Academia.edu no longer supports Internet Explorer.
To browse Academia.edu and the wider internet faster and more securely, please take a few seconds to upgrade your browser .
Enter the email address you signed up with and we'll email you a reset link.
- We're Hiring!
- Help Center
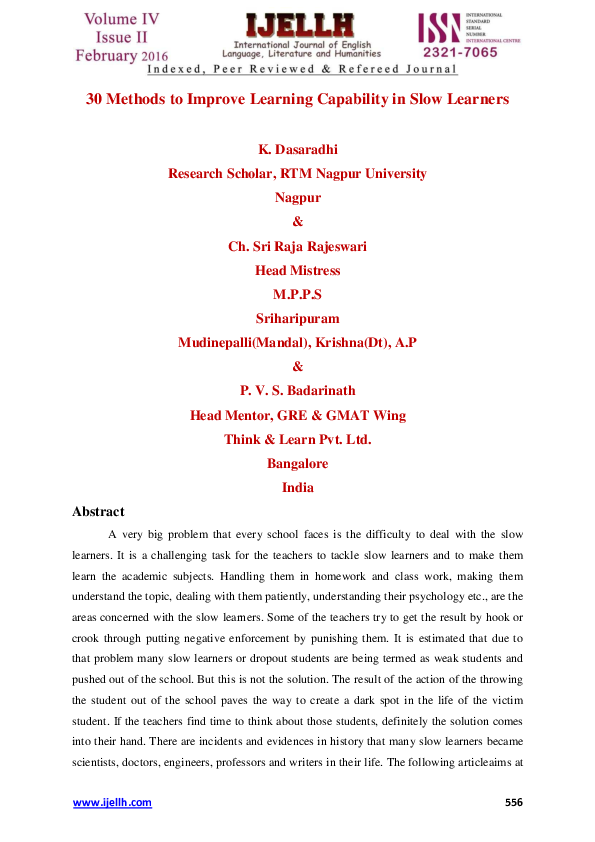
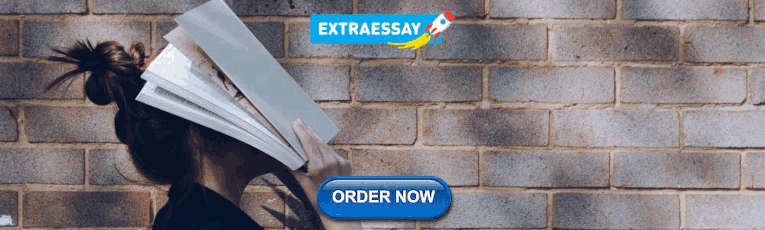
30 Methods to Improve Learning Capability in Slow Learners

A very big problem that every school faces is the difficulty to deal with the slow learners. It is a challenging task for the teachers to tackle slow learners and to make them learn the academic subjects. Handling them in homework and class work, making them understand the topic, dealing with them patiently, understanding their psychology etc., are the areas concerned with the slow learners. Some of the teachers try to get the result by hook or crook through putting negative enforcement by punishing them. It is estimated that due to that problem many slow learners or dropout students are being termed as weak students and pushed out of the school. But this is not the solution. The result of the action of the throwing the student out of the school paves the way to create a dark spot in the life of the victim student. If the teachers find time to think about those students, definitely the solution comes into their hand. There are incidents and evidences in history that many slow learners became scientists, doctors, engineers, professors and writers in their life. The following articleaims at www.ijellh.com 557 helping the teachers to handle the slow learners in an appropriate way thereby increasing the learning capability of the slow learners making teaching-learning more purposeful.
Related Papers
Galata Fekadu
Abstract A very big problem that every school faces is the difficulty to deal with the slow learners. It is a challenging task for the teachers to tackle slow learners and to make them learn the academic subjects. Handling them in homework and class work, making them understand the topic, dealing with them patiently, understanding their psychology etc., are the areas concerned with the slow learners. Some of the teachers try to get the result by hook or crook through putting negative enforcement by punishing them. It is estimated that due to that problem many slow learners or dropout students are being termed as weak students and pushed out of the school. But this is not the solution. The result of the action of the throwing the student out of the school paves or covers the way to create a dark spot in the life of the victim student. If the teachers find time to think about those students, definitely the solution comes into their hand. There are incidents and evidences in history that many slow learners became scientists, doctors, engineers, professors and writers in their life. This project or article aims helping the teachers to handle the slow learners in an appropriate way thereby increasing the learning capability or score of the slow learners making teaching-learning more purposeful. Key Words: academic, class, improve, Score, learn, method, slow learner, teacher
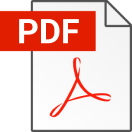
International Journal of Scientific Research in Science, Engineering and Technology IJSRSET
Allahabad Slow Learners are those children who are low achieving academic skills and often ignored by others as dull, Lazy or inept in the school setting. Slow learners log behind other students not only in academics but also in social, emotional that 5 to 15 percent of school going children, suffer from scholastic backwardness. The early identification of students who are at risk for educational failure is an important process that deserves much attention and research. Dr. S.Subramaniam says that although they have no intellectual disability, no identifiable neurological impairments and no learning disorders, slow learners perform poorly in school and exhibit discrepancy between expected achievement based on their intellectual ability assessment and actual achievement as children advance in school, There is a corresponding increase in difficult as school subject and assignments. Thus, children's performance depends progressively more on their motivation to sustain and active commitment to and efforts towards self regulated learning. 1 A pretest posttest random control group experimental group research design was followed to assess the effects of the integrated instruction on the academic performance and psychological indices of slow learners. (i) Experimental Group :-the total sample comprised of 10 slow learners as experimental groups studying in 5 th class in primary school who where giving and exposure to a specially designed integrated intervention program for the duration of 5 weeks period of time. (ii) Control group :-Another group 10 slow learners of 5 th class drawn from the same school were kept as control group. No such exposure to intervention would be given for this group. In order to have the homogeneity in the sample size, they were matched for age, gender, IQ, mother tongue, socioeconomic status etc. The slow learners were selected using the following three screening methods based on their academic performance teaches report and IQ test. A-Academic performance :-Only those students who scored less then 50 % of marks in all the subjects consistently for the first monthly and quarterly examination were selected. B-Teachers assessment:-Teacher's ratings on children's overall performance in class room and those who were found to be dull or below average were identified as slow learners. A three-tier mutually supportive integrated intervention program was administered to the slow learners. The following three types of activities such as graphic organizer, mindfulness meditation and mnemonics which had been designed exclusively to address the specific needs of the slow learners
Nurul Izzati
Human resource development should be at the focus of any educator for a developing country like India which has abundant human resources. In the Indian system of educational, it is observed that the human resources-teachers and learners are underdeveloped and perform less than their capabilities. The learners and underdeveloped in the sense that they are not achieving in tune with their capabilities. Even some of the most efficient teachers are not adequately equipped to identify and guide the backward students like slow learners to reach their optimum levels. As a result, the institutions in tune are not able to send their products into society as fully developed learners. To ensure this we need special educational programmes for backward children like slow learners. " Those who are slow to know suppose that slowness is the essence of knowledge " Friedrich Nietzsche ______________________________________________________________________________
Journal of the Research Society of Pakistan
Dr. Kashif Ishaq
The purpose of the study was to determine the classroom practices being used by teachers to teach slow learners in institutions run under the department of special education, the government of Punjab. The study was quantitative, and it was delimited to government institutions for slow learners in Punjab. The sample of the study was all the educators teaching in the institutes for slow learners and data was collected through a self-administered questionnaire consisted of 42 items. Statistical Package for Social Science (SPSS) was used to calculate Mean, Standard Deviation (SD), and t-test to measure classroom practices, gender-wise response differences. The finding showed that almost all the teachers planned the lesson according to the individual needs of students, used motivational, instructional strategies, used simple vocabulary and language, and taught modified curriculum. There was no statistically significant difference between male and female teachers applying these practices in classrooms.
International Journal for Research in Applied Science and Engineering Technology
Shafiq Khalil
The main purpose of the study is to evaluate the teacher’s perception towards slow learners academic achievement and role of guidance services at secondary school levels in district Peshawar. This was a Mixed Method research. Data was collected through administering especially designed questionnaire and used conveniently sample designed for selection of 200 Public and Private secondary schools Teachers/Head Masters, both male and female. Teachers/Head Masters were also asked to write suggestions for the improvement. It is found that positive teacher-student relation is necessary for the academic achievement of the students, especially the slow learners. Most of the teachers agreed that they take interest in the academics of their students and that they know how to teach their subject in a better way. Majority of the teachers responded positively that they can motivate the students for learning and that students feel comfortable in their class and they take feedback during the lesson...
Muhammad Sani Abdurrahman
Poor performance of students in mathematics subject has assumed a dangerous dimension. In light of this, mathematics educators need to seek suitable ways of tackling the current mass failure. It is now being recognized that there are better ways to learn mathematics than through the traditional method. Mathematics educators are beginning to show an increased awareness of the important of the way students learn. Many of our standard methods of conveying knowledge have been shown to be relatively ineffective in the slow learners ’ ability to master and then retain important concepts.To achieve this, the paper therefore examined the influence of laboratory activities and peer tutoring on slow-learners achievement and retention in senior secondary school trigonometry. Four research hypotheses were formulated to guide the study. Pre-test post-test quasi experimental design was used in the study. The population consisted of 84,573 senior secondary school students out of which 382 students...
Contrary to common belief, slow learners in the regular classroom are neither rare nor unique. The student commonly called a slow learner is one who cannot learn at an average rate from the instructional resources, texts, workbooks, and learning materials that are designed for the majority of students in the classroom. These students need special instructional pacing, frequent feedback, corrective instruction, and/or modified materials, all administered under conditions sufficiently flexible for learning to occur. Slow learners are usually taught in one of two possible instructional arrangements: 1) a class composed mostly of average students, in which case up to 20%may be slow learners, or 2) a class specially designed for slow learners. Whether you meet slow learners in a regular class or special class, you will immediately feel the challenge of meeting their learning needs. Their most obvious characteristic is a limited attention span compared to more able students. To keep these students actively engaged in the learning process requires more than the usual variation in presentation methods (direct, indirect), classroom climate (cooperative , competitive), and instructional materials (films, workbooks, cooperative games, simulations). If this variation is not part of your lesson, these students may well create their own variety in ways that disrupt your teaching. Other immediately noticeable characteristics of slow learners are their deficiencies in basic skills (reading, writing, and mathematics), their difficulty in comprehending abstract ideas, and most disconcerting, their sometimes unsystematic and careless work habits.
ETUDE: Journal of Educational Research
Amalia Febriyani
Background: Low intellect makes slow learner students feel inferior and feel that they will never learn. As a consequence, slow learner students, sometimes, have no motivation to learn in them. Motivation has an important role in children's learning activities. It can be said that motivation is the driving force for children's activities. One of them is MF, a student identified as a slow learner by their class teacher. Purpose: For this reason, this study aims to determine the extent to which the causes of changes in MF motivation levels in the teaching and learning activities. Design and methods: This research uses a case study method. This research was conducted on one of the students identified by the class teacher as a slow learner. This research data using observation, interviews, and documentation. The collected data were grouped based on various factors that influenced learning motivation initiated by Dimyati and Mudjiono. Validation is done by triangulating data sour...
Proceeding International Conference on Science and Engineering
Dr.Anagha Soman
A class comprises of students of different grasping levels and capabilities and hence it becomes a really difficult task for a teacher to teach all of them with the same pace and stipulated time period. The students in a class can be categorized on the basis of their academic excellence and then additional efforts can be taken accordingly for a group of students. Also, extra assignment can be given to fast learners. Broadly, this can be divided into three steps i.e. identifying the factors that directly have an impact on student’s performance, Individual analysis of students as per the institutional framework ( Mentor-mentee, class teacher, Head of department, Grievance cell, Principal), Using these first two steps, how to enhance the student’s performance in all aspects. This paper is a small effort to initiate the process of identifying, analyzing and providing solution for all round development of slow learners and fast learners.
International Journal of Progressive Sciences and Technologies (IJPSAT), ISSN: 2509-0119
Zareen Shakeel
Slow learners are poor at achieving academic skills and are often ignored by teachers. Slow learners lag behind other students in academics and in the areas of social, emotional, and psychological well-being. The present study aims to find out the English language teachers' perspective on teaching the English language to slow learners and how far they feel the need to modify the present curriculum on the basis of learners' ability. To serve the desired purpose, 20 teachers working at the Faculty of Arts and Women's College, Department of English, Aligarh Muslim University, have been selected as study subjects. The researchers have used a five-point Likert scale to determine their perspectives. The findings aim to identify slow learners and help them critically evaluate their learning, gain confidence and develop strategies that facilitate them to overcome fear.
RELATED PAPERS
soportetics08d01 esmeraldas
Jurnal Bioteknologi & Biosains Indonesia (JBBI)
rike oktarianti
Tonos Digital Revista Electronica De Estudios Filologicos
Maria Marcos Ramos
Eduardo Natividade
János Pipics
The Islamic University of Gaza, Palestine
Halima Benzoukh
European Journal of Public Health
Taisto Sarkola
European Journal of Combinatorics
Martin Loebl
FAVE Sección Ciencias Agrarias
Jhon Fredy Gonzalez
Tropical Animal Science Journal
Mustofa Effendi
Laurence Sigal
J-Dinamika : Jurnal Pengabdian Masyarakat
Marlina Marzuki
Activitas Nervosa Superior
Sandro Sozzo
Pflügers Archiv European Journal of Physiology
Jerneja Suput
Pakistan Journal of Ophthalmology
Tayyaba Malik
De Jure: Jurnal Hukum dan Syar'iah
Sandro wahyu Permadi
Acoustical Science and Technology
Maori Kobayashi
Journal of the architectural institute of Korea planning & design
Young Ook Kim
Chad Kerksick
Dewald Koen
Biochemical and Biophysical Research Communications
Swiany Lima
Ewa Niewiadomska-Szynkiewicz
The Journal of Experimental Medicine
Klaus Rajewsky
Virology Journal
Aekkachai Tuekprakhon
Raffles Bulletin of Zoology
Andrew Hearn
See More Documents Like This
- We're Hiring!
- Help Center
- Find new research papers in:
- Health Sciences
- Earth Sciences
- Cognitive Science
- Mathematics
- Computer Science
- Academia ©2024

An official website of the United States government
The .gov means it’s official. Federal government websites often end in .gov or .mil. Before sharing sensitive information, make sure you’re on a federal government site.
The site is secure. The https:// ensures that you are connecting to the official website and that any information you provide is encrypted and transmitted securely.
- Publications
- Account settings
Preview improvements coming to the PMC website in October 2024. Learn More or Try it out now .
- Advanced Search
- Journal List
- Dev Cogn Neurosci
- v.40; 2019 Dec
Faster learners transfer their knowledge better: Behavioral, mnemonic, and neural mechanisms of individual differences in children’s learning
Hyesang chang.
a Department of Psychiatry & Behavioral Sciences, Stanford, CA 94305, United States
Miriam Rosenberg-Lee
d Department of Psychology, Rutgers University, Newark, NJ 07102, United States
Shaozheng Qin
e State Key Laboratory of Cognitive Neuroscience and Learning & IDG/McGovern Institute for Brain Research, Faculty of Psychology at Beijing Normal University, Beijing, China
Vinod Menon
b Department of Neurology & Neurological Sciences, Stanford, CA 94305, United States
c Stanford Neurosciences Institute, Stanford University School of Medicine, Stanford, CA 94305, United States
Associated Data
- • We examined neurocognitive mechanisms underlying learning and near transfer in elementary school children.
- • A five-day tutoring led to marked differentiation of behavioral and brain responses between trained and novel problems.
- • Children who learned trained problems faster transferred their learnt knowledge to novel problems better.
- • Faster learners showed greater overlap in local neural representations between trained and novel problems.
- • More efficient learning led to greater differentiation of functional brain circuits engaged by trained and novel problems.
Why some children learn, and transfer their knowledge to novel problems, better than others remains an important unresolved question in the science of learning. Here we developed an innovative tutoring program and data analysis approach to investigate individual differences in neurocognitive mechanisms that support math learning and “near” transfer to novel, but structurally related, problems in elementary school children. Following just five days of training, children performed recently trained math problems more efficiently, with greater use of memory-retrieval-based strategies. Crucially, children who learned faster during training performed better not only on trained problems but also on novel problems, and better discriminated trained and novel problems in a subsequent recognition memory task. Faster learners exhibited increased similarity of neural representations between trained and novel problems, and greater differentiation of functional brain circuits engaged by trained and novel problems. These results suggest that learning and near transfer are characterized by parallel learning-rate dependent local integration and large-scale segregation of functional brain circuits. Our findings demonstrate that speed of learning and near transfer are interrelated and identify the neural mechanisms by which faster learners transfer their knowledge better. Our study provides new insights into the behavioral, mnemonic, and neural mechanisms underlying children’s learning.
1. Introduction
Efficient learning involves the ability to acquire knowledge quickly while also being able to transfer the newly acquired knowledge beyond specific contexts and instances. A fundamental unresolved question in the science of learning is why some individuals learn and transfer their knowledge better than others, a question first posed by Thorndike in his seminal studies of learning ( Thorndike, 1906 ). This issue is particularly relevant in childhood when transfer of knowledge is critical for successful learning and cognitive development ( Spelke, 2000 ). Here we employ an individual differences approach to address critical gaps in our understanding of brain and cognitive mechanisms that support learning and near transfer in elementary school children.
Cognitive training in adults has been shown to be effective in improving performance on specific tasks and problem sets that individuals are trained on. Whether training also improves performance on novel problems or contexts remains controversial, even for near transfer involving “transfer tasks that share many elements with the practiced tasks” ( Simons et al., 2016 ). Possible reasons are that extant studies have focused on difficult-to-achieve “far” transfer effects across disparate cognitive domains and contexts ( Melby-Lervåg and Hulme, 2013 ) and few have considered individual differences in learning that may influence transfer abilities to novel problems ( Jaeggi et al., 2011 ). Whether cognitive training facilitates “near” transfer of knowledge between trained and novel, but structurally similar, problems remains unresolved ( Jaeggi et al., 2014 ). Understanding the mechanisms underlying individual differences in learning and near transfer is thus crucial for addressing this question and, specifically, for determining why some children learn and transfer their knowledge better than others.
Investigations of the brain basis of learning and transfer are faced with a central challenge. On the one hand, learning of specific instances should lead to formation of more distinct or segregated circuits that allow trained problems to be processed differently from novel ones ( Bassett et al., 2015 ; Iuculano et al., 2015 ; Jolles et al., 2013 ). On the other hand, generalizable knowledge should depend on formation of shared neural representations and functional integration of circuits involved in processing trained and novel problems ( Dahlin et al., 2008 ; Schlichting et al., 2015 ). Thus, learning and transfer may rely on competing neural mechanisms; consequently, a major unaddressed question in the field is whether segregation and integration processes occur in parallel to support different aspects of learning. In the present study, we use multiple brain imaging analysis techniques to investigate this question by examining overlap of neural representations within specific brain areas as well as segregation of large-scale functional brain circuits during a critical stage of cognitive skill acquisition in elementary school children.
We developed a novel interactive five-day math tutoring program ( Fig. 1 ) and data analysis approach ( Fig. 2 ), which allowed us to characterize individual differences in learning profiles, its relation to near transfer, and the neural mechanisms underlying successful learning and near transfer in children. We focus on arithmetic problem solving in which learning-related brain and behavioral changes, as well as changes in problem-solving strategies, can be systematically assessed in a quantitatively rigorous manner ( Anderson et al., 2014 ; Cho et al., 2011 ; Delazer et al., 2003 ; Geary, 2011 ; Rosenberg-Lee et al., 2018 ). Understanding mechanisms underlying successful learning and near transfer in this domain is particularly relevant in today’s technologically-driven world as arithmetic knowledge provides foundational knowledge and skills gained in early childhood are strongly predictive of later academic achievement and professional success ( Butterworth and Walsh, 2011 ; Faulkner, 2008 ; Geary, 2011 ; Geary et al., 2017 ; Jordan et al., 2009 ).

Training protocol and sample tutoring materials. (a) Before training, children completed a WJ-III math fluency test, a strategy assessment, and received an introductory lesson for addition problem solving. Training sessions were spread across five days within a week and consisted of a variety of activities for solving 14 problems in the training set. The number of exposures to each problem in each activity is reported in parenthesis. Each problem was presented 14 times per day, and 70 times over the training period. At the end of training, children’s problem-solving strategies for trained and novel problems were reassessed. After training, children completed a fMRI task that involved solving trained and novel problems in the scanner. This was followed by a recognition memory task, outside of the scanner, in which children were asked to discriminate between problems they have practiced during tutoring and those they saw in the fMRI task. The type of problems presented at each stage are indicated below the training protocol. (b) Sample materials used in the tutoring . In flash cards , children verbally produced an answer to trained problems presented on a physical flash card. The tutor proceeded to the next problem once a correct answer was provided. Children completed untimed flash cards without a timer. In timed flash cards , the tutor marked the time children spent in each of three rounds (each round: full deck of trained problems) on a sailboat. Children were instructed to try to beat their previous time(s). In computerized flash cards , children typed in their answer to trained problems and received feedback on their response. In treasure hunt , children placed physical cards with trained problems on numbers corresponding to the answers in a ‘treasure map.’ Upon completion of all tutoring activities, children filled a treasure board and received a prize. (c) Sample lesson presented during tutoring . At pre-training and on training days 1–3, children reviewed a break-apart strategy with the problems in the training set and were encouraged to solve them using a method of their choice. On training days 4–5, children were asked to retrieve answers directly from memory whenever possible.

Schematic overview of analysis approach. Behavioral and neural correlates of learning outcomes were examined (i) at the group level and (ii) in terms of individual differences in learning and near transfer across trained and novel problems. Trained problems consisted of a set of 14 addition problems learned across five days; Novel problems consisted of a set of 14 novel addition problems that were matched in difficulty to Trained problems. Behavioral performance was assessed using an efficiency score (ES) in each child that was based on accuracy and reaction times. Learning rates were computed in each child using an exponential regression fit to daily ES of trained problems (see Methods for details).
Behavioral studies have shown that repeated practice is associated with improved performance and a shift from effortful procedural to memory-based retrieval strategies during arithmetic problem solving ( Barrouillet and Fayol, 1998 ; Imbo & Vandierendonck, 2008; LeFevre et al., 1996; Logan, 1988; Siegler and Shipley, 1995 ; Siegler & Shrager, 1984). Neuroimaging studies in adults have demonstrated that arithmetic training is associated with decreased activation in the fronto-parietal cortex and relative increases in the left angular gyrus ( Delazer et al., 2003 , 2005 ; Grabner et al., 2009 ; Ischebeck et al., 2006 ) and the medial temporal lobe (MTL) ( Bloechle et al., 2016 ; Klein et al., 2018 ). In children, the hippocampus has been implicated in training-related performance gain and increase in retrieval rates as well as age-related changes in brain activation and neural representational stability ( Qin et al., 2014 ; Rivera et al., 2005 ; Rosenberg-Lee et al., 2018 ; Supekar et al., 2013 ). However, there have been no systematic investigations of how children’s learning and near transfer of problem-solving knowledge and skills varies across individuals. Here we employ an individual differences approach to address critical gaps in our understanding of brain and cognitive mechanisms that support learning and near transfer of arithmetic problem-solving skills in elementary school children.
Prior to tutoring, children aged 8–10 years completed a basic arithmetic fluency test, used to control for baseline math ability, and a strategy assessment task, which assessed the relative use of retrieval and elaborate verbal and finger counting procedures during problem solving ( Wu et al., 2008 ). During tutoring, children practiced a set of double-digit plus single-digit addition problems – problems not typically solved by memory retrieval without deliberate practice and appropriate for training 8–10 years old children – across five sessions within a one-week period. The tutoring was designed to facilitate arithmetic problem-solving skills as well as rapid retrieval of math facts through interactive sessions with a tutor ( Supekar et al., 2013 ). The present study used a similar one-on-one tutoring protocol as in Supekar et al., but here we used more complex addition problems and employed a more intensive, short-term training lasting 5 days. Similar to experimental designs used in arithmetic training studies of adults ( Delazer et al., 2003 ), children’s performance on both trained and novel problems were examined post-training. Crucially, performance on trained problems was assessed by a computerized task on each of the 5 days of tutoring and individual learning profiles were determined in each child. To our knowledge, no other studies used a similar experimental design and individual differences approach in 8–10 years old children.
Following tutoring, children’s problem-solving strategies were reassessed. Children were asked to solve recently trained problems intermixed with a set of novel addition problems during a functional magnetic resonance imaging (fMRI) scanning session. Novel problems, matched to trained problems in structure and difficulty, were presented only at post-training fMRI session in order to minimize exposure to these problems, similar to the experimental design used in arithmetic training fMRI studies in adults ( Delazer et al., 2003 , 2005 ; Grabner et al., 2009 ; Ischebeck et al., 2006 ). After the fMRI scan, a recognition memory task was administered outside of the scanner to determine children’s ability to discriminate between trained and novel problems. By carefully designing matched two types of problems, trained and novel, only varying in whether or not they were practiced, the current study examined training-induced differences between these problems while minimizing practice effects on novel problems.
We first examined behavioral performance and strategies used to solve problems before and after training. We hypothesized that training would result in more efficient performance and increased reliance on memory-retrieval-based strategies for trained, compared to novel, problems, similar to past developmental and training studies ( Barrouillet and Fayol, 1998 ; Delazer et al., 2003 ; Siegler and Shipley, 1995 ). We next examined brain activation levels for trained versus novel problems. Based on previous developmental findings ( Skeide et al., 2018 ; Qin et al., 2014 ; Rosenberg-Lee et al., 2018 ) and training studies ( Bloechle et al., 2016 ; Klein et al., 2018 ), we predicted that for trained, compared to novel, problems after training, children would exhibit greater responses in the MTL, reflecting greater engagement of learning and memory systems for specific problems they had practiced, and a relative decrease in fronto-parietal network activation, due to reduced working memory demands (Chein & Schneider, 2012; Salmi et al., 2018 ) for practiced problems ( Delazer et al., 2003 ).
We then examined individual differences in learning and tested the hypothesis that children who were faster learners, with steeper learning curves across training, would, after training, solve novel problems more efficiently, reflecting near transfer. We investigated whether faster learning rate would be associated with greater ability to discriminate between trained and novel problems. To elucidate the neural mechanisms by which individuals transfer their learnt knowledge to novel problems, we then used representational similarity analysis (RSA). RSA provides a unique window into common neural patterns of information processing across task conditions and problem types within specific brain areas ( Haxby et al., 2014 ; Kriegeskorte et al., 2008 ). Here, we used RSA to assess the degree of overlap in the spatial patterns of brain activity between trained and novel problems and predicted that, after training, faster learners would exhibit greater similarity in neural representations between trained and novel problems, reflecting integration of local neural representations across structurally similar problems. To determine how learning rate influences responses to individual problems, we examined stability of neural representational patterns, across individual trained or novel problems. Building on a previous study where we found that neural representations of individual problems become more stabilized across cognitive skill development ( Qin et al., 2014 ), we hypothesized that children who learn faster would reach later stages of learning more quickly and demonstrate greater neural representational pattern stability across individual problems.
Finally, we examined whether individual differences in learning rates are associated with greater segregation of functional circuits associated with trained and novel problems. We reasoned that as a child acquires greater expertise, specific functional brain pathways would be preferentially strengthened ( Jolles et al., 2016 ). Thus, we hypothesized that faster learners would show greater differentiation between functional circuits and reconfiguration of large-scale brain networks engaged by trained and novel problems including the MTL and fronto-parietal cortical regions that support numerical problem solving in children ( Arsalidou et al., 2018 ; Cho et al., 2012 ; Menon, 2016 ). Together, this approach allowed us to uncover the neurocognitive mechanisms by which rapid learning facilitates enhanced near transfer in children.
2.1. Participants
A total of 29 children in 3rd and 4th grades (age: M = 9.49, SD = .46, 14 females) were recruited with flyers sent to schools and posted at libraries and community centers in the San Francisco Bay Area. All participants were right-handed and did not report any current neurological or psychiatric illness. All study protocols were approved by the Stanford University School of Medicine Institutional Review broad and informed consent was obtained from the parents of the children. Children received $50 for participating in the training program and $50 for completing the fMRI scanning session. Children had average to above average math abilities as reported by their parents and confirmed using the Mathematical Fluency subtest of the Woodcock Johnson Third Edition (standardized score: M = 107.50, SD = 12.96; percentile rank: M = 65.45, SD = 26.86; WJ-III, Woodcock et al., 2001 ).
fMRI data from 22 children (age: M = 9.46, SD = .48, 10 females) were investigated in the current study; data from 7 children were excluded due to (i) missing behavioral data recorded from the fMRI task (n = 3) or (ii) inadequate whole brain coverage or excessive head movement (see fMRI preprocessing section below) in the scanner (n = 4). Analysis of the influence of learning rate on brain activity and connectivity was based on data from 17 children (age: M = 9.42, SD = .53, 8 females) who had complete behavioral data on a computerized flash card task administered in each training session across the five training sessions as well as high-quality fMRI and in-scanner behavioral data. These sample sizes were determined to be adequate based on previous fMRI studies of learning in adults ( Delazer et al., 2003 , 2005 ). Critically, each child performed four runs of an event-related fMRI task (as described below) which significantly enhanced power to detect effects of interest within each individual, similar to fMRI designs used in visual neuroscience research ( Nee, 2018 ).
2.2. Training sets
There were two training sets, Group A and Group B (Supplementary Figure S5 shows all problems), counterbalanced across participants. Problems used as training set (presented across training sessions) in Group A (or Group B) was used as novel set (presented in fMRI task) in Group B (or Group A). The training (or novel) sets consisted of 14 single-digit plus double-digit problems. The stimuli were created by taking all the possible single-digit problems with operands from 2 to 9, excluding ties. This set contained 56 problems of which 28 had the smallest number first and 28 were the reverse problems with the largest digit first. We divided the 28 smallest digit first problems into two well-balanced sets of 14 such that the sum of each set equaled exactly 154. Specifically, each set had 6 ‘small’ problems with sums of 10 or less and 8 ‘large’ problems with sums of 11 or more. 1 In both sets, the sum of all the small problems was 49 and the sum of all the large problems was 105. The problems were randomly assigned to be involved in seven double-digit first or seven double-digit second problems, such that the total sum of either type of problems was 77. For each training set, the numbers 2–9 appear at least once in the single-digit spot. Decade values in the double-digit operands ranged from 20 – 80. These seven values were added to the first operand in the seven double-digit first problems and to the second operand in the seven double-digit second problems. The assignment of decades to single-digit problems had several constraints: (1) double-digit multiples of 11 were excluded; (2) amongst the set of 14 problems, at least one problem summed to a value in each of the decades from 20 to 90; (3) within the training sets, sums were separated by at least 3 units; (4) between the training sets all sums were unique.
We also created alternate novel sets that were used as novel problems in strategy assessments and unseen problems in recognition memory task (see below for task descriptions). These stimuli were created using the same single-digit problems as the A and B sets, but reversing the presentation order. Thus all 56 single-digit problems were used once. The same procedure was used to produce the double-digit operands, except that a few criteria were relaxed because there were no problems which met all of the criteria. Specifically, we allowed double-digit numbers to be multiples of 11, divisions of the stimuli into two groups had sums from 76 to 78 instead of 77 exactly and not all sums were unique between the alternate sets. Between the training sets and the alternate sets, all problems were unique (including reverses), but sums did repeat.
2.3. Training protocol
The overall training protocol is summarized in Fig. 1 . Children participated in five days of training with a tutor, after completing WJ-III Math Fluency sub-test, strategy assessment, and introductory lesson for addition problem solving.
Strategy assessment. Children completed a 28-item pre-training strategy assessment in which they were asked to solve the problems from the training set (either A or B, counterbalanced across participants) and an alternate novel set. After providing their answers, children were asked which method they used to solve the problem, including counting, decomposing the problem, or retrieving the answer from memory. Children’s responses and the category of the strategy used were recorded by trained assessors. Despite the inherent noise in this approach, this method has been shown to have good fidelity to expected response pattern across problem types ( Wu et al., 2008 ). Children solved the same problems in a different order before and after training. Half of the problems for strategy assessment consisted of problems that were later practiced during training (trained problems), and the other half were novel problems. Trained and novel problems were designed to be matched in structure and difficulty.
Introductory lesson. After the strategy assessment, all problems were drawn from the training set for that participant (A or B). To facilitate learning of complex arithmetic, children were introduced to a break-apart strategy that involved breaking down a double-digit number to a multiple of 10 plus single-digit numbers (e.g., 65 + 7 => 60 + 5 + 7 = 60 + 12 = 72). On a 14-problem worksheet, the tutor demonstrated this method on a problem not involving a carry and then asked the child to use it for the next non-carry problem. Then the tutor demonstrated the method on a carry problem and asked the child to solve two more problems using this method. Children then completed 8 problems using the method of their choice. For the final problem, they were instructed to use the break-apart rule.
2.4. Five-day training
Training consisted of the multiple activities across five days (see Supplementary Materials for details). Fig. 1 a lists the activities seen each day and the number of exposures to each problem in each activity. Tutoring gradually strengthened associations between problems and answers for trained problems through repeated practice (14 times per problem each day). For the first three days of tutoring, children practiced solving the addition problems using the break-apart method; in the last two days, children were asked to retrieve answers directly from memory whenever possible. Children accumulated stickers for completing each activity. Each tutoring session took less than one hour. Upon completion of all activities, children accumulated 20 stickers on a “treasure board” and selected a small plastic prize from a “treasure box.”
2.5. Learning rate
Each individual’s learning rate was computed by first regressing their efficiency score (ES: accuracy divided by mean reaction time for correct trials) on days of training in an exponential regression model ( y = a e b x ). Participants’ initial performance on computerized flash card task (intercept of learning curve) and their standardized score of WJ-III math fluency test were then regressed out from the slope of the learning curve. Higher scores indicated faster learning rates. Ranging from -1.72 (slowest learner) and 1.16 (fastest learner), this measure of learning rate controlled for basic arithmetic fluency and initial task performance.
2.6. fMRI task
Participants completed a delayed verification task in the scanner (Supplementary Fig. 1). Participants held a custom-made MR-compatible button box, in their right hand. Each trial began with a 500 ms fixation cross, followed by a double-digit plus single digit problem presented for 6 s. During the response phase, a possible sum was presented for up to 3 s. Participants were instructed to press the left button with their index finger if the potential solution was the correct answer or the right button with their middle finger if it was incorrect. Upon indicating their choice, a blank screen was presented until the full 10-second trial length was reached. Trials were followed by a jitter period ranging from 8 to 12 seconds.
In each run, 7 trained and 7 novel problems were drawn from the set of 14 trained problems that participants practice during training, and 14 novel problems (different from the set of novel problems used in the strategy assessment). There were 4 runs total, and participants saw each problem twice, once in the first two runs, and once in the second two runs. The problems were randomly assigned to appear in the first or second run. For the third and fourth runs, a new random distribution of the items was used. Each run lasted 4 min and 50 s, including 10 s at the beginning of each run to allow for scanner equilibration.
In each run, half the problems were presented with their correct sums and the other half were presented with incorrect sums. Incorrect sums were created by adding or subtracting 1, 2, or 10 from the correct sum 2 . In each run all possible incorrect sums were used once. One extra problem with either +1 or -1 was included to have seven incorrect problems. If the first run had an extra +1 trial, then the second run would have the -1 trial and vice versa. Problems with presented sums that differed by +10 and -10 from the correct answer were used to prevent participants from using only the ones digit to solve the problems. Within each run correct and incorrect problems were presented in a pseudo-random order where no more than 3 correct or incorrect problems appeared in a row.
2.7. Recognition memory task
After the fMRI task, participants performed a source memory test aimed at assessing their explicit knowledge of problems in the training set and problem seen in the scanner. Participants saw 42 problems in total, presented in a random order: 14 problems they practiced in tutoring (trained problems), 14 novel problems they saw in the scanner (novel problems) and 14 problems they had not seen before (unseen problems). For each problem participants were first asked: Did you see this problem in the scanner? ( seen in the scanner question ) and then asked: Did you practice this problem in tutoring? ( practiced in tutoring question ). For each problem type, children’s accuracy in identifying whether it was seen in the scanner and whether it was seen in tutoring was computed. Children’s discrimination sensitivity ( d’ : z (hit rate) – z (false alarm rate)) was measured using responses to problems recently seen and unseen for seen in the scanner question and those to trained and novel problems for practiced in tutoring question . For individuals with false alarm rates of 0, false alarm rate of 1 2 × n u m b e r o f l u r e s was used to reduce biased estimates.
2.8. fMRI data acquisition
Images were acquired on a 3 T GE Signa scanner (General Electric, Milwaukee, WI) using a custom-built head coil at the Stanford University Lucas Center. Head movement was minimized during the scan by cushions placed around the participant’s head. A total of 31 axial slices (4.0 mm thickness, 0.5 mm skip) parallel to the anterior commissure (AC)-posterior commissure (PC) line and covering the whole brain were imaged with a temporal resolution of 2 s using a T2* weighted gradient echo spiral in-out pulse sequence ( Glover and Lai, 1998 ) with the following parameters: TR =2 s, TE = 30 msec, flip angle = 80°, 1 interleave. The field of view was 22 cm, and the matrix size was 64 × 64, providing an in-plane spatial resolution of 3.4375 mm. To reduce blurring and signal loss from field inhomogeneity, an automated high-order shimming method based on spiral acquisitions was used before acquiring functional MRI scans ( Kim et al., 2002 )
2.9. fMRI preprocessing
Functional MRI data were analyzed using SPM8 ( http://www.fil.ion.ucl.ac.uk/spm/ ). The first 5 volumes were not analyzed to allow for T1 equilibration. A linear shim correction was applied separately for each slice during reconstruction ( Glover and Lai, 1998 ). Images were realigned to the first scan to correct for motion and slice acquisition timing. Following procedures similar to those used in AFNI 3dDespike ( Cox, 1996 ), deviant volumes resulting from spikes in movement greater than 0.5 voxels or spikes in the global signal greater than 5% were then interpolated using the two adjacent scans. All participants included in the analysis had less than 10% of volumes interpolated from spikes due to movement and less than 15% total volumes interpolated. Images were then spatially normalized to standard MNI space using the echo-planar imaging template provided with SPM8, resampled every 2 mm using trilinear sinc interpolation, and smoothed with a 6 mm full-width half-maximum Gaussian kernel to decrease spatial noise prior to statistical analysis.
2.10. fMRI statistical analyses
Task-related brain activation was identified using the general linear model (GLM) implemented in SPM8. In the individual subject analyses, interpolated volumes flagged at the preprocessing stage were de-weighted and did not contribute to calculating the model fit. Brain activity representing correct and incorrect trials for each trained and novel condition – a total of four conditions – was modeled using boxcar functions corresponding the 6 s where the problem was presented and convolved with a canonical hemodynamic response function and a temporal dispersion derivative to account for voxel-wise latency differences in hemodynamic response. Additionally, six movement parameters generated from the realignment procedure were included as regressors of no interest. Low-frequency drifts at each voxel were removed using a high-pass filter (.5 cycles/min). Serial correlations were accounted for by modeling the fMRI time series as a first-degree autoregressive process. Voxel-wise t-statistics maps contrasting correct trials for trained and novel problems were generated for each participant. Significant activation clusters, were identified using a height threshold of p < .01, with whole-brain family-wise error rate correction at p < .01 (cluster extent of 128 voxels) based on Monte Carlo simulations using custom Matlab scripts.
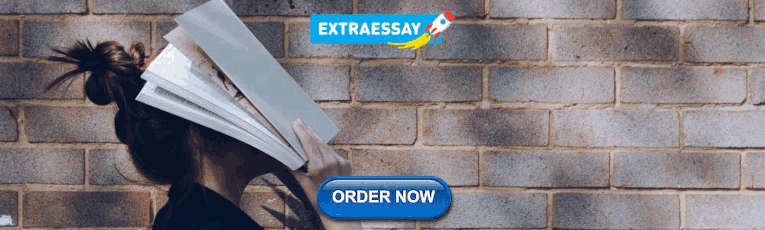
2.11. Multivoxel representational similarity analysis
To assess the neural representation shared across trained and novel problems, indicative of near transfer of knowledge, multivariate spatial correlation of patterns of brain activity between trained and novel problem solving was computed across the whole brain in each individual. Specifically, representational similarity across two conditions in the neighborhood surrounding each voxel of each individual’s brain was obtained using a searchlight mapping method ( Kriegeskorte et al., 2006 , 2008 ). First, a 6-mm spherical region centered on each voxel was selected. Next, similarity between trained and novel problems (we only included correct trials) was computed within this region using the spatial correlation between voxel-wise brain activation (beta-weights). Searchlight maps were then created for each individual by going through every voxel across the whole brain. Parametric correlation analyses were performed between similarity scores and the behavioral index of learning rate to examine whether the extent of similarity in the patterns of neural activity between trained and novel items relate to individual differences in learning. Significant clusters were determined using the same threshold criterion noted above.
2.12. Multivoxel pattern stability analysis
A trial-by-trial pattern stability analysis was implemented to obtain a measure of inter-problem pattern stability ( Qin et al., 2014 ), separately for trained and novel problems, in the neighborhood surrounding each voxel in each participant's brain. Inter-problem neural pattern stability scores only for correctly solved problems were computed using a pairwise correlation method in a 6-mm spherical region centered on each voxel. Pairwise correlation maps of two consecutive neighbor trials were excluded in order to mitigate potential collinearity because of close proximity in time. The averaged similarity scores were assigned to the central voxel and repeated across all brain voxels to create participant-specific similarity maps. These searchlight maps were subsequently entered into a second-level random effects analysis to determine variations in pattern stability in each participant. Significant clusters were determined using the same threshold criterion noted above.
2.13. Functional connectivity analysis
A seed-based generalized psychophysiological interaction (gPPI) analysis ( McLaren et al., 2012 ) was performed to examine functional connectivity of the right MTL seed with the rest of the brain during trained, compared to novel, problem solving. The seed ROI was defined as a 5-mm sphere centered at the peak of the right MTL cluster where a significant association between similarity across trained and novel items and learning was observed. Functional connectivity was modeled using a standard GLM at the individual subject level. To control for task-related activation, physiological noise, and other confounding factors, (i) the four task conditions (correct and incorrect trained and novel problems; psychological variable), (ii) deconvolved average time series from the seed ROI (physiological variable), and (iii) 6 motion parameters were included as regressors of no interest. Psychophysiological regressors of interest were defined as a product of the first two regressors (psychological and physiological variables) convolved with a canonical HRF. Contrast images corresponding to trained, compared to novel, condition were entered into a group level analysis. Learning rate was included as a regressor of interest to determine whether the functional connectivity of the seed ROI with the rest of the brain is associated with individual differences in learning. Significant clusters were determined using the same threshold criterion noted above.
2.14. Network analysis
Network analysis was performed to investigate discriminability of large-scale brain network connectivity patterns between trained and novel problems, and its relation to individual learning rates. Network nodes were defined using 5-mm spheres centered at regional peaks in brain activation for correctly solved trained and novel problems. For each participant and for each task condition (trained and novel), a 23 × 22 connectivity matrix, excluding the diagonal elements, was created using gPPI. To ensure normality, connectivity values across the two task conditions were first z-transformed. We then used a linear SVM classifier (LIBSVM: https://www.csie.ntu.edu.tw/∼cjlin/libsvm/) and leave-one-out cross validation to determine discriminability of functional connectivity patterns between trained and novel problems. For each participant we then computed the sum of the distance from the hyperplane to trained and novel problems to obtain network connectivity pattern distance between trained and novel problems and examined its relation to individual differences in learning rate, using a similar procedure to build the hyperplane separating two conditions as Uddin et al. (2011) .
3.1. Training improves behavioral performance and increases children’s use of retrieval strategy, with some children learning faster than others
We examined training-induced improvements in behavioral performance using multiple quantitative assays.
Assessment of learning over 5 days of training . Computerized flash cards were used to examine learning. To control for variations in speed-accuracy tradeoff and to lower the probability of type I errors by reducing the number of statistical tests required, we computed a composite efficiency score (ES; Townsend and Ashby, 1978 ), obtained by dividing accuracy by mean reaction time, for each child on each day. Over the course of training, participants improved significantly on trained problems from day 1 (ES: M = 0.14, SD = 0.05) to day 5 (ES: M = 0.23, SD = 0.08; F (4, 64) = 39.69, p < .001). Next, we fitted daily measures of the ES in an exponential regression model ( y = a e b x ) to determine each child’s learning rate b . Learning curves were well fit by the exponential regression ( y = 0.125 e 0.133 x , adjusted R 2 = 0.99, p < 0.001), with large individual differences in learning rates (coefficient of variation = 0.34; Fig. 3 a).

Behavioral changes with training. (a) Individual learning profiles assessed by efficiency score (ES) for trained problems in the computerized flash card task across five days of training. (b) ES for trained and novel problems after training in the fMRI task. (c) Increase in use of memory-based retrieval strategies for trained and novel problems, measured from strategy assessments before and after training. Error bars represent standard error of the mean.
fMRI task performance on trained and novel problems. After training, participants performed significantly better on trained (ES: M = 0.72, SD = 0.17), compared to novel (ES: M = 0.65, SD = 0.14), problems ( t (21) = 4.2, p < 0.001; Fig. 3 b).
Strategies used for solving trained and novel problems . We examined children’s strategies for problem solving before and after training, including direct memory-based retrieval, counting, and other procedural strategies ( Wu et al., 2008 ). Training increased children’s use of direct memory-based retrieval strategies for both trained (22%–71%) and novel (19%–43%) problems. Increases in memory-based retrieval strategies were greater for trained ( M = 0.50, SD = 0.36), compared to novel ( M = 0.24, SD = 0.30) problems ( t (20) = 3.50, p = 0.002; Fig. 3 c).
These results demonstrate that five days of training results in significant performance gains and increased use of memory-based retrieval strategies for trained problems.
3.2. Faster learners show greater transfer to novel problems
We examined how individual differences in learning rate during training influences performance on trained and novel problems. Learning rates predicted performance on both trained ( r = 0.57, p = 0.018; Fig. 4 a) and novel ( r = 0.62, p = 0.008; Fig. 4 b) problems performed during fMRI scanning. The correlation between learning rate and performance did not differ between trained and novel problems ( z = -0.53, p = 0.60). Because we controlled for arithmetic problem-solving abilities prior to training, these effects are driven by differences in training-induced learning rather than pre-existing differences. Learning rate was not significantly associated with pre-training math fluency or retrieval strategy use ( r s ≤ 0.25, p s ≥ 0.33), which further rules out the possibility that faster learners were better at arithmetic or used more retrieval strategy before training. These results suggest that faster learners perform better not only on problems they learned during training but were also able to transfer their skills to novel but structurally similar problems.

Faster learners show enhanced performance on trained and novel problems and better discrimination between trained and novel problems. (a–b) Learning rates across a 5-day training predicted greater efficiency in solving trained and novel problems in the fMRI task. (c) Learning rate predicted discrimination sensitivity ( d’ ) between trained and novel problems during a recognition memory task in which children were asked to determine whether they had practiced specific problems during training. Individual learning rates were computed as described in Fig. 2 and Methods. d’ : z (hit rate) – z (false alarm rate). Dashed lines represent 95% confidence interval.
3.3. Faster learners show better discrimination between trained and novel problems
We then investigated whether individual learning rates during training are associated with children’s ability to discriminate between trained and novel problems. Following the fMRI scan, we presented children with the same trained and novel problems that they had just solved during the fMRI scan, along with novel lures they had not seen in either the training sessions or during fMRI scanning. Children were asked to determine whether they practiced the presented problem during the 5 days of tutoring. We found that learning rates were positively correlated with discrimination sensitivity ( d’ ) between trained and novel problems ( r = 0.60, p = 0.011; Fig. 4 c). Children were also asked to determine whether the problem had been presented during fMRI scanning itself. In this case, learning rates were not significantly correlated with d’ between recently seen (trained and novel) and unseen problems ( r = 0.18, p = 0.50). These results indicate that faster learners do not simply have better recognition memory overall, as they were not better than slower learners at distinguishing between trials seen and unseen during fMRI scanning. Rather, faster learners had better recollection of which specific problems they had practiced during the five days of training.
3.4. Training-induced differences between trained and novel problems in the MTL and neocortical systems in children
To identify overall training-related differences between problems in neural activity, we contrasted brain responses between correctly solved trained and novel problems. We found that trained problems elicited significantly greater activity in the anterior and medial hippocampus (HIP), parahippocampal gyrus (PHG), the middle temporal gyrus, and the angular gyrus bilaterally, as well as the left medial prefrontal cortex and the right posterior cingulate cortex. We also observed significantly reduced activity for trained problems in the inferior frontal gyrus, intraparietal sulcus, supramarginal gyrus, supplementary motor area, middle occipital gyrus, cerebellum, and the right caudate ( Fig. 5 ; Supplementary Table S1). These results indicate that five days of training decreases engagement of fronto-parietal systems and increases involvement of the MTL and angular gyrus on trained, compared to novel, problems.

Brain areas showing differences in activation for trained versus novel problems. (a) Trained problems elicited greater activity than novel problems (shown in red-yellow) in the hippocampus (HIP), parahippocampal gyrus (PHG), angular gyrus (AG), middle temporal gyrus (MTG), medial prefrontal cortex (mPFC) and posterior cingulate cortex (PCC). Novel problems elicited greater activity (shown in blue-green) in the inferior frontal gyrus (IFG), insula, middle and superior frontal gyri, intraparietal sulcus (IPS), supramarginal gyrus (SMG), supplementary motor area (SMA), middle occipital gyrus (MOG), cerebellum (CBL), and the caudate (CAU). (b) Medial temporal lobe (MTL) activation clusters (shown in orange) overlaid on anterior (a) and medial (m) HIP (cyan) and PHG (green) anatomical boundaries from the AAL atlas. L: left; R: right.
3.5. Faster learners show greater representational similarity between trained and novel problems in the MTL and neocortex
To investigate how learning influences shared neural representations across trained and novel problems, we conducted an RSA between the two types of problems across the whole brain and then examined its relation to individual learning rates. We found that learning rates predicted representational similarity in the right MTL (both HIP and PHG) with local maximum at MNI coordinates x = 38, y = -10, z = -28 ( r = 0.59, p = 0.012; Fig. 6 ), and in multiple temporal and frontal cortical regions (Supplementary Figure S2; Supplementary Table S2). These relations were specific to multivoxel representations, as activation levels in these regions were not correlated with learning rate (Supplementary Figure S3). These results indicate that faster learners show a greater degree of shared neural representations between trained and novel problems, reflecting a key mechanism underlying near transfer.

Faster learners show increased representational similarity between trained and novel problems in the MTL. (a) Learning rate predicts multivoxel representational similarity between trained and novel problems in the R MTL region of interest (ROI, black circle). The ROI was defined using a 5-mm sphere centered at MTL cluster peak with a significant association between learning rate and representational similarity across trained and novel problems [MNI coordinates: 38 -10 -28]. (b) Learning rate predicted representational similarity across trained and novel problems in R MTL region encompassing R HIP (white) and R PHG (orange). (c) R MTL ROI overlaid on R HIP activation for trained, compared to novel, problems (purple). (d–f) Scatter plots of the relationship between learning rate and representational similarity between trained and novel problems in R MTL ROI (d) as well as R MTL region that overlapped with R HIP (e) and R PHG (f). Anatomical boundaries from the AAL atlas are shown for visualization of brain structures. Gray shaded area represents 95% confidence interval. Abbreviations as in Fig. 5 .
3.6. Faster learners show greater stability of representational patterns between individual novel problems in the MTL and IPS
To investigate how learning rate influences neural responses to individual problems, we conducted analysis on a trial-by-trial stability of multivariate representational patterns, known to be associated with cognitive skill development ( Qin et al., 2014 ), separately for trained and novel problems. We found that multivoxel representational similarity across individual novel trials in the right MTL (HIP) and the right intraparietal sulcus was positively correlated with learning rate (Supplementary Figure S4, Supplementary Table S4). This effect was specific to novel problems, as no brain region showed significant relationship between learning rate and trial-by-trial pattern stability for trained problems. These results suggest that faster learners show enhanced neural pattern stability when solving novel problems, highlighting another mechanism underlying stable near transfer.
3.7. Faster learning is associated with segregation of MTL circuitry between trained and novel problems
We next investigated the relation between learning rate and training-related differences between trained and novel problems in functional circuits, focusing on the right MTL region (MNI coordinates: x = 38, y = -10, z = -28) where faster learners showed greater neural representation similarity ( Fig. 6 , Supplementary Table S2). Seed-based generalized psychophysiological interaction (gPPI; McLaren et al., 2012 ) analysis revealed that faster learners demonstrate reduced functional connectivity between the right MTL and multiple brain regions including the HIP, bilateral supramarginal gyrus, middle temporal gyrus, left angular gyrus, and right supplementary area for trained, compared to novel, problems ( Fig. 7 , Supplementary Table S3). These results suggest that faster learners demonstrate greater differentiation of MTL circuits engaged by trained and novel problems.

Faster learners show greater differentiation of MTL functional connectivity between trained and novel problems. (a) Generalized psychophysiological interaction (gPPI) analysis revealed reduced functional connectivity between the right MTL and multiple fronto-parietal and temporal lobe regions for trained, compared to novel, problems in faster learners. MTL seed region was defined as described in Fig. 6 . (b) Scatter plots show relationship between learning rate and MTL connectivity for trained, compared to novel, problems. Dashed lines represent 95% confidence interval. Abbreviations as in Fig. 5 .
3.8. Faster learning is associated with greater network distance between trained and novel problems
Finally, we examined how short-term training alters functional network configuration to support learning gains. We used 23 regions of interest (ROIs) that showed task-related activity ( Fig. 8 a) as nodes in gPPI analysis to determine connectivity between 23 × 22 links of this network for trained and novel problems ( Fig. 8 b-c). To determine how learning induces different multivariate connectivity patterns, a linear support vector machine (SVM) classifier was performed, using links that showed significant differences in connectivity between trained and novel problems as input features ( Fig. 8 d). A network connectivity pattern distance between trained and novel problems was then computed using the sum of the distance from the hyperplane to trained and novel problems. We found that this multivariate measure of distance in connectivity patterns between trained and novel problems was significantly correlated with learning rate ( r = 0.52, p = 0.032; Fig. 8 e). These results indicate that faster learners demonstrate greater segregation of network configuration between trained and novel problems.

Faster learners show greater differentiation in large-scale brain network configuration between trained and novel problems. (a) Task-related brain activation was used to identify 23 network nodes. (b–c) Task-related effective connectivity was used to compute a network connectivity matrix for trained (b) and novel (c) problems. (d) 23 links showed significant differences in connectivity between trained and novel problems ( p < .05, in yellow). These links were used as input features in a linear SVM classifier. Leave one out cross validation and permutation tests (1000 samples) revealed a classification accuracy of 70% ( p = 0.01). (e) Learning rate predicted greater differentiation of brain networks between trained and novel problems. Connectivity pattern distance was computed using sum of absolute distance from the SVM hyperplane (separating connectivity between trained and novel problems) to each condition. Dashed lines represent 95% confidence interval. Abbreviations as in Fig. 5 .
4. Discussion
The current study investigated neurocognitive mechanisms underlying learning and near transfer of problem-solving skills in children at ages 8–10, a developmental stage crucial for knowledge acquisition. A five-day math tutoring protocol was sufficient to elicit significant learning of arithmetic facts, characterized by marked differentiation of behavioral and brain responses between trained and novel problems. Crucially, individual differences in learning rate obtained during the five-day training predicted near transfer of learning, with faster learners showing greater performance gains on novel, but similarly structured, problems. Faster learners showed greater overlap in neural representations within MTL regions implicated in memory formation, as well as greater segregation of large-scale brain circuits between trained and novel problems, indicating emergence of more distinct specialized functional circuitry with learning. Taken together, our study provides new insights into the neurocognitive mechanisms underlying learning and near transfer in a domain critical for academic and professional success ( Geary et al., 2017 ; Menon, 2016 ).
4.1. Faster learning during short-term training predicts near transfer to novel problems
Following five days of training, all children exhibited significant improvements on trained problems. Compared to novel problems, children completed trained problems more efficiently with an overall 11% greater performance efficiency. Children also showed 26% greater increase in use of sophisticated memory-based retrieval strategies for trained, relative to novel, problems. These findings demonstrate that one-week training was effective in improving task efficiency, with greater use of memory-based strategies for trained, compared to novel, problems. The finding that children also showed an increase in retrieval strategy for novel problems after tutoring indicates that children were able to transfer their strategy use to structurally similar problems that were not explicitly trained on.
Crucially, children who were faster learners showed greater transfer to novel problems. Previous behavioral research has shown that children learn single-digit math facts by progressing from frequent use of counting, through intermediate strategies, until they are eventually able to directly retrieve the answer from long-term memory ( Barrouillet and Fayol, 1998 ). However, there is a large variation between individuals in this progression as children use a mix of different strategies across different problems and contexts ( Geary et al., 2004 ; Imbo and Vandierendonck, 2007 ; Siegler and Shipley, 1995 ). In line with these developmental studies, we uncovered an important source of individual variability with respect to both learning of trained problems and near transfer of learning. Specifically, faster learning rates predicted better performance on both trained and novel problems. Learning rates also predicted better discrimination between trained and novel problems as assessed by a subsequent recognition memory task. Notably, these effects were not dependent on problem solving abilities prior to training, ruling out the possibility that faster learners are better at problem solving or mathematics in general.
Together, these behavioral results demonstrate that five days of training are sufficient to achieve significant learning of trained problems and that transfer of learning to similar but novel problems is dependent on how well a child learns. More broadly, these findings suggest that training can induce learning and near transfer – children who learn more quickly are better able to apply such knowledge and skills to novel but structurally similar problems.
4.2. Faster learners show greater similarity in neural responses between trained and novel problems
To identify neural mechanisms underlying learning and near transfer to novel problems in children, we used RSA and determined the degree of overlap in the spatial patterns of brain activity between trained and novel problems. We found that faster learners showed greater similarity of neural representations between trained and novel problems in the MTL including the right anterior hippocampus and parahippocampal gyrus, suggesting that the MTL facilitates near transfer of learning in children. The role of the MTL in learning and near transfer to structurally similar problems is consistent with a large body of evidence from the memory literature suggesting that the hippocampus, and in particular its anterior aspects, is involved in generalizable representations of recently learnt items ( Bowman and Zeithamova, 2018 ; Collin et al., 2015 ; Gerraty et al., 2014 ; Komorowski et al., 2013 ; Schlichting et al., 2015 ; Shohamy and Wagner, 2008 ; Tompary and Davachi, 2017 ). In addition to the MTL, faster learners showed greater representational similarity in the superior frontal gyrus, supplementary motor area, precentral gyrus, superior, middle, and inferior temporal gyri, middle occipital gyrus, and thalamus/brainstem, indicating that they also rely on multiple cortical systems to transfer their learning.
Next, to examine whether faster learners also show more stable representations across individual trained or novel problems, we used trial-by-trial similarity analysis, which assesses similarity of neural representations across individual problems ( Qin et al., 2014 ). This approach previously revealed that inter-problem pattern stability in the MTL increases from childhood to adulthood as individuals’ problem solving strategies and underlying neurocognitive processes become more stable with cognitive skill development ( Qin et al., 2014 ). We discovered that faster learners showed greater pattern stability across individual problems, specifically for novel problems, in the right hippocampus and right intraparietal sulcus. This finding suggests that children who learn trained problems more quickly may be able to scaffold knowledge from training sessions and apply it more consistently to novel problems, compared to those who learn more slowly. In contrast, for trained problems, all children show similar inter-trial pattern stability possibly due to item-specific representations of well-practiced instances. Interestingly, within parietal cortex, the enhanced pattern stability for novel problems were observed not in the angular gyrus, a region involved in integrating general contextual information ( Ramanan et al., 2017 ), but in the right intraparietal sulcus, a region important for representing and manipulating numerical quantity ( Cantlon, 2012 ; Menon, 2016 ; Nieder, 2016 ). As previous arithmetic training studies have not examined neural representations and their relation to individual differences in learning rate, changes in learning-rate-dependent neural representations across typical and atypical development ( Kucian, 2016 ) remains an important topic for future research. Our findings demonstrate for the first time that children who are faster learners rely on both MTL for associative learning and IPS for quantity processing to build generalizable problem-solving skills.
Although the hippocampus and parahippocampal regions of the MTL showed greater task-related activation for trained, relative to novel, problems, activation levels in these regions were not correlated with learning rate, suggesting that common neural representations underlying near transfer of learning are independent of overall engagement of the MTL. This finding, along with behavioral evidence of enhanced performance on novel problems, supports the hypothesis that children, who learn quickly during tutoring, are more likely to transfer their knowledge to novel problems - through the engagement of overlapping neural representations in the MTL and other cortical regions. Faster learning is also linked to pattern stability in the MTL across similar problems, creating a substrate that facilitates near transfer, a process that may promote stability in the MTL over development, even as task-related activation in MTL wanes ( Qin et al., 2014 ). These findings suggest that the MTL plays a crucial role in building common representations that facilitate near transfer of newly learnt skills in children.
4.3. Faster learners show more segregated brain circuits and network configuration between trained and novel problems
Beyond training-related differences between problems in isolated brain regions, we found that faster learners show more segregated brain circuits and network configuration between trained and novel problems. Network analysis revealed that faster learning children exhibit more segregated task-related brain network configurations between trained and novel problems. Specifically, faster learners showed more differentiated connectivity patterns within a large-scale brain network comprised of 23 core regions involved in arithmetic problem solving, including the MTL and fronto-parietal cortical regions. To link these findings to the MTL region that plays a key role in learning and near transfer, as identified by representational similarity analysis, we examined its task-related functional connectivity. Analysis of functional brain circuits revealed that faster learners showed lower MTL connectivity, for trained, compared to novel, problems in multiple brain regions, including bilateral hippocampus, supramarginal gyrus, middle temporal gyrus, left angular gyrus, and right supplementary motor area. Thus, MTL regions involved in learning and near transfer at the local circuit level also show functional segregation at the large-scale circuit level.
Our findings provide new evidence for greater segregation of hippocampal functional circuits and large-scale network configuration in faster learners. We suggest that increased neural efficiency for trained problems among faster learners may free up neural resources and facilitate near transfer and that children who are slower to learn, although they are able to learn specific problems that they are trained on, may struggle with near transfer. Furthermore, in an advance over previous studies on learning, our findings provide new evidence linking two parallel processes – integration of shared neural representations and segregation of large-scale brain systems anchored in the MTL – with efficient learning and near transfer in children. While these two aspects of learning are distinguishable, they may not be mutually exclusive, as observed in the current study. Item-specific learning can be represented in a larger scale in distributed brain regions, whereas more general, near transfer process may occur at the local neural representational level.
4.4. Broader implications, limitations, and future directions
Previous behavioral studies have shown that young children’s basic arithmetic skills and their memory-based strategy use predict their later mathematics achievement ( Butterworth and Walsh, 2011 ; Faulkner, 2008 ; Geary, 2011 ; Geary et al., 2017 ; Jordan et al., 2009 ). Our study advances understanding of how children learn arithmetic problems and provides insights into developing effective arithmetic training paradigms by enhancing retrieval strategy use in young children. Moreover, unlike other cognitive training studies that typically examine group-level differences between trained and novel problems, we demonstrate that individual differences in learning is a critical factor mediating near transfer. Our study provides a template for future studies of learning and transfer in children with learning disabilities. As the current work is specific to near transfer in the mathematics domain, future studies may also benefit from examining a broader range of learning and transfer across various domains and skills, using a similar individual differences approach used in the current study.
One limitation of the current study is that the sample size included in the analysis was modest. Limitations in sample size are common in neuroimaging studies of children and represent a major challenge in cognitive training studies where children’s learning profiles and fMRI data are considerably more difficult to acquire compared to studies that do not involve learning. Future studies using a larger sample are needed to address generalizability of our findings by extending the study to children at the low end of math abilities, including children with learning disabilities. Another limitation is that we acquired fMRI data after, but not before, training. This experimental design, similar to previous studies in adults ( Delazer et al., 2003 , 2005 ; Grabner et al., 2009 ; Ischebeck et al., 2006 ), allowed us to examine training-related differences between different types of problems that were carefully matched in structure and difficulty. In the current study, there were no significant associations between pre-training math fluency and learning rate or pre-training frequency of retrieval strategy use and learning rate, ruling out the possibility that faster learners were better at arithmetic or used more retrieval strategy before training. A pre-test post-test fMRI task design with a control group, using an individual differences approach, as developed here, is needed for further clarifying the specificity of training-induced brain plasticity. Such designs will also help resolve whether neural responses differ significantly in amplitude and localization of activation between before and after training, and allow disambiguation of activation and deactivation profiles in the MTL and angular gyrus ( Bloechle et al., 2016 ; Klein et al., 2019).
5. Conclusion
Our study demonstrates that two key components of efficient learning in children are related: (1) the speed of learning and (2) the “depth” of learning reflected in near transfer. We show for the first time that rapid learning facilitates near transfer and that cognitive training in young children induces two distinct brain processes that support learning and near transfer: overlap in neural representations at the level of regional circuitry that facilitates near transfer and segregation of large-scale brain networks that promotes more efficient processing of trained problems. Notably, these patterns of results are learning-rate dependent: faster learners draw on common representations in multiple brain areas to allow better performance of similar problems, while efficiently recruiting specialized brain networks for problems they have learned. These findings advance our understanding of how learning and near transfer are represented in the developing brain in a cognitive domain critical for academic success.
Declaration of Competing Interest
Acknowledgments.
This research was supported by the United States National Institutes of Health to V.M. (HD059205, MH084164, HD094623), M.R.-L. (MH101394), and S.Q. (MH105601), and by the Stanford Maternal & Child Health Research Institute Postdoctoral Support Award to H.C. We thank Emily Escovar, Amirah Khouzam, and Caitlin Tenison for assistance with the study and Drs. Daniel Abrams, Aarthi Padmanabhan, Flora Schwartz, and Kaustubh Supekar for helpful feedback on the manuscript.
1 It should be noted that carry operation was not manipulated within each problem size category: a ‘small’ problem that sums to 10 and all ‘large’ problems involve carry operations, while ‘small’ problems that sum to less than 10 do not require carry operations. Nonetheless, these problems were carefully distributed across two problem sets (trained and novel) to be matched in difficulty and counterbalanced across participants to allow comparisons between trained and novel problems. Future studies may benefit from an experimental design that manipulates both problem size and carry operations to understand whether individuals learn these problems differently.
2 Considering the possibility that the difficulty of arithmetic problems may vary depending on the distance between the correct answer and presented solution, we have carefully matched the distribution of different distances between trained and novel problems in order to minimize interaction with such distance effect. In fact, we found a significant main effect of problem type (trained, novel), F (1, 21) = 9.91, p = 0.0049, and a significant main effect of absolute distance between correct answer and presented solution (0,1,2,10), F (3, 63) = 31.27, p < .001, but did not observe a significant interaction between problem type and distance, F (3, 63) = 0.95, p = 0.42. Thus, while participants’ performance varied across problems with different distances between correct answer and presented solution, these variations were not significantly different between trained and novel problems.
Appendix A Supplementary material related to this article can be found, in the online version, at doi: https://doi.org/10.1016/j.dcn.2019.100719 .
Appendix A. Supplementary data
The following is Supplementary data to this article:
- Anderson J.R., Lee H.S., Fincham J.M. Discovering the structure of mathematical problem solving. NeuroImage. 2014; 97 :163–177. [ PubMed ] [ Google Scholar ]
- Arsalidou M., Pawliw-Levac M., Sadeghi M., Pascual-Leone J. Brain areas associated with numbers and calculations in children: meta-analyses of fMRI studies. Dev. Cogn. Neurosci. 2018; 30 :239–250. [ PMC free article ] [ PubMed ] [ Google Scholar ]
- Barrouillet P., Fayol M. From algorithmic computing to direct retrieval: evidence from number and alphabetic arithmetic in children and adults. Mem. Cognit. 1998; 26 (2):355–368. [ PubMed ] [ Google Scholar ]
- Bassett D.S., Yang M., Wymbs N.F., Grafton S.T. Learning-induced autonomy of sensorimotor systems. Nat. Neurosci. 2015; 18 (5):744–751. [ PMC free article ] [ PubMed ] [ Google Scholar ]
- Bloechle J., Huber S., Bahnmueller J., Rennig J., Willmes K., Cavdaroglu S. Fact learning in complex arithmetic—the role of the angular gyrus revisited. Hum. Brain Mapp. 2016; 37 (9):3061–3079. [ PMC free article ] [ PubMed ] [ Google Scholar ]
- Bowman C.R., Zeithamova D. Abstract memory representations in the ventromedial prefrontal cortex and Hippocampus support concept generalization. J. Neurosci. 2018; 38 (10):2605–2614. [ PMC free article ] [ PubMed ] [ Google Scholar ]
- Butterworth B., Walsh V. Neural basis of mathematical cognition. Curr. Biol. 2011; 21 :R618–621. [ PubMed ] [ Google Scholar ]
- Cantlon J.F. Math, monkeys, and the developing brain. Proc. Natl. Acad. Sci. 2012; 109 :10725–10732. [ PMC free article ] [ PubMed ] [ Google Scholar ]
- Cho S., Metcalfe A.W.S., Young C.B., Ryali S., Geary D.C., Menon V. Hippocampal–Prefrontal engagement and dynamic causal interactions in the maturation of children’s fact retrieval. J. Cogn. Neurosci. 2012; 24 (9):1849–1866. [ PMC free article ] [ PubMed ] [ Google Scholar ]
- Cho S., Ryali S., Geary D.C., Menon V. How does a child solve 7 + 8? Decoding brain activity patterns associated with counting and retrieval strategies. Dev. Sci. 2011; 5 :989–1001. [ PMC free article ] [ PubMed ] [ Google Scholar ]
- Collin S.H.P., Milivojevic B., Doeller C.F. Memory hierarchies map onto the hippocampal long axis in humans. Nat. Neurosci. 2015; 18 (11):1562–1564. [ PMC free article ] [ PubMed ] [ Google Scholar ]
- Cox R.W. AFNI: software for analysis and visualization of functional magnetic resonance neuroimages. Comput. Biomed. Res. 1996; 29 (3):162–173. [ PubMed ] [ Google Scholar ]
- Dahlin E., Neely A.S., Larsson A., Bäckman L., Nyberg L. Transfer of learning after updating training mediated by the striatum. Science. 2008; 320 (5882):1510–1512. [ PubMed ] [ Google Scholar ]
- Delazer M., Domahs F., Bartha L., Brenneis C., Lochy A., Trieb T., Benke T. Learning complex arithmetic—an fMRI study. Cogn. Brain Res. 2003; 18 (1):76–88. [ PubMed ] [ Google Scholar ]
- Delazer M., Ischebeck A., Domahs F., Zamarian L., Koppelstaetter F., Siedentopf C.M. Learning by strategies and learning by drill—evidence from an fMRI study. NeuroImage. 2005; 25 (3):838–849. [ PubMed ] [ Google Scholar ]
- Faulkner L.W. 2008. Foundations for Success: The Final Report of the National Mathematics Advisory Panel. https://www2.ed.gov/about/bdscomm/list/mathpanel/report/final-report.pdf [ Google Scholar ]
- Geary D.C. Cognitive predictors of achievement growth in mathematics: a 5-year longitudinal study. Dev. Psychol. 2011; 47 (6):1539–1552. [ PMC free article ] [ PubMed ] [ Google Scholar ]
- Geary D.C., Berch D.B., Ochsendorf R., Koepke K.M. Academic Press; 2017. Acquisition of Complex Arithmetic Skills and Higher-Order Mathematics Concepts. [ Google Scholar ]
- Geary D.C., Hoard M.K., Byrd-Craven J., Catherine DeSoto M. Strategy choices in simple and complex addition: contributions of working memory and counting knowledge for children with mathematical disability. J. Exp. Child Psychol. 2004; 88 (2):121–151. [ PubMed ] [ Google Scholar ]
- Gerraty R.T., Davidow J.Y., Wimmer G.E., Kahn I., Shohamy D. Transfer of learning relates to intrinsic connectivity between Hippocampus, ventromedial prefrontal cortex, and large-scale networks. J. Neurosci. 2014; 34 (34):11297–11303. [ PMC free article ] [ PubMed ] [ Google Scholar ]
- Glover G.H., Lai S. Self-navigated spiral fMRI: interleaved versus single-shot. Magn. Reson. Med. 1998; 39 (3):361–368. [ PubMed ] [ Google Scholar ]
- Grabner R.H., Ischebeck A., Reishofer G., Koschutnig K., Delazer M., Ebner F., Neuper C. Fact learning in complex arithmetic and figural-spatial tasks: the role of the angular gyrus and its relation to mathematical competence. Hum. Brain Mapp. 2009; 30 (9):2936–2952. [ PMC free article ] [ PubMed ] [ Google Scholar ]
- Haxby J.V., Connolly A.C., Guntupalli J.S. Decoding neural representational spaces using multivariate pattern analysis. Annu. Rev. Neurosci. 2014; 37 (1):435–456. [ PubMed ] [ Google Scholar ]
- Imbo I., Vandierendonck A. The development of strategy use in elementary school children: working memory and individual differences. J. Exp. Child Psychol. 2007; 96 (4):284–309. [ PubMed ] [ Google Scholar ]
- Ischebeck A., Zamarian L., Siedentopf C., Koppelstätter F., Benke T., Felber S., Delazer M. How specifically do we learn? Imaging the learning of multiplication and subtraction. NeuroImage. 2006; 30 (4):1365–1375. [ PubMed ] [ Google Scholar ]
- Iuculano T., Rosenberg-Lee M., Richardson J., Tenison C., Fuchs L., Supekar K., Menon V. Cognitive tutoring induces widespread neuroplasticity and remediates brain function in children with mathematical learning disabilities. Nat. Commun. 2015; 6 :8453. [ PMC free article ] [ PubMed ] [ Google Scholar ]
- Jaeggi S.M., Buschkuehl M., Jonides J., Shah P. ). Short- and long-term benefits of cognitive training. Proc. Natl. Acad. Sci. 2011; 108 (25):10081–10086. [ PMC free article ] [ PubMed ] [ Google Scholar ]
- Jaeggi S.M., Buschkuehl M., Shah P., Jonides J. The role of individual differences in cognitive training and transfer. Mem. Cognit. 2014; 42 (3):464–480. [ PubMed ] [ Google Scholar ]
- Jolles D., Ashkenazi S., Kochalka J., Evans T., Richardson J., Rosenberg-Lee M. Parietal hyper-connectivity, aberrant brain organization, and circuit-based biomarkers in children with mathematical disabilities. Dev. Sci. 2016 [ PMC free article ] [ PubMed ] [ Google Scholar ]
- Jolles D.D., Buchem M.Avan, Crone E.A., Rombouts S.A.R.B. Functional brain connectivity at rest changes after working memory training. Hum. Brain Mapp. 2013; 34 (2):396–406. [ PMC free article ] [ PubMed ] [ Google Scholar ]
- Jordan N.C., Kaplan D., Ramineni C., Locuniak M.N. Early math matters: kindergarten number competence and later mathematics outcomes. Dev. Psychol. 2009; 45 (3):850–867. [ PMC free article ] [ PubMed ] [ Google Scholar ]
- Kim D.-H., Adalsteinsson E., Glover G.H., Spielman D.M. Regularized higher-order in vivo shimming. Magn. Reson. Med. 2002; 48 (4):715–722. [ PubMed ] [ Google Scholar ]
- Klein E., Willmes K., Bieck S.M., Bloechle J., Moeller K. White matter neuro-plasticity in mental arithmetic: changes in hippocampal connectivity following arithmetic drill training. Cortex. 2018 [ PubMed ] [ Google Scholar ]
- Komorowski R.W., Garcia C.G., Wilson A., Hattori S., Howard M.W., Eichenbaum H. Ventral hippocampal neurons are shaped by experience to represent behaviorally relevant contexts. J. Neurosci. 2013; 33 (18):8079–8087. [ PMC free article ] [ PubMed ] [ Google Scholar ]
- Kriegeskorte N., Goebel R., Bandettini P. Information-based functional brain mapping. Proc. Natl. Acad. Sci. 2006; 103 (10):3863–3868. [ PMC free article ] [ PubMed ] [ Google Scholar ]
- Kriegeskorte N., Mur M., Bandettini P.A. Representational similarity analysis - connecting the branches of systems neuroscience. Front. Syst. Neurosci. 2008; 2 [ PMC free article ] [ PubMed ] [ Google Scholar ]
- Kucian K. Development of Mathematical Cognition. Elsevier; 2016. Developmental dyscalculia and the brain; pp. 165–193. [ Google Scholar ]
- McLaren D.G., Ries M.L., Xu G., Johnson S.C. A generalized form of context-dependent psychophysiological interactions (gPPI): A comparison to standard approaches. NeuroImage. 2012; 61 (4):1277–1286. [ PMC free article ] [ PubMed ] [ Google Scholar ]
- Melby-Lervåg M., Hulme C. Is working memory training effective? A meta-analytic review. Dev. Psychol. 2013; 49 (2):270–291. [ PubMed ] [ Google Scholar ]
- Menon V. Chapter 7 - memory and cognitive control circuits in mathematical cognition and learning. In: Cappelletti M., Fias W., editors. Vol. 227. Elsevier; 2016. pp. 159–186. (Progress in Brain Research). [ PMC free article ] [ PubMed ] [ Google Scholar ]
- Nee D.E. fMRI replicability depends upon sufficient individual-level data. Nat. Commun. Biol. 2018; 30 [ PMC free article ] [ PubMed ] [ Google Scholar ]
- Nieder A. The neuronal code for number. Nat. Rev. Neurosci. 2016; 17 (6):366–382. [ PubMed ] [ Google Scholar ]
- Qin S., Cho S., Chen T., Rosenberg-Lee M., Geary D.C., Menon V. Hippocampal-neocortical functional reorganization underlies children’s cognitive development. Nat. Neurosci. 2014; 17 (9):1263–1269. [ PMC free article ] [ PubMed ] [ Google Scholar ]
- Ramanan S., Piguet O., Irish M. Rethinking the role of the angular gyrus in remembering the past and imagining the future: the contextual integration model. Neuroscientist. 2017 [ PubMed ] [ Google Scholar ]
- Rivera S.M., Reiss A.L., Eckert M.A., Menon V. Developmental changes in mental arithmetic: evidence for increased functional specialization in the left inferior parietal cortex. Cereb. Cortex. 2005; 15 :1779–1790. [ PubMed ] [ Google Scholar ]
- Rosenberg-Lee M., Iuculano T., Bae S.R., Richardson J., Qin S., Jolles D., Menon V. Short-term cognitive training recapitulates hippocampal functional changes associated with one year of longitudinal skill development. Trends Neurosci. Educ. 2018; 10 :19–29. [ Google Scholar ]
- Salmi J., Nyberg L., Laine M. Working memory training mostly engages general-purpose large-scale networks for learning. Neurosci. Biobehav. Rev. 2018; 93 :108–122. [ PubMed ] [ Google Scholar ]
- Schlichting M.L., Mumford J.A., Preston A.R. Learning-related representational changes reveal dissociable integration and separation signatures in the hippocampus and prefrontal cortex. Nat. Commun. 2015; 6 :8151. [ PMC free article ] [ PubMed ] [ Google Scholar ]
- Shohamy D., Wagner A.D. Integrating memories in the human brain: hippocampal-midbrain encoding of overlapping events. Neuron. 2008; 60 (2):378–389. [ PMC free article ] [ PubMed ] [ Google Scholar ]
- Siegler R.S., Shipley C. Variation, selection, and cognitive change. In: Simon T.J., Halford G.S., editors. Developing Cognitive Competence: New Approaches to Process Modeling. Lawrence Erlbaum Associates; Hillsdale, NJ: 1995. pp. 31–76. [ Google Scholar ]
- Simons D.J., Boot W.R., Charness N., Gathercole S.E., Chabris C.F., Hambrick D.Z., Stine-Morrow E.A.L. Do “Brain-Training” programs work? Psychol. Sci. Public Interest. 2016; 17 (3):103–186. [ PubMed ] [ Google Scholar ]
- Skeide M.A., Evans T.M., Mei E.Z., Abrams D.A., Menon V. Neural signatures of co-occurring reading and mathematical difficulties. Dev. Sci. 2018; 21 (6) [ PMC free article ] [ PubMed ] [ Google Scholar ]
- Spelke E. Core knowledge. Am. Psychol. 2000; 55 (11):1233–1243. [ PubMed ] [ Google Scholar ]
- Supekar K., Swigart A.G., Tenison C., Jolles D.D., Rosenberg-lee M., Fuchs L. Neural predictors of individual differences in response to math tutoring in primary-grade school children. Proc. Natl. Acad. Sci. 2013; 110 :8230–8235. [ PMC free article ] [ PubMed ] [ Google Scholar ]
- Thorndike E.L. A. G. Seiler; New York, NY: 1906. The Principles of Teaching Based on Psychology. [ Google Scholar ]
- Tompary A., Davachi L. Consolidation promotes the emergence of representational overlap in the Hippocampus and medial prefrontal cortex. Neuron. 2017; 96 (1):228–241. e5. [ PMC free article ] [ PubMed ] [ Google Scholar ]
- Townsend J.T., Ashby F.G. Methods of modeling capacity in simple processing systems. In: Castellan J., Restle F., editors. Vol. 3. Erlbaum; Hillsdale, N.J: 1978. pp. 200–239. (Cognitive Theory). [ Google Scholar ]
- Uddin L.Q., Menon V., Young C.B., Ryali S., Chen T., Khouzam A. Multivariate searchlight classification of structural magnetic resonance imaging in children and adolescents with autism. Biol. Psychiatry. 2011; 70 (9):833–841. [ PMC free article ] [ PubMed ] [ Google Scholar ]
- Woodcock R.W., McGrew K.S., Mather N. Riverside; Istasca, IL: 2001. Woodcock–Johnson III Tests of Achievement. [ Google Scholar ]
- Wu S.S., Meyer M.L., Maeda U., Salimpoor V., Tomiyama S., Geary D.C., Menon V. Standardized assessment of strategy use and working memory in early mental arithmetic performance. Dev. Neuropsychol. 2008; 33 (3):365–393. [ PMC free article ] [ PubMed ] [ Google Scholar ]
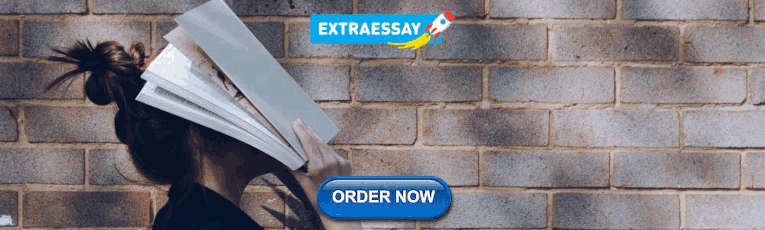
IMAGES
VIDEO
COMMENTS
The case study was conducted by keen observations of the special needed child by involving and getting ... Qustionnaire. 2. Direct observation. Etc.The study reveals the fact that the actually the child not having any slow learners like problem nor she is shy or un interested in learning by nature but she loves to read , learn , take part in ...
This study uses non-participant observation and qualitative interviews to examine the life of Mutawakilu a KG2 child of Choggu Yapalsi M/A KG and Primary School as a single case within the social ...
PDF | On Jan 1, 2021, Sutipyo Ru'iya and others published Educating with Paying Attention to Individual Differences: Case Study of Slow Learner Students in Inclusion School | Find, read and cite ...
This study examines the learning strategies of Special Assistance Teachers (GPK) for students with slow learner barriers of inclusive elementary school age. A qualitative case study approach is ...
In this way, the teacher can psychologically analyse the problem of the child and he/she can help the child get through the problem which will be helpful to the child to study better in the future. 4.2 Find out Learning Disability Slow learners may have a problem like dyslexia, sensory impairment or even an intellectual disability.
Children with slow learning problem or a slow learner are those who have low learning achievement or slightly below average of children in general. Today, the children slowly learn many encountered not only in school inclusion, but in regular schools too. Teachers who do not have adequate experience will find it difficult to deal with in the classroom. So the goal of this research is to find ...
inadequate training, mixed classes of both slow and fast learners, inability to identify slow learners, class size and lack of knowledge. It emphasizes the need to define and develop reference frameworks of teacher competencies and institutionalize in-service teacher education programmes through school-based practices and research. 1.
II. Slow Learners: Definition Slow learners are understudies with marginal knowledge and they frequently battle to adapt to the conventional scholastic requests of the customary classroom. As indicated by Lowenstein (2003), "Moderate learners are understudies with beneath normal psychological capacities who are not handicapped, but rather who
Slow learners are usually taught in one of two possible instructional arrangements: 1) a class composed mostly of average students, in which case up to 20%may be slow learners, or 2) a class specially designed for slow learners. Whether you meet slow learners in a regular class or special class, you will immediately feel the challenge of ...
DOI: 10.2991/ASSEHR.K.210304.026 Corpus ID: 233800264; Educating with Paying Attention to Individual Differences: Case Study of Slow Learner Students in Inclusion School @inproceedings{Ruiya2021EducatingWP, title={Educating with Paying Attention to Individual Differences: Case Study of Slow Learner Students in Inclusion School}, author={Sutipyo Ru'iya and Hanif Cahyo Adi Kistoro and Sutarman ...
Learning strategies are needed to help slow learners catch up. The research aims to describe the teacher's strategy for teaching slow-learning students with low grades. This type of research is qualitative research with a case study method. The research was conducted at SD Negeri Jemirahan with the participants being two grade 1 teachers.
"slow learners‟ academic performance". Beside this, the study will facilitates and guide parents in order to tackle the problems related to slow learning abilities in their child. Research Methodology The study was descriptive in nature based on qualitative research, which include a case study of ten (10) slow
The backward child or slow learner is the one who is unable to do the work where their educational attainments are lower than what they are capable of. A slow learner has the intelligence level of below average (70- ... Based on a study on slow learners conducted by Rashid et al., (2013), it was seen that slow
Challenges and Issues in Family Therapy: Case Study of a Slow Learner Citation: Amita Puri., et al. "Challenges and Issues in Family Therapy: Case Study of a Slow Learner". EC Psychology and Psychiatry 9.1 (2020): 01-05. due to the different perspectives that educationalists and clinicians are likely to have. Slow learner
The slow learners need special attention beyond school hours. If the teacher allot some extra time for the slow learners, then definitely they will improve. 8. Seating arrangement More often we see that the strong students in the class room don't wish to sit with the slow learners.
TLDR. The major finding was that slow learners were more successful by providing suitable conditions and educational opportunities to them by applying an inspiring quote from the universal scientist Albert Einstein saying "I never teach my pupils", which helps to solve this universal problem. Expand.
enhancing the developmental skills of slow learners. Although the slow learner's skill enhancement problem is discussed in [11], the presented method does not identify slow learners. In this, a random group of students is selected for the study. Kaur et al. [12] suggested a classification-based algorithm employing a predictive data mining ...
Slow learner is one who is unable to learn what the average child learns in the normal classroom. Slow learners are also called as "Backward Chi ldren". Thei r IQ ran ges from 70 to 90.
When the teacher looks at the slow learner, the smile of the teacher may change the slow learner. It makes him to feel that the teacher is for him in the class and she cares for him. So, automatically the student recognizes the teacher and obeys whatever the teacher says. Fixing a goal As a slow learner he may not be able to fix a goal in his life.
Faster learners showed greater overlap in neural representations within MTL regions implicated in memory formation, as well as greater segregation of large-scale brain circuits between trained and novel problems, indicating emergence of more distinct specialized functional circuitry with learning. Taken together, our study provides new insights ...
Each student has a uniqueness that must be considered by the teacher in the learning process, unusually slow learner children with various peculiarities. This study aims to reveal the attention of Islamic Religious Education teachers to slow learner children. This study is qualitative research with a survey approach—data sources of Islamic religious education teachers in inclusive schools ...
Slow Learner - Free download as Word Doc (.doc / .docx), PDF File (.pdf), Text File (.txt) or read online for free. Scribd is the world's largest social reading and publishing site.
Thus, the learner community can be classified into advanced, average, and slow learners. In this paper, a strategy based on Bloom s Taxonomy (revised) is proposed for identifying advanced, average ...