
- Teesside University Student & Library Services
- Learning Hub Group
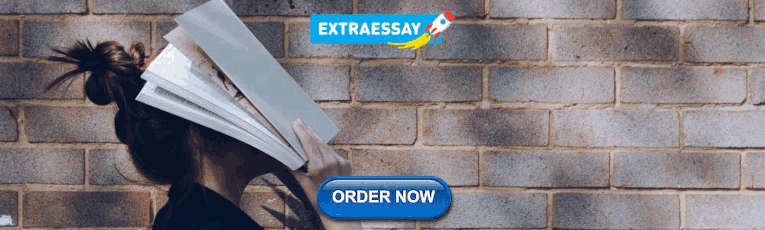
Critical Appraisal for Health Students
- Critical Appraisal of a quantitative paper
- Critical Appraisal: Help
- Critical Appraisal of a qualitative paper
- Useful resources
Appraisal of a Quantitative paper: Top tips

- Introduction
Critical appraisal of a quantitative paper (RCT)
This guide, aimed at health students, provides basic level support for appraising quantitative research papers. It's designed for students who have already attended lectures on critical appraisal. One framework for appraising quantitative research (based on reliability, internal and external validity) is provided and there is an opportunity to practise the technique on a sample article.
Please note this framework is for appraising one particular type of quantitative research a Randomised Controlled Trial (RCT) which is defined as
a trial in which participants are randomly assigned to one of two or more groups: the experimental group or groups receive the intervention or interventions being tested; the comparison group (control group) receive usual care or no treatment or a placebo. The groups are then followed up to see if there are any differences between the results. This helps in assessing the effectiveness of the intervention.(CASP, 2020)
Support materials
- Framework for reading quantitative papers (RCTs)
- Critical appraisal of a quantitative paper PowerPoint
To practise following this framework for critically appraising a quantitative article, please look at the following article:
Marrero, D.G. et al (2016) 'Comparison of commercial and self-initiated weight loss programs in people with prediabetes: a randomized control trial', AJPH Research , 106(5), pp. 949-956.
Critical Appraisal of a quantitative paper (RCT): practical example
- Internal Validity
- External Validity
- Reliability Measurement Tool
How to use this practical example
Using the framework, you can have a go at appraising a quantitative paper - we are going to look at the following article:
Marrero, d.g. et al (2016) 'comparison of commercial and self-initiated weight loss programs in people with prediabetes: a randomized control trial', ajph research , 106(5), pp. 949-956., step 1. take a quick look at the article, step 2. click on the internal validity tab above - there are questions to help you appraise the article, read the questions and look for the answers in the article. , step 3. click on each question and our answers will appear., step 4. repeat with the other aspects of external validity and reliability. , questioning the internal validity:, randomisation : how were participants allocated to each group did a randomisation process taken place, comparability of groups: how similar were the groups eg age, sex, ethnicity – is this made clear, blinding (none, single, double or triple): who was not aware of which group a patient was in (eg nobody, only patient, patient and clinician, patient, clinician and researcher) was it feasible for more blinding to have taken place , equal treatment of groups: were both groups treated in the same way , attrition : what percentage of participants dropped out did this adversely affect one group has this been evaluated, overall internal validity: does the research measure what it is supposed to be measuring, questioning the external validity:, attrition: was everyone accounted for at the end of the study was any attempt made to contact drop-outs, sampling approach: how was the sample selected was it based on probability or non-probability what was the approach (eg simple random, convenience) was this an appropriate approach, sample size (power calculation): how many participants was a sample size calculation performed did the study pass, exclusion/ inclusion criteria: were the criteria set out clearly were they based on recognised diagnostic criteria, what is the overall external validity can the results be applied to the wider population, questioning the reliability (measurement tool) internal validity:, internal consistency reliability (cronbach’s alpha). has a cronbach’s alpha score of 0.7 or above been included, test re-test reliability correlation. was the test repeated more than once were the same results received has a correlation coefficient been reported is it above 0.7 , validity of measurement tool. is it an established tool if not what has been done to check if it is reliable pilot study expert panel literature review criterion validity (test against other tools): has a criterion validity comparison been carried out was the score above 0.7, what is the overall reliability how consistent are the measurements , overall validity and reliability:, overall how valid and reliable is the paper.
- << Previous: Critical Appraisal of a qualitative paper
- Next: Useful resources >>
- Last Updated: Aug 25, 2023 2:48 PM
- URL: https://libguides.tees.ac.uk/critical_appraisal
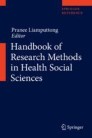
Handbook of Research Methods in Health Social Sciences pp 27–49 Cite as
Quantitative Research
- Leigh A. Wilson 2 , 3
- Reference work entry
- First Online: 13 January 2019
4075 Accesses
4 Citations
Quantitative research methods are concerned with the planning, design, and implementation of strategies to collect and analyze data. Descartes, the seventeenth-century philosopher, suggested that how the results are achieved is often more important than the results themselves, as the journey taken along the research path is a journey of discovery. High-quality quantitative research is characterized by the attention given to the methods and the reliability of the tools used to collect the data. The ability to critique research in a systematic way is an essential component of a health professional’s role in order to deliver high quality, evidence-based healthcare. This chapter is intended to provide a simple overview of the way new researchers and health practitioners can understand and employ quantitative methods. The chapter offers practical, realistic guidance in a learner-friendly way and uses a logical sequence to understand the process of hypothesis development, study design, data collection and handling, and finally data analysis and interpretation.
- Quantitative
- Epidemiology
- Data analysis
- Methodology
- Interpretation
This is a preview of subscription content, log in via an institution .
Buying options
- Available as PDF
- Read on any device
- Instant download
- Own it forever
- Available as EPUB and PDF
- Durable hardcover edition
- Dispatched in 3 to 5 business days
- Free shipping worldwide - see info
Tax calculation will be finalised at checkout
Purchases are for personal use only
Babbie ER. The practice of social research. 14th ed. Belmont: Wadsworth Cengage; 2016.
Google Scholar
Descartes. Cited in Halverston, W. (1976). In: A concise introduction to philosophy, 3rd ed. New York: Random House; 1637.
Doll R, Hill AB. The mortality of doctors in relation to their smoking habits. BMJ. 1954;328(7455):1529–33. https://doi.org/10.1136/bmj.328.7455.1529 .
Article Google Scholar
Liamputtong P. Research methods in health: foundations for evidence-based practice. 3rd ed. Melbourne: Oxford University Press; 2017.
McNabb DE. Research methods in public administration and nonprofit management: quantitative and qualitative approaches. 2nd ed. New York: Armonk; 2007.
Merriam-Webster. Dictionary. http://www.merriam-webster.com . Accessed 20th December 2017.
Olesen Larsen P, von Ins M. The rate of growth in scientific publication and the decline in coverage provided by Science Citation Index. Scientometrics. 2010;84(3):575–603.
Pannucci CJ, Wilkins EG. Identifying and avoiding bias in research. Plast Reconstr Surg. 2010;126(2):619–25. https://doi.org/10.1097/PRS.0b013e3181de24bc .
Petrie A, Sabin C. Medical statistics at a glance. 2nd ed. London: Blackwell Publishing; 2005.
Portney LG, Watkins MP. Foundations of clinical research: applications to practice. 3rd ed. New Jersey: Pearson Publishing; 2009.
Sheehan J. Aspects of research methodology. Nurse Educ Today. 1986;6:193–203.
Wilson LA, Black DA. Health, science research and research methods. Sydney: McGraw Hill; 2013.
Download references
Author information
Authors and affiliations.
School of Science and Health, Western Sydney University, Penrith, NSW, Australia
Leigh A. Wilson
Faculty of Health Science, Discipline of Behavioural and Social Sciences in Health, University of Sydney, Lidcombe, NSW, Australia
You can also search for this author in PubMed Google Scholar
Corresponding author
Correspondence to Leigh A. Wilson .
Editor information
Editors and affiliations.
Pranee Liamputtong
Rights and permissions
Reprints and permissions
Copyright information
© 2019 Springer Nature Singapore Pte Ltd.
About this entry
Cite this entry.
Wilson, L.A. (2019). Quantitative Research. In: Liamputtong, P. (eds) Handbook of Research Methods in Health Social Sciences. Springer, Singapore. https://doi.org/10.1007/978-981-10-5251-4_54
Download citation
DOI : https://doi.org/10.1007/978-981-10-5251-4_54
Published : 13 January 2019
Publisher Name : Springer, Singapore
Print ISBN : 978-981-10-5250-7
Online ISBN : 978-981-10-5251-4
eBook Packages : Social Sciences Reference Module Humanities and Social Sciences Reference Module Business, Economics and Social Sciences
Share this entry
Anyone you share the following link with will be able to read this content:
Sorry, a shareable link is not currently available for this article.
Provided by the Springer Nature SharedIt content-sharing initiative
- Publish with us
Policies and ethics
- Find a journal
- Track your research
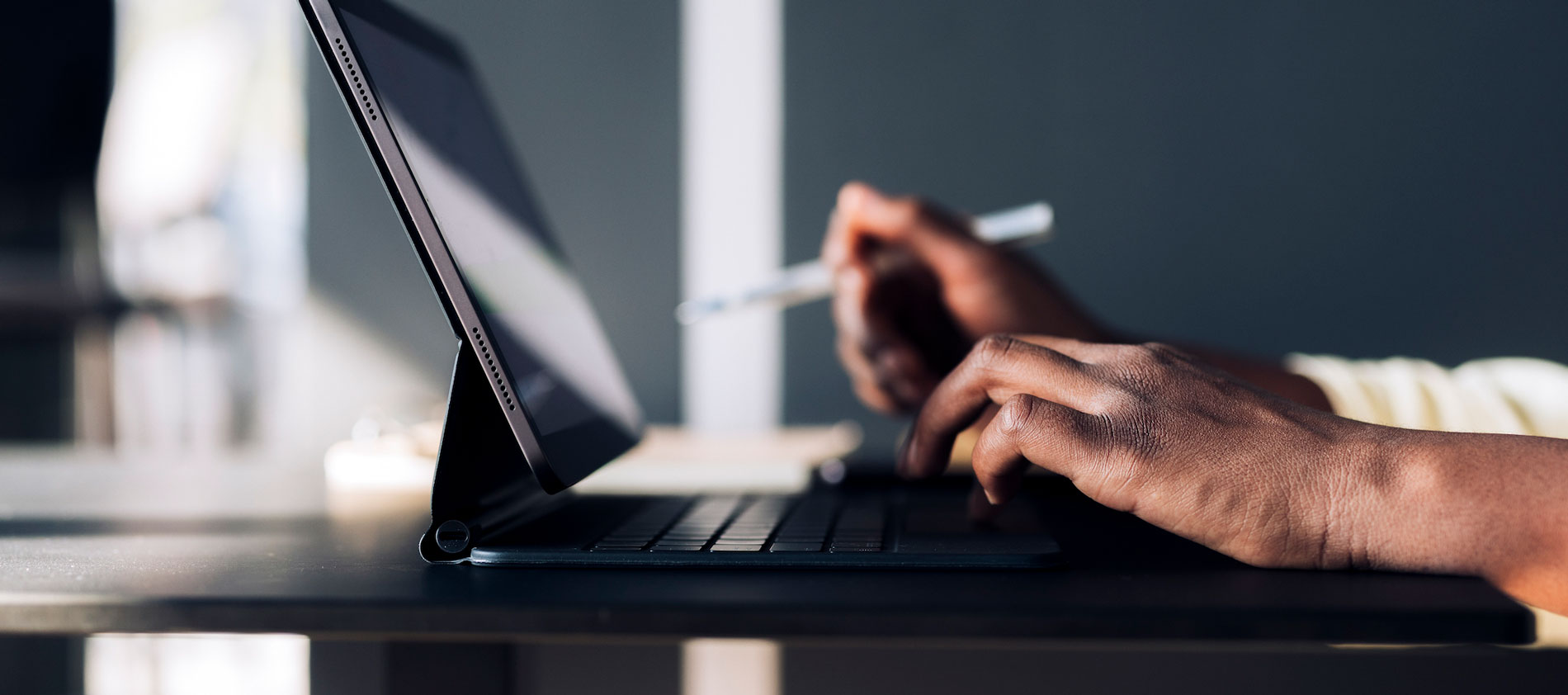
Critical Appraisal Tools
Jbi’s critical appraisal tools assist in assessing the trustworthiness, relevance and results of published papers..
These tools have been revised. Recently published articles detail the revision.
"Assessing the risk of bias of quantitative analytical studies: introducing the vision for critical appraisal within JBI systematic reviews"
"revising the jbi quantitative critical appraisal tools to improve their applicability: an overview of methods and the development process".
End to end support for developing systematic reviews
Analytical Cross Sectional Studies
Checklist for analytical cross sectional studies, how to cite, associated publication(s), case control studies , checklist for case control studies, case reports , checklist for case reports, case series , checklist for case series.
Munn Z, Barker TH, Moola S, Tufanaru C, Stern C, McArthur A, Stephenson M, Aromataris E. Methodological quality of case series studies: an introduction to the JBI critical appraisal tool. JBI Evidence Synthesis. 2020;18(10):2127-2133
Methodological quality of case series studies: an introduction to the JBI critical appraisal tool
Cohort studies , checklist for cohort studies, diagnostic test accuracy studies , checklist for diagnostic test accuracy studies.
Campbell JM, Klugar M, Ding S, Carmody DP, Hakonsen SJ, Jadotte YT, White S, Munn Z. Chapter 9: Diagnostic test accuracy systematic reviews. In: Aromataris E, Munn Z (Editors). JBI Manual for Evidence Synthesis. JBI, 2020
JBI Manual for Evidence Synthesis
Chapter 9: Diagnostic test accuracy systematic reviews
Economic Evaluations
Checklist for economic evaluations, prevalence studies , checklist for prevalence studies.
Munn Z, Moola S, Lisy K, Riitano D, Tufanaru C. Chapter 5: Systematic reviews of prevalence and incidence. In: Aromataris E, Munn Z (Editors). JBI Manual for Evidence Synthesis. JBI, 2020
Chapter 5: Systematic reviews of prevalence and incidence
Qualitative Research
Checklist for qualitative research.
Lockwood C, Munn Z, Porritt K. Qualitative research synthesis: methodological guidance for systematic reviewers utilizing meta-aggregation. Int J Evid Based Healthc. 2015;13(3):179–187
Chapter 2: Systematic reviews of qualitative evidence
Qualitative research synthesis
Methodological guidance for systematic reviewers utilizing meta-aggregation
Quasi-Experimental Studies
Checklist for quasi-experimental studies.
Barker TH, Habibi N, Aromataris E, Stone JC, Leonardi-Bee J, Sears K, et al. The revised JBI critical appraisal tool for the assessment of risk of bias quasi-experimental studies. JBI Evid Synth. 2024;22(3):378-88.
The revised JBI critical appraisal tool for the assessment of risk of bias for quasi-experimental studies
Randomized controlled trials , randomized controlled trials.
Barker TH, Stone JC, Sears K, Klugar M, Tufanaru C, Leonardi-Bee J, Aromataris E, Munn Z. The revised JBI critical appraisal tool for the assessment of risk of bias for randomized controlled trials. JBI Evidence Synthesis. 2023;21(3):494-506
The revised JBI critical appraisal tool for the assessment of risk of bias for randomized controlled trials
Randomized controlled trials checklist (archive), systematic reviews , checklist for systematic reviews.
Aromataris E, Fernandez R, Godfrey C, Holly C, Kahlil H, Tungpunkom P. Summarizing systematic reviews: methodological development, conduct and reporting of an Umbrella review approach. Int J Evid Based Healthc. 2015;13(3):132-40.
Chapter 10: Umbrella Reviews
Textual Evidence: Expert Opinion
Checklist for textual evidence: expert opinion.
McArthur A, Klugarova J, Yan H, Florescu S. Chapter 4: Systematic reviews of text and opinion. In: Aromataris E, Munn Z (Editors). JBI Manual for Evidence Synthesis. JBI, 2020
Chapter 4: Systematic reviews of text and opinion
Textual Evidence: Narrative
Checklist for textual evidence: narrative, textual evidence: policy , checklist for textual evidence: policy.
Have a language expert improve your writing
Run a free plagiarism check in 10 minutes, generate accurate citations for free.
- Knowledge Base
Methodology
- What Is Quantitative Research? | Definition, Uses & Methods
What Is Quantitative Research? | Definition, Uses & Methods
Published on June 12, 2020 by Pritha Bhandari . Revised on June 22, 2023.
Quantitative research is the process of collecting and analyzing numerical data. It can be used to find patterns and averages, make predictions, test causal relationships, and generalize results to wider populations.
Quantitative research is the opposite of qualitative research , which involves collecting and analyzing non-numerical data (e.g., text, video, or audio).
Quantitative research is widely used in the natural and social sciences: biology, chemistry, psychology, economics, sociology, marketing, etc.
- What is the demographic makeup of Singapore in 2020?
- How has the average temperature changed globally over the last century?
- Does environmental pollution affect the prevalence of honey bees?
- Does working from home increase productivity for people with long commutes?
Table of contents
Quantitative research methods, quantitative data analysis, advantages of quantitative research, disadvantages of quantitative research, other interesting articles, frequently asked questions about quantitative research.
You can use quantitative research methods for descriptive, correlational or experimental research.
- In descriptive research , you simply seek an overall summary of your study variables.
- In correlational research , you investigate relationships between your study variables.
- In experimental research , you systematically examine whether there is a cause-and-effect relationship between variables.
Correlational and experimental research can both be used to formally test hypotheses , or predictions, using statistics. The results may be generalized to broader populations based on the sampling method used.
To collect quantitative data, you will often need to use operational definitions that translate abstract concepts (e.g., mood) into observable and quantifiable measures (e.g., self-ratings of feelings and energy levels).
Note that quantitative research is at risk for certain research biases , including information bias , omitted variable bias , sampling bias , or selection bias . Be sure that you’re aware of potential biases as you collect and analyze your data to prevent them from impacting your work too much.
Here's why students love Scribbr's proofreading services
Discover proofreading & editing
Once data is collected, you may need to process it before it can be analyzed. For example, survey and test data may need to be transformed from words to numbers. Then, you can use statistical analysis to answer your research questions .
Descriptive statistics will give you a summary of your data and include measures of averages and variability. You can also use graphs, scatter plots and frequency tables to visualize your data and check for any trends or outliers.
Using inferential statistics , you can make predictions or generalizations based on your data. You can test your hypothesis or use your sample data to estimate the population parameter .
First, you use descriptive statistics to get a summary of the data. You find the mean (average) and the mode (most frequent rating) of procrastination of the two groups, and plot the data to see if there are any outliers.
You can also assess the reliability and validity of your data collection methods to indicate how consistently and accurately your methods actually measured what you wanted them to.
Quantitative research is often used to standardize data collection and generalize findings . Strengths of this approach include:
- Replication
Repeating the study is possible because of standardized data collection protocols and tangible definitions of abstract concepts.
- Direct comparisons of results
The study can be reproduced in other cultural settings, times or with different groups of participants. Results can be compared statistically.
- Large samples
Data from large samples can be processed and analyzed using reliable and consistent procedures through quantitative data analysis.
- Hypothesis testing
Using formalized and established hypothesis testing procedures means that you have to carefully consider and report your research variables, predictions, data collection and testing methods before coming to a conclusion.
Despite the benefits of quantitative research, it is sometimes inadequate in explaining complex research topics. Its limitations include:
- Superficiality
Using precise and restrictive operational definitions may inadequately represent complex concepts. For example, the concept of mood may be represented with just a number in quantitative research, but explained with elaboration in qualitative research.
- Narrow focus
Predetermined variables and measurement procedures can mean that you ignore other relevant observations.
- Structural bias
Despite standardized procedures, structural biases can still affect quantitative research. Missing data , imprecise measurements or inappropriate sampling methods are biases that can lead to the wrong conclusions.
- Lack of context
Quantitative research often uses unnatural settings like laboratories or fails to consider historical and cultural contexts that may affect data collection and results.
If you want to know more about statistics , methodology , or research bias , make sure to check out some of our other articles with explanations and examples.
- Chi square goodness of fit test
- Degrees of freedom
- Null hypothesis
- Discourse analysis
- Control groups
- Mixed methods research
- Non-probability sampling
- Inclusion and exclusion criteria
Research bias
- Rosenthal effect
- Implicit bias
- Cognitive bias
- Selection bias
- Negativity bias
- Status quo bias
Quantitative research deals with numbers and statistics, while qualitative research deals with words and meanings.
Quantitative methods allow you to systematically measure variables and test hypotheses . Qualitative methods allow you to explore concepts and experiences in more detail.
In mixed methods research , you use both qualitative and quantitative data collection and analysis methods to answer your research question .
Data collection is the systematic process by which observations or measurements are gathered in research. It is used in many different contexts by academics, governments, businesses, and other organizations.
Operationalization means turning abstract conceptual ideas into measurable observations.
For example, the concept of social anxiety isn’t directly observable, but it can be operationally defined in terms of self-rating scores, behavioral avoidance of crowded places, or physical anxiety symptoms in social situations.
Before collecting data , it’s important to consider how you will operationalize the variables that you want to measure.
Reliability and validity are both about how well a method measures something:
- Reliability refers to the consistency of a measure (whether the results can be reproduced under the same conditions).
- Validity refers to the accuracy of a measure (whether the results really do represent what they are supposed to measure).
If you are doing experimental research, you also have to consider the internal and external validity of your experiment.
Hypothesis testing is a formal procedure for investigating our ideas about the world using statistics. It is used by scientists to test specific predictions, called hypotheses , by calculating how likely it is that a pattern or relationship between variables could have arisen by chance.
Cite this Scribbr article
If you want to cite this source, you can copy and paste the citation or click the “Cite this Scribbr article” button to automatically add the citation to our free Citation Generator.
Bhandari, P. (2023, June 22). What Is Quantitative Research? | Definition, Uses & Methods. Scribbr. Retrieved April 8, 2024, from https://www.scribbr.com/methodology/quantitative-research/
Is this article helpful?
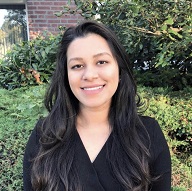
Pritha Bhandari
Other students also liked, descriptive statistics | definitions, types, examples, inferential statistics | an easy introduction & examples, unlimited academic ai-proofreading.
✔ Document error-free in 5minutes ✔ Unlimited document corrections ✔ Specialized in correcting academic texts
- Privacy Policy
Buy Me a Coffee

Home » Quantitative Research – Methods, Types and Analysis
Quantitative Research – Methods, Types and Analysis
Table of Contents

Quantitative Research
Quantitative research is a type of research that collects and analyzes numerical data to test hypotheses and answer research questions . This research typically involves a large sample size and uses statistical analysis to make inferences about a population based on the data collected. It often involves the use of surveys, experiments, or other structured data collection methods to gather quantitative data.
Quantitative Research Methods

Quantitative Research Methods are as follows:
Descriptive Research Design
Descriptive research design is used to describe the characteristics of a population or phenomenon being studied. This research method is used to answer the questions of what, where, when, and how. Descriptive research designs use a variety of methods such as observation, case studies, and surveys to collect data. The data is then analyzed using statistical tools to identify patterns and relationships.
Correlational Research Design
Correlational research design is used to investigate the relationship between two or more variables. Researchers use correlational research to determine whether a relationship exists between variables and to what extent they are related. This research method involves collecting data from a sample and analyzing it using statistical tools such as correlation coefficients.
Quasi-experimental Research Design
Quasi-experimental research design is used to investigate cause-and-effect relationships between variables. This research method is similar to experimental research design, but it lacks full control over the independent variable. Researchers use quasi-experimental research designs when it is not feasible or ethical to manipulate the independent variable.
Experimental Research Design
Experimental research design is used to investigate cause-and-effect relationships between variables. This research method involves manipulating the independent variable and observing the effects on the dependent variable. Researchers use experimental research designs to test hypotheses and establish cause-and-effect relationships.
Survey Research
Survey research involves collecting data from a sample of individuals using a standardized questionnaire. This research method is used to gather information on attitudes, beliefs, and behaviors of individuals. Researchers use survey research to collect data quickly and efficiently from a large sample size. Survey research can be conducted through various methods such as online, phone, mail, or in-person interviews.
Quantitative Research Analysis Methods
Here are some commonly used quantitative research analysis methods:
Statistical Analysis
Statistical analysis is the most common quantitative research analysis method. It involves using statistical tools and techniques to analyze the numerical data collected during the research process. Statistical analysis can be used to identify patterns, trends, and relationships between variables, and to test hypotheses and theories.
Regression Analysis
Regression analysis is a statistical technique used to analyze the relationship between one dependent variable and one or more independent variables. Researchers use regression analysis to identify and quantify the impact of independent variables on the dependent variable.
Factor Analysis
Factor analysis is a statistical technique used to identify underlying factors that explain the correlations among a set of variables. Researchers use factor analysis to reduce a large number of variables to a smaller set of factors that capture the most important information.
Structural Equation Modeling
Structural equation modeling is a statistical technique used to test complex relationships between variables. It involves specifying a model that includes both observed and unobserved variables, and then using statistical methods to test the fit of the model to the data.
Time Series Analysis
Time series analysis is a statistical technique used to analyze data that is collected over time. It involves identifying patterns and trends in the data, as well as any seasonal or cyclical variations.
Multilevel Modeling
Multilevel modeling is a statistical technique used to analyze data that is nested within multiple levels. For example, researchers might use multilevel modeling to analyze data that is collected from individuals who are nested within groups, such as students nested within schools.
Applications of Quantitative Research
Quantitative research has many applications across a wide range of fields. Here are some common examples:
- Market Research : Quantitative research is used extensively in market research to understand consumer behavior, preferences, and trends. Researchers use surveys, experiments, and other quantitative methods to collect data that can inform marketing strategies, product development, and pricing decisions.
- Health Research: Quantitative research is used in health research to study the effectiveness of medical treatments, identify risk factors for diseases, and track health outcomes over time. Researchers use statistical methods to analyze data from clinical trials, surveys, and other sources to inform medical practice and policy.
- Social Science Research: Quantitative research is used in social science research to study human behavior, attitudes, and social structures. Researchers use surveys, experiments, and other quantitative methods to collect data that can inform social policies, educational programs, and community interventions.
- Education Research: Quantitative research is used in education research to study the effectiveness of teaching methods, assess student learning outcomes, and identify factors that influence student success. Researchers use experimental and quasi-experimental designs, as well as surveys and other quantitative methods, to collect and analyze data.
- Environmental Research: Quantitative research is used in environmental research to study the impact of human activities on the environment, assess the effectiveness of conservation strategies, and identify ways to reduce environmental risks. Researchers use statistical methods to analyze data from field studies, experiments, and other sources.
Characteristics of Quantitative Research
Here are some key characteristics of quantitative research:
- Numerical data : Quantitative research involves collecting numerical data through standardized methods such as surveys, experiments, and observational studies. This data is analyzed using statistical methods to identify patterns and relationships.
- Large sample size: Quantitative research often involves collecting data from a large sample of individuals or groups in order to increase the reliability and generalizability of the findings.
- Objective approach: Quantitative research aims to be objective and impartial in its approach, focusing on the collection and analysis of data rather than personal beliefs, opinions, or experiences.
- Control over variables: Quantitative research often involves manipulating variables to test hypotheses and establish cause-and-effect relationships. Researchers aim to control for extraneous variables that may impact the results.
- Replicable : Quantitative research aims to be replicable, meaning that other researchers should be able to conduct similar studies and obtain similar results using the same methods.
- Statistical analysis: Quantitative research involves using statistical tools and techniques to analyze the numerical data collected during the research process. Statistical analysis allows researchers to identify patterns, trends, and relationships between variables, and to test hypotheses and theories.
- Generalizability: Quantitative research aims to produce findings that can be generalized to larger populations beyond the specific sample studied. This is achieved through the use of random sampling methods and statistical inference.
Examples of Quantitative Research
Here are some examples of quantitative research in different fields:
- Market Research: A company conducts a survey of 1000 consumers to determine their brand awareness and preferences. The data is analyzed using statistical methods to identify trends and patterns that can inform marketing strategies.
- Health Research : A researcher conducts a randomized controlled trial to test the effectiveness of a new drug for treating a particular medical condition. The study involves collecting data from a large sample of patients and analyzing the results using statistical methods.
- Social Science Research : A sociologist conducts a survey of 500 people to study attitudes toward immigration in a particular country. The data is analyzed using statistical methods to identify factors that influence these attitudes.
- Education Research: A researcher conducts an experiment to compare the effectiveness of two different teaching methods for improving student learning outcomes. The study involves randomly assigning students to different groups and collecting data on their performance on standardized tests.
- Environmental Research : A team of researchers conduct a study to investigate the impact of climate change on the distribution and abundance of a particular species of plant or animal. The study involves collecting data on environmental factors and population sizes over time and analyzing the results using statistical methods.
- Psychology : A researcher conducts a survey of 500 college students to investigate the relationship between social media use and mental health. The data is analyzed using statistical methods to identify correlations and potential causal relationships.
- Political Science: A team of researchers conducts a study to investigate voter behavior during an election. They use survey methods to collect data on voting patterns, demographics, and political attitudes, and analyze the results using statistical methods.
How to Conduct Quantitative Research
Here is a general overview of how to conduct quantitative research:
- Develop a research question: The first step in conducting quantitative research is to develop a clear and specific research question. This question should be based on a gap in existing knowledge, and should be answerable using quantitative methods.
- Develop a research design: Once you have a research question, you will need to develop a research design. This involves deciding on the appropriate methods to collect data, such as surveys, experiments, or observational studies. You will also need to determine the appropriate sample size, data collection instruments, and data analysis techniques.
- Collect data: The next step is to collect data. This may involve administering surveys or questionnaires, conducting experiments, or gathering data from existing sources. It is important to use standardized methods to ensure that the data is reliable and valid.
- Analyze data : Once the data has been collected, it is time to analyze it. This involves using statistical methods to identify patterns, trends, and relationships between variables. Common statistical techniques include correlation analysis, regression analysis, and hypothesis testing.
- Interpret results: After analyzing the data, you will need to interpret the results. This involves identifying the key findings, determining their significance, and drawing conclusions based on the data.
- Communicate findings: Finally, you will need to communicate your findings. This may involve writing a research report, presenting at a conference, or publishing in a peer-reviewed journal. It is important to clearly communicate the research question, methods, results, and conclusions to ensure that others can understand and replicate your research.
When to use Quantitative Research
Here are some situations when quantitative research can be appropriate:
- To test a hypothesis: Quantitative research is often used to test a hypothesis or a theory. It involves collecting numerical data and using statistical analysis to determine if the data supports or refutes the hypothesis.
- To generalize findings: If you want to generalize the findings of your study to a larger population, quantitative research can be useful. This is because it allows you to collect numerical data from a representative sample of the population and use statistical analysis to make inferences about the population as a whole.
- To measure relationships between variables: If you want to measure the relationship between two or more variables, such as the relationship between age and income, or between education level and job satisfaction, quantitative research can be useful. It allows you to collect numerical data on both variables and use statistical analysis to determine the strength and direction of the relationship.
- To identify patterns or trends: Quantitative research can be useful for identifying patterns or trends in data. For example, you can use quantitative research to identify trends in consumer behavior or to identify patterns in stock market data.
- To quantify attitudes or opinions : If you want to measure attitudes or opinions on a particular topic, quantitative research can be useful. It allows you to collect numerical data using surveys or questionnaires and analyze the data using statistical methods to determine the prevalence of certain attitudes or opinions.
Purpose of Quantitative Research
The purpose of quantitative research is to systematically investigate and measure the relationships between variables or phenomena using numerical data and statistical analysis. The main objectives of quantitative research include:
- Description : To provide a detailed and accurate description of a particular phenomenon or population.
- Explanation : To explain the reasons for the occurrence of a particular phenomenon, such as identifying the factors that influence a behavior or attitude.
- Prediction : To predict future trends or behaviors based on past patterns and relationships between variables.
- Control : To identify the best strategies for controlling or influencing a particular outcome or behavior.
Quantitative research is used in many different fields, including social sciences, business, engineering, and health sciences. It can be used to investigate a wide range of phenomena, from human behavior and attitudes to physical and biological processes. The purpose of quantitative research is to provide reliable and valid data that can be used to inform decision-making and improve understanding of the world around us.
Advantages of Quantitative Research
There are several advantages of quantitative research, including:
- Objectivity : Quantitative research is based on objective data and statistical analysis, which reduces the potential for bias or subjectivity in the research process.
- Reproducibility : Because quantitative research involves standardized methods and measurements, it is more likely to be reproducible and reliable.
- Generalizability : Quantitative research allows for generalizations to be made about a population based on a representative sample, which can inform decision-making and policy development.
- Precision : Quantitative research allows for precise measurement and analysis of data, which can provide a more accurate understanding of phenomena and relationships between variables.
- Efficiency : Quantitative research can be conducted relatively quickly and efficiently, especially when compared to qualitative research, which may involve lengthy data collection and analysis.
- Large sample sizes : Quantitative research can accommodate large sample sizes, which can increase the representativeness and generalizability of the results.
Limitations of Quantitative Research
There are several limitations of quantitative research, including:
- Limited understanding of context: Quantitative research typically focuses on numerical data and statistical analysis, which may not provide a comprehensive understanding of the context or underlying factors that influence a phenomenon.
- Simplification of complex phenomena: Quantitative research often involves simplifying complex phenomena into measurable variables, which may not capture the full complexity of the phenomenon being studied.
- Potential for researcher bias: Although quantitative research aims to be objective, there is still the potential for researcher bias in areas such as sampling, data collection, and data analysis.
- Limited ability to explore new ideas: Quantitative research is often based on pre-determined research questions and hypotheses, which may limit the ability to explore new ideas or unexpected findings.
- Limited ability to capture subjective experiences : Quantitative research is typically focused on objective data and may not capture the subjective experiences of individuals or groups being studied.
- Ethical concerns : Quantitative research may raise ethical concerns, such as invasion of privacy or the potential for harm to participants.
About the author
Muhammad Hassan
Researcher, Academic Writer, Web developer
You may also like

Questionnaire – Definition, Types, and Examples

Case Study – Methods, Examples and Guide

Observational Research – Methods and Guide

Qualitative Research Methods

Explanatory Research – Types, Methods, Guide

Survey Research – Types, Methods, Examples

An official website of the United States government
The .gov means it’s official. Federal government websites often end in .gov or .mil. Before sharing sensitive information, make sure you’re on a federal government site.
The site is secure. The https:// ensures that you are connecting to the official website and that any information you provide is encrypted and transmitted securely.
- Publications
- Account settings
Preview improvements coming to the PMC website in October 2024. Learn More or Try it out now .
- Advanced Search
- Journal List
- Indian J Crit Care Med
- v.24(Suppl 4); 2020 Sep
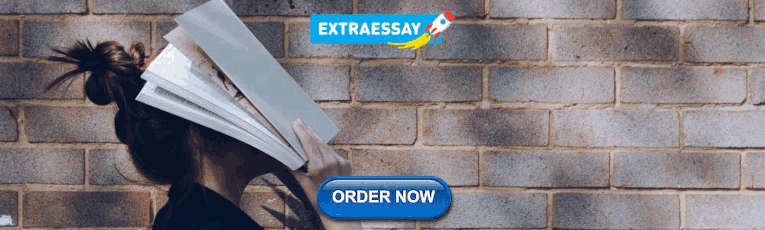
Critical Analysis of a Randomized Controlled Trial
Balkrishna d nimavat.
1 Critical Care Unit, Sir HN Reliance Hospital, Ahmedabad, Gujarat, India
Kapil G Zirpe
2,3 Department of Neuro Trauma Unit, Grant Medical Foundation, Pune, Maharashtra, India
Sushma K Gurav
In the era of evidence-based medicine, healthcare professionals are bombarded with plenty of trials and articles of which randomized control trial is considered as the epitome of all in terms of level of evidence. It is very crucial to learn the skill of balancing knowledge of randomized control trial and to avoid misinterpretation of trial result in clinical practice. There are various methods and steps to critically appraise the randomized control trial, but those are overly complex to interpret. There should be more simplified and pragmatic approach for analysis of randomized controlled trial. In this article, we like to summarize few of the practical points under 5 headings: “5 ‘Rs’ of critical analysis of randomized control trial” which encompass Right Question, Right Population, Right Study Design, Right Data, and Right Interpretation. This article gives us insight that analysis of randomized control trial should not only based on statistical findings or results but also on systematically reviewing its core question, relevant population selection, robustness of study design, and right interpretation of outcome.
How to cite this article: Nimavat BD, Zirpe KG, Gurav SK. Critical Analysis of a Randomized Controlled Trial. Indian J Crit Care Med 2020;24(Suppl 4):S215–S222.
I ntroduction
“ Statistics are like bikinis. What they reveal is suggestive, but what they conceal is vital .” [Aaron Levenstein]
Being up to date with knowledge is pivotal in world of evidence-based medicine. Sometimes, it is also crucial in terms of medicolegal aspect and to improve best current practice. In view of this background, plenty of articles and trials are emerging out in various journals every day. Among all types of study design, randomized control trial (RCT) is considered as supreme in terms of strength of evidence. Appropriately planned and vigorously conducted RCT is the best study design to see the intervention-related outcome difference, but simultaneously poorly conducted biased RCTs will misguide the reader. It is ideal to read RCTs and optimize clinical practice, but it is critical to understand strong and weak points of those RCTs before being dogmatic about their result or conclusion. There are many methods to appraise the RCTs, but in this article, I tried to simplify the points under 5 headings with mnemonic 5‘Rs’ that helps to understand things in better way ( Flowchart 1 ).

Presentation of “critical analysis of RCT”
S teps for C ritical A nalysis of R andomized C ontrol T rials
Formulate right question/address right question.
As Claude Lévi-Strauss said, “The scientist is not a person who gives the right answers, he is one who asks the right questions.”
It is crucial to look for right question that possesses characteristic such as innovative, practice changing, knowledge amplifying, and above all having some biological plausibility.
Does Randomized Control Trial Address New/Relevant Question? Does Answer to this Question Lead to More Information that will Help to Improve Current Clinical Practice or Knowledge?
Questions arises from any of topic are mostly of two types: background questions and foreground questions. RCTs are the experimental design that usually target foreground questions that are more specific to establish intervention/drug and their effect/outcome relationship. Foreground research question has four components to get relevant information like Population, Intervention, Control, and Outcome (PICO format). Whether study question and design are ethical and feasible for relevant population can be decided by FINER criterial. 1
Outcome are the variables that are monitored during study to observe presence/absence of impact of intervention on desired population. Outcome is also labeled as events or end points. Most common clinical end points are mortality, morbidity, and quality of life. It is decisive to choose right end point with their background knowledge and its relevance to formulated question ( Fig. 1 ). 2 – 4

Types of endpoint and their pros and cons
So, it is evident that no single end point is perfect, but end points should be accessed in the context of clinical question, power, and randomization.
Is Cause and Effect Having Biological Plausibility?
Biological plausibility is one of the essential components to establish that correlation means causation. Just mere association or having significant p -value without biological plausibility is like beating a dead horse (purely punitive). That means statistically significant data make least sense or should be interpreted with caution if they lack biological plausibility, and data that are unable to give statistical significance but have strong biological plausibility with vigorously conducted study should be evaluated again and discussed before rejection. 5
To determine whether correlation is equivalent to causation, many criteria and methods are available. One of such criteria is Bradford Hill criteria. It is also important to understand that knowledge of biological plausibility is dynamic and evolves with time. It is possible that there is true causation, but biological knowledge at that time is unable to explain it ( Table 1 ).
Factors help to formulate sound question 1 , 6
Right Population
Define target population/does sample truly represent population.
RCTs are usually conducted on group of people (sample) rather than whole population. It is important for the trial that selected sample truly represents the baseline characteristic of the rest of the population. Inferential leap or generalization from samples to population is also not that simple and most of the time not full proof.
External validity in RCT represents at what extent the study result can be generalized to real-world population. Internal validity gives idea about how vigorous trial is conducted and generates robust data. If RCTs have poor internal validity, result made on that trial cannot be used firmly due to higher chances poor quality data and higher chances of bias for that given sample. Limitation of external validity means trial sample or defined sample is not true representative of rest of population. In a simplified way, if internal validity is questionable, applying it on larger scale is irrelevant, and second if trial having limited external validity (by having large exclusion criteria), applicability of RCT conclusion to rest of the population should be done with caution and less reliable. External validity is improved by changing inclusion and exclusion criteria, while internal validity can be boosted by controlling more variables (reducing confounding), randomization, blinding, improving measurement technique, and by adding control/placebo group. 7
Size of Target Population/Is Sample Size Adequate?
Another important step is to choose adequate sample size that gives relevant clinical difference that is statistically significant. Sample size estimation should be done prior to trial only and should not be deviate while study is ongoing to prevent statistical error. Study size is affected by multiple factors such as acceptable level of significance (alpha error), power of study, expected effect size, event rate in population (prevalence rate), alternative hypothesis, and standard deviation in population. There are formulas to calculate sample size, but it is more important for us to understand the relationship of each factor with sample size. 8
For phenomenon or association where effect size is large, even small size sample will solve the purpose. In traditional concept, we learnt that large sample size is good but that it is not true all the time, as even clinically nonsignificant difference will be highlighted when large sample size is analyzed. For certain disease where prevalence rate is low (rare events), it is not possible to do RCTs (where observational study solve the purpose).
Tool used for sample size estimation is “Power of the study”. Power of study represents how much study population required to avoid type II error for that study. Power of study depends on variable factors such as precision and variance of measurements within any sample, effect size, type I error acceptance level, and type of statistical test we are performing. 9 Sample size also depends on expected attrition rate/dropout rate/losses to follow-up and funding capacity of trial.
Right Study Design
Experimental design considers better over observational design, as they have better grip on variables, and cause–effect hypothesis can be established. Experimental study design is again divided into preexperimental, quasi-experimental, and true experimental. Quasi-experimental and true experimental design is differentiated by absence and presence of randomization of groups. Randomized control trial is true experimental design, and it delivers higher quality of evidence over other designs as having remarkably high internal validity and presence of randomization. But RCT has its own limitations such as complex study design, costly by nature, ethical issue with limitations (as intervention/medicine use), time consuming, and difficult to apply on rare disease or conditions.
Strengthen Study Design/Are Measures Taken to Reduce Bias (Selection or Confouding Bias)?
Interventional studies/RCTs are designed to observe the efficacy and safety of new treatment for clinical condition, it is particularly important that outcome does not happen by chance. To reduce confounding factors and bias, variety of strategies such as selection of control, randomization, blinding, and allocation concealment are helpful. Control arm is used for comparison to derive the more reliable effect of intervention. Control are of four types: (1) Historical, (2) Placebo, (3) Active control (where standard treatment used), and (4) Dose–response control (where control have different dose/gradient of intervention compared to interventional arm). Randomization helps to reduce the selection bias and confounding bias. Randomization can be done by computer-generated or random number table from textbook. Randomization techniques are of different types such as simple, block randomization, stratified, and cluster randomization. Reliability of sample randomization gets compromised if used for small sample. Block randomization is better method when there is large sample size, and follow-up period is lengthy. It is also important that block size should not be disclosed to the investigator, and if possible, block size should vary with time and randomly distributed to avoid predictability. Stratified randomization is used when there are specific variables having known influence on outcome. In cluster randomization, rather than individual a group of people are randomized. Blinding is a method to reduce observation bias. Study can be open labeled/unblinded or blinded. Blinding has different types such as participant blinding, observer/investigator blinding, and data analyst blinding. Allocation concealment secures randomization and thus reduces selection bias. The difference between allocation concealment and blinding is that allocation concealment is used while recruitment and blinding after recruitment. 10
Right Data/Is Appropriate Tool/Method Used to Analyze Data?
“ We must be careful not to confuse data with the abstractions we use to analyze them .” [William James]
Study methodology should include mentioning type of research, collect and analyze data, tool/method used, and rational of using those tools. After collecting data, the next step is to decide which statistical test should be used. Choosing the right test depends on few parameters: (1) purpose/objectives of study question (whether it is to compare data or establish any correlation between them); (2) how many samples are there (one, two or multiple); (3) type of data (categorical and numerical), (4) type and number of variables? (univariate, bivariate, or multivariate); and (5) Relationship between groups (paired/dependent vs unpaired/independent). Based on these differences, possible combinations arise. Table shows different combination and methodological tests used to analyze data ( Table 2 ).
Factors/questions helps to select statistical tool to analyze data 11 , 12
In RCTs, many times we have seen subgroup analysis or post hoc analysis; for a reader, it is very important to understand limitation of those analyzes. Subgroup analyzes are usually considered as a secondary objective, but in era of personalized medicine and targeted therapies, it is well recognized that the treatment effect of a new drug/intervention might not be same among study population. Subgroup analyzes are therefore important to interpret the results of clinical trials. 13 Subgroup analyzes is helpful when (1) to evaluate safety profile in particular subgroup, (2) to access consistency of effect on different subgroup, and (3) to detect effect in subgroup in otherwise nonsignificant trial. 14 Subgroup analysis is much criticized by 2 ways: (1) Chances of high false-positive findings as multiple testing and (2) chances of false-negative when inadequate power (because of small sample size). It is exceedingly difficult to come to conclusion based on subgroup analysis and practice it. Still there are few scenarios where clinicians consider validity of subgroup analysis when prior probability of subgroup effect is more (at least more than 20% and preferably >50%), small number of subgroups (≤2) are tested, subgroup has same baseline characteristic, and when hypothesis testing of subgroup decided prior only. To reduce false-positive rate in subgroup findings, the clinician can take help of Bayes approach. 15 Post hoc analyzes, type of subgroup analysis defined by, ‘The act of examining data for findings or responses that were not specified a priori but analyzed after the study has been completed’. If possible, prespecified subgroup analyzes should be done compared to post hoc analyzes, as they are more credible. 13
Right Interpretation (Giving Meaning to Data)
“ Everything we hear is an opinion, not a fact. Everything we see is a perspective, not the truth .” [Marcus Aurelius]
Is this RCT Result Difference by Chance/Statistically Significant?
Is p-value significant? Purpose of data collection and analysis is to show whether there is difference between two groups or not. Now this difference can be due to chance or true difference. To rule out difference by chance, many tools are used in statistics: p -value is one of them. p -value is a widely used yet highly misunderstood and misinterpreted index. In Fisher's system, the p -value was used as a rough numerical guide for the strength of evidence against the null hypothesis and value of which was arbitrary selected to 0.05. In simplified way, p -value <0.05 suggests that one should repeat the experiment and word significance is merely indicating “worthy of attention”. So once p -value becomes significant, one should do more and more vigorous study rather than end of story. 16
Misperception about p-value: Most common misperception about p -value are: (1) Large p -value means no difference and (2) smaller p -value is always more significant?
(a)“Absence of evidence is not the evidence of absence.” If the p -value is above the prespecified threshold alpha error (mostly 0.05), we normally conclude that the H 0 is not rejected. But it does not mean that the H 0 is true. The better interpretation is that there is insufficient evidence to reject the H 0 . Similarly, the “not H 0 ” could mean there is something wrong with the H 0 and not necessarily that H a is right. 17 (b) p -value is affected by factors like (i) effect size (appropriate index for measuring the effect and size of effect), (ii) size of sample (larger the sample size likely a difference to be detected), and (iii) distribution of data (bigger the standard deviation, lower the p -value). 18 It is very important to understand that smaller p -values do not always mean significant findings, as larger sample size and smaller effect size can give smaller p -value.
Is multiple testing done? Another problem with p -value is multiple testing, and few of them/last testing shows p -value of <0.05.
“If you torture the data enough, nature will always confess.” [Ronald Coase] One success out of one attempt and one success out of multiple attempts have different meanings in terms of statistics and probability. The underlying mechanism of multiple described is as “File drawer problem”. Multiple testing is more about “intention” and the future likelihood of replicability of the observed finding rather than truth. 17
Is false discovery rate ruled out?/solution of multiple testing p-value: Tools used to weed out such bad data that seems good: A simple not perfect solution of multiple testing p -value is the Bonferroni adjustment, which is to use α = 0.05/5 = 0.01 for 5 (independent) tests as a new threshold and adjust the observed p -values by multiplying by 5. Problem with this adjustment is that it not only lowers the chance of detecting false-positive but also reduces true discoveries. False discovery rate (FDR) is another method that controls the number of false discoveries only in those tests having significant result. Adjusted p -values using an optimized FDR approach is known as q -value. There are other methods to overcome this phenomenon like O'Brien-Fleming for interim analyzes and empirical Bayes methods. 17 , 18
Is alternative approach to p-value used?/Bayes method: Limitation of p -value is that it does not consider prior probability and alternative hypothesis. The evidence from a given study needs to be combined with that from prior work to generate a conclusion. This purpose is solved by Bayes’ theorem/method. Bayes’ factor is the likelihood ratio of null hypothesis to alternate hypothesis. In simple terms, p -value should be compared to strongest Bayes’ factor to see the true evidence against null hypothesis 16 ( Table 3 ).
Properties and differences between Bayes’ factor and p -value 16 , 19
Is p-value backed up with confidence interval? Confidence interval (CI) describes the range of values calculated from sample observation that likely contains true population value with some degree of uncertainty. CI will help to overcome lacunae of p -value by giving more information about significance. It gives idea about size of effect rather than hypothesis testing. Width of CI will give idea about precision/reliability of estimate. CI gives insight about direction and strength of effect and thus clinical relevance rather than just statistical one. p -value is affected by type I error while CI is not. 20 , 21 Size of CI depends on sample size and standard deviation of study group. If sample size is large (leads to more confidence), it will give narrow CI. If the dispersion is wide, then certainty of conclusion is less and wider CI. Confidence interval is also affected by level of confidence that is selected by user and does not depend on sample characteristics. Most selected level is 95%, but different levels like 90% or 99% can be considered. 20 , 21 Another usefulness of CI is in equivalence/superior/non-inferior type of studies, where CI is used as intergroup comparison tool and not the p -value. 21
Is data robust? The Fragility index (FI) measures the robustness of the results of a clinical trial. In simple words, if the FI is high, statistical reproducibility of the study is high. The FI is the minimum number of patients whose status would have to change from a non-event (not experiencing the primary end point) to an event (experiencing the primary end point) to make the study lose statistical significance. For instance, an FI score of 1 means that only one patient would have to not experience the primary end point to make the trial result nonsignificant. In other words, it is a measure of how many events the statistical significance of a clinical trial result depends on. A smaller FI score indicates a more fragile, less statistically robust clinical trial result. Like other statistical tools, FI is also not free from limitations: (1) Only appropriate for RCTs; (2) Appropriate for dichotomous outcome; (3) Not appropriate for time-to event binary outcomes; (4) No specific FI value that defines an RCT outcome as robust and no FI score cut off value considered acceptable; (5) Use of FI scores to assess secondary outcome measures in studies may be limited; (6) Not reliable/difficult to interpret when number of subjects who drop out for unknown reasons is large; and (7) FI strongly related to p -value. In view of above-mentioned flaws, FI should not be used as isolated tool to measure strength of effect. Trials with lower scores are more fragile (which is usually in association with the smaller number of events, smaller sample size, and resulting lower study power), and trials with a higher FI score are less fragile (which is usually associated with larger number of events, larger sample size, and resulting higher study power). 22 – 24
Is this Statistically Significant Difference/Clinically Significant?
Another more common misinterpretation is ‘statistically significant is equivalent to clinical significant’. Statistically significant means there is true difference in the data but whether that difference is clinically significant or not depends on many factors such as size of effect (minimum important difference), any harms (risk-benefit), cost-effectiveness/feasibility, and conflict of interest/funding. 25
“ The primary product of a research inquiry is one or more measures of effect size, not p-values .” [Jacob Cohen]
p -value gives idea about whether effect exists or not but does not give idea about size of effect. It is particularly important to mention both effect size and p -value in the study. Both parameters are not alternative to each other but rather they are complementary. Unlike significance tests, effect size is independent of sample size. 26 Effect size indices can be calculated depending on the type of comparison under study ( Table 4 ).
Common effect size indices 26 – 28
Interpretation of effect size depends on the assumptions that both group (“control” and “experimental”) values are normally distributed and have same standard deviations. Relative risk and odds ratio should be interpreted in the context of absolute risk and confidence interval. Use of an effect size with a confidence interval will deliver the same information as a test of statistical significance, but it gives weightage on the significance of the effect rather than the sample size.
Minimum important difference: Most important and difficult point in clinical significance is to decide what difference is clinically important. There are 3 ways to decide MID: Anchor-based, distribution-based, and expert panel approach. 25
Is Randomized Control Trial Result Applicable/Practice Changing?
When any of new intervention or therapy launched, its acceptance and success not only depend on clinical efficacy but also on the costs associated with it. Randomized trials focus on clinical end points such as organ failure, respiratory or renal support, mortality, and morbidity, while contemporary clinical trials include economic outcomes. Therapy with good clinical outcome and low cost is considered as dominant strategy, and in such cases, there is no need of any deep analysis. But problem arises when there is one novel therapy showing some better clinical outcome but having higher cost. In such cases, the most important thing is whether improvement in outcome is worth the higher cost. So, cost-effectiveness helps in balancing cost with efficacy/outcome and comparing available alternative therapies. 29
Is any conflict of interest financial/non-financial? A conflict of interest (COI) happens when contradictory interest emerges out to on a topic/activity by an individual/institution. When conflict of interest exists, validity of RCT should be in question, independent of the behavior of the investigator. Conflict of interest can happen at different level/tier like at the level of investigator, ethics committee (EC), or at regulator level. Conflict of interest can happen with sponsors like pharmaceutical companies, contract research organization, or at multiple levels. Nowadays, most of the trials are blinded, so, it is exceedingly difficult for investigator to manipulate the data and thus the result. But it is possible to alter data unintentionally or knowingly at the level of data analysis by data management team. It is important to check at this level, as most investigators would not even know if results were altered by data analyst. In simple way, conflict of interest can be divided into non-financial type and financial type. Other classifications are negative conflict of interest and positive conflict of interest. More common is that we concern about positive conflict of interest, but negative conflict of interest is also worth observing. Negative COI happens when any investigator/sponsor willfully rejects/gives injustice to potential useful therapy or intervention, just for his own rivalry or benefit. 30
It is also very important to know that conflict of interest is not always bad thing, and sometimes it just happens because of nature of question/core problem not because of individual or sponsor. 30 , 31 Most common and best approach to handle conflict of interest is by public reporting of relevant conflicts.
Is bias present in randomized control trial? Bias is defined as systematic error in the results of individual studies or their synthesis. Cochrane Risk of Bias Tool for randomized trials mentioned that bias can happen at 6 different levels/domains: generation of allocation sequence, concealment of allocation sequence, blinding of participants(single blinding) and doctors(double blinding), blinding of data analyst (triple blinding), attrition bias, and publication bias. It is worth noticing that financial conflict of interest is not part of this but, it can be motive behind it. 31
Is randomized control trial peer reviewed or not? Another important thing about article publication and reliability is whether peer review done or not. Peer-review is the assessment of article by qualified people before publication. Peer-review helps to improve the quality of article by adding suggestion, and second it rejects the unacceptable poor-quality articles. Most of the reputed journals made their own policy about peer-review. Peer-review is not free of bias. Sometimes, quality of this process depends on selected qualified faculty and their preference on article. Like peer-review, post publication review is also especially important and should not be ignored, as it is criticized/analyzed by hundreds of experts. 32 , 33
C onclusion
In nutshell, critical analysis of RCT is all about balancing the strong and weak points of trial based on analyzing main domains such as right question, right population, right study design, right data, and right interpretation. It is also important to note that these demarcations are immensely simplified, and they are interconnected by many paths.
Source of support: Nil
Conflict of interest: None
R eferences
- - Google Chrome
Intended for healthcare professionals
- Access provided by Google Indexer
- My email alerts
- BMA member login
- Username * Password * Forgot your log in details? Need to activate BMA Member Log In Log in via OpenAthens Log in via your institution

Search form
- Advanced search
- Search responses
- Search blogs
- Quantifying possible...
Quantifying possible bias in clinical and epidemiological studies with quantitative bias analysis: common approaches and limitations
- Related content
- Peer review
- Jeremy P Brown , doctoral researcher 1 ,
- Jacob N Hunnicutt , director 2 ,
- M Sanni Ali , assistant professor 1 ,
- Krishnan Bhaskaran , professor 1 ,
- Ashley Cole , director 3 ,
- Sinead M Langan , professor 1 ,
- Dorothea Nitsch , professor 1 ,
- Christopher T Rentsch , associate professor 1 ,
- Nicholas W Galwey , statistics leader 4 ,
- Kevin Wing , assistant professor 1 ,
- Ian J Douglas , professor 1
- 1 Department of Non-Communicable Disease Epidemiology, London School of Hygiene and Tropical Medicine, London, UK
- 2 Epidemiology, Value Evidence and Outcomes, R&D Global Medical, GSK, Collegeville, PA, USA
- 3 Real World Analytics, Value Evidence and Outcomes, R&D Global Medical, GSK, Collegeville, PA, USA
- 4 R&D, GSK Medicines Research Centre, GSK, Stevenage, UK
- Correspondence to: J P Brown jeremy.brown{at}lshtm.ac.uk (or @jeremy_pbrown on X)
- Accepted 12 February 2024
Bias in epidemiological studies can adversely affect the validity of study findings. Sensitivity analyses, known as quantitative bias analyses, are available to quantify potential residual bias arising from measurement error, confounding, and selection into the study. Effective application of these methods benefits from the input of multiple parties including clinicians, epidemiologists, and statisticians. This article provides an overview of a few common methods to facilitate both the use of these methods and critical interpretation of applications in the published literature. Examples are given to describe and illustrate methods of quantitative bias analysis. This article also outlines considerations to be made when choosing between methods and discusses the limitations of quantitative bias analysis.
Bias in epidemiological studies is a major concern. Biased studies have the potential to mislead, and as a result to negatively affect clinical practice and public health. The potential for residual systematic error due to measurement bias, confounding, or selection bias is often acknowledged in publications but is seldom quantified. 1 Therefore, for many studies it is difficult to judge the extent to which residual bias could affect study findings, and how confident we should be about their conclusions. Increasingly large datasets with millions of patients are available for research, such as insurance claims data and electronic health records. With increasing dataset size, random error decreases but bias remains, potentially leading to incorrect conclusions.
Sensitivity analyses to quantify potential residual bias are available. 2 3 4 5 6 7 However, use of these methods is limited. Effective use typically requires input from multiple parties (including clinicians, epidemiologists, and statisticians) to bring together clinical and domain area knowledge, epidemiological expertise, and a statistical understanding of the methods. Improved awareness of these methods and their pitfalls will enable more frequent and effective implementation, as well as critical interpretation of their application in the medical literature.
In this article, we aim to provide an accessible introduction, description, and demonstration of three common approaches of quantitative bias analysis, and to describe their potential limitations. We briefly review bias in epidemiological studies due to measurement error, confounding, and selection. We then introduce quantitative bias analyses, methods to quantify the potential impact of residual bias (ie, bias that has not been accounted for through study design or statistical analysis). Finally, we discuss limitations and pitfalls in the application and interpretation of these methods.
Summary points
Quantitative bias analysis methods allow investigators to quantify potential residual bias and to objectively assess the sensitivity of study findings to this potential bias
Bias formulas, bounding methods, and probabilistic bias analysis can be used to assess sensitivity of results to potential residual bias; each of these approaches has strengths and limitations
Quantitative bias analysis relies on assumptions about bias parameters (eg, the strength of association between unmeasured confounder and outcome), which can be informed by substudies, secondary studies, the literature, or expert opinion
When applying, interpreting, and reporting quantitative bias analysis, it is important to transparently report assumptions, to consider multiple biases if relevant, and to account for random error
Types of bias
All clinical studies, both interventional and non-interventional, are potentially vulnerable to bias. Bias is ideally prevented or minimised through careful study design and the choice of appropriate statistical methods. In non-interventional studies, three major biases that can affect findings are measurement bias (also known as information bias) due to measurement error (referred to as misclassification for categorical variables), confounding, and selection bias.
Misclassification occurs when one or more categorical variables (such as the exposure, outcome, or covariates) are mismeasured or misreported. 8 Continuous variables might also be mismeasured leading to measurement error. As one example, misclassification occurs in some studies of alcohol consumption owing to misreporting by study participants of their alcohol intake. 9 10 As another example, studies using electronic health records or insurance claims data could have outcome misclassification if the outcome is not always reported to, or recorded by, the individual’s healthcare professional. 11 Measurement error is said to be differential when the probability of error depends on another variable (eg, differential participant recall of exposure status depending on the outcome). Errors in measurement of multiple variables could be dependent (ie, associated with each other), particularly when data are collected from one source (eg, electronic health records). Measurement error can lead to biased study findings in both descriptive and aetiological (ie, cause-effect) non-interventional studies. 12
Confounding arises in aetiological studies when the association between exposure and outcome is not solely due to the causal effect of the exposure, but rather is partly or wholly due to one or more other causes of the outcome associated with the exposure. For example, researchers have found that greater adherence to statins is associated with a reduction in motor vehicle accidents and an increase in the use of screening services. 13 However, this association is almost certainly not due to a causal effect of statins on these outcomes, but more probably because attitudes to precaution and risk that are associated with these outcomes are also associated with adherence to statins.
Selection bias occurs when non-random selection of people or person time into the study results in systematic differences between results obtained in the study population and results that would have been obtained in the population of interest. 14 15 This bias can be due to selection at study entry or due to differential loss to follow-up. For example, in a cohort study where the patients selected are those admitted to hospital in respiratory distress, covid-19 and chronic obstructive pulmonary disease might be negatively associated, even if there was no association in the overall population, because if you do not have one condition it is more likely you have the other condition in order to be admitted. 16 Selection bias can affect both descriptive and aetiological non-interventional studies.
Handling bias in practice
All three biases should ideally be minimised through study design and analysis. For example, misclassification can be reduced by the use of a more accurate measure, confounding through measurement of all relevant potential confounders and their subsequent adjustment, and selection bias through appropriate sampling from the population of interest and accounting for loss to follow-up. Other biases should also be considered, for example, immortal time bias through the appropriate choice of time zero, and sparse data bias through collection of a sample of sufficient size or by the use of penalised estimation. 17 18
Even with the best available study design and most appropriate statistical analysis, we typically cannot guarantee that residual bias will be absent. For instance, it is often not possible to perfectly measure all required variables, or it might be either impossible or impractical to collect or obtain data on every possible potential confounder. For instance, studies conducted using data collected for non-research purposes, such as insurance claims and electronic health records, are often limited to the variables previously recorded. Randomly sampling from the population of interest might also not be practically feasible, especially if individuals are not willing to participate.
To ignore potential residual biases can lead to misleading results and erroneous conclusions. Often the potential for residual bias is acknowledged qualitatively in the discussion, but these qualitative arguments are typically subjective and often downplay the impact of any bias. Heuristics are frequently relied on, but these can lead to an misestimation of the potential for residual bias. 19 Quantitative bias analysis allows both authors and readers to assess robustness of study findings to potential residual bias rigorously and quantitatively.
Quantitative bias analysis
When designing or appraising a study, several key questions related to bias should be considered ( box 1 ). 20 If, on the basis of the answers to these questions, there is potential for residual bias(es), then quantitative bias analysis methods can be considered to estimate the robustness of findings.
Key questions related to bias when designing and appraising non-interventional studies
Misclassification and measurement error: Are the exposure, outcome, and covariates likely to be measured and recorded accurately?
Confounding: Are there potential causes of the outcome, or proxies for these causes, which might differ in prevalence between exposure groups? Are these potential confounders measured and controlled through study design or analysis?
Selection bias: What is the target population? Are individuals in the study representative of this target population?
Many methods for quantitative bias analysis exist, although only a few of these are regularly applied in practice. In this article, we will introduce three straightforward, commonly applied, and general approaches 1 : bias formulas, bounding methods, and probabilistic bias analysis. Alternative methods are also available, including methods for bias adjustment of linear regression with a continuous outcome. 7 21 22 Methods for dealing with misclassification of categorical variables are outlined in this article. Corresponding methods for sensitivity analysis to deal with mismeasurement of continuous variables are available and are described in depth in the literature. 23 24
Bias formulas
We can use simple mathematical formulas to estimate the bias in a study and to estimate what the results would be in the absence of that bias. 4 25 26 27 28 Commonly applied formulas, along with details of available software to implement methods listed, are provided in the appendices. Some of these methods can be applied to the summary results (eg, risk ratio), whereas other methods require access to 2×2 tables or participant level data.
These formulas require us to specify additional information, typically not obtainable from the study data itself, in the form of bias parameters. Values for these parameters quantify the extent of bias present due to confounding, misclassification, or selection.
Bias formulas for unmeasured confounding generally require us to specify the following bias parameters: prevalence of the unmeasured confounder in the unexposed individuals, prevalence of the unmeasured confounder in the exposed individuals (or alternatively the association between exposure and unmeasured confounder), and the association between unmeasured confounder and outcome. 4 28 29
These bias formulas can be applied to the summary results (eg, risk ratios, odds ratios, risk differences, hazard ratios) and to 2×2 tables, and they produce corrected results assuming the specified bias parameters are correct. Generally, the exact bias parameters are unknown so a range of parameters can be entered into the formula, producing a range of possible bias adjusted results under more or less extreme confounding scenarios.
Bias formulas for misclassification work in a similar way, but typically require us to specify positive predictive value and negative predictive value (or sensitivity and specificity) of classification, stratified by exposure or outcome. These formulas typically require study data in the form of 2×2 tables. 7 30
Bias formulas for selection bias are applicable to the summary results (eg, risk ratios, odds ratios) or to 2×2 tables, and normally require us to specify probabilities of selection into the study for different levels of exposure and outcome. 25 When participant level data are available, a general method of bias analysis is to weight each individual by the inverse of their probability of selection. 31 Box 2 describes an example of the application of bias formulas for selection bias.
Application of bias formulas for selection bias
In a cohort study of pregnant women investigating the association between lithium use (relative to non-use) and cardiac malformations in liveborn infants, the observed covariate adjusted risk ratio was 1.65 (95% confidence interval 1.02 to 2.68). 32 Only liveborn infants were selected into the study; therefore, there was potential for selection bias if differences in the termination probabilities of fetuses with cardiac malformations existed between exposure groups.
Because the outcome is rare, the odds ratio approximates the risk ratio, and we can apply a bias formula for the odds ratio to the risk ratio. The bias parameters are selection probabilities for the unexposed group with outcome S 01 , exposed group with outcome S 11 , unexposed group without outcome S 00 , and exposed group without outcome S 10 :
OR BiasAdj = OR Obs × ((S 01 ×S 10 ) ÷ (S 00 ×S 11 ))
(Where OR BiasAdj is the bias adjusted odds ratio and OR Obs is the observed odds ratio.)
For example, if we assume that the probability of terminations is 30% among the unexposed group (ie, pregnancies with no lithium dispensation in first trimester or three months earlier) with malformations, 35% among the exposed group (ie, pregnancies with lithium dispensation in first trimester) with malformations, 20% among the unexposed group without malformations, and 25% among the exposed group without malformations, then the bias adjusted odds ratio is 1.67.
OR BiasAdj = 1.65 × ((0.7×0.75) ÷ (0.65×0.8)) = 1.67
In the study, a range of selection probabilities (stratified by exposure and outcome status) were specified, informed by the literature. Depending on assumed selection probabilities, the bias adjusted estimates of the risk ratio ranged from 1.65 to 1.80 ( fig 1 ), indicating that the estimate was robust to this selection bias under given assumptions.

Bias adjusted risk ratio for different assumed selection probabilities in cohort study investigating association between lithium use (relative to non-use) and cardiac malformations in liveborn infants. Redrawn and adapted from reference 32 with permission from Massachusetts Medical Society. Selection probability of the unexposed group without cardiac malformations was assumed to be 0.8 (ie, 20% probability of termination). Selection probabilities in the exposed group were defined relative to the unexposed group by outcome status (ie, −0%, −5%, and −10%)
- Download figure
- Open in new tab
- Download powerpoint
It is possible to incorporate measured covariates in these formulas, but specification then generally becomes more difficult because we typically have to specify bias parameters (such as the prevalence of the unmeasured confounder) within stratums of measured covariates.
Although we might not be able to estimate these unknowns from the main study itself, we can specify plausible ranges based on the published literature, clinical knowledge, or a secondary study or substudy. Secondary studies or substudies, in which additional information from a subset of study participants or from a representative external group are collected, are particularly valuable because they are more likely to accurately capture unknown values. 33 However, depending on the particular situation, they could be infeasible for a given study owing to data access limitations and resource constraints.
The published literature can be informative if there are relevant published studies and the study populations in the published studies are sufficiently similar to the population under investigation. Subjective judgments of plausible values for unknowns are vulnerable to the viewpoint of the investigator, and as a result might not accurately reflect the true unknown values. The validity of quantitative bias analysis depends critically on the validity of the assumed values. When implementing quantitative bias analysis, or appraising quantitative bias analysis in a published study, study investigators should question the choices made for these unknowns, and report these choices with transparency.
Bounding methods
Bounding methods are mathematical formulas, similar to bias formulas, that we can apply to study results to quantify sensitivity to bias due to confounding, selection, and misclassification. 5 34 35 36 However, unlike bias formulas, they require only a subset of the unknown values to be specified. While this requirement seems advantageous, one important disadvantage is that bounding methods generate a bound on the maximum possible bias, rather than an estimate of the association adjusted for bias. When values for all unknown parameters (eg, prevalence of an unmeasured confounder) can be specified and there is reasonable confidence in their validity, bias formulas or probabilistic bias analysis can generally be applied and can provide more information than bounding methods. 37
One commonly used bounding method for unmeasured confounding is the E-value. 5 35 By using E-value formulas, study investigators can calculate a bound on the bias adjusted estimate by specifying the association (eg, risk ratio) between exposure and unmeasured confounder and between unmeasured confounder and outcome, while leaving the prevalence of the unmeasured confounder unspecified. The E-value itself is the minimum value on the risk ratio scale that the association between exposure and unmeasured confounder or the association between unmeasured confounder and outcome must exceed to potentially reduce the bias adjusted findings to the null (or alternatively to some specified value, such as a protective risk ratio of 0.8). If the plausible strength of association between the unmeasured confounder and both exposure and outcome is smaller than the E-value, then that one confounder could not fully explain the observed association, providing support to the study findings. If the strength of association between the unmeasured confounder and either exposure or outcome is plausibly larger than the E-value, then we can only conclude that residual confounding might explain the observed association, but it is not possible to say whether such confounding is in truth sufficient, because we have not specified the prevalence of the unmeasured confounder. Box 3 illustrates the use of bounding methods for unmeasured confounding. Although popular, the application of E-values has been criticised, because these values have been commonly misinterpreted and have been used frequently without careful consideration of a specific unmeasured confounder or the possibility of multiple unmeasured confounders or other biases. 38
Application of bounding methods
In a cohort study investigating the association between use of proton pump inhibitors (relative to H2 receptor antagonists) and all cause mortality, investigators found evidence that individuals prescribed proton pump inhibitors were at higher risk of death after adjusting for several measured covariates including age, sex, and comorbidities (covariate adjusted hazard ratio 1.38, 95% confidence interval (CI) 1.33 to 1.44). 39 However, unmeasured differences in frailty between users of H2 receptor antagonists and users of proton pump inhibitors could bias findings. Because the prevalence of the unmeasured confounder in the different exposure groups was unclear, the E-value was calculated. Because the outcome was rare at the end of follow-up, and therefore the risk ratio approximates the hazard ratio given proportional hazards, 40 the E-value formula, which applies to the risk ratio, was applied to the hazard ratio.
E-value = RR Obs + √(RR Obs ×(RR Obs −1))
= 1.38 + √(1.38×(1.38−1))
(Where RR Obs is the observed risk ratio.)
The E-value for the point estimate of the adjusted hazard (1.38) was 2.10. Hence either the adjusted risk ratio between exposure and unmeasured confounder, or the adjusted risk ratio between unmeasured confounder and outcome, must be greater than 2.10 to potentially explain the observed association of 1.38. The E-value can be applied to the bounds of the CI to account for random error. The calculated E-value for the lower bound of the 95% CI (ie, covariate adjusted hazard ratio=1.33) was 1.99. We can plot a curve to show the values of risk ratios necessary to potentially reduce the observed association, as estimated by the point estimate and the lower bound of the CI, to the null ( fig 2 ). An unmeasured confounder with strengths of associations below the blue line could not fully explain the point estimate, and below the yellow line could not fully explain the lower bound of the confidence interval.

E-value plot for unmeasured confounding of association between use of proton pump inhibitors and all cause mortality. Curves show the values of risk ratios necessary to potentially reduce the observed association, as estimated by the point estimate and the lower bound of the confidence interval, to the null
Given risk ratios of >2 observed in the literature between frailty and mortality, unmeasured confounding could not be ruled out as a possible explanation for observed findings. However, given that we used a bounding method, and did not specify unmeasured confounder prevalence, we could not say with certainty whether such confounding was likely to explain the observed result. Additional unmeasured or partially measured confounders might have also contributed to the observed association.
Probabilistic bias analysis
Probabilistic bias analysis takes a different approach to handling uncertainty around the unknown values. Rather than specifying one value or a range of values for an unknown, a probability distribution (eg, a normal distribution) is specified for each of the unknown quantities. This distribution represents the uncertainty about the unknown values, and values are sampled repeatedly from this distribution before applying bias formulas using the sampled values. This approach can be applied to either summary or participant level data. The result is a distribution of bias adjusted estimates. Resampling should be performed a sufficient number of times (eg, 10 000 times), although this requirement can become computationally burdensome when performing corrections at the patient record level. 41
Probabilistic bias analysis can readily handle many unknowns, which makes it particularly useful for handling multiple biases simultaneously. 42 However, it can be difficult to specify a realistic distribution if little information on the unknowns is available from published studies or from additional data collection. Commonly chosen distributions include uniform, trapezoidal, triangular, beta, normal, and log-normal distributions. 7 Sensitivity analyses can be conducted by varying the distribution and assessing the sensitivity of findings to distribution chosen. When performing corrections at the patient record level, analytical methods such as regression can be applied after correction to adjust associations for measured covariates. 43 Box 4 gives an example of probabilistic bias analysis for misclassification.
Application of probabilistic bias analysis
In a cohort study of pregnant women conducted in insurance claims data, the observed covariate adjusted risk ratio for the association between antidepressant use and congenital cardiac defects among women with depression was 1.02 (95% confidence interval 0.90 to 1.15). 44
Some misclassification of the outcome, congenital cardiac defects, was expected, and therefore probabilistic bias analysis was conducted. A validation study was conducted to assess the accuracy of classification. In this validation study, full medical records were obtained and used to verify diagnoses for a subset of pregnancies with congenital cardiac defects recorded in the insurance claims data. Based on positive predictive values estimated in this validation study, triangular distributions of plausible values for sensitivity ( fig 3 ) and of specificity of outcome classification were specified and were used for probabilistic bias analysis.

Specified distribution of values for sensitivity of outcome ascertainment
Values were sampled at random 1000 times from these distributions and were used to calculate a distribution of bias adjusted estimates incorporating random error. The median bias adjusted estimate was 1.06, and the 95% simulation interval was 0.92 to 1.22. 44 This finding indicates that under the given assumptions, the results were robust to outcome misclassification, because the bias adjusted results were similar to the initial estimates. Both sets of estimates suggested no evidence of association between antidepressant use and congenital cardiac defects.
Pitfalls of methods
Incorrect assumptions.
Study investigators and readers of published research should be aware that the outputs of quantitative bias analyses are only as good as the assumptions made. These assumptions include both assumptions about the values chosen for the bias parameters ( table 1 ), and assumptions inherent to the methods. For example, applying the E-value formula directly to a hazard ratio rather than a risk ratio is an approximation, and only a good approximation when the outcome is rare. 45
Common bias parameters for bias formulas and probabilistic bias analysis
- View inline
Simplifying assumptions are required by many methods of quantitative bias analysis. For example, it is often assumed that the exposure does not modify the unmeasured confounder-outcome association. 4 If these assumptions are not met then the findings of quantitative bias analysis might be inaccurate.
Ideally, assumptions would be based on supplemental data collected in a subset of the study population (eg, internal validation studies to estimate predictive values of misclassification) or, in the case of selection bias, in the source population from which the sample was selected, but additional data collection is not always feasible. 7 Validation studies can be an important source of evidence on misclassification, although proper design is important to obtain valid estimates. 33
Multiple biases
If the results are robust to one source of bias, it is a mistake to assume that they must necessarily reflect the causal effect. Depending on the particular study, multiple residual biases could exist, and jointly quantifying the impact of all of these biases is necessary to properly assess robustness of results. 34 Bias formulas and probabilistic bias analyses can be applied for multiple biases, but specification is more complicated, and the biases should typically be accounted for in the reverse order from which they arise (appendices 2 and 3 show an applied example). 7 46 47 Bounding methods are available for multiple biases. 34
Prespecification
Prespecification of quantitative bias analysis in the study protocol is valuable so that choice of unknown values and choice to report bias analysis is not influenced by whether the results of bias analysis are in line with the investigators expectations. Clearly a large range of analyses is possible, although we would encourage judicious application of these methods to deal with biases judged to be of specific importance given the limitations of the specific study being conducted.
Accounting for random and systematic error
Both systematic errors, such as bias due to misclassification and random error due to sampling, affect study results. To accurately reflect this issue, quantitative bias analysis should jointly account for random error as well as systematic bias. 48 Bias formulas, bounding methods, and probabilistic bias analysis approaches can be adapted to account for random error (appendix 1).
Deficiencies in the reporting of quantitative bias analysis have been previously noted. 1 48 49 50 When reporting quantitative bias analysis, study investigators should state:
The method used and how it has been implemented
Details of the residual bias anticipated (eg, which specific potential confounder was unmeasured)
Any estimates for unknown values that have been used, with justification for the chosen values or distribution for these unknowns
Which simplifying assumptions (if any) have been made
Quantitative bias analysis is a valuable addition to a study, but as with any aspect of a study, should be interpreted critically and reported in sufficient detail to allow for critical interpretation.
Alternative methods
Commonly applied and broadly applicable methods have been described in this article. Other methods are available and include modified likelihood and predictive value weighting with regression analyses, 51 52 53 propensity score calibration using validation data, 54 55 multiple imputation using validation data, 56 methods for matched studies, 3 and bayesian bias analysis if a fully bayesian approach is desired. 57 58
Conclusions
Quantitative bias methods provide a means to quantitatively and rigorously assess the potential for residual bias in non-interventional studies. Increasing the appropriate use, understanding, and reporting of these methods has the potential to improve the robustness of clinical epidemiological research and reduce the likelihood of erroneous conclusions.
Contributors: This article is the product of a working group on quantitative bias analysis between the London School of Hygiene and Tropical Medicine and GSK. An iterative process of online workshops and email correspondence was used to decide by consensus the content of the manuscript. Based on these decisions, a manuscript was drafted by JPB before further comment and reviewed by all group members. JPB and IJD are the guarantors. The corresponding author attests that all listed authors meet authorship criteria and that no others meeting the criteria have been omitted.
Funding: No specific funding was given for this work. JPB was supported by a GSK PhD studentship.
Competing interests: All authors have completed the ICMJE uniform disclosure form at https://www.icmje.org/disclosure-of-interest/ and declare: AC, NWG, and JNH were paid employees of GSK at the time of the submitted work; AC, IJD, NWG, and JNH own shares in GSK; AC is currently a paid employee of McKesson Corporation in a role unrelated to the submitted work; JNH is currently a paid employee of Boehringer Ingelheim in a role unrelated to this work; DN is UK Kidney Association Director of Informatics Research; JPB was funded by a GSK studentship received by IJD and reports unrelated consultancy work for WHO Europe and CorEvitas; SML has received unrelated grants with industry collaborators from IMI Horizon, but no direct industry funding; all authors report no other relationships or activities that could appear to have influenced the submitted work.
Provenance and peer review: Not commissioned; externally peer reviewed.
- Petersen JM ,
- Ranker LR ,
- Barnard-Mayers R ,
- MacLehose RF ,
- Rosenbaum PR ,
- Rosenbaum PR
- Vanderweele TJ ,
- VanderWeele TJ
- Greenland S
- Hernán MA ,
- Zaridze D ,
- Brennan P ,
- Boreham J ,
- Gomez-Roig MD ,
- Marchei E ,
- Herrett E ,
- Thomas SL ,
- Schoonen WM ,
- Murray EJ ,
- Sealy-Jefferson S
- Dormuth CR ,
- Patrick AR ,
- Shrank WH ,
- Westreich D
- Greenland S ,
- Mansournia MA ,
- Lévesque LE ,
- Hanley JA ,
- Sterne JA ,
- Reeves BC ,
- Cinelli C ,
- D’Agostino McGowan L
- Gustafson P ,
- Carroll RJ ,
- Marshall RJ
- Schlesselman JJ
- Schmidt M ,
- Jensen AO ,
- Engebjerg MC
- Hernández-Díaz S ,
- Patorno E ,
- Huybrechts KF ,
- Bateman BT ,
- Mathur MB ,
- VanderWeele TJ ,
- Ioannidis JPA ,
- Tazare JR ,
- Williamson E ,
- Maldonado G ,
- McCandless LC ,
- Palmsten K ,
- Brendel P ,
- Collin LJ ,
- MacLehose RF
- Ioannidis JPA
- Hunnicutt JN ,
- Ulbricht CM ,
- Chrysanthopoulou SA ,
- Superak HM ,
- Stürmer T ,
- Schneeweiss S ,
- Rothman KJ ,
- Edwards JK ,
- Troester MA ,
- Richardson DB
- Shields PG ,
- Open access
- Published: 02 January 2022
The roles, activities and impacts of middle managers who function as knowledge brokers to improve care delivery and outcomes in healthcare organizations: a critical interpretive synthesis
- Faith Boutcher 1 ,
- Whitney Berta 2 ,
- Robin Urquhart 3 &
- Anna R. Gagliardi 4
BMC Health Services Research volume 22 , Article number: 11 ( 2022 ) Cite this article
3903 Accesses
5 Citations
1 Altmetric
Metrics details
Middle Managers (MMs) are thought to play a pivotal role as knowledge brokers (KBs) in healthcare organizations. However, the role of MMs who function as KBs (MM KBs) in health care is under-studied. Research is needed that contributes to our understanding of how MMs broker knowledge in health care and what factors influence their KB efforts.
We used a critical interpretive synthesis (CIS) approach to review both qualitative and quantitative studies to develop an organizing framework of how MMs enact the KB role in health care. We used compass questions to create a search strategy and electronic searches were conducted in MEDLINE, CINAHL, Social Sciences Abstracts, ABI/INFORM, EMBASE, PubMed, PsycINFO, ERIC and the Cochrane Library. Searching, sampling, and data analysis was an iterative process, using constant comparison, to synthesize the results.
We included 41 articles (38 empirical studies and 3 conceptual papers) that met the eligibility criteria. No existing review was found on this topic. A synthesis of the studies revealed 12 MM KB roles and 63 associated activities beyond existing roles hypothesized by extant theory, and we elaborate on two MM KB roles: 1) convincing others of the need for, and benefit of an innovation or evidence-based practice; and 2) functioning as a strategic influencer. We identified organizational and individual factors that may influence the efforts of MM KBs in healthcare organizations. Additionally, we found that the MM KB role was associated with enhanced provider knowledge, and skills, as well as improved organizational outcomes.
Our findings suggest that MMs do enact KB roles in healthcare settings to implement innovations and practice change. Our organizing framework offers a novel conceptualization of MM KBs that advances understanding of the emerging KB role that MMs play in healthcare organizations. In addition to roles, this study contributes to the extant literature by revealing factors that may influence the efforts and impacts of MM KBs in healthcare organizations. Future studies are required to refine and strengthen this framework.
Trial registration
A protocol for this review was not registered.
Peer Review reports
Contributions to the literature
MMs may play an important KB role in healthcare organizations.
Additional support for the MM KB role may help enhance quality of care in healthcare settings.
An improved understanding of MM KBs will contribute to this nascent area of inquiry in health care.
Health systems are under increasing pressure to improve performance including productivity, quality of care, and efficiency in service delivery. To promote optimal performance, health systems hold healthcare organizations such as hospitals accountable for the quality of care they provide through accountability agreements tied to performance targets [ 1 , 2 ]. Despite such incentives, healthcare organizations face considerable challenges in providing high-quality care and research continues to show that the quality of hospital-based care is less than ideal [ 3 , 4 , 5 ]. Some researchers contend that this is attributed, in part, to the challenges that healthcare organizations face when integrating new knowledge into practice. Some challenges include dedicating sufficient resources to adopt or implement evidence-informed innovations that enhance service delivery and optimize patient health and outcomes [ 6 ].
Healthcare organizations use knowledge translation (KT) approaches to promote the use of evidence-based practices intended to optimize quality of care. The use of knowledge brokers (KBs) is one such approach. KBs are defined as the human component of KT who work collaboratively with stakeholders to facilitate the transfer and exchange of knowledge in diverse settings, [ 7 , 8 , 9 ]. KBs that facilitate the use of knowledge between people or groups have been referred to as opinion leaders, facilitators, champions, linking agents and change agents whose roles can be formal or informal [ 10 , 11 ]. These “influencer” roles are based on the premise that interpersonal contact improves the likelihood of behavioral change associated with use or adoption of new knowledge [ 12 ]. Research shows that KBs have had a positive effect on increasing knowledge and evidence-based practices among clinicians in hospitals, and on advocating for change on behalf of clinicians to executives [ 13 , 14 , 15 ]. However, greater insight is needed on how to equip and support KBs, so they effectively promote and enable clinicians to use evidence-based practices that improve quality of care [ 13 , 16 , 17 ].
Middle managers (MMs) play a pivotal role in facilitating high quality care and may play a brokerage role in the sharing and use of knowledge in healthcare organizations [ 18 , 19 ]. MMs are managers at the mid-level of an organization supervised by senior managers, and who, in turn, supervise frontline clinicians [ 20 ]. MMs facilitate the integration of new knowledge in healthcare organizations by helping clinicians appreciate the rationale for organizational changes and translating adoption decisions into on-the-ground implementation strategies [ 18 , 19 ]. Current research suggests that MMs may play an essential role as internal KBs because of their mid-level positions in healthcare organizations. Some researchers have called for a deeper understanding of the MM role in knowledge brokering, including how MMs enact internal KB roles [ 16 , 17 , 18 , 19 , 21 ].
To this end, further research is needed on who assumes the KB role and what they do. Prior research suggests that KBs may function across five key roles: knowledge manager, linking agent, capacity builder, facilitator, and evaluator, but it is not clear whether these roles are realized in all healthcare settings [ 7 , 21 , 22 ]. KBs are often distinguished as external or internal to the practice community that they seek to influence, and most studies have focused on external KBs with comparatively little research focused on the role of internal KBs [ 7 , 9 , 17 , 23 , 24 ]. To address this gap, we will focus on internal KBs (MMs) who hold a pivotal position because their credibility and detailed knowledge of local context allows them to overcome the barriers common to external KBs. One such barrier is resistance to advice from external sources unfamiliar with the local context [ 25 ].
With respect to what KBs do, two studies explored KB roles and activities, and generated frameworks that describe KB functions, processes, and outcomes in health care [ 7 , 22 ]. However, these frameworks are not specific to MMs and are limited in detail about KB roles and functions. This knowledge is required by healthcare organizations to develop KB capacity among MMs, who can then enhance quality of care. Therefore, the focus of this study was to synthesize published research on factors that influence the KB roles, activities, and impact of MMs in healthcare settings. In doing so, we will identify key concepts, themes, and the relationships among them to generate an organizing framework that categorizes how MMs function as KBs in health care to guide future policy, practice, and research.
We used a critical interpretive synthesis (CIS) to systematically review the complex body of literature on MM KBs. This included qualitative, quantitative, and theoretical papers. CIS offers an iterative, dynamic, recursive, and reflexive approach to qualitative synthesis. CIS was well-suited to review the MM KB literature than traditional systematic review methods because it integrates findings from diverse studies into a single, coherent framework based on new theoretical insights and interpretations [ 26 , 27 ]. A key feature that distinguishes CIS from other approaches to interpretive synthesis is the critical nature of the analysis that questions the way studies conceptualize and construct the topic under study and uses this as the basis for developing synthesizing arguments [ 26 ]. We ensured rigor by complying with the Enhancing Transparency in Reporting the Synthesis of Qualitative Research (ENTREQ) criteria (Additional file 1 ) and other criteria of trustworthiness [ 28 , 29 ]. We did not register a protocol for this review.
With a medical librarian, we developed a search strategy (Additional file 2 ) that complied with the evidence-based checklist for peer review of electronic search strategies [ 30 ]. We included Medical Subject Headings and keywords that captured the concepts of MMs (e.g., nurse administrator, manager), explicit or non-explicit KB roles (e.g., diffusion of innovation, dissemination, broker, and facilitator), evidence-based practice (e.g., knowledge, evidence) and setting (e.g., hospital, healthcare, or health care). We searched MEDLINE, CINAHL, Social Sciences Abstracts, ABI/INFORM, EMBASE, PubMed, PsycINFO, ERIC, and the Cochrane Library from January 1, 2001, to August 14, 2020. We searched from 2001 onward because the field of KT did not substantially investigate KBs until 2001 [ 7 , 21 ]. We reviewed the reference lists of eligible articles for additional relevant studies not identified by searches. As is typical of CIS, this was an iterative process allowing search terms to be expanded to optimize search results [ 26 , 31 ].
Eligibility
We generated eligibility criteria based on the PICO framework (population, intervention, comparisons, and outcomes) (Additional file 3 ). Populations refer to MMs functioning as KBs in hospitals or other healthcare settings but did not necessarily use those labels. Because the MM literature is emergent, we included settings other than hospitals (e.g., public health department, Veteran Affairs Medical Centres). We included studies involving clinical and non-clinical administrators, managers, directors, or operational leaders if those studies met all other inclusion criteria. The intervention of interest was how MM KBs operated in practice for the creation, use and sharing of knowledge, implementation of evidence-based practice(s), or innovation implementation. Study comparisons may have evaluated one or more MM KB roles, approaches and associated barriers, enablers and impacts alone or in comparison with other types of approaches for the sharing or implementation of knowledge, evidence, evidence-based practices, or innovations. Outcomes included but were not limited to MM KB effectiveness (change in knowledge, skills, policies and/or practices, care delivery, satisfaction in role), behaviors, and outcomes. Searches were limited to English language quantitative, randomized, or pragmatic controlled trials, case studies, surveys, quasi-experimental, qualitative, or mixed methods studies and conceptual papers. Systematic reviews were not eligible, but we screened references for additional eligible primary studies. Publications in the form of editorials, abstracts, protocols, unpublished theses, conference proceedings were not eligible.
FB and ARG independently screened 50 titles and abstracts according to the eligibility criteria and compared and discussed results. Based on discrepancies, they modified the eligibility criteria and discussed how to apply them. Thereafter, FB screened all remaining titles, and discussed all uncertainties with ARG and the research team. FB retrieved all potentially eligible articles. FB and ARG independently screened a sample of 25 full-text articles, and again discussed selection discrepancies to further standardize how eligibility criteria were applied. Thereafter, FB screened all remaining full-text items.
Quality appraisal
We employed quality appraisal tools relevant to different research designs: Standards for Reporting Qualitative Research (SRQR) [ 32 ], the Good Reporting of a Mixed Methods Study (GRAMMS) tool [ 33 ], Critical Appraisal of a Questionnaire Study [ 34 ], Revised Standards for Quality Improvement Reporting Excellence (SQUIRE 2.0) tool [ 35 ], and the Critical Appraisal Checklist for Quasi-Experimental Studies [ 36 ]. FB and ARG independently assessed and compared the quality of a sample of seven studies each. Thereafter, FB assessed the quality of the remaining 24 studies.
Data extraction
We developed a data extraction form to extract information on study characteristics (date of publication, country, purpose, research design) and MM KB characteristics, roles, activities, enablers, barriers, and impacts. To pilot test data extraction, FB and ARG independently extracted data from the same 25 articles, then compared results and discussed how to refine data extraction. Thereafter, FB extracted data from remaining articles, which was independently checked by ARG, and then reviewed by the research team.
Data analysis
FB and ARG conducted an initial reading and coding of a sample of articles independently. Codes were assigned to significant elements of data within the results and conclusions sections of the eligible articles and grouped into relevant categories with shared characteristics and organized into preliminary themes. This was an iterative process that involved ongoing consultation with the research team, who provided feedback on the codes and themes.
We created a matrix of MM KB roles and activities from extant MM and KB theory [ 7 , 18 , 22 , 37 ] and deductively mapped themes from included studies with the matrix to help inform the analysis and interpretation of our findings. As per CIS methodology, we developed an integrative grid (matrix table) where themes pertaining to MM KB roles and activities formed columns, and themes mapped to those roles/activities from individual studies formed rows [ 31 ]. The grid helped us integrate the evidence across studies and explore relationships between concepts and themes to inductively develop synthetic constructs [ 31 , 38 ]. Using a constant comparative approach, we critiqued the synthetic constructs with the full sample of papers to identify conceptual gaps in the available evidence in relation to our aims, and to ensure that the constructs were grounded in the data [ 31 , 38 ]. Our interpretive reflections on MM KB roles, activities, factors, and impacts led us to develop “synthetic arguments” and we used the arguments to structure our findings (attributes, roles, activities, impacts, enablers, barriers) in an organizing framework to capture our interpretation of how MMs function as KBs in healthcare organizations. We used NVivo 12 software to assist with data analysis.
Search results
The initial search yielded 9936 articles. Following removal of duplicates, 9760 titles were not eligible, and 176 items were retrieved as potentially relevant. Of those, 135 were excluded because the study design was ineligible (25), they did not examine MMs (27) or MM KBs (34), were not focused on the evaluation of an MM KB role (39), were editorials (4), or the publication was a duplicate (6). We included 41 articles for review (Fig. 1 PRISMA flow diagram). Additional file 4 includes all data extracted from included studies.
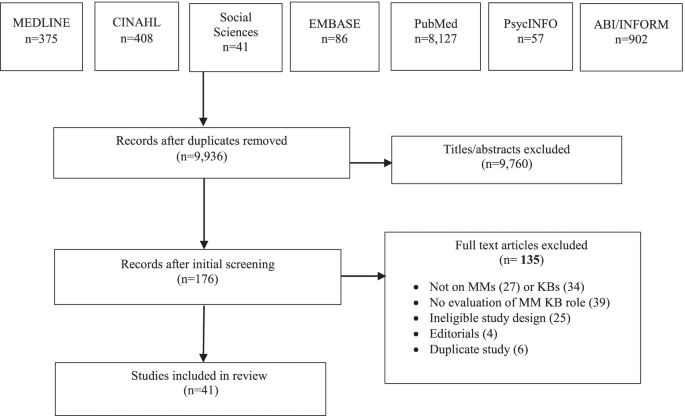
PRISMA flow diagram
Study characteristics
Eligible articles were published between 2003 and 2019. Three (7.3%) were conceptual and 38 (92.7%) were empirical studies. Conceptual articles discussed MM and KB theoretical constructs. Table 1 summarizes study characteristics. Studies examined the impacts of change efforts (47.3%), barriers to practice change (34.2%), and evaluation of KB interventions (18.4%). Most were qualitative (52.6%) and conducted in the United States (36.8%). Of study participants (34.2%) were MMs. In most studies, participants were nurses (63.1%) or allied health (13.2%) and based in hospitals (68.4%). Otherwise, (31.6%) were based in public health or occupational health departments, primary health care centers, Veterans Affairs Medical Centres, community care, and a senior’s care facility.
Quality assessment findings
A critical analysis of the included studies revealed issues related to research design, varying from data collected from heterogeneous healthcare settings and diverse types of MMs to the type of analyses completed (e.g., qualitative, mixed methods), to the strength of conclusions drawn from a few studies’ results (e.g., correlational, or causal). Fifteen (39.5%) studies met the criteria for quality. Twenty-three (60.5%) studies had minor methodological limitations (e.g., no research paradigm identified in qualitative studies, and mixed methods studies did not describe the integration of the two methods) (Additional file 5 ). These methodological flaws did not warrant exclusion of any studies as they provided relevant insights regarding the emerging framework.
MM KB attributes
Seven (18.4%) studies described MM KB attributes (Table 2 ). Of those, 4 (10.5%) identified MM attributes, 2 (5.2%) identified KB attributes, and 1 (2.6%) identified nurse knowledge broker attributes. MM KBs were described as confident, enthusiastic, and experienced with strong research skills [ 41 , 45 ]. They were also responsive and approachable, with an understanding of the complexity of an innovation and the organizational context [ 42 , 43 , 44 ].
MM KB roles and activities
Table 3 summarizes themes pertaining to roles and activities. A total of 63 activities were grouped in the following 12 MM KB roles: (1) gather data, (2) coordinate projects, (3) monitor and evaluate the progress of a project, (4) adjust implementation to organizational context, (5) disseminate information, (6) facilitate networks, (7) bridge the evidence-to-practice gap, (8) engage stakeholders, (9) convince others of the need for, and benefit of a project, (10) coach staff, (11) provide tools and resources and (12) function as a strategic influencer. Roles did not differ among MM KBs in hospital and non-hospital settings.
Table 4 summarizes the frequency of each of the 12 MM KB roles across included studies. The two most common MM KB roles were to monitor and evaluate the progress of a project (14, 36.8%) [ 40 , 41 , 47 , 48 , 49 , 50 , 51 , 54 , 57 , 60 , 63 , 64 , 65 , 66 ] and to convince others of the need for, and benefit of a project (12, 31.6%) [ 46 , 47 , 48 , 50 , 51 , 55 , 58 , 61 , 64 , 65 , 66 , 67 ]. For example, MM KBs played an important role in monitoring the progress of projects to evaluate and reinforce practice change [ 41 , 50 ]. To convince others of the need for, and benefit of a project and to promote staff buy-in, they held ongoing conversations with staff to help them understand the rationale for change, reinforce the message, and encourage staff to consistently maintain the innovations on their units [ 46 , 48 , 66 ]. The least common MM KB role was project coordination (4, 10.5%) [ 39 , 47 , 48 , 56 ].
Several of the identified MM KB roles aligned with five KB roles in prior published frameworks [ 7 , 22 ] and MM role theory [ 18 , 37 ] (Table 5 ). For example, 31 (81.6%) studies described MM KB roles of gather data, project coordination, disseminate information , and adjust implementation to organizational context , which aligned with the roles and activities of a KB knowledge manager. Twenty-nine (76.3%) studies described the MM KB roles of provide tools and resources, convince others of the need for and benefit of a project, and coach staff , which aligned with the roles and activities of a KB capacity builder. We found overlap between the MM KB roles and the four hypothesized roles in MM role theory: (1) disseminate and obtain information, (2) adapt information and the innovations, (3) mediate between strategy and day to day activities, and (4) selling innovation implementation) [ 18 , 37 ]. For example, we found that as capacity builders, MM KBs also mediated between strategy and day-to-day activities such as coaching staff and providing resources, and in the role of knowledge manager, MM KBs obtained, diffused, and synthesized information [ 18 , 37 ].
While MM KB roles identified in included studies aligned with the five previously identified KB roles, the CIS approach we employed identified 12 distinct roles that were further characterized based on corresponding activities associated with each of the 12 roles. Therefore, while this research agrees with prior work on MM KB roles, it represents a robust framework of MM KB roles and activities by elaborating the complexity of MM KB roles and activities.
We fully described two roles compared with prior frameworks: to convince others of the need for and benefit of a project, and function as a strategic influencer. To convince others of the need for and benefit of a project (e.g., a quality improvement, best practice guideline implementation, or innovation), MM KBs used tactics such as role modelling their commitment, providing the rationale for the change, being enthusiastic about its adoption, offering positive reinforcement, and providing emotional support [ 47 , 50 , 58 ]. The role of strategic influencer featured in 7 (18.4%) studies [ 39 , 48 , 52 , 56 , 62 , 65 , 68 ]. For example, MM KBs were influential at the executive level of the hospital, advocating for innovations among less involved team members and administrators, including the hospital board, were members of organizational decision-making groups for strategic planning, and served as an authoritative contact for initiatives.
Factors that influence MMs knowledge brokering
Table 6 summarizes the enablers and barriers of MM KB roles and activities, organized as individual or organizational factors. We identified four enablers at the organizational level: senior management support, availability of resources, engaged staff, and alignment to strategy. The most common was senior management support, featured in 12 (32.0%) studies. We found that senior management support enhanced the commitment of MM KBs to innovation implementation [ 16 , 17 , 19 , 44 , 45 , 52 , 61 , 63 , 66 , 67 , 68 , 69 , 70 ]. For example, senior managers empowered and supported MM KBs to make decisions by ensuring that the necessary structures and resources were in place, and by conveying that the implementation was an organizational priority [ 66 , 68 ]. We identified three individual-level facilitators: training and mentorship, personal attributes, and experience in the MM role. The most common facilitator was training and mentorship, featured in 8 (21.1%) studies. We found that training and mentorship with more experienced managers was important to the success of MM KBs and their projects, especially if they were new to their role [ 16 , 17 , 19 , 41 , 42 , 48 , 54 , 68 ].
Studies reported more barriers ( n = 8) than enablers ( n = 7). We found four organizational barriers: a lack of resources, lack of senior management support, staff resistance, and a lack of time. The most common barriers were lack of resources in 12 (32.0%) studies and lack of time in 12 (32.0%) studies. A lack of resources (budget constraints, limited staff) made it challenging for MM KBs to move their projects forward [ 39 , 42 , 44 , 47 , 52 , 55 , 57 , 64 , 68 , 69 , 70 , 71 ]. For example, inadequate funds interfered with obtaining appropriate resources and undermined the feasibility of implementing projects [ 47 , 55 ]. In addition, staffing issues created difficulty in engaging staff in project work and low staffing levels limited capacity to provide desired standards of care [ 42 , 64 ]. Additionally, a lack of protected time for data collection or other project work was identified as a significant barrier to implementing projects [ 17 , 19 , 39 , 42 , 44 , 47 , 52 , 55 , 57 , 64 , 68 , 71 ]. MM KBs also lacked the time to nurture, support and adequately coach staff [ 39 , 55 ].
We identified four individual-level barriers: lack of formal training, dissatisfaction with work life balance, being caught in the middle, and professional boundaries. The most common barriers were lack of formal training (8, 21.1%) and dissatisfaction with work life balance (8, 21.1%). For example, a lack of formal training resulted in MM KBs being unprepared for managerial roles and without the knowledge and skills to promote effective knowledge brokering and knowledge transfer with end users [ 17 , 39 , 41 , 42 , 55 , 57 , 69 , 71 ]. We also found that heavy workloads and conflicting priorities left MM KBs often dissatisfied with their work life balance and hindered their ability to successfully complete projects [ 42 , 44 , 51 , 52 , 57 , 61 , 64 , 71 ]. For example, because of multiple responsibilities and conflicting priorities, MM KBs were often pulled away to address problems or were so absorbed by administrative tasks that they had no time to complete project responsibilities [ 44 , 64 ].
Impact on service delivery and outcomes
Eight (21.1%) studies showed that MM KBs had some impact on organizational and provider outcomes [ 16 , 40 , 43 , 44 , 47 , 56 , 62 , 67 ]. One (2.6%) study reported that practice changes were greater when associated with higher MM leadership scores (OR 1.92 to 6.78) and when MMs worked to help create and sustain practice changes [ 40 ]. One (2.6%) study reported the impact of senior managers’ implementation of an evidence-based Hospital Elder Life Program on administrative outcomes (e.g., reduced length of stay and cost per patient), clinical outcomes (e.g., decreased episodes of delirium and reduced falls), and provider outcomes (e.g., increased knowledge and satisfaction) [ 67 ].
Two (5.3%) studies reported the impact of a Clinical Nurse Leader role on care processes at the service level in American hospitals. Benefits were evident in administrative outcomes such as RN hours per patient day (increased from 3.76 to 4.07) and in reduced surgical cancellation rates from 30 to 14%. There were also significantly improved patient outcomes in dementia care, pressure ulcer prevention, as well as ventilator-assisted pneumonia [ 56 , 62 ]. One (2.6%) study reported financial savings [ 56 ].
Four (10.5%) studies reported the effect of a KB strategy on health professionals’ knowledge, skills, and practices [ 16 , 43 , 44 , 47 ]. For example, Traynor et al. [ 44 ] found that participants who worked closely with a KB showed a statistically significant increase in knowledge and skill (average increase of 2.8 points out of a possible 36 (95% CI 2.0 to 3.6, p < 0.001) from baseline.
Organizing framework of MM KBs in healthcare organizations
We sought to capture the roles, activities, enablers, barriers and impacts of MM KBs across diverse healthcare settings in an organizing framework (Fig. 2 Organizing framework of MMs who function as knowledge brokers in healthcare organizations). From our interpretation of the published evidence, the findings across studies were categorized into 12 roles and 63 associated activities to represent specific ways in which MM KBs described their roles and activities during project implementation. Influencing factors were categorized into individual and organizational enablers and barriers that influence the efforts of MM KBs in healthcare organizations. While attributes were categorized as enablers, their level of importance as enablers emerged from our synthesis in how they operated in practice. The types of outcomes that we examined also varied between changes in care practice, processes, and competencies which we constructed into provider and organizational outcomes. Our emergent insights were used to construct four synthesizing arguments from the available literature: (1) MM KBs have attributes that equip and motivate them to implement practice change and innovations in healthcare organizations, (2) MMs enact KB roles and activities in healthcare organizations, (3) enablers and barriers influence the knowledge brokering efforts of MMs in healthcare settings; and (4) MM KB efforts impact healthcare service delivery. These synthesizing arguments were used to structure the organizing framework presented in Fig. 2 , which depicts how MM function as KBs in healthcare organizations and their impact on service delivery.
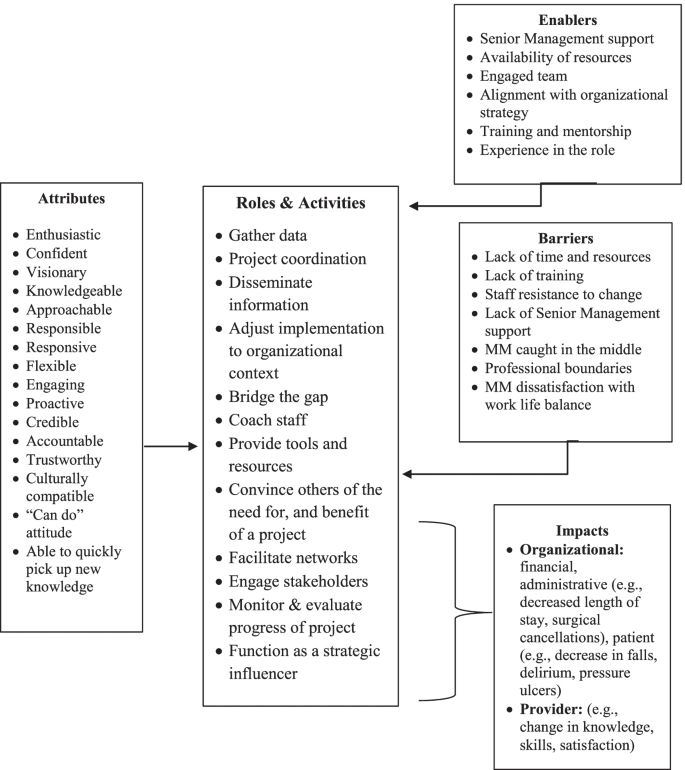
Organizing framework of MMs who function as knowledge brokers in healthcare organizations
We conducted a CIS to synthesize published research on factors that influence the roles, activities, and impacts of MM KBs in healthcare organizations. As per CIS, our output was an organizing framework (Fig. 2 ) that promotes expansive thinking about and extends knowledge of MM KBs in healthcare settings. We identified 63 activities organized within 12 distinct MM KB roles, which is far more comprehensive than any other study [ 7 , 22 ]. We build on prior frameworks and characterize further the roles of strategic influencer and convincing others of the need for, and benefit of an innovation or evidence-based practice. We identified organizational and individual enablers and barriers that may influence the efforts and impact of MM KBs in health care. Of note, a key enabler was senior leadership support while a key barrier for MM KBs was a lack of formal training in project implementation. Such factors should be closely considered when looking at how to strengthen the MM KB role in practice. Furthermore, we found that the MM KB role was associated with enhanced provider knowledge and skills, as well as improved clinical and organizational outcomes.
We offer a novel conceptualization of MM KBs in healthcare organizations that has, thus far, not been considered in the literature. Our theoretical insights (summarized in Fig. 2 ) are an important first step in understanding how individual and organizational factors may influence how MMs enact KB roles, and the impact they have on service delivery and associated outcomes. We found that the many MM KB roles and activities corresponded to the characterization of KB roles in the literature and substantiated MM role theory. Our findings corroborate previous studies and systematic reviews by confirming that MMs function as KBs and build on the MM and KB theoretical constructs previously identified in the literature [ 7 , 18 , 21 , 22 , 37 , 46 , 48 ]. Building on Birken and colleagues’ theory [ 37 ], we found significant overlap between MM and KB roles and activities. Figure 2 helps to define and analyze the intersection of these roles while distinguishing MM KB roles and activities more clearly from other administrative roles.
We contend that Fig. 2 has applicability across a range of healthcare settings and may be used by hospital administrators, policymakers, service providers, and researchers to plan projects and programs. It may be used as a resource in strategic planning, to re-structure clinical programs, build staff capacity, and optimize HR practices. For example, Fig. 2 could be used as a foundation to establish goals, objectives, or key performance indicators for a new or existing clinical program; refine job postings for MM roles to encompass optimal characteristics of candidates to enable KB activities; or identify new evaluation criteria for staff performance and training gaps in existing HR practices. It could also help decision makers take on pilot projects to formalize the KB role in healthcare.
Figure 2 is intended to foster further discussion of the role that MMs play in brokering knowledge in healthcare settings. It can be modified for specific applications, although we encourage retaining the basic structure (reflecting the synthesizing arguments). For example, the factors may change depending on specific localized healthcare contexts (i.e., acute care versus long-term care, or rehabilitation). Although the use of our framework in practice has yet to be evaluated, it may be strengthened with the results of additional mixed methods studies examining MM KBs as well as quasi-experimental studies applying adapted HR practices based upon our framework. As more studies are reported in the literature, the roles, activities, factors, and outcomes can be further refined, organized, and contextualized. Figure 2 can also be used as a guide for future studies examining how MMs enact the KB role across healthcare settings and systems, disciplines, and geographic locations.
Our synthesis provides new insights into the roles of MM KBs in healthcare settings. For example, we further elucidate two MM KB roles: 1) functioning as a strategic influencer; and 2) convincing others of the need for, and benefit of an innovation or evidence-based practice. These are important roles that MM KBs enact when preparing staff for implementation and corroborate Birken et al.’s hypothesized MM role of selling innovation implementation [ 18 , 37 ]. Our findings validate the organizational change literature that emphasizes the important information broker role MMs play in communicating with senior management and helping frontline staff achieve desired changes by bridging information gaps that might otherwise impede innovation implementation [ 37 ]. Our new conceptualization of how MM KBs navigate and enact their roles, and the impact they may have on service delivery and associated outcomes extends the findings of recent studies. These studies found that the role of MMs in organizational change is evolving and elements such as characteristics and context may influence their ability to facilitate organizational adaptation and lead the translation of new ideas [ 53 , 72 , 73 ]. However, further research is required to test and further explicate these relationships in the broader context of practice change.
Our synthesis both confirms and extends previous research by revealing organizational and individual factors that both enabled and hindered MM KBs efforts in healthcare organizations. An important organizational factor in our study was having senior management support. We found that MM KBs who had healthy supportive working relationships with their senior leaders led to project success. This support was critical because without it they experienced significant stress at being “caught in the middle” trying to address the needs of staff while also meeting the demands of senior management. Recent studies confirm our finding that senior management engagement is essential to MM KBs’ ability to implement innovations and underscores the need for senior leaders to be aware of, and acknowledge, the impact that excessive workload, competing demands, and role stress can play in their effectiveness [ 19 , 74 ].
The personal attributes of MM KBs as well as their level of experience were both important factors in how they operated in practice. We identified that key attributes of MM KBs contributed to their ability to drive implementation of initiatives and enhanced staff acceptance and motivation to implement practice change [ 75 , 76 ]. Our findings corroborate recent studies that highlight how the key attributes of effective champions (those that are intrinsic and cannot be taught) [ 77 , 78 , 79 ] may contribute to their ability to lead teams to successful implementation outcomes in healthcare organizations [ 80 , 81 , 82 ]. We also found that experienced MM KBs were well trained, knowledgeable, and better prepared to understand the practice context than novice MM KBs, but a lack of formal training in project implementation was an impediment for both. This emphasizes the importance of providing opportunities for professional development and training to prepare both novice and experienced MM KBs to successfully implement practice change. Our findings contribute to the growing knowledge base regarding what makes an effective MM KB. However, future research should focus on generating evidence, not only on the attributes of MM KBs, but also on how those attributes contribute to their organizational KB roles as well as the relationships among specific “attributes” and specific KB roles. More research is also needed to better understand how and what skills can be taught to boost the professional growth of MM KBs in health care.
Organizational theory and research may provide further insight into our findings and guidance for future research on the role of MM KBs in healthcare organizations. For example, the literature suggests that by increasing MMs’ appreciation of evidence-based practice, context, and implementation strategies may enhance their role in implementing evidence-based practices in healthcare organizations [ 18 , 83 , 84 ]. We found that MM KBs’ commitment to the implementation of an evidence-based project was influenced by the availability of resources, alignment with organizational priorities, a supportive staff and senior leadership. Extending from organizational theory and research, further investigation is needed to explore the nature of the relationship between these factors and the commitment of MM KBs to evidence-based practice implementation and subsequent outcomes.
When assessing the impact of MM KBs in hospitals, we found some evidence of changes in organizational and provider outcomes, suggesting MM KB impact on service delivery. Given that the available outcome data were limited, associational in nature, or poorly evaluated, it was challenging to identify strong thematic areas. Like our study, several systematic reviews also reported the lack of available outcome data [ 7 , 18 , 21 ]. This highlights an important area for research. Future research must include evaluation of the effectiveness of MM KBs and establish rigorous evidence of their impact on service delivery.
Our findings have important implications for policy and practice. MMs are an untapped KB resource who understand the challenges of implementing evidence-based practices in healthcare organizations. Both policy makers and administrators need to consider the preparation and training of MM KBs. As with other studies, our study found that providing MM KBs with opportunities for training and development may yield a substantial return on investment in terms of narrowing evidence-to-practice gaps in health care [ 48 ]. Thus, an argument can be made for recruiting and training MM KBs in health care. However, the lack of guidance on how to identify, determine and develop a curriculum to prepare MM KBs requires more research.
Our synthesis revealed numerous activities associated with 12 MM KB roles providing further insight into the MM role in healthcare settings. Our list of 63 activities (Table 2 ) has implications for practice. We found that MMs enact numerous KB roles and activities, in addition to their day-to day operational responsibilities, highlighting the complexity of the MM KB role. Senior leaders and administrators must acknowledge this complexity. A greater understanding of these KB roles and activities may lead to MM implementation effectiveness, to sustainable MM staffing models, and to organizational structures to support the KB efforts that many MMs are already doing informally. For example, senior leaders and administrators need to take the MM KB role seriously and explicitly include KB activities as a core function of existing MM job descriptions. To date, the KB role and associated activities are not typically or explicitly written into the formal job descriptions for MMs in healthcare settings, as their focus is primarily on operational responsibilities. A formal job description for MM KBs would improve the KB capacity of MMs by giving them the permission and recognition to implement KB-related functions. Our findings inform future research by more clearly articulating the MM KB roles and activities that may be essential to the implementation of evidence-based practice and highlights a much-needed area for future work.
Our study features both strengths and weaknesses. One strength in using CIS methodology was the ability to cast a wide net representing a range of research designs of included studies. This included studies in which MMs were required to be KBs by senior leaders or functioned explicitly as KBs. This enabled us to identify and include diverse studies that made valuable theoretical contributions to the development of an emerging framework, which goes beyond the extant theories summarized in the literature to date [ 18 ]. In contrast to prior systematic reviews of MM roles in implementing innovations [ 18 ], the CIS approach is both systematic and iterative with an interpretive approach to analysis and synthesis that allowed us to capture and critically analyze an in-depth depiction of how MMs may enact the KB role in healthcare organizations. Our synthesis also revealed numerous activities associated with the 12 identified MM KB roles. The resulting theoretical insights were merged into a new organizing framework (Fig. 2 ). These insights are an important first step in understanding how individual and organizational factors may influence how MMs enact KB roles, and the impact they have on service delivery.
Although CIS is an innovative method of synthesizing the literature and continues to evolve, it does have limitations. CIS has yet to be rigorously evaluated [ 85 , 86 ]. While there is some precedent guiding the steps to conduct a CIS, one weakness is that CIS is difficult to operationalize. Another weakness is that the steps to conduct CIS reviews are still being refined and can lack transparency. Therefore, we used standardized, evidence-based checklists and reporting tools to assess transparency and methodological quality, and an established methodology for coding and synthesis. We provided an audit trail of the interpretive process in line with the ENTREQ guidance. Still, there was a risk of methodological bias [ 28 , 85 , 86 ]. Another weakness of qualitative synthesis is its inability to access first order constructs that is the full set of participants’ accounts in each study. As reviewers, we can only work with the data provided in the papers and, therefore, the findings of any review cannot assess primary datasets [ 31 ]. Study retrieval was limited to journals that are indexed in the databases that were searched. We did not search the grey literature, assuming that most empirical research on MM KBs would be found in the indexed databases. Finally, we may have synthesized too small a sample of papers to draw definitive conclusions regarding different aspects of MMs as KBs.
Our study is a first step in advancing the theoretical and conceptual conversation regarding MM KBs by articulating the attributes, roles, activities, and factors influencing their efforts and impact. Through the generation of a novel organizing framework, we identify a potential combination of roles for those in MM positions who may also function as KBs in healthcare organizations. Our study is a timely contribution to the literature and offers an initial understanding of extant evidence of the KB role MMs play in health care. Our framework has utility for policymakers, administrators, and researchers to strengthen the MM role and, ultimately, improve quality of care.
Availability of data and materials
All data generated or analyzed during this study are included in this published article and its supplementary information files.
Abbreviations
Middle Manager
Knowledge Broker
Middle managers who function as Knowledge brokers
Knowledge Translation
Critical Interpretive Synthesis
Quality Improvement
Enhancing Transparency in Reporting the Synthesis of Qualitative Research
Berenson RA, Rice Y. Beyond measurement and reward: methods of motivating quality improvement and accountability. Health Serv Res. 2015;2(2):155–65.
Google Scholar
Nunes R, Rego G, Brandao C. Healthcare regulation as a tool for public accountability. Medical Healthcare & Philosphy. 2009;12:257–64.
Article Google Scholar
Grimshaw JM, Eccles M, Lavis JN, Hill SJ, Squires JE. Knowledge translation of research findings. Implement Sci. 2012;7(50):1–17 Available from: http://www.implementationscience.com/content/7/1/50 .
McGlynn EA, Asch SM, Adams J, Keesey J, Hicks J, DeCristofaro A. The quality of health care delivered to adults in the United States. N England Journal of Medicine. 2003;348:2635–45. https://doi.org/10.1056/NEJMsa022615 .
Squires JE, Graham ID, Grinspun D, Lavis J, Legare F, Bell R, et al. Inappropriateness of health care in Canada: a systematic review protocol. Systematic Review. 2019;8(50). https://doi.org/10.1186/s13643-019-0948-1 .
Innis J, Berta W. Routines for change: How managers can use absorptive capacity to adopt and implement evidence-based practice. Journal of Nursing Management. 2016;24(6). doi: https://doi.org/10.1111/jonm.12368 .
Bornbaum C, Kornas K, Pierson L, Rosella LC. Exploring the function and effectiveness of knowledge brokers as facilitators of knowledge translation in health-realted settings: a systematic review and thematic analysis. Implement Sci. 2015;10. https://doi.org/10.1186/s13012-015-0351-9 .
Currie G, Burgess N, White L, Lockett A, Gladman J, Waring J. A qualitative study of the knowledge-brokering role of middle-level managers in service innovation: managing the translation gap in patient safety for older persons’ care. Health Services and Delivery Research. 2014;2(32). https://doi.org/10.3310/hsdr02320 .
Canadian Health Services Research Foundation. The theory and practice of knowledge brokering in Canada’s health system. 2003. Ottawa, Ontario: www.chsrf.ca .
Flodgren G, Parmelli E, Doumit G, Gattellari M, O’Brien MA, Grimshaw J, et al. Local opinion leaders: effects on professional practice and health care outcomes. Cochrane Database Systematic Review. 2011;8. https://doi.org/10.1002/14651858.CD00125 .
Soo S, Berta W, Baker G. Role of champions in the implementation of patient safety practice change. Healthcare Quarterly. 2009;12:123–8.
Thompson GN, Estabrooks CA, Degner LF. Clarifying the concepts in knowledge transfer: a literature review. J Adv Nurs. 2006;53(6):691.
Glegg S. Knowledge brokering as an intervention in paediatric rehabilitation practice. Int J Ther Rehabil. 2010;17(4):203–9.
Rivard LM, Russell DJ, Roxborough L, Ketelaar M, Bartlett DJ, Rosenbaum P. Promoting the use of measurement tools in practice: a mixed methods study of the activities and experiences of physical therapist knowledge brokers. Phys Ther. 2010;90(11):1580–90.
Russell D, Rivard LM, Walter SD, Rosebaum PL, Roxborough L, Cameron D, et al. Using knowledge brokers to facilitate the uptake of pediatric measurement tools into clinical practice: a before-after intervention study. Implementation Science. 2010;5(92).
Dobbins M, Traynor RL, Workentine S, Yousefi-Nooraie R, Yost J. Impact of an organization-wide knowledge translation strategy to support evidence- informed public health decision making. BMC Public Health. 2018;18:1412.
Dobbins M, Greco L, Yost J, Traynor R, Decorby-Watson K, et al. A description of a tailored knowledge translation intervention delivered by knowledge brokers within public health departments in Canada. Health Research Policy and Systems. 2019;17(63):1–8. https://doi.org/10.1186/s12961-019-0460-z .
Birkin S, Clary A, Tabriz AA, Turner K, Meza R, Zizzi A, et al. Middle managers’ role in implementing evidence-based practices in healthcare: a systematic review. Implementation Science. 2018;13(149): 1-14. Available from: https://doi.org/10.1186/s13012-018-0843-5 .
Urquhart R, Kendell C, Folkes A, Reiman T, Grunfeld E, Porter GA. Factors influencing middle mangers’ commitment to the implementation of innovation in cancer care. J Health Services Res Pol. 2019;24(2):91–9. https://doi.org/10.1177/1355819618804842 .
Burgess N, Currie G. The knowledge brokering role of the hybrid middle level manager: the case of healthcare. Br J Manag. 2013;24:S132–42.
Van Eerd D, Newman K, DeForge R, Urquhart R, Cornelissen E, Dainty KN. Knowledge brokering for healthy aging: a scoping review of potential approaches. Implement Sci. 2016;11:140. https://doi.org/10.1186/s13012-016-0504-5 .
Article PubMed PubMed Central Google Scholar
Glegg SM, Hoens A. Role domains of knowledge brokering: a model for the health care setting. JNPT. 2016;40:115–23. https://doi.org/10.1097/NPT.000000000000012 .
Article PubMed Google Scholar
Schleifer Taylor J, Verrier MC, Landry MD. What do we know about knowledge brokers in pediatric rehabilitation? A systematic search and narrative summary. Physiother Can. 2014;66(2):143–52.
Ward V, House A, Hamer S. Knowledge brokering: exploring the process of transferring knowledge into action. BMC Health Serv Res. 2009;9(12):1–6.
Cranley LA, Cummings GG, Profetto-McGrath J, Toth F, Estabrooks CA. Facilitation roles and characteristics associated with research use by healthcare professionals: a scoping review. BMJ Open. 2016;7. https://doi.org/10.1136/bmjopen-2016-014384 .
Dixon-Woods M, Cavers D, Agarwal S, Annandale E, Arthur A, Harvey J, et al. Conducting a critical interpretive synthesis of the literature on access to healthcare by vulnerable groups. BMC Med Res Methodol. 2006;6:35. https://doi.org/10.1186/1471-2288-6-35 .
Kangasniemi M, Kallio H, Pietila AM. Towards environmentally responsible nursing: a critical interpretive synthesis. J Adv Nurs. 2013;70(7):1465–78.
Tong A, Flemming K, McInnes E, Oliver S, Craig J. Enhancing transparency in reporting the synthesis of qualitative research: ENTREQ. BMC Medical Research Methodology. 2012;12(181) Available from: http://www.biomedcentral.com/1471-2288/12/181 .
Shenton K. Strategies for ensuring trustworthiness in qualitative research projects. Educ Inf. 2004;22:63–75.
McGowan J, Sampson M, Lefebvre C. An evidence based checklist for the peer review of electronic search strategies (PRESS EBC). Evid Based Libr Inf Pract. 2010;5(1):149–54.
Flemming K. Synthesis of quantitiative and qualitative research: an example of critical interpretive synthesis. J Adv Nurs. 2010;66(1):201–17.
Standards for Reporting Qualitative Research. (2017, April 15). Available from: http://www.equator-network.org .
O'Cathain A, Murphy E, Nicholl J. The quality of mixed methods studies in health services research. J Health Serv Res Policy. 2008;13(2):92–8.
Roever L. Critical appraisal of a questionnaire study. Evidence Based Medicine and Practice. 2016;1(2). Available from: https://doi.org/10.4172/ebmp.1000e110 .
Ogrinc G, Davies L, Goodman D, Batalden P, Davidoff F, Stevens D. SQUIRE 2.0 (Standards for Quality Improvement Reporting Excellence): revided publication guidelines from a detailed consensus process. The Journal of Continuing Education in Nursing. 2015; 46(11):501–507. Available from: https://doi.org/10.3928/00220124-20151020-02 .
Tufanaru C, Munn Z, Aromataris E, Campbell J, Hoop L. Systematic reviews of effectiveness. In: Aromataris E, Munn Z, editors. Joanna Briggs Institute Reviewers Manual. The Joanna Briggs Institute. 2017. Available from: https://reviewersmanual.joannabriggs.org .
Birkin SA, DiMartino LD, Kirk MA, Lee S, McCelland M, Albert NA. Elaborating on theory with middle managers’ experience implementing healthcare innovations in practice. Implement Sci. 2016;11:2. https://doi.org/10.1186/s13012-015-0362-6 .
Johnson M, Tod A, Collins K, Brummell S. Prognostic communication in cancer: a critical interpretive synthesis of the literature. Eur J Oncol Nurs. 2015;19(5):554–67.
Bullock A, Morris ZS, Atwell C. Collaboration between health services managers and researchers: making a difference. Original Research. 2012;17(2):2–10. https://doi.org/10.1258/jhsrp.2011.011099 .
Donahue KE, Halladay JR, Wise A, Reiter K, Lee SD, Ward K, et al. Facilitator of transforming primary care: a look under the Hood at practice leadership. Ann Fam Med. 2013;11:527–33. https://doi.org/10.1370/afm.1492 .
Kakyo TA, Xiao LD. Nurse managers’ experiences in continuous quality improvement in resource-poor healthcare settings. Nurs Health Sci. 2017;19:244–9. https://doi.org/10.1111/nhs.12338 .
Kitson A, Silverton H, Wiechula R, Zeitz K, Marcoionni D, Page T. Clinical nursing’, team members’ and service managers’ experiences of implementing evidence at a local level. J Nurs Manag. 2011;19:542–55. https://doi.org/10.1111/j.1365-2834.2011.01258 .
Schreiber J, Marchetti GF, Racicot B, Kaminski E. The use of knowledge translation program to increase use of standardized outcome measures in outpatient pediatric physical therapy clinic: administrative case report. American Physical Therapy Association. 2015;95(4):613–29. https://doi.org/10.2522/ptj.20130434 .
Traynor R, DeCorby K, Dobbins M. Knowledge brokering in public health: a tale of two studies. The Royal Society for Public Health. 2014;128:533–544. https://doi.org/10.1016/j.puhe.2014.01.015 .
Catallo C. Should nurses be knowledge brokers? Competencies and organizational resources to support the role. Nurs Leadersh. 2015;28(1):24–37.
Engle RL, Lopez ER, Gormley KE, Chan JA, Charns MP, VanDeusen Lukas C. What roles do middle managers play in implementation of innovative practices? Health Care Manag Rev. 2017;42(1):14–27. https://doi.org/10.1097/HMR.0000000000000090 .
Hitch D, Rowan S, Nicola-Richmond K. A case study of knowledge brokerage in occupational therapy. MA Healthcare Ltd. 2014.
Urquhart R, Kendell C, Folkes A, Reiman T, Grunfeld E, Porter GA. Making it happen: middle managers’ roles in innovation implementation in health care. Worldviews Evid-Based Nurs. 2018;15(6):414–23. https://doi.org/10.1111/wvn.12324 .
Ploeg J, Skelly J, Rowan M, Edwards N, Davies B, Grinspun D. The role of nursing best practice champions in diffusing practice guidelines: a mixed methods study. Worldviews Evid-Based Nurs. 2010:238–51. https://doi.org/10.1111/j.1741-6787.2010.00202 .
Fleiszer AR, Semenic SE, Ritchie JA, Richer MC, Denis JL. Nursing unit leaders’ influence on the long-term sustainability of evidence-based practice improvements. J Nurs Manag. 2015;24:309–18. https://doi.org/10.1111/jonm.12320 .
Jeffs L, Indar A, Harvey B, McShane J, Bookey-Bassett S, Flintoft V, et al. Enabling role of manager in engaging clinicians and staff in quality improvement. J Nurs Care Qual. 2016;31(4):367–72. https://doi.org/10.1097/NCQ.0000000000000196 .
Warshawsky NE, Lake SW, Bradford A. Nurse managers describe their practice environment. Nurs Admin Q. 2013;34(4):317–25. https://doi.org/10.1097/NAQ.0b013e3182a2f9c3 .
Gutberg J, Berta W. Understanding middle managers’ influence in implementing patient safety culture. BMC Health Serv Res. 2017;17(582):1–10. https://doi.org/10.1186/s12913-017-2533-4 .
Kislov R, Hodgson D, Boarden R. Professionals as knowledge brokers: the limits of Authority in Healthcare Collaboration. Public Adm. 2016;94(2):472–89. https://doi.org/10.1111/padm.12227 .
Shaw L, McDermid J, Kothari A, Lindsay R, Brake P, Page P. Knowledge brokering with injured workers: perspective of injured worker groups and health care professionals. Work. 2010;36:89–101. https://doi.org/10.3233/WOR-20101010 .
Wilson L, Orff S, Gerry T, Shirley BR, Tabor D, Caiazzo K, et al. Evolution of an innovative role: the clinical nurse leader. J Nurs Manag. 2013;21:175–81. https://doi.org/10.1111/j.1365-2834.2012.01454 .
Currie G, Burgess NA, Hayton JC. HR practices and knowledge brokering by hybrid middle managers in hospital settings: the influence of professional hierarchy. Human Resources Management. 2015;54(5):793–812. https://doi.org/10.1002/hrm.21709 .
Waring J, Currie G, Crompton A, Bishop S. An exploratory study of knowledge sharing and learning for patient safety? Soc Sci Med. 2013;98:79–86. https://doi.org/10.1016/j.socscimed.2013.08.037 .
Girard A, Rochette A, Fillion B. Knowledge translation and improving practices in neurological rehabilitation: managers’ viewpoint. Journal of Evaluation in Clinical Practice. 2013;19:60–7 10.1111/j.1365–2753.
Williams L, Burton C, Rycroft-Malone J. What works: a realist evaluation case study of intermediaries in infection control practice. J Adv Nurs. 2012;69(4):915–26. https://doi.org/10.1111/j.1365-2648.2012.06084 .
Bradley EH, Holmboe ES, Mattera JA, Roumanis SA, Radford MJ, Krumholz HM. The roles of senior Management in Quality Improvement Efforts: what are the key components? J Healthc Manag. 2003;48(1):16–29.
Ott KM, Haddock KS, Fox SE, Shinn JK, Walters SE, Hardin JW. The clinical nurse leader: impact on practice outcomes in the veterans health administration. Nurs Econ. 2009;27(6):363–71.
PubMed Google Scholar
Schell WJ, Kuntz SW. Driving change from the middle: an exploration of the complementary roles and leadership Behaviours of clinical nurse leaders and engineers in healthcare process improvement. Eng Manag J. 2013;25(4):33–43.
Jeffs LP, Lo J, Beswick S, Campbell H. Implementing an organization-wide quality improvement initiative. Nursing Administrative Quarterly. 2013;37(3):222–30. https://doi.org/10.1097/NAQ.0b013e318295ec9f .
Lalleman PCB, Smid GAC, Lagerwey MD, Oldenhof L, Schuurmans MJ. Nurse middle managers’ dispositions of habitus: a Bourdieusian analysis of supporting role Behaviours in Dutch and American hospitals. Adv Nurs Sci. 2015;38(3):E1–E16. https://doi.org/10.1097/ANS.0000000000000083 .
Article CAS Google Scholar
Birken SA, Lee S, Weiner BJ, Chin MH, Chiu M, Schaefer CT. From strategy to action: how top managers’ support increases middle managers’ commitment to innovation implementation in health care organization. Health Care Manag Rev. 2015;40(2):159–68. https://doi.org/10.1097/HMR.0000000000000018 .
Bradley EH, Webster TR, Schlesinger M, Baker D, Inouye SK. The roles of senior Management in Improving Hospital Experiences for frail older adults. J Healthc Manag. 2006;51(5):323–37.
Balding C. Embedding organisational quality improvement through middle manager ownership. International Journal of Health Care Quality Assurance. 2005;18(4):271–88. https://doi.org/10.1108/09526860510602541 .
Uvhagen H, Hasson H, Hansson J, von Knorring M. Leading top-down implementation processes: a qualitative study on the role of managers. BMC Health Serv Res. 2018;18:562.
Fryer A-K, Tucker AL, Singer SJ. The impact of middle manager affective commitment on perceived improvement program implementation success. Health Care Manag Rev. 2018;43(3):218–28.
Chang Chen H, Jones MK, Russell C. Exploring attitudes and barriers toward the use of evidence-based nursing among nurse managers in Taiwanese residential aged care facilities. J Gerontol Nurs. 2013;39(2):36–42.
Hansell V. Identifying the prevalence of influential factors on middle manager’s ability to lead organizational change within the context of community nursing and therapy services. Int J Healthcare Management. 2018;11(3):225–32.
Buick F, Blackman D, Johnson S. Enabling middle managers as change agents: why organizational support needs to change. Aust J Public Adm. 2017;77(2):222–35.
Breed M, Downing C, Ally H. Factors influencing motivation of nurse leaders in a private hospital group in Gauteng, South Africa: A quantitative study. Curationis. 2020;43(1): Available from: https://doi.org/10.4102/curationis.v43i1.2011 .
Kallas KD. Profile of an excellent nurse manager: identifying and developing health care team leaders. Nurse Admin Quarterly. 2014;38(3):261–8. https://doi.org/10.1097/NAQ.0000000000000032 .
Sellgren S, Ekvall G, Tomson G. Leadership styles in nursing management: preferred and perceived. J Nurs Manag. 2006;14:348–55.
Dobbins M, DeCorby K, Robeson P, Ciliska D, Hanna S, Cameron R, et al. A description of a knowledge broker role implemented as part of a randomized controlled trial evaluating three knowledge translation strategies. Implementation Science. 2009;4(23). Available from: http://www.implementationscience.com/content/4/1/23 .
Cook MJ. The attributes of effective clinical nurse leaders. Nurs Stand. 2001;15(35):33–6.
Gentry H, Prince-Paul M. The nurse influencer: a concept synthesis and analysis. Nurs Forum. 2020:1–7.
Demes JAE, Nickerson N, Farand L, Montekio VB, Torres P, Dube JG, et al. What are the characteristics of the champion that influence the implementation of quality improvement programs? Evaluation and Program Planning. 2020;80:101795.
Bonawitz K, Wetmore M, Heisler M, Dalton VK, Damschroder LJ, Forman J, et al. Champions in context: which attributes matter for change efforts in healthcare? Implementation Sci. 2020;15:62.
Bunce AE, Grub I, Davis JV, Cowburn S, Cohen D, Oakley J. Lessons learned about effective operationalization of champions as an implementation strategy: results from a qualitative process evaluation of a pragmatic trial. Implementation Sci. 2020;15:87.
Birkin SA, Currie G. Using organization theory to position middle-level managers as agents of evidence-based practice implementation. Implementation Sci. 2021;16:37.
Klein KI, Sorra JS. The challenge of innovation implementation. Acad Manag Rev. 1996;21(4):1055–80.
Tricco AC, Antony J, Soobiah C, Kastner M, MacDonald H, Cogo E, et al. Knowledge synthesis methods for integrating qualitative and quantitative data: a scoping review reveals poor operationalization of the methodological steps. J Clin Epidemiol. 2016a;73:29–35.
Tricco AC, Antony J, Soobiah C, Kastner M, MacDonald H, Cogo E, et al. Knowledge synthesis methods for generating or refining theory: a scoping review reveals that little guidance is available. J Clin Epidemiol. 2016b;73:36–42.
Download references
Acknowledgements
Not applicable.
Author information
Authors and affiliations.
Baycrest Health Sciences, 3560 Bathurst Street, Toronto, Ontario, M6A 2E1, Canada
Faith Boutcher
Institute of Health Policy, Management and Evaluation, University of Toronto, Health Sciences Building Suite 425, 155 College Street, Toronto, Ontario, M5T 3M6, Canada
Whitney Berta
Department of Community Health and Epidemiology, Dalhousie University, Room 413, 5790 University Avenue, Halifax, Nova Scotia, B3H 1V7, Canada
Robin Urquhart
University Health Network, 13EN-228, 200 Elizabeth Street, Toronto, Ontario, M5G 2C4, Canada
Anna R. Gagliardi
You can also search for this author in PubMed Google Scholar
Contributions
FB, ARG, WB, and RU conceptualized and planned the study, and developed the search strategy and data collection instruments. FB and ARG screened and reviewed articles. FB, ARG, WB and RU analyzed the data. All authors read and gave approval of the final version of the manuscript.
Corresponding author
Correspondence to Faith Boutcher .
Ethics declarations
Ethics approval and consent to participate, consent for publication, competing interests.
The authors declare that they have no competing interests.
Additional information
Publisher’s note.
Springer Nature remains neutral with regard to jurisdictional claims in published maps and institutional affiliations.
Supplementary Information
Additional file 1..
ENTREQ checklist
Additional file 2.
Search strategy
Additional file 3.
Eligibility criteria
Additional file 4.
Data extraction form for eligible studies
Additional file 5.
Quality appraisal tools and findings
Rights and permissions
Open Access This article is licensed under a Creative Commons Attribution 4.0 International License, which permits use, sharing, adaptation, distribution and reproduction in any medium or format, as long as you give appropriate credit to the original author(s) and the source, provide a link to the Creative Commons licence, and indicate if changes were made. The images or other third party material in this article are included in the article's Creative Commons licence, unless indicated otherwise in a credit line to the material. If material is not included in the article's Creative Commons licence and your intended use is not permitted by statutory regulation or exceeds the permitted use, you will need to obtain permission directly from the copyright holder. To view a copy of this licence, visit http://creativecommons.org/licenses/by/4.0/ . The Creative Commons Public Domain Dedication waiver ( http://creativecommons.org/publicdomain/zero/1.0/ ) applies to the data made available in this article, unless otherwise stated in a credit line to the data.
Reprints and permissions
About this article
Cite this article.
Boutcher, F., Berta, W., Urquhart, R. et al. The roles, activities and impacts of middle managers who function as knowledge brokers to improve care delivery and outcomes in healthcare organizations: a critical interpretive synthesis. BMC Health Serv Res 22 , 11 (2022). https://doi.org/10.1186/s12913-021-07387-z
Download citation
Received : 02 September 2021
Accepted : 07 December 2021
Published : 02 January 2022
DOI : https://doi.org/10.1186/s12913-021-07387-z
Share this article
Anyone you share the following link with will be able to read this content:
Sorry, a shareable link is not currently available for this article.
Provided by the Springer Nature SharedIt content-sharing initiative
- Middle managers
- Knowledge brokers
- Critical interpretive synthesis
BMC Health Services Research
ISSN: 1472-6963
- General enquiries: [email protected]
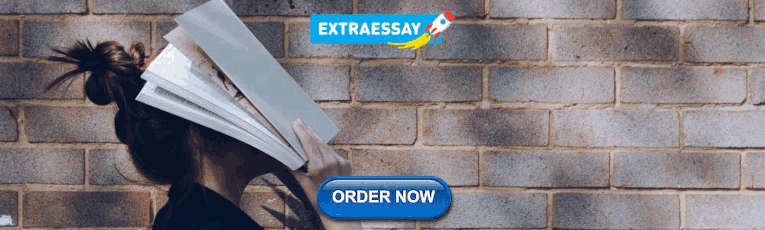
IMAGES
VIDEO
COMMENTS
quality. 1 Introduction. Critical appraisal describes the process of analyzing a study in a rigorous and. methodical way. Often, this process involves working through a series of questions. to ...
To practise following this framework for critically appraising a quantitative article, please look at the following article: Marrero, D.G. et al (2016) 'Comparison of commercial and self-initiated weight loss programs in people with prediabetes: a randomized control trial', AJPH Research, 106(5), pp. 949-956.
critiquing the literature, critical analysis, reviewing the literature, evaluation and appraisal of the literature which are in essence the same thing (Bassett and Bassett, 2003). Terminology in research can be confusing for the novice research reader where a term like 'random' refers to an organized manner of selecting items or participants ...
In this chapter, we will explore the critical appraisal process and provide you with the foundation required to start appraising quantitative research. Critical appraisal skills are important for anyone wishing to make informed decisions or improve the quality of healthcare delivery. Not all studies are carried out using rigorous methods.
The research process for conducting a critical analysis literature review has three phases ; (a) the deconstruction phase in which the individually reviewed studies are broken down into separate discreet data points or variables (e.g., breastfeeding duration, study design, sampling methods); (b) the analysis phase that includes both cross-case ...
Critical appraisal is the assessment of research studies' worth to clinical practice. Critical appraisal—the heart of evidence-based practice—involves four phases: rapid critical appraisal, evaluation, synthesis, and recommendation. This article reviews each phase and provides examples, tips, and caveats to help evidence appraisers ...
More. Critical appraisal tools and reporting guidelines are the two most important instruments available to researchers and practitioners involved in research, evidence-based practice, and policymaking. Each of these instruments has unique characteristics, and both instruments play an essential role in evidence-based practice and decision-making.
The sample size is central in quantitative research, as the findings should be able to be generalised for the wider population.10 The data analysis can be done manually or more complex analyses performed using computer software sometimes with advice of a statistician. From this analysis, results like mode, mean, median, p value, CI and so on ...
Authors reviewing qualitative research, similar to their quantitative counterparts, do not always use or optimize the use or value of their critical appraisals. Just as reviewers can undertake a sensitivity analysis on quantitative research, they can also apply the process to qualitative work (Carroll & Booth, Citation 2015). The purpose of a ...
A critical quantitative analysis of the effects of budgeting models in creating equity for high school students in a large urban school district. Doctoral dissertation, Howard University. ... (2015). Past, present, and future of critical quantitative research in higher education. New Directions for Institutional Research, 163, 103-112. https ...
This paper describes a broad framework of critical appraisal of published research literature that covers both quantitative and qualitative methodologies. The aim is the heart of a research study. It should be robust, concisely stated and specify a study factor, outcome factor(s) and reference population.
INTRODUCTION. Scientific research is usually initiated by posing evidenced-based research questions which are then explicitly restated as hypotheses.1,2 The hypotheses provide directions to guide the study, solutions, explanations, and expected results.3,4 Both research questions and hypotheses are essentially formulated based on conventional theories and real-world processes, which allow the ...
Quantitative research methods are concerned with the planning, design, and implementation of strategies to collect and analyze data. Descartes, the seventeenth-century philosopher, suggested that how the results are achieved is often more important than the results themselves, as the journey taken along the research path is a journey of discovery. . High-quality quantitative research is ...
This publication is designed to help provide practicing health educators with basic tools helpful to facilitate a better understanding of quantitative research. This article describes the major components—title, introduction, methods, analyses, results and discussion sections—of quantitative research. Readers will be introduced to ...
The first step in the critique process is for the reader to browse the abstract and article for an overview. During this initial review a great deal of information can be obtained. The abstract should provide a clear, concise overview of the study. During this review it should be noted if the title, problem statement, and research question (or ...
JBI's critical appraisal tools assist in assessing the trustworthiness, relevance and results of published papers. These tools have been revised. Recently published articles detail the revision. "Assessing the risk of bias of quantitative analytical studies: introducing the vision for critical appraisal within JBI systematic reviews".
Revised on June 22, 2023. Quantitative research is the process of collecting and analyzing numerical data. It can be used to find patterns and averages, make predictions, test causal relationships, and generalize results to wider populations. Quantitative research is the opposite of qualitative research, which involves collecting and analyzing ...
Critical appraisal is the course of action for watchfully and systematically examining research to assess its reliability, value and relevance in order to direct professionals in their vital clinical decision making [ 1 ]. Critical appraisal is essential to: Continuing Professional Development (CPD).
This handbook offers a contemporary and comprehensive review of critical research theory and methodology. Showcasing the work of contemporary critical researchers who are harnessing and building on a variety of methodological tools, this volume extends beyond qualitative methodology to also include critical quantitative and mixed-methods approaches to research.
Quantitative Research. Quantitative research is a type of research that collects and analyzes numerical data to test hypotheses and answer research questions.This research typically involves a large sample size and uses statistical analysis to make inferences about a population based on the data collected.
4. Critical Appraisal. Critical appraisal is a process which scientifically evaluate the strength and weakness of. a research paper for the application of theory, practice and education. While ...
Quantitative research in the social sciences is undergoing a change. After years of scholarship on the oppressive history of quantitative methods, quantitative scholars are grappling with the ways that our preferred methodology reinforces social injustices (Zuberi, 2001).Among others, the emerging fields of CritQuant (critical quantitative studies) and QuantCrit (quantitative critical race ...
There should be more simplified and pragmatic approach for analysis of randomized controlled trial. In this article, we like to summarize few of the practical points under 5 headings: "5 'Rs' of critical analysis of randomized control trial" which encompass Right Question, Right Population, Right Study Design, Right Data, and Right ...
Many methods for quantitative bias analysis exist, although only a few of these are regularly applied in practice. In this article, we will introduce three straightforward, commonly applied, and general approaches1: bias formulas, bounding methods, and probabilistic bias analysis.Alternative methods are also available, including methods for bias adjustment of linear regression with a ...
A critical analysis of the included studies revealed issues related to research design, varying from data collected from heterogeneous healthcare settings and diverse types of MMs to the type of analyses completed (e.g., qualitative, mixed methods), to the strength of conclusions drawn from a few studies' results (e.g., correlational, or causal).
This doctoral research delves into the water-energy-food nexus with a specific focus on India's sugar industry, which is of major importance to India, yet an underexplored sector from a nexus perspective. The thesis targets three distinct gaps in knowledge that are central to analysis of India's provision of water, energy, and food.