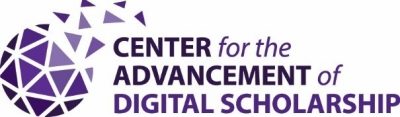
Want to create or adapt books like this? Learn more about how Pressbooks supports open publishing practices.
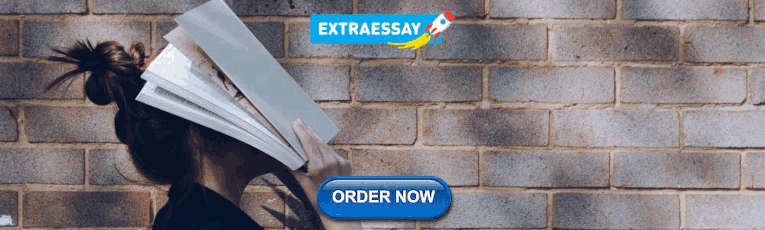
5 Collecting Data in Your Classroom
ESSENTIAL QUESTIONS
- What sort of methodological considerations are necessary to collect data in your educational context?
- What methods of data collection will be most effective for your study?
- What are the affordances and limitations associated with your data collection methods?
- What does it mean to triangulate data, and why is it necessary?
As you develop an action plan for your action research project, you will be thinking about the primary task of conducting research, and probably contemplating the data you will collect. It is likely you have asked yourself questions related to the methods you will be using, how you will organize the data collection, and how each piece of data is related within the larger project. This chapter will help you think through these questions.
Data Collection
The data collection methods used in educational research have originated from a variety of disciplines (anthropology, history, psychology, sociology), which has resulted in a variety of research frameworks to draw upon. As discussed in the previous chapter, the challenge for educator-researchers is to develop a research plan and related activities that are focused and manageable to study. While human beings like structure and definitions, especially when we encounter new experiences, educators-as-researchers frequently disregard the accepted frameworks related to research and rely on their own subjective knowledge from their own pedagogical experiences when taking on the role of educator-researcher in educational settings. Relying on subjective knowledge enables teachers to engage more effectively as researchers in their educational context. Educator-researchers especially rely on this subjective knowledge in educational contexts to modify their data collection methodologies. Subjective knowledge negotiates the traditional research frameworks with the data collection possibilities of their practice, while also considering their unique educational context. This empowers educators as researchers, utilizing action research, to be powerful agents for change in educational contexts.
Thinking about Types of Data
Whether the research design is qualitative, quantitative or mixed-methods, it will determine the methods or ways you use to collect data. Qualitative research designs focus on collecting data that is relational, interpretive, subjective, and inductive; whereas a typical quantitative study, collects data that are deductive, statistical, and objective.
In contrast, qualitative data is often in the form of language, while quantitative data typically involves numbers. Quantitative researchers require large numbers of participants for validity, while qualitative researchers use a smaller number of participants, and can even use one (Hatch, 2002). In the past, quantitative and qualitative educational researchers rarely interacted, sometimes holding contempt for each other’s work; and even published articles in separate journals based on having distinct theoretical orientations in terms of data collection. Overall, there is a greater appreciation for both quantitative and qualitative approaches, with scholars finding distinct value in each approach, yet in many circles the debate continues over which approach is more beneficial for educational research and in educational contexts.
The goal of qualitative data collection is to build a complex and nuanced description of social or human problems from multiple perspectives. The flexibility and ability to use a variety of data collection techniques encompasses a distinct stance on research. Qualitative researchers are able to capture conversations and everyday language, as well as situational attitudes and beliefs. Qualitative data collection is able to be fitted to the study, with the goal of collecting the most authentic data, not necessarily the most objective. To researchers who strictly use quantitative methods, qualitative methods may seem wholly unstructured, eclectic, and idiosyncratic; however, for qualitative researchers these characteristics are advantageous to their purpose. Quantitative research depends upon structure and is bounded to find relationship among variables and units of measurement. Quantitative research helps make sense of large amounts of data. Both quantitative and qualitative research help us address education challenges by better identifying what is happening, with the goal of identifying why it is happening, and how we can address it.
Most educator-researchers who engage in research projects in schools and classrooms utilize qualitative methodologies for their data collection. Educator-researchers also use mixed methods that focus on qualitative methods, but also use quantitative methods, such as surveys, to provide a multidimensional approach to inquiring about their topic. While qualitative methods may feel more comfortable, there is a methodological rationale for using quantitative research.
Research methodologists use two distinct forms of logic to describe research: induction and deduction. Inductive approaches are focused on developing new or emerging theories, by explaining the accumulation of evidence that provides meaning to similar circumstances. Deductive approaches move in the opposite direction, and create meaning about a particular situation by reasoning from a general idea or theory about the particular circumstances. While qualitative approaches are inductive – observe and then generate theories, for example – qualitative researchers will typically initiate studies with some preconceived notions of potential theories to support their work.
Flexible Research Design
A researcher’s decisions about data collection and activities involve a personal choice, yet the choice of data sources must be responsive to the proposed project and topic. Logically, researchers will use whatever validated methods help them to address the issue they are researching and will develop a research plan around activities to implement those methods. While a research plan is important to conducting valid research in schools and classrooms, a research plan should also be flexible in design to allow data to emerge and find the best data to address research questions. In this way, a research plan is recommended, but data collection methods are not always known in advance. As you, the educator-researcher, interacts with participants, you may find it necessary to continue the research with additional data sources to better address the question at the center of your research. When educators are researchers and a participant in their study, it is especially important to keep an open mind to the wide range of research methodologies. All-in-all educator-researchers should understand that there are varied and multiple paths to move from research questions to addressing those questions.
Mixed Methods
As mentioned above, mixed methods is the use of both qualitative and quantitative methods. Researchers generally use mixed methods to clarify findings from the initial method of data collection. In mixed-methods research, the educator-researcher has increased flexibility in data collection. Mixed methods studies often result in a combination of precise measurements (e.g., grades, test scores, survey, etc.) along with in-depth qualitative data that provide meaningful detail to those measurements. The key advantage of using mixed methods is that quantitative details enhance qualitative data sources that involve conclusions and use terms such as usually, some, or most which can be substituted with a number or quantity, such as percentages or averages, or the mean, the median, and/or the mode. One challenge to educator-researchers is that mixed methods require more time and resources to complete the study, and more familiarity about both qualitative and quantitative data collection methods.
Mixed methods in educator research, even if quantitative methods are only used minimally, provide an opportunity to clarify findings, fill gaps in understanding, and cross-check data. For example, if you are looking at the use of math journals to better engage students and improve their math scores, it would be helpful to understand their abilities in math and reading before analyzing the math journals. Therefore, looking at their test scores might give you some nuanced understanding of why some students improved more than others after using the math journals. Pre- and post-surveys would also provide valuable information in terms of students’ attitudes and beliefs about math and writing. In line with thinking about pre- and post-surveys, some researchers suggest using either qualitative or quantitative approaches in different phases of the research process. In the previous example, pre- and post test scores may quantitatively demonstrate growth or improvement after implementing the math journal; however, the qualitative data would provide detailed evidence as to why the math journals contributed to growth or improvement in math. Quantitative methods can establish relationships among variables, while qualitative methods can explain factors underlying those same relationships.
I caution the reader at this point to not simply think of qualitative methodologies as anecdotal details to quantitative reports. I only highlight mixed methods to introduce the strength of such studies, and to aid in moving educational research methodology away from the binary thinking of quantitative vs. qualitative. In thinking about data collection, possible data sources include questionnaires or surveys, observations (video or written notes), collaboration (meetings, peer coaching), interviews, tests and records, pictures, diaries, transcripts of video and audio recordings, personal journals, student work samples, e-mail and online communication, and any other pertinent documents and reports. As you begin to think about data collection you will consider the available materials and think about aspects discussed in the previous chapter: who, what, where, when, and how. Specifically:
- Who are the subjects or participants for the study?
- What data is vital evidence for this study?
- Where will the data be collected?
- When will the data be collected?
- How will the data be collected?
If you find you are having trouble identifying data sources that support your initial question, you may need to revise your research question – and make sure what you are asking is researchable or measurable. The research question can always change throughout the study, but it should only be in relation the data being collected.
Participant Data
As an educator, your possible participants selection pool is narrower than most researchers encounter – however, it is important to be clear about their role in the data design and collection. A study can involve one participant or multiple participants, and participants often serve as the primary source of data in the research process. Most studies by educator-researchers utilize purposeful sampling, or in other words, they select participants who will be able to provide the most relevant information to the study. Therefore, the study design relies upon the participants and the information they can provide. The following is a description of some data collection methods, which include: surveys or questionnaires, individual or group interviews, observations, field notes or diaries, narratives, documents, and elicitation.
Surveys, or questionnaires, are a research instrument frequently used to receive data about participants’ feelings, beliefs, and attitudes in regard to the research topic or activities. Surveys are often used for large sample sizes with the intent of generalizing from a sample population to a larger population. Surveys are used with any number of participants and can be administered at different times during the study, such as pre-activity and post-activity, with the same participants to determine if changes have occurred over the course of the activity time, or simply change over time. Researchers like surveys and questionnaires as an instrument because they can be distributed and collected easily – especially with all of the recent online application possibilities (e.g., Google, Facebook, etc.). Surveys come in several forms, closed-ended, open-ended, or a mix of the two. Closed-ended surveys are typically multiple-choice questions or scales (e.g. 1-5, most likely–least likely) that allow participants to rate or select a response for each question. These responses can easily be tabulated into meaningful number representations, like percentages. For example, Likert scales are often used with a five-point range, with options such as strongly agree, agree, neutral, disagree, and strongly disagree. Open-ended surveys consist of prompts for participants to add their own perspectives in short answer or limited word responses. Open-ended surveys are not always as easy to tabulate, but can provide more detail and description.
Interviews and Focus Groups
Interviews are frequently used by researchers because they often produce some of the most worthwhile data. Interviews allow researchers to obtain candid verbal perspectives through structured or semi-structured questioning. Interview questions, either structured or semi-structured, are related to the research question or research activities to gauge the participants’ thoughts, feelings, motivations, and reflections. Some research relies on interviewing as the primary data source, but most often interviews are used to strengthen and support other data sources. Interviews can be time consuming, but interviews are worthwhile in that you can gather richer and more revealing information than other methods that could be utilized (Koshy, 2010). Lincoln and Guba (1985) identified five outcomes of interviewing:
Outcomes of Interviewing
- Here and now explanations;
- Reconstructions of past events and experiences;
- Projections of anticipated experiences;
- Verification of information from other sources;
- Verification of information (p. 268).
As mentioned above, interviews typically take two forms: structured and semi-structured. In terms of interviews, structured means that the researcher identifies a certain number of questions, in a prescribed sequence, and the researcher asks each participant these questions in the same order. Structured interviews qualitatively resemble surveys and questionnaires because they are consistent, easy to administer, provide direct responses, and make tabulation and analysis more consistent. Structured interviews use an interview protocol to organize questions, and maintain consistency.
Semi-structured interviews have a prescribed set of questions and protocol, just like structured interviews, but the researcher does not have to follow those questions or order explicitly. The researcher should ask the same questions to each participant for comparison reasons, but semi-structured interviews allow the researcher to ask follow-up questions that stray from the protocol. The semi-structured interview is intended to allow for new, emerging topics to be obtained from participants. Semi-structured questions can be included in more structured protocols, which allows for the participant to add additional information beyond the formal questions and for the researcher to return to preplanned formal questions after the participant responds. Participants can be interviewed individually or collectively, and while individual interviews are time-consuming, they can provide more in-depth information.
When considering more than two participants for an interview, researchers will often use a focus group interview format. Focus group interviews typically involve three to ten participants and seek to gain socially dependent perspectives or organizational viewpoints. When using focus group interviews with students, researchers often find them beneficial because they allow student reflection and ideas to build off of each other. This is important because often times students feel shy or hesitant to share their ideas with adults, but once another student sparks or confirms their idea, belief, or opinion they are more willing to share. Focus group interviews are very effective as pre- and post-activity data sources. Researchers can use either a structured or semi-structured interview protocol for focus group interviews; however, with multiple participants it may be difficult to maintain the integrity of a structured protocol.
Observations
One of the simplest, and most natural, forms of data collection is to engage in formal observation. Observing humans in a setting provides us contextual understanding of the complexity of human behavior and interrelationships among groups in that setting. If a researcher wants to examine the ways teachers approach a particular area of pedagogical practice, then observation would be a viable data collection tool. Formal observations are truly unique and allow the researcher to collect data that cannot be obtained through other data sources. Ethnography is a qualitative research design that provides a descriptive account based on researchers’ observations and explorations to examine the social dynamics present in cultures and social systems – which includes classrooms and schools. Taken from anthropology, the ethnographer uses observations and detailed note taking, along with other forms of mapping or making sense of the context and relationships within. For Creswell (2007), several guidelines provide structure to an observation:
Structuring Observations
- Identify what to observe
- Determine the role you will assume — observer or participant
- Design observational protocol for recording notes
- Record information such as physical situation, particular events and activities
- Thank participants and inform them of the use of and their accessibility to the data (pp. 132– 134)
As an educator-researcher, you may take on a role that exceeds that of an observer and participate as a member of the research setting. In this case, the data sources would be called participant observation to clearly identify the degree of involvement you have in the study. In participant observation, the researcher embeds themselves in the actions of the participants. It is important to understand that participant observation will provide completely different data, in comparison to simply observing someone else. Ethnographies, or studies focused completely on observation as a data source, often extend longer than other data sources, ranging from several months to even years. Extended time provides the researcher the ability to obtain more detailed and accurate information, because it takes time to observe patterns and other details that are significant to the study. Self-study is another consideration for educators, if they want to use observation and be a participant observer. They can use video and audio recordings of their activities to use as data sources and use those as the source of observation.
Field Diaries and Notes
Utilizing a field dairy, or keeping field notes, can be a very effective and practical data collection method. In purpose, a field diary or notes keep a record of what happens during the research activities. It can be useful in tracking how and why your ideas and the research process evolved. Many educators keep daily notes about their classes, and in many ways, this is a more focused and narrower version of documenting the daily happenings of a class. A field diary or notes can also serve as an account of your reflections and commentary on your study, and can be a starting place for your data analysis and interpretations. A field diary or notes are typically valuable when researchers begin to write about their project because it allows them to draw upon their authentic voice. The reflective process that represents a diary can also serve as an additional layer of professional learning for researchers. The format and length of a field diary or notes will vary depending on the researching and the topic; however, the ultimate goal should be to facilitate data collection and analysis.
Data narratives and stories are a fairly new form of formalized data. While researchers have collected bits and pieces of narratives in other forms of data, asking participants to compose a narrative (either written, spoken, or performed) as a whole allows researchers to examine how participants embrace the complexities of the context and social interactions. Humans are programmed to engage with and share narratives to develop meaningful and experiential knowledge. Educator autobiographies bring to life personal stories shaped by knowledge, values, and feelings that developed from their classroom experiences. Narrative data includes three primary areas: temporality, sociality, and place (Clandinin & Conolley, 2000). In terms of temporality, narratives have a past, present, and future because stories are time-based and transitional. Sociality highlights the social relationships in narratives as well as the personal and moral dispositions. Place includes the spaces where the narratives happen. Furthermore, bell hooks (1991) notes that narratives, or storytelling, as inquiry can be a powerful way to study how contexts are influenced by power structures, often linking and intersecting the structural dynamics of social class, race, and gender to highlight the struggle.
Documents provide a way to collect data that is unobtrusive to the participant. Documents are unobtrusive data because it is collected without modifying or distracting the research context when gathered. Educational settings maintain records on all sorts of activities in schools: content standards, state mandates, student discipline records, student attendance, student assessments, performance records, parental engagement, records of how teachers spend PTO money, etc. Documents often provide background and contextual material providing a snapshot of school policies, demographic information, ongoing records over a period of time, and contextual details from the site of the research study. Documents can be characterized similarly to historical research, as primary and secondary. Examples of primary materials are first-hand sources from someone in the educational context, such as minutes from a school board or faculty meeting, photographs, video recordings, and letters. Examples of secondary sources typically include analysis or interpretations of a primary source by others, such as texts, critiques, and reviews. Both types of sources are especially valuable in action research.
Elicitation Methods
We have talked about several methods of data collection that each have useful ways of documenting, inquiring, and thinking about the research question. However, how does a researcher engage participants in ways that allow them to demonstrate what they know, feel, think, or believe? Asking participants directly about their thinking, feeling, or beliefs will only take you so far depending on the comfort and rapport the participant has with the researcher. There are always a variety of hurdles in extracting participants’ knowledge. Even the manner in which questions are framed and the way researchers use materials in the research process are equally important in getting participants to provide reliable, comparable, and valid responses. Furthermore, all individuals who participate in research studies vary in their ability to recall and report what they know, and this affects the value of traditional data collection, especially structured and semi-structured interviewing. In particular, participants’ knowledge or other thinking of interest may be implicit and difficult for them to explicate in simple discussion.
Elicitation methods help researchers uncover unarticulated participant knowledge through a potential variety of activities. Researchers will employ elicitation methods and document the participants’ actions and typically the description of why they took those particular actions. Educators may be able to relate the process of elicitation methods to a “think aloud” activity in which the researcher wants to record or document the activity. Elicitation methods can take many forms. What follows are some basic ideas and formats for elicitation methods.
Brainstorming/Concept Map
Most educators are probably familiar with the process of brainstorming or creating a concept map. These can be very effective elicitation methods when the researcher asks the participant to create a concept map or representation of brainstorming, and then asks the participant to explain the connections between concepts or ideas on the brainstorming or concept map.
Sorting provides an engaging way to gather data from your participants. Sorting, as you can imagine, involves participants sorting, grouping, or categorizing objects or photographs in meaningful ways. Once participants have sorted the objects or photographs, the researcher records or documents the participant explaining why they sorted or grouped the objects or photographs in the way that they did. As a former history teacher, I would often use sorting to assess my students’ understanding of related concepts and events in a world history class. I would use pictures too as the means for students to sort and demonstrate what they understood from the unit. For broader discussion of elicitation techniques in history education see Barton (2015).
Listing/ Ranking
Listing can be an effective way to examine participants’ thinking about a topic. Researchers can have participants construct a list in many different ways to fit the focus of the study and then have the participants explain their list. For example, if an educator was studying middle school student perceptions of careers, they could ask them to complete three lists: Careers in Most Demand; Careers with Most Education/Training; Careers of most Interest.
Then, once participants have filled out the lists, the most important part is documenting them explaining their thinking, and why they filled out the lists the way they did. As you may imagine, in this example, every participant would have a list that is different based on their personal interests.
Researchers can also elicit responses by simply giving participants a prompt, and then asking them to recall whatever they know about that prompt. Researchers will have the participants do this in some sort of demonstrative activity. For example, at the end of a world history course, I might ask students to explain what “culture” means to them and to explain their thinking.
Re-articulation (writing or drawing)
A unique way to engage participants in elicitation methods is to have them write about, rewrite, or draw visual representations of either life experiences or literature that they have read. For example, you could ask them to rewrite a part of the literature they did not like, add a part they thought should be there, or simply extend the ending. Participants can either write or draw these re-articulations. I find that drawing works just as well because, again, the goal is to have participant describe their thinking based on the activity.
Scenario Decision-Making
Elicitation methods can also examine skills. Researchers can provide participants scenarios and ask them to make decisions. The researchers can document those decisions and analyze the extent to which the participant understands the skill.
Document, Photograph, or Video Analysis
This is the most basic elicitation in which the researcher provides a document, photograph, or video for the participant to examine. Then, the researcher asks questions about the participants interpretations of the document, photograph, or video. One method that would support this sort of elicitation is to ask the participants to provide images from their everyday words. For example, asking students to document the literacy examples in their homes (i.e., pictures of calendars, bookshelves etc.). With the availability of one-to-one tech, and iPads, participant documentation is easier.
There are many more methods of data collection also, as well as many variations of the methods described above. The goal for you is to find the data collection methods that are going to give you the best data to answer your research question. If you are unsure, there is nothing wrong with collecting more data than you need to make sure you use effective methods – the only thing you have to lose is time!
Use of Case Studies
Case studies are a popular way for studying phenomena in settings using qualitative methodology. Case studies typically encompass qualitative studies which look closely at what happens when researchers collect data, analyze the data, and present the results. Case studies can focus on a single case or examine a phenomenon across multiple cases. Case studies frame research in a way that allows for rich description of data and depth of analysis.
An advantage of using case study design is that the reader often identifies with the case or phenomena, as well as the participants in the study. Yin (2003) describes case study methodology as inquiry that investigates a contemporary phenomenon within its authentic context. Case studies are particularly appropriate when the boundaries and relationship between the phenomenon and the context are not clear. Case studies relate well with the processes involved in action research. Critics of action research case studies sometimes criticize the inevitable subjectivity, just like general criticisms of action research. Case studies provide researchers opportunities to explore both the how and the why of phenomena in context, while being both exploratory and descriptive.
We want to clarify the differences between methodologies and methods of research. There are methodologies of research, like case study and action research, and methods of data collection. Methodologies like ethnography, narrative inquiry, and case study draw from some similar methods of data collecting that include interviews, collection of artifacts (writings, drawings, images), and observations. The differences between the methodologies include the time-frame for research; the boundaries of the research; and the epistemology.
Triangulation of Data
Triangulation is a method used by qualitative researchers to check and establish trustworthiness in their studies by using and analyzing multiple (three or more) data collection methods to address a research question and develop a consistency of evidence from data sources or approaches. Thus, triangulation facilitates trustworthiness of data through cross verification of evidence, to support claims, from more than two data collection sources. Triangulation also tests the consistency of findings obtained through different data sources and instruments, while minimizing bias in the researcher’s interpretations of the data.
If we think about the example of studying the use of math journals in an elementary classroom, the researcher would want to collect at least three sources of data – the journal prompts, assessment scores, and interviews. When the researcher is analyzing the data, they will want to find themes or evidence across all three data sources to address their research question. In a very basic analysis, if the students demonstrated a deeper level of reflection about math in the journals, their assessment scores improved, and their interviews demonstrated they had more confidence in their number sense and math abilities – then, the researcher could conclude, on a very general level, that math journals improved their students’ math skills, confidence, or abilities. Ideally, the study would examine specific aspects of math to enable deeper analysis of math journals, but this example demonstrates the basic idea of triangulation. In this example, all of the data provided evidence that the intervention of a math journal improved students’ understanding of math, and the three data sources provided trustworthiness for this claim.
Data Collection Checklist
- Based on your research question, what data might you need ?
- What are the multiple ways you could collect that data ?
- How might you document this data , or organize it so that it can be analyzed?
- What methods are most appropriate for your context and timeframe ?
- How much time will your data collection require? How much time can you allow for?
- Will you need to create any data sources (e.g., interview protocol, elicitation materials)?
- Do your data sources all logically support the research question, and each other?
- Does your data collection provide for multiple perspectives ?
- How will your data achieve triangulation in addressing the research question?
- Will you need more than three data sources to ensure triangulation of data?
Action Research Copyright © by J. Spencer Clark; Suzanne Porath; Julie Thiele; and Morgan Jobe is licensed under a Creative Commons Attribution-NonCommercial 4.0 International License , except where otherwise noted.
Share This Book
Qualitative vs. Quantitative Research: Comparing the Methods and Strategies for Education Research
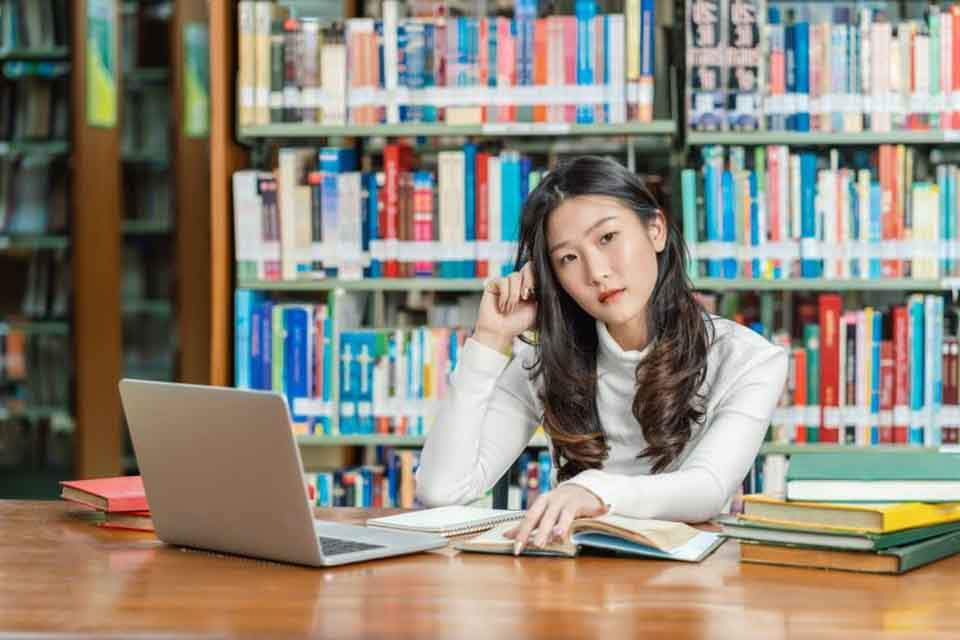
No matter the field of study, all research can be divided into two distinct methodologies: qualitative and quantitative research. Both methodologies offer education researchers important insights.
Education research assesses problems in policy, practices, and curriculum design, and it helps administrators identify solutions. Researchers can conduct small-scale studies to learn more about topics related to instruction or larger-scale ones to gain insight into school systems and investigate how to improve student outcomes.
Education research often relies on the quantitative methodology. Quantitative research in education provides numerical data that can prove or disprove a theory, and administrators can easily share the number-based results with other schools and districts. And while the research may speak to a relatively small sample size, educators and researchers can scale the results from quantifiable data to predict outcomes in larger student populations and groups.
Qualitative vs. Quantitative Research in Education: Definitions
Although there are many overlaps in the objectives of qualitative and quantitative research in education, researchers must understand the fundamental functions of each methodology in order to design and carry out an impactful research study. In addition, they must understand the differences that set qualitative and quantitative research apart in order to determine which methodology is better suited to specific education research topics.
Generate Hypotheses with Qualitative Research
Qualitative research focuses on thoughts, concepts, or experiences. The data collected often comes in narrative form and concentrates on unearthing insights that can lead to testable hypotheses. Educators use qualitative research in a study’s exploratory stages to uncover patterns or new angles.
Form Strong Conclusions with Quantitative Research
Quantitative research in education and other fields of inquiry is expressed in numbers and measurements. This type of research aims to find data to confirm or test a hypothesis.
Differences in Data Collection Methods
Keeping in mind the main distinction in qualitative vs. quantitative research—gathering descriptive information as opposed to numerical data—it stands to reason that there are different ways to acquire data for each research methodology. While certain approaches do overlap, the way researchers apply these collection techniques depends on their goal.
Interviews, for example, are common in both modes of research. An interview with students that features open-ended questions intended to reveal ideas and beliefs around attendance will provide qualitative data. This data may reveal a problem among students, such as a lack of access to transportation, that schools can help address.
An interview can also include questions posed to receive numerical answers. A case in point: how many days a week do students have trouble getting to school, and of those days, how often is a transportation-related issue the cause? In this example, qualitative and quantitative methodologies can lead to similar conclusions, but the research will differ in intent, design, and form.
Taking a look at behavioral observation, another common method used for both qualitative and quantitative research, qualitative data may consider a variety of factors, such as facial expressions, verbal responses, and body language.
On the other hand, a quantitative approach will create a coding scheme for certain predetermined behaviors and observe these in a quantifiable manner.
Qualitative Research Methods
- Case Studies : Researchers conduct in-depth investigations into an individual, group, event, or community, typically gathering data through observation and interviews.
- Focus Groups : A moderator (or researcher) guides conversation around a specific topic among a group of participants.
- Ethnography : Researchers interact with and observe a specific societal or ethnic group in their real-life environment.
- Interviews : Researchers ask participants questions to learn about their perspectives on a particular subject.
Quantitative Research Methods
- Questionnaires and Surveys : Participants receive a list of questions, either closed-ended or multiple choice, which are directed around a particular topic.
- Experiments : Researchers control and test variables to demonstrate cause-and-effect relationships.
- Observations : Researchers look at quantifiable patterns and behavior.
- Structured Interviews : Using a predetermined structure, researchers ask participants a fixed set of questions to acquire numerical data.
Choosing a Research Strategy
When choosing which research strategy to employ for a project or study, a number of considerations apply. One key piece of information to help determine whether to use a qualitative vs. quantitative research method is which phase of development the study is in.
For example, if a project is in its early stages and requires more research to find a testable hypothesis, qualitative research methods might prove most helpful. On the other hand, if the research team has already established a hypothesis or theory, quantitative research methods will provide data that can validate the theory or refine it for further testing.
It’s also important to understand a project’s research goals. For instance, do researchers aim to produce findings that reveal how to best encourage student engagement in math? Or is the goal to determine how many students are passing geometry? These two scenarios require distinct sets of data, which will determine the best methodology to employ.
In some situations, studies will benefit from a mixed-methods approach. Using the goals in the above example, one set of data could find the percentage of students passing geometry, which would be quantitative. The research team could also lead a focus group with the students achieving success to discuss which techniques and teaching practices they find most helpful, which would produce qualitative data.
Learn How to Put Education Research into Action
Those with an interest in learning how to harness research to develop innovative ideas to improve education systems may want to consider pursuing a doctoral degree. American University’s School of Education Online offers a Doctor of Education (EdD) in Education Policy and Leadership that prepares future educators, school administrators, and other education professionals to become leaders who effect positive changes in schools. Courses such as Applied Research Methods I: Enacting Critical Research provides students with the techniques and research skills needed to begin conducting research exploring new ways to enhance education. Learn more about American’ University’s EdD in Education Policy and Leadership .
What’s the Difference Between Educational Equity and Equality?
EdD vs. PhD in Education: Requirements, Career Outlook, and Salary
Top Education Technology Jobs for Doctorate in Education Graduates
American University, EdD in Education Policy and Leadership
Edutopia, “2019 Education Research Highlights”
Formplus, “Qualitative vs. Quantitative Data: 15 Key Differences and Similarities”
iMotion, “Qualitative vs. Quantitative Research: What Is What?”
Scribbr, “Qualitative vs. Quantitative Research”
Simply Psychology, “What’s the Difference Between Quantitative and Qualitative Research?”
Typeform, “A Simple Guide to Qualitative and Quantitative Research”
Request Information
Have a language expert improve your writing
Run a free plagiarism check in 10 minutes, generate accurate citations for free.
- Knowledge Base
Methodology
- What Is Quantitative Research? | Definition, Uses & Methods
What Is Quantitative Research? | Definition, Uses & Methods
Published on June 12, 2020 by Pritha Bhandari . Revised on June 22, 2023.
Quantitative research is the process of collecting and analyzing numerical data. It can be used to find patterns and averages, make predictions, test causal relationships, and generalize results to wider populations.
Quantitative research is the opposite of qualitative research , which involves collecting and analyzing non-numerical data (e.g., text, video, or audio).
Quantitative research is widely used in the natural and social sciences: biology, chemistry, psychology, economics, sociology, marketing, etc.
- What is the demographic makeup of Singapore in 2020?
- How has the average temperature changed globally over the last century?
- Does environmental pollution affect the prevalence of honey bees?
- Does working from home increase productivity for people with long commutes?
Table of contents
Quantitative research methods, quantitative data analysis, advantages of quantitative research, disadvantages of quantitative research, other interesting articles, frequently asked questions about quantitative research.
You can use quantitative research methods for descriptive, correlational or experimental research.
- In descriptive research , you simply seek an overall summary of your study variables.
- In correlational research , you investigate relationships between your study variables.
- In experimental research , you systematically examine whether there is a cause-and-effect relationship between variables.
Correlational and experimental research can both be used to formally test hypotheses , or predictions, using statistics. The results may be generalized to broader populations based on the sampling method used.
To collect quantitative data, you will often need to use operational definitions that translate abstract concepts (e.g., mood) into observable and quantifiable measures (e.g., self-ratings of feelings and energy levels).
Note that quantitative research is at risk for certain research biases , including information bias , omitted variable bias , sampling bias , or selection bias . Be sure that you’re aware of potential biases as you collect and analyze your data to prevent them from impacting your work too much.
Receive feedback on language, structure, and formatting
Professional editors proofread and edit your paper by focusing on:
- Academic style
- Vague sentences
- Style consistency
See an example
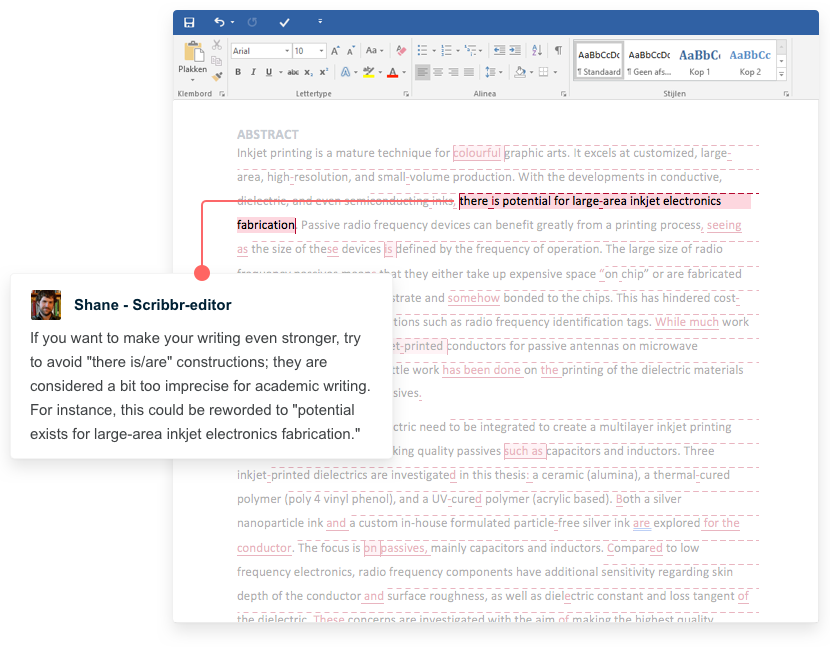
Once data is collected, you may need to process it before it can be analyzed. For example, survey and test data may need to be transformed from words to numbers. Then, you can use statistical analysis to answer your research questions .
Descriptive statistics will give you a summary of your data and include measures of averages and variability. You can also use graphs, scatter plots and frequency tables to visualize your data and check for any trends or outliers.
Using inferential statistics , you can make predictions or generalizations based on your data. You can test your hypothesis or use your sample data to estimate the population parameter .
First, you use descriptive statistics to get a summary of the data. You find the mean (average) and the mode (most frequent rating) of procrastination of the two groups, and plot the data to see if there are any outliers.
You can also assess the reliability and validity of your data collection methods to indicate how consistently and accurately your methods actually measured what you wanted them to.
Quantitative research is often used to standardize data collection and generalize findings . Strengths of this approach include:
- Replication
Repeating the study is possible because of standardized data collection protocols and tangible definitions of abstract concepts.
- Direct comparisons of results
The study can be reproduced in other cultural settings, times or with different groups of participants. Results can be compared statistically.
- Large samples
Data from large samples can be processed and analyzed using reliable and consistent procedures through quantitative data analysis.
- Hypothesis testing
Using formalized and established hypothesis testing procedures means that you have to carefully consider and report your research variables, predictions, data collection and testing methods before coming to a conclusion.
Despite the benefits of quantitative research, it is sometimes inadequate in explaining complex research topics. Its limitations include:
- Superficiality
Using precise and restrictive operational definitions may inadequately represent complex concepts. For example, the concept of mood may be represented with just a number in quantitative research, but explained with elaboration in qualitative research.
- Narrow focus
Predetermined variables and measurement procedures can mean that you ignore other relevant observations.
- Structural bias
Despite standardized procedures, structural biases can still affect quantitative research. Missing data , imprecise measurements or inappropriate sampling methods are biases that can lead to the wrong conclusions.
- Lack of context
Quantitative research often uses unnatural settings like laboratories or fails to consider historical and cultural contexts that may affect data collection and results.
Here's why students love Scribbr's proofreading services
Discover proofreading & editing
If you want to know more about statistics , methodology , or research bias , make sure to check out some of our other articles with explanations and examples.
- Chi square goodness of fit test
- Degrees of freedom
- Null hypothesis
- Discourse analysis
- Control groups
- Mixed methods research
- Non-probability sampling
- Inclusion and exclusion criteria
Research bias
- Rosenthal effect
- Implicit bias
- Cognitive bias
- Selection bias
- Negativity bias
- Status quo bias
Quantitative research deals with numbers and statistics, while qualitative research deals with words and meanings.
Quantitative methods allow you to systematically measure variables and test hypotheses . Qualitative methods allow you to explore concepts and experiences in more detail.
In mixed methods research , you use both qualitative and quantitative data collection and analysis methods to answer your research question .
Data collection is the systematic process by which observations or measurements are gathered in research. It is used in many different contexts by academics, governments, businesses, and other organizations.
Operationalization means turning abstract conceptual ideas into measurable observations.
For example, the concept of social anxiety isn’t directly observable, but it can be operationally defined in terms of self-rating scores, behavioral avoidance of crowded places, or physical anxiety symptoms in social situations.
Before collecting data , it’s important to consider how you will operationalize the variables that you want to measure.
Reliability and validity are both about how well a method measures something:
- Reliability refers to the consistency of a measure (whether the results can be reproduced under the same conditions).
- Validity refers to the accuracy of a measure (whether the results really do represent what they are supposed to measure).
If you are doing experimental research, you also have to consider the internal and external validity of your experiment.
Hypothesis testing is a formal procedure for investigating our ideas about the world using statistics. It is used by scientists to test specific predictions, called hypotheses , by calculating how likely it is that a pattern or relationship between variables could have arisen by chance.
Cite this Scribbr article
If you want to cite this source, you can copy and paste the citation or click the “Cite this Scribbr article” button to automatically add the citation to our free Citation Generator.
Bhandari, P. (2023, June 22). What Is Quantitative Research? | Definition, Uses & Methods. Scribbr. Retrieved March 27, 2024, from https://www.scribbr.com/methodology/quantitative-research/
Is this article helpful?
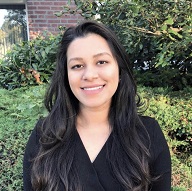
Pritha Bhandari
Other students also liked, descriptive statistics | definitions, types, examples, inferential statistics | an easy introduction & examples, unlimited academic ai-proofreading.
✔ Document error-free in 5minutes ✔ Unlimited document corrections ✔ Specialized in correcting academic texts
- Technical Support
- Find My Rep
You are here
Understanding Quantitative Data in Educational Research
- Nicoleta Gaciu - Oxford Brookes University, UK
- Description
This book is designed to help Education students gain confidence in analysing and interpreting quantitative data and using appropriate statistical tests, by exploring, in plain language, a variety of data analysis methods.
Highly practical, each chapter includes step-by-step instructions on how to run specific statistical tests using R, practical tips on how to interpret results correctly and exercises to put into practice what students have learned.
It also includes guidance on how to use R and RStudio, how to visualise quantitative data, and the fundamentals of inferential statistics, estimations and hypothesis testing.
Nicoleta Gaciu is Senior Lecturer in Education at Oxford Brookes University.
See what’s new to this edition by selecting the Features tab on this page. Should you need additional information or have questions regarding the HEOA information provided for this title, including what is new to this edition, please email [email protected] . Please include your name, contact information, and the name of the title for which you would like more information. For information on the HEOA, please go to http://ed.gov/policy/highered/leg/hea08/index.html .
For assistance with your order: Please email us at [email protected] or connect with your SAGE representative.
SAGE 2455 Teller Road Thousand Oaks, CA 91320 www.sagepub.com
Supplements
Preview this book, for instructors, select a purchasing option, related products.

- Subject List
- Take a Tour
- For Authors
- Subscriber Services
- Publications
- African American Studies
- African Studies
- American Literature
- Anthropology
- Architecture Planning and Preservation
- Art History
- Atlantic History
- Biblical Studies
- British and Irish Literature
- Childhood Studies
- Chinese Studies
- Cinema and Media Studies
- Communication
- Criminology
- Environmental Science
- Evolutionary Biology
- International Law
- International Relations
- Islamic Studies
- Jewish Studies
- Latin American Studies
- Latino Studies
- Linguistics
- Literary and Critical Theory
- Medieval Studies
- Military History
- Political Science
- Public Health
- Renaissance and Reformation
- Social Work
- Urban Studies
- Victorian Literature
- Browse All Subjects
How to Subscribe
- Free Trials
In This Article Expand or collapse the "in this article" section Data Collection in Educational Research
Introduction, general overviews.
- General Quantitative Overviews
- Questionnaires
- Quantitative Interviewing
- Quantitative Observation
- Technical Properties
- General Qualitative Overviews
- In-Depth Interviewing
- Focus Groups
- Qualitative Observation
- Qualitative Document Analysis
- Visual Analysis
Related Articles Expand or collapse the "related articles" section about
About related articles close popup.
Lorem Ipsum Sit Dolor Amet
Vestibulum ante ipsum primis in faucibus orci luctus et ultrices posuere cubilia Curae; Aliquam ligula odio, euismod ut aliquam et, vestibulum nec risus. Nulla viverra, arcu et iaculis consequat, justo diam ornare tellus, semper ultrices tellus nunc eu tellus.
- Case Study in Education Research
- Grounded Theory
- Methodologies for Conducting Education Research
- Mixed Methods Research
- Qualitative Research Design
- Statistical Assumptions
- Using Ethnography in Educational Research
Other Subject Areas
Forthcoming articles expand or collapse the "forthcoming articles" section.
- Gender, Power, and Politics in the Academy
- Girls' Education in the Developing World
- Non-Formal & Informal Environmental Education
- Find more forthcoming articles...
- Export Citations
- Share This Facebook LinkedIn Twitter
Data Collection in Educational Research by James H. McMillan , Laura P. Gogia LAST REVIEWED: 05 August 2020 LAST MODIFIED: 30 June 2014 DOI: 10.1093/obo/9780199756810-0087
Data collection methods in educational research are used to gather information that is then analyzed and interpreted. As such, data collection is a very important step in conducting research and can influence results significantly. Once the research question and sources of data are identified, appropriate methods of data collection are determined. Data collection includes a broad range of more specific techniques. Historically, much of the data collection performed in educational research depended on methods developed for studies in the field of psychology, a discipline which took what is termed a “quantitative” approach. This involves using instruments, scales, Tests , and structured observation and interviewing. By the mid- to late twentieth centuries, other disciplines such as anthropology and sociology began to influence educational researchers. Forms of data collection broadened to include what is now called “qualitative” methods, with an emphasis on narratives, participant perspectives, and less structured observation and interviewing. As contemporary educational researchers also draw from fields such as business, political science, and medicine, data collection in education has become a multidisciplinary phenomenon. Because data collection is such a broad topic, General Overviews that attempt to cover all or most techniques tend to offer introductory treatments. Few texts, however, provide comprehensive coverage of every data collection technique. Instead, some cover techniques appropriate for either quantitative or qualitative research approaches. Even more focus on one or two data collection methods within those two research contexts. Consequently, after presenting general overviews, this entry is categorized by data collection appropriate for quantitative and Qualitative Data Collection . These sections, in turn, are subdivided into the major types of quantitative and qualitative data collection techniques. While there are some data collection techniques specific to mixed method research design, which implies a combination of qualitative and quantitative research methodologies, these specific procedures are not emphasized in the present article—readers are referred to the Oxford Bibliography article Mixed Methods Research by Nancy Leech for a comprehensive treatment of mixed method data collection techniques. To locate sources for this article, extensive searches were performed using general-use Internet search engines and educational, psychological, and social science research databases. These searches included keywords around data collection and research methods, as well as specific data collection techniques such as surveys, Tests , Focus Groups , and observation. Frequently cited texts and articles, most recent editions at the time, and sources specific to educational research were given priority. Once these sources were identified, their suggested readings and reference lists were mined for other potential sources. Works or scholars found in multiple reference lists were investigated. When applicable, book reviews in peer-reviewed journals were located and taken into account when curating sources. Sources that demonstrated a high level of impact or offered unique coverage of the topic were included.
General educational research overviews typically include several chapters on data collection, organized into qualitative and quantitative approaches. As a rule they are updated frequently so that they offer timely discussions of methodological trends. Most of them are introductory in nature, written for student researchers. Because of the influence of psychology and other social sciences on the development of data collection in educational research, representative works of psychology ( Trochim 2006 ) and of general social sciences ( Robson 2011 ) are included. Available online, Trochim 2006 is a reader-friendly introduction that provides succinct explanations of most quantitative and qualitative approaches. Olsen 2012 is helpful in showing how data collection techniques used in other disciplines have implications for educational studies. Specific to education, Gall, et al. 2007 is a frequently cited text that contains most educational data collection techniques, although it tends to emphasize more traditional quantitative approaches. Johnson and Christensen 2014 offers a more balanced treatment meant for novice researchers and educational research consumers. Cohen, et al. 2011 also provides a balanced approach, but from a British perspective. Fielding, et al. 2008 offer practical advice on recently developed forms of online data collection, with special attention given to the ethical ramifications of Internet-based data collection. Finally, Arthur, et al. 2012 is unique in this section in that it is an edited work offering short overviews of data collection techniques authored by contemporary leading experts.
Arthur, James, Michael Waring, Robert Coe, and Larry Hedges, eds. 2012. Research methods and methodologies in education . London: SAGE.
A diverse edited text discussing trends in study designs, data collection, and data analysis. It includes twelve chapters devoted to different forms of data collection, written by authors who have recently published extensively on the topic. Annotated bibliographies found at the end of each chapter provide guidance for further reading.
Cohen, Louis, Lawrence Manion, and Keith Morrison. 2011. Research methods in education . 7th ed. London: Routledge.
This long-running, bestselling, comprehensive source offers practical advice with clear theoretical foundations. The newest edition has undergone significant revision. Specific to data collection, revisions include new chapters devoted to data collection via the Internet and visual media. Slides highlighting main points are available on a supplementary website.
Fielding, Nigel, Raymond Lee, and Grant Blank. 2008. The SAGE handbook of online research methods . Thousand Oaks, CA: SAGE.
This extensive handbook presents chapters on Internet research design and data collection written by leading scholars in the field. It discusses using the Internet as an archival resource and a research tool, focusing on the most recent trends in multidisciplinary Internet research.
Gall, Meredith, Joyce Gall, and Walter Borg. 2007. Educational research: An introduction . 8th ed. White Plains, NY: Pearson.
A long-standing, well-respected, nuts-and-bolts perspective on data collection meant to prepare students for conducting original research. Although it tends to emphasize quantitative research methodologies, it has a uniquely rich chapter on historical document analysis.
Johnson, Burke, and Larry Christensen. 2014. Educational research: Quantitative, qualitative, and mixed approaches . 5th ed. Thousand Oaks, CA: SAGE.
A comprehensive introductory text for the consumer and the would-be researcher, with extensive lists of additional resources for gathering all types of data. It discusses quantitative and qualitative research methodologies and data collection evenly but provides extended coverage of questionnaire construction.
Olsen, Wendy. 2012. Data collection: Key debates and methods in social research . London: SAGE.
This recently published toolkit of quantitative, qualitative, and mixed method approaches to data collection provides a more contemporary introduction for both students and research professionals. It offers a helpful overview of data collection as an integral part of research in several different fields of study.
Robson, Colin. 2011. Real world research: A resource for users of social research methods in applied settings . West Sussex, UK: Wiley
This introductory text is intended for all social science. There is an applied, integrated emphasis on contemporary quantitative and qualitative data collection techniques in a separate section of the book, including individual and focus group observations, surveys, unstructured and structured interviewing, and tests.
Trochim, William. 2006. Research methods knowledge base
A free online hypertext textbook on applied social research methods. Data collection techniques associated with qualitative and quantitative research are covered comprehensively. Foundational information appropriate for undergraduates and early graduate students is presented through a series of easy-to-navigate and intuitively ordered webpages. Printed editions are available for purchase in an edition written with James Donnelly (Atomic Dog/Cengage Learning, 2008).
back to top
Users without a subscription are not able to see the full content on this page. Please subscribe or login .
Oxford Bibliographies Online is available by subscription and perpetual access to institutions. For more information or to contact an Oxford Sales Representative click here .
- About Education »
- Meet the Editorial Board »
- Academic Achievement
- Academic Audit for Universities
- Academic Freedom and Tenure in the United States
- Action Research in Education
- Adjuncts in Higher Education in the United States
- Administrator Preparation
- Adolescence
- Advanced Placement and International Baccalaureate Courses
- Advocacy and Activism in Early Childhood
- African American Racial Identity and Learning
- Alaska Native Education
- Alternative Certification Programs for Educators
- Alternative Schools
- American Indian Education
- Animals in Environmental Education
- Art Education
- Artificial Intelligence and Learning
- Assessing School Leader Effectiveness
- Assessment, Behavioral
- Assessment, Educational
- Assessment in Early Childhood Education
- Assistive Technology
- Augmented Reality in Education
- Beginning-Teacher Induction
- Bilingual Education and Bilingualism
- Black Undergraduate Women: Critical Race and Gender Perspe...
- Blended Learning
- Changing Professional and Academic Identities
- Character Education
- Children’s and Young Adult Literature
- Children's Beliefs about Intelligence
- Children's Rights in Early Childhood Education
- Citizenship Education
- Civic and Social Engagement of Higher Education
- Classroom Learning Environments: Assessing and Investigati...
- Classroom Management
- Coherent Instructional Systems at the School and School Sy...
- College Admissions in the United States
- College Athletics in the United States
- Community Relations
- Comparative Education
- Computer-Assisted Language Learning
- Computer-Based Testing
- Conceptualizing, Measuring, and Evaluating Improvement Net...
- Continuous Improvement and "High Leverage" Educational Pro...
- Counseling in Schools
- Critical Approaches to Gender in Higher Education
- Critical Perspectives on Educational Innovation and Improv...
- Critical Race Theory
- Crossborder and Transnational Higher Education
- Cross-National Research on Continuous Improvement
- Cross-Sector Research on Continuous Learning and Improveme...
- Cultural Diversity in Early Childhood Education
- Culturally Responsive Leadership
- Culturally Responsive Pedagogies
- Culturally Responsive Teacher Education in the United Stat...
- Curriculum Design
- Data Collection in Educational Research
- Data-driven Decision Making in the United States
- Deaf Education
- Desegregation and Integration
- Design Thinking and the Learning Sciences: Theoretical, Pr...
- Development, Moral
- Dialogic Pedagogy
- Digital Age Teacher, The
- Digital Citizenship
- Digital Divides
- Disabilities
- Distance Learning
- Distributed Leadership
- Doctoral Education and Training
- Early Childhood Education and Care (ECEC) in Denmark
- Early Childhood Education and Development in Mexico
- Early Childhood Education in Aotearoa New Zealand
- Early Childhood Education in Australia
- Early Childhood Education in China
- Early Childhood Education in Europe
- Early Childhood Education in Sub-Saharan Africa
- Early Childhood Education in Sweden
- Early Childhood Education Pedagogy
- Early Childhood Education Policy
- Early Childhood Education, The Arts in
- Early Childhood Mathematics
- Early Childhood Science
- Early Childhood Teacher Education
- Early Childhood Teachers in Aotearoa New Zealand
- Early Years Professionalism and Professionalization Polici...
- Economics of Education
- Education For Children with Autism
- Education for Sustainable Development
- Education Leadership, Empirical Perspectives in
- Education of Native Hawaiian Students
- Education Reform and School Change
- Educational Statistics for Longitudinal Research
- Educator Partnerships with Parents and Families with a Foc...
- Emotional and Affective Issues in Environmental and Sustai...
- Emotional and Behavioral Disorders
- Environmental and Science Education: Overlaps and Issues
- Environmental Education
- Environmental Education in Brazil
- Epistemic Beliefs
- Equity and Improvement: Engaging Communities in Educationa...
- Equity, Ethnicity, Diversity, and Excellence in Education
- Ethical Research with Young Children
- Ethics and Education
- Ethics of Teaching
- Ethnic Studies
- Evidence-Based Communication Assessment and Intervention
- Family and Community Partnerships in Education
- Family Day Care
- Federal Government Programs and Issues
- Feminization of Labor in Academia
- Finance, Education
- Financial Aid
- Formative Assessment
- Future-Focused Education
- Gender and Achievement
- Gender and Alternative Education
- Gender-Based Violence on University Campuses
- Gifted Education
- Global Mindedness and Global Citizenship Education
- Global University Rankings
- Governance, Education
- Growth of Effective Mental Health Services in Schools in t...
- Higher Education and Globalization
- Higher Education and the Developing World
- Higher Education Faculty Characteristics and Trends in the...
- Higher Education Finance
- Higher Education Governance
- Higher Education Graduate Outcomes and Destinations
- Higher Education in Africa
- Higher Education in China
- Higher Education in Latin America
- Higher Education in the United States, Historical Evolutio...
- Higher Education, International Issues in
- Higher Education Management
- Higher Education Policy
- Higher Education Research
- Higher Education Student Assessment
- High-stakes Testing
- History of Early Childhood Education in the United States
- History of Education in the United States
- History of Technology Integration in Education
- Homeschooling
- Inclusion in Early Childhood: Difference, Disability, and ...
- Inclusive Education
- Indigenous Education in a Global Context
- Indigenous Learning Environments
- Indigenous Students in Higher Education in the United Stat...
- Infant and Toddler Pedagogy
- Inservice Teacher Education
- Integrating Art across the Curriculum
- Intelligence
- Intensive Interventions for Children and Adolescents with ...
- International Perspectives on Academic Freedom
- Intersectionality and Education
- Knowledge Development in Early Childhood
- Leadership Development, Coaching and Feedback for
- Leadership in Early Childhood Education
- Leadership Training with an Emphasis on the United States
- Learning Analytics in Higher Education
- Learning Difficulties
- Learning, Lifelong
- Learning, Multimedia
- Learning Strategies
- Legal Matters and Education Law
- LGBT Youth in Schools
- Linguistic Diversity
- Linguistically Inclusive Pedagogy
- Literacy Development and Language Acquisition
- Literature Reviews
- Mathematics Identity
- Mathematics Instruction and Interventions for Students wit...
- Mathematics Teacher Education
- Measurement for Improvement in Education
- Measurement in Education in the United States
- Meta-Analysis and Research Synthesis in Education
- Methodological Approaches for Impact Evaluation in Educati...
- Mindfulness, Learning, and Education
- Motherscholars
- Multiliteracies in Early Childhood Education
- Multiple Documents Literacy: Theory, Research, and Applica...
- Multivariate Research Methodology
- Museums, Education, and Curriculum
- Music Education
- Narrative Research in Education
- Native American Studies
- Note-Taking
- Numeracy Education
- One-to-One Technology in the K-12 Classroom
- Online Education
- Open Education
- Organizing for Continuous Improvement in Education
- Organizing Schools for the Inclusion of Students with Disa...
- Outdoor Play and Learning
- Outdoor Play and Learning in Early Childhood Education
- Pedagogical Leadership
- Pedagogy of Teacher Education, A
- Performance Objectives and Measurement
- Performance-based Research Assessment in Higher Education
- Performance-based Research Funding
- Phenomenology in Educational Research
- Philosophy of Education
- Physical Education
- Podcasts in Education
- Policy Context of United States Educational Innovation and...
- Politics of Education
- Portable Technology Use in Special Education Programs and ...
- Post-humanism and Environmental Education
- Pre-Service Teacher Education
- Problem Solving
- Productivity and Higher Education
- Professional Development
- Professional Learning Communities
- Program Evaluation
- Programs and Services for Students with Emotional or Behav...
- Psychology Learning and Teaching
- Psychometric Issues in the Assessment of English Language ...
- Qualitative Data Analysis Techniques
- Qualitative, Quantitative, and Mixed Methods Research Samp...
- Quantitative Research Designs in Educational Research
- Queering the English Language Arts (ELA) Writing Classroom
- Race and Affirmative Action in Higher Education
- Reading Education
- Refugee and New Immigrant Learners
- Relational and Developmental Trauma and Schools
- Relational Pedagogies in Early Childhood Education
- Reliability in Educational Assessments
- Religion in Elementary and Secondary Education in the Unit...
- Researcher Development and Skills Training within the Cont...
- Research-Practice Partnerships in Education within the Uni...
- Response to Intervention
- Restorative Practices
- Risky Play in Early Childhood Education
- Scale and Sustainability of Education Innovation and Impro...
- Scaling Up Research-based Educational Practices
- School Accreditation
- School Choice
- School Culture
- School District Budgeting and Financial Management in the ...
- School Improvement through Inclusive Education
- School Reform
- Schools, Private and Independent
- School-Wide Positive Behavior Support
- Science Education
- Secondary to Postsecondary Transition Issues
- Self-Regulated Learning
- Self-Study of Teacher Education Practices
- Service-Learning
- Severe Disabilities
- Single Salary Schedule
- Single-sex Education
- Single-Subject Research Design
- Social Context of Education
- Social Justice
- Social Network Analysis
- Social Pedagogy
- Social Science and Education Research
- Social Studies Education
- Sociology of Education
- Standards-Based Education
- Student Access, Equity, and Diversity in Higher Education
- Student Assignment Policy
- Student Engagement in Tertiary Education
- Student Learning, Development, Engagement, and Motivation ...
- Student Participation
- Student Voice in Teacher Development
- Sustainability Education in Early Childhood Education
- Sustainability in Early Childhood Education
- Sustainability in Higher Education
- Teacher Beliefs and Epistemologies
- Teacher Collaboration in School Improvement
- Teacher Evaluation and Teacher Effectiveness
- Teacher Preparation
- Teacher Training and Development
- Teacher Unions and Associations
- Teacher-Student Relationships
- Teaching Critical Thinking
- Technologies, Teaching, and Learning in Higher Education
- Technology Education in Early Childhood
- Technology, Educational
- Technology-based Assessment
- The Bologna Process
- The Regulation of Standards in Higher Education
- Theories of Educational Leadership
- Three Conceptions of Literacy: Media, Narrative, and Gamin...
- Tracking and Detracking
- Traditions of Quality Improvement in Education
- Transformative Learning
- Transitions in Early Childhood Education
- Tribally Controlled Colleges and Universities in the Unite...
- Understanding the Psycho-Social Dimensions of Schools and ...
- University Faculty Roles and Responsibilities in the Unite...
- Value of Higher Education for Students and Other Stakehold...
- Virtual Learning Environments
- Vocational and Technical Education
- Wellness and Well-Being in Education
- Women's and Gender Studies
- Young Children and Spirituality
- Young Children's Learning Dispositions
- Young Children's Working Theories
- Privacy Policy
- Cookie Policy
- Legal Notice
- Accessibility
Powered by:
- [66.249.64.20|81.177.182.136]
- 81.177.182.136
Quantitative research in education : Background information
- Background information
- SAGE researchmethods SAGE Research Methods is a tool created to help researchers, faculty and students with their research projects. Users can explore methods concepts to help them design research projects, understand particular methods or identify a new method, conduct their research, and write up their findings. Since SAGE Research Methods focuses on methodology rather than disciplines, it can be used across the social sciences, health sciences, and other areas of research.
- The American freshman, national norms for ... From the Higher Education Research Institute, University of California, Los Angeles
- Education at a glance : OECD indicators
- Global education digest From UNESCO
- Next: Recent e-books >>
- Recent e-books
- Recent print books
- Connect to Stanford e-resources

- Last Updated: Jan 23, 2024 12:46 PM
- URL: https://guides.library.stanford.edu/quantitative_research_in_ed

Quantitative Data Analysis 101
The lingo, methods and techniques, explained simply.
By: Derek Jansen (MBA) and Kerryn Warren (PhD) | December 2020
Quantitative data analysis is one of those things that often strikes fear in students. It’s totally understandable – quantitative analysis is a complex topic, full of daunting lingo , like medians, modes, correlation and regression. Suddenly we’re all wishing we’d paid a little more attention in math class…
The good news is that while quantitative data analysis is a mammoth topic, gaining a working understanding of the basics isn’t that hard , even for those of us who avoid numbers and math . In this post, we’ll break quantitative analysis down into simple , bite-sized chunks so you can approach your research with confidence.
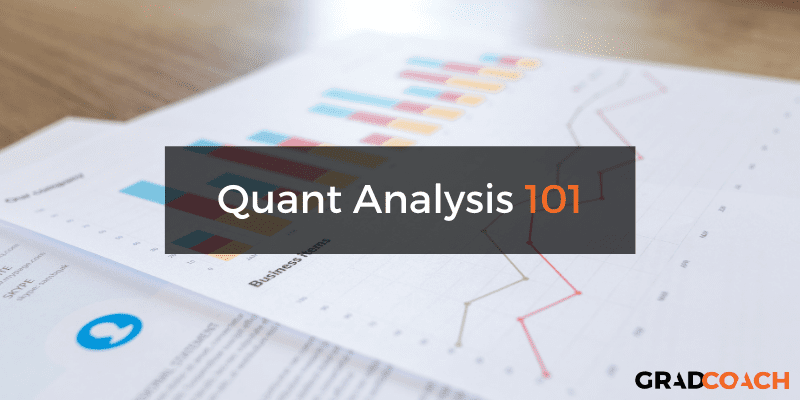
Overview: Quantitative Data Analysis 101
- What (exactly) is quantitative data analysis?
- When to use quantitative analysis
- How quantitative analysis works
The two “branches” of quantitative analysis
- Descriptive statistics 101
- Inferential statistics 101
- How to choose the right quantitative methods
- Recap & summary
What is quantitative data analysis?
Despite being a mouthful, quantitative data analysis simply means analysing data that is numbers-based – or data that can be easily “converted” into numbers without losing any meaning.
For example, category-based variables like gender, ethnicity, or native language could all be “converted” into numbers without losing meaning – for example, English could equal 1, French 2, etc.
This contrasts against qualitative data analysis, where the focus is on words, phrases and expressions that can’t be reduced to numbers. If you’re interested in learning about qualitative analysis, check out our post and video here .
What is quantitative analysis used for?
Quantitative analysis is generally used for three purposes.
- Firstly, it’s used to measure differences between groups . For example, the popularity of different clothing colours or brands.
- Secondly, it’s used to assess relationships between variables . For example, the relationship between weather temperature and voter turnout.
- And third, it’s used to test hypotheses in a scientifically rigorous way. For example, a hypothesis about the impact of a certain vaccine.
Again, this contrasts with qualitative analysis , which can be used to analyse people’s perceptions and feelings about an event or situation. In other words, things that can’t be reduced to numbers.
How does quantitative analysis work?
Well, since quantitative data analysis is all about analysing numbers , it’s no surprise that it involves statistics . Statistical analysis methods form the engine that powers quantitative analysis, and these methods can vary from pretty basic calculations (for example, averages and medians) to more sophisticated analyses (for example, correlations and regressions).
Sounds like gibberish? Don’t worry. We’ll explain all of that in this post. Importantly, you don’t need to be a statistician or math wiz to pull off a good quantitative analysis. We’ll break down all the technical mumbo jumbo in this post.
Need a helping hand?
As I mentioned, quantitative analysis is powered by statistical analysis methods . There are two main “branches” of statistical methods that are used – descriptive statistics and inferential statistics . In your research, you might only use descriptive statistics, or you might use a mix of both , depending on what you’re trying to figure out. In other words, depending on your research questions, aims and objectives . I’ll explain how to choose your methods later.
So, what are descriptive and inferential statistics?
Well, before I can explain that, we need to take a quick detour to explain some lingo. To understand the difference between these two branches of statistics, you need to understand two important words. These words are population and sample .
First up, population . In statistics, the population is the entire group of people (or animals or organisations or whatever) that you’re interested in researching. For example, if you were interested in researching Tesla owners in the US, then the population would be all Tesla owners in the US.
However, it’s extremely unlikely that you’re going to be able to interview or survey every single Tesla owner in the US. Realistically, you’ll likely only get access to a few hundred, or maybe a few thousand owners using an online survey. This smaller group of accessible people whose data you actually collect is called your sample .
So, to recap – the population is the entire group of people you’re interested in, and the sample is the subset of the population that you can actually get access to. In other words, the population is the full chocolate cake , whereas the sample is a slice of that cake.
So, why is this sample-population thing important?
Well, descriptive statistics focus on describing the sample , while inferential statistics aim to make predictions about the population, based on the findings within the sample. In other words, we use one group of statistical methods – descriptive statistics – to investigate the slice of cake, and another group of methods – inferential statistics – to draw conclusions about the entire cake. There I go with the cake analogy again…
With that out the way, let’s take a closer look at each of these branches in more detail.
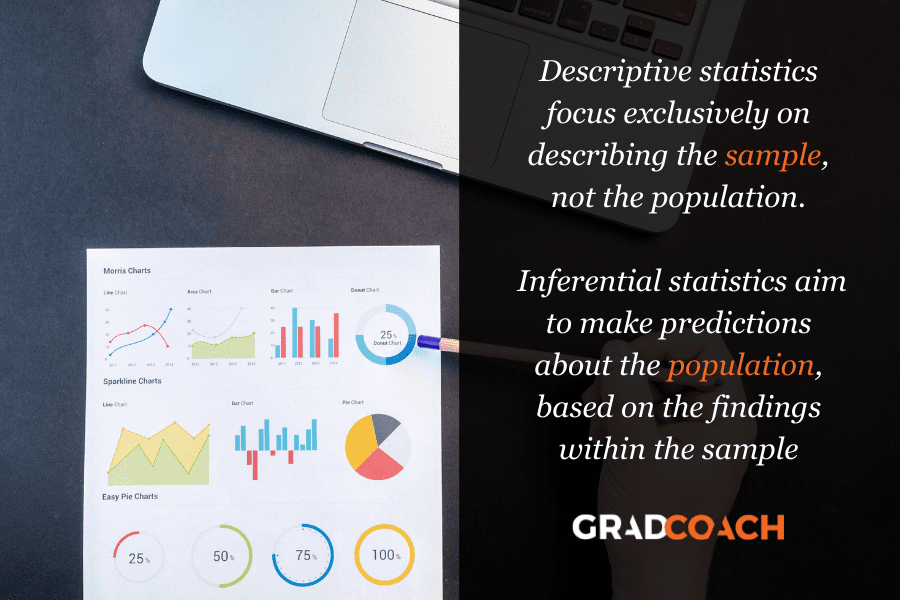
Branch 1: Descriptive Statistics
Descriptive statistics serve a simple but critically important role in your research – to describe your data set – hence the name. In other words, they help you understand the details of your sample . Unlike inferential statistics (which we’ll get to soon), descriptive statistics don’t aim to make inferences or predictions about the entire population – they’re purely interested in the details of your specific sample .
When you’re writing up your analysis, descriptive statistics are the first set of stats you’ll cover, before moving on to inferential statistics. But, that said, depending on your research objectives and research questions , they may be the only type of statistics you use. We’ll explore that a little later.
So, what kind of statistics are usually covered in this section?
Some common statistical tests used in this branch include the following:
- Mean – this is simply the mathematical average of a range of numbers.
- Median – this is the midpoint in a range of numbers when the numbers are arranged in numerical order. If the data set makes up an odd number, then the median is the number right in the middle of the set. If the data set makes up an even number, then the median is the midpoint between the two middle numbers.
- Mode – this is simply the most commonly occurring number in the data set.
- In cases where most of the numbers are quite close to the average, the standard deviation will be relatively low.
- Conversely, in cases where the numbers are scattered all over the place, the standard deviation will be relatively high.
- Skewness . As the name suggests, skewness indicates how symmetrical a range of numbers is. In other words, do they tend to cluster into a smooth bell curve shape in the middle of the graph, or do they skew to the left or right?
Feeling a bit confused? Let’s look at a practical example using a small data set.
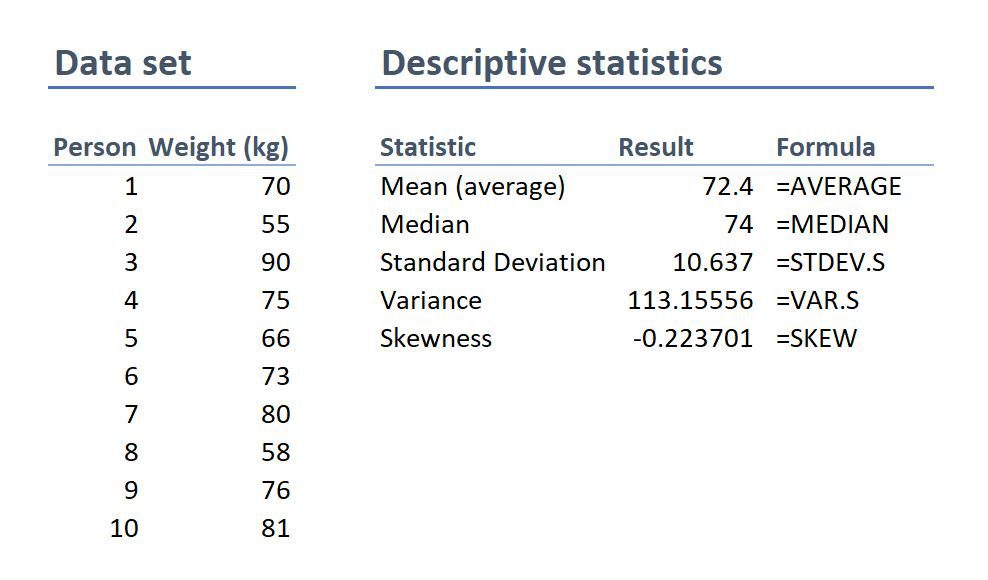
On the left-hand side is the data set. This details the bodyweight of a sample of 10 people. On the right-hand side, we have the descriptive statistics. Let’s take a look at each of them.
First, we can see that the mean weight is 72.4 kilograms. In other words, the average weight across the sample is 72.4 kilograms. Straightforward.
Next, we can see that the median is very similar to the mean (the average). This suggests that this data set has a reasonably symmetrical distribution (in other words, a relatively smooth, centred distribution of weights, clustered towards the centre).
In terms of the mode , there is no mode in this data set. This is because each number is present only once and so there cannot be a “most common number”. If there were two people who were both 65 kilograms, for example, then the mode would be 65.
Next up is the standard deviation . 10.6 indicates that there’s quite a wide spread of numbers. We can see this quite easily by looking at the numbers themselves, which range from 55 to 90, which is quite a stretch from the mean of 72.4.
And lastly, the skewness of -0.2 tells us that the data is very slightly negatively skewed. This makes sense since the mean and the median are slightly different.
As you can see, these descriptive statistics give us some useful insight into the data set. Of course, this is a very small data set (only 10 records), so we can’t read into these statistics too much. Also, keep in mind that this is not a list of all possible descriptive statistics – just the most common ones.
But why do all of these numbers matter?
While these descriptive statistics are all fairly basic, they’re important for a few reasons:
- Firstly, they help you get both a macro and micro-level view of your data. In other words, they help you understand both the big picture and the finer details.
- Secondly, they help you spot potential errors in the data – for example, if an average is way higher than you’d expect, or responses to a question are highly varied, this can act as a warning sign that you need to double-check the data.
- And lastly, these descriptive statistics help inform which inferential statistical techniques you can use, as those techniques depend on the skewness (in other words, the symmetry and normality) of the data.
Simply put, descriptive statistics are really important , even though the statistical techniques used are fairly basic. All too often at Grad Coach, we see students skimming over the descriptives in their eagerness to get to the more exciting inferential methods, and then landing up with some very flawed results.
Don’t be a sucker – give your descriptive statistics the love and attention they deserve!
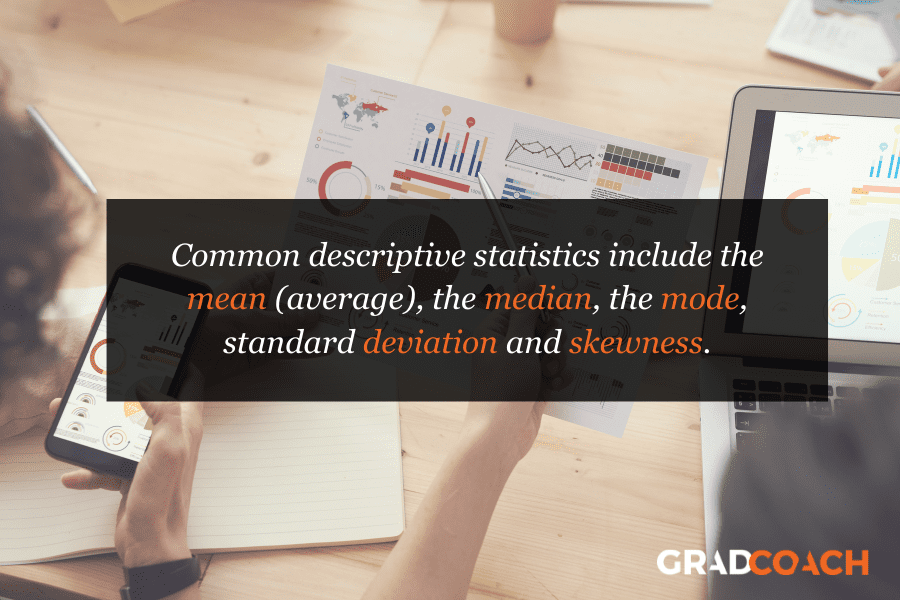
Branch 2: Inferential Statistics
As I mentioned, while descriptive statistics are all about the details of your specific data set – your sample – inferential statistics aim to make inferences about the population . In other words, you’ll use inferential statistics to make predictions about what you’d expect to find in the full population.
What kind of predictions, you ask? Well, there are two common types of predictions that researchers try to make using inferential stats:
- Firstly, predictions about differences between groups – for example, height differences between children grouped by their favourite meal or gender.
- And secondly, relationships between variables – for example, the relationship between body weight and the number of hours a week a person does yoga.
In other words, inferential statistics (when done correctly), allow you to connect the dots and make predictions about what you expect to see in the real world population, based on what you observe in your sample data. For this reason, inferential statistics are used for hypothesis testing – in other words, to test hypotheses that predict changes or differences.
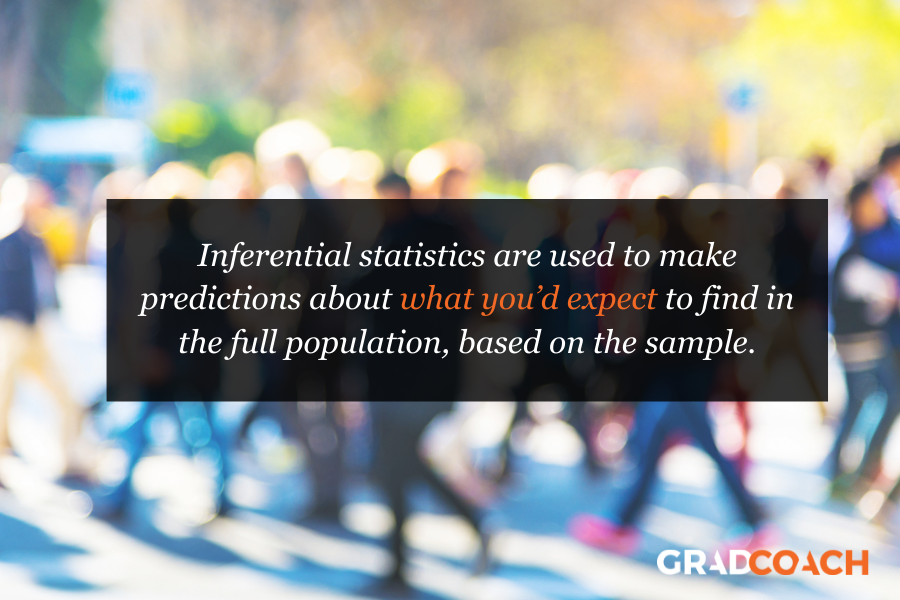
Of course, when you’re working with inferential statistics, the composition of your sample is really important. In other words, if your sample doesn’t accurately represent the population you’re researching, then your findings won’t necessarily be very useful.
For example, if your population of interest is a mix of 50% male and 50% female , but your sample is 80% male , you can’t make inferences about the population based on your sample, since it’s not representative. This area of statistics is called sampling, but we won’t go down that rabbit hole here (it’s a deep one!) – we’ll save that for another post .
What statistics are usually used in this branch?
There are many, many different statistical analysis methods within the inferential branch and it’d be impossible for us to discuss them all here. So we’ll just take a look at some of the most common inferential statistical methods so that you have a solid starting point.
First up are T-Tests . T-tests compare the means (the averages) of two groups of data to assess whether they’re statistically significantly different. In other words, do they have significantly different means, standard deviations and skewness.
This type of testing is very useful for understanding just how similar or different two groups of data are. For example, you might want to compare the mean blood pressure between two groups of people – one that has taken a new medication and one that hasn’t – to assess whether they are significantly different.
Kicking things up a level, we have ANOVA, which stands for “analysis of variance”. This test is similar to a T-test in that it compares the means of various groups, but ANOVA allows you to analyse multiple groups , not just two groups So it’s basically a t-test on steroids…
Next, we have correlation analysis . This type of analysis assesses the relationship between two variables. In other words, if one variable increases, does the other variable also increase, decrease or stay the same. For example, if the average temperature goes up, do average ice creams sales increase too? We’d expect some sort of relationship between these two variables intuitively , but correlation analysis allows us to measure that relationship scientifically .
Lastly, we have regression analysis – this is quite similar to correlation in that it assesses the relationship between variables, but it goes a step further to understand cause and effect between variables, not just whether they move together. In other words, does the one variable actually cause the other one to move, or do they just happen to move together naturally thanks to another force? Just because two variables correlate doesn’t necessarily mean that one causes the other.
Stats overload…
I hear you. To make this all a little more tangible, let’s take a look at an example of a correlation in action.
Here’s a scatter plot demonstrating the correlation (relationship) between weight and height. Intuitively, we’d expect there to be some relationship between these two variables, which is what we see in this scatter plot. In other words, the results tend to cluster together in a diagonal line from bottom left to top right.
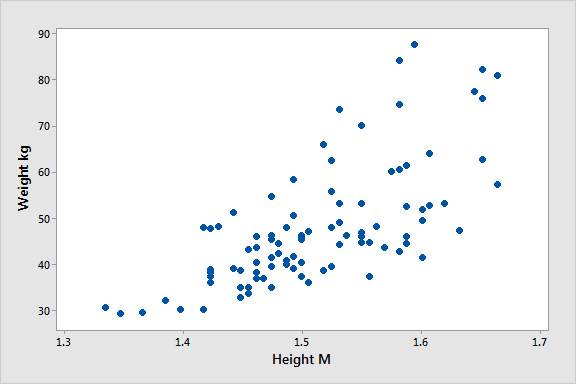
As I mentioned, these are are just a handful of inferential techniques – there are many, many more. Importantly, each statistical method has its own assumptions and limitations.
For example, some methods only work with normally distributed (parametric) data, while other methods are designed specifically for non-parametric data. And that’s exactly why descriptive statistics are so important – they’re the first step to knowing which inferential techniques you can and can’t use.
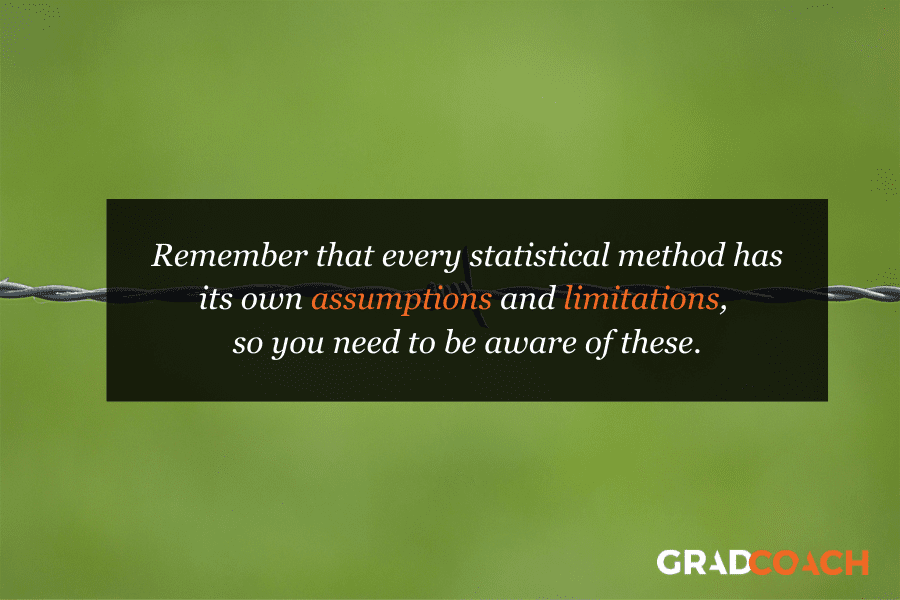
How to choose the right analysis method
To choose the right statistical methods, you need to think about two important factors :
- The type of quantitative data you have (specifically, level of measurement and the shape of the data). And,
- Your research questions and hypotheses
Let’s take a closer look at each of these.
Factor 1 – Data type
The first thing you need to consider is the type of data you’ve collected (or the type of data you will collect). By data types, I’m referring to the four levels of measurement – namely, nominal, ordinal, interval and ratio. If you’re not familiar with this lingo, check out the video below.
Why does this matter?
Well, because different statistical methods and techniques require different types of data. This is one of the “assumptions” I mentioned earlier – every method has its assumptions regarding the type of data.
For example, some techniques work with categorical data (for example, yes/no type questions, or gender or ethnicity), while others work with continuous numerical data (for example, age, weight or income) – and, of course, some work with multiple data types.
If you try to use a statistical method that doesn’t support the data type you have, your results will be largely meaningless . So, make sure that you have a clear understanding of what types of data you’ve collected (or will collect). Once you have this, you can then check which statistical methods would support your data types here .
If you haven’t collected your data yet, you can work in reverse and look at which statistical method would give you the most useful insights, and then design your data collection strategy to collect the correct data types.
Another important factor to consider is the shape of your data . Specifically, does it have a normal distribution (in other words, is it a bell-shaped curve, centred in the middle) or is it very skewed to the left or the right? Again, different statistical techniques work for different shapes of data – some are designed for symmetrical data while others are designed for skewed data.
This is another reminder of why descriptive statistics are so important – they tell you all about the shape of your data.
Factor 2: Your research questions
The next thing you need to consider is your specific research questions, as well as your hypotheses (if you have some). The nature of your research questions and research hypotheses will heavily influence which statistical methods and techniques you should use.
If you’re just interested in understanding the attributes of your sample (as opposed to the entire population), then descriptive statistics are probably all you need. For example, if you just want to assess the means (averages) and medians (centre points) of variables in a group of people.
On the other hand, if you aim to understand differences between groups or relationships between variables and to infer or predict outcomes in the population, then you’ll likely need both descriptive statistics and inferential statistics.
So, it’s really important to get very clear about your research aims and research questions, as well your hypotheses – before you start looking at which statistical techniques to use.
Never shoehorn a specific statistical technique into your research just because you like it or have some experience with it. Your choice of methods must align with all the factors we’ve covered here.
Time to recap…
You’re still with me? That’s impressive. We’ve covered a lot of ground here, so let’s recap on the key points:
- Quantitative data analysis is all about analysing number-based data (which includes categorical and numerical data) using various statistical techniques.
- The two main branches of statistics are descriptive statistics and inferential statistics . Descriptives describe your sample, whereas inferentials make predictions about what you’ll find in the population.
- Common descriptive statistical methods include mean (average), median , standard deviation and skewness .
- Common inferential statistical methods include t-tests , ANOVA , correlation and regression analysis.
- To choose the right statistical methods and techniques, you need to consider the type of data you’re working with , as well as your research questions and hypotheses.
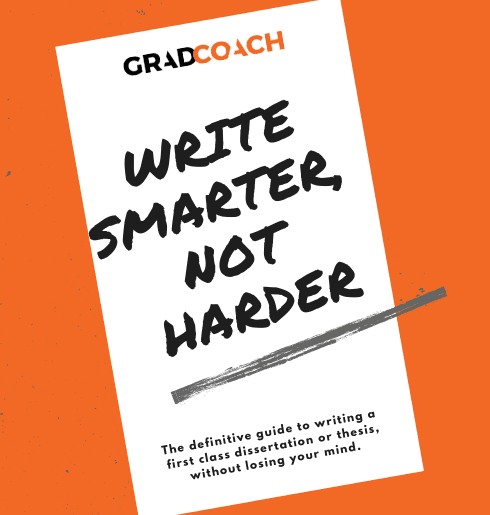
Psst… there’s more (for free)
This post is part of our dissertation mini-course, which covers everything you need to get started with your dissertation, thesis or research project.
You Might Also Like:
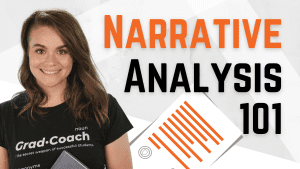
73 Comments
Hi, I have read your article. Such a brilliant post you have created.
Thank you for the feedback. Good luck with your quantitative analysis.
Thank you so much.
Thank you so much. I learnt much well. I love your summaries of the concepts. I had love you to explain how to input data using SPSS
Amazing and simple way of breaking down quantitative methods.
This is beautiful….especially for non-statisticians. I have skimmed through but I wish to read again. and please include me in other articles of the same nature when you do post. I am interested. I am sure, I could easily learn from you and get off the fear that I have had in the past. Thank you sincerely.
Send me every new information you might have.
i need every new information
Thank you for the blog. It is quite informative. Dr Peter Nemaenzhe PhD
It is wonderful. l’ve understood some of the concepts in a more compréhensive manner
Your article is so good! However, I am still a bit lost. I am doing a secondary research on Gun control in the US and increase in crime rates and I am not sure which analysis method I should use?
Based on the given learning points, this is inferential analysis, thus, use ‘t-tests, ANOVA, correlation and regression analysis’
Well explained notes. Am an MPH student and currently working on my thesis proposal, this has really helped me understand some of the things I didn’t know.
I like your page..helpful
wonderful i got my concept crystal clear. thankyou!!
This is really helpful , thank you
Thank you so much this helped
Wonderfully explained
thank u so much, it was so informative
THANKYOU, this was very informative and very helpful
This is great GRADACOACH I am not a statistician but I require more of this in my thesis
Include me in your posts.
This is so great and fully useful. I would like to thank you again and again.
Glad to read this article. I’ve read lot of articles but this article is clear on all concepts. Thanks for sharing.
Thank you so much. This is a very good foundation and intro into quantitative data analysis. Appreciate!
You have a very impressive, simple but concise explanation of data analysis for Quantitative Research here. This is a God-send link for me to appreciate research more. Thank you so much!
Avery good presentation followed by the write up. yes you simplified statistics to make sense even to a layman like me. Thank so much keep it up. The presenter did ell too. i would like more of this for Qualitative and exhaust more of the test example like the Anova.
This is a very helpful article, couldn’t have been clearer. Thank you.
Awesome and phenomenal information.Well done
The video with the accompanying article is super helpful to demystify this topic. Very well done. Thank you so much.
thank you so much, your presentation helped me a lot
I don’t know how should I express that ur article is saviour for me 🥺😍
It is well defined information and thanks for sharing. It helps me a lot in understanding the statistical data.
I gain a lot and thanks for sharing brilliant ideas, so wish to be linked on your email update.
Very helpful and clear .Thank you Gradcoach.
Thank for sharing this article, well organized and information presented are very clear.
VERY INTERESTING AND SUPPORTIVE TO NEW RESEARCHERS LIKE ME. AT LEAST SOME BASICS ABOUT QUANTITATIVE.
An outstanding, well explained and helpful article. This will help me so much with my data analysis for my research project. Thank you!
wow this has just simplified everything i was scared of how i am gonna analyse my data but thanks to you i will be able to do so
simple and constant direction to research. thanks
This is helpful
Great writing!! Comprehensive and very helpful.
Do you provide any assistance for other steps of research methodology like making research problem testing hypothesis report and thesis writing?
Thank you so much for such useful article!
Amazing article. So nicely explained. Wow
Very insightfull. Thanks
I am doing a quality improvement project to determine if the implementation of a protocol will change prescribing habits. Would this be a t-test?
The is a very helpful blog, however, I’m still not sure how to analyze my data collected. I’m doing a research on “Free Education at the University of Guyana”
tnx. fruitful blog!
So I am writing exams and would like to know how do establish which method of data analysis to use from the below research questions: I am a bit lost as to how I determine the data analysis method from the research questions.
Do female employees report higher job satisfaction than male employees with similar job descriptions across the South African telecommunications sector? – I though that maybe Chi Square could be used here. – Is there a gender difference in talented employees’ actual turnover decisions across the South African telecommunications sector? T-tests or Correlation in this one. – Is there a gender difference in the cost of actual turnover decisions across the South African telecommunications sector? T-tests or Correlation in this one. – What practical recommendations can be made to the management of South African telecommunications companies on leveraging gender to mitigate employee turnover decisions?
Your assistance will be appreciated if I could get a response as early as possible tomorrow
This was quite helpful. Thank you so much.
wow I got a lot from this article, thank you very much, keep it up
Thanks for yhe guidance. Can you send me this guidance on my email? To enable offline reading?
Thank you very much, this service is very helpful.
Every novice researcher needs to read this article as it puts things so clear and easy to follow. Its been very helpful.
Wonderful!!!! you explained everything in a way that anyone can learn. Thank you!!
I really enjoyed reading though this. Very easy to follow. Thank you
Many thanks for your useful lecture, I would be really appreciated if you could possibly share with me the PPT of presentation related to Data type?
Thank you very much for sharing, I got much from this article
This is a very informative write-up. Kindly include me in your latest posts.
Very interesting mostly for social scientists
Thank you so much, very helpfull
You’re welcome 🙂
woow, its great, its very informative and well understood because of your way of writing like teaching in front of me in simple languages.
I have been struggling to understand a lot of these concepts. Thank you for the informative piece which is written with outstanding clarity.
very informative article. Easy to understand
Beautiful read, much needed.
Always greet intro and summary. I learn so much from GradCoach
Quite informative. Simple and clear summary.
I thoroughly enjoyed reading your informative and inspiring piece. Your profound insights into this topic truly provide a better understanding of its complexity. I agree with the points you raised, especially when you delved into the specifics of the article. In my opinion, that aspect is often overlooked and deserves further attention.
Absolutely!!! Thank you
Submit a Comment Cancel reply
Your email address will not be published. Required fields are marked *
Save my name, email, and website in this browser for the next time I comment.
- Print Friendly
ENCYCLOPEDIC ENTRY
Quantitative data.
Science wouldn't be science without the facts, research, and numbers to back up all those theories
Mathematics
Loading ...
Science wouldn't be science without the facts, research, and numbers to back up all those theories. Can you imagine Albert Einstein trying to promote his famous theory of relativity without any numbers, research, or the famous formula E=MC ²? The hard numbers behind any good research project are called quantitative data . Quantitative data is the language of science. It uses mathematical models, theories, and hypotheses. Thanks to this type of data, we know things like the distance between the sun and the moon , and why we have seasons , days, and nights. Quantitative data depends on the fact that you can put everything in terms of numbers. "This hot chocolate is hotter than that hot chocolate" might be a true statement. But it is not as informative as "This hot chocolate is 100 degrees Fahrenheit; therefore, it is 30 degrees hotter than this other cup of hot chocolate, which is 70 degrees Fahrenheit." Quantitative data and qualitative data , in which you observe the non-numerical qualities of your subject, go hand-in-hand. Measurements are very important to science and observation. But knowing and testing how something feels and smells is also important. In fact, knowing how something feels and smells may lead you to wonder why it feels and smells that way. Before you know it, you're using numbers and formulas to find your answer. A frequent traveler might make a qualitative observation that the Hartsfield-Jackson Atlanta International Airport, in the U.S. state of Georgia, is very busy. The quantitative data about passenger traffic at Hartsfield-Jackson would prove that the airport is, in fact, the busiest in the world: In 2008, more than 90 million passengers used Hartsfield-Jackson. Scientists determined this by comparing quantitative data from other airports. The next-busiest airport was Chicago O'Hare International Airport, in the U.S. state of Illinois, which handled 69.4 million passengers. The busiest airport outside the United States was Heathrow Airport in London, England. It handled 67 million passengers. Each of the airports provides that quantitative data, also known as numerical data, to the public.
Media Credits
The audio, illustrations, photos, and videos are credited beneath the media asset, except for promotional images, which generally link to another page that contains the media credit. The Rights Holder for media is the person or group credited.
Illustrators
Educator reviewer, last updated.
October 19, 2023
User Permissions
For information on user permissions, please read our Terms of Service. If you have questions about how to cite anything on our website in your project or classroom presentation, please contact your teacher. They will best know the preferred format. When you reach out to them, you will need the page title, URL, and the date you accessed the resource.
If a media asset is downloadable, a download button appears in the corner of the media viewer. If no button appears, you cannot download or save the media.
Text on this page is printable and can be used according to our Terms of Service .
Interactives
Any interactives on this page can only be played while you are visiting our website. You cannot download interactives.
Related Resources
- Privacy Policy
Buy Me a Coffee

Home » Quantitative Data – Types, Methods and Examples
Quantitative Data – Types, Methods and Examples
Table of Contents

Quantitative Data
Definition:
Quantitative data refers to numerical data that can be measured or counted. This type of data is often used in scientific research and is typically collected through methods such as surveys, experiments, and statistical analysis.
Quantitative Data Types
There are two main types of quantitative data: discrete and continuous.
- Discrete data: Discrete data refers to numerical values that can only take on specific, distinct values. This type of data is typically represented as whole numbers and cannot be broken down into smaller units. Examples of discrete data include the number of students in a class, the number of cars in a parking lot, and the number of children in a family.
- Continuous data: Continuous data refers to numerical values that can take on any value within a certain range or interval. This type of data is typically represented as decimal or fractional values and can be broken down into smaller units. Examples of continuous data include measurements of height, weight, temperature, and time.
Quantitative Data Collection Methods
There are several common methods for collecting quantitative data. Some of these methods include:
- Surveys : Surveys involve asking a set of standardized questions to a large number of people. Surveys can be conducted in person, over the phone, via email or online, and can be used to collect data on a wide range of topics.
- Experiments : Experiments involve manipulating one or more variables and observing the effects on a specific outcome. Experiments can be conducted in a controlled laboratory setting or in the real world.
- Observational studies : Observational studies involve observing and collecting data on a specific phenomenon without intervening or manipulating any variables. Observational studies can be conducted in a natural setting or in a laboratory.
- Secondary data analysis : Secondary data analysis involves using existing data that was collected for a different purpose to answer a new research question. This method can be cost-effective and efficient, but it is important to ensure that the data is appropriate for the research question being studied.
- Physiological measures: Physiological measures involve collecting data on biological or physiological processes, such as heart rate, blood pressure, or brain activity.
- Computerized tracking: Computerized tracking involves collecting data automatically from electronic sources, such as social media, online purchases, or website analytics.
Quantitative Data Analysis Methods
There are several methods for analyzing quantitative data, including:
- Descriptive statistics: Descriptive statistics are used to summarize and describe the basic features of the data, such as the mean, median, mode, standard deviation, and range.
- Inferential statistics : Inferential statistics are used to make generalizations about a population based on a sample of data. These methods include hypothesis testing, confidence intervals, and regression analysis.
- Data visualization: Data visualization involves creating charts, graphs, and other visual representations of the data to help identify patterns and trends. Common types of data visualization include histograms, scatterplots, and bar charts.
- Time series analysis: Time series analysis involves analyzing data that is collected over time to identify patterns and trends in the data.
- Multivariate analysis : Multivariate analysis involves analyzing data with multiple variables to identify relationships between the variables.
- Factor analysis : Factor analysis involves identifying underlying factors or dimensions that explain the variation in the data.
- Cluster analysis: Cluster analysis involves identifying groups or clusters of observations that are similar to each other based on multiple variables.
Quantitative Data Formats
Quantitative data can be represented in different formats, depending on the nature of the data and the purpose of the analysis. Here are some common formats:
- Tables : Tables are a common way to present quantitative data, particularly when the data involves multiple variables. Tables can be used to show the frequency or percentage of data in different categories or to display summary statistics.
- Charts and graphs: Charts and graphs are useful for visualizing quantitative data and can be used to highlight patterns and trends in the data. Some common types of charts and graphs include line charts, bar charts, scatterplots, and pie charts.
- Databases : Quantitative data can be stored in databases, which allow for easy sorting, filtering, and analysis of large amounts of data.
- Spreadsheets : Spreadsheets can be used to organize and analyze quantitative data, particularly when the data is relatively small in size. Spreadsheets allow for calculations and data manipulation, as well as the creation of charts and graphs.
- Statistical software : Statistical software, such as SPSS, R, and SAS, can be used to analyze quantitative data. These programs allow for more advanced statistical analyses and data modeling, as well as the creation of charts and graphs.
Quantitative Data Gathering Guide
Here is a basic guide for gathering quantitative data:
- Define the research question: The first step in gathering quantitative data is to clearly define the research question. This will help determine the type of data to be collected, the sample size, and the methods of data analysis.
- Choose the data collection method: Select the appropriate method for collecting data based on the research question and available resources. This could include surveys, experiments, observational studies, or other methods.
- Determine the sample size: Determine the appropriate sample size for the research question. This will depend on the level of precision needed and the variability of the population being studied.
- Develop the data collection instrument: Develop a questionnaire or survey instrument that will be used to collect the data. The instrument should be designed to gather the specific information needed to answer the research question.
- Pilot test the data collection instrument : Before collecting data from the entire sample, pilot test the instrument on a small group to identify any potential problems or issues.
- Collect the data: Collect the data from the selected sample using the chosen data collection method.
- Clean and organize the data : Organize the data into a format that can be easily analyzed. This may involve checking for missing data, outliers, or errors.
- Analyze the data: Analyze the data using appropriate statistical methods. This may involve descriptive statistics, inferential statistics, or other types of analysis.
- Interpret the results: Interpret the results of the analysis in the context of the research question. Identify any patterns, trends, or relationships in the data and draw conclusions based on the findings.
- Communicate the findings: Communicate the findings of the analysis in a clear and concise manner, using appropriate tables, graphs, and other visual aids as necessary. The results should be presented in a way that is accessible to the intended audience.
Examples of Quantitative Data
Here are some examples of quantitative data:
- Height of a person (measured in inches or centimeters)
- Weight of a person (measured in pounds or kilograms)
- Temperature (measured in Fahrenheit or Celsius)
- Age of a person (measured in years)
- Number of cars sold in a month
- Amount of rainfall in a specific area (measured in inches or millimeters)
- Number of hours worked in a week
- GPA (grade point average) of a student
- Sales figures for a product
- Time taken to complete a task.
- Distance traveled (measured in miles or kilometers)
- Speed of an object (measured in miles per hour or kilometers per hour)
- Number of people attending an event
- Price of a product (measured in dollars or other currency)
- Blood pressure (measured in millimeters of mercury)
- Amount of sugar in a food item (measured in grams)
- Test scores (measured on a numerical scale)
- Number of website visitors per day
- Stock prices (measured in dollars)
- Crime rates (measured by the number of crimes per 100,000 people)
Applications of Quantitative Data
Quantitative data has a wide range of applications across various fields, including:
- Scientific research: Quantitative data is used extensively in scientific research to test hypotheses and draw conclusions. For example, in biology, researchers might use quantitative data to measure the growth rate of cells or the effectiveness of a drug treatment.
- Business and economics: Quantitative data is used to analyze business and economic trends, forecast future performance, and make data-driven decisions. For example, a company might use quantitative data to analyze sales figures and customer demographics to determine which products are most popular among which segments of their customer base.
- Education: Quantitative data is used in education to measure student performance, evaluate teaching methods, and identify areas where improvement is needed. For example, a teacher might use quantitative data to track the progress of their students over the course of a semester and adjust their teaching methods accordingly.
- Public policy: Quantitative data is used in public policy to evaluate the effectiveness of policies and programs, identify areas where improvement is needed, and develop evidence-based solutions. For example, a government agency might use quantitative data to evaluate the impact of a social welfare program on poverty rates.
- Healthcare : Quantitative data is used in healthcare to evaluate the effectiveness of medical treatments, track the spread of diseases, and identify risk factors for various health conditions. For example, a doctor might use quantitative data to monitor the blood pressure levels of their patients over time and adjust their treatment plan accordingly.
Purpose of Quantitative Data
The purpose of quantitative data is to provide a numerical representation of a phenomenon or observation. Quantitative data is used to measure and describe the characteristics of a population or sample, and to test hypotheses and draw conclusions based on statistical analysis. Some of the key purposes of quantitative data include:
- Measuring and describing : Quantitative data is used to measure and describe the characteristics of a population or sample, such as age, income, or education level. This allows researchers to better understand the population they are studying.
- Testing hypotheses: Quantitative data is often used to test hypotheses and theories by collecting numerical data and analyzing it using statistical methods. This can help researchers determine whether there is a statistically significant relationship between variables or whether there is support for a particular theory.
- Making predictions : Quantitative data can be used to make predictions about future events or trends based on past data. This is often done through statistical modeling or time series analysis.
- Evaluating programs and policies: Quantitative data is often used to evaluate the effectiveness of programs and policies. This can help policymakers and program managers identify areas where improvements can be made and make evidence-based decisions about future programs and policies.
When to use Quantitative Data
Quantitative data is appropriate to use when you want to collect and analyze numerical data that can be measured and analyzed using statistical methods. Here are some situations where quantitative data is typically used:
- When you want to measure a characteristic or behavior : If you want to measure something like the height or weight of a population or the number of people who smoke, you would use quantitative data to collect this information.
- When you want to compare groups: If you want to compare two or more groups, such as comparing the effectiveness of two different medical treatments, you would use quantitative data to collect and analyze the data.
- When you want to test a hypothesis : If you have a hypothesis or theory that you want to test, you would use quantitative data to collect data that can be analyzed statistically to determine whether your hypothesis is supported by the data.
- When you want to make predictions: If you want to make predictions about future trends or events, such as predicting sales for a new product, you would use quantitative data to collect and analyze data from past trends to make your prediction.
- When you want to evaluate a program or policy : If you want to evaluate the effectiveness of a program or policy, you would use quantitative data to collect data about the program or policy and analyze it statistically to determine whether it has had the intended effect.
Characteristics of Quantitative Data
Quantitative data is characterized by several key features, including:
- Numerical values : Quantitative data consists of numerical values that can be measured and counted. These values are often expressed in terms of units, such as dollars, centimeters, or kilograms.
- Continuous or discrete : Quantitative data can be either continuous or discrete. Continuous data can take on any value within a certain range, while discrete data can only take on certain values.
- Objective: Quantitative data is objective, meaning that it is not influenced by personal biases or opinions. It is based on empirical evidence that can be measured and analyzed using statistical methods.
- Large sample size: Quantitative data is often collected from a large sample size in order to ensure that the results are statistically significant and representative of the population being studied.
- Statistical analysis: Quantitative data is typically analyzed using statistical methods to determine patterns, relationships, and other characteristics of the data. This allows researchers to make more objective conclusions based on empirical evidence.
- Precision : Quantitative data is often very precise, with measurements taken to multiple decimal points or significant figures. This precision allows for more accurate analysis and interpretation of the data.
Advantages of Quantitative Data
Some advantages of quantitative data are:
- Objectivity : Quantitative data is usually objective because it is based on measurable and observable variables. This means that different people who collect the same data will generally get the same results.
- Precision : Quantitative data provides precise measurements of variables. This means that it is easier to make comparisons and draw conclusions from quantitative data.
- Replicability : Since quantitative data is based on objective measurements, it is often easier to replicate research studies using the same or similar data.
- Generalizability : Quantitative data allows researchers to generalize findings to a larger population. This is because quantitative data is often collected using random sampling methods, which help to ensure that the data is representative of the population being studied.
- Statistical analysis : Quantitative data can be analyzed using statistical methods, which allows researchers to test hypotheses and draw conclusions about the relationships between variables.
- Efficiency : Quantitative data can often be collected quickly and efficiently using surveys or other standardized instruments, which makes it a cost-effective way to gather large amounts of data.
Limitations of Quantitative Data
Some Limitations of Quantitative Data are as follows:
- Limited context: Quantitative data does not provide information about the context in which the data was collected. This can make it difficult to understand the meaning behind the numbers.
- Limited depth: Quantitative data is often limited to predetermined variables and questions, which may not capture the complexity of the phenomenon being studied.
- Difficulty in capturing qualitative aspects: Quantitative data is unable to capture the subjective experiences and qualitative aspects of human behavior, such as emotions, attitudes, and motivations.
- Possibility of bias: The collection and interpretation of quantitative data can be influenced by biases, such as sampling bias, measurement bias, or researcher bias.
- Simplification of complex phenomena: Quantitative data may oversimplify complex phenomena by reducing them to numerical measurements and statistical analyses.
- Lack of flexibility: Quantitative data collection methods may not allow for changes or adaptations in the research process, which can limit the ability to respond to unexpected findings or new insights.
About the author
Muhammad Hassan
Researcher, Academic Writer, Web developer
You may also like

Primary Data – Types, Methods and Examples

Qualitative Data – Types, Methods and Examples

Research Data – Types Methods and Examples

Secondary Data – Types, Methods and Examples

Information in Research – Types and Examples

Quantitative and Qualitative Research
- I NEED TO . . .
What is Quantitative Research?
- What is Qualitative Research?
- Quantitative vs Qualitative
- Step 1: Accessing CINAHL
- Step 2: Create a Keyword Search
- Step 3: Create a Subject Heading Search
- Step 4: Repeat Steps 1-3 for Second Concept
- Step 5: Repeat Steps 1-3 for Quantitative Terms
- Step 6: Combining All Searches
- Step 7: Adding Limiters
- Step 8: Save Your Search!
- What Kind of Article is This?
- More Research Help This link opens in a new window
Quantitative methodology is the dominant research framework in the social sciences. It refers to a set of strategies, techniques and assumptions used to study psychological, social and economic processes through the exploration of numeric patterns . Quantitative research gathers a range of numeric data. Some of the numeric data is intrinsically quantitative (e.g. personal income), while in other cases the numeric structure is imposed (e.g. ‘On a scale from 1 to 10, how depressed did you feel last week?’). The collection of quantitative information allows researchers to conduct simple to extremely sophisticated statistical analyses that aggregate the data (e.g. averages, percentages), show relationships among the data (e.g. ‘Students with lower grade point averages tend to score lower on a depression scale’) or compare across aggregated data (e.g. the USA has a higher gross domestic product than Spain). Quantitative research includes methodologies such as questionnaires, structured observations or experiments and stands in contrast to qualitative research. Qualitative research involves the collection and analysis of narratives and/or open-ended observations through methodologies such as interviews, focus groups or ethnographies.
Coghlan, D., Brydon-Miller, M. (2014). The SAGE encyclopedia of action research (Vols. 1-2). London, : SAGE Publications Ltd doi: 10.4135/9781446294406
What is the purpose of quantitative research?
The purpose of quantitative research is to generate knowledge and create understanding about the social world. Quantitative research is used by social scientists, including communication researchers, to observe phenomena or occurrences affecting individuals. Social scientists are concerned with the study of people. Quantitative research is a way to learn about a particular group of people, known as a sample population. Using scientific inquiry, quantitative research relies on data that are observed or measured to examine questions about the sample population.
Allen, M. (2017). The SAGE encyclopedia of communication research methods (Vols. 1-4). Thousand Oaks, CA: SAGE Publications, Inc doi: 10.4135/9781483381411
How do I know if the study is a quantitative design? What type of quantitative study is it?
Quantitative Research Designs: Descriptive non-experimental, Quasi-experimental or Experimental?
Studies do not always explicitly state what kind of research design is being used. You will need to know how to decipher which design type is used. The following video will help you determine the quantitative design type.
- << Previous: I NEED TO . . .
- Next: What is Qualitative Research? >>
- Last Updated: Dec 8, 2023 10:05 PM
- URL: https://libguides.uta.edu/quantitative_and_qualitative_research
University of Texas Arlington Libraries 702 Planetarium Place · Arlington, TX 76019 · 817-272-3000
- Internet Privacy
- Accessibility
- Problems with a guide? Contact Us.
- Find My Rep
You are here
Understanding Quantitative Data in Educational Research
- Nicoleta Gaciu - Oxford Brookes University, UK
- Description
This book is designed to help Education students gain confidence in analysing and interpreting quantitative data and using appropriate statistical tests, by exploring, in plain language, a variety of data analysis methods.
Highly practical, each chapter includes step-by-step instructions on how to run specific statistical tests using R, practical tips on how to interpret results correctly and exercises to put into practice what students have learned.
It also includes guidance on how to use R and RStudio, how to visualise quantitative data, and the fundamentals of inferential statistics, estimations and hypothesis testing.
Nicoleta Gaciu is Senior Lecturer in Education at Oxford Brookes University.
Supplements
Preview this book, for instructors.
Please select a format:
Select a Purchasing Option
- Electronic Order Options VitalSource Amazon Kindle Google Play eBooks.com Kobo
Related Products

What is Quantitative Data?
Data professionals work with two types of data: quantitative and qualitative. What is quantitative data? What is qualitative data? In simple terms, quantitative data is measurable while qualitative data is descriptive—think numbers versus words.
If you plan on working as a data analyst or a data scientist (or in any field that involves conducting research, like psychology), you’ll need to get to grips with both. In this post, we’ll focus on quantitative data. We’ll explain exactly what quantitative data is, including plenty of useful examples. We’ll also show you what methods you can use to collect and analyze quantitative data.
By the end of this post, you’ll have a clear understanding of quantitative data and how it’s used.
We’ll cover:
- What is quantitative data? (Definition)
- What are some examples of quantitative data?
- What’s the difference between quantitative and qualitative data?
- What are the different types of quantitative data?
- How is quantitative data collected?
- What methods are used to analyze quantitative data?
- What are the advantages and disadvantages of quantitative data?
- Should I use quantitative or qualitative data in my research?
- What are some common quantitative data analysis tools?
- What is quantitative data? FAQs
- Key takeaways
So: what is quantitative data? Let’s find out.
1. What is quantitative data? (Definition)
Quantitative data is, quite simply, information that can be quantified. It can be counted or measured, and given a numerical value—such as length in centimeters or revenue in dollars. Quantitative data tends to be structured in nature and is suitable for statistical analysis. If you have questions such as “How many?”, “How often?” or “How much?”, you’ll find the answers in quantitative data.
2. What are some examples of quantitative data?
Some examples of quantitative data include:
- Revenue in dollars
- Weight in kilograms
- Age in months or years
- Length in centimeters
- Distance in kilometers
- Height in feet or inches
- Number of weeks in a year
3. What is the difference between quantitative and qualitative data?
It’s hard to define quantitative data without comparing it to qualitative data—so what’s the difference between the two?
While quantitative data can be counted and measured, qualitative data is descriptive and, typically, unstructured. It usually takes the form of words and text—for example, a status posted on Facebook or an interview transcript are both forms of qualitative data. You can also think of qualitative data in terms of the “descriptors” you would use to describe certain attributes. For example, if you were to describe someone’s hair color as auburn, or an ice cream flavor as vanilla, these labels count as qualitative data.
Qualitative data cannot be used for statistical analysis; to make sense of such data, researchers and analysts will instead try to identify meaningful groups and themes.
You’ll find a detailed exploration of the differences between qualitative and quantitative data in this post . But, to summarize:
- Quantitative data is countable or measurable, relating to numbers; qualitative data is descriptive, relating to words.
- Quantitative data lends itself to statistical analysis; qualitative data is grouped and categorized according to themes.
- Examples of quantitative data include numerical values such as measurements, cost, and weight; examples of qualitative data include descriptions (or labels) of certain attributes, such as “brown eyes” or “vanilla flavored ice cream”.
Now we know the difference between the two, let’s get back to quantitative data.
4. What are the different types of quantitative data?
There are two main types of quantitative data: discrete and continuous .
Discrete data
Discrete data is quantitative data that can only take on certain numerical values. These values are fixed and cannot be broken down. When you count something, you get discrete data. For example, if a person has three children, this is an example of discrete data. The number of children is fixed—it’s not possible for them to have, say, 3.2 children.
Another example of discrete quantitative data could be the number of visits to your website; you could have 150 visits in one day, but not 150.6 visits. Discrete data is usually visualized using tally charts, bar charts, and pie charts.
Continuous data
Continuous data, on the other hand, can be infinitely broken down into smaller parts. This type of quantitative data can be placed on a measurement scale; for example, the length of a piece of string in centimeters, or the temperature in degrees Celsius. Essentially, continuous data can take any value; it’s not limited to fixed values. What’s more, continuous data can also fluctuate over time—the room temperature will vary throughout the day, for example. Continuous data is usually represented using a line graph.
Continuous data can be further classified depending on whether it’s interval data or ratio data . Let’s take a look at those now.
Interval vs. ratio data
Interval data can be measured along a continuum, where there is an equal distance between each point on the scale. For example: The difference between 30 and 31 degrees C is equal to the difference between 99 and 100 degrees. Another thing to bear in mind is that interval data has no true or meaningful zero value . Temperature is a good example; a temperature of zero degrees does not mean that there is “no temperature”—it just means that it’s extremely cold!
Ratio data is the same as interval data in terms of equally spaced points on a scale, but unlike interval data, ratio data does have a true zero . Weight in grams would be classified as ratio data; the difference between 20 grams and 21 grams is equal to the difference between 8 and 9 grams, and if something weighs zero grams, it truly weighs nothing.
Beyond the distinction between discrete and continuous data, quantitative data can also be broken down into several different types:
- Measurements: This type of data refers to the measurement of physical objects. For example, you might measure the length and width of your living room before ordering new sofas.
- Sensors: A sensor is a device or system which detects changes in the surrounding environment and sends this information to another electronic device, usually a computer. This information is then converted into numbers—that’s your quantitative data. For example, a smart temperature sensor will provide you with a stream of data about the temperature of the room throughout the day.
- Counts: As the name suggests, this is the quantitative data you get when you count things. You might count the number of people who attended an event, or the number of visits to your website in one week.
- Quantification of qualitative data: This is when qualitative data is converted into numbers. Take the example of customer satisfaction. If a customer said “I’m really happy with this product”, that would count as qualitative data. You could turn this into quantitative data by asking them to rate their satisfaction on a scale of 1-10.
- Calculations: This is any quantitative data that results from mathematical calculations, such as calculating your final profit at the end of the month.
- Projections: Analysts may estimate or predict quantities using algorithms, artificial intelligence, or “manual” analysis. For example, you might predict how many sales you expect to make in the next quarter. The figure you come up with is a projection of quantitative data.
Knowing what type of quantitative data you’re working with helps you to apply the correct type of statistical analysis. We’ll look at how quantitative data is analyzed in section five.
5. How is quantitative data collected?
Now we know what quantitative data is, we can start to think about how analysts actually work with it in the real world. Before the data can be analyzed, it first needs to be generated or collected. So how is this done?
Researchers (for example, psychologists or scientists) will often conduct experiments and studies in order to gather quantitative data and test certain hypotheses. A psychologist investigating the relationship between social media usage and self-esteem might devise a questionnaire with various scales—for example, asking participants to rate, on a scale of one to five, the extent to which they agree with certain statements.
If the survey reaches enough people, the psychologist ends up with a large sample of quantitative data (for example, an overall self-esteem score for each participant) which they can then analyze.
Data analysts and data scientists are less likely to conduct experiments, but they may send out questionnaires and surveys—it all depends on the sector they’re working in. Usually, data professionals will work with “naturally occurring” quantitative data, such as the number of sales per quarter, or how often a customer uses a particular service.
Some common methods of data collection include:
- Analytics tools, such as Google Analytics
- Probability sampling
Questionnaires and surveys
- Open-source datasets on the web
Analytics tools
Data analysts and data scientists rely on specialist tools to gather quantitative data from various sources. Google Analytics, for example, will gather data pertaining to your website; at a glance, you can see metrics such as how much traffic you got in one week, how many page views per minute, and average session length—all useful insights if you want to optimize the performance of your site.
Aside from Google Analytics, which tends to be used within the marketing sector, there are loads of tools out there which can be connected to multiple data sources at once. Tools like RapidMiner, Knime, Qlik, and Splunk can be integrated with internal databases, data lakes, cloud storage, business apps, social media, and IoT devices, allowing you to access data from multiple sources all in one place.
You can learn more about the top tools used by data analysts in this guide
Sampling is when, instead of analyzing an entire dataset, you select a sample or “section” of the data. Sampling may be used to save time and money, and in cases where it’s simply not possible to study an entire population. For example, if you wanted to analyze data pertaining to the residents of New York, it’s unlikely that you’d be able to get hold of data for every single person in the state. Instead, you’d analyze a representative sample.
There are two types of sampling: Random probability sampling, where each unit within the overall dataset has the same chance of being selected (i.e. included in the sample), and non-probability sampling, where the sample is actively selected by the researcher or analyst—not at random. Data analysts and scientists may use Python (the popular programming language) and various algorithms to extract samples from large datasets.
Another way to collect quantitative data is through questionnaires and surveys. Nowadays, it’s easy to create a survey and distribute it online—with tools like Typeform , SurveyMonkey , and Qualtrics , practically anyone can collect quantitative data. Surveys are a useful tool for gathering customer or user feedback, and generally finding out how people feel about certain products or services.
To make sure you gather quantitative data from your surveys, it’s important that you ask respondents to quantify their feelings—for example, asking them to rate their satisfaction on a scale of one to ten.
Open-source datasets online
In addition to analyzing data from internal databases, data analysts might also collect quantitative data from external sources. Again, it all depends on the field you’re working in and what kind of data you need. The internet is full of free and open datasets spanning a range of sectors, from government, business and finance, to science, transport, film, and entertainment—pretty much anything you can think of! We’ve put together a list of places where you can find free datasets here .
6. How is quantitative data analyzed?
A defining characteristic of quantitative data is that it’s suitable for statistical analysis. There are many different methods and techniques used for quantitative data analysis, and how you analyze your data depends on what you hope to find out.
Before we go into some specific methods of analysis, it’s important to distinguish between descriptive and inferential analysis .
What’s the difference between descriptive and inferential analysis of quantitative data?
Descriptive analysis does exactly what it says on the tin; it describes the data. This is useful as it allows you to see, at a glance, what the basic qualities of your data are and what you’re working with. Some commonly used descriptive statistics include the range (the difference between the highest and lowest scores), the minimum and maximum (the lowest and highest scores in a dataset), and frequency (how often a certain value appears in the dataset).
You might also calculate various measures of central tendency in order to gauge the general trend of your data. Measures of central tendency include the mean (the sum of all values divided by the number of values, otherwise known as the average), the median (the middle score when all scores are ordered numerically), and the mode (the most frequently occurring score). Another useful calculation is standard deviation . This tells you how representative of the entire dataset the mean value actually is.
While descriptive statistics give you an initial read on your quantitative data, they don’t allow you to draw definitive conclusions. That’s where inferential analysis comes in. With inferential statistics, you can make inferences and predictions. This allows you to test various hypotheses and to predict future outcomes based on probability theory.
Quantitative data analysis methods
When it comes to deriving insights from your quantitative data, there’s a whole host of techniques at your disposal. Some of the most common (and useful) methods of quantitative data analysis include:
- Regression analysis: This is used to estimate the relationship between a set of variables, and to see if there’s any kind of correlation between the two. Regression is especially useful for making predictions and forecasting future trends.
- Monte Carlo simulation : The Monte Carlo method is a computerized technique used to generate models of possible outcomes and their probability distributions based on your dataset. It essentially considers a range of possible outcomes and then calculates how likely it is that each particular outcome will occur. It’s used by data analysts to conduct advanced risk analysis, allowing them to accurately predict what might happen in the future.
- Cohort analysis: A cohort is a group of people who share a common attribute or behavior during a given time period—for example, a cohort of students who all started university in 2020, or a cohort of customers who purchased via your app in the month of February. Cohort analysis essentially divides your dataset into cohorts and analyzes how these cohorts behave over time. This is especially useful for identifying patterns in customer behavior and tailoring your products and services accordingly.
- Cluster analysis : This is an exploratory technique used to identify structures within a dataset. The aim of cluster analysis is to sort different data points into groups that are internally homogenous and externally heterogeneous—in other words, data points within a cluster are similar to each other, but dissimilar to data points in other clusters. Clustering is used to see how data is distributed in a given dataset, or as a preprocessing step for other algorithms.
- Time series analysis : This is used to identify trends and cycles over time. Time series data is a sequence of data points which measure the same variable at different points in time, such as weekly sales figures or monthly email sign-ups. By looking at time-related trends, analysts can forecast how the variable of interest may fluctuate in the future. Extremely handy when it comes to making business decisions!
Above is just a very brief introduction to how you might analyze your quantitative data. For a more in-depth look, check out this comprehensive guide to some of the most useful data analysis techniques .
7. What are the advantages and disadvantages of quantitative data?
As with anything, there are both advantages and disadvantages of using quantitative data. So what are they? Let’s take a look.
Advantages of quantitative data
The main advantages of working with quantitative data are as follows:
- Quantitative data is relatively quick and easy to collect , allowing you to gather a large sample size. And, the larger your sample size, the more accurate your conclusions are likely to be.
- Quantitative data is less susceptible to bias. The use of random sampling helps to ensure that a given dataset is as representative as possible, and protects the sample from bias. This is crucial for drawing reliable conclusions.
- Quantitative data is analyzed objectively. Because quantitative data is suitable for statistical analysis, it can be analyzed according to mathematical rules and principles. This greatly reduces the impact of analyst or researcher bias on how the results are interpreted.
Disadvantages of quantitative data
There are two main drawbacks to be aware of when working with quantitative data, especially within a research context:
- Quantitative data can lack context. In some cases, context is key; for example, if you’re conducting a questionnaire to find out how customers feel about a new product. The quantitative data may tell you that 60% of customers are unhappy with the product, but that figure alone will not tell you why. Sometimes, you’ll need to delve deeper to gain valuable insights beyond the numbers.
- There is a risk of bias when using surveys and questionnaires. Again, this point relates more to a research context, but it’s important to bear in mind when creating surveys and questionnaires. The way in which questions are worded can allow researcher bias to seep in, so it’s important to make sure that surveys are devised carefully. You can learn all about how to reduce survey bias in this post .
8. Should I use quantitative or qualitative data in my research?
Okay—so now we know what the difference between quantitative and qualitative data is, as well as other aspects of quantitative data. But when should you make use of quantitative or qualitative research? This answer to this question will depend on the type of project you’re working on—or client you’re working for—specifically. But use these simple criteria as a guide:
- When to use quantitative research: when you want to confirm or test something, like a theory or hypothesis. When the data can be shown clearly in numbers. Think of a city census that shows the whole number of people living there, as well as their ages, incomes, and other useful information that makes up a city’s demographic.
- When to use qualitative research: when you want to understand something—for example, a concept, experience, or opinions. Maybe you’re testing out a run of experiences for your company, and need to gather reviews for a specific time period. This would be an example of qualitative research.
- When to use both quantitative and qualitative research: when you’re taking on a research project that demands both numerical and non-numerical data.
9. What are some common quantitative analysis tools?
The tools used for quantitative data collection and analysis should come as no surprise to the budding data analyst. You may end up using one tool per project, or a combination of tools:
- Microsoft Power BI
10. What is quantitative data? FAQs
Who uses quantitative data.
Quantitative data is used in many fields—not just data analytics (though, you could argue that all of these fields are at least data-analytics-adjacent)! Those working in the fields of economics, epidemiology, psychology, sociology, and health—to name a few—would make great use of quantitative data in their work. You would be less likely to see quantitative data being used in fields such as anthropology and history.
Is quantitative data better than qualitative data?
It would be hard to make a solid argument of which form of data collection is “better”, as it really depends on the type of project you’re working on. However, quantitative research provides more “hard and fast” information that can be used to make informed, objective decisions.
Where is quantitative data used?
Quantitative data is used when a problem needs to be quantified. That is, to answer the questions that start with “how many…” or “how often…”, for example.
What is quantitative data in statistics?
As statistics is an umbrella term of a discipline concerning the collection, organization and analysis of data, it’s only natural that quantitative data falls under that umbrella—the practice of counting and measuring data sets according to a research question or set of research needs.
Can quantitative data be ordinal?
Ordinal data is a type of statistical data where the variables are sorted into ranges, and the distance between the ranges are not known. Think of the pain scale they sometimes use in the hospital, where you judge the level of pain you have on a scale of 1-10, with 1 being low and 10 being the highest. However, you can’t really quantify the difference between 1-10—it’s a matter of how you feel!
By that logic, ordinal data falls under qualitative data, not quantitative. You can learn more about the data levels of measurement in this post .
Is quantitative data objective?
Due to the nature of how quantitative data is produced—that is, using methods that are verifiable and replicable—it is objective.
11. Key takeaways and further reading
In this post, we answered the question: what is quantitative data? We looked at how it differs from qualitative data, and how it’s collected and analyzed. To recap what we’ve learned:
- Quantitative data is data that can be quantified. It can be counted or measured, and given a numerical value.
- Quantitative data lends itself to statistical analysis, while qualitative data is grouped according to themes.
- Quantitative data can be discrete or continuous. Discrete data takes on fixed values (e.g. a person has three children), while continuous data can be infinitely broken down into smaller parts.
- Quantitative data has several advantages: It is relatively quick and easy to collect, and it is analyzed subjectively.
Collecting and analyzing quantitative data is just one aspect of the data analyst’s work. To learn more about what it’s like to work as a data analyst, check out the following guides. And, if you’d like to dabble in some analytics yourself, why not try our free five-day introductory short course ?
- What is data analytics? A beginner’s guide
- A step-by-step guide to the data analysis process
- Where could a career in data analytics take you?
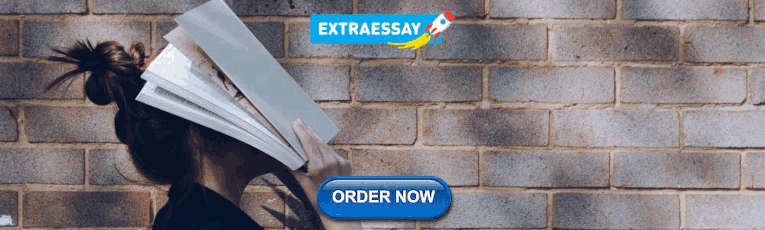
Qualitative vs Quantitative Research Methods & Data Analysis
Saul Mcleod, PhD
Editor-in-Chief for Simply Psychology
BSc (Hons) Psychology, MRes, PhD, University of Manchester
Saul Mcleod, PhD., is a qualified psychology teacher with over 18 years of experience in further and higher education. He has been published in peer-reviewed journals, including the Journal of Clinical Psychology.
Learn about our Editorial Process
Olivia Guy-Evans, MSc
Associate Editor for Simply Psychology
BSc (Hons) Psychology, MSc Psychology of Education
Olivia Guy-Evans is a writer and associate editor for Simply Psychology. She has previously worked in healthcare and educational sectors.
On This Page:
What is the difference between quantitative and qualitative?
The main difference between quantitative and qualitative research is the type of data they collect and analyze.
Quantitative research collects numerical data and analyzes it using statistical methods. The aim is to produce objective, empirical data that can be measured and expressed in numerical terms. Quantitative research is often used to test hypotheses, identify patterns, and make predictions.
Qualitative research, on the other hand, collects non-numerical data such as words, images, and sounds. The focus is on exploring subjective experiences, opinions, and attitudes, often through observation and interviews.
Qualitative research aims to produce rich and detailed descriptions of the phenomenon being studied, and to uncover new insights and meanings.
Quantitative data is information about quantities, and therefore numbers, and qualitative data is descriptive, and regards phenomenon which can be observed but not measured, such as language.
What Is Qualitative Research?
Qualitative research is the process of collecting, analyzing, and interpreting non-numerical data, such as language. Qualitative research can be used to understand how an individual subjectively perceives and gives meaning to their social reality.
Qualitative data is non-numerical data, such as text, video, photographs, or audio recordings. This type of data can be collected using diary accounts or in-depth interviews and analyzed using grounded theory or thematic analysis.
Qualitative research is multimethod in focus, involving an interpretive, naturalistic approach to its subject matter. This means that qualitative researchers study things in their natural settings, attempting to make sense of, or interpret, phenomena in terms of the meanings people bring to them. Denzin and Lincoln (1994, p. 2)
Interest in qualitative data came about as the result of the dissatisfaction of some psychologists (e.g., Carl Rogers) with the scientific study of psychologists such as behaviorists (e.g., Skinner ).
Since psychologists study people, the traditional approach to science is not seen as an appropriate way of carrying out research since it fails to capture the totality of human experience and the essence of being human. Exploring participants’ experiences is known as a phenomenological approach (re: Humanism ).
Qualitative research is primarily concerned with meaning, subjectivity, and lived experience. The goal is to understand the quality and texture of people’s experiences, how they make sense of them, and the implications for their lives.
Qualitative research aims to understand the social reality of individuals, groups, and cultures as nearly as possible as participants feel or live it. Thus, people and groups are studied in their natural setting.
Some examples of qualitative research questions are provided, such as what an experience feels like, how people talk about something, how they make sense of an experience, and how events unfold for people.
Research following a qualitative approach is exploratory and seeks to explain ‘how’ and ‘why’ a particular phenomenon, or behavior, operates as it does in a particular context. It can be used to generate hypotheses and theories from the data.
Qualitative Methods
There are different types of qualitative research methods, including diary accounts, in-depth interviews , documents, focus groups , case study research , and ethnography.
The results of qualitative methods provide a deep understanding of how people perceive their social realities and in consequence, how they act within the social world.
The researcher has several methods for collecting empirical materials, ranging from the interview to direct observation, to the analysis of artifacts, documents, and cultural records, to the use of visual materials or personal experience. Denzin and Lincoln (1994, p. 14)
Here are some examples of qualitative data:
Interview transcripts : Verbatim records of what participants said during an interview or focus group. They allow researchers to identify common themes and patterns, and draw conclusions based on the data. Interview transcripts can also be useful in providing direct quotes and examples to support research findings.
Observations : The researcher typically takes detailed notes on what they observe, including any contextual information, nonverbal cues, or other relevant details. The resulting observational data can be analyzed to gain insights into social phenomena, such as human behavior, social interactions, and cultural practices.
Unstructured interviews : generate qualitative data through the use of open questions. This allows the respondent to talk in some depth, choosing their own words. This helps the researcher develop a real sense of a person’s understanding of a situation.
Diaries or journals : Written accounts of personal experiences or reflections.
Notice that qualitative data could be much more than just words or text. Photographs, videos, sound recordings, and so on, can be considered qualitative data. Visual data can be used to understand behaviors, environments, and social interactions.
Qualitative Data Analysis
Qualitative research is endlessly creative and interpretive. The researcher does not just leave the field with mountains of empirical data and then easily write up his or her findings.
Qualitative interpretations are constructed, and various techniques can be used to make sense of the data, such as content analysis, grounded theory (Glaser & Strauss, 1967), thematic analysis (Braun & Clarke, 2006), or discourse analysis.
For example, thematic analysis is a qualitative approach that involves identifying implicit or explicit ideas within the data. Themes will often emerge once the data has been coded.
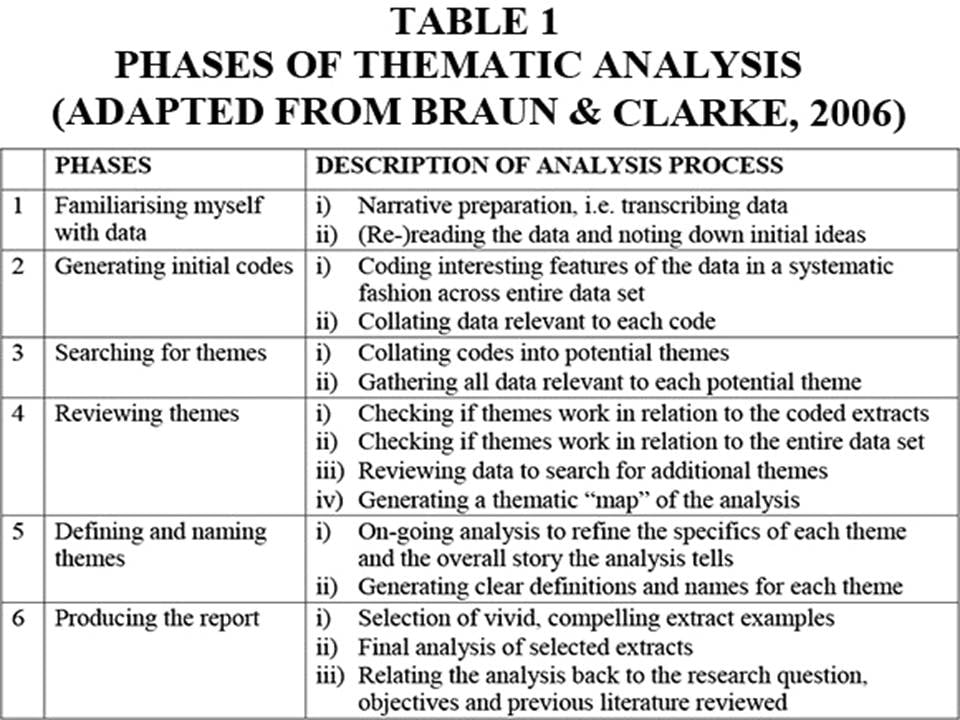
Key Features
- Events can be understood adequately only if they are seen in context. Therefore, a qualitative researcher immerses her/himself in the field, in natural surroundings. The contexts of inquiry are not contrived; they are natural. Nothing is predefined or taken for granted.
- Qualitative researchers want those who are studied to speak for themselves, to provide their perspectives in words and other actions. Therefore, qualitative research is an interactive process in which the persons studied teach the researcher about their lives.
- The qualitative researcher is an integral part of the data; without the active participation of the researcher, no data exists.
- The study’s design evolves during the research and can be adjusted or changed as it progresses. For the qualitative researcher, there is no single reality. It is subjective and exists only in reference to the observer.
- The theory is data-driven and emerges as part of the research process, evolving from the data as they are collected.
Limitations of Qualitative Research
- Because of the time and costs involved, qualitative designs do not generally draw samples from large-scale data sets.
- The problem of adequate validity or reliability is a major criticism. Because of the subjective nature of qualitative data and its origin in single contexts, it is difficult to apply conventional standards of reliability and validity. For example, because of the central role played by the researcher in the generation of data, it is not possible to replicate qualitative studies.
- Also, contexts, situations, events, conditions, and interactions cannot be replicated to any extent, nor can generalizations be made to a wider context than the one studied with confidence.
- The time required for data collection, analysis, and interpretation is lengthy. Analysis of qualitative data is difficult, and expert knowledge of an area is necessary to interpret qualitative data. Great care must be taken when doing so, for example, looking for mental illness symptoms.
Advantages of Qualitative Research
- Because of close researcher involvement, the researcher gains an insider’s view of the field. This allows the researcher to find issues that are often missed (such as subtleties and complexities) by the scientific, more positivistic inquiries.
- Qualitative descriptions can be important in suggesting possible relationships, causes, effects, and dynamic processes.
- Qualitative analysis allows for ambiguities/contradictions in the data, which reflect social reality (Denscombe, 2010).
- Qualitative research uses a descriptive, narrative style; this research might be of particular benefit to the practitioner as she or he could turn to qualitative reports to examine forms of knowledge that might otherwise be unavailable, thereby gaining new insight.
What Is Quantitative Research?
Quantitative research involves the process of objectively collecting and analyzing numerical data to describe, predict, or control variables of interest.
The goals of quantitative research are to test causal relationships between variables , make predictions, and generalize results to wider populations.
Quantitative researchers aim to establish general laws of behavior and phenomenon across different settings/contexts. Research is used to test a theory and ultimately support or reject it.
Quantitative Methods
Experiments typically yield quantitative data, as they are concerned with measuring things. However, other research methods, such as controlled observations and questionnaires , can produce both quantitative information.
For example, a rating scale or closed questions on a questionnaire would generate quantitative data as these produce either numerical data or data that can be put into categories (e.g., “yes,” “no” answers).
Experimental methods limit how research participants react to and express appropriate social behavior.
Findings are, therefore, likely to be context-bound and simply a reflection of the assumptions that the researcher brings to the investigation.
There are numerous examples of quantitative data in psychological research, including mental health. Here are a few examples:
Another example is the Experience in Close Relationships Scale (ECR), a self-report questionnaire widely used to assess adult attachment styles .
The ECR provides quantitative data that can be used to assess attachment styles and predict relationship outcomes.
Neuroimaging data : Neuroimaging techniques, such as MRI and fMRI, provide quantitative data on brain structure and function.
This data can be analyzed to identify brain regions involved in specific mental processes or disorders.
For example, the Beck Depression Inventory (BDI) is a clinician-administered questionnaire widely used to assess the severity of depressive symptoms in individuals.
The BDI consists of 21 questions, each scored on a scale of 0 to 3, with higher scores indicating more severe depressive symptoms.
Quantitative Data Analysis
Statistics help us turn quantitative data into useful information to help with decision-making. We can use statistics to summarize our data, describing patterns, relationships, and connections. Statistics can be descriptive or inferential.
Descriptive statistics help us to summarize our data. In contrast, inferential statistics are used to identify statistically significant differences between groups of data (such as intervention and control groups in a randomized control study).
- Quantitative researchers try to control extraneous variables by conducting their studies in the lab.
- The research aims for objectivity (i.e., without bias) and is separated from the data.
- The design of the study is determined before it begins.
- For the quantitative researcher, the reality is objective, exists separately from the researcher, and can be seen by anyone.
- Research is used to test a theory and ultimately support or reject it.
Limitations of Quantitative Research
- Context: Quantitative experiments do not take place in natural settings. In addition, they do not allow participants to explain their choices or the meaning of the questions they may have for those participants (Carr, 1994).
- Researcher expertise: Poor knowledge of the application of statistical analysis may negatively affect analysis and subsequent interpretation (Black, 1999).
- Variability of data quantity: Large sample sizes are needed for more accurate analysis. Small-scale quantitative studies may be less reliable because of the low quantity of data (Denscombe, 2010). This also affects the ability to generalize study findings to wider populations.
- Confirmation bias: The researcher might miss observing phenomena because of focus on theory or hypothesis testing rather than on the theory of hypothesis generation.
Advantages of Quantitative Research
- Scientific objectivity: Quantitative data can be interpreted with statistical analysis, and since statistics are based on the principles of mathematics, the quantitative approach is viewed as scientifically objective and rational (Carr, 1994; Denscombe, 2010).
- Useful for testing and validating already constructed theories.
- Rapid analysis: Sophisticated software removes much of the need for prolonged data analysis, especially with large volumes of data involved (Antonius, 2003).
- Replication: Quantitative data is based on measured values and can be checked by others because numerical data is less open to ambiguities of interpretation.
- Hypotheses can also be tested because of statistical analysis (Antonius, 2003).
Antonius, R. (2003). Interpreting quantitative data with SPSS . Sage.
Black, T. R. (1999). Doing quantitative research in the social sciences: An integrated approach to research design, measurement and statistics . Sage.
Braun, V. & Clarke, V. (2006). Using thematic analysis in psychology . Qualitative Research in Psychology , 3, 77–101.
Carr, L. T. (1994). The strengths and weaknesses of quantitative and qualitative research : what method for nursing? Journal of advanced nursing, 20(4) , 716-721.
Denscombe, M. (2010). The Good Research Guide: for small-scale social research. McGraw Hill.
Denzin, N., & Lincoln. Y. (1994). Handbook of Qualitative Research. Thousand Oaks, CA, US: Sage Publications Inc.
Glaser, B. G., Strauss, A. L., & Strutzel, E. (1968). The discovery of grounded theory; strategies for qualitative research. Nursing research, 17(4) , 364.
Minichiello, V. (1990). In-Depth Interviewing: Researching People. Longman Cheshire.
Punch, K. (1998). Introduction to Social Research: Quantitative and Qualitative Approaches. London: Sage
Further Information
- Designing qualitative research
- Methods of data collection and analysis
- Introduction to quantitative and qualitative research
- Checklists for improving rigour in qualitative research: a case of the tail wagging the dog?
- Qualitative research in health care: Analysing qualitative data
- Qualitative data analysis: the framework approach
- Using the framework method for the analysis of
- Qualitative data in multi-disciplinary health research
- Content Analysis
- Grounded Theory
- Thematic Analysis


An official website of the United States government
The .gov means it’s official. Federal government websites often end in .gov or .mil. Before sharing sensitive information, make sure you’re on a federal government site.
The site is secure. The https:// ensures that you are connecting to the official website and that any information you provide is encrypted and transmitted securely.
- Publications
- Account settings
Preview improvements coming to the PMC website in October 2024. Learn More or Try it out now .
- Advanced Search
- Journal List
- J Korean Med Sci
- v.37(16); 2022 Apr 25

A Practical Guide to Writing Quantitative and Qualitative Research Questions and Hypotheses in Scholarly Articles
Edward barroga.
1 Department of General Education, Graduate School of Nursing Science, St. Luke’s International University, Tokyo, Japan.
Glafera Janet Matanguihan
2 Department of Biological Sciences, Messiah University, Mechanicsburg, PA, USA.
The development of research questions and the subsequent hypotheses are prerequisites to defining the main research purpose and specific objectives of a study. Consequently, these objectives determine the study design and research outcome. The development of research questions is a process based on knowledge of current trends, cutting-edge studies, and technological advances in the research field. Excellent research questions are focused and require a comprehensive literature search and in-depth understanding of the problem being investigated. Initially, research questions may be written as descriptive questions which could be developed into inferential questions. These questions must be specific and concise to provide a clear foundation for developing hypotheses. Hypotheses are more formal predictions about the research outcomes. These specify the possible results that may or may not be expected regarding the relationship between groups. Thus, research questions and hypotheses clarify the main purpose and specific objectives of the study, which in turn dictate the design of the study, its direction, and outcome. Studies developed from good research questions and hypotheses will have trustworthy outcomes with wide-ranging social and health implications.
INTRODUCTION
Scientific research is usually initiated by posing evidenced-based research questions which are then explicitly restated as hypotheses. 1 , 2 The hypotheses provide directions to guide the study, solutions, explanations, and expected results. 3 , 4 Both research questions and hypotheses are essentially formulated based on conventional theories and real-world processes, which allow the inception of novel studies and the ethical testing of ideas. 5 , 6
It is crucial to have knowledge of both quantitative and qualitative research 2 as both types of research involve writing research questions and hypotheses. 7 However, these crucial elements of research are sometimes overlooked; if not overlooked, then framed without the forethought and meticulous attention it needs. Planning and careful consideration are needed when developing quantitative or qualitative research, particularly when conceptualizing research questions and hypotheses. 4
There is a continuing need to support researchers in the creation of innovative research questions and hypotheses, as well as for journal articles that carefully review these elements. 1 When research questions and hypotheses are not carefully thought of, unethical studies and poor outcomes usually ensue. Carefully formulated research questions and hypotheses define well-founded objectives, which in turn determine the appropriate design, course, and outcome of the study. This article then aims to discuss in detail the various aspects of crafting research questions and hypotheses, with the goal of guiding researchers as they develop their own. Examples from the authors and peer-reviewed scientific articles in the healthcare field are provided to illustrate key points.
DEFINITIONS AND RELATIONSHIP OF RESEARCH QUESTIONS AND HYPOTHESES
A research question is what a study aims to answer after data analysis and interpretation. The answer is written in length in the discussion section of the paper. Thus, the research question gives a preview of the different parts and variables of the study meant to address the problem posed in the research question. 1 An excellent research question clarifies the research writing while facilitating understanding of the research topic, objective, scope, and limitations of the study. 5
On the other hand, a research hypothesis is an educated statement of an expected outcome. This statement is based on background research and current knowledge. 8 , 9 The research hypothesis makes a specific prediction about a new phenomenon 10 or a formal statement on the expected relationship between an independent variable and a dependent variable. 3 , 11 It provides a tentative answer to the research question to be tested or explored. 4
Hypotheses employ reasoning to predict a theory-based outcome. 10 These can also be developed from theories by focusing on components of theories that have not yet been observed. 10 The validity of hypotheses is often based on the testability of the prediction made in a reproducible experiment. 8
Conversely, hypotheses can also be rephrased as research questions. Several hypotheses based on existing theories and knowledge may be needed to answer a research question. Developing ethical research questions and hypotheses creates a research design that has logical relationships among variables. These relationships serve as a solid foundation for the conduct of the study. 4 , 11 Haphazardly constructed research questions can result in poorly formulated hypotheses and improper study designs, leading to unreliable results. Thus, the formulations of relevant research questions and verifiable hypotheses are crucial when beginning research. 12
CHARACTERISTICS OF GOOD RESEARCH QUESTIONS AND HYPOTHESES
Excellent research questions are specific and focused. These integrate collective data and observations to confirm or refute the subsequent hypotheses. Well-constructed hypotheses are based on previous reports and verify the research context. These are realistic, in-depth, sufficiently complex, and reproducible. More importantly, these hypotheses can be addressed and tested. 13
There are several characteristics of well-developed hypotheses. Good hypotheses are 1) empirically testable 7 , 10 , 11 , 13 ; 2) backed by preliminary evidence 9 ; 3) testable by ethical research 7 , 9 ; 4) based on original ideas 9 ; 5) have evidenced-based logical reasoning 10 ; and 6) can be predicted. 11 Good hypotheses can infer ethical and positive implications, indicating the presence of a relationship or effect relevant to the research theme. 7 , 11 These are initially developed from a general theory and branch into specific hypotheses by deductive reasoning. In the absence of a theory to base the hypotheses, inductive reasoning based on specific observations or findings form more general hypotheses. 10
TYPES OF RESEARCH QUESTIONS AND HYPOTHESES
Research questions and hypotheses are developed according to the type of research, which can be broadly classified into quantitative and qualitative research. We provide a summary of the types of research questions and hypotheses under quantitative and qualitative research categories in Table 1 .
Research questions in quantitative research
In quantitative research, research questions inquire about the relationships among variables being investigated and are usually framed at the start of the study. These are precise and typically linked to the subject population, dependent and independent variables, and research design. 1 Research questions may also attempt to describe the behavior of a population in relation to one or more variables, or describe the characteristics of variables to be measured ( descriptive research questions ). 1 , 5 , 14 These questions may also aim to discover differences between groups within the context of an outcome variable ( comparative research questions ), 1 , 5 , 14 or elucidate trends and interactions among variables ( relationship research questions ). 1 , 5 We provide examples of descriptive, comparative, and relationship research questions in quantitative research in Table 2 .
Hypotheses in quantitative research
In quantitative research, hypotheses predict the expected relationships among variables. 15 Relationships among variables that can be predicted include 1) between a single dependent variable and a single independent variable ( simple hypothesis ) or 2) between two or more independent and dependent variables ( complex hypothesis ). 4 , 11 Hypotheses may also specify the expected direction to be followed and imply an intellectual commitment to a particular outcome ( directional hypothesis ) 4 . On the other hand, hypotheses may not predict the exact direction and are used in the absence of a theory, or when findings contradict previous studies ( non-directional hypothesis ). 4 In addition, hypotheses can 1) define interdependency between variables ( associative hypothesis ), 4 2) propose an effect on the dependent variable from manipulation of the independent variable ( causal hypothesis ), 4 3) state a negative relationship between two variables ( null hypothesis ), 4 , 11 , 15 4) replace the working hypothesis if rejected ( alternative hypothesis ), 15 explain the relationship of phenomena to possibly generate a theory ( working hypothesis ), 11 5) involve quantifiable variables that can be tested statistically ( statistical hypothesis ), 11 6) or express a relationship whose interlinks can be verified logically ( logical hypothesis ). 11 We provide examples of simple, complex, directional, non-directional, associative, causal, null, alternative, working, statistical, and logical hypotheses in quantitative research, as well as the definition of quantitative hypothesis-testing research in Table 3 .
Research questions in qualitative research
Unlike research questions in quantitative research, research questions in qualitative research are usually continuously reviewed and reformulated. The central question and associated subquestions are stated more than the hypotheses. 15 The central question broadly explores a complex set of factors surrounding the central phenomenon, aiming to present the varied perspectives of participants. 15
There are varied goals for which qualitative research questions are developed. These questions can function in several ways, such as to 1) identify and describe existing conditions ( contextual research question s); 2) describe a phenomenon ( descriptive research questions ); 3) assess the effectiveness of existing methods, protocols, theories, or procedures ( evaluation research questions ); 4) examine a phenomenon or analyze the reasons or relationships between subjects or phenomena ( explanatory research questions ); or 5) focus on unknown aspects of a particular topic ( exploratory research questions ). 5 In addition, some qualitative research questions provide new ideas for the development of theories and actions ( generative research questions ) or advance specific ideologies of a position ( ideological research questions ). 1 Other qualitative research questions may build on a body of existing literature and become working guidelines ( ethnographic research questions ). Research questions may also be broadly stated without specific reference to the existing literature or a typology of questions ( phenomenological research questions ), may be directed towards generating a theory of some process ( grounded theory questions ), or may address a description of the case and the emerging themes ( qualitative case study questions ). 15 We provide examples of contextual, descriptive, evaluation, explanatory, exploratory, generative, ideological, ethnographic, phenomenological, grounded theory, and qualitative case study research questions in qualitative research in Table 4 , and the definition of qualitative hypothesis-generating research in Table 5 .
Qualitative studies usually pose at least one central research question and several subquestions starting with How or What . These research questions use exploratory verbs such as explore or describe . These also focus on one central phenomenon of interest, and may mention the participants and research site. 15
Hypotheses in qualitative research
Hypotheses in qualitative research are stated in the form of a clear statement concerning the problem to be investigated. Unlike in quantitative research where hypotheses are usually developed to be tested, qualitative research can lead to both hypothesis-testing and hypothesis-generating outcomes. 2 When studies require both quantitative and qualitative research questions, this suggests an integrative process between both research methods wherein a single mixed-methods research question can be developed. 1
FRAMEWORKS FOR DEVELOPING RESEARCH QUESTIONS AND HYPOTHESES
Research questions followed by hypotheses should be developed before the start of the study. 1 , 12 , 14 It is crucial to develop feasible research questions on a topic that is interesting to both the researcher and the scientific community. This can be achieved by a meticulous review of previous and current studies to establish a novel topic. Specific areas are subsequently focused on to generate ethical research questions. The relevance of the research questions is evaluated in terms of clarity of the resulting data, specificity of the methodology, objectivity of the outcome, depth of the research, and impact of the study. 1 , 5 These aspects constitute the FINER criteria (i.e., Feasible, Interesting, Novel, Ethical, and Relevant). 1 Clarity and effectiveness are achieved if research questions meet the FINER criteria. In addition to the FINER criteria, Ratan et al. described focus, complexity, novelty, feasibility, and measurability for evaluating the effectiveness of research questions. 14
The PICOT and PEO frameworks are also used when developing research questions. 1 The following elements are addressed in these frameworks, PICOT: P-population/patients/problem, I-intervention or indicator being studied, C-comparison group, O-outcome of interest, and T-timeframe of the study; PEO: P-population being studied, E-exposure to preexisting conditions, and O-outcome of interest. 1 Research questions are also considered good if these meet the “FINERMAPS” framework: Feasible, Interesting, Novel, Ethical, Relevant, Manageable, Appropriate, Potential value/publishable, and Systematic. 14
As we indicated earlier, research questions and hypotheses that are not carefully formulated result in unethical studies or poor outcomes. To illustrate this, we provide some examples of ambiguous research question and hypotheses that result in unclear and weak research objectives in quantitative research ( Table 6 ) 16 and qualitative research ( Table 7 ) 17 , and how to transform these ambiguous research question(s) and hypothesis(es) into clear and good statements.
a These statements were composed for comparison and illustrative purposes only.
b These statements are direct quotes from Higashihara and Horiuchi. 16
a This statement is a direct quote from Shimoda et al. 17
The other statements were composed for comparison and illustrative purposes only.
CONSTRUCTING RESEARCH QUESTIONS AND HYPOTHESES
To construct effective research questions and hypotheses, it is very important to 1) clarify the background and 2) identify the research problem at the outset of the research, within a specific timeframe. 9 Then, 3) review or conduct preliminary research to collect all available knowledge about the possible research questions by studying theories and previous studies. 18 Afterwards, 4) construct research questions to investigate the research problem. Identify variables to be accessed from the research questions 4 and make operational definitions of constructs from the research problem and questions. Thereafter, 5) construct specific deductive or inductive predictions in the form of hypotheses. 4 Finally, 6) state the study aims . This general flow for constructing effective research questions and hypotheses prior to conducting research is shown in Fig. 1 .

Research questions are used more frequently in qualitative research than objectives or hypotheses. 3 These questions seek to discover, understand, explore or describe experiences by asking “What” or “How.” The questions are open-ended to elicit a description rather than to relate variables or compare groups. The questions are continually reviewed, reformulated, and changed during the qualitative study. 3 Research questions are also used more frequently in survey projects than hypotheses in experiments in quantitative research to compare variables and their relationships.
Hypotheses are constructed based on the variables identified and as an if-then statement, following the template, ‘If a specific action is taken, then a certain outcome is expected.’ At this stage, some ideas regarding expectations from the research to be conducted must be drawn. 18 Then, the variables to be manipulated (independent) and influenced (dependent) are defined. 4 Thereafter, the hypothesis is stated and refined, and reproducible data tailored to the hypothesis are identified, collected, and analyzed. 4 The hypotheses must be testable and specific, 18 and should describe the variables and their relationships, the specific group being studied, and the predicted research outcome. 18 Hypotheses construction involves a testable proposition to be deduced from theory, and independent and dependent variables to be separated and measured separately. 3 Therefore, good hypotheses must be based on good research questions constructed at the start of a study or trial. 12
In summary, research questions are constructed after establishing the background of the study. Hypotheses are then developed based on the research questions. Thus, it is crucial to have excellent research questions to generate superior hypotheses. In turn, these would determine the research objectives and the design of the study, and ultimately, the outcome of the research. 12 Algorithms for building research questions and hypotheses are shown in Fig. 2 for quantitative research and in Fig. 3 for qualitative research.

EXAMPLES OF RESEARCH QUESTIONS FROM PUBLISHED ARTICLES
- EXAMPLE 1. Descriptive research question (quantitative research)
- - Presents research variables to be assessed (distinct phenotypes and subphenotypes)
- “BACKGROUND: Since COVID-19 was identified, its clinical and biological heterogeneity has been recognized. Identifying COVID-19 phenotypes might help guide basic, clinical, and translational research efforts.
- RESEARCH QUESTION: Does the clinical spectrum of patients with COVID-19 contain distinct phenotypes and subphenotypes? ” 19
- EXAMPLE 2. Relationship research question (quantitative research)
- - Shows interactions between dependent variable (static postural control) and independent variable (peripheral visual field loss)
- “Background: Integration of visual, vestibular, and proprioceptive sensations contributes to postural control. People with peripheral visual field loss have serious postural instability. However, the directional specificity of postural stability and sensory reweighting caused by gradual peripheral visual field loss remain unclear.
- Research question: What are the effects of peripheral visual field loss on static postural control ?” 20
- EXAMPLE 3. Comparative research question (quantitative research)
- - Clarifies the difference among groups with an outcome variable (patients enrolled in COMPERA with moderate PH or severe PH in COPD) and another group without the outcome variable (patients with idiopathic pulmonary arterial hypertension (IPAH))
- “BACKGROUND: Pulmonary hypertension (PH) in COPD is a poorly investigated clinical condition.
- RESEARCH QUESTION: Which factors determine the outcome of PH in COPD?
- STUDY DESIGN AND METHODS: We analyzed the characteristics and outcome of patients enrolled in the Comparative, Prospective Registry of Newly Initiated Therapies for Pulmonary Hypertension (COMPERA) with moderate or severe PH in COPD as defined during the 6th PH World Symposium who received medical therapy for PH and compared them with patients with idiopathic pulmonary arterial hypertension (IPAH) .” 21
- EXAMPLE 4. Exploratory research question (qualitative research)
- - Explores areas that have not been fully investigated (perspectives of families and children who receive care in clinic-based child obesity treatment) to have a deeper understanding of the research problem
- “Problem: Interventions for children with obesity lead to only modest improvements in BMI and long-term outcomes, and data are limited on the perspectives of families of children with obesity in clinic-based treatment. This scoping review seeks to answer the question: What is known about the perspectives of families and children who receive care in clinic-based child obesity treatment? This review aims to explore the scope of perspectives reported by families of children with obesity who have received individualized outpatient clinic-based obesity treatment.” 22
- EXAMPLE 5. Relationship research question (quantitative research)
- - Defines interactions between dependent variable (use of ankle strategies) and independent variable (changes in muscle tone)
- “Background: To maintain an upright standing posture against external disturbances, the human body mainly employs two types of postural control strategies: “ankle strategy” and “hip strategy.” While it has been reported that the magnitude of the disturbance alters the use of postural control strategies, it has not been elucidated how the level of muscle tone, one of the crucial parameters of bodily function, determines the use of each strategy. We have previously confirmed using forward dynamics simulations of human musculoskeletal models that an increased muscle tone promotes the use of ankle strategies. The objective of the present study was to experimentally evaluate a hypothesis: an increased muscle tone promotes the use of ankle strategies. Research question: Do changes in the muscle tone affect the use of ankle strategies ?” 23
EXAMPLES OF HYPOTHESES IN PUBLISHED ARTICLES
- EXAMPLE 1. Working hypothesis (quantitative research)
- - A hypothesis that is initially accepted for further research to produce a feasible theory
- “As fever may have benefit in shortening the duration of viral illness, it is plausible to hypothesize that the antipyretic efficacy of ibuprofen may be hindering the benefits of a fever response when taken during the early stages of COVID-19 illness .” 24
- “In conclusion, it is plausible to hypothesize that the antipyretic efficacy of ibuprofen may be hindering the benefits of a fever response . The difference in perceived safety of these agents in COVID-19 illness could be related to the more potent efficacy to reduce fever with ibuprofen compared to acetaminophen. Compelling data on the benefit of fever warrant further research and review to determine when to treat or withhold ibuprofen for early stage fever for COVID-19 and other related viral illnesses .” 24
- EXAMPLE 2. Exploratory hypothesis (qualitative research)
- - Explores particular areas deeper to clarify subjective experience and develop a formal hypothesis potentially testable in a future quantitative approach
- “We hypothesized that when thinking about a past experience of help-seeking, a self distancing prompt would cause increased help-seeking intentions and more favorable help-seeking outcome expectations .” 25
- “Conclusion
- Although a priori hypotheses were not supported, further research is warranted as results indicate the potential for using self-distancing approaches to increasing help-seeking among some people with depressive symptomatology.” 25
- EXAMPLE 3. Hypothesis-generating research to establish a framework for hypothesis testing (qualitative research)
- “We hypothesize that compassionate care is beneficial for patients (better outcomes), healthcare systems and payers (lower costs), and healthcare providers (lower burnout). ” 26
- Compassionomics is the branch of knowledge and scientific study of the effects of compassionate healthcare. Our main hypotheses are that compassionate healthcare is beneficial for (1) patients, by improving clinical outcomes, (2) healthcare systems and payers, by supporting financial sustainability, and (3) HCPs, by lowering burnout and promoting resilience and well-being. The purpose of this paper is to establish a scientific framework for testing the hypotheses above . If these hypotheses are confirmed through rigorous research, compassionomics will belong in the science of evidence-based medicine, with major implications for all healthcare domains.” 26
- EXAMPLE 4. Statistical hypothesis (quantitative research)
- - An assumption is made about the relationship among several population characteristics ( gender differences in sociodemographic and clinical characteristics of adults with ADHD ). Validity is tested by statistical experiment or analysis ( chi-square test, Students t-test, and logistic regression analysis)
- “Our research investigated gender differences in sociodemographic and clinical characteristics of adults with ADHD in a Japanese clinical sample. Due to unique Japanese cultural ideals and expectations of women's behavior that are in opposition to ADHD symptoms, we hypothesized that women with ADHD experience more difficulties and present more dysfunctions than men . We tested the following hypotheses: first, women with ADHD have more comorbidities than men with ADHD; second, women with ADHD experience more social hardships than men, such as having less full-time employment and being more likely to be divorced.” 27
- “Statistical Analysis
- ( text omitted ) Between-gender comparisons were made using the chi-squared test for categorical variables and Students t-test for continuous variables…( text omitted ). A logistic regression analysis was performed for employment status, marital status, and comorbidity to evaluate the independent effects of gender on these dependent variables.” 27
EXAMPLES OF HYPOTHESIS AS WRITTEN IN PUBLISHED ARTICLES IN RELATION TO OTHER PARTS
- EXAMPLE 1. Background, hypotheses, and aims are provided
- “Pregnant women need skilled care during pregnancy and childbirth, but that skilled care is often delayed in some countries …( text omitted ). The focused antenatal care (FANC) model of WHO recommends that nurses provide information or counseling to all pregnant women …( text omitted ). Job aids are visual support materials that provide the right kind of information using graphics and words in a simple and yet effective manner. When nurses are not highly trained or have many work details to attend to, these job aids can serve as a content reminder for the nurses and can be used for educating their patients (Jennings, Yebadokpo, Affo, & Agbogbe, 2010) ( text omitted ). Importantly, additional evidence is needed to confirm how job aids can further improve the quality of ANC counseling by health workers in maternal care …( text omitted )” 28
- “ This has led us to hypothesize that the quality of ANC counseling would be better if supported by job aids. Consequently, a better quality of ANC counseling is expected to produce higher levels of awareness concerning the danger signs of pregnancy and a more favorable impression of the caring behavior of nurses .” 28
- “This study aimed to examine the differences in the responses of pregnant women to a job aid-supported intervention during ANC visit in terms of 1) their understanding of the danger signs of pregnancy and 2) their impression of the caring behaviors of nurses to pregnant women in rural Tanzania.” 28
- EXAMPLE 2. Background, hypotheses, and aims are provided
- “We conducted a two-arm randomized controlled trial (RCT) to evaluate and compare changes in salivary cortisol and oxytocin levels of first-time pregnant women between experimental and control groups. The women in the experimental group touched and held an infant for 30 min (experimental intervention protocol), whereas those in the control group watched a DVD movie of an infant (control intervention protocol). The primary outcome was salivary cortisol level and the secondary outcome was salivary oxytocin level.” 29
- “ We hypothesize that at 30 min after touching and holding an infant, the salivary cortisol level will significantly decrease and the salivary oxytocin level will increase in the experimental group compared with the control group .” 29
- EXAMPLE 3. Background, aim, and hypothesis are provided
- “In countries where the maternal mortality ratio remains high, antenatal education to increase Birth Preparedness and Complication Readiness (BPCR) is considered one of the top priorities [1]. BPCR includes birth plans during the antenatal period, such as the birthplace, birth attendant, transportation, health facility for complications, expenses, and birth materials, as well as family coordination to achieve such birth plans. In Tanzania, although increasing, only about half of all pregnant women attend an antenatal clinic more than four times [4]. Moreover, the information provided during antenatal care (ANC) is insufficient. In the resource-poor settings, antenatal group education is a potential approach because of the limited time for individual counseling at antenatal clinics.” 30
- “This study aimed to evaluate an antenatal group education program among pregnant women and their families with respect to birth-preparedness and maternal and infant outcomes in rural villages of Tanzania.” 30
- “ The study hypothesis was if Tanzanian pregnant women and their families received a family-oriented antenatal group education, they would (1) have a higher level of BPCR, (2) attend antenatal clinic four or more times, (3) give birth in a health facility, (4) have less complications of women at birth, and (5) have less complications and deaths of infants than those who did not receive the education .” 30
Research questions and hypotheses are crucial components to any type of research, whether quantitative or qualitative. These questions should be developed at the very beginning of the study. Excellent research questions lead to superior hypotheses, which, like a compass, set the direction of research, and can often determine the successful conduct of the study. Many research studies have floundered because the development of research questions and subsequent hypotheses was not given the thought and meticulous attention needed. The development of research questions and hypotheses is an iterative process based on extensive knowledge of the literature and insightful grasp of the knowledge gap. Focused, concise, and specific research questions provide a strong foundation for constructing hypotheses which serve as formal predictions about the research outcomes. Research questions and hypotheses are crucial elements of research that should not be overlooked. They should be carefully thought of and constructed when planning research. This avoids unethical studies and poor outcomes by defining well-founded objectives that determine the design, course, and outcome of the study.
Disclosure: The authors have no potential conflicts of interest to disclose.
Author Contributions:
- Conceptualization: Barroga E, Matanguihan GJ.
- Methodology: Barroga E, Matanguihan GJ.
- Writing - original draft: Barroga E, Matanguihan GJ.
- Writing - review & editing: Barroga E, Matanguihan GJ.
What is Data in Education? The Ultimate Guide
Data is everywhere in education today. From classroom assessments to school-wide evaluations, data plays a crucial role in shaping educational practices and policies. In this complete guide, we’ll explore what data in education is, how it’s evolved over time, the different types of data used, essential tools for data collection and analysis, and round off with some future trends and predictions for data in the field of education.
Let’s get started!
- What is Data in Education?
- How Has Data in Education Evolved?
- Types of Data in Education: Qualitative vs. Quantitative
- Tools for Data Collection and Analysis
- Ethical Considerations for Handling Data in Education
- Trends and Predictions for the Future of Data in Education
1. What is Data in Education?
At its core, data in education refers to the collection, analysis, and interpretation of information to make informed decisions and improve educational outcomes. It encompasses a vast range of information, including student performance, attendance records, demographics, curriculum content, and more. Data helps educators identify patterns and trends, evaluate the effectiveness of instructional strategies, and monitor student progress.
The ultimate goal of using data in education is to enhance teaching and learning experiences, personalize instruction, and provide meaningful insights to support evidence-based decision-making. To learn more, we’ve written an in-depth blog post exploring why data is so important in education .
2. How Has Data in Education Evolved?
Data in education has come a long way from traditional paper-based records and manual calculations. In the past, educators relied heavily on subjective observations and standardized assessments to make instructional decisions. Today, digital tools and learning management systems provide real-time data, allowing educators to instantly identify areas of strength and weakness, tailor instruction to individual needs, and adjust teaching strategies accordingly.
One of the key advancements in data collection and analysis in education is the use of learning analytics. Learning analytics is the measurement, collection, analysis, and reporting of data about learners and their contexts, for the purposes of understanding and optimizing learning and the environments in which it occurs. This approach allows educators to gain insights into student behavior, engagement, and performance, enabling them to make data-driven decisions to improve learning outcomes.
Another significant development in data-driven education is the emergence of data dashboards and visualizations. These tools provide educators with a visual representation of data, making it easier to interpret and understand complex information. Data dashboards can display various metrics, such as student progress, assessment results, and attendance rates, allowing educators to quickly identify areas of concern and take appropriate action.
Read next: How data analytics is reshaping the education industry
3. Types of Data in Education: Qualitative vs. Quantitative
There are two overarching types of data in education : Qualitative and quantitative data. While both types are essential for gaining a comprehensive understanding of educational practices, they offer different perspectives and insights.
Qualitative Data
Qualitative data in education provides rich, descriptive information about educational experiences, beliefs, attitudes, and behaviors. It is typically collected through methods such as interviews, observations, focus groups, and open-ended surveys. Qualitative data allows for in-depth exploration of complex educational phenomena, offering a holistic view of students’ experiences and perspectives.
For example, through interviews with students, researchers can gain insights into their motivations, aspirations, and challenges. These interviews can uncover valuable information about the factors that influence student engagement and academic success. Observations in classrooms can provide researchers with a deeper understanding of teaching practices, classroom dynamics, and student interactions. By immersing themselves in the educational environment, researchers can capture the nuances and complexities that quantitative data alone may not reveal.
Read next: 5 examples of qualitative data in education
Quantitative Data
Quantitative data in education , on the other hand, focuses on numerical information and measurable outcomes. It includes data points such as test scores, attendance records, and demographic information. Quantitative data is collected using surveys, standardized assessments, and statistical analyses. It provides objective measurements and trends, enabling educators to track progress, identify achievement gaps, and evaluate the effectiveness of educational interventions.
For instance, by analyzing test scores, educators can identify areas of strength and weakness in students’ academic performance. They can use this information to tailor instruction and provide targeted support to students who may be struggling. Attendance records can help identify patterns of absenteeism and inform strategies to improve student attendance rates. Demographic data, such as gender, ethnicity, and socioeconomic status, can provide insights into disparities in educational outcomes and guide efforts to promote equity and inclusivity . To learn more, check out our roundup of examples of quantitative data in education .
4. Tools for Data Collection and Analysis
Collecting and analyzing data in education requires effective tools and technologies. These generally fall into three categories:
- Learning Management Systems (LMS): LMS platforms allow educators to track student progress, deliver online assessments, and gather data on student engagement and performance. They provide a centralized hub for managing educational resources, tracking learning outcomes, and facilitating data-driven decision-making. These can also include student data management systems .
- Data Visualization Tools: Data visualization tools like charts, graphs, and dashboards make it easier to interpret complex data sets. Tools like Inno™ Starter Kits transform raw data into visual representations that are easy to understand, enabling educators to identify trends, patterns, and outliers at a glance.
- Analytical Software: Analytical software, like statistical analysis tools and predictive modeling software, helps educators make sense of large volumes of data. These tools use algorithms and data mining techniques to identify correlations, predict outcomes, and generate actionable insights.
5. Ethical Considerations for Handling Data in Education
While data can significantly enhance educational practices, its collection and use must be done ethically and responsibly. Safeguarding the privacy and confidentiality of students and educators is of utmost importance. Here are some ethical considerations to keep in mind:
- Student Data Security : Implement robust security measures to protect sensitive data from unauthorized access or breaches.
- Informed Consent: Obtain informed consent from students or their guardians before collecting any personally identifiable information.
- Anonymization: Ensure that data is sufficiently anonymized and de-identified to protect individuals’ privacy.
- Data Ownership: Clarify who owns and has access to the collected data, ensuring transparency and accountability.
- Data Retention: Establish clear policies on data retention and disposal to avoid unnecessary data storage and potential privacy risks.
6. Trends and Predictions for the Future of Data in Education
The field of data in education continues to evolve and hold great promise for improving educational outcomes. Here are some key trends and predictions for the future:
- Personalized Learning: Data-driven insights will play a vital role in tailoring instruction to individual students’ needs, preferences, and learning styles.
- Predictive Analytics: Predictive modeling and machine learning algorithms will help identify early warning signs and intervene proactively to prevent potential learning difficulties.
- Big Data Analytics: Advanced data analytics techniques will enable educators to gain deeper insights by analyzing vast amounts of complex educational data.
- Artificial Intelligence: AI-powered adaptive learning systems will provide personalized learning experiences driven by real-time data analysis and student performance.
- Ethical Data Use: Ethical considerations and responsible data practices will become more prominent, ensuring data privacy, equity, and inclusivity in educational decision-making.
Final Thoughts
Data has revolutionized teaching and learning. It empowers educators with invaluable insights, fuels evidence-based decision-making, and supercharges educational practices. By unlocking the true value of data in education, educators can create a profound impact on students’ lives and drive remarkable educational outcomes.
Having delved into the world of educational data, now it’s time to equip yourself with tools that make this knowledge actionable. Inno™ Starter Kits are purposefully crafted to bridge the gap between understanding and application, ensuring educators have a seamless journey in the realm of educational data. If you’re ready to take the next step and harness the power of data-driven insights in real-time, explore the Inno™ Starter Kits and elevate your educational strategies to the next level.
Thank you for sharing!
You may also be interested in
Why interactive data visualization is the key to better student outcomes.
Discover the power of interactive data visualization for student data with this in-depth guide.
by Innovare | Dec 4, 2023 | Data in K-12 Schools
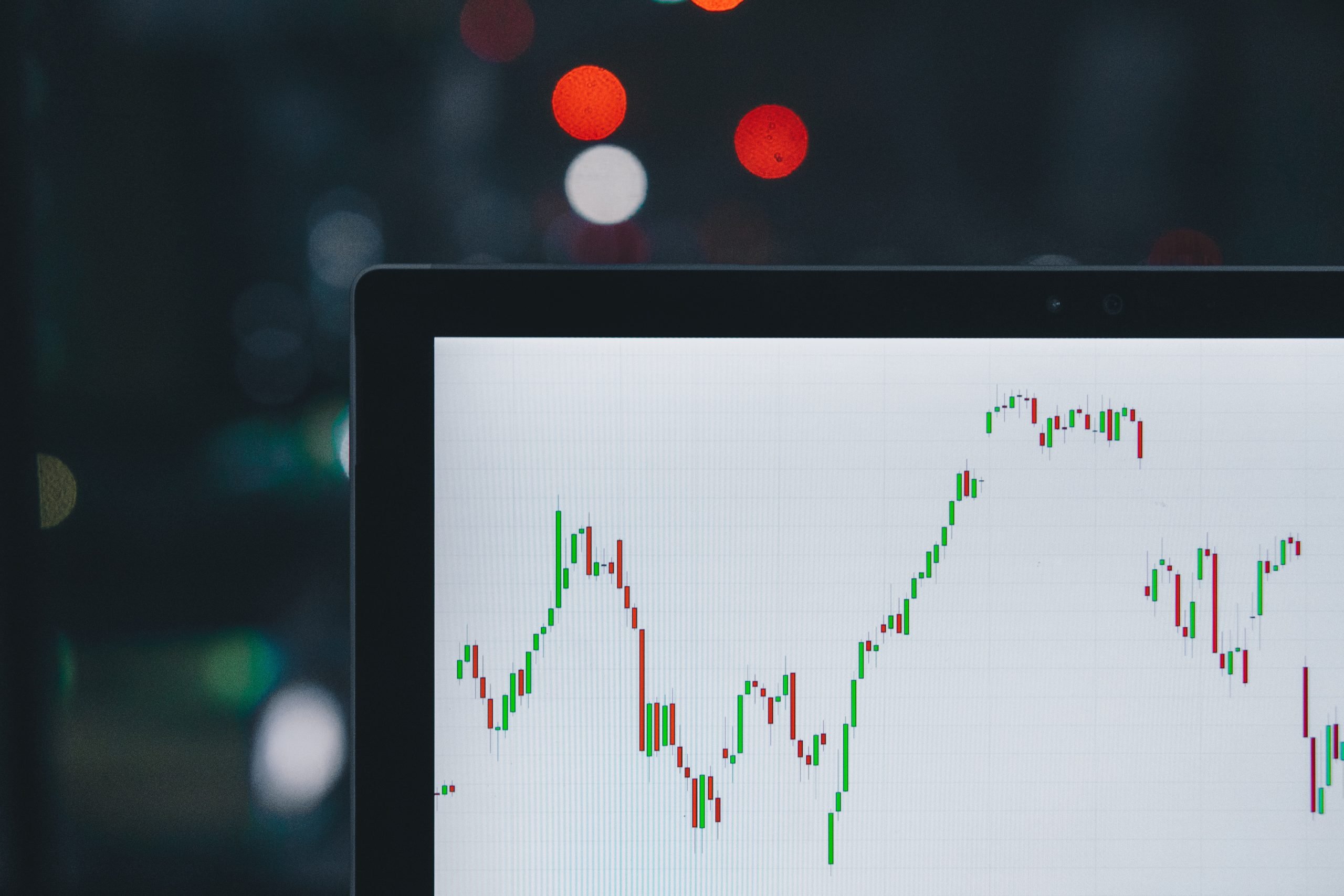
Data in K-12 Schools
Why is Data Important in Education? A 2023 Educators’ Guide
Discover the crucial role of data in education and how it empowers educators in our comprehensive guide.
by Innovare | Sep 18, 2023 | Data in K-12 Schools

This website uses cookies to improve your experience. See our Privacy Policy to learn more. Accept
- Skip to main content
- Skip to primary sidebar
- Skip to footer
- QuestionPro

- Solutions Industries Gaming Automotive Sports and events Education Government Travel & Hospitality Financial Services Healthcare Cannabis Technology Use Case NPS+ Communities Audience Contactless surveys Mobile LivePolls Member Experience GDPR Positive People Science 360 Feedback Surveys
- Resources Blog eBooks Survey Templates Case Studies Training Help center

Home Surveys Academic
Examples of Qualitative Data in Education: How to Use
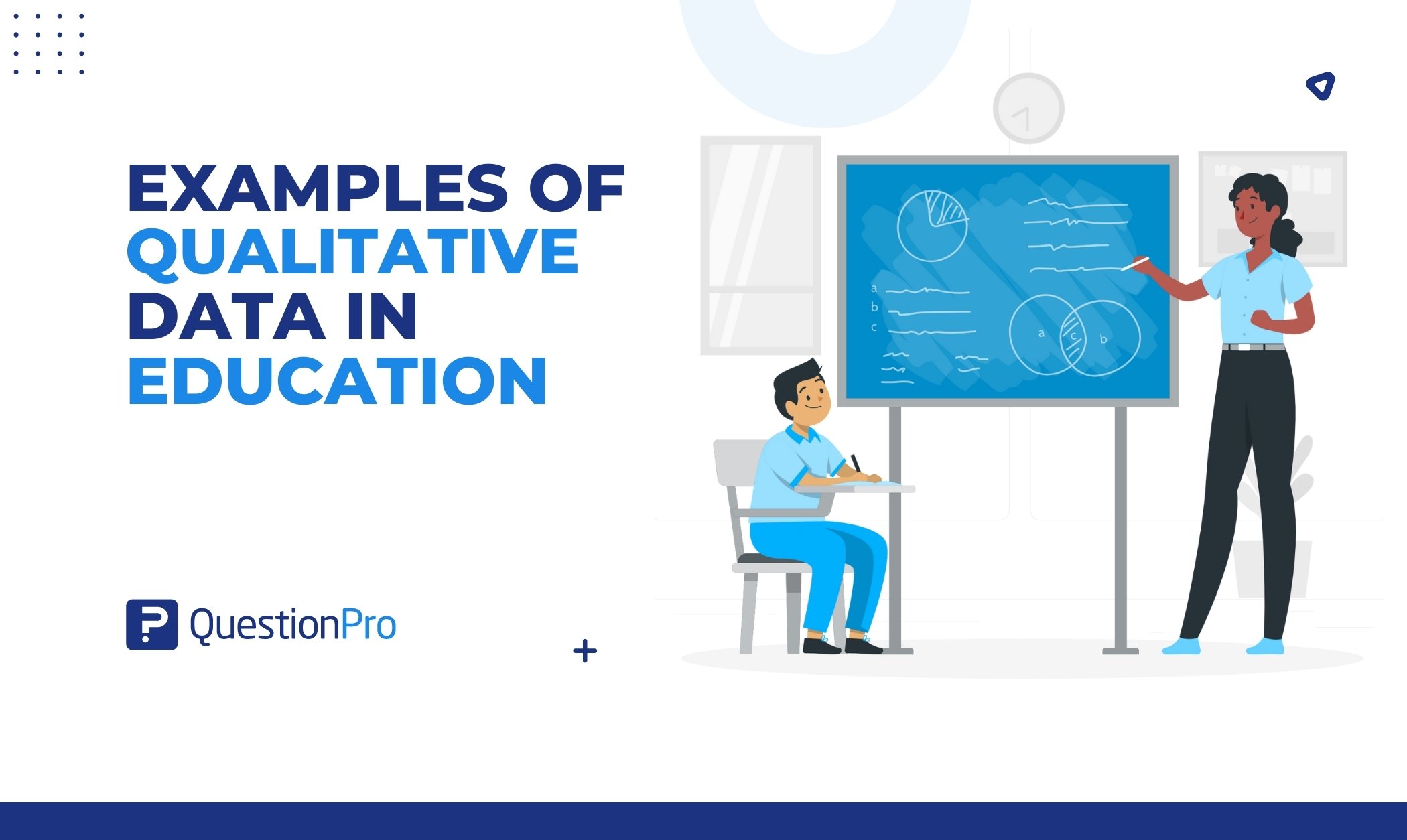
The phrase “Examples of Qualitative Data in Education” reveals rich and detailed knowledge in the field of education, where understanding fuels creativity and insights drive advancement. While quantitative data frequently grabs the spotlight, its qualitative counterpart is just as important for understanding how complicated learning environments work.
In this blog, we will delve into the significance of qualitative data in education and provide a range of examples showcasing its applications in fostering educational excellence.
What is qualitative data?
Qualitative data is a type of data that is open to interpretation and can be used in a variety of ways- both as a measure of quality and as the basis for analysis.
It describes the way things are and tells you why something is happening, rather than what is happening (for example, if a student isn’t doing well in math, qualitative data would tell you their reasons for this), rather than describing its characteristics or how much of it there is.
Qualitative data is not numerical and does not have a set meaning, which makes it difficult to analyze. Understanding how to use qualitative data collection in education effectively can be crucial for educational institutions.
LEARN ABOUT: Qualitative Interview
Using qualitative data in educational settings
Qualitative data provides insight into the learning experience that cannot always be expressed through numbers. It allows you to better understand how students learn by asking open-ended questions and listening carefully to their answers.
When you use qualitative data, you can investigate particular areas of concern for your organization and formulate action plans as needed. Also, qualitative data addresses a number of the shortcomings of quantitative research.
For instance, quantitative data can indicate that a particular school district’s test scores have outperformed those of other regional school districts but cannot explain why this is the case.
Examples of qualitative data in education
Here are some examples of qualitative data in education:
Field observations
Teachers and administrators could observe the classroom during different times of day, at different points during the year, or when a special event is happening.
Documentary research
School organizations can spend time looking closely at their current documents to learn more about students.
Focus groups
Conducting focus group discussions with students, teachers, or parents can provide qualitative insights into their perceptions, experiences, and opinions related to educational practices and policies.
Student portfolios
Reviewing student portfolios that showcase their work, assignments, and projects over time offers qualitative data on their progress, growth, and learning journey.
Peer review and feedback
Encouraging students to provide peer reviews and feedback on each other’s work generates qualitative data on their ability to critically assess and provide constructive input.
Learning diaries
Similar to journals, learning diaries encourage students to document their daily experiences, challenges, and triumphs, offering qualitative insights into their engagement and progress.
Parent-teacher conferences
Conversations during parent-teacher conferences provide qualitative data about a student’s strengths, weaknesses, and overall development from both home and school perspectives.
Online discussion forums
Analyzing interactions on online platforms where students and educators discuss topics related to coursework offers qualitative insights into their understanding, questions, and collaboration and is one of the best examples of qualitative data in education.
Classroom artifacts
Examining classroom artifacts like bulletin boards, student artwork, and project displays provides qualitative data on the learning environment, student creativity, and the integration of various subjects.
Audio and video recordings
Recording classroom discussions, presentations, or group activities captures qualitative data on communication skills, collaboration, and the depth of student understanding.
Student surveys with open-ended questions
Incorporating open-ended questions into student surveys enables them to express their thoughts, opinions, and suggestions in their own words, yielding qualitative data that complements quantitative results.
Teacher reflective journals
Teachers maintaining reflective journals about their teaching experiences, challenges, and innovative approaches generate qualitative data on professional growth and instructional strategies.
Student interviews
One-on-one interviews with students are one of the most common examples of qualitative data in education. It can provide qualitative insights into their learning experiences, interests, and motivations, helping educators tailor instruction to individual needs.
How can a survey tool help with qualitative data analysis in education?
A survey tool is a useful research tool that can help with qualitative data analysis in education. Qualitative data is best analyzed through close inspection and asking questions to understand the root causes of phenomena, but this is a time-consuming process.
A well-designed survey questionnaire can simplify the qualitative analysis by giving you insight into what most concerns your group and helping you to prioritize your responses.
Key steps to using a survey tool: In order to successfully use a survey tool, you’ll need to:
Define your goal
What are you trying to accomplish? If you don’t know where you want the findings of your Qualitative research project to lead, it will be difficult for people to provide feedback and difficult for you to analyze the results.
Choose your qualitative research method
What are your options? How will people be invited to give feedback, and where will this feedback come from? Identifying how participants/respondents/users will be asked about their experiences is an important first step.
Design survey questions
Craft thoughtful and relevant survey questions that align with your research goals. Ensure a mix of closed-ended questions to gather quantitative data and open-ended questions to gather qualitative insights.
Use clear and concise language to avoid ambiguity, and consider using skip logic or branching to tailor the survey experience based on participants’ responses. Well-designed questions will make the data analysis process smoother.
Distribute and collect responses
Utilize the survey tool to distribute the survey to your target audience, whether it’s students, teachers, parents, or administrators. You can use various distribution channels such as email, social media, or school websites.
Track the responses as they come in and monitor the data collection process. Keep the survey open for an appropriate amount of time to ensure a diverse range of responses.
Analyze qualitative data
Once you’ve collected a sufficient number of responses, begin the qualitative data analysis process. Start by categorizing and coding open-ended responses. Look for recurring themes, patterns, and trends within the qualitative data.
You can use tools like thematic analysis to identify key themes that emerge from participants’ responses. Software like NVivo or even Excel can help organize and analyze qualitative data effectively.
Triangulate with quantitative data
If your survey included closed-ended questions with quantitative responses (e.g., Likert scales), you can enrich your analysis by comparing qualitative insights with quantitative data. This triangulation can provide a more comprehensive understanding of the research topic.
For example, if participants express negative sentiments about a particular aspect of education, you can cross-reference this sentiment with the corresponding quantitative rating to see if there’s a correlation.
LEARN ABOUT: Steps in Qualitative Research
Methods to analyze qualitative data
Qualitative research methods form the cornerstone of understanding human experiences, utilizing an array of data collection methods to delve into the nuances of perspectives. From interviews and focus groups to ethnographic studies and content analysis, these qualitative methods harmonize to reveal the intricate tapestry of human narratives.
Now, we will unveil how these methods converge, creating a symphony of insights that deepen our comprehension of the human condition.
Content analysis
The content of the data is analyzed by scrutinizing and interpreting texts, pictures, video, audio, and other materials. This involves looking at the words in a document, for example, and deciding their meaning.
Grounded theory
To create a grounded theory, you study what is happening in a particular situation and try to formulate a theory about why it happens. This process often begins with an initial assumption or question, which will be tested out over time. For example: “How do we know when this analysis process is finished?”
Phenomenology
Phenomenology looks at experiences from the perspective of those who experience them. It tries to understand what these experiences mean to people rather than the events themselves. This is relevant for understanding students’ learning experiences in an educational setting.
Framework analysis
Framework analysis is a conversation with participants and then using the content of that discussion to analyze the data. It could involve asking individuals, “What was the knowledge you gained from this project?” and then anonymizing their answers in order to avoid starting your article with personal stories.
Discourse analysis
Discourse analysis looks at how individuals use language and what the implications of those uses are. This can be helpful in a classroom setting where students use their voices to express themselves about their learning process within the walls of academia.
Interpretative phenomenological analysis
Interpretive phenomenological analysis (IPA) helps you understand that qualitative data is a type of open-ended and interpretable data that can be used in various ways. Whether you’re trying to learn more about your customer’s experiences or the educational process, qualitative analysis will help get insights into what’s important for your project.
Learn more about academic surveys here
Learn more about academic research services for students here
Qualitative data in education is a treasure trove of insights that empowers educators and administrators to create holistic learning experiences.
By leveraging methods such as interviews, observations, and reflections, educational institutions can gain a deeper understanding of student needs, teaching strategies, and program effectiveness. The fusion of quantitative and qualitative data enriches decision-making processes and paves the way for continuous improvement in education.
If you want help analyzing the qualitative aspects of your research projects, we’re here to provide assistance with our survey tool ! Let us know if there are any other information needs, and we’ll work on providing an answer as soon as possible.
FREE TRIAL LEARN MORE
MORE LIKE THIS
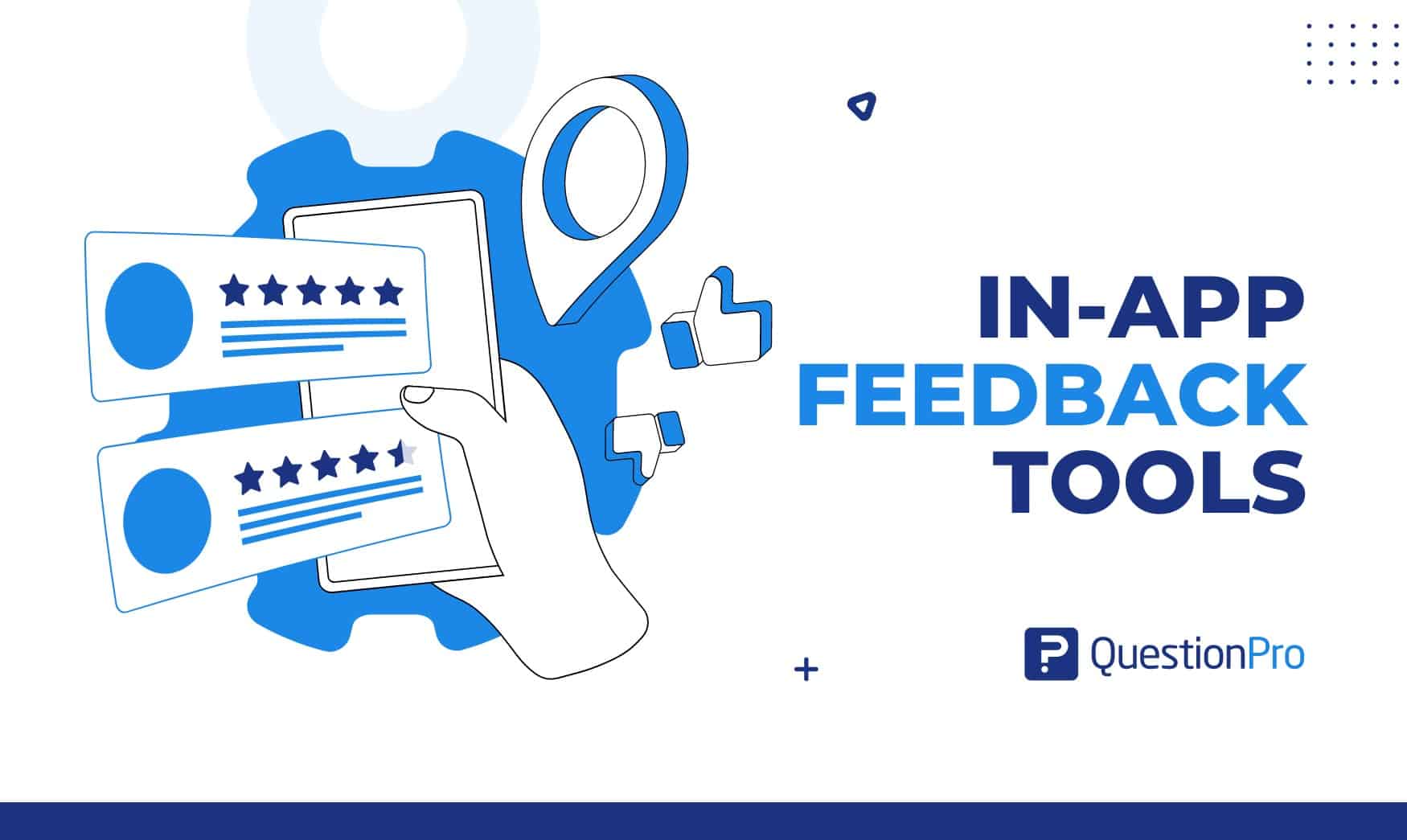
In-App Feedback Tools: How to Collect, Uses & 14 Best Tools
Mar 29, 2024
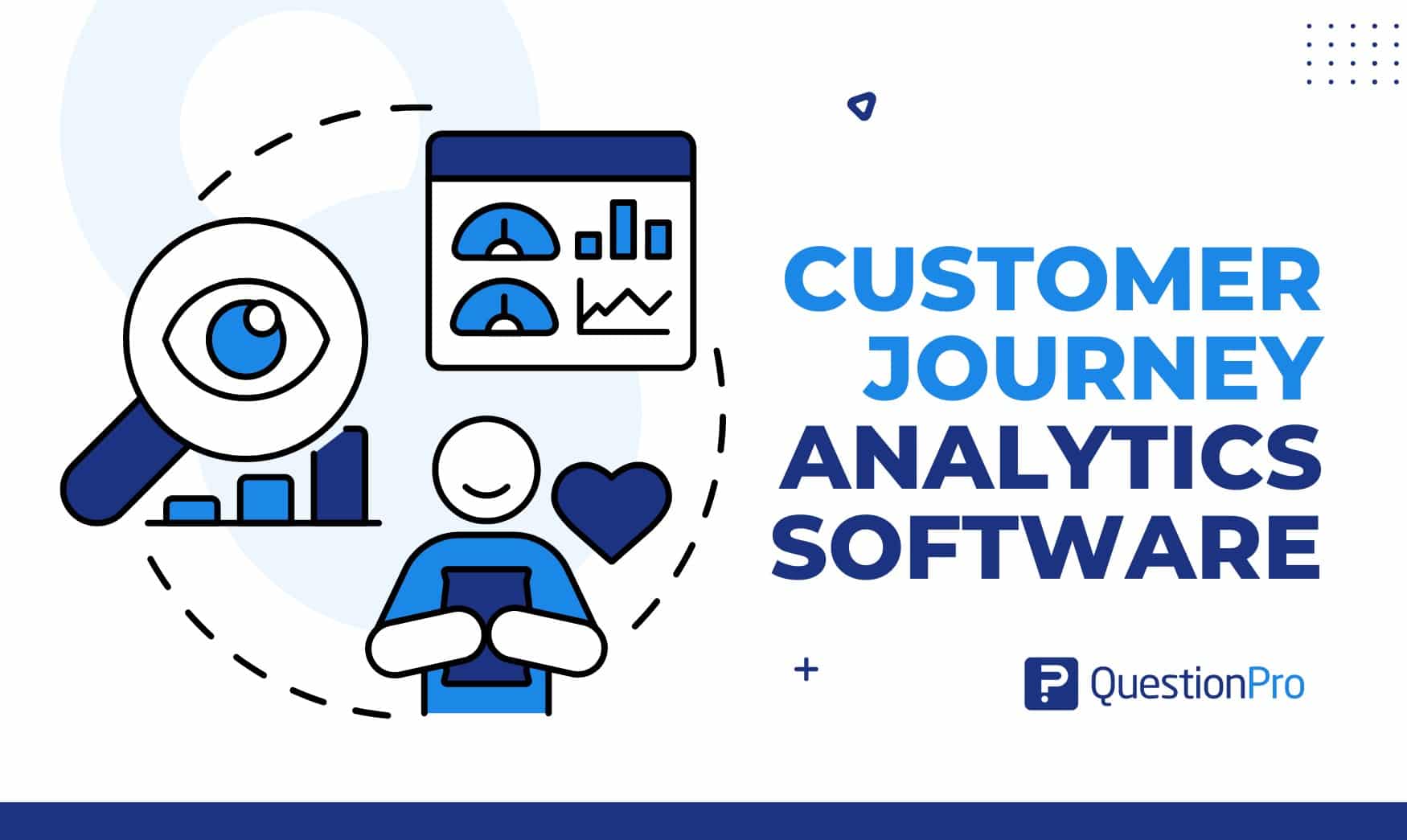
11 Best Customer Journey Analytics Software in 2024
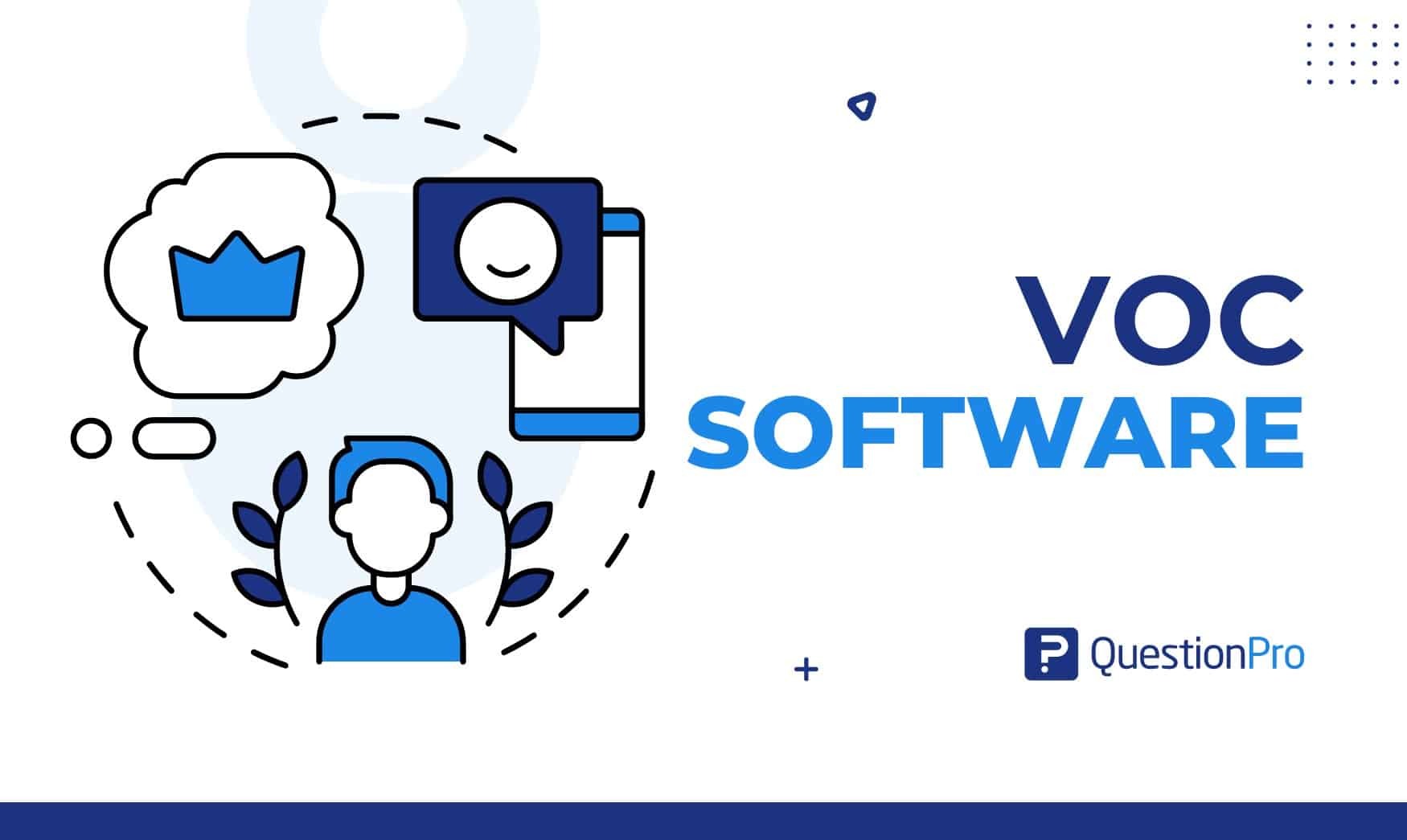
17 Best VOC Software for Customer Experience in 2024
Mar 28, 2024
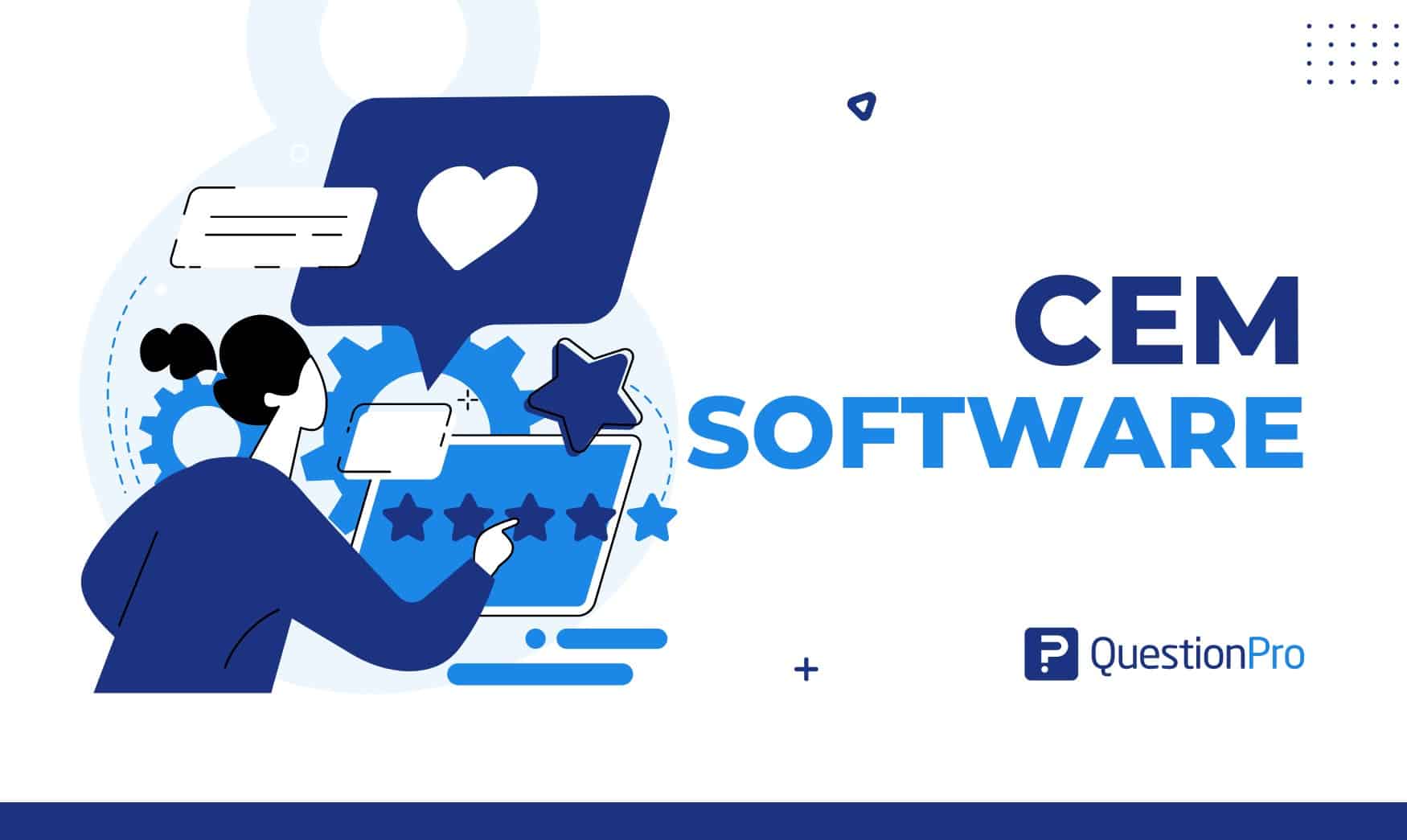
CEM Software: What it is, 7 Best CEM Software in 2024
Other categories.
- Academic Research
- Artificial Intelligence
- Assessments
- Brand Awareness
- Case Studies
- Communities
- Consumer Insights
- Customer effort score
- Customer Engagement
- Customer Experience
- Customer Loyalty
- Customer Research
- Customer Satisfaction
- Employee Benefits
- Employee Engagement
- Employee Retention
- Friday Five
- General Data Protection Regulation
- Insights Hub
- Life@QuestionPro
- Market Research
- Mobile diaries
- Mobile Surveys
- New Features
- Online Communities
- Question Types
- Questionnaire
- QuestionPro Products
- Release Notes
- Research Tools and Apps
- Revenue at Risk
- Survey Templates
- Training Tips
- Uncategorized
- Video Learning Series
- What’s Coming Up
- Workforce Intelligence
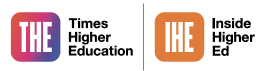
The what, why and how of data visualisation for higher education
Wondering how to get started with data visualisation? Andrew Moles lays out the basics and offers his advice
.jpg?h=d72ff27f&itok=Pjj75seA)
Andrew Moles
Additional links.
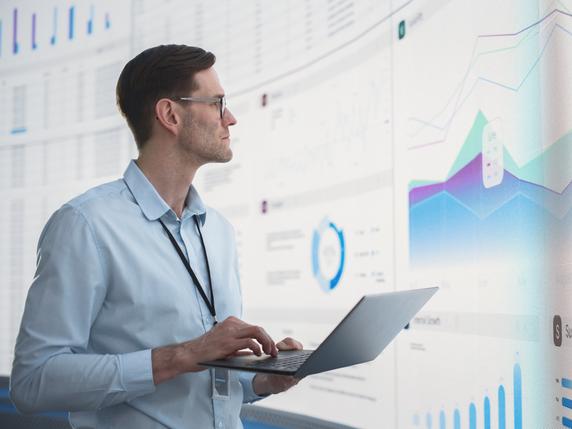
Created in partnership with
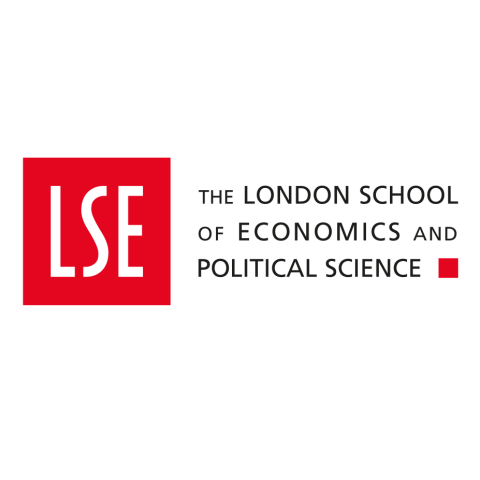
You may also like
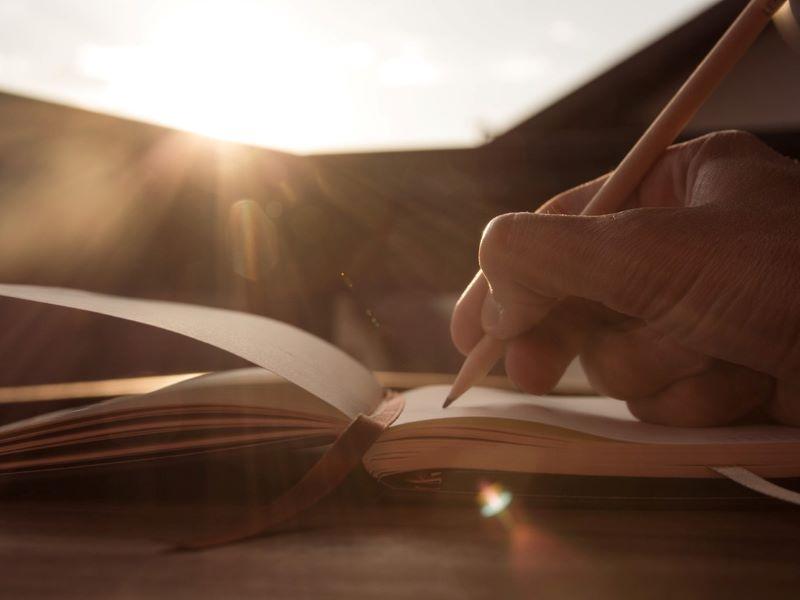
Popular resources
.css-1txxx8u{overflow:hidden;max-height:81px;text-indent:0px;} Emotions and learning: what role do emotions play in how and why students learn?
A diy guide to starting your own journal, universities, ai and the common good, artificial intelligence and academic integrity: striking a balance, create an onboarding programme for neurodivergent students.
Visualising data can help students and staff in higher education get useful insights into otherwise opaque data sets – impacting decision-making, improving understanding when teaching and effectively communicating research findings both within and outside of academia. Here, I’ll take a tour of data visualisation and offer my tips to help you get started.
What is data visualisation?
In the simplest terms, data visualisation is the act of conveying information from data as a visual representation. You might be asking yourself: “What does that even mean?” I’m being vague because data visualisation comes in so many different forms and styles, with a lot of jargon attached. To get a feel for different styles, take a look at the visualisations people have made for the Information is Beautiful Awards .
Why is data visualisation important?
Data visualisation makes it much easier to get the information you need or to tell others about the insights you have found. A classic example from the 1850s is when Florence Nightingale used data visualisation to convey that poor sanitary conditions were causing high mortality in hospitals. The visual provided a clear message that change was needed.
- How data from digital learning tools can refine teaching
- DIY learning analytics: using data to improve online teaching
- Collecting data on our students is the only way forward
At LSE, we often use data visualisation to explain and explore research findings or illustrate student journeys , for example. From a more statistical standpoint, data visualisation allows you to see trends in your data that would otherwise be invisible. An excellent example of this can be seen in this article by Justin Matejka and George Fitzmaurice, where several datasets with the same statistics, such as averages, result in very different-looking visuals.
This all sounds great, but we should still think critically about what we are seeing! Charts can be misleading, demonstrated by this article by Statistics How To . The New York Times has a great resource to help here called What’s Going on in This Graph ?, which I recommend taking a look at.
How do I make all these exciting graphics?
There are four things you need to get started: a data visualisation tool, some data, an idea of what visualisation to make and a can-do attitude. If you’ve not done much data work before, Excel or Google Sheets are good tools to start with, depending on what you have access to – but there are many tools to pick from.
Getting yourself some data to play around with is relatively straightforward, as many datasets are available online. I’ve been collating my favourite sources over the years, which you can find on my GitHub . Finally, knowing what visual to make can be overwhelming when there are so many options. I recommend From Data to Viz to ease this process, which provides interactive maps of what visual to use based on your data.
It’s important to note that when seeing some excellent data visualisations, like this example from Our World in Data , or static and infographic examples from LSE, you might think: “Wow, those are great, but how easy is it to do that?” I won’t lie, it is hard to get to that level. But that doesn’t mean you can’t create some valuable visuals. Have fun and make good and useful stuff!
Let’s talk tools
There are a lot of different options when it comes to tools. To help, I’ve provided a (non-exhaustive) list and categorised them by non-coding and coding tools. Coding tools require you to write some programming syntax to make a visualisation.
- Excel: most higher education institutions have access to this
- Google Sheets: you’ll get this if you have a Google account
- Tableau: it’s costly but has a free version called Tableau Public
- Power BI: currently cheaper than Tableau, and has a free public version . But be warned, Power BI only works on Windows.
- RAWGraphs : a non-coding visualisation tool without any cost
- Datawrapper : a lightweight free version is available, with other paid models
- Flourish : similar set-up to Datawrapper
Coding, all of which you can use without cost
- R using ggplot2 or plotly . R is the easiest of the coding tools to use for visualisation.
- Python has many visualisation libraries. Matplotlib and Seaborn are popular, as is plotly , but I find Lets Plot the best.
- JavaScript using the D3 , React , highcharts (also available in R) or Observable libraries.
My advice here is to try out several tools and see what sticks. Trying out programming languages like R, Python or JavaScript is more of a learning endeavour but worth it if you get serious about data work.
Data visualisation and being able to think critically about visualisations you see in the wild is a useful skill – not just in higher education, but in many aspects of life. Hopefully reading this has given you a taste of the use and potential it offers.
But let’s remember that data visualisation isn’t just about charts – it is about design and creativity, which I think makes it more fun! Even so, when getting started it can be helpful to follow some general guidelines and get a bit of theory too. I would recommend Claus Wilke’s book Fundamentals of Data Visualisation .
Look for inspiration or a project that is interesting to you because this will give you the drive to keep learning – and hopefully enjoy yourself along the way.
Andrew Moles is learning developer for data science tools at the London School of Economics and Political Science.
If you would like advice and insight from academics and university staff delivered direct to your inbox each week, sign up for the Campus newsletter .
- A good place to start to learn R is the R for Data Science book .
- My favourite book for learning Python for data work is the Python for Data Science book .
- For a nice community try joining, for free, the Data Visualisation Society .
Emotions and learning: what role do emotions play in how and why students learn?
Global perspectives: navigating challenges in higher education across borders, how to help young women see themselves as coders, contextual learning: linking learning to the real world, authentic assessment in higher education and the role of digital creative technologies, how hard can it be testing ai detection tools.
Register for free
and unlock a host of features on the THE site
- Open access
- Published: 26 March 2024
Incorporating a situational judgement test in residency selections: clinical, educational and organizational outcomes
- Anurag Saxena ORCID: orcid.org/0000-0002-9375-9790 1 ,
- Loni Desanghere 1 ,
- Kelly Dore 2 , 3 &
- Harold Reiter 4
BMC Medical Education volume 24 , Article number: 339 ( 2024 ) Cite this article
41 Accesses
Metrics details
Computer-based assessment for sampling personal characteristics (Casper), an online situational judgement test, is a broad measure of personal and professional qualities. We examined the impact of Casper in the residency selection process on professionalism concerns, learning interventions and resource utilization at an institution.
In 2022, admissions data and information in the files of residents in difficulty (over three years pre- and post- Casper implementation) was used to determine the number of residents in difficulty, CanMEDS roles requiring a learning intervention, types of learning interventions (informal learning plans vs. formal remediation or probation), and impact on the utilization of institutional resource (costs and time). Professionalism concerns were mapped to the 4I domains of a professionalism framework, and their severity was considered in mild, moderate, and major categories. Descriptive statistics and between group comparisons were used for quantitative data.
In the pre- and post- Casper cohorts the number of residents in difficulty (16 vs. 15) and the number of learning interventions (18 vs. 16) were similar. Professionalism concerns as an outcome measure decreased by 35% from 12/16 to 6/15 ( p < 0.05), were reduced in all 4I domains (involvement, integrity, interaction, introspection) and in their severity. Formal learning interventions (15 vs. 5) and informal learning plans (3 vs. 11) were significantly different in the pre- and post-Casper cohorts respectively ( p < 0.05). This reduction in formal learning interventions was associated with a 96% reduction in costs f(rom hundreds to tens of thousands of dollars and a reduction in time for learning interventions (from years to months).
Conclusions
Justifiable from multiple stakeholder perspectives, use of an SJT (Casper) improves a clinical performance measure (professionalism concerns) and permits the institution to redirect its limited resources (cost savings and time) to enhance institutional endeavors and improve learner well-being and quality of programs.
Peer Review reports
Admission into medical school and residency training programs is a high-stakes process as it affects the careers and well-being of the individuals who apply, care and health of the patients and communities, and the quality, costs and reputation of the institutions involved in medical education. Selections into medicine are aimed at identifying candidates with desirable attributes and deselecting those who are unlikely to be successful in that specialty or serve the society as physicians [ 1 ]. Further, there are many considerations that play a significant role in the complex process of selections. These include perspectives of different stakeholders that may not always be aligned [ 2 , 3 ] and sometimes be in conflict. These include societal considerations that underlie the move towards a diverse workforce to meet culturally aligned healthcare needs of population and an equitable representation of societal segments in medicine. The applicants desire a process that is transparent, less expensive, maximizes their chances for selection into a specialty of their choice, and assesses those aspects over which they have some control. Institutions are motivated by their vision and mission including the social accountability mandate, differentiation, scope of influence, quality of education, selection of best possible candidates, reputation, operational efficiency, and resource utilization.
Selection processes into medical schools and postgraduate residency programs involve assessment of both cognitive abilities (CAs) and non-cognitive abilities (NCAs). The cognitive abilities (CAs) refer to general intelligence and learned knowledge [ 4 ] and the non-cognitive abilities (NCAs), sometimes referred to as socio-emotional skills [ 5 ], personal and professional characteristics [ 6 ], personal qualities [ 7 ], non-academic attributes [ 8 ], soft skills, or character skills are rather broad and include mindsets, attitudes, integrity, personality traits, learning strategies and social skills including communication and empathy. The CAs and NCAs are not mutually exclusive [ 9 ], are somewhat overlapping constructs [ 10 ] and both are required for effective practice of medicine [ 11 ]. The NCAs are considered essential for good physicians [ 12 ], better patient outcomes [ 13 , 14 ], and underlie excellence by residents and physicians [ 15 , 16 ].
Selection processes consider CAs and NCAs along with prior achievements, expression of interests and other factors in a somewhat structured and deliberate but quite variable manner, through a multitude of tools (e.g., interviews, computerized tests, personal letters, references, and others) aimed at identifying candidates with the highest likelihood of success. The importance of reliable tools [ 17 ] and assessment of wide-ranging attributes [ 18 ], is well-accepted. CAs are assessed using prior academic performance and tests of medical knowledge and weighted variably along with the assessment of NCAs in the processes to make final decisions [ 18 ].
The assessment of NCAs is considered worthwhile [ 19 , 20 , 21 ]. Indeed, a few studies have shown the relevance of personal characteristics to future success, including clinical performance and professionalism lapses [ 22 ] and overall competency [ 23 ]. Even more relevant is the demonstration of incremental validity of NCA assessment over and above academic assessment [ 22 , 24 ]. Assessment of NCAs is highly variable and largely suboptimal [ 1 ], except when utilized through a validated process e.g., structured personal essays [ 25 ], multiple-mini interviews (MMIs) [ 26 ], or online situational judgment tests (SJTs) [ 27 ].
Computer-based assessment for sampling personal characteristics (Casper) is an online SJT that measures personal and professional qualities (computer-based video generated written response in an open-ended manner) in a contextualized manner and is devoid of medical knowledge assessment [ 28 ]. Contextualized assessment is essential since context is important for behavioral manifestations [ 29 ]. Casper has been used in selections in medical education settings [ 30 , 31 ]. Casper has been generally used in undergraduate medical school admissions and only more recently for selections in postgraduate residency programs. It is used for assessment of NCAs e.g., receptivity to feedback empathy, teamwork, communication, collaboration, resilience, self-awareness, problem solving and ethics. It has good psychometric properties. Construct validity is ensured as the components of the test are based on the non-medical expert roles captured in various competency frameworks for medical education. The overall test generalizability and interrater reliability are high (G = 0.78–0.87) and (G = 0.81–0.92) respectively; and concurrent validity with MMI after correcting for dis-attenuation is 0.6, although the it varies based on MMI format [ 28 ]. Casper’s predictive validity for personal/professional components of national licensure examinations three-six years post-medical school admission has also been demonstrated ( r = 0.3–0.5) [ 6 ]. Its discriminant validity is shown by a consistent absence or negative correlation with cognitive aspects of national licensure examinations [ 6 ].
However, the need for “a more solid empirical basis” [ 32 ] for the assessment of NCAs at the time of selections has been asserted from an incremental validity and utilitarian perspective. This includes demonstration of incremental validity (over and above assessment of academic performance) for predicting performance, especially clinical performance, and not just academic performance. The literature on predictive validity for performance in practice is extremely limited [ 33 ], with the work linking unprofessional behaviors to future adverse actions, being a rare example of how powerful such research can be. From the utilitarian perspective, there needs to be an immediate practical effect of using a selection instrument. For example, economic consequences of implementing selection tests are important considerations for medical institutions [ 34 , 35 ]. There is a paucity of data linking Casper to future professionalism behavior. Further, we are not aware of any study that has addressed the impact of Casper on institutional resources and operations involved in remediations. Against the background of the need to uphold multiple stakeholder perspectives, the purpose of this study was to determine the impact of Casper in postgraduate residency selections (incorporated as a mandatory component of selections at our institution in 2017) on a clinical performance outcome measure - professional behavior, and the impact on institutional resources. This study adds to the literature by addressing these considerations and is one of the first forays into these areas and sets the stage for future research in this area.
This study was deemed exempt by the University of Saskatchewan Ethics Review Board.
Canadian residency selections are a two-iteration “match” process through the Canadian Resident Matching Service (CaRMS) and involve assessment of the applicants by the programs and the evaluation of the programs by the applicants to determine compatibility. Following rank order list generation, CaRMS algorithms find a match. Our institution is a participant in this national process. The reason to explore Casper was to assess all applicants for the NCAs in a standardized manner. The institution has no financial gains through this test offering.
Over a three-year period following a review of literature discussions were held with multiple stakeholders. This included the senior leadership of the College of Medicine, educational administrators of residency programs, townhalls with undergraduate students, presentations by the Casper team to the formal postgraduate education committee meetings and a formal voting process to adopt Casper. After this decision, a blueprinting exercise with the program directors to determine attributes considered necessary for success during residency and clinical practice. The findings were taken into consideration by Altus Assessments (now Acuity Insights) to determine if their current offering met these requirements or anything else needed to be added to their test menu. The first offering (2016) was voluntary (to conduct correlation studies with other selection methods) and results were not used in selection decisions. A small number of candidates ( n = 56) took this test and there was concordance in the predictive ability of Casper scores for rank ordered lists generated by the traditional selection process. The test was then mandated for all programs commencing next year. The test results are used at our institution in the following manner. The raw and z scores (both institution-wide and program-specific), percentiles, and any narrative comments (highlighting any concerns) for all applicants are sent to the University and shared with the residency program selection committees. The programs must use Casper results in their deliberations in any one of the following three ways – either (a) for screening or (b) for quantitative part of their deliberations or (c) use it as a part of the overall qualitative discussion. Of the 25 programs, all programs use it for screening, 18 use it as a quantitative variable in their deliberations and 13 use it part of the overall discussion about the candidate (because overall assessment of the applicant involves interviews with SJT and BI components).
The components of Casper for postgraduate medical education (PGME) residency selections at our institution include collaboration, communication, empathy, equity, ethics, motivation, problem-solving, professionalism, resilience and self-awareness, receptivity to feedback, judgement, and teamwork. The examinations are marked by independent fully trained assessors situated remotely.
Participants
The study pool included residents selected each year as first year residents in direct entry programs over a three-year pre- Casper (2013–2015) and three-year post- Casper (2017–2019) time-period. Although follow-up data was available for many years (ranging from 3 to 9 years), only three-year post-selection data was used for each cohort as this was the minimum time of follow-up data for the 2019 cohort at the time of the study.
Data collection and outcome measures
Data for this study was obtained from admission documents and education files of residents who were in difficulty (RID), defined as those who needed a learning intervention during their residency training. This study is based on secondary use of admissions data and information in the files of residents in difficulty, as the information was not primarily collected for this purpose and therefore required a review and subsequent exemption by the Ethics Board of the institution. RID files were compiled within the PGME office by the academic processes and policies coordinator and only anonymized and summarized information was conveyed to the project team.
We utilize a systematic tiered approach (addressing learners, teachers and system issues) reflecting the principles outlined in the graduated interventions for disruptive behavior model [ 36 ] and educational practices in different zones [ 37 ]. Criteria for learning interventions were reviewed subsequent to implementation. Changes to CBME policies spanned programmatic approach to assessments, resource allocation, modification of learning experiences including simulation to acquire competencies, and program evaluation. Changes to assessment involved a more comprehensive evaluation of all assessment data (including daily feedback that specifically required identification of professionalism and patient safety concerns and a more robust approach to assessment in clinical and academic settings). The criteria for R and P were reviewed and were not changed with the implementation of competency-based medical education (CBME); these remained same for pre- and post-Casper groups.
The educational interventions for RID could be either an enhanced learning plan (ELP), remediation ( R) or probation (P). The ELPs are informal and for those issues that do not meet the threshold for remediation or probation and are less intensive; these are usually 3 months in duration. The criteria for a formal learning intervention (R and P) are stringent reflecting the intensity of deficits, egregiousness of behavior and impact on the safety of the patients and learners. An ELP is a deliberately designed and structured learning plan intended to guide the resident towards successful attainment of specific competencies when it has been assessed that the deficiencies are mild and can be addressed with more focused attention. Remediation is a more rigorous intervention with clearly defined learning and assessment measures, and outcomes for residents experiencing considerable difficulties, according to our established criteria. Probation is the formal modification of the residency training to address specific identified weaknesses and where the extent of weaknesses is such where the resident’s ability to continue training is likely to be significantly compromised. R and P are usually six months in duration and can be as along as 12 months. The identification of areas for improvement / concerns are documented systematically through a formal programmatic assessment process and discussions and decisions by a resident assessment committee (prior to the adoption of competency-based medical education (CBME) and by the competence committee (after the implementation of CBME). The learning plans are developed at the program level involving the residency program committee utilizing standardized templates and these plans are discussed with the resident, faculty supervisors and mentors. These are then reviewed by the PGME office for adherence to policies and procedures, academic rigor and well-being and support for the resident. After formal approval these are implemented by the program. Regular monitoring and a review of the final report are done by the PGME office including approval of the actions based on the outcomes.
The following outcome measures were collected from resident files; (a) residents in difficulty (number of RID, frequency of different CanMEDS roles requiring interventions), (b) category of learning intervention (ELP, R, or P), (c) outcome of learning interventions (successful completion, adverse outcome including another learning intervention or termination, and appeal of the decision), and (d) costs and time associated with addressing RID (summary data provided by the PGME Resident Resource Office, Education Coordinator and the Finance Coordinator).
Professionalism concerns were considered using the 4I framework [ 38 , 39 ], which originated in the undergraduate medical education setting and with minor changes has been shown to be relevant to the PGME settings [ 40 ]. The 4I framework articulates 30 specific unprofessional behaviors and maps these to four categories (involvement, integrity, interaction and introspection). The behavioral descriptors provide a guide to the assessors and educators on what to observe and document [ 38 ]. Based on another study [ 40 ], two descriptors were added to the Introspection category; these included absences related to perceived workload complaints (nervous exhaustion) and a nine-to-five mentality. Professionalism concerns were mapped onto the 4I domains and specific behavioral descriptors based upon the documentation in the program and the central PGME office files and the frequency of the specific behaviors recorded in the pre- and post-Casper cohort. The general categorization of professionalism concerns into mild, moderate and major was according to the descriptors from the Baylor College of Medicine [ 41 ].
Descriptive statistics was used for frequencies of quantitative data within groups e.g., number of residents, CanMEDS roles requiring intervention, category of learning interventions, and learner outcomes. Between group comparisons were performed using two-proportion z-tests on the number of learning interventions observed between the pre- and post-Casper groups, frequency of CanMEDS roles identified and the type of outcome.
The results are presented to reflect the two main questions of this study, a) association between Casper and professionalism behavior and the impact on institution (costs and time required for learning interventions).
Number of residents
The number of first-year residents admitted into PGME pre- (2013–2015) and post (2017–2019) Casper were 361 and 343 respectively. Amongst these, the number of RID were similar (16 (4.4%) in the pre-Casper cohort and 15 (4.4%) in the post-Casper cohort.
CanMEDS roles requiring attention
The medical expert domain was the most frequent role requiring attention in both the pre- and post- Casper cohorts. Professionalism concerns were identified in 75% of the pre- Casper cohort and 40% in the post- Casper group; a 35% reduction. The differences in the two groups across CanMEDS roles are shown in Table 1 .
Professionalism concerns
Using the 4I framework the identified themes/ categories and specific behavioral descriptors of professionalism concerns are shown in Table 2 . In addition to the overall reduction in the number of residents with professionalism concerns (35% reduction), there was a decrease in the number of professionalism concerns in all four domains; 17% each in the involvement, interaction, and introspection domains, and a 25% reduction in the integrity domain. Further the “severity” of the concerns in the post- Casper group was considered to be mild to moderate and not major.
Changes in the type of learning interventions required
Formal learning interventions (15 vs. 5) and informal learning plans (3 vs. 11) were significantly different in the pre- and post- Casper cohorts respectively ( p < 0.05).
Outcomes of intervention
In the pre- Casper cohort, more than one formal intervention (remediation or conversion of remediation to probation or ELP followed by remediation or probation) occurred in 3 cases; while in the post- Casper cohort, none of the residents required another learning intervention.There were no terminations in the post- Casper cohort compared to three in the pre- Casper group (two dismissals and one leave of absence and resignation).
Financial impact on the publicly funded PGME
The costs associated with salary for additional training, preceptor remunerations, additional assessments to tailor interventions, logistics (vacations, leaves, travel), and resident resource office support were reduced by 96% (from hundreds of thousands to tens of thousands of dollars). Addition of legal costs to this equation reduced it by approximately 99%. The savings were redirected to enhance residency education; specifically, improve the quality of the programs (infrastructure support – technical, physical, materials, simulation, academic programming – a wider access to courses and conferences), and resident well-being. Some of the funds were utilized to support institutional endeavors e.g., the continuing implementation of CBME.
Time required for learning interventions
The time spent on all learning interventions was measured in years for the pre-Casper cohort and in months for the post-Casper cohort. The “time” included actual faculty time during learning interventions and time for development of plans, and processes and administrative oversight. It did not include time for appeals and legal processes.
The introduction of Casper as a mandatory requirement for entry into postgraduate residency programs led to a reduction in the number of professionalism concerns (and various domains and specific behavioral descriptors as outlined in van der Vossen framework), reduction in the number of formal learning interventions (remediations and probations), correction of identified professionalism deficiencies with less intense enhanced learning plans and a marked reduction in the use of institutional resources (time required and institutional costs).
The two most important contributions in the assessment of personal characteristics have been incorporation of behavioral and situational judgment aspects in various methods including interviews [ 26 ], personal essays [ 25 ], online tests [ 6 , 42 ], and selection centres [ 43 ]. Predicting future performance through outcome variable(s) is often used to justify the use of a selection method, tool or an approach. Professionalism concern(s) are a useful clinical performance outcome measure as a decrease in professionalism concerns is relevant to the practice of medicine and its role in professional identity formation [ 44 ]. Further, there is a linkage between unprofessional behaviors during medical education and subsequent adverse actions by the regulatory bodies [ 33 ]. Using an SJT derived test is useful to assess NCAs, as SJTs are predictive of both performance at later stages of selection process itself, e.g., performance in selection centres [ 45 ] and predicting rank order list in a manner similar to traditional faculty assessments of applicants [ 46 ], and performance upon entry into clinical practice [ 47 ].
One confounding variable in our study was the early stages of CBME implementation at our institution in the same timeframe. It is possible that CBME had an effect, especially for those residents who in the post-Casper cohort were in programs that were in the early stages of CBME implementation, however, the effect would have been in the opposite direction, i.e., more concerns are likely to have been identified due to enhanced supervision and increased rigor (frequency and deliberate focus including on professionalism and patient safety) of feedback. Almost all residents in difficulty in the post- Casper cohort demonstrated willingness to take responsibility and address the issues and ranked high on both reflectivity and adaptability; [ 48 ] this, along with less severe concerns – mild and moderate only [ 41 ] - contributed to the development of enhanced learning plans as opposed to remediations and probations.
Selections into medical school and later into residency programs need to balance the needs of the applicants, institutions, and the society at large. The process must be fair and transparent for the applicants and the institutions. The applicants’ perceptions of selection practices are important [ 49 ]; if perceived to be unfair, many excellent candidates may choose not to apply. Since our institution was the first to mandate this test in Canada in PGME admissions, there was a 3% decrease in the number of applicants in the pilot year (2016), but from the next year onwards it has been consistently increasing. Our goal was for all applicants to have an opportunity to be assessed for personal characteristics and not just those who have been screened by either academic records or personal letters that are limited by low reliability coefficients [ 50 ] or reference letters that have significant interpretive variability [ 51 ]. Offering an opportunity to all applicants to be assessed for the abilities essential for the practice of medicine “job” is in keeping with an organizational justice perspective [ 52 ]. This objective assessment is even more important if the interviews at the program level are not structured, and the interviewers have not been trained who then engage in assessing NCAs in a variable manner, especially in view of the low reliability of the interviews [ 53 ]. Finally, the test itself should not be subject to improvements due to coaching; as an SJT, coaching (for performing on the test) does not cause any more effect [ 54 ] that it does on cognitive tests [ 55 ].
An additional consideration is the cost of the test to the applicants and the institutions. There is indeed a cost to write another examination, however it is probably the cheapest (cost approximately 60 CAD) exam to write (compared to other exams). Further, from an institutional perspective SJTs are cost effective [ 56 ]. The institutions need to be prudent in the use and allocation of resources (especially when using public money), operationally efficient to achieve intended outcomes. The cost of residency training is high, estimated to be approximately $ 100,000 / year / resident (approximation from our data). The real and hidden costs of remediation and not remediating a resident who needs it are high [ 57 ], and if professionalism is a reason then these tend to be even higher. The costs of unprofessional behavior in the healthcare system are also very high [ 58 ] and addressing these issues at selection and during residency is worthwhile. However, the resources that are not required for these corrections can be and have been utilized to improve the overall quality of the educational programs, large-scale endeavors to improve well-being and by investing in efforts to make good students even better.
There are additional tangible and intangible costs, and among others include time (additional teaching, supervision, administrative processes and oversight, and appeals), and morale. There are other considerations for institutions as well, including the risk to institutional reputation, and the political impact of going alone. There was considerable opposition at the beginning and the necessary strong leadership support was provided by the Dean and Vice Dean of our institution to sustain the efforts to implement this endeavor. Following our lead, many other institutions in postgraduate settings have adopted Casper.
Working towards equitable representation in medicine is one consideration when thinking of societal implications. Inclusion of non-academic data (to supplement academic data) is even more important for disadvantaged and minority applicants [ 59 ]. SJTs have been shown to have a relatively low adverse impact on minority applicants and have the potential to reduce the disadvantage of the lower socio economic status but not across ethnicity [ 27 ]. Further SJTs are generally perceived to be fair by the applicants [ 56 ]. The evidence for bias of Casper across societal segments is conflicting and evolving. On the one hand there is no evidence of bias for age, gender and aboriginal status for Casper [ 60 ] and the differences between groups across intersectionality variables are smaller for Casper and MMI (compared to academic assessments) and a higher weighting of Casper has been proposed to increase diversity across race, gender and ethnicity [ 61 ]. On the other hand, studies have identified Casper percentiles to be significantly different for gender [ 62 ], race [ 62 ], language use (bilingual vs. English only or English as a second language) [ 63 ], and socio-economic status [ 63 ]. In general, between group differences between groups across societal segments are smaller in the SJTs that what is typically observed with other academic evaluations [ 8 , 61 , 63 ]. Importance of group differences highlights the need to consider adjusting testing to minimize those differences. For example, to reduce bias in Casper, altering response format from typed to a video response, was recently shown to substantially lower group differences across race, socioeconomic status, language use and rural vs. non-rural background (assessed by determining effect size using Cohen’s d) [ 64 ].
Simultaneously addressing multiple stakeholder perspectives is a balancing act between meeting applicant, societal and institutional needs, and accountability to our funders for the most prudent use of money for the highest quality education. Our study has added to the literature that online assessment of personal characteristics in a standardized manner resulted in improvements in an important outcome measure – the incidence and severity of professionalism concerns were reduced, required informal ELPs to address the deficiencies and were associated with a marked reduction in the institutional resources (financial and time) required. The limitations of this study include a relatively small sample size, limited follow-up (three years for each of the six cohorts), a single institutional perspective and absence of details on systemic factors affecting professionalism behaviors, and any unaccounted pre-existing differences between cohorts.
The implications of our study include suggestions for a process for adoption of a new test(s) for selections in residency programs, integration of a validated and objective tool for assessment of NCAs in a comprehensive approach for selections, training of reviewers to consider its use in applicant deliberations, and the use of institutional data to determine the impact and decision to continue the use. Future research aimed at exploring resident outcome analysis of longitudinal data over a longer time-period focused on predictive validities of selection measures is needed. It will also be helpful to include a wider array of outcome measures of both academic and clinical performance to more fully explore the impact of incorporating SJTs (e.g., Casper) in the selection processes in PGME. There are significant advantages from postgraduate programs in terms of collection, analysis, interpretation, and dissemination of postgraduate data compared to undergraduate counterparts. These advantages include greater trainee homogeneity and thus easier interpretation; greater degree of longitudinal data available for analysis, as at this point it includes the complete undergraduate data set in addition to at least part of the postgraduate; less lead time to performance in practice, thus shortening time to obtaining interpretable and actionable results; and fewer confounding factors when seeking correlation in post-training outcome measures of performance in practice.
Availability of data and materials
The datasets used and/or analysed during this study are available from the corresponding author on reasonable request.
Abbreviations
Computerized assessment of personal attributes
Cognitive abilities
Canadian Medical Education Directives for Specialists
Canadian resident matching service
Competency-based medical education
Enhanced learning plan
Multiple-mini interviews
Non-cognitive abilities
Postgraduate Medical Education
Remediation
- Situational judgement test
Powis D, Munro D, Bore M, Eley D. Why is it so hard to consider personal qualities when selecting medical students? Med Teach. 2020;42(4):366–71.
Article Google Scholar
Tulsky A, Aultman JM, Zirwas MJ. Personality testing in resident selection. Virtual Mentor. 2012;14(12):937–45.
Google Scholar
Wiliam D, Millar M, Bartholomew H. Selection for medical education: a review of the literature. London: King’s College London; 2004.
Carroll JB. Human cognitive abilities: a survey of factor analytic studies. New York: Cambridge University Press; 1993.
Book Google Scholar
Heckman JJ, Kautz T. Fostering and measuring skills: interventions that improve character and cognition. Working Paper. 2013. Contract No.: Working Paper 19656.
Dore KL, Reiter HI, Kreuger S, Norman GR. Casper, an online pre-interview screen for personal/professional characteristics: prediction of national licensure scores. Adv Health Sci Educ. 2017;22(5):1321–2.
Dowell J, Lumsden MA, Powis D, Munro D, Bore M, Makubate B, et al. Predictive validity of the personal qualities assessment for selection of medical students in Scotland. Med Teach. 2011;33(9):E485–488.
Patterson F, Ashworth V, Zibarras L, Coan P, Kerrin M, O’Neill P. Evaluations of situational judgement tests to assess non-academic attributes in selection. Med Educ. 2012;46(9):850–68.
Eva KW, Reiter HI. Where judgement fails: pitfalls in the selection process for medical personnel. Adv Health Sci Educ. 2004;9(2):161–74.
Borghans L, Duckworth AL, Heckman JJ, Ter Weel B. The economics and psychology of personality traits. J Hum Resour. 2008;43(4):972–1059.
Eva KW, Reiter HI, Trinh K, Wasi P, Rosenfeld J, Norman GR. Predictive validity of the multiple mini-interview for selecting medical trainees. Med Educ. 2009;43(8):767–75.
Warm EJ, Kinnear B, Lance S, Schauer DP, Brenner J. What behaviors define a good physician? Assessing and communicating about noncognitive skills. Acad Med. 2022;97(2):193–9.
Zolnierek KB, Dimatteo MR. Physician communication and patient adherence to treatment: a meta-analysis. Med Care. 2009;47(8):826–34.
Tavakoly Sany SB, Behzhad F, Ferns G, Peyman N. Communication skills training for physicians improves health literacy and medical outcomes among patients with hypertension: a randomized controlled trial. BMC Health Serv Res. 2020;20(1):60.
Khawar A, Frederiks F, Nasori M, Mak M, Visser M, van Etten-Jamaludin F, et al. What are the characteristics of excellent physicians and residents in the clinical workplace? A systematic review. BMJ Open. 2022;12(9):e065333.
Saxena A, Desanghere L. A framework for residents’ pursuit of excellence based upon non-cognitive and cognitive attributes. Postgrad Med J. 2023;99(1167):17–24.
Kreiter CD, Axelson RD. A perspective on medical school admission research and practice over the last 25 years. Teach Learn Med. 2013;25(Suppl 1):S50–56.
Patterson F, Knight A, Dowell J, Nicholson S, Cousans F, Cleland J. How effective are selection methods in medical education? A systematic review. Med Educ. 2016;50(1):36–60.
Powis DA. Selecting medical students. Med Educ. 2003;37(12):1064–5.
AAMC. Deciding on a career in medicine Washington, DC: Association of American Medical Colleges. 2019. Available from: https://students-residents.aamc.org/choosing-medical-career/deciding-career-medicine .
GMC. Becoming a doctor in the UK: General Medical Council. 2019. Available from: https://www.gmc-uk.org/education/becoming-a-doctor-in-the-uk .
Adam J, Bore M, Childs R, Dunn J, McKendree J, Munro D, et al. Predictors of professional behaviour and academic outcomes in a UK medical school: a longitudinal cohort study. Med Teach. 2015;37(9):868–80.
Park E, Ha PK, Eisele DW, Francis HW, Kim YJ. Personal characteristics of residents may predict competency improvement. Laryngoscope. 2016;126(8):1746–52.
Lievens F. Adjusting medical school admission: assessing interpersonal skills using situational judgement tests. Med Educ. 2013;47(2):182–9.
White JS, Lemay J-F, Brownell K, Lockyer J. A chance to show yourself – how do applicants approach medical school admission essays? Med Teach. 2011;33(10):e541–548.
Dore KL, Kreuger S, Ladhani M, Rolfson D, Kurtz D, Kulasegaram K, et al. The reliability and acceptability of the multiple Mini-interview as a selection instrument for postgraduate admissions. Acad Med. 2010;85(10 Suppl):S60–63.
Lievens F, Patterson F, Corstjens J, Martin S, Nicholson S. Widening access in selection using situational judgement tests: evidence from the UKCAT. Med Educ. 2016;50(6):624–36.
Dore KL, Reiter HI, Eva KW, Krueger S, Scriven E, Siu E, et al. Extending the interview to all medical school candidates-computer-based multiple sample evaluation of noncognitive skills (CMSENS). Acad Med. 2009;84:S9–12.
Ginsburg S, Regehr G, Hatala R, McNaughton N, Frohna A, Hodges B, et al. Context, conflict, and resolution: a new conceptual framework for evaluating professionalism. Acad Med. 2000;75(10 Suppl):S6–11.
Reiter H, Dore K, Kreuger S, Edge w, Murray T, Dietrich J. How small concerns Loom large when changing medium: from multiple mini-interview (MMI) to computer-based assessment for sampling personal characteristics (Casper). Med Educ. 2011;45(Suppl 1):48.
Reiter H, Dore K, Edge W, Kreuger S, Dietrich J. How can web-based Casper (Computer-based Assessment for Sampling Personal characteristics) best meet your needs in assessing your medical school and residency program applicants? Med Educ. 2012;46(1Suppl 1):21.
Niessen ASM, Meijer RR. Selection of medical students on the basis of nonacademic skills: is it worth the trouble? Clin Med. 2016;16(4):339–42.
Papadakis MA, Hodgson CS, Teherani A, Kohatsu ND. Unprofessional behavior in medical school is associated with subsequent disciplinary action by a state medical board. Acad Med. 2004;79(3):244–9.
Brogden HE. When Testing pays off. Pers Psychol. 1949;2(2):171–83.
Cascio WF. Responding to the demand for accountability: a critical analysis of three utility models. Org Behav Hum Perform. 1980;25(1):32–45.
Hickson GB, Pichert JW, Webb LE, Gabbe SG. A complementary approach to promoting professionalism: identifying, measuring, and addressing unprofessional behaviors. Acad Med. 2007;82(11):1040–8.
Ellaway RH, Chou CL, Kalet AL. Situating remediation: accommodating success and failure in medical education systems. Acad Med. 2018;93(3):391–8.
Mak-van der Vossen M, Teherani A, van Mook W, Croiset G, Kusurkar RA. How to identify, address and report students’ unprofessional behaviour in medical school. Med Teach. 2020;42(4):372–9.
Mak-van der Vossen M, van Mook W, van der Burgt S, Kors J, Ket JCF, Croiset G, et al. Descriptors for unprofessional behaviours of medical students: a systematic review and categorisation. BMC Med Educ. 2017;17(1):164.
Barnhoorn PC, Nierkens V, Mak-van der Vossen MC, Numans ME, van Mook WNKA, Kramer AWM. Unprofessional behaviour of GP residents and its remediation: a qualitative study among supervisors and faculty. BMC Fam Pract. 2021;22(1):249.
Gill AC, Nelson EA, Mian AI, Raphael JL, Rowley DR, McGuire AL. Responding to moderate breaches in professionalism: an intervention for medical students. Med Teach. 2015;37(2):136–9.
Lievens F, Sackett PR. The validity of interpersonal skills assessment via situational judgment tests for predicting academic success and job performance. J Appl Psychol. 2012;97(2):460–8.
Gale TCE, Roberts MJ, Sice PJ, Langton JA, Patterson FC, Carr AS, et al. Predictive validity of a selection centre testing non-technical skills for recruitment to training in anaesthesia. Brit J Anaesth. 2010;105(5):603–9.
Cruess RL, Cruess SR, Boudreau JD, Snell L, Steinert Y. A schematic representation of the professional identity formation and socialization of medical students and residents: a guide for medical educators. Acad Med. 2015;90(6):718–25.
Koczwara A, Patterson F, Zibarras L, Kerrin M, Irish B, Wilkinson M. Evaluating cognitive ability, knowledge tests and situational judgement tests for postgraduate selection. Med Educ. 2012;46(4):399–408.
Shipper ES, Mazer LM, Merrell SB, Lin DT, Lau JN, Melcher ML. Pilot evaluation of the computer-based assessment for sampling personal characteristics test. J Surg Res. 2017;215:211–8.
Patterson F, Zibarras L, Ashworth V. Situational judgement tests in medical education and training: research, theory and practice: AMEE Guide 100. Med Teach. 2016;38(1):3–17.
der Mak-van MC, de la Croix A, Teherani A, van Mook W, Croiset G, Kusurkar RA. Developing a two-dimensional model of unprofessional behaviour profiles in medical students. Adv Health Sci Educ Theory Pract. 2019;24(2):215–32.
Patterson F, Zibarras L, Carr V, Irish B, Gregory S. Evaluating candidate reactions to selection practices using organisational justice theory. Med Educ. 2011;45(3):289–97.
Wright S. Medical school personal statements: a measure of motivation or proxy for cultural privilege? Adv Health Sci Educ Theory Pract. 2015;20(3):627–43.
Dirschl DR, Adams GL. Reliability in evaluating letters of recommendation. Acad Med. 2000;75(10):1029.
Gilliland SW. The Perceived fairness of selection systems: an organizational justice perspective. Acad Manage Rev. 1993;18(4):694–734.
Kreiter CD, Yin P, Solow C, Brennan RL. Investigating the reliability of the medical school admissions interview. Adv Health Sci Educ Theory Pract. 2004;9(2):147–59.
Cullen M, Sackett P, Lievens F. Threats to the operational use of situational judgment tests in the college admission process. Int J Select Assess. 2006;14:142–55.
Hausknecht J, Trevor C, Farr J. Retaking ability tests in a selection setting: implications for practice effects, training performance, and turnover. J Appl Psychol. 2002;87:243–54.
Lievens F, Peeters H, Schollaert E. Situational judgment tests: a review of recent research. Personnel Rev. 2008;37(4):426–41.
Roberts NK, Williams RG. The hidden costs of failing to fail residents. J Grad Med Educ. 2011;3(2):127–9.
Rawson J, Thompson N, Sostre G, Deitte L. The cost of disruptive and unprofessional behaviors in Health Care. Acad Radiol. 2013;20:1074–6.
Mitchell KJ. Traditional predictors of performance in medical school. Acad Med. 1990;65(3):149–58.
Dore K, Reiter HI, Edge W, Fowler N, Whyte R, Kreuger S. Is there any evidence of Casper bias for age, gender, and aboriginal status on video-based SJT scores? Med Educ. 2013;47(Suppl 1):66.
Juster FR, Baum RC, Zou C, Risucci D, Ly A, Reiter H, et al. Addressing the diversity-validity dilemma using situational judgment tests. Acad Med. 2019;94(8):1197–203.
Gustafson CE, Johnson CJ, Beck Dallaghan GL, Knight OJ, Malloy KM, Nichols KR, et al. Evaluating situational judgment test use and diversity in admissions at a southern US medical school. PLoS One. 2023;18(2):e0280205.
Pan X, Huang V, Laumbach S, Copeland HL, Akinola M, Rosenbaum D, et al. Impact of patterns of language use and socio-economic status on a constructed response situational judgment test (SJT). PLoS One. 2023;18(8):e0289420.
Robb C, Sitarenios G, Dore K, Au Yong C, Derby J, Davidson H, Improving fairness and equity in a situational judgmenet test: An alternate response format. Canadian Conference on Medical Education;, Ottawa ON et al. Calgary, AB: Canadian Medical Education Journal; 2022. p. 111.
Download references
Acknowledgements
Not applicable.
Author information
Authors and affiliations.
College of Medicine, University of Saskatchewan, Room 3A10, Health Sciences Bldg., 107 Wiggins Road, Saskatoon, SK, S7N 5E5, Canada
Anurag Saxena & Loni Desanghere
College of Medicine, University of Saskatchewan, Saskatoon, Canada
Department of Medicine, McMaster University, Hamilton, Canada, Science and Innovation at Acuity Insights, Toronto, ON, Canada
Department of Oncology, McMaster University, Hamilton, ON, Canada
Harold Reiter
You can also search for this author in PubMed Google Scholar
Contributions
AS conceptualized and designed this study, analyzed and interpreted the data and drafted the manuscript.LD designed the study, collected, analyzed and interpreted data and drafted the manuscript.KD designed the study, reviewed analyzed data and revised the manuscript for important intellectual content.HR designed the study, reviewed analyzed data and revised the manuscript for important intellectual content.All authors approved the final submitted version of the manuscript and agreed to be accountable for all aspects of the work.
Corresponding author
Correspondence to Anurag Saxena .
Ethics declarations
Ethics approval and consent to participate.
This study was deemed exempt by the University of Saskatchewan Ethics Review Board, and University of Saskatchewan Ethics Review Board waived the need for informed consent.
Consent for publication
Competing interests.
Anurag Saxena: The lead and corresponding author is Associate Dean, Postgraduate Medical education of the office where this study was conducted. He was on the Advisory Board of Altus Assessments (there was no financial compensation).
Loni Desanghere: None.
Kelly Dore: Co-Founder and current VP of Science and Innovation at Acuity Insights ( formerly Altus Assessments), the company that provides Casper. She was not involved in primary data analysis.
Harold Reiter: Co-founder, non-voting Board member and shareholder in Acuity Insights ( formerly Altus Assessments), the company that provides Casper. He was not involved in primary data analysis.
Additional information
Publisher’s note.
Springer Nature remains neutral with regard to jurisdictional claims in published maps and institutional affiliations.
Rights and permissions
Open Access This article is licensed under a Creative Commons Attribution 4.0 International License, which permits use, sharing, adaptation, distribution and reproduction in any medium or format, as long as you give appropriate credit to the original author(s) and the source, provide a link to the Creative Commons licence, and indicate if changes were made. The images or other third party material in this article are included in the article's Creative Commons licence, unless indicated otherwise in a credit line to the material. If material is not included in the article's Creative Commons licence and your intended use is not permitted by statutory regulation or exceeds the permitted use, you will need to obtain permission directly from the copyright holder. To view a copy of this licence, visit http://creativecommons.org/licenses/by/4.0/ . The Creative Commons Public Domain Dedication waiver ( http://creativecommons.org/publicdomain/zero/1.0/ ) applies to the data made available in this article, unless otherwise stated in a credit line to the data.
Reprints and permissions
About this article
Cite this article.
Saxena, A., Desanghere, L., Dore, K. et al. Incorporating a situational judgement test in residency selections: clinical, educational and organizational outcomes. BMC Med Educ 24 , 339 (2024). https://doi.org/10.1186/s12909-024-05310-8
Download citation
Received : 05 January 2024
Accepted : 13 March 2024
Published : 26 March 2024
DOI : https://doi.org/10.1186/s12909-024-05310-8
Share this article
Anyone you share the following link with will be able to read this content:
Sorry, a shareable link is not currently available for this article.
Provided by the Springer Nature SharedIt content-sharing initiative
- Professionalism
- Resident selection
- Learner outcomes
- Organizational outcomes
BMC Medical Education
ISSN: 1472-6920
- Submission enquiries: [email protected]
- General enquiries: [email protected]
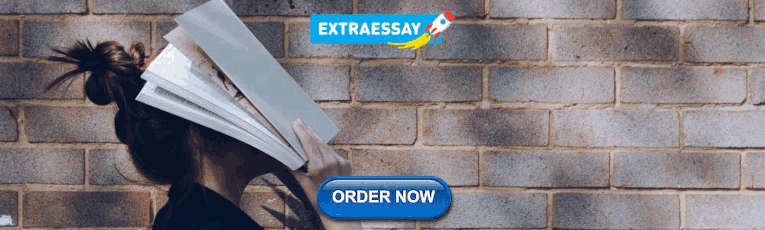
IMAGES
VIDEO
COMMENTS
Quantitative data refers to numerical information that can be measured and analyzed statistically. It provides educators with objective measurements that help identify patterns, trends, and correlations in student performance. Quantitative data plays a crucial role in education as it allows educators to make data-driven decisions.
Qualitative research designs focus on collecting data that is relational, interpretive, subjective, and inductive; whereas a typical quantitative study, collects data that are deductive, statistical, and objective. In contrast, qualitative data is often in the form of language, while quantitative data typically involves numbers.
Quantitative research in education provides numerical data that can prove or disprove a theory, and administrators can easily share the number-based results with other schools and districts. And while the research may speak to a relatively small sample size, educators and researchers can scale the results from quantifiable data to predict ...
Revised on June 22, 2023. Quantitative research is the process of collecting and analyzing numerical data. It can be used to find patterns and averages, make predictions, test causal relationships, and generalize results to wider populations. Quantitative research is the opposite of qualitative research, which involves collecting and analyzing ...
In addition to the above four types of data in education, you also have qualitative and quantitative data. Quantitative data uses statistical and mathematical analysis to measure variables (i.e., student achievement, attendance, and demographic characteristics). Educational quantitative data is collected through numerical methods, like surveys ...
Quantitative research is 'Explaining phenomena by collecting numerical data that are analysed using mathematically based methods (in particu-lar statistics)'. Let's go through this definition step by step. The first element is explaining phenomena. This is a key element of all research, be it quantitative or quali-tative.
Understanding Quantitative Data in Educational Research. This book is designed to help Education students gain confidence in analysing and interpreting quantitative data and using appropriate statistical tests, by exploring, in plain language, a variety of data analysis methods. Highly practical, each chapter includes step-by-step instructions ...
This book is designed to help Education students gain confidence in analysing and interpreting quantitative data and using appropriate statistical tests, by exploring, in plain language, a variety ...
Historically, much of the data collection performed in educational research depended on methods developed for studies in the field of psychology, a discipline which took what is termed a "quantitative" approach. This involves using instruments, scales, Tests, and structured observation and interviewing. By the mid- to late twentieth ...
Educational research has a strong tradition of employing state-of-the-art statistical and psychometric (psychological measurement) techniques. Commonly referred to as quantitative methods, these techniques cover a range of statistical tests and tools. The Sage encyclopedia of educational research, measurement, and evaluation by Bruce B. Frey (Ed.)
Data Analysis: Strengthening Inferences in Quantitative Education Studies Conducted by Novice Researchers Abstract Data analysis is a significant methodological component when conducting quantitative education studies. Guidelines for conducting data analyses in quantitative education studies are common but often underemphasize four important
Quantitative data analysis is one of those things that often strikes fear in students. It's totally understandable - quantitative analysis is a complex topic, full of daunting lingo, like medians, modes, correlation and regression.Suddenly we're all wishing we'd paid a little more attention in math class…. The good news is that while quantitative data analysis is a mammoth topic ...
Quantitative data is the language of science. It uses mathematical models, theories, and hypotheses. Thanks to this type of data, we know things like the distance between the sun and the moon, and why we have seasons, days, and nights. Quantitative data depends on the fact that you can put everything in terms of numbers.
Quantitative data plays a crucial role in education, providing valuable insights into various aspects of the learning process. By analyzing numerical information, educators can make informed decisions and implement effective strategies to improve educational outcomes. But what exactly is quantitative data in education, and why is it essential ...
Education: Quantitative data is used in education to measure student performance, evaluate teaching methods, and identify areas where improvement is needed. For example, a teacher might use quantitative data to track the progress of their students over the course of a semester and adjust their teaching methods accordingly.
What is Quantitative Research? Quantitative methodology is the dominant research framework in the social sciences. It refers to a set of strategies, techniques and assumptions used to study psychological, social and economic processes through the exploration of numeric patterns.Quantitative research gathers a range of numeric data.
Understanding Quantitative Data in Educational Research. This book is designed to help Education students gain confidence in analysing and interpreting quantitative data and using appropriate statistical tests, by exploring, in plain language, a variety of data analysis methods. Highly practical, each chapter includes step-by-step instructions ...
Quantitative data can be discrete or continuous. Discrete data takes on fixed values (e.g. a person has three children), while continuous data can be infinitely broken down into smaller parts. Quantitative data has several advantages: It is relatively quick and easy to collect, and it is analyzed subjectively.
The main difference between quantitative and qualitative research is the type of data they collect and analyze. Quantitative research collects numerical data and analyzes it using statistical methods. The aim is to produce objective, empirical data that can be measured and expressed in numerical terms.
education data to improve student achievement. What Factors Hinder Effective Data Use? Although many national, state, district, and school ... • Use quantitative and qualitative data sources to improve instruction and better understand student thinking and learning, including test results, portfolios, homework, student conferences, ...
Hypothesis-testing (Quantitative hypothesis-testing research) - Quantitative research uses deductive reasoning. - This involves the formation of a hypothesis, collection of data in the investigation of the problem, analysis and use of the data from the investigation, and drawing of conclusions to validate or nullify the hypotheses.
Read next: 5 examples of qualitative data in education. Quantitative Data. Quantitative data in education, on the other hand, focuses on numerical information and measurable outcomes. It includes data points such as test scores, attendance records, and demographic information. Quantitative data is collected using surveys, standardized ...
Also, qualitative data addresses a number of the shortcomings of quantitative research. For instance, quantitative data can indicate that a particular school district's test scores have outperformed those of other regional school districts but cannot explain why this is the case. Examples of qualitative data in education
How data from digital learning tools can refine teaching; DIY learning analytics: using data to improve online teaching; Collecting data on our students is the only way forward; At LSE, we often use data visualisation to explain and explore research findings or illustrate student journeys, for example. From a more statistical standpoint, data ...
Computer-based assessment for sampling personal characteristics (Casper), an online situational judgement test, is a broad measure of personal and professional qualities. We examined the impact of Casper in the residency selection process on professionalism concerns, learning interventions and resource utilization at an institution. In 2022, admissions data and information in the files of ...