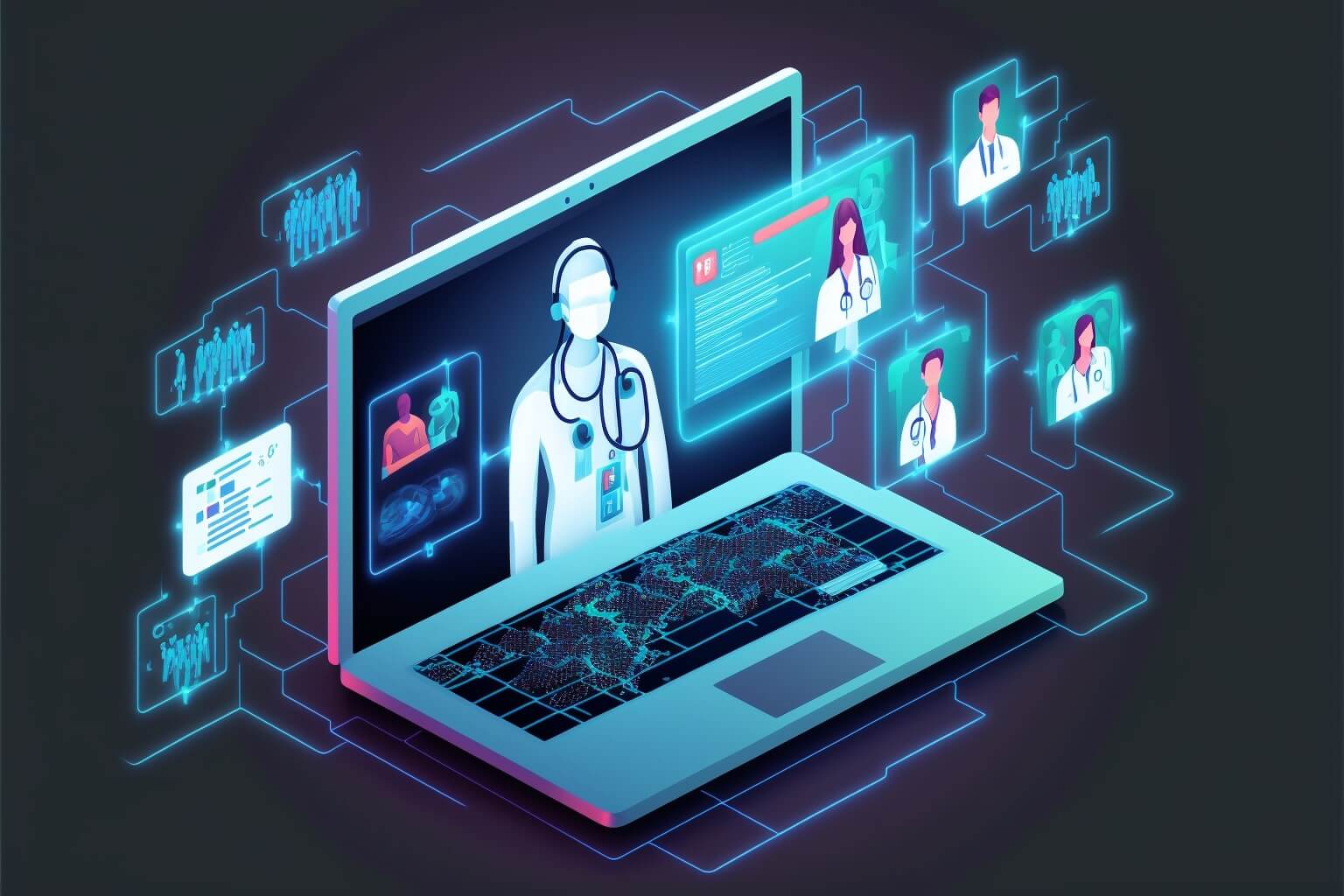
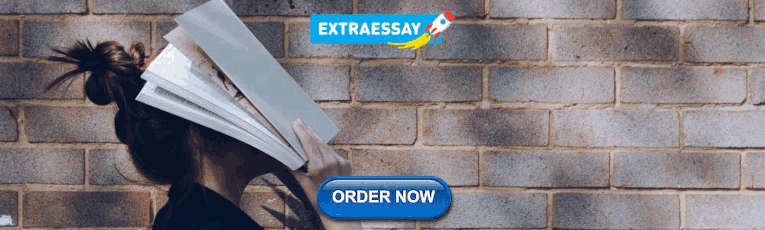
Data Mining Techniques in Healthcare: A Comprehensive Guide

data mining techniques have the potential to transform the healthcare industry by providing insights that were previously impossible to obtain.
As technology continues to advance, it is likely that data mining techniques will become even more sophisticated and powerful, enabling healthcare professionals to provide better care to patients and improve overall health outcomes.
Are you curious about how data mining techniques can improve healthcare? With the vast amount of data generated in the healthcare industry, data mining has become an essential tool for extracting valuable insights and improving patient outcomes.
Data mining techniques can help healthcare providers identify patterns and trends in large datasets, which can lead to more accurate diagnoses, better treatment plans, and more efficient operations.
Data mining involves analyzing large datasets to identify patterns, relationships, and anomalies. In the healthcare industry, data mining can be used to analyze patient data, clinical trials, medical claims, and electronic health records (EHRs).
By using data mining techniques, healthcare providers can identify risk factors for diseases, predict patient outcomes, and improve the quality of care. Data mining can also be used to identify fraudulent claims and reduce healthcare costs.
Data Mining Techniques in Healthcare
Healthcare organizations are increasingly using data mining techniques to improve efficiency, quality, and patient outcomes. Data mining is the process of discovering patterns and associations in large datasets, and applying it to make better decisions.
In healthcare, data mining can be used to identify drug interactions, detect fraudulent insurance claims, predict treatment efficiency, and improve patient safety.
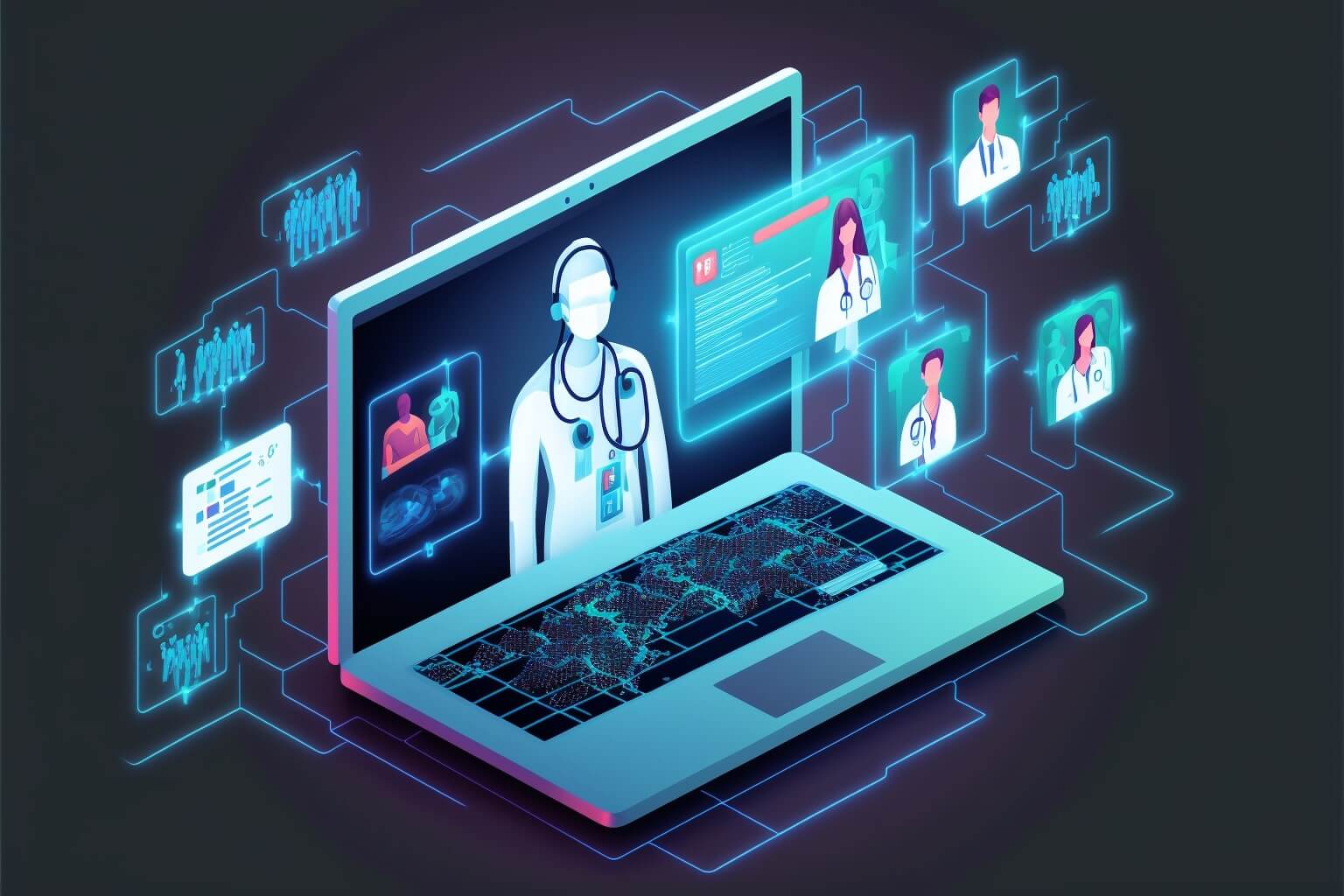
Data mining techniques in healthcare involve the use of various technologies such as neural networks, machine learning , clustering , and decision trees. These technologies enable healthcare organizations to analyze large amounts of data from electronic health records, medical images such as X-rays and MRIs, and other sources.
The data can be used to identify patterns and associations that can be used to improve patient care and outcomes.
Applications
The applications of data mining in healthcare are numerous and varied. One of the most common applications is in predictive analytics , where data mining is used to identify patients who are at risk of developing certain conditions.
This information can be used to develop treatment plans that are tailored to the patient’s needs. Data mining can also be used to identify fraudulent insurance claims, which can save healthcare organizations millions of dollars each year.
The benefits of data mining in healthcare are many. One of the biggest benefits is improved patient outcomes. By identifying patterns and associations in large datasets, healthcare organizations can develop more effective treatment plans that are tailored to the patient’s needs.
Data mining can also help reduce medical errors, which can improve patient safety. Additionally, data mining can help healthcare organizations identify areas where they can improve efficiency, which can lead to cost savings.
Despite the many benefits of data mining in healthcare, there are also some challenges that must be addressed. One of the biggest challenges is the need for skilled professionals who can analyze the data and develop effective treatment plans.
Another challenge is the need for healthcare organizations to protect patient privacy while still being able to use the data effectively. Finally, there is the challenge of keeping up with the latest trends and technologies in data mining and healthcare.
In conclusion, data mining techniques have become an essential tool in healthcare organizations, enabling them to improve efficiency, quality, and patient outcomes.
By using data mining to identify patterns and associations in large datasets, healthcare organizations can develop more effective treatment plans, reduce medical errors, and improve patient safety. However, healthcare organizations must also address the challenges of skilled professionals, patient privacy, and keeping up with the latest trends and technologies.
If you are curios to learn more about analytics and data science with potential use cases, then check out all of our post related to data & analytics or data science
Data Mining Techniques
Data mining techniques are used in healthcare to extract valuable information and patterns from large datasets. These techniques can assist in clinical decision-making, predicting disease outbreaks, and improving treatment efficiency.
Here are some of the most commonly used data mining techniques in healthcare:
Clustering is a technique used to group similar data points together . In healthcare, clustering can be used to identify groups of patients with similar characteristics or diseases. This can help doctors and researchers understand disease patterns and develop more effective treatments.

Image source: Becris | Flaticon
Classification
Classification is used to categorize data into predefined classes. In healthcare, classification can be used to identify patients with specific diseases or conditions. This can help doctors diagnose diseases more accurately and develop personalized treatment plans.

Image source: Freepik | Flaticon
Association Rule Mining
Association rule mining is used to identify relationships between different variables in a dataset. In healthcare, association rule mining can be used to identify drug interactions or to identify factors that contribute to medical errors. This can help doctors and researchers develop more effective treatment plans and reduce the risk of medical errors.
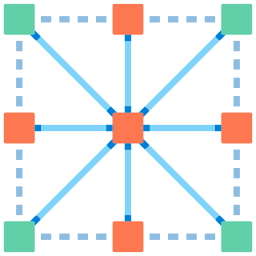
Image source: Chanut is Industries | Flaticon
Prediction is used to forecast future outcomes based on historical data. In healthcare, prediction can be used to predict disease outbreaks or to predict the effectiveness of different treatments. This can help doctors and researchers develop more effective treatment plans and improve patient outcomes.

Image source: Paul J | Flaticon
Visualization
Visualization is used to represent data in a graphical format. In healthcare, visualization can be used to represent data patterns or to visualize X-ray and MRI images. This can help doctors and researchers better understand disease patterns and develop more effective treatments.
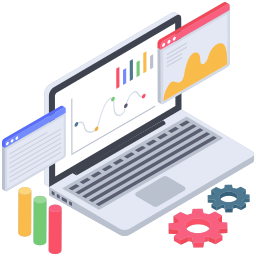
Summary Data Mining Techniques
Overall, data mining techniques are an important tool in healthcare. They can help doctors and researchers better understand disease patterns, develop more effective treatments, and improve patient outcomes. By using data mining techniques, healthcare professionals can make more informed decisions and provide better care to their patients.
Applications of Data Mining in Healthcare
Data mining techniques are increasingly being applied in the healthcare industry to improve the quality and efficiency of care , and to reduce costs. Here are some of the key applications of data mining in healthcare:
Disease Diagnosis
Data mining can be used to analyze electronic health records (EHRs) and other healthcare data to identify patterns and associations that can help with disease diagnosis.
For example, machine learning algorithms can be trained on large datasets of patient data to identify early warning signs of cancer or other diseases. Neural networks can be used to identify complex relationships between different disease symptoms, genetic markers, and other factors that can help with diagnosis.

Patient Safety
Data mining can also be used to identify potential safety issues in healthcare. For example, clustering algorithms can be used to identify groups of patients who are at high risk of medical errors or adverse events.
Visualization tools can be used to help doctors and nurses better understand patient data and identify potential safety issues.
Treatment Efficiency
Data mining can also be used to analyze treatment outcomes and identify the most effective treatments for different diseases.
For example, predictive analytics can be used to identify patients who are at high risk of developing complications or adverse reactions to certain treatments. This can help doctors to adjust treatment plans and improve patient outcomes.

Fraudulent Insurance
Data mining can also be used to identify cases of insurance fraud in healthcare. For example, clustering algorithms can be used to identify groups of patients who are submitting fraudulent insurance claims.
Predictive models can be used to identify patients who are at high risk of submitting fraudulent claims in the future.
Clinical Decision-making
Data mining can be used to support clinical decision-making by providing doctors and nurses with real-time access to patient data and predictive models.
For example, decision trees can be used to guide doctors through the diagnostic process and recommend the most appropriate treatment options for each patient. Artificial intelligence models can be used to analyze medical images and provide doctors with real-time feedback on potential diagnoses.
Medical Imaging
Data mining can also be used to analyze medical images and identify patterns and associations that can help with diagnosis and treatment.
For example, machine learning algorithms can be trained on large datasets of X-ray or MRI images to identify early warning signs of cancer or other diseases. Clustering algorithms can be used to identify groups of patients who are at high risk of developing certain conditions based on their medical images.
Overall, data mining techniques have the potential to revolutionize healthcare by improving the quality and efficiency of care, and reducing costs. By analyzing large datasets of patient data and identifying patterns and associations, healthcare providers can make more informed decisions and provide better care to their patients.
Examples of Healthcare Using Data Mining
Data mining is a powerful tool that has been used in healthcare to improve efficiency, quality, and patient satisfaction. Here are a few examples of how data mining techniques have been used in healthcare:
Example 1: Cancer Diagnosis and Treatment Efficiency
Data mining techniques have been used to analyze electronic health records (EHR) to identify patterns that can help improve cancer diagnosis and treatment efficiency.
By analyzing patient data, including X-ray and MRI images, researchers can develop predictive models that can help identify patients who are at risk for developing cancer or who may respond better to certain treatments.
Additionally, data mining can help identify drug interactions and potential side effects, allowing doctors to create more effective treatment plans.
Example 2: Insurance Fraud Detection
Data mining techniques have also been used to detect fraudulent insurance claims.
By analyzing large amounts of data, including claims data, medical records, and other information, data mining algorithms can detect patterns and anomalies that may indicate fraudulent activity.
This can help insurance companies save money and improve the overall quality of care for their patients.
Example 3: Clinical Decision Support Systems
Data mining techniques have been used to develop clinical decision support systems (CDSS) that can help doctors make more informed decisions.
By analyzing patient data, including medical history, lab results, and other information, CDSS can provide doctors with real-time recommendations for diagnosis and treatment. This can help improve patient safety and reduce medical errors.
In conclusion, data mining techniques have been used in healthcare to improve efficiency, quality, and patient satisfaction. By analyzing large amounts of data, including medical big data, data mining algorithms can identify data patterns, associations, and forecasting that can help doctors make more informed decisions. It is important for healthcare providers to continue to explore the use of data mining techniques in order to improve patient outcomes and provide the best possible care.
Conclusion: Data Mining Techniques in Healthcare
In conclusion, data mining techniques have revolutionized the healthcare industry by enabling healthcare professionals to analyze and interpret large volumes of data to make informed decisions.
By leveraging data mining techniques, healthcare professionals can identify patterns, trends, and relationships in patient data, which can be used to improve patient outcomes, reduce costs, and enhance the overall quality of care.
Benefits with Data Mining in Healthcare
One of the key benefits of data mining in healthcare is its ability to identify at-risk patients and predict potential health problems before they occur. This allows healthcare professionals to intervene early and provide targeted interventions to prevent adverse outcomes.
Additionally, data mining techniques can be used to identify new treatments and therapies, as well as to optimize existing treatments by tailoring them to individual patients.
However, it is important to note that data mining techniques are not a panacea for all healthcare challenges. They require careful planning, implementation, and interpretation to ensure that the results are accurate and meaningful.
Furthermore, ethical considerations must be taken into account to ensure that patient privacy and confidentiality are protected.
Overall, data mining techniques have the potential to transform the healthcare industry by providing insights that were previously impossible to obtain. As technology continues to advance, it is likely that data mining techniques will become even more sophisticated and powerful, enabling healthcare professionals to provide better care to patients and improve overall health outcomes.
FAQ: Data Mining in Healthcare
What is data mining in healthcare.
Data mining in healthcare is the process of extracting useful information from large datasets in order to improve patient care, reduce costs, and identify patterns and trends. u003cbru003eu003cbru003eThis can include anything from analyzing electronic health records (EHRs) to identifying risk factors for certain diseases.
What are some examples of data mining in healthcare?
There are many examples of data mining in healthcare, including:u003cbru003eu003cbru003eu003cstrongu003eu003cstrongu003e•u003c/strongu003eu003c/strongu003e Predictive modeling to identify patients at risk for hospital readmissionu003cbru003eu003cstrongu003eu003cstrongu003e•u003c/strongu003eu003c/strongu003e Analysis of EHRs to identify patterns in medication errorsu003cbru003eu003cstrongu003eu003cstrongu003e•u003c/strongu003eu003c/strongu003e Mining social media data to identify outbreaks of infectious diseasesu003cbru003eu003cstrongu003eu003cstrongu003e•u003c/strongu003eu003c/strongu003e Identifying patterns in patient data to improve clinical decision making
What are the benefits of data mining in healthcare?
Data mining in healthcare can have many benefits, including:u003cbru003eu003cbru003eu003cstrongu003eu003cstrongu003e•u003c/strongu003eu003c/strongu003e Improved patient outcomes through more accurate diagnoses and treatment plansu003cbru003eu003cstrongu003eu003cstrongu003e•u003c/strongu003eu003c/strongu003e Reduced costs through more efficient use of resourcesu003cbru003eu003cstrongu003eu003cstrongu003e•u003c/strongu003eu003c/strongu003e Improved population health through better disease prevention and managementu003cbru003eu003cstrongu003eu003cstrongu003e•u003c/strongu003eu003c/strongu003e Increased efficiency and productivity for healthcare providers
What are some challenges of data mining in healthcare?
There are also some challenges associated with data mining in healthcare, including:u003cbru003eu003cbru003eu003cstrongu003eu003cstrongu003e•u003c/strongu003eu003c/strongu003e Ensuring patient privacy and confidentialityu003cbru003eu003cstrongu003eu003cstrongu003e•u003c/strongu003eu003c/strongu003e Dealing with large amounts of data and ensuring data qualityu003cbru003eu003cstrongu003eu003cstrongu003e•u003c/strongu003eu003c/strongu003e Overcoming technical barriers, such as integrating data from different sourcesu003cbru003eu003cstrongu003eu003cstrongu003e•u003c/strongu003eu003c/strongu003e Ensuring that data mining results are accurate and reliable

Meet Eric, the data "guru" behind Datarundown. When he's not crunching numbers, you can find him running marathons, playing video games, and trying to win the Fantasy Premier League using his predictions model (not going so well).
Eric passionate about helping businesses make sense of their data and turning it into actionable insights. Follow along on Datarundown for all the latest insights and analysis from the data world.
Related Posts
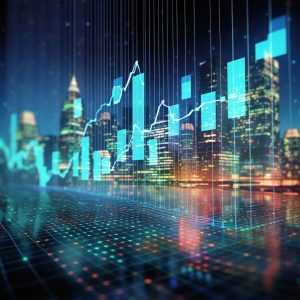
Data Mining for Business Analytics: Your Complete Manual
- Data Mining

Robotic Process Automation (RPA) in Clinical Trials
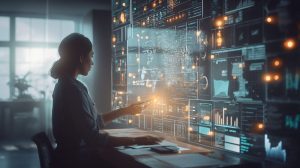
RPA as a Service (RPAaaS): 5 Key Benefits and Components
- Open access
- Published: 11 August 2021
Data mining in clinical big data: the frequently used databases, steps, and methodological models
- Wen-Tao Wu 1 , 2 na1 ,
- Yuan-Jie Li 3 na1 ,
- Ao-Zi Feng 1 ,
- Tao Huang 1 ,
- An-Ding Xu 4 &
- Jun Lyu ORCID: orcid.org/0000-0002-2237-8771 1
Military Medical Research volume 8 , Article number: 44 ( 2021 ) Cite this article
40k Accesses
160 Citations
2 Altmetric
Metrics details
Many high quality studies have emerged from public databases, such as Surveillance, Epidemiology, and End Results (SEER), National Health and Nutrition Examination Survey (NHANES), The Cancer Genome Atlas (TCGA), and Medical Information Mart for Intensive Care (MIMIC); however, these data are often characterized by a high degree of dimensional heterogeneity, timeliness, scarcity, irregularity, and other characteristics, resulting in the value of these data not being fully utilized. Data-mining technology has been a frontier field in medical research, as it demonstrates excellent performance in evaluating patient risks and assisting clinical decision-making in building disease-prediction models. Therefore, data mining has unique advantages in clinical big-data research, especially in large-scale medical public databases. This article introduced the main medical public database and described the steps, tasks, and models of data mining in simple language. Additionally, we described data-mining methods along with their practical applications. The goal of this work was to aid clinical researchers in gaining a clear and intuitive understanding of the application of data-mining technology on clinical big-data in order to promote the production of research results that are beneficial to doctors and patients.
With the rapid development of computer software/hardware and internet technology, the amount of data has increased at an amazing speed. “Big data” as an abstract concept currently affects all walks of life [ 1 ], and although its importance has been recognized, its definition varies slightly from field to field. In the field of computer science, big data refers to a dataset that cannot be perceived, acquired, managed, processed, or served within a tolerable time by using traditional IT and software and hardware tools. Generally, big data refers to a dataset that exceeds the scope of a simple database and data-processing architecture used in the early days of computing and is characterized by high-volume and -dimensional data that is rapidly updated represents a phenomenon or feature that has emerged in the digital age. Across the medical industry, various types of medical data are generated at a high speed, and trends indicate that applying big data in the medical field helps improve the quality of medical care and optimizes medical processes and management strategies [ 2 , 3 ]. Currently, this trend is shifting from civilian medicine to military medicine. For example, the United States is exploring the potential to use of one of its largest healthcare systems (the Military Healthcare System) to provide healthcare to eligible veterans in order to potentially benefit > 9 million eligible personnel [ 4 ]. Another data-management system has been developed to assess the physical and mental health of active-duty personnel, with this expected to yield significant economic benefits to the military medical system [ 5 ]. However, in medical research, the wide variety of clinical data and differences between several medical concepts in different classification standards results in a high degree of dimensionality heterogeneity, timeliness, scarcity, and irregularity to existing clinical data [ 6 , 7 ]. Furthermore, new data analysis techniques have yet to be popularized in medical research [ 8 ]. These reasons hinder the full realization of the value of existing data, and the intensive exploration of the value of clinical data remains a challenging problem.
Computer scientists have made outstanding contributions to the application of big data and introduced the concept of data mining to solve difficulties associated with such applications. Data mining (also known as knowledge discovery in databases) refers to the process of extracting potentially useful information and knowledge hidden in a large amount of incomplete, noisy, fuzzy, and random practical application data [ 9 ]. Unlike traditional research methods, several data-mining technologies mine information to discover knowledge based on the premise of unclear assumptions (i.e., they are directly applied without prior research design). The obtained information should have previously unknown, valid, and practical characteristics [ 9 ]. Data-mining technology does not aim to replace traditional statistical analysis techniques, but it does seek to extend and expand statistical analysis methodologies. From a practical point of view, machine learning (ML) is the main analytical method in data mining, as it represents a method of training models by using data and then using those models for predicting outcomes. Given the rapid progress of data-mining technology and its excellent performance in other industries and fields, it has introduced new opportunities and prospects to clinical big-data research [ 10 ]. Large amounts of high quality medical data are available to researchers in the form of public databases, which enable more researchers to participate in the process of medical data mining in the hope that the generated results can further guide clinical practice.
This article provided a valuable overview to medical researchers interested in studying the application of data mining on clinical big data. To allow a clearer understanding of the application of data-mining technology on clinical big data, the second part of this paper introduced the concept of public databases and summarized those commonly used in medical research. In the third part of the paper, we offered an overview of data mining, including introducing an appropriate model, tasks, and processes, and summarized the specific methods of data mining. In the fourth and fifth parts of this paper, we introduced data-mining algorithms commonly used in clinical practice along with specific cases in order to help clinical researchers clearly and intuitively understand the application of data-mining technology on clinical big data. Finally, we discussed the advantages and disadvantages of data mining in clinical analysis and offered insight into possible future applications.
Overview of common public medical databases
A public database describes a data repository used for research and dedicated to housing data related to scientific research on an open platform. Such databases collect and store heterogeneous and multi-dimensional health, medical, scientific research in a structured form and characteristics of mass/multi-ownership, complexity, and security. These databases cover a wide range of data, including those related to cancer research, disease burden, nutrition and health, and genetics and the environment. Table 1 summarizes the main public medical databases [ 11 , 12 , 13 , 14 , 15 , 16 , 17 , 18 , 19 , 20 , 21 , 22 , 23 , 24 , 25 , 26 ]. Researchers can apply for access to data based on the scope of the database and the application procedures required to perform relevant medical research.
Data mining: an overview
Data mining is a multidisciplinary field at the intersection of database technology, statistics, ML, and pattern recognition that profits from all these disciplines [ 27 ]. Although this approach is not yet widespread in the field of medical research, several studies have demonstrated the promise of data mining in building disease-prediction models, assessing patient risk, and helping physicians make clinical decisions [ 28 , 29 , 30 , 31 ].
Data-mining models
Data-mining has two kinds of models: descriptive and predictive. Predictive models are used to predict unknown or future values of other variables of interest, whereas descriptive models are often used to find patterns that describe data that can be interpreted by humans [ 32 ].
Data-mining tasks
A model is usually implemented by a task, with the goal of description being to generalize patterns of potential associations in the data. Therefore, using a descriptive model usually results in a few collections with the same or similar attributes. Prediction mainly refers to estimation of the variable value of a specific attribute based on the variable values of other attributes, including classification and regression [ 33 ].
Data-mining methods
After defining the data-mining model and task, the data mining methods required to build the approach based on the discipline involved are then defined. The data-mining method depends on whether or not dependent variables (labels) are present in the analysis. Predictions with dependent variables (labels) are generated through supervised learning, which can be performed by the use of linear regression, generalized linear regression, a proportional hazards model (the Cox regression model), a competitive risk model, decision trees, the random forest (RF) algorithm, and support vector machines (SVMs). In contrast, unsupervised learning involves no labels. The learning model infers some internal data structure. Common unsupervised learning methods include principal component analysis (PCA), association analysis, and clustering analysis.
Data-mining algorithms for clinical big data
Data mining based on clinical big data can produce effective and valuable knowledge, which is essential for accurate clinical decision-making and risk assessment [ 34 ]. Data-mining algorithms enable realization of these goals.
Supervised learning
A concept often mentioned in supervised learning is the partitioning of datasets. To prevent overfitting of a model, a dataset can generally be divided into two or three parts: a training set, validation set, and test set. Ripley [ 35 ] defined these parts as a set of examples used for learning and used to fit the parameters (i.e., weights) of the classifier, a set of examples used to tune the parameters (i.e., architecture) of a classifier, and a set of examples used only to assess the performance (generalized) of a fully-specified classifier, respectively. Briefly, the training set is used to train the model or determine the model parameters, the validation set is used to perform model selection, and the test set is used to verify model performance. In practice, data are generally divided into training and test sets, whereas the verification set is less involved. It should be emphasized that the results of the test set do not guarantee model correctness but only show that similar data can obtain similar results using the model. Therefore, the applicability of a model should be analysed in combination with specific problems in the research. Classical statistical methods, such as linear regression, generalized linear regression, and a proportional risk model, have been widely used in medical research. Notably, most of these classical statistical methods have certain data requirements or assumptions; however, in face of complicated clinical data, assumptions about data distribution are difficult to make. In contrast, some ML methods (algorithmic models) make no assumptions about the data and cross-verify the results; thus, they are likely to be favoured by clinical researchers [ 36 ]. For these reasons, this chapter focuses on ML methods that do not require assumptions about data distribution and classical statistical methods that are used in specific situations.
Decision tree
A decision tree is a basic classification and regression method that generates a result similar to the tree structure of a flowchart, where each tree node represents a test on an attribute, each branch represents the output of an attribute, each leaf node (decision node) represents a class or class distribution, and the topmost part of the tree is the root node [ 37 ]. The decision tree model is called a classification tree when used for classification and a regression tree when used for regression. Studies have demonstrated the utility of the decision tree model in clinical applications. In a study on the prognosis of breast cancer patients, a decision tree model and a classical logistic regression model were constructed, respectively, with the predictive performance of the different models indicating that the decision tree model showed stronger predictive power when using real clinical data [ 38 ]. Similarly, the decision tree model has been applied to other areas of clinical medicine, including diagnosis of kidney stones [ 39 ], predicting the risk of sudden cardiac arrest [ 40 ], and exploration of the risk factors of type II diabetes [ 41 ]. A common feature of these studies is the use of a decision tree model to explore the interaction between variables and classify subjects into homogeneous categories based on their observed characteristics. In fact, because the decision tree accounts for the strong interaction between variables, it is more suitable for use with decision algorithms that follow the same structure [ 42 ]. In the construction of clinical prediction models and exploration of disease risk factors and patient prognosis, the decision tree model might offer more advantages and practical application value than some classical algorithms. Although the decision tree has many advantages, it recursively separates observations into branches to construct a tree; therefore, in terms of data imbalance, the precision of decision tree models needs improvement.
The RF method
The RF algorithm was developed as an application of an ensemble-learning method based on a collection of decision trees. The bootstrap method [ 43 ] is used to randomly retrieve sample sets from the training set, with decision trees generated by the bootstrap method constituting a “random forest” and predictions based on this derived from an ensemble average or majority vote. The biggest advantage of the RF method is that the random sampling of predictor variables at each decision tree node decreases the correlation among the trees in the forest, thereby improving the precision of ensemble predictions [ 44 ]. Given that a single decision tree model might encounter the problem of overfitting [ 45 ], the initial application of RF minimizes overfitting in classification and regression and improves predictive accuracy [ 44 ]. Taylor et al. [ 46 ] highlighted the potential of RF in correctly differentiating in-hospital mortality in patients experiencing sepsis after admission to the emergency department. Nowhere in the healthcare system is the need more pressing to find methods to reduce uncertainty than in the fast, chaotic environment of the emergency department. The authors demonstrated that the predictive performance of the RF method was superior to that of traditional emergency medicine methods and the methods enabled evaluation of more clinical variables than traditional modelling methods, which subsequently allowed the discovery of clinical variables not expected to be of predictive value or which otherwise would have been omitted as a rare predictor [ 46 ]. Another study based on the Medical Information Mart for Intensive Care (MIMIC) II database [ 47 ] found that RF had excellent predictive power regarding intensive care unit (ICU) mortality [ 48 ]. These studies showed that the application of RF to big data stored in the hospital healthcare system provided a new data-driven method for predictive analysis in critical care. Additionally, random survival forests have recently been developed to analyse survival data, especially right-censored survival data [ 49 , 50 ], which can help researchers conduct survival analyses in clinical oncology and help develop personalized treatment regimens that benefit patients [ 51 ].
The SVM is a relatively new classification or prediction method developed by Cortes and Vapnik and represents a data-driven approach that does not require assumptions about data distribution [ 52 ]. The core purpose of an SVM is to identify a separation boundary (called a hyperplane) to help classify cases; thus, the advantages of SVMs are obvious when classifying and predicting cases based on high dimensional data or data with a small sample size [ 53 , 54 ].
In a study of drug compliance in patients with heart failure, researchers used an SVM to build a predictive model for patient compliance in order to overcome the problem of a large number of input variables relative to the number of available observations [ 55 ]. Additionally, the mechanisms of certain chronic and complex diseases observed in clinical practice remain unclear, and many risk factors, including gene–gene interactions and gene-environment interactions, must be considered in the research of such diseases [ 55 , 56 ]. SVMs are capable of addressing these issues. Yu et al. [ 54 ] applied an SVM for predicting diabetes onset based on data from the National Health and Nutrition Examination Survey (NHANES). Furthermore, these models have strong discrimination ability, making SVMs a promising classification approach for detecting individuals with chronic and complex diseases. However, a disadvantage of SVMs is that when the number of observation samples is large, the method becomes time- and resource-intensive, which is often highly inefficient.
Competitive risk model
Kaplan–Meier marginal regression and the Cox proportional hazards model are widely used in survival analysis in clinical studies. Classical survival analysis usually considers only one endpoint, such as the impact of patient survival time. However, in clinical medical research, multiple endpoints usually coexist, and these endpoints compete with one another to generate competitive risk data [ 57 ]. In the case of multiple endpoint events, the use of a single endpoint-analysis method can lead to a biased estimation of the probability of endpoint events due to the existence of competitive risks [ 58 ]. The competitive risk model is a classical statistical model based on the hypothesis of data distribution. Its main advantage is its accurate estimation of the cumulative incidence of outcomes for right-censored survival data with multiple endpoints [ 59 ]. In data analysis, the cumulative risk rate is estimated using the cumulative incidence function in single-factor analysis, and Gray’s test is used for between-group comparisons [ 60 ].
Multifactor analysis uses the Fine-Gray and cause-specific (CS) risk models to explore the cumulative risk rate [ 61 ]. The difference between the Fine-Gray and CS models is that the former is applicable to establishing a clinical prediction model and predicting the risk of a single endpoint of interest [ 62 ], whereas the latter is suitable for answering etiological questions, where the regression coefficient reflects the relative effect of covariates on the increased incidence of the main endpoint in the target event-free risk set [ 63 ]. Currently, in databases with CS records, such as Surveillance, Epidemiology, and End Results (SEER), competitive risk models exhibit good performance in exploring disease-risk factors and prognosis [ 64 ]. A study of prognosis in patients with oesophageal cancer from SEER showed that Cox proportional risk models might misestimate the effects of age and disease location on patient prognosis, whereas competitive risk models provide more accurate estimates of factors affecting patient prognosis [ 65 ]. In another study of the prognosis of penile cancer patients, researchers found that using a competitive risk model was more helpful in developing personalized treatment plans [ 66 ].
Unsupervised learning
In many data-analysis processes, the amount of usable identified data is small, and identifying data is a tedious process [ 67 ]. Unsupervised learning is necessary to judge and categorize data according to similarities, characteristics, and correlations and has three main applications: data clustering, association analysis, and dimensionality reduction. Therefore, the unsupervised learning methods introduced in this section include clustering analysis, association rules, and PCA.
Clustering analysis
The classification algorithm needs to “know” information concerning each category in advance, with all of the data to be classified having corresponding categories. When the above conditions cannot be met, cluster analysis can be applied to solve the problem [ 68 ]. Clustering places similar objects into different categories or subsets through the process of static classification. Consequently, objects in the same subset have similar properties. Many kinds of clustering techniques exist. Here, we introduced the four most commonly used clustering techniques.
Partition clustering
The core idea of this clustering method regards the centre of the data point as the centre of the cluster. The k-means method [ 69 ] is a representative example of this technique. The k-means method takes n observations and an integer, k , and outputs a partition of the n observations into k sets such that each observation belongs to the cluster with the nearest mean [ 70 ]. The k-means method exhibits low time complexity and high computing efficiency but has a poor processing effect on high dimensional data and cannot identify nonspherical clusters.
Hierarchical clustering
The hierarchical clustering algorithm decomposes a dataset hierarchically to facilitate the subsequent clustering [ 71 ]. Common algorithms for hierarchical clustering include BIRCH [ 72 ], CURE [ 73 ], and ROCK [ 74 ]. The algorithm starts by treating every point as a cluster, with clusters grouped according to closeness. When further combinations result in unexpected results under multiple causes or only one cluster remains, the grouping process ends. This method has wide applicability, and the relationship between clusters is easy to detect; however, the time complexity is high [ 75 ].
Clustering according to density
The density algorithm takes areas presenting a high degree of data density and defines these as belonging to the same cluster [ 76 ]. This method aims to find arbitrarily-shaped clusters, with the most representative algorithm being DBSCAN [ 77 ]. In practice, DBSCAN does not need to input the number of clusters to be partitioned and can handle clusters of various shapes; however, the time complexity of the algorithm is high. Furthermore, when data density is irregular, the quality of the clusters decreases; thus, DBSCAN cannot process high dimensional data [ 75 ].
Clustering according to a grid
Neither partition nor hierarchical clustering can identify clusters with nonconvex shapes. Although a dimension-based algorithm can accomplish this task, the time complexity is high. To address this problem, data-mining researchers proposed grid-based algorithms that changed the original data space into a grid structure of a certain size. A representative algorithm is STING, which divides the data space into several square cells according to different resolutions and clusters the data of different structure levels [ 78 ]. The main advantage of this method is its high processing speed and its exclusive dependence on the number of units in each dimension of the quantized space.
In clinical studies, subjects tend to be actual patients. Although researchers adopt complex inclusion and exclusion criteria before determining the subjects to be included in the analyses, heterogeneity among different patients cannot be avoided [ 79 , 80 ]. The most common application of cluster analysis in clinical big data is in classifying heterogeneous mixed groups into homogeneous groups according to the characteristics of existing data (i.e., “subgroups” of patients or observed objects are identified) [ 81 , 82 ]. This new information can then be used in the future to develop patient-oriented medical-management strategies. Docampo et al. [ 81 ] used hierarchical clustering to reduce heterogeneity and identify subgroups of clinical fibromyalgia, which aided the evaluation and management of fibromyalgia. Additionally, Guo et al. [ 83 ] used k-means clustering to divide patients with essential hypertension into four subgroups, which revealed that the potential risk of coronary heart disease differed between different subgroups. On the other hand, density- and grid-based clustering algorithms have mostly been used to process large numbers of images generated in basic research and clinical practice, with current studies focused on developing new tools to help clinical research and practices based on these technologies [ 84 , 85 ]. Cluster analysis will continue to have extensive application prospects along with the increasing emphasis on personalized treatment.
Association rules
Association rules discover interesting associations and correlations between item sets in large amounts of data. These rules were first proposed by Agrawal et al. [ 86 ] and applied to analyse customer buying habits to help retailers create sales plans. Data-mining based on association rules identifies association rules in a two-step process: 1) all high frequency items in the collection are listed and 2) frequent association rules are generated based on the high frequency items [ 87 ]. Therefore, before association rules can be obtained, sets of frequent items must be calculated using certain algorithms. The Apriori algorithm is based on the a priori principle of finding all relevant adjustment items in a database transaction that meet a minimum set of rules and restrictions or other restrictions [ 88 ]. Other algorithms are mostly variants of the Apriori algorithm [ 64 ]. The Apriori algorithm must scan the entire database every time it scans the transaction; therefore, algorithm performance deteriorates as database size increases [ 89 ], making it potentially unsuitable for analysing large databases. The frequent pattern (FP) growth algorithm was proposed to improve efficiency. After the first scan, the FP algorithm compresses the frequency set in the database into a FP tree while retaining the associated information and then mines the conditional libraries separately [ 90 ]. Association-rule technology is often used in medical research to identify association rules between disease risk factors (i.e., exploration of the joint effects of disease risk factors and combinations of other risk factors). For example, Li et al. [ 91 ] used the association-rule algorithm to identify the most important stroke risk factor as atrial fibrillation, followed by diabetes and a family history of stroke. Based on the same principle, association rules can also be used to evaluate treatment effects and other aspects. For example, Guo et al. [ 92 ] used the FP algorithm to generate association rules and evaluate individual characteristics and treatment effects of patients with diabetes, thereby reducing the readability rate of patients with diabetes. Association rules reveal a connection between premises and conclusions; however, the reasonable and reliable application of information can only be achieved through validation by experienced medical professionals and through extensive causal research [ 92 ].
PCA is a widely used data-mining method that aims to reduce data dimensionality in an interpretable way while retaining most of the information present in the data [ 93 , 94 ]. The main purpose of PCA is descriptive, as it requires no assumptions about data distribution and is, therefore, an adaptive and exploratory method. During the process of data analysis, the main steps of PCA include standardization of the original data, calculation of a correlation coefficient matrix, calculation of eigenvalues and eigenvectors, selection of principal components, and calculation of the comprehensive evaluation value. PCA does not often appear as a separate method, as it is often combined with other statistical methods [ 95 ]. In practical clinical studies, the existence of multicollinearity often leads to deviation from multivariate analysis. A feasible solution is to construct a regression model by PCA, which replaces the original independent variables with each principal component as a new independent variable for regression analysis, with this most commonly seen in the analysis of dietary patterns in nutritional epidemiology [ 96 ]. In a study of socioeconomic status and child-developmental delays, PCA was used to derive a new variable (the household wealth index) from a series of household property reports and incorporate this new variable as the main analytical variable into the logistic regression model [ 97 ]. Additionally, PCA can be combined with cluster analysis. Burgel et al. [ 98 ] used PCA to transform clinical data to address the lack of independence between existing variables used to explore the heterogeneity of different subtypes of chronic obstructive pulmonary disease. Therefore, in the study of subtypes and heterogeneity of clinical diseases, PCA can eliminate noisy variables that can potentially corrupt the cluster structure, thereby increasing the accuracy of the results of clustering analysis [ 98 , 99 ].
The data-mining process and examples of its application using common public databases
Open-access databases have the advantages of large volumes of data, wide data coverage, rich data information, and a cost-efficient method of research, making them beneficial to medical researchers. In this chapter, we introduced the data-mining process and methods and their application in research based on examples of utilizing public databases and data-mining algorithms.
The data-mining process
Figure 1 shows a series of research concepts. The data-mining process is divided into several steps: (1) database selection according to the research purpose; (2) data extraction and integration, including downloading the required data and combining data from multiple sources; (3) data cleaning and transformation, including removal of incorrect data, filling in missing data, generating new variables, converting data format, and ensuring data consistency; (4) data mining, involving extraction of implicit relational patterns through traditional statistics or ML; (5) pattern evaluation, which focuses on the validity parameters and values of the relationship patterns of the extracted data; and (6) assessment of the results, involving translation of the extracted data-relationship model into comprehensible knowledge made available to the public.
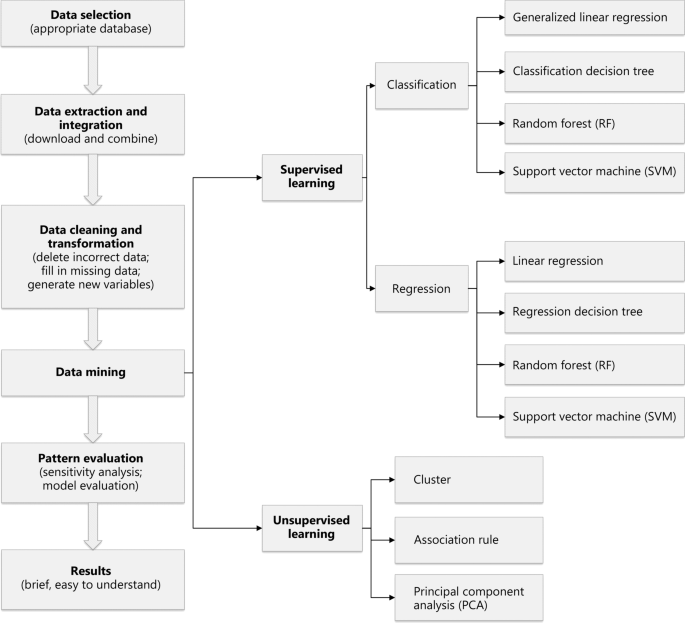
The steps of data mining in medical public database
Examples of data-mining applied using public databases
Establishment of warning models for the early prediction of disease.
A previous study identified sepsis as a major cause of death in ICU patients [ 100 ]. The authors noted that the predictive model developed previously used a limited number of variables, and that model performance required improvement. The data-mining process applied to address these issues was, as follows: (1) data selection using the MIMIC III database; (2) extraction and integration of three types of data, including multivariate features (demographic information and clinical biochemical indicators), time series data (temperature, blood pressure, and heart rate), and clinical latent features (various scores related to disease); (3) data cleaning and transformation, including fixing irregular time series measurements, estimating missing values, deleting outliers, and addressing data imbalance; (4) data mining through the use of logical regression, generation of a decision tree, application of the RF algorithm, an SVM, and an ensemble algorithm (a combination of multiple classifiers) to established the prediction model; (5) pattern evaluation using sensitivity, precision, and the area under the receiver operating characteristic curve to evaluate model performance; and (6) evaluation of the results, in this case the potential to predicting the prognosis of patients with sepsis and whether the model outperformed current scoring systems.
Exploring prognostic risk factors in cancer patients
Wu et al. [ 101 ] noted that traditional survival-analysis methods often ignored the influence of competitive risk events, such as suicide and car accident, on outcomes, leading to deviations and misjudgements in estimating the effect of risk factors. They used the SEER database, which offers cause-of-death data for cancer patients, and a competitive risk model to address this problem according to the following process: (1) data were obtained from the SEER database; (2) demography, clinical characteristics, treatment modality, and cause of death of cecum cancer patients were extracted from the database; (3) patient data were deleted when there were no demographic, clinical, therapeutic, or cause-of-death variables; (4) Cox regression and two kinds of competitive risk models were applied for survival analysis; (5) the results were compared between three different models; and (6) the results revealed that for survival data with multiple endpoints, the competitive risk model was more favourable.
Derivation of dietary patterns
A study by Martínez Steele et al. [ 102 ] applied PCA for nutritional epidemiological analysis to determine dietary patterns and evaluate the overall nutritional quality of the population based on those patterns. Their process involved the following: (1) data were extracted from the NHANES database covering the years 2009–2010; (2) demographic characteristics and two 24 h dietary recall interviews were obtained; (3) data were weighted and excluded based on subjects not meeting specific criteria; (4) PCA was used to determine dietary patterns in the United States population, and Gaussian regression and restricted cubic splines were used to assess associations between ultra-processed foods and nutritional balance; (5) eigenvalues, scree plots, and the interpretability of the principal components were reviewed to screen and evaluate the results; and (6) the results revealed a negative association between ultra-processed food intake and overall dietary quality. Their findings indicated that a nutritionally balanced eating pattern was characterized by a diet high in fibre, potassium, magnesium, and vitamin C intake along with low sugar and saturated fat consumption.
The use of “big data” has changed multiple aspects of modern life, with its use combined with data-mining methods capable of improving the status quo [ 86 ]. The aim of this study was to aid clinical researchers in understanding the application of data-mining technology on clinical big data and public medical databases to further their research goals in order to benefit clinicians and patients. The examples provided offer insight into the data-mining process applied for the purposes of clinical research. Notably, researchers have raised concerns that big data and data-mining methods were not a perfect fit for adequately replicating actual clinical conditions, with the results potentially capable of misleading doctors and patients [ 86 ]. Therefore, given the rate at which new technologies and trends progress, it is necessary to maintain a positive attitude concerning their potential impact while remaining cautious in examining the results provided by their application.
In the future, the healthcare system will need to utilize increasingly larger volumes of big data with higher dimensionality. The tasks and objectives of data analysis will also have higher demands, including higher degrees of visualization, results with increased accuracy, and stronger real-time performance. As a result, the methods used to mine and process big data will continue to improve. Furthermore, to increase the formality and standardization of data-mining methods, it is possible that a new programming language specifically for this purpose will need to be developed, as well as novel methods capable of addressing unstructured data, such as graphics, audio, and text represented by handwriting. In terms of application, the development of data-management and disease-screening systems for large-scale populations, such as the military, will help determine the best interventions and formulation of auxiliary standards capable of benefitting both cost-efficiency and personnel. Data-mining technology can also be applied to hospital management in order to improve patient satisfaction, detect medical-insurance fraud and abuse, and reduce costs and losses while improving management efficiency. Currently, this technology is being applied for predicting patient disease, with further improvements resulting in the increased accuracy and speed of these predictions. Moreover, it is worth noting that technological development will concomitantly require higher quality data, which will be a prerequisite for accurate application of the technology.
Finally, the ultimate goal of this study was to explain the methods associated with data mining and commonly used to process clinical big data. This review will potentially promote further study and aid doctors and patients.
Abbreviations
Biologic Specimen and Data Repositories Information Coordinating Center
China Health and Retirement Longitudinal Study
China Health and Nutrition Survey
China Kadoorie Biobank
Cause-specific risk
Comparative Toxicogenomics Database
EICU Collaborative Research Database
Frequent pattern
Global burden of disease
Gene expression omnibus
Health and Retirement Study
International Cancer Genome Consortium
Medical Information Mart for Intensive Care
- Machine learning
National Health and Nutrition Examination Survey
Principal component analysis
Paediatric intensive care
Random forest
Surveillance, epidemiology, and end results
Support vector machine
The Cancer Genome Atlas
Herland M, Khoshgoftaar TM, Wald R. A review of data mining using big data in health informatics. J Big Data. 2014;1(1):1–35.
Article Google Scholar
Wang F, Zhang P, Wang X, Hu J. Clinical risk prediction by exploring high-order feature correlations. AMIA Annu Symp Proc. 2014;2014:1170–9.
PubMed PubMed Central Google Scholar
Xu R, Li L, Wang Q. dRiskKB: a large-scale disease-disease risk relationship knowledge base constructed from biomedical text. BMC Bioinform. 2014;15:105. https://doi.org/10.1186/1471-2105-15-105 .
Article CAS Google Scholar
Ramachandran S, Erraguntla M, Mayer R, Benjamin P, Editors. Data mining in military health systems-clinical and administrative applications. In: 2007 IEEE international conference on automation science and engineering; 2007. https://doi.org/10.1109/COASE.2007.4341764 .
Vie LL, Scheier LM, Lester PB, Ho TE, Labarthe DR, Seligman MEP. The US army person-event data environment: a military-civilian big data enterprise. Big Data. 2015;3(2):67–79. https://doi.org/10.1089/big.2014.0055 .
Article PubMed Google Scholar
Mohan A, Blough DM, Kurc T, Post A, Saltz J. Detection of conflicts and inconsistencies in taxonomy-based authorization policies. IEEE Int Conf Bioinform Biomed. 2012;2011:590–4. https://doi.org/10.1109/BIBM.2011.79 .
Luo J, Wu M, Gopukumar D, Zhao Y. Big data application in biomedical research and health care: a literature review. Biomed Inform Insights. 2016;8:1–10. https://doi.org/10.4137/BII.S31559 .
Article CAS PubMed PubMed Central Google Scholar
Bellazzi R, Zupan B. Predictive data mining in clinical medicine: current issues and guidelines. Int J Med Inform. 2008;77(2):81–97.
Sahu H, Shrma S, Gondhalakar S. A brief overview on data mining survey. Int J Comput Technol Electron Eng. 2011;1(3):114–21.
Google Scholar
Obermeyer Z, Emanuel EJ. Predicting the future - big data, machine learning, and clinical medicine. N Engl J Med. 2016;375(13):1216–9.
Article PubMed PubMed Central Google Scholar
Doll KM, Rademaker A, Sosa JA. Practical guide to surgical data sets: surveillance, epidemiology, and end results (SEER) database. JAMA Surg. 2018;153(6):588–9.
Johnson AE, Pollard TJ, Shen L, Lehman LW, Feng M, Ghassemi M, et al. MIMIC-III, a freely accessible critical care database. Sci Data. 2016;3: 160035. https://doi.org/10.1038/sdata.2016.35 .
Ahluwalia N, Dwyer J, Terry A, Moshfegh A, Johnson C. Update on NHANES dietary data: focus on collection, release, analytical considerations, and uses to inform public policy. Adv Nutr. 2016;7(1):121–34.
Vos T, Lim SS, Abbafati C, Abbas KM, Abbasi M, Abbasifard M, et al. Global burden of 369 diseases and injuries in 204 countries and territories, 1990–2019: a systematic analysis for the Global Burden of Disease Study 2019. Lancet. 2020;396(10258):1204–22. https://doi.org/10.1016/S0140-6736(20)30925-9 .
Palmer LJ. UK Biobank: Bank on it. Lancet. 2007;369(9578):1980–2. https://doi.org/10.1016/S0140-6736(07)60924-6 .
Cancer Genome Atlas Research Network, Weinstein JN, Collisson EA, Mills GB, Shaw KR, Ozenberger BA, et al. The cancer genome atlas pan-cancer analysis project. Nat Genet. 2013;45(10):1113–20. https://doi.org/10.1038/ng.2764 .
Davis S, Meltzer PS. GEOquery: a bridge between the Gene Expression Omnibus (GEO) and BioConductor. Bioinformatics. 2007;23(14):1846–7.
Article PubMed CAS Google Scholar
Zhang J, Bajari R, Andric D, Gerthoffert F, Lepsa A, Nahal-Bose H, et al. The international cancer genome consortium data portal. Nat Biotechnol. 2019;37(4):367–9.
Article CAS PubMed Google Scholar
Chen Z, Chen J, Collins R, Guo Y, Peto R, Wu F, et al. China Kadoorie Biobank of 0.5 million people: survey methods, baseline characteristics and long-term follow-up. Int J Epidemiol. 2011;40(6):1652–66.
Davis AP, Grondin CJ, Johnson RJ, Sciaky D, McMorran R, Wiegers J, et al. The comparative toxicogenomics database: update 2019. Nucleic Acids Res. 2019;47(D1):D948–54. https://doi.org/10.1093/nar/gky868 .
Zeng X, Yu G, Lu Y, Tan L, Wu X, Shi S, et al. PIC, a paediatric-specific intensive care database. Sci Data. 2020;7(1):14.
Giffen CA, Carroll LE, Adams JT, Brennan SP, Coady SA, Wagner EL. Providing contemporary access to historical biospecimen collections: development of the NHLBI Biologic Specimen and Data Repository Information Coordinating Center (BioLINCC). Biopreserv Biobank. 2015;13(4):271–9.
Zhang B, Zhai FY, Du SF, Popkin BM. The China Health and Nutrition Survey, 1989–2011. Obes Rev. 2014;15(Suppl 1):2–7. https://doi.org/10.1111/obr.12119 .
Zhao Y, Hu Y, Smith JP, Strauss J, Yang G. Cohort profile: the China Health and Retirement Longitudinal Study (CHARLS). Int J Epidemiol. 2014;43(1):61–8.
Pollard TJ, Johnson AEW, Raffa JD, Celi LA, Mark RG, Badawi O. The eICU collaborative research database, a freely available multi-centre database for critical care research. Sci Data. 2018;5:180178. https://doi.org/10.1038/sdata.2018.178 .
Fisher GG, Ryan LH. Overview of the health and retirement study and introduction to the special issue. Work Aging Retire. 2018;4(1):1–9.
Iavindrasana J, Cohen G, Depeursinge A, Müller H, Meyer R, Geissbuhler A. Clinical data mining: a review. Yearb Med Inform. 2009:121–33.
Zhang Y, Guo SL, Han LN, Li TL. Application and exploration of big data mining in clinical medicine. Chin Med J. 2016;129(6):731–8. https://doi.org/10.4103/0366-6999.178019 .
Ngiam KY, Khor IW. Big data and machine learning algorithms for health-care delivery. Lancet Oncol. 2019;20(5):e262–73.
Huang C, Murugiah K, Mahajan S, Li S-X, Dhruva SS, Haimovich JS, et al. Enhancing the prediction of acute kidney injury risk after percutaneous coronary intervention using machine learning techniques: a retrospective cohort study. PLoS Med. 2018;15(11):e1002703.
Rahimian F, Salimi-Khorshidi G, Payberah AH, Tran J, Ayala Solares R, Raimondi F, et al. Predicting the risk of emergency admission with machine learning: development and validation using linked electronic health records. PLoS Med. 2018;15(11):e1002695.
Kantardzic M. Data Mining: concepts, models, methods, and algorithms. Technometrics. 2003;45(3):277.
Jothi N, Husain W. Data mining in healthcare—a review. Procedia Comput Sci. 2015;72:306–13.
Piatetsky-Shapiro G, Tamayo P. Microarray data mining: facing the challenges. SIGKDD. 2003;5(2):1–5. https://doi.org/10.1145/980972.980974 .
Ripley BD. Pattern recognition and neural networks. Cambridge: Cambridge University Press; 1996.
Book Google Scholar
Arlot S, Celisse A. A survey of cross-validation procedures for model selection. Stat Surv. 2010;4:40–79. https://doi.org/10.1214/09-SS054 .
Shouval R, Bondi O, Mishan H, Shimoni A, Unger R, Nagler A. Application of machine learning algorithms for clinical predictive modelling: a data-mining approach in SCT. Bone Marrow Transp. 2014;49(3):332–7.
Momenyan S, Baghestani AR, Momenyan N, Naseri P, Akbari ME. Survival prediction of patients with breast cancer: comparisons of decision tree and logistic regression analysis. Int J Cancer Manag. 2018;11(7):e9176.
Topaloğlu M, Malkoç G. Decision tree application for renal calculi diagnosis. Int J Appl Math Electron Comput. 2016. https://doi.org/10.18100/ijamec.281134.
Li H, Wu TT, Yang DL, Guo YS, Liu PC, Chen Y, et al. Decision tree model for predicting in-hospital cardiac arrest among patients admitted with acute coronary syndrome. Clin Cardiol. 2019;42(11):1087–93.
Ramezankhani A, Hadavandi E, Pournik O, Shahrabi J, Azizi F, Hadaegh F. Decision tree-based modelling for identification of potential interactions between type 2 diabetes risk factors: a decade follow-up in a Middle East prospective cohort study. BMJ Open. 2016;6(12):e013336.
Carmona-Bayonas A, Jiménez-Fonseca P, Font C, Fenoy F, Otero R, Beato C, et al. Predicting serious complications in patients with cancer and pulmonary embolism using decision tree modelling: the EPIPHANY Index. Br J Cancer. 2017;116(8):994–1001.
Efron B. Bootstrap methods: another look at the jackknife. In: Kotz S, Johnson NL, editors. Breakthroughs in statistics. New York: Springer; 1992. p. 569–93.
Chapter Google Scholar
Breima L. Random forests. Mach Learn. 2010;1(45):5–32. https://doi.org/10.1023/A:1010933404324 .
Franklin J. The elements of statistical learning: data mining, inference and prediction. Math Intell. 2005;27(2):83–5.
Taylor RA, Pare JR, Venkatesh AK, Mowafi H, Melnick ER, Fleischman W, et al. Prediction of in-hospital mortality in emergency department patients with sepsis: a local big data-driven, machine learning approach. Acad Emerg Med. 2016;23(3):269–78.
Lee J, Scott DJ, Villarroel M, Clifford GD, Saeed M, Mark RG. Open-access MIMIC-II database for intensive care research. Annu Int Conf IEEE Eng Med Biol Soc. 2011:8315–8. https://doi.org/10.1109/IEMBS.2011.6092050 .
Lee J. Patient-specific predictive modelling using random forests: an observational study for the critically Ill. JMIR Med Inform. 2017;5(1):e3.
Wongvibulsin S, Wu KC, Zeger SL. Clinical risk prediction with random forests for survival, longitudinal, and multivariate (RF-SLAM) data analysis. BMC Med Res Methodol. 2019;20(1):1.
Taylor JMG. Random survival forests. J Thorac Oncol. 2011;6(12):1974–5.
Hu C, Steingrimsson JA. Personalized risk prediction in clinical oncology research: applications and practical issues using survival trees and random forests. J Biopharm Stat. 2018;28(2):333–49.
Dietrich R, Opper M, Sompolinsky H. Statistical mechanics of support vector networks. Phys Rev Lett. 1999;82(14):2975.
Verplancke T, Van Looy S, Benoit D, Vansteelandt S, Depuydt P, De Turck F, et al. Support vector machine versus logistic regression modelling for prediction of hospital mortality in critically ill patients with haematological malignancies. BMC Med Inform Decis Mak. 2008;8:56. https://doi.org/10.1186/1472-6947-8-56 .
Yu W, Liu T, Valdez R, Gwinn M, Khoury MJ. Application of support vector machine modelling for prediction of common diseases: the case of diabetes and pre-diabetes. BMC Med Inform Decis Mak. 2010;10:16. https://doi.org/10.1186/1472-6947-10-16 .
Son YJ, Kim HG, Kim EH, Choi S, Lee SK. Application of support vector machine for prediction of medication adherence in heart failure patients. Healthc Inform Res. 2010;16(4):253–9.
Schadt EE, Friend SH, Shaywitz DA. A network view of disease and compound screening. Nat Rev Drug Discov. 2009;8(4):286–95.
Austin PC, Lee DS, Fine JP. Introduction to the analysis of survival data in the presence of competing risks. Circulation. 2016;133(6):601–9.
Putter H, Fiocco M, Geskus RB. Tutorial in biostatistics: competing risks and multi-state models. Stat Med. 2007;26(11):2389–430. https://doi.org/10.1002/sim.2712 .
Klein JP. Competing risks. WIREs Comp Stat. 2010;2(3):333–9. https://doi.org/10.1002/wics.83 .
Haller B, Schmidt G, Ulm K. Applying competing risks regression models: an overview. Lifetime Data Anal. 2013;19(1):33–58. https://doi.org/10.1007/s10985-012-9230-8 .
Fine JP, Gray RJ. A proportional hazards model for the subdistribution of a competing risk. J Am Stat Assoc. 1999;94(446):496–509.
Koller MT, Raatz H, Steyerberg EW, Wolbers M. Competing risks and the clinical community: irrelevance or ignorance? Stat Med. 2012;31(11–12):1089–97.
Lau B, Cole SR, Gange SJ. Competing risk regression models for epidemiologic data. Am J Epidemiol. 2009;170(2):244–56.
Yang J, Li Y, Liu Q, Li L, Feng A, Wang T, et al. Brief introduction of medical database and data mining technology in big data era. J Evid Based Med. 2020;13(1):57–69.
Yu Z, Yang J, Gao L, Huang Q, Zi H, Li X. A competing risk analysis study of prognosis in patients with esophageal carcinoma 2006–2015 using data from the surveillance, epidemiology, and end results (SEER) database. Med Sci Monit. 2020;26:e918686.
Yang J, Pan Z, He Y, Zhao F, Feng X, Liu Q, et al. Competing-risks model for predicting the prognosis of penile cancer based on the SEER database. Cancer Med. 2019;8(18):7881–9.
Miotto R, Wang F, Wang S, Jiang X, Dudley JT. Deep learning for healthcare: review, opportunities and challenges. Brief Bioinform. 2018;19(6):1236–46.
Alashwal H, El Halaby M, Crouse JJ, Abdalla A, Moustafa AA. The application of unsupervised clustering methods to Alzheimer’s disease. Front Comput Neurosci. 2019;13:31.
Macqueen J. Some methods for classification and analysis of multivariate observations. In: Proceedings of the fifth Berkeley symposium on mathematical statistics and probability, Oakland, CA: University of California Press;1967.
Forgy EW. Cluster analysis of multivariate data: efficiency versus interpretability of classifications. Biometrics. 1965;21:768–9.
Johnson SC. Hierarchical clustering schemes. Psychometrika. 1967;32(3):241–54.
Zhang T, Ramakrishnan R, Livny M. BIRCH: an efficient data clustering method for very large databases. ACM SIGMOD Rec. 1996;25(2):103–14.
Guha S, Rastogi R, Shim K. CURE: an efficient clustering algorithm for large databases. ACM SIGMOD Rec. 1998;27(2):73–84.
Guha S, Rastogi R, Shim K. ROCK: a robust clustering algorithm for categorical attributes. Inf Syst. 2000;25(5):345–66.
Xu D, Tian Y. A comprehensive survey of clustering algorithms. Ann Data Sci. 2015;2(2):165–93.
Kriegel HP, Kröger P, Sander J, Zimek A. Density-based clustering. WIRES Data Min Knowl. 2011;1(3):231–40. https://doi.org/10.1002/widm.30 .
Ester M, Kriegel HP, Sander J, Xu X, editors. A density-based algorithm for discovering clusters in large spatial databases with noise. In: Proceedings of 2nd international conference on knowledge discovery and data mining Portland, Oregon: AAAI Press; 1996. p. 226–31.
Wang W, Yang J, Muntz RR. STING: a statistical information grid approach to spatial data mining. In: Proceedings of the 23rd international conference on very large data bases, Morgan Kaufmann Publishers Inc.; 1997. p. 186–95.
Iwashyna TJ, Burke JF, Sussman JB, Prescott HC, Hayward RA, Angus DC. Implications of heterogeneity of treatment effect for reporting and analysis of randomized trials in critical care. Am J Respir Crit Care Med. 2015;192(9):1045–51.
Ruan S, Lin H, Huang C, Kuo P, Wu H, Yu C. Exploring the heterogeneity of effects of corticosteroids on acute respiratory distress syndrome: a systematic review and meta-analysis. Crit Care. 2014;18(2):R63.
Docampo E, Collado A, Escaramís G, Carbonell J, Rivera J, Vidal J, et al. Cluster analysis of clinical data identifies fibromyalgia subgroups. PLoS ONE. 2013;8(9):e74873.
Sutherland ER, Goleva E, King TS, Lehman E, Stevens AD, Jackson LP, et al. Cluster analysis of obesity and asthma phenotypes. PLoS ONE. 2012;7(5):e36631.
Guo Q, Lu X, Gao Y, Zhang J, Yan B, Su D, et al. Cluster analysis: a new approach for identification of underlying risk factors for coronary artery disease in essential hypertensive patients. Sci Rep. 2017;7:43965.
Hastings S, Oster S, Langella S, Kurc TM, Pan T, Catalyurek UV, et al. A grid-based image archival and analysis system. J Am Med Inform Assoc. 2005;12(3):286–95.
Celebi ME, Aslandogan YA, Bergstresser PR. Mining biomedical images with density-based clustering. In: International conference on information technology: coding and computing (ITCC’05), vol II. Washington, DC, USA: IEEE; 2005. https://doi.org/10.1109/ITCC.2005.196 .
Agrawal R, Imieliński T, Swami A, editors. Mining association rules between sets of items in large databases. In: Proceedings of the ACM SIGMOD conference on management of data. Washington, DC, USA: Association for Computing Machinery; 1993. p. 207–16. https://doi.org/10.1145/170035.170072 .
Sethi A, Mahajan P. Association rule mining: A review. TIJCSA. 2012;1(9):72–83.
Kotsiantis S, Kanellopoulos D. Association rules mining: a recent overview. GESTS Int Trans Comput Sci Eng. 2006;32(1):71–82.
Narvekar M, Syed SF. An optimized algorithm for association rule mining using FP tree. Procedia Computer Sci. 2015;45:101–10.
Verhein F. Frequent pattern growth (FP-growth) algorithm. Sydney: The University of Sydney; 2008. p. 1–16.
Li Q, Zhang Y, Kang H, Xin Y, Shi C. Mining association rules between stroke risk factors based on the Apriori algorithm. Technol Health Care. 2017;25(S1):197–205.
Guo A, Zhang W, Xu S. Exploring the treatment effect in diabetes patients using association rule mining. Int J Inf Pro Manage. 2016;7(3):1–9.
Pearson K. On lines and planes of closest fit to systems of points in space. Lond Edinb Dublin Philos Mag J Sci. 1901;2(11):559–72.
Hotelling H. Analysis of a complex of statistical variables into principal components. J Educ Psychol. 1933;24(6):417.
Jolliffe IT, Cadima J. Principal component analysis: a review and recent developments. Philos Trans A Math Phys Eng Sci. 2016;374(2065):20150202.
Zhang Z, Castelló A. Principal components analysis in clinical studies. Ann Transl Med. 2017;5(17):351.
Apio BRS, Mawa R, Lawoko S, Sharma KN. Socio-economic inequality in stunting among children aged 6–59 months in a Ugandan population based cross-sectional study. Am J Pediatri. 2019;5(3):125–32.
Burgel PR, Paillasseur JL, Caillaud D, Tillie-Leblond I, Chanez P, Escamilla R, et al. Clinical COPD phenotypes: a novel approach using principal component and cluster analyses. Eur Respir J. 2010;36(3):531–9.
Vogt W, Nagel D. Cluster analysis in diagnosis. Clin Chem. 1992;38(2):182–98.
Layeghian Javan S, Sepehri MM, Layeghian Javan M, Khatibi T. An intelligent warning model for early prediction of cardiac arrest in sepsis patients. Comput Methods Programs Biomed. 2019;178:47–58. https://doi.org/10.1016/j.cmpb.2019.06.010 .
Wu W, Yang J, Li D, Huang Q, Zhao F, Feng X, et al. Competitive risk analysis of prognosis in patients with cecum cancer: a population-based study. Cancer Control. 2021;28:1073274821989316. https://doi.org/10.1177/1073274821989316 .
Martínez Steele E, Popkin BM, Swinburn B, Monteiro CA. The share of ultra-processed foods and the overall nutritional quality of diets in the US: evidence from a nationally representative cross-sectional study. Popul Health Metr. 2017;15(1):6.
Download references
This study was supported by the National Social Science Foundation of China (No. 16BGL183).
Author information
Wen-Tao Wu and Yuan-Jie Li have contributed equally to this work
Authors and Affiliations
Department of Clinical Research, The First Affiliated Hospital of Jinan University, Tianhe District, 613 W. Huangpu Avenue, Guangzhou, 510632, Guangdong, China
Wen-Tao Wu, Ao-Zi Feng, Li Li, Tao Huang & Jun Lyu
School of Public Health, Xi’an Jiaotong University Health Science Center, Xi’an, 710061, Shaanxi, China
Department of Human Anatomy, Histology and Embryology, School of Basic Medical Sciences, Xi’an Jiaotong University Health Science Center, Xi’an, 710061, Shaanxi, China
Yuan-Jie Li
Department of Neurology, The First Affiliated Hospital of Jinan University, Tianhe District, 613 W. Huangpu Avenue, Guangzhou, 510632, Guangdong, China
You can also search for this author in PubMed Google Scholar
Contributions
WTW, YJL and JL designed the review. JL, AZF, TH, LL and ADX reviewed and criticized the original paper. All authors read and approved the final manuscript.
Corresponding authors
Correspondence to An-Ding Xu or Jun Lyu .
Ethics declarations
Ethics approval and consent to participate.
Not applicable.
Consent for publication
Competing interests.
The authors declare that they have no competing interests.
Rights and permissions
Open Access This article is licensed under a Creative Commons Attribution 4.0 International License, which permits use, sharing, adaptation, distribution and reproduction in any medium or format, as long as you give appropriate credit to the original author(s) and the source, provide a link to the Creative Commons licence, and indicate if changes were made. The images or other third party material in this article are included in the article's Creative Commons licence, unless indicated otherwise in a credit line to the material. If material is not included in the article's Creative Commons licence and your intended use is not permitted by statutory regulation or exceeds the permitted use, you will need to obtain permission directly from the copyright holder. To view a copy of this licence, visit http://creativecommons.org/licenses/by/4.0/ . The Creative Commons Public Domain Dedication waiver ( http://creativecommons.org/publicdomain/zero/1.0/ ) applies to the data made available in this article, unless otherwise stated in a credit line to the data.
Reprints and permissions
About this article
Cite this article.
Wu, WT., Li, YJ., Feng, AZ. et al. Data mining in clinical big data: the frequently used databases, steps, and methodological models. Military Med Res 8 , 44 (2021). https://doi.org/10.1186/s40779-021-00338-z
Download citation
Received : 24 January 2020
Accepted : 03 August 2021
Published : 11 August 2021
DOI : https://doi.org/10.1186/s40779-021-00338-z
Share this article
Anyone you share the following link with will be able to read this content:
Sorry, a shareable link is not currently available for this article.
Provided by the Springer Nature SharedIt content-sharing initiative
- Clinical big data
- Data mining
- Medical public database
Military Medical Research
ISSN: 2054-9369
- Submission enquiries: Access here and click Contact Us
- General enquiries: [email protected]

Healthcare Data Mining: Examples, Techniques & Benefits
Medical data mining is a set of data science methods and instruments used to generate evidence-based medical information that clinicians and scientists can trust.
Healthcare data mining techniques are used in many health-related areas, including biotech, pharmaceutical research, and medical science. The main health technologies and tech components involved in the clinical data mining process include…
- Connected EHR and EMR solutions
- Hospital information management systems
- ML and AI-driven data-mining systems
- Medical data visualization modules
- Advanced role-based admin panels
- External platform and tool integration.
How is data mining used in healthcare?
👨⚕️💻 Modern healthcare activities generate an enormous volume of medical information, which is usually captured and organized with the help of custom EHR & EMR systems ( electronic health/medical records ). This information includes…
- personal details (protected by HIPAA and similar regulations)
- patient biological parameters
- patient health conditions and diagnoses
- individual treatment plans
- health specifics, like allergies or chronic disease
- lab tests and scans
- treatment feedback
- …and so on.
When you accumulate this type of data for thousands of patients and cases, it is called medical Big Data, and it is a real treasure chest for healthcare researchers!
Requirements: Data mining in healthcare is a resource-intensive process that requires considerable computing and data-warehousing capacity, developed mathematical skills, and data science specialists. Moreover, Big Data and data mining in healthcare are closely interrelated, and their combined use can bring exceptional benefits to the industry. 📊🚀
Objectives: Why should we mine healthcare data? Clinical data mining in healthcare databases helps medical scientists and experts reveal data patterns, trends, associations, and other fact correlations enabling them to formulate…
- Important observations and conclusions
- Healthcare issues and strategies for prevention
- Pattern-based predictions and complex forecasting
- Data artifacts, abnormalities, and phenomena in medical practice
- Best algorithms and recommendations for a wide range of healthcare situations.
Is healthcare data mining a new practice?
Methods for data mining and analysis of healthcare data have been known for years; however, they were only accessible to a narrow circle of medical statisticians, clinical scientists, and healthcare experts because of numerous formal barriers and practical complications.
Nowadays, since informational technologies (including personal workstations) are adopted in every doctor’s office or ER, clinical data mining is no longer a rare or privileged practice. See the table below for a detailed explanation of this statement…
Before we delve into more detail of data mining processes for healthcare management and improvement, read more about our team and capabilities: 👇
Table of Contents
Why Data Mining Is So Important in Healthcare
Healthcare data-mining applications can offer a wide spectrum of benefits. These advantages boost every sphere of activities and operations in medical facilities to make patient service faster, more cost-efficient, and safer.
Let’s learn more about the benefits of data mining in healthcare…
⚕️ Evidence-based decision-making in clinical settings
We are seeing a booming practice of building clinical decision support systems (CDSS) for medical organizations and facilities that want to support their decisions with sophisticated evidence. These systems can include a combination of data-mining applications in healthcare operations: for example, EMR/EHR database + connected medical Big Data management platforms + a set of integrated modules (like an admin panel with medical data graphs and charts , medical personnel access portals, and more).
With the help of this complex tool, physicians can…
- Compare symptoms across customized groups of patients with similar attributes and identify the most probable scenarios of treatment/outcomes.
- Get digital assistance with interpretation, diagnosis, and treatment of both unusual and typical patients based upon previous medical experience.
- Shape a consistent patient safety environment in which medical decision-makers are provided with alerts, reminders, prompts, and health recommendations in the context of each individual patient.
- Conduct quick clinical research and dive deeper into healthcare service history and your patient base.
- Make use of predictive analysis in medical practice: for example, predict possible health complications and outcomes in a patient in relation to certain conditions, their personal medical history, and individualized treatment scenarios.

Slava Khristich
Healthtech cto.
Based in San Diego, Slava knows how to design an efficient software solution for healthcare, including IoT, Cloud, and embedded systems.
📝 Increased Accuracy of Diagnosis and Treatment Plans
There are many ways in which medical data-mining tools can improve daily healthcare. More sophisticated solutions with AI or machine-learning components can help physicians (even highly qualified ones) to process tests, X-rays, MRI images, and other materials faster. They can also detect subtle details to identify a different diagnosis or previously undiscovered concomitant disease in a patient.
This allows care providers to avoid clinical mistakes and lets patients receive more accurate treatment plans and scenarios based on evidence previously unspotted by specialists.
💊 Avoiding Harmful Drug Interactions
The FDA has approved more than 20K prescription medications for distribution in the U.S. With the huge number of prescription drugs available, a patient can get several different courses of medication at one time, which sets them up for interactions between drugs and potential consequences.
Sometimes, patients can be allergic to or intolerant of specific chemicals generated by combinations of drugs. Computer-assisted data mining can help physicians and pharmacists decide on prescriptions and prevent dangerous combinations of drugs based on digital drug and food interaction modeling and patient health factors.
⚠️ Detection of Insurance Fraud in Healthcare
We are all human, and our world is still far from perfect. Fraud involving insurance claims happens frequently, to the tune of many billions of dollars annually, according to the FBI . With the help of clinical data-mining techniques and associated software solutions, specialists can automatically detect inconsistent data or suspicious signs and patterns in health insurance claims to prevent or reject fabricated claim submissions.
In this way, medical data mining can potentially save huge financial resources that can be otherwise assigned to better purposes. A fraud detection system can also become an integral part of a custom health insurance application .
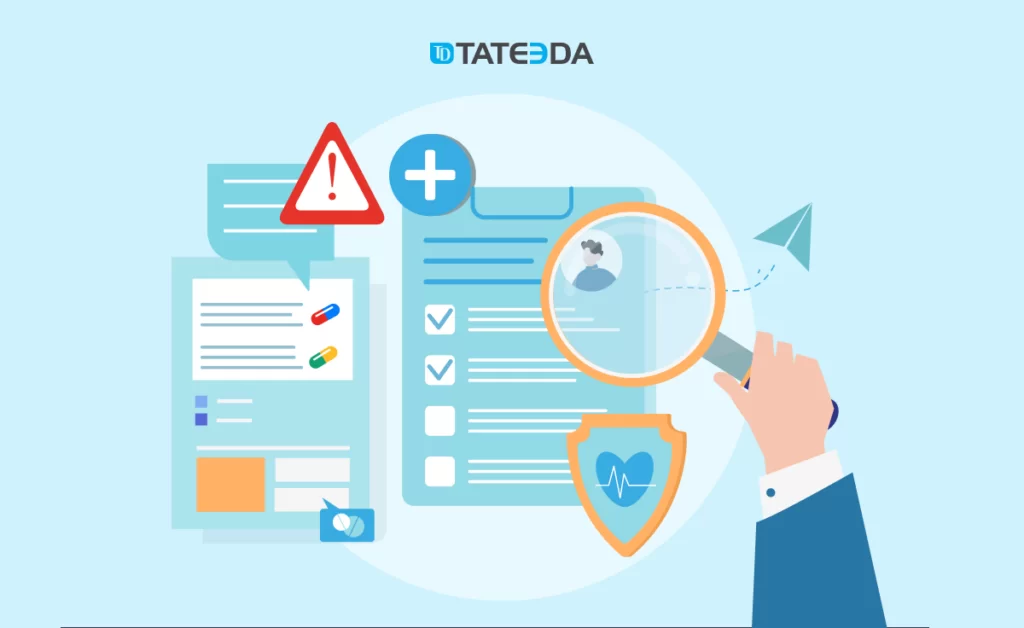
How Data Mining Works in the Healthcare Sector
📈 ⛏️ There exist several types of data mining objectives in healthcare. For example, we can distinguish between descriptive and predictive models in data mining for medical activities. The first type of research ( descriptive ) focuses on capturing and interpreting the current state of affairs, while the second ( predictive ) concentrates on medical forecasting based on typical patterns of events and previous interpretations.
The two models can be combined. Neural networks with self-learning abilities can scan medical Big Data and be trained to recognize typical or as-yet-unnoticed/uninterpreted data compositions like patterns, trends, associations, or clusters. You can find a number of Big Data and EHR/EMR platforms providing data-mining tools for healthcare companies and organizations.
When you have all the required steps in place, clinical data mining goes through the following stages:
- Data selection and acquisition : In this stage, parameters and healthcare datasets for data mining are identified within the whole array of medical data—usually an EHR/EMR database, aggregated documents, healthcare ERP systems , or specific types, like Cardiology EHR or Mental Health EMR .
- Data preprocessing and transformation : At this step, data is properly formatted and normalized in order to clean up invalid records and prepare data objects according to predefined methods and standards.
- Data-mining process : Data-mining algorithms and techniques with predefined parameters are applied to the medical database to derive conclusions and knowledge.
- Data interpretation : After specific data products are extracted from available data arrays with the help of selected data-mining methods, it’s time to interpret the results and formulate valuable insights.

Do you need IT consulting services on hospital data-mining system development or implementation?
Custom healthcare solutions.
See how we can engineer healthcare software, validate your ideas, and manage project costs for you.

Data Mining Techniques in Healthcare
Hospital data-mining techniques can be combined to obtain precise results, providing you with a vast array of options. Only an experienced data-mining expert can identify the best strategy or best set of methods to fulfill specific goals in your healthcare organization. Here is a brief explanation of some popular data-mining techniques in medical research…
Association or relationship analysis 🔀
This healthcare data-mining technique is focused on finding associations and relations between groups of specific events, facts, or attributes in the database ( data patterns ). It aims to discover and study logical links between these events, objects, and datasets while identifying the ways in which these associations can be interpreted and extrapolated.
For example, if a group of patients with specific symptoms is steadily associated with certain prescribed medications they acquire in pharmacies during a preset season , pharmacists can use this information to manage their stock.
Sequence analysis 🔄
This technique also helps analyze another type of pattern: consequential flows of facts or events. One can then study the logic and interrelationships between the steps.
For example, data mining of multiple patient records can identify that increased occurrence of certain symptoms or patient complaints precedes the development or diagnosis of specific ailments within a period of time . These conclusions can be deepened with the help of association analysis to discover additional data dependencies: for example, all patients of the group also share similar lifestyles, chronic diseases, or other health features. With this knowledge, physicians can offer preventive care.
Classification 📁
This method of medical data mining includes classifying datasets or complex data objects (a patient, case, etc.) into categories (for example, “COVID-19” and “bird flu” classes). Cases can be compared with each other to be verified as falling within a certain class, to identify differences and apply necessary algorithms and protocols, or to screen out and readdress unmatching data.
For example , suppose we have a sufficient number of patient cases whose symptoms and other parameters match up to 90% or more. In that case, we can be pretty sure we’re dealing with the same disease, and it can be treated according to a standardized protocol . If the degree of matching is lower, the case falls out of the class, and additional research or reclassification is required.

Learn more: ➡️ Healthcare Payroll Software Development
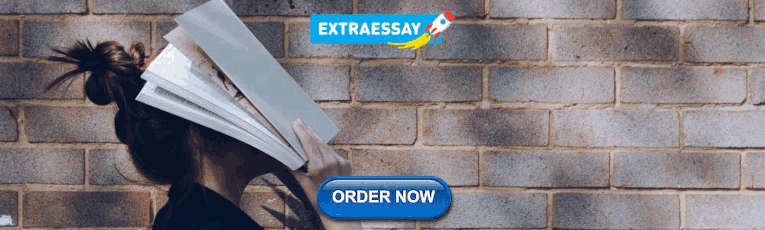
Visualization 📈
Building different charts and graphs, such as Gantt charts, pie charts, bubble charts, treemaps, scatter plots, density maps, and more helps physicians, medical administrators, and scholars identify trends, patterns, spikes, and declines in certain healthcare parameters or events. Some visualization types can be used together with other data mining methods like logic trees or block diagrams to depict associations and/or relationships between events and datasets. Visualizations can be executed with the help of specific tools implemented in healthcare mobile applications, medical admin panels for EHR or ERP systems, and more. Here’s an example of how the health data visual representation method is used in data mining: this graph shows a comprehensive relationship between certain medical actions and their consequences , which provides physicians and epidemiologists with the evidence they require…

Clustering 🟢🟠
One data mining method usually carried out with the help of a visualization technique is a cluster chart. Here, data points are grouped according to their parameters and statistical distribution. Once multiple data points are put on a graph, they appear clustered in dense neighborhoods falling within certain borders. In this way, they can be interpreted as being a part of one class. Clustering is a method that is usually used for classification in healthcare.
Forecasting and predicting 🔮
There are several methods of forecasting in medical data mining. Some are pretty simple, like presuming that a certain data pattern will perpetuate itself into the future; other models are more complicated, and are supported by AI or machine learning. This includes testing multiple hypotheses by running cascades of statistical examinations and emulating multiple scenarios. Machine-supported predictions require integration of your EHR or EMR system (or any other Big Data repository you use) with a third-party platform offering data-mining and forecasting tools. Do not forget about healthcare IT interoperability standards and technologies to be established between different systems.
Do you want to make use of healthcare data mining applications?
Delivered healthcare software portfolio.
The leading American healthcare companies benefit from working with us.

Data Mining in Healthcare: Examples
How can you make use of data mining in healthcare? There exist myriad ways to adopt and leverage this practice in hospitals, public medical centers, and private clinics. When authorized medical experts use digital data mining techniques in healthcare, they obtain valuable insights in a matter of seconds without lengthy research or daunting calculations. Let’s take a look at a few healthcare data mining examples:
😷 Epidemiology patterns: discovery and prognosis
Epidemics and pandemics are not rare these days. With the help of medical data mining, doctors and other healthcare specialists can…
- Monitor the number of disease occurrences and see how an epidemic or disease outbreak scales over a period of time.
- Mark considerable fluctuations in correlation with seasons, location, selected patient groups (i.e., gender, age, health features), and other datasets.
- Predict changes in the epidemiologic situation and manage response according to prior experience and/or educated expectations.
- Study hidden trends, relationships, or patterns in epidemiological situations.
- Research and predict the spread of disease in terms of expected timelines, affected areas, risks, potential numbers of severe vs. mild cases, and epidemic endpoints.

Learn more: ➡️ Cloud Computing in Healthcare: 3 Use Cases, Benefits, Features & Best Practices
🌡️ Personalized disease course and treatment forecast
When it comes to individualized patient care, with clinical data mining, it’s easy to…
- Classify patients into separate groups and identify the most frequent and/or severe symptoms and complaints relevant to every specific group.
- Identify the most successful treatment protocols and helpful approaches in the context of every patient group.
- Correlate health events (like a chronic disease) with the frequency of specific symptoms and their severity.
- Forecast the development of a disease in an individual patient based upon their health conditions and medical background.
- Find and study new and significant relationships between health conditions, symptoms, treatment plans, medical methods, and outcomes.
🤖 Medical knowledge research and automatic diagnostics
When you cater sufficient medical data to AI or machine learning algorithms, this sophisticated software can be trained to automatically…
- Discover and report all possible relationships between facts in the healthcare database while identifying interesting phenomena and developing valuable conclusions.
- Recognize the most probable diagnoses in a specific patient and offer physicians individualized treatment approaches and recommendations.
- Process MRI scans in bulk to mine visual and technical data barely noticed by human physicians and help them quickly detect even the slightest signs of disease.
- Research DNA data on tumor segmentation and sequencing, and execute other DNA-related medical examinations and/or scientific studies.
- Suggest personalized patient insurance plans and custom health policies based on health risk.
- Suggest new approaches and tweak existing healthcare plans and medical protocols to increase treatment efficiency and bring better outcomes.

Learn more: ➡️ How to Build a Lab Information Management System (LIMS)
🏥 Pharmacy and hospital management insights
What are examples of data mining for healthcare management? Smart planning, tracking, and forecasting of assets: Hospital resources and pharmacy stock-management applications can be enhanced with the help of data mining, which offers the following capabilities…
- Identify seasonal spikes or declines in patient symptoms and drug prescriptions.
- Dig into a pharmacy’s CRM system or Hospital Information Management System (HIMS) to classify, cluster, visualize, and analyze current customer data.
- Predict future demand for beds, medication stock, workforce, and various resources found in hospitals, pharmacies, and other medical institutions.
- Use accurate insights to manage pharmacy stock and hospital beds to be reserved prior to seasonal disease outbreaks.
- Correlate seasonal epidemics and environmental changes with the way in which risk is spread among different patient groups.
- Integrate data-mining tools into hospital management apps to provide clinical staff with access to medical evidence-supported insights.
- Derive patient insights and reports from custom healthcare CRM systems to identify new marketing opportunities and deliver tailored offerings through cross-selling channels.
🍔 Dietary pattern exploration
The potential influence of dietary and nutritional habits remains largely unexplored. Studies suggest that certain products can lead to the development of chronic diseases and even cancer. Clinical data mining can help us find out.
- Dig into your database to find the right patients and form focus groups of patients to be supervised under dietary pattern research in your medical organization.
- Control their meals and collect data. Once a sufficient volume of data is accumulated, it can be mined by data analysts to discover relationships and patterns.
- Employ self-reporting apps for patients. People can report their meals and access nutrition plans or suggestions from the clinic, which can follow them and provide notifications..

TATEEDA GLOBAL: Our Custom Project with Data Mining Components
TATEEDA GLOBAL has been involved in a number of custom software development projects for pharma businesses and partners. For example, we built crucial online medication fulfillment features for a major pharma distribution company. The solution included automated processing of pharmacy insurance claims. Our client demanded the development of data-mining functionalities with specific algorithms analyzing their database of pharma products and operations and providing results in PDF reports and spreadsheets. This data-mining module helped them improve the results of their trade activities. Learn more: ➡️ Custom Online Medication-fulfillment System Development .

What are the prospects for data mining in the healthcare sector?
Data-mining methods and techniques will contribute further breakthroughs in medical science and practice, providing a foundation for evidence-based change and research in healthcare. It is recommended that all medical specialists benefit from this approach.
How is data mining changing healthcare?
Data mining helps physicians get an overview of healthcare activities and improve their interpretation of results and current situations in terms of numbers. As stated earlier, data mining allows them to make educated (backed by evidence and calculations) decisions and conclusions, so it’s recommended to provide medical specialists with role-based access to EHR/EMR systems with data-mining panels and toolboxes.
How can data mining improve healthcare business performance?
Data mining is a reliable instrument of operational management in all types of healthcare companies and organizations. With data-mining modules integrated into your patient and business process databases (patient CRM, hospital system, EHR, etc.) you can analyze the data in a variety of ways and obtain conclusions on the efficiency of your work and available opportunities.f
How can you integrate data mining into your healthcare solution?
This can be done in a variety of ways…
- Build a custom data-mining application or software module connected to your medical data database.
- Use a third-party solution provider with excellent data-mining functionality (this approach still requires a software engineer who can customize and integrate this component.)
- Seek the acquisition of an additional module or improved functionality from your current EHR/EMR provider, if available.
How should you hire a team with experience in data-mining algorithms?
Consider TATEEDA GLOBAL. We have experienced software development engineers and coders who can help you develop a custom solution for data mining. If you want to learn more about our expertise and skills, please contact us for a free consultation ! We will help you identify the best solution for your situation.
Rate this article!

CTO at TATEEDA GLOBAL
Expert in IT Staff Augmentation Services and Healthtech projects. Contact me for a free consultation!
View 60 more posts

BOOk a Consultation
with our Senior Healthcare Solutions Architect
Let's build something great together!
Why choose tateeda.
We treat our clients and our own team members like family. We build relationships with our clients based on trust and loyalty.
We are industry experts. Most of our team members are senior software engineers.
We deliver quality software, on spec and on time . We follow through on our promises to our clients.
We continue to learn and grow as professionals. We are better today than we were yesterday, and tomorrow we will be better still.
We will contact you within one business day
Related Posts

Top-17 Healthcare Technology Trends in 2024

Virtual Nurse App Development Guide: How to Create Custom Health Assistant Software

How to Develop Hospital Management Software in 2024: Features, Modules, and Concepts
Our Latest Posts

The Top 7 IT Staff Augmentation Companies for the U.S. Market

IT Outsourcing Trends 2024: Expectations and Challenges

How to Hire Offshore Developers in 2024: Avoid Common Mistakes in Remote IT Outsourcing
Contact us to start
We normally respond within 24 hours
If you need immediate attention, please give us a call at 619-630-7568
Use our free estimator to find out your approximate cost.
GET A FREE QUOTE

Dave Churchville
Principal, ventrilink.
TATEEDA helped us get some key projects finished on time when our internal team was already at capacity. They gave us a way to do more without needing to add more staff or deal with more management overhead by handling the day to day details. If you’re looking for flexible and cost effective development resources that can work with your existing team, I’d highly recommend TATEEDA.

Sal Saldivar
Cto, la maestra community health centers, san diego, california, us.
TATEEDA had a very methodical approach in helping up develop our mobile app. Besides just developing the software, it required managing my team (as the customer) to provide the required information and decision making. TATEEDA’s always had our best interest in mind and made sure we have a realistic expectation.

We have 100+ in-house developers in the U.S.A., Ukraine, Poland, Brazil, Colombia.
Privacy Overview
Implementation of Data Mining Techniques in Healthcare
Ieee account.
- Change Username/Password
- Update Address
Purchase Details
- Payment Options
- Order History
- View Purchased Documents
Profile Information
- Communications Preferences
- Profession and Education
- Technical Interests
- US & Canada: +1 800 678 4333
- Worldwide: +1 732 981 0060
- Contact & Support
- About IEEE Xplore
- Accessibility
- Terms of Use
- Nondiscrimination Policy
- Privacy & Opting Out of Cookies
A not-for-profit organization, IEEE is the world's largest technical professional organization dedicated to advancing technology for the benefit of humanity. © Copyright 2024 IEEE - All rights reserved. Use of this web site signifies your agreement to the terms and conditions.
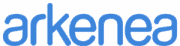
Data Mining in Healthcare: Techniques, Process, and Benefits
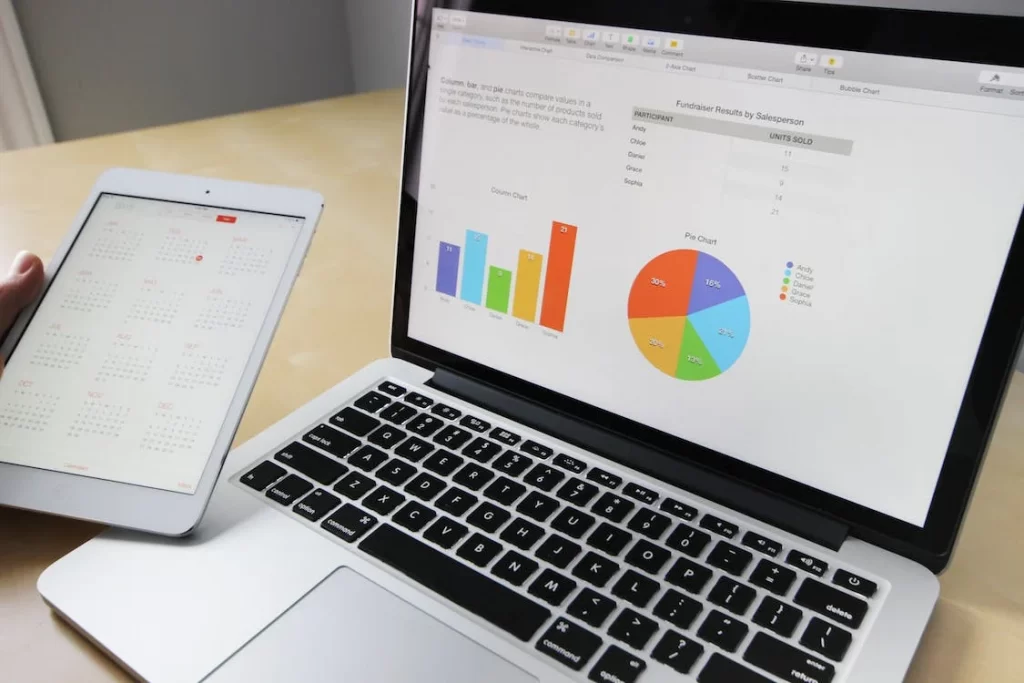
Data mining involves collecting, sorting, searching, and analyzing raw data to extract useful information. The data mining process identifies patterns, trends, and relationships between data. In healthcare, data mining is used for fraud detection, clinical decision-making, treatment, diagnosis, and more.
Healthcare data mining includes techniques such as clustering, classification, or regression analysis, and these techniques help to scrutinize information. Furthermore, the data mining market is predicted to reach $1.03 billion by 2023 at a CAGR of 11.9 percent during the 2018 to 2023 forecast period . This article offers a comprehensive lookout for healthcare data mining, including benefits, techniques, and processes.
Techniques of Data Mining in Healthcare
Data mining encompasses a variety of techniques to extract valuable insights from large datasets. These techniques are as follows:
- Clustering: Grouping similar data points to identify patterns and relationships within the data. Examples: population health management, chronic disease prevention, and identifying fatal diseases before their onset.
- Classification: Categorizing data into predefined classes or labels based on their features. Classification plays a vital role in segregating medical files and documents, making it easier for doctors to navigate through records.
- Association Rule Mining: Discovering relationships or associations between variables in the dataset. In healthcare this method can be used to find the impact of one disease over the other, or one medical situation over the other. For instance, impact of obesity on cardiovascular health or how exercise affects mental health.
- Regression Analysis: Predicting numeric values based on the relationships between variables. This method helps to comprehend the impact of one variable on another. This is a bit similar to the association rule mining technique.
- Anomaly Detection: Detecting unusual instances in the data deviating from the norm and this technique is useful for fraud detection and quality control. For example, anomaly detection and spot fraudulent messages sent to doctors via patient portals or healthcare apps and it can also detect anomalies in payments.
- Text Mining: Extracting meaningful information from text data, including sentiment analysis, topic modeling, and document categorization. Text mining makes use of NLP (Natural Language Processing) to extract useful data. Physicians can use this method to take notes in real time.
- Time Series Analysis: Scrutinizing data points ordered by time to uncover patterns and trends. Example: predicting epidemics and pandemics, to take preventive action against them.
- Neural Networks: Deep learning models that can discover complex patterns and relationships in data, commonly used in image and speech recognition. Radiologists use this method because it can examine images in bulk, thereby saving ample time.
- Decision Trees: Hierarchical structures that aid in decision-making by mapping out possible outcomes based on input variables. Through decision trees method, physicians can arrive at a conclusion based on diagnosis and medical history.
- Dimensionality Reduction: Reducing the number of features in a dataset while preserving important information, improving processing efficiency, and reducing noise.
- Ensemble Methods: The ensemble method combines the results of multiple models and enhances the prediction accuracy.
- Sequential Pattern Mining: Identifying sequential patterns in data, often used in analyzing patient behaviors over time.
- Collaborative Filtering: Recommending items to users based on the preferences and behaviors of similar users. For example, patient referrals.
These techniques, among others, contribute to the versatility and power of data mining in uncovering valuable insights from diverse datasets.
Process of Data Mining in Healthcare
The process of data mining in healthcare involves several key stages, each contributing to the extraction of valuable insights from medical data. Here’s an overview of the process:
- Problem Definition and Goal Setting: Identify the specific healthcare problem or question you want to address using data mining techniques. Define clear objectives and outcomes you aim to achieve.
- Data Collection: Gather relevant healthcare data from various sources, such as electronic health records (EHRs), medical devices, clinical trials, and patient surveys. This data can include patient demographics, medical history, test results, and treatment records.
- Data Preprocessing: P reprocess the collected data to ensure its quality and consistency. This step involves handling missing values, removing outliers, and standardizing data formats.
- Data Integration: If working with data from multiple sources, integrate and combine datasets to create a unified and comprehensive dataset for analysis.
- Feature Selection/Extraction: Identify the relevant variables (features) that will be used for analysis. This step may involve selecting important features or transforming the data to extract meaningful patterns.
- Data Transformation: Convert data into a suitable format for analysis. This could involve scaling numerical data, encoding categorical variables, and normalizing data distributions.
- Data Mining Algorithms Selection: Choose appropriate data mining algorithms based on the nature of the problem and the goals. Common algorithms include decision trees, neural networks, clustering algorithms, and association rule mining.
- Model Building: Apply selected algorithms to the preprocessed and transformed data to build predictive or descriptive models. For instance, predictive models might help forecast disease outcomes, while descriptive models might reveal patterns in patient populations.
- Model Evaluation: Assess the performance of the data mining models using relevant evaluation metrics. This helps ensure the models are accurate and effective in addressing the healthcare problem.
- Interpretation of Results: Analyze the patterns, trends, and insights obtained from the models. Understand the implications of the findings for medical decision-making and patient care.
- Deployment: Implement the insights and models into clinical practice or clinical decision-making processes. This might involve creating tools for healthcare professionals to use, integrating findings into electronic health records, or developing predictive models for disease prevention .
- Monitoring and Iteration: Continuously monitor the performance and effectiveness of the deployed models. Update and refine the models as new data becomes available or as the healthcare landscape evolves.
It’s essential to consider ethical and privacy considerations throughout the process, as healthcare data often contains sensitive patient information. Compliance with regulations like HIPAA (Health Insurance Portability and Accountability Act) is crucial to ensure patient privacy and data security.
By following these steps, data mining in healthcare can lead to improved patient outcomes, personalized treatment plans, disease prevention strategies, and enhanced healthcare delivery.
Importance of Data Mining in Healthcare
Data mining plays a crucial role in healthcare by extracting valuable insights from large and complex medical datasets, contributing to improved patient care, research advancements, and healthcare system efficiency. Its importance is evident in several key areas:
- Clinical Decision-Making: Data mining helps healthcare practitioners make more informed decisions by identifying patterns and correlations in patient data. This helps in accurate diagnosis, treatment selection, and personalized patient care.
- Disease Detection and Prevention: Data mining can detect early signs of diseases, enabling timely intervention and prevention. It’s particularly valuable in predicting outbreaks, tracking disease progression, and identifying high-risk populations.
- Patient Risk Assessment: Data mining assists in assessing patient risk factors and predicting adverse events, such as hospital readmissions. This allows healthcare providers to allocate resources effectively and proactively address potential complications.
- Drug Discovery and Development: By scrutinizing molecular and genetic data, data mining accelerates drug discovery by identifying potential drug candidates and predicting their effectiveness.
- Genomic Analysis: Data mining aids in understanding the genetic basis of diseases, identifying genetic markers, and guiding personalized treatments based on an individual’s genetic makeup.
- Healthcare Fraud Detection: Data mining helps identify fraudulent activities in billing and insurance claims, ensuring that resources are used efficiently and fraud is minimized.
- Public Health Surveillance: Monitoring and analyzing healthcare data in real-time supports public health surveillance efforts, enabling early detection of disease outbreaks and effective response planning.
- Clinical Research: Data mining helps researchers uncover insights from clinical trials, patient records, and research databases, leading to the discovery of new treatments and medical knowledge.
- Personalized Medicine: Data mining tailors treatment plans to individual patient characteristics, optimizing outcomes and reducing adverse effects by considering factors like genetics, lifestyle, and medical history.
- Operational Efficiency: Healthcare organizations use data mining to optimize resource allocation, improve patient flow, and enhance operational processes, resulting in cost savings and improved patient experiences.
- Healthcare Management: Analyzing administrative data helps healthcare administrators make strategic decisions, allocate resources, and plan for future healthcare needs.
- Patient Engagement: Data mining can identify patient preferences and behaviors, helping providers deliver personalized communication and care plans, thus improving patient engagement and satisfaction.
- Predictive Analytics: By forecasting patient needs and resource demands, data mining enhances healthcare system preparedness and resource allocation.
In essence, data mining transforms healthcare data into actionable insights, driving evidence-based decision-making, patient-centric care, and research breakthroughs. As technology advances and healthcare generates more data, data mining continues to evolve, enabling the healthcare industry to harness its full potential for the benefit of patients and society as a whole.
Arkenea is one of the leading healthcare software development companies that also specializes in AI. We offer a range of solutions from chatbots to predictive modeling and deliver top-notch products to our clients. If you’re looking for something similar for your healthcare organization, then connect with Arkenea.
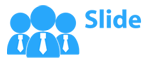
Powerpoint Templates
Icon Bundle
Kpi Dashboard
Professional
Business Plans
Swot Analysis
Gantt Chart
Business Proposal
Marketing Plan
Project Management
Business Case
Business Model
Cyber Security
Business PPT
Digital Marketing
Digital Transformation
Human Resources
Product Management
Artificial Intelligence
Company Profile
Acknowledgement PPT
PPT Presentation
Reports Brochures
One Page Pitch
Interview PPT
All Categories

Data Mining Techniques Used In Healthcare
The following slide enumerates data mining techniques used in healthcare industry to identify effective treatments and best practices for patients.This covers four methods such as classification, clustering, association and outlier detection for transforming huge data into useful information.
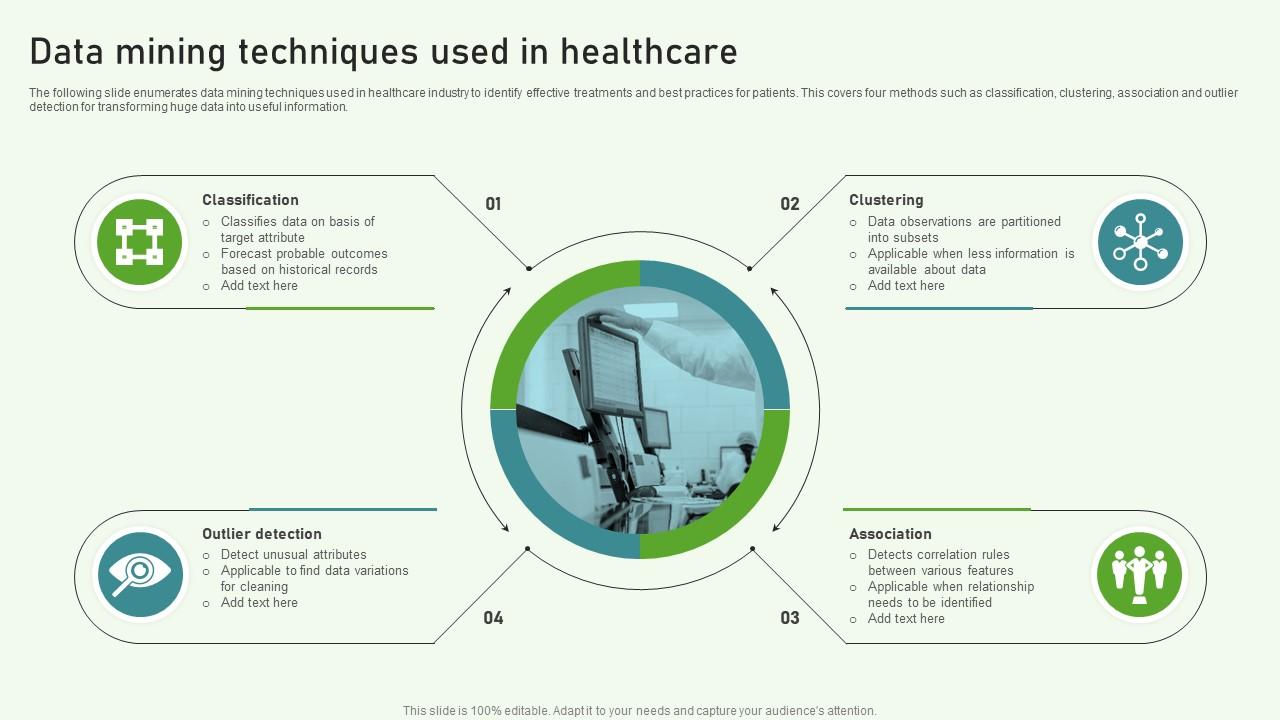
These PPT Slides are compatible with Google Slides
Compatible With Google Slides
- Google Slides is a new FREE Presentation software from Google.
- All our content is 100% compatible with Google Slides.
- Just download our designs, and upload them to Google Slides and they will work automatically.
- Amaze your audience with SlideTeam and Google Slides.

Want Changes to This PPT Slide? Check out our Presentation Design Services

Get Presentation Slides in WideScreen
Get This In WideScreen
- WideScreen Aspect ratio is becoming a very popular format. When you download this product, the downloaded ZIP will contain this product in both standard and widescreen format.

- Some older products that we have may only be in standard format, but they can easily be converted to widescreen.
- To do this, please open the SlideTeam product in Powerpoint, and go to
- Design ( On the top bar) -> Page Setup -> and select "On-screen Show (16:9)” in the drop down for "Slides Sized for".
- The slide or theme will change to widescreen, and all graphics will adjust automatically. You can similarly convert our content to any other desired screen aspect ratio.
- Add a user to your subscription for free
You must be logged in to download this presentation.
Do you want to remove this product from your favourites?
PowerPoint presentation slides
The following slide enumerates data mining techniques used in healthcare industry to identify effective treatments and best practices for patients. This covers four methods such as classification, clustering, association and outlier detection for transforming huge data into useful information. Introducing our premium set of slides with Data Mining Techniques Used In Healthcare. Ellicudate the four stages and present information using this PPT slide. This is a completely adaptable PowerPoint template design that can be used to interpret topics like Classification, Outlier Detection, Association. So download instantly and tailor it with your information.
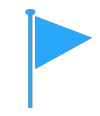
People who downloaded this PowerPoint presentation also viewed the following :
- Diagrams , Business , Strategy , Icons , Business Slides , Flat Designs , Technology and Communication , Software Development
- Classification ,
- Outlier Detection ,
- Association
Data Mining Techniques Used In Healthcare with all 6 slides:
Use our Data Mining Techniques Used In Healthcare to effectively help you save your valuable time. They are readymade to fit into any presentation structure.
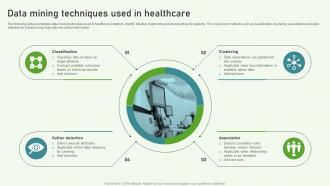
Ratings and Reviews
by Denver Fox
April 20, 2023
by John Walker

Smart Healthcare Support Using Data Mining and Machine Learning
- First Online: 31 May 2022
Cite this chapter
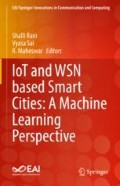
- Theodora Chatzinikolaou 5 ,
- Eleni Vogiatzi 5 ,
- Anestis Kousis 5 &
- Christos Tjortjis ORCID: orcid.org/0000-0001-8263-9024 5
Part of the book series: EAI/Springer Innovations in Communication and Computing ((EAISICC))
402 Accesses
8 Citations
Ever since the first cities were created, they have been dependent on technology to sustain life. The smart city paradigm integrates advanced monitoring, sensing, communication, and control technologies, aiming at providing real-time, interactive, and intelligent city services to citizens. Thus, the healthcare sector, as an essential part of our lives, could not remain unaffected. The advances in technology provided great potential for many aspects of the health system. In this chapter, we focus on popular machine learning (ML) and data mining (DM) predictive and descriptive techniques and their most prominent applications in the healthcare domain. First, we introduce the process of mining data to extract healthcare relevant knowledge. We briefly review key techniques used, including classification, clustering, and association rule mining. We then focus on specific smart healthcare applications including but not limited to (i) assisting diagnosis and treatment, (ii) health management, (iii) disease prevention and risk monitoring, (iv) virtual assistant and wearable sensors, and (v) drug research. The chapter concludes with a running example of applying well-known ML and DM techniques to a publicly available dataset related to diabetes and a discussion on the impact of DM in healthcare support.
- Smart cities
- Data mining (DM)
- Machine learning
This is a preview of subscription content, log in via an institution to check access.
Access this chapter
- Available as PDF
- Read on any device
- Instant download
- Own it forever
- Available as EPUB and PDF
- Compact, lightweight edition
- Dispatched in 3 to 5 business days
- Free shipping worldwide - see info
- Durable hardcover edition
Tax calculation will be finalised at checkout
Purchases are for personal use only
Institutional subscriptions
https://www.ibm.com/watson
https://www.cs.waikato.ac.nz/ml/weka/
A.M. Townsend, Smart cities: big data, civic hackers, and the Quest for a New Utopia (W.W. Norton & Company, New York, 2013)
Google Scholar
M. Bermudez-Edo, P. Barnaghi, K. Moessner, Analysing real world data streams with spatio-temporal correlations: entropy vs. pearson correlation. Automation in Construction 88 , 87–100 (2018)
Article Google Scholar
Q. Le-Dang, T. Le-Ngog, Internet of Things (IoT) Infrastructures for Smart Cities, in Handbook of Smart Cities: Software Services and Cyber Infrastructure , (Cham, Springer, 2018), pp. 1–30
P. Anatharam, P. Barnaghi, K. Thirunarayan, A. Sheth, Extracting city traffic events from social streams. ACM Trans. Intell. Syst. Technol. 6 (4), 43.:1-43:27, (2015)
A.K. Kar, S.Z. Mustafa, M.P. Gupta, P.V. Ilavarasan, Y.K. Dwivedi, Understanding Smart Cities: Inputs for Research and Practice, in Advances in Smart Cities: Smarter People, Governence, and Solutions , (CRC Press, Boca Ralton, 2017), p. 1
Chapter Google Scholar
A. Yassine, S. Singh, A. Alamri, Mining human activity patterns from smart home big data for health care applications. IEEE Access 5 , 13131–13141 (2017)
B. Liu, K. He, G. Zhi, The impact of big data and artificial intelligence on the future medical model. Med. Philos. 39 (22), 1–4. (in Chinese), (2018)
S. Tian, W. Yang, J.M.L. Grange, P. Wang, W. Huang, Z. Ye, Smart healthcare: making medical care more intelligent. Glob. Health J. 3 , 62–65 (2019)
K. Kasikumar, M.M. Najumuddeen, R. Suresh, Applications of data mining techniques in healthcare and prediction of heart attacks. Int. J. Data Min. Tech. Appl. 7 , 172–176 (2018)
G.R. Pooja, M.B. Trinath, K. Vasanthi, K.S. Ramireddy, R.K. Tenali, Smart E-health prediction system using data mining. Int. J. Innov. Technol. Explor. Eng 8 (6), 787–791 (2019)
D.K. Singh, M. Ashraf, An experimental approach for prediction of disease in smart health system using data mining technique. Int. J. Adv. Sci. Technol. 27 , 112–119 (2019). Retrieved from http://sersc.org/journals/index.php/IJAST/article/view/102
B. Kantarci, K.G. Carr, C.D. Pearsall, SONATA: Social Network Assisted Trustworthiness Assurance in Smart City Crowdsensing, in The Internet of Things: Breakthroughs in Research and Practice , (Hershey, IGI Global, 2017), pp. 278–299
J.A. Rodriguez, F.J. Fernadez, P. Arboleya, Study of the architecture of a smart city. Proceedings 2 , 1–5 (2018)
P. Lombardi, S. Giordano, Evaluating the Smart and Sustainable Built Environment in Urban Planning, in Handbook of Research on Social, Economic, and Environmental Sustainability in the Development of Smart Cities , (Hershey, IGI Global, 2015), pp. 44–59
H. Habibzadeh, A. Boggio-Dandry, Z. Qin, T. Soyata, B. Kantarci, H. Mouftah, Soft sensing in smart cities: handling 3Vs using recommender systems, machine intelligence, and data analytics. IEEE Commun. Mag. 56 , 78–86 (2018)
A. Souza, M. Figueredo, N. Cacho, D. Araujo, C.A. Prolo, Using big data and real-time analytics to support smart city initiatives. IFAC-Papers Online 49 (30), 257–262 (2016)
P. Koukaras, C. Tjortjis, D. Roussidis, Social media types: introducing a data driven taxonomy. Computing 102 (1), 295–340 (2020)
A. Mystakidis, C. Tjortjis, Big Data Mining for Smart Cities: Predicting Traffic Congestion using Classification, in The 11th International Conference on Information, Intelligence, Systems and Applications, Piraeus, 2020
S. Mohanty, U. Choppali, E. Kougianos, Everything you wanted to know about smart cities: the Internet of Things is the backbone. IEEE Consum. Electron. Mag. 5 (3), 60–70 (2016)
M.V. Moreno, F. Terroso-Saenz, A. Gonzalez-Vidal, M. Vldez-Vela, A. Skarmeta, M.A. Zamora, V. Chang, Applicability of big data techniques to smart cities deployments. IEEE Trans. Ind. Inf. 13 (2), 800–809 (April 2017)
J. Massana, C. Pous, L. Burgas, J. Melendez, J. Colomer, Identifying services for short-term load forecasting using data driven models in a Smart City platform. Sustain. Cities Soc. 28 , 108–117 (2017)
L. DeRen, C. JianJun, Y. Yuan, Big data in smart cities. Sci. China Inf. Sci. 58 (12) (2015)
D.J. Cook, G. Duncan, G. Sprint, R. Fritz, Using smart city technology to make healthcare smarter. Proc. IEEE 106 , 708–722 (April 2018)
K. Joo-Chang, C. Kyungyong, Depression index service using knowledge based crowdsourcing in smart health. Wirel. Pers. Commun. 93 , 255–268 (March 2017)
A. Copie, V.I. Munteanu, B. Manate, T.-F. Fortis, An Internet of Things Governance Architecture with Applications in Healthcare, in The Internet of Things: Breakthroughs in Research and Practice , (Hershey, IGI Global, 2017), pp. 112–136
A.A. Obinikpo, B. Kantarci, Big sensed data meets deep learning for smarter health care in smart cities. J. Sens. Actuator Netw. 6 , 1–22 (2017)
J. Dhar, A. Ranganathan, Machine learning capabilities in medical diagnosis applications: computational results for hepatitis disease. Int. J. Biomed. Eng. Technol. 17 , 330–340 (2015)
K. Polat, S. Gunes, Principles component analysis, fuzzy weighting pre-processing and artificial immune recognition system based diagnostic system for diagnosis of lung cancer, in Expert Systems with Applications , vol. 34, 1st edn., (2008), pp. 214–221
A. Esteva, B. Kuprel, R.A. Novoa, J. Ko, S.M. Swetter, H.M. Blau, S. Thrun, Dermatologist-level classification of skin cancer with deep neural networks. Nature 542 , 115–118 (2017)
S. Wang, R. Summers, Mechine Learning and Radiology. Med. Image Anal. 16 (5), 933–951 (2012)
S.P. Somashekhar, M.-J. Sepúlveda, S. Puglielli, A.E.H. Shortliffe, C. Kumar, A. Rauthan, N. Kumar, P. Patil, K. Rhee, Y. Ramya, Watson for oncology and breast cancer treatment recommendations: agreement with an expert multidisciplinary tumor board, in Annals of Oncology , vol. 29, 2nd edn., (2018), pp. 418–423
K. Kincade, Data mining: digging for healthcare gold. Insur. Technol. 23 ( 2 ), IM2 – IM7 (1998)
A. Milley, Healthcare and data mining. Health Manag. Technol. 21 (8), 44–47 (2000)
J. Andreu-Perez, D. Leff, H. Ip, G. Yang, From wearable sensors to smart Implants—toward pervasive and personalized healthcare. IEEE Trans. Biomed. Eng. 62 , 2750–2762 (2015)
M. Chan, E. Campo, D. Estève, J.-Y. Fourniols, Smart homes—current features and future perspectives. Maturitas 64 (2), 90–97 (2009)
L. Liu, E. Strouli, I. Nikolaidis, A. Miguel-Cruz, A.R. Rincon, Smart homes and home health monitoring technologies for older adults: a systematic review. Int. J. Med. Inf. 91 , 44–59 (July 2016)
J. Zhang, Y. Li, L. Cao, Y. Zhang, Research on the construction of smart hospitals at home and abroad. Chin. Hos. Manag, 64–66 (2018)
K. Li, J. Wang, T. Li, F. Dou, K. He, Application of internet of things in supplies logistics of intelligent hospital. Chin. Med. Equip., 172–176 (2018)
H. Demirkan, A Smart Healthcare Systems Framework, IT Prof. no. 5, pp. 38–45, Sept–Oct 2013
Q. Chen, Y. Lu, Construction, and application effect evaluation of integrated manage-ment platform of intelligent hospital based on big data analysis. Chin. Med. Herald., 161–164 (2018)
P. Piazza, Health alerts to fight bioterror, Secur. Manag. p. 40, 2002.
J. Redfern, Smart health and innovation: facilitating health-related behaviour change. Proc. Nutr. Soc., 328–332 (2017)
M. Ridinger, American healthways uses SAS to improve patient care, DM Rev. no. 12, p. 139, 2002.
T.S. Brisimi, T. Xu, T. Wang, W. Dai, W.G. Adams, I.C. Paschalidis, Predicting chronic disease hospitalizations from electronic health records: an interpretable classification approach. Proc. IEEE 106 (4), 690–707 (2018)
S. Zhang, C. Tjortjis, X. Zeng, H. Qiao, B. Iain, J. Keane, Comparing data mining methods with logistic regression in childhood obesity prediction. Inf. Syst. Front. J. Springer 11 (4), 449–460 (2009)
C. Tjortjis, M. Saraee, B. Theodoulidis, J. Keane, Using T3, an improved decision tree classifier, for mining stroke related medical data. Method Inf Med Schattauer GmbH 46 (5), 523–529 (2007)
H. Banaee, M.U. Ahmed, A. Loutfi, Data mining for wearable sensors in health monitoring systems: a review of recent trends and challenges. Sensors 13 , 17472–17500 (2013)
T. Nef, P. Urwuler, M. Buchler, I. Tarnanas, R. Stucki, D. Cazzoli, R. Muri, U. Mosimann, Evaluation of three state-of-the-art classifiers for recognition of activities of daily living from smart home ambient data. Sensors 15 , 11725–11740 (2015)
B. Lin, Y. Huangfu, N. Lima, B. Lobson, M. Kirk, P. O'Keeffe, S. Pressley, V. Walden, B. Lamb, D. Cook, Analyzing the relationship between human behavior and indoor air quality. J. Sens. Actuator Netw. 6 , 1–18 (2017)
M. Islam, M. Hasan, X. Wang, H. Germack, M. Noor-E-Alam, A systematic review on healthcare analytics: application and theoretical perspective of data mining. Healthcare 6 (2), 54 (2018)
N. Jothi, N.A. Rashid, W. Husain, Data Mining in Healthcare – A Review, in The Third Information Systems International Conference, 2015.
M. Durairaj, V. Ranjani, Data Mining Applications In Healthcare Sector: A Study. Int. J. Sci. Technol. Res. 2 (10), 29–35 (2013)
V. Tatsis, C. Tjortjis, P. Tzirakis, Evaluating data mining algorithms using molecular dynamics trajectories. Int. J. Data Min. Bioinf. Indersci. 8 (2), 169–187 (2013)
P. Ahmad, S. Qamar, S.Q.A. Rizvi, Techniques of Data Mining In Healthcare: A Review. Int. J. Comp. Appl. 120 (15), 38–50 (2015)
H. Chung, P. Gray, Data mining. J. Manag. Inf. Syst. 16 (1), 11–16 (1999)
M. Aggarwal, Medium.com , 7 January 2018. [Online]. Available: https://medium.com/@thecodingcookie/cross-industry-process-for-data-mining-286c407132d0
I. Parvathi, S. Rautaray, Survey on data mining techniques for the diagnosis of diseases in medical domain. Int. J. Comp. Sci. Inf. Technol. 5 (1), 838–846 (2014)
R. Martinez-Espana, A. Bueno-Crespo, I. Timon, J. Soto, A. Munoz, J.M. Cecilia, Air-pollution prediction in smart cities through machine learning methods: a case study in Murcia, Spain. J. Univer. Comp. Sci. 24 (3), 261–276 (2018)
P. Tzirakis and C. Tjortjis, "T3C: Improving a Decision Tree Classification Algorithm’s Interval Splits on Continuous Attributes," Advances in Data Analysis and Classification, Springer , vol. 11, no. 2, pp. 353-370, 2017.
MATH Google Scholar
S. Mohapatra, P.K. Patra, S. Mohanty, B. Pati, Smart Health Care System using Data Mining, in International Conference on Information Technology, 2018.
D. Tomar, S. Agarwal, A survey on data mining approaches for Healthcare. Allahabad Int. J. Biosci. Biotechnol. 5 (5), 241–266 (2013)
Y. Kanellopoulos, P. Antonellis, C. Tjortjis, C. Makris, N. Tsirakis, k-Attractors: a partitional clustering algorithm for numeric data analysis. Appl. Artif. Intell. Taylor Francis 25 (2), 97–115 (2011)
A. Kelati, J. Plosila, H. Tenhunen, Smart Meter Load Profiling for e-Health Monitoring System, in 7th International Conference on Smart Energy Grid Engineering, 2019.
R. Agrawal and R. Srikant, "Apriori algorithm". 1994.
S.M. Ghafari, and C. Tjortjis, Association Rules Mining by improving the Imperialism Competitive Algorithm (ARMICA), in IFIP AICT Proceeding of 12th International Conference on Artificial Intelligence Applications and Innovations (AIAI 2016). Springer,, 2016.
S. Yakhchi, S. M. Ghafari, C. Tjortjis, M. Fazeli, ARMICA-Improved: A New Approach for Association Rule Mining, in Proceeding of 10th International Conference on Knowledge Science, Engineering and Management (KSEM 17), Springer LNAI, vol. 10412, pp. 296–306, 2017.
J. Han, H. Pei, Y. Yin, Mining Frequent Patterns without Candidate Generation, in Proceeding of Conference on the Management of Data (SIGMOD’00, Dallas, TX) , (ACM Press, New York, 2000)
S.M. Ghafari, C. Tjortjis, A survey on association rules mining using Heuristics. WIREs Data Min. Knowl. Discov. 9 (4) (2019)
Y. Ji, H. Ying, J. Tran, P. Dews, A. Mansour, M.R. Massanari, Mining Infrequent Causal Associations in Electronic Health Databases, in 2011 IEEE 11th Int’l Conf. on Data Mining Workshops, 2011.
A. Asuncion, D. Newman, UCI Machine Learning Repository, 2007. [Online]. Available: https://archive.ics.uci.edu/ml/datasets.php
H. Ian, E. Frank, A.H. Mark, J.P. Christopher, Data Mining: Practical Machine Learning Tools and Techniques , 3rd edn. (Morgan Kaufmann, San Francisco, 2011)
R.L. Thorndike, Who belongs in the family? Psychometrika 18 , 267–276 (1953)
Download references
Author information
Authors and affiliations.
The Data Mining and Analytics research group, School of Science and Technology, International Hellenic University, Thermi, Greece
Theodora Chatzinikolaou, Eleni Vogiatzi, Anestis Kousis & Christos Tjortjis
You can also search for this author in PubMed Google Scholar
Corresponding author
Correspondence to Christos Tjortjis .
Editor information
Editors and affiliations.
Chitkara University Institute of Engineering and Technology, Chitkara University, Rajpura, Punjab, India
Shalli Rani
Intel Corporation, Folsom, CA, USA
Department of ECE, KPR Institute of Engineering and Technology, Coimbatore, India
R. Maheswar
Rights and permissions
Reprints and permissions
Copyright information
© 2022 The Author(s), under exclusive license to Springer Nature Switzerland AG
About this chapter
Chatzinikolaou, T., Vogiatzi, E., Kousis, A., Tjortjis, C. (2022). Smart Healthcare Support Using Data Mining and Machine Learning. In: Rani, S., Sai, V., Maheswar, R. (eds) IoT and WSN based Smart Cities: A Machine Learning Perspective. EAI/Springer Innovations in Communication and Computing. Springer, Cham. https://doi.org/10.1007/978-3-030-84182-9_3
Download citation
DOI : https://doi.org/10.1007/978-3-030-84182-9_3
Published : 31 May 2022
Publisher Name : Springer, Cham
Print ISBN : 978-3-030-84181-2
Online ISBN : 978-3-030-84182-9
eBook Packages : Engineering Engineering (R0)
Share this chapter
Anyone you share the following link with will be able to read this content:
Sorry, a shareable link is not currently available for this article.
Provided by the Springer Nature SharedIt content-sharing initiative
- Publish with us
Policies and ethics
- Find a journal
- Track your research
Next Application Deadline: 4/12
Home > Resources > Healthcare Analytics > Data Mining In Healthcare
Data Mining In Healthcare
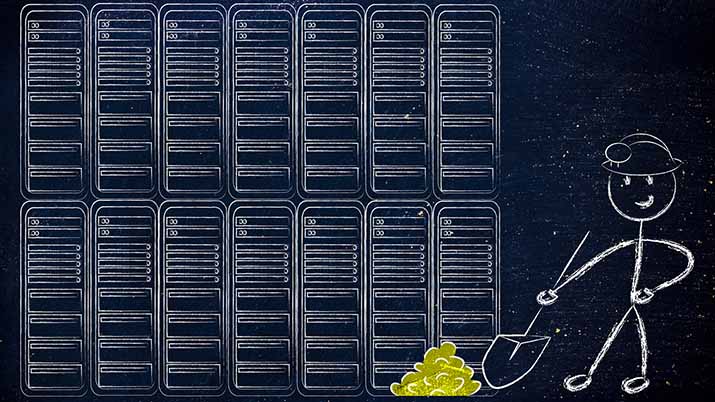
- Published February 15, 2017
- Updated February 28, 2023
Since the 1990s, businesses have used data mining for things like credit scoring and fraud detection. With the increase in accessibility to large amounts of patient data for providers today, the use of data mining in healthcare is being adopted by organizations with a focus on optimizing the efficiency and quality of their predictive analytics.
What is Data Mining?
The purpose of data mining, whether it’s being used in healthcare or business, is to identify useful and understandable patterns by analyzing large sets of data . These data patterns help predict industry or information trends, and then determine what to do about them.
In the healthcare industry specifically, data mining can be used to decrease costs by increasing efficiencies, improve patient quality of life, and perhaps most importantly, save the lives of more patients.
Data Mining in Healthcare Examples
Data mining has been used in many industries to improve customer experience and satisfaction, and increase product safety and usability. Data mining in healthcare has proven effective in areas such as predictive medicine, customer relationship management, detection of fraud and abuse, management of healthcare and measuring the effectiveness of certain treatments.
Here is a short breakdown of two healthcare data mining applications with real-world examples of their use.
Measuring Treatment Effectiveness
This application of healthcare data mining involves comparing and contrasting symptoms, causes and courses of treatment to find the most effective course of action for a certain illness or condition. For example, patient groups who are treated with different drug regimens can be compared to determine which treatment plans work best and save the most money. Furthermore, the continued use of this data mining application could help standardize a method of treatment for specific diseases, thus making the diagnosis and treatment process quicker and easier.
Detecting Fraud and Abuse
This application of data mining in healthcare involves establishing normal patterns, then identifying unusual patterns of medical claims by clinics, physicians, labs, or others. This application can also be used to identify inappropriate referrals or prescriptions and insurance fraud and fraudulent medical claims. The Texas Medicaid Fraud and Abuse Detection System is a good example of a business using data mining to detect fraud. In 1998, the organization recovered $2.2 million in stolen funds and identified 1,400 suspects for investigation. To recognize its success, the Texas system received a national award for its innovative use of technology .
Healthcare Data Mining and its Effect on Patient Privacy
Data mining is proving beneficial for healthcare, but it has also come with a few patient privacy concerns. Massive amounts of patient data being shared during the data mining process increases patient concerns that their personal information could fall into the wrong hands. However, experts argue that this is a risk worth taking.
“There will be criminals. There will be people who are bad actors. At some point, something is going to get out,” Thomas Graf, chief medical officer at Geisinger Health System told The Washington Post . “It’s not an irrational fear. At the same time, people die driving every year and we still choose to drive cars, or most of us do. It’s a risk every person has to decide where they fall on the line.”
Others have suggested letting patients choose whether their information can be used for data mining purposes and then providing a tax break benefit to encourage patients to get involved.
“The goal in healthcare is not to protect privacy, the goal is to save lives,” David Castro, Director of the Center for Data Innovation told The Washington Post.
The Future of Data Mining in Healthcare
The shift from written to electronic health records has played a huge part in the push to use patient data to improve areas of the healthcare industry. The adoption of electronic health records have allowed healthcare professionals to distribute the knowledge across all sectors of healthcare, which in turn, helps reduce medical errors and improve patient care and satisfaction.
Data mining is also projected to help cut costs. If the U.S. healthcare industry continues to use big data to drive efficiency and quality, the value could be significant. According to research from McKinsey and Company , system wide data analytics efforts could cut overall healthcare costs by 12-17%.
According to spending data reported by the Centers for Medicare and Medicaid Services , the United States national healthcare expenditure reached $3.5 trillion in 2017. Applying a 12-17% savings to that number, the estimated cost reduction from system wide data analytics efforts could reach between $420 billion and $595 billion.
The future of healthcare may well depend on using data mining to decrease healthcare costs, identify treatment plans and best practices, measure effectiveness, detect fraudulent insurance and medical claims, and ultimately, improve the standard of patient care.
Related Articles
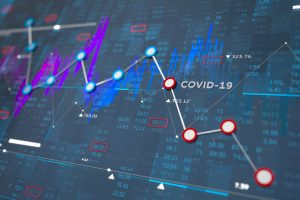
Analytics, AI and the Power of Data in a Pandemic
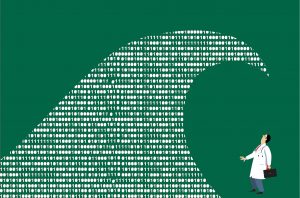
Big Data and the U.S. Healthcare System
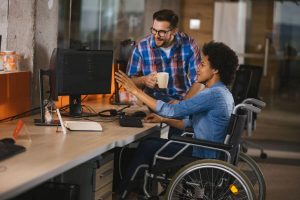
Medical Coder: Job Description and Career Outlook
Academic calendar, get our program guide, if you are ready to learn more about our programs, get started by downloading our program guide now..
How can we help you?
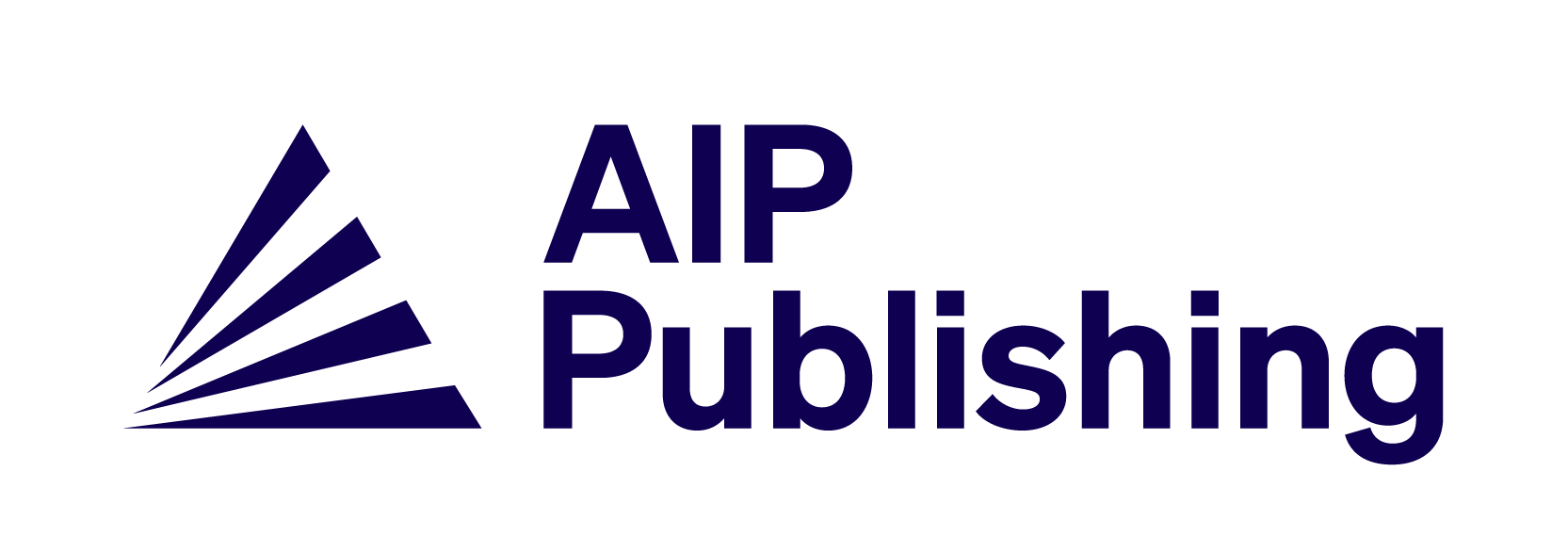
A review: Data mining techniques in healthcare
- Article contents
- Figures & tables
- Supplementary Data
- Peer Review
- Reprints and Permissions
- Cite Icon Cite
- Search Site
Priya Chaudhary , Priyanka Sharma , Aditya Gataum; A review: Data mining techniques in healthcare. AIP Conf. Proc. 27 July 2023; 2721 (1): 070036. https://doi.org/10.1063/5.0153916
Download citation file:
- Ris (Zotero)
- Reference Manager
Data mining is the process of obtaining valuable data from the big collection of raw data. The healthcare industry is onewhich collects a large amount of data that is not properly used so as to find hidden patterns and correlation important for early diagnosis. In this study, a survey of data mining applications in healthcare has been presented using different mining techniques,to analyze and predict deadly human diseases also epidemics like recent one that is COVID-19. Prediction of the disease is critical to help the health practitioners to make decisions to cure it effectively. “Now it is obvious that world needs a speedy and quicker way to tackle the further spread of the deadly diseases which can only be possible by data mining approaches and artificial intelligence techniques so as to lessen the load onhealthcare system by providing best feasible mode for diagnosis and prognosis of SARS-CoV-2.”(1).
Sign in via your Institution
Citing articles via, publish with us - request a quote.
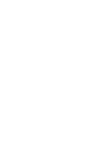
Sign up for alerts
- Online ISSN 1551-7616
- Print ISSN 0094-243X
- For Researchers
- For Librarians
- For Advertisers
- Our Publishing Partners
- Physics Today
- Conference Proceedings
- Special Topics
pubs.aip.org
- Privacy Policy
- Terms of Use
Connect with AIP Publishing
This feature is available to subscribers only.
Sign In or Create an Account
Case study: how to apply data mining techniques in a healthcare data warehouse
Affiliation.
- 1 Rush Medical College, USA.
- PMID: 11452577
Healthcare provider organizations are faced with a rising number of financial pressures. Both administrators and physicians need help analyzing large numbers of clinical and financial data when making decisions. To assist them, Rush-Presbyterian-St. Luke's Medical Center and Hitachi America, Ltd. (HAL), Inc., have partnered to build an enterprise data warehouse and perform a series of case study analyses. This article focuses on one analysis, which was performed by a team of physicians and computer science researchers, using a commercially available on-line analytical processing (OLAP) tool in conjunction with proprietary data mining techniques developed by HAL researchers. The initial objective of the analysis was to discover how to use data mining techniques to make business decisions that can influence cost, revenue, and operational efficiency while maintaining a high level of care. Another objective was to understand how to apply these techniques appropriately and to find a repeatable method for analyzing data and finding business insights. The process used to identify opportunities and effect changes is described.
- Database Management Systems / organization & administration*
- Decision Support Systems, Management*
- Diagnosis-Related Groups / economics*
- Efficiency, Organizational / economics
- Hospital Costs
- Hospitals, Teaching / economics
- Hospitals, Teaching / statistics & numerical data*
- Information Centers / organization & administration*
- Information Storage and Retrieval / methods*
- Middle Aged
- Organizational Case Studies
- Systems Integration
- User-Computer Interface

An official website of the United States government
The .gov means it’s official. Federal government websites often end in .gov or .mil. Before sharing sensitive information, make sure you’re on a federal government site.
The site is secure. The https:// ensures that you are connecting to the official website and that any information you provide is encrypted and transmitted securely.
- Publications
- Account settings
Preview improvements coming to the PMC website in October 2024. Learn More or Try it out now .
- Advanced Search
- Journal List
- Iran J Public Health
- v.50(11); 2021 Nov
Introduction of Health Information Technology Professionals for Data Mining in Hospitals
Elham aalipour.
1. Department of Health Information Management, School of Allied Medical Sciences, Tehran University of Medical Sciences, Tehran, Iran
2. Department of Health Information Technology, School of Allied Medical Sciences, Ahvaz Jundishapur University of Medical Sciences, Ahvaz, Iran
Marjan Ghazisaeedi
Dear editor-in-chief.
The databases of the computer-based information systems in hospitals contain data which could be used well to assess the performance of hospital staff especially healthcare professionals by analyzing appropriately. According to the performance analysis results, the hospital staff performance can be maintained in a high level more than ever. Definitely, improvement of hospital staff performance has a key role in improving the provision of healthcare services to the patients and ultimately clients referred to healthcare centers ( 1 – 2 ).
In addition, the complete, accurate and appropriate data represent the personnel performance truly and can be utilized in scientific researches confidently. After ensuring that the data existing in the databases are regarded appropriate, complete and exact for an issue under study, the data mining techniques can be used to discover knowledge among the huge data existing in the databases of the computer-based information systems in hospitals in order to make timely and correct decisions about a specific issue or access to new knowledge in the field of apparent and hidden challenges in hospitals ( 3 ).
Regarding different steps of knowledge discovery, first non-assimilated and redundant data are removed among huge data (data cleaning), various data resources are combined (data integration), and target-related data are retrieved from databases (data selection). Further, data are converted to appropriate forms for extraction by using various operations (data transformation) such as summarizing. Furthermore, data patterns are extracted by using logical and wise methods (data mining). Finally, patterns are evaluated and knowledge presentation are performed ( 4 – 5 ).
Data mining step is considered as the auto search among large data resources to find patterns or data characteristics, which cannot be conducted by simple statistical analysis. Some techniques such as bayesian, decision tree, neural networks, nearest neighbor, fuzzy logic, and genetic algorithms models are used in the data mining step for discovering patterns and the relationships between data ( 5 – 6 ).
The extraction of knowledge among huge relevant data for identifying the methods of diseases prevention, the cause, diagnosis, anticipation and treatment of illness, the effectiveness of drugs and other relevant issues are considered as some purposes of data mining related to health area, which ultimately result in increasing longevity and making peace of mind among community individuals ( 7 ).
Hospital officials can utilize the skills of the professionals in health information technology department to analyze healthcare and managerial data. The professionals of health information technology department in hospital monitor the performance of hardware, software and network equipment in the electronic environment for the management and security of hospital information. These professionals with their specialty in software, hardware, networking, and health information technologies, play an important role in helping hospital officials regarding monitoring data collection, storage, analysis, and then presenting and disseminating information and knowledge. If professionals of health information technology department can increase their experiences and skills in data mining and its related subcategories such as text mining, web mining, and ultimately, data science thus they can have more valuable effects in the health area ( 8 – 9 ).
A number of abilities of health information technology professionals related to explore in data on the basis of their job titles ( 10 ) are presented in Table 1 .
Some job titles of health information technology professionals related to explore in data
Conflict of interest
The author declares that there is no conflict of interest.
We couldn’t find any results matching your search.
Please try using other words for your search or explore other sections of the website for relevant information.
We’re sorry, we are currently experiencing some issues, please try again later.
Our team is working diligently to resolve the issue. Thank you for your patience and understanding.
News & Insights

Unearthing Efficiency: How the Mining Industry is Using AI to Make Data-driven Discoveries

April 05, 2024 — 01:00 pm EDT
Written by Dean Belder for Investing News Network ->
Since OpenAI launched ChatGPT to the public in November 2022, artificial intelligence (AI) has exploded into the mainstream, turning into a gold mine for companies that have become early adopters.
What are the implications of AI for the mining sector? Can AI help revitalize investment in the chronically underfunded exploration stage? Can it provide the tools companies need to improve operational efficiency?
This year at the Prospectors & Developers Association of Canada (PDAC) convention, AI and machine learning were broadly featured in several presentations, with participants aiming to answer those and other questions, as well as provide insight into how AI is being deployed and what it means for the future of the mining industry.
Mining sector no stranger to technology
Terms like AI and machine learning might seem like they've exploded onto the scene recently, but the reality is they’ve been around since the 1940s. So it should come as no surprise that an industry rooted in science has been using these technologies for decades, not only to improve extraction and processing, but also to aid in discovery.
This idea was discussed during a PDAC panel hosted by Steve de Jong, CEO of AI company VRIFY.
Chris Taylor, former president and CEO of Great Bear Resources, which was acquired by Kinross Gold (TSX: K ,NYSE:KGC) in 2022, said the company's use of machine learning tools was instrumental in making the district-scale discovery of the Dixie gold deposit in Ontario, which sent waves through the industry in the late 2010s.
Taylor said he believed he was included on the panel to provide a contrarian point of view.
“Every geologist that I know, every person that was instrumental in the Great Bear discovery, was already doing both computer modeling and interpretation and traditional field geology. So it’s not like there’s a dichotomy. These are tools that we’ve been using for a long time," he explained to listeners.
Specifically, geographic information system (GIS) programs such as Esri's ArcGIS have been used by the mining industry to help model and visualize exploration data since the mid-1980s. Taylor detailed how the tools used by Great Bear worked by having a geologist input a mathematical equation into GIS software.
“It all came down to the brain of the geologist and what factors you thought were most important. So you’d build an equation, and you’d wait for the equation and that would give you a number answer of zero or one,” he said. The results would help build a model that would provide the most prospective targets on the property.
How is the mining industry using AI today?
The data modeling tools used by Great Bear are still widely employed in the mining industry, but are beginning a new phase of evolution as AI and machine learning are more widely adopted and more closely integrated into GIS tools.
While some resource companies have approached AI cautiously, preferring to stick with the standard methods of exploration they are accustomed to, others have embraced the technology.
With backing from the likes of billionaires Bill Gates and Jeff Bezos, privately owned KoBold Metals has taken the second approach. In fact, the exploration company has been mistaken for a tech company due to the software side of its operations and its close connection with Silicon Valley capital. Even so, KoBold is emphatic that it is an exploration company first — just one that has fully integrated machine learning into its processes.
The company, which currently holds interests in more than 60 projects, made headlines in December 2022 , when it agreed to pay US$115 million to EMR Capital, a private equity firm with an 80 percent stake in the Lubambe copper mine in Zambia. In return, Kobold received a 52 percent stake in the Lubambe extension project, which is now known as the Mingomba deposit. As part of the agreement, the company also committed to investing an additional US$35 million for exploration work at the site, which it has been carrying out since then.
In February of this year, KoBold confirmed that Mingomba hosts a large resource, calling it the largest copper discovery in a century, and said it intends to fast track mine development at the site.
Some media reports have credited the discovery to the team’s software. However, KoBold’s co-founder and CEO, Kurt House, who was also part of the VRIFY panel, described it as part of a larger process. KoBold’s software is a type of machine learning called a neural net — a set of processing nodes modeled after the human brain — that can put together a model based on billions of parameters. This requires integrated teams that provide the AI with enhanced data from drill results plus broader geological data, which it then uses to better target resource deposits.
“Every exploration program we have worldwide is co-led by a geoscientist and a data scientist, every single one,” House said at PDAC. “They’re glued together.” This is in contrast to the standard exploration process, whereby a more limited set of parameters would be fed to a GIS program by a geoscientist without the aid of a data scientist.
VRIFY's de Jong was similarly positive about how AI tools have evolved in the mining space.
In 2017, his company began the development of its namesake tool, which allowed improved communication between companies and their investors. The program uses AI to aid in the production of presentations that marry easy-to-read data on exploration activities, financials and company activities with intuitive 3D models of deposits and drill sites. Since then, VRIFY has gone on to be used by 180 companies in the mining industry.
Much like AI tools, VRIFY as a company has also evolved. In an interview with the Investing News Network, de Jong said his company is working with four mining companies to beta test its new AI-powered VRIFY.ai mineral exploration tool.
De Jong said VRIFY’s approach differs from KoBold’s; it's more granular and works by applying a company’s own data sets to VRIFY’s trained AI model to see patterns and identify mineralization that might otherwise be missed.
“If I give you a database, even if it’s just drill holes or rock samples from the surface, but there are positive assay hits of the type of mineral you’re looking for within that, then we can take that, then grab every other data set available and train it to look for more occurrences of those positive hits,” he explained.
So far, de Jong said the tools have revealed targets that are encouraging, and he’s excited about the next steps when companies go out to drill the areas identified by VRIFY’s tool and begin to validate the data.
What does AI mean for mining investors?
Mining industry investment has lagged for many years now. While the rewards of exploration have the potential to be high, the risks are even higher. In the “Where Will the Money Come From?” panel at PDAC, Franco-Nevada (TSX: FNV ,NYSE:FNV) Founder and Chair Emeritus Pierre Lassonde explained how rare successful projects are.
“I took a 10 year span from 1983 to 1993 and looked at 3,000 exploration companies and what happened to them,” he told the audience at the convention. “Of those 3,000, only five companies actually delivered mines that opened and made money. The ratio is appalling, and it got worse in the last 20 years.”
Lassonde went on to discuss how AI has the potential to revolutionize the exploration process, but added the caveat that to be effective it needs vast amounts of data gleaned from drill programs and assay results, making it less accessible for the earliest-stage explorers or those operating in underexplored regions.
“AI is going to help incredibly, but you have to understand that AI is fed by data,” he said. “So if you have a project that already has 300,000 meters of drilling, AI is going to be incredibly useful to you because you’re feeding it massive amounts of information, and it will be helpful. But if you have a totally new discovery with two drill holes, it's not going to be very helpful because it has no information.”
In the VRIFY panel, Taylor spoke about how AI tools are helping make operations more efficient, which in turn leads to lower costs and ultimately provides investors with better returns. “What it will do is put the power back in the exploration geologist to make those decisions efficiently, and keep that return coming for investors,” he said.
For de Jong, efficiency is more of a by-product of AI’s true potential, which is helping companies maximize their chance at making a greater discovery, whether it's aiding in resource expansion or finding a completely unknown deposit.
Of course, it's not just exploration that is benefiting from what AI and machine learning have to offer.
During another PDAC presentation, Denise Johnson, a group president at Caterpillar (NYSE: CAT ), talked about how the company has been investing in new technologies like battery electric mining vehicles and AI.
On the production side, Johnson painted a picture of how companies are already deploying AI to operate mines more efficiently, decrease mining waste and ultimately drive productivity.
She said leveraging AI at remote mining sites can be particularly advantageous, noting that optimization is essential when getting labor and equipment to challenging locations. “We’re focused right now also on combining data and sensors and intelligence to really improve the understanding of the orebody so that customers can make more precise real-time decisions, which really enables that end-to-end value chain optimization,” she said.
Whether AI improves operational efficiency, unlocks greater value from resources or both, the end result is a benefit to investors as it helps reduce risk in a naturally high-risk part of the industry.
That's one reason why de Jong sees early adopters in the industry faring well compared to their counterparts who continue on a more standard path to exploration.
“I do think you’re going to see the companies that are out there and loudly embracing this start to get a premium in the market, because investors are going to say, ‘This is a tool that’s going to help you increase the potential (return on investment) on every dollar that I invest in your company. Why wouldn’t I reward you for that in the market?’” he said.
However, like Lassonde, de Jong noted that AI isn’t a panacea that can come in and magically find targets — it still takes work and data and time to develop tools. When asked how investors can determine if companies are just trying to ride the attention AI has been getting without properly employing the technology, he was straightforward.
“The best way to tell if someone’s just looking for buzzwords and to kind of pump a share price versus actually doing something or standing behind it is whether or not they’re drilling those targets,” he said.
Right now, AI seems to be making inroads in mining. If it holds even half the potential its proponents suggest, it should aid in driving discovery and attracting new investment to an industry that has lacked both for some time.
Don't forget to follow us @INN_Resource for real-time updates!
Securities Disclosure: I, Dean Belder, hold no direct investment interest in any company mentioned in this article.
Editorial Disclosure: The Investing News Network does not guarantee the accuracy or thoroughness of the information reported in the interviews it conducts. The opinions expressed in these interviews do not reflect the opinions of the Investing News Network and do not constitute investment advice. All readers are encouraged to perform their own due diligence.
The views and opinions expressed herein are the views and opinions of the author and do not necessarily reflect those of Nasdaq, Inc.

More Related Articles
This data feed is not available at this time.
Sign up for the TradeTalks newsletter to receive your weekly dose of trading news, trends and education. Delivered Wednesdays.
To add symbols:
- Type a symbol or company name. When the symbol you want to add appears, add it to My Quotes by selecting it and pressing Enter/Return.
- Copy and paste multiple symbols separated by spaces.
These symbols will be available throughout the site during your session.
Your symbols have been updated
Edit watchlist.
- Type a symbol or company name. When the symbol you want to add appears, add it to Watchlist by selecting it and pressing Enter/Return.
Opt in to Smart Portfolio
Smart Portfolio is supported by our partner TipRanks. By connecting my portfolio to TipRanks Smart Portfolio I agree to their Terms of Use .
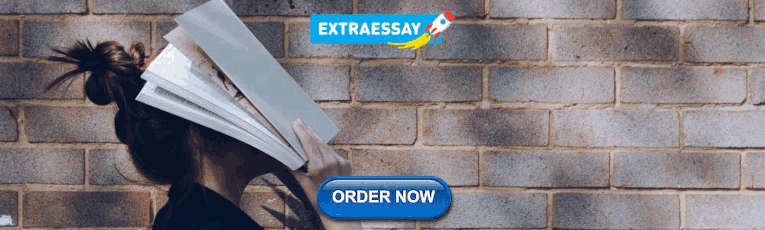
COMMENTS
1. Introduction. Deriving from Industry 4.0 that pursues the expansion of its autonomy and efficiency through data-driven automatization and artificial intelligence employing cyber-physical spaces, the Healthcare 4.0 portrays the overhaul of medical business models towards a data-driven management [].In akin environments, substantial amounts of information associated to organizational ...
Overview. Data mining techniques in healthcare involve the use of various technologies such as neural networks, machine learning, clustering, and decision trees. These technologies enable healthcare organizations to analyze large amounts of data from electronic health records, medical images such as X-rays and MRIs, and other sources.
Data mining is a multidisciplinary field at the intersection of database technology, statistics, ML, and pattern recognition that profits from all these disciplines [].Although this approach is not yet widespread in the field of medical research, several studies have demonstrated the promise of data mining in building disease-prediction models, assessing patient risk, and helping physicians ...
Medical data mining is a set of data science methods and instruments used to generate evidence-based medical information that clinicians and scientists can trust. Healthcare data mining techniques are used in many health-related areas, including biotech, pharmaceutical research, and medical science. The main health technologies and tech components involved in the clinical data mining process
Data mining have a great potential to enable healthcare systems to use data more efficiently and effectively. Hence, it improves care and reduces costs. This paper reviews various Data Mining ...
The past data mining techniques and its function tools for healthcare organisations are also discussed. ... The presentation of artificial neural network's collective technique and rough set ... A.S., Jayakarthik, R. (2022). Data Mining in Health Care: Application Perspective. In: Ramu, A., Chee Onn, C., Sumithra, M. (eds) International ...
In order to identify the strategic topics and the thematic evolution structure of data mining applied to healthcare, in this paper, a bibliometric performance and network analysis (BPNA) was conducted. For this purpose, 6138 articles were sourced from the Web of Science covering the period from 1995 to July 2020 and the SciMAT software was used. Our results present a strategic diagram composed ...
1. Introduction. Big data analytics (BDA) is an emerging topic among scholars and it is a holistic scheme to supervise, practice and analyze the 5 V data-associated dimensions [1].BDA is comprised of various applications including healthcare units, business and industrial sectors [2].The high volume data that is produced at higher velocities and assortments in healthcare augment complexity.
Healthcare sector is having lots of data about various disease and newly discovered viruses and bacteria's and so on. The data has been resided for a long at various data warehouses of each country's healthcare department but due to lack of collaboration and employment of advanced machine learning algorithms the meaningful insights which may conclude from data research and association ...
Healthcare data mining includes techniques such as clustering, classification, or regression analysis, and these techniques help to scrutinize information. Furthermore, the data mining market is predicted to reach $1.03 billion by 2023 at a CAGR of 11.9 percent during the 2018 to 2023 forecast period. This article offers a comprehensive lookout ...
Applying Data Mining Techniques in Healthcare. September 2016; Studies in Informatics and Control 25(3):385-394 ... there is a growing demand for the healthcare community to transform the existing ...
Data mining is a powerful new tec hnology with gr eat potential t o help c ompanies. focus on the m ost important information in the data they have collected about the behavior. of their customers ...
PowerPoint presentation slides: The following slide enumerates data mining techniques used in healthcare industry to identify effective treatments and best practices for patients. This covers four methods such as classification, clustering, association and outlier detection for transforming huge data into useful information.
Islam et al. claim that classification techniques are the most dominant for analyzing health data and widely used in the literature for clinical decision support and healthcare administration. Some of the best known classification algorithms are K-nearest neighbor (k-NN) [ 58 ], decision trees (DT) [ 59 ], support vector machines (SVM) [ 23 ...
Detecting Fraud and Abuse. This application of data mining in healthcare involves establishing normal patterns, then identifying unusual patterns of medical claims by clinics, physicians, labs, or others. This application can also be used to identify inappropriate referrals or prescriptions and insurance fraud and fraudulent medical claims.
Data mining is the process of obtaining valuable data from the big collection of raw data. The healthcare industry is onewhich collects a large amount of data that is not properly used so as to find hidden patterns and correlation important for early diagnosis. In this study, a survey of data mining applications in healthcare has been presented ...
The existing works on bibliometric analysis of data mining in health care in the Web of Science are shown in Table1, where it is depicted that only three studies have been ... the themes were then manually classified between data mining techniques and medical research concepts. Figure 1. Strategic diagram (a). Thematic network structure (b ...
The initial objective of the analysis was to discover how to use data mining techniques to make business decisions that can influence cost, revenue, and operational efficiency while maintaining a high level of care. Another objective was to understand how to apply these techniques appropriately and to find a repeatable method for analyzing data ...
Several studies in healthcare have explored data mining techniques to predict incidence [6] and characteristics of patients in pandemic scenarios [7], ... The existing works on bibliometric analysis of data mining in health care in the Web of Science are shown in Table 1, where it is depicted that only three studies have been ...
Generally, data visualization involves representing data and information in various forms, such as graphs, charts, diagrams, and pictures [ 1 ]. These visualization techniques can provide healthcare providers with an easy way to identify and understand data trends, outliers, and patterns [ 2 ]. Visualization techniques have been essential in ...
Furthermore, data patterns are extracted by using logical and wise methods (data mining). Finally, patterns are evaluated and knowledge presentation are performed (4-5). Data mining step is considered as the auto search among large data resources to find patterns or data characteristics, which cannot be conducted by simple statistical analysis.
The data modeling tools used by Great Bear are still widely employed in the mining industry, but are beginning a new phase of evolution as AI and machine learning are more widely adopted and more ...