Change Password
Your password must have 6 characters or more:.
- a lower case character,
- an upper case character,
- a special character
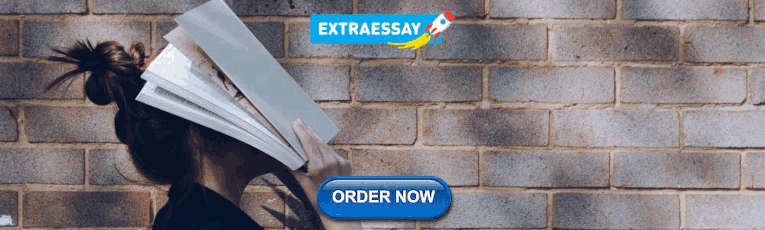
Password Changed Successfully
Your password has been changed
Create your account
Forget yout password.
Enter your email address below and we will send you the reset instructions
If the address matches an existing account you will receive an email with instructions to reset your password
Forgot your Username?
Enter your email address below and we will send you your username
If the address matches an existing account you will receive an email with instructions to retrieve your username

- April 01, 2024 | VOL. 181, NO. 4 CURRENT ISSUE pp.255-346
- March 01, 2024 | VOL. 181, NO. 3 pp.171-254
- February 01, 2024 | VOL. 181, NO. 2 pp.83-170
- January 01, 2024 | VOL. 181, NO. 1 pp.1-82
The American Psychiatric Association (APA) has updated its Privacy Policy and Terms of Use , including with new information specifically addressed to individuals in the European Economic Area. As described in the Privacy Policy and Terms of Use, this website utilizes cookies, including for the purpose of offering an optimal online experience and services tailored to your preferences.
Please read the entire Privacy Policy and Terms of Use. By closing this message, browsing this website, continuing the navigation, or otherwise continuing to use the APA's websites, you confirm that you understand and accept the terms of the Privacy Policy and Terms of Use, including the utilization of cookies.
The Critical Relationship Between Anxiety and Depression
- Ned H. Kalin , M.D.
Search for more papers by this author
Anxiety and depressive disorders are among the most common psychiatric illnesses; they are highly comorbid with each other, and together they are considered to belong to the broader category of internalizing disorders. Based on statistics from the Substance Abuse and Mental Health Services Administration, the 12-month prevalence of major depressive disorder in 2017 was estimated to be 7.1% for adults and 13.3% for adolescents ( 1 ). Data for anxiety disorders are less current, but in 2001–2003, their 12-month prevalence was estimated to be 19.1% in adults, and 2001–2004 data estimated that the lifetime prevalence in adolescents was 31.9% ( 2 , 3 ). Both anxiety and depressive disorders are more prevalent in women, with an approximate 2:1 ratio in women compared with men during women’s reproductive years ( 1 , 2 ).
Across all psychiatric disorders, comorbidity is the rule ( 4 ), which is definitely the case for anxiety and depressive disorders, as well as their symptoms. With respect to major depression, a worldwide survey reported that 45.7% of individuals with lifetime major depressive disorder had a lifetime history of one or more anxiety disorder ( 5 ). These disorders also commonly coexist during the same time frame, as 41.6% of individuals with 12-month major depression also had one or more anxiety disorder over the same 12-month period. From the perspective of anxiety disorders, the lifetime comorbidity with depression is estimated to range from 20% to 70% for patients with social anxiety disorder ( 6 ), 50% for patients with panic disorder ( 6 ), 48% for patients with posttraumatic stress disorder (PTSD) ( 7 ), and 43% for patients with generalized anxiety disorder ( 8 ). Data from the well-known Sequenced Treatment Alternatives to Relieve Depression (STAR*D) study demonstrate comorbidity at the symptom level, as 53% of the patients with major depression had significant anxiety and were considered to have an anxious depression ( 9 ).
Anxiety and depressive disorders are moderately heritable (approximately 40%), and evidence suggests shared genetic risk across the internalizing disorders ( 10 ). Among internalizing disorders, the highest level of shared genetic risk appears to be between major depressive disorder and generalized anxiety disorder. Neuroticism is a personality trait or temperamental characteristic that is associated with the development of both anxiety and depression, and the genetic risk for developing neuroticism also appears to be shared with that of the internalizing disorders ( 11 ). Common nongenetic risk factors associated with the development of anxiety and depression include earlier life adversity, such as trauma or neglect, as well as parenting style and current stress exposure. At the level of neural circuits, alterations in prefrontal-limbic pathways that mediate emotion regulatory processes are common to anxiety and depressive disorders ( 12 , 13 ). These findings are consistent with meta-analyses that reveal shared structural and functional brain alterations across various psychiatric illnesses, including anxiety and major depression, in circuits involving emotion regulation ( 13 ), executive function ( 14 ), and cognitive control ( 15 ).
Anxiety disorders and major depression occur during development, with anxiety disorders commonly beginning during preadolescence and early adolescence and major depression tending to emerge during adolescence and early to mid-adulthood ( 16 – 18 ). In relation to the evolution of their comorbidity, studies demonstrate that anxiety disorders generally precede the presentation of major depressive disorder ( 17 ). A European community-based study revealed, beginning at age 15, the developmental relation between comorbid anxiety and major depression by specifically focusing on social phobia (based on DSM-IV criteria) and then asking the question regarding concurrent major depressive disorder ( 18 ). The findings revealed a 19% concurrent comorbidity between these disorders, and in 65% of the cases, social phobia preceded major depressive disorder by at least 2 years. In addition, initial presentation with social phobia was associated with a 5.7-fold increased risk of developing major depressive disorder. These associations between anxiety and depression can be traced back even earlier in life. For example, childhood behavioral inhibition in response to novelty or strangers, or an extreme anxious temperament, is associated with a three- to fourfold increase in the likelihood of developing social anxiety disorder, which in turn is associated with an increased risk to develop major depressive disorder and substance abuse ( 19 ).
It is important to emphasize that the presence of comor‐bid anxiety symptoms and disorders matters in relation to treatment. Across psychiatric disorders, the presence of significant anxiety symptoms generally predicts worse outcomes, and this has been well demonstrated for depression. In the STAR*D study, patients with anxious major depressive disorder were more likely to be severely depressed and to have more suicidal ideation ( 9 ). This is consistent with the study by Kessler and colleagues ( 5 ), in which patients with anxious major depressive disorder, compared with patients with nonanxious major depressive disorder, were found to have more severe role impairment and more suicidal ideation. Data from level 1 of the STAR*D study (citalopram treatment) nicely illustrate the impact of comorbid anxiety symptoms on treatment. Compared with patients with nonanxious major depressive disorder, those 53% of patients with an anxious depression were less likely to remit and also had a greater side effect burden ( 20 ). Other data examining patients with major depressive disorder and comorbid anxiety disorders support the greater difficulty and challenge in treating patients with these comorbidities ( 21 ).
This issue of the Journal presents new findings relevant to the issues discussed above in relation to understanding and treating anxiety and depressive disorders. Drs. Conor Liston and Timothy Spellman, from Weill Cornell Medicine, provide an overview for this issue ( 22 ) that is focused on understanding mechanisms at the neural circuit level that underlie the pathophysiology of depression. Their piece nicely integrates human neuroimaging studies with complementary data from animal models that allow for the manipulation of selective circuits to test hypotheses generated from the human data. Also included in this issue is a review of the data addressing the reemergence of the use of psychedelic drugs in psychiatry, particularly for the treatment of depression, anxiety, and PTSD ( 23 ). This timely piece, authored by Dr. Collin Reiff along with a subgroup from the APA Council of Research, provides the current state of evidence supporting the further exploration of these interventions. Dr. Alan Schatzberg, from Stanford University, contributes an editorial in which he comments on where the field is in relation to clinical trials with psychedelics and to some of the difficulties, such as adequate blinding, in reliably studying the efficacy of these drugs ( 24 ).
In an article by McTeague et al. ( 25 ), the authors use meta-analytic strategies to understand the neural alterations that are related to aberrant emotion processing that are shared across psychiatric disorders. Findings support alterations in the salience, reward, and lateral orbital nonreward networks as common across disorders, including anxiety and depressive disorders. These findings add to the growing body of work that supports the concept that there are common underlying factors across all types of psychopathology that include internalizing, externalizing, and thought disorder dimensions ( 26 ). Dr. Deanna Barch, from Washington University in St. Louis, writes an editorial commenting on these findings and, importantly, discusses criteria that should be met when we consider whether the findings are actually transdiagnostic ( 27 ).
Another article, from Gray and colleagues ( 28 ), addresses whether there is a convergence of findings, specifically in major depression, when examining data from different structural and functional neuroimaging modalities. The authors report that, consistent with what we know about regions involved in emotion processing, the subgenual anterior cingulate cortex, hippocampus, and amygdala were among the regions that showed convergence across multimodal imaging modalities.
In relation to treatment and building on our understanding of neural circuit alterations, Siddiqi et al. ( 29 ) present data suggesting that transcranial magnetic stimulation (TMS) targeting can be linked to symptom-specific treatments. Their findings identify different TMS targets in the left dorsolateral prefrontal cortex that modulate different downstream networks. The modulation of these different networks appears to be associated with a reduction in different types of symptoms. In an editorial, Drs. Sean Nestor and Daniel Blumberger, from the University of Toronto ( 30 ), comment on the novel approach used in this study to link the TMS-related engagement of circuits with symptom improvement. They also provide a perspective on how we can view these and other circuit-based findings in relation to conceptualizing personalized treatment approaches.
Kendler et al. ( 31 ), in this issue, contribute an article that demonstrates the important role of the rearing environment in the risk to develop major depression. Using a unique design from a Swedish sample, the analytic strategy involves comparing outcomes from high-risk full sibships and high-risk half sibships where at least one of the siblings was home reared and one was adopted out of the home. The findings support the importance of the quality of the rearing environment as well as the presence of parental depression in mitigating or enhancing the likelihood of developing major depression. In an accompanying editorial ( 32 ), Dr. Myrna Weissman, from Columbia University, reviews the methods and findings of the Kendler et al. article and also emphasizes the critical significance of the early nurturing environment in relation to general health.
This issue concludes with an intriguing article on anxiety disorders, by Gold and colleagues ( 33 ), that demonstrates neural alterations during extinction recall that differ in children relative to adults. With increasing age, and in relation to fear and safety cues, nonanxious adults demonstrated greater connectivity between the amygdala and the ventromedial prefrontal cortex compared with anxious adults, as the cues were being perceived as safer. In contrast, neural differences between anxious and nonanxious youths were more robust when rating the memory of faces that were associated with threat. Specifically, these differences were observed in the activation of the inferior temporal cortex. In their editorial ( 34 ), Dr. Dylan Gee and Sahana Kribakaran, from Yale University, emphasize the importance of developmental work in relation to understanding anxiety disorders, place these findings into the context of other work, and suggest the possibility that these and other data point to neuroscientifically informed age-specific interventions.
Taken together, the papers in this issue of the Journal present new findings that shed light onto alterations in neural function that underlie major depressive disorder and anxiety disorders. It is important to remember that these disorders are highly comorbid and that their symptoms are frequently not separable. The papers in this issue also provide a developmental perspective emphasizing the importance of early rearing in the risk to develop depression and age-related findings important for understanding threat processing in patients with anxiety disorders. From a treatment perspective, the papers introduce data supporting more selective prefrontal cortical TMS targeting in relation to different symptoms, address the potential and drawbacks for considering the future use of psychedelics in our treatments, and present new ideas supporting age-specific interventions for youths and adults with anxiety disorders.
Disclosures of Editors’ financial relationships appear in the April 2020 issue of the Journal .
1 Substance Abuse and Mental Health Services Administration (SAMHSA): Key substance use and mental health indicators in the United States: results from the 2017 National Survey on Drug Use and Health (HHS Publication No. SMA 18-5068, NSDUH Series H-53). Rockville, Md, Center for Behavioral Health Statistics and Quality, SAMHSA, 2018. https://www.samhsa.gov/data/sites/default/files/cbhsq-reports/NSDUHFFR2017/NSDUHFFR2017.htm Google Scholar
2 Kessler RC, Chiu WT, Demler O, et al. : Prevalence, severity, and comorbidity of 12-month DSM-IV disorders in the National Comorbidity Survey Replication . Arch Gen Psychiatry 2005 ; 62:617–627, correction, 62:709 Crossref , Medline , Google Scholar
3 Merikangas KR, He JP, Burstein M, et al. : Lifetime prevalence of mental disorders in U.S. adolescents: results from the National Comorbidity Survey Replication–Adolescent Supplement (NCS-A) . J Am Acad Child Adolesc Psychiatry 2010 ; 49:980–989 Crossref , Medline , Google Scholar
4 Kessler RC, McGonagle KA, Zhao S, et al. : Lifetime and 12-month prevalence of DSM-III-R psychiatric disorders in the United States: results from the National Comorbidity Survey . Arch Gen Psychiatry 1994 ; 51:8–19 Crossref , Medline , Google Scholar
5 Kessler RC, Sampson NA, Berglund P, et al. : Anxious and non-anxious major depressive disorder in the World Health Organization World Mental Health Surveys . Epidemiol Psychiatr Sci 2015 ; 24:210–226 Crossref , Medline , Google Scholar
6 Dunner DL : Management of anxiety disorders: the added challenge of comorbidity . Depress Anxiety 2001 ; 13:57–71 Crossref , Medline , Google Scholar
7 Kessler RC, Sonnega A, Bromet E, et al. : Posttraumatic stress disorder in the National Comorbidity Survey . Arch Gen Psychiatry 1995 ; 52:1048–1060 Crossref , Medline , Google Scholar
8 Brawman-Mintzer O, Lydiard RB, Emmanuel N, et al. : Psychiatric comorbidity in patients with generalized anxiety disorder . Am J Psychiatry 1993 ; 150:1216–1218 Link , Google Scholar
9 Fava M, Alpert JE, Carmin CN, et al. : Clinical correlates and symptom patterns of anxious depression among patients with major depressive disorder in STAR*D . Psychol Med 2004 ; 34:1299–1308 Crossref , Medline , Google Scholar
10 Hettema JM : What is the genetic relationship between anxiety and depression? Am J Med Genet C Semin Med Genet 2008 ; 148C:140–146 Crossref , Medline , Google Scholar
11 Hettema JM, Neale MC, Myers JM, et al. : A population-based twin study of the relationship between neuroticism and internalizing disorders . Am J Psychiatry 2006 ; 163:857–864 Link , Google Scholar
12 Kovner R, Oler JA, Kalin NH : Cortico-limbic interactions mediate adaptive and maladaptive responses relevant to psychopathology . Am J Psychiatry 2019 ; 176:987–999 Link , Google Scholar
13 Etkin A, Schatzberg AF : Common abnormalities and disorder-specific compensation during implicit regulation of emotional processing in generalized anxiety and major depressive disorders . Am J Psychiatry 2011 ; 168:968–978 Link , Google Scholar
14 Goodkind M, Eickhoff SB, Oathes DJ, et al. : Identification of a common neurobiological substrate for mental illness . JAMA Psychiatry 2015 ; 72:305–315 Crossref , Medline , Google Scholar
15 McTeague LM, Huemer J, Carreon DM, et al. : Identification of common neural circuit disruptions in cognitive control across psychiatric disorders . Am J Psychiatry 2017 ; 174:676–685 Link , Google Scholar
16 Beesdo K, Knappe S, Pine DS : Anxiety and anxiety disorders in children and adolescents: developmental issues and implications for DSM-V . Psychiatr Clin North Am 2009 ; 32:483–524 Crossref , Medline , Google Scholar
17 Kessler RC, Wang PS : The descriptive epidemiology of commonly occurring mental disorders in the United States . Annu Rev Public Health 2008 ; 29:115–129 Crossref , Medline , Google Scholar
18 Ohayon MM, Schatzberg AF : Social phobia and depression: prevalence and comorbidity . J Psychosom Res 2010 ; 68:235–243 Crossref , Medline , Google Scholar
19 Clauss JA, Blackford JU : Behavioral inhibition and risk for developing social anxiety disorder: a meta-analytic study . J Am Acad Child Adolesc Psychiatry 2012 ; 51:1066–1075 Crossref , Medline , Google Scholar
20 Fava M, Rush AJ, Alpert JE, et al. : Difference in treatment outcome in outpatients with anxious versus nonanxious depression: a STAR*D report . Am J Psychiatry 2008 ; 165:342–351 Link , Google Scholar
21 Dold M, Bartova L, Souery D, et al. : Clinical characteristics and treatment outcomes of patients with major depressive disorder and comorbid anxiety disorders: results from a European multicenter study . J Psychiatr Res 2017 ; 91:1–13 Crossref , Medline , Google Scholar
22 Spellman T, Liston C : Toward circuit mechanisms of pathophysiology in depression . Am J Psychiatry 2020 ; 177:381–390 Link , Google Scholar
23 Reiff CM, Richman EE, Nemeroff CB, et al. : Psychedelics and psychedelic-assisted psychotherapy . Am J Psychiatry 2020 ; 177:391–410 Link , Google Scholar
24 Schatzberg AF : Some comments on psychedelic research (editorial). Am J Psychiatry 2020 ; 177:368–369 Link , Google Scholar
25 McTeague LM, Rosenberg BM, Lopez JW, et al. : Identification of common neural circuit disruptions in emotional processing across psychiatric disorders . Am J Psychiatry 2020 ; 177:411–421 Link , Google Scholar
26 Caspi A, Moffitt TE : All for one and one for all: mental disorders in one dimension . Am J Psychiatry 2018 ; 175:831–844 Link , Google Scholar
27 Barch DM : What does it mean to be transdiagnostic and how would we know? (editorial). Am J Psychiatry 2020 ; 177:370–372 Abstract , Google Scholar
28 Gray JP, Müller VI, Eickhoff SB, et al. : Multimodal abnormalities of brain structure and function in major depressive disorder: a meta-analysis of neuroimaging studies . Am J Psychiatry 2020 ; 177:422–434 Link , Google Scholar
29 Siddiqi SH, Taylor SF, Cooke D, et al. : Distinct symptom-specific treatment targets for circuit-based neuromodulation . Am J Psychiatry 2020 ; 177:435–446 Link , Google Scholar
30 Nestor SM, Blumberger DM : Mapping symptom clusters to circuits: toward personalizing TMS targets to improve treatment outcomes in depression (editorial). Am J Psychiatry 2020 ; 177:373–375 Abstract , Google Scholar
31 Kendler KS, Ohlsson H, Sundquist J, et al. : The rearing environment and risk for major depression: a Swedish national high-risk home-reared and adopted-away co-sibling control study . Am J Psychiatry 2020 ; 177:447–453 Abstract , Google Scholar
32 Weissman MM : Is depression nature or nurture? Yes (editorial). Am J Psychiatry 2020 ; 177:376–377 Abstract , Google Scholar
33 Gold AL, Abend R, Britton JC, et al. : Age differences in the neural correlates of anxiety disorders: an fMRI study of response to learned threat . Am J Psychiatry 2020 ; 177:454–463 Link , Google Scholar
34 Gee DG, Kribakaran S : Developmental differences in neural responding to threat and safety: implications for treating youths with anxiety (editorial). Am J Psychiatry 2020 ; 177:378–380 Abstract , Google Scholar
- Cited by None
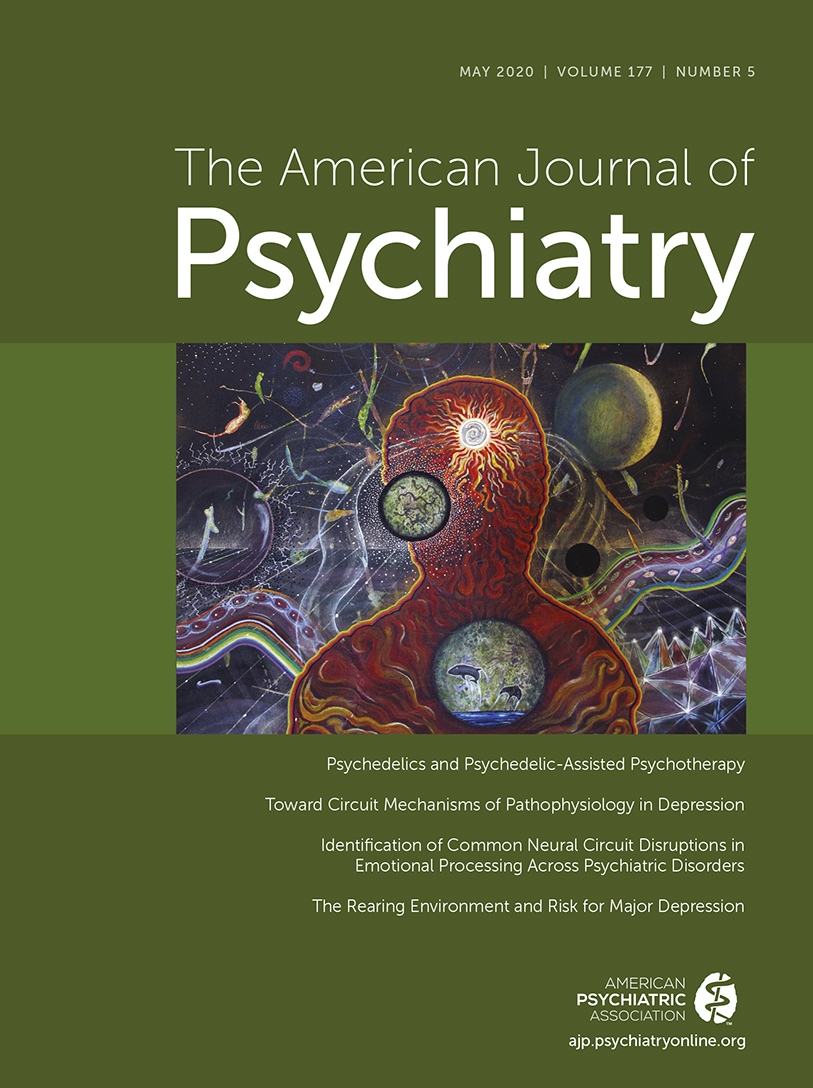
- Neuroanatomy
- Neurochemistry
- Neuroendocrinology
- Other Research Areas
- Search Menu
- Advance Articles
- Editor's Choice
- Supplements
- E-Collections
- Virtual Roundtables
- Author Videos
- Author Guidelines
- Submission Site
- Open Access Options
- About The European Journal of Public Health
- About the European Public Health Association
- Editorial Board
- Advertising and Corporate Services
- Journals Career Network
- Self-Archiving Policy
- Terms and Conditions
- Explore Publishing with EJPH
- Journals on Oxford Academic
- Books on Oxford Academic
E-collection: Public Mental Health
Mental health and mental disorder in the european journal of public health.
Jutta Lindert President of the EUPHA Section on Public Mental Health
Mental Health and mental disorder including suicide and suicidal behavior have been a neglected issue in Public Health for many years. Yet, mental disorders rank among the disorders which contribute enormous suffering for affected persons and their families, high burden of disability adjusted life years (DALYS), and high economic and societal direct and indirect costs. People with severe mental illness have increased risk for premature mortality and thus a shorter life expectancy ( Ösby U ).
The fact that mental disorders are the leading causes of the burden of disease make research in mental disorders and policies to promote mental health a Public Health priority, worldwide. More knowledge on the scope and extent of mental health and mental disorders, the relationship between mental health and mental disorder and the determinants of mental health and mental disorder is highly needed. As examples of determinants a variety of determinants of mental disorders (e.g., economic, social factors, relationship factors, factors related to the physical environment) have been identified. Economic factors such as relative deprivation ( Gunnarsdóttir H ), social factors such as social adversities ( Rajaleid K ) and working related factors, relationship factors such as violence and victimization, and factors related to the physical environment have been identified.
Social adversities over the life course have not only short term but also long-term effects on mental health and social adversities in adolescence predict trajectories of internalized mental ill-health symptoms. Working related factors related to mental disorders are employment status ( Katikireddi S ), working conditions ( Kouvonen A ), and employment history ( von Bonsdorff MB ). In the study from the Netherlands by von Bonsdorf discontinuous employment during mid-career was associated with poorer self-reported physical and mental functioning around the age of retirement. Herewith the long term effects of exposures to social adversities such as financial stress and interrupted employment histories were highlighted. Many studies have investigated how unemployment history influences health, less attention has been paid to the reverse causal direction; how health may influence the risk of employment history and the risk of becoming unemployed. However, an interrupted employment history might be both an indicator for mental disorders and a determinant of mental disorders as people with poor mental and physical health are at increased risk of job loss. ( Kaspersen SL )
Additionally, and importantly, studies investigated relationship factors and mental disorders and mental health. Relationships might include relationships between individuals, groups and communities. A study by Palm et al. showed the significant impact violence and abuse has on women`s mental health. In this study young women visiting youth health centers in Sweden answered a questionnaire constructed from standardized instruments addressing violence victimization (emotional, physical, sexual and family violence), socio-demographics, substance use and physical and mental health ( Palm A ). Yet the relationships between violence and health need further investigation, might it be the impact of war on mental health ( Lindert J ) or the impact of family relationships, physical abuse and early adversities, gun violence, domestic violence, bullying and cyber-bullying?
Besides economic, social or relationship factors environment related factors may have a significant contribution for mental health, such as exposure to asbestos. The results obtained in the Asbestos-Related Diseases Cohort (ARDCO) study confirm that environment related factors need to be investigated and linked to the field of Public Mental Health. ( Mounchetrou Njoya I ).
Yet the relationships of mental health and mental disorders need further investigation. However, we need more longitudinal population based studies on trajectories of mental disorders, determinants and mechanisms of mental health and mental disorders and how positive trajectories of mental health can be supported. If we want to promote mental health, reduce mental disorders and improve Public Mental Health, we need to produce studies with data from more countries ( Bøe T ). Studies published in the EJPH on mental disorder and mental health trajectories are good examples and will allow us not only to better understand variations between and within countries but mental disorders' trajectories and develop effective and cost-effective interventions.
Financial difficulties in childhood and adult depression in Europe Tormod Bøe, Mirza Balaj, Terje A. Eikemo, Courtney L. McNamara, Erling F. Solheim Eur J Public Health (2017) 27 (suppl_1): 96-101.
Suicide mortality in Belgium at the beginning of the 21st century: differences according to migrant background Mariska Bauwelinck, Patrick Deboosere, Didier Willaert, Hadewijch Vandenheede Eur J Public Health (2017) 27 (1): 111-111
Relative deprivation in the Nordic countries-child mental health problems in relation to parental financial stress Hrafnhildur Gunnarsdóttir, Gunnel Hensing, Lene Povlsen, Max Petzold Eur J Public Health (2016) 26 (2): 277-282
Health and unemployment: 14 years of follow-up on job loss in the Norwegian HUNT Study Silje L Kaspersen, Kristine Pape, Gunnhild Å. Vie, Solveig O. Ose, Steinar Krokstad, David Gunnell, Johan H. Bjørngaard Eur J Public Health (2016) 26 (2): 312-317.
Employment status and income as potential mediators of educational inequalities in population mental health Srinivasa Vittal Katikireddi, Claire L. Niedzwiedz CL, Frank Popham Eur J Public Health (2016) 26 (5): 814-816
Changes in psychosocial and physical working conditions and common mental disorders Anne Kouvonen, Minna Mänty, Tea Lallukka, Eero Lahelma, Ossi Rahkonen Eur J Public Health (2016). pii: ckw019. [Epub ahead of print])
Refugees mental health-A public mental health challenge Jutta Lindert, Mauro G. Carta, Ingo Schäfer, Richard F. Mollica Eur J Public Health (2016) 26 (3): 374-375
Anxious and depressive symptoms in the French Asbestos Related Diseases Cohort: risk factors and self-perception of risk Ibrahim Mounchetrou Njoya, Christophe Paris, Jerome Dinet, Amadine Luc, Joelle Lighezzolo-Alnot, Jean-Claude Pairon, Isabelle Thaon Eur J Public Health (2017) 27 (2): 359-366
Mortality trends in cardiovascular causes in schizophrenia, bipolar and unipolar mood disorder in Sweden 1987-2010 Urban Ösby, Jeanette Westman, Jonas Hällgren, Mika Gissler Eur J Public Health (2016) 26 (5): 867-871
Violence victimisation-a watershed for young women's mental and physical health Anna Palm, Ingela Danielsson, Alkistis Skalkidou, Niclas Olofsson, Ulf Högberg Eur J Public Health (2016) 26 (5): 861-867
Social adversities in adolescence predict unfavourable trajectories of internalized mental health symptoms until middle age: results from the Northern Swedish Cohort Kristiina Rajaleid, Tapio Nummi, Hugo Westerlund, Pekka Virtanen, Per E. Gustafsson, Anne Hammarström Eur J Public Health (2016) 26 (1): 23-29
Mid-career work patterns and physical and mental functioning at age 60-64: evidence from the 1946 British birth cohort Mikaela B. von Bonsdorff, Diana Kuh, Monika E. von Bonsdorff, Rachel Cooper Eur J Public Health (2016) 26 (3): 486-491
- Contact EUPHA
- Recommend to your Library
Affiliations
- Online ISSN 1464-360X
- Print ISSN 1101-1262
- Copyright © 2024 European Public Health Association
- About Oxford Academic
- Publish journals with us
- University press partners
- What we publish
- New features
- Open access
- Institutional account management
- Rights and permissions
- Get help with access
- Accessibility
- Advertising
- Media enquiries
- Oxford University Press
- Oxford Languages
- University of Oxford
Oxford University Press is a department of the University of Oxford. It furthers the University's objective of excellence in research, scholarship, and education by publishing worldwide
- Copyright © 2024 Oxford University Press
- Cookie settings
- Cookie policy
- Privacy policy
- Legal notice
This Feature Is Available To Subscribers Only
Sign In or Create an Account
This PDF is available to Subscribers Only
For full access to this pdf, sign in to an existing account, or purchase an annual subscription.
Thank you for visiting nature.com. You are using a browser version with limited support for CSS. To obtain the best experience, we recommend you use a more up to date browser (or turn off compatibility mode in Internet Explorer). In the meantime, to ensure continued support, we are displaying the site without styles and JavaScript.
- View all journals
- My Account Login
- Explore content
- About the journal
- Publish with us
- Sign up for alerts
- Review Article
- Open access
- Published: 08 April 2022
Natural language processing applied to mental illness detection: a narrative review
- Tianlin Zhang ORCID: orcid.org/0000-0003-0843-1916 1 ,
- Annika M. Schoene 1 ,
- Shaoxiong Ji ORCID: orcid.org/0000-0003-3281-8002 2 &
- Sophia Ananiadou 1 , 3
npj Digital Medicine volume 5 , Article number: 46 ( 2022 ) Cite this article
42k Accesses
82 Citations
54 Altmetric
Metrics details
- Disease prevention
- Psychiatric disorders
Mental illness is highly prevalent nowadays, constituting a major cause of distress in people’s life with impact on society’s health and well-being. Mental illness is a complex multi-factorial disease associated with individual risk factors and a variety of socioeconomic, clinical associations. In order to capture these complex associations expressed in a wide variety of textual data, including social media posts, interviews, and clinical notes, natural language processing (NLP) methods demonstrate promising improvements to empower proactive mental healthcare and assist early diagnosis. We provide a narrative review of mental illness detection using NLP in the past decade, to understand methods, trends, challenges and future directions. A total of 399 studies from 10,467 records were included. The review reveals that there is an upward trend in mental illness detection NLP research. Deep learning methods receive more attention and perform better than traditional machine learning methods. We also provide some recommendations for future studies, including the development of novel detection methods, deep learning paradigms and interpretable models.
Similar content being viewed by others
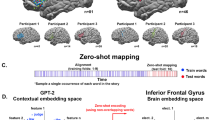
Alignment of brain embeddings and artificial contextual embeddings in natural language points to common geometric patterns
Ariel Goldstein, Avigail Grinstein-Dabush, … Uri Hasson
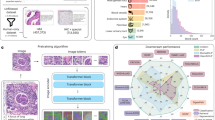
A visual-language foundation model for computational pathology
Ming Y. Lu, Bowen Chen, … Faisal Mahmood
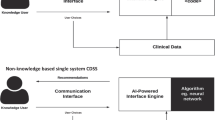
An overview of clinical decision support systems: benefits, risks, and strategies for success
Reed T. Sutton, David Pincock, … Karen I. Kroeker
Introduction
Mental illnesses, also called mental health disorders, are highly prevalent worldwide, and have been one of the most serious public health concerns 1 . There are many different mental illnesses, including depression, suicidal ideation, bipolar disorder, autism spectrum disorder (ASD), anxiety disorder, schizophrenia, etc., any of which can have a negative influence on an individual’s physical health and well-being with the problem exacerbated due to Covid-19 2 . According to the latest statistics, millions of people worldwide suffer from one or more mental disorders 1 . If mental illness is detected at an early stage, it can be beneficial to overall disease progression and treatment.
There are different text types, in which people express their mood, such as social media messages on social media platforms, transcripts of interviews and clinical notes including the description of patients’ mental states. In recent years, natural language processing (NLP), a branch of artificial intelligence (AI) technologies, has played an essential role in supporting the analysis and management of large scale textual data and facilitating various tasks such as information extraction, sentiment analysis 3 , emotion detection, and mental health surveillance 4 , 5 , 6 . Detecting mental illness from text can be cast as a text classification or sentiment analysis task, where we can leverage NLP techniques to automatically identify early indicators of mental illness to support early detection, prevention and treatment.
Existing reviews introduce mainly the computational methods for mental health illness detection, they mostly focus on specific mental illnesses (suicide 7 , 8 , 9 , depression 10 , 11 , 12 ), or specific data sources (social media 13 , 14 , 15 , non-clinical texts 16 ). To the best of our knowledge, there is no review of NLP techniques applied to mental illness detection from textual sources recently. We present a broader scope of mental illness detection using NLP that covers a decade of research, different types of mental illness and a variety of data sources. Our review aims to provide a comprehensive overview of the latest trends and recent NLP methodologies used for text-based mental illness detection, and also points at the future challenges and directions. Our review seeks to answer the following questions:
What are the main NLP trends and approaches for mental illness detection?
Which features have been used for mental health detection in traditional machine learning-based models?
Which neural architectures have been commonly used to detect mental illness?
What are the main challenges and future directions in NLP for mental illness?
Search methodology
Search strategy.
A comprehensive search was conducted in multiple scientific databases for articles written in English and published between January 2012 and December 2021. The databases include PubMed, Scopus, Web of Science, DBLP computer science bibliography, IEEE Xplore, and ACM Digital Library.
The search query we used was based on four sets of keywords shown in Table 1 . For mental illness, 15 terms were identified, related to general terms for mental health and disorders (e.g., mental disorder and mental health), and common specific mental illnesses (e.g., depression, suicide, anxiety). For data source, we searched for general terms about text types (e.g., social media, text, and notes) as well as for names of popular social media platforms, including Twitter and Reddit. The methods and detection sets refer to NLP methods used for mental illness identification.
The keywords of each sets were combined using Boolean operator “OR", and the four sets were combined using Boolean operator “AND". We conducted the searches in December 2021.
Filtering strategy
A total of 10,467 bibliographic records were retrieved from six databases, of which 7536 records were retained after removing duplication. Then, we used RobotAnalyst 17 , a tool that minimizes the human workload involved in the screening phase of reviews, by prioritizing the most relevant articles for mental illness based on relevancy feedback and active learning 18 , 19 .
Each of the 7536 records was screened based on title and abstract. Records were removed if the following exclusion criteria were met: (1) the full text was not available in English; (2) the abstract was not relevant to mental illness detection; (3) the method did not use textual experimental data, but speech or image data.
After the screening process, 611 records were retained for further review. An additional manual full-text review was conducted to retain only articles focusing on the description of NLP methods only. The final inclusion criteria were established as follow:
Articles must study textual data such as contents from social media, electronic health records or transcription of interviews.
They must focus on NLP methods for mental illness detection, including machine learning-based methods (in this paper, the machine learning methods refer to traditional feature engineering-based machine learning) and deep learning-based methods. We exclude review and data analysis papers.
They must provide a methodology contribution by (1) proposing a new feature extraction method, a neural architecture, or a novel NLP pipeline; or (2) applying the learning methods to a specific mental health detection domain or task.
Following the full-text screening process, 399 articles were selected. The flow diagram of the article selection process is shown in Fig. 1 .
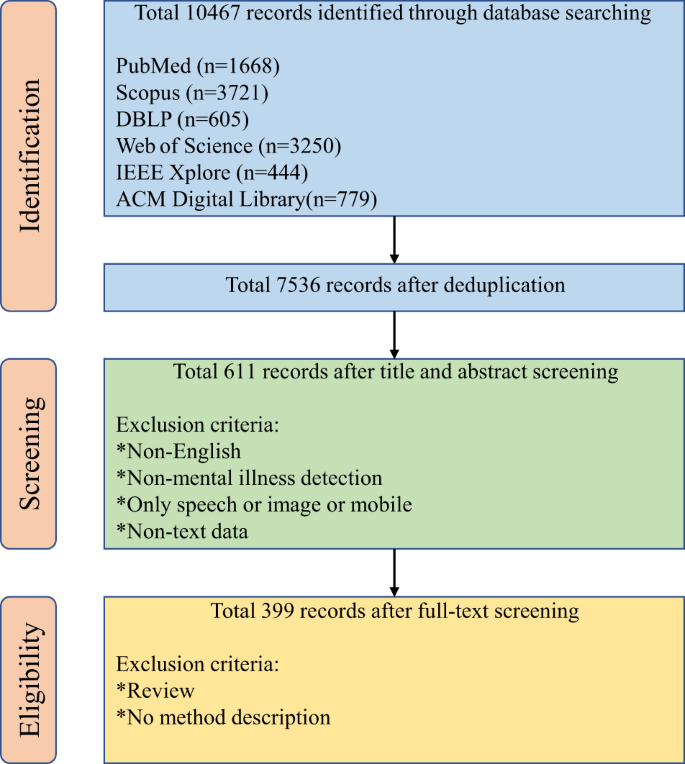
Six databases (PubMed, Scopus, Web of Science, DBLP computer science bibliography, IEEE Xplore, and ACM Digital Library) were searched. The flowchart lists reasons for excluding the study from the data extraction and quality assessment.
Data extraction
For each selected article, we extracted the following types of metadata and other information:
Year of publication.
The aim of research.
The dataset used, including type of mental illness (e.g., depression, suicide, and eating disorder), language, and data sources (e.g., Twitter, electronic health records (EHRs) and interviews).
The NLP method (e.g., machine learning and deep learning) and types of features used (e.g., semantic, syntactic, and topic).
We show in Fig. 2 the number of publications retrieved and the methods used in our review, reflecting the trends of the past 10 years. We can observe that: (1) there is an upward trend in NLP-driven mental illness detection research, suggesting the great research value and prospects for automatic mental illness detection from text (2) deep learning-based methods have increased in popularity in the last couple of years.
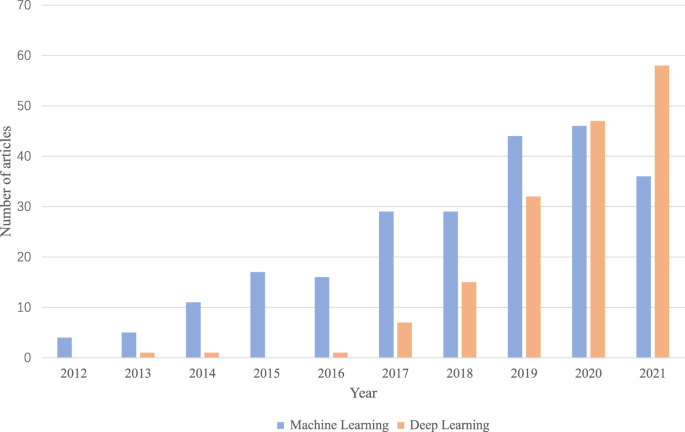
The trend of the number of articles containing machine learning-based and deep learning-based methods for detecting mental illness from 2012 to 2021.
In the following subsections, we provide an overview of the datasets and the methods used. In section Datesets, we introduce the different types of datasets, which include different mental illness applications, languages and sources. Section NLP methods used to extract data provides an overview of the approaches and summarizes the features for NLP development.
In order to better train mental illness detection models, reliable and accurate datasets are necessary. There are several sources from which we can collect text data related to mental health, including social media posts, screening surveys, narrative writing, interviews and EHRs. At the same time, for different detection tasks, the datasets also differ in the types of illness they focus on and language. We show a comprehensive mapping of each method with its associated application using a Sankey diagram (Fig. 3 ).
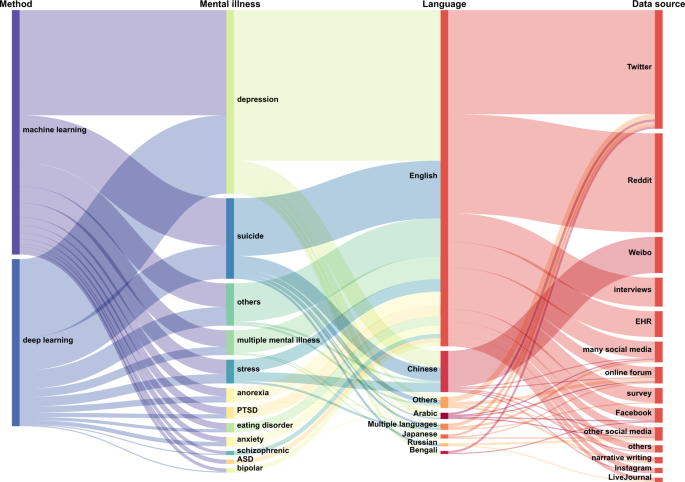
The different methods with their associated application are represented via flows. Nodes are represented as rectangles, and the height represents their value. The width of each curved line is proportional to their values.
Data sources
Figure 4 illustrates the distribution of the different data sources. It can be seen that, among the 399 reviewed papers, social media posts (81%) constitute the majority of sources, followed by interviews (7%), EHRs (6%), screening surveys (4%), and narrative writing (2%).
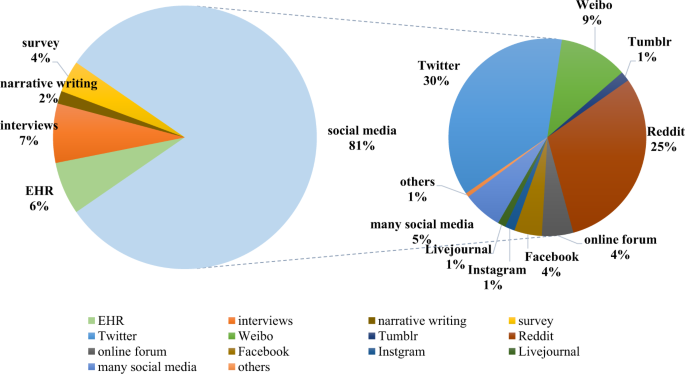
The pie chart depicts the percentages of different textual data sources based on their numbers.
Social media posts
The use of social media has become increasingly popular for people to express their emotions and thoughts 20 . In addition, people with mental illness often share their mental states or discuss mental health issues with others through these platforms by posting text messages, photos, videos and other links. Prominent social media platforms are Twitter, Reddit, Tumblr, Chinese microblogs, and other online forums. We briefly introduce some popular social media platforms.
Twitter. Twitter is a popular social networking service with over 300 million active users monthly, in which users can post their tweets (the posts on Twitter) or retweet others’ posts. Researchers can collect tweets using available Twitter application programming interfaces (API). For example, Sinha et al. created a manually annotated dataset to identify suicidal ideation in Twitter 21 . Hu et al. used a rule-based approach to label users’ depression status from the Twitter 22 . However, normally Twitter does not allow the texts of downloaded tweets to be publicly shared, only the tweet identifiers—some/many of which may then disappear over time, so many datasets of actual tweets are not made publicly available 23 .
Reddit . Reddit is also a popular social media platform for publishing posts and comments. The difference between Reddit and other data sources is that posts are grouped into different subreddits according to the topics (i.e., depression and suicide). Because of Reddit’s open policy, their datasets are publicly available. Yates et al. established a depression dataset named “Reddit Self-reported Depression Diagnosis" (RSDD) 24 , which contains about 9k depressed users and 100k control users. Similarly, CLEF risk 2019 shared task 25 also proposed an anorexia and self-harm detection task based on the Reddit platform.
Online forums. People can discuss their mental health conditions and seek mental help from online forums (also called online communities). There are various forms of online forums, such as chat rooms, discussion rooms (recoveryourlife, endthislife). For example, Saleem et al. designed a psychological distress detection model on 512 discussion threads downloaded from an online forum for veterans 26 . Franz et al. used the text data from TeenHelp.org, an Internet support forum, to train a self-harm detection system 27 .
Electronic health records
EHRs, a rich source of secondary health care data, have been widely used to document patients’ historical medical records 28 . EHRs often contain several different data types, including patients’ profile information, medications, diagnosis history, images. In addition, most EHRs related to mental illness include clinical notes written in narrative form 29 . Therefore, it is appropriate to use NLP techniques to assist in disease diagnosis on EHRs datasets, such as suicide screening 30 , depressive disorder identification 31 , and mental condition prediction 32 .
Some work has been carried out to detect mental illness by interviewing users and then analyzing the linguistic information extracted from transcribed clinical interviews 33 , 34 . The main datasets include the DAIC-WoZ depression database 35 that involves transcriptions of 142 participants, the AViD-Corpus 36 with 48 participants, and the schizophrenic identification corpus 37 collected from 109 participants.
Screening surveys
In order to evaluate participants’ mental health conditions, some researchers post questionnaires for clinician-patient diagnosis of patients or self-measurement. After participants are asked to fill in a survey from crowd-sourcing platforms (like Crowd Flower, Amazon’s Mechanical Turk) or online platforms, the data is collected and labeled. There are different survey contents to measure different psychiatric symptoms. For depression, the PHQ-9 (Patient Health Questionnaire) 38 or Beck Depression Inventory (BDI) questionnaire 39 are widely used for assessing the severity of depressive symptoms. The Scale Center for Epidemiological Studies Depression Scale (CES-D) questionnaire 40 with 20 multiple-choice questions is also designed for testing depression. For suicide ideation, there are many questionnaires such as the Holmes-Rahe Social Readjustment Rating Scale (SRRS) 41 or the Depressive Symptom Inventory-Suicide Subscale (DSI-SS) 42 .
Narrative writing
There are other types of texts written for specific experiments, as well as narrative texts that are not published on social media platforms, which we classify as narrative writing. For example, in one study, children were asked to write a story about a time that they had a problem or fought with other people, where researchers then analyzed their personal narrative to detect ASD 43 . In addition, a case study on Greek poetry of the 20th century was carried out for predicting suicidal tendencies 44 .
Types of mental illness
There are many applications for the detection of different types of mental illness, where depression (45%) and suicide (20%) account for the largest proportion; stress, anorexia, eating disorders, PTSD, bipolar disorder, anxiety, ASD and schizophrenia have corresponding datasets and have been analyzed using NLP (Fig. 5 ). This shows that there is a demand for NLP technology in different mental illness detection applications.
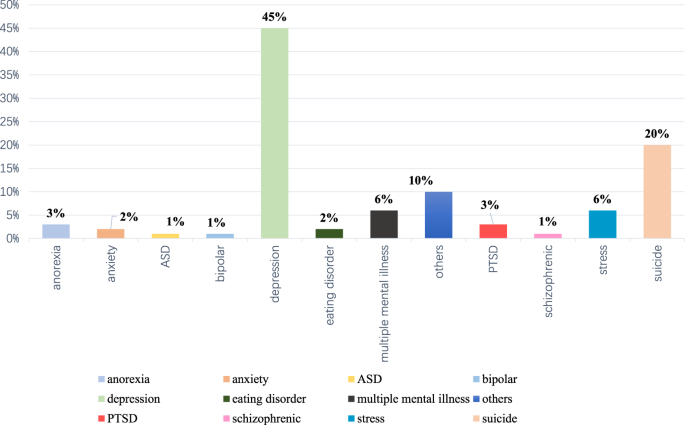
The chart depicts the percentages of different mental illness types based on their numbers.
The amount of datasets in English dominates (81%), followed by datasets in Chinese (10%), Arabic (1.5%). When using non-English language datasets, the main difference lies in the pre-processing pipline, such as word segmentation, sentence splitting and other language-dependent text processing, while the methods and model architectures are language-agnostic.
NLP methods used to extract data
Machine learning methods.
Traditional machine learning methods such as support vector machine (SVM), Adaptive Boosting (AdaBoost), Decision Trees, etc. have been used for NLP downstream tasks. Figure 3 shows that 59% of the methods used for mental illness detection are based on traditional machine learning, typically following a pipeline approach of data pre-processing, feature extraction, modeling, optimization, and evaluation.
In order to train a good ML model, it is important to select the main contributing features, which also help us to find the key predictors of illness. Table 2 shows an overview of commonly used features in machine learning. We further classify these features into linguistic features, statistical features, domain knowledge features, and other auxiliary features. The most frequently used features are mainly based on basic linguistic patterns (Part-of-Speech (POS) 45 , 46 , 47 , Bag-of-words (BoW) 48 , 49 , 50 , Linguistic Inquiry and Word Count (LIWC) 51 , 52 , 53 ) and statistics (n-gram 54 , 55 , 56 , term frequency-inverse document frequency (TF-IDF) 57 , 58 , 59 , length of sentences or passages 60 , 61 , 62 ) because these features can be easily obtained through text processing tools and are widely used in many NLP tasks. Furthermore, emotion and topic features have been shown empirically to be effective for mental illness detection 63 , 64 , 65 . Domain specific ontologies, dictionaries and social attributes in social networks also have the potential to improve accuracy 65 , 66 , 67 , 68 . Research conducted on social media data often leverages other auxiliary features to aid detection, such as social behavioral features 65 , 69 , user’s profile 70 , 71 , or time features 72 , 73 .
Machine learning models have been designed based on a combination of various extracted features. The majority of the papers based on machine learning methods used supervised learning, where they described one or more methods employed to detect mental illness: SVM 26 , 74 , 75 , 76 , 77 , Adaptive Boosting (AdaBoost) 71 , 78 , 79 , 80 , k-Nearest Neighbors (KNN) 38 , 81 , 82 , 83 , Decision Tree 84 , 85 , 86 , 87 , Random Forest 75 , 88 , 89 , 90 , Logistic Model Tree (LMT) 47 , 47 , 91 , 92 , Naive Bayes (NB) 64 , 86 , 93 , 94 , Logistic Regression 37 , 95 , 96 , 97 , XGBoost 38 , 55 , 98 , 99 , and some ensemble models combining several methods 75 , 100 , 101 , 102 . The advantage of such supervised learning lies in the model’s ability to learn patterns from labeled data, thus ensuring better performance. However, labeling the large amount of data at a high quality level is time-consuming and challenging, although there are methods that help reduce the human annotation burden 103 . Thus, we need to use other methods which do not rely on labeled data or need only a small amount of data to train a classifier.
Unsupervised learning methods to discover patterns from unlabeled data, such as clustering data 55 , 104 , 105 , or by using LDA topic model 27 . However, in most cases, we can apply these unsupervised models to extract additional features for developing supervised learning classifiers 56 , 85 , 106 , 107 . Across all papers, few papers 108 , 109 used semi-supervised learning (models trained from large unlabeled data as additional information), including statistical model ssToT (semi-supervised topic modeling over time) 108 and classic semi-supervised algorithms (YATSI 110 and LLGC 111 ).
Deep learning methods
As mentioned above, machine learning-based models rely heavily on feature engineering and feature extraction. Using deep learning frameworks allows models to capture valuable features automatically without feature engineering, which helps achieve notable improvements 112 . Advances in deep learning methods have brought breakthroughs in many fields including computer vision 113 , NLP 114 , and signal processing 115 . For the task of mental illness detection from text, deep learning techniques have recently attracted more attention and shown better performance compared to machine learning ones 116 .
Deep learning-based frameworks mainly contain two layers: an embedding layer and a classification layer. By using an embedding layer, the inputs are embedded from sparse one-hot encoded vectors (where only one member of a vector is ‘1’, all others are ‘0’, leading to the sparsity) into dense vectors which can preserve semantic and syntactic information such that deep learning models can be better trained 117 . There are many different embedding techniques, such as ELMo, GloVe word embedding 118 , word2vec 119 and contextual language encoder representations (e.g., bidirectional encoder representations from transformers (BERT) 120 and ALBERT[ 121 ).
According to the structures of different classification layer’s structures, we have divided the deep learning-based methods into the following categories for this review: convolutional neural networks (CNN)-based methods (17%), recurrent neural networks (RNN)-based methods (36%), transformer-based methods (17%) and hybrid-based methods (30%) that combine multiple neural networks with different structures, as shown in Table 3 .
CNN-based methods. The standard CNN structure is composed of a convolutional layer and a pooling layer, followed by a fully-connected layer. Some studies 122 , 123 , 124 , 125 , 126 , 127 utilized standard CNN to construct classification models, and combined other features such as LIWC, TF-IDF, BOW, and POS. In order to capture sentiment information, Rao et al. proposed a hierarchical MGL-CNN model based on CNN 128 . Lin et al. designed a CNN framework combined with a graph model to leverage tweet content and social interaction information 129 .
RNN-based methods . The architecture of RNNs allows previous outputs to be used as inputs, which is beneficial when using sequential data such as text. Generally, long short-term memory (LSTM) 130 and gated recurrent (GRU) 131 networks models that can deal with the vanishing gradient problem 132 of the traditional RNN are effectively used in NLP field. There are many studies (e.g., 133 , 134 ) based on LSTM or GRU, and some of them 135 , 136 exploited an attention mechanism 137 to find significant word information from text. Some also used a hierarchical attention network based on LSTM or GRU structure to better exploit the different-level semantic information 138 , 139 .
Moreover, many other deep learning strategies are introduced, including transfer learning, multi-task learning, reinforcement learning and multiple instance learning (MIL). Rutowski et al. made use of transfer learning to pre-train a model on an open dataset, and the results illustrated the effectiveness of pre-training 140 , 141 . Ghosh et al. developed a deep multi-task method 142 that modeled emotion recognition as a primary task and depression detection as a secondary task. The experimental results showed that multi-task frameworks can improve the performance of all tasks when jointly learning. Reinforcement learning was also used in depression detection 143 , 144 to enable the model to pay more attention to useful information rather than noisy data by selecting indicator posts. MIL is a machine learning paradigm, which aims to learn features from bags’ labels of the training set instead of individual labels. Wongkoblap et al. used MIL to predict users with depression task 145 , 146 .
Transformer-based methods. Recently, transformer architectures 147 were able to solve long-range dependencies using attention and recurrence. Wang et al. proposed the C-Attention network 148 by using a transformer encoder block with multi-head self-attention and convolution processing. Zhang et al. also presented their TransformerRNN with multi-head self-attention 149 . Additionally, many researchers leveraged transformer-based pre-trained language representation models, including BERT 150 , 151 , DistilBERT 152 , Roberta 153 , ALBERT 150 , BioClinical BERT for clinical notes 31 , XLNET 154 , and GPT model 155 . The usage and development of these BERT-based models prove the potential value of large-scale pre-training models in the application of mental illness detection.
Hybrid-based methods. Some methods combining several neural networks for mental illness detection have been used. For examples, the hybrid frameworks of CNN and LSTM models 156 , 157 , 158 , 159 , 160 are able to obtain both local features and long-dependency features, which outperform the individual CNN or LSTM classifiers used individually. Sawhney et al. proposed STATENet 161 , a time-aware model, which contains an individual tweet transformer and a Plutchik-based emotion 162 transformer to jointly learn the linguistic and emotional patterns. Inspired by the improved performance of using sub-emotions representations 163 , Aragon et al. presented a deep emotion attention model 164 which consists of sub-emotion embedding, CNN, GRU as well as an attention mechanism, and Lara et al. also proposed Deep Bag of Sub-Emotions (DeepBose) model 165 . Furthermore, Sawhney et al. introduced the PHASE model 166 , which learns the chronological emotional progression of a user by a new time-sensitive emotion LSTM and also Hyperbolic Graph Convolution Networks 167 . It also learns the chronological emotional spectrum of a user by using BERT fine-tuned for emotions as well as a heterogeneous social network graph.
Evaluation metrics
Evaluation metrics are used to compare the performance of different models for mental illness detection tasks. Some tasks can be regarded as a classification problem, thus the most widely used standard evaluation metrics are Accuracy (AC), Precision (P), Recall (R), and F1-score (F1) 149 , 168 , 169 , 170 . Similarly, the area under the ROC curve (AUC-ROC) 60 , 171 , 172 is also used as a classification metric which can measure the true positive rate and false positive rate. In some studies, they can not only detect mental illness, but also score its severity 122 , 139 , 155 , 173 . Therefore, metrics of mean error (e.g., mean absolute error, mean square error, root mean squared error) 173 and other new metrics (e.g., graded precision, graded recall, average hit rate, average closeness rate, average difference between overall depression levels) 139 , 174 are sometimes needed to indicate the difference between the predicted severity and the actual severity in a dataset. Meanwhile, taking into account the timeliness of mental illness detection, where early detection is significant for early prevention, an error metric called early risk detection error was proposed 175 to measure the delay in decision.
Although promising results have been obtained using both machine and deep learning methods, several challenges remain for the mental illness detection task that require further research. Herein, we introduce some key challenges and future research directions:
Data volume and quality: Most of the methods covered in this review used supervised learning models. The success of these methods is attributed to the number of training datasets available. These training datasets often require human annotation, which is usually a time-consuming and expensive process. However, in the mental illness detection task, there are not enough annotated public datasets. For training reliable models, the quality of datasets is concerning. Some datasets have annotation bias because the annotators can not confirm a definitive action has taken place in relation to a disorder (e.g., if actual suicide has occurred) and can only label them within the constraints of their predefined annotation rules 9 . In addition, some imbalanced datasets have many negative instances (individuals without mental disorders), which is not conducive to training comprehensive and robust models. Therefore, it is important to explore how to train a detection model by using a small quantity of labeled training data or not using training data. Semi-supervised learning 176 incorporates few labeled data and large amounts of unlabeled data into the training process, which can be used to facilitate annotation 177 or improve classification performance when labeled data is scarce. Additionally, unsupervised methods can also be applied in mental disorders detection. For instance, unsupervised topic modeling 178 increases the explainability of results and aids the extraction of latent features for developing further supervised models. 179 , 180
Performance and instability: There are some causes of model instability, including class imbalance, noisy labels, and extremely long or extremely short text samples text. Performance is not robust when training on the datasets from different data sources due to diverse writing styles and semantic heterogeneity. Thus, the performance of some detection models is not good. With the advances of deep learning techniques, various learning techniques have emerged and accelerated NLP research, such as adversarial training 181 , contrastive learning 182 , joint learning 183 , reinforcement learning 184 and transfer learning 185 , which can also be utilized for the mental illness detection task. For example, pre-trained Transformer-based models can be transferred to anorexia detection in Spanish 186 , and reinforcement networks can be used to find the sentence that best reflects the mental state. Other emerging techniques like attention mechanism 187 , knowledge graph 188 , and commonsense reasoning 189 , can also be introduced for textual feature extraction. In addition, feature enrichment and data augmentation 190 are useful to achieve comparable results. For example, many studies use multi-modal data resources, such as image 191 , 192 , 193 , and audio 194 , 195 , 196 , which perform better than the single-modal text-based model.
Interpretability: The goal of representation learning for mental health is to understand the causes or explanatory factors of mental illness in order to boost detection performance and empower decision-making. The evaluation of a successful model does not only rely on performance, but also on its interpretability 197 , which is significant for guiding clinicians to understand not only what has been extracted from text but the reasoning underlying some prediction 198 , 199 , 200 . Deep learning-based methods achieve good performance by utilizing feature extraction and complex neural network structures for illness detection. Nevertheless, they are still treated as black boxes 201 and fail to explain the predictions. Therefore, in future work, the explainability of the deep learning models will become an important research direction.
Ethical considerations: It is of greater importance to discuss ethical concerns when using mental health-related textual data, since the privacy and security of personal data is significant and health data is particularly sensitive. During the research, the researchers should follow strict protocols similar to the guidelines 202 introduced by Bentan et al., to ensure the data is properly applied in healthcare research while protecting privacy to avoid further psychological distress. Furthermore, when using some publicly available data, researchers need to acquire ethical approvals from institutional review boards and human research ethics committees 203 , 204 .
There has been growing research interest in the detection of mental illness from text. Early detection of mental disorders is an important and effective way to improve mental health diagnosis. In our review, we report the latest research trends, cover different data sources and illness types, and summarize existing machine learning methods and deep learning methods used on this task.
We find that there are many applications for different data sources, mental illnesses, even languages, which shows the importance and value of the task. Our findings also indicate that deep learning methods now receive more attention and perform better than traditional machine learning methods.
We discuss some challenges and propose some future directions. In the future, the development of new methods including different learning strategies, novel deep learning paradigms, interpretable models and multi-modal methods will support mental illness detection, with an emphasis on interpretability being crucial for uptake of detection applications by clinicians.
Reporting summary
Further information on research design is available in the Nature Research Reporting Summary linked to this article.
Data availability
The data that support the findings of this study are available from the corresponding author upon reasonable request.
Rehm, J. & Shield, K. D. Global burden of disease and the impact of mental and addictive disorders. Curr. Psychiatry Rep. 21 , 1–7 (2019).
Article Google Scholar
Santomauro, D. F. et al. Global prevalence and burden of depressive and anxiety disorders in 204 countries and territories in 2020 due to the covid-19 pandemic. The Lancet 398 , 1700–1712 (2021).
Nadkarni, P. M., Ohno-Machado, L. & Chapman, W. W. Natural language processing: an introduction. J. Am. Med. Inform. Assoc. 18 , 544–551 (2011).
Article PubMed PubMed Central Google Scholar
Ive, J. Generation and evaluation of artificial mental health records for natural language processing. NPJ Digital Med. 3 , 1–9 (2020).
Mukherjee, S. S. et al. Natural language processing-based quantification of the mental state of psychiatric patients. Comput. Psychiatry 4 , 76–106 (2020).
Jackson, R. G. Natural language processing to extract symptoms of severe mental illness from clinical text: the clinical record interactive search comprehensive data extraction (cris-code) project. BMJ Open 7 , 012012 (2017).
Castillo-Sánchez, G. Suicide risk assessment using machine learning and social networks: a scoping review. J. Med. Syst. 44 , 1–15 (2020).
Franco-Martín, M. A. A systematic literature review of technologies for suicidal behavior prevention. J. Med. Syst. 42 , 1–7 (2018).
Ji, S. Suicidal ideation detection: a review of machine learning methods and applications. IEEE Trans. Comput. Soc. Syst. 8 , 214–226 (2021).
Giuntini, F. T. A review on recognizing depression in social networks: challenges and opportunities. J. Ambient Intell. Human. Comput. 11 , 4713–4729 (2020).
Mahdy, N., Magdi, D. A., Dahroug, A. & Rizka, M. A. Comparative study: different techniques to detect depression using social media. in Internet of Things-Applications and Future, pp. 441–452 (2020).
Khan, A., Husain, M. S. & Khan, A. Analysis of mental state of users using social media to predict depression! a survey. Int. J. Adv. Res. Comput. Sci. 9 , 100–106 (2018).
Google Scholar
Skaik, R. & Inkpen, D. Using social media for mental health surveillance: a review. ACM Comput. Surv. 53 , 1–31 (2020).
Chancellor, S. & De Choudhury, M. Methods in predictive techniques for mental health status on social media: a critical review. NPJ Digital Med. 3 , 1–11 (2020).
Ríssola, E. A., Losada, D. E. & Crestani, F. A survey of computational methods for online mental state assessment on social media. ACM Trans. Comput. Healthc. 2 , 1–31 (2021).
Calvo, R. A., Milne, D. N., Hussain, M. S. & Christensen, H. Natural language processing in mental health applications using non-clinical texts. Nat. Lang. Eng. 23 , 649–685 (2017).
Przybyła, P. Prioritising references for systematic reviews with robotanalyst: a user study. Res. Synth. Methods 9 , 470–488 (2018).
O’Mara-Eves, A., Thomas, J., McNaught, J., Miwa, M. & Ananiadou, S. Using text mining for study identification in systematic reviews: a systematic review of current approaches. Syst. Rev. 4 , 1–22 (2015).
Miwa, M., Thomas, J., O’Mara-Eves, A. & Ananiadou, S. Reducing systematic review workload through certainty-based screening. J. Biomed. Inform. 51 , 242–253 (2014).
Kemp, S. Digital 2020: 3.8 billion people use social media. We Are Social 30 , (2020). https://wearesocial.com/uk/blog/2020/01/digital-2020-3-8-billion-people-use-social-media/ .
Sinha, P. P. et al. Suicidal-a multipronged approach to identify and explore suicidal ideation in twitter. In Proc. 28th ACM International Conference on Information and Knowledge Management , pp. 941–950 (2019).
Hu, P. et al. Bluememo: depression analysis through twitter posts. In IJCAI , pp. 5252–5254 (2020).
Golder, S., Ahmed, S., Norman, G. & Booth, A. Attitudes toward the ethics of research using social media: a systematic review. J. Med. Internet Res. 19 , 7082 (2017).
Yates, A., Cohan, A. & Goharian, N. Depression and self-harm risk assessment in online forums. In Proc. 2017 Conference on Empirical Methods in Natural Language Processing (2017).
Naderi, N., Gobeill, J., Teodoro, D., Pasche, E. & Ruch, P. A baseline approach for early detection of signs of anorexia and self-harm in reddit posts. In CLEF (Working Notes) (2019).
Saleem, S. et al. Automatic detection of psychological distress indicators in online forum posts. In Proc. 2012 Asia Pacific Signal and Information Processing Association Annual Summit and Conference , pp. 1–4 (2012).
Franz, P. J., Nook, E. C., Mair, P. & Nock, M. K. Using topic modeling to detect and describe self-injurious and related content on a large-scale digital platform. Suicide Life Threat. Behav. 50 , 5–18 (2020).
Article PubMed Google Scholar
Menachemi, N. & Collum, T. H. Benefits and drawbacks of electronic health record systems. Risk Manag. Healthc. Policy 4 , 47 (2011).
Kho, A. N. Practical challenges in integrating genomic data into the electronic health record. Genet. Med. 15 , 772–778 (2013).
Downs, J. et al. Detection of suicidality in adolescents with autism spectrum disorders: developing a natural language processing approach for use in electronic health records. In AMIA Annual Symposium Proceedings , vol. 2017, p. 641 (2017).
Kshatriya, B. S. A. et al. Neural language models with distant supervision to identify major depressive disorder from clinical notes. Preprint at arXiv https://arxiv.org/abs/2104.09644 (2021).
Tran, T. & Kavuluru, R. Predicting mental conditions based on “history of present illness" in psychiatric notes with deep neural networks. J. Biomed. Inform. 75 , 138–148 (2017).
Morales, M. R. & Levitan, R. Speech vs. text: a comparative analysis of features for depression detection systems. In 2016 IEEE Spoken Language Technology Workshop (SLT) , pp. 136–143 (2016).
Arseniev-Koehler, A., Mozgai, S. & Scherer, S. What type of happiness are you looking for?-a closer look at detecting mental health from language. In Proc. Fifth Workshop on Computational Linguistics and Clinical Psychology: From Keyboard to Clinic , pp. 1–12 (2018).
Ringeval, F. et al. Avec 2017: real-life depression, and affect recognition workshop and challenge. In Proc. 7th Annual Workshop on Audio/Visual Emotion Challenge , pp. 3–9 (2017).
Valstar, M. et al. Avec 2014: 3d dimensional affect and depression recognition challenge. In Proc. 4th International Workshop on Audio/visual Emotion Challenge , pp. 3–10 (2014).
Voleti, R. et al. Objective assessment of social skills using automated language analysis for identification of schizophrenia and bipolar disorder. In Proc. Interspeech , pp. 1433–1437 (2019).
Tlachac, M., Toto, E. & Rundensteiner, E. You’re making me depressed: Leveraging texts from contact subsets to predict depression. In 2019 IEEE EMBS International Conference on Biomedical & Health Informatics (BHI) , pp. 1–4 (2019).
Stankevich, M., Smirnov, I., Kiselnikova, N. & Ushakova, A. Depression detection from social media profiles. In International Conference on Data Analytics and Management in Data Intensive Domains , pp. 181–194 (2019).
Wongkoblap, A., Vadillo, M. A. & Curcin, V. A multilevel predictive model for detecting social network users with depression. In 2018 IEEE International Conference on Healthcare Informatics (ICHI) , pp. 130–135 (2018).
Delgado-Gomez, D., Blasco-Fontecilla, H., Sukno, F., Ramos-Plasencia, M. S. & Baca-Garcia, E. Suicide attempters classification: toward predictive models of suicidal behavior. Neurocomputing 92 , 3–8 (2012).
von Glischinski, M., Teismann, T., Prinz, S., Gebauer, J. E. & Hirschfeld, G. Depressive symptom inventory suicidality subscale: optimal cut points for clinical and non-clinical samples. Clin. Psychol. Psychother. 23 , 543–549 (2016).
Hilvert, E., Davidson, D. & Gámez, P. B. Assessment of personal narrative writing in children with and without autism spectrum disorder. Res. Autism Spectr. Disord. 69 , 101453 (2020).
Zervopoulos, A. D. et al. Language processing for predicting suicidal tendencies: a case study in greek poetry. In IFIP International Conference on Artificial Intelligence Applications and Innovations , pp. 173–183 (2019).
Birjali, M., Beni-Hssane, A. & Erritali, M. Machine learning and semantic sentiment analysis based algorithms for suicide sentiment prediction in social networks. Proc. Computer. Sci. 113 , 65–72 (2017).
Trifan, A., Antunes, R., Matos, S. & Oliveira, J. L. Understanding depression from psycholinguistic patterns in social media texts. Adv. Inf. Retr. 12036 , 402 (2020).
Briand, A., Almeida, H. & Meurs, M. -J. Analysis of social media posts for early detection of mental health conditions. In Canadian Conference on Artificial Intelligence , pp. 133–143 (2018).
Trifan, A. & Oliveira, J. L. Bioinfo@ uavr at erisk 2019: delving into social media texts for the early detection of mental and food disorders. In CLEF (Working Notes) (2019).
Lin, W., Ji, D. & Lu, Y. Disorder recognition in clinical texts using multi-label structured svm. BMC Bioinform. 18 , 1–11 (2017).
Chomutare, T. Text classification to automatically identify online patients vulnerable to depression. In International Symposium on Pervasive Computing Paradigms for Mental Health , pp. 125–130 (2014).
Islam, M. R. Depression detection from social network data using machine learning techniques. Health Inf. Sci. Syst. 6 , 1–12 (2018).
Su, Y., Zheng, H., Liu, X. & Zhu, T. Depressive emotion recognition based on behavioral data. In International Conference on Human Centered Computing , pp. 257–268 (2018).
Simms, T. et al. Detecting cognitive distortions through machine learning text analytics. In 2017 IEEE International Conference on Healthcare Informatics (ICHI) , pp. 508–512 (2017).
He, Q., Veldkamp, B. P., Glas, C. A. & de Vries, T. Automated assessment of patients’ self-narratives for posttraumatic stress disorder screening using natural language processing and text mining. Assessment 24 , 157–172 (2017).
Shickel, B., Siegel, S., Heesacker, M., Benton, S. & Rashidi, P. Automatic detection and classification of cognitive distortions in mental health text. In 2020 IEEE 20th International Conference on Bioinformatics and Bioengineering (BIBE) , pp. 275–280 (2020).
Guntuku, S. C., Giorgi, S. & Ungar, L. Current and future psychological health prediction using language and socio-demographics of children for the clpysch 2018 shared task. In Proc. Fifth Workshop on Computational Linguistics and Clinical Psychology: From Keyboard to Clinic , pp. 98–106 (2018).
Stankevich, M., Isakov, V., Devyatkin, D. & Smirnov, I. V. Feature engineering for depression detection in social media. In ICPRAM , pp. 426–431 (2018).
Boag, W. Hard for humans, hard for machines: predicting readmission after psychiatric hospitalization using narrative notes. Transl. Psychiatry 11 , 1–6 (2021).
Adamou, M. et al. Mining free-text medical notes for suicide risk assessment. In Proc. 10th Hellenic Conference on Artificial Intelligence , pp. 1–8 (2018).
Saleem, S. et al. Automatic detection of psychological distress indicators and severity assessment from online forum posts. In Proc. COLING 2012 , pp. 2375–2388 (2012).
Trifan, A. & Oliveira, J. L. Cross-evaluation of social mining for classification of depressed online personas. J. Integr. Bioinform . (2021)
Balani, S. & De Choudhury, M. Detecting and characterizing mental health related self-disclosure in social media. In Proc. 33rd Annual ACM Conference Extended Abstracts on Human Factors in Computing Systems , pp. 1373–1378 (2015).
Delahunty, F., Wood, I. D. & Arcan, M. First insights on a passive major depressive disorder prediction system with incorporated conversational chatbot. In Irish Conference on Artificial Intelligence and Cognitive Science (2018).
Deshpande, M. & Rao, V. Depression detection using emotion artificial intelligence. In 2017 International Conference on Intelligent Sustainable Systems (iciss) , pp. 858–862 (2017).
Hwang, Y., Kim, H. J., Choi, H. J. & Lee, J. Exploring abnormal behavior patterns of online users with emotional eating behavior: topic modeling study. J. Med. Internet Res. 22 , 15700 (2020).
Alam, M. A. U. & Kapadia, D. Laxary: a trustworthy explainable twitter analysis model for post-traumatic stress disorder assessment. In 2020 IEEE International Conference on Smart Computing (SMARTCOMP) , pp. 308–313 (2020).
Plaza-del Arco, F. M., López-Úbeda, P., Diaz-Galiano, M. C., Urena-López, L. A. & Martin-Valdivia, M.-T. Integrating Umls for Early Detection of Sings of Anorexia . (Universidad de Jaen, Campus Las Lagunillas: Jaen, Spain, 2019).
Dao, B., Nguyen, T., Phung, D. & Venkatesh, S. Effect of mood, social connectivity and age in online depression community via topic and linguistic analysis. In International Conference on Web Information Systems Engineering , pp. 398–407 (2014).
Katchapakirin, K., Wongpatikaseree, K., Yomaboot, P. & Kaewpitakkun, Y. Facebook social media for depression detection in the thai community. In 2018 15th International Joint Conference on Computer Science and Software Engineering (JCSSE) , pp. 1–6 (2018).
Chang, M. -Y. & Tseng, C. -Y. Detecting social anxiety with online social network data. In 2020 21st IEEE International Conference on Mobile Data Management (MDM) , pp. 333–336 (2020).
Tong. L. et al. Cost-sensitive boosting pruning trees for depression detection on Twitter. In IEEE Transactions on Affective Computing , https://doi.org/10.1109/TAFFC.2022.3145634 (2019).
Guntuku, S. C., Buffone, A., Jaidka, K., Eichstaedt, J. C. & Ungar, L. H. Understanding and measuring psychological stress using social media. In Proc. International AAAI Conference on Web and Social Media , vol. 13, pp. 214–225 (2019).
Zhao, L., Jia, J. & Feng, L. Teenagers’ stress detection based on time-sensitive micro-blog comment/response actions. In IFIP International Conference on Artificial Intelligence in Theory and Practice , pp. 26–36 (2015).
Ziwei, B. Y. & Chua, H. N. An application for classifying depression in tweets. In Proc. 2nd International Conference on Computing and Big Data , pp. 37–41 (2019).
Prakash, A., Agarwal, K., Shekhar, S., Mutreja, T. & Chakraborty, P. S. An ensemble learning approach for the detection of depression and mental illness over twitter data. In 2021 8th International Conference on Computing for Sustainable Global Development (INDIACom) , pp. 565–570 (2021).
Coello-Guilarte, L., Ortega-Mendoza, R. M., Villaseñor-Pineda, L. & Montes-y-Gómez, M. Crosslingual depression detection in twitter using bilingual word alignments. In International Conference of the Cross-Language Evaluation Forum for European Languages , pp. 49–61 (2019).
Qiu, J. & Gao, J. Depression tendency recognition model based on college student’s microblog text. In International Conference on Intelligence Science , pp. 351–359 (2017).
Almouzini, S. et al. Detecting arabic depressed users from twitter data. Proc. Comput. Sci. 163 , 257–265 (2019).
Mbarek, A., Jamoussi, S., Charfi, A. & Hamadou, A. B. Suicidal profiles detection in twitter. In WEBIST , pp. 289–296 (2019).
Xu, S. et al. Automatic verbal analysis of interviews with schizophrenic patients. In 2018 IEEE 23rd International Conference on Digital Signal Processing (DSP) , pp. 1–5 (2018).
Verma, P., Sharma, K. & Walia, G. S. Depression detection among social media users using machine learning. In International Conference on Innovative Computing and Communications , pp. 865–874 (2021).
Shrestha, A. & Spezzano, F. Detecting depressed users in online forums. In Proc. 2019 IEEE/ACM International Conference on Advances in Social Networks Analysis and Mining , pp. 945–951 (2019).
Desmet, B. & Hoste, V. Recognising suicidal messages in dutch social media. In 9th International Conference on Language Resources and Evaluation (LREC) , pp. 830–835 (2014).
He, L. & Luo, J. “What makes a pro eating disorder hashtag”: using hashtags to identify pro eating disorder tumblr posts and twitter users. In 2016 IEEE International Conference on Big Data (Big Data) , pp. 3977–3979 (2016).
Marerngsit, S. & Thammaboosadee, S. A two-stage text-to-emotion depressive disorder screening assistance based on contents from online community. In 2020 8th International Electrical Engineering Congress (iEECON) , pp. 1–4 (2020).
Nadeem, M. Identifying depression on twitter. Preprint at arXiv https://arxiv.org/abs/1607.07384 (2016).
Fodeh, S. et al. Using machine learning algorithms to detect suicide risk factors on twitter. In 2019 International Conference on Data Mining Workshops (ICDMW) , pp. 941–948 (2019).
Tariq, S. A novel co-training-based approach for the classification of mental illnesses using social media posts. IEEE Access 7 , 166165–166172 (2019).
Mittal, A., Goyal, A. & Mittal, M. Data preprocessing based connecting suicidal and help-seeking behaviours. In 2021 5th International Conference on Computing Methodologies and Communication (ICCMC) , pp. 1824–1830 (2021).
Kamite, S. R. & Kamble, V. Detection of depression in social media via twitter using machine learning approach. In 2020 International Conference on Smart Innovations in Design, Environment, Management, Planning and Computing (ICSIDEMPC) , pp. 122–125 (2020).
Schoene, A. M. & Dethlefs, N. Automatic identification of suicide notes from linguistic and sentiment features. In Proc. 10th SIGHUM Workshop on Language Technology for Cultural Heritage, Social Sciences, and Humanities , pp. 128–133 (2016).
Almeida, H., Briand, A. & Meurs, M.- J. Detecting early risk of depression from social media user-generated content. In CLEF (Working Notes) (2017).
Govindasamy, K. A. & Palanichamy, N. Depression detection using machine learning techniques on twitter data. In 2021 5th International Conference on Intelligent Computing and Control Systems (ICICCS) , pp. 960–966 (2021).
Baheti, R. & Kinariwala, S. Detection and analysis of stress using machine learning techniques. Int. J. Eng. Adv. Technol. 9 , 335–342 (2019).
Németh, R., Sik, D. & Máté, F. Machine learning of concepts hard even for humans: the case of online depression forums. Int. J. Qualitative Methods 19 , 1609406920949338 (2020).
Benton, A., Mitchell, M. & Hovy, D. Multitask learning for mental health conditions with limited social media data. In Proc. 15th Conference of the European Chapter of the Association for Computational Linguistics: Vol. 1 , pp. 152–162 (2017).
Hiraga, M. Predicting depression for japanese blog text. In Proc. ACL 2017, Student Research Workshop , pp. 107–113 (2017).
Nasir, A., A slam, K., Tariq, S. & Ullah, M. F. Predicting mental illness using social media posts and comments. International Journal of Advanced Computer Science and Applications(IJACSA) , vol. 11 (2020).
Skaik, R. & Inkpen, D. Using twitter social media for depression detection in the canadian population. In 2020 3rd Artificial Intelligence and Cloud Computing Conference , pp. 109–114 (2020).
Chadha, A. & Kaushik, B. Machine learning based dataset for finding suicidal ideation on twitter. In 2021 Third International Conference on Intelligent Communication Technologies and Virtual Mobile Networks (ICICV) , pp. 823–828 (2021).
Sekulić, I., Gjurković, M. & Šnajder, J. Not Just Depressed: Bipolar Disorder Prediction on Reddit. In Proc, the 9th Workshop on Computational Approaches to Subjectivity, Sentiment and Social Media Analysis , pp. 72–78 (2018).
Kumar, A., Sharma, A. & Arora, A. Anxious depression prediction in real-time social data. In International Conference on Advances in Engineering Science Management & Technology (ICAESMT)-2019. (Uttaranchal University, Dehradun, India, 2019).
Nghiem, M. -Q., Baylis, P. & Ananiadou, S. Paladin: an annotation tool based on active and proactive learning. In Proc. 16th Conference of the European Chapter of the Association for Computational Linguistics: System Demonstrations , pp. 238–243 (2021).
Park, A., Conway, M. & Chen, A. T. Examining thematic similarity, difference, and membership in three online mental health communities from reddit: a text mining and visualization approach. Comput. Hum. Behav. 78 , 98–112 (2018).
Shrestha, A., Serra, E. & Spezzano, F. Multi-modal social and psycho-linguistic embedding via recurrent neural networks to identify depressed users in online forums. Netw. Modeling Anal. Health Inform. Bioinforma. 9 , 1–11 (2020).
Friedenberg, M., Amiri, H., Daumé III, H. & Resnik, P. The umd clpsych 2016 shared task system: text representation for predicting triage of forum posts about mental health. In Proc. Third Workshop on Computational Linguistics and Clinical Psychology , pp. 158–161 (2016).
Nguyen, T. Using linguistic and topic analysis to classify sub-groups of online depression communities. Multimed. Tools Appl. 76 , 10653–10676 (2017).
Yazdavar, A. H. et al. Semi-supervised approach to monitoring clinical depressive symptoms in social media. In Proc. 2017 IEEE/ACM International Conference on Advances in Social Networks Analysis and Mining 2017 , pp. 1191–1198 (2017).
Sarsam, S. M., Al-Samarraie, H., Alzahrani, A. I., Alnumay, W. & Smith, A. P. A lexicon-based approach to detecting suicide-related messages on twitter. Biomed. Signal Process. Control 65 , 102355 (2021).
Driessens, K., Reutemann, P., Pfahringer, B. & Leschi, C. Using weighted nearest neighbor to benefit from unlabeled data. In Pacific-Asia Conference on Knowledge Discovery and Data Mining , pp. 60–69 (2006).
Zhou, D., Bousquet, O., Lal, T. N., Weston, J. & Schölkopf, B. Learning with local and global consistency. In Advances in Neural Information Processing Systems , pp. 321–328 (2004).
LeCun, Y., Bengio, Y. & Hinton, G. Deep learning. Nature 521 , 436–444 (2015).
Article CAS PubMed Google Scholar
Voulodimos, A., Doulamis, N., Doulamis, A. & Protopapadakis, E. Deep learning for computer vision: a brief review. Comput. Intell. Neurosci. 2018 1–13 (2018).
Young, T., Hazarika, D., Poria, S. & Cambria, E. Recent trends in deep learning based natural language processing. IEEE Comput. Intell. Mag. 13 , 55–75 (2018).
Deng, L. & Yu, D. Deep learning: methods and applications. Found. Trends Signal Process. 7 , 197–387 (2014).
Su, C., Xu, Z., Pathak, J. & Wang, F. Deep learning in mental health outcome research: a scoping review. Transl. Psychiatry 10 , 1–26 (2020).
Ghannay, S., Favre, B., Esteve, Y. & Camelin, N. Word embedding evaluation and combination. In Proc. Tenth International Conference on Language Resources and Evaluation (LREC’16) , pp. 300–305 (2016).
Pennington, J., Socher, R. & Manning, C. D. Glove: Global vectors for word representation. In Proc. 2014 Conference on Empirical Methods in Natural Language Processing (EMNLP) , pp. 1532–1543 (2014).
Mikolov, T., Chen, K., Corrado, G. & Dean, J. Efficient estimation of word representations in vector space. In Proc. 1st International Conference on Learning Representations (ICLR) Workshops Track . (2013).
Devlin, J., Chang, M. W., Lee, K. & Toutanova, K. Bert: Pre-training of deep bidirectional transformers for languageunderstanding. In Proc. NAACL-HLT , pp. 4171–4186 (2019).
Lan, Z. et al. Albert: a lite bert for self-supervised learning of language representations. In Proc. 8th International Conference on Learning Representations (ICLR) (2020).
Gaur, M. et al. Knowledge-aware assessment of severity of suicide risk for early intervention. In The World Wide Web Conference , pp. 514–525 (2019).
Boukil, S., El Adnani, F., Cherrat, L., El Moutaouakkil, A. E. & Ezziyyani, M. Deep learning algorithm for suicide sentiment prediction. In International Conference on Advanced Intelligent Systems for Sustainable Development , pp. 261–272 (2018).
Phan, H. T., Tran, V. C., Nguyen, N. T. & Hwang, D. A framework for detecting user’s psychological tendencies on twitter based on tweets sentiment analysis. In International Conference on Industrial, Engineering and Other Applications of Applied Intelligent Systems , pp. 357–372 (2020).
Wang, Y. -T., Huang, H. -H., Chen, H. -H. & Chen, H. A neural network approach to early risk detection of depression and anorexia on social media text. In CLEF (Working Notes) (2018).
Trotzek, M., Koitka, S. & Friedrich, C. M. Utilizing neural networks and linguistic metadata for early detection of depression indications in text sequences. IEEE Trans. Knowl. Data Eng. 32 , 588–601 (2018).
Obeid, J. S. Automated detection of altered mental status in emergency department clinical notes: a deep learning approach. BMC Med. Inform. Decis. Mak. 19 , 1–9 (2019).
Rao, G., Zhang, Y., Zhang, L., Cong, Q. & Feng, Z. Mgl-cnn: a hierarchical posts representations model for identifying depressed individuals in online forums. IEEE Access 8 , 32395–32403 (2020).
Lin, H. Detecting stress based on social interactions in social networks. IEEE Trans. Knowl. Data Eng. 29 , 1820–1833 (2017).
Hochreiter, S. & Schmidhuber, J. Long short-term memory. Neural Comput. 9 , 1735–1780 (1997).
Cho, K. et al. Learning phrase representations using rnn encoder-decoder for statistical machine translation. In Proc. the 2014 Conference on Empirical Methods in Natural Language Processing (EMNLP) , pp. 1724–1734 (2014).
Pascanu, R., Mikolov, T. & Bengio, Y. On the difficulty of training recurrent neural networks. In International Conference on Machine Learning , pp. 1310–1318 (2013).
Ghosh, S. & Anwar, T. Depression intensity estimation via social media: a deep learning approach. IEEE Trans. Comput. Soc. Syst. 8 , 1465–1474 (2021).
Uddin, A. H., Bapery, D. & Arif, A. S. M. Depression analysis of bangla social media data using gated recurrent neural network. In 2019 1st International Conference on Advances in Science, Engineering and Robotics Technology (ICASERT) , pp. 1–6 (2019).
Yao, H. Detection of suicidality among opioid users on reddit: machine learning–based approach. J. Med. Internet Res. 22 , 15293 (2020).
Ahmed, U., Mukhiya, S. K., Srivastava, G., Lamo, Y. & Lin, J. C. -W. Attention-based deep entropy active learning using lexical algorithm for mental health treatment. Front. Psychol. 12 , 471 (2021).
Bahdanau, D., Cho, K. & Bengio, Y. Neural machine translation by jointly learning to align and translate. In Proc. 3rd International Conference on Learning Representations (ICLR) (2015).
Sekulić, I. & Strube, M. Adapting deep learning methods for mental health prediction on social media. In Proc. the 5th Workshop on Noisy User-generated Text (W-NUT) , pp. 322–327 (2019).
Sawhney, R., Joshi, H., Gandhi, S. & Shah, R. R. Towards ordinal suicide ideation detection on social media. in: Proc. 14th ACM International Conference on Web Search and Data Mining , pp. 22–30 (2021).
Rutowski, T. et al. Cross-demographic portability of deep nlp-based depression models. In 2021 IEEE Spoken Language Technology Workshop (SLT) , pp. 1052–1057 (2021).
Rutowski, T. et al. Depression and anxiety prediction using deep language models and transfer learning. In 2020 7th International Conference on Behavioural and Social Computing (BESC) , pp. 1–6 (2020).
Ghosh, S., Ekbal, A. & Bhattacharyya, P. A multitask framework to detect depression, sentiment and multi-label emotion from suicide notes. Cognit. Comput. 14 , 110–129 (2022).
Gui, T. et al. Cooperative multimodal approach to depression detection in twitter. In Proc. AAAI Conference on Artificial Intelligence , vol. 33, pp. 110–117 (2019).
Gui, T. et al. Depression detection on social media with reinforcement learning. In China National Conference on Chinese Computational Linguistics , pp. 613–624 (2019).
Wongkoblap, A., Vadillo, M. A. & Curcin, V. Predicting social network users with depression from simulated temporal data. In IEEE EUROCON 2019-18th International Conference on Smart Technologies , pp. 1–6 (2019).
Wongkoblap, A., Vadillo, M. A. & Curcin, V. Modeling depression symptoms from social network data through multiple instance learning. AMIA Summits Transl. Sci. Proc. 2019 , 44 (2019).
PubMed PubMed Central Google Scholar
Vaswani, A. et al. Attention is all you need. In Advances in Neural Information Processing Systems , pp. 5998–6008 (2017).
Wang, N. et al. Learning models for suicide prediction from social media posts. In Proc. the Seventh Workshop on Computational Linguistics and Clinical Psychology , pp. 87–92 (2021).
Zhang, T., Schoene, A. M. & Ananiadou, S. Automatic identification of suicide notes with a transformer-based deep learning model. Internet Interv. 25 , 100422 (2021).
Haque, F., Nur, R. U., Al Jahan, S., Mahmud, Z. & Shah, F. M. A transformer based approach to detect suicidal ideation using pre-trained language models. In 2020 23rd International Conference on Computer and Information Technology (ICCIT) , pp. 1–5 (2020).
Chaurasia, A. et al. Predicting mental health of scholars using contextual word embedding. In 2021 8th International Conference on Computing for Sustainable Global Development (INDIACom) , pp. 923–930 (2021).
Malviya, K., Roy, B. & Saritha, S. A transformers approach to detect depression in social media. In 2021 International Conference on Artificial Intelligence and Smart Systems (ICAIS) , pp. 718–723 (2021).
Murarka, A., Radhakrishnan, B. & Ravichandran, S. Detection and classification of mental illnesses on social media using roberta. Preprint arXiv https://arxiv.org/abs/2011.11226 (2020).
Wang, X. Depression risk prediction for chinese microblogs via deep-learning methods: content analysis. JMIR Med. Inform. 8 , 17958 (2020).
Abed-Esfahani, P. et al. Transfer learning for depression: early detection and severity prediction from social media postings. In CLEF (Working Notes) (2019).
Gaur, M. Characterization of time-variant and time-invariant assessment of suicidality on reddit using c-ssrs. PloS ONE 16 , 0250448 (2021).
Tadesse, M. M., Lin, H., Xu, B. & Yang, L. Detection of suicide ideation in social media forums using deep learning. Algorithms 13 , 7 (2020).
Zhou, S., Zhao, Y., Bian, J., Haynos, A. F. & Zhang, R. Exploring eating disorder topics on twitter: machine learning approach. JMIR Med. Inform. 8 , 18273 (2020).
Deshpande, S. & Warren, J. Self-harm detection for mental health chatbots. In Public Health and Informatics , pp. 48–52. (IOS Press, 2021).
Solieman, H. & Pustozerov, E. A. The detection of depression using multimodal models based on text and voice quality features. In 2021 IEEE Conference of Russian Young Researchers in Electrical and Electronic Engineering (ElConRus) , pp. 1843–1848 (2021).
Sawhney, R., Joshi, H., Gandhi, S. & Shah, R. A time-aware transformer based model for suicide ideation detection on social media. In Proc. 2020 Conference on Empirical Methods in Natural Language Processing (EMNLP) , pp. 7685–7697 (2020).
Plutchik, R. A general psychoevolutionary theory of emotion. In Theories of Emotion , pp. 3–33. (Elsevier, 1980).
Aragón, M. E., López-Monroy, A. P., González-Gurrola, L. C. & Montes, M. Detecting depression in social media using fine-grained emotions. in: Proc. 2019 Conference of the North American Chapter of the Association for Computational Linguistics: Human Language Technologies, Volume 1 (Long and Short Papers) , pp. 1481–1486 (2019).
Aragón, M. E., López-Monroy, A. P., González, L. C. & Montes-y-Gómez, M. Attention to emotions: detecting mental disorders in social media. In International Conference on Text, Speech, and Dialogue , pp. 231–239 (2020).
Lara, J. S., Aragon, M. E., Gonzalez, F. A. & Montes-y-Gomez, M. Deep bag-of-sub-emotions for depression detection in social media. In Proc. International Conference on Text, Speech, and Dialogue . pp. 60–72 (2021).
Sawhney, R., Joshi, H., Flek, L. & Shah, R. Phase: Learning emotional phase-aware representations for suicide ideation detection on social media. In Proc. 16th Conference of the European Chapter of the Association for Computational Linguistics: Main Volume , pp. 2415–2428 (2021).
Sawhney, R., Joshi, H., Shah, R. & Flek, L. Suicide ideation detection via social and temporal user representations using hyperbolic learning. In Proc. 2021 Conference of the North American Chapter of the Association for Computational Linguistics: Human Language Technologies , pp. 2176–2190 (2021).
Thorstad, R. & Wolff, P. Predicting future mental illness from social media: a big-data approach. Behav. Res. Methods 51 , 1586–1600 (2019).
Aladağ, A. E., Muderrisoglu, S., Akbas, N. B., Zahmacioglu, O. & Bingol, H. O. Detecting suicidal ideation on forums: proof-of-concept study. J. Med. Internet Res. 20 , 9840 (2018).
Desmet, B. & Hoste, V. Online suicide prevention through optimised text classification. Inf. Sci. 439 , 61–78 (2018).
Cheng, Q., Li, T. M., Kwok, C.-L., Zhu, T. & Yip, P. S. Assessing suicide risk and emotional distress in chinese social media: a text mining and machine learning study. J. Med. internet Res. 19 , 243 (2017).
Roy, A. A machine learning approach predicts future risk to suicidal ideation from social media data. NPJ Digital Med. 3 , 1–12 (2020).
Rios, A. & Kavuluru, R. Ordinal convolutional neural networks for predicting rdoc positive valence psychiatric symptom severity scores. J. Biomed. Inform. 75 , 85–93 (2017).
Losada, D. E., Crestani, F. & Parapar, J. Overview of erisk 2019 early risk prediction on the internet. In International Conference of the Cross-Language Evaluation Forum for European Languages , pp. 340–357 (2019).
Losada, D. E. & Crestani, F. A test collection for research on depression and language use. In International Conference of the Cross-Language Evaluation Forum for European Languages , pp. 28–39 (2016).
Van Engelen, J. E. & Hoos, H. H. A survey on semi-supervised learning. Mach. Learn. 109 , 373–440 (2020).
Settles, B. Closing the loop: fast, interactive semi-supervised annotation with queries on features and instances. In Proc. 2011 Conference on Empirical Methods in Natural Language Processing , pp. 1467–1478 (2011).
Maupomé, D. & Meurs, M. -J. Using topic extraction on social media content for the early detection of depression. In CLEF (Working Notes) vol. 2125 (2018)
Gaur, M. et al. “Let me tell you about your mental health!” contextualized classification of reddit posts to dsm-5 for web-based intervention. In Proc. 27th ACM International Conference on Information and Knowledge Management , pp. 753–762 (2018).
Galiatsatos, D. et al. Classification of the most significant psychological symptoms in mental patients with depression using bayesian network. In Proc. 16th International Conference on Engineering Applications of Neural Networks (INNS) , pp. 1–8 (2015).
Wang, W. Y., Singh, S. & Li, J. Deep adversarial learning for nlp. In Proc. 2019 Conference of the North American Chapter of the Association for Computational Linguistics: Tutorials , pp. 1–5 (2019).
Le-Khac, P. H., Healy, G. & Smeaton, A. F. Contrastive representation learning: a framework and review. IEEE Access 8 , 193907–193934 (2020).
Li, Y., Tian, X., Liu, T. & Tao, D. Multi-task model and feature joint learning. In Twenty-Fourth International Joint Conference on Artificial Intelligence (2015).
Sharma, A. R. & Kaushik, P. Literature survey of statistical, deep and reinforcement learning in natural language processing. In 2017 International Conference on Computing, Communication and Automation (ICCCA) , pp. 350–354 (2017).
Ruder, S., Peters, M. E., Swayamdipta, S. & Wolf, T. Transfer learning in natural language processing. In Proc. 2019 Conference of the North American Chapter of the Association for Computational Linguistics: Tutorials , pp. 15–18 (2019).
López-Úbeda, P., Plaza-del-Arco, F. M., Díaz-Galiano, M. C. & Martín-Valdivia, M.-T. How successful is transfer learning for detecting anorexia on social media? Appl. Sci. 11 , 1838 (2021).
Hu, D. An introductory survey on attention mechanisms in nlp problems. In Proc. SAI Intelligent Systems Conference , pp. 432–448 (2019).
Wang, Z., Zhang, J., Feng, J. & Chen, Z. Knowledge graph and text jointly embedding. In Proc. 2014 Conference on Empirical Methods in Natural Language Processing (EMNLP) , pp. 1591–1601 (2014).
Sap, M., Shwartz, V., Bosselut, A., Choi, Y. & Roth, D. Commonsense reasoning for natural language processing. In Proc. 58th Annual Meeting of the Association for Computational Linguistics: Tutorial Abstracts , pp. 27–33 (2020).
Feng, S. Y. et al. A survey of data augmentation approaches for nlp. In Proc. Findings of the Association for Computational Linguistics: ACL-IJCNLP , pp. 968–988 (2021).
Lin, C. et al. Sensemood: depression detection on social media. In Proc. 2020 International Conference on Multimedia Retrieval , pp. 407–411 (2020).
Mann, P., Paes, A. & Matsushima, E. H. See and read: detecting depression symptoms in higher education students using multimodal social media data. In Proc. International AAAI Conference on Web and Social Media , vol. 14, pp. 440–451 (2020).
Xu, Z., Pérez-Rosas, V. & Mihalcea, R. Inferring social media users’ mental health status from multimodal information. In Proc. 12th Language Resources and Evaluation Conference , pp. 6292–6299 (2020).
Wang, B. et al. Learning to detect bipolar disorder and borderline personality disorder with language and speech in non-clinical interviews. In Proc. Interspeech 2020 , pp. 437–441 (2020).
Rodrigues Makiuchi, M., Warnita, T., Uto, K. & Shinoda, K. Multimodal fusion of bert-cnn and gated cnn representations for depression detection. In Proc. 9th International on Audio/Visual Emotion Challenge and Workshop , pp. 55–63 (2019).
Mittal, A. et al. Multi-modal detection of alzheimer’s disease from speech and text. In Proc. BIOKDD'21 (2021).
Ribeiro, M. T., Singh, S. & Guestrin, C. “Why should i trust you?” explaining the predictions of any classifier. In Proc. 22nd ACM SIGKDD International Conference on Knowledge Discovery and Data Mining , pp. 1135–1144 (2016).
Du, M., Liu, N. & Hu, X. Techniques for interpretable machine learning. Commun. ACM 63 , 68–77 (2019).
Song, H., You, J., Chung, J. -W. & Park, J. C. Feature attention network: Interpretable depression detection from social media. In Proc. 32nd Pacific Asia Conference on Language, Information and Computation (2018).
Zogan, H., Razzak, I., Wang, X., Jameel, S. & Xu, G. Explainable depression detection with multi-modalities using a hybrid deep learning model on social media. Preprint at arXiv https://arxiv.org/abs/2007.02847 (2020).
Castelvecchi, D. Can we open the black box of AI? Nat. N. 538 , 20 (2016).
Article CAS Google Scholar
Benton, A., Coppersmith, G. & Dredze, M. Ethical research protocols for social media health research. In Proc. First ACL Workshop on Ethics in Natural Language Processing , pp. 94–102 (2017).
Nicholas, J., Onie, S. & Larsen, M. E. Ethics and privacy in social media research for mental health. Curr. Psychiatry Rep. 22 , 1–7 (2020).
McKee, R. Ethical issues in using social media for health and health care research. Health Policy 110 , 298–301 (2013).
Tadisetty, S. & Ghazinour, K. Anonymous prediction of mental illness in social media. In 2021 IEEE 11th Annual Computing and Communication Workshop and Conference (CCWC) , pp. 0954–0960 (2021).
Doan, S. Extracting health-related causality from twitter messages using natural language processing. BMC Med. Inform. Decis. Mak. 19 , 71–77 (2019).
Hutto, C. & Gilbert, E. Vader: a parsimonious rule-based model for sentiment analysis of social media text. In Proc. International AAAI Conference on Web and Social Media , vol. 8 (2014).
Cambria, E., Poria, S., Hazarika, D. & Kwok, K. Senticnet 5: Discovering conceptual primitives for sentiment analysis by means of context embeddings. In Proc. AAAI Conference on Artificial Intelligence , vol. 32 (2018).
Nielsen, F. Å. A new anew: evaluation of a word list for sentiment analysis in microblogs. In Proc. CEUR Workshop Proceedings, vol. 718, pp. 93–98 (2011).
Wang, X. et al. A depression detection model based on sentiment analysis in micro-blog social network. In Pacific-Asia Conference on Knowledge Discovery and Data Mining , pp. 201–213 (2013).
Leiva, V. & Freire, A. Towards suicide prevention: early detection of depression on social media. In International Conference on Internet Science , pp. 428–436 (2017).
Stephen, J. J. & Prabu, P. Detecting the magnitude of depression in twitter users using sentiment analysis. Int. J. Electr. Comput. Eng. 9 , 3247 (2019).
Mohammad, S. M. & Turney, P. D. Nrc emotion lexicon. National Research Council, Canada 2 (2013).
Zhou, T. H., Hu, G. L. & Wang, L. Psychological disorder identifying method based on emotion perception over social networks. Int. J. Environ. Res. Public Health 16 , 953 (2019).
Article PubMed Central Google Scholar
Saloun, P., Ondrejka, A., Malčík, M. & Zelinka, I. Personality disorders identification in written texts. In AETA 2015: Recent Advances in Electrical Engineering and Related Sciences , pp. 143–154 (Springer, 2016).
Blei, D. M., Ng, A. Y. & Jordan, M. I. Latent dirichlet allocation. J. Mach. Learn. Res. 3 , 993–1022 (2003).
Dumais, S. T. Latent semantic analysis. Annu. Rev. Inf. Sci. Technol. 38 , 188–230 (2004).
Xu, W., Liu, X. & Gong, Y. Document clustering based on non-negative matrix factorization. In Proc. 26th Annual International ACM SIGIR Conference on Research and Development in Informaion Retrieval , pp. 267–273 (2003).
Desmet, B., Jacobs, G. & Hoste, V. Mental distress detection and triage in forum posts: the lt3 clpsych 2016 shared task system. In Proc. Third Workshop on Computational Linguistics and Clinical Psychology , pp. 148–152 (2016).
Tausczik, Y. R. & Pennebaker, J. W. The psychological meaning of words: Liwc and computerized text analysis methods. J. Lang. Soc. Psychol. 29 , 24–54 (2010).
Rodrigues, R. G., das Dores, R. M., Camilo-Junior, C. G. & Rosa, T. C. Sentihealth-cancer: a sentiment analysis tool to help detecting mood of patients in online social networks. Int. J. Med. Inform. 85 , 80–95 (2016).
Yoo, M., Lee, S. & Ha, T. Semantic network analysis for understanding user experiences of bipolar and depressive disorders on reddit. Inf. Process. Manag. 56 , 1565–1575 (2019).
Ricard, B. J., Marsch, L. A., Crosier, B. & Hassanpour, S. Exploring the utility of community-generated social media content for detecting depression: an analytical study on instagram. J. Med. Internet Res. 20 , 11817 (2018).
Mikolov, T., Sutskever, I., Chen, K., Corrado, G. S. & Dean, J. Distributed representations of words and phrases and their compositionality. In Advances in Neural Information Processing Systems , pp. 3111–3119 (2013).
Hemmatirad, K., Bagherzadeh, H., Fazl-Ersi, E. & Vahedian, A. Detection of mental illness risk on social media through multi-level svms. In 2020 8th Iranian Joint Congress on Fuzzy and Intelligent Systems (CFIS) , pp. 116–120 (2020).
Bandyopadhyay, A., Achilles, L., Mandl, T., Mitra, M. & Saha, S. K. Identification of depression strength for users of online platforms: a comparison of text retrieval approaches. In Proc. CEUR Workshop Proceedings, vol. 2454, pp. 331–342 (2019).
Zhong, Q. -Y. Screening pregnant women for suicidal behavior in electronic medical records: diagnostic codes vs. clinical notes processed by natural language processing. BMC Med. Inform. Decis. Mak. 18 , 1–11 (2018).
Huang, Y., Liu, X. & Zhu, T. Suicidal ideation detection via social media analytics. In International Conference on Human Centered Computing , pp. 166–174 (2019).
Lv, M., Li, A., Liu, T. & Zhu, T. Creating a chinese suicide dictionary for identifying suicide risk on social media. PeerJ 3 , 1455 (2015).
Nguyen, T., Phung, D., Adams, B. & Venkatesh, S. Prediction of age, sentiment, and connectivity from social media text. In International Conference on Web Information Systems Engineering , pp. 227–240 (2011).
Peng, Z., Hu, Q. & Dang, J. Multi-kernel svm based depression recognition using social media data. Int. J. Mach. Learn. Cybern. 10 , 43–57 (2019).
Wu, M. Y., Shen, C.-Y., Wang, E. T. & Chen, A. L. A deep architecture for depression detection using posting, behavior, and living environment data. J. Intell. Inf. Syst. 54 , 225–244 (2020).
Zogan, H., Wang, X., Jameel, S. & Xu, G. Depression detection with multi-modalities using a hybrid deep learning model on social media. Preprint at arXiv https://arxiv.org/abs/2007.02847 (2020).
Yao, X., Yu, G., Tang, J. & Zhang, J. Extracting depressive symptoms and their associations from an online depression community. Comput. Hum. Behav. 120 , 106734 (2021).
Dinkel, H., Wu, M. & Yu, K. Text-based depression detection on sparse data. Preprint at arXiv https://arxiv.org/abs/1904.05154 (2019).
Zhou, Y., Glenn, C. & Luo, J. Understanding and predicting multiple risky behaviors from social media. In Workshops at the Thirty-First AAAI Conference on Artificial Intelligence (2017).
Wang, Y., Wang, Z., Li, C., Zhang, Y. & Wang, H. A multitask deep learning approach for user depression detection on sina weibo. Preprint at arXiv https://arxiv.org/abs/2008.11708 (2020).
Aragon, M. E., Lopez-Monroy, A. P., Gonzalez-Gurrola, L. -C. G. & Montes, M. Detecting mental disorders in social media through emotional patterns-the case of anorexia and depression. IEEE Trans. Affect. Comput . (2021).
Li, N., Zhang, H. & Feng, L. Incorporating forthcoming events and personality traits in social media based stress prediction. IEEE Trans. Affect. Comput. (2021).
Download references
Acknowledgements
This research was partially funded by the Alan Turing Institute and the H2020 EPHOR project, grant agreement No. 874703.
Author information
Authors and affiliations.
Department of Computer Science, The University of Manchester, National Centre for Text Mining, Manchester, UK
Tianlin Zhang, Annika M. Schoene & Sophia Ananiadou
Department of Computer Science, Aalto University, Helsinki, Finland
Shaoxiong Ji
The Alan Turing Institute, London, UK
Sophia Ananiadou
You can also search for this author in PubMed Google Scholar
Contributions
T.Z. conducted the review, prepared figures, and wrote the initial draft. A.M.S., S.J., and S.A. revised the paper. S.A. supervised the project. All authors reviewed the paper.
Corresponding author
Correspondence to Sophia Ananiadou .
Ethics declarations
Competing interests.
The authors declare no competing interests.
Additional information
Publisher’s note Springer Nature remains neutral with regard to jurisdictional claims in published maps and institutional affiliations.
Supplementary information
Reporting summary, rights and permissions.
Open Access This article is licensed under a Creative Commons Attribution 4.0 International License, which permits use, sharing, adaptation, distribution and reproduction in any medium or format, as long as you give appropriate credit to the original author(s) and the source, provide a link to the Creative Commons license, and indicate if changes were made. The images or other third party material in this article are included in the article’s Creative Commons license, unless indicated otherwise in a credit line to the material. If material is not included in the article’s Creative Commons license and your intended use is not permitted by statutory regulation or exceeds the permitted use, you will need to obtain permission directly from the copyright holder. To view a copy of this license, visit http://creativecommons.org/licenses/by/4.0/ .
Reprints and permissions
About this article
Cite this article.
Zhang, T., Schoene, A.M., Ji, S. et al. Natural language processing applied to mental illness detection: a narrative review. npj Digit. Med. 5 , 46 (2022). https://doi.org/10.1038/s41746-022-00589-7
Download citation
Received : 26 October 2021
Accepted : 23 February 2022
Published : 08 April 2022
DOI : https://doi.org/10.1038/s41746-022-00589-7
Share this article
Anyone you share the following link with will be able to read this content:
Sorry, a shareable link is not currently available for this article.
Provided by the Springer Nature SharedIt content-sharing initiative
This article is cited by
Toward efficient, sustainable, and scalable methods of treatment characterization: an investigation of coding clinical practice from chart notes.
- Benjamin M. Isenberg
- Kimberly D. Becker
- Bruce F. Chorpita
Administration and Policy in Mental Health and Mental Health Services Research (2024)
Development of intelligent system based on synthesis of affective signals and deep neural networks to foster mental health of the Indian virtual community
- Mandeep Kaur Arora
- Jaspreet Singh
Social Network Analysis and Mining (2024)
Identifying patients in need of psychological treatment with language representation models
- İrfan Aygün
- Mehmet Kaya
Multimedia Tools and Applications (2024)
Unraveling minds in the digital era: a review on mapping mental health disorders through machine learning techniques using online social media
An automatic speech analytics program for digital assessment of stress burden and psychosocial health.
- Amanda M. Y. Chu
- Benson S. Y. Lam
- Mike K. P. So
npj Mental Health Research (2023)
Quick links
- Explore articles by subject
- Guide to authors
- Editorial policies
Sign up for the Nature Briefing newsletter — what matters in science, free to your inbox daily.

- Open access
- Published: 05 September 2022
Psychiatric and medical comorbidities of eating disorders: findings from a rapid review of the literature
- Ashlea Hambleton 1 ,
- Genevieve Pepin 2 ,
- Anvi Le 3 ,
- Danielle Maloney 1 , 4 ,
- National Eating Disorder Research Consortium ,
- Stephen Touyz 1 , 4 &
- Sarah Maguire 1 , 4
Journal of Eating Disorders volume 10 , Article number: 132 ( 2022 ) Cite this article
17k Accesses
40 Citations
65 Altmetric
Metrics details
Eating disorders (EDs) are potentially severe, complex, and life-threatening illnesses. The mortality rate of EDs is significantly elevated compared to other psychiatric conditions, primarily due to medical complications and suicide. The current rapid review aimed to summarise the literature and identify gaps in knowledge relating to any psychiatric and medical comorbidities of eating disorders.
This paper forms part of a rapid review) series scoping the evidence base for the field of EDs, conducted to inform the Australian National Eating Disorders Research and Translation Strategy 2021–2031, funded and released by the Australian Government. ScienceDirect, PubMed and Ovid/Medline were searched for English-language studies focused on the psychiatric and medical comorbidities of EDs, published between 2009 and 2021. High-level evidence such as meta-analyses, large population studies and Randomised Control Trials were prioritised.
A total of 202 studies were included in this review, with 58% pertaining to psychiatric comorbidities and 42% to medical comorbidities. For EDs in general, the most prevalent psychiatric comorbidities were anxiety (up to 62%), mood (up to 54%) and substance use and post-traumatic stress disorders (similar comorbidity rates up to 27%). The review also noted associations between specific EDs and non-suicidal self-injury, personality disorders, and neurodevelopmental disorders. EDs were complicated by medical comorbidities across the neuroendocrine, skeletal, nutritional, gastrointestinal, dental, and reproductive systems. Medical comorbidities can precede, occur alongside or emerge as a complication of the ED.
Conclusions
This review provides a thorough overview of the comorbid psychiatric and medical conditions co-occurring with EDs. High psychiatric and medical comorbidity rates were observed in people with EDs, with comorbidities contributing to increased ED symptom severity, maintenance of some ED behaviours, and poorer functioning as well as treatment outcomes. Early identification and management of psychiatric and medical comorbidities in people with an ED may improve response to treatment and overall outcomes.
Plain English Summary
The mortality rate of eating disorders is significantly elevated compared to other psychiatric conditions, primarily due to medical complications and suicide. Further, individuals with eating disorders often meet the diagnostic criteria of at least one comorbid psychiatric or medical disorder, that is, the individual simultaneously experiences both an ED and at least one other condition. This has significant consequences for researchers and health care providers – medical and psychiatric comorbidities impact ED symptoms and treatment effectiveness. The current review is part of a larger Rapid Review series conducted to inform the development of Australia’s National Eating Disorders Research and Translation Strategy 2021–2031. A Rapid Review is designed to comprehensively summarise a body of literature in a short timeframe, often to guide policymaking and address urgent health concerns. The Rapid Review synthesises the current evidence base and identifies gaps in eating disorder research and care. This paper gives a critical overview of the scientific literature relating to the psychiatric and medical comorbidities of eating disorders. It covers recent literature regarding psychiatric comorbidities including anxiety disorders, mood disorders, substance use disorders, trauma and personality disorders and neurodevelopmental disorders. Further, the review discusses the impact and associations between EDs and medical comorbidities, some of which precede the eating disorder, occur alongside, or as a consequence of the eating disorder.
Introduction
Eating Disorders (EDs) are often severe, complex, life-threatening illnesses with significant physiological and psychiatric impacts. EDs impact individuals across the entire lifespan, affecting all age groups (although most often they emerge in childhood and adolescence), genders, socioeconomic groups and cultures [ 1 ]. EDs have some of the highest mortality rates of all psychiatric illnesses and carry a significant personal, interpersonal, social and economic burdens [ 2 , 3 ].
Adding to the innate complexity of EDs, it is not uncommon for people living with an ED to experience associated problems such as psychological, social, and functional limitations [ 2 ] in addition to psychiatric and medical comorbidities [ 4 , 5 , 6 ]. Comorbidity is defined as conditions or illnesses that occur concurrently to the ED. Evidence suggests that between 55 and 95% of people diagnosed with an ED will also experience a comorbid psychiatric disorder in their lifetime [ 4 , 6 ]. Identifying psychiatric comorbidities is essential because of their potential impact on the severity of ED symptomatology, the individual’s distress and treatment effectiveness [ 7 , 8 ].
The mortality rate of EDs is significantly higher than the general population, with the highest occurring in Anorexia Nervosa (AN) due to impacts on the cardiovascular system [ 9 ] and suicide. [ 10 ] Mortality rates are also heightened in Bulimia Nervosa (BN) and Other Specified Feeding and Eating Disorder (OSFED) [ 11 ]. Suicide rates are elevated across the ED spectrum, and higher rates are observed in patients with a comorbid psychiatric disorder [ 10 , 12 ]. Of concern, the proportion of people with an ED not accessing treatment is estimated to be as high as 75% [ 13 ], potentially a consequence of comorbidities which impact on motivation, the ability to schedule appointments or require clinical prioritisation (i.e., self-harm or suicidal behaviours) [ 14 ]. Further, for many of those diagnosed with an ED who access treatment, recovery is a lengthy process. A longitudinal study found approximately two-thirds of participants with AN or BN had recovered by 22 years follow-up [ 15 ]. Although recovery occurred earlier for those with BN, illness duration was lengthy for both groups with quality of life and physical health impacts [ 15 ]. Further, less is known regarding the illness trajectory for those who do not receive treatment.
Medical comorbidities associated with EDs can range from mild to severe and life-threatening, with complications observed across all body systems, including the cardiac, metabolic and gastrointestinal, and reproductive systems [ 5 ]. These comorbidities and complications can place people at increased risk of medical instability and death [ 5 ]. Therefore, understanding how co-occurring medical comorbidities and complications impact EDs is critical to treatment and recovery.
In addition to ED-associated medical comorbidities, EDs often present alongside other psychiatric conditions. Psychiatric comorbidities in people with EDs are associated with higher health system costs, emergency department presentations and admissions [ 16 ]. Comorbidities may precede the onset of the ED, be co-occurring, or result from symptoms and behaviours associated with the ED [ 17 , 18 ]. Individuals with an ED, their carers and care providers often face a complex and important dilemma; the individual with an ED requires treatment for their ED but also for their psychiatric comorbidities, and it can be difficult for treatment providers to determine which is the clinical priority [ 19 ]. This is further complicated by the fact that EDs and comorbidities may have a reciprocal relationship, whereby the presence of one impact the pathology, treatment and outcomes of the other.
The current Rapid Review (RR) forms part of a series of reviews commissioned by the Australian Federal Government to inform the Australian National Eating Disorders Research and Translation Strategy 2021–2031 [ 20 ]. In response to the impact of psychiatric and medical comorbidities on outcomes, this rapid review summarises the recent literature on the nature and implications of psychiatric and medical comorbidities associated with EDs.
The Australian Government Commonwealth Department of Health funded the InsideOut Institute for Eating Disorders (IOI) to develop the Australian Eating Disorders Research and Translation Strategy 2021–2031 [ 20 ] under the Psych Services for Hard to Reach Groups initiative (ID 4-8MSSLE). The strategy was developed in partnership with state and national stakeholders including clinicians, service providers, researchers, and experts by lived experience (both consumers and families/carers). Developed through a two-year national consultation and collaboration process, the strategy provides the roadmap to establishing EDs as a national research priority and is the first disorder-specific strategy to be developed in consultation with the National Mental Health Commission. To inform the strategy, IOI commissioned Healthcare Management Advisors (HMA) to conduct a series of RRs to assess all available peer-reviewed literature on all DSM-5 listed EDs.
A RR Protocol [ 21 ] was utilised to allow swift synthesis of the evidence in order to guide public policy and decision-making [ 22 ]. This approach has been adopted by several leading health organisations including the World Health Organisation [ 17 ] and the Canadian Agency for Drugs and Technologies in Health Rapid Response Service [ 18 ], to build a strong evidence base in a timely and accelerated manner, without compromising quality. A RR is not designed to be as comprehensive as a systematic review—it is purposive rather than exhaustive and provides actionable evidence to guide health policy [ 23 ].
The RR is a narrative synthesis adhering to the PRISMA guidelines [ 24 ]. It is divided by topic area and presented as a series of papers. Three research databases were searched: ScienceDirect, PubMed and Ovid/Medline. To establish a broad understanding of the progress made in the field of EDs, and to capture the largest evidence base from the past 12 years (originally 2009–2019, but expanded to include the preceding two years), the eligibility criteria for included studies were kept broad. Therefore, included studies were published between 2009 and 2021, written in English, and conducted within Western healthcare systems or health systems comparable to Australia in terms of structure and resourcing. The initial search and review process was conducted by three reviewers between 5 December 2019 and 16 January 2020. The re-run for the years 2020–2021 was conducted by two reviewers at the end of May 2021.
The RR had a translational research focus with the objective of identifying evidence relevant to developing optimal care pathways. Searches therefore used a Population, Intervention, Comparison, Outcome (PICO) approach to identify literature relating to population impact, prevention and early intervention, treatment, and long-term outcomes. Purposive sampling focused on high-level evidence studies encompassing meta-analyses; systematic reviews; moderately sized randomised controlled studies (RCTs) (n > 50); moderately sized controlled-cohort studies (n > 50); and population studies (n > 500). However, the diagnoses ARFID and UFED necessitated less stringent eligibility criteria due to a paucity of published articles. As these diagnoses are newly captured in the DSM-5 (released in 2013, within the allocated search timeframe), the evidence base is still emerging, and few studies have been conducted. Thus, smaller studies (n = ≤ 20) and narrative reviews were also considered and included. Grey literature, such as clinical or practice guidelines, protocol papers (without results) and Masters’ theses or dissertations, were excluded. Other sources (which may not be replicable when applying the current methodology) included the personal libraries of authors, yielding two additional studies (see Additional file 1 ). This extra step was conducted in line with the PRISMA-S: an extension to the PRISMA Statement for Reporting Literature Searches in Systematic Reviews [ 25 ].
Full methodological details including eligibility criteria, search strategy and terms and data analysis are published in a separate protocol paper, which included a total of 1320 studies [ 26 ] (see Additional file 1 : Fig. S1 for PRISMA flow diagram). Data from included studies relating to psychiatric and medical comorbidities of EDs were synthesised and are presented in the current review. No further analyses were conducted.
The search included articles published in the period January 2009 to May 2021. The RR identified 202 studies for inclusion. Of these, 58% related to psychiatric comorbidities (n = 117) and 42% to medical comorbidities (n = 85). A full list of the studies included in this review and information about population, aims and results can be found in Additional file 2 : Tables S3, S4. Results are subdivided into two categories: (1) psychiatric comorbidities and (2) medical complications. Tables 1 and 2 provide high-level summaries of the results.
Psychiatric comorbidities
The study of psychiatric comorbidities can assist with developing models of ED aetiology, conceptualising psychopathology and has relevance for treatment development and outcomes. Given that common psychological factors are observed across psychiatric disorders [ 87 ], it is not surprising that there are high prevalence rates of co-occurring psychiatric conditions with EDs. Comorbidity rates of EDs and other psychiatric conditions are elevated further in ethnic/racial minority groups [ 88 ]. When looking at the evidence from studies conducted with children and young people, one study of children with ARFID found that 53% of the population had a lifetime comorbid psychiatric disorder [ 89 ]. It emerged from the RR that research regarding psychiatric comorbidities generally focussed on the prevalence rates of comorbidities among certain ED subgroups, with some also exploring implications for treatment and ED psychopathology.
Anxiety disorders
Research indicates that EDs and anxiety disorders frequently co-occur [ 8 , 27 ]. The high prevalence rates of anxiety disorders in the general population are also observed in people with EDs; with a large population study finding anxiety disorders were the most frequently comorbid conditions reported [ 8 ]. In a study of women presenting for ED treatment, 65% also met the criteria for at least one comorbid anxiety disorder [ 28 ]. Of note, 69% of those endorsing the comorbidity also reported that the anxiety disorder preceded the onset of the ED [ 28 ]. Another study explored anxiety across individuals with an ED categorised by three weight ranges (individuals whose weight is in the ‘healthy weight’ range, individuals in the ‘overweight’ range and individuals in the ‘obese’ range). While anxiety was elevated across all groups, the authors did note that individuals in the overweight group reported significantly higher rates of anxiety than individuals within the healthy weight group [ 90 ]. One study that explored temperamental factors provided some insight into factors that may mediate this association; anxiety sensitivity (a predictor of anxiety disorders) was associated with greater ED severity among individuals in a residential ED treatment facility [ 29 ]. Further, this association was mediated by a tendency to engage in experiential avoidance—the authors noting that individuals with greater ED symptoms were more likely to avoid distressing experiences [ 29 ].
Generalised anxiety disorder (GAD)
Studies have noted the potential genetic links between EDs and GAD, noting that the presence of one significantly increases the likelihood of the other [ 8 , 30 ]. Further, there appears to be a relationship between the severity of ED behaviours and the co-occurrence of GAD, with comorbidity more likely when fasting and excessive exercise are present, as well as a lower BMI [ 30 ]. The authors noted the particularly pernicious comorbidity of EDs (specifically AN) and GAD may be amplified by the jointly anxiolytic and weight loss effects of food restriction and excessive exercise [ 30 ].
Social anxiety
A meta-analysis of 12 studies found higher rates of social anxiety across all ED diagnoses, with patients with BN demonstrating the highest rate of comorbidity at 84.5%, followed by both BED and AN-BP both at 75% [ 31 ]. High levels of social anxiety were also associated with more severe ED psychopathology [ 31 ] and higher body weight [ 91 ]. This particular comorbidity may also impact on access to treatment for the ED; a large follow-up study of adolescents found that self-reported social phobia predicted not seeking treatment for BN symptoms [ 32 ]. Interestingly, two studies noted that anxiety symptoms improved following psychological treatments that targeted ED symptoms, possibly due to a shared symptom profile [ 29 , 31 ].
Obsessive–compulsive disorder
Similarities between the symptoms of Obsessive–Compulsive Disorder (OCD) and EDs, such as cognitive rigidity, obsessiveness, detail focus, perfectionism and compulsive routines have long been reported in the literature [ 34 ]. Given the symptom overlap, a meta-analysis sought to clarify the lifetime and current (that is, a current diagnosis at the time of data collection) comorbidity rates of OCD and EDs, noting the lifetime comorbidity rate was 18% and current comorbidity rate was 15% [ 33 ]. However, the authors noted that this prevalence may double over longer periods of observation, with some follow-up data demonstrating comorbidity rates of 33% [ 33 ]. Prevalence rates of OCD seemed to be highest among people with AN (lifetime = 19% and current = 14%) compared to other ED subtypes. In addition to the symptom crossover, this RR found evidence of a complex relationship between OCD and EDs, including a potential association between OCD and greater ED severity [ 34 ].
Network analysis found that doubts about simple everyday things and repeating things over and over bridged between ED and OCD symptoms. Further, a pathway was observed between restricting and checking compulsions and food rigidity as well as binge eating and hoarding. However, as the data was cross-sectional, directional inferences could not be made [ 36 ]. An earlier study explored how changes in OCD symptoms impact ED symptoms among an inpatient sample [ 35 ]. As was hypothesised, decreases in OCD symptoms accounted for significant variance in decreases in ED symptoms, and this effect was strongest among ED patients with comorbid OCD. The study also found that irrespective of whether patients had comorbid OCD or not, when ED symptoms improved, so did symptoms of OCD [ 35 ]. The authors concluded that perhaps there is a reciprocal relationship between OCD and ED symptoms, whereby symptoms of both conditions interact in a synergistic, bidirectional manner, meaning that improvement in one domain can lead to improvement in another [ 35 ]. These findings were somewhat supported in a study by Simpson and colleagues (2013), which found exposure and response prevention (a specialised OCD treatment) resulted in a significant reduction in OCD severity, as was expected, and an improvement in ED symptoms. In their study, individuals with BN showed more improvement than those with AN–nevertheless, BMI still increased among those underweight [ 92 ].
Mood disorders
Depression and major depressive disorder (mdd).
This RR also found high levels of comorbidity between major depression and EDs. A longitudinal study of disordered eating behaviours among adolescents found that disordered eating behaviours and depressive symptoms developed concurrently [ 37 ]. Among the sample, over half the adolescent sample had a depressive disorder. Prevalence rates were similar for AN (51.5%) and BN (54%) [ 37 ]. The study also explored the neurological predictors of comorbid depression in individuals with EDs, noting that lower grey matter volumes in the medial orbitofrontal, dorsomedial, and dorsolateral prefrontal cortices predicted the concurrent development of purging and depressive symptoms [ 37 ]. The results suggested that alterations in frontal brain circuits were part of a neural aetiology common to EDs and depression [ 37 ].
This RR found much support for a strong relationship between depression and ED symptomatology. In a study of patients with AN, comorbid MDD was associated with a greater AN symptom severity [ 93 ], and this relationship between the symptoms of MDD and AN was bidirectional in a study of adolescents undergoing treatment for AN, whereby dietary restraint predicted increased guilt and hostility (symptoms of low mood) and fear predicted further food restriction [ 94 ]. Further studies noted the association between BN, BED and NES, with a higher prevalence of depression and more significant depression symptoms [ 95 , 96 , 97 ]. However, other studies have failed to find support for this association–for example, a Swedish twin study found no association between NES and other mental health disorders [ 98 ].
The impact of the relationship between depression and EDs on treatment outcomes was variable across the studies identified by the RR. One study noted the impact of depression on attrition; patients with BN and comorbid depression attending a university clinic had the highest rates of treatment drop-out [ 99 ]. However, in a sample of patients with AN, the comorbidity of depression (or lack of) did not impact treatment outcome and the severity of depression was not associated with changes in ED symptoms [ 100 ]. This finding was supported in another study of inpatients with AN; pre-treatment depression level did not predict treatment outcome or BMI [ 101 ].
Bipolar disorders
Notable comorbidity rates between bipolar disorders (BD) and EDs were reported in the literature reviewed, however evidence about the frequency of this association was mixed. Studies noted comorbidity rates of BD and EDs ranging between 1.9% to as high as 35.8% [ 38 , 39 , 40 ]. In order to better understand the nature of comorbidity, a recent systematic review and meta-analysis found BD (including bipolar 1 disorder and bipolar 2 disorder) and ED comorbidity varied across different ED diagnostic groups (BED—12.5%, BN—7.4%, AN—3.8%) [ 102 ]. However, the authors noted the scant longitudinal studies available, particularly in paediatric samples. An analysis of comorbidity within a sample of patients with BD identified that 27% of participants also met criteria for an ED; 15% had BN, 12% had BED, and 0.2% had AN [ 103 ]. Two other studies noted considerable comorbidity rates of BD; 18.6% for binge eating [ 104 ] and 8.8% for NES [ 105 ]. Some studies suggested the co-occurrence of BD and EDs were seen most in people with AN-BP, BN and BED—all of which share a binge and/or purge symptom profile [ 38 , 106 ]. Specifically, BED and BN were the most common co-occurring EDs with BD [ 40 ], however, these EDs are also the most prevalent in the population. Therefore, it is unclear if this finding is reflective of the increased prevalence of BN and BED, or if it reflects a shared underlying psychopathology between BD and these EDs [ 40 ].
Comorbid ED-BD patients appear to experience increased ED symptom severity, poorer daily and neuropsychological functioning than patients with only a ED or BD diagnosis [ 107 ]. In an effort to understand which shared features in ED-BD relate to quality of life, one study assessed an adult sample with BD [ 108 ]. Binge eating, restriction, overevaluation of weight and shape, purging and driven exercise were associated with poorer clinical outcomes, quality of life and mood regulation [ 108 ]. Additionally, a study of patients undergoing treatment for BD noted patients with a comorbid ED had significantly poorer clinical outcomes and higher scores of depression [ 109 ]. Further, quality of life was significantly lower among patients with comorbid ED-BD [ 109 ]. The comorbidity of ED and BD has implications for intervention and clinical management, as at least one study observed higher rates of alcohol abuse and suicidality among patients with comorbid ED and BD compared to those with BD only [ 40 ].
Personality disorders
This RR identified limited research regarding the comorbidity between personality disorders (PD) and EDs. A meta-analysis sought to summarise the proportion of comorbid PDs among patients with AN and BN [ 41 ]. There was a heightened association between any type of ED and PDs, and this was significantly different to the general population. For specific PDs, the proportions of paranoid, borderline, avoidant, dependant and obsessive–compulsive PD were significantly higher in EDs than in the general population. For both AN and BN, Cluster C PDs (avoidant, dependant and obsessive–compulsive) were most frequent. The authors noted that the specific comorbidity between specific EDs and PDs appears to be associated with common traits—constriction/perfectionism and rigidity is present in both AN and obsessive–compulsive PD (which had a heightened association), as was the case with impulsivity, a characteristic of both BN and borderline PD [ 41 ]. This symptom association was also observed in a study of adolescents admitted to an ED inpatient unit whereby a significant interaction between binge-purge EDs (AN-BP and BN), childhood emotional abuse (a risk factor for PD) and borderline personality style was found [ 110 ].
This comorbidity may be associated with greater patient distress and have implications for patient outcomes [ 41 , 42 ]. Data from a nine-year observational study of individuals with BN reported that comorbidity with a PD was strongly associated with elevated mortality risk [ 111 ]. In terms of treatment outcomes, an RCT compared the one- and three-year treatment outcomes of four subgroups of women with BN, defined by PD complexity; no comorbid PD (health control), personality difficulties, simple PD and complex PD [ 112 ]. At pre-treatment, the complex PD group had greater ED psychopathology than the other three groups. Despite this initial difference, there were no differences in outcomes between groups at one-year and three-year follow up [ 112 ]. The authors suggested this result could be due to the targeting of the shared symptoms of BN and PD by the intervention delivered in this study, and that as ED symptoms improve, so do PD symptoms [ 112 ]. Suggesting that beyond symptom overlap, perhaps some symptoms attributed to the PD are better explained by the ED. This was consistent with Brietzke and colleagues’ (2011) recommendation that for individuals with ED and a comorbid PD, treatment approaches should target both conditions where possible [ 113 ].
Substance use disorders
Comorbid substance use disorders (SUDs) are also often noted in the literature as an issue that complicates treatment and outcomes of EDs [ 114 ]. A meta-analysis reported the lifetime prevalence of EDs and comorbid SUD was 27.9%, [ 43 ] with a lifetime prevalence of comorbid illicit drug use of 17.2% for AN and 18.6% for BN [ 115 ]. Alcohol, caffeine and tobacco were the most frequently reported comorbidities [ 43 ]. Further analysis of SUDs by substance type in a population-based twin sample indicated that the lifetime prevalence of an alcohol use disorder among individuals with AN was 22.4% [ 115 ]. For BN, the prevalence rate was slightly higher at 24.0% [ 115 ].
The comorbidity of SUD is considered far more common among individuals with binge/purge type EDs, evidenced by a meta-analysis finding higher rates of comorbid SUD among patients with AN-BP and BN than AN-R [ 44 ]. This trend was also observed in population data [ 116 ]. Further, a multi-site study found that patients with BN had higher rates of comorbid SUD than patients with AN, BED and Eating Disorder Not Otherwise Specific (EDNOS) (utilised DSM-IV criteria) [ 117 ]. Behaviourally, there was an association between higher frequencies of binge/purge behaviours with high rates of substance use [ 117 ]. The higher risk of substance abuse among patients with binge/purge symptomology was also associated with younger age of binge eating onset [ 118 ]. A study explored whether BN and ED subtypes with binge/purge symptoms predicted adverse outcomes and found that adolescent girls with purging disorder were significantly more likely to use drugs or frequently binge drink [ 119 ]. This association was again observed in a network analysis of college students, whereby there was an association between binge drinking and increased ED cognitions [ 120 ].
Psychosis and schizophrenia
The RR identified a small body of literature with mixed results regarding the comorbidity of ED and psychosis-spectrum symptoms. A study of patients with schizophrenia found that 12% of participants met full diagnostic criteria for NES, with a further 10% meeting partial criteria [ 45 ]. Miotto and colleagues’ (2010) study noted higher rates of paranoid ideation and psychotic symptoms in ED patients than those observed in healthy controls [ 121 ]. However, the authors concluded that these symptoms were better explained by the participant's ED diagnosis than a psychotic disorder [ 121 ]. At a large population level, an English national survey noted associations between psychotic-like experiences and uncontrolled eating, food dominance and potential EDs [ 122 ]. In particular, these associations were stronger in males [ 122 ]. However, the true comorbidity between psychotic disorders and ED remains unclear and further research is needed.
Body dysmorphic disorder
While body image disturbances common to AN, BN and BED are primarily related to weight and shape concerns, individuals with body dysmorphic disorder (BDD) have additional concerns regarding other aspects of their appearance, such as facial features and skin blemishes [ 46 , 123 ]. AN and BDD share similar psychopathology and both have a peak onset period in adolescence, although BDD development typically precedes AN [ 46 ]. The prevalence rates of BDD among individuals with AN are variable. In one clinical sample of female AN patients, 26% met BDD diagnostic criteria [ 124 ]. However, much higher rates were observed in another clinical sample of adults with AN, where 62% of patients reported clinically significant 'dysmorphic concern' [ 125 ].
As the RR has found with other mental health comorbidities, BDD contributes to greater symptom severity in individuals with AN, making the disorder more difficult to treat. However, some research suggested that improved long-term outcomes from treatments for AN are associated with the integration of strategies that address dysmorphic concerns [ 124 , 126 ]. However, there remains little research on the similarities, differences and co-occurrence of BDD and AN, and with even less research on the cooccurrence of BDD and other EDs.
Neurodevelopmental disorders
Attention deficit hyperactivity disorder
Several studies noted the comorbidity between Attention Deficit Hyperactivity Disorder (ADHD) and EDs. A systematic review found moderate evidence for a positive association between ADHD and disordered eating, particularly between overeating and ADHD [ 47 ]. The impulsivity symptoms of ADHD were particularly associated with BN for all genders, and weaker evidence was found for the association between hyperactivity and restrictive EDs (AN and ARFID) for males, but not females [ 47 ]. Another meta-analysis reported a two-fold increased risk of ADHD in individuals with an ED [ 48 ] and studies have noted particularly strong associations between ADHD and BN [ 49 , 50 ]. In a cohort of adults with a diagnosis of an ED, 31.3% had a 'possible' ADHD [ 127 ]. Another study considered sex differences; women with ADHD had a significantly higher lifetime prevalence of both AN and BN than women without ADHD [ 128 ]. Further, the comorbidity rates for BED were considerably higher among individuals with ADHD for both genders [ 128 ].
Further evidence for a significant association between ADHD and EDs was reported in a population study of children [ 51 ]. Results revealed that children with ADHD were more like to experience an ED or binge, purge, or restrictive behaviours above clinical threshold [ 51 ]. Another study of children with ADHD considered gender differences; boys with ADHD had a greater risk of binge eating than girls [ 129 ]. However, the study found no significant difference in AN's prevalence between ADHD and non-ADHD groups. Further, among patients attending an ED specialist clinic, those with comorbid ADHD symptoms had poorer outcomes at one-year follow-up [ 130 ].
Autism spectrum disorder
There is evidence of heightened prevalence rates of autism spectrum disorder (ASD) among individuals with EDs. A systematic review found an average prevalence of ASD with EDs of 22.9% compared with 2% observed in the general population [ 52 ]. With regards to AN, several studies have found symptoms of ASD to be frequently exhibited by patients with AN [ 53 , 54 ]. An assessment of common phenomena between ARFID and ASD in children found a shared symptom profile of eating difficulties, behavioural problems and sensory hypersensitivity beyond what is observed in typically developing children (the control group) [ 55 ]. While research in this area is developing, the findings indicated these comorbidities would likely have implications for the treatment and management of both conditions [ 55 ].
Post traumatic stress disorder
Many individuals with EDs report historical traumatic experiences, and for a proportion of the population, symptoms of post traumatic stress disorder (PTSD). A broad range of prevalence rates between PTSD and EDs have been reported; between 16.1–22.7% for AN, 32.4–66.2% for BN and 24.02–31.6% for BED [ 56 ]. A review noted self-criticism, low self-worth, guilt, shame, depression, anxiety, emotion dysregulation, anger and impulsivity were linked to the association between EDs and trauma [ 57 ]. It was suggested that for individuals with trauma/PTSD, EDs might have a functional role to manage PTSD symptoms and reduce negative affect [ 57 ]. Further, some ED behaviours such as restriction, binge eating, and purging may be used to avoid hyperarousal, in turn maintaining the association between EDs and PTSD [ 57 ].
Few studies have explored the impact of comorbid PTSD on ED treatment outcomes. A study of inpatients admitted to a residential ED treatment service investigated whether PTSD diagnosis at admission was associated with symptom changes [ 56 ]. Cognitive and behavioural symptoms related to the ED had decreased at discharge, however, they increased again at six-month follow up. In contrast, while PTSD diagnosis was associated with higher baseline ED symptoms, it was not related to symptom change throughout treatment or treatment dropout [ 56 ]. Given previous research identified that PTSD and EDs tend to relate to more complex courses of illness, greater rates of drop out and poorer outcomes, a study by Brewerton and colleagues [ 131 ], explored the presence of EDs in patients with PTSD admitted to a residential setting. Results showed that patients with PTSD had significantly higher scores of ED psychopathology, as well as depression, anxiety and quality of life. [ 131 ]. Further, those with PTSD had a greater tendency for binge-type EDs.
Suicidality
Suicide is one of the leading causes of death for individuals with EDs [ 58 ]. In a longitudinal study of adolescents, almost one quarter had attempted suicide, and 65% reported suicidal ideation within the past 6 months [ 37 ]. EDs are a significant risk factor for suicide, with some evidence suggesting a genetic association between suicide risk and EDs [ 59 , 60 ]. This association was supported in the analysis of Swedish population registry data, which found that individuals with a sibling with an ED had an increased risk of suicide attempts with an odds ratio of 1.4 (relative cohort n = 1,680,658) [ 61 ]. For suicide attempts, this study found an even higher odds ratio of 5.28 (relative cohort n = 2,268,786) for individuals with an ED and 5.39 (relative cohort n = 1,919,114) for death by suicide [ 61 ]. A comparison of individuals with AN and BN indicated that risk for suicide attempts was higher for those with BN compared to AN [ 61 ]. However, the opposite was true for death by suicide; which was higher in AN compared to BN [ 61 ]. This result is consistent with the findings of a meta-analysis—the incidence of suicide was higher among patients with AN compared to those with BN or BED [ 62 ].
The higher incidence of suicide in adults with AN [ 132 ] is potentially explained by the findings from Guillaume and colleagues (2011), which suggested that comparative to BN, AN patients are more likely to have more serious suicide attempts resulting in a higher risk of death [ 133 ]. However, death by suicide remains a significant risk for both diagnoses. As an example, Udo and colleagues (2019) study reported that suicide attempts were more common in those with an AN-BP subtype (44.1%) than AN-R (15.7%), or BN (31.4%) [ 134 ]. Further, in a large cohort of transgender college students with EDs, rates of past-year suicidal ideation (a significant risk factor for suicide attempts) was 75.2%, and suicide attempts were 74.8%, significantly higher than cisgender students with EDs and transgender students without EDs [ 135 ]. The RR found that the risk of suicidal ideation and behaviour was associated with ED diagnosis and the presence of other comorbidities. Among a community-based sample of female college students diagnosed with an ED, 25.6% reported suicidal ideation, and this was positively correlated with depression, anxiety and purging [ 136 ]. In support of this evidence, Sagiv and Gvion (2020) proposed a dual pathway model of risk of suicide attempt in individuals with ED, which implicates trait impulsivity and comorbid depression [ 137 ]. In two large transdiagnostic ED patient samples, suicidal ideation was associated with different aspects of self-image between ED diagnoses. For example, suicidal ideation was associated with higher levels of self-blame among individuals with BED, while among patients with AN and OSFED, increased suicidal ideation was associated with a lack of self-love [ 138 , 139 ].
Anorexia nervosa
Amongst adults with AN, higher rates of suicide have been reported amongst those with a binge-purge subtype (25%) than restrictive subtype (8.65%) [ 58 , 140 ]. Further, comorbid depression and prolonged starvation were strongly associated with elevated suicide attempts for both subtypes [ 58 , 140 ]. In another study, the risk of attempted suicide was associated with depression, but it was moderated by hospital treatment [ 93 ]. Further, suicidal ideation was related to depression. A significant 'acquired' suicide risk in individuals with AN has been identified by Selby et al. (2010) through an increased tolerance for pain and discomfort resultant from repeated exposure to painful restricting and purging behaviours [ 141 ].
Bulimia nervosa
Further research among individuals diagnosed with BN found an increased level of suicide risk [ 142 ]. Results from an extensive study of women with BN indicated that the lifetime prevalence of suicide attempts in this cohort was 26.9% [ 143 ]. In one study of individuals diagnosed with severe BN, 60% of deaths were attributed to suicide [ 144 ]. The mean age at the time of death was 29.6 years, and predictive factors included previous suicide attempts and low BMI. Further, in a sample of children and adolescents aged 7 to 18 years, higher rates of suicidal ideation were associated with BN, self-induced vomiting and a history of trauma [ 12 ].
A large population-based study of adolescents and adults explored the frequency and correlates of suicidal ideation and attempts in those who met the criteria for BN [ 145 ]. Suicidal ideation was highest in adolescents with BN (53%), followed by BED (34.4%), other non-ED psychopathology (21.3%) or no psychopathology (3.8%). A similar trend was observed for suicide plans and attempts [ 145 ]. However, for adults, suicidality was more prevalent in the BN group compared to no psychopathology, but not statistically different to the AN, BED or other psychopathology groups [ 145 ].
Consistent with Crow and colleagues’ (2014) results, in a sample of women with BN, depression had the strongest association with lifetime suicide attempts [ 146 ]. There were also associations between identity problems, cognitive dysregulation, anxiousness, insecure attachment and lifetime suicide attempts among the sample. Depression was the most pertinent association, suggesting that potential comorbid depression should be a focus of assessment and treatment among individuals with BN due to the elevated suicide risk for this group [ 146 ]. Insecure attachment is associated with childhood trauma, and a systematic review found that suicide attempts in women with BN were significantly associated with childhood abuse and familial history of EDs [ 58 ].
Binge eating disorder
The RR found mixed evidence for the association between suicidal behaviour and BED. A meta-analysis found no suicides for patients with BED [ 62 ]. However, evidence from two separate large national surveys found that a significant proportion of individuals who had a suicide attempt also had a diagnosis of BED [ 134 , 147 ].
Non-suicidal self injury
Non-suicidal self-injury (NSSI), broadly defined, is the intentional harm inflicted to one’s body without intent to die [ 148 ]. Recognising NSSI is often a precursor for suicidal ideation and behaviour [ 149 ], together with the already heightened mortality rate for EDs, several studies have examined the association between EDs and NSSI. Up to one-third of patients with EDs report NSSI at some stage in their lifetime, with over one quarter having engaged in NSSI within the previous year [ 63 ]. Similarly, a cohort study [ 148 ] found elevated rates of historical NSSI amongst patients with DSM-IV EDs; specifically EDNOS (49%), BN (41%) and AN (26%). In a Spanish sample of ED patients, the most prevalent form of NSSI was banging (64.6%) and cutting (56.9%) [ 63 ].
Further research has explored the individual factors associated with heightened rates of NSSI. Higher levels of impulsivity among patients with EDs have been associated with concomitant NSSI [ 64 ]. This was demonstrated in a longitudinal study of female students, whereby NSSI preceded purging, marking it a potential risk factor for ED onset [ 65 ]. In a study of a large clinical sample of patients with EDs and co-occurring NSSI, significantly higher levels of emotional reactivity were observed [ 150 ]. The highest levels of emotional reactivity were reported by individuals with a diagnosis of BN, who were also more likely to engage in NSSI than those with AN [ 150 ]. In Olatunji and colleagues’ (2015) cohort study, NSSI was used to regulate difficult emotions, much like other ED behaviours. NSSI functioning as a means to manage negative affect associated with EDs was further supported by Muehlenkamp and colleagues’ [ 66 ] study exploring the risk factors in inpatients admitted for an ED. The authors found significant differences in the prevalence of NSSI across ED diagnoses, although patients with binge/purge subtype EDs were more likely to engage in poly-NSSI (multiple types of NSSI). Consistent with these findings, a study of patients admitted to an ED inpatient unit found that 45% of patients displayed at least one type of NSSI [ 151 ]. The function of NSSI among ED patients was explored in two studies, one noting that avoiding or suppressing negative feelings was the most frequently reported reason for NSSI [ 151 ]. The other analysed a series of interviews and self-report questionnaires and found patients with ED and comorbid Borderline Personality Disorder (BPD) engaged in NSSI as a means of emotion regulation [ 152 ].
Medical comorbidities
The impact of EDs on physical health and the consequential medical comorbidities has been a focus of research. Many studies reported medical comorbidities resulting from prolonged malnutrition, as well as excessive exercise, binging and purging behaviours.
Cardiovascular complications
As discussed above, although suicide is a significant contributor to the mortality rate of EDs, physical and medical complications remain the primary cause of death, particularly in AN, with a high proportion of deaths thought to result from cardiovascular complications [ 153 ]. AN has attracted the most research focus given its increased risk of cardiac failure due to severe malnutrition, dehydration and electrolyte imbalances [ 67 ].
Cardiovascular complications in AN can be divided by conduction, structural and ischemic diseases. A review found that up to 87% of patients experience cardiovascular compromise shortly following onset of AN [ 153 ]. Within conduction disease, bradycardia and QT prolongation occur at a high frequency, largely due to low body weight and resultant decreased venous return to the heart. Whereas, atrioventricular block and ventricular arrhythmia are more rare [ 153 ]. Various structural cardiomyopathies are observed in AN, such as low left ventricular mass index (occurs frequently), mitral prolapse and percardial effusion (occurs moderately). Ischemic diseases such as dyslipidemia or acute myocardial infarction are more rare.
Another review identified cardiopulmonary abnormalities that are frequently observed in AN; mitral valve prolapse occurred in 25% of patients, sinus bradycardia was the most common arrhythmia, and pericardial effusion prevalence rates ranged from 15 to 30%. [ 68 ] Sudden cardiac death is thought to occur due to increased QT interval dispersion and heart rate variability. [ 68 ] A review of an inpatient database in a large retrospective cohort study found that coronary artery disease (CAD) was lower in AN patients than the general population (4.4% and 18.4%, respectively). Consistent with trends in the general population, the risk of cardiac arrest, arrhythmias and heart failure was higher in males with AN than females with AN [ 69 ].
Given that individuals with AN have compromised biology, may avoid medical care, and have higher rates of substance use, research has examined cancer incidence and prognosis among individuals with AN. A retrospective study noted higher mortality from melanoma, cancers of genital organs and cancers of unspecified sites among individuals with AN, however, there was no statistically significant difference compared to the general population [ 70 ]. No further studies of cancer in EDs were identified.
Gastrointestinal disorders
The gastrointestinal (GI) system plays a pivotal role in the development, maintenance, and treatment outcomes for EDs, with changes and implications present throughout the GI tract. More than 90% of AN patients report fullness, early satiety, abdominal distention, pain and nausea [ 68 ]. Although it is well understood that GI system complaints are complicated and exacerbated by malnutrition, purging and binge eating [ 154 , 155 ], the actual cause of the increased prevalence of GI disorders and their contribution to ED maintenance remain poorly understood.
To this end, a review aimed to determine the GI symptoms reported in two restrictive disorders (AN and ARFID), as well as the physiologic changes as a result of malnutrition and function of low body weight and the contribution of GI diseases to the disordered eating observed in AN and ARFID [ 156 ]. The review found mixed evidence regarding whether GI issues were increased in patients with AN and ARFID. This was partly due to the relatively limited amount of research in this area and mixed results across the literature. The review noted that patients with AN and ARFID reported a higher frequency of symptoms of gastroparesis. Further, there was evidence for a bidirectional relationship between AN and functional gastrointestinal disorders (FGIDs) contributing to ongoing disordered eating. The review found that GI symptoms observed in EDs develop due to (1) poorly treated medical conditions with GI-predominant symptoms, (2) the physiological and anatomical changes that develop due to malnutrition or (3) FGIDs.
There was a high rate of comorbidity (93%) between ED and FGIDs, including oesophageal, bowel and anorectal disorders, in a patient sample with AN, BN and EDNOS [ 157 ]. A retrospective study investigating increased rates of oesophageal cancer in individuals with a history of EDs could not conclude that risk was associated with purging over other confounding factors such as alcohol abuse and smoking [ 158 ].
Given that gut peptides like ghrelin, cholecystokinin (CCK), peptide tyrosine (PYY) and glucagon-like peptide 1 (GLP-1) are known to influence food intake, attention has focussed on the dysregulation of gut peptide signalling in EDs [ 159 ]. A review aimed to discuss how these peptides or the signals triggered by their release are dysregulated in EDs and whether they are normalised following weight restoration or weight loss (in the case of people with higher body weight) [ 159 ]. The results were inconsistent, with significant variability in peptide dysregulation observed across EDs [ 159 ]. A systematic review and meta-analysis explored whether ghrelin is increased in restrictive AN. The review found that all forms of ghrelin were raised in AN’s acute state during fasting [ 160 ]. In addition, the data did not support differences in ghrelin levels between AN subtypes [ 160 ]. Another study examined levels of orexigenic ghrelin and anorexigenic peptide YY (PYY) in young females with ARFID, AN and healthy controls (HC) [ 161 ]. Results demonstrated that fasting and postprandial ghrelin were lower in ARFID than AN, but there was no difference between ARFID and AN for fasting and postprandial PYY [ 161 ].
Oesophageal and gastrointestinal dysfunction have been observed in patients with AN and complicate nutritional and refeeding interventions [ 155 ]. Findings from a systematic review indicated that structural changes that occurred in the GI tract of patients with AN impacted their ability to swallow and absorb nutrients [ 162 ]. Interestingly, no differences in the severity of gastrointestinal symptoms were observed between AN-R and AN-BP subtypes [ 155 ].
A systematic review of thirteen studies aimed to identify the most effective treatment approaches for GI disorders and AN [ 163 ]. An improvement in at least one or more GI symptoms was reported in 11 of the 13 studies, with all studies including nutritional rehabilitation, and half also included concurrent psychological treatment [ 163 ]. Emerging evidence on ED comorbidity with chronic GI disorders suggested that EDs are often misdiagnosed in children and adolescents due to the crossover of symptoms. Therefore, clinicians treating children and adolescents for GI dysfunction should be aware of potential EDs and conduct appropriate screening [ 164 ]. There has been an emerging focus on the role of the gut microbiome in the regulation of core ED symptoms and psychophysiology. Increased attention is being paid to how the macronutrient composition of nutritional rehabilitation should be considered to maximise treatment outcomes. A review found that high fibre consumption in addition to prebiotic and probiotic supplementation helped balance the gut microbiome and maintained the results of refeeding [ 165 ].
Bone health
The RR found evidence for bone loss/poor bone mineral density (BMD) and EDs, particularly in AN. The high rates of bone resorption observed in patients with AN is a consequence of chronic malnutrition leading to osteoporosis (weak and brittle bones), increased fracture risk and scoliosis [ 166 ]. The negative impacts of bone loss are more pronounced in individuals with early-onset AN when the skeleton is still developing [ 67 ] and among those who have very low BMI [ 71 ], with comorbidity rates as high as 46.9% [ 71 ]. However, lowered BMD was also observed among patients with BN [ 72 ].
A review [ 167 ] explored the prevalence and differences in pathophysiology of osteoporosis and fractures in patients with AN-R and AN-BP. AN-R patients had a higher prevalence of osteoporosis, and AN-BP patients had a higher prevalence of osteopenia (loss of BMD) [ 167 ]. Further, the authors noted the significant increase in fracture risk that starts at disease onset and lasts throughout AN, with some evidence that risk remains increased beyond remission and recovery [ 167 ]. Findings from a longitudinal study of female patients with a history of adolescent AN found long-term bone thinning at five and ten-year follow-up despite these patients achieving weight restoration [ 168 ].
Given this, treatment to increase BMD in individuals with AN has been the objective of many pharmacotherapy trials, mainly investigating the efficacy of hormone replacement [ 169 , 170 ]. Treatments include oestrogen and oral contraceptives [ 169 , 170 , 171 , 172 ]; bisphosphonates [ 169 , 173 ]; other hormonal treatment [ 174 , 175 , 176 , 177 ] and vitamin D [ 178 ]. However, the outcomes of these studies were mixed.
Refeeding syndrome
Nutritional rehabilitation of severely malnourished individuals is central to routine care and medical stabilisation of patients with EDs [ 179 ]. Within inpatient treatment settings, reversing severe malnutrition is achieved using oral, or nasogastric tube feeding. However, following a period of starvation, initiating/commencing feeding has been associated with ‘refeeding syndrome’ (RFS), a potentially fatal electrolyte imbalance caused by the body's response to introducing nutritional restoration [ 180 , 181 ]. The studies identified in the RR focused predominantly on restrictive EDs/on this population group—results regarding RFS risk were mixed [ 73 ].
A retrospective cohort study of inpatients diagnosed with AN with a very low BMI implemented a nasogastric feeding routine with vitamin, potassium and phosphate supplementation [ 182 ]. All patients achieved a significant increase in body weight. None developed RFS [ 182 ], suggesting that even with extreme undernutrition, cautious feeding within a specialised unit can be done safely without RFS. For adults with AN, aminotransferases are often high upon admission, however are normalised following four weeks of enteral feeding [ 183 , 184 ]. Further, the RR identified several studies demonstrating the provision of a higher caloric diet at intake to adolescents with AN led to faster recoveries and fewer days in the hospital with no observed increased risk for RFS [ 75 , 76 , 77 ]. These findings were also noted in a study of adults with AN [ 179 ].
However, the prevalence of RFS among inpatients is highly variable, with one systematic review noting rates ranging from 0 to 62% [ 74 ]. This variability was largely a reflection of the different definitions of RFS used across the literature [ 74 ]. A retrospective review of medical records of patients with AN admitted to Intensive Care Units (ICUs) aimed to evaluate complications, particularly RFS, that occurred during the ICU stay and the impact of these complications on treatment outcomes [ 185 ]. Of the 68 patients (62 female), seven developed RFS (10.3%) [ 185 ].
Although easily detectable and treatable, hypophosphatemia (a low serum phosphate concentration) may lead to RFS which is the term used to describe severe fluid and electrolyte shifts that can occur when nutrition support is introduced after a period of starvation. Untreated hypophosphatemia may lead to characteristic signs of the RFS such as respiratory failure, heart failure, and seizures [ 76 , 179 , 186 , 187 , 188 ]. A retrospective case–control study of inpatients with severe AN identified [ 189 ]. A retrospective study of AN and atypical AN patients undergoing refeeding found that the risk of hypophosphatemia was associated with a higher level of total weight loss and recent weight loss rather than the patient’s weight at admission [ 190 ]. The safe and effective use of prophylactic phosphate supplementation during refeeding was supported by the results from Agostino and colleagues’ chart review study [ 191 ], where 90% of inpatients received supplementation during admission.
Higher calorie refeeding approaches are considered safe in most cases, however the steps necessitated to monitor health status are costly to health services [ 192 ]. The most cost-effective approach would likely involve prophylactic electrolyte supplementation in addition to high calorie refeeding, which would decrease the need for daily laboratory monitoring as well as shortening hospital stays [ 75 , 191 , 192 ]. A systematic review noted that much of the research regarding refeeding, particularly in children and young people, has been limited by small sample sizes, single-site studies and heterogeneous designs [ 181 ]. Further, the differing definitions of RFS, recovery, remission and outcomes leading to variable results. While RFS appears safe for many people requiring feeding, the risk and benefits of it are unclear [ 193 ] due to the limited research on this topic. Following current clinical practice guidelines on the safe introduction of nutrition is recommended.
Metabolic syndrome
Metabolic syndrome refers to a group of factors that increase risks for heart disease, diabetes, stroke and other related conditions [ 194 ]. Metabolic syndrome is conceptualised as five key criteria; (1) elevated waist circumference, (2) elevated triglyceride levels, (3) reduced HDL-C, (4) elevated blood pressure and (5) elevated fasting glucose. The binge eating behaviours exhibited in BN, BED and NES have been linked to the higher rates of metabolic syndrome observed in these ED patients [ 78 , 195 ].
An analysis of population data of medical comorbidities with BED noted the strongest associations were with diabetes and circulatory systems, likely indexing components of metabolic syndrome [ 196 ]. While type 1 diabetes is considered a risk factor for ED development, both BN and BED have increased risk for type 2 diabetes [ 78 ]. A 16-year observation study found that the risk of type 2 diabetes was significantly increased in male patients with BED compared to the community controls [ 78 ]. By the end of the observation period, 33% of patients with BED had developed type 2 diabetes compared to 1.7% of the control group. The prevalence of type 2 diabetes among patients with BN was also slightly elevated at 4.4% [ 78 ]. Importantly, the authors were not able to control for BMI in this study. In another study, BED was the most prevalent ED in a cohort of type 2 diabetes patients [ 197 ]. Conversely, the prevalence of AN among patients with type 2 diabetes is significantly lower, with a review of national data reporting comorbidity rates to be 0.06% [ 198 ].
Metabolic dysfunction was observed in a relatively large sample of individuals with NES, including metabolic syndrome and type 2 diabetes, with women reporting slightly higher rates (13%) than men (11%) [ 199 ]. In another group of adults with type 2 diabetes, 7% met the diagnostic criteria for NES [ 200 ]. These findings suggested a need for increased monitoring and treatment of type 2 diabetes in individuals with EDs, particularly BED and NES. Another study found BED had a significant impact on metabolic abnormalities, including elevated cholesterol and poor glycaemic control [ 201 ].
The RR identified one intervention study, which examined an intervention to address medical comorbidities associated with BN and BED [ 195 ]. The study compared cognitive behaviour therapy (CBT) to an exercise and nutrition intervention to increase physical fitness, decrease body fat percentage and reduce the risk for metabolic syndrome. While the exercise intervention improved participants' physical fitness and body composition, neither group reduced cardiovascular risk at one-year follow-up [ 195 ].
Oral health
Purging behaviour, particularly self-induced vomiting, has been associated with several oral health and gastrointestinal dysfunctions in patients with EDs. A case–control study of ED patients with binge/purge symptomology found that despite ED patients reporting an increased concern for dental issues and engaging in more frequent brushing, their oral health was poorer than controls. [ 79 ] Further, a systematic review and meta-analysis aimed to explore whether EDs increase the risk of tooth erosion [ 80 ]. The analysis found that patients with EDs had more risk of dental erosion, especially among those who self-induced vomiting [ 80 ]. These findings were also found in a large cohort study, where the increased risk for BN was associated with higher rates of dental erosion but not dental cavities [ 81 ].
However, a systematic review of 10 studies suggested that poor oral health may be common among ED patients irrespective of whether self-induced vomiting forms part of their psychopathology [ 202 ]. One study reported that AN-R patients had poorer oral health outcomes and tooth decay than BN patients [ 203 ]. Two studies identified associations between NES and poor oral health, including higher rates of missing teeth, periodontal disease [ 204 , 205 ]. Another study of a group of patients with AN, BN and EDNOS, demonstrated the impact of ED behaviours on dental soft tissue, whereby 94% of patients had oral mucosal lesions, and 3% were found to have dental erosion [ 206 ].
Vitamin deficiencies
The prolonged periods of starvation, food restriction (of caloric intake and/or food groups), purging and excessive exercise observed across the ED spectrum have detrimental impacts on micronutrient balances [ 207 ]. The impact of prolonged vitamin deficiencies in early-onset EDs can also impair brain development, substantially reducing neurocognitive function in some younger patients even after weight restoration [ 82 ]. Common micronutrient deficiencies include calcium, fat soluble vitamins, essential fatty acids selenium, zinc and B vitamins [ 183 ]. One included study looked at prevalence rates of cerebral atrophy and neurological conditions, specifically Wernicke's encephalopathy in EDs and found that these neurological conditions were very rare in people with EDs [ 208 ].
Cognitive functioning
The literature included in RR regarding the cognitive changes in ED patients with AN following weight gain was sparse. It appears that some cognitive functions affected by EDs recover following nutritional restoration, whereas others persist. Cognitive functions, such as flexibility, central coherence, decision making, attention, processing speed and memory, are hypothesised to be impacted by, and influence the maintenance of EDs. A systematic review explored whether cognitive functions improved in AN following weight gain [ 83 ]. Weight gain appeared to be associated with improved processing speed in children and adolescents. However, no improvement was observed in cognitive flexibility following weight gain. Further, the results for adults were inconclusive [ 83 ].
Reproductive health
Infertility and higher rates of poor reproductive health are strongly associated with EDs, including miscarriages, induced abortions, obstetric complications, and poorer birth outcomes [ 84 , 85 ]. Although amenorrhea is a known consequence of AN, oligomenorrhea (irregular periods) was common among individuals with BN and BED [ 86 ]. A twin study found women diagnosed with BN and BED were also more likely to have poly cystic ovarian syndrome (PCOS), leading to menstrual irregularities [ 209 ]. The prevalence of lifetime amenorrhea in this sample was 10.4%, and lifetime oligomenorrhea was 33.7%. An epidemiological study explored the association of premenstrual syndrome (PMS) and premenstrual dysphoric disorder (PMDD) in women with BN and BED and found prevalence rates as high as 42.4% for PMS and 4.2% for PMDD [ 210 ].
Given the increased rates of menstrual irregularities and issues, questions have been raised regarding whether this complication is reversed or improves with recovery. A review of five studies monitoring reproductive functions during recovery over a 6- to 18-year follow up period [ 211 ] noted no significant difference between the pooled odds of childbirth rates between the AN and general population—demonstrating that if patients undergo treatment for AN, achieve weight restoration, and continue to maintain wellness, reproductive functions can renormalise [ 211 ].
An observational study of women with AN, BN or EDNOS found higher rates of low birth rate, pre-term deliveries, caesarean deliveries, and intrauterine growth restrictions [ 84 ]. Increased caesarean delivery was also observed in a large cohort of women diagnosed with BED [ 212 ]. However, these women had higher birth weight babies [ 212 ]. Further, women with comorbid ED and epilepsy were found to have an increased risk of pregnancy-related comorbidities, including preeclampsia (gestational hypertension and signs of damage to the liver and kidneys ) , gestational diabetes and perinatal depression [ 213 ].
The results from this review identified that the symptomology and outcomes of EDs are impacted by both psychiatric and medical factors. Further, EDs have a mortality rate substantially higher than the general population, with a significant proportion of those who die from an ED dying by suicide or as a result of severe medical complications.
This RR noted high rates of psychiatric and medical comorbidities in people with EDs, with comorbidities contributing to increased ED symptom severity, maintenance of some ED behaviours, compromised functioning, and adverse treatment outcomes. Evidence suggested that early identification and management of psychiatric and medical comorbidities in people with an ED may improve response to treatment and outcomes [ 29 , 35 , 83 ].
EDs and other psychiatric conditions often shared symptoms and high levels of psychopathology crossover were noted. The most prevalent psychiatric comorbidities were anxiety disorders, mood disorders and substance use disorders [ 8 , 100 , 119 ]. perhaps unsurprising given the prevalence of these illnesses in the general population. Of concern is the elevated suicide rate noted across the ED spectrum, the highest observed in AN [ 58 , 140 , 149 ]. For people with AN, suicide attempts were mostly associated with comorbid mood and anxiety disorders [ 136 ]. The review noted elevated rates of NSSI were particularly associated with binge/purge subtype EDs [ 150 ], impulsivity and emotional dysregulation (again, an example of psychopathological overlap).
With regards to PDs, studies were limited to EDs with binge-purge symptomology. Of those included, the presence of a comorbid personality disorder and ED was associated with childhood trauma [ 110 ] and elevated mortality risk [ 111 ]. There appeared to be a link between the clinical characteristics of the ED (e.g., impulsivity, rigidity) and the comorbid PD (cluster B PDs were more associated with BN/BED and cluster C PDs were more associated with AN). There was mixed (albeit limited) evidence regarding the comorbidity between EDs and psychosis and schizophrenia, with some studies noting an association between EDs and psychotic experiences [ 45 ]. Specifically, there was an association between psychotic experiences and uncontrolled eating and food dominance, which were stronger in males [ 122 ]. In addition, the review noted the association between EDs and neurodevelopmental disorders-specifically ADHD—was associated with features of BN and ASD was more prevalent among individuals with AN [ 53 , 54 ] and ARFID [ 55 ].
EDs are complicated by medical comorbidities across the neuroendocrine, skeletal, nutritional, gastrointestinal, dental, and reproductive systems that can occur alongside, or result from the ED. The RR noted mixed evidence regarding the effectiveness and safety of enteral feeding [ 180 , 181 ], with some studies noting that RFS could be safely managed with supplementation [ 191 ]. Research also described the impacts of restrictive EDs on BMD and binge eating behaviour on metabolic disorders [ 78 , 195 ]. Purging behaviours, particularly self-induced vomiting [ 79 ], were found to increase the risk of tooth erosion [ 81 ] and damage to soft tissue within the gastrointestinal tract [ 206 ]. Further, EDs were associated with a range of reproductive health issues in women, including infertility and birth complications [ 84 ].
Whilst the RR achieved its aim of synthesising a broad scope of literature, the absence of particular ED diagnoses and other key research gaps are worth noting. A large portion of the studies identified focused on AN, for both psychiatric and medical comorbidities. This reflects the stark lack of research exploring the comorbidities for ARFID, NES, and OSFED compared to that seen with AN, BN and BED. There were no studies identified exploring the psychiatric and medical comorbidities of Pica. These gaps could in part be due to the timeline utilised in the RR search strategy, which included the transition from DSM-IV to DSM-5. The update in the DSM had significant implications for psychiatric diagnosis, with the addition of new disorders (such as Autism Spectrum Disorder and various Depressive Disorders), reorganisation (for example, moving OCD and PTSD out of anxiety disorders and into newly defined chapters) and changes in diagnostic criteria (including for AN and BN, and establishing BED as a discrete disorder). Although current understanding suggests EDs are more prevalent in females, research is increasingly demonstrating that males are not immune to ED symptoms, and the RR highlighted the disproportionate lack of male subjects included in recent ED research, particularly in the domain of psychiatric and medical comorbidities.
As the RR was broad in scope and policy-driven in intent, limitations as a result of this methodology ought to be considered. The RR only considered ‘Western’ studies, leading to the potential of important pieces of work not being included in the synthesis. In the interest of achieving a rapid synthesis, grey literature, qualitative and theoretical works, case studies or implementation research were not included, risking a loss of nuance in developing fields, such as the association and prevalence of complex/developmental trauma with EDs (most research on this comorbidity focuses on PTSD, not complex or developmental trauma) or body image dissatisfaction among different gender groups. No studies regarding the association between dissociative disorders and EDs were included in the review. However, dissociation can co-occur with EDs, particularly AN-BP and among those with a trauma history [ 214 ]. Future studies would benefit from exploring this association further, particularly as trauma becomes more recognised as a risk factor for ED development.
The review was not designed to be an exhaustive summary of all medical comorbidities. Thus, some areas of medical comorbidity may not be included, or there may be variability in the level of detail included (such as, limited studies regarding the association between cancer and EDs). Studies that explored the association between other autoimmune disorders (such as Type 1 Diabetes, Crohn’s disease, Addison’s disease, ulcerative colitis, and coeliac disease) and EDs [ 215 , 216 ] were not included. Future reviews and research should examine the associations between autoimmune disorders and the subsequent increased risk of EDs, and likewise, the association between EDs and the subsequent risk of autoimmune disorders.
An important challenge for future research is to explore the impact of comorbidity on ED identification, development and treatment processes and outcomes. Insights could be gained from exploring shared psychiatric symptomology (i.e., ARFID and ASD, BN/BED and personality disorders, and food addiction). Particularly in disorders where the psychiatric comorbidity appears to precede the ED diagnosis (as may be the case in anxiety disorders [ 28 ]) and the unique physiological complications of these EDs (e.g., the impact of ARFID on childhood development and growth). Further, treatment outcomes would benefit from future research exploring the nature of the proposed reciprocal nature between EDs and comorbidities, particularly in those instances where there is significant shared psychopathology, or the presence of ED symptoms appears to exacerbate the symptoms of the other condition—and vice versa.
The majority of research regarding the newly introduced EDs has focused on understanding their aetiology, psychopathology, and what treatments demonstrate efficacy. Further, some areas included in the review had limited included studies, for example cancer and EDs. Thus, in addition to the already discussed need for further review regarding the association between EDs and autoimmune disorders, future research should explore the nature and prevalence of comorbidity between cancers and EDs. There was variability regarding the balance of child/adolescent and adult studies across the various comorbidities. Some comorbidities are heavily researched in child and adolescent populations (such as refeeding syndrome) and others there is stark child and adolescent inclusion, with included studies only looking at adult samples. Future studies should also address specific comorbidities as they apply to groups underrepresented in current research. This includes but is not limited to gender, sexual and racial minorities, whereby prevalence rates of psychiatric comorbidities are elevated. [ 88 ] In addition, future research would benefit from considering the nature of psychiatric and medical comorbidity for subthreshold and subclinical EDs, particularly as it pertains to an opportunity to identify EDs early within certain comorbidities where ED risk is heightened.
This review has identified the psychiatric and medical comorbidities of EDs, for which there is a substantial level of literature, as well as other areas requiring further investigation. EDs are associated with a myriad of psychiatric and medical comorbidities which have significant impacts on the symptomology and outcomes of an already difficult to treat, and burdensome illness.
Availability of data and materials
Not applicable—all citations provided.
Abbreviations
Anorexia nervosa—restricting type
Anorexia nervosa—binge-purge type
Avoidant restrictive food intake disorder
Body mass index
Borderline personality disorder
Diagnostic and statistical manual of mental disorders, 5th edition
Eating disorder
Generalised anxiety disorder
International classification of diseases, 11th edition
Major depressive disorder
Night eating syndrome
Other specified feeding or eating disorder
Post-traumatic stress disorder
Rapid review
Brandsma L. Eating disorders across the lifespan. J Women Aging. 2007;19(1–2):155–72.
Article PubMed Google Scholar
van Hoeken D, Hoek HW. Review of the burden of eating disorders: mortality, disability, costs, quality of life, and family burden. Curr Opin Psychiatry. 2020;33(6):521–7.
Article PubMed PubMed Central Google Scholar
Weigel A, Löwe B, Kohlmann S. Severity of somatic symptoms in outpatients with anorexia and bulimia nervosa. Eur Eat Disord Rev. 2019;27(2):195–204.
Hudson JI, Hiripi E, Pope HG Jr, Kessler RC. The prevalence and correlates of eating disorders in the National Comorbidity Survey Replication. Biol Psychiatry. 2007;61(3):348–58.
Jahraus J. Medical complications of eating disorders. Psychiatr Ann. 2018;48(10):463–7.
Article Google Scholar
Udo T, Grilo CM. Psychiatric and medical correlates of DSM-5 eating disorders in a nationally representative sample of adults in the United States. Int J Eat Disord. 2019;52(1):42–50.
Grenon R, Tasca GA, Cwinn E, Coyle D, Sumner A, Gick M, et al. Depressive symptoms are associated with medication use and lower health-related quality of life in overweight women with binge eating disorder. Womens Health Issues. 2010;20(6):435–40.
Ulfvebrand S, Birgegård A, Norring C, Högdahl L, von Hausswolff-Juhlin Y. Psychiatric comorbidity in women and men with eating disorders results from a large clinical database. Psychiatry Res. 2015;230(2):294–9.
Sachs KV, Harnke B, Mehler PS, Krantz MJ. Cardiovascular complications of anorexia nervosa: a systematic review. Int J Eat Disord. 2016;49(3):238–48.
Smith AR, Zuromski KL, Dodd DR. Eating disorders and suicidality: what we know, what we don’t know, and suggestions for future research. Curr Opin Psychol. 2018;22:63–7.
Arcelus J, Mitchell AJ, Wales J, Nielsen S. Mortality rates in patients with anorexia nervosa and other eating disorders: a meta-analysis of 36 studies. Arch Gen Psychiatry. 2011;68(7):724–31.
Mayes SD, Fernandez-Mendoza J, Baweja R, Calhoun S, Mahr F, Aggarwal R, et al. Correlates of suicide ideation and attempts in children and adolescents with eating disorders. Eat Disord. 2014;22(4):352–66.
Hart LM, Granillo MT, Jorm AF, Paxton SJ. Unmet need for treatment in the eating disorders: a systematic review of eating disorder specific treatment seeking among community cases. Clin Psychol Rev. 2011;31(5):727–35.
Kaplan AS, Garfinkel PE. Difficulties in treating patients with eating disorders: A review of patient and clinician variables. Can J Psychiatry. 1999;44(7):665–70.
Eddy KT, Tabri N, Thomas JJ, Murray HB, Keshaviah A, Hastings E, et al. Recovery from anorexia nervosa and bulimia nervosa at 22-year follow-up. J Clin Psychiatry. 2017;78(2):184–9.
John A, Marchant A, Demmler J, Tan J, DelPozo-Banos M. Clinical management and mortality risk in those with eating disorders and self-harm: e-cohort study using the SAIL databank. BJPsych Open. 2021;7(2):1–8.
Monteleone P, Brambilla F. Multiple comorbidities in people with eating disorders. In: Comorbidity of mental and physical disorders. vol. 179. Karger Publishers; 2015. p. 66-80.
Van Alsten SC, Duncan AE. Lifetime patterns of comorbidity in eating disorders: an approach using sequence analysis. Eur Eat Disord Rev. 2020;28(6):709–23.
National Institute of Health and Care Excellence. Managing comorbid health problems in people with eating disorders. United Kingdom: National Institute of Health and Care Excellence. 2019.
Institute InsideOut. Australian Eating Disorders Research and Translation Strategy 2021–2031. Sydney: The University of Sydney; 2021.
Google Scholar
Virginia Commonwealth University. Rapid review protocol. 2018.
Brooks SK, Webster RK, Smith LE, Woodland L, Wessely S, Greenberg N, et al. The psychological impact of quarantine and how to reduce it: rapid review of the evidence. Lancet. 2020;395(10227):912–20.
Hamel C, Michaud A, Thuku M, Skidmore B, Stevens A, Nussbaumer-Streit B, et al. Defining rapid reviews: a systematic scoping review and thematic analysis of definitions and defining characteristics of rapid reviews. J Clin Epidemiol. 2020;129:74–85.
Moher D, Liberati A, Tetzlaff J, Altman DG. Preferred reporting items for systematic reviews and meta-analyses: the PRISMA statement. PLOS Med. 2009;6(7):1–6.
Rethlefsen ML, Kirtley S, Waffenschmidt S, Ayala AP, Moher D, Page MJ, et al. PRISMA-S: an extension to the PRISMA statement for reporting literature searches in systematic reviews. Syst Rev. 2021;10(1):39.
Aouad P, Bryant E, Maloney D, Marks P, Le A, Russell H, et al. Informing the development of Australia’s national eating disorders research and translation strategy: a rapid review methodology. J Eat Disord. 2022;10(1):31.
Godart N, Radon L, Curt F, Duclos J, Perdereau F, Lang F, et al. Mood disorders in eating disorder patients: prevalence and chronology of ONSET. J Affect Disord. 2015;185:115–22.
Swinbourne J, Hunt C, Abbott M, Russell J, St Clare T, Touyz S. The comorbidity between eating disorders and anxiety disorders: Prevalence in an eating disorder sample and anxiety disorder sample. Aust N Z J Psychiatry. 2012;46(2):118–31.
Espel-Huynh HM, Muratore AF, Virzi N, Brooks G, Zandberg LJ. Mediating role of experiential avoidance in the relationship between anxiety sensitivity and eating disorder psychopathology: a clinical replication. Eat Behav. 2019;34:101308.
Thornton LM, Dellava JE, Root TL, Lichtenstein P, Bulik CM. Anorexia nervosa and generalized anxiety disorder: further explorations of the relation between anxiety and body mass index. J Anxiety Disord. 2011;25(5):727–30.
Kerr-Gaffney J, Harrison A, Tchanturia K. Social anxiety in the eating disorders: a systematic review and meta-analysis. Psychol Med. 2018;48(15):2477–91.
Ranta K, Väänänen J, Fröjd S, Isomaa R, Kaltiala-Heino R, Marttunen M. Social phobia, depression and eating disorders during middle adolescence: longitudinal associations and treatment seeking. Nord J Psychiatry. 2017;71(8):605–13.
Mandelli L, Draghetti S, Albert U, De Ronchi D, Atti A-R. Rates of comorbid obsessive-compulsive disorder in eating disorders: a meta-analysis of the literature. J Affect Disord. 2020;277:927–39.
Finzi-Dottan R, Zubery E. The role of depression and anxiety in impulsive and obsessive-compulsive behaviors among anorexic and bulimic patients. Eat Disord. 2009;17(2):162–82.
Olatunji BO, Tart CD, Shewmaker S, Wall D, Smits JA. Mediation of symptom changes during inpatient treatment for eating disorders: the role of obsessive–compulsive features. J Psychiatr Res. 2010;44(14):910–6.
Vanzhula IA, Kinkel-Ram SS, Levinson CA. Perfectionism and difficulty controlling thoughts bridge eating disorder and obsessive-compulsive disorder symptoms: a network analysis. J Affect Disord. 2021;283:302–9.
Zhang Z, Robinson L, Jia T, Quinlan EB, Tay N, Chu C, et al. Development of disordered eating behaviors and comorbid depressive symptoms in adolescence: neural and psychopathological predictors. Biol Psychiatry. 2020;90(12):853–62.
Thiebaut S, Godart N, Radon L, Courtet P, Guillaume S. Crossed prevalence results between subtypes of eating disorder and bipolar disorder: a systematic review of the literature. L’encephale. 2019;45(1):60–73.
Crow S, Blom TJ, Sim L, Cuellar-Barboza AB, Biernacka JM, Frye MA, et al. Factor analysis of the eating disorder diagnostic scale in individuals with bipolar disorder. Eat Behav. 2019;33:30–3.
McDonald CE, Rossell SL, Phillipou A. The comorbidity of eating disorders in bipolar disorder and associated clinical correlates characterised by emotion dysregulation and impulsivity: a systematic review. J Affect Disord. 2019;259:228–43.
Martinussen M, Friborg O, Schmierer P, Kaiser S, Øvergård KT, Neunhoeffer A-L, et al. The comorbidity of personality disorders in eating disorders: a meta-analysis. Eat Weight Disord Stud Anorex Bulim Obes. 2017;22(2):201–9.
Vrabel KR, Rø Ø, Martinsen EW, Hoffart A, Rosenvinge JH. Five-year prospective study of personality disorders in adults with longstanding eating disorders. Int J Eat Disord. 2010;43(1):22–8.
PubMed Google Scholar
Bahji A, Mazhar MN, Hudson CC, Nadkarni P, MacNeil BA, Hawken E. Prevalence of substance use disorder comorbidity among individuals with eating disorders: A systematic review and meta-analysis. Psychiatry Res. 2019;273:58–66.
Calero-Elvira A, Krug I, Davis K, Lopez C, Fernández-Aranda F, Treasure J. Meta-analysis on drugs in people with eating disorders. Eur Eat Disord Rev Prof J Eat Disord Assoc. 2009;17(4):243–59.
Palmese LB, Ratliff JC, Reutenauer EL, Tonizzo KM, Grilo CM, Tek C. Prevalence of night eating in obese individuals with schizophrenia and schizoaffective disorder. Compr Psychiatry. 2013;54(3):276–81.
Hartmann AS, Greenberg JL, Wilhelm S. The relationship between anorexia nervosa and body dysmorphic disorder. Clin Psychol Rev. 2013;33(5):675–85.
Kaisari P, Dourish CT, Higgs S. Attention deficit hyperactivity disorder (ADHD) and disordered eating behaviour: a systematic review and a framework for future research. Clin Psychol Rev. 2017;53:109–21.
Nazar BP, Bernardes C, Peachey G, Sergeant J, Mattos P, Treasure J. The risk of eating disorders comorbid with attention-deficit/hyperactivity disorder: a systematic review and meta-analysis. Int J Eat Disord. 2016;49(12):1045–57.
Seitz J, Kahraman-Lanzerath B, Legenbauer T, Sarrar L, Herpertz S, Salbach-Andrae H, et al. The role of impulsivity, inattention and comorbid ADHD in patients with bulimia nervosa. PLoS ONE. 2013;8(5):e63891.
Ziobrowski H, Brewerton TD, Duncan AE. Associations between ADHD and eating disorders in relation to comorbid psychiatric disorders in a nationally representative sample. Psychiatry Res. 2018;260:53–9.
Bleck JR, DeBate RD, Olivardia R. The comorbidity of ADHD and eating disorders in a nationally representative sample. J Behav Health Serv Res. 2015;42(4):437–51.
Huke V, Turk J, Saeidi S, Kent A, Morgan JF. Autism spectrum disorders in eating disorder populations: a systematic review. Eur Eat Disord Rev. 2013;21(5):345–51.
Westwood H, Mandy W, Tchanturia K. Clinical evaluation of autistic symptoms in women with anorexia nervosa. Mol Autism. 2017;8(1):1–9.
Dell’Osso L, Carpita B, Gesi C, Cremone I, Corsi M, Massimetti E, et al. Subthreshold autism spectrum disorder in patients with eating disorders. Compr Psychiatry. 2018;81:66–72.
Dovey TM, Kumari V, Blissett J. Eating behaviour, behavioural problems and sensory profiles of children with avoidant/restrictive food intake disorder (ARFID), autistic spectrum disorders or picky eating: same or different? Eur Psychiatry. 2019;61:56–62.
Mitchell KS, Singh S, Hardin S, Thompson-Brenner H. The impact of comorbid posttraumatic stress disorder on eating disorder treatment outcomes: investigating the unified treatment model. Int J Eat Disord. 2021;54(7):1260–9.
Mitchell KS, Scioli ER, Galovski T, Belfer PL, Cooper Z. Posttraumatic stress disorder and eating disorders: maintaining mechanisms and treatment targets. Eat Disord. 2021;29(3):292–306.
Goldstein A, Gvion Y. Socio-demographic and psychological risk factors for suicidal behavior among individuals with anorexia and bulimia nervosa: a systematic review. J Affect Disord. 2019;245:1149–67.
Pisetsky EM, Peterson CB, Mitchell JE, Wonderlich SA, Crosby RD, Le Grange D, et al. A comparison of the frequency of familial suicide attempts across eating disorder diagnoses. Int J Eat Disord. 2017;50(6):707–10.
Thornton LM, Welch E, Munn-Chernoff MA, Lichtenstein P, Bulik CM. Anorexia nervosa, major depression, and suicide attempts: shared genetic factors. Suicide Life Threat Behav. 2016;46(5):525–34.
Yao S, Kuja-Halkola R, Thornton LM, Runfola CD, D’Onofrio BM, Almqvist C, et al. Familial liability for eating disorders and suicide attempts: evidence from a population registry in Sweden. JAMA Psychiatry. 2016;73(3):284–91.
Preti A, Rocchi MBL, Sisti D, Camboni M, Miotto P. A comprehensive meta-analysis of the risk of suicide in eating disorders. Acta Psychiatr Scand. 2011;124(1):6–17.
Pérez S, Marco JH, Cañabate M. Non-suicidal self-injury in patients with eating disorders: prevalence, forms, functions, and body image correlates. Compr Psychiatry. 2018;84:32–8.
Claes L, Islam MA, Fagundo AB, Jimenez-Murcia S, Granero R, Agüera Z, et al. The relationship between non-suicidal self-injury and the UPPS-P impulsivity facets in eating disorders and healthy controls. PLoS ONE. 2015;10(5):e0126083.
Riley EN, Davis HA, Combs JL, Jordan CE, Smith GT. Nonsuicidal self-injury as a risk factor for purging onset: Negatively reinforced behaviours that reduce emotional distress. Eur Eat Disord Rev. 2016;24(1):78–82.
Muehlenkamp JJ, Claes L, Smits D, Peat CM, Vandereycken W. Non-suicidal self-injury in eating disordered patients: a test of a conceptual model. Psychiatry Res. 2011;188(1):102–8.
Gosseaume C, Dicembre M, Bemer P, Melchior J-C, Hanachi M. Somatic complications and nutritional management of anorexia nervosa. Clin Nutr Exp. 2019;28:2–10.
Cass K, McGuire C, Bjork I, Sobotka N, Walsh K, Mehler PS. Medical complications of anorexia nervosa. Psychosomatics. 2020;61(6):625–31.
Kalla A, Krishnamoorthy P, Gopalakrishnan A, Garg J, Patel N, Figueredo V. Gender and age differences in cardiovascular complications in anorexia nervosa patients. Int J Cardiol. 2017;227:55–7.
Karamanis G, Skalkidou A, Tsakonas G, Brandt L, Ekbom A, Ekselius L, et al. Cancer incidence and mortality patterns in women with anorexia nervosa. Int J Cancer. 2014;134(7):1751–7.
Hofman M, Landewé-Cleuren S, Wojciechowski F, Kruseman AN. Prevalence and clinical determinants of low bone mineral density in anorexia nervosa. Eur J Intern Med. 2009;20(1):80–4.
Robinson L, Aldridge V, Clark E, Misra M, Micali N. A systematic review and meta-analysis of the association between eating disorders and bone density. Osteoporos Int. 2016;27(6):1953–66.
Rizzo SM, Douglas JW, Lawrence JC. Enteral nutrition via nasogastric tube for refeeding patients with anorexia nervosa: a systematic review. Nutr Clin Pract. 2019;34(3):359–70.
Cioffi I, Ponzo V, Pellegrini M, Evangelista A, Bioletto F, Ciccone G, et al. The incidence of the refeeding syndrome. A systematic review and meta-analyses of literature. Clin Nutr. 2021;40(6):3688–701.
Golden NH, Keane-Miller C, Sainani KL, Kapphahn CJ. Higher caloric intake in hospitalized adolescents with anorexia nervosa is associated with reduced length of stay and no increased rate of refeeding syndrome. J Adolesc Health. 2013;53(5):573–8.
Garber AK, Mauldin K, Michihata N, Buckelew SM, Shafer M-A, Moscicki A-B. Higher calorie diets increase rate of weight gain and shorten hospital stay in hospitalized adolescents with anorexia nervosa. J Adolesc Health. 2013;53(5):579–84.
O’Connor G, Nicholls D, Hudson L, Singhal A. Refeeding low weight hospitalized adolescents with anorexia nervosa: a multicenter randomized controlled trial. Nutr Clin Pract. 2016;31(5):681–9.
Raevuori A, Suokas J, Haukka J, Gissler M, Linna M, Grainger M, et al. Highly increased risk of type 2 diabetes in patients with binge eating disorder and bulimia nervosa. Int J Eat Disord. 2015;48(6):555–62.
Conviser JH, Fisher SD, Mitchell KB. Oral care behavior after purging in a sample of women with bulimia nervosa. J Am Dent Assoc. 2014;145(4):352–4.
Hermont AP, Oliveira PA, Martins CC, Paiva SM, Pordeus IA, Auad SM. Tooth erosion and eating disorders: a systematic review and meta-analysis. PLoS ONE. 2014;9(11):e111123.
Hermont AP, Pordeus IA, Paiva SM, Abreu MHNG, Auad SM. Eating disorder risk behavior and dental implications among adolescents. Int J Eat Disord. 2013;46(7):677–83.
Peebles R, Sieke EH. Medical complications of eating disorders in youth. Child Adolesc Psychiatr Clin. 2019;28(4):593–615.
Hemmingsen SD, Wesselhoeft R, Lichtenstein MB, Sjögren JM, Støving RK. Cognitive improvement following weight gain in patients with anorexia nervosa: a systematic review. Eur Eat Disord Rev. 2021;29(3):402–26.
Pasternak Y, Weintraub AY, Shoham-Vardi I, Sergienko R, Guez J, Wiznitzer A, et al. Obstetric and perinatal outcomes in women with eating disorders. J Womens Health. 2012;21(1):61–5.
Linna MS, Raevuori A, Haukka J, Suvisaari JM, Suokas JT, Gissler M. Reproductive health outcomes in eating disorders. Int J Eat Disord. 2013;46(8):826–33.
Martini MG, Solmi F, Krug I, Karwautz A, Wagner G, Fernandez-Aranda F, et al. Associations between eating disorder diagnoses, behaviors, and menstrual dysfunction in a clinical sample. Arch Womens Ment Health. 2016;19(3):553–7.
Clarke E, Kiropoulos LA. Mediating the relationship between neuroticism and depressive, anxiety and eating disorder symptoms: The role of intolerance of uncertainty and cognitive flexibility. J Affect Disord Rep. 2021;4:100101.
Grilo CM, White MA, Barnes RD, Masheb RM. Psychiatric disorder co-morbidity and correlates in an ethnically diverse sample of obese patients with binge eating disorder in primary care settings. Compr Psychiatry. 2013;54(3):209–16.
Kambanis PE, Kuhnle MC, Wons OB, Jo JH, Keshishian AC, Hauser K, et al. Prevalence and correlates of psychiatric comorbidities in children and adolescents with full and subthreshold avoidant/restrictive food intake disorder. Int J Eat Disord. 2020;53(2):256–65.
Balantekin KN, Grammer AC, Fitzsimmons-Craft EE, Eichen DE, Graham AK, Monterubio GE, et al. Overweight and obesity are associated with increased eating disorder correlates and general psychopathology in university women with eating disorders. Eat Behav. 2021;41:101482.
Spettigue W, Obeid N, Santos A, Norris M, Hamati R, Hadjiyannakis S, et al. Binge eating and social anxiety in treatment-seeking adolescents with eating disorders or severe obesity. Eat Weight Disord Stud Anorex Bulim Obes. 2020;25(3):787–93.
Simpson HB, Wetterneck CT, Cahill SP, Steinglass JE, Franklin ME, Leonard RC, et al. Treatment of obsessive-compulsive disorder complicated by comorbid eating disorders. Cogn Behav Ther. 2013;42(1):64–76.
Fennig S, Hadas A. Suicidal behavior and depression in adolescents with eating disorders. Nord J Psychiatry. 2010;64(1):32–9.
Pila E, Murray SB, Le Grange D, Sawyer SM, Hughes EK. Reciprocal relations between dietary restraint and negative affect in adolescents receiving treatment for anorexia nervosa. J Abnorm Psychol. 2019;128(2):129–39.
Touchette E, Henegar A, Godart NT, Pryor L, Falissard B, Tremblay RE, et al. Subclinical eating disorders and their comorbidity with mood and anxiety disorders in adolescent girls. Psychiatry Res. 2011;185(1–2):185–92.
Carriere C, Michel G, Féart C, Pellay H, Onorato O, Barat P, et al. Relationships between emotional disorders, personality dimensions, and binge eating disorder in French obese adolescents. Arch Pediatr. 2019;26(3):138–44.
Kucukgoncu S, Tek C, Bestepe E, Musket C, Guloksuz S. Clinical features of night eating syndrome among depressed patients. Eur Eat Disord Rev. 2014;22(2):102–8.
Lundgren JD, Allison KC, Stunkard AJ, Bulik CM, Thornton LM, Lindroos AK, et al. Lifetime medical and psychiatric comorbidity of night eating behavior in the Swedish Twin Study of Adults: Genes and Environment (STAGE). Psychiatry Res. 2012;199(2):145–9.
Schnicker K, Hiller W, Legenbauer T. Drop-out and treatment outcome of outpatient cognitive–behavioral therapy for anorexia nervosa and bulimia nervosa. Compr Psychiatry. 2013;54(7):812–23.
Calugi S, El Ghoch M, Conti M, Dalle GR. Depression and treatment outcome in anorexia nervosa. Psychiatry Res. 2014;218(1–2):195–200.
Voderholzer U, Witte S, Schlegl S, Koch S, Cuntz U, Schwartz C. Association between depressive symptoms, weight and treatment outcome in a very large anorexia nervosa sample. Eat Weight Disord Stud Anorex Bulim Obes. 2016;21(1):127–31.
Fornaro M, Daray FM, Hunter F, Anastasia A, Stubbs B, De Berardis D, et al. The prevalence, odds and predictors of lifespan comorbid eating disorder among people with a primary diagnosis of bipolar disorders, and vice-versa: systematic review and meta-analysis. J Affect Disord. 2021;280:409–31.
McElroy SL, Crow S, Blom TJ, Biernacka JM, Winham SJ, Geske J, et al. Prevalence and correlates of DSM-5 eating disorders in patients with bipolar disorder. J Affect Disord. 2016;191:216–21.
Boulanger H, Tebeka S, Girod C, Lloret-Linares C, Meheust J, Scott J, et al. Binge eating behaviours in bipolar disorders. J Affect Disord. 2018;225:482–8.
Melo MCA, de Oliveira RM, de Araújo CFC, de Mesquita LMF, de Bruin PFC, de Bruin VMS. Night eating in bipolar disorder. Sleep Med. 2018;48:49–52.
McElroy SL, Frye MA, Hellemann G, Altshuler L, Leverich GS, Suppes T, et al. Prevalence and correlates of eating disorders in 875 patients with bipolar disorder. J Affect Disord. 2011;128(3):191–8.
Thiebaut S, Jaussent I, Maïmoun L, Beziat S, Seneque M, Hamroun D, et al. Impact of bipolar disorder on eating disorders severity in real-life settings. J Affect Disord. 2019;246:867–72.
McAulay C, Mond J, Outhred T, Malhi GS, Touyz S. Eating disorder features in bipolar disorder: clinical implications. J Mental Health. 2021:1–11.
Seixas C, Miranda-Scippa Â, Nery-Fernandes F, Andrade-Nascimento M, Quarantini LC, Kapczinski F, et al. Prevalence and clinical impact of eating disorders in bipolar patients. Braz J Psychiatry. 2012;34(1):66–70.
Spiegel J, Arnold S, Salbach H, Gotti E, Pfeiffer E, Lehmkuhl U, et al. Emotional abuse interacts with borderline personality in adolescent inpatients with binge-purging eating disorders. Eat Weight Disord Stud Anorex Bulim Obes. 2021;27:131–8.
Himmerich H, Hotopf M, Shetty H, Schmidt U, Treasure J, Hayes RD, et al. Psychiatric comorbidity as a risk factor for the mortality of people with bulimia nervosa. Soc Psychiatry Psychiatr Epidemiol. 2019;54(7):813–21.
Rowe SL, Jordan J, McIntosh VV, Carter FA, Frampton C, Bulik CM, et al. Complex personality disorder in bulimia nervosa. Compr Psychiatry. 2010;51(6):592–8.
Brietzke E, Moreira CL, Toniolo RA, Lafer B. Clinical correlates of eating disorder comorbidity in women with bipolar disorder type I. J Affect Disord. 2011;130(1–2):162–5.
Harrop EN, Marlatt GA. The comorbidity of substance use disorders and eating disorders in women: prevalence, etiology, and treatment. Addict Behav. 2010;35(5):392–8.
Baker JH, Mitchell KS, Neale MC, Kendler KS. Eating disorder symptomatology and substance use disorders: prevalence and shared risk in a population based twin sample. Int J Eat Disord. 2010;43(7):648–58.
Root TL, Pisetsky EM, Thornton L, Lichtenstein P, Pedersen NL, Bulik CM. Patterns of co-morbidity of eating disorders and substance use in Swedish females. Psychol Med. 2010;40(1):105–15.
Fouladi F, Mitchell JE, Crosby RD, Engel SG, Crow S, Hill L, et al. Prevalence of alcohol and other substance use in patients with eating disorders. Eur Eat Disord Rev. 2015;23(6):531–6.
Brewerton TD, Rance SJ, Dansky BS, O’Neil PM, Kilpatrick DG. A comparison of women with child-adolescent versus adult onset binge eating: Results from the national women’s study. Int J Eat Disord. 2014;47(7):836–43.
Field AE, Sonneville KR, Micali N, Crosby RD, Swanson SA, Laird NM, et al. Prospective association of common eating disorders and adverse outcomes. Pediatrics. 2012;130(2):e289–95.
Cusack CE, Christian C, Drake JE, Levinson CA. A network analysis of eating disorder symptoms and co-occurring alcohol misuse among heterosexual and sexual minority college women. Addict Behav. 2021;118:106867.
Miotto P, Pollini B, Restaneo A, Favaretto G, Sisti D, Rocchi MB, et al. Symptoms of psychosis in anorexia and bulimia nervosa. Psychiatry Res. 2010;175(3):237–43.
Koyanagi A, Stickley A, Haro JM. Psychotic-like experiences and disordered eating in the English general population. Psychiatry Res. 2016;241:26–34.
Phillipou A, Castle DJ, Rossell SL. Direct comparisons of anorexia nervosa and body dysmorphic disorder: a systematic review. Psychiatry Res. 2019;274:129–37.
Cerea S, Bottesi G, Grisham JR, Ghisi M. Non-weight-related body image concerns and body dysmorphic disorder prevalence in patients with anorexia nervosa. Psychiatry Res. 2018;267:120–5.
Beilharz F, Phillipou A, Castle D, Jenkins Z, Cistullo L, Rossell S. Dysmorphic concern in anorexia nervosa: implications for recovery. Psychiatry Res. 2019;273:657–61.
Beilharz F, Castle D, Grace S, Rossell S. A systematic review of visual processing and associated treatments in body dysmorphic disorder. Acta Psychiatr Scand. 2017;136(1):16–36.
Svedlund NE, Norring C, Ginsberg Y, von Hausswolff-Juhlin Y. Symptoms of attention deficit hyperactivity disorder (ADHD) among adult eating disorder patients. BMC Psychiatry. 2017;17(1):1–9.
Brewerton TD, Duncan AE. Associations between attention deficit hyperactivity disorder and eating disorders by gender: results from the national comorbidity survey replication. Eur Eat Disord Rev. 2016;24(6):536–40.
Bisset M, Rinehart N, Sciberras E. DSM-5 eating disorder symptoms in adolescents with and without attention-deficit/hyperactivity disorder: a population-based study. Int J Eat Disord. 2019;52(7):855–62.
Svedlund NE, Norring C, Ginsberg Y, von Hausswolff-Juhlin Y. Are treatment results for eating disorders affected by ADHD symptoms? A one-year follow-up of adult females. Eur Eat Disord Rev. 2018;26(4):337–45.
Brewerton TD, Perlman MM, Gavidia I, Suro G, Genet J, Bunnell DW. The association of traumatic events and posttraumatic stress disorder with greater eating disorder and comorbid symptom severity in residential eating disorder treatment centers. Int J Eat Disord. 2020;53(12):2061–6.
Bühren K, Schwarte R, Fluck F, Timmesfeld N, Krei M, Egberts K, et al. Comorbid psychiatric disorders in female adolescents with first-onset anorexia nervosa. Eur Eat Disord Rev. 2014;22(1):39–44.
Guillaume S, Jaussent I, Olie E, Genty C, Bringer J, Courtet P, et al. Characteristics of suicide attempts in anorexia and bulimia nervosa: a case–control study. PLoS ONE. 2011;6(8):e23578.
Udo T, Bitley S, Grilo CM. Suicide attempts in US adults with lifetime DSM-5 eating disorders. BMC Med. 2019;17(1):1–13.
Duffy ME, Henkel KE, Joiner TE. Prevalence of self-injurious thoughts and behaviors in transgender individuals with eating disorders: a national study. J Adolesc Health. 2019;64(4):461–6.
Goel NJ, Sadeh-Sharvit S, Flatt RE, Trockel M, Balantekin KN, Fitzsimmons-Craft EE, et al. Correlates of suicidal ideation in college women with eating disorders. Int J Eat Disord. 2018;51(6):579–84.
Sagiv E, Gvion Y. A multi factorial model of self-harm behaviors in Anorexia-nervosa and Bulimia-nervosa. Compr Psychiatry. 2020;96:152142.
Andersén M, Birgegård A. D iagnosis-specific self-image predicts longitudinal suicidal ideation in adult eating disorders. Int J Eat Disord. 2017;50(8):970–8.
Runfola CD, Thornton LM, Pisetsky EM, Bulik CM, Birgegård A. Self-image and suicide in a Swedish national eating disorders clinical register. Compr Psychiatry. 2014;55(3):439–49.
Forcano L, Álvarez E, Santamaría JJ, Jimenez-Murcia S, Granero R, Penelo E, et al. Suicide attempts in anorexia nervosa subtypes. Compr Psychiatry. 2011;52(4):352–8.
Selby EA, Smith AR, Bulik CM, Olmsted MP, Thornton L, McFarlane TL, et al. Habitual starvation and provocative behaviors: two potential routes to extreme suicidal behavior in anorexia nervosa. Behav Res Ther. 2010;48(7):634–45.
Bodell LP, Joiner TE, Keel PK. Comorbidity-independent risk for suicidality increases with bulimia nervosa but not with anorexia nervosa. J Psychiatr Res. 2013;47(5):617–21.
Forcano L, Fernández-Aranda F, Alvarez-Moya E, Bulik C, Granero R, Gratacos M, et al. Suicide attempts in bulimia nervosa: personality and psychopathological correlates. Eur Psychiatry. 2009;24(2):91–7.
Huas C, Godart N, Caille A, Pham-Scottez A, Foulon C, Divac SM, et al. Mortality and its predictors in severe bulimia nervosa patients. Eur Eat Disord Rev. 2013;21(1):15–9.
Crow SJ, Swanson SA, le Grange D, Feig EH, Merikangas KR. Suicidal behavior in adolescents and adults with bulimia nervosa. Compr Psychiatry. 2014;55(7):1534–9.
Pisetsky EM, Wonderlich SA, Crosby RD, Peterson CB, Mitchell JE, Engel SG, et al. Depression and personality traits associated with emotion dysregulation: correlates of suicide attempts in women with bulimia nervosa. Eur Eat Disord Rev. 2015;23(6):537–44.
Brown KL, LaRose JG, Mezuk B. The relationship between body mass index, binge eating disorder and suicidality. BMC Psychiatry. 2018;18(1):1–9.
Olatunji BO, Cox R, Ebesutani C, Wall D. Self-harm history predicts resistance to inpatient treatment of body shape aversion in women with eating disorders: The role of negative affect. J Psychiatr Res. 2015;65:37–46.
Pérez S, Ros MC, Folgado JEL, Marco JH. Non-suicidal self-injury differentiates suicide ideators and attempters and predicts future suicide attempts in patients with eating disorders. Suicide Life Threat Behav. 2019;49(5):1220–31.
Smith KE, Hayes NA, Styer DM, Washburn JJ. Emotional reactivity in a clinical sample of patients with eating disorders and nonsuicidal self-injury. Psychiatry Res. 2017;257:519–25.
Claes L, Klonsky ED, Muehlenkamp J, Kuppens P, Vandereycken W. The affect-regulation function of nonsuicidal self-injury in eating-disordered patients: which affect states are regulated? Compr Psychiatry. 2010;51(4):386–92.
Navarro-Haro MV, Wessman I, Botella C, García-Palacios A. The role of emotion regulation strategies and dissociation in non-suicidal self-injury for women with borderline personality disorder and comorbid eating disorder. Compr Psychiatry. 2015;63:123–30.
Giovinazzo S, Sukkar S, Rosa G, Zappi A, Bezante G, Balbi M, et al. Anorexia nervosa and heart disease: a systematic review. Eat Weight Disord Stud Anorex Bulim Obes. 2019;24(2):199–207.
Bouquegneau A, Dubois BE, Krzesinski J-M, Delanaye P. Anorexia nervosa and the kidney. Am J Kidney Dis. 2012;60(2):299–307.
Benini L, Todesco T, Frulloni L, Dalle Grave R, Campagnola P, Agugiaro F, et al. Esophageal motility and symptoms in restricting and binge-eating/purging anorexia. Dig Liver Dis. 2010;42(11):767–72.
Gibson D, Watters A, Mehler PS. The intersect of gastrointestinal symptoms and malnutrition associated with anorexia nervosa and avoidant/restrictive food intake disorder: Functional or pathophysiologic? A systematic review. Int J Eat Disord. 2021.
Abraham S, Kellow J. Exploring eating disorder quality of life and functional gastrointestinal disorders among eating disorder patients. J Psychosom Res. 2011;70(4):372–7.
Brewster DH, Nowell SL, Clark DN. Risk of oesophageal cancer among patients previously hospitalised with eating disorder. Cancer Epidemiol. 2015;39(3):313–20.
Smith KR, Moran TH. Gastrointestinal peptides in eating-related disorders. Physiol Behav. 2021;238:113456.
Seidel M, Markmann Jensen S, Healy D, Dureja A, Watson HJ, Holst B, et al. A systematic review and meta-analysis finds increased blood levels of all forms of ghrelin in both restricting and binge-eating/purging subtypes of anorexia nervosa. Nutrients. 2021;13(2):709.
Becker KR, Mancuso C, Dreier MJ, Asanza E, Breithaupt L, Slattery M, et al. Ghrelin and PYY in low-weight females with avoidant/restrictive food intake disorder compared to anorexia nervosa and healthy controls. Psychoneuroendocrinology. 2021;129:105243.
Schalla MA, Stengel A. Gastrointestinal alterations in anorexia nervosa—A systematic review. Eur Eat Disord Rev. 2019;27(5):447–61.
West M, McMaster CM, Staudacher HM, Hart S, Jacka FN, Stewart T, et al. Gastrointestinal symptoms following treatment for anorexia nervosa: A systematic literature review. Int J Eat Disord. 2021;54(6):936–51.
Avila JT, Park K, Golden NH. Eating disorders in adolescents with chronic gastrointestinal and endocrine diseases. Lancet Child Adolesc Health. 2019;3(3):181–9.
Ruusunen A, Rocks T, Jacka F, Loughman A. The gut microbiome in anorexia nervosa: relevance for nutritional rehabilitation. Psychopharmacology. 2019;236(5):1545–58.
Zaina F, Pesenti F, Persani L, Capodaglio P, Negrini S, Polli N. Prevalence of idiopathic scoliosis in anorexia nervosa patients: results from a cross-sectional study. Eur Spine J. 2018;27(2):293–7.
Hung C, Muñoz M, Shibli-Rahhal A. Anorexia nervosa and osteoporosis. Calcif Tissue Int. 2021;110(5):562–75.
Mumford J, Kohn M, Briody J, Miskovic-Wheatley J, Madden S, Clarke S, et al. Long-term outcomes of adolescent anorexia nervosa on bone. J Adolesc Health. 2019;64(3):305–10.
Robinson L, Aldridge V, Clark EM, Misra M, Micali N. Pharmacological treatment options for low bone mineral density and secondary osteoporosis in anorexia nervosa: a systematic review of the literature. J Psychosom Res. 2017;98:87–97.
Sim LA, McGovern L, Elamin MB, Swiglo BA, Erwin PJ, Montori VM. Effect on bone health of estrogen preparations in premenopausal women with anorexia nervosa: A systematic review and meta-analyses. Int J Eat Disord. 2010;43(3):218–25.
Lebow J, Sim L. The influence of estrogen therapies on bone mineral density in premenopausal women with anorexia nervosa and amenorrhea. Vitam Horm. 2013;92:243–57.
Maïmoun L, Renard E, Lefebvre P, Bertet H, Philibert P, Sénèque M, et al. Oral contraceptives partially protect from bone loss in young women with anorexia nervosa. Fertil Steril. 2019;111(5):1020–9.
Miller KK, Meenaghan E, Lawson EA, Misra M, Gleysteen S, Schoenfeld D, et al. Effects of risedronate and low-dose transdermal testosterone on bone mineral density in women with anorexia nervosa: a randomized, placebo-controlled study. J Clin Endocrinol Metab. 2011;96(7):2081–8.
Bloch M, Ish-Shalom S, Greenman Y, Klein E, Latzer Y. Dehydroepiandrosterone treatment effects on weight, bone density, bone metabolism and mood in women suffering from anorexia nervosa—a pilot study. Psychiatry Res. 2012;200(2–3):544–9.
Vajapeyam S, Ecklund K, Mulkern RV, Feldman HA, O’Donnell JM, DiVasta AD, et al. Magnetic resonance imaging and spectroscopy evidence of efficacy for adrenal and gonadal hormone replacement therapy in anorexia nervosa. Bone. 2018;110:335–42.
DiVasta AD, Feldman HA, Beck TJ, LeBoff MS, Gordon CM. Does hormone replacement normalize bone geometry in adolescents with anorexia nervosa? J Bone Miner Res. 2014;29(1):151–7.
Fazeli PK, Wang IS, Miller KK, Herzog DB, Misra M, Lee H, et al. Teriparatide increases bone formation and bone mineral density in adult women with anorexia nervosa. J Clin Endocrinol Metab. 2014;99(4):1322–9.
Giollo A, Idolazzi L, Caimmi C, Fassio A, Bertoldo F, Dalle Grave R, et al. V itamin D levels strongly influence bone mineral density and bone turnover markers during weight gain in female patients with anorexia nervosa. Int J Eat Disord. 2017;50(9):1041–9.
Davies JE, Cockfield A, Brown A, Corr J, Smith D, Munro C. The medical risks of severe anorexia nervosa during initial re-feeding and medical stabilisation. Clin Nutr ESPEN. 2017;17:92–9.
Hale MD, Logomarsino JV. The use of enteral nutrition in the treatment of eating disorders: a systematic review. Eat Weight Disord Stud Anorex Bulim Obes. 2019;24(2):179–98.
Rocks T, Pelly F, Wilkinson P. Nutrition therapy during initiation of refeeding in underweight children and adolescent inpatients with anorexia nervosa: a systematic review of the evidence. J Acad Nutr Diet. 2014;114(6):897–907.
Gentile MG, Pastorelli P, Ciceri R, Manna GM, Collimedaglia S. Specialized refeeding treatment for anorexia nervosa patients suffering from extreme undernutrition. Clin Nutr. 2010;29(5):627–32.
Hanachi M, Melchior JC, Crenn P. Hypertransaminasemia in severely malnourished adult anorexia nervosa patients: risk factors and evolution under enteral nutrition. Clin Nutr. 2013;32(3):391–5.
Rosen E, Sabel AL, Brinton JT, Catanach B, Gaudiani JL, Mehler PS. Liver dysfunction in patients with severe anorexia nervosa. Int J Eat Disord. 2016;49(2):151–8.
Vignaud M, Constantin J-M, Ruivard M, Villemeyre-Plane M, Futier E, Bazin J-E, et al. Refeeding syndrome influences outcome of anorexia nervosa patients in intensive care unit: an observational study. Crit Care. 2010;14(5):R172.
Whitelaw M, Gilbertson H, Lam P-Y, Sawyer SM. Does aggressive refeeding in hospitalized adolescents with anorexia nervosa result in increased hypophosphatemia? J Adolesc Health. 2010;46(6):577–82.
Leclerc A, Turrini T, Sherwood K, Katzman DK. Evaluation of a nutrition rehabilitation protocol in hospitalized adolescents with restrictive eating disorders. J Adolesc Health. 2013;53(5):585–9.
Leitner M, Burstein B, Agostino H. Prophylactic phosphate supplementation for the inpatient treatment of restrictive eating disorders. J Adolesc Health. 2016;58(6):616–20.
Brown C, Sabel A, Gaudiani J, Mehler PS. Predictors of hypophosphatemia during refeeding of patients with severe anorexia nervosa. Int J Eat Disord. 2015;48(7):898–904.
Whitelaw M, Lee KJ, Gilbertson H, Sawyer SM. Predictors of complications in anorexia nervosa and atypical anorexia nervosa: degree of underweight or extent and recency of weight loss? J Adolesc Health. 2018;63(6):717–23.
Agostino H, Erdstein J, Di Meglio G. Shifting paradigms: continuous nasogastric feeding with high caloric intakes in anorexia nervosa. J Adolesc Health. 2013;53(5):590–4.
Ridout KK, Kole J, Fitzgerald KL, Ridout SJ, Donaldson AA, Alverson B. Daily laboratory monitoring is of poor health care value in adolescents acutely hospitalized for eating disorders. J Adolesc Health. 2016;59(1):104–9.
Nehring I, Kewitz K, Von Kries R, Thyen U. Long-term effects of enteral feeding on growth and mental health in adolescents with anorexia nervosa—results of a retrospective German cohort study. Eur J Clin Nutr. 2014;68(2):171–7.
National Heat LaBI. Metabolic syndrome: US Department of Health and Human Services. 2020.
Mathisen TF, Sundgot-Borgen J, Rosenvinge JH, Bratland-Sanda S. Managing risk of non-communicable diseases in women with bulimia nervosa or binge eating disorders: A randomized trial with 12 months follow-up. Nutrients. 2018;10(12):1887.
Article PubMed Central Google Scholar
Thornton LM, Watson HJ, Jangmo A, Welch E, Wiklund C, von Hausswolff-Juhlin Y, et al. Binge-eating disorder in the Swedish national registers: Somatic comorbidity. Int J Eat Disord. 2017;50(1):58–65.
Nicolau J, Simó R, Sanchís P, Ayala L, Fortuny R, Zubillaga I, et al. Eating disorders are frequent among type 2 diabetic patients and are associated with worse metabolic and psychological outcomes: results from a cross-sectional study in primary and secondary care settings. Acta Diabetol. 2015;52(6):1037–44.
Jaworski M, Panczyk M, Śliwczyński AM, Brzozowska M, Janaszek K, Małkowski P, et al. A ten-year longitudinal study of prevalence of eating disorders in the general polish type 2 diabetes population. Med Sci Monit Int Med J Exp Clin Res. 2018;24:9204.
Gallant A, Drapeau V, Allison KC, Tremblay A, Lambert M, O’Loughlin J, et al. Night eating behavior and metabolic heath in mothers and fathers enrolled in the QUALITY cohort study. Eat Behav. 2014;15(2):186–91.
Hood MM, Reutrakul S, Crowley SJ. Night eating in patients with type 2 diabetes. Associations with glycemic control, eating patterns, sleep, and mood. Appetite. 2014;79:91–6.
Udo T, McKee SA, White MA, Masheb RM, Barnes RD, Grilo CM. Menopause and metabolic syndrome in obese individuals with binge eating disorder. Eat Behav. 2014;15(2):182–5.
Kisely S, Baghaie H, Lalloo R, Johnson NW. Association between poor oral health and eating disorders: systematic review and meta-analysis. Br J Psychiatry. 2015;207(4):299–305.
Pallier A, Karimova A, Boillot A, Colon P, Ringuenet D, Bouchard P, et al. Dental and periodontal health in adults with eating disorders: a case-control study. J Dent. 2019;84:55–9.
Lundgren JD, Smith BM, Spresser C, Harkins P, Zolton L, Williams K. The relationship of night eating to oral health and obesity in community dental clinic patients. Age (Years). 2010;57(15):12.
Lundgren JD, Williams KB, Heitmann BL. Nocturnal eating predicts tooth loss among adults: results from the Danish MONICA study. Eat Behav. 2010;11(3):170–4.
Panico R, Piemonte E, Lazos J, Gilligan G, Zampini A, Lanfranchi H. Oral mucosal lesions in anorexia nervosa, bulimia nervosa and EDNOS. J Psychiatr Res. 2018;96:178–82.
Setnick J. Micronutrient deficiencies and supplementation in anorexia and bulimia nervosa: a review of literature. Nutr Clin Pract. 2010;25(2):137–42.
Oudman E, Wijnia JW, Oey MJ, van Dam MJ, Postma A. Preventing Wernicke’s encephalopathy in anorexia nervosa: A systematic review. Psychiatry Clin Neurosci. 2018;72(10):774–9.
Ålgars M, Huang L, Von Holle AF, Peat CM, Thornton LM, Lichtenstein P, et al. Binge eating and menstrual dysfunction. J Psychosom Res. 2014;76(1):19–22.
Nobles CJ, Thomas JJ, Valentine SE, Gerber MW, Vaewsorn AS, Marques L. Association of premenstrual syndrome and premenstrual dysphoric disorder with bulimia nervosa and binge-eating disorder in a nationally representative epidemiological sample. Int J Eat Disord. 2016;49(7):641–50.
Chaer R, Nakouzi N, Itani L, Tannir H, Kreidieh D, El Masri D, et al. Fertility and Reproduction after recovery from anorexia nervosa: a systematic review and meta-analysis of long-term follow-up studies. Diseases. 2020;8(4):46.
Bulik CM, Von Holle A, Siega-Riz AM, Torgersen L, Lie KK, Hamer RM, et al. Birth outcomes in women with eating disorders in the Norwegian Mother and Child cohort study (MoBa). Int J Eat Disord. 2009;42(1):9–18.
Kolstad E, Gilhus NE, Veiby G, Reiter SF, Lossius MI, Bjørk M. Epilepsy and eating disorders during pregnancy: prevalence, complications and birth outcome. Seizure. 2015;28:81–4.
Longo P, Panero M, Amodeo L, Demarchi M, Abbate-Daga G, Marzola E. Psychoform and somatoform dissociation in anorexia nervosa: a systematic review. Clin Psychol Psychother. 2021;28(2):295–312.
Zerwas S, Larsen JT, Petersen L, Thornton LM, Quaranta M, Koch SV, et al. Eating disorders, autoimmune, and autoinflammatory disease. Pediatrics. 2017;140(6):e20162089.
Wotton CJ, James A, Goldacre MJ. Coexistence of eating disorders and autoimmune diseases: record linkage cohort study, UK. Int J Eat Disord. 2016;49(7):663–72.
Download references
Acknowledgements
The authors would like to thank and acknowledge the hard work of Healthcare Management Advisors (HMA) who were commissioned to undertake the Rapid Review. Additionally, the authors would like to thank all members of the consortium and consultation committees for their advice, input, and considerations during the development process. Further, a special thank you to the carers, consumers and lived experience consultants that provided input to the development of the Rapid Review and wider national Eating Disorders Research & Translation Strategy. Finally, thank you to the Australian Government—Department of Health for their support of the current project.
National Eating Disorder Research Consortium: Phillip Aouad, Sarah Barakat, Robert Boakes, Leah Brennan, Emma Bryant, Susan Byrne, Belinda Caldwell, Shannon Calvert, Bronny Carroll, David Castle, Ian Caterson, Belinda Chelius, Lyn Chiem, Simon Clarke, Janet Conti, Lexi Crouch, Genevieve Dammery, Natasha Dzajkovski, Jasmine Fardouly, Carmen Felicia, John Feneley, Amber-Marie Firriolo, Nasim Foroughi, Mathew Fuller-Tyszkiewicz, Anthea Fursland, Veronica Gonzalez-Arce, Bethanie Gouldthorp, Kelly Griffin, Scott Griffiths, Ashlea Hambleton, Amy Hannigan, Mel Hart, Susan Hart, Phillipa Hay, Ian Hickie, Francis Kay-Lambkin, Ross King, Michael Kohn, Eyza Koreshe, Isabel Krug, Anvi Le, Jake Linardon, Randall Long, Amanda Long, Sloane Madden, Sarah Maguire, Danielle Maloney, Peta Marks, Sian McLean, Thy Meddick, Jane Miskovic-Wheatley, Deborah Mitchison, Richard O’Kearney, Shu Hwa Ong, Roger Paterson, Susan Paxton, Melissa Pehlivan, Genevieve Pepin, Andrea Phillipou, Judith Piccone, Rebecca Pinkus, Bronwyn Raykos, Paul Rhodes, Elizabeth Rieger, Sarah Rodan, Karen Rockett, Janice Russell, Haley Russell, Fiona Salter, Susan Sawyer, Beth Shelton, Urvashnee Singh, Sophie Smith, Evelyn Smith, Karen Spielman, Sarah Squire, Juliette Thomson, Marika Tiggemann, Stephen Touyz, Ranjani Utpala, Lenny Vartanian, Andrew Wallis, Warren Ward, Sarah Wells, Eleanor Wertheim, Simon Wilksch & Michelle Williams
The RR was in-part funded by the Australian Government Department of Health in partnership with other national and jurisdictional stakeholders. As the organisation responsible for overseeing the National Eating Disorder Research & Translation Strategy, InsideOut Institute commissioned Healthcare Management Advisors to undertake the RR as part of a larger, ongoing, project. Role of Funder: The funder was not directly involved in informing the development of the current review.
Author information
Authors and affiliations.
InsideOut Institute, Central Clinical School, Faculty of Medicine and Health, Charles Perkins Centre (D17), University of Sydney, Camperdown, NSW, 2006, Australia
Ashlea Hambleton, Danielle Maloney, Stephen Touyz & Sarah Maguire
School of Health and Social Development, Faculty of Health, Deakin University, Geelong, VIC, 3220, Australia
Genevieve Pepin
Healthcare Management Advisors, Melbourne, VIC, Australia
Sydney Local Health District, Camperdown, NSW, Australia
Danielle Maloney, Stephen Touyz & Sarah Maguire
You can also search for this author in PubMed Google Scholar
National Eating Disorder Research Consortium
- Phillip Aouad
- , Sarah Barakat
- , Robert Boakes
- , Leah Brennan
- , Emma Bryant
- , Susan Byrne
- , Belinda Caldwell
- , Shannon Calvert
- , Bronny Carroll
- , David Castle
- , Ian Caterson
- , Belinda Chelius
- , Lyn Chiem
- , Simon Clarke
- , Janet Conti
- , Lexi Crouch
- , Genevieve Dammery
- , Natasha Dzajkovski
- , Jasmine Fardouly
- , Carmen Felicia
- , John Feneley
- , Amber-Marie Firriolo
- , Nasim Foroughi
- , Mathew Fuller-Tyszkiewicz
- , Anthea Fursland
- , Veronica Gonzalez-Arce
- , Bethanie Gouldthorp
- , Kelly Griffin
- , Scott Griffiths
- , Ashlea Hambleton
- , Amy Hannigan
- , Susan Hart
- , Phillipa Hay
- , Ian Hickie
- , Francis Kay-Lambkin
- , Ross King
- , Michael Kohn
- , Eyza Koreshe
- , Isabel Krug
- , Jake Linardon
- , Randall Long
- , Amanda Long
- , Sloane Madden
- , Sarah Maguire
- , Danielle Maloney
- , Peta Marks
- , Sian McLean
- , Thy Meddick
- , Jane Miskovic-Wheatley
- , Deborah Mitchison
- , Richard O’Kearney
- , Shu Hwa Ong
- , Roger Paterson
- , Susan Paxton
- , Melissa Pehlivan
- , Genevieve Pepin
- , Andrea Phillipou
- , Judith Piccone
- , Rebecca Pinkus
- , Bronwyn Raykos
- , Paul Rhodes
- , Elizabeth Rieger
- , Sarah Rodan
- , Karen Rockett
- , Janice Russell
- , Haley Russell
- , Fiona Salter
- , Susan Sawyer
- , Beth Shelton
- , Urvashnee Singh
- , Sophie Smith
- , Evelyn Smith
- , Karen Spielman
- , Sarah Squire
- , Juliette Thomson
- , Marika Tiggemann
- , Stephen Touyz
- , Ranjani Utpala
- , Lenny Vartanian
- , Andrew Wallis
- , Warren Ward
- , Sarah Wells
- , Eleanor Wertheim
- , Simon Wilksch
- & Michelle Williams
Contributions
DM, PM, ST and SM oversaw the Rapid Review process; AL carried out and wrote the initial review; AH and GP wrote the first manuscript; all authors edited and approved the final manuscript.
Corresponding author
Correspondence to Ashlea Hambleton .
Ethics declarations
Ethics approval and consent to participate.
Not applicable.
Consent for publication
Competing interests.
ST receives royalties from Hogrefe and Huber, McGraw Hill and Taylor and Francis for published books/book chapters. He has received honoraria from the Takeda Group of Companies for consultative work, public speaking engagements and commissioned reports. He has chaired their Clinical Advisory Committee for Binge Eating Disorder. He is the Editor in Chief of the Journal of Eating Disorders. ST is a committee member of the National Eating Disorders Collaboration as well as the Technical Advisory Group for Eating Disorders. AL undertook work on this RR while employed by HMA. A/Prof Sarah Maguire is a guest editor of the special issue “Improving the future by understanding the present: evidence reviews for the field of eating disorders.”
Additional information
Publisher's note.
Springer Nature remains neutral with regard to jurisdictional claims in published maps and institutional affiliations.
Supplementary Information
Additional file 1..
PRISMA diagram.
Additional file 2.
Studies included in the Rapid Review.
Rights and permissions
Open Access This article is licensed under a Creative Commons Attribution 4.0 International License, which permits use, sharing, adaptation, distribution and reproduction in any medium or format, as long as you give appropriate credit to the original author(s) and the source, provide a link to the Creative Commons licence, and indicate if changes were made. The images or other third party material in this article are included in the article's Creative Commons licence, unless indicated otherwise in a credit line to the material. If material is not included in the article's Creative Commons licence and your intended use is not permitted by statutory regulation or exceeds the permitted use, you will need to obtain permission directly from the copyright holder. To view a copy of this licence, visit http://creativecommons.org/licenses/by/4.0/ . The Creative Commons Public Domain Dedication waiver ( http://creativecommons.org/publicdomain/zero/1.0/ ) applies to the data made available in this article, unless otherwise stated in a credit line to the data.
Reprints and permissions
About this article
Cite this article.
Hambleton, A., Pepin, G., Le, A. et al. Psychiatric and medical comorbidities of eating disorders: findings from a rapid review of the literature. J Eat Disord 10 , 132 (2022). https://doi.org/10.1186/s40337-022-00654-2
Download citation
Received : 08 July 2022
Accepted : 15 August 2022
Published : 05 September 2022
DOI : https://doi.org/10.1186/s40337-022-00654-2
Share this article
Anyone you share the following link with will be able to read this content:
Sorry, a shareable link is not currently available for this article.
Provided by the Springer Nature SharedIt content-sharing initiative
- Psychiatric
- Comorbidities
- Eating disorders
Journal of Eating Disorders
ISSN: 2050-2974
- General enquiries: [email protected]
Factors predicting long-term weight maintenance in anorexia nervosa: a systematic review
- Open access
- Published: 06 April 2024
- Volume 29 , article number 24 , ( 2024 )
Cite this article
You have full access to this open access article
- Lydia Maurel 1 ,
- Molly MacKean 1 &
- J. Hubert Lacey ORCID: orcid.org/0000-0001-7302-6496 2 , 3
321 Accesses
13 Altmetric
Explore all metrics
Eating disorder recovery is a poorly defined concept, with large variations among researchers’ definitions. Weight maintenance is a key aspect of recovery that remains relatively underexplored in the literature. Understanding the role of weight maintenance may help guide the development of treatments. This paper aims to address this by (1) investigating the factors predicting long-term weight maintenance in anorexia nervosa (AN) patients; (2) exploring differences in predictive factors between adolescent and adult populations; and (3) exploring how weight maintenance is conceptualised in the literature. Methods: We conducted a systematic review following PRISMA guidelines to address our research questions. Five databases were searched and filtered according to our exclusion criteria.
From the search, 1059 studies were yielded, and 13 studies were included for review. A range of weight, biological and psychological factors were found to predict weight maintenance among these papers. BMI at admission and discharge from inpatient treatment was the most common predictor among the papers. Few studies investigated biological factors and mixed evidence was found for psychological factors. We found no observable differences between adult and adolescent populations. Finally, weight maintenance was defined and measured differently across studies.
This review’s findings can help contribute to a well-rounded understanding of weight maintenance, and ultimately, of recovery. This can help support clinicians in tailoring interventions to improve long-term outcomes in AN. Future research should aim to replicate studies to better understand the relationship between the factors identified and weight maintenance.
Systematic review.
Avoid common mistakes on your manuscript.
Introduction
Eating disorders are severe mental health conditions that negatively impact an individual’s physical, psychological and social functioning [ 1 ]. The prevalence and severity of eating disorder presentations have increased significantly over the last few years, with hospital admissions in the UK increasing by 84% in the last five years [ 2 ]. The recent COVID-19 pandemic further contributed, in part, to this increase, whereby individuals with eating disorders faced significant challenges such as increased social isolation, a reduced sense of control, and limited access to healthcare services [ 3 , 4 ]. Taken together, these pressures have meant that eating disorder services have struggled to meet demand and healthcare providers face the ongoing need to develop and adapt treatments accordingly.
Clinicians have highlighted concerns around long-term outcomes for patients following eating disorder treatment, in particular relapse. For example, relapse rates of 31% have been reported in anorexia nervosa (AN) [ 5 ], highlighting the importance of understanding contributing factors. Studies have explored possible mechanisms behind AN relapse and have found a wide range of possible factors. Frostad et al. [ 6 ] found BMI at discharge was a significant predictor of relapse in adults and adolescents. This lies in contrast to other studies that have found factors such as weight and shape concerns [ 5 ] having the binge–purge subtype of AN, having more motivation to recover at different points in treatment, and the severity of pre-treatment checking behaviour [ 7 ] to be significant predictors of relapse. Whilst these findings may support the adaptations of future treatments, a drawback of focusing on relapse is the heavy emphasis on preventing negative outcomes, rather than promoting positive change. These are two separate facets of long-term AN outcomes, and a substantial focus on preventing relapse may disempower an individual in their journey.
The promotion of positive outcomes in AN can be viewed through a recovery-focused lens. Numerous factors have been identified as predictors of recovery or positive outcomes, including personality traits [ 8 , 9 ], family relations [ 10 ], impulsivity [ 9 ], selflessness [ 11 ], and self-esteem [ 12 , 13 ]. As the aim for patients, families and clinicians is full recovery from AN, this has led to a comprehensive literature base on factors impacting AN recovery, and subsequently, a vast landscape of possible definitions of recovery [ 14 ]. Many researchers have attempted to operationalise ‘recovery’, with a widely accepted modern view that this should include a combination of biological, physical, and cognitive constructs [ 15 ], as well as measures of psychological and social wellbeing [ 16 ]. However, the concept of recovery remains somewhat abstract due to the variability in the individual’s experience and the personal nature of recovery for each person, which together have led to difficulties with measuring recovery, its predictors and with producing replicable studies [ 8 ].
An important aspect of recovery is weight maintenance, which refers to the sustained management of weight within a healthy range over time. Underweight individuals with AN have a twofold challenge when it comes to weight: weight gain and weight maintenance. Research has investigated factors that contribute to weight gain in various clinical settings [ 17 , 18 ]. Byrne et al. [ 17 ] found that parental self-efficacy was a significant predictor of weight gain for adolescents undergoing family-based treatment for AN. Nyman-Carlsson et al. [ 18 ] investigated pre-treatment factors predicting weight gain in a sample of young adult women and found that different predictors were significant depending on the type of treatment received. These factors included levels of emotion dysregulation and deficits in one’s ability to understand and cope with emotions. Research has also demonstrated early weight gain during treatment is a strong predictor of overall weight gain, as well as full recovery [ 19 , 20 ]. Whilst studies have investigated weight gain, there is little research on factors that impact weight maintenance. This is surprising given weight maintenance is a primary aim of AN treatments. Furthermore, research has found that weight maintenance is an essential part of full recovery outcomes [ 21 , 22 ]; for example, Rigaud et al. [ 22 ] found in a sample of adult inpatients with AN that more years spent relapse-free increased the probability of reaching full recovery with each year.
Better understanding the factors that impact weight maintenance can provide a focus on the positive aspects of AN trajectories and may support services to sustain existing improvement, including maximising current successful aspects of treatment. Furthermore, this perspective would allow us to focus on weight as an important aspect of positive change, whilst acknowledging that there are other relevant factors within recovery. This specific focus prevents researchers from becoming lost in an abstract world of ‘recovery’. In this context, recovery lacks a clear conceptualisation due to the wide number of relevant factors and its personal nature. Investigating weight maintenance in more depth can contribute to establishing a better understanding of recovery, and at the same time, the specificity of weight maintenance may allow findings to be more effectively applied in clinical settings.
This study reviews the current literature on factors associated with long-term weight maintenance in AN specifically, rather than eating disorders as a whole, given the heterogeneity of eating disorders and the likelihood of different factors affecting weight maintenance. Long-term weight maintenance is considered as defined by the papers included in the review and its definition will be further discussed in this paper.
This study further aims to investigate whether any identified factors vary between adult and adolescent populations. AN impacts adolescents and adults differently due to differences across developmental stages; for example, younger patients may have poorer medical outcomes as compared with adults [ 23 ]. Furthermore, therapeutic approaches differ according to age [ 24 ]. It is therefore possible that the factors maintaining long-term weight maintenance may also differ for each population. Another important reason to investigate differences between adults and adolescents is their differences in treatment outcome. Despite poorer morbidity, research suggests that adolescents have overall better outcomes after treatment for their eating disorder, and that the effect of factors such as early weight gain is larger for children than for adults [ 25 , 26 ]. This suggests that it is important to investigate patterns in factors that predict aspects of recovery, such as weight maintenance, as this may help explain overall differences in recovery rates for each group.
This paper aims to answer the following questions:
What are the factors associated with long-term weight maintenance following weight restoration in AN?
What are the similarities and differences in the factors associated with long-term weight maintenance between adult and adolescent AN populations?
How is long-term weight maintenance conceptualised in AN literature?
Search strategy
A systematic search of the literature was conducted by one reviewer, following PRISMA guidelines, between February and August 2022 using PubMed, MEDLINE, PsycINFO, Cochrane Library and Wiley Online Library. A pre-defined list of search terms was used to generate the literature search, including a combination of: “eating disorders”; “long term weight restoration”; “long term weight maintenance”; “anorexia nervosa”; “weight maintenance” and “restrictive eating disorder”.
Eligibility criteria and selection process
Due to the lack of available studies in this research area, no limits were placed on the patient demographics, type of intervention or study design. Studies were excluded using the following primary criteria:
Measuring weight maintenance after weight loss, in obesity and binge eating populations;
Examining factors that predict poor outcomes;
Examining factors that predict global outcomes, including psychological improvement; and
Measuring factors that predict long-term improvements in weight as a continuous variable.
Inclusion criteria included:
Studies measuring factors associated with and/or predicting weight maintenance in AN populations; and
English language studies.
For the purpose of this paper, studies met the condition of weight maintenance if they identified a weight or weight range to be sustained over a period of time. No limits were placed on the defined length of time required for a participant’s weight to be considered maintained, and patterns in these definitions will be discussed in the results.
The reviewer screened abstracts and retrieved full-texts of appropriate studies using the eligibility criteria above. Reference lists of reports that were assessed for eligibility were also searched for any appropriate studies. Reference lists were searched at this point in the retrieval process as the reports retrieved thus far were likely to be the most appropriate and may refer to other studies that contribute to their reports on weight maintenance. Figure 1 outlines the selection process for the studies included in this review, including full exclusion criteria. The third author was consulted regarding any studies that required further consultation to determine if they met inclusion or exclusion criteria.
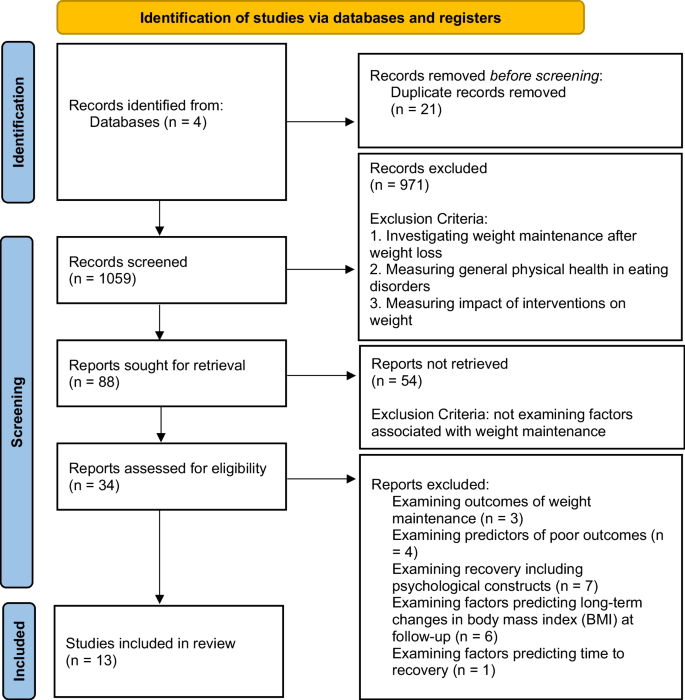
PRISMA 2020 flow diagram
A data extraction sheet was used by two reviewers to independently gather data on study purpose and design, intervention details, participant characteristics, definition of weight maintenance, any other measures and results/outcomes were sought from the retrieved full-text studies. These studies were then examined by the third author for clarification on study details and outcomes where needed.
The quality of the included studies was evaluated using the Quality Assessment Tool (QAT) [ 25 ].
The initial search yielded 1059 studies which were then screened for eligibility. Eighty-eight studies remained, and their abstracts were reviewed, with any papers that did not investigate factors associated with weight maintenance in AN being excluded at this stage. Thirteen studies remained, and the full texts were retrieved and assessed for eligibility. In addition, the reference lists of these 13 studies were reviewed, alongside any papers that had cited them, yielding a total of 21 additional studies. Combined, this resulted in the retrieval of full-texts for 34 studies. Twenty-one studies were excluded because they met the exclusion criteria, resulting in 13 studies for review in this paper. The process of selecting studies for review, following PRISMA guidelines, is depicted in Fig. 1 .
Sample characteristics of all included studies, as well as results from the quality assessment, are presented in Table 1 . All studies examined factors that influence long-term weight maintenance in individuals with AN as part of their research, although some studies did not investigate this as a primary aim. All the included studies were published between 2007 – 2021. In all 13 studies, the samples consisted of patients who had been admitted to inpatient or day patient programmes [ 26 , 27 , 28 , 29 , 30 , 31 , 32 , 33 , 34 , 35 , 36 , 37 , 38 ] and in 6 of these studies, patients had been discharged to outpatient programmes [ 26 , 27 , 28 , 29 , 30 , 38 ].
The mean age of participants across all studies ranged from 14.40 to 32.55 years. Data from studies show that the average weight maintenance rate for the participants ranged from 32.10% to 62.50%. Six studies included only adults [ 26 , 28 , 33 , 35 , 37 , 38 ], two studies included only adolescents [ 29 , 32 ], and five studies included both adults and adolescents [ 27 , 30 , 31 , 34 , 36 ]. The combined sample size across all the studies was 1689. Significant findings and p-values from the included papers are presented in Table 2 .
Definition of weight maintenance
There was a range of weight maintenance definitions across the studies, with different definitions for both adult and adolescent samples. Eleven studies used a measure of between BMI ≥18 and 19.5 [ 26 , 27 , 28 , 29 , 30 , 31 , 32 , 33 , 35 , 36 , 37 ], whilst Forman [ 34 ] used > 85% median BMI (%mBMI).
All studies took measures at three or more time points: admission, discharge and one or more follow-up points. Follow-up ranged from 6 months to 5 years. Six studies provided measures of weight between discharge and follow-up to ensure weight maintenance was sustained during the given time period [ 27 , 28 , 30 , 31 , 35 , 38 ]. From these, two studies used only in-person measures [ 27 , 30 ], two studies used only online/phone measures [ 31 , 35 ], and two studies used a combination of both [ 28 , 38 ]. Furthermore, three studies defined the time in weight maintenance needed for a patient to be considered in ‘maintenance’, specifying a requirement ranging from 4 to 8 consecutive weeks [ 27 , 28 , 30 ].
After reviewing the included articles, our findings can be grouped into three themes: BMI/weight variables; biological markers; and psychological markers.
Weight variables—BMI
Overall, BMI was most commonly investigated as a predictor of weight maintenance across the studies [ 26 , 29 , 30 , 31 , 32 , 35 , 37 ].
The most common finding was that BMI at discharge from inpatient treatment significantly predicted weight maintenance at follow-up [ 26 , 30 , 31 , 35 , 37 ]. Kaplan [ 30 ] found that women with a higher BMI at discharge from intensive treatment (inpatient or day patient) were more likely to maintain their weight at 6- and 12-month follow-up. El Ghoch [ 26 ] found discharge BMI significantly predicted weight maintenance at 12-month follow-up in a sample of inpatient women. Redgrave [ 37 ] also found similar results using a more stringent measure of maintenance (BMI ≥ 19 kg/m 2 ) at 6-month follow-up, and two studies found similar findings at longer term follow-up, namely up to 5 years [ 31 , 35 ]. These five studies reported that participants had a discharge BMI between 19.0 ± 3.3 kg/m 2 and 20.3 ± 0.5 kg/m 2 .
Alternatively, only two studies found that a higher BMI at admission to inpatient treatment significantly predicted weight maintenance up to 1-year follow-up [ 29 , 31 ]. Castro-Fornieles [ 29 ] found that admission BMI predicted weight maintenance at 9-month follow-up. Glasofer [ 31 ] found that BMI at admission predicted weight maintenance at 18.5 kg/m 2 , but not at a more stringent cut off at 19.5 kg/m 2 , whereas discharge BMI predicted maintenance in both maintenance at 18.5 kg/m 2 and at 19.5 kg/m 2 . These studies reported a BMI at admission between 15.5 ± 1.4 kg/m 2 and 16.0 ± 1.86 kg/m 2 .
However, not all studies found BMI to be a significant predictor. Boehm [ 32 ] used a different measure of BMI and found that increases in BMI standard deviation scores did not significantly predict weight maintenance at follow-up, which was a mean of 3.7 years after the start of inpatient treatment.
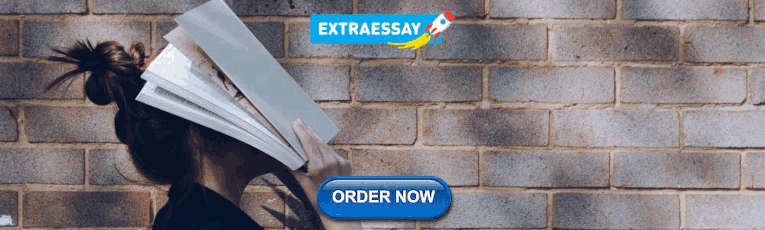
Other weight variables
Some studies investigated the predictive value of other weight-related variables on long-term weight maintenance. Forman [ 34 ] found that %MBMI was a significant predictor of weight maintenance at 1-year follow-up in a sample of adolescents and young adults, such that for each 5% increase in baseline %MBMI, patients were 1.69 times more likely to reach weight maintenance.
Uniacke [ 27 ] investigated the impact of weight suppression using data from Kaplan’s [ 30 ] study. Weight suppression refers to the difference between a person’s previous highest weight and their current weight [ 40 ]. Whilst previous research has found that weight suppression predicts weight gain outcomes [ 40 ], Uniacke [ 27 ] found neither weight suppression, nor the interaction between weight suppression and BMI (measured at start of outpatient treatment), significantly predicted weight maintenance at follow-up.
One study investigated the predictive value of early weight gain on long-term outcomes. Kaplan [ 30 ] found that the rate of weight change, namely a lower rate of weekly weight loss, in the first 28 days of outpatient CBT treatment was a significant predictor of weight maintenance.
Biological markers
Three studies investigated the impact of biological factors related to weight [ 26 , 28 , 38 ]. Two studies found that body fat percentage, measured using a whole-body DXA scan and MRI imaging, did not significantly predict weight maintenance at 12-month follow-up [ 26 , 38 ]. However, Kim [ 28 ] found that both body fat percentage and higher levels of leptin (fat-adjusted) pre-discharge from inpatient treatment, measured using whole-body MRI imaging, significantly predicted weight maintenance at 12-month follow-up.
Psychological markers
The identified studies in this paper investigated a range of psychological markers with mixed findings. Some studies found significant findings for predictors related to motivation and belief in oneself to change [ 29 , 36 ]. Castro-Fornieles [ 29 ] found that readiness to recover significantly predicted weight maintenance at 9-month follow-up in a sample of adolescents. Cooper [ 36 ] found that normative eating self-efficacy at admission was significantly associated with long-term weight maintenance, with participants 4.65 times more likely to have maintained weight at 6-month follow-up for each one-unit increase in normative eating self-efficacy scores from admission to follow-up.
Three studies investigated the impact of body image concern components on weight maintenance outcomes namely: ‘fear of weight gain’, ‘preoccupation with weight or shape’, ‘feeling fat’, body image distortion, and body image self-efficacy [ 32 , 33 , 36 ]. Calugi [ 33 ] explored a range of variables, measured by using items taken from the Eating Disorder Examination 12.0D at the end of treatment, and found that lower scores for ‘fear of weight gain’ at baseline were associated with a higher likelihood of maintaining weight at 6- and 12-month follow-up in a sample of young women. Calugi [ 33 ] also found that lower scores of ‘preoccupation with shape or weight’, and ‘feeling fat’ predicted weight maintenance at 6-month follow-up, but not at 12-month follow-up. However, the authors did not include significance values in their findings therefore it is unclear whether these measures are significant predictors. In contrast, Boehm [ 32 ] investigated a separate facet of body image, specifically perceptual body image distortion, referring to the accuracy of comparison between one’s perceived and actual body size [ 41 ], and found this did not significantly predict weight maintenance, although it was a significant predictor of long-term global outcome (including psychological outcomes). In line with this, Cooper [ 36 ] found that improvements in body dissatisfaction and body image self-efficacy from admission to follow-up, namely the belief in oneself to complete everyday tasks without being held back by body image concerns [ 42 ], did not significantly predict long-term weight maintenance either.
Other studies found that psychological variables, including anxiety symptoms, depression symptoms, eating disorder psychopathology, expectations for recovery, personality traits and quality of life did not significantly predict long-term weight maintenance [ 30 , 35 , 36 ].
Difference between adult and adolescent samples
The studies were reviewed to examine whether any predictors differentiated the adult and adolescent patient groups. None of the studies with both adolescent and adult samples analysed differences in predictors between these two groups. Studies looking at biological markers used only adult samples. Otherwise, there were no observable patterns in the data to suggest that any variable has been found to predict weight maintenance more consistently in adult or adolescent samples.
The overarching aim of this review was to explore the factors predicting long-term weight maintenance in adults and adolescents with AN, and then compare any differences between adults and adolescents. Another aim was to evaluate how weight maintenance is defined and measured among these papers. A literature review was conducted following the PRISMA framework, resulting in 13 studies. The review identified a range of weight, biological and psychological factors investigated in relation to weight maintenance, but also found that the concept of weight maintenance varied among the studies.
BMI at admission and discharge
The most common significant finding across studies was that BMI at admission and discharge from inpatient treatment significantly predicted weight maintenance across both adult and adolescent samples.
Our finding that admission BMI predicts weight maintenance is mirrored in the literature on recovery, as admission BMI has been found to significantly predict treatment outcome and recovery [ 43 , 44 ]. However, we found that BMI at discharge from treatment was a more common significant predictor of weight maintenance than admission BMI among the included studies. This finding is important as there is little research on the predictive value of discharge BMI in the recovery and relapse literature. A possible reason for this finding is that patients with a higher discharge BMI would have a wider margin for some weight loss to remain in the ‘maintenance’ category as compared to those with a lower discharge BMI, making maintenance easier from a weight perspective. It is also possible that higher BMI at discharge correlates with increased cognitive function recovery, which is linked to increased cognitive flexibility [ 45 ]. This may support individuals in their efforts to maintain their weight after treatment, although further research is needed to investigate these relationships.
Research on recovery has investigated changes in BMI during inpatient treatment, rather than BMI before or after treatment, and found that larger changes in BMI between admission and discharge were a significant predictor of remission at follow-up, whereas admission BMI was not a significant predictor [ 46 ]. It would be interesting to investigate this construct further in relation to long-term weight maintenance, in order to better understand the predictive value and relationships between admission BMI, discharge BMI and weight gain during treatment.
Some papers included in this review investigated the predictive value of other weight-related variables, such as weight suppression and rate of weight change in treatment [ 27 , 28 ]. Whilst some findings are significant, given that there has been little replication of any significant findings on these weight variables, future research is needed.
Biological factors
This review also identified studies that investigated biological factors predicting weight maintenance, namely body fat and leptin levels. Whilst studies investigating body fat found this was not a significant predictor of weight maintenance [ 26 , 38 ], one study found that body fat percentage and leptin levels at discharge significantly predicted weight maintenance at follow-up [ 28 ]. This may correlate with our finding that discharge BMI predicts weight maintenance, as identified in this review. Given the relationship between body fat percentage and leptin levels with a person’s weight for height, these findings may be linked [ 6 ].
Psychological factors
The present study found a range of psychological factors affecting weight maintenance among the literature. Some studies found that factors related to self-efficacy and motivation to change significantly predicted weight maintenance [ 36 , 39 ]. Research suggests that increased self-efficacy predicts end-of-treatment outcomes in eating disorder populations [ 12 , 47 ], and it is possible that this may help to support individuals with AN in maintaining their weight after treatment, helping them cope with difficulties and challenges they may face during this process [ 48 ]. However, the other studies looking at body image constructs in this review found mixed results [ 32 , 33 , 36 ]. Research suggests that psychological factors tend to take longer than physical factors to improve [ 49 ], and body image disturbance is suggested to shift in the later stages of recovery [ 12 ]. This may explain these inconsistent findings in the present review, though this should be interpreted with caution.
Adult vs adolescent samples
Our second aim was to explore whether there were any differences between adult and adolescent samples in factors that predict weight maintenance. We found no observable patterns in results from the included studies between age groups. This may be due to the limited number of studies in this review, which will have impacted our ability to observe patterns; it would be important for future research to investigate this further. An understanding of the role of different factors in weight maintenance may help clinicians to tailor interventions according to age group, as adolescents and adults face different challenges when overcoming eating disorders. [ 50 ].
Conceptualisation of weight maintenance
We found that many studies used different definitions of weight maintenance, including different weight cut-offs and time periods required for weight restoration to be considered maintenance. This makes it difficult to compare findings across studies because different factors may have varying predictive value. For example, one study using a different measure of BMI, namely BMI-SDS, found this did not predict weight maintenance [ 32 ], which may suggest that the measurements used may impact findings. Further, one study with a notably longer-term follow-up period [ 32 ] had non-significant findings regarding a range of variables. This highlights the need for more consistent measurements and follow-up periods, to gain a better understanding of predictive variables in weight maintenance.
Despite this, many studies used the weight criterion from the Morgan–Russell scale [ 51 ]. These studies also included menstrual recovery as part of their weight maintenance definition. This dilutes the definition of weight maintenance, which cannot be used across wider samples, including men.
Furthermore, most studies took one measure of weight at follow-up and used a weight cut off to establish whether participants had maintained their weight throughout this time period, instead of taking multiple measurements to ascertain sustained weight maintenance. This approach does not necessarily represent a true measure of ‘maintenance’, as it is possible that participants may have lost weight in between follow-up measures.
Taken together, there is a lack of consensus between researchers in the definition of weight maintenance, as well as a need for more robust and consistent measurement methods. We hope this paper stimulates the debate. It is important to improve this before trying to explore more complex concepts, such as recovery.
Strengths and limits
This study gives voice to the lack of clarity around the concept of eating disorders recovery, alongside the impact that this could have on treatment. To our knowledge this is the first systematic review on papers looking at factors affecting weight maintenance.
The present study has several limitations. Findings should be treated with caution given the small number of available studies, as well as the heterogeneity in their design, intervention and follow-up durations. The differences make it difficult to make comparisons between studies and find patterns in results, highlighting the need for a common definition of weight maintenance across studies [ 45 ]. We included studies that included menstruation as part of their criteria. It is possible that this may have skewed findings, for having an additional criterion for maintenance may reduce the likelihood that certain predictors are found significant, or alternatively, other factors may hold more importance. In addition, most studies had primarily white female samples, particularly so in the studies that included menstruation resumption as part of their criteria. Men and non-white samples are more likely to have poorer outcomes [ 44 ], therefore significant predictors identified in this review may not apply to those populations.
Implications
Future research must focus on developing a clear concept of weight maintenance as it pertains to the eating disorders and particularly AN. Research and common clinical observation suggests that weight maintenance is the first step to full psychological recovery [ 19 ]. In addition, there lacks a clear consensus on the definitions of recovery and relapse, and better understanding weight maintenance may help contribute to rectifying this. Understanding the factors that predict weight maintenance can help clinicians adapt existing treatments to focus on targeting these factors, with the aim of supporting patients to maintain their weight after treatment and work towards full recovery.
Avenues that may be explored by future research include replicating studies looking at BMI throughout treatment, in order to increase reliability in the findings around weight variables. Future research should also investigate further the relationship between body image and long-term weight maintenance, given this review’s mixed findings.
The present study aimed to scope the literature on the factors predicting weight maintenance after acknowledging that this is a critical factor for recovery, and the inconsistent findings and definitions of recovery.
The current literature on weight maintenance suggests that a higher BMI at admission and discharge are the strongest predictors of long-term weight maintenance. Mixed findings have been found for biological and psychological factors. It is important for readers to interpret these findings with care, and to combine this with a wider understanding of what is important for AN patients, rather than using these results in isolation to promote a purely medical model of recovery. The findings provide important implications for future research as they highlight the need for a common definition of weight maintenance, as well as the need to compare differences between adult and adolescent samples so we can ensure that treatments are tailored to their individual needs. Further research should aim to develop a clear definition of weight maintenance and investigate predictive factors, including how BMI and weight gain processes account for weight maintenance, and elucidate the role of psychological processes in weight maintenance.
What is already known on this subject?
There exists extensive research on eating disorder recovery, but there are different views on how this should be defined and measured. Several factors have been suggested to predict long-term recovery, yet the recovery landscape remains unclear due to the lack of consensus on the definition of recovery and on the factors deemed to predict recovery.
What this study adds?
This study adds an understanding of how weight maintenance is conceptualised in eating disorder research and an initial understanding of factors predicting weight maintenance, upon which future research can build.
Data availability
The datasets used in this study are available from the corresponding author on reasonable request.
De la Rie SM, Noordenbos G, Van Furth EF (2005) Quality of life and eating disorders. Qual Life Res 14(6):1511–1521. https://doi.org/10.1007/s11136-005-0585-0
Article PubMed Google Scholar
Royal College of Psychiatrists (2022) Hospital admissions for eating disorders increased by 84% in the last five years. https://www.rcpsych.ac.uk/news-and-features/latest-news/detail/2022/05/18/hospital-admissions-for-eating-disorders-increased-by-84-in-the-last-five-years . Accessed 14 March 2023
Branley-Bell D, Talbot CV (2020) Exploring the impact of the COVID-19 pandemic and UK lockdown on individuals with experience of eating disorders. J Eat Disord 8(44):1–12. https://doi.org/10.1186/s40337-020-00319-y
Article Google Scholar
Devoe DJ, Han A, Anderson A, Katzman DK, Patten SB, Soumbasis A, Flanagan J, Paslakis G, Vyver E, Marcoux G, Dimitropoulos G (2023) The impact of the COVID-19 pandemic on eating disorders: a systematic review. Int J Eat Disord 56(1):5–25. https://doi.org/10.1002/eat.23704
Berends T, Boonstra N, Van Elburg A (2018) Relapse in anorexia nervosa: a systematic review and meta-analysis. Curr Opin Psychiatry 31:445–455. https://doi.org/10.1097/YCO.0000000000000453
Frostad S, Rozakou-Soumalia N, Dârvariu Ş, Foruzesh B, Azkia H, Larsen MP, Rowshandel E, Sjögren JM (2022) BMI at discharge from treatment predicts relapse in anorexia nervosa: a systematic scoping review. J Pers Med 12:836. https://doi.org/10.3390/jpm12050836
Article PubMed PubMed Central Google Scholar
Carter JC, Mercer-Lynn KB, Norwood SJ, Bewell-Weiss CV, Crosby RD, Woodside DB, Olmsted MP (2012) A prospective study of predictors of relapse in anorexia nervosa: implications for relapse prevention. Psychiatry Res 200(2–3):518–523. https://doi.org/10.1016/j.psychres.2012.04.037
Bardone-Cone AM, Hunt RA, Watson HJ (2018) An overview of conceptualizations of eating disorder recovery, recent findings, and future directions. Curr Psychiatry Rep 20(9):1–18. https://doi.org/10.1007/s11920-018-0932-9
Zerwas S, Lund BC, Von Holle A, Thornton LM, Berrettini WH, Brandt H, Crawford S, Fichter MM, Halmi KA, Johnson C, Kaplan AS, La Via M, Mitchell J, Rotondo A, Strober M, Woodside DB, Kaye WH, Bulik CM (2013) Factors associated with recovery from anorexia nervosa. J Psychiatry Res 47(7):972–979. https://doi.org/10.1016/j.jpsychires.2013.02.011
Lock J, Couturier J, Bryson S, Agras S (2006) Predictors of dropout and remission in family therapy for adolescent anorexia nervosa in a randomised clinical trial. Int J Eat Disord 39:639–647. https://doi.org/10.1002/eat.20328
Pinius U, Canetti L, Bonne O, Bachar E (2019) Selflessness as a predictor of remission from an eating disorder: 1–4 year outcomes from an adolescent day-care unit. Eat Weight Disord 24:777–786. https://doi.org/10.1007/s40519-017-0444-3
Bardone-Cone AM, Miller AJ, Thompson KA, Walsh EC (2020) Predicting a comprehensive operationalization of eating disorder recovery: examining self-concept, personality, and negative affect. Int J Eat Disord 53(6):987–996. https://doi.org/10.1002/eat.23281
Wild B, Friederich H-C, Zipfel S, Resmark G, Giel K, Teufel M, Schelberg D, Löwe B, de Zwaan M, Zeeck A, Herpertz S, Burgmer M, von Wietersheim J, Tagay S, Dinkel A, Herzog W (2016) Predictors of outcomes in outpatients with anorexia nervosa—results from the ANTOP study. Psychiatry Res 244:45–50. https://doi.org/10.1016/j.psychres.2016.07.002
Eaton CM (2020) Eating disorder recovery: a metaethnography. J Am Psychiatric Nurses Assoc 26(4):373–388. https://doi.org/10.1177/1078390319849106
Bardone-Cone AM, Alvarez A, Gorlick J, Koller KA, Thompson KA, Miller AJ (2019) Longitudinal follow-up of a comprehensive operationalization of eating disorder recovery: concurrent and predictive validity. Int J Eat Disord 52(9):1052–1057. https://doi.org/10.1002/eat.23128
de Vos JA, LaMarre A, Radstaak M, Bijkerk CA, Bohlmeijer ET, Westerhof GJ (2017) Identifying fundamental criteria for eating disorder recovery: a systematic review and qualitative meta-analysis. J Eat Disord 5(1):1–14. https://doi.org/10.1186/s40337-017-0164-0
Byrne CE, Accurso EC, Arnow KD, Lock J, Le Grange D (2015) An exploratory examination of patient and parental self-efficacy as predictors of weight gain in adolescents with anorexia nervosa. Int J Eat Disord 48(7):883–888. https://doi.org/10.1002/eat.22376
Nyman-Carlsson E, Birgegård A, Engström I, Gustafsson SA, Nevonen L (2019) Predictors of outcome among young adult patients with anorexia nervosa in a randomised controlled trial. Eur Eat Disord Rev: J Eat Disord Assoc 27(1):76–85. https://doi.org/10.1002/erv.2630
Accurso EC, Ciao AC, Fitzsimmons-Craft EE, Lock JD, Le Grange D (2014) Is weight gain really a catalyst for broader recovery? The impact of weight gain on psychological symptoms in the treatment of adolescent anorexia nervosa. Behav Res Ther 56:1–6. https://doi.org/10.1016/j.brat.2014.02.006
Le Grange D, Accurso EC, Lock J, Agras S, Bryson SW (2014) Early weight gain predicts outcome in two treatments for adolescent anorexia nervosa. Int J Eat Disord 47(2):124–129. https://doi.org/10.1002/eat.22221
Franko D, Tabri N, Keshaviah A, Murray HB, Herzog DB, Thomas JJ, Coniglio K, Keel PK, Eddy KT (2018) Predictors of long-term recovery in anorexia nervosa and bulimia nervosa: data from a 22-year longitudinal study. J Psychiatry Res 96:183–188. https://doi.org/10.1016/j.jpsychires.2017.10.008
Rigaud D, Pennacchio H, Bizeul C, Reveillard V, Vergès B (2011) Outcome in AN adult patients: a 13-year follow-up in 484 patients. Diabetes Metab 37(4):305–311. https://doi.org/10.1016/j.diabet.2010.11.020
Article CAS PubMed Google Scholar
Winston AP, Paul M, Juanola-Borrat Y (2011) The same but different? treatment of anorexia nervosa in adolescents and adults. Eur Eat Disord Rev 20(2):89–93. https://doi.org/10.1002/erv.1137
Dalle Grave R, Sartirana M, Sermattei S, Calugi S (2021) Treatment of eating disorders in adults versus adolescents: similarities and differences. Clin Ther 43(1):70–84. https://doi.org/10.1016/j.clinthera.2020.10.015
National Heart Lung and Blood Institute (2021) Quality assessment tool for observational cohort and cross-sectional studies. Study Quality Assessment Tools. https://www.nhlbi.nih.gov/health-topics/study-quality-assessment-tools . Accessed 26 July 2023
El Ghoch M, Calugi S, Chignola E, Bazzani PV, Dalle Grave R (2016) Body mass index, body fat and risk factor of relapse in anorexia nervosa. Eur J Clin Nutr 70(2):194–198. https://doi.org/10.1038/ejcn.2015.164
Uniacke B, Attia E, Kaplan A, Walsh BT (2020) Weight suppression and weight maintenance following treatment of anorexia nervosa. Int J Eat Disord 53(6):1002–1006. https://doi.org/10.1002/eat.23269
Kim Y, Hersch J, Bodell LP, Schebendach J, Hildebrandt T, Walsh BT, Mayer LE (2021) The association between leptin and weight maintenance outcome in anorexia nervosa. Int J Eat Disord 54(4):527–534. https://doi.org/10.1002/eat.23407
Castro-Fornieles J, Casulà V, Saura B, Martínez E, Lazaro L, Vila M, Plana MT, Toro J (2007) Predictors of weight maintenance after hospital discharge in adolescent anorexia nervosa. Int J Eat Disord 40(2):129–135. https://doi.org/10.1002/eat.20340
Kaplan AS, Walsh BT, Olmsted M, Attia E, Carter JC, Devlin MJ, Pike KM, Woodside B, Rockert W, Roberto CA, Parides M (2009) The slippery slope: prediction of successful weight maintenance in anorexia nervosa. Psychol Med 39(6):1037–1045. https://doi.org/10.1017/S003329170800442X
Glasofer DR, Muratore AF, Attia E, Wu P, Wang Y, Minkoff H, Rufin T, Walsh BT, Steinglass JE (2020) Predictors of illness course and health maintenance following inpatient treatment among patients with anorexia nervosa. J Eat Disord 8(1):1–10. https://doi.org/10.1186/s40337-020-00348-7
Boehm I, Finke B, Tam FI, Fittig E, Scholz M, Gantchev K, Roessner V, Ehrlich S (2016) Effects of perceptual body image distortion and early weight gain on long-term outcome of adolescent anorexia nervosa. Eur Child Adolesc Psychiatry 25(12):1319–1326. https://doi.org/10.1007/s00787-016-0854-1
Calugi S, El Ghoch M, Conti M, Dalle Grave R (2018) Preoccupation with shape or weight, fear of weight gain, feeling fat and treatment outcomes in patients with anorexia nervosa: a longitudinal study. Behav Res Ther 105:63–68. https://doi.org/10.1016/j.brat.2018.04.001
Forman SF, McKenzie N, Hehn R, Monge MC, Kapphahn CJ, Mammel KA, Callahan ST, Sigel EJ, Bravender T, Romano M, Rome ES, Robinson KA, Fisher M, Malizio JB, Rosen DS, Hergenroeder C, Bucklelew SM, Jay MS, Lindenbaum J, Rickert VI, Garber A, Golden NH, Woods ER (2014) Predictors of outcome at 1 year in adolescents with DSM-5 restrictive eating disorders: report of the national eating disorders quality improvement collaborative. J Adolesc Health 55(6):750–756. https://doi.org/10.1016/j.jadohealth.2014.06.014
Brewerton TD, Costin C (2011) Long-term outcome of residential treatment for anorexia nervosa and bulimia nervosa. Eat Disord 19(2):132–144. https://doi.org/10.1080/10640266.2011.551632
Cooper M, Guarda AS, Petterway F, Schreyer CC (2021) Change in normative eating self-efficacy is associated with six-month weight restoration following inpatient treatment for anorexia nervosa. Eat Behav 42:101518. https://doi.org/10.1016/j.eatbeh.2021.101518
Redgrave GW, Schreyer CC, Coughlin JW, Fischer LK, Pletch A, Guarda AS (2021) Discharge body mass index, not illness chronicity, predicts 6-month weight outcome in patients hospitalized with anorexia nervosa. Front Psychiatry 12:641861. https://doi.org/10.3389/fpsyt.2021.641861
Bodell LP, Mayer LE (2011) Percent body fat is a risk factor for relapse in anorexia nervosa: a replication study. Int J Eat Disord 44(2):118–123. https://doi.org/10.1002/eat.20801
Mayer LES, Roberto CA, Glasofer DR, Fischer Etu S, Gallagher D, Wang J, Heymsfeld SB, Pierson RN Jr, Attia E, Devlin MJ, Walsh BT (2007) Does percent body fat predict outcome in anorexia nervosa? Am J Psychiatry 164(6):970–972. https://doi.org/10.1176/appi.ajp.164.6.970
Gorrell S, Reilly EE, Schaumberg K, Anderson LM, Donahue JM (2019) Weight suppression and its relation to eating disorder and weight outcomes: a narrative review. Eat Disord 27(1):52–81. https://doi.org/10.1080/10640266.2018.1499297
Gardner RM (2012) Measurement of perceptual body image. Encycl Body Image Hum Appear 2:526–532. https://doi.org/10.1016/B978-0-12-384925-0.00083-3
Pinto AM, Heinberg LJ, Coughlin JW, Fava JL, Guarda AS (2008) The eating disorder recovery self-efficacy questionnaire (EDRSQ): change with treatment and prediction of outcome. Eat Behav 9(2):143–153. https://doi.org/10.1016/j.eatbeh.2007.07.001
Wales J, Brewin N, Cashmore R, Haycraft E, Baggott J, Cooper A, Arcelus J (2016) Predictors of positive treatment outcome in people with anorexia nervosa treated in a specialized inpatient unit: the role of early response to treatment. Eur Eat Disord Rev 24(5):417–424. https://doi.org/10.1002/erv.2443
Miskovic-Wheatley J, Bryant E, Ong SH, Vatter S, Le A, Touyz S, Maguire S (2023) Eating disorder outcomes: findings from a rapid review of over a decade of research. J Eat Disord 11(1):85. https://doi.org/10.1186/s40337-023-00801-3
Lozano-Serra E, Andrés-Perpiña S, Lázaro-García L, Castro-Fornieles J (2014) Adolescent anorexia nervosa: cognitive performance after weight recovery. J Psychosom Res 76(1):6–11. https://doi.org/10.1016/j.jpsychores.2013.10.009
Danielsen M, Bjørnelv S, Weider S, Myklebust TÅ, Lundh H, Rø Ø (2020) The outcome at follow-up after inpatient eating disorder treatment: a naturalistic study. J Eat Disord 8:1–12. https://doi.org/10.1186/s40337-020-00349-6
Keshen A, Helson T, Town J, Warren K (2017) Self-efficacy as a predictor of treatment outcome in an outpatient eating disorder program. Eat Disord 25(5):406–419. https://doi.org/10.1080/10640266.2017.1324073
Fogarty S, Ramjan LM (2016) Factors impacting treatment and recovery in anorexia nervosa: qualitative findings from an online questionnaire. J Eat Disord 4(1):1–9. https://doi.org/10.1186/s40337-016-0107-1
Richmond TK, Woolverton GA, Mammel K, Ornstein RM, Spalding A, Woods ER, Forman SF (2020) How do you define recovery? A qualitative study of patients with eating disorders, their parents, and clinicians. Int J Eat Disord 53(8):1209–1218. https://doi.org/10.1002/eat.23294
McGorry P, Bates T, Birchwood M (2013) Designing youth mental health services for the 21 st century: examples from Australia, Ireland and the UK. Br J Psychiatry 202(54):30–35. https://doi.org/10.1192/bjp.bp.112.119214
Morgan HG, Hayward AE (1988) Clinical assessment of anorexia nervosa: the Morgan-Russell outcome assessment schedule. Br J Psychiatry 152(3):367–371. https://doi.org/10.1192/bjp.152.3.367
Download references
The authors declare that no financial support or funding was received during the preparation of this manuscript.
Author information
Authors and affiliations.
Schoen Clinic Chelsea, London, UK
Lydia Maurel & Molly MacKean
Schoen, Birmingham, UK
J. Hubert Lacey
St George’s, University of London, London, UK
You can also search for this author in PubMed Google Scholar
Contributions
All authors contributed to the development of the study and manuscript. Data collection, synthesis and analysis were performed by LM and MM. JHL supervised the project. The first draft of the manuscript was written by LM. All authors commented on all versions of the manuscript. All authors read and approved the final manuscript.
Corresponding author
Correspondence to J. Hubert Lacey .
Ethics declarations
Competing interests.
The authors declare no competing interests.
Ethics approval
This paper did not require formal ethics approval. Nonetheless the research was done in accordance with the ethical supervision of the Schoen Research and Ethics Committee, based on the ethical standards laid down by the 1964 Declaration of Helsinki.
Informed consent
For this type of study, formal consent is not required.
Additional information
Publisher's note.
Springer Nature remains neutral with regard to jurisdictional claims in published maps and institutional affiliations.
Rights and permissions
Open Access This article is licensed under a Creative Commons Attribution 4.0 International License, which permits use, sharing, adaptation, distribution and reproduction in any medium or format, as long as you give appropriate credit to the original author(s) and the source, provide a link to the Creative Commons licence, and indicate if changes were made. The images or other third party material in this article are included in the article's Creative Commons licence, unless indicated otherwise in a credit line to the material. If material is not included in the article's Creative Commons licence and your intended use is not permitted by statutory regulation or exceeds the permitted use, you will need to obtain permission directly from the copyright holder. To view a copy of this licence, visit http://creativecommons.org/licenses/by/4.0/ .
Reprints and permissions
About this article
Maurel, L., MacKean, M. & Lacey, J.H. Factors predicting long-term weight maintenance in anorexia nervosa: a systematic review. Eat Weight Disord 29 , 24 (2024). https://doi.org/10.1007/s40519-024-01649-5
Download citation
Received : 19 October 2023
Accepted : 03 March 2024
Published : 06 April 2024
DOI : https://doi.org/10.1007/s40519-024-01649-5
Share this article
Anyone you share the following link with will be able to read this content:
Sorry, a shareable link is not currently available for this article.
Provided by the Springer Nature SharedIt content-sharing initiative
- Eating disorders
- Anorexia nervosa
- Weight maintenance
- Weight restoration
- Find a journal
- Publish with us
- Track your research
This paper is in the following e-collection/theme issue:
Published on 9.4.2024 in Vol 26 (2024)
Moderating Effect of Coping Strategies on the Association Between the Infodemic-Driven Overuse of Health Care Services and Cyberchondria and Anxiety: Partial Least Squares Structural Equation Modeling Study
Authors of this article:

Original Paper
- Richard Huan Xu 1 , PhD ;
- Caiyun Chen 2 , PhD
1 Department of Rehabilitation Sciences, Faculty of Health and Social Sciences, Hong Kong Polytechnic University, Hung Hom, China (Hong Kong)
2 Nanjing Academy of Administration, Nanjing, China
Corresponding Author:
Richard Huan Xu, PhD
Department of Rehabilitation Sciences
Faculty of Health and Social Sciences
Hong Kong Polytechnic University
11 Yuk Choi Rd
China (Hong Kong)
Phone: 852 27664199
Email: [email protected]
Background: The COVID-19 pandemic has led to a substantial increase in health information, which has, in turn, caused a significant rise in cyberchondria and anxiety among individuals who search for web-based medical information. To cope with this information overload and safeguard their mental well-being, individuals may adopt various strategies. However, the effectiveness of these strategies in mitigating the negative effects of information overload and promoting overall well-being remains uncertain.
Objective: This study aimed to investigate the moderating effect of coping strategies on the relationship between the infodemic-driven misuse of health care and depression and cyberchondria. The findings could add a new dimension to our understanding of the psychological impacts of the infodemic, especially in the context of a global health crisis, and the moderating effect of different coping strategies on the relationship between the overuse of health care and cyberchondria and anxiety.
Methods: The data used in this study were obtained from a cross-sectional web-based survey. A professional survey company was contracted to collect the data using its web-based panel. The survey was completed by Chinese individuals aged 18 years or older without cognitive problems. Model parameters of the relationships between infodemic-driven overuse of health care, cyberchondria, and anxiety were analyzed using bootstrapped partial least squares structural equation modeling. Additionally, the moderating effects of coping strategies on the aforementioned relationships were also examined.
Results: A total of 986 respondents completed the web-based survey. The mean scores of the Generalized Anxiety Disorder-7 and Cyberchondria Severity Scale-12 were 8.4 (SD 3.8) and 39.7 (SD 7.5), respectively. The mean score of problem-focused coping was higher than those of emotion- and avoidant-focused coping. There was a significantly positive relationship between a high level of infodemic and increased overuse of health care (bootstrapped mean 0.21, SD 0.03; 95% CI 0.1581-0.271). The overuse of health care resulted in more severe cyberchondria (bootstrapped mean 0.107, SD 0.032) and higher anxiety levels (bootstrapped mean 0.282, SD 0.032) in all the models. Emotion (bootstrapped mean 0.02, SD 0.008 and 0.037, SD 0.015)- and avoidant (bootstrapped mean 0.026, SD 0.009 and 0.049, SD 0.016)-focused coping strategies significantly moderated the relationship between the overuse of health care and cyberchondria and that between the overuse of health care and anxiety, respectively. Regarding the problem-based model, the moderating effect was significant for the relationship between the overuse of health care and anxiety (bootstrapped mean 0.007, SD 0.011; 95% CI 0.005-0.027).
Conclusions: This study provides empirical evidence about the impact of coping strategies on the relationship between infodemic-related overuse of health care services and cyberchondria and anxiety. Future research can build on the findings of this study to further explore these relationships and develop and test interventions aimed at mitigating the negative impact of the infodemic on mental health.
Introduction
Covid-19–related mental health problems.
In today’s technologically advancing society, widespread and rapid digitization has led to a substantial increase in the use of social media and the internet. This, in turn, has facilitated the rapid dissemination of all types of information. Although this can be beneficial in filling information gaps quickly, it has its drawbacks. A prominent drawback is the amplification of harmful messages, which can have negative effects on individuals [ 1 , 2 ]. The World Health Organization (WHO) acknowledged the presence of an infodemic during the COVID-19 pandemic and subsequent responses. WHO defines an infodemic as an excessive amount of information, including both accurate and inaccurate content [ 3 ]. This abundance of information makes it difficult for individuals to distinguish reliable sources from unreliable sources and to find trustworthy guidance when they need it.
Excessive use of health care services can have adverse effects on individuals and the overall sustainability of health care systems. Although challenges associated with the overuse of health care services were evident before the COVID-19 pandemic [ 4 , 5 ], the urgent need for sustainable health care systems was exacerbated by the pandemic. Because large portions of the population were instructed to self-isolate at home and had limited access to health care professionals during the pandemic, the internet became the primary source of information for numerous individuals seeking answers to health-related questions. However, the abundance of web-based information, including both true and false content, can leave individuals feeling overwhelmed and struggling to make informed choices. This information overload can lead to depression because individuals bombarded with conflicting messages may feel unsure of what to believe [ 6 - 10 ].
Besides depression, cyberchondria has also emerged as a significant public health challenge since the onset of the COVID-19 pandemic. This refers to the repeated and excessive search for health-related information on the internet, leading to a significant increase in distress or anxiety [ 11 ]. Although the global emergency caused by the COVID-19 pandemic is over, telehealth remains a growing trend. An increasing number of studies have indicated that telehealth can improve health care access, outcomes, and affordability by offering a bridge to care and an opportunity to reinvent web-based care models [ 12 ]. However, increasing internet exposure increases the risk of cyberchondria, especially under conditions of uncertainty and increased risk, due to the large volume of information it contains. Thus, it is crucial to understand how to provide support and guidance to help people adopt appropriate strategies for using web-based resources safely in the context of an infodemic.
Current Research on the COVID-19–Related Infodemic
The harms of infodemic are well documented. An Italian study suggested developing early warning signals for an infodemic, which can provide important cues for implementing effective communication strategies to mitigate misinformation [ 13 ]. Other studies have shown that successful use of coping strategies can help individuals manage stressful events and reduce negative emotions during a pandemic. For example, Yang [ 14 ] found a positive correlation between emotion-focused coping and cyberbullying and depression during the COVID-19 pandemic. A large-scale UK study indicated that supportive coping was associated with a faster decrease in depression and anxiety symptoms [ 15 ]. Shigeto et al [ 16 ] emphasized the importance of training young adults to develop resilience, flexibility, and specific coping skills to offset the psychological effects of significant lifestyle changes resulting from pandemics or other health crises in the future. A recent study used machine learning technology to enhance the accuracy and efficiency of automated fact-checking and infodemic risk management at a strategic level [ 17 ]. However, the impact of coping strategies on the relationship among the infodemic, cyberchondria, and anxiety at an individual level during the COVID-19 pandemic is still unknown.
Importance of Coping Strategies
The ability of individuals to discern and adopt appropriate coping strategies can have a profound impact on their mental health, particularly in relation to conditions such as depression and anxiety. The ability to select and implement coping strategies is not uniform across all individuals, and these differences can significantly influence the trajectory of their mental health outcomes. For some, the ability to effectively choose and implement coping strategies can serve as a protective factor, mitigating the severity of the symptoms of depression or anxiety and promoting overall health and well-being. Conversely, for others, inability or difficulty in selecting and implementing effective coping strategies can exacerbate mental health conditions, leading to increased severity of depression and anxiety. This, in turn, can have detrimental effects on individuals’ overall health and well-being. Therefore, understanding the factors that influence individuals’ ability to select and implement effective coping strategies is of paramount importance in the field of mental health research and intervention [ 18 ].
Research has demonstrated the importance of appropriate coping mechanisms in managing mental health problems. Coping strategies, which are essential for dealing with stress or challenging situations, can be categorized into 3 primary types: emotion focused, problem focused, and avoidant focused [ 19 ]. Emotion-focused strategies are centered around managing and regulating emotions. They serve as a means to cope with stress or difficult situations. These strategies might involve seeking emotional support from others, using relaxation techniques, or practicing mindfulness. In contrast, problem-focused strategies actively address the problem or stressor. These strategies might encompass problem-solving, devising a plan of action, or seeking information and resources to effectively tackle the situation. Avoidant-focused strategies involve evading or distancing oneself from the stressor or problem. These strategies might include denial, distraction, or engaging in activities to escape or avoid contemplating the issue [ 18 ]. The effectiveness of different coping strategies can vary depending on the situation. Individuals often use different or a combination of strategies, tailoring their approach to their circumstances.
Coping Strategies in the COVID-19–Related Infodemic
From a social perspective, this study underscores the importance of mental health in the context of public health emergencies such as the COVID-19 pandemic. It highlights the need for society to recognize and address the mental health burden that such emergencies can place on individuals, particularly in relation to the phenomenon of cyberchondria, which is the unfounded escalation of concerns about common symptoms based on reviews of web-based literature and resources.
Practically, this study provides valuable insights for policy makers and practitioners. It emphasizes the need for the development of effective coping strategies and programs to manage the negative impact of an overload of misinformation and disinformation on mental health. This is particularly relevant in the digital age, where individuals have access to a plethora of information, not all of which is accurate or reliable. Policy makers and practitioners can use the findings of this study to design interventions that not only provide accurate information but also equip individuals with the skills to distinguish reliable sources from unreliable sources and to cope with the anxiety that misinformation can cause. From a research standpoint, this study fills a gap in the literature by assessing the impact of the infodemic on cyberchondria and the moderating effect of coping strategies in this relationship. It opens up new avenues of research into the complex interplay among public health emergencies, infodemic, cyberchondria, and coping strategies. Future research could build on the findings of this study to further explore these relationships and develop and test interventions aimed at mitigating the negative impact of infodemic on mental health.
Objective of the Study
Currently, the association between the overuse of health care services and mental health problems in the context of an infodemic remains unclear, as is the moderating effect of different coping strategies on this association. Thus, this study investigated the moderating effect of coping strategies on the relationship between the infodemic-driven misuse of health care and depression and cyberchondria.
Hypotheses of the Study
The study used a hypothesis-driven format. Specifically, there are five hypotheses: (1) a positive relationship exists between infodemic and the misuse of health care, (2) a positive relationship exists between the misuse of health care and depressive disorders, (3) a positive relationship exists between the misuse of health care and cyberchondria, (4) coping strategies mitigate the negative effect of the misuse of health care on depression, and (5) coping strategies mitigate the negative effect of the misuse of health care on cyberchondria. Hypotheses 2-5 are separately evaluated for the three types of coping strategies: problem focused (H2.1), emotion focused (H2.2), and avoidant focused (H2.3).
Study Design and Sample Size
The data used in this study were obtained from a cross-sectional and web-based survey conducted between April and May 2023 in China.
There is no gold standard for sample estimation in partial least squares structural equation modeling (PLS-SEM). Following Hair et al [ 20 ], we set the significance level at 5% and the minimum path coefficients to between 0.05 and 0.1. Based on these criteria, a minimum sample size of 619 was determined.
Data Source and Collection
A professional surveying company, WenJuanXing, was invited to collect the data through its web-based panel. The panel of WenJuanXing consists of 2.6 million members, with an average of over 1 million questionnaire respondents daily. At the beginning of the project, a survey manager collaborated with the research team to screen and recruit participants using the company’s internal social network platform. All of the eligible panel members received a survey invitation, and a voluntary response sampling method was used. The survey manager checked the data quality using WenJuanXing’s artificial intelligence data quality control system to ensure that respondents met our inclusion criteria and provided valid responses, thus ensuring a high level of data accuracy and integrity. The inclusion criteria were (1) aged older than 18 years, (2) able to understand and read Chinese, and (3) agreed to provide informed consent. All eligible respondents were invited to participate in a web-based survey. The first section of the survey was the informed consent, which the participants were required to read and agree to before proceeding. All the participants who agreed to participate in the survey were asked to complete six questionnaires covering (1) demographics and socioeconomic status, (2) COVID-19 information–related questions, (3) a cyberchondria questionnaire, (4) an eHealth literacy questionnaire, (5) an anxiety questionnaire, and (6) a coping strategy questionnaire. The English translations of the questionnaires are presented in Multimedia Appendix 1 . To ensure data quality, we collaborated with the survey company and implemented various indicators. We monitored completion time, excluding responses that took less than 6 minutes. We also tracked ID addresses, ensuring that each ID address could only complete the questionnaire once. To minimize random errors, we used an artificial intelligence formula developed by the survey company to identify and filter any response patterns that appeared to be generated in parallel.
Ethical Considerations
The study protocol and informed consent process were approved by the institutional review board of the Hong Kong Polytechnic University (HSEARS20230502006). Informed consent was collected from all participants. The survey was conducted anonymously, and no personally identifiable information was collected. No compensation was provided by the research team.
Instruments
Cyberchondria severity scale-12.
The Cyberchondria Severity Scale-12 (CSS-12), derived from the 33-item CSS, was used to measure the severity of cyberchondria. The CSS-12 exhibited equally good psychometric properties as the original version and has been validated in Chinese populations [ 21 ]. The CSS-12 items are scored on a Likert-type scale ranging from 1=“never” to 5=“always,” giving total scores ranging from 12 to 60. A higher score indicates a higher severity of suspected cyberchondria. The psychometric properties of the Chinese version of the CSS-12 were reported by Peng et al [ 22 ].
Generalized Anxiety Disorder Assessment
The Generalized Anxiety Disorder Assessment-7 (GAD-7) was used to screen for generalized anxiety disorder and related anxiety disorders [ 23 ]. This scale consists of 7 items designed to assess the frequency of anxiety symptoms during the 2 weeks preceding the survey. The GAD-7 score is calculated by assigning scores of 0, 1, 2, and 3 to the response categories of “not at all,” “several days,” “more than half the days,” and “nearly every day,” respectively. The scores of the 7 questions are then summed, giving a total ranging from 0 to 21, with higher scores indicating a higher severity of anxiety disorders. Many studies have reported the psychometric properties of the GAD-7 in Chinese populations, such as that conducted by Sun et al [ 24 ].
Coping Orientation to Problems Experienced Inventory
The Coping Orientation to Problems Experienced Inventory (Brief-COPE) is a 28-item self-report questionnaire used to measure effective and ineffective strategies for coping with a stressful life event [ 25 ]. The Brief-COPE assesses how a person deals with stressors in their daily life. The questionnaire measures 3 coping strategy dimensions: problem focused, emotion focused, and avoidant focused [ 26 ]. Each item is rated on a 4-point scale. The scores for the 3 overarching coping styles are calculated as average scores. This is done by dividing the sum of the item scores by the number of items. These average scores indicate the extent to which the respondent engages in each coping style. A higher score indicates that the respondent does not have many coping skills. The Chinese version of the Brief-COPE and its psychometric properties in Chinese populations were reported by Wang et al [ 27 ].
Infodemic- and Misinformation-Driven Overuse of Health Care Services
The COVID-19–related infodemic and misinformation-driven medical misbehavior were assessed using 2 self-developed items. The first item was “Do you believe there is an excessive amount of information regarding the COVID virus and vaccine on a daily basis?” The second item was “Has misinformation or disinformation about COVID-19 led you to engage in the overuse of health care services (eg, frequently visiting the doctor/psychiatrist or buying unnecessary medicine)?” The respondents were required to indicate their response to these 2 questions by selecting 1 of 2 options presented dichotomously: yes or no.
Statistical Analysis
Descriptive statistics were used to describe the participants’ background characteristics. Continuous variables (eg, age) were calculated as means and SDs. Categorical variables (eg, sex) were calculated as frequencies and proportions. The Pearson correlation coefficient ( r ) was used to examine the association between measures, where r ≥0.3 and r ≥0.5 indicated moderate and large effects, respectively [ 28 , 29 ].
In this study, we used PLS-SEM to estimate the research model parameters, as it works efficiently with small samples and complex models. Compared with covariance-based structural equation modeling, PLS-SEM has several advantages, such as the ability to handle non-normal data and small samples [ 30 ]. Unlike covariance-based structural equation modeling, which focuses on confirming theories, PLS-SEM is a causal-predictive approach that explains variance in the model’s dependent variables [ 31 ]. To improve the model fit, we used the bootstrapping method with 10,000 replications to obtain the estimates of the mean coefficients and 95% CIs [ 32 ]. Composite reliability rho_a (>0.7), composite reliability rho_c (>0.7), and average variance extracted (>0.5) were used to examine the model performance.
PLS-SEM encompasses measurement models that define the relationship between constructs (instruments) and indicator variables and a structural model. The structural model used in this study is presented in Figure 1 . We hypothesized that the infodemic significantly affects misinformation-driven medical misbehavior, resulting in cyberchondria and high anxiety levels. Furthermore, we speculated that coping strategies significantly modify this relationship. To test these hypotheses, we used 3 models that used the full sample to separately investigate the moderating effect of the 3 types of coping strategies (problem focused, emotion focused, and avoidant focused). We analyzed the data and estimated the PLS-SEM parameters using the “SEMinR” package in R (R Foundation for Statistical Computing). A P value of ≤.05 was considered statistically significant.

Background Characteristics of Participants
A total of 986 respondents completed the web-based survey and provided valid responses, resulting in a response rate of 84%. Among the participants, 51.7% (n=510) were female, approximately 95% (n=933) had completed tertiary education or above, and 71.2% (n=702) resided in urban areas. The participants’ background characteristics are listed in Table 1 .
a A currency exchange rate of 7.23 CNY=US $1 applies.
Mean Scores and Frequency of Responses
The mean score of the GAD-7 was 8.4 (SD 3.8), while the mean score of the CSS-12 was 39.7 (SD 7.5). Problem-focused coping had a higher mean score than emotion- and avoidant-focused coping. Respondents with active employment reported statistically significantly higher mean scores on the GAD and avoidant-focused coping subscale compared to those with nonactive employment. A higher proportion of respondents with chronic diseases experienced an infodemic and exhibited the overuse of health care services relative to those without chronic diseases ( Table 2 ). The correlations between all of the measures are presented in Multimedia Appendix 2 .
a GAD-7: Generalized Anxiety Disorder Assessment-7.
b CSS-12: Cyberchondria Severity Scale-12.
c COPE: Coping Orientation to Problems Experienced Inventory.
g P <.001.
Measurement Models
Tables 3 - 5 present the performance of the measurement models for the 3 coping strategies. The values of rho_C and rho_A were above 0.7, indicating acceptable construct reliability. All 3 constructs had Cronbach α values exceeding the cutoff of 0.7, indicating adequate reliability. Table 2 presents the models’ convergent validity. All the bootstrapped item loadings exceeded 0.3 and were significant at <.05 for the problem- and avoidant-focused models. However, for cyberchondria and the Brief-COPE, none of the average variance extracted values were above 0.5, indicating unsatisfactory model convergent validity.
a AVE: average variance extracted.
b GAD-7: Generalized Anxiety Disorder-7.
d HC: health care.
b GAD-7: Generalized Anxiety Disorder.
Structural Models
The structural model analysis involved estimating path coefficients for the conceptual model. We performed PLS-SEM on the research model 3 times to estimate path coefficients for the models with different coping strategies. We found that H1 was supported. A significant and positive relationship was observed between a high level of infodemic exposure and increased overuse of health care services (coefficient=0.212, 95% CI 0.151-0.271). In addition, the overuse of health care services was correlated with more severe cyberchondria and higher anxiety levels in all the 3 models, supporting H2 and H3. The effect of the overuse of health care services on cyberchondria was larger than its effect on anxiety. All these relationships were statistically significant ( Tables 3 - 5 ).
Moderating Effects
In our moderation analyses ( Figure 2 and Tables 6 and 7 ), we found that emotion- and avoidant-focused coping strategies significantly moderated the relationship between the overuse of health care services and cyberchondria and that between the overuse of health care services and anxiety, respectively, supporting H5 and H6. For the problem-based model (H4), the moderating effect was not significant for the relationship between the overuse of health care services and cyberchondria (coefficient=0.002, 95% CI −0.011 to 0.006), indicating that H4.1 was not supported. Compared with the direct effects on the relationship between the overuse of health care services and cyberchondria or anxiety, a strong ability to cope with difficulties can effectively mitigate the negative effects of the infodemic-driven overuse of health care services on cyberchondria and anxiety.

a HC: health care.
b GAD: Generalized Anxiety Disorder Assessment.
b CS: coping strategy.
c GAD: Generalized Anxiety Disorder Assessment.
Principal Findings
We performed a series of PLS-SEM analyses to examine the relationships between the infodemic-driven overuse of health care services and cyberchondria and anxiety and determine the moderating effects of 3 types of coping strategies on these relationships. We observed that the individuals who were exposed to an overload of COVID-19–related information were more likely to seek and use extra and unnecessary health care services during the pandemic. Such behavior may lead to a considerable wastage of health resources that are particularly limited during a public health crisis. Although some studies have indicated that during the COVID-19 pandemic individuals with increasing mental health symptoms rarely used mental health services [ 33 - 35 ], we found that the overuse of health care services may contribute to higher levels of depression and cyberchondria during a pandemic. This finding has never been reported before. However, we did not differentiate between the types of health care services, either physical or mental, that the individuals overused during the pandemic. This limitation may affect the implications of our findings for policy making purposes.
Comparisons With Previous Studies
We observed that enhanced coping strategies can mitigate the adverse effects of overusing health care on depression and cyberchondria. Studies have confirmed the association between pandemics and depression, have identified several sources of depression [ 6 , 7 , 10 , 36 , 37 ], and have determined the relationship between depression and cyberchondria [ 38 ]. However, few studies have investigated the relationship between depression or cyberchondria and the infodemic-driven overuse of health care services. Our findings demonstrate that the adverse effects of the pandemic are diverse and require the investigation of individuals’ health from multiple perspectives (ie, infodemic in health communication, the use of health care in health service research, and depression in psychiatry). These effects might not be immediately apparent, but they are all linked to each other and collectively cause harm. Thus, policy makers should develop a comprehensive and cost-effective strategy to address the potential adverse effects of pandemics on people’s health and well-being and better prepare for the next public health crisis.
This study offers new insights into the role of coping strategies in mediating the relationship between health care overuse and depression or cyberchondria during the COVID-19 pandemic. Overall, individuals with strong coping abilities were more likely to report lower levels of depression or cyberchondria than those with weak coping abilities. However, the moderating effects of different coping strategies varied slightly. We discovered that problem-focused coping strategies resulted in lower levels of depression and cyberchondria than avoidant-focused coping strategies. Additionally, emotion-focused coping strategies led to lower levels of depression than the other 2 types of coping strategies. These findings partially align with previous studies. For instance, Li [ 39 ] demonstrated that using both problem-focused and emotion-focused coping strategies was beneficial for psychological well-being. However, previous studies have reported mixed findings. For example, AlHadi et al [ 40 ] indicated that emotion-focused coping strategies were associated with increased depression, anxiety, and sleep disorders in the Saudi Arabian population. Few studies have examined the effect of avoidant-focused coping strategies. In this study, we found that respondents who reported living with chronic diseases exhibited a higher ability to use avoidant-focused coping. This finding is partially consistent with a previous study that found a positive relationship between avoidance-focused coping strategies and mental health in women with heart disease [ 41 ]. Individuals with medical conditions are more likely to adopt avoidant coping strategies. Firouzbakht et al [ 42 ] explained that avoidance is an effective strategy for handling short-term stress and is more likely to be adopted by certain patient groups.
We found that individuals who favor emotion-focused coping strategies to overcome difficulties are able to effectively mitigate the adverse effects of excessive health care use on depression and cyberchondria relative to those who opt for the other 2 coping strategies. This finding is not entirely surprising or unexpected. It is, in fact, quite reasonable when one considers that scholars and researchers in the field have previously indicated that people have a tendency to adopt emotion-focused strategies, especially when they find themselves in situations that are uncontrollable or unpredictable, such as the ongoing global pandemic [ 43 ]. Some studies have found that age can have a significant impact on an individual’s coping strategy preferences. For instance, younger adults were more likely to use emotion-focused coping strategies during the acute phase of the SARS outbreak, whereas older adults used this particular strategy several months after the outbreak had initially occurred [ 44 ]. This suggests that the coping strategies adopted by individuals can vary greatly depending on their age and the stage of the crisis they are experiencing. However, in the context of this study, we did not observe any significant differences in the coping strategy preferences of the different age groups. This could be due to a variety of factors, but a possible explanation is that our model incorporated the COVID-19 infodemic. In this context, it is understandable that providing emotional support might be more important than providing real solutions. This is particularly true in the current digital age, where the internet offers unlimited information sources for people to explore, which can often lead to information overload and increased anxiety. Therefore, emotion-focused coping strategies could be more beneficial in helping individuals navigate the sea of information and manage their emotional responses effectively.
In this study, we used self-developed items to measure the infodemic and overuse of health care services. While this approach allowed us to collect data that were directly related to the research questions, it may have introduced some potential issues. First, self-developed items may have less validity and reliability than standardized questionnaires. This could affect the accuracy of measurements and the validity of findings. Second, using self-developed items may limit comparability with other studies that use standardized questionnaires. Standardized questionnaires allow for easy comparison across studies and populations. The lack of a common metric may make it challenging to compare the findings of this study to other studies or to aggregate them in future meta-analyses. Finally, self-developed items may be more susceptible to response bias. They may not have considered factors like social desirability bias or acquiescence bias as standardized questionnaires do. This could have skewed the responses and affected the accuracy of the findings. Despite these limitations, the study’s findings provide valuable insights and pave the way for future research in this area.
Main Contributions of This Study
The importance of preparedness, prevention, and emergency response to infodemiology is highly encouraged by the WHO [ 45 ]. This study makes a significant contribution by exploring and empirically evaluating the relationship between the infodemic, the overuse of health care services, cyberchondria, and anxiety in the context of the COVID-19 pandemic. It provides empirical evidence supporting the assertion that a high level of infodemic can lead to the increased overuse of health care services, resulting in more severe cyberchondria and heightened anxiety levels. This finding adds a new dimension to our understanding of the psychological impacts of the infodemic, especially in the context of a global public health crisis. Additionally, this study highlights that adopting appropriate coping strategies can potentially reduce the severity of cyberchondria and anxiety, even among people facing high levels of the infodemic and the overuse of health care services.
Future Research
The study’s findings emphasize the importance of coping strategies in reducing the negative effects of the infodemic and the excessive use of health care. Future research could focus on developing and testing interventions to improve coping skills, such as cognitive-behavioral, mindfulness-based, or psychoeducational approaches. Additionally, other factors like social support, personality traits, or health literacy may moderate the relationship between infodemic, health care overuse, cyberchondria, and anxiety. Future research could further explore these variables. This study’s findings may not apply to all populations, so future research could investigate these relationships in different groups, including those with pre-existing mental health conditions, health care professionals, or diverse cultural contexts. By pursuing these future directions, researchers could build on this study’s findings, thereby enhancing our understanding of the psychological impact of infodemic and developing effective interventions.
Limitations
This study has several limitations that need to be addressed. A primary limitation is that the data were cross-sectional and self-reporting, which can introduce several biases. Social desirability bias may occur when respondents provide answers they believe are socially acceptable rather than truthful. Recall bias may also be present, as the respondents were asked to recall experiences from months or even a year ago. The data are also prone to response bias, as respondents may agree or disagree with statements regardless of their content. These biases may have affected the accuracy of the findings. In the future, we will try to collect data at multiple time points to reduce the biases and identify changes over time. Second, the data used in this analysis were obtained from a web-based survey, which excluded individuals who are not familiar with web-based surveys or do not have access to the internet. This could have resulted in selection bias. Additionally, due to the nature of the web-based survey, the demographic information of our sample was highly skewed. The majority of the respondents were young and highly educated and were frequent internet users who may have experienced more infodemic effects than older and less educated individuals. This may have affected the reliability of our findings. A quota sampling method could be used in future studies to improve the representativeness of the sample. Third, the study was conducted in China; thus, it is important to consider the unique context of China when interpreting the results. It is necessary to conduct further research in different cultural and regional contexts to determine the generalizability of the results. Finally, the evaluation of health care service overuse and strength of the infodemic relied on 2 self-developed items, which may have affected the measurement properties and limited the reliability of our findings. The development of standardized questionnaires to measure the infodemic and the overuse of health care services during a pandemic would be a valuable contribution to future research in this field.
Conclusions
This study is the first to demonstrate a significant correlation between the infodemic-driven overuse of health care services and high levels of depression and cyberchondria in the Chinese population during the COVID-19 pandemic. We find that 3 types of coping strategies can effectively mitigate the adverse effects of infodemic-driven health care overuse on depression and cyberchondria. Among them, emotion-focused coping strategies have stronger moderating effects than the other 2 types of coping strategies. These findings provide empirical evidence that can guide policy makers in developing strategies to reduce cyberchondria, provide accurate information about public health crises, and promote adaptive coping strategies to effectively manage future public health crises.
Data Availability
The data sets generated and analyzed during this study are available from the corresponding author on reasonable request.
Authors' Contributions
RHX contributed to developing the study concept and design, data analysis and interpretation, software, writing the original draft, and review and editing. CC contributed to data collection, software, and review and editing. Both authors approved the submitted version.
Conflicts of Interest
None declared.
English-translated questionnaire.
Correlations between measures.
- Joseph AM, Fernandez V, Kritzman S, Eaddy I, Cook OM, Lambros S, et al. COVID-19 misinformation on social media: a scoping review. Cureus. 2022;14(4):e24601. [ FREE Full text ] [ CrossRef ] [ Medline ]
- Farsi D, Martinez-Menchaca HR, Ahmed M, Farsi N. Social media and health care (Part II): narrative review of social media use by patients. J Med Internet Res. 2022;24(1):e30379. [ FREE Full text ] [ CrossRef ] [ Medline ]
- Health topic - Infodemic. World Health Organization. 2023. URL: https://www.who.int/health-topics/infodemic#tab=tab_1 [accessed 2023-05-14]
- Gogol M, Siebenhofer A. [Choosing wisely—against overuse in healthcare systems—activities in Germany and Austria in geriatric medicine]. Wien Med Wochenschr. 2016;166(5-6):155-160. [ FREE Full text ] [ CrossRef ] [ Medline ]
- Morgan DJ, Leppin AL, Smith CD, Korenstein D. A practical framework for understanding and reducing medical overuse: conceptualizing overuse through the patient-clinician interaction. J Hosp Med. 2017;12(5):346-351. [ FREE Full text ] [ CrossRef ] [ Medline ]
- Alnazly E, Khraisat OM, Al-Bashaireh AM, Bryant CL. Anxiety, depression, stress, fear and social support during COVID-19 pandemic among Jordanian healthcare workers. PLoS One. 2021;16(3):e0247679. [ FREE Full text ] [ CrossRef ] [ Medline ]
- Choi EPH, Hui BPH, Wan EYF. Depression and anxiety in Hong Kong during COVID-19. Int J Environ Res Public Health. 2020;17(10):3740. [ FREE Full text ] [ CrossRef ] [ Medline ]
- Tomasoni D, Bai F, Castoldi R, Barbanotti D, Falcinella C, Mulè G, et al. Anxiety and depression symptoms after virological clearance of COVID-19: a cross-sectional study in Milan, Italy. J Med Virol. 2021;93(2):1175-1179. [ FREE Full text ] [ CrossRef ] [ Medline ]
- Tran HTT, Nguyen MH, Pham TTM, Kim GB, Nguyen HT, Nguyen NM, et al. Predictors of eHealth literacy and its associations with preventive behaviors, fear of COVID-19, anxiety, and depression among undergraduate nursing students: a cross-sectional survey. Int J Environ Res Public Health. 2022;19(7):3766. [ FREE Full text ] [ CrossRef ] [ Medline ]
- Bareeqa SB, Ahmed SI, Samar SS, Yasin W, Zehra S, Monese GM, et al. Prevalence of depression, anxiety and stress in China during COVID-19 pandemic: a systematic review with meta-analysis. Int J Psychiatry Med. 2021;56(4):210-227. [ FREE Full text ] [ CrossRef ] [ Medline ]
- Starcevic V, Berle D. Cyberchondria: towards a better understanding of excessive health-related internet use. Expert Rev Neurother. 2013;13(2):205-213. [ FREE Full text ] [ CrossRef ] [ Medline ]
- Smith AC, Thomas E, Snoswell CL, Haydon H, Mehrotra A, Clemensen J, et al. Telehealth for global emergencies: implications for coronavirus disease 2019 (COVID-19). J Telemed Telecare. 2020;26(5):309-313. [ FREE Full text ] [ CrossRef ] [ Medline ]
- Gallotti R, Valle F, Castaldo N, Sacco P, De Domenico M. Assessing the risks of 'infodemics' in response to COVID-19 epidemics. Nat Hum Behav. 2020;4(12):1285-1293. [ FREE Full text ] [ CrossRef ] [ Medline ]
- Yang F. Coping strategies, cyberbullying behaviors, and depression among Chinese netizens during the COVID-19 pandemic: a web-based nationwide survey. J Affect Disord. 2021;281:138-144. [ FREE Full text ] [ CrossRef ] [ Medline ]
- Fluharty M, Bu F, Steptoe A, Fancourt D. Coping strategies and mental health trajectories during the first 21 weeks of COVID-19 lockdown in the United Kingdom. Soc Sci Med. 2021;279:113958. [ FREE Full text ] [ CrossRef ] [ Medline ]
- Shigeto A, Laxman DJ, Landy JF, Scheier LM. Typologies of coping in young adults in the context of the COVID-19 pandemic. J Gen Psychol. 2021;148(3):272-304. [ FREE Full text ] [ CrossRef ] [ Medline ]
- Hang CN, Yu PD, Chen S, Tan CW, Chen G. MEGA: Machine Learning-Enhanced Graph Analytics for infodemic risk management. IEEE J Biomed Health Inform. 2023;27(12):6100-6111. [ FREE Full text ] [ CrossRef ] [ Medline ]
- Lazarus RS. Coping theory and research: past, present, and future. Psychosom Med. 1993;55(3):234-247. [ FREE Full text ] [ CrossRef ] [ Medline ]
- Wechsler B. Coping and coping strategies: a behavioural view. Appl Anim Behav Sci. 1995;43(2):123-134. [ FREE Full text ] [ CrossRef ]
- Hair JF, Hair J, Hult GTM, Ringle CM, Sarstedt M. A Primer on Partial Least Squares Structural Equation Modeling (PLS-SEM). Thousand Oaks, CA. SAGE; 2022.
- McElroy E, Kearney M, Touhey J, Evans J, Cooke Y, Shevlin M. The CSS-12: development and validation of a short-form version of the cyberchondria severity scale. Cyberpsychol Behav Soc Netw. 2019;22(5):330-335. [ FREE Full text ] [ CrossRef ] [ Medline ]
- Peng XQ, Chen Y, Zhang YC, Liu F, He HY, Luo T, et al. The status and influencing factors of cyberchondria during the COVID-19 epidemic. a cross-sectional study in Nanyang City of China. Front Psychol. 2021;12:712703. [ FREE Full text ] [ CrossRef ] [ Medline ]
- Spitzer RL, Kroenke K, Williams JBW, Löwe B. A brief measure for assessing generalized anxiety disorder: the GAD-7. Arch Intern Med. 2006;166(10):1092-1097. [ FREE Full text ] [ CrossRef ] [ Medline ]
- Sun J, Liang K, Chi X, Chen S. Psychometric properties of the Generalized Anxiety Disorder Scale-7 Item (GAD-7) in a large sample of Chinese Adolescents. Healthcare (Basel). 2021;9(12):1709. [ FREE Full text ] [ CrossRef ] [ Medline ]
- Carver CS. You want to measure coping but your protocol's too long: consider the brief COPE. Int J Behav Med. 1997;4(1):92-100. [ FREE Full text ] [ CrossRef ] [ Medline ]
- Poulus D, Coulter TJ, Trotter MG, Polman R. Stress and coping in Esports and the influence of mental toughness. Front Psychol. 2020;11:628. [ FREE Full text ] [ CrossRef ] [ Medline ]
- Wang AWT, Cheng CP, Chang CS, Chen DR, Chen ST, Shieh V, et al. Does the factor structure of the brief COPE fit different types of traumatic events? Eur J Psychol Assess. 2018;34(3):162-173. [ FREE Full text ] [ CrossRef ]
- Brown TA. Confirmatory Factor Analysis for Applied Research, Second Edition. New York, NY. Guilford Publications; 2015.
- Nunnally JC. Psychometric Theory, 3rd Edition. New York, NY. Tata McGraw-Hill Education; 1994.
- Hair JF, Sarstedt M, Pieper TM, Ringle CM. The use of partial least squares structural equation modeling in strategic management research: a review of past practices and recommendations for future applications. Long Range Plann. 2012;45(5-6):320-340. [ FREE Full text ] [ CrossRef ]
- Streukens S, Leroi-Werelds S. Bootstrapping and PLS-SEM: a step-by-step guide to get more out of your bootstrap results. Eur Manag J. 2016;34(6):618-632. [ FREE Full text ] [ CrossRef ]
- Hair JF, Hult GTM, Ringle CM, Sarstedt M, Danks NP, Ray S. Partial Least Squares Structural Equation Modeling (PLS-SEM) Using R: A Workbook. Cham, Switzerland. Springer International Publishing; 2021.
- Liberman JN, Bhattacharjee S, Rui P, Ruetsch C, Rothman B, Kulkarni A, et al. Impact of the COVID-19 pandemic on healthcare resource utilization in individuals with major depressive disorder. Health Serv Res Manag Epidemiol. 2022;9:23333928221111864. [ FREE Full text ] [ CrossRef ] [ Medline ]
- Saunders R, Buckman JEJ, Leibowitz J, Cape J, Pilling S. Trends in depression and anxiety symptom severity among mental health service attendees during the COVID-19 pandemic. J Affect Disord. 2021;289:105-109. [ FREE Full text ] [ CrossRef ] [ Medline ]
- Lee J, Jeong HJ, Kim S. Stress, anxiety, and depression among undergraduate students during the COVID-19 pandemic and their use of mental health services. Innov High Educ. 2021;46(5):519-538. [ FREE Full text ] [ CrossRef ] [ Medline ]
- Luceño-Moreno L, Talavera-Velasco B, García-Albuerne Y, Martín-García J. Symptoms of posttraumatic stress, anxiety, depression, levels of resilience and burnout in Spanish health personnel during the COVID-19 pandemic. Int J Environ Res Public Health. 2020;17(15):5514. [ FREE Full text ] [ CrossRef ] [ Medline ]
- Chen J, Liu X, Wang D, Jin Y, He M, Ma Y, et al. Risk factors for depression and anxiety in healthcare workers deployed during the COVID-19 outbreak in China. Soc Psychiatry Psychiatr Epidemiol. 2021;56(1):47-55. [ FREE Full text ] [ CrossRef ] [ Medline ]
- Jungmann SM, Witthöft M. Health anxiety, cyberchondria, and coping in the current COVID-19 pandemic: which factors are related to coronavirus anxiety? J Anxiety Disord. 2020;73:102239. [ FREE Full text ] [ CrossRef ] [ Medline ]
- Li Q. Psychosocial and coping responses toward 2019 coronavirus diseases (COVID-19): a cross-sectional study within the Chinese general population. QJM. 2020;113(10):731-738. [ FREE Full text ] [ CrossRef ] [ Medline ]
- AlHadi AN, Alarabi MA, AlMansoor KM. Mental health and its association with coping strategies and intolerance of uncertainty during the COVID-19 pandemic among the general population in Saudi Arabia: cross-sectional study. BMC Psychiatry. 2021;21(1):382. [ FREE Full text ] [ CrossRef ] [ Medline ]
- Sidhu A, Arora AK. A study of mental health and coping among women with coronary heart disease and women without coronary heart disease. Int J Adv Res Dev. 2017;2(6):1-3. [ FREE Full text ]
- Firouzbakht M, Rahmani N, Nia HS, Omidvar S. Coping strategies and depression during the COVID-19 pandemic in pregnant women: a cross sectional study. BMC Psychiatry. 2022;22(1):153. [ FREE Full text ] [ CrossRef ] [ Medline ]
- Chew QH, Wei KC, Vasoo S, Chua HC, Sim K. Narrative synthesis of psychological and coping responses towards emerging infectious disease outbreaks in the general population: practical considerations for the COVID-19 pandemic. Singapore Med J. 2020;61(7):350-356. [ FREE Full text ] [ CrossRef ] [ Medline ]
- Yeung DYL, Fung HH. Age differences in coping and emotional responses toward SARS: a longitudinal study of Hong Kong Chinese. Aging Ment Health. 2007;11(5):579-587. [ FREE Full text ] [ CrossRef ] [ Medline ]
- Wilhelm E, Ballalai I, Belanger ME, Benjamin P, Bertrand-Ferrandis C, Bezbaruah S, et al. Measuring the burden of infodemics: summary of the methods and results of the Fifth WHO Infodemic Management Conference. JMIR Infodemiology. 2023;3:e44207. [ FREE Full text ] [ CrossRef ] [ Medline ]
Abbreviations
Edited by G Eysenbach, T de Azevedo Cardoso; submitted 05.10.23; peer-reviewed by K Wang, J Chen, CN Hang, E Vashishtha, D Liu; comments to author 06.11.23; revised version received 14.11.23; accepted 22.03.24; published 09.04.24.
©Richard Huan Xu, Caiyun Chen. Originally published in the Journal of Medical Internet Research (https://www.jmir.org), 09.04.2024.
This is an open-access article distributed under the terms of the Creative Commons Attribution License (https://creativecommons.org/licenses/by/4.0/), which permits unrestricted use, distribution, and reproduction in any medium, provided the original work, first published in the Journal of Medical Internet Research, is properly cited. The complete bibliographic information, a link to the original publication on https://www.jmir.org/, as well as this copyright and license information must be included.
- COVID-19 and your mental health
Worries and anxiety about COVID-19 can be overwhelming. Learn ways to cope as COVID-19 spreads.
At the start of the COVID-19 pandemic, life for many people changed very quickly. Worry and concern were natural partners of all that change — getting used to new routines, loneliness and financial pressure, among other issues. Information overload, rumor and misinformation didn't help.
Worldwide surveys done in 2020 and 2021 found higher than typical levels of stress, insomnia, anxiety and depression. By 2022, levels had lowered but were still higher than before 2020.
Though feelings of distress about COVID-19 may come and go, they are still an issue for many people. You aren't alone if you feel distress due to COVID-19. And you're not alone if you've coped with the stress in less than healthy ways, such as substance use.
But healthier self-care choices can help you cope with COVID-19 or any other challenge you may face.
And knowing when to get help can be the most essential self-care action of all.
Recognize what's typical and what's not
Stress and worry are common during a crisis. But something like the COVID-19 pandemic can push people beyond their ability to cope.
In surveys, the most common symptoms reported were trouble sleeping and feeling anxiety or nervous. The number of people noting those symptoms went up and down in surveys given over time. Depression and loneliness were less common than nervousness or sleep problems, but more consistent across surveys given over time. Among adults, use of drugs, alcohol and other intoxicating substances has increased over time as well.
The first step is to notice how often you feel helpless, sad, angry, irritable, hopeless, anxious or afraid. Some people may feel numb.
Keep track of how often you have trouble focusing on daily tasks or doing routine chores. Are there things that you used to enjoy doing that you stopped doing because of how you feel? Note any big changes in appetite, any substance use, body aches and pains, and problems with sleep.
These feelings may come and go over time. But if these feelings don't go away or make it hard to do your daily tasks, it's time to ask for help.
Get help when you need it
If you're feeling suicidal or thinking of hurting yourself, seek help.
- Contact your healthcare professional or a mental health professional.
- Contact a suicide hotline. In the U.S., call or text 988 to reach the 988 Suicide & Crisis Lifeline , available 24 hours a day, seven days a week. Or use the Lifeline Chat . Services are free and confidential.
If you are worried about yourself or someone else, contact your healthcare professional or mental health professional. Some may be able to see you in person or talk over the phone or online.
You also can reach out to a friend or loved one. Someone in your faith community also could help.
And you may be able to get counseling or a mental health appointment through an employer's employee assistance program.
Another option is information and treatment options from groups such as:
- National Alliance on Mental Illness (NAMI).
- Substance Abuse and Mental Health Services Administration (SAMHSA).
- Anxiety and Depression Association of America.
Self-care tips
Some people may use unhealthy ways to cope with anxiety around COVID-19. These unhealthy choices may include things such as misuse of medicines or legal drugs and use of illegal drugs. Unhealthy coping choices also can be things such as sleeping too much or too little, or overeating. It also can include avoiding other people and focusing on only one soothing thing, such as work, television or gaming.
Unhealthy coping methods can worsen mental and physical health. And that is particularly true if you're trying to manage or recover from COVID-19.
Self-care actions can help you restore a healthy balance in your life. They can lessen everyday stress or significant anxiety linked to events such as the COVID-19 pandemic. Self-care actions give your body and mind a chance to heal from the problems long-term stress can cause.
Take care of your body
Healthy self-care tips start with the basics. Give your body what it needs and avoid what it doesn't need. Some tips are:
- Get the right amount of sleep for you. A regular sleep schedule, when you go to bed and get up at similar times each day, can help avoid sleep problems.
- Move your body. Regular physical activity and exercise can help reduce anxiety and improve mood. Any activity you can do regularly is a good choice. That may be a scheduled workout, a walk or even dancing to your favorite music.
- Choose healthy food and drinks. Foods that are high in nutrients, such as protein, vitamins and minerals are healthy choices. Avoid food or drink with added sugar, fat or salt.
- Avoid tobacco, alcohol and drugs. If you smoke tobacco or if you vape, you're already at higher risk of lung disease. Because COVID-19 affects the lungs, your risk increases even more. Using alcohol to manage how you feel can make matters worse and reduce your coping skills. Avoid taking illegal drugs or misusing prescriptions to manage your feelings.
Take care of your mind
Healthy coping actions for your brain start with deciding how much news and social media is right for you. Staying informed, especially during a pandemic, helps you make the best choices but do it carefully.
Set aside a specific amount of time to find information in the news or on social media, stay limited to that time, and choose reliable sources. For example, give yourself up to 20 or 30 minutes a day of news and social media. That amount keeps people informed but not overwhelmed.
For COVID-19, consider reliable health sources. Examples are the U.S. Centers for Disease Control and Prevention (CDC) and the World Health Organization (WHO).
Other healthy self-care tips are:
- Relax and recharge. Many people benefit from relaxation exercises such as mindfulness, deep breathing, meditation and yoga. Find an activity that helps you relax and try to do it every day at least for a short time. Fitting time in for hobbies or activities you enjoy can help manage feelings of stress too.
- Stick to your health routine. If you see a healthcare professional for mental health services, keep up with your appointments. And stay up to date with all your wellness tests and screenings.
- Stay in touch and connect with others. Family, friends and your community are part of a healthy mental outlook. Together, you form a healthy support network for concerns or challenges. Social interactions, over time, are linked to a healthier and longer life.
Avoid stigma and discrimination
Stigma can make people feel isolated and even abandoned. They may feel sad, hurt and angry when people in their community avoid them for fear of getting COVID-19. People who have experienced stigma related to COVID-19 include people of Asian descent, health care workers and people with COVID-19.
Treating people differently because of their medical condition, called medical discrimination, isn't new to the COVID-19 pandemic. Stigma has long been a problem for people with various conditions such as Hansen's disease (leprosy), HIV, diabetes and many mental illnesses.
People who experience stigma may be left out or shunned, treated differently, or denied job and school options. They also may be targets of verbal, emotional and physical abuse.
Communication can help end stigma or discrimination. You can address stigma when you:
- Get to know people as more than just an illness. Using respectful language can go a long way toward making people comfortable talking about a health issue.
- Get the facts about COVID-19 or other medical issues from reputable sources such as the CDC and WHO.
- Speak up if you hear or see myths about an illness or people with an illness.
COVID-19 and health
The virus that causes COVID-19 is still a concern for many people. By recognizing when to get help and taking time for your health, life challenges such as COVID-19 can be managed.
- Mental health during the COVID-19 pandemic. National Institutes of Health. https://covid19.nih.gov/covid-19-topics/mental-health. Accessed March 12, 2024.
- Mental Health and COVID-19: Early evidence of the pandemic's impact: Scientific brief, 2 March 2022. World Health Organization. https://www.who.int/publications/i/item/WHO-2019-nCoV-Sci_Brief-Mental_health-2022.1. Accessed March 12, 2024.
- Mental health and the pandemic: What U.S. surveys have found. Pew Research Center. https://www.pewresearch.org/short-reads/2023/03/02/mental-health-and-the-pandemic-what-u-s-surveys-have-found/. Accessed March 12, 2024.
- Taking care of your emotional health. Centers for Disease Control and Prevention. https://emergency.cdc.gov/coping/selfcare.asp. Accessed March 12, 2024.
- #HealthyAtHome—Mental health. World Health Organization. www.who.int/campaigns/connecting-the-world-to-combat-coronavirus/healthyathome/healthyathome---mental-health. Accessed March 12, 2024.
- Coping with stress. Centers for Disease Control and Prevention. www.cdc.gov/mentalhealth/stress-coping/cope-with-stress/. Accessed March 12, 2024.
- Manage stress. U.S. Department of Health and Human Services. https://health.gov/myhealthfinder/topics/health-conditions/heart-health/manage-stress. Accessed March 20, 2020.
- COVID-19 and substance abuse. National Institute on Drug Abuse. https://nida.nih.gov/research-topics/covid-19-substance-use#health-outcomes. Accessed March 12, 2024.
- COVID-19 resource and information guide. National Alliance on Mental Illness. https://www.nami.org/Support-Education/NAMI-HelpLine/COVID-19-Information-and-Resources/COVID-19-Resource-and-Information-Guide. Accessed March 15, 2024.
- Negative coping and PTSD. U.S. Department of Veterans Affairs. https://www.ptsd.va.gov/gethelp/negative_coping.asp. Accessed March 15, 2024.
- Health effects of cigarette smoking. Centers for Disease Control and Prevention. https://www.cdc.gov/tobacco/data_statistics/fact_sheets/health_effects/effects_cig_smoking/index.htm#respiratory. Accessed March 15, 2024.
- People with certain medical conditions. Centers for Disease Control and Prevention. https://www.cdc.gov/coronavirus/2019-ncov/need-extra-precautions/people-with-medical-conditions.html. Accessed March 15, 2024.
- Your healthiest self: Emotional wellness toolkit. National Institutes of Health. https://www.nih.gov/health-information/emotional-wellness-toolkit. Accessed March 15, 2024.
- World leprosy day: Bust the myths, learn the facts. Centers for Disease Control and Prevention. https://www.cdc.gov/leprosy/world-leprosy-day/. Accessed March 15, 2024.
- HIV stigma and discrimination. Centers for Disease Control and Prevention. https://www.cdc.gov/hiv/basics/hiv-stigma/. Accessed March 15, 2024.
- Diabetes stigma: Learn about it, recognize it, reduce it. Centers for Disease Control and Prevention. https://www.cdc.gov/diabetes/library/features/diabetes_stigma.html. Accessed March 15, 2024.
- Phelan SM, et al. Patient and health care professional perspectives on stigma in integrated behavioral health: Barriers and recommendations. Annals of Family Medicine. 2023; doi:10.1370/afm.2924.
- Stigma reduction. Centers for Disease Control and Prevention. https://www.cdc.gov/drugoverdose/od2a/case-studies/stigma-reduction.html. Accessed March 15, 2024.
- Nyblade L, et al. Stigma in health facilities: Why it matters and how we can change it. BMC Medicine. 2019; doi:10.1186/s12916-019-1256-2.
- Combating bias and stigma related to COVID-19. American Psychological Association. https://www.apa.org/topics/covid-19-bias. Accessed March 15, 2024.
- Yashadhana A, et al. Pandemic-related racial discrimination and its health impact among non-Indigenous racially minoritized peoples in high-income contexts: A systematic review. Health Promotion International. 2021; doi:10.1093/heapro/daab144.
- Sawchuk CN (expert opinion). Mayo Clinic. March 25, 2024.
Products and Services
- A Book: Endemic - A Post-Pandemic Playbook
- Begin Exploring Women's Health Solutions at Mayo Clinic Store
- A Book: Future Care
- Antibiotics: Are you misusing them?
- COVID-19 and vitamin D
- Convalescent plasma therapy
- Coronavirus disease 2019 (COVID-19)
- COVID-19: How can I protect myself?
- Herd immunity and coronavirus
- COVID-19 and pets
- COVID-19 antibody testing
- COVID-19, cold, allergies and the flu
- COVID-19 drugs: Are there any that work?
- Long-term effects of COVID-19
- COVID-19 tests
- COVID-19 in babies and children
- Coronavirus infection by race
- COVID-19 travel advice
- COVID-19 vaccine: Should I reschedule my mammogram?
- COVID-19 vaccines for kids: What you need to know
- COVID-19 vaccines
- COVID-19 variant
- COVID-19 vs. flu: Similarities and differences
- COVID-19: Who's at higher risk of serious symptoms?
- Debunking coronavirus myths
- Different COVID-19 vaccines
- Extracorporeal membrane oxygenation (ECMO)
- Fever: First aid
- Fever treatment: Quick guide to treating a fever
- Fight coronavirus (COVID-19) transmission at home
- Honey: An effective cough remedy?
- How do COVID-19 antibody tests differ from diagnostic tests?
- How to take your pulse
- How to measure your respiratory rate
- How to take your temperature
- How well do face masks protect against COVID-19?
- Is hydroxychloroquine a treatment for COVID-19?
- Loss of smell
- Mayo Clinic Minute: You're washing your hands all wrong
- Mayo Clinic Minute: How dirty are common surfaces?
- Multisystem inflammatory syndrome in children (MIS-C)
- Nausea and vomiting
- Pregnancy and COVID-19
- Safe outdoor activities during the COVID-19 pandemic
- Safety tips for attending school during COVID-19
- Sex and COVID-19
- Shortness of breath
- Thermometers: Understand the options
- Treating COVID-19 at home
- Unusual symptoms of coronavirus
- Vaccine guidance from Mayo Clinic
- Watery eyes
Related information
- Mental health: What's normal, what's not - Related information Mental health: What's normal, what's not
- Mental illness - Related information Mental illness
Your gift holds great power – donate today!
Make your tax-deductible gift and be a part of the cutting-edge research and care that's changing medicine.

An official website of the United States government
The .gov means it’s official. Federal government websites often end in .gov or .mil. Before sharing sensitive information, make sure you’re on a federal government site.
The site is secure. The https:// ensures that you are connecting to the official website and that any information you provide is encrypted and transmitted securely.
- Publications
- Account settings
Preview improvements coming to the PMC website in October 2024. Learn More or Try it out now .
- Advanced Search
- Journal List
- HHS Author Manuscripts

Estimating the association between mental health disorders and suicide: a review of common sources of bias and challenges and opportunities for US-based research
Purpose of review:.
The purpose of this review is to 1) illuminate prevalent methodological approaches and estimates of association between mental health diagnoses and suicide from the meta-analytic literature; 2) discuss key internal and external validity concerns with these estimates; and 3) highlight some of the unique attributes and challenges in US-based suicide research and opportunities to move the evidence base forward.
Recent findings:
Globally, there is considerable variability in measures of association between mental health disorders and suicide and a growing debate over methodological approaches to this research. A high suicide incidence makes the US an outlier, and the decentralized nature of US administrative data poses a unique challenge to data linkage that could otherwise advance this research.
We offer methodological considerations for future research and discuss opportunities made possible by the recent expansion of the US National Violent Death Reporting System to a nationwide registry.
Introduction
Suicide is a leading cause of death internationally and within the United States (US). While global suicide mortality trends have declined over the last several years,( 1 ) the US suicide rate increased 35% between 1999 and 2018, from 10.5 to 14.2 deaths per 100,000 population.( 2 ) Suicide is now the 10 th leading cause of death in the US, accounting for 48,344 deaths in 2018.( 3 )
Acknowledging this concerning trend, in 2012 the US Surgeon General released a National Strategy for Suicide Prevention Plan, which advocated for a comprehensive approach to suicide prevention involving both community and clinical action.( 4 ) The Plan highlighted the widespread consensus that mental health disorders (MHDs), including mood disorders, anxiety disorders, borderline personality disorder, schizophrenia, and substance use disorders (SUD), are leading risk factors for suicide and suicidal behavior.( 4 )
The MHD-suicide association is widely assumed to be established and strong. Perhaps as a result, many publications in this area adopt a seemingly relaxed approach to the methods used to estimate the MHD-suicide association in the literature, with little attention to study specifics (e.g., populations studied, exposure definitions) and variability in magnitudes of associations. For example, it is quite common to find an introductory paragraph in suicide-related studies citing one eye-catching finding that 90% of suicide decedents had a diagnosable MHD, despite the literature’s wide variability in estimates and debate generated by this statistic.( 5 – 10 )
While estimate variability is not automatically a research shortcoming, it becomes problematic when 1) estimates are biased due to internal validity issues or 2) consumers of research attempt to generalize findings that may hold in certain populations, contexts, and circumstances and not others (e.g., external validity issues). Therefore, as researchers and practitioners develop and discuss suicide prevention interventions and policies, it is critical to have a clear understanding of the population characteristics, design assumptions, analytic methodologies, and potential biases underlying the estimates utilized. As such, the purpose of this review is to 1) illuminate some of the prevalent methodological approaches and estimates of association between MHDs and suicide, as captured in the meta-analytic literature, and 2) discuss key internal and external validity concerns with these estimates. Moreover, because the US has an increasing suicide trend characterized by unique risk factors, like firearm ownership and availability, we 3) also highlight some of the unique attributes and challenges in US-based suicide research and opportunities to move the evidence base forward.
Mental health disorders and suicide: findings from the meta-analytic literature
Many meta-analyses have examined the relationship between MHDs and suicide or suicidal behavior ( Table 1 ). While these studies have contributed considerably to the field, they also illuminate some key limitations in the evidence base.
Select meta-analyses and large cohort studies examining associations between mental health and substance use disorders and suicide
OR=odds ratio; SMR=standardized mortality ratio; IRR=incidence rate ratio; SUD=substance use disorder; OCD=obsessive compulsive disorder
In an early example, Harris et al. (1997) reported standardized mortality ratios (SMRs) for suicide based on 249 studies from a range of countries dating back to the 1950s, with the majority published in the 1980s and 1990s.( 11 ) This study appears regularly in suicide-related literature, with over 3,000 citations. Despite its landmark status, the lack of specificity in methods exemplifies common deficiencies in this literature. In explaining the inclusion and exclusion criteria for the study, the authors state that they selected papers with “defined disorders” without giving a clear source of the definitions. While it appears that these disorders are likely based on International Classification of Disease, version 9 (ICD-9-CM) codes, the ICD-9-CM codes utilized by individual investigators may not have been consistent across studies and information is lacking on when the patients were diagnosed or the setting of diagnosis. Although the authors suggest that they only included longitudinal designs, more specific information on the study populations and the rigor of the study designs is warranted. Without this synthesized information, it is difficult to form an impression of which patients are at highest risk or how these associations have trended over time and populations.
Meta-analyses and reviews published since Harris et al. (1997) have generally focused on the associations between specific MHDs, SUDs, and other conditions and suicide, often with highly divergent results.( 12 ) Chesney et al. (2014) reviewed 20 such meta-analyses and published results for many MHDs and SUDs, along with a score reflecting the rigor of the publication in question ( Table 1 ). Unfortunately, Chesney fails to distinguish between analyses of secondary (e.g., specialist) vs. primary care or general population samples, though suicide risks and associations may be quite different across these populations.( 13 ) For example, in another meta-analysis, this one examining MHD prevalence among suicide victims, Bertolote et al. (2004) reported that among those who suicided, the risk of a mood disorder was higher in general population samples than in studies of psychiatric inpatients (44.4% vs. 20.8%).( 14 ) This was also true of SUDs (19.2% vs 9.8%) but not of anxiety/somatoform disorders (2.7% vs 2.5%).( 14 ) While attention to study population sources in this analysis is thorough, the authors included studies with extremely diverse methods of MHD ascertainment, which might also explain some of the population-level variability in their findings.( 14 )
Another common limitation of these meta-analyses is that they reflect data almost exclusively from “Western,” industrialized countries. Too et al. (2019) acknowledged evidence suggesting that MHD-suicide associations may be different in Asian countries, and further found that in their meta-analysis of mostly Scandinavian countries, the study location accounted for a great deal of the heterogeneity in effect estimates.( 15 ) This underscores the importance of context in interpreting these estimates. In the following sections, we delve more deeply into these issues, highlighting common internal and external validity considerations in the MHD and suicide literature.
Internal validity concerns
Internal validity refers to the amount of systematic error in a study’s effect estimate(s). Estimates that have little or no systematic error are described as internally valid. There are three general categories of systematic error that can reduce a study’s internal validity: confounding, selection bias, and measurement or misclassification bias.( 16 ) Below, we highlight key concerns prevalent in the MHD-suicide literature within each of these general categories.
Confounding control
Confounding occurs when effects of extraneous factors are blended with the actual effect of the exposure.( 16 ) There are a number of factors that can and do get blended when attempting to estimate the effect of MHDs on suicide, and these factors can be illuminated using Directed Acyclic Graphs (DAGs).( 17 , 18 ) DAGs are graphs that make explicit researchers’ assumptions about relationships between the outcome, exposure, and covariates.
Figure 1 illustrates a possible DAG for the causal relationship between MHD and suicide, which is informed by scientific findings and underlying suicide theory—the Stress-Diathesis Model is a popular theory that explains suicide as the result of an interaction between 1) distal factors and personal vulnerabilities and 2) proximal stressors from life events.( 19 , 20 ) While DAGs, like the example in Figure 1 , can and should be shaped by the best available evidence and theory, even when they illuminate a minimally sufficient set of factors that should be accounted for in the study design or analyses in order to remove bias, measurement of such factors (e.g., “personality traits”) might be very difficult or impossible to achieve on a population scale. Despite these limitations, DAGs support analysts in pinpointing and addressing known, measured confounders (e.g., by adjusting for them in a statistical model); in thinking through potential quantitative bias analyses for unmeasured confounders; and in helping identify mediators (e.g., factors on a causal pathway between the exposure and outcome) that if included in the model, could induce bias. In the DAG in Figure 1 , “history of self-harm” and “history of suicide attempts” are considered mediators.

Example Directed Acyclic Graph (DAG) depicting potential causal and biasing pathways between mental health and substance use disorders and suicide
ACEs=Adverse Childhood Experiences; MHD=Mental Health Disorder; SUD=Substance Use Diagnosis
Unfortunately, to date, suicide models generally have not taken advantage of the abundant theory regarding causal pathways between MHDs and suicide. This may be due to at least two reasons: unfamiliarity with robust causal inference methods, such as DAGs, and/or an inability to adjust for a minimally sufficient set of factors because they are not measured in the data sources most commonly used, such as administrative data. Instead, it is the norm for MHD-suicide studies to report SMRs that adjust estimates to reflect solely the age and gender distribution of some broader population of interest and to ignore other confounders, such as socioeconomic status, race, or comorbidities.( 12 ) This common reliance on age/sex-adjusted SMRs has hindered the research community’s conversation on confounding control. Moreover, the lack of causal inference theory underlying much of this research may make it more likely for mediators to be considered appropriate members of the adjustment set.( 21 ) While inherent restrictions exist by data source, a much greater degree of attention needs to be paid to the topic of robust confounding control and causal inference in MHD-suicide research in order to inform future data collection, estimation approaches, and quantitative bias analyses meant to address the issue of residual confounding.
Selection bias
Selection bias occurs when the exposure-outcome relationship for a population under study differs from the population that should have been eligible for the study. It results from selecting the study population based on factors related to the exposure and/or outcome and can create a distortion in effect estimates.( 16 ) Many MHD-suicide studies rely on diagnosis codes in health insurance administrative data and medical records to measure both the exposure and outcome. These may be problematic for many reasons. For example, in the US, where health insurance is largely privatized, different populations may have different levels of consistency in their health care coverage and utilization, which means some persons are more likely to be diagnosed (and therefore selected into the study) than others. Additionally, because suicidal behaviors and nonfatal suicide injuries are more likely to trigger a medical visit, the outcome can also increase the chance of selection in some studies. This differential selection according to exposure and outcome is commonly referred to as Berkson’s bias.( 22 ) Tools are available to assess the magnitude of such bias,( 16 ) and researchers should carefully consider how the population they are including in their study might differ from the population they are trying to make inferences about.
Measurement bias or misclassification
Measurement of the exposure and outcome can be a third challenge to internal validity in estimating the effect of MHDs on suicide. Below, we highlight some common sources of exposure and outcome misclassification in this research.
Exposure measurement
As noted above, diagnosis codes are an extremely common means of assessing MHDs and suicidal behaviors; however, in addition to the role they can play in selection bias, researchers should also evaluate their potential measurement bias. First, healthcare providers can be subject to social desirability bias and stigma surrounding MHDs and at times may not be willing to “label” a patient with a MHD, leading to under ascertainment of exposure in diagnosis codes. Second, for those with a diagnosable MHD, patients’ records are not always linked across providers, so a diagnosis such as a MHD, which is often treated in an outpatient setting, may not be present in hospital or primary care records. In this scenario, combining claims with medical records may address some of the missingness in MHD exposure. However, even in this case, because diagnoses are not associated with reimbursement, providers are poorly incentivized to be accurate and specific in their claims documentation. Finally, not all diagnosed illnesses are associated with a claim.
Another likely source of measurement error arises from the use of psychological autopsy (PA) methods for ascertaining MHD history. PA involves conducting interviews with a “proxy” (e.g., next of kin) following a death and is an extremely common study design in suicide research.( 8 ) Several PA meta-analyses have been conducted ( Table 1 ). The PA approach was first developed to assist medical examiners and coroners in their work and was never standardized for research purposes. It is vulnerable to inconsistencies and biases, including inconsistent numbers and types of proxies, lack of standardized proxy interview tools, and a lack of consensus on the source of controls (living or dead) for decedent suicide cases.( 8 ) Recall bias, including bias introduced by interviewing people close to the recently deceased, has not been fully evaluated in these studies, and the time lapse between the death and interviews is often inconsistent or lengthy.( 8 , 13 ) The PA approach was used by Cavanah et al. (2003) to support the previously discussed (and debated) claim that 90% of people who suicide had a diagnosable MHD.( 5 ) In a critique of the PA approach, Hjelmeland et al. (2012) question the reliability and validity of the approach, calling for the use of other, more systematic approaches for interviewing a high number of key informants around each suicide and taking into account the relationship with the deceased during data collection and analysis stages.( 10 )
Finally, exposure misclassification may be more common for specific types of suicide, such as firearm-related suicides. Studies show that individuals with easy access to highly lethal means are more likely to suffer a fatal outcome in the event of a suicide attempt. For example, research indicates that firearm owners are at increased risk of suicide,( 23 – 27 ) often attributed to the fact that firearms are extremely lethal (85% of people who attempt suicide with a firearm die).( 28 ) On average, very little time lapses between the decision to self-inflict lethal force and the act of suicide and time may be even shorter for those with access to highly lethal means. Boggs et al. (2018), using a linkage between US medical records and death records, found that out of 35 MHD and general medicine diagnoses thought to be predictive of suicide, 32 were more predictive of non-firearm suicide than firearm suicide ( Table 1 ).( 29 ) However, this could be a function of opportunities for accurate exposure measurement and, therefore, potential exposure misclassification. Because the time from onset of suicide ideation until death may be shorter among those using firearms, there may be a shorter window of time for these individuals to be seen by health professionals and diagnosed with MHD-related conditions. In a population like the US, where about half of suicides are by firearm, the effects of MHDs on suicide may, therefore, look different than in a country with a different distribution of methods used.
Outcome measurement
The outcome of suicide also may be prone to misclassification for several reasons. First, there may not be enough evidence to determine intent of death (e.g., unintentional overdose vs. suicide by overdose).( 30 ) Second, deaths may be misclassified due to social desirability bias because suicide is stigmatized, and officials may rule them accidental or of undetermined intent to avoid causing embarrassment or financial penalty to family members. Third, officials may be less likely to classify the death as a suicide if the decedent does not fit the typical race and gender profile. The likelihood for suicide among minorities to be classified as “undetermined” is well-documented.( 31 )
External validity concerns
In addition to internal validity concerns, there are a number of external validity concerns that researchers and practitioners should note when trying to apply or “transport” study findings from one context to another. External validity refers to the generalizability of a study to the population of interest. The population of interest may be clinic-based, hospital-based, health systems-based, or geographically-based.
The presence of national health registries and more lenient policies surrounding personal health information in European countries often allow for study designs and results that are applicable to larger populations, such as an entire nation.( 15 , 21 , 32 – 39 ) In comparison, investigators in countries like the US, which lack similar national health registries, tend to focus on subpopulations, such as the military or members of a common health insurance plan. Relatedly, the majority of suicide research appears to take place in European countries. For instance, in Berotlote et al.’s (2004) international meta-analysis, 45% of the suicide deaths came from the United Kingdom, and an additional 26% were contributed by Denmark.( 14 ) As is commonly observed in meta-analyses on this topic, the US contributed just 4% of deaths.
However, as noted earlier, effect estimates of the association between MHDs and suicide vary considerably by population, highlighting the importance of context-specific studies and inferences, as opposed to smoothing over estimates from heterogeneous populations. Connor et al. (2019), demonstrated that major depression was much more predictive of suicide in Asia than in Western regions, while SUDs were less predictive ( Table 1 ).( 40 ) With that in mind, we agree with Rothman that, taken too far, “the pursuit of representativeness can defeat the goal of validly identifying causal relationships.”( 16 ) Thus, some balance must be achieved between these two tensions, and researchers should consider that a small well-defined population with strong internal validity may be of more use than an estimate of effect for a larger population but with little confounding control.
United States-based research challenges
The US faces several distinct challenges in its suicide research and prevention efforts, given its unique firearm culture and fragmented data structures. The increasing rate of suicide in this country is likely attributable to a confluence of factors and could benefit from a larger body of rigorous research. We highlight key characteristics and challenges of US suicide-related research and follow with a discussion of promising paths forward.
Recent suicide research from the US is summarized in a review by Steele et al. (2018)( 41 ), which underscores that US studies frequently focus on suicide risk factors for very specific populations (e.g., youth,( 42 – 44 ) people of advanced age,( 45 , 46 ) prisoners,( 47 ) veterans,( 48 – 54 ) patients with specific diagnoses,( 55 ) twins,( 56 ) patients who otherwise screen as high risk for suicide( 57 – 61 ), or Medicaid beneficiaries( 58 , 59 , 62 , 63 )). Because national registries do not exist and the capacity to link data is often limited, much US research has targeted such smaller, high risk populations. Sources such as schools, prisons, the Veterans’ Health Administration, medical records, and senior care programs facilitate easier study population sampling and data collection than studies of the general population. As a result, relatively few US studies reflect the general population. However, the US suicide rate is increasing among a diverse range of demographic groups, not just these specialized populations.( 64 ) Thus, there is a critical need for studies that assess relationships between MHDs and other risk factors and suicide in a wider swath of the population.
Another characteristic of US suicide research is a focus on risk factors for suicidal behavior (e.g., suicidal ideation, attempts, plans), as opposed to suicide itself. Again, this reflects the available data: while probabilistic linkages between medical records or claims data and death data certainly exist,( 44 , 58 , 59 , 63 , 65 – 67 ) they do not approach the scope or detail available in other countries.( 21 , 34 , 37 ) Meanwhile, US resources such as the National Comorbidity Survey( 68 – 71 ) and The National Epidemiologic Survey on Alcohol and Related Conditions( 72 ) offer nationally representative survey data but investigate non-fatal outcomes.
While US suicide-related research is generally typified by studies of smaller, specific populations and risk factors for suicidal behaviors, there are a few notable exceptions of larger, population-based suicide studies. The Southern Community Cohort Study was a longitudinal prospective study from 2002–2009 of 85,000 low income Black and White American patients in the Southeast and between the ages of 40–79 years.( 73 ) In this sample, patients who reported that they had been diagnosed with or treated for depression had a hazard ratio for suicide of 3.05 (95% confidence interval (CI): 1.70, 5.48) compared to those who had not. Unfortunately, other MHDs were not assessed, and given depression assessment procedures, measurement of depression may have been subject to recall bias ( Table 1 ).
The Mental Health Research Network is a more recent example of the potential for large, longitudinal, civilian data linkages in the US.( 74 ) Using 2,674 suicides cases and 100 matched controls per case in eight health care systems,( 75 ) the authors estimated associations between different healthcare settings and suicide based on the timing, number of visits, and history of MHD or SUD diagnoses. Results indicated an increased odds of suicide in every sub-category; for example, the adjusted odds of suicide within one week of being discharged from the hospital with a SUD diagnosis was 103.68 (95% CI: 66.38, 161.92) times the odds of suicide among outpatients who did not have an SUD.( 75 ) While these examples constitute important suicide research efforts in the US, predictors of suicide are still vastly understudied, relative to the considerable public health burden of suicide.
Data linkage studies utilizing the National Violent Death Reporting System: A promising path forward
The National Violent Death Reporting System (NVDRS) is a unique US surveillance system with linkage potential that offers an opportunity to substantially strengthen the suicide-related evidence base. The NVDRS was developed by the US Centers for Disease Control and Prevention (CDC) in 2002, beginning with 6 states (Massachusetts, Maryland, New Jersey, Oregon, South Carolina, and Virginia).( 76 ) It gradually expanded to include all 50 states, Puerto Rico, and the District of Columbia by 2018. The purpose of the system is to integrate detailed proximal death information on violent deaths, including suicide, homicide, deaths caused by law enforcement, deaths of undetermined intent, and unintentional firearm deaths in order to inform prevention efforts. For these deaths, death certificates, law enforcement reports, medical examiner reports, and toxicology reports are collected and linked, and detailed data from each of these sources is mined by state-level abstractors.
Linkages to NVDRS data offer the potential to overcome several of the biases discussed previously. Because it offers general population surveillance data, there is the potential to link NVDRS to general population controls from other surveillance systems (such as statewide hospital discharge data), which may reduce selection bias while affording sufficient power to evaluate for heterogeneity of effects. The rigorous data design instituted by NVDRS make this resource robust to outcome misclassification; however, for some exposures and confounders (i.e., those based on police statements collected from living associates), this resource is likely subject to the same bias introduced by PA studies.
Studies have linked health data to state VDRS data sources to more fully capture risk factors and trajectories leading to violent deaths, like suicides ( Table 2 ). One Oregon study linked VDRS records on 112 veterans who suicided in 2000–2005 to Veteran’s Health Administration records in order to better understand health contacts in the year prior to death.( 65 ) Rather than diagnoses, the researchers searched for screenings for depression, SUD, post-traumatic stress disorder, or suicidal ideation. They found that 68% of veterans were screened for depression and 81% for a SUD in the year prior to death. The authors also described health care utilization before the suicide, noting that almost half had a mental health-related contact and 55% were seen in a primary care setting in the year prior to death, revealing potentially important opportunities for intervention. In another notable example, Weis et al. (2006) linked South Carolina VDRS data to state hospital inpatient and emergency room billing records, as well as State Department of Mental Health data and criminal justice data.( 66 ) Key findings indicated that 57% of suicide victims were hospitalized or had an emergency department visit, and 19% were seen by the Department of Mental Health in the year prior to death. As a final example, a study using Kentucky’s VDRS linked to statewide emergency department visit data (2008–2010) described events leading up to the suicide and estimated the impact of critical risk factors, like MHDs, on suicide.( 77 ) Results indicated that the odds of suicide among those with MHDs was 1.93 (95% CI: 1.52, 2.45) times the odds of suicide among those without MHDs.
Studies involving linkage with state Violent Death Reporting System data
VDRS=Violent Death Reporting System; DOB=date of birth; DOD=date of death; SSN=social security number; VA=Veterans Administration; SI=suicidal ideation; ED=emergency department; CI=confidence interval
In sum, these examples demonstrate the feasibility of linking state-level VDRS data to health data. In doing so, they have provided a template, including linkage variables and approaches for “fuzzy” linkages, which can be replicated in future studies. However, one limitation of these studies is that they generally have not made use of the rich circumstantial information that VDRS provides surrounding suicides. VDRS data captures (in many cases) the decedent’s recent struggles, mental state, and conflicts prior to death. While this PA-like data come with limitations, these data could be leveraged strategically to flesh out or validate health care backgrounds provided through linkage. Presumably, similar circumstantial information could be collected from controls, or a selection of controls, in order to provide comparisons that could yield a more internally valid measure of association.
Conclusions
Suicide is a leading cause of death around the world, with increasing rates in the US. This likely stems from the US’s firearm culture, which has contributed to high availability of this extremely lethal option. In addition to ease of access to lethal force means, MHDs have long been recognized as key risk factors in the global setting. However, estimates of association between MHDs and suicide have typically been generated by studies situated in other countries. Moreover, many of these studies suffer from a variety of internal validity issues, including inappropriate confounding control, selection bias concerns, and potential exposure and outcome mismeasurement. This is due in part to limitations in population-level data resources globally and particularly in the US.
The NVDRS holds considerable potential for US-based research, offering the potential for larger study populations and more complete variable measurement and corresponding confounding control, particularly when linked with other large health-related databases (e.g., Medicaid, Medicare, medical records, etc.). Understanding unbiased, context-specific relationships between MHDs and death by suicide can help researchers and practitioners identify and appropriately customize interventions for populations at greatest risk.
Acknowledgements:
Financial support for this work was provided by the University of North Carolina at Chapel Hill Injury Prevention Research Center through an award (R49/CE0042479) from the Centers for Disease Control and Prevention, National Center for Injury Prevention and Control. In addition, this research was partially supported by a National Research Service Award Pre-Doctoral/Post-Doctoral Traineeship from the Agency for Healthcare Research and Quality sponsored by The Cecil G. Sheps Center for Health Services Research, The University of North Carolina at Chapel Hill, Grant No. T32-HS000032.
Josie Caves Sivaraman has nothing to disclose.
Dr. Naumann has nothing to disclose.
Human and animal rights: This article does not contain any studies with human or animal subjects performed by any of the authors.
- International edition
- Australia edition
- Europe edition
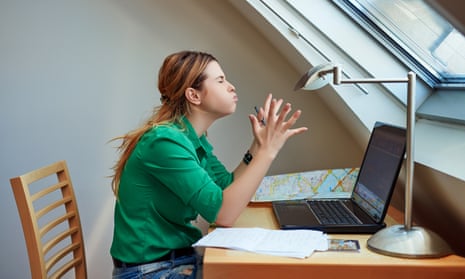
Write down your thoughts and shred them to relieve anger, researchers say
Writing negative reactions on paper and shredding it or scrunching and throwing in the bin eliminates angry feelings, study finds
Since time immemorial humans have tried to devise anger management techniques.
In ancient Rome, the Stoic philosopher Seneca believed “my anger is likely to do me more harm than your wrong” and offered avoidance tips in his AD45 work De Ira (On Anger).
More modern methods include a workout on the gym punchbag or exercise bike. But the humble paper shredder may be a more effective – and accessible – way to decompress, according to research.
A study in Japan has found that writing down your reaction to a negative incident on a piece of paper and then shredding it, or scrunching it into a ball and throwing it in the bin, gets rid of anger.
“We expected that our method would suppress anger to some extent,” said Nobuyuki Kawai, lead researcher of the study at Nagoya University. “However, we were amazed that anger was eliminated almost entirely.”
The study, published in Scientific Reports on Nature , builds on research on the association between the written word and anger reduction as well as studies showing how interactions with physical objects can control a person’s mood. For instance, those wanting revenge on an ex-partner may burn letters or destroy gifts.
Researchers believe the shredder results may be related to the phenomenon of “backward magical contagion”, which is the belief that actions taken on an object associated with a person can affect the individuals themselves. In this case, getting rid of the negative physical entity, the piece of paper, causes the original emotion to also disappear.
This is a reversal of “magical contagion” or “celebrity contagion” – the belief that the “essence” of an individual can be transferred through their physical possessions.
Fifty student participants were asked to write brief opinions about an important social problem, such as whether smoking in public should be outlawed. Evaluators then deliberately scored the papers low on intelligence, interest, friendliness, logic, and rationality. For good measure, evaluators added insulting comments such as: “I cannot believe an educated person would think like this. I hope this person learns something while at the university.”
The wound-up participants then wrote down their angry thoughts on the negative feedback on a piece of paper. One group was told to either roll up the paper and throw it in a bin or keep it in a file on their desk. A second group was told to shred the paper, or put it in a plastic box.
Anger levels of the individuals who discarded their paper in the bin or shredded it returned to their initial state, while those who retained a hard copy of the paper experienced only a small decrease in their overall anger.
Researchers concluded that “the meaning (interpretation) of disposal plays a critical role” in reducing anger.
“This technique could be applied in the moment by writing down the source of anger as if taking a memo and then throwing it away,” said Kawai.
Along with its practical benefits, this discovery may shed light on the origins of the Japanese cultural tradition known as hakidashisara ( hakidashi sara refers to a dish or plate) at the Hiyoshi shrine in Kiyosu, just outside Nagoya. Hakidashisara is an annual festival where people smash small discs representing things that make them angry. The study’s findings may explain the feeling of relief that participants report after leaving the festival, the paper concluded.
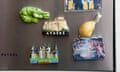
Fridge magnets can be cool aid to holiday memory recall, study finds
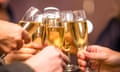
Want to skip that Christmas party? The host probably won’t mind, study shows
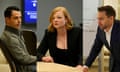
‘Succession syndrome’ prevalent among wealthy households, psychiatrists warn
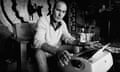
Drugs and alcohol do not make you more creative, research finds
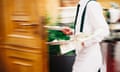
Why being rude to the waiter (or other staff) is the worst strategy
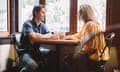
Authors of original dating profiles rated more attractive, research finds
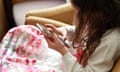
I fear my children are overexposed to technology. Experts say I’m right to worry
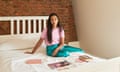
I used to be ashamed of being a fangirl. Now I see how joyous and creative it was
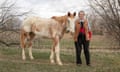
Autistic scholar Temple Grandin: ‘The education system is screening out visual thinkers’
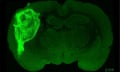
Human neurons transplanted into rats to help study brain disorders
Most viewed.
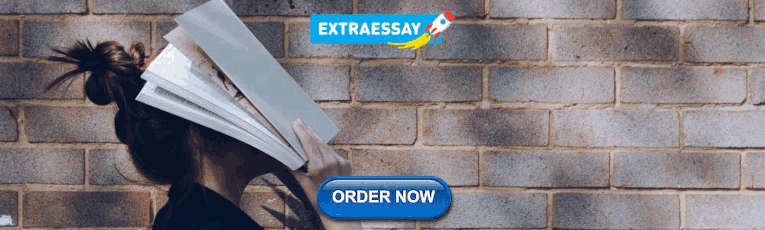
IMAGES
VIDEO
COMMENTS
Overall, the position papers on psychological perspectives converge on several strengths of the European research field: i.e. a substantial body of expertise and knowledge in both basic and clinical research, strong and increasingly more intimate collaborative ties to the biomedical field, and a broader coverage of mental health issues as ...
research findings concerning the etiology and treatment of psychological disorders are based on criteria set 554 Chapter 15 Psychological Disorders This content is available for free at https ...
The findings revealed a 19% concurrent comorbidity between these disorders, and in 65% of the cases, social phobia preceded major depressive disorder by at least 2 years. In addition, initial presentation with social phobia was associated with a 5.7-fold increased risk of developing major depressive disorder. These associations between anxiety ...
In this essay, we have briefly reviewed a selected range of key discoveries that neuroscientific research has made on the topic of depressive disorders in the last decades. ... Garvey M, et al. (2010) Research domain criteria (RDoC): Toward a new classification framework for research on mental disorders. American Journal of Psychiatry 167(7 ...
Anxiety disorders form the most common group of mental disorders and generally start before or in early adulthood. Core features include excessive fear and anxiety or avoidance of perceived threats that are persistent and impairing. Anxiety disorders involve dysfunction in brain circuits that respond to danger. Risk for anxiety disorders is influenced by genetic factors, environmental factors ...
For example, improvement in mental wellbeing over a 10-year period is associated with reducing the risk of developing mental illness by up to 8.2 times in people without mental illness 5,6 and ...
Although traumatic stress has been known for over 100 years by a number of terms, including "shell shock", "battle fatigue", or "soldier's heart" 1, it was only in the 1980s that persistent stress reactions were recognized in psychiatric nosology.In the wake of the mental health problems evident in many troops returning from deployment in Vietnam, the DSM-III introduced the ...
This article discusses the approaches to describing and classifying mental disorders taken by three key organizations: the World Health Organization (WHO), 2 which is in the process of developing the 11th revision of the International Classification of Diseases (ICD), scheduled to be released for use by WHO member states in 2018; the American Psychiatric Association (APA), which published the ...
Explore the latest full-text research PDFs, articles, conference papers, preprints and more on PSYCHOLOGICAL DISORDERS. Find methods information, sources, references or conduct a literature review ...
The fact that mental disorders are the leading causes of the burden of disease make research in mental disorders and policies to promote mental health a Public Health priority, worldwide. ... The Papers. Financial difficulties in childhood and adult depression in Europe Tormod Bøe, Mirza Balaj, Terje A. Eikemo, Courtney L. McNamara, Erling F ...
Research Open Access 08 Apr 2024 Translational Psychiatry. Volume: 14, P: 182. ... Wastewater analysis can quantify the pharmaceuticals used to treat mental health disorders, providing data for ...
1. Introduction and historical overview. Like much research in applied psychology, the history of treating anxiety in young people 1 can be traced back over a century. For the most part, the history of evaluating treatment for anxiety disorders in young people can be described as rigorous, highly informative, and an exemplar of incremental science.
In each issue of Personality Disorders: Theory, Research, and Treatment one accepted manuscript will be selected to serve as an "Editor's Choice" paper. Selection will follow discussion by the editor and associate editor and consider issues such as overall contribution, clinical implications, or the highlighting of important theoretical ...
There are many different mental illnesses, including depression, suicidal ideation, bipolar disorder, autism spectrum disorder (ASD), anxiety disorder, schizophrenia, etc., any of which can have a ...
Personality disorders are highly prevalent in clinical practice. With the introduction of the Diagnostic Statistical Manual - 5th Edition (DSM-5) the multi-axial system of diagnosis for mental disorders has disappeared, which has led to an increase in research on personality disorders (e.g. Emmelkamp & Meyerbröker, 2020).In this Special Issues, several recent developments are discussed.
Recently published articles from subdisciplines of psychology covered by more than 90 APA Journals™ publications. For additional free resources (such as article summaries, podcasts, and more), please visit the Highlights in Psychological Research page. Browse and read free articles from APA Journals across the field of psychology, selected by ...
The current rapid review aimed to summarise the literature and identify gaps in knowledge relating to any psychiatric and medical comorbidities of eating disorders. This paper forms part of a rapid review) series scoping the evidence base for the field of EDs, conducted to inform the Australian National Eating Disorders Research and Translation ...
Binge eating disorder (BED) is a complex and heritable mental health disorder, with genetic, neurobiological, neuroendocrinological, environmental and developmental factors all demonstrated to contribute to the aetiology of this illness. Although psychotherapy is the gold standard for treating BED, a significant subgroup of those treated do not recover. Neurobiological research highlights ...
Purpose Eating disorder recovery is a poorly defined concept, with large variations among researchers' definitions. Weight maintenance is a key aspect of recovery that remains relatively underexplored in the literature. Understanding the role of weight maintenance may help guide the development of treatments. This paper aims to address this by (1) investigating the factors predicting long ...
Background: The COVID-19 pandemic has led to a substantial increase in health information, which has, in turn, caused a significant rise in cyberchondria and anxiety among individuals who search for web-based medical information. To cope with this information overload and safeguard their mental well-being, individuals may adopt various strategies.
Worldwide surveys done in 2020 and 2021 found higher than typical levels of stress, insomnia, anxiety and depression. By 2022, levels had lowered but were still higher than before 2020. Though feelings of distress about COVID-19 may come and go, they are still an issue for many people. You aren't alone if you feel distress due to COVID-19.
Purpose of review: The purpose of this review is to 1) illuminate prevalent methodological approaches and estimates of association between mental health diagnoses and suicide from the meta-analytic literature; 2) discuss key internal and external validity concerns with these estimates; and 3) highlight some of the unique attributes and challenges in US-based suicide research and opportunities ...
41%. Percentage of teens with the highest social media use who rate their overall mental health as poor or very poor, compared with 23% of those with the lowest use. For example, 10% of the highest use group expressed suicidal intent or self-harm in the past 12 months compared with 5% of the lowest use group, and 17% of the highest users expressed poor body image compared with 6% of the lowest ...
A study in Japan has found that writing down your reaction to a negative incident on a piece of paper then shredding it, or scrunching it into a ball and throwing it in the bin, gets rid of anger ...