What is the NIST Cloud Computing Reference Architecture?
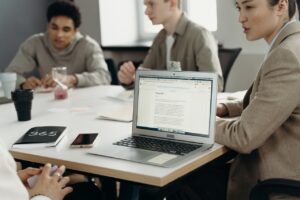
In September 2011, The National Institute for Standard and Technology (NIST) created Special Publication (SP) 500-292, “NIST Cloud Computing Reference Architecture,” to establish a baseline cloud computing architecture. NIST SP 500-292 defines services and relationships between cloud service providers, consumers, and other stakeholders. When preparing to implement or revisit your cloud computing architecture , you’ll want to review the specifics of NIST SP 500-292.
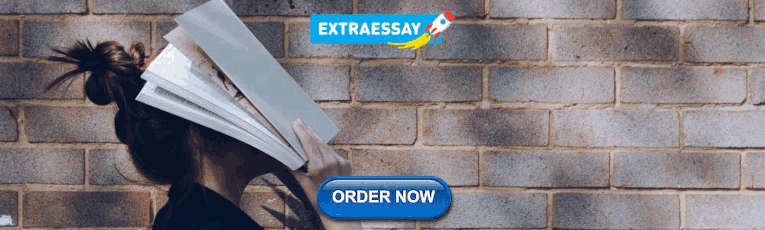
What is the NIST Architecture in Cloud Computing
The NIST SP 500-292 breaks down into several sections that define and explain all elements of cloud computing . These form a taxonomy with four distinct levels, each representing a more nuanced, niche set of terms. The first two levels define the most essential terms:
- The Level 1 terms – A set of Roles that collectively comprise the cloud Reference Model
- The Level 2 terms – A set of Activities that define the model’s Architectural Components
By understanding these terms and the relationships between them, any company can begin to optimize its cloud computing security architecture in response to ever-evolving cloud threats.
The NIST’s Cloud Computing Architecture Model
The first portion of NIST SP 500-292 defines the relationships between all stakeholders involved in cloud computing . There are five major roles detailed within NIST SP 500-292:
- Cloud Consumer
- Cloud Provider
- Cloud Auditor
- Cloud Broker
- Cloud Carrier
As a disclaimer, these roles may be less stable today than they were in 2011, as providers and consumers alike have changed drastically in nature and scale. Still, the definitions are useful as templates for understanding the basis of stakeholders’ differing roles and responsibilities.
Download Our Comprehensive Guide to NIST Implementation
Cloud consumers in the nist cloud computing reference architecture.
NIST designates Cloud Consumers as the principal stakeholders for cloud computing services. The category includes three Cloud Consumer distinctions according to the services used:
- Software as a service (SaaS) consumers who rely on cloud computing for general office or productivity services (e.g., HR and accounting tasks)
- Platform as a service (PaaS) consumers who rely on cloud computing for their business intelligence needs (e.g., database management and application integration)
- Information technology as a service (ITaaS) consumers who rely on cloud computing for IT needs (e.g., storage, backups, content delivery, and other general computing tasks)
Cloud Providers in the NIST Cloud Computing Reference Architecture
Cloud providers are the parties most closely associated with cloud consumers. They are responsible for making cloud services available. Cloud providers’ offerings correspond to the types of consumers, along with the “Activities” or “Components.”
SaaS cloud providers generally deploy or manage the configuration of given software on cloud infrastructure. PaaS cloud providers generally manage the cloud infrastructure while also developing tools for optimizing workflows. ITaaS cloud providers generally facilitate distribution, maintenance, and monitoring of cloud infrastructure.
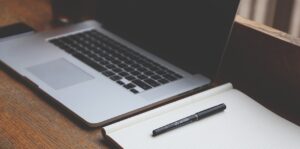
Cloud Auditors in the NIST Cloud Computing Reference Architecture
The NIST defines cloud auditors as parties who can execute independent audits or assessments on a company’s cloud infrastructure. Audits are typically done to determine whether the infrastructure meets cybersecurity or compliance benchmarks. Critically, auditing services must be delivered separately from any cloud services when partnering with the same vendor or by another third party.
However, in the contemporary cloud environment, a provider may integrate a secure and logically separate auditing functionality into a suite of services. As a result, consumers might seek out providers who integrate this functionality for efficiency’s sake.
Cloud Brokers in the NIST Cloud Computing Reference Architecture
Cloud brokers are defined as managing service providers. Consumers may contact cloud brokers instead of cloud providers. Brokers tend to handle three cloud categories:
- Intermediation – Enhancing access, performance monitoring, identity management, etc.
- Aggregation – Integrating a provider’s cloud services into a comprehensive cloud suite
- Arbitrage – Integrating services from multiple providers into a uniform service suite
These parties may be distinct from providers, but providers may also conduct such activity.
Cloud Carriers in the NIST Cloud Computing Reference Architecture
The NIST defines cloud carriers as the parties facilitating consumers’ and providers’ data transmissions and their connectivity to cloud services.
Cloud carriers’ responsibilities include the production and distribution of all physical and virtual resources needed to maintain cloud computing. Responsibilities pertain to all the servers and hardware needed to keep cloud networks up and running, along with endpoints or network access devices used to access cloud data safely.
The NIST Cloud Computing Reference Architecture Components
The most critical stakeholders in the NIST Cloud Computing Reference Architecture are consumers and providers. The entire architecture, comprising five “Architectural Components,” can be understood as a way of defining the relationships between them.
The five functionalities explained below overlap with the SaaS, PaaS, and ITaaS models detailed above.
Deployment in the NIST Cloud Computing Reference Architecture
The first Architectural Component is Deployment, which follows one of four distinct models:
- Public – Most cloud infrastructure and resources are available or accessible to a diverse audience, including the general public and a wide range of subscription-level consumers.
- Private – The cloud infrastructure and resources are available or accessible to only an individual consumer. These are hosted on-site by the provider or off-site by a third party.
- Community – Most cloud infrastructure and resources are available or accessible to a group of consumers within the same industry or with similar security needs or concerns.
- Hybrid – Cloud infrastructure and resources are available via distinct, packaged distribution models (e.g., through a cloud broker).

Orchestration in the NIST Cloud Computing Reference Architecture
The second Architectural Component is Orchestration, which refers to three hierarchical layers of system components that providers require to deliver services:
- Service layer – This layer determines service type, corresponding to the SaaS, PaaS, and ITaaS categories of providers.
- Resource abstraction and control – This layer determines the internal software assets and systems needed to abstract data (e.g., virtual machines) and those needed for control (e.g., dynamic allocation) to communicate with system hardware.
- Physical resource layer – This layer concerns physical resources (e.g., endpoints, servers).
Management in the NIST Cloud Computing Reference Architecture
The third Architectural Component is Management, which breaks down into three categories:
- General business support – Cloud-based management of business processes (e.g., client, inventory, contract management, accounting, and reporting)
- Provisioning / configuration – Cloud-based management of logistical processes (e.g., deployment or adjustment of cloud systems or service-level agreements)
- Portability / interoperability – Cloud-based management of information-related tasks (e.g., optimization across various formats and wide-scale security and accessibility)
Cloud Security in the NIST Cloud Computing Reference Architecture
The fourth Architectural Component is Cloud Security, which includes the oversight and advisory for cloud infrastructure and all interacting physical or virtual resources. This component may involve general security architecture implementation , security control development, cloud patch monitoring , or various regulatory compliance framework implementations.
Critically, all stakeholders in a given service relationship must contend with the intricacies of their own security implications. Providers’ and consumers’ respective security practices may impact each other, as hackers may leverage a weaker consumer network to attack a provider or vice versa.
Cloud Privacy in the NIST Cloud Computing Reference Architecture
The fifth and final Architectural Component is Cloud Privacy, which is closely related to Cloud Security. NIST specifically designates that cloud providers must protect consumers’ data processed or stored via cloud services. In particular, providers must safeguard any personal information (PI) or personally identifiable information (PII).
There is a significant overlap between Cloud Privacy and compliance concerns, though it is generally addressed in particular compliance frameworks rather than in NIST SP 500-292. For example, the HIPAA framework’s Privacy and Security Rules detail controls to ensure protected health information (PHI) is private, but NIST doesn’t define any specific protocols.
Professional Cyberdefense Architecture with RSI Security
Cybersecurity threats evolve each year, and companies across all industries must update their cloud protections to maintain security and privacy.
Despite the NIST SP 500-292 being a decade old, it still defines cloud architecture in cloud computing. If you’re ready to optimize your cloud computing architecture and rethink your cybersecurity, contact RSI Security today .
We’ll show you just how simple and secure your cloud architecture can be.
RSI Security
RSI Security is the nation’s premier cybersecurity and compliance provider dedicated to helping organizations achieve risk-management success. We work with some of the world’s leading companies, institution and governments to ensure the safety of their information and their compliance with applicable regulation. We also are a security and compliance software ISV and stay at the forefront of innovative tools to save assessment time, increase compliance and provide additional safeguard assurance. With a unique blend of software based automation and managed services, RSI Security can assist all sizes of organizations in managing IT governance, risk management and compliance efforts (GRC). RSI Security is an Approved Scanning Vendor (ASV) and Qualified Security Assessor (QSA).
SOC 2 Type 1 vs. Type 2: What’s the Difference?
How to detect pegasus spyware, you may also like, cloud security architecture best practices, cloud security for fintech companies, what is cloud encryption, 10 tips for keeping private information secure on..., how to prevent cloud data breaches, importance of encryption in the business world, cloud encryption: top tips & best practices, top benefits of cloud computing, is it safe to store personal data on..., challenges with cloud encryption, leave a comment cancel reply.
Save my name, email, and website in this browser for the next time I comment.
This website uses cookies to improve your experience. If you have any questions about our policy, we invite you to read more. Accept Read More
- Trending Now
- Foundational Courses
- Data Science
- Practice Problem
- Machine Learning
- System Design
- DevOps Tutorial
- Web Browser
- Benefits of Transitioning to Cloud Computing
- Business Drivers in Cloud Computing
- What is OpenStack Telemetry Service?
- Host-Based Evidence
- Could Computing | Service classes and system codes of conduct in IDaaS
- Identity as a Service (IDaaS) as a cloud Based service
- Anatomy of Cloud Computing
- Introduction to OpenStack
- Energy Efficiency in Cloud Computing
- Service level agreements in Cloud computing
- Compliance as a Service (CaaS) in Cloud Computing
- Components, Requirements and interoperability of IDaaS
- Cloud Deployment Model
- Load balancing in Cloud Computing
- Scalability and Elasticity in Cloud Computing
- Issues in Cloud Computing
- What is OpenStack Heat Service?
- Serverless Computing and FaaS Model - The Next Stage in Cloud Computing
- Cloud Computing Services in Financial Market
Cloud Stakeholders as per NIST
NIST Cloud Computing reference architecture defines five major performers:
- Cloud Provider
- Cloud Carrier
- Cloud Broker
- Cloud Auditor
- Cloud Consumer
Each performer is an object (a person or an organization) that contributes to a transaction or method and/or performs tasks in Cloud computing. There are five major actors defined in the NIST cloud computing reference architecture, which are described below:

1. Cloud Service Providers: A group or object that delivers cloud services to cloud consumers or end-users. It offers various components of cloud computing. Cloud computing consumers purchase a growing variety of cloud services from cloud service providers. There are various categories of cloud-based services mentioned below:
- IaaS Providers: In this model, the cloud service providers offer infrastructure components that would exist in an on-premises data center. These components consist of servers, networking, and storage as well as the virtualization layer.
- SaaS Providers: In Software as a Service (SaaS), vendors provide a wide sequence of business technologies, such as Human resources management (HRM) software, customer relationship management (CRM) software, all of which the SaaS vendor hosts and provides services through the internet.
- PaaS Providers: In Platform as a Service (PaaS), vendors offer cloud infrastructure and services that can access to perform many functions. In PaaS, services and products are mostly utilized in software development. PaaS providers offer more services than IaaS providers. PaaS providers provide operating system and middleware along with application stack, to the underlying infrastructure.
2. Cloud Carrier: The mediator who provides offers connectivity and transport of cloud services within cloud service providers and cloud consumers. It allows access to the services of the cloud through Internet networks, telecommunication, and other access devices. Network and telecom carriers or a transport agent can provide distribution. A consistent level of services is provided when cloud providers set up Service Level Agreements (SLA) with a cloud carrier. In general, Carrier may be required to offer dedicated and encrypted connections.
3. Cloud Broker: An organization or a unit that manages the performance, use, and delivery of cloud services by enhancing specific capability and offers value-added services to cloud consumers. It combines and integrates various services into one or more new services. They provide service arbitrage which allows flexibility and opportunistic choices. There are major three services offered by a cloud broker:
- Service Intermediation.
- Service Aggregation.
- Service Arbitrage.
4. Cloud Auditor: An entity that can conduct independent assessment of cloud services, security, performance, and information system operations of the cloud implementations. The services that are provided by Cloud Service Providers (CSP) can be evaluated by service auditors in terms of privacy impact, security control, and performance, etc. Cloud Auditor can make an assessment of the security controls in the information system to determine the extent to which the controls are implemented correctly, operating as planned and constructing the desired outcome with respect to meeting the security necessities for the system. There are three major roles of Cloud Auditor which are mentioned below:
- Security Audit.
- Privacy Impact Audit.
- Performance Audit.
5. Cloud Consumer: A cloud consumer is the end-user who browses or utilizes the services provided by Cloud Service Providers (CSP), sets up service contracts with the cloud provider. The cloud consumer pays per use of the service provisioned. Measured services utilized by the consumer. In this, a set of organizations having mutual regulatory constraints performs a security and risk assessment for each use case of Cloud migrations and deployments. Cloud consumers use Service-Level Agreement (SLAs) to specify the technical performance requirements to be fulfilled by a cloud provider. SLAs can cover terms concerning the quality of service, security, and remedies for performance failures. A cloud provider may also list in the SLAs a set of limitations or boundaries, and obligations that cloud consumers must accept. In a mature market environment, a cloud consumer can freely pick a cloud provider with better pricing and more favourable terms. Typically, a cloud provider’s public pricing policy and SLAs are non-negotiable, although a cloud consumer who assumes to have substantial usage might be able to negotiate for better contracts.
Please Login to comment...
- Cloud-Computing
- WhatsApp To Launch New App Lock Feature
- Node.js 21 is here: What’s new
- Zoom: World’s Most Innovative Companies of 2024
- 10 Best Skillshare Alternatives in 2024
- 30 OOPs Interview Questions and Answers (2024)
Improve your Coding Skills with Practice
What kind of Experience do you want to share?
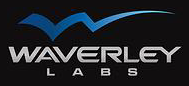
NIST Cloud Computing Security Reference Architecture

NIST Special Publication 500-299
Authors Waverley Labs’ Juanita Koilpillai is a contributing member of the NIST Cloud Computing Security Working Group (NCC SWG).
Abstract The National Institute of Standards and Technology (NIST), along with other agencies, was tasked by the U.S. Chief Information Officer with specific activities aimed at accelerating the adoption of cloud computing. These include the delivery of a US Government Cloud Computing Technology Roadmap and the creation of other NIST Special Publications (NIST SPs) that address the definitions, security aspects, and reference architecture of Cloud Computing.
This document was developed as part of a collective effort by the NIST Cloud Computing Public Security Working Group in response to the priority action plans for the early USG cloud computing adoption identified in NIST SP 500-293: US Government Cloud Computing Technology Roadmap Volume 1, High-Priority Requirements to Further USG Agency Cloud Computing Adoption. NIST SP 500-293 highlights concerns around the protection and control of cloud Consumer data.
This document introduces the NIST Cloud Computing Security Reference Architecture (NCC-SRA or, for the sake of brevity, SRA), providing a comprehensive formal model to serve as security overlay to the architecture described in NIST SP 500-292: NIST Cloud Computing Reference Architecture.
This document also describes a methodology for applying a Cloud-adapted Risk Management Framework (CRMF) using the formal model and an associated set of Security Components (derived from the capabilities identified in the Cloud Security Alliance’s Trusted Cloud Initiative – Reference Architecture [TCI-RA]) to orchestrate a secure cloud Ecosystem by applying the Risk Management Framework described in NIST SP 800-37 (Rev. 1): Guide for Applying the Risk Management Framework to Federal Information Systems: A Security Life Cycle Approach.
The study upon which the NCC-SRA is based collected, aggregated, and validated data for a Public cloud, considering all three cloud service models – Software as a Service (SaaS), Platform as a Service (PaaS), and Infrastructure as a Service (IaaS) – and all cloud Actors (i.e., Consumer, Provider, Broker, Carrier, and Auditor). While this document focuses on a Public cloud deployment model because it best supports illustrative examples of all of the NCC-SRA Security Components and security considerations, the NCC-SRA (the formal model, the set of Security Components and the methodology for applying the CRMF) is agnostic with respect to cloud deployment model, and its methodology can easily be applied to Private, Community, or Hybrid clouds.
The NCC-SRA introduces a risk-based approach to determine each cloud Actor’s responsibility for implementing specific controls throughout the life cycle of the cloud Ecosystem. Specifically, for each instance of the cloud Ecosystem, the security components are analyzed to identify the level of involvement of each cloud Actor in implementing those components. The ultimate objective of this document is to demystify the process of describing, identifying, categorizing, analyzing, and selecting cloud-based services for the cloud Consumer seeking to determine which cloud service offering most effectively addresses their cloud computing requirement(s) and supports their business and mission-critical processes and services in the most secure and efficient manner.
Download the PDF >
View source >

- Privacy Overview
- Strictly Necessary Cookies
This website uses cookies so that we can provide you with the best user experience possible. Cookie information is stored in your browser and performs functions such as recognising you when you return to our website and helping our team to understand which sections of the website you find most interesting and useful.
Strictly Necessary Cookie should be enabled at all times so that we can save your preferences for cookie settings.
If you disable this cookie, we will not be able to save your preferences. This means that every time you visit this website you will need to enable or disable cookies again.
This is a potential security issue, you are being redirected to https://csrc.nist.gov .
You have JavaScript disabled. This site requires JavaScript to be enabled for complete site functionality.
An official website of the United States government
Here’s how you know
Official websites use .gov A .gov website belongs to an official government organization in the United States.
Secure .gov websites use HTTPS A lock ( Lock Locked padlock icon ) or https:// means you’ve safely connected to the .gov website. Share sensitive information only on official, secure websites.
NIST Releases Cybersecurity White Paper: Planning for a Zero Trust Architecture May 06, 2022
NIST announces the publication of a Cybersecurity White Paper (CSWP), Planning for a Zero Trust Architecture: A Guide for Federal Administrators , which describes processes for migrating to a zero trust architecture using the NIST Risk Management Framework (RMF).
Zero trust is a set of principles designed to reduce or remove implicit trust in networked systems by addressing network identity, endpoint health, and data flows. This white paper helps system administrators and operators use the RMF when designing and implementing zero trust architecture by describing how the steps in the RMF map to similar steps described in NIST Special Publication (SP) 800-207, Zero Trust Architecture . The document also provides an abstract logical architecture on which to map gaps and solutions, as well as additional resources for federal agency administrators, planners, and managers.
Related Topics
Security and Privacy: planning , risk management , zero trust
Technologies: networks
Applications: enterprise
A security review of local government using NIST CSF: a case study
- Open access
- Published: 12 July 2018
- Volume 74 , pages 5171–5186, ( 2018 )
Cite this article
You have full access to this open access article
- Ahmed Ibrahim ORCID: orcid.org/0000-0002-4760-3533 1 ,
- Craig Valli 1 ,
- Ian McAteer 1 &
- Junaid Chaudhry 2
13k Accesses
17 Citations
Explore all metrics
A Correction to this article was published on 02 September 2019
This article has been updated
Evaluating cyber security risk is a challenging task regardless of an organisation’s nature of business or size, however, an essential activity. This paper uses the National Institute of Standards and Technology (NIST) cyber security framework (CSF) to assess the cyber security posture of a local government organisation in Western Australia. Our approach enabled the quantification of risks for specific NIST CSF core functions and respective categories and allowed making recommendations to address the gaps discovered to attain the desired level of compliance. This has led the organisation to strategically target areas related to their people, processes, and technologies, thus mitigating current and future threats.
Similar content being viewed by others
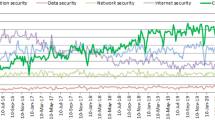
AI-Driven Cybersecurity: An Overview, Security Intelligence Modeling and Research Directions
Iqbal H. Sarker, Md Hasan Furhad & Raza Nowrozy
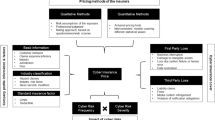
Cyber risk and cybersecurity: a systematic review of data availability
Frank Cremer, Barry Sheehan, … Stefan Materne
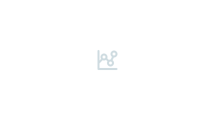
An overview of cybercrime law in South Africa
Sizwe Snail ka Mtuze & Melody Musoni
Avoid common mistakes on your manuscript.
1 Introduction
The National Institute of Standards and Technology (NIST) Cyber Security Framework (CSF) [ 28 ] is a risk-based approach to manage risks organisations face from a cyber security perspective. Similarly, several frameworks such as NIST SP 800-53 [ 27 ], COBIT5 [ 17 ], ISO/IEC 27001:2013 [ 23 ], ISA 62443-2-1:2009 [ 21 ], and ISA 62443-3-3:2013 [ 22 ] are being used to assess cyber security risk from different perspectives and outcomes are measured using different yardsticks. Often, navigating the various frameworks can be challenging for organisations, especially if such expertise are not present internally. Given the rapidly changing technology and threat landscape, assessing the cyber security posture of an organisation, regardless of their business or size, is paramount.
Our focus of this paper is to demonstrate the application (Sect. 3 ) of NIST CSF in a local government organisation and provide recommendations (Sect. 5 ) based on our findings (Sect. 4 ).
The main contributions of this paper are:
The adoption of the NIST CSF as an Assessment Tool and targeting different levels of the organisation, depending on their level of expertise and job function to obtain responses to facilitate assessment.
Quantification of the assessment to reflect severity of actual risk, which in turn enabled the organisation to effectively address the issues to attain desired level of compliance.
A detailed review of similar frameworks used in the industry and relevant case studies (Sect. 6 ).
The next section provides a background of the NIST CSF and its components. We recommend the reader to refer to NIST [ 28 ] for additional details and strategies for suitable approaches to implement, which would vary from organisation to organisation.
2 The NIST CSF
The NIST CSF [ 28 ] consists of the Framework Core , the Framework Implementation Tiers , and the Framework Profiles . The Framework Core consists of five concurrent and continuous functions; Identify , Protect , Detect , Respond , and Recover . We designed an Assessment Tool for our investigation based on these functions, which provided a systematic approach to ascertain the organisations cyber security risk management practices and processes.
The Framework Implementation Tiers describe the level an organisations cyber security risk management practices that comply with the framework. Tiers provide context and degree to which cyber security risks are managed and extent to which business needs are considered in cyber security risk management. The Assessment Tool enabled the determination of the organisations Current Tier based on various internal and external factors such as their risk management practices, threat environment, legal and regulatory requirements, business/mission objectives, and organisational constraints. Organisations should also determine the Desired Tier , provided it is feasible to implement, reduces cyber security risks, and meets the organisational goals. The following are descriptions of the tier levels [ 28 ]:
Tier-1 (Partial): risk management practices are not formalised and managed in an ad hoc manner, lack awareness of cyber security risks organisation wide, and do not have processes in place to collaborate with external entities.
Tier-2 (Risk Informed): risk management practices are formalised but not integrated organisation wide, but cyber security activities are prioritised based on risks with adequate means to perform related duties, with informal means to communicate cyber security information internally and externally.
Tier-3 (Repeatable): risk management practices are formalised and policies are in place and are adaptable to cyber threats. Organisation-wide approach is required to manage cyber security with skilled and knowledgeable personnel to respond and understand dependencies and role of external partners.
Tier-4 (Adaptive): cyber security practices are based on lessons learnt and predictive indicators, with continuous improvement, adaptability, and timely response. Organisation-wide approach to manage cyber security risks is part of the organisational culture and actively shares with external partners.
The Framework Profile represents the outcomes based on the business needs the organisation characterised from the Framework Core and determined using the Assessment Tool. Consequently, a Current Profile (the “as is” state) and a Target Profile (the “to be” state) can be used to identify opportunities for improving the cyber security of the organisation [ 28 ]. Framework profiles can be determined based on particular implementation scenarios, and therefore, the gap between Current Profile and Target Profile would vary as per scenario. In this paper, a local government-specific approach to CSF was adapted. However, industry-specific tailoring may be performed for the CSF.
3 Methodology
The NIST CSF allowed us to design an Assessment Tool targeted at three levels of participants within the organisation, i.e. executive, management and technical. The rationale was to ascertain organisation-wide understanding of cyber security risks. Hence, the Assessment Tool comprised of questions addressing the requirements outlined as per the NIST CSF.
The questions were selected based on the nature and relevance to the level of participant. This is because the NIST CSF comprised of questions that were both technical and non-technical. Therefore, it would have been unrealistic to expect deep knowledge of technical operations or implementation level details from a policy level executive.
In order to assist us determine a baseline (i.e. the Desired Tier), additional questions were included in the Assessment Tool to determine the nature of the organisation and its business. This was then followed by the remaining requirements comprised in the NIST CSF.
3.1 Determining compliance
The compliance for each measure was based on the responses provided by the participants. They were graded as either, Complaint , Partially Compliant , or Non-Compliant ; and each was assigned scores of either 10, 5, or 0, respectively, for each core function’s subcategory. Any subcategory that was not applicable depending on the Desired Tier level was excluded from the compliance score calculation.
Given the number of security requirements for each Core Function’s subcategory is N , then the number of applicable requirements in each subcategory given the Desired Tier level is \(N^{\prime }\) . Therefore, the total compliance score C for each core function’s category can be defined as:
where R is the compliance score for each category of the respective Core Function.
Additionally, a detailed document audit was conducted on existing policies and procedures. The Information Technology (IT) infrastructure (internal, remote locations, and cloud) were reviewed, and a detailed internal vulnerability assessment was also conducted during our investigation.
The responses provided by the Executive, Management, and Technical participants gave insight into the organisation’s cyber security posture. Table 1 shows the summary of the compliance of NIST CSF assessment. The compliance scores were determined based on Eq. 1 presented previously.
For Identify core function, the organisation scored 36%. Their ability to track assets centrally, keep management informed, and understand operational risks from a cyber security perspective was limited, while a strategy to manage such risks did not exist. However, the organisation understood its business well and were able set priorities to support risk management decisions.
Access to physical/virtual assets were through authorisation and well-defined processes. The staff were trained and informed adequately of information security related duties and responsibilities. Certain aspects of data security related to confidentiality and availability were done reasonably well, however, assuring integrity of data needed improvement. Similarly, local maintenance and remote maintenance of IT infrastructure were carried out in a manner consistent to policies and procedures. However, relevant policies, processes, and procedures, as well as technology to assist the protection of information systems and relevant assets, were lacking. Therefore, in aggregate, the organisation scored 45% compliance for Protect core function.
The organisation scored weakest in the detection of cyber security incidents with a score of 25%. Although certain monitoring activities were in place to track physical security and malicious code, timely detection of anomalous activities and detection processes were lacking or non-existent.
Despite the lack of a specific response plan to respond to a cyber security events, the organisation had measures in place to report incidents and coordinate activities to respond adequately, which resulted in a 38% compliance score for Respond core function. These practices are updated from time to time; however, mechanism to perform post-incident analysis or to mitigate future cyber security events has not been implemented presently.
Interestingly, the organisation was well prepared to deal with recovery and resumption of core services after a cyber security event. The recovery plans in place are tested, updated, and improved periodically, thus receiving full compliance for Recover core functionality of the framework.
5 Recommendations
Based on the findings, the following recommendations were made with respect to each core function of the NIST CSF.
5.1 Identify
Establish a central inventory of assets, including physical devices and systems, software, and external systems with all required information and prioritise based on classification, criticality, and business value.
Identify the organisations role in the supply chain (i.e. producer-consumer model) as it captures and retains public data, collects revenue, and provides services to its stakeholders.
Establish an Information Security policy and reference relevant federal and state policies regarding cyber security to ensure legal and regulatory requirements are understood and managed.
Identify and prioritise threats and vulnerabilities, both internal and external, to determine cyber security risks to the organisations operations, assets, and individuals.
Establish risk management processes that are managed and agreed to by stakeholders to support operational risk decisions.
5.2 Protect
Strengthen the Access Control policy and procedures for organisation-wide assets that require both physical and remote access.
Sensitise and increase awareness about cyber security throughout the workforce more comprehensively and provide adequate cyber security training based on roles and responsibilities. In this regard, clearly describe cyber security roles and responsibilities for relevant staff and external stakeholders.
Enforce required provisions for data security in the policy and implement data-at-rest and data-in-transit security, and integrity-checking mechanisms to ensure confidentiality, integrity, and availability of information and data.
Establish required policies, processes, and procedures to manage protection of information assets. This include establishment of lacking policies and processes, particularly for configuration management, data destruction, and physical operating environment; identification of security baselines; SDLC for system management; formulate vulnerability, response, and recovery plans.
Strengthen processes that control and log remote access to organisational assets by external maintenance contractors.
Establish a central log of organisation-wide information systems and devices, establish Removable Media policy, and strengthen network segregation to protect communications and controls networks.
Determine baselines for network operations and data flows, implement appropriate activities to detect and analyse events based on event data aggregated from multiple sources and sensors. Determine incident impact and threshold to prepare and allocate resources appropriately.
Implement tools to monitor cyber and physical environments to detect unauthorised mobile code, external service provider activities, and unauthorised access. Perform organisation-wide vulnerabilities regularly.
Outline detection requirements in Information Security policy and continuously improve these processes to ensure timely and adequate awareness of anomalous events.
5.4 Respond
Establish processes and procedures to respond to cyber security events in a timely manner.
Define cyber security roles and responsibilities in Information Security policy to ensure activities are coordinated for internal and external stakeholders including law enforcement in response to cyber security events.
Implement required cyber security events notification and detection systems to ensure adequate information is available to analyse and understand the impact to support recovery activities.
Implement required cyber security controls to detect, report, and contain incidents to prevent escalation of an incident, mitigate its effect, and eradicate the incidents.
Each of the above recommendations also had specific internal stakeholder(s) identified to indicate ownership and responsibility for addressing the issues associated. Consequently, the organisation was then able to develop strategies to address the issues identified, and assign specific tasks to individuals. For this purpose, the organisation established an internal document using Microsoft Power BI [ 25 ] (typically referred to as a Power BI site) to track and visualise the status of the NIST CSF assessment (Fig. 1 ).
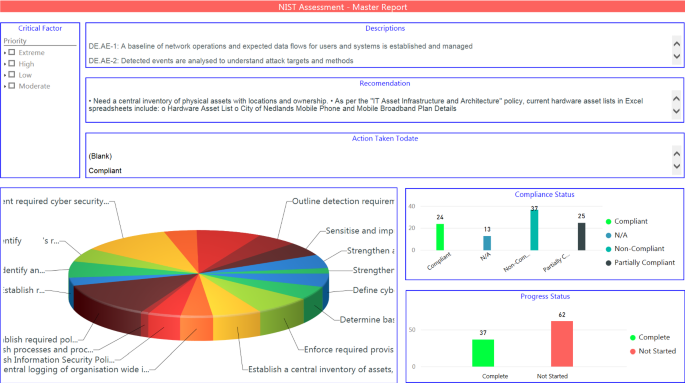
Microsoft Power BI Internal Site for tracking, visualising, and reporting NIST CSF assessment findings, courtesy of the participating local government organisation
The Power BI site facilitated transparency, visibility, and central reporting throughout the organisation. Intuitively, this resulted in a rapid and responsive drive for the organisation to address and prioritise issues based on severity and cost, with the goal of achieving Tier-2 compliance.
Furthermore, a desire to achieve a higher compliance level such as Tier-3 was expressed. Such aspiration is encouraged, however, with caution. Even though a higher level of compliance will improve the cyber security posture of the organisation, it will also affect other aspects such as resources and cost. For example, when contrasting the Risk Management Process between Tier-2 and Tier-3 as defined in the NIST CSF [ 28 ]:
Implementation of risk management practices are not mandatory in Tier-2, whereas these have to be implemented as organisation-wide policies in Tier-3. Thus, Tier-3 organisations should have procedures, processes, technology, and human resources to implement relevant policies.
The cyber security activities’ priorities are updated in a passive nature in Tier-2 as opposed to regular active updates and constant re-evaluation of priorities for Tier-3 compliance. To acquire such capability, an organisation requires adequate technology, skilled human resources, and relevant policies that would enable keeping pace with the changes in the technology and threat landscape.
In addition to the two points highlighted above, considering both Integrated Risk Management Program and External Participation [ 28 ], significant investment in resources and human skills development or acquisition is needed to make the transition from Tier-2 to Tier-3. Moreover, this should only be considered carefully based on the organisation’s business requirements, strategic objectives, budget, risk appetite, and current and future threats.
6 Related frameworks
The diversity and complexity of Information Technology (IT) system components have increased significantly in recent years. Consequently, in order for businesses to adequately secure these systems, several standards and frameworks have been developed [ 2 ]. Such frameworks need to be applicable to all manner of business sectors, be they government or private, enterprise or small-business. Tables 2 and 3 provide a summary of useful examples of how both NIST SP 800-53 and ISO/IEC 27001:2013 frameworks have been applied in practice.
Since NIST CSF can be considered as a high-level abstraction of related frameworks, it provides references to other related frameworks for specific implementation guidelines. These referenced frameworks include:
NIST SP 800-53 Rev. 4.
Control Objectives for Information and Related Technologies (COBIT5).
ISO/IEC 27001:2013.
ISA 62443-2-1:2009.
ISA 62443-3-3:2013.
These are further described below.
6.1 NIST SP 800-53 Rev. 4
NIST SP 800-53 [ 27 ] revisions are made according to changes in responsibilities under the Federal Information Security Management Act (FISMA), Public Law (P.L.) 107-347. The latest version of this framework consists of five functions (Identify, Protect, Detect, Respond, and Recover), 22 categories, and 98 subcategories. This framework utilises a four-tier security model (Partial, Risk Informed, Repeatable, and Adaptive) and a seven-step process (Prioritise and Scope, Orient, Create a Current Profile, Conduct a Risk Assessment, Create a Target Profile, Determine, Analyse and Prioritise Gaps, and Implement Action Plan). It focuses on assessing the current situation by determining how to assess security, how to consider risk, and how to resolve the security threats.
6.2 Control Objectives for Information and Related Technologies (COBIT5)
COBIT5 [ 17 ] is a business CSF designed for the governance and maintenance of enterprise IT systems. It consists of five domains and 37 processes in line with the responsibility areas of plan, build, run, and monitor. COBIT5 is aligned and coordinated with other recognised IT standards and good practices, such as NIST, ISO 27000, COSO, ITIL, BiSL, CMMI, TOGAF and PMBOK. It is built around the following considerations:
The need to meet stakeholder expectations.
The end-to-end process control of the enterprise.
To work as a single integrated framework.
Recognising that “Management” and “Governance” are two different things.
6.3 ISO/IEC 27001:2013
ISO/IEC 27001:2013 [ 23 ] is an international information security standard published by the International Organisation for Standardisation (ISO) and the International Electrotechnical Commission (IEC), which originated from the British Standard, BS 7799. This framework consists of 114 controls in 14 groups describing the requirements needed to design and implement an Information Security Management Systems (ISMS). Version 2 released in 2013 replaces the 2005 version 1 edition. It is a standard that should be instigated by all businesses where information security is a critical factor, but in particular, applies to software development, managed service providers/hosting services providers, IT, banking and insurance, information management, government agencies and their service providers, and E-commerce merchants [ 23 ].
6.4 ISA 62443-2-1:2009
ISA 62443-2-1:2009 [ 21 ] is an International Standards on Auditing (ISA) standard covering the elements required to develop an Industrial Automation Control System Security Management System (IACS-SMS). It consists of three categories, three element groups, and 22 elements. The framework is the first of four ISA policy and procedure products that identifies the essentials necessary to establish an effective cyber security management system (CSMS). However, the step-by-step approach as to how this is achieved is company-specific and according to their own business culture. These essentials are:
Risk analysis.
Addressing risk with the CSMS.
Monitoring and improving the CSMS.
6.5 ISA 62443-3-3:2013
ISA 62443-3-3:2013 [ 22 ] is an International Standards on Auditing (ISA) standard covering the elements required for cyber security controls of industrial control systems (ICS). It consists of seven Foundation Requirements and 51 System Requirements.
ISA 62443-3-3:2013 is the third of three ISA systems products, that outlines system security requirements and security levels [ 22 ].
6.6 Other frameworks
In addition to the above, other frameworks used in the industry include:
Committee of Sponsoring Organizations of the Treadway Commission (COSO) is an enterprise risk management standard, designed jointly by five leading associations, with the aim of integrating strategy and performance [ 13 ].
Council on CyberSecurity Top 20 Critical Security Controls (CCS CSC) consists of a prioritised set of actions, originally developed by the SANS Institute, to protect assets from cyber attack [ 12 ].
ISF Standard of Good Practice (SoGP) is a standard aimed at providing controls and guidance on all aspects of information security [ 20 ].
ETSI Cyber Security Technical Committee (TC Cyber) was developed to improve standards within the European telecommunications sector [ 15 ].
Sherwood Applied Business Security Architecture (SABSA) Enhanced NIST Cybersecurity (SENC) project enhances the five core levels of the NIST CSF into a SABSA model consisting of a six-level security architecture [ 30 ].
IASME Consortium (IASME) is an information assurance standard based on ISO 27000, but aimed at small businesses [ 18 ].
RFC 2196 - Site Security Handbook (SSH) represents a guide on how to develop computer security policies and procedures [ 19 ].
Health Information Trust Alliance (HITRUST) is the first IT security CSF designed specifically for the healthcare sector. It is based on existing NIST standards and is aimed at healthcare and information security professionals [ 16 ].
North American Electric Reliability Corporation Critical Infrastructure Protection (NERC-CIP) version 5 is a set of requirements needed to secure the assets of the North American bulk electric system [ 14 ].
Open Security Architecture (OSA) is a free community-owned resource of advice on the selection, design, and integration of devices required to provide security and control of an IT network [ 29 ].
Good Practice Guide 13 (GPG13) is a UK government CSF related to Code of Connection (CoCo) compliance for businesses to secure IT systems [ 10 ].
7 Conclusion
In this paper we have used the NIST CSF to evaluate the cyber security risks of a local government organisation in Western Australia. Our approach can be used to derive measurable metrics for each Framework Core function and respective categories, thus enabling the organisation to ascertain the cyber security preparedness to actual risk.
Our findings suggest that evaluating the Desired Tier compliance to the NIST CSF helps identify the specific people, process, and technology areas that require improvement (i.e. gaps), which directly influence threat mitigation. The application of CSF helped us understand the current security context of the organisation while identifying the risks and future growth areas to improve. While higher tier compliance maybe desired, we have also recommended that the organisation’s business requirements, strategic goals, budget, risk appetite, and current and future threats to be considered carefully.
Furthermore, as we have presented several related frameworks, navigating such frameworks for self assessment can be challenging, often not intended by design even, but not impossible. We have observed that the NIST CSF offers an advantage over other frameworks in this regard. However, there is still room for developing additional tools that would simplify the implementation process and speed up adoption.
Therefore, our future work will aim to improve the current Assessment Tool we have used, with a focus of making it adaptable and accessible to a wider audience and measurable for accurate quantification of cyber preparedness.
Change history
02 september 2019.
The original version of this article was revised due to a retrospective Open Access order.
Abrams M, Weiss J (2008) Malicious control system cyber security attack case study: Maroochy water services, Australia. https://www.mitre.org/sites/default/files/pdf/08_1145.pdf . Accessed 29 Jan 2018
Angelini M, Lenti S, Santucci G (2017) Crumbs: a cyber security framework browser. In: 2017 IEEE Symposium on Visualization for Cyber Security (VizSec). IEEE, pp 1–8
BSI Group (2011) Case study Thames Security Shredding (TSS) Ltd. https://www.bsigroup.com/Documents/iso-27001/case-studies/BSI-ISO-IEC-27001-case-study-Thames-Security-UK-EN.pdf?epslanguage=en-MY . Accessed 15 Feb 2018
BSI Group (2012) How Fredrickson has reduced third party scrutiny and protected its reputation with ISO 27001 certification. https://www.bsigroup.com/Documents/iso-27001/case-studies/BSI-ISO-IEC-27001-case-study-Fredrickson-International-EN-UK.pdf?epslanguage=en-MY . Accessed 15 Feb 2018
BSI Group (2013) Implementing best practice and improving client confidence with ISO/IEC 27001. https://www.bsigroup.com/Documents/iso-27001/case-studies/BSI-ISO-IEC-27001-case-study-Legal-Ombudsman-UK-EN.pdf . Accessed 15 Feb 2018
BSI Group (2013) Infoview case study. https://www.bsigroup.com/LocalFiles/EN-AU/_Case%20Studies/BSI%20Infoview%20Case%20Study.pdf . Accessed 15 Feb 2018
BSI Group (2013) Supporting business growth with ISO/IEC 27001. https://www.bsigroup.com/Documents/iso-27001/case-studies/BSI-ISO-IEC-27001-case-study-SVM-UK-EN.pdf . Accessed 15 Feb 2018
BSI Group (2014) Using ISO/IEC 27001 certification to increase resilience, reassure clients and gain a competitive edge. https://www.bsigroup.com/Documents/iso-27001/case-studies/BSI-ISO-IEC-27001-case-study-Capgemini-UK-EN.pdf . Accessed 15 Feb 2018
BSI Group (2015) Integrating management systems to improve business performance and achieve sustained competitive advantage. https://www.bsigroup.com/Documents/iso-22301/case-studies/Costain-case-study-UK-EN.pdf . Accessed 15 Feb 2018
Cabinet Office (2010) Gpg13: Protective monitoring controls. http://gpg13.com/executive-summary/ . Accessed 13 Mar 2018
Casey T, Fiftal K, Landfield K, Miller J, Morgan D, Willis B (2015) The cybersecurity framework in action: an Intel use case. Intel Corporation, pp 1–10. https://supplier.intel.com/static/governance/documents/The-cybersecurity-framework-in-action-an-intel-use-case-brief.pdf . Accessed 30 Jan 2018
Center for Internet Security (2018) CIS controls. https://www.cisecurity.org/controls/ . Accessed 6 Mar 2018
COSO (2017) Guidance on enterprise risk management. https://www.coso.org/Pages/erm.aspx . Accessed 6 Mar 2018
Elkins V (2014) Summary of CIP version 5 standards. http://www.velaw.com/uploadedfiles/vesite/resources/summarycipversion5standards2014.pdf . Accessed 12 Feb 2018
ETSI (2017) Overview of cybersecurity. https://www.enisa.europa.eu/events/enisa-cscg-2017/presentations/brookson . Accessed 7 Mar 2018
HITRUST (2017) Introduction to the HITRUST CSF. https://hitrustalliance.net/documents/csf_rmf_related/v9/CSFv9Introduction.pdf . Accessed 21 Mar 2018
IASCA (2012) Cobit 5. https://cobitonline.isaca.org/ . Accessed 01 Feb 2018
IASME Consortium (2014) About cyber essentials. https://www.iasme.co.uk/cyberessentials/about-cyber-essentials/ . Accessed 07 Mar 2018
IETF (1997) Rfc 2196: site security handbook. https://www.ietf.org/rfc/rfc2196.txt . Accessed 8 Mar 2018
Information Security Forum (2016) The ISF standard of good practice for information security. https://www.securityforum.org/tool/the-isf-standardrmation-security/ . Accessed 8 Mar 2018
ISA (2009) ANSI/ISA-99.02.01-2009. http://www.icsdefender.ir/files/scadadefender-ir/paygahdaHrBnesh/standards/ISA-62443-2-1-Public.pdfHrB . Accessed 13 Mar 2018
ISA (2012) ANSI/ISA-62443-3-3 (99.03.03)-2013. http://www.icsdefender.ir/files/scadadefender-ir/paygahdanesh/standards/ISA-62443-3-3-Public.pdf . Accessed 13 Mar 2018
ISO (2013) ISO/IEC 27001:2013. https://www.iso.org/standard/54534.html . Accessed 1 Feb 2018
Kim EB (2014) Recommendations for information security awareness training for college students. Inf Manag Comput Secur 22(1):115–126. https://doi.org/10.1108/IMCS-01-2013-0005
Article Google Scholar
Microsoft (2018) Power BI. https://powerbi.microsoft.com/en-us/ . Accessed 12 Apr 2018
Montesino R, Fenz S, Baluja W (2012) Siem-based framework for security controls automation. Inf Manag Comput Secur 20(4):248–263. https://doi.org/10.1108/09685221211267639
NIST (2014) Assessing security and privacy controls in federal information systems and organizations. http://nvlpubs.nist.gov/nistpubs/SpecialPublications/NIST.SP.800-53Ar4.pdf . Accessed 1 Feb 2018
NIST (2014) Framework for improving critical infrastructure cybersecurity: Version 1.0. https://www.nist.gov/sites/default/files/documents/cyberframework/cybersecurity-framework-021214.pdf . Accessed 30 Jan 2018
OSA (2007) Osa landscape. http://www.opensecurityarchitecture.org/cms/foundations/osa-landscape . Accessed 15 Mar 2018
SABSA (2015) Project charter for the development of a SABSA enhanced nist cybersecurity framework. https://sabsa.org/sabsa-nist-framework-project/ . Accessed 21 Mar 2018
Sweeney S (2015) How the University of Pittsburgh is using the NIST cybersecurity framework. https://www.sei.cmu.edu/podcasts/podcast_episode.cfm?episodeid=445056&autostarter=1&wtpodcast=howtheuniversityofpittsburghisusingthenistcybersecurityframework . Accessed 1 Feb 2018
University of Chicago (2016) Applying the cybersecurity framework at the university of Chicago: an education case study. http://security.bsd.uchicago.edu/wp-content/uploads/sites/2/2016/04/BSD-Framework-Implementation-Case-Study_final_edition.pdf . Accessed 31 Jan 2018
Download references
Acknowledgements
We would like to thank the Western Australia local government organisation for sharing their case study for this research. We would also like to thank their staff for their support and cooperation during the assessment.
Author information
Authors and affiliations.
Security Research Institute, School of Science, Edith Cowan University, 270 Joondalup Drive, Perth, WA, 6027, Australia
Ahmed Ibrahim, Craig Valli & Ian McAteer
College of Security and Intelligence, Embry-Riddle Aeronautical University, Prescott, AZ, USA
Junaid Chaudhry
You can also search for this author in PubMed Google Scholar
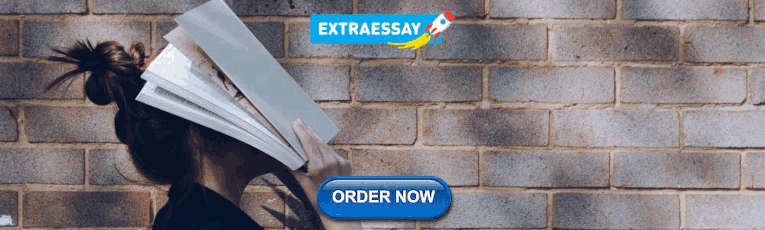
Corresponding author
Correspondence to Ahmed Ibrahim .
Additional information
Rights and permissions.
Open Access This article is distributed under the terms of the Creative Commons Attribution 4.0 International License ( http://creativecommons.org/licenses/by/4.0/ ), which permits use, duplication, adaptation, distribution and reproduction in any medium or format, as long as you give appropriate credit to the original author(s) and the source, provide a link to the Creative Commons license and indicate if changes were made.
Reprints and permissions
About this article
Ibrahim, A., Valli, C., McAteer, I. et al. A security review of local government using NIST CSF: a case study. J Supercomput 74 , 5171–5186 (2018). https://doi.org/10.1007/s11227-018-2479-2
Download citation
Published : 12 July 2018
Issue Date : October 2018
DOI : https://doi.org/10.1007/s11227-018-2479-2
Share this article
Anyone you share the following link with will be able to read this content:
Sorry, a shareable link is not currently available for this article.
Provided by the Springer Nature SharedIt content-sharing initiative
- NIST cyber security framework
- Local government
- Cyber security
- Risk assessment
- Find a journal
- Publish with us
- Track your research

Can I hire someone to write essay?
Student life is associated with great stress and nervous breakdowns, so young guys and girls urgently need outside help. There are sites that take all the responsibility for themselves. You can turn to such companies for help and they will do all the work while clients relax and enjoy a carefree life.
Take the choice of such sites very seriously, because now you can meet scammers and low-skilled workers.
On our website, polite managers will advise you on all the details of cooperation and sign an agreement so that you are confident in the agency. In this case, the user is the boss who hires the employee to delegate responsibilities and devote themselves to more important tasks. You can correct the work of the writer at all stages, observe that all special wishes are implemented and give advice. You pay for the work only if you liked the essay and passed the plagiarism check.
We will be happy to help you complete a task of any complexity and volume, we will listen to special requirements and make sure that you will be the best student in your group.
Artikel & Berita
Write my essay for me.

BitterSweet: Building machine learning models for predicting the bitter and sweet taste of small molecules
Rudraksh Tuwani, Somin Wadhwa & Ganesh Bagler
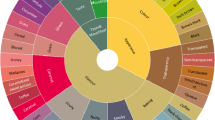
Sensory lexicon and aroma volatiles analysis of brewing malt
Xiaoxia Su, Miao Yu, … Tianyi Du
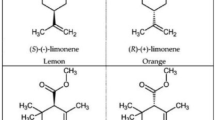
Predicting odor from molecular structure: a multi-label classification approach
Kushagra Saini & Venkatnarayan Ramanathan
Introduction
Predicting and understanding food perception and appreciation is one of the major challenges in food science. Accurate modeling of food flavor and appreciation could yield important opportunities for both producers and consumers, including quality control, product fingerprinting, counterfeit detection, spoilage detection, and the development of new products and product combinations (food pairing) 1 , 2 , 3 , 4 , 5 , 6 . Accurate models for flavor and consumer appreciation would contribute greatly to our scientific understanding of how humans perceive and appreciate flavor. Moreover, accurate predictive models would also facilitate and standardize existing food assessment methods and could supplement or replace assessments by trained and consumer tasting panels, which are variable, expensive and time-consuming 7 , 8 , 9 . Lastly, apart from providing objective, quantitative, accurate and contextual information that can help producers, models can also guide consumers in understanding their personal preferences 10 .
Despite the myriad of applications, predicting food flavor and appreciation from its chemical properties remains a largely elusive goal in sensory science, especially for complex food and beverages 11 , 12 . A key obstacle is the immense number of flavor-active chemicals underlying food flavor. Flavor compounds can vary widely in chemical structure and concentration, making them technically challenging and labor-intensive to quantify, even in the face of innovations in metabolomics, such as non-targeted metabolic fingerprinting 13 , 14 . Moreover, sensory analysis is perhaps even more complicated. Flavor perception is highly complex, resulting from hundreds of different molecules interacting at the physiochemical and sensorial level. Sensory perception is often non-linear, characterized by complex and concentration-dependent synergistic and antagonistic effects 15 , 16 , 17 , 18 , 19 , 20 , 21 that are further convoluted by the genetics, environment, culture and psychology of consumers 22 , 23 , 24 . Perceived flavor is therefore difficult to measure, with problems of sensitivity, accuracy, and reproducibility that can only be resolved by gathering sufficiently large datasets 25 . Trained tasting panels are considered the prime source of quality sensory data, but require meticulous training, are low throughput and high cost. Public databases containing consumer reviews of food products could provide a valuable alternative, especially for studying appreciation scores, which do not require formal training 25 . Public databases offer the advantage of amassing large amounts of data, increasing the statistical power to identify potential drivers of appreciation. However, public datasets suffer from biases, including a bias in the volunteers that contribute to the database, as well as confounding factors such as price, cult status and psychological conformity towards previous ratings of the product.
Classical multivariate statistics and machine learning methods have been used to predict flavor of specific compounds by, for example, linking structural properties of a compound to its potential biological activities or linking concentrations of specific compounds to sensory profiles 1 , 26 . Importantly, most previous studies focused on predicting organoleptic properties of single compounds (often based on their chemical structure) 27 , 28 , 29 , 30 , 31 , 32 , 33 , thus ignoring the fact that these compounds are present in a complex matrix in food or beverages and excluding complex interactions between compounds. Moreover, the classical statistics commonly used in sensory science 34 , 35 , 36 , 37 , 38 , 39 require a large sample size and sufficient variance amongst predictors to create accurate models. They are not fit for studying an extensive set of hundreds of interacting flavor compounds, since they are sensitive to outliers, have a high tendency to overfit and are less suited for non-linear and discontinuous relationships 40 .
In this study, we combine extensive chemical analyses and sensory data of a set of different commercial beers with machine learning approaches to develop models that predict taste, smell, mouthfeel and appreciation from compound concentrations. Beer is particularly suited to model the relationship between chemistry, flavor and appreciation. First, beer is a complex product, consisting of thousands of flavor compounds that partake in complex sensory interactions 41 , 42 , 43 . This chemical diversity arises from the raw materials (malt, yeast, hops, water and spices) and biochemical conversions during the brewing process (kilning, mashing, boiling, fermentation, maturation and aging) 44 , 45 . Second, the advent of the internet saw beer consumers embrace online review platforms, such as RateBeer (ZX Ventures, Anheuser-Busch InBev SA/NV) and BeerAdvocate (Next Glass, inc.). In this way, the beer community provides massive data sets of beer flavor and appreciation scores, creating extraordinarily large sensory databases to complement the analyses of our professional sensory panel. Specifically, we characterize over 200 chemical properties of 250 commercial beers, spread across 22 beer styles, and link these to the descriptive sensory profiling data of a 16-person in-house trained tasting panel and data acquired from over 180,000 public consumer reviews. These unique and extensive datasets enable us to train a suite of machine learning models to predict flavor and appreciation from a beer’s chemical profile. Dissection of the best-performing models allows us to pinpoint specific compounds as potential drivers of beer flavor and appreciation. Follow-up experiments confirm the importance of these compounds and ultimately allow us to significantly improve the flavor and appreciation of selected commercial beers. Together, our study represents a significant step towards understanding complex flavors and reinforces the value of machine learning to develop and refine complex foods. In this way, it represents a stepping stone for further computer-aided food engineering applications 46 .
To generate a comprehensive dataset on beer flavor, we selected 250 commercial Belgian beers across 22 different beer styles (Supplementary Fig. S1 ). Beers with ≤ 4.2% alcohol by volume (ABV) were classified as non-alcoholic and low-alcoholic. Blonds and Tripels constitute a significant portion of the dataset (12.4% and 11.2%, respectively) reflecting their presence on the Belgian beer market and the heterogeneity of beers within these styles. By contrast, lager beers are less diverse and dominated by a handful of brands. Rare styles such as Brut or Faro make up only a small fraction of the dataset (2% and 1%, respectively) because fewer of these beers are produced and because they are dominated by distinct characteristics in terms of flavor and chemical composition.
Extensive analysis identifies relationships between chemical compounds in beer
For each beer, we measured 226 different chemical properties, including common brewing parameters such as alcohol content, iso-alpha acids, pH, sugar concentration 47 , and over 200 flavor compounds (Methods, Supplementary Table S1 ). A large portion (37.2%) are terpenoids arising from hopping, responsible for herbal and fruity flavors 16 , 48 . A second major category are yeast metabolites, such as esters and alcohols, that result in fruity and solvent notes 48 , 49 , 50 . Other measured compounds are primarily derived from malt, or other microbes such as non- Saccharomyces yeasts and bacteria (‘wild flora’). Compounds that arise from spices or staling are labeled under ‘Others’. Five attributes (caloric value, total acids and total ester, hop aroma and sulfur compounds) are calculated from multiple individually measured compounds.
As a first step in identifying relationships between chemical properties, we determined correlations between the concentrations of the compounds (Fig. 1 , upper panel, Supplementary Data 1 and 2 , and Supplementary Fig. S2 . For the sake of clarity, only a subset of the measured compounds is shown in Fig. 1 ). Compounds of the same origin typically show a positive correlation, while absence of correlation hints at parameters varying independently. For example, the hop aroma compounds citronellol, and alpha-terpineol show moderate correlations with each other (Spearman’s rho=0.39 and 0.57), but not with the bittering hop component iso-alpha acids (Spearman’s rho=0.16 and −0.07). This illustrates how brewers can independently modify hop aroma and bitterness by selecting hop varieties and dosage time. If hops are added early in the boiling phase, chemical conversions increase bitterness while aromas evaporate, conversely, late addition of hops preserves aroma but limits bitterness 51 . Similarly, hop-derived iso-alpha acids show a strong anti-correlation with lactic acid and acetic acid, likely reflecting growth inhibition of lactic acid and acetic acid bacteria, or the consequent use of fewer hops in sour beer styles, such as West Flanders ales and Fruit beers, that rely on these bacteria for their distinct flavors 52 . Finally, yeast-derived esters (ethyl acetate, ethyl decanoate, ethyl hexanoate, ethyl octanoate) and alcohols (ethanol, isoamyl alcohol, isobutanol, and glycerol), correlate with Spearman coefficients above 0.5, suggesting that these secondary metabolites are correlated with the yeast genetic background and/or fermentation parameters and may be difficult to influence individually, although the choice of yeast strain may offer some control 53 .
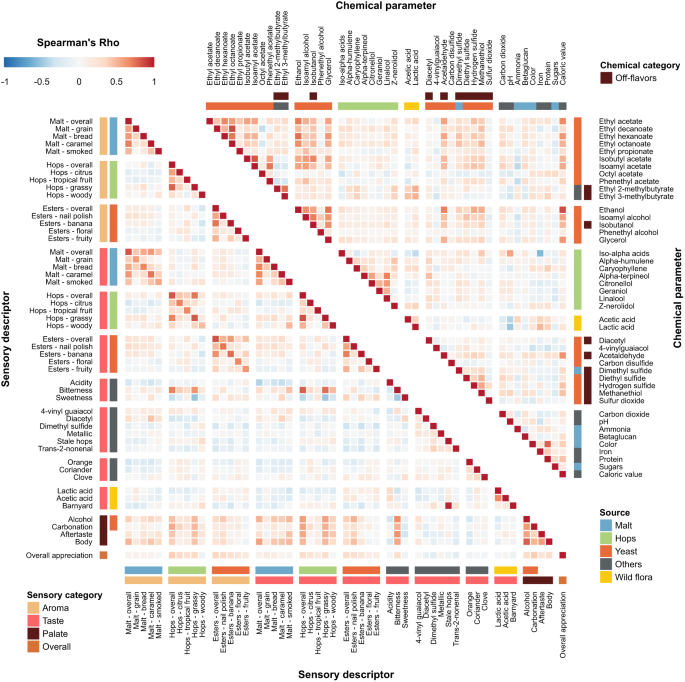
Spearman rank correlations are shown. Descriptors are grouped according to their origin (malt (blue), hops (green), yeast (red), wild flora (yellow), Others (black)), and sensory aspect (aroma, taste, palate, and overall appreciation). Please note that for the chemical compounds, for the sake of clarity, only a subset of the total number of measured compounds is shown, with an emphasis on the key compounds for each source. For more details, see the main text and Methods section. Chemical data can be found in Supplementary Data 1 , correlations between all chemical compounds are depicted in Supplementary Fig. S2 and correlation values can be found in Supplementary Data 2 . See Supplementary Data 4 for sensory panel assessments and Supplementary Data 5 for correlation values between all sensory descriptors.
Interestingly, different beer styles show distinct patterns for some flavor compounds (Supplementary Fig. S3 ). These observations agree with expectations for key beer styles, and serve as a control for our measurements. For instance, Stouts generally show high values for color (darker), while hoppy beers contain elevated levels of iso-alpha acids, compounds associated with bitter hop taste. Acetic and lactic acid are not prevalent in most beers, with notable exceptions such as Kriek, Lambic, Faro, West Flanders ales and Flanders Old Brown, which use acid-producing bacteria ( Lactobacillus and Pediococcus ) or unconventional yeast ( Brettanomyces ) 54 , 55 . Glycerol, ethanol and esters show similar distributions across all beer styles, reflecting their common origin as products of yeast metabolism during fermentation 45 , 53 . Finally, low/no-alcohol beers contain low concentrations of glycerol and esters. This is in line with the production process for most of the low/no-alcohol beers in our dataset, which are produced through limiting fermentation or by stripping away alcohol via evaporation or dialysis, with both methods having the unintended side-effect of reducing the amount of flavor compounds in the final beer 56 , 57 .
Besides expected associations, our data also reveals less trivial associations between beer styles and specific parameters. For example, geraniol and citronellol, two monoterpenoids responsible for citrus, floral and rose flavors and characteristic of Citra hops, are found in relatively high amounts in Christmas, Saison, and Brett/co-fermented beers, where they may originate from terpenoid-rich spices such as coriander seeds instead of hops 58 .
Tasting panel assessments reveal sensorial relationships in beer
To assess the sensory profile of each beer, a trained tasting panel evaluated each of the 250 beers for 50 sensory attributes, including different hop, malt and yeast flavors, off-flavors and spices. Panelists used a tasting sheet (Supplementary Data 3 ) to score the different attributes. Panel consistency was evaluated by repeating 12 samples across different sessions and performing ANOVA. In 95% of cases no significant difference was found across sessions ( p > 0.05), indicating good panel consistency (Supplementary Table S2 ).
Aroma and taste perception reported by the trained panel are often linked (Fig. 1 , bottom left panel and Supplementary Data 4 and 5 ), with high correlations between hops aroma and taste (Spearman’s rho=0.83). Bitter taste was found to correlate with hop aroma and taste in general (Spearman’s rho=0.80 and 0.69), and particularly with “grassy” noble hops (Spearman’s rho=0.75). Barnyard flavor, most often associated with sour beers, is identified together with stale hops (Spearman’s rho=0.97) that are used in these beers. Lactic and acetic acid, which often co-occur, are correlated (Spearman’s rho=0.66). Interestingly, sweetness and bitterness are anti-correlated (Spearman’s rho = −0.48), confirming the hypothesis that they mask each other 59 , 60 . Beer body is highly correlated with alcohol (Spearman’s rho = 0.79), and overall appreciation is found to correlate with multiple aspects that describe beer mouthfeel (alcohol, carbonation; Spearman’s rho= 0.32, 0.39), as well as with hop and ester aroma intensity (Spearman’s rho=0.39 and 0.35).
Similar to the chemical analyses, sensorial analyses confirmed typical features of specific beer styles (Supplementary Fig. S4 ). For example, sour beers (Faro, Flanders Old Brown, Fruit beer, Kriek, Lambic, West Flanders ale) were rated acidic, with flavors of both acetic and lactic acid. Hoppy beers were found to be bitter and showed hop-associated aromas like citrus and tropical fruit. Malt taste is most detected among scotch, stout/porters, and strong ales, while low/no-alcohol beers, which often have a reputation for being ‘worty’ (reminiscent of unfermented, sweet malt extract) appear in the middle. Unsurprisingly, hop aromas are most strongly detected among hoppy beers. Like its chemical counterpart (Supplementary Fig. S3 ), acidity shows a right-skewed distribution, with the most acidic beers being Krieks, Lambics, and West Flanders ales.
Tasting panel assessments of specific flavors correlate with chemical composition
We find that the concentrations of several chemical compounds strongly correlate with specific aroma or taste, as evaluated by the tasting panel (Fig. 2 , Supplementary Fig. S5 , Supplementary Data 6 ). In some cases, these correlations confirm expectations and serve as a useful control for data quality. For example, iso-alpha acids, the bittering compounds in hops, strongly correlate with bitterness (Spearman’s rho=0.68), while ethanol and glycerol correlate with tasters’ perceptions of alcohol and body, the mouthfeel sensation of fullness (Spearman’s rho=0.82/0.62 and 0.72/0.57 respectively) and darker color from roasted malts is a good indication of malt perception (Spearman’s rho=0.54).
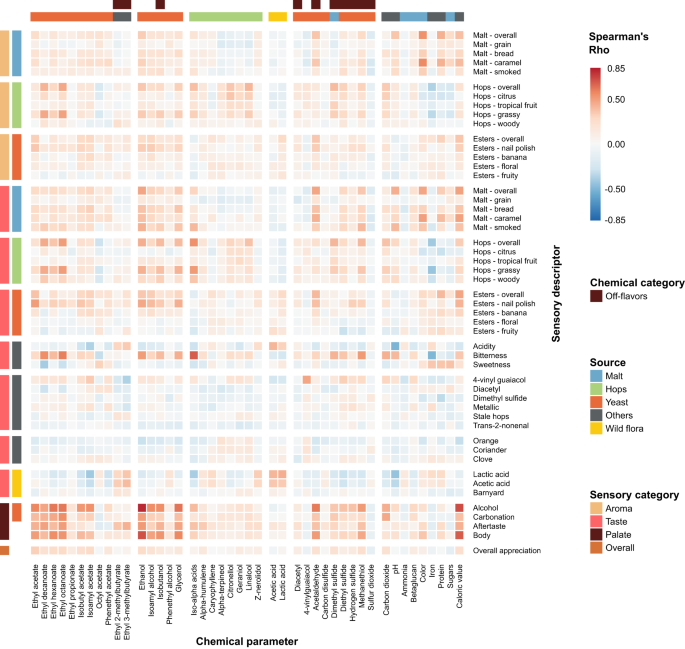
Heatmap colors indicate Spearman’s Rho. Axes are organized according to sensory categories (aroma, taste, mouthfeel, overall), chemical categories and chemical sources in beer (malt (blue), hops (green), yeast (red), wild flora (yellow), Others (black)). See Supplementary Data 6 for all correlation values.
Interestingly, for some relationships between chemical compounds and perceived flavor, correlations are weaker than expected. For example, the rose-smelling phenethyl acetate only weakly correlates with floral aroma. This hints at more complex relationships and interactions between compounds and suggests a need for a more complex model than simple correlations. Lastly, we uncovered unexpected correlations. For instance, the esters ethyl decanoate and ethyl octanoate appear to correlate slightly with hop perception and bitterness, possibly due to their fruity flavor. Iron is anti-correlated with hop aromas and bitterness, most likely because it is also anti-correlated with iso-alpha acids. This could be a sign of metal chelation of hop acids 61 , given that our analyses measure unbound hop acids and total iron content, or could result from the higher iron content in dark and Fruit beers, which typically have less hoppy and bitter flavors 62 .
Public consumer reviews complement expert panel data
To complement and expand the sensory data of our trained tasting panel, we collected 180,000 reviews of our 250 beers from the online consumer review platform RateBeer. This provided numerical scores for beer appearance, aroma, taste, palate, overall quality as well as the average overall score.
Public datasets are known to suffer from biases, such as price, cult status and psychological conformity towards previous ratings of a product. For example, prices correlate with appreciation scores for these online consumer reviews (rho=0.49, Supplementary Fig. S6 ), but not for our trained tasting panel (rho=0.19). This suggests that prices affect consumer appreciation, which has been reported in wine 63 , while blind tastings are unaffected. Moreover, we observe that some beer styles, like lagers and non-alcoholic beers, generally receive lower scores, reflecting that online reviewers are mostly beer aficionados with a preference for specialty beers over lager beers. In general, we find a modest correlation between our trained panel’s overall appreciation score and the online consumer appreciation scores (Fig. 3 , rho=0.29). Apart from the aforementioned biases in the online datasets, serving temperature, sample freshness and surroundings, which are all tightly controlled during the tasting panel sessions, can vary tremendously across online consumers and can further contribute to (among others, appreciation) differences between the two categories of tasters. Importantly, in contrast to the overall appreciation scores, for many sensory aspects the results from the professional panel correlated well with results obtained from RateBeer reviews. Correlations were highest for features that are relatively easy to recognize even for untrained tasters, like bitterness, sweetness, alcohol and malt aroma (Fig. 3 and below).
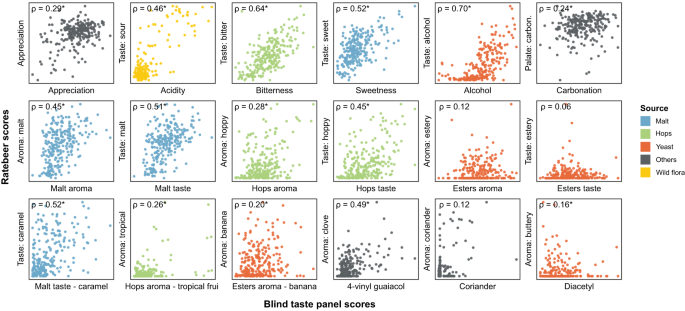
RateBeer text mining results can be found in Supplementary Data 7 . Rho values shown are Spearman correlation values, with asterisks indicating significant correlations ( p < 0.05, two-sided). All p values were smaller than 0.001, except for Esters aroma (0.0553), Esters taste (0.3275), Esters aroma—banana (0.0019), Coriander (0.0508) and Diacetyl (0.0134).
Besides collecting consumer appreciation from these online reviews, we developed automated text analysis tools to gather additional data from review texts (Supplementary Data 7 ). Processing review texts on the RateBeer database yielded comparable results to the scores given by the trained panel for many common sensory aspects, including acidity, bitterness, sweetness, alcohol, malt, and hop tastes (Fig. 3 ). This is in line with what would be expected, since these attributes require less training for accurate assessment and are less influenced by environmental factors such as temperature, serving glass and odors in the environment. Consumer reviews also correlate well with our trained panel for 4-vinyl guaiacol, a compound associated with a very characteristic aroma. By contrast, correlations for more specific aromas like ester, coriander or diacetyl are underrepresented in the online reviews, underscoring the importance of using a trained tasting panel and standardized tasting sheets with explicit factors to be scored for evaluating specific aspects of a beer. Taken together, our results suggest that public reviews are trustworthy for some, but not all, flavor features and can complement or substitute taste panel data for these sensory aspects.
Models can predict beer sensory profiles from chemical data
The rich datasets of chemical analyses, tasting panel assessments and public reviews gathered in the first part of this study provided us with a unique opportunity to develop predictive models that link chemical data to sensorial features. Given the complexity of beer flavor, basic statistical tools such as correlations or linear regression may not always be the most suitable for making accurate predictions. Instead, we applied different machine learning models that can model both simple linear and complex interactive relationships. Specifically, we constructed a set of regression models to predict (a) trained panel scores for beer flavor and quality and (b) public reviews’ appreciation scores from beer chemical profiles. We trained and tested 10 different models (Methods), 3 linear regression-based models (simple linear regression with first-order interactions (LR), lasso regression with first-order interactions (Lasso), partial least squares regressor (PLSR)), 5 decision tree models (AdaBoost regressor (ABR), extra trees (ET), gradient boosting regressor (GBR), random forest (RF) and XGBoost regressor (XGBR)), 1 support vector regression (SVR), and 1 artificial neural network (ANN) model.
To compare the performance of our machine learning models, the dataset was randomly split into a training and test set, stratified by beer style. After a model was trained on data in the training set, its performance was evaluated on its ability to predict the test dataset obtained from multi-output models (based on the coefficient of determination, see Methods). Additionally, individual-attribute models were ranked per descriptor and the average rank was calculated, as proposed by Korneva et al. 64 . Importantly, both ways of evaluating the models’ performance agreed in general. Performance of the different models varied (Table 1 ). It should be noted that all models perform better at predicting RateBeer results than results from our trained tasting panel. One reason could be that sensory data is inherently variable, and this variability is averaged out with the large number of public reviews from RateBeer. Additionally, all tree-based models perform better at predicting taste than aroma. Linear models (LR) performed particularly poorly, with negative R 2 values, due to severe overfitting (training set R 2 = 1). Overfitting is a common issue in linear models with many parameters and limited samples, especially with interaction terms further amplifying the number of parameters. L1 regularization (Lasso) successfully overcomes this overfitting, out-competing multiple tree-based models on the RateBeer dataset. Similarly, the dimensionality reduction of PLSR avoids overfitting and improves performance, to some extent. Still, tree-based models (ABR, ET, GBR, RF and XGBR) show the best performance, out-competing the linear models (LR, Lasso, PLSR) commonly used in sensory science 65 .
GBR models showed the best overall performance in predicting sensory responses from chemical information, with R 2 values up to 0.75 depending on the predicted sensory feature (Supplementary Table S4 ). The GBR models predict consumer appreciation (RateBeer) better than our trained panel’s appreciation (R 2 value of 0.67 compared to R 2 value of 0.09) (Supplementary Table S3 and Supplementary Table S4 ). ANN models showed intermediate performance, likely because neural networks typically perform best with larger datasets 66 . The SVR shows intermediate performance, mostly due to the weak predictions of specific attributes that lower the overall performance (Supplementary Table S4 ).
Model dissection identifies specific, unexpected compounds as drivers of consumer appreciation
Next, we leveraged our models to infer important contributors to sensory perception and consumer appreciation. Consumer preference is a crucial sensory aspects, because a product that shows low consumer appreciation scores often does not succeed commercially 25 . Additionally, the requirement for a large number of representative evaluators makes consumer trials one of the more costly and time-consuming aspects of product development. Hence, a model for predicting chemical drivers of overall appreciation would be a welcome addition to the available toolbox for food development and optimization.
Since GBR models on our RateBeer dataset showed the best overall performance, we focused on these models. Specifically, we used two approaches to identify important contributors. First, rankings of the most important predictors for each sensorial trait in the GBR models were obtained based on impurity-based feature importance (mean decrease in impurity). High-ranked parameters were hypothesized to be either the true causal chemical properties underlying the trait, to correlate with the actual causal properties, or to take part in sensory interactions affecting the trait 67 (Fig. 4A ). In a second approach, we used SHAP 68 to determine which parameters contributed most to the model for making predictions of consumer appreciation (Fig. 4B ). SHAP calculates parameter contributions to model predictions on a per-sample basis, which can be aggregated into an importance score.
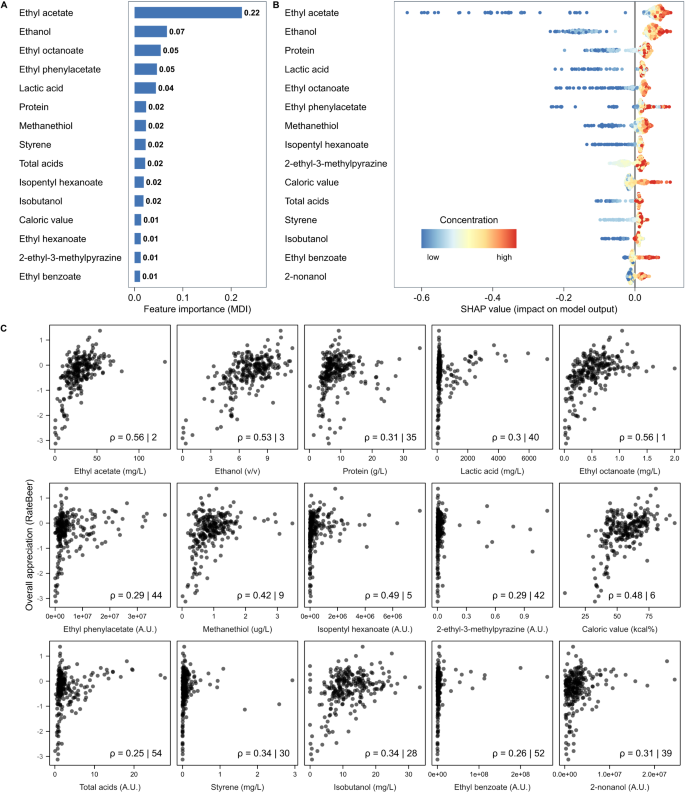
A The impurity-based feature importance (mean deviance in impurity, MDI) calculated from the Gradient Boosting Regression (GBR) model predicting RateBeer appreciation scores. The top 15 highest ranked chemical properties are shown. B SHAP summary plot for the top 15 parameters contributing to our GBR model. Each point on the graph represents a sample from our dataset. The color represents the concentration of that parameter, with bluer colors representing low values and redder colors representing higher values. Greater absolute values on the horizontal axis indicate a higher impact of the parameter on the prediction of the model. C Spearman correlations between the 15 most important chemical properties and consumer overall appreciation. Numbers indicate the Spearman Rho correlation coefficient, and the rank of this correlation compared to all other correlations. The top 15 important compounds were determined using SHAP (panel B).
Both approaches identified ethyl acetate as the most predictive parameter for beer appreciation (Fig. 4 ). Ethyl acetate is the most abundant ester in beer with a typical ‘fruity’, ‘solvent’ and ‘alcoholic’ flavor, but is often considered less important than other esters like isoamyl acetate. The second most important parameter identified by SHAP is ethanol, the most abundant beer compound after water. Apart from directly contributing to beer flavor and mouthfeel, ethanol drastically influences the physical properties of beer, dictating how easily volatile compounds escape the beer matrix to contribute to beer aroma 69 . Importantly, it should also be noted that the importance of ethanol for appreciation is likely inflated by the very low appreciation scores of non-alcoholic beers (Supplementary Fig. S4 ). Despite not often being considered a driver of beer appreciation, protein level also ranks highly in both approaches, possibly due to its effect on mouthfeel and body 70 . Lactic acid, which contributes to the tart taste of sour beers, is the fourth most important parameter identified by SHAP, possibly due to the generally high appreciation of sour beers in our dataset.
Interestingly, some of the most important predictive parameters for our model are not well-established as beer flavors or are even commonly regarded as being negative for beer quality. For example, our models identify methanethiol and ethyl phenyl acetate, an ester commonly linked to beer staling 71 , as a key factor contributing to beer appreciation. Although there is no doubt that high concentrations of these compounds are considered unpleasant, the positive effects of modest concentrations are not yet known 72 , 73 .
To compare our approach to conventional statistics, we evaluated how well the 15 most important SHAP-derived parameters correlate with consumer appreciation (Fig. 4C ). Interestingly, only 6 of the properties derived by SHAP rank amongst the top 15 most correlated parameters. For some chemical compounds, the correlations are so low that they would have likely been considered unimportant. For example, lactic acid, the fourth most important parameter, shows a bimodal distribution for appreciation, with sour beers forming a separate cluster, that is missed entirely by the Spearman correlation. Additionally, the correlation plots reveal outliers, emphasizing the need for robust analysis tools. Together, this highlights the need for alternative models, like the Gradient Boosting model, that better grasp the complexity of (beer) flavor.
Finally, to observe the relationships between these chemical properties and their predicted targets, partial dependence plots were constructed for the six most important predictors of consumer appreciation 74 , 75 , 76 (Supplementary Fig. S7 ). One-way partial dependence plots show how a change in concentration affects the predicted appreciation. These plots reveal an important limitation of our models: appreciation predictions remain constant at ever-increasing concentrations. This implies that once a threshold concentration is reached, further increasing the concentration does not affect appreciation. This is false, as it is well-documented that certain compounds become unpleasant at high concentrations, including ethyl acetate (‘nail polish’) 77 and methanethiol (‘sulfury’ and ‘rotten cabbage’) 78 . The inability of our models to grasp that flavor compounds have optimal levels, above which they become negative, is a consequence of working with commercial beer brands where (off-)flavors are rarely too high to negatively impact the product. The two-way partial dependence plots show how changing the concentration of two compounds influences predicted appreciation, visualizing their interactions (Supplementary Fig. S7 ). In our case, the top 5 parameters are dominated by additive or synergistic interactions, with high concentrations for both compounds resulting in the highest predicted appreciation.
To assess the robustness of our best-performing models and model predictions, we performed 100 iterations of the GBR, RF and ET models. In general, all iterations of the models yielded similar performance (Supplementary Fig. S8 ). Moreover, the main predictors (including the top predictors ethanol and ethyl acetate) remained virtually the same, especially for GBR and RF. For the iterations of the ET model, we did observe more variation in the top predictors, which is likely a consequence of the model’s inherent random architecture in combination with co-correlations between certain predictors. However, even in this case, several of the top predictors (ethanol and ethyl acetate) remain unchanged, although their rank in importance changes (Supplementary Fig. S8 ).
Next, we investigated if a combination of RateBeer and trained panel data into one consolidated dataset would lead to stronger models, under the hypothesis that such a model would suffer less from bias in the datasets. A GBR model was trained to predict appreciation on the combined dataset. This model underperformed compared to the RateBeer model, both in the native case and when including a dataset identifier (R 2 = 0.67, 0.26 and 0.42 respectively). For the latter, the dataset identifier is the most important feature (Supplementary Fig. S9 ), while most of the feature importance remains unchanged, with ethyl acetate and ethanol ranking highest, like in the original model trained only on RateBeer data. It seems that the large variation in the panel dataset introduces noise, weakening the models’ performances and reliability. In addition, it seems reasonable to assume that both datasets are fundamentally different, with the panel dataset obtained by blind tastings by a trained professional panel.
Lastly, we evaluated whether beer style identifiers would further enhance the model’s performance. A GBR model was trained with parameters that explicitly encoded the styles of the samples. This did not improve model performance (R2 = 0.66 with style information vs R2 = 0.67). The most important chemical features are consistent with the model trained without style information (eg. ethanol and ethyl acetate), and with the exception of the most preferred (strong ale) and least preferred (low/no-alcohol) styles, none of the styles were among the most important features (Supplementary Fig. S9 , Supplementary Table S5 and S6 ). This is likely due to a combination of style-specific chemical signatures, such as iso-alpha acids and lactic acid, that implicitly convey style information to the original models, as well as the low number of samples belonging to some styles, making it difficult for the model to learn style-specific patterns. Moreover, beer styles are not rigorously defined, with some styles overlapping in features and some beers being misattributed to a specific style, all of which leads to more noise in models that use style parameters.
Model validation
To test if our predictive models give insight into beer appreciation, we set up experiments aimed at improving existing commercial beers. We specifically selected overall appreciation as the trait to be examined because of its complexity and commercial relevance. Beer flavor comprises a complex bouquet rather than single aromas and tastes 53 . Hence, adding a single compound to the extent that a difference is noticeable may lead to an unbalanced, artificial flavor. Therefore, we evaluated the effect of combinations of compounds. Because Blond beers represent the most extensive style in our dataset, we selected a beer from this style as the starting material for these experiments (Beer 64 in Supplementary Data 1 ).
In the first set of experiments, we adjusted the concentrations of compounds that made up the most important predictors of overall appreciation (ethyl acetate, ethanol, lactic acid, ethyl phenyl acetate) together with correlated compounds (ethyl hexanoate, isoamyl acetate, glycerol), bringing them up to 95 th percentile ethanol-normalized concentrations (Methods) within the Blond group (‘Spiked’ concentration in Fig. 5A ). Compared to controls, the spiked beers were found to have significantly improved overall appreciation among trained panelists, with panelist noting increased intensity of ester flavors, sweetness, alcohol, and body fullness (Fig. 5B ). To disentangle the contribution of ethanol to these results, a second experiment was performed without the addition of ethanol. This resulted in a similar outcome, including increased perception of alcohol and overall appreciation.
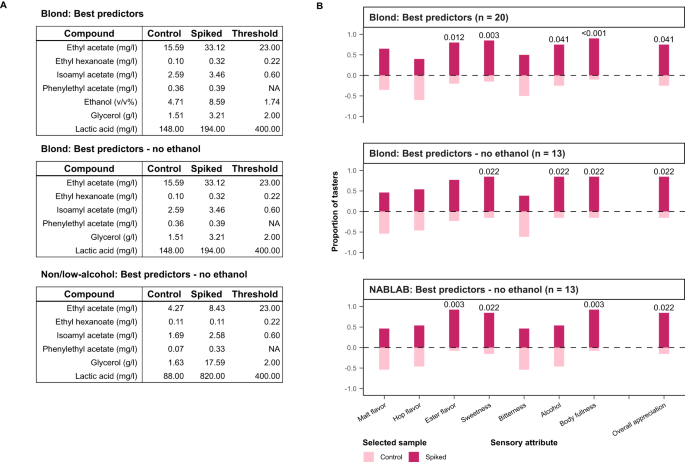
Adding the top chemical compounds, identified as best predictors of appreciation by our model, into poorly appreciated beers results in increased appreciation from our trained panel. Results of sensory tests between base beers and those spiked with compounds identified as the best predictors by the model. A Blond and Non/Low-alcohol (0.0% ABV) base beers were brought up to 95th-percentile ethanol-normalized concentrations within each style. B For each sensory attribute, tasters indicated the more intense sample and selected the sample they preferred. The numbers above the bars correspond to the p values that indicate significant changes in perceived flavor (two-sided binomial test: alpha 0.05, n = 20 or 13).
In a last experiment, we tested whether using the model’s predictions can boost the appreciation of a non-alcoholic beer (beer 223 in Supplementary Data 1 ). Again, the addition of a mixture of predicted compounds (omitting ethanol, in this case) resulted in a significant increase in appreciation, body, ester flavor and sweetness.
Predicting flavor and consumer appreciation from chemical composition is one of the ultimate goals of sensory science. A reliable, systematic and unbiased way to link chemical profiles to flavor and food appreciation would be a significant asset to the food and beverage industry. Such tools would substantially aid in quality control and recipe development, offer an efficient and cost-effective alternative to pilot studies and consumer trials and would ultimately allow food manufacturers to produce superior, tailor-made products that better meet the demands of specific consumer groups more efficiently.
A limited set of studies have previously tried, to varying degrees of success, to predict beer flavor and beer popularity based on (a limited set of) chemical compounds and flavors 79 , 80 . Current sensitive, high-throughput technologies allow measuring an unprecedented number of chemical compounds and properties in a large set of samples, yielding a dataset that can train models that help close the gaps between chemistry and flavor, even for a complex natural product like beer. To our knowledge, no previous research gathered data at this scale (250 samples, 226 chemical parameters, 50 sensory attributes and 5 consumer scores) to disentangle and validate the chemical aspects driving beer preference using various machine-learning techniques. We find that modern machine learning models outperform conventional statistical tools, such as correlations and linear models, and can successfully predict flavor appreciation from chemical composition. This could be attributed to the natural incorporation of interactions and non-linear or discontinuous effects in machine learning models, which are not easily grasped by the linear model architecture. While linear models and partial least squares regression represent the most widespread statistical approaches in sensory science, in part because they allow interpretation 65 , 81 , 82 , modern machine learning methods allow for building better predictive models while preserving the possibility to dissect and exploit the underlying patterns. Of the 10 different models we trained, tree-based models, such as our best performing GBR, showed the best overall performance in predicting sensory responses from chemical information, outcompeting artificial neural networks. This agrees with previous reports for models trained on tabular data 83 . Our results are in line with the findings of Colantonio et al. who also identified the gradient boosting architecture as performing best at predicting appreciation and flavor (of tomatoes and blueberries, in their specific study) 26 . Importantly, besides our larger experimental scale, we were able to directly confirm our models’ predictions in vivo.
Our study confirms that flavor compound concentration does not always correlate with perception, suggesting complex interactions that are often missed by more conventional statistics and simple models. Specifically, we find that tree-based algorithms may perform best in developing models that link complex food chemistry with aroma. Furthermore, we show that massive datasets of untrained consumer reviews provide a valuable source of data, that can complement or even replace trained tasting panels, especially for appreciation and basic flavors, such as sweetness and bitterness. This holds despite biases that are known to occur in such datasets, such as price or conformity bias. Moreover, GBR models predict taste better than aroma. This is likely because taste (e.g. bitterness) often directly relates to the corresponding chemical measurements (e.g., iso-alpha acids), whereas such a link is less clear for aromas, which often result from the interplay between multiple volatile compounds. We also find that our models are best at predicting acidity and alcohol, likely because there is a direct relation between the measured chemical compounds (acids and ethanol) and the corresponding perceived sensorial attribute (acidity and alcohol), and because even untrained consumers are generally able to recognize these flavors and aromas.
The predictions of our final models, trained on review data, hold even for blind tastings with small groups of trained tasters, as demonstrated by our ability to validate specific compounds as drivers of beer flavor and appreciation. Since adding a single compound to the extent of a noticeable difference may result in an unbalanced flavor profile, we specifically tested our identified key drivers as a combination of compounds. While this approach does not allow us to validate if a particular single compound would affect flavor and/or appreciation, our experiments do show that this combination of compounds increases consumer appreciation.
It is important to stress that, while it represents an important step forward, our approach still has several major limitations. A key weakness of the GBR model architecture is that amongst co-correlating variables, the largest main effect is consistently preferred for model building. As a result, co-correlating variables often have artificially low importance scores, both for impurity and SHAP-based methods, like we observed in the comparison to the more randomized Extra Trees models. This implies that chemicals identified as key drivers of a specific sensory feature by GBR might not be the true causative compounds, but rather co-correlate with the actual causative chemical. For example, the high importance of ethyl acetate could be (partially) attributed to the total ester content, ethanol or ethyl hexanoate (rho=0.77, rho=0.72 and rho=0.68), while ethyl phenylacetate could hide the importance of prenyl isobutyrate and ethyl benzoate (rho=0.77 and rho=0.76). Expanding our GBR model to include beer style as a parameter did not yield additional power or insight. This is likely due to style-specific chemical signatures, such as iso-alpha acids and lactic acid, that implicitly convey style information to the original model, as well as the smaller sample size per style, limiting the power to uncover style-specific patterns. This can be partly attributed to the curse of dimensionality, where the high number of parameters results in the models mainly incorporating single parameter effects, rather than complex interactions such as style-dependent effects 67 . A larger number of samples may overcome some of these limitations and offer more insight into style-specific effects. On the other hand, beer style is not a rigid scientific classification, and beers within one style often differ a lot, which further complicates the analysis of style as a model factor.
Our study is limited to beers from Belgian breweries. Although these beers cover a large portion of the beer styles available globally, some beer styles and consumer patterns may be missing, while other features might be overrepresented. For example, many Belgian ales exhibit yeast-driven flavor profiles, which is reflected in the chemical drivers of appreciation discovered by this study. In future work, expanding the scope to include diverse markets and beer styles could lead to the identification of even more drivers of appreciation and better models for special niche products that were not present in our beer set.
In addition to inherent limitations of GBR models, there are also some limitations associated with studying food aroma. Even if our chemical analyses measured most of the known aroma compounds, the total number of flavor compounds in complex foods like beer is still larger than the subset we were able to measure in this study. For example, hop-derived thiols, that influence flavor at very low concentrations, are notoriously difficult to measure in a high-throughput experiment. Moreover, consumer perception remains subjective and prone to biases that are difficult to avoid. It is also important to stress that the models are still immature and that more extensive datasets will be crucial for developing more complete models in the future. Besides more samples and parameters, our dataset does not include any demographic information about the tasters. Including such data could lead to better models that grasp external factors like age and culture. Another limitation is that our set of beers consists of high-quality end-products and lacks beers that are unfit for sale, which limits the current model in accurately predicting products that are appreciated very badly. Finally, while models could be readily applied in quality control, their use in sensory science and product development is restrained by their inability to discern causal relationships. Given that the models cannot distinguish compounds that genuinely drive consumer perception from those that merely correlate, validation experiments are essential to identify true causative compounds.
Despite the inherent limitations, dissection of our models enabled us to pinpoint specific molecules as potential drivers of beer aroma and consumer appreciation, including compounds that were unexpected and would not have been identified using standard approaches. Important drivers of beer appreciation uncovered by our models include protein levels, ethyl acetate, ethyl phenyl acetate and lactic acid. Currently, many brewers already use lactic acid to acidify their brewing water and ensure optimal pH for enzymatic activity during the mashing process. Our results suggest that adding lactic acid can also improve beer appreciation, although its individual effect remains to be tested. Interestingly, ethanol appears to be unnecessary to improve beer appreciation, both for blond beer and alcohol-free beer. Given the growing consumer interest in alcohol-free beer, with a predicted annual market growth of >7% 84 , it is relevant for brewers to know what compounds can further increase consumer appreciation of these beers. Hence, our model may readily provide avenues to further improve the flavor and consumer appreciation of both alcoholic and non-alcoholic beers, which is generally considered one of the key challenges for future beer production.
Whereas we see a direct implementation of our results for the development of superior alcohol-free beverages and other food products, our study can also serve as a stepping stone for the development of novel alcohol-containing beverages. We want to echo the growing body of scientific evidence for the negative effects of alcohol consumption, both on the individual level by the mutagenic, teratogenic and carcinogenic effects of ethanol 85 , 86 , as well as the burden on society caused by alcohol abuse and addiction. We encourage the use of our results for the production of healthier, tastier products, including novel and improved beverages with lower alcohol contents. Furthermore, we strongly discourage the use of these technologies to improve the appreciation or addictive properties of harmful substances.
The present work demonstrates that despite some important remaining hurdles, combining the latest developments in chemical analyses, sensory analysis and modern machine learning methods offers exciting avenues for food chemistry and engineering. Soon, these tools may provide solutions in quality control and recipe development, as well as new approaches to sensory science and flavor research.
Beer selection
250 commercial Belgian beers were selected to cover the broad diversity of beer styles and corresponding diversity in chemical composition and aroma. See Supplementary Fig. S1 .
Chemical dataset
Sample preparation.
Beers within their expiration date were purchased from commercial retailers. Samples were prepared in biological duplicates at room temperature, unless explicitly stated otherwise. Bottle pressure was measured with a manual pressure device (Steinfurth Mess-Systeme GmbH) and used to calculate CO 2 concentration. The beer was poured through two filter papers (Macherey-Nagel, 500713032 MN 713 ¼) to remove carbon dioxide and prevent spontaneous foaming. Samples were then prepared for measurements by targeted Headspace-Gas Chromatography-Flame Ionization Detector/Flame Photometric Detector (HS-GC-FID/FPD), Headspace-Solid Phase Microextraction-Gas Chromatography-Mass Spectrometry (HS-SPME-GC-MS), colorimetric analysis, enzymatic analysis, Near-Infrared (NIR) analysis, as described in the sections below. The mean values of biological duplicates are reported for each compound.
HS-GC-FID/FPD
HS-GC-FID/FPD (Shimadzu GC 2010 Plus) was used to measure higher alcohols, acetaldehyde, esters, 4-vinyl guaicol, and sulfur compounds. Each measurement comprised 5 ml of sample pipetted into a 20 ml glass vial containing 1.75 g NaCl (VWR, 27810.295). 100 µl of 2-heptanol (Sigma-Aldrich, H3003) (internal standard) solution in ethanol (Fisher Chemical, E/0650DF/C17) was added for a final concentration of 2.44 mg/L. Samples were flushed with nitrogen for 10 s, sealed with a silicone septum, stored at −80 °C and analyzed in batches of 20.
The GC was equipped with a DB-WAXetr column (length, 30 m; internal diameter, 0.32 mm; layer thickness, 0.50 µm; Agilent Technologies, Santa Clara, CA, USA) to the FID and an HP-5 column (length, 30 m; internal diameter, 0.25 mm; layer thickness, 0.25 µm; Agilent Technologies, Santa Clara, CA, USA) to the FPD. N 2 was used as the carrier gas. Samples were incubated for 20 min at 70 °C in the headspace autosampler (Flow rate, 35 cm/s; Injection volume, 1000 µL; Injection mode, split; Combi PAL autosampler, CTC analytics, Switzerland). The injector, FID and FPD temperatures were kept at 250 °C. The GC oven temperature was first held at 50 °C for 5 min and then allowed to rise to 80 °C at a rate of 5 °C/min, followed by a second ramp of 4 °C/min until 200 °C kept for 3 min and a final ramp of (4 °C/min) until 230 °C for 1 min. Results were analyzed with the GCSolution software version 2.4 (Shimadzu, Kyoto, Japan). The GC was calibrated with a 5% EtOH solution (VWR International) containing the volatiles under study (Supplementary Table S7 ).
HS-SPME-GC-MS
HS-SPME-GC-MS (Shimadzu GCMS-QP-2010 Ultra) was used to measure additional volatile compounds, mainly comprising terpenoids and esters. Samples were analyzed by HS-SPME using a triphase DVB/Carboxen/PDMS 50/30 μm SPME fiber (Supelco Co., Bellefonte, PA, USA) followed by gas chromatography (Thermo Fisher Scientific Trace 1300 series, USA) coupled to a mass spectrometer (Thermo Fisher Scientific ISQ series MS) equipped with a TriPlus RSH autosampler. 5 ml of degassed beer sample was placed in 20 ml vials containing 1.75 g NaCl (VWR, 27810.295). 5 µl internal standard mix was added, containing 2-heptanol (1 g/L) (Sigma-Aldrich, H3003), 4-fluorobenzaldehyde (1 g/L) (Sigma-Aldrich, 128376), 2,3-hexanedione (1 g/L) (Sigma-Aldrich, 144169) and guaiacol (1 g/L) (Sigma-Aldrich, W253200) in ethanol (Fisher Chemical, E/0650DF/C17). Each sample was incubated at 60 °C in the autosampler oven with constant agitation. After 5 min equilibration, the SPME fiber was exposed to the sample headspace for 30 min. The compounds trapped on the fiber were thermally desorbed in the injection port of the chromatograph by heating the fiber for 15 min at 270 °C.
The GC-MS was equipped with a low polarity RXi-5Sil MS column (length, 20 m; internal diameter, 0.18 mm; layer thickness, 0.18 µm; Restek, Bellefonte, PA, USA). Injection was performed in splitless mode at 320 °C, a split flow of 9 ml/min, a purge flow of 5 ml/min and an open valve time of 3 min. To obtain a pulsed injection, a programmed gas flow was used whereby the helium gas flow was set at 2.7 mL/min for 0.1 min, followed by a decrease in flow of 20 ml/min to the normal 0.9 mL/min. The temperature was first held at 30 °C for 3 min and then allowed to rise to 80 °C at a rate of 7 °C/min, followed by a second ramp of 2 °C/min till 125 °C and a final ramp of 8 °C/min with a final temperature of 270 °C.
Mass acquisition range was 33 to 550 amu at a scan rate of 5 scans/s. Electron impact ionization energy was 70 eV. The interface and ion source were kept at 275 °C and 250 °C, respectively. A mix of linear n-alkanes (from C7 to C40, Supelco Co.) was injected into the GC-MS under identical conditions to serve as external retention index markers. Identification and quantification of the compounds were performed using an in-house developed R script as described in Goelen et al. and Reher et al. 87 , 88 (for package information, see Supplementary Table S8 ). Briefly, chromatograms were analyzed using AMDIS (v2.71) 89 to separate overlapping peaks and obtain pure compound spectra. The NIST MS Search software (v2.0 g) in combination with the NIST2017, FFNSC3 and Adams4 libraries were used to manually identify the empirical spectra, taking into account the expected retention time. After background subtraction and correcting for retention time shifts between samples run on different days based on alkane ladders, compound elution profiles were extracted and integrated using a file with 284 target compounds of interest, which were either recovered in our identified AMDIS list of spectra or were known to occur in beer. Compound elution profiles were estimated for every peak in every chromatogram over a time-restricted window using weighted non-negative least square analysis after which peak areas were integrated 87 , 88 . Batch effect correction was performed by normalizing against the most stable internal standard compound, 4-fluorobenzaldehyde. Out of all 284 target compounds that were analyzed, 167 were visually judged to have reliable elution profiles and were used for final analysis.
Discrete photometric and enzymatic analysis
Discrete photometric and enzymatic analysis (Thermo Scientific TM Gallery TM Plus Beermaster Discrete Analyzer) was used to measure acetic acid, ammonia, beta-glucan, iso-alpha acids, color, sugars, glycerol, iron, pH, protein, and sulfite. 2 ml of sample volume was used for the analyses. Information regarding the reagents and standard solutions used for analyses and calibrations is included in Supplementary Table S7 and Supplementary Table S9 .
NIR analyses
NIR analysis (Anton Paar Alcolyzer Beer ME System) was used to measure ethanol. Measurements comprised 50 ml of sample, and a 10% EtOH solution was used for calibration.
Correlation calculations
Pairwise Spearman Rank correlations were calculated between all chemical properties.
Sensory dataset
Trained panel.
Our trained tasting panel consisted of volunteers who gave prior verbal informed consent. All compounds used for the validation experiment were of food-grade quality. The tasting sessions were approved by the Social and Societal Ethics Committee of the KU Leuven (G-2022-5677-R2(MAR)). All online reviewers agreed to the Terms and Conditions of the RateBeer website.
Sensory analysis was performed according to the American Society of Brewing Chemists (ASBC) Sensory Analysis Methods 90 . 30 volunteers were screened through a series of triangle tests. The sixteen most sensitive and consistent tasters were retained as taste panel members. The resulting panel was diverse in age [22–42, mean: 29], sex [56% male] and nationality [7 different countries]. The panel developed a consensus vocabulary to describe beer aroma, taste and mouthfeel. Panelists were trained to identify and score 50 different attributes, using a 7-point scale to rate attributes’ intensity. The scoring sheet is included as Supplementary Data 3 . Sensory assessments took place between 10–12 a.m. The beers were served in black-colored glasses. Per session, between 5 and 12 beers of the same style were tasted at 12 °C to 16 °C. Two reference beers were added to each set and indicated as ‘Reference 1 & 2’, allowing panel members to calibrate their ratings. Not all panelists were present at every tasting. Scores were scaled by standard deviation and mean-centered per taster. Values are represented as z-scores and clustered by Euclidean distance. Pairwise Spearman correlations were calculated between taste and aroma sensory attributes. Panel consistency was evaluated by repeating samples on different sessions and performing ANOVA to identify differences, using the ‘stats’ package (v4.2.2) in R (for package information, see Supplementary Table S8 ).
Online reviews from a public database
The ‘scrapy’ package in Python (v3.6) (for package information, see Supplementary Table S8 ). was used to collect 232,288 online reviews (mean=922, min=6, max=5343) from RateBeer, an online beer review database. Each review entry comprised 5 numerical scores (appearance, aroma, taste, palate and overall quality) and an optional review text. The total number of reviews per reviewer was collected separately. Numerical scores were scaled and centered per rater, and mean scores were calculated per beer.
For the review texts, the language was estimated using the packages ‘langdetect’ and ‘langid’ in Python. Reviews that were classified as English by both packages were kept. Reviewers with fewer than 100 entries overall were discarded. 181,025 reviews from >6000 reviewers from >40 countries remained. Text processing was done using the ‘nltk’ package in Python. Texts were corrected for slang and misspellings; proper nouns and rare words that are relevant to the beer context were specified and kept as-is (‘Chimay’,’Lambic’, etc.). A dictionary of semantically similar sensorial terms, for example ‘floral’ and ‘flower’, was created and collapsed together into one term. Words were stemmed and lemmatized to avoid identifying words such as ‘acid’ and ‘acidity’ as separate terms. Numbers and punctuation were removed.
Sentences from up to 50 randomly chosen reviews per beer were manually categorized according to the aspect of beer they describe (appearance, aroma, taste, palate, overall quality—not to be confused with the 5 numerical scores described above) or flagged as irrelevant if they contained no useful information. If a beer contained fewer than 50 reviews, all reviews were manually classified. This labeled data set was used to train a model that classified the rest of the sentences for all beers 91 . Sentences describing taste and aroma were extracted, and term frequency–inverse document frequency (TFIDF) was implemented to calculate enrichment scores for sensorial words per beer.
The sex of the tasting subject was not considered when building our sensory database. Instead, results from different panelists were averaged, both for our trained panel (56% male, 44% female) and the RateBeer reviews (70% male, 30% female for RateBeer as a whole).
Beer price collection and processing
Beer prices were collected from the following stores: Colruyt, Delhaize, Total Wine, BeerHawk, The Belgian Beer Shop, The Belgian Shop, and Beer of Belgium. Where applicable, prices were converted to Euros and normalized per liter. Spearman correlations were calculated between these prices and mean overall appreciation scores from RateBeer and the taste panel, respectively.
Pairwise Spearman Rank correlations were calculated between all sensory properties.
Machine learning models
Predictive modeling of sensory profiles from chemical data.
Regression models were constructed to predict (a) trained panel scores for beer flavors and quality from beer chemical profiles and (b) public reviews’ appreciation scores from beer chemical profiles. Z-scores were used to represent sensory attributes in both data sets. Chemical properties with log-normal distributions (Shapiro-Wilk test, p < 0.05 ) were log-transformed. Missing chemical measurements (0.1% of all data) were replaced with mean values per attribute. Observations from 250 beers were randomly separated into a training set (70%, 175 beers) and a test set (30%, 75 beers), stratified per beer style. Chemical measurements (p = 231) were normalized based on the training set average and standard deviation. In total, three linear regression-based models: linear regression with first-order interaction terms (LR), lasso regression with first-order interaction terms (Lasso) and partial least squares regression (PLSR); five decision tree models, Adaboost regressor (ABR), Extra Trees (ET), Gradient Boosting regressor (GBR), Random Forest (RF) and XGBoost regressor (XGBR); one support vector machine model (SVR) and one artificial neural network model (ANN) were trained. The models were implemented using the ‘scikit-learn’ package (v1.2.2) and ‘xgboost’ package (v1.7.3) in Python (v3.9.16). Models were trained, and hyperparameters optimized, using five-fold cross-validated grid search with the coefficient of determination (R 2 ) as the evaluation metric. The ANN (scikit-learn’s MLPRegressor) was optimized using Bayesian Tree-Structured Parzen Estimator optimization with the ‘Optuna’ Python package (v3.2.0). Individual models were trained per attribute, and a multi-output model was trained on all attributes simultaneously.
Model dissection
GBR was found to outperform other methods, resulting in models with the highest average R 2 values in both trained panel and public review data sets. Impurity-based rankings of the most important predictors for each predicted sensorial trait were obtained using the ‘scikit-learn’ package. To observe the relationships between these chemical properties and their predicted targets, partial dependence plots (PDP) were constructed for the six most important predictors of consumer appreciation 74 , 75 .
The ‘SHAP’ package in Python (v0.41.0) was implemented to provide an alternative ranking of predictor importance and to visualize the predictors’ effects as a function of their concentration 68 .
Validation of causal chemical properties
To validate the effects of the most important model features on predicted sensory attributes, beers were spiked with the chemical compounds identified by the models and descriptive sensory analyses were carried out according to the American Society of Brewing Chemists (ASBC) protocol 90 .
Compound spiking was done 30 min before tasting. Compounds were spiked into fresh beer bottles, that were immediately resealed and inverted three times. Fresh bottles of beer were opened for the same duration, resealed, and inverted thrice, to serve as controls. Pairs of spiked samples and controls were served simultaneously, chilled and in dark glasses as outlined in the Trained panel section above. Tasters were instructed to select the glass with the higher flavor intensity for each attribute (directional difference test 92 ) and to select the glass they prefer.
The final concentration after spiking was equal to the within-style average, after normalizing by ethanol concentration. This was done to ensure balanced flavor profiles in the final spiked beer. The same methods were applied to improve a non-alcoholic beer. Compounds were the following: ethyl acetate (Merck KGaA, W241415), ethyl hexanoate (Merck KGaA, W243906), isoamyl acetate (Merck KGaA, W205508), phenethyl acetate (Merck KGaA, W285706), ethanol (96%, Colruyt), glycerol (Merck KGaA, W252506), lactic acid (Merck KGaA, 261106).
Significant differences in preference or perceived intensity were determined by performing the two-sided binomial test on each attribute.
Reporting summary
Further information on research design is available in the Nature Portfolio Reporting Summary linked to this article.
Data availability
The data that support the findings of this work are available in the Supplementary Data files and have been deposited to Zenodo under accession code 10653704 93 . The RateBeer scores data are under restricted access, they are not publicly available as they are property of RateBeer (ZX Ventures, USA). Access can be obtained from the authors upon reasonable request and with permission of RateBeer (ZX Ventures, USA). Source data are provided with this paper.
Code availability
The code for training the machine learning models, analyzing the models, and generating the figures has been deposited to Zenodo under accession code 10653704 93 .
Tieman, D. et al. A chemical genetic roadmap to improved tomato flavor. Science 355 , 391–394 (2017).
Article ADS CAS PubMed Google Scholar
Plutowska, B. & Wardencki, W. Application of gas chromatography–olfactometry (GC–O) in analysis and quality assessment of alcoholic beverages – A review. Food Chem. 107 , 449–463 (2008).
Article CAS Google Scholar
Legin, A., Rudnitskaya, A., Seleznev, B. & Vlasov, Y. Electronic tongue for quality assessment of ethanol, vodka and eau-de-vie. Anal. Chim. Acta 534 , 129–135 (2005).
Loutfi, A., Coradeschi, S., Mani, G. K., Shankar, P. & Rayappan, J. B. B. Electronic noses for food quality: A review. J. Food Eng. 144 , 103–111 (2015).
Ahn, Y.-Y., Ahnert, S. E., Bagrow, J. P. & Barabási, A.-L. Flavor network and the principles of food pairing. Sci. Rep. 1 , 196 (2011).
Article CAS PubMed PubMed Central Google Scholar
Bartoshuk, L. M. & Klee, H. J. Better fruits and vegetables through sensory analysis. Curr. Biol. 23 , R374–R378 (2013).
Article CAS PubMed Google Scholar
Piggott, J. R. Design questions in sensory and consumer science. Food Qual. Prefer. 3293 , 217–220 (1995).
Article Google Scholar
Kermit, M. & Lengard, V. Assessing the performance of a sensory panel-panellist monitoring and tracking. J. Chemom. 19 , 154–161 (2005).
Cook, D. J., Hollowood, T. A., Linforth, R. S. T. & Taylor, A. J. Correlating instrumental measurements of texture and flavour release with human perception. Int. J. Food Sci. Technol. 40 , 631–641 (2005).
Chinchanachokchai, S., Thontirawong, P. & Chinchanachokchai, P. A tale of two recommender systems: The moderating role of consumer expertise on artificial intelligence based product recommendations. J. Retail. Consum. Serv. 61 , 1–12 (2021).
Ross, C. F. Sensory science at the human-machine interface. Trends Food Sci. Technol. 20 , 63–72 (2009).
Chambers, E. IV & Koppel, K. Associations of volatile compounds with sensory aroma and flavor: The complex nature of flavor. Molecules 18 , 4887–4905 (2013).
Pinu, F. R. Metabolomics—The new frontier in food safety and quality research. Food Res. Int. 72 , 80–81 (2015).
Danezis, G. P., Tsagkaris, A. S., Brusic, V. & Georgiou, C. A. Food authentication: state of the art and prospects. Curr. Opin. Food Sci. 10 , 22–31 (2016).
Shepherd, G. M. Smell images and the flavour system in the human brain. Nature 444 , 316–321 (2006).
Meilgaard, M. C. Prediction of flavor differences between beers from their chemical composition. J. Agric. Food Chem. 30 , 1009–1017 (1982).
Xu, L. et al. Widespread receptor-driven modulation in peripheral olfactory coding. Science 368 , eaaz5390 (2020).
Kupferschmidt, K. Following the flavor. Science 340 , 808–809 (2013).
Billesbølle, C. B. et al. Structural basis of odorant recognition by a human odorant receptor. Nature 615 , 742–749 (2023).
Article ADS PubMed PubMed Central Google Scholar
Smith, B. Perspective: Complexities of flavour. Nature 486 , S6–S6 (2012).
Pfister, P. et al. Odorant receptor inhibition is fundamental to odor encoding. Curr. Biol. 30 , 2574–2587 (2020).
Moskowitz, H. W., Kumaraiah, V., Sharma, K. N., Jacobs, H. L. & Sharma, S. D. Cross-cultural differences in simple taste preferences. Science 190 , 1217–1218 (1975).
Eriksson, N. et al. A genetic variant near olfactory receptor genes influences cilantro preference. Flavour 1 , 22 (2012).
Ferdenzi, C. et al. Variability of affective responses to odors: Culture, gender, and olfactory knowledge. Chem. Senses 38 , 175–186 (2013).
Article PubMed Google Scholar
Lawless, H. T. & Heymann, H. Sensory evaluation of food: Principles and practices. (Springer, New York, NY). https://doi.org/10.1007/978-1-4419-6488-5 (2010).
Colantonio, V. et al. Metabolomic selection for enhanced fruit flavor. Proc. Natl. Acad. Sci. 119 , e2115865119 (2022).
Fritz, F., Preissner, R. & Banerjee, P. VirtualTaste: a web server for the prediction of organoleptic properties of chemical compounds. Nucleic Acids Res 49 , W679–W684 (2021).
Tuwani, R., Wadhwa, S. & Bagler, G. BitterSweet: Building machine learning models for predicting the bitter and sweet taste of small molecules. Sci. Rep. 9 , 1–13 (2019).
Dagan-Wiener, A. et al. Bitter or not? BitterPredict, a tool for predicting taste from chemical structure. Sci. Rep. 7 , 1–13 (2017).
Pallante, L. et al. Toward a general and interpretable umami taste predictor using a multi-objective machine learning approach. Sci. Rep. 12 , 1–11 (2022).
Malavolta, M. et al. A survey on computational taste predictors. Eur. Food Res. Technol. 248 , 2215–2235 (2022).
Lee, B. K. et al. A principal odor map unifies diverse tasks in olfactory perception. Science 381 , 999–1006 (2023).
Mayhew, E. J. et al. Transport features predict if a molecule is odorous. Proc. Natl. Acad. Sci. 119 , e2116576119 (2022).
Niu, Y. et al. Sensory evaluation of the synergism among ester odorants in light aroma-type liquor by odor threshold, aroma intensity and flash GC electronic nose. Food Res. Int. 113 , 102–114 (2018).
Yu, P., Low, M. Y. & Zhou, W. Design of experiments and regression modelling in food flavour and sensory analysis: A review. Trends Food Sci. Technol. 71 , 202–215 (2018).
Oladokun, O. et al. The impact of hop bitter acid and polyphenol profiles on the perceived bitterness of beer. Food Chem. 205 , 212–220 (2016).
Linforth, R., Cabannes, M., Hewson, L., Yang, N. & Taylor, A. Effect of fat content on flavor delivery during consumption: An in vivo model. J. Agric. Food Chem. 58 , 6905–6911 (2010).
Guo, S., Na Jom, K. & Ge, Y. Influence of roasting condition on flavor profile of sunflower seeds: A flavoromics approach. Sci. Rep. 9 , 11295 (2019).
Ren, Q. et al. The changes of microbial community and flavor compound in the fermentation process of Chinese rice wine using Fagopyrum tataricum grain as feedstock. Sci. Rep. 9 , 3365 (2019).
Hastie, T., Friedman, J. & Tibshirani, R. The Elements of Statistical Learning. (Springer, New York, NY). https://doi.org/10.1007/978-0-387-21606-5 (2001).
Dietz, C., Cook, D., Huismann, M., Wilson, C. & Ford, R. The multisensory perception of hop essential oil: a review. J. Inst. Brew. 126 , 320–342 (2020).
CAS Google Scholar
Roncoroni, Miguel & Verstrepen, Kevin Joan. Belgian Beer: Tested and Tasted. (Lannoo, 2018).
Meilgaard, M. Flavor chemistry of beer: Part II: Flavor and threshold of 239 aroma volatiles. in (1975).
Bokulich, N. A. & Bamforth, C. W. The microbiology of malting and brewing. Microbiol. Mol. Biol. Rev. MMBR 77 , 157–172 (2013).
Dzialo, M. C., Park, R., Steensels, J., Lievens, B. & Verstrepen, K. J. Physiology, ecology and industrial applications of aroma formation in yeast. FEMS Microbiol. Rev. 41 , S95–S128 (2017).
Article PubMed PubMed Central Google Scholar
Datta, A. et al. Computer-aided food engineering. Nat. Food 3 , 894–904 (2022).
American Society of Brewing Chemists. Beer Methods. (American Society of Brewing Chemists, St. Paul, MN, U.S.A.).
Olaniran, A. O., Hiralal, L., Mokoena, M. P. & Pillay, B. Flavour-active volatile compounds in beer: production, regulation and control. J. Inst. Brew. 123 , 13–23 (2017).
Verstrepen, K. J. et al. Flavor-active esters: Adding fruitiness to beer. J. Biosci. Bioeng. 96 , 110–118 (2003).
Meilgaard, M. C. Flavour chemistry of beer. part I: flavour interaction between principal volatiles. Master Brew. Assoc. Am. Tech. Q 12 , 107–117 (1975).
Briggs, D. E., Boulton, C. A., Brookes, P. A. & Stevens, R. Brewing 227–254. (Woodhead Publishing). https://doi.org/10.1533/9781855739062.227 (2004).
Bossaert, S., Crauwels, S., De Rouck, G. & Lievens, B. The power of sour - A review: Old traditions, new opportunities. BrewingScience 72 , 78–88 (2019).
Google Scholar
Verstrepen, K. J. et al. Flavor active esters: Adding fruitiness to beer. J. Biosci. Bioeng. 96 , 110–118 (2003).
Snauwaert, I. et al. Microbial diversity and metabolite composition of Belgian red-brown acidic ales. Int. J. Food Microbiol. 221 , 1–11 (2016).
Spitaels, F. et al. The microbial diversity of traditional spontaneously fermented lambic beer. PLoS ONE 9 , e95384 (2014).
Blanco, C. A., Andrés-Iglesias, C. & Montero, O. Low-alcohol Beers: Flavor Compounds, Defects, and Improvement Strategies. Crit. Rev. Food Sci. Nutr. 56 , 1379–1388 (2016).
Jackowski, M. & Trusek, A. Non-Alcohol. beer Prod. – Overv. 20 , 32–38 (2018).
Takoi, K. et al. The contribution of geraniol metabolism to the citrus flavour of beer: Synergy of geraniol and β-citronellol under coexistence with excess linalool. J. Inst. Brew. 116 , 251–260 (2010).
Kroeze, J. H. & Bartoshuk, L. M. Bitterness suppression as revealed by split-tongue taste stimulation in humans. Physiol. Behav. 35 , 779–783 (1985).
Mennella, J. A. et al. A spoonful of sugar helps the medicine go down”: Bitter masking bysucrose among children and adults. Chem. Senses 40 , 17–25 (2015).
Wietstock, P., Kunz, T., Perreira, F. & Methner, F.-J. Metal chelation behavior of hop acids in buffered model systems. BrewingScience 69 , 56–63 (2016).
Sancho, D., Blanco, C. A., Caballero, I. & Pascual, A. Free iron in pale, dark and alcohol-free commercial lager beers. J. Sci. Food Agric. 91 , 1142–1147 (2011).
Rodrigues, H. & Parr, W. V. Contribution of cross-cultural studies to understanding wine appreciation: A review. Food Res. Int. 115 , 251–258 (2019).
Korneva, E. & Blockeel, H. Towards better evaluation of multi-target regression models. in ECML PKDD 2020 Workshops (eds. Koprinska, I. et al.) 353–362 (Springer International Publishing, Cham, 2020). https://doi.org/10.1007/978-3-030-65965-3_23 .
Gastón Ares. Mathematical and Statistical Methods in Food Science and Technology. (Wiley, 2013).
Grinsztajn, L., Oyallon, E. & Varoquaux, G. Why do tree-based models still outperform deep learning on tabular data? Preprint at http://arxiv.org/abs/2207.08815 (2022).
Gries, S. T. Statistics for Linguistics with R: A Practical Introduction. in Statistics for Linguistics with R (De Gruyter Mouton, 2021). https://doi.org/10.1515/9783110718256 .
Lundberg, S. M. et al. From local explanations to global understanding with explainable AI for trees. Nat. Mach. Intell. 2 , 56–67 (2020).
Ickes, C. M. & Cadwallader, K. R. Effects of ethanol on flavor perception in alcoholic beverages. Chemosens. Percept. 10 , 119–134 (2017).
Kato, M. et al. Influence of high molecular weight polypeptides on the mouthfeel of commercial beer. J. Inst. Brew. 127 , 27–40 (2021).
Wauters, R. et al. Novel Saccharomyces cerevisiae variants slow down the accumulation of staling aldehydes and improve beer shelf-life. Food Chem. 398 , 1–11 (2023).
Li, H., Jia, S. & Zhang, W. Rapid determination of low-level sulfur compounds in beer by headspace gas chromatography with a pulsed flame photometric detector. J. Am. Soc. Brew. Chem. 66 , 188–191 (2008).
Dercksen, A., Laurens, J., Torline, P., Axcell, B. C. & Rohwer, E. Quantitative analysis of volatile sulfur compounds in beer using a membrane extraction interface. J. Am. Soc. Brew. Chem. 54 , 228–233 (1996).
Molnar, C. Interpretable Machine Learning: A Guide for Making Black-Box Models Interpretable. (2020).
Zhao, Q. & Hastie, T. Causal interpretations of black-box models. J. Bus. Econ. Stat. Publ. Am. Stat. Assoc. 39 , 272–281 (2019).
Article MathSciNet Google Scholar
Hastie, T., Tibshirani, R. & Friedman, J. The Elements of Statistical Learning. (Springer, 2019).
Labrado, D. et al. Identification by NMR of key compounds present in beer distillates and residual phases after dealcoholization by vacuum distillation. J. Sci. Food Agric. 100 , 3971–3978 (2020).
Lusk, L. T., Kay, S. B., Porubcan, A. & Ryder, D. S. Key olfactory cues for beer oxidation. J. Am. Soc. Brew. Chem. 70 , 257–261 (2012).
Gonzalez Viejo, C., Torrico, D. D., Dunshea, F. R. & Fuentes, S. Development of artificial neural network models to assess beer acceptability based on sensory properties using a robotic pourer: A comparative model approach to achieve an artificial intelligence system. Beverages 5 , 33 (2019).
Gonzalez Viejo, C., Fuentes, S., Torrico, D. D., Godbole, A. & Dunshea, F. R. Chemical characterization of aromas in beer and their effect on consumers liking. Food Chem. 293 , 479–485 (2019).
Gilbert, J. L. et al. Identifying breeding priorities for blueberry flavor using biochemical, sensory, and genotype by environment analyses. PLOS ONE 10 , 1–21 (2015).
Goulet, C. et al. Role of an esterase in flavor volatile variation within the tomato clade. Proc. Natl. Acad. Sci. 109 , 19009–19014 (2012).
Article ADS CAS PubMed PubMed Central Google Scholar
Borisov, V. et al. Deep Neural Networks and Tabular Data: A Survey. IEEE Trans. Neural Netw. Learn. Syst. 1–21 https://doi.org/10.1109/TNNLS.2022.3229161 (2022).
Statista. Statista Consumer Market Outlook: Beer - Worldwide.
Seitz, H. K. & Stickel, F. Molecular mechanisms of alcoholmediated carcinogenesis. Nat. Rev. Cancer 7 , 599–612 (2007).
Voordeckers, K. et al. Ethanol exposure increases mutation rate through error-prone polymerases. Nat. Commun. 11 , 3664 (2020).
Goelen, T. et al. Bacterial phylogeny predicts volatile organic compound composition and olfactory response of an aphid parasitoid. Oikos 129 , 1415–1428 (2020).
Article ADS Google Scholar
Reher, T. et al. Evaluation of hop (Humulus lupulus) as a repellent for the management of Drosophila suzukii. Crop Prot. 124 , 104839 (2019).
Stein, S. E. An integrated method for spectrum extraction and compound identification from gas chromatography/mass spectrometry data. J. Am. Soc. Mass Spectrom. 10 , 770–781 (1999).
American Society of Brewing Chemists. Sensory Analysis Methods. (American Society of Brewing Chemists, St. Paul, MN, U.S.A., 1992).
McAuley, J., Leskovec, J. & Jurafsky, D. Learning Attitudes and Attributes from Multi-Aspect Reviews. Preprint at https://doi.org/10.48550/arXiv.1210.3926 (2012).
Meilgaard, M. C., Carr, B. T. & Carr, B. T. Sensory Evaluation Techniques. (CRC Press, Boca Raton). https://doi.org/10.1201/b16452 (2014).
Schreurs, M. et al. Data from: Predicting and improving complex beer flavor through machine learning. Zenodo https://doi.org/10.5281/zenodo.10653704 (2024).
Download references
Acknowledgements
We thank all lab members for their discussions and thank all tasting panel members for their contributions. Special thanks go out to Dr. Karin Voordeckers for her tremendous help in proofreading and improving the manuscript. M.S. was supported by a Baillet-Latour fellowship, L.C. acknowledges financial support from KU Leuven (C16/17/006), F.A.T. was supported by a PhD fellowship from FWO (1S08821N). Research in the lab of K.J.V. is supported by KU Leuven, FWO, VIB, VLAIO and the Brewing Science Serves Health Fund. Research in the lab of T.W. is supported by FWO (G.0A51.15) and KU Leuven (C16/17/006).
Author information
These authors contributed equally: Michiel Schreurs, Supinya Piampongsant, Miguel Roncoroni.
Authors and Affiliations
VIB—KU Leuven Center for Microbiology, Gaston Geenslaan 1, B-3001, Leuven, Belgium
Michiel Schreurs, Supinya Piampongsant, Miguel Roncoroni, Lloyd Cool, Beatriz Herrera-Malaver, Florian A. Theßeling & Kevin J. Verstrepen
CMPG Laboratory of Genetics and Genomics, KU Leuven, Gaston Geenslaan 1, B-3001, Leuven, Belgium
Leuven Institute for Beer Research (LIBR), Gaston Geenslaan 1, B-3001, Leuven, Belgium
Laboratory of Socioecology and Social Evolution, KU Leuven, Naamsestraat 59, B-3000, Leuven, Belgium
Lloyd Cool, Christophe Vanderaa & Tom Wenseleers
VIB Bioinformatics Core, VIB, Rijvisschestraat 120, B-9052, Ghent, Belgium
Łukasz Kreft & Alexander Botzki
AB InBev SA/NV, Brouwerijplein 1, B-3000, Leuven, Belgium
Philippe Malcorps & Luk Daenen
You can also search for this author in PubMed Google Scholar
Contributions
S.P., M.S. and K.J.V. conceived the experiments. S.P., M.S. and K.J.V. designed the experiments. S.P., M.S., M.R., B.H. and F.A.T. performed the experiments. S.P., M.S., L.C., C.V., L.K., A.B., P.M., L.D., T.W. and K.J.V. contributed analysis ideas. S.P., M.S., L.C., C.V., T.W. and K.J.V. analyzed the data. All authors contributed to writing the manuscript.
Corresponding author
Correspondence to Kevin J. Verstrepen .
Ethics declarations
Competing interests.
K.J.V. is affiliated with bar.on. The other authors declare no competing interests.
Peer review
Peer review information.
Nature Communications thanks Florian Bauer, Andrew John Macintosh and the other, anonymous, reviewer(s) for their contribution to the peer review of this work. A peer review file is available.
Additional information
Publisher’s note Springer Nature remains neutral with regard to jurisdictional claims in published maps and institutional affiliations.
Supplementary information
Supplementary information, peer review file, description of additional supplementary files, supplementary data 1, supplementary data 2, supplementary data 3, supplementary data 4, supplementary data 5, supplementary data 6, supplementary data 7, reporting summary, source data, source data, rights and permissions.
Open Access This article is licensed under a Creative Commons Attribution 4.0 International License, which permits use, sharing, adaptation, distribution and reproduction in any medium or format, as long as you give appropriate credit to the original author(s) and the source, provide a link to the Creative Commons licence, and indicate if changes were made. The images or other third party material in this article are included in the article’s Creative Commons licence, unless indicated otherwise in a credit line to the material. If material is not included in the article’s Creative Commons licence and your intended use is not permitted by statutory regulation or exceeds the permitted use, you will need to obtain permission directly from the copyright holder. To view a copy of this licence, visit http://creativecommons.org/licenses/by/4.0/ .
Reprints and permissions
About this article
Cite this article.
Schreurs, M., Piampongsant, S., Roncoroni, M. et al. Predicting and improving complex beer flavor through machine learning. Nat Commun 15 , 2368 (2024). https://doi.org/10.1038/s41467-024-46346-0
Download citation
Received : 30 October 2023
Accepted : 21 February 2024
Published : 26 March 2024
DOI : https://doi.org/10.1038/s41467-024-46346-0
Share this article
Anyone you share the following link with will be able to read this content:
Sorry, a shareable link is not currently available for this article.
Provided by the Springer Nature SharedIt content-sharing initiative
By submitting a comment you agree to abide by our Terms and Community Guidelines . If you find something abusive or that does not comply with our terms or guidelines please flag it as inappropriate.
Quick links
- Explore articles by subject
- Guide to authors
- Editorial policies
Sign up for the Nature Briefing: Translational Research newsletter — top stories in biotechnology, drug discovery and pharma.


An official website of the United States government
Here’s how you know
Official websites use .gov A .gov website belongs to an official government organization in the United States.
Secure .gov websites use HTTPS A lock ( Lock A locked padlock ) or https:// means you’ve safely connected to the .gov website. Share sensitive information only on official, secure websites.
https://www.nist.gov/publications/case-study-multi-modal-multi-institutional-data-management-combinatorial-materials
A case study of multi-modal, multi-institutional data management for the combinatorial materials science community
Download paper, additional citation formats.
- Google Scholar
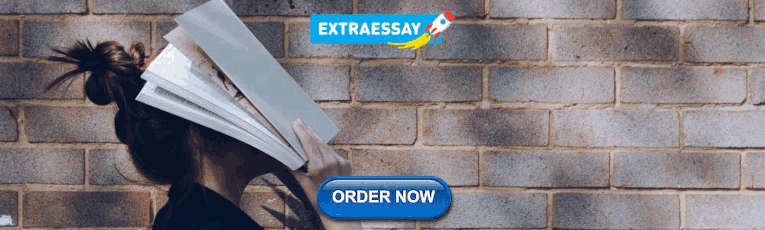
IMAGES
VIDEO
COMMENTS
written by RSI Security September 14, 2023. In September 2011, The National Institute for Standard and Technology (NIST) created Special Publication (SP) 500-292, "NIST Cloud Computing Reference Architecture," to establish a baseline cloud computing architecture. NIST SP 500-292 defines services and relationships between cloud service ...
This Special Publication 800-series reports on ITL‟s research, guidance, and outreach efforts in computer security and its collaborative activities with industry, government, and academic organizations. National Institute of Standards and Technology Special Publication 500-292 Natl. Inst. Stand. Technol. Spec.
NIST SP 800-171. Defense Federal Acquisition Regulation Supplement (DFARS) 252.204-7012 - Network Penetration Reporting and Contracting for Cloud Services. Effective October 21, 2016. Department of Defense (DoD) Requires the implementation of the security requirements in NIST SP 800-171. Deadline is December 31, 2017.
NIST Cloud Computing reference architecture defines five major performers: Cloud Provider. Cloud Carrier. Cloud Broker. Cloud Auditor. Cloud Consumer. Each performer is an object (a person or an organization) that contributes to a transaction or method and/or performs tasks in Cloud computing. There are five major actors defined in the NIST ...
The NIST Cloud Computing Security Reference Architecture provides a case study that walks readers through steps an agency follows using the cloud-adapted Risk Management Framework while deploying a typical application to the cloud—migrating existing email, calendar and document-sharing systems as a unified, cloud-based messaging system.
This document introduces the NIST Cloud Computing Security Reference Architecture (NCC-SRA or, for the sake of brevity, SRA), providing a comprehensive formal model to serve as security overlay to the architecture described in NIST SP 500-292: NIST Cloud Computing Reference Architecture. This document also describes a methodology for applying a ...
Zero trust (ZT) is the term for an evolving set of cybersecurity paradigms that move defenses from static, network- based perimeters to focus on users, assets, and resources. A zero trust architecture (ZTA) uses zero trust principles to plan industrial and enterprise infrastructure and workflows. Zero trust assumes there is no implicit trust ...
The NIST Cloud Federation Reference Architecture (CFRA) is presented in ten parts: a complete overview of the actors and their roles, and the necessary architectural components for managing and providing cloud services such as service deployment, service orchestration, cloud service management, security and privacy.
The purpose of this document is to define a NIST Cloud Computing Security Reference Architecture (NCC-SRA)--a framework that: i) identifies a core set of Security Components that can be implemented in a Cloud Ecosystem to secure the environment, the operations, and the data migrated to the cloud; ii) provides, for each Cloud Actor, the core set of Security Components that fall under their ...
vice-based approaches such as Service-Oriented Architecture or Pervasive Com-1 E. Simmon ( ) National Institute of Standards and Technology, 100 Bureau Drive Stop 8120, Gaithersburg, MD 20899-8970, USA e-mail: [email protected] 2 R. Bohn National Institute of Standards and Technology J. Stjepandic´ et al. (eds.),
NIST announces the publication of a Cybersecurity White Paper (CSWP), Planning for a Zero Trust Architecture: A Guide for Federal Administrators, which describes processes for migrating to a zero trust architecture using the NIST Risk Management Framework (RMF). Zero trust is a set of principles designed to reduce or remove implicit trust in networked systems by addressing network identity ...
The NIST Enterprise Architecture Model is a five-layered model for enterprise architecture, designed for organizing, planning, and building an integrated set of information and information technology architectures. The five layers are defined separately but are interrelated and interwoven. [2] The model defined the interrelation as follows: [3 ...
Solution. A zero trust architecture is the perfect solution for highly complex, distributed, multi levels-of-maturity organizations because zero trust is a design principle or framework rather than a defined technology stack or tool. This means that decisions by Cimpress Security that are rolled down to its business units must adhere to the ...
Abstract. Evaluating cyber security risk is a challenging task regardless of an organisation's nature of business or size, however, an essential activity. This paper uses the National Institute of Standards and Technology (NIST) cyber security framework (CSF) to assess the cyber security posture of a local government organisation in Western ...
Case Study: Cloud-Native Security Using Zero Trust. For most organizations, cloud usage is now the norm. Much like the adoption path of many technologies, the road to cloud normalization started with an initial reluctance and has only recently begun to gain wider acceptance. More specifically, many organizations had initial reservations about ...
In the case study document provided with this course, you'll read numerous details about Red30 Tech. Use those as you're working through the different functions and phases of the NIST RMF.
Framework for Manufacturing" to provide a generic guideline and a reference architecture for case-specific digital twin implementations (ISO 2020). Currently, many case studies of digital twin implementations are within a laboratory environment. For example, an Automated Guided Vehicle (AGV) or Cyber Guided Vehicle (CGV) with self-adapting ...
By the end of the course, you will have a deep understanding of the NIST CSF and how to use it to improve your organization's cybersecurity posture. In summary, this NIST Cyber Security Framework Online Training + Case Studies is designed to help professionals gain the knowledge and skills needed to understand, implement and comply with NIST CSF.
Case Study Of Nist Architecture - Writing. User ID: 407841. For expository writing, our writers investigate a given idea, evaluate its various evidence, set forth interesting arguments by expounding on the idea, and that too concisely and clearly. Our online essay writing service has the eligibility to write marvelous expository essays for you. ...
Case Studies. CONTAM and other multizone modeling software is often used in the field of building ventilation and IAQ research. However, the use of multizone modeling in more practical applications is increasing. Along with building research, multizone modeling can be applied to areas of building design and operation.
President Barack Obama Executive Order 13636, Feb. 12, 2013. • NIST was directed to work with stakeholders to develop a voluntary framework for reducing cybersecurity risks to critical infrastructure. • Version 1.0 of the framework was released on Feb. 12, 2014, along with a roadmap for future work. About the Process.
POMPANO BEACH, Fla., March 28, 2024 /PRNewswire-PRWeb/ -- Case Study House #18. Modern-style architecture was very popular in the US and Europe between the 1930s and '60s, marked by a stark ...
Download Nero Tapware's case study to learn more about the luxurious amenities and modern features of Project Bordeaux in Melbourne's Bentleigh East, crafted by Elation Group. Project Bordeaux, a ...
The following Case Studies were created by the National Cyber Security Alliance, with a grant from NIST, and should prove useful in stimulating ongoing learning for all business owners and their employees. Case 1: A Business Trip to South America Goes South Topic: ATM Skimming and Bank Fraud; Case 2: A Construction Company Gets Hammered by a ...
The NIST MS Search software (v2.0 g) in combination with the NIST2017, FFNSC3 and Adams4 libraries were used to manually identify the empirical spectra, taking into account the expected retention ...
In this paper we describe a case study in the use of the OMA to integrate legacy software components into a distributed object system. We assess the OMA in this problem context, and indicate strengths and weaknesses of the specification and current implementations.
Here, we present a case study for the low-barrier development of such a dashboard that enables standardized organization, analysis, and visualization of a large data lake consisting of combinatorial datasets of synthesis and processing conditions, X-ray diffraction patterns, and materials property measurements generated at several different ...