engineering do not take into consideration all the relevant issues.
This is an arguable claim because it would be possible to argue against it by saying that Michael Sandel’s arguments do take all of the relevant issues into consideration. But the claim is too broad. Because the thesis does not specify which “issues” it is focused on—or why it matters if they are considered—readers won’t know what the rest of the essay will argue, and the writer won’t know what to focus on. If there is a particular issue that Sandel does not address, then a more specific version of the thesis would include that issue—hand an explanation of why it is important.
Arguable thesis with analytical claim
While Sandel argues persuasively that our instinct to “remake” (54) ourselves into something ever more perfect is a problem, his belief that we can always draw a line between what is medically necessary and what makes us simply “better than well” (51) is less convincing.
This is an arguable analytical claim. To argue for this claim, the essay writer will need to show how evidence from the article itself points to this interpretation. It’s also a reasonable scope for a thesis because it can be supported with evidence available in the text and is neither too broad nor too narrow.
Arguable thesis with normative claim
Given Sandel’s argument against genetic enhancement, we should not allow parents to decide on using Human Growth Hormone for their children.
This thesis tells us what we should do about a particular issue discussed in Sandel’s article, but it does not tell us how we should understand Sandel’s argument.
Questions to ask about your thesis
- Is the thesis truly arguable? Does it speak to a genuine dilemma in the source, or would most readers automatically agree with it?
- Is the thesis too obvious? Again, would most or all readers agree with it without needing to see your argument?
- Is the thesis complex enough to require a whole essay's worth of argument?
- Is the thesis supportable with evidence from the text rather than with generalizations or outside research?
- Would anyone want to read a paper in which this thesis was developed? That is, can you explain what this paper is adding to our understanding of a problem, question, or topic?
- picture_as_pdf Thesis
What is a framework? Understanding their purpose, value, development and use
- Articles with Attitude
- Open access
- Published: 14 April 2023
- Volume 13 , pages 510–519, ( 2023 )
Cite this article
You have full access to this open access article
- Stefan Partelow ORCID: orcid.org/0000-0002-7751-4005 1 , 2
13k Accesses
4 Citations
18 Altmetric
Explore all metrics
Many frameworks exist across the sciences and science-policy interface, but it is not always clear how they are developed or can be applied. It is also often vague how new or existing frameworks are positioned in a theory of science to advance a specific theory or paradigm. This article examines these questions and positions the role of frameworks as integral but often vague scientific tools, highlighting benefits and critiques. While frameworks can be useful for synthesizing and communicating core concepts in a field, they often lack transparency in how they were developed and how they can be applied. Positioning frameworks within a theory of science can aid in knowing the purpose and value of framework use. This article provides a meta-framework for visualizing and engaging the four mediating processes for framework development and application: (1) empirical generalization, (2) theoretical fitting, (3) application, and (4) hypothesizing. Guiding points for scholars and policymakers using or developing frameworks in their research are provided in closing.
Similar content being viewed by others
Introduction
Reflections on Methodological Issues
Looking Back
Avoid common mistakes on your manuscript.
The development of ‘frameworks’ is at present probably the most common strategy in the field of natural resources management to achieve integration and interdisciplinarity. Mollinga , 2008
…it is not clear what the role of a scientific framework should be, and relatedly, what makes for a successful scientific framework. Ban and Cox, 2017
Frameworks are important research tools across nearly all fields of science. They are critically important for structuring empirical inquiry and theoretical development in the environmental social sciences, governance research and practice, the sustainability sciences and fields of social-ecological systems research in tangent with the associated disciplines of those fields (Binder et al. 2013 ; Pulver et al. 2018 ; Colding and Barthel 2019 ). Many well-established frameworks are regularly applied to collect new data or to structure entire research programs such as the Ecosystem Services (ES) framework (Potschin-Young et al. 2018 ), the Social-Ecological Systems Framework (SESF) (McGinnis and Ostrom 2014a ), Earth Systems Governance (ESG) (Biermann et al. 2010 ), the Driver-Impact-Pressure-State-Response (DIPSR) framework, and the Life Cycle Assessment (LCA) framework. Frameworks are also put forth by major scientific organizing bodies to steer scientific and policy agendas at regional and global levels such as the Intergovernmental Science-Policy Platform on Biodiversity and Ecosystem Services (IPBES) (Díaz et al. 2015 ) and the Global Sustainable Development Report’s transformational levers and fields (UN 2019 ).
Despite the countless frameworks, it is not always clear how a framework can be developed or applied (Ban and Cox 2017 ; Partelow 2018 ; Nagel and Partelow 2022 ). Development may occur through empirically backed synthesis or by scholars based on their own knowledge, values, or interests. These diverse development pathways do, however, result in common trends. The structure of most frameworks is the identification of a set of concepts and their general relationships — often in the form box-and-arrow diagrams — that are loosely defined or unspecified. This hallmark has both benefits and challenges. On one hand, this is arguably the purpose of frameworks, to structure the basic ideas of theory or conceptual thinking, and if they were more detailed they would be models. On the other hand, there is often a “black box” nature to frameworks. It is often unclear why some sets of concepts and relationships are chosen for integration into frameworks, and others not. As argued below, these choices are often the result of the positionality of the framework’s creators. Publications of frameworks, furthermore, often lack descriptions of their value and potential uses compared to other frameworks or analytical tools that exist in the field.
Now shifting focus to how frameworks are applied. Some frameworks provide measureable indicators as the key variables in the framework, but many only suggest general concepts. This creates the need to link concepts and their relationships to data through other more tangible indicators. Methods to measure such indicators will also be needed in new empirical studies. These methodological and study design steps necessary to associate data to framework concepts is often referred to as “operationalizing” a framework. However, without guidance on how to do this, scholars are often left with developing their own strategies, which can lead to heterogeneous and idiosyncratic methods and data. These challenges can be referred to as methodological gaps (Partelow 2018 ), where the details of how to move from concept to indicator to measurement to data transformation, are not always detailed in a way that welcomes replicability or learning. This is not necessarily a problem if the purpose of a framework is to only guide the analysis of individual cases or synthesis activities in isolation, for example to inform local management, but it hinders meta-analyses, cross-case learning and data interpretability for others.
In this article, a brief overview of framework definitions and current synthesis literature are reviewed in the “ What is a framework? ” section. This is coupled with the argument that frameworks often lack clarity in their development and application because their positioning within a theory of science is unclear. In the “ Mechanisms of framework development and use: a meta-framework ” section, a meta-framework is proposed to assist in clarifying the four major levers with which frameworks are developed and applied: (1) empirical generalization, (2) theoretical fitting, (3) hypothesizing, and (4) application. The meta-framework aims to position individual frameworks into a theory of science, which can enable scholars to take a conceptual “step back” in order to view how their engagement with a framework contributes to their broader scientific goal and field. Two case studies of different frameworks are provided to explore how the meta-framework can aid in comparing them. This is followed by a discussion of what makes a good framework, along with explicit guiding points for the use of frameworks in research and policy practice.
What is a framework?
The definition and purpose of a framework is likely to vary across disciplines and thematic fields (Cox et al. 2016 ). There is no universal definition of a framework, but it is useful to provide a brief overview of different definitions for orientation. The Cambridge Dictionary states that frameworks are “a supporting structure around which something can be built; a system of rules, ideas, or beliefs that is used to plan or decide something.” Schlager ( 2007 , 293) states that “frameworks provide a foundation for inquiry,” and Cumming ( 2014 , 5) adds that this “does not necessarily depend on deductive logic to connect different ideas.” Importantly, Binder et al., ( 2013 , 2) note that “a framework provides a set of assumptions, concepts, values and practices,” emphasizing the normative or inherently subjective logic to framework development. A core theme being plurality and connectivity. Similarly, McGinnis and Ostrom ( 2014a , 1) define frameworks as “the basic vocabulary of concepts and terms that may be used to construct the kinds of causal explanations expected of a theory. Frameworks organize diagnostic, descriptive, and prescriptive inquiry.” In a review comparing ten commonly used frameworks in social-ecological systems (SES) research, Binder et al., ( 2013 , 1) state that frameworks are useful for developing “a common language, to structure research on SES, and to provide guidance toward a more sustainable development of SES.” In a similar review, Pulver et al., ( 2018 , 1) suggest that frameworks “assist scholars and practitioners to analyze the complex, nonlinear interdependencies that characterize interactions between biophysical and social arenas and to navigate the new epistemological, ontological, analytical, and practical horizons of integrating knowledge for sustainability solutions.” It is important to recognize that the above claims often suggest the dualistic or bridging positions held by frameworks, in both theory building and for guiding empirical observations. However, there is relatively little discussion in the above literature on how frameworks act as bridging tools within a theory of science or how frameworks add value as positioning tools in a field.
Every framework has a position, meaning it is located within a specific context of a scientific field. As positioning tools, frameworks seem to “populate the scientist’s world with a set of conceptual objects and (non-causal) relationships among them,” shaping (and sometimes limiting) the way we think about problems and potential solutions (Cox et al. 2016 , 47). Thus, using a specific framework helps in part to position the work of a researcher in a field and its related concepts, theories and paradigms.
Four factors can be considered to evaluate the positioning of a framework: (a) who developed it, (b) the values being put forth by those researchers, (c) the research questions engaged with, and (d) the field in which it is embedded. For example, the Social-Ecological Systems Framework (SESF) (Ostrom 2009 ) was developed by (a) Elinor Ostrom who developed the framework studying common-pool resource and public goods governance from the 1960s until the 2000s. Ostrom’s overall goal was (b) to examine the hindering and enabling conditions for governance to guide the use and provision common goods towards sustainability outcomes. Her primary research questions (c) related to collective action theory, unpacking how and why people cooperate with each other or not. The field her work is embedded in (d) is an interdisciplinary mix between public policy, behavioral and institutional economics. Scholars who use Ostrom’s SESF today, carry this history with them and therefore position themselves, whether implicitly or explicitly, as part of this research landscape as systems thinkers and interdisciplinarians, even if they have other scholarly positions.
Frameworks are positioned within a theory of science. Understanding this positioning can guide scholars in comprehending how their engagement with frameworks contributes to the overall advancement of their field. To do this, taking a conceptual “step back” is necessary, to distinguish between different levels of theory in science. From the conceptually broadest to the most empirically specific, we can identify the following levels of theory: paradigms, frameworks, specific theories, models/archetypes and cases (Table 1 ). Knowledge production processes flow up and down these levels of theory. For example, as argued by Kuhn ( 1962 ), the purpose of a scientific field is to advance its paradigm. Thus, the study of empirical observations (e.g., case studies) — and the development of models or theories resulting from those data — are aimed at advancing the overarching paradigm. Such paradigms could be conservation, democracy, sustainable development or social-ecological systems.
There is a need to connect cases, models and specific theory up to the overall paradigms of a field to make aggregate knowledge gains. Here, the role of frameworks becomes more clear, as bridging tools that enable connections between levels of knowledge. From the top down, frameworks can specify paradigms with more tangible conceptual features and relationships, which can then guide empirical inquiry. For example, the Driver-Pressure-State-Impact-Response (DPSIR) framework (Smeets and Weterings 1999 ; Ness, Anderberg, and Olsson 2010 ) specifies how to evaluate policy options and their effects by focusing on the five embedded concepts in a relational order. Scholars can then generate more specific indicators and methods to measure the five specified features of the framework, and their relationships, to generate empirical insights that now have a direct link to the paradigm of sustainable policy development via the framework.
Furthermore, frameworks can also emerge from the bottom up, by distilling empirical data across cases and thus creating a knowledge bridge of more specified conceptual features and relationships that connect to a paradigm. In both top-down and bottom-up mechanism, frameworks can play a vital role in synthesizing and communicating ideas among scholars in a field — from empirical data to a paradigm. A challenge may be, however, that multiple frameworks have emerged attempting to specify the core conceptual features and relationships in a paradigm. A mature scientific field is likely to have many frameworks to guide research and debate. There is, however, a lack of research and tools available to compare frameworks and their added value.
Beyond their use as positioning tools, frameworks make day-to-day science easier. They can guide researchers in designing new empirical research by indicating which core concepts and relationships are of interest to be measured and compared. Scientific fields also need common fires to huddle around, meaning that we need reference points to initiate scholarly debates, coordinate disparate empirical efforts and to communicate findings and novel advancements through a common language (McGinnis and Ostrom 2014a ; Ban and Cox 2017 ). As such, frameworks are useful for synthesis research, focusing the attention of reviews and meta-analyses around core sets of concepts and relationships.
There is, however, a tension between frameworks that aim to capture complexity and those that aim to simplify core principles. Complexity oriented frameworks often advance systems thinking at the risk of including too many variables. They often have long lists of variables which makes empirical orientation and synthesis difficult. On the other hand, simplification frameworks face the challenge of leaving important things out, with the benefit of clarifying what may be important and giving clear direction.
From a more critical perspective, the “criteria for comparing frameworks are not well developed,” (Schlager, 2007 , 312), and the positionality of frameworks has not been rigorously explored outside of smaller studies. Nonetheless, numerous classifications or typologies of frameworks within specific fields have been suggested (Table 2 ), although not with reference to positionality (Spangenberg 2011 ; Binder et al. 2013 ; Cumming 2014 ; Schlager 2007 ; Ness et al. 2007 ; Potschin-Young et al. 2018 ; Cox et al. 2021 ; Louder et al. 2021 ; Chofreh and Goni 2017 ; Alaoui et al. 2022 ; Tapio and Willamo 2008 ). These studies point to the question of: what makes a good framework? Are there certain quality criteria that make some frameworks more useful than others? There has undoubtedly been a rise in the number of frameworks, but as expressed by Ban and Cox ( 2017 , 2), “it is not clear what the role of a scientific framework should be, and relatedly, what makes for a successful scientific framework. Although there are many frameworks […] there is little discussion on what their scientific role ought to be, other than providing a common scientific language.” The meta-framework presented below serves as a tool for answering these questions and provides guidance for developing and implementing frameworks in a range of settings.
Mechanisms of framework development and use: a meta-framework
This section presents a meta-framework detailing the mechanisms of framework development and use (Fig. 1 ). The meta-framework illustrates the role of frameworks as bridging tools for knowledge synthesis and communication. Therefore, the purpose of the meta-framework is to demonstrate how the mechanisms of framework development and use act as levers of knowledge flow across levels within a theory of science, doing so by enabling the communication and synthesis of knowledge. Introducing the meta-framework has two parts, outlined below.
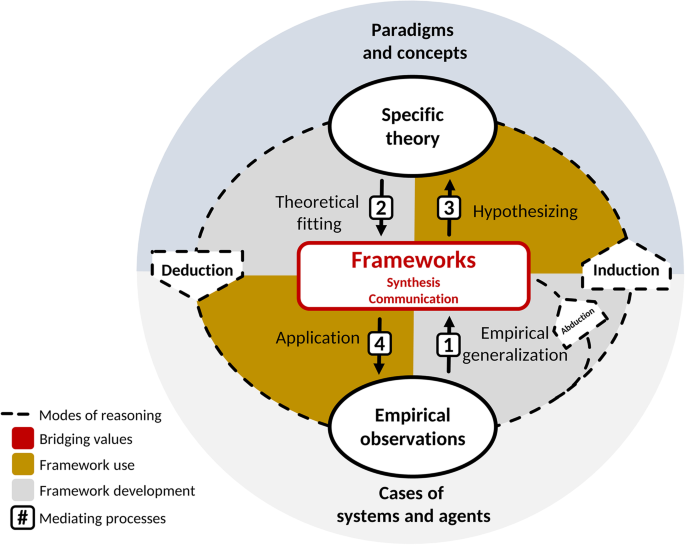
A meta-framework outlining the central role frameworks play in scientific advancement through their development and use. In the center, frameworks provide two core bridging values: knowledge synthesis and knowledge communication. Three modes of logical reasoning contribute to framework development: induction, deduction and abduction. Frameworks are used and developed through four mediating processes: (1) empirical generalization, (2) theoretical fitting, (3) application, and (4) hypothesizing
First, the meta-framework visualizes the levels along the scale of scientific theory including paradigms, frameworks, specific theory and empirical observations, introduced above. Along this scale, three mechanisms of logical reasoning are typical: induction, deduction, and abduction. Induction is a mode of logical reasoning based on sets of empirical observations, which, when patterns within those observations emerge, can inform more generalized theory formation. Induction, in its pure form, is reasoning without prior assumptions about what we think is happening. In contrast, deduction is a mode of logical reasoning based on testing a claim or hypothesis, often based on a body of theory, against an observation to infer whether or not a claim is true. In contrast to induction, which always leads to probable or fuzzy conclusions, deductive logic provides true or false conclusions. A third mode of logical reasoning is abduction. Abduction starts with a single or limited set of observations, and assumes the most likely cause as a conclusion. Abduction can only provide probable conclusions. Knowledge claims from all three modes of logical reasoning are part of the nexus of potential framework creation or modification.
Second, the meta-framework has four iterative mediating processes that directly enable the development and/or application of frameworks (Fig. 1 ). Two of the four mediating processes relate to framework development: (1) empirical generalization and (2) theoretical fitting. The other two relate to framework application: (3) hypothesizing, and (4) application (Fig. 1 , Table 3 ). The details of the specific mediating pathways are outlined in Table 3 , including the processes involved in each. There are numerous potential benefits and challenges associated with each (Table 3 ).
The value of a meta-framework
The presented meta-framework (Fig. 1 ) allows us to assess the values different frameworks can provide. If a framework provides a novel synthesis of key ideas or new developments in a field, and communicates those insights well in its composition, it likely adds notable value. If a framework coordinates scientific inquiry across the 1 or more of the four mediating processes, it likely acts as an important gatekeeper and boundary object for what may otherwise be disparate or tangential research. If it contributes substantial advances in 3 or 4 of the mediating processes, the value of the framework is likely higher.
The meta-framework can further help identify the positioning of framework such as the type of logical reasoning processes used to create it, as well as help clarify the role of a framework along the scale of knowledge production (i.e., from data to paradigm). It might be clear, for example, what paradigm or specific theory a framework contributes to. The meta-framework can add value by guiding the assessment of how frameworks fit into the bigger picture of knowledge contribution in their field. Furthermore, many scholars and practitioners are interested in developing new frameworks. The meta-framework outlines the mechanisms that can be considered in creating the framework as well as help developers of new frameworks communicate how their frameworks add value. For example, to link empirical data collection to theoretical work in their field.
The meta-framework can help compare frameworks, to assess strengths and weaknesses in terms of their positioning and knowledge production mechanisms. It can also help elucidate the need for, or value of, new frameworks. This challenge is noted by Cumming ( 2014 , 18) in the field of social-ecological systems, reflecting that “the tendency of researchers to develop “new” frameworks without fully explaining how they relate to other existing frameworks and what new elements they bring to the problem is another obvious reason for the lack of a single dominant, unifying framework.” To showcase such as comparison, two brief examples are provided. The first example features the Driver-Pressure-State-Impact-Response (DPSIR) framework developed by the European Environmental Agency (EEA) (Box 1 ) (Smeets and Weterings 1999 ; Ness, Anderberg, and Olsson 2010 ). The DPSIR framework exemplifies a framework developed from the top-down (theoretical fitting) approach, to better organize the policy goal and paradigm of environmental sustainability to the indicators collected by EU member states. The second example highlights the Social-Ecological Systems Framework (SESF) developed by Elinor Ostrom (Box 2 ) (Ostrom 2009 ; McGinnis and Ostrom 2014a ). The SESF exemplifies a framework developed from the bottom up (empirical generalization) to aggregate data into common variables to enable data standardization and comparison towards theory building to improve environmental governance. In the case examples (Box 1 ; Box 2 ), we can see the value of both frameworks from different perspectives. The examples briefly illustrate how the positionality of each framework dictates how others use them to produce knowledge towards a paradigm. In the case of the DPSIR framework, from the top-down towards a policy goal, and with the SESF, from the bottom-up towards a theoretical goal.
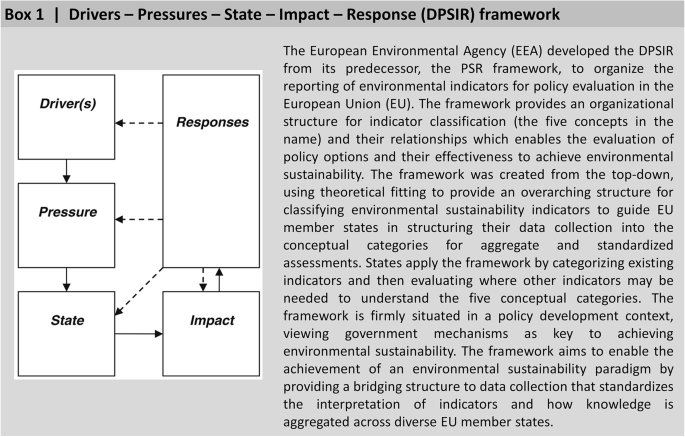
Drivers – Pressures – State – Impact - Response (DPSIR) framework
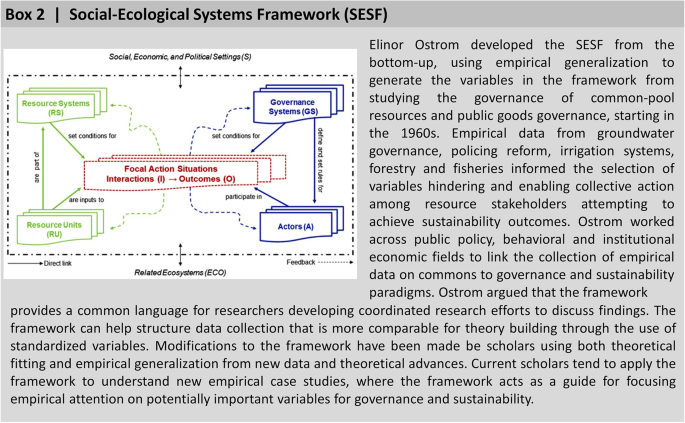
Social-Ecological Systems Framework (SESF)
Discussion and directions forward
Frameworks are commons objects to huddle around in academic and practitioner communities, providing identity and guiding our effort. They focus scholarly attention on important issues, stimulate cognitive energy and provide fodder for discussion. However, reflection on the role and purpose of the frameworks we use needs to be a more common practice in science. The proposed meta-framework aims to showcase the role of frameworks as boundary objects that connect ideas and concepts to data in constructive and actionable ways, enabling knowledge to be built up and aggregated within scientific fields through using common languages and concepts (Mollinga 2008 ; Klein 1996 ).
Boundary objects such as frameworks can be especially important for inter- and transdisciplinary collaboration, where there may be few prior shared points of conceptual understanding or terminology beyond a problem context. Mollinga ( 2008 , 33) reflects that “frameworks are typical examples of boundary objects, building connections between the worlds of science and that of policy, and between different knowledge domains,” and that “the development of frameworks is at present probably the most common strategy in the field of natural resources management to achieve integration and interdisciplinarity,” (Mollinga, 2008 , 31). They are, however, critically important for both disciplinary specific fundamental research, as well as for bridging science-society gaps through translating often esoteric academic concepts and findings into digestible and often visual objects. For example, the DPSIR framework (Box 1 ) attempts to better organize the analysis of environmental indicators for policy evaluation processes in the EU. Furthermore, Partelow et al., ( 2019 ) and Gurney et al., ( 2019 ) both use Ostrom’s SESF (Box 2 ) as a boundary object at the science-society interface to visually communicate systems thinking and social-ecological interactions to fishers and coastal stakeholders involved in local management decision-making.
An important feature of frameworks is that the very contestation over their nature is perhaps their main value. A framework can only be an effective boundary object if it catalyzes deliberation and scholarly debate — thus contestation over what it is and its value is seeded into the toolbox and identity of a scholarly field. Although most frameworks are likely to have shortcomings, flaws or controversial features, the fact that they motivate engagement around common problems and stimulate scholarly engagement is a value of its own. In doing so, frameworks often become symbols of individual and community identity in contested spaces. This is evidenced in how frameworks are often used to stamp our research as valid, relevant and important to the field, even if done passively. Citing a framework both communicates the general purpose of what a scholar is attempting to achieve to others, and orients science towards a common synthetic object for future knowledge synthesis and debate. These positioning actions are essential for science and practitioner communities to understand a research or policy project, its aims and assumptions. Historically, disciplines have provided this value – signaling the problems, methods and theories one is likely to engage with. Frameworks can act as tools for bridging disciplines, helping to catalyze interdisciplinary engagement (Mollinga 2008 ; Klein 1996 ). As many scientific communities shift focus towards solving real-world problems (e.g., climate change, gender equality), tools that can help scientists’ cooperate and communicate, such as a framework, will continue to play a vital role in achieving knowledge co-production goals.
Guiding points for framework engagement
An aim of this article is not only to reflect on the purpose, value and positioning of frameworks, but to provide some take-away advice for engaging with frameworks in current or future work. Over the course of this article, the question of “What makes a good framework?” has been explored. The meta-framework outlines mechanisms of useful frameworks and can help understand the positioning of frameworks. Nonetheless, more detailed guiding points can be specified for both the use and development of frameworks going forward. A series of guiding points are outlined in Table 4 , generated from the literature cited throughout this article, feedback from colleagues and personal experiences applying and developing numerous frameworks. The guiding points focus on the two types of mediating processes, framework development and use (Table 4 ).
In conclusion, we need to know our academic tools in order make the best use of them in our own research, practice and knowledge communities. Frameworks have gained substantial popularity for the communication and synthesis of academic ideas, and as tools we all have the ability to create and perhaps the responsibility to steward. However, frameworks have struggled to find roots in a theory of science which grounds their contributions in relation to other scientific tools such as models, specific theories and empirical data. There is also a lack of discussion about what makes a good framework and how to apply frameworks in a way to makes those applications of integrative value to an overall community of scholars positioned around it. The meta-framework provided in this article offers insights into how to understand the purpose and positionality of frameworks, as well as the mechanisms for understanding the creation and application of frameworks. The meta-framework further allows for the comparison of frameworks to assess their value.
Alaoui A, Barão L, Ferreira CSS, Hessel R (2022) An Overview of sustainability assessment frameworks in agriculture. Land 11(4):1–26. https://doi.org/10.3390/land11040537
Article Google Scholar
Ban NC, Cox M (2017) Advancing social-ecological research through teaching: summary, observations, and challenges. Ecol Soc 22(1):1–3
Biermann F, Betsill MM, Gupta J, Kanie N, Lebel L, Liverman D, Schroeder H, Siebenhüner B, Zondervan R (2010) Earth system governance: a research framework. Int Environ Agreem Polit 10(4):277–298. https://doi.org/10.1007/s10784-010-9137-3
Binder CR, Hinkel J, Bots PWG, Pahl-Wostl C (2013) Comparison of Frameworks for analyzing social-ecological systems. Ecol Soc 18(4):26. https://doi.org/10.5751/ES-05551-180426
Chofreh AG, Goni FA (2017) Review of frameworks for sustainability implementation. Sustain Dev 25(3):180–188. https://doi.org/10.1002/sd.1658
Colding J, Barthel S (2019) Exploring the social-ecological systems discourse 20 years later. Ecol Soc 24(1). https://doi.org/10.5751/es-10598-240102
Cox M, Gurney GG, Anderies JM, Coleman E, Darling E, Epstein G, Frey UJ (2021) Lessons learned from synthetic research projects based on the ostrom workshop frameworks. Ecol Soc 26(1)
Cox M, Villamayor-tomas S, Epstein G, Evans L, Ban NC, Fleischman F, Nenadovic M, Garcia-lopez G (2016) Synthesizing theories of natural resource management and governance. Glob Environ Chang 39(January):45–56. https://doi.org/10.1016/j.gloenvcha.2016.04.011
Cumming GS (2014) Theoretical Frameworks for the analysis of social-ecological systems. In: Sakai S, Umetsu C (eds) Social-Ecological Systems in Transition . Springer, Japan. https://doi.org/10.1007/978-4-431-54910-9
Chapter Google Scholar
Díaz S, Demissew S, Carabias J, Joly C, Lonsdale M, Ash N, Larigauderie A et al (2015) The IPBES conceptual framework - connecting nature and people. Curr Opin Environ Sustain 14:1–16. https://doi.org/10.1016/j.cosust.2014.11.002
Gurney GG, Darling ES, Jupiter SD, Mangubhai S, McClanahan TR, Lestari P, Pardede S et al (2019) Implementing a social-ecological systems framework for conservation monitoring: lessons from a multi-country coral reef program. Biol Conserv 240(August). https://doi.org/10.1016/j.biocon.2019.108298
Kuhn T (1962) The structure of scientific revolutions (University of Chicago Press)
Klein JT (1996) Crossing boundaries: knowledge, disciplinarities, and interdisciplinarities . University of Virginia Press, Charlottesville and London
Google Scholar
Louder E, Wyborn C, Cvitanovic C, Bednarek AT (2021) A Synthesis of the frameworks available to guide evaluations at the interface of environmental science on policy and practice. Environ Sci Policy 116(July 2020):1–27. https://doi.org/10.1016/j.envsci.2020.12.006
McGinnis MD, Ostrom E (2014a) Social-Ecological system framework: initial changes and continuing challenges. Ecol Soc 19(2). https://doi.org/10.5751/ES-06387-190230
Mollinga PP (2008) In: Evers H-D, Gerke S, Mollinga P, Schetter C (eds) The rational organisation of dissent: boundary concepts, boundary objects and boundary settings in the interdisciplinary Study of Natural Resources Management. University of Bonn, Bonn
Nagel B, Partelow S (2022) A methodological guide for applying the ses framework: a review of quantitative approaches. Ecol Soc
Ness B, Anderberg S, Olsson L (2010) Structuring problems in sustainability science: the multi-Level DPSIR framework. Geoforum 41(3):479–488. https://doi.org/10.1016/j.geoforum.2009.12.005
Ness B, Urbel-Piirsalu E, Anderberg S, Olsson L (2007) Categorising tools for sustainability assessment. Ecol Econ 60(3):498–508. https://doi.org/10.1016/j.ecolecon.2006.07.023
Ostrom E (2009) A general framework for analyzing sustainability of social-ecological systems. Science 325(5939):419–422. https://doi.org/10.1126/science.1172133
Article CAS Google Scholar
Partelow S (2018) A review of the social-ecological systems framework: applications, methods, modifications and challenges. Ecol Soc
Partelow S, Fujitani M, Soundararajan V, Schlüter A (2019) Transforming the social-ecological systems framework into a knowledge exchange and deliberation tool for comanagement. Ecol Soc 24(1). https://doi.org/10.5751/ES-10724-240115
Potschin-Young M, Haines-Young R, Görg C, Heink U, Jax K, Schleyer C (2018) Understanding the role of conceptual frameworks: reading the ecosystem service cascade. Ecosyst Serv. https://doi.org/10.1016/j.ecoser.2017.05.015
Pulver S, Ulibarri N, Sobocinski KL, Alexander SM, Johnson ML, Mccord PF (2018) Frontiers in socio-environmental research: components, connections, scale, and context. Ecol Soc 23(3)
Schlager E (2007) A Comparison of frameworks, theories, and models of policy processes. In: Sabatier PA (ed) Theories of the Policy Process , 1st edn. Routledge, pp 293–319
Smeets E, Weterings R (1999) Environmental indicators: typology and overview. In: Bosch P, Büchele M, Gee D (eds) European Environment Agency (EEA) , vol 50. European Environment Agency, Copenhagan
Spangenberg JH (2011) Sustainability Science: a review, an analysis and some empirical lessons. Environ Conserv 38(3):275–287. https://doi.org/10.1017/S0376892911000270
Tapio P, Willamo R (2008) Developing Interdisciplinary environmental frameworks. Ambio 37(2):125–133. https://doi.org/10.1579/0044-7447(2008)37[125:DIEF]2.0.CO;2
UN (2019) Independent Group of Scientists appointed by the Secretary-General, Global Sustainable Development Report 2019: The Future is Now – Science for Achieving Sustainable Development. United Nations, New York
Download references
Acknowledgements
I would like to thank Michael Cox and Achim Schlüter for their helpful feedback on previous versions of the manuscript and the ideas within it. I am grateful to the Leibniz Centre for Tropical Marine Research (ZMT) in Bremen, and the Center for Life Ethics at the University of Bonn for support.
Open Access funding enabled and organized by Projekt DEAL.
Author information
Authors and affiliations.
Leibniz Centre for Tropical Marine Research (ZMT), Bremen, Germany
Stefan Partelow
Center for Life Ethics, University of Bonn, Bonn, Germany
You can also search for this author in PubMed Google Scholar
Corresponding author
Correspondence to Stefan Partelow .
Ethics declarations
Competing interests.
The authors declares that he has no competing interests.
Additional information
Publisher's note.
Springer Nature remains neutral with regard to jurisdictional claims in published maps and institutional affiliations.
Rights and permissions
Open Access This article is licensed under a Creative Commons Attribution 4.0 International License, which permits use, sharing, adaptation, distribution and reproduction in any medium or format, as long as you give appropriate credit to the original author(s) and the source, provide a link to the Creative Commons licence, and indicate if changes were made. The images or other third party material in this article are included in the article's Creative Commons licence, unless indicated otherwise in a credit line to the material. If material is not included in the article's Creative Commons licence and your intended use is not permitted by statutory regulation or exceeds the permitted use, you will need to obtain permission directly from the copyright holder. To view a copy of this licence, visit http://creativecommons.org/licenses/by/4.0/ .
Reprints and permissions
About this article
Partelow, S. What is a framework? Understanding their purpose, value, development and use. J Environ Stud Sci 13 , 510–519 (2023). https://doi.org/10.1007/s13412-023-00833-w
Download citation
Accepted : 29 March 2023
Published : 14 April 2023
Issue Date : September 2023
DOI : https://doi.org/10.1007/s13412-023-00833-w
Share this article
Anyone you share the following link with will be able to read this content:
Sorry, a shareable link is not currently available for this article.
Provided by the Springer Nature SharedIt content-sharing initiative
- Theory of science
- Methodology
- Social science
- Find a journal
- Publish with us
- Track your research
Have a language expert improve your writing
Run a free plagiarism check in 10 minutes, automatically generate references for free.
- Knowledge Base
- Dissertation
- What Is a Thesis? | Ultimate Guide & Examples
What Is a Thesis? | Ultimate Guide & Examples
Published on 15 September 2022 by Tegan George . Revised on 5 December 2023.
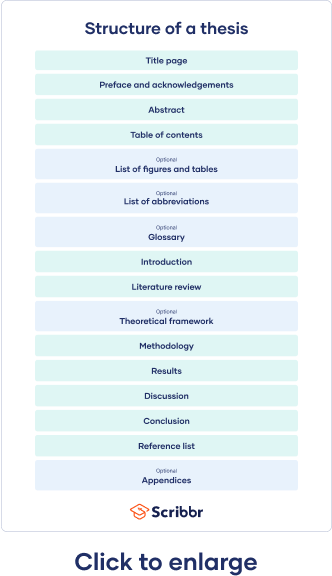
A thesis is a type of research paper based on your original research. It is usually submitted as the final step of a PhD program in the UK.
Writing a thesis can be a daunting experience. Indeed, alongside a dissertation , it is the longest piece of writing students typically complete. It relies on your ability to conduct research from start to finish: designing your research , collecting data , developing a robust analysis, drawing strong conclusions , and writing concisely .
Thesis template
You can also download our full thesis template in the format of your choice below. Our template includes a ready-made table of contents , as well as guidance for what each chapter should include. It’s easy to make it your own, and can help you get started.
Download Word template Download Google Docs template
Instantly correct all language mistakes in your text
Be assured that you'll submit flawless writing. Upload your document to correct all your mistakes.
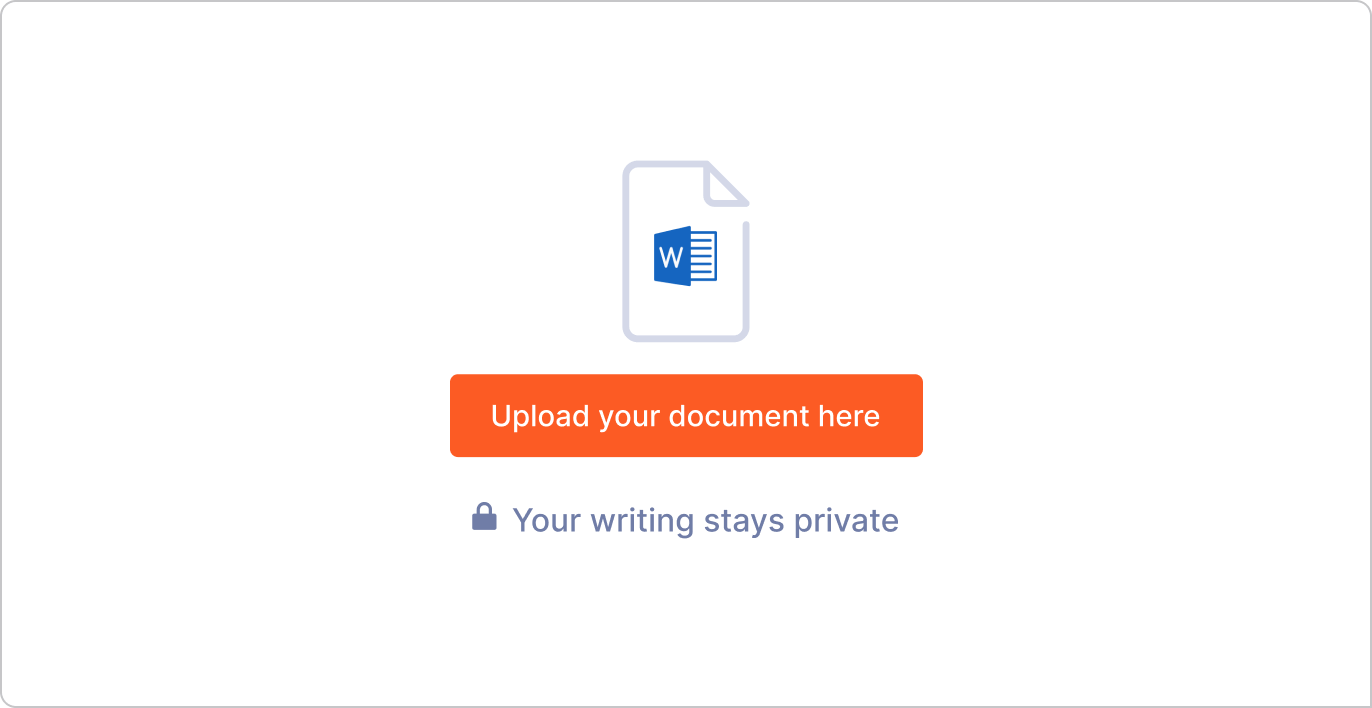
Table of contents
Thesis vs. thesis statement, how to structure a thesis, acknowledgements or preface, list of figures and tables, list of abbreviations, introduction, literature review, methodology, reference list, proofreading and editing, defending your thesis, frequently asked questions about theses.
You may have heard the word thesis as a standalone term or as a component of academic writing called a thesis statement . Keep in mind that these are two very different things.
- A thesis statement is a very common component of an essay, particularly in the humanities. It usually comprises 1 or 2 sentences in the introduction of your essay , and should clearly and concisely summarise the central points of your academic essay .
- A thesis is a long-form piece of academic writing, often taking more than a full semester to complete. It is generally a degree requirement to complete a PhD program.
- In many countries, particularly the UK, a dissertation is generally written at the bachelor’s or master’s level.
- In the US, a dissertation is generally written as a final step toward obtaining a PhD.
Prevent plagiarism, run a free check.
The final structure of your thesis depends on a variety of components, such as:
- Your discipline
- Your theoretical approach
Humanities theses are often structured more like a longer-form essay . Just like in an essay, you build an argument to support a central thesis.
In both hard and social sciences, theses typically include an introduction , literature review , methodology section , results section , discussion section , and conclusion section . These are each presented in their own dedicated section or chapter. In some cases, you might want to add an appendix .
Thesis examples
We’ve compiled a short list of thesis examples to help you get started.
- Example thesis #1: ‘Abolition, Africans, and Abstraction: the Influence of the “Noble Savage” on British and French Antislavery Thought, 1787-1807’ by Suchait Kahlon.
- Example thesis #2: ‘”A Starving Man Helping Another Starving Man”: UNRRA, India, and the Genesis of Global Relief, 1943-1947’ by Julian Saint Reiman.
The very first page of your thesis contains all necessary identifying information, including:
- Your full title
- Your full name
- Your department
- Your institution and degree program
- Your submission date.
Sometimes the title page also includes your student ID, the name of your supervisor, or the university’s logo. Check out your university’s guidelines if you’re not sure.
Read more about title pages
The acknowledgements section is usually optional. Its main point is to allow you to thank everyone who helped you in your thesis journey, such as supervisors, friends, or family. You can also choose to write a preface , but it’s typically one or the other, not both.
Read more about acknowledgements Read more about prefaces
The only proofreading tool specialized in correcting academic writing
The academic proofreading tool has been trained on 1000s of academic texts and by native English editors. Making it the most accurate and reliable proofreading tool for students.
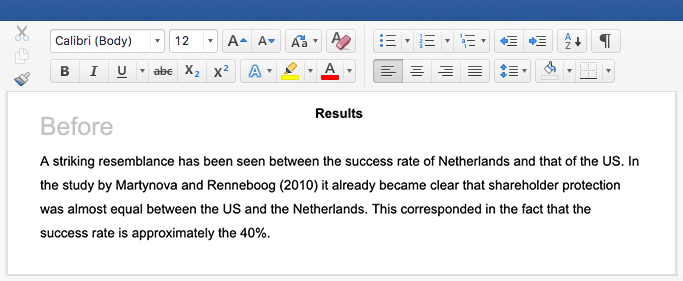
Correct my document today
An abstract is a short summary of your thesis. Usually a maximum of 300 words long, it’s should include brief descriptions of your research objectives , methods, results, and conclusions. Though it may seem short, it introduces your work to your audience, serving as a first impression of your thesis.
Read more about abstracts
A table of contents lists all of your sections, plus their corresponding page numbers and subheadings if you have them. This helps your reader seamlessly navigate your document.
Your table of contents should include all the major parts of your thesis. In particular, don’t forget the the appendices. If you used heading styles, it’s easy to generate an automatic table Microsoft Word.
Read more about tables of contents
While not mandatory, if you used a lot of tables and/or figures, it’s nice to include a list of them to help guide your reader. It’s also easy to generate one of these in Word: just use the ‘Insert Caption’ feature.
Read more about lists of figures and tables
If you have used a lot of industry- or field-specific abbreviations in your thesis, you should include them in an alphabetised list of abbreviations . This way, your readers can easily look up any meanings they aren’t familiar with.
Read more about lists of abbreviations
Relatedly, if you find yourself using a lot of very specialised or field-specific terms that may not be familiar to your reader, consider including a glossary . Alphabetise the terms you want to include with a brief definition.
Read more about glossaries
An introduction sets up the topic, purpose, and relevance of your thesis, as well as expectations for your reader. This should:
- Ground your research topic , sharing any background information your reader may need
- Define the scope of your work
- Introduce any existing research on your topic, situating your work within a broader problem or debate
- State your research question(s)
- Outline (briefly) how the remainder of your work will proceed
In other words, your introduction should clearly and concisely show your reader the “what, why, and how” of your research.
Read more about introductions
A literature review helps you gain a robust understanding of any extant academic work on your topic, encompassing:
- Selecting relevant sources
- Determining the credibility of your sources
- Critically evaluating each of your sources
- Drawing connections between sources, including any themes, patterns, conflicts, or gaps
A literature review is not merely a summary of existing work. Rather, your literature review should ultimately lead to a clear justification for your own research, perhaps via:
- Addressing a gap in the literature
- Building on existing knowledge to draw new conclusions
- Exploring a new theoretical or methodological approach
- Introducing a new solution to an unresolved problem
- Definitively advocating for one side of a theoretical debate
Read more about literature reviews
Theoretical framework
Your literature review can often form the basis for your theoretical framework, but these are not the same thing. A theoretical framework defines and analyses the concepts and theories that your research hinges on.
Read more about theoretical frameworks
Your methodology chapter shows your reader how you conducted your research. It should be written clearly and methodically, easily allowing your reader to critically assess the credibility of your argument. Furthermore, your methods section should convince your reader that your method was the best way to answer your research question.
A methodology section should generally include:
- Your overall approach ( quantitative vs. qualitative )
- Your research methods (e.g., a longitudinal study )
- Your data collection methods (e.g., interviews or a controlled experiment
- Any tools or materials you used (e.g., computer software)
- The data analysis methods you chose (e.g., statistical analysis , discourse analysis )
- A strong, but not defensive justification of your methods
Read more about methodology sections
Your results section should highlight what your methodology discovered. These two sections work in tandem, but shouldn’t repeat each other. While your results section can include hypotheses or themes, don’t include any speculation or new arguments here.
Your results section should:
- State each (relevant) result with any (relevant) descriptive statistics (e.g., mean , standard deviation ) and inferential statistics (e.g., test statistics , p values )
- Explain how each result relates to the research question
- Determine whether the hypothesis was supported
Additional data (like raw numbers or interview transcripts ) can be included as an appendix . You can include tables and figures, but only if they help the reader better understand your results.
Read more about results sections
Your discussion section is where you can interpret your results in detail. Did they meet your expectations? How well do they fit within the framework that you built? You can refer back to any relevant source material to situate your results within your field, but leave most of that analysis in your literature review.
For any unexpected results, offer explanations or alternative interpretations of your data.
Read more about discussion sections
Your thesis conclusion should concisely answer your main research question. It should leave your reader with an ultra-clear understanding of your central argument, and emphasise what your research specifically has contributed to your field.
Why does your research matter? What recommendations for future research do you have? Lastly, wrap up your work with any concluding remarks.
Read more about conclusions
In order to avoid plagiarism , don’t forget to include a full reference list at the end of your thesis, citing the sources that you used. Choose one citation style and follow it consistently throughout your thesis, taking note of the formatting requirements of each style.
Which style you choose is often set by your department or your field, but common styles include MLA , Chicago , and APA.
Create APA citations Create MLA citations
In order to stay clear and concise, your thesis should include the most essential information needed to answer your research question. However, chances are you have many contributing documents, like interview transcripts or survey questions . These can be added as appendices , to save space in the main body.
Read more about appendices
Once you’re done writing, the next part of your editing process begins. Leave plenty of time for proofreading and editing prior to submission. Nothing looks worse than grammar mistakes or sloppy spelling errors!
Consider using a professional thesis editing service to make sure your final project is perfect.
Once you’ve submitted your final product, it’s common practice to have a thesis defense, an oral component of your finished work. This is scheduled by your advisor or committee, and usually entails a presentation and Q&A session.
After your defense, your committee will meet to determine if you deserve any departmental honors or accolades. However, keep in mind that defenses are usually just a formality. If there are any serious issues with your work, these should be resolved with your advisor way before a defense.
The conclusion of your thesis or dissertation shouldn’t take up more than 5-7% of your overall word count.
When you mention different chapters within your text, it’s considered best to use Roman numerals for most citation styles. However, the most important thing here is to remain consistent whenever using numbers in your dissertation .
If you only used a few abbreviations in your thesis or dissertation, you don’t necessarily need to include a list of abbreviations .
If your abbreviations are numerous, or if you think they won’t be known to your audience, it’s never a bad idea to add one. They can also improve readability, minimising confusion about abbreviations unfamiliar to your reader.
A thesis or dissertation outline is one of the most critical first steps in your writing process. It helps you to lay out and organise your ideas and can provide you with a roadmap for deciding what kind of research you’d like to undertake.
Generally, an outline contains information on the different sections included in your thesis or dissertation, such as:
- Your anticipated title
- Your abstract
- Your chapters (sometimes subdivided into further topics like literature review, research methods, avenues for future research, etc.)
Cite this Scribbr article
If you want to cite this source, you can copy and paste the citation or click the ‘Cite this Scribbr article’ button to automatically add the citation to our free Reference Generator.
George, T. (2023, December 05). What Is a Thesis? | Ultimate Guide & Examples. Scribbr. Retrieved 2 April 2024, from https://www.scribbr.co.uk/thesis-dissertation/thesis-ultimate-guide/
Is this article helpful?
Tegan George
Other students also liked, dissertation & thesis outline | example & free templates, how to write a thesis or dissertation conclusion, how to write a thesis or dissertation introduction.
- Open access
- Published: 26 September 2023
Deep learning-based lung sound analysis for intelligent stethoscope
- Dong-Min Huang 1 ,
- Jia Huang 2 ,
- Kun Qiao 2 ,
- Nan-Shan Zhong 3 ,
- Hong-Zhou Lu 2 &
- Wen-Jin Wang 1
Military Medical Research volume 10 , Article number: 44 ( 2023 ) Cite this article
5060 Accesses
3 Citations
3 Altmetric
Metrics details
Auscultation is crucial for the diagnosis of respiratory system diseases. However, traditional stethoscopes have inherent limitations, such as inter-listener variability and subjectivity, and they cannot record respiratory sounds for offline/retrospective diagnosis or remote prescriptions in telemedicine. The emergence of digital stethoscopes has overcome these limitations by allowing physicians to store and share respiratory sounds for consultation and education. On this basis, machine learning, particularly deep learning, enables the fully-automatic analysis of lung sounds that may pave the way for intelligent stethoscopes. This review thus aims to provide a comprehensive overview of deep learning algorithms used for lung sound analysis to emphasize the significance of artificial intelligence (AI) in this field. We focus on each component of deep learning-based lung sound analysis systems, including the task categories, public datasets, denoising methods, and, most importantly, existing deep learning methods, i.e., the state-of-the-art approaches to convert lung sounds into two-dimensional (2D) spectrograms and use convolutional neural networks for the end-to-end recognition of respiratory diseases or abnormal lung sounds. Additionally, this review highlights current challenges in this field, including the variety of devices, noise sensitivity, and poor interpretability of deep models. To address the poor reproducibility and variety of deep learning in this field, this review also provides a scalable and flexible open-source framework that aims to standardize the algorithmic workflow and provide a solid basis for replication and future extension: https://github.com/contactless-healthcare/Deep-Learning-for-Lung-Sound-Analysis .
Lung disease has been a leading cause of mortality worldwide for many years, especially since the onset of corona virus disease 2019 (COVID-19) [ 1 , 2 , 3 ]. Various clinical methods have been developed to diagnose and evaluate lung health conditions, including computed tomographic scans, chest X-rays, and pulmonary function tests (PFTs) [ 4 , 5 ]. However, these methods are often limited to high-end clinics due to their complexity and high costs [ 6 ]. In contrast, auscultation offers a non-invasive, low-cost, and portable way of working where paramedics use a conventional acoustic stethoscope to diagnose lung diseases, including asthma, chronic obstructive pulmonary disease (COPD), and pneumonia [ 7 , 8 , 9 ], based on the patient's lung sound.
Although the stethoscope has been widely used in clinics, it has several associated challenges. First, the interpretation of lung sounds requires a trained paramedic, limiting stethoscope use in low-resource areas [ 10 ]. Second, the medical-decisions made based on auscultation are subject to inter-listener variability in proficiency [ 11 ]. The subjectivity of the diagnosis is further amplified by the lack of a recording function in the conventional stethoscope that prevents other personnel from analyzing the sounds heard during the consultation [ 12 ]. These challenges need to be resolved to improve the quality and efficiency of lung disease diagnosis.
To this end, the digital stethoscope has been developed to record lung sounds by digitizing acoustic signals [ 13 ]. It enables the visualization and retrospective analysis of lung sounds. In addition, wireless transmission (e.g., Bluetooth or WiFi) allows it to be used for remote diagnosis, further increasing the convenience of application [ 14 , 15 , 16 ]. The emergence of digital stethoscopes combined with related physics study [ 17 ] has contributed to our understanding of lung sounds including, their production, transmission, and characteristics under healthy and pathological conditions [ 18 ].
Based on this understanding, the recognition of lung sound patterns using machine learning has been achieved, providing an objective and quantitative method for lung health assessment [ 19 ]. Earlier studies focused on the feature engineering of lung sounds and exploitation of shallow machine learning tools for abnormal lung sound detection [ 20 ]. Zhang et al. [ 21 ] conducted a clinical trial showing that support vector machine (SVM)-based diagnosis performed better than general pediatricians in abnormal lung sound detection, achieving an accuracy of 77.7% and 59.9% for crackles and wheezes, respectively. This demonstrates the potential of machine learning in intelligent lung sound recognition.
More recently, deep learning-based models were proposed to detect the patterns related to lung diseases and distinguish abnormal lung sounds from normal ones and have shown promising performance [ 22 ]. Compared with shallow machine learning, most deep learning-based methods adopt an end-to-end learning approach to automatically learn the representation of lung sounds from raw acoustic signals without the need for handcrafted feature engineering. They can also leverage transfer learning to increase the adaptability of the learned models in new environments, which reduces the amount of data needed for training [ 23 , 24 ]. It is important for clinical applications due to the difficulty of acquiring a large amount of patient data. Pham et al. [ 25 ] applied convolutional neural networks (CNNs) to learn temporal-frequency information from spectrograms, and achieved 89% specificity and 82% sensitivity in normal and abnormal lung sound classification. Perna et al. [ 26 ] used recurrent neural networks (RNNs) to mine the context information of lung sounds over time, obtaining an accuracy of 99% in recognizing COPD patients. In addition, Altan et al. [ 27 ] proposed a deep belief network-based model combined with a three-dimensional (3D)-second order difference plot of lung sound signals to distinguish the severity of COPD patients. These methods demonstrate the feasibility of implementing deep learning-based intelligent stethoscopes that can automate the detection of pulmonary disease and its severity. Moreover, deep learning-based quantitative results overcome the disadvantages of subjective auscultation diagnosis caused by inter-listener difference and the need for clinical proficiency, thus supporting medical diagnosis and treatment. Thus, deep learning-based approaches can significantly improve the quality of healthcare in underdeveloped countries with limited clinical resources; examples of their applications include community-acquired pneumonia detection and the domiciliary management of COPD.
To increase the understanding of deep learning-based lung sound analysis, in this paper, we systematically review deep learning methods proposed for lung sound analysis. This review, organized as shown in Fig. 1 , outlines the system of lung sound analysis, including the pathological fundamentals of lung sounds, existing digital stethoscopes, and deep learning-based methods. The fundamentals of lung sounds guide and motivate the design of reasonable deep learning methods, and in turn, the application of digital stethoscope-based deep learning methods verifies the understanding of observations. In contrast to previous reviews [ 6 , 19 , 28 , 29 , 30 , 31 ], this paper emphasizes the applications of deep learning-based lung sound analysis, including the system framework, basic model selection, and the advancement of deep methods in respiratory medical tasks, also highlighting the challenges that need to be overcome. The main contributions of this review are as follows: (1) It provides an in-depth review of the fundamentals of lung sounds under normal and pathological conditions that motivates the design of deep-learning models and guides the design of signal processing algorithms (spectrograms, typical signatures, and their definitions); (2) It provides a thorough overview of the algorithmic framework of deep learning-based lung sound analysis, with a detailed introduction to each processing step, including the pros and cons of deep models and challenges they face; and (3) It provides a unified open-source deep learning-based framework that aims to standardize algorithmic components and establish a strong base that facilitates replication, benchmarking, and future extension.
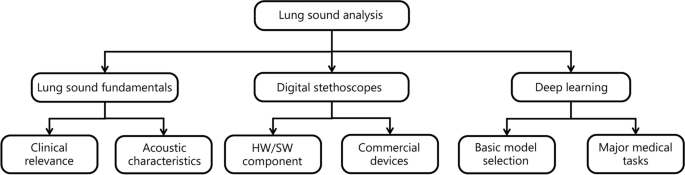
An overview of deep learning in lung sound analysis. The fundamentals of lung sounds include clinically relevant knowledge and its acoustic characteristic, which guides and motivates the design of the digital stethoscope in hardware and software. In turn, the application of digital stethoscope-based deep learning methods verifies the understanding of observations
The remainder of this paper is structured as follows. First, the fundamentals of lung sounds are presented. Then, the existing digital and wireless stethoscopes that can be used for clinical purposes are described, followed by an overview of the framework of deep learning in lung sound analysis including the main tasks, preprocessing, public datasets, and related research. Furthermore, an open-source framework for deep learning-based lung sound analysis is introduced. Finally, the conclusions of this review are presented.
Fundamentals of lung sounds
This section provides an overview of lung sound to improve our understanding of its definitions, as summarized in Table 1 , which is important for designing and implementing methods for lung sound analysis.
Lung sound, also termed respiratory sound, can be categorized into two types according to the health condition: (1) normal lung sound, which refers to the sounds generated by the airflow passing through the healthy respiratory system [ 32 ]; (2) abnormal lung sound, which is generally caused by lung diseases, exemplified by the presence of additional sounds overlaying the normal lung sound, the absence or reduction of normal lung sound, and asymmetry between left and right lung sounds [ 28 ]. Figure 2 portrays these separately.
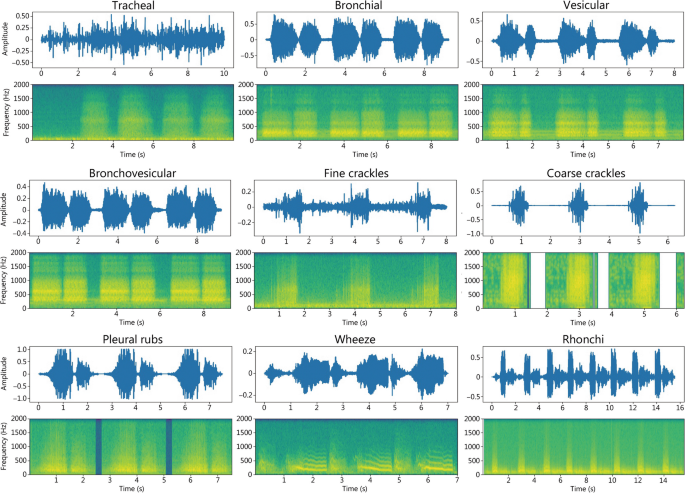
Lung sound demo. In each example, the upper panel shows the acoustic signal and the lower panel shows the corresponding spectrogram
Normal lung sound
Normal lung sound mostly consists of tracheal, bronchial, vesicular, and bronchovesicular sounds [ 33 ]. The differences between regarding the mechanism of generation, auscultation location, appearance timing, and acoustic characteristics are shown in Table 1 .
Tracheal sound is produced by the turbulent airflow passing the tracheal tissues of the respiratory system [ 34 ]. When auscultation is carried out over the trachea, particularly above the sternum, this sound can be heard clearly during both the inspiratory and expiratory phases. The tracheal sound lasts for a similar duration in both phases, and the pause between the two phases is obvious [ 35 ]. Since its transport occurs in the straighter part of the trachea with a larger diameter, the tracheal sound is typically high-pitched, hollow, non-musical, harsh, and louder than other normal lung sounds [ 36 , 37 ]. The normal tracheal sound has a wide energy distribution of 100–5000 Hz, and the energy usually drops at 800 Hz [ 38 ].
Bronchial sound is generated by the airflow traversing from the trachea to the main airways, and can usually be heard near the second and third intercostal spaces [ 37 ]. Like the tracheal sound, it appears in both phases but mainly in the expiratory phase, twice as long as in the inspiratory phase [ 39 ]. In general, the bronchial sound is generally soft, non-musical, loud, high-pitched, and tubular, with a similar frequency energy distribution as the tracheal sound [ 28 , 40 ].
Vesicular sound is created by the airflow passing through the smaller airways and alveoli (tiny air sacs) in the lungs [ 41 ]. It is audible in most of the lung fields across the whole inspiration phase and the early expiration phase [ 35 , 42 , 43 ]. The vesicular sound is typically soft, non-musical, and low-pitched and its frequency range is from below 100–1000 Hz with an energy drop at 200 Hz [ 40 , 44 ].
Bronchovesicular sound can be heard between the scapulae in the posterior chest, and in the central region of the anterior chest [ 40 ]. It has a similar duration in the expiratory and inspiratory phases [ 39 ]. In sound analysis, the bronchovesicular sound is softer than the bronchial sound but approximates the tubular sound, similar to the sound between the bronchial and vesicular sounds. Additionally, the frequency band of bronchovesicular sounds is between that of vesicular and bronchial sounds [ 44 ].
Abnormal lung sound
Abnormal lung sounds can be distinguished as discontinuous and continuous abnormal sounds according to their acoustic properties. The former has a shorter duration of less than 25 ms including fine crackle, coarse crackle, and pleural rub, whereas the latter typically has a longer duration of more than 250 ms [ 28 ], including wheeze, rhonchi, and stridor. Table 1 presents a description of these lung sounds in terms of their causes, appearance timing, clinical characteristics, acoustic characteristics, and the associated diseases.
Fine crackle arises due to the explosive opening of small airways or alveoli that were previously collapsed or closed [ 45 ]. It is commonly audible in mid-to-late inspiration and sometimes in the expiration phase, changing or disappearing with the body position [ 35 ]. Clinical study has reported that fine crackle is caused by several diseases, such as interstitial lung fibrosis and pneumonia [ 35 ]. It can be used as a biomarker for detecting specific diseases such as idiopathic pulmonary fibrosis and asbestosis, showing good sensitivity and specificity [ 46 ]. Fine crackle presents as high-pitched (close to 650 Hz), non-musical, and explosive, with a duration of nearly 5 ms [ 47 ].
Coarse crackle is probably caused by air bubbles in larger airways that open and close intermittently [ 48 ]. Upon auscultation, it can be heard in both phases, mostly in the early inspiratory phase [ 49 ]. Due to intermittent airway opening, it is associated with some obstructive diseases, for example, COPD, bronchiectasis, and asthma [ 28 , 50 ]. In contrast to fine crackle, coarse crackle is low-pitched (close to 350 Hz) and has an approximative duration of 15 ms [ 51 ].
Pleural rub is generated by the rubbing of the pleural membranes against each other and is relevant to pleural inflammation and pleural tumors [ 35 ]. It is typically biphasic with the expiratory sequence of sounds mirroring the inspiratory sequence [ 37 ]. Pleural rub is non-musical, rhythmic, and low-pitched (< 350 Hz). Its duration is longer than 15 ms.
Wheeze is produced by airflow limitations due to airway narrowing and is normally detected in both phases, mostly in the expiration phase [ 52 ]. Wheezing sounds are typically caused in asthma and COPD, possibly by a foreign body (e.g., a tumor) blocking the airway [ 35 ]. In general, wheeze is musical, sibilant, and high-pitched (more than 100 Hz). Its duration is generally more than 80 ms [ 53 ].
Rhonchi are related to the thickening of secretions in the bronchial tree and can be heard mostly in the expiration phase and sometimes in the inspiratory phase. Rhonchi are reported to be associated with bronchitis and COPD [ 35 ]. The acoustic characteristics of rhonchi are similar to those of wheeze sounds but with a relatively low pitch (< 200 Hz) [ 53 ].
Stridor is created by the turbulent airflow in the bronchial tree, which is relevant to upper airway obstruction. Upon auscultation, it can be detected mostly in the inspiration phase, but in certain situations, it can be heard in both phases [ 28 ]. Diseases related to upper airway obstruction may cause stridor, including croup and laryngeal edema. Stridor is a sibilant and musical sound that has a high pitch above 500 Hz with a duration longer than 250 ms.
Digital stethoscopes
For deep learning-based lung sound analysis, the data acquisition process depends on digital stethoscopes that record the lung sound by converting acoustic waves into electrical signals. Thus, this section focuses on digital stethoscopes currently available in the market and widely used in clinics, with an emphasis on their limitations and potential directions for improvement.
Implementation of digital stethoscopes
A digital stethoscope generally consists of a diaphragm, sensor, pre-amplifier, microcontroller, and transmission module [ 54 , 55 ], as shown in Fig. 3 . Its workflow is as follows in Fig. 3 a, b: first, the diaphragm is placed on the chest piece to capture the sound wave of the internal body [ 56 ]. Then, either piezoelectric sensors or electret microphones are commonly used to convert the sound waves into electrical signals [ 57 , 58 ]. The pre-amplifier enhances the extremely weak acoustic signal that is picked up by the sensor [ 59 ]. Next, the microcontroller processes the amplified signal, which includes controlling the audio processing circuitry and managing the user interface and display. Finally, under the control of the microcontroller, the transmission module (e.g., Bluetooth), transmits data to the terminals in a lossless way as far as possible [ 60 , 61 ].
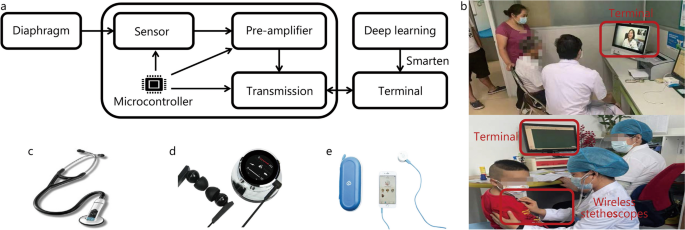
Digital stethoscopes. a Implementation of wireless stethoscopes; b Telemedicine; c 3 M LITTMAN 3200; d Thinklabs; e Clinicloud
Available digital stethoscopes
Here, we focus on digital stethoscopes that have been used as clinical devices, including 3 M LITTMAN 3200, Thinklabs digital stethoscope, and Clinicloud digital stethoscope, as shown in Fig. 3 c–e.
3M LITTMAN 3200
The most popular stethoscope, it amplifies 24 times for acoustic signals with a denoised module and offers a mobile applications system for lung health management. A clinical trial showed that the diagnostic accuracy of medical interns was improved upon using LITTMAN 3200 compared to the traditional acoustic stethoscope [ 62 ]. Some studies also used machine learning to automatically detect abnormal lung sounds and diagnose lung diseases in offline clinical studies, wherein the 3M LITTMAN 3200 was applied to collect and transmit lung sounds [ 10 , 63 , 64 ].
Thinklabs digital stethoscope
This is a tube-free device that can amplify acoustic signals 100-fold, remove noises that have different frequency bands by using multiple frequency filters, and provide a mobile APP. This stethoscope has been clinically investigated for pneumonia detection [ 65 ] and the analysis of the frequency characteristics of normal lung sounds [ 66 ].
Clinicloud digital stethoscope
This stethoscope has been designed without the function of signal amplification. It was used in a clinical trial at Melbourne Hospital and showed accurate abnormal sound detection (ASD) in children [ 67 ].
Limitations and future improvements
Although the abovementioned stethoscopes are capable of recording and transmitting lung sounds, they still face some challenges. First, the high price of existing digital stethoscopes limits their scope of application in low-resource areas. Such areas desperately need low-cost and easy-to-operate medical devices since they cannot afford expensive equipment and manpower. Second, the available commercial digital stethoscopes are single-channel devices, making it difficult to monitor the left and right lungs synchronously. The diagnostic accuracy of single-channel devices can be improved by extending them to multiple channels [ 68 , 69 , 70 ]. Third, the difference in sound quality between these stethoscopes may cause deviations in the performance of algorithms for lung sound analysis [ 71 ]. Gairola et al. [ 72 ] performed device-based fine-tuning to improve the quality of detection; however, it is not practical to tune all these devices.
To solve these challenges, future research should focus on the implementation of low-cost and highly-reliable digital stethoscopes. Specifically, the development of each component of the device can facilitate this goal. For example, the expensive commercial diaphragm can be replaced with 3D-printed materials [ 73 ]. For signal transmission, the lung sound signal can be transmitted by matured technologies such as Bluetooth Low Energy [ 74 ] and Zigbee [ 75 ], allowing stethoscopes to be a part of the Internet of Medical Things to provide more comprehensive lung health assessments [ 76 ]. Furthermore, the development of wearable devices is also conducive to all-weather lung health monitoring. Meanwhile, the endurance and intelligence of digital stethoscopes need to be improved by introducing new technologies regarding the battery, processor, and embedded algorithms to cope with medical situations in low-resource areas.
Deep learning in lung sound analysis
This section reviews deep learning studies for lung sound analysis including the system framework, common datasets, preprocessing, feature extraction, and deep learning methods designed for different medical tasks, as shown in Fig. 4 .
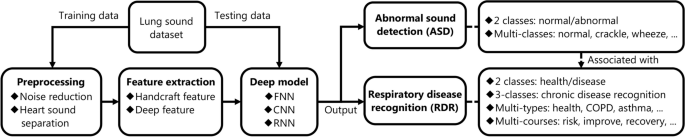
Deep learning-based framework for lung sound analysis. For two different medical tasks (ASD and RDR), the training set is used to construct the model including the steps of preprocessing, feature extraction, model selection. Finally, the test set is used to evaluate the performance of model. FNN fully connected neural network, CNN convolutional neural network, RNN recurrent neural network, COPD chronic obstructive pulmonary disease
System framework
Clinically, auscultation results depend on the doctor's interpretations of lung sounds, which are often subjective based on the proficiency of the listener. As a result, the clinical decisions made for the same patient may vary between physicians, promoting misdiagnosis and missed diagnosis. To solve this issue, machine learning methods (SVM, CNN, and random under-sampling boosting) have been proposed in different clinical contexts to provide quantitative and objective results on different types and degrees of lung disease [ 21 , 77 , 78 ]. However, most shallow machine learning-based lung sound analysis methods were evaluated based on a self-collected dataset of only a few subjects that was saturated at a low accuracy of approximately 80% [ 79 , 80 , 81 ].
Recently, deep learning has shown great potential in lung sound analysis, with a more accurate and robust performance compared with shallow machine learning [ 82 ]. Its improved performance may be attributed to the following features. (1) Representation: deep learning methods automatically learn task-relevant features in a data-driven manner without the need for manual feature engineering, and the learned features can capture complex patterns and structures in the raw data [ 22 ]; (2) Context information: deep learning methods show the advantages of capturing temporal context information, such as RNNs, which is significant for lung sound analysis in mining periodic lung sound changes caused by disease [ 26 ]; (3) Transfer learning: deep learning methods can use the common knowledge shared with related fields (e.g., AudioSet [ 83 ], a large audio dataset) to improve lung sound analysis, which reduces the amount of data required for training [ 24 ]. This property is significant for clinical applications since clinical data are often scarce due to the challenge of organizing clinical trials.
Generally, most deep learning-based lung sound analyses follow the paradigm of sequentially executing data acquisition and preprocessing, feature extraction, and classification. First, a digital stethoscope is used to collect lung sound data, following which preprocessing is applied to suppress environmental noise in the recorded lung sound signals. Thereafter, feature extraction is used to convert high-dimensional preprocessed lung sound data into a lower-dimensional space to obtain a more discriminative representation. Finally, the classifier is designed to create a mapping between the features and classes of relevant diseases.
Datasets for lung sound analysis
To evaluate performance, many deep learning-based lung sound analysis methods were benchmarked on public datasets for a fair comparison. The public lung sound datasets [ 84 , 85 , 86 , 87 , 88 ] are summarized in Table 2 . The most widely used dataset is the ICBHI 2017 Respiratory Sound Database [ 84 ] which consists of 920 recordings from 126 subjects who were diagnosed with respiratory pathological conditions, such as pneumonia, bronchiectasis, bronchiolitis, and COPD. Those recordings had different sampling rates (e.g., 4000 Hz, 10,000 Hz, and 44,100 Hz) and their duration ranged from 10 to 90 s. For annotation, the medical teams labeled the beginning and end of the breathing cycles in each recording as well as the presence/absence of crackles and wheezes. This dataset collected 6898 breath cycles, with 3642 normal cycles, 1864 with crackles, 886 with wheezes, and 506 with both, where the cycle duration of all recordings varied from 0.2 to 16 s, with a mean duration of 2.7 s.
Recently, many new datasets have emerged for lung sound analysis. Fraiwan et al. [ 85 ] collected 112 lung sound recordings from 112 subjects who were healthy or diagnosed with asthma, pneumonia, COPD, bronchitis, heart failure, lung fibrosis, and pleural effusion. Each recording was annotated according to the different lung sound events, including normal, inspiratory, expiratory, crepitations, crackles, and wheezes. Hsu et al. [ 86 ] proposed a new dataset called HF_Lung_V1, which consists of 9765 lung sound recordings with a duration of 15 s from 261 subjects. These recordings were collected using a single-channel device (3 M LITTMAN 3200) and a multi-channel device (self-customized device, HF-Type-1). HF_Lung_V1 marked 34,095 inspiratory segments, 18,349 expiratory segments, 13,883 continuous adventitious sound segments, and 15,606 discontinuous adventitious sound segments. Moreover, Hsu et al. [ 87 ] collected lung sounds from 42 new subjects to expand HF_Lung_V1 into a new dataset, namely HF_Lung_V2. More details about these public datasets are given in Table 2 .
In addition, the need for the management of chronic pulmonary disease like COPD has also gradually attracted the attention of clinicians and researchers [ 89 ], where the assessment of disease severity is a prerequisite for determining medical interventions [ 90 ]. Altan et al. [ 88 ] released a dataset called RespiratoryDatabase@TR that collected lung sounds from patients diagnosed with asthma, bronchitis, and different severities of COPD (0–5). In the trial, each subject underwent the examinations of chest X-rays, PFTs, and cardiopulmonary auscultation. The resulting dataset consists of 77 recordings from 77 subjects, with each recording sampled at 4000 Hz and containing 4 channels of heart sounds and 12 channels of lung sounds. For annotation, two pulmonologists validated and labeled the sound records as murmur, crackle, or wheezing, with reference to the gold standards of chest X-rays and PFTs. RespiratoryDatabase@TR has been widely used to assess the severity of COPD [ 27 , 91 , 92 ].
Data acquisition and preprocessing
In the clinical procedure for acquiring lung sound data, the digital stethoscope should be placed on specific parts of the thoracic surface for certain durations (e.g., 15 s, 30 s, or even longer) to depict the overall lung condition. As shown in Fig. 5 , the monitoring of the superior lung lobe requires the digital stethoscope to be placed on both the left and right second intercostal spaces on the anterior chest, along with the suprascapular region at the equivalent horizontal level. The fourth intercostal space and the interscapular region are correspondingly affiliated with the superior lobe of the left lung (the lingular segment) and the middle lobe of the right lung. To assess the inferior lobes of the lung, auscultation should be performed on the left and right eighth intercostal spaces as well as the infrascapular region. Through this process, the lung sound data from the audio recorded by the stethoscope are extracted in the form of electrical signals. However, since lung sound is fragile to environmental noise and the disturbance caused by internal heartbeat sounds, it is necessary to preprocess the raw recordings to ensure that lung sound is the dominant component of the recordings [ 93 ]. According to the different noise sources, the preprocessing can be subdivided into two types, namely external noise reduction and heart sound separation.
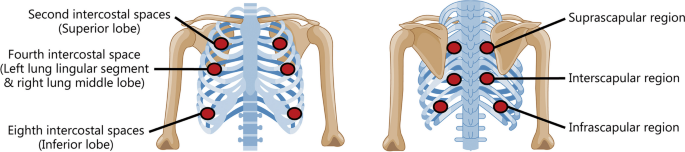
Auscultation sites. The red dots indicate auscultation. Typically, doctors monitor the lungs in a symmetrical way, up and down
External noise reduction methods are generally based on three different technologies. (1) Filter-based: this technology has the ability to quickly process a large amount of data but it is difficult to remove noise, with frequency information overlapping with lung sounds [ 94 , 95 , 96 ]; (2) Wavelet-based: this can decompose the mixed signal based on its time–frequency information to obtain the denoised signal; however, its denoising effect is easily affected by the selection in the wavelet basis function and threshold function [ 97 , 98 , 99 ]; (3) Empirical mode decomposition (EMD) based: this eliminates different types of noise in the audio signal but requires high computational complexity and reasonable parameter selection [ 100 , 101 ]. For example, Meng et al. [ 102 ] decomposed the noisy signal into seven sub-signals using wavelet decomposition and located the position of the lung sound in each sub-signal using autocorrelation coefficients to extract the effective lung sound components. Haider et al. [ 103 ] used EMD to decompose the noisy signal and integrated Hurst analysis for intrinsic mode function (IMF) selection to reduce the noise from the lung sound recording. Based on prior knowledge of lung sound signals, Emmanouilidou et al. [ 11 ] processed the noisy signal in short-time windows and used the current frame’s signal-to-noise information to dynamically extract the interested components of lung sound.
To separate the lung sound and heart sound, various methods have been proposed based on blind source separation (BSS), such as filter-based methods, independent component analysis (ICA), wavelet-based methods, and non-negative matrix factorization (NMF) [ 104 , 105 , 106 , 107 , 108 , 109 ]. Grooby et al. [ 110 ] presented an NMF-based method that separates the raw sound recording into both the heart sound and lung sound. Although these methods have shown their effectiveness, the results of ICA-based separation are varied due to the selection of the number of iterations and convergence criteria, resulting in uncertainties in the phase, amplitude, or ranking order of separated signals. In the NMF-based method, the spectrogram of mixed signals is decomposed into two non-negative matrices, minimizing the difference between the product of the two non-negative matrices and the original matrix. Since the minimization process involves non-convex optimization, the decomposed signal is easily limited to the local optimal solution, resulting in poor noise reduction. In addition, the periodicity of heart sound has been applied to differentiate heart sound from lung sound [ 111 , 112 ]. For example, Ghaderi et al. [ 113 ] applied singular spectrum analysis to locate and separate different trends of heart sound and lung sound.
Feature extraction
The high variability of lung sound is caused by many factors, such as age, sex, lung disease, and body position. The feature extraction method is important for obtaining distinctive feature representations for classification. As shown in Fig. 6 , the representations of lung sound rely on two different types of feature extraction: traditional handcrafted feature extraction and deep learning-based feature extraction [ 114 ], which are discussed below.
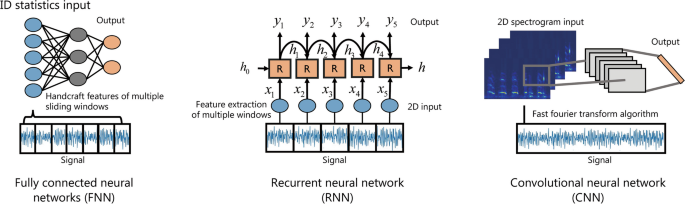
Design procedure of deep learning models. FNN makes predictions based on 1-D statistical features extracted from multiple windows, and RNN predict the health states based on the 2-D features of each window. CNN learns the deep features from the 2D spectrogram input to predict the health states. 1D one-dimensional, 2D two-dimensional
The traditional handcrafted features have quantifiable characteristics of audio signals that can be used to differentiate various sounds, which can be subdivided as follows: (1) time-domain features, which capture information related to lung sound variations over time, such as zero-crossing rate, root mean square, and signal envelope; (2) frequency-domain features, which provide information about the distribution of energy across various frequency bands, such as spectral centroid, spectral roll-off, and spectral flux. Mel-frequency cepstral coefficients (MFCCs) are a commonly used feature in lung sound analysis derived from the Fourier transform, which can capture the distribution of energy in different frequency bands [ 115 , 116 ]; and (3) time–frequency domain features, which record the distribution of energy across different frequency bands over time, providing valuable insights into the non-stationary and transient nature of lung sounds, such as wavelet transform and spectrogram [ 117 , 118 , 119 ]. Researchers generally use a combination of multiple-domain handcrafted features as representations for lung sound analysis [ 120 ]. Among them, the statistical feature is a commonly used combination representation derived from a short temporal sliding window that divides the signal into multiple segments to extract multi-domain features. The statistical values of each feature across multiple segments, such as mean, variance, skewness, and kurtosis, are calculated as the representation. Deep learning-based feature extraction is a data-driven approach that learns features directly from the raw data without the need to design manual features [ 121 , 122 , 123 ]. The CNN, with the input of the spectrogram, is commonly used to capture complex and hierarchical patterns within data and can learn more discriminative and robust representations. Pham et al. [ 124 ] explored the effect of different types of spectrograms and the spectral-time resolution in deep learning-based lung disease detection. Long short-term memory (LSTM) is another important method for feature extraction based on raw data or frequency-domain features. Fraiwan et al. [ 125 ] used CNN to extract the time–frequency information of multiple windows from the raw signal, then used LSTM to mine the continuous time–frequency change information for pulmonary disease recognition.
In summary, traditional handcrafted features are manually designed based on the human understanding of audio signals that emphasize different characteristics of lung sounds in different targeting domains. These handcrafted features are usually easy to interpret and computationally efficient. Initially, the 1D handcrafted features combined with fully connected neural networks (FNNs) were often used for lung sound analysis by projecting the feature vectors into the specified task space [ 117 ]. However, handcrafted features are more sensitive to noise, suffering from quality drops when unexpected events emerge (e.g., talking, footsteps, and coughing) [ 93 ]. Unlike handcrafted features, deep learning-based feature extraction does not fully rely on the human understanding of acoustics or audio content, but automatically learns the task-relevant features from a large amount of lung sound data. Here, CNN combined with the input of 2D spectrogram representation is the most commonly used method, wherein the spectrogram records the raw signal information in the time–frequency domain, and the convolutional kernel is used to integrate the frequency and time domain features to generate high-level semantic representations. The features learned by the deep learning model have the clear advantage of high complexity and dimensionality; however, they lack interpretability since the procedure of network optimization (e.g., backpropagation) is not transparent. Furthermore, this approach requires more computing resources.
Deep learning methods
This section outlines the existing deep-learning methods for lung sound analysis [ 10 , 22 , 23 , 24 , 25 , 26 , 27 , 33 , 72 , 77 , 82 , 91 , 92 , 117 , 122 , 123 , 124 , 125 , 126 , 127 , 128 , 129 , 130 , 131 , 132 , 133 , 134 , 135 , 136 , 137 , 138 , 139 , 140 , 141 , 142 , 143 , 144 , 145 , 146 , 147 , 148 , 149 , 150 , 151 , 152 , 153 , 154 , 155 , 156 , 157 ], as shown in Table 3 . Many aspects of deep learning-based lung analysis are overviewed: basic model selection, the advancement of medical tasks, and limitations and future directions.
Basic model selection
The construction of a specific deep-learning model is based on the structure of input data, as shown in Fig. 6 . FNNs can be used to extract information from a 1D representation, such as the 1D statistical features of lung sound data. For RNNs, the lung sounds will be divided into continuous time windows, and the acoustic features will be extracted from each window to form a 2D lung sound representation. Then, the RNN uses the hidden layer to learn the temporal changes of lung sounds for disease classification. CNNs are more suitable for 2D data representation, such as images (e.g., 2D spectrograms of lung sound). Therefore, the construction of deep learning can be done based on the selection of a specific deep learning model according to its input structure. The basic models can be referred to [ 33 , 126 , 127 ]. Preferably, the model undergoes some tailoring or tuning of its structure based on the classification task and optimization strategy [ 24 , 128 , 129 ]. For example, the FNN-based method transforms the lung sound into a combination representation of acoustic characteristics, then feeds it to the FNN for abnormal sound identification [ 18 ]. Charleston-Villalobos et al. [ 118 ] extracted power spectral density as the representation of lung sound, then used a FNN to distinguish between healthy subjects and interstitial lung disease (ILD) patients, achieving a mean accuracy of 84% with a self-collected dataset. The RNN-based method analyzes the temporal dynamics of lung sounds, which provides insight into the progression of respiratory diseases over time [ 127 ]. Perna et al. [ 26 ] exploited the temporal information of lung sounds by using an RNN to recognize abnormal lung sounds, achieving 85% specificity and 62% sensitivity. The CNN-based method learns the temporal-frequency features from the 2D spectrogram of lung sounds to detect abnormal patterns and infer health conditions [ 33 , 121 ]. Based on the ICBHI 2017 dataset, Yu et al. [ 130 ] extracted global and local features from the Mel spectrogram with a CNN to recognize normal lung sounds, crackle, wheeze, and both, achieving 84.9% specificity and 84.5% sensitivity.
Advancement of medical tasks using lung sound analysis
For medical purposes, deep learning methods can be sorted for two main tasks. (1) ASD: this is a diagnostic auxiliary task that involves the detection of specific abnormal lung sounds, usually crackling and wheezing, as the basis for the diagnosis of specific diseases; and (2) respiratory disease recognition (RDR): this is an automated diagnostic task that directly distinguishes respiratory patients from healthy subjects or identifies patients with different types of respiratory diseases, such as patients with COPD, pneumonia, and asthma. The relationship between them is shown in Fig. 4 .
ASD consists of two sub-tasks:
2-classes abnormal lung sound detection. As a binary classification, this focuses on distinguishing abnormal lung sounds from normal lung sounds without concrete labels or on detecting one type of abnormal lung sound (e.g., crackle, wheeze, and stridor). Serbes et al. [ 126 ] explored the effect of different wavelet types and window sizes in FNN-based crackle detection, where Gaussian, Hanning, Hamming, and Rectangular windows were considered, while Morlet, Mexican Hat, and Paul wavelets were applied to lung sound recognition. Nguyen et al. [ 131 ] proposed the methods of temporal stretching and vocal tract length perturbation for data augmentation to solve the issue of limited training samples, then used a CNN as the backbone for abnormal lung sound detection.
Multi-classes abnormal lung sound recognition. This is used to distinguish between specific abnormal sounds including crackles, wheezes, and rhonchi, where the number of classes is dependent on the number of types of abnormal sounds. Sengupta et al. [ 132 ] extracted statistical features based on MFCCs for lung sound, then fed a FNN to distinguish normal, wheeze, and crackle sounds. Their experiment was carried out on 30 subjects and showed that MFCC-based statistical features outperformed wavelet-based features in finding abnormal sounds. Bardou et al. [ 33 ] extended the types of abnormal lung sounds to include normal, coarse crackle, fine crackle, monophonic wheeze, polyphonic wheeze, squawk, and stridor, then used a spectrogram-based CNN to identify these types. Grzywalski et al. [ 133 ] conducted a clinical trial to compare the accuracy of abnormal lung sound detection between an artificial intelligence (AI) algorithm and doctors, where a CNN was trained to detect four types of lung sound: wheezes, rhonchi, and fine and coarse crackles. This trial suggested that CNN-based abnormal lung sound detection is more accurate than doctors in regard to the metrics of sensitivity and F1-score. With the release of the ICBHI 2017 dataset, the number of studies on ASD for detecting normal sound, crackles, wheezes, and both crackles and wheezes exploded [ 23 , 130 , 134 , 135 ]. Rocha et al. [ 136 ] separately trained a classifier for crackle detection, wheeze detection, and mixture detection (crackle, wheeze, and others) and used four different machine learning methods to evaluate its effectiveness (e.g., boosted trees, SVM, and CNN). Gairola et al. [ 72 ] proposed a concatenation-based augmentation to solve the unbalanced class issue, and used the ResNet block for abnormal lung sound detection. For a limited training sample, Song et al. [ 22 ] proposed an abnormal lung sound detection method that encourages intra-class compactness and inter-class separability by comparing samples from different classes during the training phase. To explore the temporal and frequency information of lung sound, Petmezas et al. [ 137 ] integrated a CNN and an RNN for abnormal lung sound detection, where the former extracts the deep temporal-frequency features from spectrograms, and the latter uses the deep features to mine the change of lung sound over the time.
For RDR, most studies were evaluated on ICBHI 2017 and focused on four sub-tasks:
2-classes respiratory pathology recognition. This is used to distinguish patients from healthy people. Messner et al. [ 122 ] collected lung sounds from healthy subjects and patients with idiopathic pulmonary fibrosis, then applied a convolutional RNN to lung sound analysis for binary classification (e.g., healthy vs. pathological). Mondal et al. [ 138 ] extracted the statistical feature combination of kurtosis, sample entropy, and skewness from lung sounds and used FNN to infer lung health conditions.
3-classes respiratory chronic disease recognition. This divides populations into three groups: healthy subjects, chronic patients (e.g., COPD, bronchiectasis, and asthma patients), and non-chronic patients (e.g., those with upper and lower respiratory tract infection, pneumonia, and bronchiolitis). García-Ordás et al. [ 139 ] converted lung sounds into Mel spectrogram representations to train CNNs to recognize respiratory pathologies, meanwhile using variational autoencoders to generate new samples for minority classes to solve the issues of unbalanced data. Shuvo et al. [ 140 ] decomposed the preprocessed signal using EMD to obtain an IMF signal that had a high correlation with the lung sound signal, then applied the continuous wavelet transform to extract a discriminative representation for training a lightweight CNN model. Their proposed method was evaluated on ICBHI 2017 and outperformed other lightweight models. Shi et al. [ 141 ] explored the temporal-frequency information of different scales with the dual wavelet analysis module, and used the attention module to extract the salient difference information for respiratory chronic disease recognition.
Multi-types specific RDR. This task is used to distinguish between specific respiratory diseases (e.g., COPD, asthma, and pneumonia), where the number of classes depends on the total class of the disease. Tariq et al. [ 123 ] applied a variety of data augmentation methods to solve the issue of unbalanced classes (e.g., time stretching, pitch shifting, and dynamic range compression) and used a CNN to extract pathological features from the spectrogram to recognize seven respiratory diseases. Kwon et al. [ 142 ] explored the performance of different combinations of feature extraction methods and classifiers in detecting lung conditions (e.g., healthy lungs, Upper respiratory tract infection, COPD, pneumonia, and bronchiolitis).
Multi-courses respiratory disease severity recognition. This task aims to distinguish the severity of respiratory diseases, in which the number of classes generally depends on the medical definition of disease progression. Morillo et al. [ 158 ] adopted principal component analysis and FNN to detect whether COPD patients were aggravated by pneumonia, with a sensitivity and specificity of 72.0% and 81.8%, respectively. Based on the RespiratoryDatabase@TR dataset, Altan et al. [ 27 ] proposed the method of using a 3D-second order difference plot to analyze lung sound signals, then using pre-trained deep belief networks to distinguish the risk level from the interior level for COPD patients. This approach demonstrated the validity of pre-trained deep-learning architectures in RDR. Huang et al. [ 10 ] proposed a hybrid model based on pre-trained VGGish networks and BiLSTM to identify the severity of community-acquired pneumonia among children, including pneumonia-confirmation, spontaneous resolution, and recovery. Altan et al. [ 143 ] adopted the cuboid and octant-based quantization methods to extract characteristic abnormalities from a 3D-second order difference plot, then used a deep extreme learning machine classifier to separate five COPD severities. Yu et al. [ 144 ] explored the ability of multiple methods (SVM, decision tree, and deep belief network) to identify the severity of COPD, where the deep belief network achieved 93.67% accuracy in distinguishing between patients with mild, moderate, and severe COPD.
More recently, some studies proposed deep learning-based methods that can be used for both RDR and ASD [ 25 , 124 , 145 ], as shown in Table 3 . Perna et al. [ 26 ] extracted the MFCCs of multi-window from lung sound signals to generate representations, then used an RNN-based model. Li et al. [ 128 ] proposed a knowledge distillation-based method that transfers the weights of a CNN learned from multiple centers into a fuzzy decision tree, which provides an interpretable model for abnormal lung sound detection and chronic RDR. Nguyen et al. [ 24 ] introduced different methods to adapt a pre-trained model to a new environment, including fine-tuning, co-tuning, stochastic normalization, and their combination, for ASD and RDR. In their experiments, the authors noted that varying performance was caused by differences in equipment and introduced spectrum correction to solve this issue [ 159 ].
Limitations and future directions
Table 3 summarizes the state-of-the-art deep learning approaches for ASD and RDR. It shows that most methods use specificity, sensitivity, and the confounding index between the two for ASD, while evaluation metrics (e.g., accuracy, precision, recall, and F1) are added based on the evaluation metrics of ASD for RDR. In terms of the model, a CNN with the input of a spectrogram and Mel spectrogram is currently the most widely-used method for both tasks, achieving over 80% specificity and 60% sensitivity in the ICBHI 2017 dataset for ASD and having over 90% accuracy, recall, precision, and F1 for RDR. In addition, most methods recently used a structure that applies a CNN to extract deep features from multiple consecutive temporal windows, then uses the deep features of successive windows as the input of RNN to learn the contextual information for RDR. Table 3 shows that deep learning has made progress regarding lung sound-based medical tasks, demonstrating the capability to identify different abnormal sounds, pulmonary diseases, and disease severity. However, the clinical application of deep learning-based lung sound analysis still faces some challenges, as discussed below.
The main challenge is that most deep learning-based lung sound analysis methods have poor interpretability [ 128 ]; thus deep learning-based methods currently only play a supporting role in clinical applications. Specifically, physicians rely on the interpretation of lung sounds for medical decision-making. However, the black-box operation of deep learning makes it difficult for physicians to understand how the model works in the diagnosis, that is its mechanism is not fully clear. As a result, physicians cannot fully trust or rely on the results given by the model. Potential solutions to improve interpretability include the following. (1) Symptom localization: intuitively, the segmentation network can highlight the segments of lung sound in the respiratory cycle to locate the symptoms caused by the disease. These segments can be used not only for disease diagnosis, but also for physicians to confirm the final outcome based on intermediate supporting results [ 160 ]. The appearance and localization of abnormal sounds in specific respiratory diseases can be exploited as the trigger of intelligibility by combining them with clinical knowledge; (2) Input visualization: Gradient-weighted class activation mapping analyzes input and gradients to generate interpretable heatmaps that can be used to understand which regions the model focuses on when making decisions [ 161 ]. This can present the intermediate results of the model during the decision-making process, which may convince the clinician of its reliability [ 162 ]; (3) Knowledge distillation: this can distill the knowledge learned from complex models to another model with interpretability, such as decision trees or linear regression, to achieve an interpretable recognition process with high performance [ 128 ]; (4) Surrogate model: this generates a simple, interpretable local model for each specific input to approximate the behavior of the original complex model given the input, such as local interpretable model-agnostic explanations (LIME) [ 163 ]. Thus, LIME can help explain the predictions of complex models on specific inputs.
Another challenge is that deep learning-based lung sound analysis lacks robustness under some conditions. (1) Noise sensitivity: most methods have performance degradation due to an increased noise level [ 136 ], meaning that the reliability of deep learning methods will be compromised in disease diagnosis due to distortions, resulting in misdiagnosis and missed diagnosis; (2) Device difference: due to the difference between devices regarding sensors, timbre, and sound quality, the performance of a model trained on a single device will fluctuate or drop when tested on other devices [ 23 , 24 ]; (3) Physiological diversification: Fernandes et al. [ 146 ] reported that physiological differences between patients, including age, sex, and body mass index, caused deviations in the performance of models for ASD. To address this problem, transfer learning which mines invariant features under different factors (e.g., noise, devices, and physiological differences) for lung sound analysis, may be an option. It can map the data with differences into aligned data distributions to improve generalizability [ 164 , 165 ]. Moreover, multi-input models that take these differences as input and force the model to dynamically adjust its weight based on the input to improve generalizability may be effective.
In addition, due to differences in the morbidity of pulmonary diseases, the data distribution of lung sound is a long-tail distribution, which may cause the poor recognition ability of models for rare categories. Most methods adopt data augmentations to address this issue [ 22 , 72 , 139 ]; however, they are still unreliable in real clinical applications since the data augmented by perturbations are different from patient data in practice. To address this issue, few-shot learning might be a useful tool that aims to extract the representative features from a limited number of training samples to exhibit good generalization when faced with new, unseen data [ 166 ]. For example, prototypical networks achieved remarkable results in audio event classification with the long-tail distribution [ 167 , 168 ]. The key idea is to learn the prototype representation of each class, then perform the classification by calculating the distance between the new sample and each prototype [ 169 ]. In addition, contrastive learning can be applied to lessen long-tail distribution issues by increasing the distance between different classes in the feature space. Li et al. [ 170 ] integrated the idea of prototypical networks to first generate a set of targets uniformly distributed on a feature space, then make the features of different classes converge to these distinct and uniformly distributed targets during training. This forces all classes, including a few, to remain uniformly distributed by the constraints of targeted supervised contrastive learning on the feature space during the optimization process to improve class boundaries.
It is worth noting that most existing lung sound studies only focus on accuracy rather than taking computational resource consumption into account, tending to use models with a large number of parameters that demand more memory and high computational resources [ 6 , 14 , 122 ]. This poses challenges to implementation on the chips of portable devices with limited computation power as compared to servers or personal computers, especially considering the cost-effective hardware solutions that are important for large-scale deployment in poor-resource areas for healthcare improvement. The edge computing of intelligent stethoscopes allows the processing of lung sound data on the device, which reduces the time delay in decision-making and monitoring caused by data transmission in cloud computing, protects the privacy of patients, and reduces the cost of maintaining the cloud server. Such a device is also suitable for disease or well-being management at home by tracking and predicting recovery. Therefore, we consider portable digital stethoscopes equipped with deep learning methods to be a major research direction in this field. Here, we present three strategies to embed deep learning models into the chip of a stethoscope for edge computing. (1) Lightweight model: a large number of methods, such as knowledge distillation and pruning, have been used to lightweight large-scale models to reduce computational requirements [ 171 ]; (2) Hardware acceleration: characteristics of hardware, such as parallel processing capabilities, high-speed memory access, and customized computation units, are proven to accelerate computation in deep models [ 172 ]; and (3) Operational optimization: the complexity and computation of deep models can be dropped by optimizing basic operators (e.g., depthwise separable convolution decomposes the convolution operation into two separate layers, a depthwise convolution layer and a pointwise convolution layer) [ 173 ]. With the above three strategies, deep learning models can be implemented in the chips of digital stethoscopes in the near future, turning the devices into intelligent stethoscopes that not only make recordings of lung sounds, but also give prompt predictions on potential diseases, which can better assist clinicians in consultation.
Open-source framework
Due to the poor reproducibility caused by the variety of deep learning methods, an open-source framework intended to build a solid foundation for replication and extension has been released to facilitate progress in this field. This framework provides the commonly used methods (e.g., FNN with acoustic feature input and CNN with spectrogram input) and demonstrates them on the ICBHI 2017 dataset as an example of benchmarking. In addition, the framework decomposes the algorithm into four major modules: preprocessing for segmentation and noise reduction, feature extraction for input representation, evaluation metrics for performance assessment, and classifier design for training and testing. Thus, researchers can focus on improving specific steps while keeping the rest identical, which can largely improve the efficiency and agreement of the benchmark. This framework was developed based on PyTorch, and each module contains a main function that is called upon to execute the corresponding task.
The preprocessing module consists of two main operations: (1) Noise suppression. Since lung sounds are easily contaminated in the real environment, this framework executes basic noise suppression based on the band-pass filter to retain the frequency band information of interest for lung sounds. In addition, it provides candidates for noise suppression, including EMD, wavelet denoising, ICA, etc. (2) Segmentation. This step segments the input audio recording into intervals to form a uniform input to train the deep model. For the ICBHI 2017 dataset, each audio recording has each respiratory cycle annotated, i.e., the cycles with abnormal lung sounds (crackles and wheezes) are annotated as 1 and the other as 0. This module splits the recording with such labels. If the duration of the segment is insufficient, smart padding [ 131 ] or zero padding is used.
The feature extraction module transforms the 1D sound signal into a representation suitable for the model input. For FNNs and RNNs, lung sound analysis methods adopt the statistical features extracted from segmentation as the representation to train and test the model. This framework performs extraction using pyAudioAnalysis [ 174 ]. For CNNs, spectrogram-based input is generally employed for training and testing, where the framework uses the Librosa library to extract different spectrograms, including the Mel spectrogram.
The evaluation metrics module provides the data-splitting strategies and the commonly used evaluation metrics for the experiment setting. To date, there are two data-splitting strategies for lung sound analysis: (1) subject-dependent experiment [ 22 , 130 , 131 ] that randomly splits the entire dataset into training and testing sets. Here, the data from one subject exist in both the training set and the testing set; and (2) subject-independent experiment [ 10 , 24 , 175 ] that splits the entire dataset into training and testing sets in a subject-wise manner. Here, the data from one subject only appear in the training set or testing set to implement the cross-subject benchmark. The choice of evaluation metrics has been referred to [ 84 ], including accuracy, specificity, sensitivity, and ICBHI score.
The classifier design module is based on PyTorch to automate lung sound analysis, where the training and testing set is loaded based on different dataset splitting strategies. This module is formed by the model design, evaluation metrics, training and testing function, and recording function. For model design, a commonly used basic model is implemented (e.g., FNN, CNN, and RNN). For evaluation metrics, specificity, sensitivity, and the ICBHI score (the mean of specificity and sensitivity) are applied to evaluate the performance of the model according to previous studies [ 84 ]. The recording function is applied to visualize the training information including loss, specificity, and sensitivity.
To develop and evaluate deep learning methods, the above modules can be used as a basis or starting point, providing general functional performance as demonstrated on the ICBHI 2017 dataset. Customized functions can be added on top of each module in future research.
Conclusions
This review provides a systemic overview of the development of deep learning-based lung sound analysis for intelligent stethoscopes. Deep learning has shown effective performance in detecting, classifying, and assessing respiratory conditions from lung sound recordings, especially the CNN model with 2D spectrogram-based input. While there are still challenges to be addressed, including noise reduction, the interpretability of the model, and the robustness of performance, the potential benefits of deep learning-based lung sound analysis are significant regarding the intelligent stethoscope. With further development and refinement, we expect deep learning to empower the digital stethoscope for automatic and intelligent diagnosis. In addition, it can be a part of 5G telemedicine based on video and audio streams, where deep learning-based intelligent stethoscopes provide in-body information (e.g., lung sound and heart sound) and the video provides out-body information (e.g., affective and pain level).
Abbreviations
Average score of specificity and sensitivity
Abnormal sound detection
Blind source separation
Chronic obstructive pulmonary disease
Convolutional neural network
Empirical mode decomposition
Fully connected neural network
Interstitial lung disease
Independent component analysis
Linear frequency cepstral coefficients
Long short-term memory
Mel-frequency cepstral coefficients
Non-negative matrix factorization
Negative percent agreement
Positive percent agreement
Respiratory disease recognition
Recurrent neural network
Support vector machine
Sensitivity
Specificity
One-dimensional
Two-dimensional
Three-dimensional
Williamson EJ, Walker AJ, Bhaskaran K, Bacon S, Bates C, Morton C, et al. Factors associated with COVID-19-related death using OpenSAFELY. Nature. 2020;584(7821):430–6.
Article CAS PubMed PubMed Central Google Scholar
Wu Y, Wang X, Li X, Song L, Yu S, Fang Z, et al. Common mtDNA variations at C5178a and A249d/T6392C/G10310A decrease the risk of severe COVID-19 in a Han Chinese population from Central China. Mil Med Res. 2021;8(1):1–10.
Google Scholar
Jin Y, Cai L, Cheng Z, Cheng H, Deng T, Fan Y, et al. A rapid advice guideline for the diagnosis and treatment of 2019 novel coronavirus (2019-nCoV) infected pneumonia (standard version). Mil Med Res. 2020;7(1):1–23.
Singh D, Agusti A, Anzueto A, Barnes PJ, Bourbeau J, Celli BR, et al. Chronic obstructive lung disease: the GOLD science committee report 2019. Eur Respir J. 2019;53(5):1900164.
Article CAS PubMed Google Scholar
Wu K, Jelfs B, Ma X, Ke R, Tan X, Fang Q. Weakly-supervised lesion analysis with a CNN-based framework for COVID-19. Phys Med Biol. 2021;66(24):245027.
Article CAS Google Scholar
Landge K, Kidambi BR, Singhal A, Basha A, et al. Electronic stethoscopes: brief review of clinical utility, evidence, and future implications. J Pract Cardiovasc Sci. 2018;4(2):65.
Article Google Scholar
Palaniappan R, Sundaraj K, Sundaraj S. A comparative study of the SVM and k-NN machine learning algorithms for the diagnosis of respiratory pathologies using pulmonary acoustic signals. BMC Bioinform. 2014;15:223.
Sakai T, Kato M, Miyahara S, Kiyasu S. Robust detection of adventitious lung sounds in electronic auscultation signals. In: Proceedings of the 21st International Conference on Pattern Recognition (ICPR2012). Tsukuba, Japan; 2012, p. 1993–6.
Oweis RJ, Abdulhay EW, Khayal A, Awad A. An alternative respiratory sounds classification system utilizing artificial neural networks. Biomed J. 2015;38(2):152–61.
Huang D, Wang L, Wang W. A multi-center clinical trial for wireless stethoscope-based diagnosis and prognosis of children community-acquired pneumonia. IEEE Trans Biomed Eng. 2023;70(7):2215–26.
Article PubMed Google Scholar
Emmanouilidou D, McCollum ED, Park DE, Elhilali M. Adaptive noise suppression of pediatric lung auscultations with real applications to noisy clinical settings in developing countries. IEEE Trans Biomed Eng. 2015;62(9):2279–88.
Article PubMed PubMed Central Google Scholar
Mills GA, Nketia TA, Oppong IA, Kaufmann EE. Wireless digital stethoscope using Bluetooth technology. Intern J Eng Sci Technol. 2012;4(8):3961–9.
Leng S, Tan RS, Chai KTC, Wang C, Ghista D, Zhong L. The electronic stethoscope. Biomed Eng Online. 2015;14:66.
Lee SH, Kim YS, Yeo MK, Mahmood M, Zavanelli N, Chung C, et al. Fully portable continuous real-time auscultation with a soft wearable stethoscope designed for automated disease diagnosis. Sci Adv. 2022;8(21):eabo5867.
Hirosawa T, Harada Y, Ikenoya K, Kakimoto S, Aizawa Y, et al. The utility of real-time remote auscultation using a bluetooth-connected electronic stethoscope: open-label randomized controlled pilot trial. JMIR Mhealth Uhealth. 2021;9(7):e23109.
Yilmaz G, Rapin M, Pessoa D, Rocha BM, de Sousa AM, Rusconi R, et al. A wearable stethoscope for long-term ambulatory respiratory health monitoring. Sensors (Basel). 2020;20(18):5124.
Dai Z, Peng Y, Mansy HA, Sandler RH, Royston TJ. Comparison of poroviscoelastic models for sound and vibration in the lungs. J Vib Acoust. 2014;136(5):0510121–5101211.
İçer S, Gengeç Ş. Classification and analysis of non-stationary characteristics of crackle and rhonchus lung adventitious sounds. Digit Signal Process. 2014;28:18–27.
Palaniappan R, Sundaraj K, Ahamed NU. Machine learning in lung sound analysis: a systematic review. Biocybern Biomed Eng. 2013;33(3):129–35.
Sen I, Saraclar M, Kahya YP. A comparison of SVM and GMM-based classifier configurations for diagnostic classification of pulmonary sounds. IEEE Trans Biomed Eng. 2015;62(7):1768–76.
Zhang J, Wang HS, Zhou HY, Dong B, Zhang L, Zhang F, et al. Real-world verification of artificial intelligence algorithm-assisted auscultation of breath sounds in children. Front Pediatr. 2021;9:627337.
Song W, Han J, Song H. Contrastive embeddind learning method for respiratory sound classification. In: ICASSP 2021–2021 IEEE International Conference on Acoustics, Speech and Signal Processing (ICASSP). Toronto, ON, Canada; 2021. p. 1275–79.
Acharya J, Basu A. Deep neural network for respiratory sound classification in wearable devices enabled by patient specific model tuning. IEEE Trans Biomed Circuits Syst. 2020;14(3):535–44.
PubMed Google Scholar
Nguyen T, Pernkopf F. Lung sound classification using co-tuning and stochastic normalization. IEEE Trans Biomed Eng. 2022;69(9):2872–82.
Pham L, McLoughlin I, Phan H, Tran M, Nguyen T, Palaniappan R. Robust deep learning framework for predicting respiratory anomalies and diseases. In: 2020 42nd Annual International Conference of the IEEE Engineering in Medicine & Biology Society (EMBC). Montreal, QC, Canada; 2020. p. 164–7.
Perna D, Tagarelli A. Deep auscultation: predicting respiratory anomalies and diseases via recurrent neural networks. In: 2019 IEEE 32nd International Symposium on Computer-Based Medical Systems (CBMS). Cordoba, Spain; 2019. p. 50–5.
Altan G, Kutlu Y, Pekmezci AÖ, Nural S. Deep learning with 3D-second order difference plot on respiratory sounds. Biomed Signal Process Control. 2018;45:58–69.
Pramono RXA, Bowyer S, Rodriguez-Villegas E. Automatic adventitious respiratory sound analysis: a systematic review. PLoS One. 2017;12(5):e0177926.
Palaniappan R, Sundaraj K, Ahamed NU, Arjunan A, Sundaraj S. Computer-based respiratory sound analysis: a systematic review. IETE Tech Rev. 2013;30(3):248–56.
Jácome C, Marques A. Computerized respiratory sounds in patients with COPD: a systematic review. J Chronic Obstr Pulm Dis. 2015;12(1):104–12.
Rao A, Huynh E, Royston TJ, Kornblith A, Roy S. Acoustic methods for pulmonary diagnosis. IEEE Rev Biomed Eng. 2018;12:221–39.
Chang GC, Lai YF. Performance evaluation and enhancement of lung sound recognition system in two real noisy environments. Comput Methods Programs Biomed. 2010;97(2):141–50.
Bardou D, Zhang K, Ahmad SM. Lung sounds classification using convolutional neural networks. Artif Intell Med. 2018;88:58–69.
Pasterkamp H, Kraman SS, Wodicka GR. Respiratory sounds: advances beyond the stethoscope. Am J Respir Crit Care Med. 1997;156(3):974–87.
Bohadana A, Izbicki G, Kraman SS. Fundamentals of lung auscultation. N Engl J Med. 2014;370(8):744–51.
Olson DE, Hammersley JR. Mechanisms of lung sound generation. Semin Respir Crit Care Med. 1985;6(3):171–9.
Sarkar M, Madabhavi I, Niranjan N, Dogra M. Auscultation of the respiratory system. Ann Thorac Med. 2015;10(3):158–68.
Gavriely N, Palti Y, Alroy G. Spectral characteristics of normal breath sounds. J Appl Physiol. 1981;50(2):307–14.
Weiss EB, Carlson CJ. Recording of breath sounds. Am Rev Respir Dis. 1972;105(5):835–9.
CAS PubMed Google Scholar
Forgacs P, Nathoo AR, Richardson HD. Breath sounds. Thorax. 1971;26(3):288–95.
Kraman SS. Vesicular (normal) lung sounds: how are they made, where do they come from, and what do they mean? Semin Respir Crit Care Med. 1985;6(3):183–91.
Kraman SS. Determination of the site of production of respiratory sounds by subtraction phonopneumography. Am Rev Respir Dis. 1980;122(2):303–9.
Kraman SS. Does laryngeal noise contribute to the vesicular lung sound? Am Rev Respir Dis. 1981;124(3):292–4.
Gavriely N, Nissan M, Rubin AH, Cugell DW. Spectral characteristics of chest wall breath sounds in normal subjects. Thorax. 1995;50(12):1292–300.
Vyshedskiy A, Alhashem RM, Paciej R, Ebril M, Rudman I, Fredberg JJ, et al. Mechanism of inspiratory and expiratory crackles. Chest. 2009;135(1):156–64.
Flietstra B, Markuzon N, Vyshedskiy A, Murphy R. Automated analysis of crackles in patients with interstitial pulmonary fibrosis. Pulm Med. 2011;2011:590506.
Munakata M, Ukita H, Doi I, Ohtsuka Y, Masaki Y, Homma Y, et al. Spectral and waveform characteristics of fine and coarse crackles. Thorax. 1991;46(9):651–7.
Forgacs P. The functional basis of pulmonary sounds. Chest. 1978;73(3):399–405.
Jones A. A brief overview of the analysis of lung sounds. Physiotherapy. 1995;81(1):37–42.
Murphy R, Vyshedskiy A. Acoustic findings in a patient with radiation pneumonitis. N Engl J Med. 2010;363(20):e31.
Bohadana AB, Peslin R, Uffholtz H. Breath sounds in the clinical assessment of airflow obstruction. Thorax. 1978;33(3):345–51.
Nagasaka Y. Lung sounds in bronchial asthma. Allergol Int. 2012;61(3):353–63.
American Thoracic Society, et al. Updated nomenclature for membership reaction. ATS News. 1977;3:5–6.
Luo Y. Portable bluetooth visual electrical stethoscope research. In: 2008 11th IEEE International Conference on Communication Technology. Hangzhou, China; 2008. p. 634–6.
Chamberlain D, Mofor J, Fletcher R, Kodgule R. Mobile stethoscope and signal processing algorithms for pulmonary screening and diagnostics. In: 2015 IEEE Global Humanitarian Technology Conference (GHTC). Seattle, WA, USA; 2015. p. 385–92.
Schuman AJ. Electronic stethoscopes: what’s new for auscultation. Contemp Pediatr. 2015;32(2):37–41.
Behere S, Baffa JM, Penfil S, Slamon N. Real-world evaluation of the eko electronic teleauscultation system. Pediatr Cardiol. 2019;40:154–60.
Wang W, Xu Q, Zhang G, Lian Y, Zhang L, Zhang X, et al. A bat-shape piezoresistor electronic stethoscope based on MEMS technology. Measurement. 2019;147:106850.
Kajor M, Grochala D, Iwaniec M, Kantoch E, Kucharski D. A prototype of the mobile stethoscope for telemedical application. In: 2018 XIV-th International Conference on Perspective Technologies and Methods in MEMS Design (MEMSTECH). Lviv, Ukraine; 2018. p. 5–8.
Lakhe A, Sodhi I, Warrier J, Sinha V. Development of digital stethoscope for telemedicine. J Med Eng Technol. 2016;40(1):20–4.
Vasudevan RS, Horiuchi Y, Torriani FJ, Cotter B, Maisel SM, et al. Persistent value of the stethoscope in the age of COVID-19. Am J Med. 2020;133(10):1143–50.
Mesquita CT, dos Reis JC, Simões LS, de Moura EC, Rodrigues GA, Athayde CC, et al. Digital stethoscope as an innovative tool on the teaching of auscultatory skills. Arq Bras Cardiol. 2013;100(2):187–9.
Elgendi M, Bobhate P, Jain S, Guo L, Rutledge J, Coe Y, et al. Spectral analysis of the heart sounds in children with and without pulmonary artery hypertension. Int J Cardiol. 2014;173(1):92–9.
Elgendi M, Bobhate P, Jain S, Rutledge J, Coe JY, Zemp R, et al. Time-domain analysis of heart sound intensity in children with and without pulmonary artery hypertension: a pilot study using a digital stethoscope. Pulm Circ. 2014;4(4):685–95.
Scrafford C, Basnet S, Ansari I, Shrestha L, Shrestha S, Ghimire R, et al. Evaluation of digital auscultation to diagnose pneumonia in children 2 to 35 months of age in a clinical setting in Kathmandu, Nepal: a prospective case–control study. J Pediatr Infect Dis. 2016;11(2):28–36.
Ellington LE, Emmanouilidou D, Elhilali M, Gilman RH, Tielsch JM, Chavez MA, et al. Developing a reference of normal lung sounds in healthy Peruvian children. Lung. 2014;192(5):765–73.
Kevat AC, Kalirajah A, Roseby R. Digital stethoscopes compared to standard auscultation for detecting abnormal paediatric breath sounds. Eur J Pediatr. 2017;176:989–92.
Zheng L, Li Y, Chen W, Wang Q, Jiang Q, Liu G. Detection of respiration movement asymmetry between the left and right lungs using mutual information and transfer entropy. IEEE Access. 2017;6:605–13.
Jean S, Cinel I, Tay C, Parrillo JE, Dellinger RP. Assessment of asymmetric lung disease in intensive care unit patients using vibration response imaging. Anesth Analg. 2008;107(4):1243–7.
Ren S, Li Y, Li W, Zhao Z, Jin C, Zhang D. Fatal asymmetric interstitial lung disease after erlotinib for lung cancer. Respiration. 2012;84(5):431–5.
Rennoll V, McLane I, Emmanouilidou D, West J, Elhilali M. Electronic stethoscope filtering mimics the perceived sound characteristics of acoustic stethoscope. IEEE J Biomed Health Inform. 2021;25(5):1542–9.
Gairola S, Tom F, Kwatra N, Jain M. RespireNet: a deep neural network for accurately detecting abnormal lung sounds in limited data setting. In: 2021 43rd Annual International Conference of the IEEE Engineering in Medicine & Biology Society (EMBC). Mexico; 2021. p. 527–30.
Pavlosky A, Glauche J, Chambers S, Al-Alawi M, Yanev K, Loubani T. Validation of an effective, low cost, free/open access 3D-printed stethoscope. PLoS ONE. 2018;13(3):e0193087.
Tosi J, Taffoni F, Santacatterina M, Sannino R, Formica D. Performance evaluation of Bluetooth Low Energy: a systematic review. Sensors (Basel). 2017;17(12):2898.
Memon S, Soothar KK, Memon KA, Magsi AH, Laghari AA, Abbas M, ul Ain N. The design of wireless portable electrocardiograph monitoring system based on ZigBee. EAI Endorsed Trans Scalable Inf Syst. 2020;7(28):e6.
Wang J, Huang D, Fan S, Han K, Jeon G, Rodrigues JJ. PSDCE: physiological signal-based double chaotic encryption for instantaneous E-healthcare services. Future Gener Comput Syst. 2023;141:116–28.
Kim Y, Hyon Y, Jung SS, Lee S, Yoo G, Chung C, et al. Respiratory sound classification for crackles, wheezes, and rhonchi in the clinical field using deep learning. Sci Rep. 2021;11(1):17186.
Grooby E, Sitaula C, Tan K, Zhou L, King A, Ramanathan A, et al. Prediction of neonatal respiratory distress in term babies at birth from digital stethoscope recorded chest sounds. In: 2022 44th Annual International Conference of the IEEE Engineering in Medicine & Biology Society (EMBC). Glasgow, Scotland, United Kingdom; 2022. p. 4996–9.
Oud M, Dooijes EH, van der Zee JS. Asthmatic airways obstruction assessment based on detailed analysis of respiratory sound spectra. IEEE Trans Biomed Eng. 2000;47(11):1450–5.
Mayorga P, Druzgalski C, Morelos R, Gonzalez O, Vidales J. Acoustics based assessment of respiratory diseases using GMM classification. In: 2010 Annual International Conference of the IEEE Engineering in Medicine and Biology. Buenos Aires, Argentina; 2010. p. 6312–6.
Kahya YP, Guler EC, Sahin S. Respiratory disease diagnosis using lung sounds. In: Proceedings of the 19th Annual International Conference of the IEEE Engineering in Medicine and Biology Society. 'Magnificent Milestones and Emerging Opportunities in Medical Engineering' (Cat. No. 97CH36136), Chicago, IL, USA; 1997;5:2051-3.
Cinyol F, Baysal U, Köksal D, Babaoğlu E, Ulaşlı SS. Incorporating support vector machine to the classification of respiratory sounds by convolutional neural network. Biomed Signal Process Control. 2023;79:104093.
Gemmeke JF, Ellis DP, Freedman D, Jansen A, Lawrence W, Moore RC, et al. Audio set: an ontology and human-labeled dataset for audio events. In: 2017 IEEE International Conference on Acoustics, Speech and Signal Processing (ICASSP). New Orleans, LA, USA; 2017. p. 776–80.
Rocha BM, Filos D, Mendes L, Serbes G, Ulukaya S, Kahya YP, et al. An open access database for the evaluation of respiratory sound classification algorithms. Physiol Meas. 2019;40(3):035001.
Fraiwan M, Fraiwan L, Khassawneh B, Ibnian A. A dataset of lung sounds recorded from the chest wall using an electronic stethoscope. Data Brief. 2021;35:106913.
Hsu FS, Huang SR, Huang CW, Huang CJ, Cheng YR, Chen CC, et al. Benchmarking of eight recurrent neural network variants for breath phase and adventitious sound detection on a self-developed open-access lung sound database-HF_Lung_V1. PLoS One. 2021;16(7):e0254134.
Hsu FS, Huang SR, Huang CW, Cheng YR, Chen CC, Hsiao J, et al. An update on a progressively expanded database for automated lung sound analysis. arXiv. 2021. https://arxiv.org/abs/2102.04062 .
Altan G, Kutlu Y, Garbİ Y, Pekmezci AÖ, Nural S. Multimedia respiratory database (RespiratoryDatabase@TR): auscultation sounds and chest X-rays. Nat Eng Sci. 2017;2(3):59–72.
World Health Organization. World health statistics 2017: monitoring health for the SDGs, sustainable development goals. https://api.semanticscholar.org/CorpusID:203489275?utm_source=wikipedia . Accessed 8 May 2018.
Guide P, Copd T. Global initiative for chronic obstructive lung a guide for health care professionals global initiative for chronic obstructive disease. Glob Initiative Chronic Obstr Lung Dis. 2010;22(4):1–30.
Altan G, Kutlu Y. Hessenberg elm autoencoder kernel for deep learning. J Eng Technol Appl Sci. 2018;3(2):141–51.
Roy A, Satija U. A novel melspectrogram snippet representation learning framework for severity detection of chronic obstructive pulmonary diseases. IEEE Trans Instrum Meas. 2023;72:1–11.
Emmanouilidou D, McCollum ED, Park DE, Elhilali M. Computerized lung sound screening for pediatric auscultation in noisy field environments. IEEE Trans Biomed Eng. 2018;65(7):1564–74.
Meng F, Wang Y, Shi Y, Zhao H. A kind of integrated serial algorithms for noise reduction and characteristics expanding in respiratory sound. Int J Biol Sci. 2019;15(9):1921.
Haider NS, Behera AK. Respiratory sound denoising using sparsity-assisted signal smoothing algorithm. Biocybern Biomed Eng. 2022;42(2):481–93.
Singh D, Singh BK, Behera AK. Comparitive study of different iir filter for denoising lung sound. In: 2021 6th International Conference for Convergence in Technology (I2CT). Maharashtra, India; 2021. p. 1–3.
Pouyani MF, Vali M, Ghasemi MA. Lung sound signal denoising using discrete wavelet transform and artificial neural network. Biomed Signal Process Control. 2022;72:103329.
Singh D, Singh BK, Behera AK. Comparative analysis of lung sound denoising technique. In: 2020 First International Conference on Power, Control and Computing Technologies (ICPC2T). Raipur, India; 2020. p. 406–10.
Syahputra M, Situmeang S, Rahmat R, Budiarto R. Noise reduction in breath sound files using wavelet transform based filter. In: IOP Conference Series: Materials Science and Engineering. Semarang, Indonesia; 2017;190:012040.
Sangeetha B, Periyasamy R. Performance metrics analysis of adaptive threshold empirical mode decomposition denoising method for suppression of noise in lung sounds. In: 2021 Seventh International Conference on Bio Signals, Images, and Instrumentation (ICBSII). Chennai, India; 2021. p. 1–6.
Gupta S, Agrawal M, Deepak D. Gammatonegram based triple classification of lung sounds using deep convolutional neural network with transfer learning. Biomed Signal Process Control. 2021;70:102947.
Meng F, Wang Y, Shi Y, Cai M, Yang L, Shen D. A new type of wavelet de-noising algorithm for lung sound signals. In: 2018 IEEE International Conference on Bioinformatics and Biomedicine (BIBM). Madrid, Spain; 2018. p. 2544–2548.
Haider NS. Respiratory sound denoising using empirical mode decomposition, hurst analysis and spectral subtraction. Biomed Signal Process Control. 2021;64:102313.
Nersisson R, Noel MM. Heart sound and lung sound separation algorithms: a review. J Med Eng Technol. 2017;41(1):13–21.
Khan TEA, Vijayakumar P. Separating heart sound from lung sound using labVIEW. Int J Comput Electr Eng. 2010;2(3):524–33.
Ayari F, Ksouri M, Alouani AT. Lung sound extraction from mixed lung and heart sounds fastica algorithm. In: 2012 16th IEEE Mediterranean Electrotechnical Conference. Yasmine Hammamet, Tunisia; 2012. p. 339–42.
Lin C, Hasting E. Blind source separation of heart and lung sounds based on nonnegative matrix factorization. In: 2013 International Symposium on Intelligent Signal Processing and Communication Systems. Naha, Japan; 2013. p. 731–6.
Mondal A, Banerjee P, Somkuwar A. Enhancement of lung sounds based on empirical mode decomposition and Fourier transform algorithm. Comput Methods Programs Biomed. 2017;139:119–36.
Grooby E, Sitaula C, Fattahi D, Sameni R, Tan K, Zhou L, et al. Noisy neonatal chest sound separation for high-quality heart and lung sounds. IEEE J Biomed Health Inform. 2023;27(6):2635–46.
Grooby E, He J, Fattahi D, Zhou L, King A, Ramanathan A, et al. A new non-negative matrix co-factorisation approach for noisy neonatal chest sound separation. In: 2021 43rd Annual International Conference of the IEEE Engineering in Medicine & Biology Society (EMBC). Mexico; 2021. p. 5668–73.
Li T, Tang H, Qiu T, Park Y. Heart sound cancellation from lung sound record using cyclostationarity. Med Eng Phys. 2013;35(12):1831–6.
Tsai KH, Wang WC, Cheng CH, Tsai CY, Wang JK, Lin TH, et al. Blind monaural source separation on heart and lung sounds based on periodic-coded deep autoencoder. IEEE J Biomed Health Inform. 2020;24(11):3203–14.
Ghaderi F, Mohseni HR, Sanei S. Localizing heart sounds in respiratory signals using singular spectrum analysis. IEEE Trans Biomed Eng. 2011;58(12):3360–7.
Kim Y, Hyon Y, Lee S, Woo SD, Ha T, Chung C. The coming era of a new auscultation system for analyzing respiratory sounds. BMC Pulm Med. 2022;22(1):119.
Bahoura M, Pelletier C. Respiratory sounds classification using cepstral analysis and Gaussian mixture models. In: The 26th Annual International Conference of the IEEE Engineering in Medicine and Biology Society. San Francisco, CA, USA; 2004. p. 9–12.
Haider NS, Singh BK, Periyasamy R, Behera AK. Respiratory sound based classification of chronic obstructive pulmonary disease: a risk stratification approach in machine learning paradigm. J Med Syst. 2019;43(8):255.
Tocchetto MA, Bazanella AS, Guimaraes L, Fragoso J, Parraga A. An embedded classifier of lung sounds based on the wavelet packet transform and ANN. IFAC Proc. 2014;47(3):2975–80.
Charleston-Villalobos S, Martinez-Hernandez G, Gonzalez-Camarena R, Chi-Lem G, Carrillo JG, Aljama-Corrales T. Assessment of multichannel lung sounds parameterization for two-class classification in interstitial lung disease patients. Comput Biol Med. 2011;41(7):473–82.
Lozano M, Fiz JA, Jané R. Automatic differentiation of normal and continuous adventitious respiratory sounds using ensemble empirical mode decomposition and instantaneous frequency. IEEE J Biomed Health Inform. 2016;20(2):486–97.
Datta S, Choudhury AD, Deshpande P, Bhattacharya S, Pal A. Automated lung sound analysis for detecting pulmonary abnormalities. In: 2017 39th Annual International Conference of the IEEE Engineering in Medicine and Biology Society (EMBC). Jeju, Korea (South); 2017. p. 4594–8.
Aykanat M, Kılıç Ö, Kurt B, Saryal S. Classification of lung sounds using convolutional neural networks. EURASIP J Image Video Process. 2017;2017(1):65.
Messner E, Fediuk M, Swatek P, Scheidl S, Smolle-Jüttner FM, Olschewski H, et al. Multi-channel lung sound classification with convolutional recurrent neural networks. Comput Biol Med. 2020;122:103831.
Tariq Z, Shah SK, Lee Y. Lung disease classification using deep convolutional neural network. In: 2019 IEEE International Conference on Bioinformatics and Biomedicine (BIBM). San Diego, CA, USA; 2019. p. 732–5.
Pham L, Phan H, Palaniappan R, Mertins A, McLoughlin I. CNN-MoE based framework for classification of respiratory anomalies and lung disease detection. IEEE J Biomed Health Inform. 2021;25(8):2938–47.
Fraiwan M, Fraiwan L, Alkhodari M, Hassanin O. Recognition of pulmonary diseases from lung sounds using convolutional neural networks and long short-term memory. J Ambient Intell Humaniz Comput. 2022;13(10):4759–71.
Serbes G, Sakar CO, Kahya YP, Aydin N. Pulmonary crackle detection using time–frequency and time–scale analysis. Digit Signal Process. 2013;23(3):1012–21.
Messner E, Fediuk M, Swatek P, Scheidl S, Smolle-Juttner FM, et al. Crackle and breathing phase detection in lung sounds with deep bidirectional gated recurrent neural networks. In: 2018 40th Annual International Conference of the IEEE Engineering in Medicine and Biology Society (EMBC). Honolulu, HI, USA; 2018. p. 356–9.
Li J, Wang C, Chen J, Zhang H, Dai Y, Wang L, et al. Explainable CNN with fuzzy tree regularization for respiratory sound analysis. IEEE Trans Fuzzy Syst. 2022;30(6):1516–28.
Choi Y, Lee H. Interpretation of lung disease classification with light attention connected module. Biomed Signal Process Control. 2023;84:104695.
Yu S, Ding Y, Qian K, Hu B, Li W, Schuller BW. A glance-and-gaze network for respiratory sound classification. In: ICASSP 2022–2022 IEEE International Conference on Acoustics, Speech and Signal Processing (ICASSP). Singapore, Singapore; 2022. p. 9007–11.
Nguyen T, Pernkopf F. Lung sound classification using snapshot ensemble of convolutional neural networks. In: 2020 42nd Annual International Conference of the IEEE Engineering in Medicine & Biology Society (EMBC). Montreal, QC, Canada; 2020. p. 760-3.
Sengupta N, Sahidullah M, Saha G. Lung sound classification using cepstral-based statistical features. Comput Biol Med. 2016;75:118–29.
Grzywalski T, Piecuch M, Szajek M, Bręborowicz A, Hafke-Dys H, Kociński J, et al. Practical implementation of artificial intelligence algorithms in pulmonary auscultation examination. Eur J Pediatr. 2019;178(6):883–90.
Pham L, Ngo D, Tran K, Hoang T, Schindler A, McLoughlin I. An ensemble of deep learning frameworks for predicting respiratory anomalies. In: 2022 44th Annual International Conference of the IEEE Engineering in Medicine & Biology Society (EMBC). Glasgow, Scotland, United Kingdom; 2022. p. 4595–8.
Zhao Z, Gong Z, Niu M, Ma J, Wang H, Zhang Z, et al. Automatic respiratory sound classification via multi-branch temporal convolutional network. In: ICASSP 2022–2022 IEEE International Conference on Acoustics, Speech and Signal Processing (ICASSP). Singapore, Singapore; 2022. p. 9102–6.
Rocha BM, Pessoa D, Marques A, Carvalho P, Paiva RP. Automatic classification of adventitious respiratory sounds: a (un)solved problem? Sensors (Basel). 2020;21(1):57.
Petmezas G, Cheimariotis GA, Stefanopoulos L, Rocha B, Paiva RP, Katsaggelos AK, et al. Automated lung sound classification using a hybrid CNN-LSTM network and focal loss function. Sensors (Basel). 2022;22(3):1232.
Mondal A, Bhattacharya P, Saha G. Detection of lungs status using morphological complexities of respiratory sounds. Sci World J. 2014;2014:182938.
García-Ordás MT, Benítez-Andrades JA, García-Rodríguez I, Benavides C, Alaiz-Moretón H. Detecting respiratory pathologies using convolutional neural networks and variational autoencoders for unbalancing data. Sensors. 2020;20(4):1214.
Shuvo SB, Ali SN, Swapnil SI, Hasan T, Bhuiyan MIH. A lightweight CNN model for detecting respiratory diseases from lung auscultation sounds using EMD-CWT-based hybrid scalogram. IEEE J Biomed Health Inform. 2021;25(7):2595–603.
Shi L, Zhang Y, Zhang J. Lung sound recognition method based on wavelet feature enhancement and time-frequency synchronous modeling. IEEE J Biomed Health Inform. 2023;27(1):308–18.
Kwon AM, Kang K. A temporal dependency feature in lower dimension for lung sound signal classification. Sci Rep. 2022;12:7889.
Altan G, Kutlu Y, Gökçen A. Chronic obstructive pulmonary disease severity analysis using deep learning on multi-channel lung sounds. Turk J Elec Eng Co. 2020;28(5):2979–96.
Yu H, Zhao J, Liu D, Chen Z, Sun J, Zhao X. Multi-channel lung sounds intelligent diagnosis of chronic obstructive pulmonary disease. BMC Pulm Med. 2021;21(1):1–13.
Pham L, Phan H, Schindler A, King R, Mertins A, McLoughlin I. Inception-based network and multi-spectrogram ensemble applied to predict respiratory anomalies and lung diseases. In: 2021 43rd Annual International Conference of the IEEE Engineering in Medicine & Biology Society (EMBC). Mexico; 2021. p. 253–6.
Fernandes T, Rocha BM, Pessoa D, de Carvalho P, Paiva RP. Classification of adventitious respiratory sound events: A stratified analysis. In: 2022 IEEE-EMBS International Conference on Biomedical and Health Informatics (BHI). Ioannina, Greece; 2022. p. 1–5.
Kochetov K, Putin E, Balashov M, Filchenkov A, Shalyto A. Noise masking recurrent neural network for respiratory sound classification. In: Artificial Neural Networks and Machine Learning–ICANN 2018. Cham: Springer International Publishing; 2018. p. 208–17.
Ma Y, Xu X, Yu Q, Zhang Y, Li Y, Zhao J, et al. LungBRN: a smart digital stethoscope for detecting respiratory disease using bi-ResNet deep learning algorithm. In: 2019 IEEE Biomedical Circuits and Systems Conference (BioCAS). Nara, Japan; 2019. p. 1–4.
Hsiao CH, Lin TW, Lin CW, Hsu FS, Lin FYS, Chen CW, et al. Breathing sound segmentation and detection using transfer learning techniques on an attention-based encoder-decoder architecture. In: 2020 42nd Annual International Conference of the IEEE Engineering in Medicine & Biology Society (EMBC). Montreal, QC, Canada; 2020. p. 754–9.
Kevat A, Kalirajah A, Roseby R. Artificial intelligence accuracy in detecting pathological breath sounds in children using digital stethoscopes. Respir Res. 2020;21(1):1–6.
Jayalakshmy S, Sudha GF. Scalogram based prediction model for respiratory disorders using optimized convolutional neural networks. Artif Intell Med. 2020;103:101809.
Ngo D, Pham L, Nguyen A, Phan B, Tran K, Nguyen T. Deep learning framework applied for predicting anomaly of respiratory sounds. In: 2021 International Symposium on Electrical and Electronics Engineering (ISEE). Ho Chi Minh, Vietnam; 2021. p. 42–7.
Becker K, Scheffer C, Blanckenberg M, Diacon A. Analysis of adventitious lung sounds originating from pulmonary tuberculosis. In: 2013 35th Annual International Conference of the IEEE Engineering in Medicine and Biology Society (EMBC). Osaka, Japan; 2013. p. 4334–7.
Altan G, Kutlu Y, Pekmezci AÖ, Yayık A. Diagnosis of chronic obstructive pulmonary disease using deep extreme learning machines with lu autoencoder kernel. In: 7th International Conference on Advanced Technologies (ICAT’18). Antalya; 2018. p. 618–22.
Altan G, Kutlu Y, Allahverdi N. Deep learning on computerized analysis of chronic obstructive pulmonary disease. IEEE J Biomed Health Inform. 2020;24(5):1344–50.
Monaco A, Amoroso N, Bellantuono L, Pantaleo E, Tangaro S, Bellotti R. Multi-time-scale features for accurate respiratory sound classification. Appl Sci. 2020;10(23):8606.
Brunese L, Mercaldo F, Reginelli A, Santone A. A neural network-based method for respiratory sound analysis and lung disease detection. Appl Sci. 2022;12(8):3877.
Morillo DS, León Jiménez A, Moreno SA. Computer-aided diagnosis of pneumonia in patients with chronic obstructive pulmonary disease. J Am Med Inform Assoc. 2013;20(e1):e111–7.
Nguyen T, Pernkopf F, Kosmider M. Acoustic scene classification for mismatched recording devices using heated-up softmax and spectrum correction. In: ICASSP 2020–2020 IEEE International Conference on Acoustics, Speech and Signal Processing (ICASSP). Barcelona, Spain; 2020. p. 126–30.
Fernando T, Sridharan S, Denman S, Ghaemmaghami H, Fookes C. Robust and interpretable temporal convolution network for event detection in lung sound recordings. IEEE J Biomed Health Inform. 2022;26(7):2898–908.
Selvaraju RR, Cogswell M, Das A, Vedantam R, Parikh D, Batra D. Grad-CAM: visual explanations from deep networks via gradient-based localization. In: Proceedings of the IEEE International Conference on Computer Vision. Venice, Italy; 2017. p. 618–26.
Altan G. DeepOCT: An explainable deep learning architecture to analyze macular edema on oct images. Eng Sci Technol Int J. 2022;34:101091.
Mishra S, Sturm BL, Dixon S. Local interpretable model-agnostic explanations for music content analysis. In: ISMIR. 2017. p. 537–43.
Ganin Y, Ustinova E, Ajakan H, Germain P, Larochelle H, Laviolette F, et al. Domain-adversarial training of neural networks. J Mach Learn Res. 2016;17:1–35.
Tzeng E, Hoffman J, Saenko K, Darrell T. Adversarial discriminative domain adaptation. In: Proceedings of the IEEE Conference on Computer Vision and Pattern Recognition (CVPR). Honolulu, HI, USA; 2017. p. 7167–76.
Wang Y, Yao Q, Kwok JT, Ni LM. Generalizing from a few examples: a survey on few-shot learning. ACM Comput Surv. 2020;53(3):63.
Pons J, Serrà J, Serra X. Training neural audio classifiers with few data. In: ICASSP 2019–2019 IEEE International Conference on Acoustics, Speech and Signal Processing (ICASSP). Brighton, UK; 2019. p. 16–20.
Wolters P, Careaga C, Hutchinson B, Phillips L. A study of few-shot audio classification. arXiv. 2020. https://arxiv.org/abs/2012.01573 .
Snell J, Swersky K, Zemel R. Prototypical networks for few-shot learning. arXiv. 2017. https://arxiv.org/abs/1703.05175 .
Li T, Cao P, Yuan Y, Fan L, Yang Y, Feris RS, et al. Targeted supervised contrastive learning for long-tailed recognition. In: Proceedings of the IEEE/CVF Conference on Computer Vision and Pattern Recognition. New Orleans, LA, USA; 2022. p. 6908–28.
Gou J, Yu B, Maybank SJ, Tao D. Knowledge distillation: a survey. Int J Comput Vis. 2021;129(6):1789–819.
Ding W, Huang Z, Huang Z, Tian L, Wang H, Feng S. Designing efficient accelerator of depthwise separable convolutional neural network on FPGA. J Syst Archit. 2019;97:278–86.
Howard AG, Zhu M, Chen B, Kalenichenko D, Wang W, Weyand T, et al. MobileNets: efficient convolutional neural networks for mobile vision applications. arXiv. 2017. https://arxiv.org/abs/1704.04861 .
Giannakopoulos T. pyAudioAnalysis: an open-source python library for audio signal analysis. PLoS ONE. 2015;10(12):e0144610.
Huang D, Wang L, Lu H, Wang W. A contrastive embedding-based domain adaptation method for lung sound recognition in children community-acquired pneumonia. In: ICASSP 2023–2023 IEEE International Conference on Acoustics, Speech and Signal Processing (ICASSP). Rhodes, Greece; 2023. p. 1–5.
Download references
Acknowledgements
Not applicable.
This work is supported by the National Key Research and Development Program of China (2022YFC2407800), the General Program of National Natural Science Foundation of China (62271241), the Guangdong Basic and Applied Basic Research Foundation (2023A1515012983), and the Shenzhen Fundamental Research Program (JCYJ20220530112601003).
Author information
Authors and affiliations.
Department of Biomedical Engineering, Southern University of Science and Technology, Shenzhen, 518055, Guangdong, China
Dong-Min Huang & Wen-Jin Wang
The Third People’s Hospital of Shenzhen, Shenzhen, 518112, Guangdong, China
Jia Huang, Kun Qiao & Hong-Zhou Lu
Guangzhou Institute of Respiratory Health, China State Key Laboratory of Respiratory Disease, National Clinical Research Center for Respiratory Disease, The First Affiliated Hospital of Guangzhou Medical University, Guangzhou, 510120, China
Nan-Shan Zhong
You can also search for this author in PubMed Google Scholar
Corresponding authors
Correspondence to Nan-Shan Zhong , Hong-Zhou Lu or Wen-Jin Wang .
Ethics declarations
Ethics approval and consent to participate, consent for publication, competing interests.
The authors declare that they have no competing interests.
Rights and permissions
Open Access This article is licensed under a Creative Commons Attribution 4.0 International License, which permits use, sharing, adaptation, distribution and reproduction in any medium or format, as long as you give appropriate credit to the original author(s) and the source, provide a link to the Creative Commons licence, and indicate if changes were made. The images or other third party material in this article are included in the article's Creative Commons licence, unless indicated otherwise in a credit line to the material. If material is not included in the article's Creative Commons licence and your intended use is not permitted by statutory regulation or exceeds the permitted use, you will need to obtain permission directly from the copyright holder. To view a copy of this licence, visit http://creativecommons.org/licenses/by/4.0/ . The Creative Commons Public Domain Dedication waiver ( http://creativecommons.org/publicdomain/zero/1.0/ ) applies to the data made available in this article, unless otherwise stated in a credit line to the data.
Reprints and permissions
About this article
Cite this article.
Huang, DM., Huang, J., Qiao, K. et al. Deep learning-based lung sound analysis for intelligent stethoscope. Military Med Res 10 , 44 (2023). https://doi.org/10.1186/s40779-023-00479-3
Download citation
Received : 29 March 2023
Accepted : 05 September 2023
Published : 26 September 2023
DOI : https://doi.org/10.1186/s40779-023-00479-3
Share this article
Anyone you share the following link with will be able to read this content:
Sorry, a shareable link is not currently available for this article.
Provided by the Springer Nature SharedIt content-sharing initiative
- Deep learning
- Lung sound analysis
- Respiratory sounds
Military Medical Research
ISSN: 2054-9369
- Submission enquiries: Access here and click Contact Us
- General enquiries: [email protected]
Have a language expert improve your writing
Run a free plagiarism check in 10 minutes, generate accurate citations for free.
- Knowledge Base
- How to Write a Thesis Statement | 4 Steps & Examples
How to Write a Thesis Statement | 4 Steps & Examples
Published on January 11, 2019 by Shona McCombes . Revised on August 15, 2023 by Eoghan Ryan.
A thesis statement is a sentence that sums up the central point of your paper or essay . It usually comes near the end of your introduction .
Your thesis will look a bit different depending on the type of essay you’re writing. But the thesis statement should always clearly state the main idea you want to get across. Everything else in your essay should relate back to this idea.
You can write your thesis statement by following four simple steps:
- Start with a question
- Write your initial answer
- Develop your answer
- Refine your thesis statement
Instantly correct all language mistakes in your text
Upload your document to correct all your mistakes in minutes
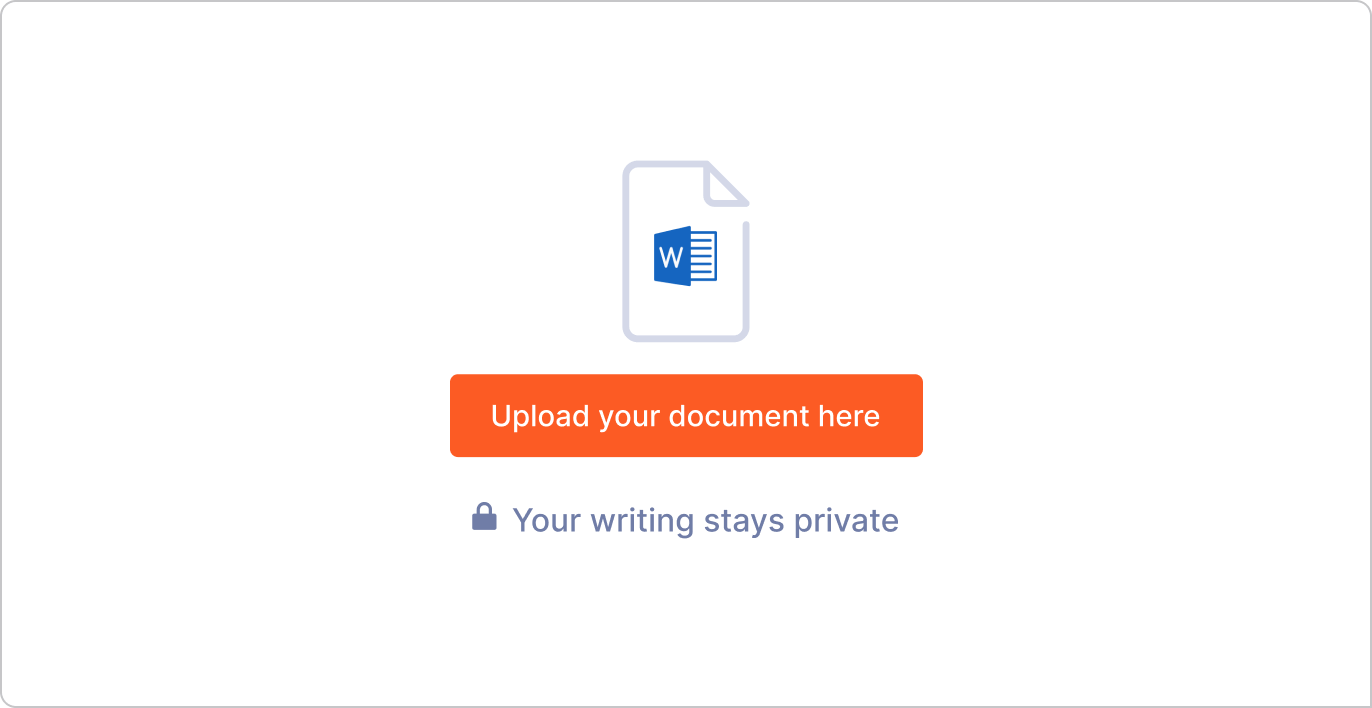
Table of contents
What is a thesis statement, placement of the thesis statement, step 1: start with a question, step 2: write your initial answer, step 3: develop your answer, step 4: refine your thesis statement, types of thesis statements, other interesting articles, frequently asked questions about thesis statements.
A thesis statement summarizes the central points of your essay. It is a signpost telling the reader what the essay will argue and why.
The best thesis statements are:
- Concise: A good thesis statement is short and sweet—don’t use more words than necessary. State your point clearly and directly in one or two sentences.
- Contentious: Your thesis shouldn’t be a simple statement of fact that everyone already knows. A good thesis statement is a claim that requires further evidence or analysis to back it up.
- Coherent: Everything mentioned in your thesis statement must be supported and explained in the rest of your paper.
Receive feedback on language, structure, and formatting
Professional editors proofread and edit your paper by focusing on:
- Academic style
- Vague sentences
- Style consistency
See an example
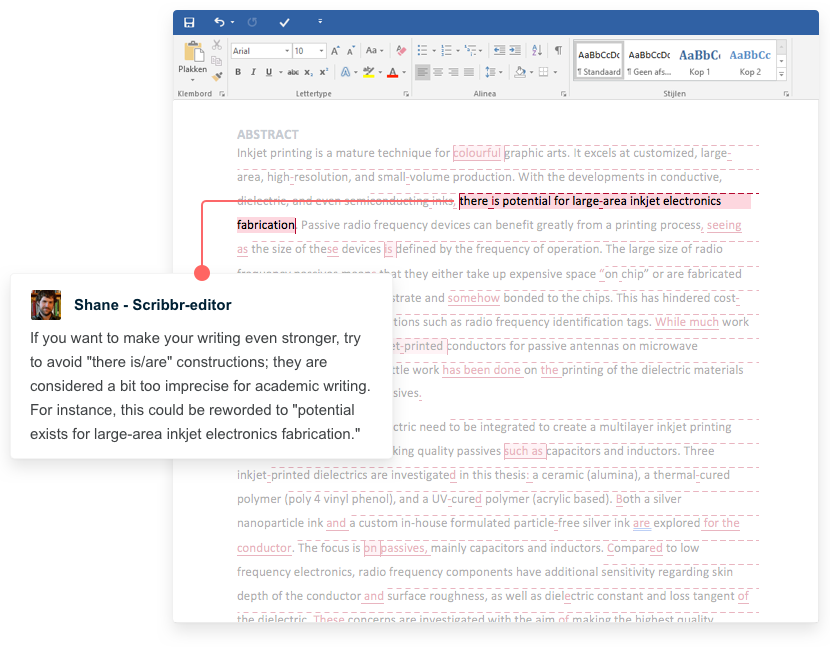
The thesis statement generally appears at the end of your essay introduction or research paper introduction .
The spread of the internet has had a world-changing effect, not least on the world of education. The use of the internet in academic contexts and among young people more generally is hotly debated. For many who did not grow up with this technology, its effects seem alarming and potentially harmful. This concern, while understandable, is misguided. The negatives of internet use are outweighed by its many benefits for education: the internet facilitates easier access to information, exposure to different perspectives, and a flexible learning environment for both students and teachers.
You should come up with an initial thesis, sometimes called a working thesis , early in the writing process . As soon as you’ve decided on your essay topic , you need to work out what you want to say about it—a clear thesis will give your essay direction and structure.
You might already have a question in your assignment, but if not, try to come up with your own. What would you like to find out or decide about your topic?
For example, you might ask:
After some initial research, you can formulate a tentative answer to this question. At this stage it can be simple, and it should guide the research process and writing process .
Prevent plagiarism. Run a free check.
Now you need to consider why this is your answer and how you will convince your reader to agree with you. As you read more about your topic and begin writing, your answer should get more detailed.
In your essay about the internet and education, the thesis states your position and sketches out the key arguments you’ll use to support it.
The negatives of internet use are outweighed by its many benefits for education because it facilitates easier access to information.
In your essay about braille, the thesis statement summarizes the key historical development that you’ll explain.
The invention of braille in the 19th century transformed the lives of blind people, allowing them to participate more actively in public life.
A strong thesis statement should tell the reader:
- Why you hold this position
- What they’ll learn from your essay
- The key points of your argument or narrative
The final thesis statement doesn’t just state your position, but summarizes your overall argument or the entire topic you’re going to explain. To strengthen a weak thesis statement, it can help to consider the broader context of your topic.
These examples are more specific and show that you’ll explore your topic in depth.
Your thesis statement should match the goals of your essay, which vary depending on the type of essay you’re writing:
- In an argumentative essay , your thesis statement should take a strong position. Your aim in the essay is to convince your reader of this thesis based on evidence and logical reasoning.
- In an expository essay , you’ll aim to explain the facts of a topic or process. Your thesis statement doesn’t have to include a strong opinion in this case, but it should clearly state the central point you want to make, and mention the key elements you’ll explain.
If you want to know more about AI tools , college essays , or fallacies make sure to check out some of our other articles with explanations and examples or go directly to our tools!
- Ad hominem fallacy
- Post hoc fallacy
- Appeal to authority fallacy
- False cause fallacy
- Sunk cost fallacy
College essays
- Choosing Essay Topic
- Write a College Essay
- Write a Diversity Essay
- College Essay Format & Structure
- Comparing and Contrasting in an Essay
(AI) Tools
- Grammar Checker
- Paraphrasing Tool
- Text Summarizer
- AI Detector
- Plagiarism Checker
- Citation Generator
A thesis statement is a sentence that sums up the central point of your paper or essay . Everything else you write should relate to this key idea.
The thesis statement is essential in any academic essay or research paper for two main reasons:
- It gives your writing direction and focus.
- It gives the reader a concise summary of your main point.
Without a clear thesis statement, an essay can end up rambling and unfocused, leaving your reader unsure of exactly what you want to say.
Follow these four steps to come up with a thesis statement :
- Ask a question about your topic .
- Write your initial answer.
- Develop your answer by including reasons.
- Refine your answer, adding more detail and nuance.
The thesis statement should be placed at the end of your essay introduction .
Cite this Scribbr article
If you want to cite this source, you can copy and paste the citation or click the “Cite this Scribbr article” button to automatically add the citation to our free Citation Generator.
McCombes, S. (2023, August 15). How to Write a Thesis Statement | 4 Steps & Examples. Scribbr. Retrieved April 5, 2024, from https://www.scribbr.com/academic-essay/thesis-statement/
Is this article helpful?
Shona McCombes
Other students also liked, how to write an essay introduction | 4 steps & examples, how to write topic sentences | 4 steps, examples & purpose, academic paragraph structure | step-by-step guide & examples, what is your plagiarism score.
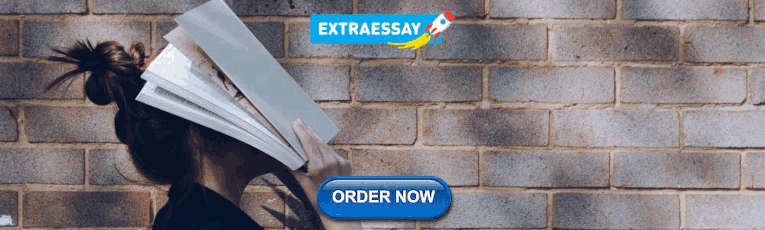
IMAGES
VIDEO
COMMENTS
A theoretical framework is a foundational review of existing theories that serves as a roadmap for developing the arguments you will use in your own work. Theories are developed by researchers to explain phenomena, draw connections, and make predictions. In a theoretical framework, you explain the existing theories that support your research ...
A theoretical framework guides the research process like a roadmap for the study, so you need to get this right. Theoretical framework 1,2 is the structure that supports and describes a theory. A theory is a set of interrelated concepts and definitions that present a systematic view of phenomena by describing the relationship among the variables for explaining these phenomena.
A theoretical framework is a foundational review of existing theories that serves as a roadmap for developing the arguments you will use in your own work. Theories are developed by researchers to explain phenomena, draw connections, and make predictions. In a theoretical framework, you explain the existing theories that support your research ...
Sample theoretical framework. Below is a simplified example showing how you can describe and compare theories in your thesis or dissertation. In this example, we focus on the concept of customer satisfaction introduced above. Example.
framework is the foundation from which all knowledge is constructed (metaphorically and literally) for a research study. It serves as the structure and support for the rationale for the study, the problem statement, the purpose, the significance, and the research questions. The theoretical framework provides a grounding base, or an anchor, for
Conclusion. A robust conceptual or theoretical framework is crucial when writing a thesis/dissertation. It defines your research gap, identifies your approach, and guides the interpretation of your results. A thesis is the most important document you will write during your academic studies.
In a thesis or dissertation, a theoretical framework is a section where the writer evaluates or discusses the most relevant theories to their study. The purpose of this section is to: Define the key concepts. Combine and evaluate relevant models and theories. Explain expectations and assumptions that guide the project.
Your theoretical framework is based on: A new boutique downtown is struggling with the fact that many of their online customers do not return to make subsequent purchases. This is a big issue for the otherwise fast-growing shop.Management wants to increase customer loyalty. They believe that improved customer satisfaction will play a major role ...
Theoretical Framework. Definition: Theoretical framework refers to a set of concepts, theories, ideas, and assumptions that serve as a foundation for understanding a particular phenomenon or problem.It provides a conceptual framework that helps researchers to design and conduct their research, as well as to analyze and interpret their findings.. In research, a theoretical framework explains ...
Resources About Theory and Theoretical Frameworks. Challenging Ideas: Theory and Empirical Research in the Social Sciences and humanities Edited by Maren Lytje, Torben K. Nielsen, and Martin Ottovay Jørgensen. Call Number: Ebook, click link to view. ISBN: 9781443887373. Publication Date: 2015.
Your theoretical framework is the blueprint for your entire dissertation and will guide you during the process of writing it. Photo by Daniel McCullough on Unsplash. With this in mind, I'm going to discuss six steps to mastering the theoretical framework section—the "blueprint" for your dissertation.
Theoretical framework vs conceptual framework. As you can see, the theoretical framework and the conceptual framework are closely related concepts, but they differ in terms of focus and purpose. The theoretical framework is used to lay down a foundation of theory on which your study will be built, whereas the conceptual framework visualises ...
Steps to Developing the Perfect Conceptual Framework. Pick a question. Conduct a literature review. Identify your variables. Create your conceptual framework. 1. Pick a Question. You should already have some idea of the broad area of your research project. Try to narrow down your research field to a manageable topic in terms of time and resources.
A thesis is a type of research paper based on your original research. It is usually submitted as the final step of a master's program or a capstone to a bachelor's degree. Writing a thesis can be a daunting experience. Other than a dissertation, it is one of the longest pieces of writing students typically complete.
A thesis is an in-depth research study that identifies a particular topic of inquiry and presents a clear argument or perspective about that topic using evidence and logic. Writing a thesis showcases your ability of critical thinking, gathering evidence, and making a compelling argument. Integral to these competencies is thorough research ...
A theoretical framework, the way I define it, is comprised of the different theories and theoretical constructs that help explain a phenomenon. A theoretical framework sets out the various expectations that a theory posits and how they would apply to a specific case under analysis, and how one would use theory to explain a particular phenomenon.
Thesis. Your thesis is the central claim in your essay—your main insight or idea about your source or topic. Your thesis should appear early in an academic essay, followed by a logically constructed argument that supports this central claim. A strong thesis is arguable, which means a thoughtful reader could disagree with it and therefore ...
A theoretical framework illuminates the phenomenon of study and the corresponding assumptions adopted by the researcher. Frameworks can take on different orientations. The conceptual framework is created by the researcher(s), includes the presumed relationships among concepts, and addresses needed areas of study discovered in literature reviews.
Developing a conceptual framework in research. Step 1: Choose your research question. Step 2: Select your independent and dependent variables. Step 3: Visualize your cause-and-effect relationship. Step 4: Identify other influencing variables. Frequently asked questions about conceptual models.
The theoretical framework is a structure that supports the theory that explains why the research problem you are studying exists. It encompasses concepts, definitions, existing theories, and other literature that you have referred to in your study. The theoretical framework demonstrates your understanding of the concepts related to the research ...
Many frameworks exist across the sciences and science-policy interface, but it is not always clear how they are developed or can be applied. It is also often vague how new or existing frameworks are positioned in a theory of science to advance a specific theory or paradigm. This article examines these questions and positions the role of frameworks as integral but often vague scientific tools ...
A thesis is a type of research paper based on your original research. It is usually submitted as the final step of a PhD program in the UK. Writing a thesis can be a daunting experience. Indeed, alongside a dissertation, it is the longest piece of writing students typically complete. It relies on your ability to conduct research from start to ...
The John Gokongwei School of Management invites you to a Brown Bag Session on "COVID-19 Blood Collection Volatility in the Philippines: A Data-Driven Disaster Preparedness and Recovery Policy Framework". Our presenter is Joel Patrick D. Caballa, Lecturer at the Department of Quantitative Methods and Information Technology.
In this thesis, I investigate various aspects of one of the most fundamental questions in thermodynamics: what state transformations can quantum systems undergo while interacting with a thermal bath under specific constraints? These constraints may involve total energy conservation, memory effects, or finite-size considerations. Addressing this question leads to (i) a characterisation of the ...
The remainder of this paper is structured as follows. First, the fundamentals of lung sounds are presented. Then, the existing digital and wireless stethoscopes that can be used for clinical purposes are described, followed by an overview of the framework of deep learning in lung sound analysis including the main tasks, preprocessing, public datasets, and related research.
Step 2: Write your initial answer. After some initial research, you can formulate a tentative answer to this question. At this stage it can be simple, and it should guide the research process and writing process. The internet has had more of a positive than a negative effect on education.