The human disease network
Affiliation.
- 1 Center for Complex Network Research and Department of Physics, University of Notre Dame, Notre Dame, IN 46556, USA.
- PMID: 17502601
- PMCID: PMC1885563
- DOI: 10.1073/pnas.0701361104
A network of disorders and disease genes linked by known disorder-gene associations offers a platform to explore in a single graph-theoretic framework all known phenotype and disease gene associations, indicating the common genetic origin of many diseases. Genes associated with similar disorders show both higher likelihood of physical interactions between their products and higher expression profiling similarity for their transcripts, supporting the existence of distinct disease-specific functional modules. We find that essential human genes are likely to encode hub proteins and are expressed widely in most tissues. This suggests that disease genes also would play a central role in the human interactome. In contrast, we find that the vast majority of disease genes are nonessential and show no tendency to encode hub proteins, and their expression pattern indicates that they are localized in the functional periphery of the network. A selection-based model explains the observed difference between essential and disease genes and also suggests that diseases caused by somatic mutations should not be peripheral, a prediction we confirm for cancer genes.
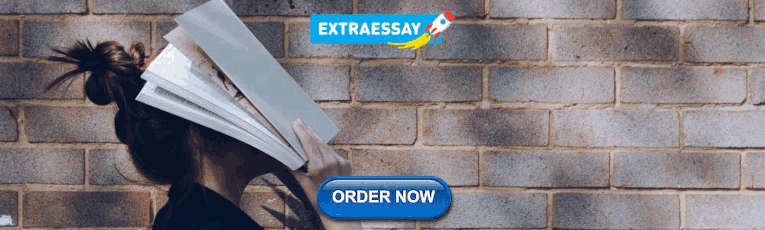
Publication types
- Research Support, N.I.H., Extramural
- Research Support, Non-U.S. Gov't
- Research Support, U.S. Gov't, Non-P.H.S.
- Computer Simulation
- Gene Expression Regulation
- Genetic Predisposition to Disease / genetics*
Grants and funding
- U56 CA113004/CA/NCI NIH HHS/United States
- IH U01 A1070499-01/PHS HHS/United States
- Search Menu
- Chemical Biology and Nucleic Acid Chemistry
- Computational Biology
- Critical Reviews and Perspectives
- Data Resources and Analyses
- Gene Regulation, Chromatin and Epigenetics
- Genome Integrity, Repair and Replication
- Methods Online
- Molecular Biology
- Nucleic Acid Enzymes
- RNA and RNA-protein complexes
- Structural Biology
- Synthetic Biology and Bioengineering
- Advance Articles
- Breakthrough Articles
- Special Collections
- Scope and Criteria for Consideration
- Author Guidelines
- Data Deposition Policy
- Database Issue Guidelines
- Web Server Issue Guidelines
- Submission Site
- About Nucleic Acids Research
- Editors & Editorial Board
- Information of Referees
- Self-Archiving Policy
- Dispatch Dates
- Advertising and Corporate Services
- Journals Career Network
- Journals on Oxford Academic
- Books on Oxford Academic
Article Contents
Introduction, network database improvement, web interface improvement, supplementary data.
- < Previous
HumanNet v2: human gene networks for disease research

The authors wish it to be known that, in their opinion, the first two authors should be regarded as Joint First Authors.
- Article contents
- Figures & tables
- Supplementary Data
Sohyun Hwang, Chan Yeong Kim, Sunmo Yang, Eiru Kim, Traver Hart, Edward M Marcotte, Insuk Lee, HumanNet v2: human gene networks for disease research, Nucleic Acids Research , Volume 47, Issue D1, 08 January 2019, Pages D573–D580, https://doi.org/10.1093/nar/gky1126
- Permissions Icon Permissions
Human gene networks have proven useful in many aspects of disease research, with numerous network-based strategies developed for generating hypotheses about gene-disease-drug associations. The ability to predict and organize genes most relevant to a specific disease has proven especially important. We previously developed a human functional gene network, HumanNet, by integrating diverse types of omics data using Bayesian statistics framework and demonstrated its ability to retrieve disease genes. Here, we present HumanNet v2 ( http://www.inetbio.org/humannet ), a database of human gene networks, which was updated by incorporating new data types, extending data sources and improving network inference algorithms. HumanNet now comprises a hierarchy of human gene networks, allowing for more flexible incorporation of network information into studies. HumanNet performs well in ranking disease-linked gene sets with minimal literature-dependent biases. We observe that incorporating model organisms’ protein–protein interactions does not markedly improve disease gene predictions, suggesting that many of the disease gene associations are now captured directly in human-derived datasets. With an improved interactive user interface for disease network analysis, we expect HumanNet will be a useful resource for network medicine.
Human gene networks have been widely used to investigate genetic factors of diseases and therapeutic targets ( 1 ). Gene networks can also augment disease genomics information derived from expression profiles ( 2–4 ), whole exome sequencing ( 5 , 6 ) and genome-wide association studies (GWAS) ( 7 , 8 ) for the discovery of disease-associated genes. Edges of the gene networks may represent diverse types of associations between genes which can be mapped by both experimental and computational methods. Because appropriately integrating interaction information from diverse sources can improve the breadth and accuracy of a network, many integrated human gene networks have been developed and a variety of topological analysis algorithms have been applied to generate new hypotheses about gene-disease-drug associations.
We previously developed an integrated human functional gene network, HumanNet, and demonstrated its capability of disease gene predictions ( 9 ). In order to construct the network, we inferred functional associations between human genes from protein–protein interactions (PPI), co-citation of human genes across PubMed abstracts, co-occurrence of protein domains, co-expression of genes across samples and genomic context associations. In addition, interactions between evolutionarily conserved proteins of model organisms were transferred to the human gene network. Those networks, inferred from different types of data, were evaluated and integrated using a Bayesian statistical framework. Since the first release of HumanNet, the amount of publicly available omics data has increased substantially and network inference algorithms have also improved significantly, and thus we expected that updating HumanNet could provide a greatly enhanced resource for network medicine.
In this report, we present HumanNet v2, which offers substantial performance improvements over v1, especially for the disease gene predictions. A new feature of the updated HumanNet is a four level inclusive hierarchy of the human gene networks: the first level has two networks, HumanNet-PI comprising human-derived PPIs and HumanNet-CF based on co-functional links inferred from various types of genomics data; the integration of HumanNet-PI and HumanNet-CF produces the second level network HumanNet-FN which is an integrated functional gene network; the third level has two extended functional networks by either co-citation (HumanNet-XC) or interologs ( 10 ) from other species (HumanNet-XI); and the fourth level network is the fully extended network (HumanNet-XN) that contains all above functional links (Figure 1A ).

( A ) Overview of the four level hierarchy of human gene networks in the HumanNet database. ( B ) Assessment of the six human gene networks at different levels of the hierarchy, based on measuring the precision of identifying gene pairs linked to the same human diseases (defined by DisGeNET or GWAS catalog with timestamp filtration) as a function of the coverage of the database genes.
We benchmarked each of the networks for their ability to prioritize disease-linked gene sets with two different network-based algorithms. We observed HumanNet-XC and HumanNet-XN to have equally good or better performance than STRING v10.5 ( 11 ) and significantly better performance than other integrated human gene networks such as ConsensusPathDB (CPDB) ( 12 ), GIANT ( 7 ), GeneMANIA ( 13 ) and FunCoup ( 14 ). Time-stamped benchmarking strategy demonstrated that the improvements in performance of HumanNet extended beyond the incorporation of literature-based information. Interestingly, while we offer networks extended by IL for completeness, we observed no gains in disease gene prediction quality by their incorporation, suggesting that data measured directly in humans has reached a high level of predictive power for the disease gene network. Users can download edge information of various human gene networks and perform disease gene predictions and disease network analysis via a highly interactive user interface on the HumanNet web server ( www.inetbio.org/humannet ).
Four-level inclusive hierarchy of human gene networks
To provide flexibility in utilizing the network's information for various purposes, we designed HumanNet v2 with a four-level inclusive hierarchy of human gene networks comprising networks based on 10 distinct types of data (Figure 1A and Supplemental Table 1 ). The previous version of HumanNet was constructed based on only functional associations between genes, which can be supported by various types of biological data. The PPI assay was a traditional approach for mapping the functional associations between genes. Human gene networks based on only PPIs generally have a limited network coverage, because there are many functional associations that are not mediated by physical interactions between proteins. However, PPI networks have advantages in terms of the mechanistic interpretation of disease-associated mutations ( 15 ). Therefore, we decided to maintain a human gene network based on only PPIs separately as one of the first-level networks, HumanNet-PI, which contains 158 499 links among 15 352 genes, based on PPIs by high-throughput assays (HT) and literature-curated PPIs (LC).
In contrast to the PPI network, functional gene networks can be supported by diverse types of data ( 16 ), including PPIs. Despite lacking mechanistic information for the network links due to the broad edge definition, the typically high comprehensiveness of functional gene networks provides advantages in terms of generating functional hypotheses. We inferred co-functional associations between genes from six additional types of data: co-essentiality (CE) ( 17 ), co-expression (CX) ( 18 ), associations by pathway database (DB), associations between protein domain profiles (DP) ( 19 ), associations by gene neighborhood (GN) ( 20 ) and associations between phylogenetic profiles (PG) ( 21 ). Network inference methods for each type of data are described in the Supplemental Methods . We integrated the six co-functional gene networks to generate another first-level network based on only inferred co-functional links from omics data, HumanNet-CF that contains 14 739 genes and 252 590 links. Integration of these two first-level networks produces the second-level network HumanNet-FN, an integrated functional gene network that contains 17 247 genes and 371 502 links.
Two networks at third-level were constructed based on the extended information of the functional associations by either co-citations (CC) across approximately 300 000 full-text articles of PubMed Central (HumanNet-XC) or interologs (IL) transferred from nine other species (HumanNet-XI). Co-citation made a significant contribution to the mapping of functional associations for several human gene networks, including HumanNet and STRING. However, the functional network by co-citation may cause biased benchmarking performance for disease gene discovery, because benchmarking data are also based on the literature. Some users may want to exclude the influence of co-citation during disease gene predictions. Therefore, we decided to maintain a human gene network extended by co-citation data separately. HumanNet-XC contains 17 790 genes and 424 501 links. In contrast to the HumanNet-XC, which contains only human-derived functional networks, HumanNet-XI includes interologs derived from five laboratory model organisms ( Caenorhabditis elegans, Drosophila melanogaster, Danio rerio, Mus musculus and Saccharomyces cerevisiae ) and four additional vertebrates: Canis lupus familiaris (dog), Bos taurus (cattle), Rattus norvegicus (Rat) and Gallus gallus (chicken). HumanNet-XI contains 17 303 genes and 418 525 links.
The fourth level network, HumanNet-XN, is a fully extended functional gene network by both co-citation and interologs. Interologs derived from non-human species provided 101 036 more links to HumanNet-XC, yet its genome coverage only increased from 94.6 to 95.3%. The most comprehensive network, HumanNet-XN, contains 17 929 genes and 525 537 links.
New types of data used for HumanNet v2
We incorporated functional associations inferred from two new types of data to the updated version of HumanNet. We inferred functional associations from co-annotations by pathway database. If a gene is involved in many different pathways, it may not belong to a specific pathway. Similarly, co-annotation involving such genes would be only weak indication of functional coupling. Thus, we measured the significance of functional association for given co-annotations by Fisher’s exact test, giving more weight on gene pairs that share larger proportion of annotated pathways for each gene. We used pathway annotations by KEGG ( 22 ), BioCarta ( 23 ) and Recactome ( 24 ) databases. Network inference from pathway databases resulted in 125 550 links among 7512 human genes.
Another new type of data used for updating HumanNet was co-essentiality. Recently, several large-scale essential gene screens were conducted across hundreds of cancer cell lines using the shRNA and CRISPR-Cas9 systems. Functionally associated human genes tend to have correlations of essentiality profiles across many cancer cell lines ( 17 ). We obtained the functional links inferred from associations between essentiality profiles based on over 100 genome-scale pooled-library shRNA screens and over 400 CRISPR-Cas9 screens from cancer cell lines, which are downloadable from the PICKLES database ( 25 ). Network inference from co-essentiality resulted in 71 243 links among 4052 human genes.
Data source extensions
To improve HumanNet, we also extended the sources of each data type (summarized in Supplemental Table 1 ). The co-citation network of HumanNet v2 is based on ∼300 000 full-text articles from PubMed Central, whereas ∼750 000 Medline abstracts were used for the co-citation network of the previous version of HumanNet. Sources of PPI data were also substantially extended. The number of database and high-throughput assay sets ( Supplemental Table 2 ) used for human-derived PPI networks increased from 5 to 14 and 3 to 12, respectively. As a result, the number of non-redundant PPIs of HumanNet v2 is 158 499 (connecting 15 352 genes), whereas the PPI network of HumanNet v1 has 60 287 links among 9428 genes. Given that PPIs generally provide high-quality functional associations, this substantially expanded PPI network will significantly improve the generation of functional hypotheses. To update the co-expression networks, we used 125 microarray-based and 33 RNA-seq-based gene expression omnibus (GEO) ( 26 ) series (GSEs) (16 220 samples in total) ( Supplemental Table 3 ), whereas only 21 microarray-based GSEs (1603 samples in total) were used in the previous version. Thus, the amount of expression profile data for co-expression analysis has been increased by more than 10-fold. HumanNet includes networks based on genomic context associations (GN and PG). We utilized 1748 prokaryotic (1626 bacterial and 122 archaeal) genomes and 996 metagenomes (754 from human and 242 from ocean) ( 27 , 28 ) to analyze the genomic context associations for HumanNet v2, whereas only 432 prokaryotic (393 bacterial and 31 archaeal) genomes were used for HumanNet v1.
Network inference algorithm enhancement
Since the release of the first version of HumanNet, we have significantly improved the network inference algorithms for each data type. We found that associations between the phylogenetic profiles of proteins showed a higher correlation with functional association within each domain of life: Archaea, Bacteria, and Eukaryota ( 29 ). Thus, for HumanNet v2, we measured the associations between phylogenetic profiles that comprise reference genomes from each domain of life, then integrated the networks based on domain-specific profiles into a single network (PG).
For the previous version of HumanNet, we inferred functional associations by gene neighborhood using only probability-based measures ( 30 ). We later found that probability-based and distance-based measures ( 31 ) of gene neighborhood are complementary and that their integration could significantly improve network quality ( 20 ). Thus, we generated two functional networks using probability- and distance-based measures of gene neighborhood. We also found that distance-based gene neighborhoods across metagenomes correlated with functional associations ( 32 ). We could infer two functional networks by gene neighborhood analysis using 754 human microbiomes ( 27 ) and 242 ocean metagenomes ( 28 ). The final gene neighborhood network (GN) was constructed by integrating the four networks.
The human gene network based on protein domain profiles for HumanNet v2 was improved by using a weighted mutual information (WMI) score that measured the mutual information (MI) between domain profiles of proteins by giving a higher weight to rarer protein domains ( 19 ).
Systematic network evaluation for disease gene discovery
Recently, a systematic network evaluation for their ability to retrieve disease gene sets was conducted for 21 human gene networks, including the previous version of HumanNet ( 33 ). The study reported that CPDB ( 12 ), GeneMania ( 13 ), GIANT ( 7 ) and STRING ( 11 ) had the best performance in terms of retrieval of literature-curated disease gene sets by DisGeNET ( 34 ) and sets of disease candidate genes mapped by P < 5e-08 from the GWAS catalog ( 35 ). To confirm these results and to evaluate the new human gene networks of HumanNet v2, we evaluated the four best performed gene networks reported by the aforementioned study, another large-scale human functional network, FunCoup ( 14 ), and HumanNet v2 for disease gene predictions. Importantly, we used ‘time-stamped benchmarking’ strategy ( 36 ) to avoid biased evaluation by co-citation links of HumanNet and STRING. Co-citation links of HumanNet v2 were captured from papers published until 2015. Thus, we used disease-associated genes identified via GWAS published only after 2016 for each trait of the GWAS catalog. With this timestamp filtration, we could obtain 231 traits that contain more than 10 genes mapped by P < 5e-08 from the GWAS catalog. Since the latest version of STRING was published in 2016, we expected that the same gene sets could be used for unbiased evaluation of STRING.
We first assessed network accuracy for identifying two genes involved in the same human diseases. We found that two first level networks, HumanNet-PI and HumanNet-CF, to have the worse accuracy than the integrated functional network, HumanNet-FN in terms of connecting gene pairs linked to the same diseases annotated by DisGeNET or GWAS catalog with timestamp filtration as a function of the coverage of the database genes (Figure 1B ). This result is consistent with the observation that all of the best performing human gene networks reported by the aforementioned study were functional networks rather than PPI networks ( 33 ). We found HumanNet-XC to have the best performance in identifying gene pairs for the same diseases. Notably, incorporating interologs into HumanNet-FN and HumanNet-XC did not notably improve network precision compared with HumanNet-XI and HumanNet-XN, respectively. To evaluate contribution of each evidence to the integrated gene network, accuracy and genome coverage of networks by each data type were also assessed based on the same disease annotations ( Supplemental Figure 1 ).
Next, we compared the best performing HumanNet-XC with the previous HumanNet (v1) as well as five other human gene networks, and found that HumanNet-XC outperformed all the other human gene networks (Figure 2A ). In addition, we observed that HumanNet-PI has overall higher accuracy than another scored human PPI network, InWeb (Figure 2B ). These results indicate that HumanNet v2 might provide the most appropriate networks for disease research by utilizing protein physical interactions as well as functional associations.
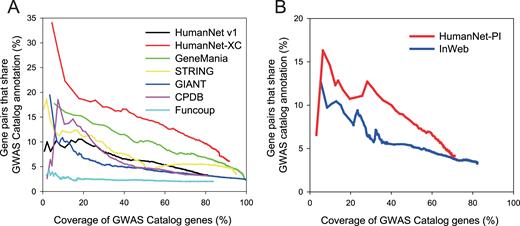
Assessment of human functional gene networks ( A ) and PPI networks ( B ) for genes linked to the same human diseases (defined by GWAS catalog with timestamp filtration) as a function of the coverage of the database genes.
Next, we evaluated the networks for their ability to retrieve disease gene sets. The network performance for disease gene recovery correlates with the efficiency of disease gene discovery by network-based gene prioritization. Network-based gene prioritization for diseases can use two alternative strategies: direct neighborhood and network diffusion ( 37 ). Direct neighborhood methods prioritize genes using the disease information of their directly connected network neighbors only ( 38 , 39 ). In contrast, network diffusion methods prioritize genes by propagating disease information throughout the entire network ( 40 ). Recently, network diffusion methods have increased in popularity, and the web server of the previous HumanNet version also employed network diffusion for disease gene prioritization. However, more recently, multiple studies have shown that direct neighborhood is generally more efficient than network diffusion in obtaining disease genes in the top predictions ( 41 , 42 ). Because typically only a few hundred candidates at most are considered for the follow-up functional analysis, we benchmarked the retrieval efficiency of disease genes by the area under the receiver operating characteristic curve (AUROC) until a false positive rate of 1% (FPR < 0.01). With this benchmarking analysis, we found HumanNet-XC and HumanNet-XN to have significantly better performance than all other networks by direct neighborhood with the unbiased disease gene sets (Figure 3A ). We observed similar results for AUROC until FPR of 2% and 5% ( Supplemental Figure 2 ). In consistent with the results of previous systematic evaluation, HumanNet v1 showed worse performance than STRING, GeneMania, and GIANT with the time-stamped benchmarking, indicating large influence of co-citation information on the earlier version of HumanNet ( 33 ).
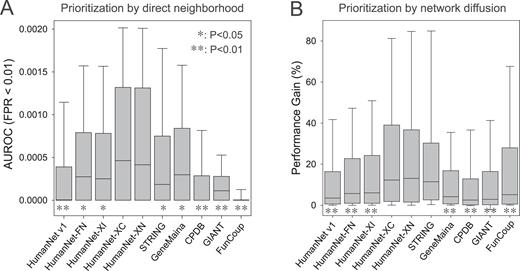
Assessment of predictive ability of networks for unbiased GWAS catalog disease gene sets based on the distribution of ( A ) the area under receiver operating characteristic curve (AUROC) until 1% of false positive rate (FPR < 0.01) and ( B ) performance gain scores based on area under precision recall curve (AUPRC). For each box-and-whisker plot, the boundaries of the box represent the first and third quartiles and the whiskers represent the 10th and 90th percentiles. Significance of performance difference from that of HumanNet-XC is indicated by asterisk (*: P < 0.05, **: P < 0.01, Wilcoxon rank sum test).
It is also possible to prioritize disease genes with network diffusion techniques such as random walk with the restart model ( 40 ). For benchmarking the retrieval efficiency of disease gene sets by network diffusion, we used ‘performance gain’ scores based on the area under the precision recall curve (AUPRC) as described in a previous study on systematic network evaluations ( 33 ). With this benchmarking analysis, we found HumanNet-XC, HumanNet-XN, and STRING to have significantly better performance than other networks (Figure 3B ).
Notably, in all of the above benchmarking analysis, we did not observe a significant increase in performance by incorporating interologs ( P > 0.05 for HumanNet-FN versus HumanNet-XI and for HumanNet-XC versus HumanNet-XN, Wilcoxon rank sum test). These results suggest that many of the evolutionarily conserved gene links for the same diseases are now captured directly in human-derived data. However, we cannot exclude the possibility that intrologs can improve gene prioritization for non-pathogenic cellular processes such as core metabolic pathways.
Implementation of a new user interface
We implemented back-end and front-end servers for HumanNet v2 to facilitate effective interactions with users. For the back-end server implementation, we used Redis ( https://redis.io ), an in-memory DB which reduces the data loading time significantly compared with that from a hard drive. We designed the back-end interface as an Application Programming Interface (API) to communicate with the front-end server and also job requests from users. We employed several open-sourced Cascading Style Sheet components and JavaScript libraries for front-end server implementation. We designed the website layout using Bootstrap4 and its components ( https://getbootstrap.com ). Cytoscape.js ( 43 ) and its extensions, ‘cytoscape.js-cose-bilkent’ (from https://doi.org/10.5281/zenodo.1098231 ) and ‘cytoscape.js-panzoom’ (from http://doi.org/10.5281/zenodo.835037 ) were employed to provide the graph and network visualization.
Disease-focused hypothesis generation
The HumanNet v2 web server facilitates human disease research by predicting disease genes or disease annotations. Network-based disease gene predictions are generally based on the network connections to the genes known to be involved in the disease. We dubbed these known disease genes ‘guide genes’ because they guide the network-based predictions of new disease gene candidates. We can estimate the predictive performance of networks based on the efficiency of guide gene recovery. The HumanNet v2 server uses a direct neighborhood approach rather than network diffusion for network-based gene prioritization, because at most a few hundred candidates are considered for follow-up functional analysis and direct neighborhood generally outperforms network diffusion methods for the early retrieval of guide genes ( 41 , 42 ). The HumanNet v2 server uses HumanNet-XC as a default network, because it showed the best performance for disease gene recovery in our benchmarking analyses.
Using multiple guide genes for network-based predictions is desirable, because predictions based on multiple network connections are more confident due to the ensemble effect. The functional coherence of guide genes would be a meaningful indicator of their effectiveness. Therefore, the HumanNet server reports on the significance of within-group connectivity of guide genes using 10 000 random gene sets of the same size (Figure 4 , lower panel). The HumanNet server also reports on ROC plots, which indicate the predictive performance of networks for a disease based on the efficiency of guide gene recovery. To evaluate statistical significance of the observed AUROC score, HumanNet v2 server generates null models using 10 000 random gene sets of the same size. Users can submit pre-defined disease gene sets from DisGeNET ( 34 ), DISEASES ( 44 ), Disease Ontology Annotation Framework (DOAF) ( 45 ), GWAS catalog ( 35 ) and Human Phenotype Ontology (HPO) ( 46 ). Users can also submit a set of genes targeted by a drug based on DiSigDB ( 47 ). Thus, predictions guided by the DiSigDB gene set are likely candidates of novel targets for the same drug. HumanNet users can also predict disease annotations of a gene based on the network-neighbors. For a query gene, the HumanNet server collects disease annotations from its network neighbors and lists them starting from the most enriched one.
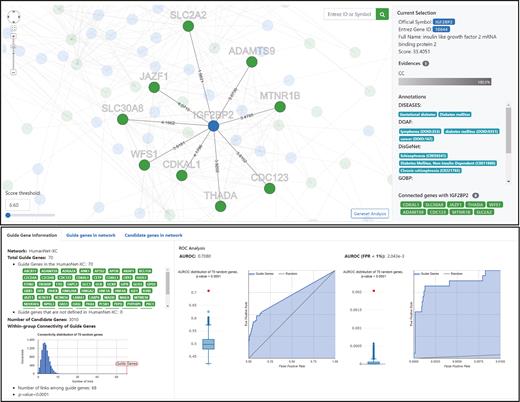
Screenshots of the HumanNet reports page for the network-based disease gene prediction using HumanNet-XC based on submission of 70 genes for type 2 diabetes mellitus (defined by DISEASES) as guide (query) genes. The upper panel shows the interactive network viewer, visualizing a network of guide genes (green nodes) and their top 100 direct neighbors, which can be interpreted as putative candidate genes (blue nodes). Here, the local subnetwork of the third ranked candidate, IGF2BP2 and its neighbors is highlighted. The retrieved gene IGF2BP2 is already annotated for diabetes mellitus by DISEASES, DOAF and DisGeNET, serving to validate the specific prediction result. The lower panel reports data on the guide genes, including the statistical significance of within group connectivity of guide genes, and the observed network performance for guide gene recovery reported as ROC curves.
Interactive network viewer
Network-based disease gene prediction generates a network of guide genes and new candidate genes for disease. Further investigation of the disease gene network would provide functional insights which might be useful for narrowing down final candidates and for mode-of-action studies. Therefore, we designed a network viewer enabling users to conduct interactive analyses of the disease gene network. The HumanNet v2 server generates a network of guide genes and the top 100 candidate genes for the disease. Initially, the entire network appears in the viewer to give a brief idea of the disease gene network, but soon after, all the candidate genes disappear. Then, users can select different numbers of top candidate genes for a new disease network by thresholding the prediction score (Figure 4 , upper panel). Users can select a particular gene of the disease network not only from the network viewer but also from the table of candidate genes. The network viewer highlights a local subnetwork of the choice of gene and its network neighbors. Users can also see additional information such as annotations of the GO biological process and diseases for the chosen gene and supporting evidence for the local network connections. The interactive thresholding for candidate gene selection allows users to consider various disease gene networks with different trade-offs between degree of confidence and coverage. Disease-association for the selected group of candidate genes can be summarized by gene-set analysis (GSA). Users can select the top N candidate genes and run GSA with not only GO biological processes but also annotated disease genes from DisGeNET ( 34 ), DISEASES ( 44 ), DOAF ( 45 ) and HOP ( 46 ).
In this report, we present an updated HumanNet by incorporating new types of data, extending data sources and improving network inference algorithms. The new HumanNet was designed to have an inclusive, four level hierarchy of human gene networks. Based on our benchmarking results for their performance of disease gene recovery, we conclude that HumanNet serves as one of the better human gene networks for prioritizing disease-linked genes and reconstructing disease-relevant gene modules. We recommend HumanNet-XC for most network-based disease research, but other networks will be useful for other purposes. For example, HumanNet-PI is recommended for the mode-of-action studies of disease mutation, HumanNet-FN for more conservative predictions of disease genes and HumanNet-XN for studies requiring the most comprehensive networks. Due to the continuous growth of omics data repositories and the advent of new types of functional genomics data such as single cell transcriptome profiles, we might be able to keep improving HumanNet in the future. With a highly interactive web server for generating hypotheses, we expect HumanNet to be a highly useful in silico resource for the study of human diseases.
Supplementary Data are available at NAR Online.
National Research Foundation of Korea (NRF) grant funded by the Korean Government (MSIT) [NRF-2018M3C9A5064709, NRF-2018R1A5A2025079 to I.L., NRF-2018R1C1B5032617 to S.H.]; Brain Korea 21 (BK21) PLUS Program (to I.L.); NIH (to E.M.M.); NSF (to E.M.M.); Welch Foundation (F-1515) (to E.M.M.); CPRIT Grant [RR160032 to E.K., T.H.]; NIH Grants [R35GM130119, P30 CA016672 to T.H.]. Funding for open access charge: National Research Foundation of Korea.
Conflict of interest statement . None declared.
Barabasi A.L. , Gulbahce N. , Loscalzo J. Network medicine: a network-based approach to human disease . Nat. Rev. Genet. 2011 ; 12 : 56 – 68 .
Google Scholar
Jiang P. , Wang H. , Li W. , Zang C. , Li B. , Wong Y.J. , Meyer C. , Liu J.S. , Aster J.C. , Liu X.S. Network analysis of gene essentiality in functional genomics experiments . Genome Biol. 2015 ; 16 : 239 .
Nitsch D. , Tranchevent L.C. , Thienpont B. , Thorrez L. , Van Esch H. , Devriendt K. , Moreau Y. Network analysis of differential expression for the identification of disease-causing genes . PLoS One . 2009 ; 4 : e5526 .
Gwinner F. , Boulday G. , Vandiedonck C. , Arnould M. , Cardoso C. , Nikolayeva I. , Guitart-Pla O. , Denis C.V. , Christophe O.D. , Beghain J. et al. Network-based analysis of omics data: the LEAN method . Bioinformatics . 2017 ; 33 : 701 – 709 .
Cho A. , Shim J.E. , Kim E. , Supek F. , Lehner B. , Lee I. MUFFINN: cancer gene discovery via network analysis of somatic mutation data . Genome Biol. 2016 ; 17 : 129 .
Horn H. , Lawrence M.S. , Chouinard C.R. , Shrestha Y. , Hu J.X. , Worstell E. , Shea E. , Ilic N. , Kim E. , Kamburov A. et al. NetSig: network-based discovery from cancer genomes . Nat. Methods . 2018 ; 15 : 61 – 66 .
Greene C.S. , Krishnan A. , Wong A.K. , Ricciotti E. , Zelaya R.A. , Himmelstein D.S. , Zhang R. , Hartmann B.M. , Zaslavsky E. , Sealfon S.C. et al. Understanding multicellular function and disease with human tissue-specific networks . Nat. Genet. 2015 ; 47 : 569 – 576 .
Shim J.E. , Bang C. , Yang S. , Lee T. , Hwang S. , Kim C.Y. , Singh-Blom U.M. , Marcotte E.M. , Lee I. GWAB: a web server for the network-based boosting of human genome-wide association data . Nucleic Acids Res. 2017 ; 45 : W154 – W161 .
Lee I. , Blom U.M. , Wang P.I. , Shim J.E. , Marcotte E.M. Prioritizing candidate disease genes by network-based boosting of genome-wide association data . Genome Res. 2011 ; 21 : 1109 – 1121 .
Yu H. , Luscombe N.M. , Lu H.X. , Zhu X. , Xia Y. , Han J.D. , Bertin N. , Chung S. , Vidal M. , Gerstein M. Annotation transfer between genomes: protein-protein interologs and protein-DNA regulogs . Genome Res. 2004 ; 14 : 1107 – 1118 .
Szklarczyk D. , Morris J.H. , Cook H. , Kuhn M. , Wyder S. , Simonovic M. , Santos A. , Doncheva N.T. , Roth A. , Bork P. et al. The STRING database in 2017: quality-controlled protein-protein association networks, made broadly accessible . Nucleic Acids Res. 2017 ; 45 : D362 – D368 .
Herwig R. , Hardt C. , Lienhard M. , Kamburov A. Analyzing and interpreting genome data at the network level with ConsensusPathDB . Nat. Protoc. 2016 ; 11 : 1889 – 1907 .
Franz M. , Rodriguez H. , Lopes C. , Zuberi K. , Montojo J. , Bader G.D. , Morris Q. GeneMANIA update 2018 . Nucleic Acids Res. 2018 ; 46 : W60 – W64 .
Ogris C. , Guala D. , Sonnhammer E.L.L. FunCoup 4: new species, data, and visualization . Nucleic Acids Res. 2018 ; 46 : D601 – D607 .
Sahni N. , Yi S. , Taipale M. , Fuxman Bass J.I. , Coulombe-Huntington J. , Yang F. , Peng J. , Weile J. , Karras G.I. , Wang Y. et al. Widespread macromolecular interaction perturbations in human genetic disorders . Cell . 2015 ; 161 : 647 – 660 .
Shim J.E. , Lee T. , Lee I. From sequencing data to gene functions: co-functional network approaches . Anim. Cells Syst. 2017 ; 21 : 77 – 83 .
Wang T. , Yu H. , Hughes N.W. , Liu B. , Kendirli A. , Klein K. , Chen W.W. , Lander E.S. , Sabatini D.M. Gene essentiality profiling reveals gene networks and synthetic lethal interactions with oncogenic ras . Cell . 2017 ; 168 : 890 – 903 .
Yang S. , Kim C.Y. , Hwang S. , Kim E. , Kim H. , Shim H. , Lee I. COEXPEDIA: exploring biomedical hypotheses via co-expressions associated with medical subject headings (MeSH) . Nucleic Acids Res. 2017 ; 45 : D389 – D396 .
Shim J.E. , Lee I. Weighted mutual information analysis substantially improves domain-based functional network models . Bioinformatics . 2016 ; 32 : 2824 – 2830 .
Shin J. , Lee T. , Kim H. , Lee I. Complementarity between distance- and probability-based methods of gene neighbourhood identification for pathway reconstruction . Mol. Biosyst. 2014 ; 10 : 24 – 29 .
Shin J. , Lee I. Construction of functional gene networks using phylogenetic profiles . Methods Mol. Biol. 2017 ; 1526 : 87 – 98 .
Kanehisa M. , Furumichi M. , Tanabe M. , Sato Y. , Morishima K. KEGG: new perspectives on genomes, pathways, diseases and drugs . Nucleic Acids Res. 2017 ; 45 : D353 – D361 .
Nishimura D. BioCarta . Biotech Softw. Int. Rep. 2001 ; 2 : 117 – 120 .
Fabregat A. , Jupe S. , Matthews L. , Sidiropoulos K. , Gillespie M. , Garapati P. , Haw R. , Jassal B. , Korninger F. , May B. et al. The reactome pathway knowledgebase . Nucleic Acids Res. 2018 ; 46 : D649 – D655 .
Lenoir W.F. , Lim T.L. , Hart T. PICKLES: the database of pooled in-vitro CRISPR knockout library essentiality screens . Nucleic Acids Res. 2018 ; 46 : D776 – D780 .
Barrett T. , Wilhite S.E. , Ledoux P. , Evangelista C. , Kim I.F. , Tomashevsky M. , Marshall K.A. , Phillippy K.H. , Sherman P.M. , Holko M. et al. NCBI GEO: archive for functional genomics data sets–update . Nucleic Acids Res. 2013 ; 41 : D991 – D995 .
Human Microbiome Project Consortium Structure, function and diversity of the healthy human microbiome . Nature . 2012 ; 486 : 207 – 214 .
Sunagawa S. , Coelho L.P. , Chaffron S. , Kultima J.R. , Labadie K. , Salazar G. , Djahanschiri B. , Zeller G. , Mende D.R. , Alberti A. et al. Ocean plankton. Structure and function of the global ocean microbiome . Science . 2015 ; 348 : 1261359 .
Shin J. , Lee I. Co-Inheritance analysis within the domains of life substantially improves network inference by phylogenetic profiling . PLoS One . 2015 ; 10 : e0139006 .
Bowers P.M. , Pellegrini M. , Thompson M.J. , Fierro J. , Yeates T.O. , Eisenberg D. Prolinks: a database of protein functional linkages derived from coevolution . Genome Biol. 2004 ; 5 : R35 .
Korbel J.O. , Jensen L.J. , von Mering C. , Bork P. Analysis of genomic context: prediction of functional associations from conserved bidirectionally transcribed gene pairs . Nat. Biotechnol. 2004 ; 22 : 911 – 917 .
Kim C.Y. , Lee I. Functional gene networks based on the gene neighborhood in metagenomes . Anim. Cells Syst. 2017 ; 21 : 301 – 306 .
Huang J.K. , Carlin D.E. , Yu M.K. , Zhang W. , Kreisberg J.F. , Tamayo P. , Ideker T. Systematic evaluation of molecular networks for discovery of disease genes . Cell Syst. 2018 ; 6 : 484 – 495 .
Pinero J. , Bravo A. , Queralt-Rosinach N. , Gutierrez-Sacristan A. , Deu-Pons J. , Centeno E. , Garcia-Garcia J. , Sanz F. , Furlong L.I. DisGeNET: a comprehensive platform integrating information on human disease-associated genes and variants . Nucleic Acids Res. 2017 ; 45 : D833 – D839 .
MacArthur J. , Bowler E. , Cerezo M. , Gil L. , Hall P. , Hastings E. , Junkins H. , McMahon A. , Milano A. , Morales J. et al. The new NHGRI-EBI Catalog of published genome-wide association studies (GWAS Catalog) . Nucleic Acids Res. 2017 ; 45 : D896 – D901 .
Bornigen D. , Tranchevent L.C. , Bonachela-Capdevila F. , Devriendt K. , De Moor B. , De Causmaecker P. , Moreau Y. An unbiased evaluation of gene prioritization tools . Bioinformatics . 2012 ; 28 : 3081 – 3088 .
Wang P.I. , Marcotte E.M. It's the machine that matters: Predicting gene function and phenotype from protein networks . J. Proteomics . 2010 ; 73 : 2277 – 2289 .
Guala D. , Sjolund E. , Sonnhammer E.L. MaxLink: network-based prioritization of genes tightly linked to a disease seed set . Bioinformatics . 2014 ; 30 : 2689 – 2690 .
Lee I. , Li Z. , Marcotte E.M. An improved, bias-reduced probabilistic functional gene network of baker's yeast, Saccharomyces cerevisiae . PLoS One . 2007 ; 2 : e988 .
Kohler S. , Bauer S. , Horn D. , Robinson P.N. Walking the interactome for prioritization of candidate disease genes . Am. J. Hum. Genet. 2008 ; 82 : 949 – 958 .
Shim J.E. , Hwang S. , Lee I. Pathway-dependent effectiveness of network algorithms for gene prioritization . PLoS One . 2015 ; 10 : e0130589 .
Guala D. , Sonnhammer E.L.L. A large-scale benchmark of gene prioritization methods . Sci. Rep. 2017 ; 7 : 46598 .
Franz M. , Lopes C.T. , Huck G. , Dong Y. , Sumer O. , Bader G.D. Cytoscape.js: a graph theory library for visualisation and analysis . Bioinformatics . 2016 ; 32 : 309 – 311 .
Pletscher-Frankild S. , Palleja A. , Tsafou K. , Binder J.X. , Jensen L.J. DISEASES: text mining and data integration of disease-gene associations . Methods . 2015 ; 74 : 83 – 89 .
Xu W. , Wang H. , Cheng W. , Fu D. , Xia T. , Kibbe W.A. , Lin S.M. A framework for annotating human genome in disease context . PLoS One . 2012 ; 7 : e49686 .
Kohler S. , Vasilevsky N.A. , Engelstad M. , Foster E. , McMurry J. , Ayme S. , Baynam G. , Bello S.M. , Boerkoel C.F. , Boycott K.M. et al. The human phenotype ontology in 2017 . Nucleic Acids Res. 2017 ; 45 : D865 – D876 .
Yoo M. , Shin J. , Kim J. , Ryall K.A. , Lee K. , Lee S. , Jeon M. , Kang J. , Tan A.C. DSigDB: drug signatures database for gene set analysis . Bioinformatics . 2015 ; 31 : 3069 – 3071 .
Author notes
Supplementary data, email alerts, citing articles via.
- Editorial Board
Affiliations
- Online ISSN 1362-4962
- Print ISSN 0305-1048
- Copyright © 2024 Oxford University Press
- About Oxford Academic
- Publish journals with us
- University press partners
- What we publish
- New features
- Open access
- Institutional account management
- Rights and permissions
- Get help with access
- Accessibility
- Advertising
- Media enquiries
- Oxford University Press
- Oxford Languages
- University of Oxford
Oxford University Press is a department of the University of Oxford. It furthers the University's objective of excellence in research, scholarship, and education by publishing worldwide
- Copyright © 2024 Oxford University Press
- Cookie settings
- Cookie policy
- Privacy policy
- Legal notice
This Feature Is Available To Subscribers Only
Sign In or Create an Account
This PDF is available to Subscribers Only
For full access to this pdf, sign in to an existing account, or purchase an annual subscription.
Europe PMC requires Javascript to function effectively.
Either your web browser doesn't support Javascript or it is currently turned off. In the latter case, please turn on Javascript support in your web browser and reload this page.
Search life-sciences literature (43,954,836 articles, preprints and more)
- Free full text
- Citations & impact
- Similar Articles
The human disease network.
Author information, affiliations, orcids linked to this article.
- Barabási AL | 0000-0002-4028-3522
Proceedings of the National Academy of Sciences of the United States of America , 14 May 2007 , 104(21): 8685-8690 https://doi.org/10.1073/pnas.0701361104 PMID: 17502601 PMCID: PMC1885563
Free full text in Europe PMC
Abstract
Free full text .

The human disease network
Kwang-il goh.
*Center for Complex Network Research and Department of Physics, University of Notre Dame, Notre Dame, IN 46556;
† Center for Cancer Systems Biology (CCSB) and
‡ Department of Genetics, Harvard Medical School, 77 Avenue Louis Pasteur, Boston, MA 02115;
§ Department of Physics, Korea University, Seoul 136-713, Korea; and
Michael E. Cusick
¶ Department of Cancer Biology, Dana–Farber Cancer Institute, 44 Binney Street, Boston, MA 02115;
David Valle
‖ Department of Pediatrics and the McKusick–Nathans Institute of Genetic Medicine, Johns Hopkins University School of Medicine, Baltimore, MD 21205
Barton Childs
Albert-lászló barabási.
Author contributions: D.V., B.C., M.V., and A.-L.B. designed research; K.-I.G. and M.E.C. performed research; K.-I.G. and M.E.C. analyzed data; and K.-I.G., M.E.C., D.V., M.V., and A.-L.B. wrote the paper.
- Associated Data
A network of disorders and disease genes linked by known disorder–gene associations offers a platform to explore in a single graph-theoretic framework all known phenotype and disease gene associations, indicating the common genetic origin of many diseases. Genes associated with similar disorders show both higher likelihood of physical interactions between their products and higher expression profiling similarity for their transcripts, supporting the existence of distinct disease-specific functional modules. We find that essential human genes are likely to encode hub proteins and are expressed widely in most tissues. This suggests that disease genes also would play a central role in the human interactome. In contrast, we find that the vast majority of disease genes are nonessential and show no tendency to encode hub proteins, and their expression pattern indicates that they are localized in the functional periphery of the network. A selection-based model explains the observed difference between essential and disease genes and also suggests that diseases caused by somatic mutations should not be peripheral, a prediction we confirm for cancer genes.
Decades-long efforts to map human disease loci, at first genetically and later physically ( 1 ), followed by recent positional cloning of many disease genes ( 2 ) and genome-wide association studies ( 3 ), have generated an impressive list of disorder–gene association pairs ( 4 , 5 ). In addition, recent efforts to map the protein–protein interactions in humans ( 6 , 7 ), together with efforts to curate an extensive map of human metabolism ( 8 ) and regulatory networks offer increasingly detailed maps of the relationships between different disease genes. Most of the successful studies building on these new approaches have focused, however, on a single disease, using network-based tools to gain a better understanding of the relationship between the genes implicated in a selected disorder ( 9 ).
Here we take a conceptually different approach, exploring whether human genetic disorders and the corresponding disease genes might be related to each other at a higher level of cellular and organismal organization. Support for the validity of this approach is provided by examples of genetic disorders that arise from mutations in more than a single gene (locus heterogeneity). For example, Zellweger syndrome is caused by mutations in any of at least 11 genes, all associated with peroxisome biogenesis ( 10 ). Similarly, there are many examples of different mutations in the same gene (allelic heterogeneity) giving rise to phenotypes currently classified as different disorders. For example, mutations in TP53 have been linked to 11 clinically distinguishable cancer-related disorders ( 11 ). Given the highly interlinked internal organization of the cell ( 12 – 17 ), it should be possible to improve the single gene–single disorder approach by developing a conceptual framework to link systematically all genetic disorders (the human “disease phenome”) with the complete list of disease genes (the “disease genome”), resulting in a global view of the “diseasome,” the combined set of all known disorder/disease gene associations.
Construction of the Diseasome.
We constructed a bipartite graph consisting of two disjoint sets of nodes. One set corresponds to all known genetic disorders, whereas the other set corresponds to all known disease genes in the human genome ( Fig. 1 ). A disorder and a gene are then connected by a link if mutations in that gene are implicated in that disorder. The list of disorders, disease genes, and associations between them was obtained from the Online Mendelian Inheritance in Man (OMIM; ref. 18 ), a compendium of human disease genes and phenotypes. As of December 2005, this list contained 1,284 disorders and 1,777 disease genes. OMIM initially focused on monogenic disorders but in recent years has expanded to include complex traits and the associated genetic mutations that confer susceptibility to these common disorders ( 18 ). Although this history introduces some biases, and the disease gene record is far from complete, OMIM represents the most complete and up-to-date repository of all known disease genes and the disorders they confer. We manually classified each disorder into one of 22 disorder classes based on the physiological system affected [see supporting information (SI) Text , SI Fig. 5, and SI Table 1 for details].

Construction of the diseasome bipartite network. ( Center ) A small subset of OMIM-based disorder–disease gene associations ( 18 ), where circles and rectangles correspond to disorders and disease genes, respectively. A link is placed between a disorder and a disease gene if mutations in that gene lead to the specific disorder. The size of a circle is proportional to the number of genes participating in the corresponding disorder, and the color corresponds to the disorder class to which the disease belongs. ( Left ) The HDN projection of the diseasome bipartite graph, in which two disorders are connected if there is a gene that is implicated in both. The width of a link is proportional to the number of genes that are implicated in both diseases. For example, three genes are implicated in both breast cancer and prostate cancer, resulting in a link of weight three between them. ( Right ) The DGN projection where two genes are connected if they are involved in the same disorder. The width of a link is proportional to the number of diseases with which the two genes are commonly associated. A full diseasome bipartite map is provided as SI Fig. 13 .
Starting from the diseasome bipartite graph we generated two biologically relevant network projections ( Fig. 1 ). In the “human disease network” (HDN) nodes represent disorders, and two disorders are connected to each other if they share at least one gene in which mutations are associated with both disorders ( Figs. 1 and and2 2 a ). In the “disease gene network” (DGN) nodes represent disease genes, and two genes are connected if they are associated with the same disorder ( Figs. 1 and and2 2 b ). Next, we discuss the potential of these networks to help us understand and represent in a single framework all known disease gene and phenotype associations.

The HDN and the DGN. ( a ) In the HDN, each node corresponds to a distinct disorder, colored based on the disorder class to which it belongs, the name of the 22 disorder classes being shown on the right. A link between disorders in the same disorder class is colored with the corresponding dimmer color and links connecting different disorder classes are gray. The size of each node is proportional to the number of genes participating in the corresponding disorder (see key), and the link thickness is proportional to the number of genes shared by the disorders it connects. We indicate the name of disorders with >10 associated genes, as well as those mentioned in the text. For a complete set of names, see SI Fig. 13 . ( b ) In the DGN, each node is a gene, with two genes being connected if they are implicated in the same disorder. The size of each node is proportional to the number of disorders in which the gene is implicated (see key). Nodes are light gray if the corresponding genes are associated with more than one disorder class. Genes associated with more than five disorders, and those mentioned in the text, are indicated with the gene symbol. Only nodes with at least one link are shown.
Properties of the HDN.
If each human disorder tends to have a distinct and unique genetic origin, then the HDN would be disconnected into many single nodes corresponding to specific disorders or grouped into small clusters of a few closely related disorders. In contrast, the obtained HDN displays many connections between both individual disorders and disorder classes ( Fig. 2 a ). Of 1,284 disorders, 867 have at least one link to other disorders, and 516 disorders form a giant component, suggesting that the genetic origins of most diseases, to some extent, are shared with other diseases. The number of genes associated with a disorder, s , has a broad distribution (see SI Fig. 6 a ), indicating that most disorders relate to a few disease genes, whereas a handful of phenotypes, such as deafness ( s = 41), leukemia ( s = 37), and colon cancer ( s = 34), relate to dozens of genes ( Fig. 2 a ). The degree ( k ) distribution of HDN ( SI Fig. 6 b ) indicates that most disorders are linked to only a few other disorders, whereas a few phenotypes such as colon cancer (linked to k = 50 other disorders) or breast cancer ( k = 30) represent hubs that are connected to a large number of distinct disorders. The prominence of cancer among the most connected disorders arises in part from the many clinically distinct cancer subtypes tightly connected with each other through common tumor repressor genes such as TP53 and PTEN .
Although the HDN layout was generated independently of any knowledge on disorder classes, the resulting network is naturally and visibly clustered according to major disorder classes. Yet, there are visible differences between different classes of disorders. Whereas the large cancer cluster is tightly interconnected due to the many genes associated with multiple types of cancer ( TP53 , KRAS , ERBB2 , NF1 , etc.) and includes several diseases with strong predisposition to cancer, such as Fanconi anemia and ataxia telangiectasia, metabolic disorders do not appear to form a single distinct cluster but are underrepresented in the giant component and overrepresented in the small connected components ( Fig. 2 a ). To quantify this difference, we measured the locus heterogeneity of each disorder class and the fraction of disorders that are connected to each other in the HDN (see SI Text ). We find that cancer and neurological disorders show high locus heterogeneity and also represent the most connected disease classes, in contrast with metabolic, skeletal, and multiple disorders that have low genetic heterogeneity and are the least connected ( SI Fig. 7 ).
Properties of the DGN.
In the DGN, two disease genes are connected if they are associated with the same disorder, providing a complementary, gene-centered view of the diseasome. Given that the links signify related phenotypic association between two genes, they represent a measure of their phenotypic relatedness, which could be used in future studies, in conjunction with protein–protein interactions ( 6 , 7 , 19 ), transcription factor-promoter interactions ( 20 ), and metabolic reactions ( 8 ), to discover novel genetic interactions. In the DGN, 1,377 of 1,777 disease genes are connected to other disease genes, and 903 genes belong to a giant component ( Fig. 2 b ). Whereas the number of genes involved in multiple diseases decreases rapidly ( SI Fig. 6 d ; light gray nodes in Fig. 2 b ), several disease genes (e.g., TP53 , PAX6 ) are involved in as many as 10 disorders, representing major hubs in the network.
Functional Clustering of HDN and DGN.
To probe how the topology of the HDN and GDN deviates from random, we randomly shuffled the associations between disorders and genes, while keeping the number of links per each disorder and disease gene in the bipartite network unchanged. Interestingly, the average size of the giant component of 10 4 randomized disease networks is 643 ± 16, significantly larger than 516 ( P < 10 −4 ; for details of statistical analyses of the results reported hereafter, see SI Text ), the actual size of the HDN ( SI Fig. 6 c ). Similarly, the average size of the giant component from randomized gene networks is 1,087 ± 20 genes, significantly larger than 903 ( P < 10 −4 ), the actual size of the DGN ( SI Fig. 6 e ). These differences suggest important pathophysiological clustering of disorders and disease genes. Indeed, in the actual networks disorders (genes) are more likely linked to disorders (genes) of the same disorder class. For example, in the HDN there are 812 links between disorders of the same class, an 8-fold enrichment with respect to 107 ± 10 links obtained between the same set of nodes in the randomized networks. This local functional clustering accounts for the small size of the giant components observed in the actual networks.
Disease-Associated Genes Identify Distinct Functional Modules.
For several disorders known to arise from mutations in any one of a few distinct genes, the corresponding protein products have been shown to participate in the same cellular pathway, molecular complex, or functional module ( 21 , 22 ). For example, Fanconi anemia arises from mutations in a set of genes encoding proteins involved in DNA repair, many of them forming a single heteromeric complex ( 23 ). Yet, the extent to which most disorders and disorder classes correspond to distinct functional modules in the cellular network has remained largely unclear. If genes linked by disorder associations encode proteins that interact in functionally distinguishable modules, then the proteins within such disease modules should more likely interact with one another than with other proteins. To test this hypothesis, we overlaid the DGN on a network of physical protein–protein interactions derived from high-quality systematic interactome mapping ( 6 , 7 ) and literature curation ( 6 ). We found that 290 interactions overlap between the two networks, a 10-fold increase relative to random expectation ( P < 10 −6 ; Fig. 3 a ).

Characterizing the disease modules. ( a ) Number of observed physical interactions between the products of genes within the same disorder (red arrow) and the distribution of the expected number of interactions for the random control (blue) ( P < 10 −6 ). ( b ) Distribution of the tissue-homogeneity of a disorder (red). Random control (blue) with the same number of genes chosen randomly is shown for comparison. ( c ) The distribution of PCC ρ ij values of the expression profiles of each disease gene pair that belongs to the same disorder (red) and the control (blue), representing the PCC distribution between all gene pairs ( P < 10 −6 ). ( d ) Distribution of the average PCC between expression profiles of all genes associated with the same disorder (red) is also shifted toward higher values than the random control (blue) with the same number of genes chosen randomly ( P < 10 −6 ).
Genes associated with the same disorder share common cellular and functional characteristics, as annotated in the Gene Ontology (GO) ( 24 ). If the HDN shows modular organization, then a group of genes associated with the same common disorder should share similar cellular and functional characteristics, as annotated in GO. To investigate the validity of this hypothesis, we measured the GO homogeneity of each disorder (see SI Text ) separately for each branch of GO, biological process, molecular function, and cellular component, finding significant elevation of GO homogeneity with respect to random controls in all three branches ( SI Fig. 8 ).
Disease genes encoding proteins that interact within common functional modules should tend to be expressed in the same tissue. To measure this, we introduced the tissue-homogeneity coefficient of a disorder, defined as the maximum fraction of genes among those belonging to a common disorder that are expressed in a specific tissue in a microarray data set obtained for 10,594 genes across 36 healthy tissues ( 25 ). We found that 68% of disorders exhibited almost perfect tissue-homogeneity ( Fig. 3 b ), compared with 51% expected by chance ( P < 10 −5 ).
Finally, disease genes that participate in a common functional module should also show high expression profiling correlation ( 26 ). The distribution of Pearson correlation coefficients (PCCs) for the coexpression profiles of pairs of genes associated with the same disorder was shifted toward higher values compared with that of a random control ( Fig. 3 c ; P < 10 −6 , χ 2 test). Similarly, the average PCC over all pairs of genes within a given disorder shows a significant shift from the random reference ( Fig. 3 d ), with a small but clearly distinguishable peak in the distribution around PCC ≈ 0.75. This peak corresponds to ≈33 disorders with average PCC > 0.6 for which all genes are highly coexpressed in most tissues, including Heinz body anemia (PCC = 0.935), Bethlem myopathy (PCC = 0.835), and spherocytosis (PCC = 0.656).
In summary, genes that contribute to a common disorder ( i ) show an increased tendency for their products to interact with each other through protein–protein interactions, ( ii ) have a tendency to be expressed together in specific tissues, ( iii ) tend to display high coexpression levels, ( iv ) exhibit synchronized expression as a group, and ( v ) tend to share GO terms. Together, these findings support the hypothesis of a global functional relatedness for disease genes and their products and offer a network-based model for the diseasome. Cellular networks are modular, consisting of groups of highly interconnected proteins responsible for specific cellular functions ( 21 , 22 ). A disorder then represents the perturbation or breakdown of a specific functional module caused by variation in one or more of the components producing recognizable developmental and/or physiological abnormalities.
This model offers a network-based explanation for the emergence of complex or polygenic disorders: a phenotype often correlates with the inability of a particular functional module to carry out its basic functions. For extended modules, many different combinations of perturbed genes could incapacitate the module, as a result of which mutations in different genes will appear to lead to the same phenotype. This correlation between disease and functional modules can also inform our understanding of cellular networks by helping us to identify which genes are involved in the same cellular function or network module ( 21 , 22 ).
Centrality and Peripherality.
An early indication of the connection between the structure of a cellular network and its functional properties was the finding that in Saccharomyces cerevisiae highly connected proteins or “hubs” are more likely encoded by essential genes ( 15 , 16 ). This prompted a number of recent studies ( 27 , 28 ) to formulate the hypothesis that human disease genes should also have a tendency to encode hubs. Yet, previous measurements found only a weak correlation between disease genes and hubs ( 29 ), resulting in an important mystery: what is the role, if any, of the cellular network in human diseases? Are disease genes more likely to encode hubs in the cellular network?
Our initial analysis appears to support the hypothesis that disease genes, given their impact on the organism, display a tendency to encode hubs in the interactome ( 27 , 28 ), finding that disease related proteins have a 32% larger number of interactions ( 6 , 7 ) with other proteins (average degree) than the nondisease proteins (see SI Fig 9 ) and that high-degree proteins are more likely to be encoded by genes associated with diseases than proteins with few interactions ( P = 1.6 × 10 −17 ; Fig. 4 a ). Next, we show, however, that despite this apparent correlation, the relationship between diseases and hubs hides deep differences between various disease genes.

When exploring whether disease genes encode hubs, we, and authors of other earlier studies ( 27 – 29 ), ignored the fact that some human genes are essential in early development and functional changes in these contribute to the high rate of first-trimester spontaneous abortions, which might be as much as 20% of recognized pregnancies. One strategy to explore the impact of this in utero essential segment of human disease is to consider human orthologs of mouse genes that result in embryonic or postnatal lethality when disrupted by homologous recombination (Mouse Genome Informatics; www.informatics.jax.org ). All together, we find 1,267 such mouse lethal orthologs of human genes, of which 398 are associated with human diseases, representing 22% of all known human disease genes. This allows us to distinguish between two classes of human genes: 1,267 “essential genes” and 1,379 “nonessential disease genes,” the latter obtained by removing from the full list of 1,777 OMIM disease genes the 398 that are also essential ( Fig. 4 b ). Next, we show that these two classes of genes play quite different roles in the human interactome.
First, we find that essential proteins show a tendency to be associated with hubs ( P = 1.3 × 10 −17 ; Fig. 4 c ), displaying a much stronger trend than the one observed for all disease proteins ( Fig. 4 a ). This raises an important question: Could the observed correlation between disease genes and hubs ( Fig. 4 a ) be the sole consequence of the fact that a small fraction (22%) of disease genes is also essential? To address this question we measured the degree dependence of the nonessential disease proteins ( Fig. 4 d ). Surprisingly, the correlation between hubs and disease proteins entirely disappears. Thus, the vast majority of disease genes (78%), those that are nonessential, do not show a tendency to encode hubs, indicating that the observed weak correlations between hubs and disease genes ( Fig. 4 a ) was entirely due to the few essential genes within the disease gene class.
Finally, we asked whether housekeeping genes, expressed in all tissues, have a tendency to encode disease genes. We find that the more tissues in which a gene is expressed, the higher the likelihood that it will be essential ( P = 2.8 × 10 −16 ; Fig. 4 g ). The opposite is true for nonessential disease genes: they have a tendency to be expressed in a few tissues ( P = 1.4 × 10 −6 ; Fig. 4 h ). Similarly, we found that only 9.9% of housekeeping genes correspond to disease genes, compared with 13.5% of nonhousekeeping genes, a significant 36% difference ( P = 3.6 × 10 −6 ). In contrast, 59.8% of housekeeping genes annotated with mouse phenotype were essential, compared with 40.5% for nonhousekeeping genes ( P < 10 −4 ).
These results support the somewhat unexpected conclusion that nonessential disease genes are not associated with hubs ( 27 , 28 ), show smaller correlation in their expression pattern with the rest of the genes in the cell than expected from random, and have a tendency to be expressed in only a few tissues. Therefore, contrary to earlier hypotheses and our expectations, the vast majority of nonessential disease genes occupy functionally peripheral and topologically neutral positions in the cellular network. In stark contrast, essential genes are likely to encode hubs, show highly synchronized expression with the rest of the genes, and are expressed in most tissues, being overrepresented among housekeeping genes. Thus, essential genes are topologically and functionally central.
This unexpected peripherality of most disease genes can be best explained by using an evolutionary argument. Mutations in topologically central, widely expressed genes are more likely to result in severe impairment of normal developmental and/or physiological function, leading to lethality in utero or early extrauterine life and to eventual deletion from the population. Only mutations compatible with survival into the reproductive years are likely to be maintained in a population. Therefore, disease-related mutations in the functionally and topologically peripheral regions of the cell give a higher chance of viability.
Disease genes whose mutations are somatic should not be subject to the selective pressure discussed above. Instead, somatic mutations that lead to severe disease phenotypes should more likely affect the functional center. To test the predictive power of this selection-based argument, we studied separately the properties of somatic cancer genes (Cancer Genome Census; www.sanger.ac.uk/genetics/CGP/Census ) and found that they ( i ) are more likely to encode hubs, ( ii ) show higher coexpression with the rest of the genes in the cell, and ( iii ) are more represented among housekeeping genes ( SI Fig. 10 ). The observed functional and topological centrality of somatic cancer genes fits well with our current understanding that many cancer genes play critical roles in cellular development and growth ( 11 ).
Throughout history, clinicians and medical researchers have focused on a few disorder(s) sharing commonalities in etiology or pathology. Recent progress in genetics and genomics has led to an appreciation of the effects of gene mutations in virtually all disorders and provides the opportunity to study human diseases all at once rather than one at a time ( 4 , 30 ). This unique approach offers the possibility of discerning general patterns and principles of human disease not readily apparent from the study of individual disorders.
An important tool in this quest is the HDN that represents a genome-wide roadmap for future studies on disease associations. The accompanying detailed diseasome map ( SI Fig. 13 ), showing all disorders and the genes associated with different disorders, offers a rapid visual reference of the genetic links between disorders and disease genes, a valuable global perspective for physicians, genetic counselors, and biomedical researchers alike.
To test whether the conclusions obtained in this work are robust to the incompleteness of the OMIM coverage, we expanded our study to include not only genes with identified mutations linked to the specific disease phenotype, but also those that satisfy the less stringent criterion that the phenotype has not been mapped to a specific locus ( 18 ). This expansion increased the number of disease-associated genes from 1,777 to 2,765, but also introduced noise in the data, because the link between many of the newly added genes and diseases is less stringent. Yet, the overall organization of the expanded diseasome map remains largely unaltered ( SI Fig. 11 ), and none of the trends uncovered in Fig. 4 are affected by this extension ( SI Fig. 12 ), supporting the robustness of our findings to further expansion of the OMIM database. Thus, although the maps shown in Fig. 2 and SI Fig. 13 will inevitably undergo local changes with the discovery of new disease genes, this will not change the overall organization and layout of the HDN significantly, because the HDN reflects the underlying cellular network-based relationship between genes and functional modules.
- Supplementary Material
- Acknowledgments
We thank Victor McKusick, Ada Hamosh, Joanna Amberger, and the rest of the OMIM team for their hard work and dedication and Tom Deisboeck, Zoltán Oltvai, Joanna Amberger, Todd Golub, Gerardo Jimenez-Sanchez and the members of the M.V. laboratory and the Center for Cancer Systems Biology, especially David E. Hill, for useful discussions. K.-I.G. and A.-L.B. were supported by National Institutes of Health (NIH) Grants IH U01 A1070499-01 and U56 CA113004 and National Science Foundation Grant ITR DMR-0926737 IIS-0513650. This work was supported by the Dana–Farber Cancer Institute (DFCI) Strategic Initiative (M.V.) and grants from the W. M. Keck Foundation (to M.V.) and the NIH/National Human Genome Research Institute and NIH/National Institute of General Medical Sciences (to M.V.).
- Abbreviations
The authors declare no conflict of interest.
This article is a PNAS Direct Submission.
This article contains supporting information online at www.pnas.org/cgi/content/full/0701361104/DC1 .
Full text links
Read article at publisher's site: https://doi.org/10.1073/pnas.0701361104
Citations & impact
Impact metrics, citations of article over time, alternative metrics.

Smart citations by scite.ai Smart citations by scite.ai include citation statements extracted from the full text of the citing article. The number of the statements may be higher than the number of citations provided by EuropePMC if one paper cites another multiple times or lower if scite has not yet processed some of the citing articles. Explore citation contexts and check if this article has been supported or disputed. https://scite.ai/reports/10.1073/pnas.0701361104
Article citations, drug repurposing for cancer therapy..
Xia Y , Sun M , Huang H , Jin WL
Signal Transduct Target Ther , 9(1):92, 19 Apr 2024
Cited by: 0 articles | PMID: 38637540 | PMCID: PMC11026526
Evaluation of network-guided random forest for disease gene discovery.
Hu J , Szymczak S
BioData Min , 17(1):10, 16 Apr 2024
Cited by: 0 articles | PMID: 38627770 | PMCID: PMC11020917
Quantifying the global film festival circuit: Networks, diversity, and public value creation.
Zemaityte V , Karjus A , Rohn U , Schich M , Ibrus I
PLoS One , 19(3):e0297404, 06 Mar 2024
Cited by: 0 articles | PMID: 38446758
Network topology mapping of chemical compounds space.
Tsekenis G , Cimini G , Kalafatis M , Giacometti A , Gili T , Caldarelli G
Sci Rep , 14(1):5266, 04 Mar 2024
Cited by: 0 articles | PMID: 38438443 | PMCID: PMC10912673
Uncovering genetic associations in the human diseasome using an endophenotype-augmented disease network.
Woerner J , Sriram V , Nam Y , Verma A , Kim D
Bioinformatics , 40(3):btae126, 01 Mar 2024
Cited by: 0 articles | PMID: 38527901
Other citations
Wikipedia (2).
- https://en.wikipedia.org/wiki/Metabolism
- https://en.wikipedia.org/wiki/Phenotypic_disease_network_(PDN)
Data behind the article
This data has been text mined from the article, or deposited into data resources.
BioStudies: supplemental material and supporting data
- http://www.ebi.ac.uk/biostudies/studies/S-EPMC1885563?xr=true
Similar Articles
To arrive at the top five similar articles we use a word-weighted algorithm to compare words from the Title and Abstract of each citation.
A network-based approach to identify disease-associated gene modules through integrating DNA methylation and gene expression.
Zhang Y , Zhang J , Liu Z , Liu Y , Tuo S
Biochem Biophys Res Commun , 465(3):437-442, 14 Aug 2015
Cited by: 5 articles | PMID: 26282201
A human phenome-interactome network of protein complexes implicated in genetic disorders.
Lage K , Karlberg EO , Størling ZM , Olason PI , Pedersen AG , Rigina O , Hinsby AM , Tümer Z , Pociot F , Tommerup N , Moreau Y , Brunak S
Nat Biotechnol , 25(3):309-316, 01 Mar 2007
Cited by: 550 articles | PMID: 17344885
Network properties of genes harboring inherited disease mutations.
Feldman I , Rzhetsky A , Vitkup D
Proc Natl Acad Sci U S A , 105(11):4323-4328, 07 Mar 2008
Cited by: 175 articles | PMID: 18326631 | PMCID: PMC2393821
[Posttraumatic stress disorder (PTSD) as a consequence of the interaction between an individual genetic susceptibility, a traumatogenic event and a social context].
Encephale , 38(5):373-380, 24 Jan 2012
Cited by: 36 articles | PMID: 23062450
The essentiality of drug targets: an analysis of current literature and genomic databases.
Ji X , Rajpal DK , Freudenberg JM
Drug Discov Today , 24(2):544-550, 13 Nov 2018
Cited by: 1 article | PMID: 30439449
Funding
Funders who supported this work.
NCI NIH HHS (1)
Grant ID: U56 CA113004
55 publication s
PHS HHS (1)
Grant ID: IH U01 A1070499-01
1 publication
Europe PMC is part of the ELIXIR infrastructure
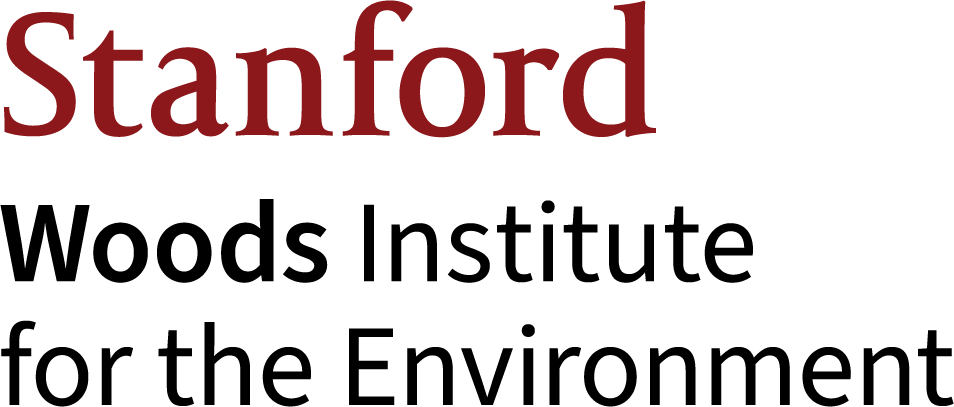
Planet versus Plastics
Plastic waste has infiltrated every corner of our planet, from oceans and waterways to the food chain and even our bodies. Only 9% of plastic is recycled due to factors including poor infrastructure, technical challenges, lack of incentives, and low market demand.
“We need legislation that disincentivizes big oil from producing plastic in the first place, coupled with enforced single use plastic taxes and fines,” says Desiree LaBeaud , professor of pediatric infectious diseases and senior fellow at Stanford Woods Institute for the Environment . “We also need truly compostable alternatives that maintain the convenient lifestyle that plastic allows us now."
Plastic presents a problem like no other. Stanford scholars are approaching it from many angles: exploring the connection between plastic and disease, rethinking how plastic could be reused, and uncovering new ways of breaking down waste. In honor of Earth Day and this year’s theme – Planet vs. Plastics – we’ve highlighted stories about promising solutions to the plastics challenge.
Environmental changes are altering the risk for mosquito-borne diseases

Our changing climate is dramatically altering the landscape for mosquito-borne diseases, but other changes to the physical environment - like the proliferation of plastic trash - also make an impact, as mosquitos can breed in the plastic waste we discard.
Since this study published, HERI-Kenya , a nonprofit started by Stanford infectious disease physician Desiree LaBeaud , has launched HERI Hub , a brick and mortar education hub that educates, empowers and inspires community members to improve the local environment to promote health.
Using plastic waste to build roads, buildings, and more

Stanford engineers Michael Lepech and Zhiye Li have a unique vision of the future: buildings and roads made from plastic waste. In this story, they discuss obstacles, opportunities, and other aspects of transforming or upcycling plastic waste into valuable materials.
Since this white paper was published, students in Lepech's life cycle assessment course have explored the environmental and economic impacts of waste management, emissions, and energy efficiency of building materials for the San Francisco Museum of Modern Arts. In addition to recycled plastic, they proposed a photovoltaic system and conducted comparison studies to maximize the system’s life cycle. This work is being translated into an upcoming publication.
Stanford researchers show that mealworms can safely consume toxic additive-containing plastic

Mealworms are not only able to eat various forms of plastic, as previous research has shown, they can also consume potentially toxic plastic additives in polystyrene with no ill effects. The worms can then be used as a safe, protein-rich feed supplement.
Since this study published, it has inspired students across the world to learn about and experiment with mealworms and plastic waste. Stanford researchers involved with this and related studies have been inundated with requests for more information and guidance from people inspired by the potential solution.
Grants tackle the plastics problem
Stanford Woods Institute has awarded more than $23 million in funding to research projects that seek to identify solutions to pressing environment and sustainability challenges, including new approaches to plastic waste management.
Converting polyethylene into palm oil

This project is developing a new technology to convert polyethylene — by far the most discarded plastic — into palm oil. The approach could add value to the plastic waste management chain while sourcing palm oil through a less destructive route.
Improving plastic waste management

This project aims to radically change the way plastic waste is processed via a new biotechnology paradigm: engineering highly active enzymes and microbes capable of breaking down polyesters in a decentralized network of “living” waste receptacles.
More stories from Stanford
Eight simple but meaningful things you can do for the environment.

A new, artistic perspective on plastic waste

Whales eat colossal amounts of microplastics

Event | Pollution and Health

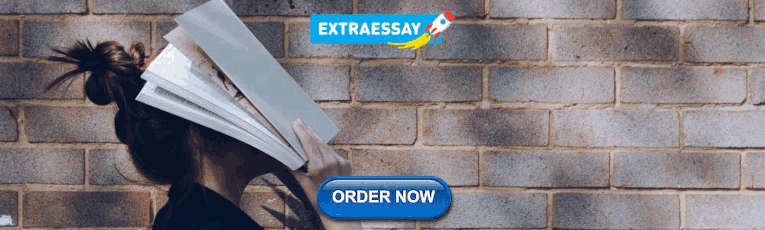
A greener future begins with small steps

Mosquito diseases on the move

Last straw: The path to reducing plastic pollution

Plastic ingestion by fish a growing problem

Stanford infectious disease expert Desiree LaBeaud talks trash, literally, on Stanford Engineering's The Future of Everything podcast.
Thank you for visiting nature.com. You are using a browser version with limited support for CSS. To obtain the best experience, we recommend you use a more up to date browser (or turn off compatibility mode in Internet Explorer). In the meantime, to ensure continued support, we are displaying the site without styles and JavaScript.
- View all journals
- Explore content
- About the journal
- Publish with us
- Sign up for alerts
- Published: 15 November 2023
Approaching disease transmission with network science
- Shivkumar Vishnempet Shridhar ORCID: orcid.org/0000-0001-6094-3041 1 , 2 &
- Nicholas A. Christakis ORCID: orcid.org/0000-0001-5547-1086 1 , 2 , 3
Nature Reviews Bioengineering volume 2 , pages 6–7 ( 2024 ) Cite this article
202 Accesses
2 Altmetric
Metrics details
- Bioinformatics
- Complex networks
- Infectious diseases
Social connections are an important means for people to cope with adversity and illness. Thus, technologies, such as social network analysis, that can leverage close, face-to-face social networks could help optimize healthcare interventions and reduce healthcare-related costs, particularly in low-resource settings.
This is a preview of subscription content, access via your institution
Access options
Subscribe to this journal
Receive 12 digital issues and online access to articles
92,52 € per year
only 7,71 € per issue
Buy this article
- Purchase on Springer Link
- Instant access to full article PDF
Prices may be subject to local taxes which are calculated during checkout
Christakis, N. A. & Fowler, J. H. The collective dynamics of smoking in a large social network. N. Engl. J. Med. 358 , 2249–2258 (2008).
Article Google Scholar
Valente, T. W., Watkins, S. C., Jato, M. N., van der Straten, A. & Tsitsol, L. P. M. Social network associations with contraceptive use among Cameroonian women in voluntary associations. Soc. Sci. Med. 45 , 677–687 (1997).
Fu, F., Christakis, N. A. & Fowler, J. H. Dueling biological and social contagions. Sci. Rep. 7 , 43634 (2017).
Brito, I. L. et al. Transmission of human-associated microbiota along family and social networks. Nat. Microbiol. 4 , 964–971 (2019).
Shridhar, S. V., Alexander, M. & Christakis, N. A. Characterizing super-spreaders using population-level weighted social networks in rural communities. Philos. Trans. R. Soc. A 380 , 20210123 (2022).
Banerjee, A., Chandrasekhar, A. G., Duflo, E. & Jackson, M. O. Using gossips to spread information: theory and evidence from two randomized controlled trials. Rev. Econ. Stud. 86 , 2453–2490 (2019).
Article MathSciNet Google Scholar
Alexander, M., Forastiere, L., Gupta, S. & Christakis, N. A. Algorithms for seeding social networks can enhance the adoption of a public health intervention in urban India. PNAS 119 , e2120742119 (2022).
Lungeanu, A. et al. Using Trellis software to enhance high-quality large-scale network data collection in the field. Soc. Netw. 66 , 171–184 (2021).
Nishi, A., Dewey, G., Endo, A. & Young, S. D. Network interventions for managing the COVID-19 pandemic and sustaining economy. Proc. Natl Acad. Sci. USA 117 , 30285–30294 (2020).
Vogels, C. B. F. et al. SalivaDirect: a simplified and flexible platform to enhance SARS-CoV-2 testing capacity. Medicine 2 , 263–280 (2021).
Yang, F. et al. Pay-it-forward gonorrhoea and chlamydia testing among men who have sex with men in China: a randomised controlled trial. Lancet Infect. Dis. 20 , 976–982 (2020).
Download references
Acknowledgements
Our work is supported by the NOMIS Foundation.
Author information
Authors and affiliations.
Yale Institute for Network Science, Yale University, New Haven, CT, USA
Shivkumar Vishnempet Shridhar & Nicholas A. Christakis
Department of Biomedical Engineering, Yale University, New Haven, CT, USA
Department of Medicine, Yale School of Medicine, New Haven, CT, USA
Nicholas A. Christakis
You can also search for this author in PubMed Google Scholar
Corresponding author
Correspondence to Nicholas A. Christakis .
Ethics declarations
Competing interests.
The authors declare no competing interests.
Rights and permissions
Reprints and permissions
About this article
Cite this article.
Vishnempet Shridhar, S., Christakis, N.A. Approaching disease transmission with network science. Nat Rev Bioeng 2 , 6–7 (2024). https://doi.org/10.1038/s44222-023-00139-0
Download citation
Published : 15 November 2023
Issue Date : January 2024
DOI : https://doi.org/10.1038/s44222-023-00139-0
Share this article
Anyone you share the following link with will be able to read this content:
Sorry, a shareable link is not currently available for this article.
Provided by the Springer Nature SharedIt content-sharing initiative
Quick links
- Explore articles by subject
- Guide to authors
- Editorial policies
Sign up for the Nature Briefing newsletter — what matters in science, free to your inbox daily.


An official website of the United States government
The .gov means it’s official. Federal government websites often end in .gov or .mil. Before sharing sensitive information, make sure you’re on a federal government site.
The site is secure. The https:// ensures that you are connecting to the official website and that any information you provide is encrypted and transmitted securely.
- Publications
- Account settings
Preview improvements coming to the PMC website in October 2024. Learn More or Try it out now .
- Advanced Search
- Journal List
- Healthcare (Basel)

Machine-Learning-Based Disease Diagnosis: A Comprehensive Review
Md manjurul ahsan.
1 School of Industrial and Systems Engineering, University of Oklahoma, Norman, OK 73019, USA
Shahana Akter Luna
2 Medicine & Surgery, Dhaka Medical College & Hospital, Dhaka 1000, Bangladesh; [email protected]
Zahed Siddique
3 Department of Aerospace and Mechanical Engineering, University of Oklahoma, Norman, OK 73019, USA; ude.uo@euqiddisz
Globally, there is a substantial unmet need to diagnose various diseases effectively. The complexity of the different disease mechanisms and underlying symptoms of the patient population presents massive challenges in developing the early diagnosis tool and effective treatment. Machine learning (ML), an area of artificial intelligence (AI), enables researchers, physicians, and patients to solve some of these issues. Based on relevant research, this review explains how machine learning (ML) is being used to help in the early identification of numerous diseases. Initially, a bibliometric analysis of the publication is carried out using data from the Scopus and Web of Science (WOS) databases. The bibliometric study of 1216 publications was undertaken to determine the most prolific authors, nations, organizations, and most cited articles. The review then summarizes the most recent trends and approaches in machine-learning-based disease diagnosis (MLBDD), considering the following factors: algorithm, disease types, data type, application, and evaluation metrics. Finally, in this paper, we highlight key results and provides insight into future trends and opportunities in the MLBDD area.
1. Introduction
In medical domains, artificial intelligence (AI) primarily focuses on developing the algorithms and techniques to determine whether a system’s behavior is correct in disease diagnosis. Medical diagnosis identifies the disease or conditions that explain a person’s symptoms and signs. Typically, diagnostic information is gathered from the patient’s history and physical examination [ 1 ]. It is frequently difficult due to the fact that many indications and symptoms are ambiguous and can only be diagnosed by trained health experts. Therefore, countries that lack enough health professionals for their populations, such as developing countries like Bangladesh and India, face difficulty providing proper diagnostic procedures for their maximum population of patients [ 2 ]. Moreover, diagnosis procedures often require medical tests, which low-income people often find expensive and difficult to afford.
As humans are prone to error, it is not surprising that a patient may have overdiagnosis occur more often. If overdiagnosis, problems such as unnecessary treatment will arise, impacting individuals’ health and economy [ 3 ]. According to the National Academics of Science, Engineering, and Medicine report of 2015, the majority of people will encounter at least one diagnostic mistake during their lifespan [ 4 ]. Various factors may influence the misdiagnosis, which includes:
- lack of proper symptoms, which often unnoticeable
- the condition of rare disease
- the disease is omitted mistakenly from the consideration
Machine learning (ML) is used practically everywhere, from cutting-edge technology (such as mobile phones, computers, and robotics) to health care (i.e., disease diagnosis, safety). ML is gaining popularity in various fields, including disease diagnosis in health care. Many researchers and practitioners illustrate the promise of machine-learning-based disease diagnosis (MLBDD), which is inexpensive and time-efficient [ 5 ]. Traditional diagnosis processes are costly, time-consuming, and often require human intervention. While the individual’s ability restricts traditional diagnosis techniques, ML-based systems have no such limitations, and machines do not get exhausted as humans do. As a result, a method to diagnose disease with outnumbered patients’ unexpected presence in health care may be developed. To create MLBDD systems, health care data such as images (i.e., X-ray, MRI) and tabular data (i.e., patients’ conditions, age, and gender) are employed [ 6 ].
Machine learning (ML) is a subset of AI that uses data as an input resource [ 7 ]. The use of predetermined mathematical functions yields a result (classification or regression) that is frequently difficult for humans to accomplish. For example, using ML, locating malignant cells in a microscopic image is frequently simpler, which is typically challenging to conduct just by looking at the images. Furthermore, since advances in deep learning (a form of machine learning), the most current study shows MLBDD accuracy of above 90% [ 5 ]. Alzheimer’s disease, heart failure, breast cancer, and pneumonia are just a few of the diseases that may be identified with ML. The emergence of machine learning (ML) algorithms in disease diagnosis domains illustrates the technology’s utility in medical fields.
Recent breakthroughs in ML difficulties, such as imbalanced data, ML interpretation, and ML ethics in medical domains, are only a few of the many challenging fields to handle in a nutshell [ 8 ]. In this paper, we provide a review that highlights the novel uses of ML and DL in disease diagnosis and gives an overview of development in this field in order to shed some light on this current trend, approaches, and issues connected with ML in disease diagnosis. We begin by outlining several methods to machine learning and deep learning techniques and particular architecture for detecting and categorizing various forms of disease diagnosis.
The purpose of this review is to provide insights to recent and future researchers and practitioners regarding machine-learning-based disease diagnosis (MLBDD) that will aid and enable them to choose the most appropriate and superior machine learning/deep learning methods, thereby increasing the likelihood of rapid and reliable disease detection and classification in diagnosis. Additionally, the review aims to identify potential studies related to the MLBDD. In general, the scope of this study is to provide the proper explanation for the following questions:
- 1. What are some of the diseases that researchers and practitioners are particularly interested in when evaluating data-driven machine learning approaches?
- 2. Which MLBDD datasets are the most widely used?
- 3. Which machine learning and deep learning approaches are presently used in health care to classify various forms of disease?
- 4. Which architecture of convolutional neural networks (CNNs) is widely employed in disease diagnosis?
- 5. How is the model’s performance evaluated? Is that sufficient?
In this paper, we summarize the different machine learning (ML) and deep learning (DL) methods utilized in various disease diagnosis applications. The remainder of the paper is structured as follows. In Section 2 , we discuss the background and overview of ML and DL, whereas in Section 3 , we detail the article selection technique. Section 4 includes bibliometric analysis. In Section 5 , we discuss the use of machine learning in various disease diagnoses, and in Section 6 , we identify the most frequently utilized ML methods and datatypes based on the linked research. In Section 7 , we discuss the findings, anticipated trends, and problems. Finally, Section 9 concludes the article with a general conclusion.
2. Basics and Background
Machine learning (ML) is an approach that analyzes data samples to create main conclusions using mathematical and statistical approaches, allowing machines to learn without programming. Arthur Samuel presented machine learning in games and pattern recognition algorithms to learn from experience in 1959, which was the first time the important advancement was recognized. The core principle of ML is to learn from data in order to forecast or make decisions depending on the assigned task [ 9 ]. Thanks to machine learning (ML) technology, many time-consuming jobs may now be completed swiftly and with minimal effort. With the exponential expansion of computer power and data capacity, it is becoming simpler to train data-driven ML models to predict outcomes with near-perfect accuracy. Several papers offer various sorts of ML approaches [ 10 , 11 ].
The ML algorithms are generally classified into three categories such as supervised, unsupervised, and semisupervised [ 10 ]. However, ML algorithms can be divided into several subgroups based on different learning approaches, as shown in Figure 1 . Some of the popular ML algorithms include linear regression, logistic regression, support vector machines (SVM), random forest (RF), and naïve Bayes (NB) [ 10 ].

Different types of machine learning algorithms.
2.1. Machine Learning Algorithms
This section provides a comprehensive review of the most frequently used machine learning algorithms in disease diagnosis.
2.1.1. Decision Tree
The decision tree (DT) algorithm follows divide-and-conquer rules. In DT models, the attribute may take on various values known as classification trees; leaves indicate distinct classes, whereas branches reflect the combination of characteristics that result in those class labels. On the other hand, DT can take continuous variables called regression trees. C4.5 and EC4.5 are the two famous and most widely used DT algorithms [ 12 ]. DT is used extensively by following reference literature: [ 13 , 14 , 15 , 16 ].
2.1.2. Support Vector Machine
For classification and regression-related challenges, support vector machine (SVM) is a popular ML approach. SVM was introduced by Vapnik in the late twentieth century [ 17 ]. Apart from disease diagnosis, SVMs have been extensively employed in various other disciplines, including facial expression recognition, protein fold, distant homology discovery, speech recognition, and text classification. For unlabeled data, supervised ML algorithms are unable to perform. Using a hyperplane to find the clustering among the data, SVM can categorize unlabeled data. However, SVM output is not nonlinearly separable. To overcome such problems, selecting appropriate kernel and parameters is two key factors when applying SVM in data analysis [ 11 ].
2.1.3. K -Nearest Neighbor
K -nearest neighbor (KNN) classification is a nonparametric classification technique invented in 1951 by Evelyn Fix and Joseph Hodges. KNN is suitable for classification as well as regression analysis. The outcome of KNN classification is class membership. Voting mechanisms are used to classify the item. Euclidean distance techniques are utilized to determine the distance between two data samples. The projected value in regression analysis is the average of the values of the KNN [ 18 ].
2.1.4. Naïve Bayes
The naïve Bayes (NB) classifier is a Bayesian-based probabilistic classifier. Based on a given record or data point, it forecasts membership probability for each class. The most probable class is the one having the greatest probability. Instead of predictions, the NB classifier is used to project likelihood [ 11 ].
2.1.5. Logistic Regression
Logistic regression (LR) is an ML approach that is used to solve classification issues. The LR model has a probabilistic framework, with projected values ranging from 0 to 1. Examples of LR-based ML include spam email identification, online fraud transaction detection, and malignant tumor detection. The cost function, often known as the sigmoid function, is used by LR. The sigmoid function transforms every real number between 0 and 1 [ 19 ].
2.1.6. AdaBoost
Yoav Freund and Robert Schapire developed Adaptive Boosting, popularly known as AdaBoost. AdaBoost is a classifier that combines multiple weak classifiers into a single classifier. AdaBoost works by giving greater weight to samples that are harder to classify and less weight to those that are already well categorized. It may be used for categorization as well as regression analysis [ 20 ].
2.2. Deep Learning Overview
Deep learning (DL) is a subfield of machine learning (ML) that employs multiple layers to extract both higher and lower-level information from input (i.e., images, numerical value, categorical values). The majority of contemporary DL models are built on artificial neural networks (ANN), notably convolutional neural networks (CNN), which may be integrated with other DL models, including generative models, deep belief networks, and the Boltzmann machine. Deep learning may be classified into three types: supervised, semisupervised, and unsupervised. Deep neural networks (DNN), reinforcement learning, and recurrent neural networks (RNN) are some of the most prominent DL architectures (RNN) [ 21 ].
Each level in DL learns to convert its input data to the succeeding layers while learning distinct data attributes. For example, the raw input may be a pixel matrix in image recognition applications, and the first layers may detect the image’s edges. On the other hand, the second layer will construct and encode the nose and eyes, and the third layer may recognize the face by merging all of the information gathered from the previous two layers [ 6 ].
In medical fields, DL has enormous promise. Radiology and pathology are two well-known medical fields that have widely used DL in disease diagnosis over the years [ 22 ]. Furthermore, collecting valuable information from molecular state and determining disease progression or therapy sensitivity are practical uses of DL that are frequently unidentified by human investigations [ 23 ].
Convolutional Neural Network
Convolutional neural networks (CNNs) are a subclass of artificial neural networks (ANNs) that are extensively used in image processing. CNN is widely employed in face identification, text analysis, human organ localization, and biological image detection or recognition [ 24 ]. Since the initial development of CNN in 1989, a different type of CNN has been proposed that has performed exceptionally well in disease diagnosis over the last three decades. A CNN architecture comprises three parts: input layer, hidden layer, and output layer. The intermediate levels of any feedforward network are known as hidden layers, and the number of hidden layers varies depending on the type of architecture. Convolutions are performed in hidden layers, which contain dot products of the convolution kernel with the input matrix. Each convolutional layer provides feature maps used as input by the subsequent layers. Following the concealed layer are more layers, such as pooling and fully connected layers [ 21 ]. Several CNN models have been proposed throughout the years, and the most extensively used and popular CNN models are shown in Figure 2 .

Some of the most well-known CNN models, along with their development time frames.
In general, it may be considered that ML and DL have grown substantially throughout the years. The increased computational capability of computers and the enormous number of data available inspire academics and practitioners to employ ML/DL more efficiently. A schematic overview of machine learning and deep learning algorithms and their development chronology is shown in Figure 3 , which may be a helpful resource for future researchers and practitioner.

Illustration of machine learning and deep learning algorithms development timeline.
2.3. Performance Evaluations
This section describes the performance measures used in reference literature. Performance indicators, including accuracy, precision, recall, and F1 score, are widely employed in disease diagnosis. For example, lung cancer can be categorized as true positive ( T P ) or true-negative ( T N ) if individuals are diagnosed correctly, while it can be categorized into false positive ( F P ) or false negative ( F N ) if misdiagnosed. The most widely used metrics are described below [ 10 ].
Accuracy (Acc) : The accuracy denotes total correctly identifying instances among all of the instances. Accuracy can be calculated using following formulas:
Precision ( P n ): Precision is measured as the proportion of precisely predicted to all expected positive observations.
Recall ( R c ): The proportion of overall relevant results that the algorithm properly recognizes is referred to as recall.
Sensitivity ( S n ) : Sensitivity denotes only true positive measure considering total instances and can be measured as follows:
Specificity ( S p ): It identifies how many true negatives are appropriately identified and calculated as follows:
F-measure: The F1 score is the mean of accuracy and recall in a harmonic manner. The highest F score is 1, indicating perfect precision and recall score.
Area under curve (AUC): The area under the curve represents the models’ behaviors in different situations. The AUC can be calculated as follows:
where l p and l n denotes positive and negative data samples and R i is the rating of the i th positive samples.
3. Article Selection
3.1. identification.
The Scopus and Web of Science (WOS) databases are utilized to find original research publications. Due to their high quality and peer review paper index, Scopus and WOS are prominent databases for article searching, as many academics and scholars utilized them for systematic review [ 25 , 26 ]. Using keywords along with Boolean operators, the title search was carried out as follows:
“disease” AND (“diagnsois” OR “Supprot vector machine” OR “SVM” OR “KNN” OR “K-nearest neighbor” OR “logistic regression” OR “K-means clustering” OR “random forest” OR “RF” OR “adaboost” OR “XGBoost”, “decision tree” OR “neural network” OR “NN” OR “artificial neural network” OR “ANN” OR “convolutional neural network” OR “CNN” OR “deep neural network” OR “DNN” OR “machine learning" or “adversarial network” or “GAN”).
The initial search yielded 16,209 and 2129 items, respectively, from Scopus and Web of Science (WOS).
3.2. Screening
Once the search period was narrowed to 2012–2021 and only peer-reviewed English papers were evaluated, the total number of articles decreased to 9117 for Scopus and 1803 for WOS, respectively.
3.3. Eligibility and Inclusion
These publications were chosen for further examination if they are open access and are journal articles. There were 1216 full-text articles (724 from the Scopus database and 492 from WOS). Bibliographic analysis was performed on all 1216 publications. One investigator (Z.S.) imported the 1216 article information as excel CSV data for future analysis. Excel duplication functions were used to identify and eliminate duplicates. Two independent reviewers (M.A. and Z.S.) examined the titles and abstracts of 1192 publications. Disagreements were settled through conversation. We omitted studies that were not relevant to machine learning but were relevant to disease diagnosis or vice versa.
After screening the titles and abstracts, the complete text of 102 papers was examined, and all 102 articles satisfied all inclusion requirements. Factors that contributed to the article’s exclusion from the full-text screening includes:
- 1. Inaccessibility of the entire text
- 2. Nonhuman studies, book chapters, reviews
- 3. Incomplete information related to test result
Figure 4 shows the flow diagram of the systematic article selection procedure used in this study.

MLBDD article selection procedure used in this study.
4. Bibliometric Analysis
The bibliometric study in this section was carried out using reference literature gathered from the Scopus and WOS databases. The bibliometric study examines publications in terms of the subject area, co-occurrence network, year of publication, journal, citations, countries, and authors.
4.1. Subject Area
Many research disciplines have uncovered machine learning-based disease diagnostics throughout the years. Figure 5 depicts a schematic representation of machine learning-based disease detection spread across several research fields. According to the graph, computer science (40%) and engineering (31.2%) are two dominating fields that vigorously concentrated on MLBDD.

Distribution of articles by subject area.
4.2. Co-Occurrence Network
Co-occurrence of keywords provides an overview of how the keywords are interconnected or used by the researchers. Figure 6 displays the co-occurrence network of the article’s keywords and their connection, developed by VOSviewer software. The figure shows that some of the significant clusters include neural networks (NN), decision trees (DT), machine learning (ML), and logistic regression (LR). Each cluster is also connected with other keywords that fall under that category. For instance, the NN cluster contains support vector machine (SVM), Parkinson’s disease, and classification.

Bibliometric map representing co-occurrence analysis of keywords in network visualization.
4.3. Publication by Year
The exponential growth of journal publications is observed from 2017. Figure 7 displays the number of publications between 2012 to 2021 based on the Scopus and WOS data. Note that while the image may not accurately depict the MLBDD’s real contribution, it does illustrate the influence of MLBDD over time.

Publications of machine-learning-based disease diagnosis (MLBDD) by year.
4.4. Publication by Journal
We investigated the most prolific journals in MLBDD domains based on our referred literature.The top ten journals and the number of articles published in the last ten years are depicted in Figure 8 . IEEE Access and Scientific Reports are the most productive journals that published 171 and 133 MLBDD articles, respectively.

Publications by journals.
4.5. Publication by Citations
Citations are one of the primary indicators of an article’s effect. Here, we have identified the top ten cited articles using the R Studio tool. Table 1 summarizes the top articles that achieved the highest citation during the year between 2012 to 2021. Note that Google Scholar and other online databases may have various indexing procedures and times; therefore, the citations in this manuscript may differ from the number of citations shown in this study. The table shows that published articles by [ 27 ] earned the most citations (257), with 51.4 citations per year, followed by Gray [ 28 ]’s article, which obtained 218 citations. It is assumed that all the authors included in Table 1 are among those prominent authors that contributed to MLBDD.
Top ten cited papers published in MLBDD in between 2012–2021 based on Scopus and WOS database.
4.6. Publication by Countries
Figure 9 displayed that China published the most publications in MLBDD, total 259 articles. USA and India are placed 2nd and 3rd, respectively, as they published 139 and 103 papers related to MLBDD. Interestingly, four out of the top ten productive countries are from Asia: China, India, Korea, and Japan.

Top ten countries that contributed to MLBDD literature.
4.7. Publication by Author
According to Table 2 , author Kim J published the most publications (20 out of 1216). Wang Y and Li J Ranked 2nd and 3rd by publishing 19 and 18 articles, respectively. As shown in Table 2 , the number of papers produced by the top 10 authors ranges between 15–20.
Top ten authors based on total number of publications.
5. Machine Learning Techniques for Different Disease Diagnosis
Many academics and practitioners have used machine learning (ML) approaches in disease diagnosis. This section describes many types of machine-learning-based disease diagnosis (MLBDD) that have received much attention because of their importance and severity. For example, due to the global relevance of COVID-19, several studies concentrated on COVID-19 disease detection using ML from 2020 to the present, which also received greater priority in our study. Severe diseases such as heart disease, kidney disease, breast cancer, diabetes, Parkinson’s, Alzheimer’s, and COVID-19 are discussed briefly, while other diseases are covered briefly under the “other disease”.
5.1. Heart Disease
Most researchers and practitioners use machine learning (ML) approaches to identify cardiac disease [ 37 , 38 ]. Ansari et al. (2011), for example, offered an automated coronary heart disease diagnosis system based on neurofuzzy integrated systems that yield around 89% accuracy [ 37 ]. One of the study’s significant weaknesses is the lack of a clear explanation for how their proposed technique would work in various scenarios such as multiclass classification, big data analysis, and unbalanced class distribution. Furthermore, there is no explanation about the credibility of the model’s accuracy, which has lately been highly encouraged in medical domains, particularly to assist users who are not from the medical domains in understanding the approach.
Rubin et al. (2017) uses deep-convolutional-neural-network-based approaches to detect irregular cardiac sounds. The authors of this study adjusted the loss function to improve the training dataset’s sensitivity and specificity. Their suggested model was tested in the 2016 PhysioNet computing competition. They finished second in the competition, with a final prediction of 0.95 specificity and 0.73 sensitivity [ 39 ].
Aside from that, deep-learning (DL)-based algorithms have lately received attention in detecting cardiac disease. Miao and Miao et al. (2018), for example, offered a DL-based technique to diagnosing cardiotocographic fetal health based on a multiclass morphologic pattern. The created model is used to differentiate and categorize the morphologic pattern of individuals suffering from pregnancy complications. Their preliminary computational findings include accuracy of 88.02%, a precision of 85.01%, and an F-score of 0.85 [ 40 ]. During that study, they employed multiple dropout strategies to address overfitting problems, which finally increased training time, which they acknowledged as a tradeoff for higher accuracy.
Although ML applications have been widely employed in heart disease diagnosis, no research has been conducted that addressed the issues associated with unbalanced data with multiclass classification. Furthermore, the model’s explainability during final prediction is lacking in most cases. Table 3 summarizes some of the cited publications that employed ML and DL approaches in the diagnosis of cardiac disease. However, further information about machine-learning-based cardiac disease diagnosis can be found in [ 5 ].
Referenced literature that considered machine-learning-based heart disease diagnosis.
5.2. Kidney Disease
Kidney disease, often known as renal disease, refers to nephropathy or kidney damage. Patients with kidney disease have decreased kidney functional activity, which can lead to kidney failure if not treated promptly. According to the National Kidney Foundation, 10% of the world’s population has chronic kidney disease (CKD), and millions die each year due to insufficient treatment. The recent advancement of ML- and DL-based kidney disease diagnosis may provide a possibility for those countries that are unable to handle the kidney disease diagnostic-related tests [ 49 ]. For instance, Charleonnan et al. (2016) used publicly available datasets to evaluate four different ML algorithms: K -nearest neighbors (KNN), support vector machine (SVM), logistic regression (LR), and decision tree classifiers and received the accuracy of 98.1%, 98.3%, 96.55%, and 94.8%, respectively [ 50 ]. Aljaaf et al. (2018) conducted a similar study. The authors tested different ML algorithms, including RPART, SVM, LOGR, and MLP, using a comparable dataset, CKD, as used by [ 50 ], and found that MLP performed best (98.1 percent) in identifying chronic kidney disease [ 51 ]. To identify chronic kidney disease, Ma et al. (2020) utilizes a collection of datasets containing data from many sources [ 52 ]. Their suggested heterogeneous modified artificial neural network (HMANN) model obtained an accuracy of 87–99%.
Table 4 summarizes some of the cited publications that employed ML and DL approaches to diagnose kidney disease.
Referenced literature that considered machine-learning-based kidney disease diagnosis.
5.3. Breast Cancer
Many scholars in the medical field have proposed machine-learning (ML)-based breast cancer analysis as a potential solution to early-stage diagnosis. Miranda and Felipe (2015), for example, proposed fuzzy-logic-based computer-aided diagnosis systems for breast cancer categorization. The advantage of fuzzy logic over other classic ML techniques is that it can minimize computational complexity while simulating the expert radiologist’s reasoning and style. If the user inputs parameters such as contour, form, and density, the algorithm offers a cancer categorization based on their preferred method [ 57 ]. Miranda and Felipe (2015)’s proposed model had an accuracy of roughly 83.34%. The authors employed an approximately equal ratio of images for the experiment, which resulted in improved accuracy and unbiased performance. However, as the study did not examine the interpretation of their results in an explainable manner, it may be difficult to conclude that accuracy, in general, indicates true accuracy for both benign and malignant classifications. Furthermore, no confusion matrix is presented to demonstrate the models’ actual prediction for the each class.
Zheng et al. (2014) presented hybrid strategies for diagnosing breast cancer disease utilizing k -means clustering (KMC) and SVM. Their proposed model considerably decreased the dimensional difficulties and attained an accuracy of 97.38% using Wisconsin Diagnostic Breast Cancer (WDBC) dataset [ 58 ]. The dataset is normally distributed and has 32 features divided into 10 categories. It is difficult to conclude that their suggested model will outperform in a dataset with an unequal class ratio, which may contain missing value as well.
To determine the best ML models, Asri et al. (2016) applied various ML approaches such as SVM, DT (C4.5), NB, and KNN on the Wisconsin Breast Cancer (WBC) datasets. According to their findings, SVM outperformed all other ML algorithms, obtaining an accuracy of 97.13% [ 59 ]. However, if a same experiment is repeated in a different database, the results may differ. Furthermore, experimental results accompanied by ground truth values may provide a more precise estimate in determining which ML model is the best or not.
Mohammed et al. (2020) conducted a nearly identical study. The authors employ three ML algorithms to find the best ML methods: DT (J48), NB, and sequential minimal optimization (SMO), and the experiment was conducted on two popular datasets: WBC and breast cancer datasets. One of the interesting aspects of this research is that they focused on data imbalance issues and minimized the imbalance problem through the use of resampling data labeling procedures. Their findings showed that the SMO algorithms exceeded the other two classifiers, attaining more than 95% accuracy on both datasets [ 60 ]. However, in order to reduce the imbalance ratio, they used resampling procedures numerous times, potentially lowering the possibility of data diversity. As a result, the performance of those three ML methods may suffer on a dataset that is not normally distributed or imbalanced.
Assegie (2021) used the grid search approach to identify the best k -nearest neighbor (KNN) settings. Their investigation showed that parameter adjustment had a considerable impact on the model’s performance. They demonstrated that by fine-tuning the settings, it is feasible to get 94.35% accuracy, whereas the default KNN achieved around 90% accuracy [ 61 ].
To detect breast cancer, Bhattacherjee et al. (2020) employed a backpropagation neural network (BNN). The experiment was carried out in the WBC dataset with nine features, and they achieved 99.27% accuracy [ 62 ]. Alshayeji et al. (2021) used the WBCD and WDBI datasets to develop a shallow ANN model for classifying breast cancer tumors. The authors demonstrated that the suggested model could classify tumors up to 99.85% properly without selecting characteristics or tweaking the algorithms [ 63 ].
Sultana et al. (2021) detect breast cancer using a different ANN architecture on the WBC dataset. They employed a variety of NN architectures, including the multilayer perceptron (MLP) neural network, the Jordan/Elman NN, the modular neural network (MNN), the generalized feedforward neural network (GFFNN), the self-organizing feature map (SOFM), the SVM neural network, the probabilistic neural network (PNN), and the recurrent neural network (RNN). Their final computational result demonstrates that the PNN with 98.24% accuracy outperforms the other NN models utilized in that study [ 64 ]. However, this study lacks the interpretability as of many other investigations because it does not indicate which features are most important during the prediction phase.
Deep learning (DL) was also used by Ghosh et al. (2021). The WBC dataset was used by the authors to train seven deep learning (DL) models: ANN, CNN, GRU, LSTM, MLP, PNN, and RNN. Long short-term memory (LSTM) and gated recurrent unit (GRU) demonstrated the best performance among all DL models, achieving an accuracy of roughly 99% [ 65 ]. Table 5 summarizes some of the referenced literature that used ML and DL techniques in breast cancer diagnosis.
Referenced literature that considered machine-learning-based breast cancer disease diagnosis.
5.4. Diabetes
According to the International Diabetes Federation (IDF), there are currently over 382 million individuals worldwide who have diabetes, with that number anticipated to increase to 629 million by 2045 [ 71 ]. Numerous studies widely presented ML-based systems for diabetes patient detection. For example, Kandhasamy and Balamurali (2015) compared ML classifiers (J48 DT, KNN, RF, and SVM) for classifying patients with diabetes mellitus. The experiment was conducted on the UCI Diabetes dataset, and the KNN (K = 1) and RF classifiers obtained near-perfect accuracy [ 72 ]. However, one disadvantage of this work is that it used a simplified Diabetes dataset with only eight binary-classified parameters. As a result, getting 100% accuracy with a less difficult dataset is unsurprising. Furthermore, there is no discussion of how the algorithms influence the final prediction or how the result should be viewed from a nontechnical position in the experiment.
Yahyaoui et al. (2019) presented a Clinical Decision Support Systems (CDSS) to aid physicians or practitioners with Diabetes diagnosis. To reach this goal, the study utilized a variety of ML techniques, including SVM, RF, and deep convolutional neural network (CNN). RF outperformed all other algorithms in their computations, obtaining an accuracy of 83.67%, while DL and SVM scored 76.81% and 65.38% accuracy, respectively [ 73 ].
Naz and Ahuja (2020) employed a variety of ML techniques, including artificial neural networks (ANN), NB, DT, and DL, to analyze open-source PIMA Diabetes datasets. Their study indicates that DL is the most accurate method for detecting the development of diabetes, with an accuracy of approximately 98.07% [ 71 ]. The PIMA dataset is one of the most thoroughly investigated and primary datasets, making it easy to perform conventional and sophisticated ML-based algorithms. As a result, gaining greater accuracy with the PIMA Indian dataset is not surprising. Furthermore, the paper makes no mention of interpretability issues and how the model would perform with an unbalanced dataset or one with a significant number of missing variables. As is widely recognized in healthcare, several types of data can be created that are not always labeled, categorized, and preprocessed in the same way as the PIMA Indian dataset. As a result, it is critical to examine the algorithms’ fairness, unbiasedness, dependability, and interpretability while developing a CDSS, especially when a considerable amount of information is missing in a multiclass classification dataset.
Ashiquzzaman et al. (2017) developed a deep learning strategy to address the issue of overfitting in diabetes datasets. The experiment was carried out on the PIMA Indian dataset and yielded an accuracy of 88.41%. The authors claimed that performance improved significantly when dropout techniques were utilized and the overfitting problems were reduced [ 74 ]. Overuse of the dropout approach, on the other hand, lengthens overall training duration. As a result, as they did not address these concerns in their study, assessing whether their proposed model is optimum in terms of computational time is difficult.
Alhassan et al. (2018) introduced the King Abdullah International Research Center for Diabetes (KAIMRCD) dataset, which includes data from 14k people and is the world’s largest diabetic dataset. During that experiment, the author presented a CDSS architecture based on LSTM and GRU-based deep neural networks, which obtained up to 97% accuracy [ 75 ]. Table 6 highlights some of the relevant publications that employed ML and DL approaches in the diagnosis of diabetic disease.
Referenced literature that considered machine-learning-based diabetic disease diagnosis.
5.5. Parkinson’s Disease
Parkinson’s disease is one of the conditions that has received a great amount of attention in the ML literature. It is a slow-progressing chronic neurological disorder. When dopamine-producing neurons in certain parts of the brain are harmed or die, people have difficulty speaking, writing, walking, and doing other core activities [ 80 ]. There are several ML-based approaches have been proposed. For instance, Sriram et al. (2013) used KNN, SVM, NB, and RF algorithms to develop intelligent Parkinson’s disease diagnosis systems. Their computational result shows that, among all other algorithms, RF shows the best performance (90.26% accuracy), and NB demonstrate the worst performance (69.23% accuracy) [ 81 ].
Esmaeilzadeh et al. (2018) proposed a deep CNN-based model to diagnose Parkinson’s disease and achieved almost 100% accuracy on train and test set [ 82 ]. However, there was no mention of any overfitting difficulties in the trial. Furthermore, the experimental results do not provide a good interpretation of the final classification and regression, which is now widely expected, particularly in CDSS. Grover et al. (2018) also used DL-based approaches on UCI’s Parkinson’s telemonitoring voice dataset. Their experiment using DNN has achieved around 81.67% accuracy in diagnosing patients with Parkinson’s disease symptoms [ 80 ].
Warjurkar and Ridhorkar (2021) conducted a thorough study on the performance of the ML-based approach in decision support systems that can detect both brain tumors and diagnose Parkinson’s patients. Based on their findings, it was obvious that, when compared to other algorithms, boosted logistic regression surpassed all other models, attaining 97.15% accuracy in identifying Parkinson’s disease patients. In tumor segmentation, however, the Markov random technique performed best, obtaining an accuracy of 97.4% [ 83 ]. Parkinson’s disease diagnosis using ML and DL approaches is summarized in Table 7 , which includes a number of references to the relevant research.
Referenced literature that considered machine-learning-based Parkinson’s disease diagnosis.
5.6. COVID-19
The new severe acute respiratory syndrome coronavirus 2 (SARS-CoV-2), also known as COVID-19, pandemic has become humanity’s greatest challenge in contemporary history. Despite the fact that a vaccine had been advanced in distribution because to the global emergency, it was unavailable to the majority of people for the duration of the crisis [ 88 ]. Because of the new COVID-19 Omicron strain’s high transmission rates and vaccine-related resistance, there is an extra layer of concern. The gold standard for diagnosing COVID-19 infection is now Real-Time Reverse Transcription-Polymerase Chain Reaction (RT-PCR) [ 89 , 90 ]. Throughout the epidemic, the researcher advocated other technologies including as chest X-rays and Computed Tomography (CT) combined with Machine Learning and Artificial Intelligence to aid in the early detection of people who might be infected. For example, Chen et al. (2020) proposed a UNet++ model employing CT images from 51 COVID-19 and 82 non-COVID-19 patients and achieved an accuracy of 98.5% [ 91 ]. Ardakani et al. (2020) used a small dataset of 108 COVID-19 and 86 non-COVID-19 patients to evaluate ten different DL models and achieved a 99% overall accuracy [ 92 ]. Wang et al. (2020) built an inception-based model with a large dataset, containing 453 CT scan images, and achieved 73.1% accuracy. However, the model’s network activity and region of interest were poorly explained [ 93 ]. Li et al. (2020) suggested the COVNet model and obtain 96% accuracy utilizing a large dataset of 4356 chest CT images of Pneumonia patients, 1296 of which were verified COVID-19 cases [ 94 ].
Several studies investigated and advised screening COVID-19 patients utilizing chest X-ray images in parallel, with major contributions in [ 95 , 96 , 97 ]. For example, Hemdan et al. (2020) used a small dataset of only 50 images to identify COVID-19 patients from chest X-ray images with an accuracy of 90% and 95%, respectively, using VGG19 and ResNet50 models [ 95 ]. Using a dataset of 100 chest X-ray images, Narin et al. (2021) distinguished COVID-19 patients from those with Pneumonia with 86% accuracy [ 97 ].
In addition, in order to develop more robust and better screening systems, other studies considered larger datasets. For example, Brunese et al. (2020) employed 6505 images with a data ratio of 1:1.17, with 3003 images classified as COVID-19 symptoms and 3520 as “other patients” for the objectives of that study [ 98 ]. With a dataset of 5941 images, Ghoshal and Tucker (2020) achieved 92.9% accuracy [ 99 ]. However, neither study looked at how their proposed models would work with data that was severely unbalanced and had mismatched class ratios. Apostolopoulos and Mpesiana (2020) employed a CNN-based Xception model on an imbalanced dataset of 284 COVID-19 and 967 non-COVID-19 patient chest X-ray images and achieved 89.6% accuracy [ 100 ].
The following Table 8 summarizes some of the relevant literature that employed ML and DL approaches to diagnose COVID-19 disease.
Referenced literature that considered machine-learning-based COVID-19 disease diagnosis.
5.7. Alzheimer’s Disease
Alzheimer is a brain illness that often begins slowly but progresses over time, and it affects 60–70% of those who are diagnosed with dementia [ 103 ]. Alzheimer’s disease symptoms include language problems, confusion, mood changes, and other behavioral disorders. Body functions gradually deteriorated, and the usual life expectancy is three to nine years after diagnosis. Early diagnosis, on the other hand, may assist to avoid and take required actions to enter into suitable treatment as soon as possible, which will also raise the possibility of life expectancy. Machine learning and deep learning have shown promising outcomes in detecting Alzheimer’s disease patients throughout the years. For instance, Neelaveni and Devasana (2020) proposed a model that can detect Alzheimer patients using SVM and DT, and achieved an accuracy of 85% and 83% respectively [ 104 ]. Collij et al. (2016) also used SVM to detect single-subject Alzheimer’s disease and mild cognitive impairment (MCI) prediction and achieved an accuracy of 82% [ 105 ].
Multiple algorithms have been adopted and tested in developing ML based Alzheimer disease diagnosis. For example, Vidushi and Shrivastava (2019) experimented using Logistic Regression (LR), SVM, DT, ensemble Random Forest (RF), and Boosting Adaboost and achieved an accuracy of 78.95%, 81.58%, 81.58%, 84.21%, and 84.21% respectively [ 106 ]. Many of the study adopted CNN based approach to detect Alzheimer patients as CNN demonstrates robust results in image processing compared to other existing algorithms. As a consequence, Ahmed et al. (2020) proposed a CNN model for earlier diagnosis and classification of Alzheimer disease. Within the dataset consists of 6628 MRI images, the proposed model achieved 99% accuracy [ 107 ]. Nawaz et al. (2020) proposed deep feature-based models and achieved an accuracy of 99.12% [ 108 ]. Additionally, Studies conducted by Haft-Javaherian et al. (2019) [ 109 ] and Aderghal et al. (2017) [ 110 ] are some of the CNN based study that also demonstrates the robustness of CNN based approach in Alzheimer disease diagnosis. ML and DL approaches employed in the diagnosis of Alzheimer’s disease are summarized in Table 9 .
Referenced literature that considered Machine Learning-based Alzheimer disease diagnosis.
5.8. Other Diseases
Beyond the disease mentioned above, ML and DL have been used to identify various other diseases. Big data and increasing computer processing power are two key reasons for this increased use. For example, Mao et al. (2020) used Decision Tree (DT) and Random Forest (RF) to disease classification based on eye movement [ 114 ]. Nosseir and Shawky (2019) evaluated KNN and SVM to develop automatic skin disease classification systems, and the best performance was observed using KNN by achieving an accuracy of 98.22% [ 115 ]. Khan et al. (2020) employed CNN-based approaches such as VGG16 and VGG19 to classify multimodal Brain tumors. The experiment was carried out using publicly available three image datasets: BraTs2015, BraTs2017, and BraTs2018, and achieved 97.8%, 96.9%, and 92.5% accuracy, respectively [ 116 ]. Amin et al. (2018) conducted a similar experiment utilizing the RF classifier for tumor segmentation. The authors achieved 98.7%, 98.7%, 98.4%, 90.2%, and 90.2% accuracy using BRATS 2012, BRATS 2013, BRATS 2014, BRATS 2015, and ISLES 2015 dataset, respectively [ 117 ].
Dai et al. (2019) proposed a CNN-based model to develop an application to detect Skin cancer. The authors used a publicly available dataset, HAM10000, to experiment and achieved 75.2% accuracy [ 118 ]. Daghrir et al. (2020) evaluated KNN, SVM, CNN, Majority Voting using ISIC (International Skin Imaging Collaboration) dataset to detect Melanoma skin cancer. The best result was found using Majority Voting (88.4% accuracy) [ 119 ]. Table 10 summarizes some of the referenced literature that used ML and DL techniques in various disease diagnosis.
Referenced literature that considered Machine Learning on various disease diagnoses.
6. Algorithm and Dataset Analysis
Most of the referenced literature considered multiple algorithms in MLBDD approaches. Here we have addressed multiple algorithms as hybrid approaches. For instance, Sun et al. (2021) used hybrid approaches to predict coronary Heart disease using Gaussian Naïve Bayes, Bernoulli Naïve Bayes, and Random Forest (RF) algorithms [ 111 ]. Bemando et al. (2021) adopted CNN and SVM to automate the diagnosis of Alzheimer’s disease and mild cognitive impairment [ 41 ]. Saxena et al. (2019) used KNN and Decision Tree (DT) in Heart disease diagnosis [ 131 ]; Elsalamony (2018) employed Neural Networks (NN) and SVM in detecting Anaemia disease in human red blood cells [ 132 ]. One of the key benefits of using the hybrid technique is that it is more accurate than using single ML models.
According to the relevant literature, the most extensively utilized induvial algorithms in developing MLBDD models are CNN, SVM, and LR. For instance, Kalaiselvi et al. (2020) proposed CNN based approach in Brain tumor diagnosis [ 123 ]; Dai et al. (2019) used CNN in developing a device inference app for Skin cancer detection [ 118 ]; Fathi et al. (2020) used SVM to classify liver diseases [ 121 ]; Sing et al. (2019) used SVM to classify the patients with Heart disease symptoms [ 43 ]; and Basheer et al. (2019) used Logistic Regression to detect Heart disease [ 133 ].
Figure 10 depicts the most commonly used Machine Learning algorithms in disease diagnosis. The bolder and larger font emphasizes the importance and frequency with which the algorithms in MLBDD are used. Based on the Figure, we can observe that Neural Networks, CNN, SVM, and Logistic Regression are the most commonly employed algorithms by MLBDD researchers.

Word cloud for most frequently used ML algorithms in MLBDD publications.
Most MLBDD researchers utilize publically accessible datasets since they do not require permission and provide sufficient information to do the entire study. Manually gathering data from patients, on the other hand, is time-consuming; yet, numerous research utilized privately collected/owned data, either owing to their special necessity based on their experiment setup or to produce a result with actual data [ 46 , 55 , 56 , 68 , 70 ]. The Cleveland Heart disease dataset, PIMA dataset, and Parkinson dataset are the most often utilized datasets in disease diagnosis areas. Table 11 lists publicly available datasets and sources that may be useful to future academics and practitioners.
Most widely used disease diagnosis dataset URL along with the referenced literature (accessed on 16 December 2021).
7. Discussion
In the last 10 years, Machine Learning (ML) and Deep Learning (DL) have grown in prominence in disease diagnosis, which the annotated literature has strengthened in this study. The review began with specific research questions and attempted to answer them using the reference literature. According to the overall research, CNN is one of the most emerging algorithms, outperforming all other ML algorithms due to its solid performance with both image and tabular data [ 94 , 123 , 128 , 137 ]. Transfer learning is also gaining popularity since it does not necessitate constructing a CNN model from scratch and produces better results than typical ML methods [ 47 , 91 ]. Aside from CNN, the reference literature lists SVM, RF, and DT as some of the most common algorithms utilized widely in MLBDD. Furthermore, several researchers are emphasizing ensemble techniques in MLBDD [ 127 , 130 ]. Nonetheless, when compared to other ML algorithms, CNN is the most dominating. VGG16, VGG19, ResNet50, and UNet++ are among of the most prominent CNN architectures utilized widely in disease diagnosis.
In terms of databases, it was discovered that UCI repository data is the preferred option of academics and practitioners for constructing a Machine Learning-based Disease Diagnosis (MLBDD) model. However, while the current dataset frequently has shortcomings, several researchers have recently relied on additional data acquired from the hospital or clinic (i.e., imbalance data, missing data). To assist future researchers and practitioners interested in studying MLBDD, we have included a list of some of the most common datasets utilized in the reference literature in Table 11 , along with the link to the repository.
As previously indicated, there were several inconsistencies in terms of assessment measures published by the literature. For example, some research reported their results with accuracy [ 45 ]; others provided with accuracy, precision, recall, and F1-score [ 42 ]; while a few studies emphasized sensitivity, specificity, and true positive [ 67 ]. As a result, there were no criteria for the authors to follow in order to report their findings correctly and genuinely. Nonetheless, of all assessment criteria, accuracy is the most extensively utilized and recognized by academics.
With the emergence of COVID-19, MLBDD research switched mostly on Pneumonia and COVID-19 patient detection beginning in 2020, and COVID-19 remains a popular subject as the globe continues to battle this disease. As a result, it is projected that the application of ML and DL in the medical sphere for disease diagnosis would expand significantly in this domain in the future as well. Many questions have been raised due to the progress of ML and DL-based disease diagnosis. For example, if a doctor or other health practitioner incorrectly diagnoses a patient, he or she will be held accountable. However, if the machine does, who will be held accountable? Furthermore, fairness is an issue in ML because most ML models are skewed towards the majority class. As a result, future research should concentrate on ML ethics and fairness.
Model interpretation is absent in nearly all investigations, which is surprising. Interpreting machine learning models used to be difficult, but explainable and interpretable XAI have made it much easier. Despite the fact that the previous MLBDD lacked sufficient interpretations, it is projected that future researchers and practitioners would devote more attention to interpreting the machine learning model due to the growing demand for model interpretability.
The idea that ML alone will enough to construct an MLBDD model is a flawed one. To make the MLBDD model more dynamic, it may be anticipated that the model will need to be developed and stored on a cloud system, as the heath care industry generates a lot of data that is typically kept in cloud systems. As a result, the adversarial attack will focus on patients’ data, which is very sensitive. For future ML-based models, the data bridge and security challenges must be taken into consideration.
It is a major issue to analyze data if there is a large disparity in the data. As the ML-based diagnostic model deals with human life, every misdiagnosis is a possible danger to one’s health. However, despite the fact that many study used the imbalance dataset to perform their experiment, none of the cited literature highlights issues related to imbalance data. Thus, future work should demonstrate the validity of any ML models while developing with imbalanced data.
Within the many scopes this review paper also have some limitations which can be summarized as follows:
- 1. The study first searched the Scopus and WOS databases for relevant papers and then examined other papers that were pertinent to this investigation. If other databases like Google Scholar and Pubmed were used, the findings might be somewhat different. As a result, our study may provide some insight into MLBDD, but there is still a great deal of information that is outside of our control.
- 2. ML algorithms, DL algorithms, dataset, disease classifications, and evaluation metrics are highlighted in the review. Though the suggested ML process is thoroughly examined in reference literature, this paper does not go into that level of detail.
- 3. Only those publications that adhered to a systematic literature review technique were included in the study’s paper selection process. Using a more comprehensive range of keywords, on the other hand, might lead to higher search activity. However, our SLR approach will provide researchers and practitioners with a more thorough understanding of MLBDD.
8. Research Challenges and Future Agenda
While machine learning-based applications have been used extensively in disease diagnosis, researchers and practitioners still face several challenges when deploying them as a practical application in healthcare. In this section, the key challenges associated with ML in disease diagnosis have been summarized as follows:
8.1. Data Related Challenges
- 1. Data scarcity: Even though many patients’ data has been recorded by different hospitals and healthcare, due to the data privacy act, real-world data is not often available for global research purposes.
- 2. Noisy data: Frequently, the clinical data contains noise or missing values; therefore, such kind of data takes a reasonable amount of time to make it trainable.
- 3. Adversarial attack: Adversarial attack is one of the key issues in the disease dataset. Adversarial attack means the manipulation of training data, testing data, or machine learning model to result in wrong output from ML.
8.2. Disease Diagnosis-Related Challenges
- 1. Misclassification: While the machine learning model can be used to develop as a disease diagnosis model, any misclassification for a particular disease might bring severe damage. For instance, if a patient with stomach cancer is diagnosed as a non-cancer patient, it will have a huge impact.
- 2. Wrong image segmentation: One of the key challenges with the ML model is that the model often identifies the wrong region as an infected region. For instance, author Ahsan et al. (2020) shows that even though the accuracy is around 100% in detecting COVID-19 and non-COVID-19 patients, the pre-trained CNN models such as VGG16 and VGG19 often pay attention to the wrong region during the training process [ 2 ]. As a result, it also raises the question of the validity of the MLBDD.
- 3. Confusion: Some of the diseases such as COVID-19, pneumonia, edema in the chest often demonstrate similar symptoms; in these particular cases, many CNN models detect all of the data samples into one class, i.e., COVID-19.
8.3. Algorithm Related Challenges
- 1. Supervised vs. unsupervised: Most ML models (Linear regression, logistic regression) performed very well with the labeled data. However, similar algorithms’ performance was significantly reduced with the unlabeled data. On the other hand, popular algorithms that can perform well with unlabeled data such as K-means clustering, SVM, and KNNs performance also degraded with multidimensional data.
- 2. Blackbox-related challenges: One of the most widely used ML algorithms is convolutional neural networks. However, one of the key challenges associated with this algorithm is that it is often hard to interpret how the model adjusts internal parameters such as learning rate and weights. In healthcare, implementing such an algorithm-related model needs proper explanations.
8.4. Future Directions
The challenges addressed in the above section might give some future direction to future researchers and practitioners. Here we have introduced some of the possible algorithms and applications that might overcome existing MLBDD challenges.
- 1. GAN-based approach: Generative adversarial network is one of the most popular approaches in deep learning fields. Using this approach, it is possible to generate synthetic data which looks almost similar to the real data. Therefore, GAN might be a good option for handling data scarcity issues. Moreover, it will also reduce the dependency on real data and also will help to follow the data privacy act.
- 2. Explainable AI: Explainable AI is a popular domain that is now widely used to explain the algorithms’ behavior during training and prediction. Still, the explainable AI domains face many challenges; however, the implementation of interpretability and explainability clarifies the ML models’ deployment in the real world.
- 3. Ensemble-based approach: With the advancement of modern technology, we can now capture high resolutions and multidimensional data. While the traditional ML approach might not perform well with high-quality data, a combination of several machine learning models might be an excellent option to handle such high-dimensional data.
9. Conclusions and Potential Remarks
This study reviewed the papers published between 2012–2021 that focused on Machine Learning-based Disease Diagnosis (MLBDD). Researchers are particularly interested in some diseases, such as Heart disease, Breast cancer, Kidney disease, Diabetes, Alzheimer’s, and Parkinson’s diseases, which are discussed considering machine learning/deep learning-based techniques. Additionally, some other ML-based disease diagnosis approaches are discussed as well. Prior to that, A bibliometric analysis was performed, taking into account a variety of parameters such as subject area, publication year, journal, country, and identifying the most prominent contributors in the MLBDD field. According to our bibliometric research, machine learning applications in disease diagnosis have grown at an exponential rate since 2017. In terms of overall number of publications over the years, IEEE Access, Scientific Reports, and the International Journal of advanced computer science and applications are the three most productive journals. The three most-cited publications on MLBDD are those by Motwani et al. (2017), Gray et al. (2013), and Mohan et al. (2019). In terms of overall publications, China, the United States, and India are the three most productive countries. Kim J, the most influential author, published around 20 publications between 2012 and 2021, followed by Wang Y and Li J, who came in second and third place, respectively. Around 40% of the publication are from computer science domains and around 31% from engineering fields, demonstrating their domination in the MLBDD field.
Finally, we have systematically selected 102 papers for in-depth analysis. Our overall findings were highlighted in the discussion sections. Because of its remarkable performance in constructing a robust model, our primary conclusion implies that deep learning is the most popular method for researchers. Despite the fact that deep learning is widely applied in MLBDD fields, the majority of the research lacks sufficient explanations of the final predictions. As a result, future research in MLBDD needs focus on pre and post hoc analysis and model interpretation in order to use the ML model in healthcare.
Physical patient services are increasingly dangerous as a result of the emergence of COVID-19. At the same time, the health-care system must be maintained. While telemedicine and online consultation are becoming more popular, it is still important to consider an alternate strategy that may also highlight the importance of in-person health facilities. Many recent studies recommend home-robot service for patient care rather than hospitalization [ 138 ].
Many countries are increasingly worried about the privacy of patients’ data. Many nations have also raised legal concerns about the ethics of AI and ML when used with real-world patient data [ 139 ]. As a result, rather of depending on data gathering and processing, future study could try producing synthetic data. Some of the techniques that future researchers and practitioners may be interested in to produce synthetic data for the experiment include generative adversarial networks, ADASYN, SMOTE, and SVM-SMOTE.
Cloud systems are becoming potential threats as a result of data storage in it. As a result, any built ML models must safeguard patient access and transaction concerns. Many academics exploited blockchain technology to access and distribute data [ 140 , 141 ]. As a result, blockchain technology paired with deep learning and machine learning might be a promising study subject for constructing safe diagnostic systems.
We anticipate that our review will guide both novice and expert research and practitioners in MLBDD. It would be interesting to see some research work based on the limitations addressed in the discussion and conclusion section. Additionally, future works in MLBDD might focus on multiclass classification with highly imbalanced data along with highly missing data, explanation and Interpretation of multiclass data classification using XAI, and optimizing the big data containing numerical, categorical, and image data.
Author Contributions
Conceptualization—M.M.A.; methodology—M.M.A. and Z.S.; software—M.M.A.; validation—Z.S. and S.A.L.; formal analysis—S.A.L.; investigation—Z.S.; writing—original draft preparation—M.M.A.; writing—review and editing—M.M.A., S.A.L., Z.S.; supervision—Z.S. All authors have read and agreed to the published version of the manuscript.
This research received no external funding.
Conflicts of Interest
The authors declare no conflict of interest.
Publisher’s Note: MDPI stays neutral with regard to jurisdictional claims in published maps and institutional affiliations.
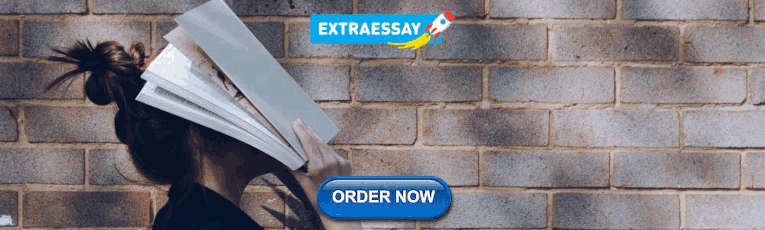
IMAGES
VIDEO
COMMENTS
In the "disease gene network" (DGN) nodes represent disease genes, and two genes are connected if they are associated with the same disorder ( Figs. 1 and 2 b ). Next, we discuss the potential of these networks to help us understand and represent in a single framework all known disease gene and phenotype associations. Fig. 2.
The human disease network. In this paper, we review the construction, the application, the meaning and the interpretation of the Diseasome network, which enables a systematic connection between the molecular and the phenotype level, and derived models like the human disease network. Further, we are surveying recent conceptual and methodological ...
In this paper, we use large-scale medical bibliographic records and the related Medical Subject Headings (MeSH) metadata 31 from PubMed 32, to generate a symptom-based network of human diseases ...
A breakthrough is the pioneering human disease network (HDN) research 2,3,4,5,6, under which a large number of diseases are simultaneously considered, and their interconnections are modelled.
Abstract. A network of disorders and disease genes linked by known disorder-gene associations offers a platform to explore in a single graph-theoretic framework all known phenotype and disease gene associations, indicating the common genetic origin of many diseases. Genes associated with similar disorders show both higher likelihood of physical ...
(A) Meaning and interpretation of different types of networks used in network biology and medicine. (B) Constructing the human disease and disease gene network.
A systematic study by Huang et al. 158 evaluated 21 human interaction networks on their ability to predict disease genes and found ConsensusPathDB 159, GIANT 148 and STRING 147 to have the best ...
A network of disorders and disease genes linked by known disorder-gene associations offers a platform to explore in a single graph-theoretic framework all known phenotype and disease gene associations, indicating the common genetic origin of many diseases. Genes associated with similar disorders show both higher likelihood of physical ...
One can also construct the human disease gene network, the network of human genes, connected by implicating common human disorders ... This work is supported by the Basic Science Research Program (No. 2011-0014191) funded by National Research Foundation (NRF), Ministry of Education, Science and Technology (MEST) (to K.- ... Papers. Download all ...
The construction, the application, the meaning and the interpretation of the Diseasome network, which enables a systematic connection between the molecular and the phenotype level, and derived models like the human disease network are reviewed. In this paper, we review the construction, the application, the meaning and the interpretation of the Diseasome network, which enables a systematic ...
In the ''disease gene network'' (DGN) nodes represent disease genes, and two genes are connected if they are associated with the same disorder (Figs. 1 and 2b). Next, we discuss the potential of these networks to help us understand and represent in a single framework all known disease gene and phenotype associations.
It is found that essential human genes are likely to encode hub proteins and are expressed widely in most tissues, suggesting that disease genes also would play a central role in the human interactome, and that diseases caused by somatic mutations should not be peripheral. A network of disorders and disease genes linked by known disorder-gene associations offers a platform to explore in a ...
While the bulk of research on biological networks has focused on E. coli and S. cerevisiae, following the human genome project, the amount of data pertaining to networks in the human cells exceeds in richness and diversity the data available for model organisms.In the following, we briefly discuss the most studied network maps and their limitations, but remind the reader to exercise caution as ...
Identification of genes causing diseases is a primary goal in human health research for accurate disease diagnosis, treatment, and prevention [1,2]. In the process of cloning and dividing genes, structural changes can occur in a gene that can transform biological processes and cause diseases. ... A disease network was formed by adding the ...
With inpatient and outpatient treatment data on close to 1 million randomly selected subjects and collected during the period of 2000 to 2013, the human disease cost network is constructed using a novel copula-based approach and the weighted correlation-based network construction technique. Extensive analysis is conducted, and the results are ...
HumanNet-XI contains 17 303 genes and 418 525 links. The fourth level network, HumanNet-XN, is a fully extended functional gene network by both co-citation and interologs. Interologs derived from non-human species provided 101 036 more links to HumanNet-XC, yet its genome coverage only increased from 94.6 to 95.3%.
The human disease network - PMC. Journal List. Proc Natl Acad Sci U S A. v.104 (21); 2007 May 22. PMC1885563. As a library, NLM provides access to scientific literature. Inclusion in an NLM database does not imply endorsement of, or agreement with, the contents by NLM or the National Institutes of Health.
Abstract. A plethora of network-based approaches within the Systems Biology universe have been applied, to date, to investigate the underlying molecular mechanisms of various human diseases. In the present study, we perform a bipartite, topological and clustering graph analysis in order to gain a better understanding of the relationships ...
The Human Disease Network. No comments yet. A network of disorders and disease genes linked by known disorder-gene associations offers a platform to explore in a single graph-theoretic framework ...
We found that 68% of disorders exhibited almost perfect tissue-homogeneity ( Fig. 3b ), compared with 51% expected by chance ( P < 10 −5 ). Finally, disease genes that participate in a common functional module should also show high expression profiling correlation ( 26 ). The distribution of Pearson correlation coefficients (PCCs) for the ...
Planet versus Plastics. Plastic waste has infiltrated every corner of our planet, from oceans and waterways to the food chain and even our bodies. Only 9% of plastic is recycled due to factors including poor infrastructure, technical challenges, lack of incentives, and low market demand. "We need legislation that disincentivizes big oil from ...
1. Introduction. With the rapid development of high-throughput biological experiment and the wide application of bioinformatics (Guingab-Cagmat et al., 2013), the identification of genes related to human diseases becomes more and more important in understanding the mechanism of disease pathogenesis.Many biological networks (Raval and Ray, 2013) have been used to identify disease related genes ...
In this paper, a new automatic modulation recognition (AMR) method named CCLDNN (complex-valued convolution long short-term memory deep neural network) is proposed. It is designed to significantly improve the recognition accuracy of modulation modes in low signal-to-noise ratio (SNR) environments. The model integrates the advantages of existing mainstream neural networks. The phase and ...
Approaching disease transmission with network science. Shivkumar Vishnempet Shridhar &. Nicholas A. Christakis. Nature Reviews Bioengineering 2 , 6-7 ( 2024) Cite this article. 202 Accesses. 2 ...
A Feature Paper should be a substantial original Article that involves several techniques or approaches, provides an outlook for future research directions and describes possible research applications. Feature papers are submitted upon individual invitation or recommendation by the scientific editors and must receive positive feedback from the ...
the disease is omitted mistakenly from the consideration. Machine learning (ML) is used practically everywhere, from cutting-edge technology (such as mobile phones, computers, and robotics) to health care (i.e., disease diagnosis, safety). ML is gaining popularity in various fields, including disease diagnosis in health care.