- Privacy Policy
Buy Me a Coffee

Home » Research Data – Types Methods and Examples
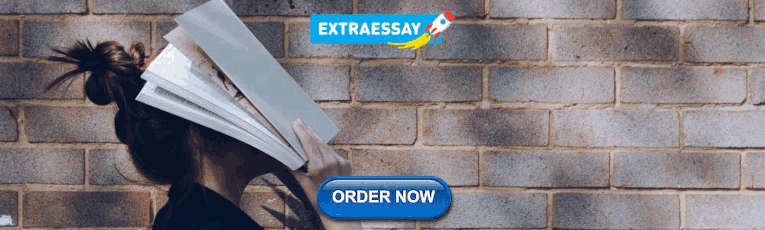
Research Data – Types Methods and Examples
Table of Contents

Research Data
Research data refers to any information or evidence gathered through systematic investigation or experimentation to support or refute a hypothesis or answer a research question.
It includes both primary and secondary data, and can be in various formats such as numerical, textual, audiovisual, or visual. Research data plays a critical role in scientific inquiry and is often subject to rigorous analysis, interpretation, and dissemination to advance knowledge and inform decision-making.
Types of Research Data
There are generally four types of research data:
Quantitative Data
This type of data involves the collection and analysis of numerical data. It is often gathered through surveys, experiments, or other types of structured data collection methods. Quantitative data can be analyzed using statistical techniques to identify patterns or relationships in the data.
Qualitative Data
This type of data is non-numerical and often involves the collection and analysis of words, images, or sounds. It is often gathered through methods such as interviews, focus groups, or observation. Qualitative data can be analyzed using techniques such as content analysis, thematic analysis, or discourse analysis.
Primary Data
This type of data is collected by the researcher directly from the source. It can include data gathered through surveys, experiments, interviews, or observation. Primary data is often used to answer specific research questions or to test hypotheses.
Secondary Data
This type of data is collected by someone other than the researcher. It can include data from sources such as government reports, academic journals, or industry publications. Secondary data is often used to supplement or support primary data or to provide context for a research project.
Research Data Formates
There are several formats in which research data can be collected and stored. Some common formats include:
- Text : This format includes any type of written data, such as interview transcripts, survey responses, or open-ended questionnaire answers.
- Numeric : This format includes any data that can be expressed as numerical values, such as measurements or counts.
- Audio : This format includes any recorded data in an audio form, such as interviews or focus group discussions.
- Video : This format includes any recorded data in a video form, such as observations of behavior or experimental procedures.
- Images : This format includes any visual data, such as photographs, drawings, or scans of documents.
- Mixed media: This format includes any combination of the above formats, such as a survey response that includes both text and numeric data, or an observation study that includes both video and audio recordings.
- Sensor Data: This format includes data collected from various sensors or devices, such as GPS, accelerometers, or heart rate monitors.
- Social Media Data: This format includes data collected from social media platforms, such as tweets, posts, or comments.
- Geographic Information System (GIS) Data: This format includes data with a spatial component, such as maps or satellite imagery.
- Machine-Readable Data : This format includes data that can be read and processed by machines, such as data in XML or JSON format.
- Metadata: This format includes data that describes other data, such as information about the source, format, or content of a dataset.
Data Collection Methods
Some common research data collection methods include:
- Surveys : Surveys involve asking participants to answer a series of questions about a particular topic. Surveys can be conducted online, over the phone, or in person.
- Interviews : Interviews involve asking participants a series of open-ended questions in order to gather detailed information about their experiences or perspectives. Interviews can be conducted in person, over the phone, or via video conferencing.
- Focus groups: Focus groups involve bringing together a small group of participants to discuss a particular topic or issue in depth. The group is typically led by a moderator who asks questions and encourages discussion among the participants.
- Observations : Observations involve watching and recording behaviors or events as they naturally occur. Observations can be conducted in person or through the use of video or audio recordings.
- Experiments : Experiments involve manipulating one or more variables in order to measure the effect on an outcome of interest. Experiments can be conducted in a laboratory or in the field.
- Case studies: Case studies involve conducting an in-depth analysis of a particular individual, group, or organization. Case studies typically involve gathering data from multiple sources, including interviews, observations, and document analysis.
- Secondary data analysis: Secondary data analysis involves analyzing existing data that was collected for another purpose. Examples of secondary data sources include government records, academic research studies, and market research reports.
Analysis Methods
Some common research data analysis methods include:
- Descriptive statistics: Descriptive statistics involve summarizing and describing the main features of a dataset, such as the mean, median, and standard deviation. Descriptive statistics are often used to provide an initial overview of the data.
- Inferential statistics: Inferential statistics involve using statistical techniques to draw conclusions about a population based on a sample of data. Inferential statistics are often used to test hypotheses and determine the statistical significance of relationships between variables.
- Content analysis : Content analysis involves analyzing the content of text, audio, or video data to identify patterns, themes, or other meaningful features. Content analysis is often used in qualitative research to analyze open-ended survey responses, interviews, or other types of text data.
- Discourse analysis: Discourse analysis involves analyzing the language used in text, audio, or video data to understand how meaning is constructed and communicated. Discourse analysis is often used in qualitative research to analyze interviews, focus group discussions, or other types of text data.
- Grounded theory : Grounded theory involves developing a theory or model based on an analysis of qualitative data. Grounded theory is often used in exploratory research to generate new insights and hypotheses.
- Network analysis: Network analysis involves analyzing the relationships between entities, such as individuals or organizations, in a network. Network analysis is often used in social network analysis to understand the structure and dynamics of social networks.
- Structural equation modeling: Structural equation modeling involves using statistical techniques to test complex models that include multiple variables and relationships. Structural equation modeling is often used in social science research to test theories about the relationships between variables.
Purpose of Research Data
Research data serves several important purposes, including:
- Supporting scientific discoveries : Research data provides the basis for scientific discoveries and innovations. Researchers use data to test hypotheses, develop new theories, and advance scientific knowledge in their field.
- Validating research findings: Research data provides the evidence necessary to validate research findings. By analyzing and interpreting data, researchers can determine the statistical significance of relationships between variables and draw conclusions about the research question.
- Informing policy decisions: Research data can be used to inform policy decisions by providing evidence about the effectiveness of different policies or interventions. Policymakers can use data to make informed decisions about how to allocate resources and address social or economic challenges.
- Promoting transparency and accountability: Research data promotes transparency and accountability by allowing other researchers to verify and replicate research findings. Data sharing also promotes transparency by allowing others to examine the methods used to collect and analyze data.
- Supporting education and training: Research data can be used to support education and training by providing examples of research methods, data analysis techniques, and research findings. Students and researchers can use data to learn new research skills and to develop their own research projects.
Applications of Research Data
Research data has numerous applications across various fields, including social sciences, natural sciences, engineering, and health sciences. The applications of research data can be broadly classified into the following categories:
- Academic research: Research data is widely used in academic research to test hypotheses, develop new theories, and advance scientific knowledge. Researchers use data to explore complex relationships between variables, identify patterns, and make predictions.
- Business and industry: Research data is used in business and industry to make informed decisions about product development, marketing, and customer engagement. Data analysis techniques such as market research, customer analytics, and financial analysis are widely used to gain insights and inform strategic decision-making.
- Healthcare: Research data is used in healthcare to improve patient outcomes, develop new treatments, and identify health risks. Researchers use data to analyze health trends, track disease outbreaks, and develop evidence-based treatment protocols.
- Education : Research data is used in education to improve teaching and learning outcomes. Data analysis techniques such as assessments, surveys, and evaluations are used to measure student progress, evaluate program effectiveness, and inform policy decisions.
- Government and public policy: Research data is used in government and public policy to inform decision-making and policy development. Data analysis techniques such as demographic analysis, cost-benefit analysis, and impact evaluation are widely used to evaluate policy effectiveness, identify social or economic challenges, and develop evidence-based policy solutions.
- Environmental management: Research data is used in environmental management to monitor environmental conditions, track changes, and identify emerging threats. Data analysis techniques such as spatial analysis, remote sensing, and modeling are used to map environmental features, monitor ecosystem health, and inform policy decisions.
Advantages of Research Data
Research data has numerous advantages, including:
- Empirical evidence: Research data provides empirical evidence that can be used to support or refute theories, test hypotheses, and inform decision-making. This evidence-based approach helps to ensure that decisions are based on objective, measurable data rather than subjective opinions or assumptions.
- Accuracy and reliability : Research data is typically collected using rigorous scientific methods and protocols, which helps to ensure its accuracy and reliability. Data can be validated and verified using statistical methods, which further enhances its credibility.
- Replicability: Research data can be replicated and validated by other researchers, which helps to promote transparency and accountability in research. By making data available for others to analyze and interpret, researchers can ensure that their findings are robust and reliable.
- Insights and discoveries : Research data can provide insights into complex relationships between variables, identify patterns and trends, and reveal new discoveries. These insights can lead to the development of new theories, treatments, and interventions that can improve outcomes in various fields.
- Informed decision-making: Research data can inform decision-making in a range of fields, including healthcare, business, education, and public policy. Data analysis techniques can be used to identify trends, evaluate the effectiveness of interventions, and inform policy decisions.
- Efficiency and cost-effectiveness: Research data can help to improve efficiency and cost-effectiveness by identifying areas where resources can be directed most effectively. By using data to identify the most promising approaches or interventions, researchers can optimize the use of resources and improve outcomes.
Limitations of Research Data
Research data has several limitations that researchers should be aware of, including:
- Bias and subjectivity: Research data can be influenced by biases and subjectivity, which can affect the accuracy and reliability of the data. Researchers must take steps to minimize bias and subjectivity in data collection and analysis.
- Incomplete data : Research data can be incomplete or missing, which can affect the validity of the findings. Researchers must ensure that data is complete and representative to ensure that their findings are reliable.
- Limited scope: Research data may be limited in scope, which can limit the generalizability of the findings. Researchers must carefully consider the scope of their research and ensure that their findings are applicable to the broader population.
- Data quality: Research data can be affected by issues such as measurement error, data entry errors, and missing data, which can affect the quality of the data. Researchers must ensure that data is collected and analyzed using rigorous methods to minimize these issues.
- Ethical concerns: Research data can raise ethical concerns, particularly when it involves human subjects. Researchers must ensure that their research complies with ethical standards and protects the rights and privacy of human subjects.
- Data security: Research data must be protected to prevent unauthorized access or use. Researchers must ensure that data is stored and transmitted securely to protect the confidentiality and integrity of the data.
About the author
Muhammad Hassan
Researcher, Academic Writer, Web developer
You may also like

Primary Data – Types, Methods and Examples

Qualitative Data – Types, Methods and Examples

Quantitative Data – Types, Methods and Examples

Secondary Data – Types, Methods and Examples

Information in Research – Types and Examples
6 Types of Data in Statistics & Research: Key in Data Science
Understanding the different types of data (in statistics, marketing research, or data science) allows you to pick the data type that most closely matches your needs and goals.
Whether you are a businessman, marketer, data scientist, or another professional who works with some kinds of data, you should be familiar with the key list of data types.
Why? Because the various data classifications allow you to correctly use measurements and thus to correctly make decisions.
On this page:
- The most common data types (with examples) in statistics, research, and data science. Simply explained.
- Infographics in PDF
Qualitative vs Quantitative Data
1. Quantitative data
Quantitative data seems to be the easiest to explain. It answers key questions such as “how many, “how much” and “how often”.
Quantitative data can be expressed as a number or can be quantified. Simply put, it can be measured by numerical variables.
Quantitative data are easily amenable to statistical manipulation and can be represented by a wide variety of statistical types of graphs and charts such as line, bar graph, scatter plot, and etc.
Examples of quantitative data:
- Scores on tests and exams e.g. 85, 67, 90 and etc.
- The weight of a person or a subject.
- Your shoe size.
- The temperature in a room.
There are 2 general types of quantitative data: discrete data and continuous data. We will explain them later in this article.
2. Qualitative data
Qualitative data can’t be expressed as a number and can’t be measured. Qualitative data consist of words, pictures, and symbols, not numbers.
Qualitative data is also called categorical data because the information can be sorted by category, not by number.
Qualitative data can answer questions such as “how this has happened” or and “why this has happened”.
Examples of qualitative data:
- Colors e.g. the color of the sea
- Your favorite holiday destination such as Hawaii, New Zealand and etc.
- Names as John, Patricia,…..
- Ethnicity such as American Indian, Asian, etc.
More you can see on our post qualitative vs quantitative data .
There are 2 general types of qualitative data: nominal data and ordinal data. We will explain them after a while.
Download the following infographic in PDF
Nominal vs Ordinal Data
3. Nominal data
Nominal data is used just for labeling variables, without any type of quantitative value. The name ‘nominal’ comes from the Latin word “nomen” which means ‘name’.
The nominal data just name a thing without applying it to order. Actually, the nominal data could just be called “labels.”
Examples of Nominal Data:
- Gender (Women, Men)
- Hair color (Blonde, Brown, Brunette, Red, etc.)
- Marital status (Married, Single, Widowed)
- Ethnicity (Hispanic, Asian)
As you see from the examples there is no intrinsic ordering to the variables.
Eye color is a nominal variable having a few categories (Blue, Green, Brown) and there is no way to order these categories from highest to lowest.
4. Ordinal data
Ordinal data shows where a number is in order. This is the crucial difference from nominal types of data.
Ordinal data is data which is placed into some kind of order by their position on a scale. Ordinal data may indicate superiority.
However, you cannot do arithmetic with ordinal numbers because they only show sequence.
Ordinal variables are considered as “in between” qualitative and quantitative variables.
In other words, the ordinal data is qualitative data for which the values are ordered.
In comparison with nominal data, the second one is qualitative data for which the values cannot be placed in an ordered.
We can also assign numbers to ordinal data to show their relative position. But we cannot do math with those numbers. For example: “first, second, third…etc.”
Examples of Ordinal Data:
- The first, second and third person in a competition.
- Letter grades: A, B, C, and etc.
- When a company asks a customer to rate the sales experience on a scale of 1-10.
- Economic status: low, medium and high.
Much more on the topic plus a quiz, you can learn in our post: nominal vs ordinal data .
Discrete vs Continuous Data
As we mentioned above discrete and continuous data are the two key types of quantitative data.
In statistics, marketing research, and data science, many decisions depend on whether the basic data is discrete or continuous.
5. Discrete data
Discrete data is a count that involves only integers. The discrete values cannot be subdivided into parts.
For example, the number of children in a class is discrete data. You can count whole individuals. You can’t count 1.5 kids.
To put in other words, discrete data can take only certain values. The data variables cannot be divided into smaller parts.
It has a limited number of possible values e.g. days of the month.
Examples of discrete data:
- The number of students in a class.
- The number of workers in a company.
- The number of home runs in a baseball game.
- The number of test questions you answered correctly
6. Continuous data
Continuous data is information that could be meaningfully divided into finer levels. It can be measured on a scale or continuum and can have almost any numeric value.
For example, you can measure your height at very precise scales — meters, centimeters, millimeters and etc.
You can record continuous data at so many different measurements – width, temperature, time, and etc. This is where the key difference from discrete types of data lies.
The continuous variables can take any value between two numbers. For example, between 50 and 72 inches, there are literally millions of possible heights: 52.04762 inches, 69.948376 inches and etc.
A good great rule for defining if a data is continuous or discrete is that if the point of measurement can be reduced in half and still make sense, the data is continuous.
Examples of continuous data:
- The amount of time required to complete a project.
- The height of children.
- The square footage of a two-bedroom house.
- The speed of cars.
Much more on the topic you can see in our detailed post discrete vs continuous data : with a comparison chart.
All of the different types of data have a critical place in statistics, research, and data science.
Data types work great together to help organizations and businesses from all industries build successful data-driven decision-making process .
Working in the data management area and having a good range of data science skills involves a deep understanding of various types of data and when to apply them. If you’re looking to enhance your data analysis skills, taking the best data science courses online can provide you with a solid foundation in understanding these essential data types.
About The Author

Silvia Valcheva
Silvia Valcheva is a digital marketer with over a decade of experience creating content for the tech industry. She has a strong passion for writing about emerging software and technologies such as big data, AI (Artificial Intelligence), IoT (Internet of Things), process automation, etc.
It’s a great blog. A great blog. Thanks for sharing this helpful post.
The blog is very informative and useful. Great article.
Brilliant, thanks
Educative article indeed
Wow! Well explained.
Thank you for taking the time to explain with such clarity.This has been of great help to me.
Informative and Easy
excellent explanation. you provided clarity on this topic. thanks to you i am saved from endless search of a simple and concise explanation. kudos
Leave a Reply Cancel Reply
This site uses Akismet to reduce spam. Learn how your comment data is processed .
- Skip to main content
- Skip to primary sidebar
- Skip to footer
- QuestionPro

- Solutions Industries Gaming Automotive Sports and events Education Government Travel & Hospitality Financial Services Healthcare Cannabis Technology Use Case NPS+ Communities Audience Contactless surveys Mobile LivePolls Member Experience GDPR Positive People Science 360 Feedback Surveys
- Resources Blog eBooks Survey Templates Case Studies Training Help center

Home Market Research
Data Analysis in Research: Types & Methods
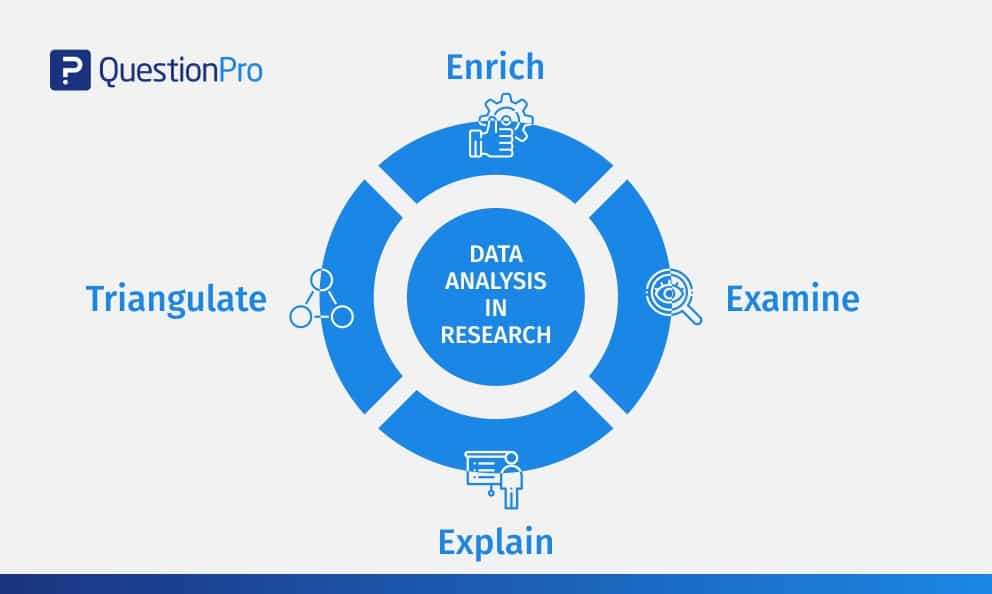
Content Index
Why analyze data in research?
Types of data in research, finding patterns in the qualitative data, methods used for data analysis in qualitative research, preparing data for analysis, methods used for data analysis in quantitative research, considerations in research data analysis, what is data analysis in research.
Definition of research in data analysis: According to LeCompte and Schensul, research data analysis is a process used by researchers to reduce data to a story and interpret it to derive insights. The data analysis process helps reduce a large chunk of data into smaller fragments, which makes sense.
Three essential things occur during the data analysis process — the first is data organization . Summarization and categorization together contribute to becoming the second known method used for data reduction. It helps find patterns and themes in the data for easy identification and linking. The third and last way is data analysis – researchers do it in both top-down and bottom-up fashion.
LEARN ABOUT: Research Process Steps
On the other hand, Marshall and Rossman describe data analysis as a messy, ambiguous, and time-consuming but creative and fascinating process through which a mass of collected data is brought to order, structure and meaning.
We can say that “the data analysis and data interpretation is a process representing the application of deductive and inductive logic to the research and data analysis.”
Researchers rely heavily on data as they have a story to tell or research problems to solve. It starts with a question, and data is nothing but an answer to that question. But, what if there is no question to ask? Well! It is possible to explore data even without a problem – we call it ‘Data Mining’, which often reveals some interesting patterns within the data that are worth exploring.
Irrelevant to the type of data researchers explore, their mission and audiences’ vision guide them to find the patterns to shape the story they want to tell. One of the essential things expected from researchers while analyzing data is to stay open and remain unbiased toward unexpected patterns, expressions, and results. Remember, sometimes, data analysis tells the most unforeseen yet exciting stories that were not expected when initiating data analysis. Therefore, rely on the data you have at hand and enjoy the journey of exploratory research.
Create a Free Account
Every kind of data has a rare quality of describing things after assigning a specific value to it. For analysis, you need to organize these values, processed and presented in a given context, to make it useful. Data can be in different forms; here are the primary data types.
- Qualitative data: When the data presented has words and descriptions, then we call it qualitative data . Although you can observe this data, it is subjective and harder to analyze data in research, especially for comparison. Example: Quality data represents everything describing taste, experience, texture, or an opinion that is considered quality data. This type of data is usually collected through focus groups, personal qualitative interviews , qualitative observation or using open-ended questions in surveys.
- Quantitative data: Any data expressed in numbers of numerical figures are called quantitative data . This type of data can be distinguished into categories, grouped, measured, calculated, or ranked. Example: questions such as age, rank, cost, length, weight, scores, etc. everything comes under this type of data. You can present such data in graphical format, charts, or apply statistical analysis methods to this data. The (Outcomes Measurement Systems) OMS questionnaires in surveys are a significant source of collecting numeric data.
- Categorical data: It is data presented in groups. However, an item included in the categorical data cannot belong to more than one group. Example: A person responding to a survey by telling his living style, marital status, smoking habit, or drinking habit comes under the categorical data. A chi-square test is a standard method used to analyze this data.
Learn More : Examples of Qualitative Data in Education
Data analysis in qualitative research
Data analysis and qualitative data research work a little differently from the numerical data as the quality data is made up of words, descriptions, images, objects, and sometimes symbols. Getting insight from such complicated information is a complicated process. Hence it is typically used for exploratory research and data analysis .
Although there are several ways to find patterns in the textual information, a word-based method is the most relied and widely used global technique for research and data analysis. Notably, the data analysis process in qualitative research is manual. Here the researchers usually read the available data and find repetitive or commonly used words.
For example, while studying data collected from African countries to understand the most pressing issues people face, researchers might find “food” and “hunger” are the most commonly used words and will highlight them for further analysis.
LEARN ABOUT: Level of Analysis
The keyword context is another widely used word-based technique. In this method, the researcher tries to understand the concept by analyzing the context in which the participants use a particular keyword.
For example , researchers conducting research and data analysis for studying the concept of ‘diabetes’ amongst respondents might analyze the context of when and how the respondent has used or referred to the word ‘diabetes.’
The scrutiny-based technique is also one of the highly recommended text analysis methods used to identify a quality data pattern. Compare and contrast is the widely used method under this technique to differentiate how a specific text is similar or different from each other.
For example: To find out the “importance of resident doctor in a company,” the collected data is divided into people who think it is necessary to hire a resident doctor and those who think it is unnecessary. Compare and contrast is the best method that can be used to analyze the polls having single-answer questions types .
Metaphors can be used to reduce the data pile and find patterns in it so that it becomes easier to connect data with theory.
Variable Partitioning is another technique used to split variables so that researchers can find more coherent descriptions and explanations from the enormous data.
LEARN ABOUT: Qualitative Research Questions and Questionnaires
There are several techniques to analyze the data in qualitative research, but here are some commonly used methods,
- Content Analysis: It is widely accepted and the most frequently employed technique for data analysis in research methodology. It can be used to analyze the documented information from text, images, and sometimes from the physical items. It depends on the research questions to predict when and where to use this method.
- Narrative Analysis: This method is used to analyze content gathered from various sources such as personal interviews, field observation, and surveys . The majority of times, stories, or opinions shared by people are focused on finding answers to the research questions.
- Discourse Analysis: Similar to narrative analysis, discourse analysis is used to analyze the interactions with people. Nevertheless, this particular method considers the social context under which or within which the communication between the researcher and respondent takes place. In addition to that, discourse analysis also focuses on the lifestyle and day-to-day environment while deriving any conclusion.
- Grounded Theory: When you want to explain why a particular phenomenon happened, then using grounded theory for analyzing quality data is the best resort. Grounded theory is applied to study data about the host of similar cases occurring in different settings. When researchers are using this method, they might alter explanations or produce new ones until they arrive at some conclusion.
LEARN ABOUT: 12 Best Tools for Researchers
Data analysis in quantitative research
The first stage in research and data analysis is to make it for the analysis so that the nominal data can be converted into something meaningful. Data preparation consists of the below phases.
Phase I: Data Validation
Data validation is done to understand if the collected data sample is per the pre-set standards, or it is a biased data sample again divided into four different stages
- Fraud: To ensure an actual human being records each response to the survey or the questionnaire
- Screening: To make sure each participant or respondent is selected or chosen in compliance with the research criteria
- Procedure: To ensure ethical standards were maintained while collecting the data sample
- Completeness: To ensure that the respondent has answered all the questions in an online survey. Else, the interviewer had asked all the questions devised in the questionnaire.
Phase II: Data Editing
More often, an extensive research data sample comes loaded with errors. Respondents sometimes fill in some fields incorrectly or sometimes skip them accidentally. Data editing is a process wherein the researchers have to confirm that the provided data is free of such errors. They need to conduct necessary checks and outlier checks to edit the raw edit and make it ready for analysis.
Phase III: Data Coding
Out of all three, this is the most critical phase of data preparation associated with grouping and assigning values to the survey responses . If a survey is completed with a 1000 sample size, the researcher will create an age bracket to distinguish the respondents based on their age. Thus, it becomes easier to analyze small data buckets rather than deal with the massive data pile.
LEARN ABOUT: Steps in Qualitative Research
After the data is prepared for analysis, researchers are open to using different research and data analysis methods to derive meaningful insights. For sure, statistical analysis plans are the most favored to analyze numerical data. In statistical analysis, distinguishing between categorical data and numerical data is essential, as categorical data involves distinct categories or labels, while numerical data consists of measurable quantities. The method is again classified into two groups. First, ‘Descriptive Statistics’ used to describe data. Second, ‘Inferential statistics’ that helps in comparing the data .
Descriptive statistics
This method is used to describe the basic features of versatile types of data in research. It presents the data in such a meaningful way that pattern in the data starts making sense. Nevertheless, the descriptive analysis does not go beyond making conclusions. The conclusions are again based on the hypothesis researchers have formulated so far. Here are a few major types of descriptive analysis methods.
Measures of Frequency
- Count, Percent, Frequency
- It is used to denote home often a particular event occurs.
- Researchers use it when they want to showcase how often a response is given.
Measures of Central Tendency
- Mean, Median, Mode
- The method is widely used to demonstrate distribution by various points.
- Researchers use this method when they want to showcase the most commonly or averagely indicated response.
Measures of Dispersion or Variation
- Range, Variance, Standard deviation
- Here the field equals high/low points.
- Variance standard deviation = difference between the observed score and mean
- It is used to identify the spread of scores by stating intervals.
- Researchers use this method to showcase data spread out. It helps them identify the depth until which the data is spread out that it directly affects the mean.
Measures of Position
- Percentile ranks, Quartile ranks
- It relies on standardized scores helping researchers to identify the relationship between different scores.
- It is often used when researchers want to compare scores with the average count.
For quantitative research use of descriptive analysis often give absolute numbers, but the in-depth analysis is never sufficient to demonstrate the rationale behind those numbers. Nevertheless, it is necessary to think of the best method for research and data analysis suiting your survey questionnaire and what story researchers want to tell. For example, the mean is the best way to demonstrate the students’ average scores in schools. It is better to rely on the descriptive statistics when the researchers intend to keep the research or outcome limited to the provided sample without generalizing it. For example, when you want to compare average voting done in two different cities, differential statistics are enough.
Descriptive analysis is also called a ‘univariate analysis’ since it is commonly used to analyze a single variable.
Inferential statistics
Inferential statistics are used to make predictions about a larger population after research and data analysis of the representing population’s collected sample. For example, you can ask some odd 100 audiences at a movie theater if they like the movie they are watching. Researchers then use inferential statistics on the collected sample to reason that about 80-90% of people like the movie.
Here are two significant areas of inferential statistics.
- Estimating parameters: It takes statistics from the sample research data and demonstrates something about the population parameter.
- Hypothesis test: I t’s about sampling research data to answer the survey research questions. For example, researchers might be interested to understand if the new shade of lipstick recently launched is good or not, or if the multivitamin capsules help children to perform better at games.
These are sophisticated analysis methods used to showcase the relationship between different variables instead of describing a single variable. It is often used when researchers want something beyond absolute numbers to understand the relationship between variables.
Here are some of the commonly used methods for data analysis in research.
- Correlation: When researchers are not conducting experimental research or quasi-experimental research wherein the researchers are interested to understand the relationship between two or more variables, they opt for correlational research methods.
- Cross-tabulation: Also called contingency tables, cross-tabulation is used to analyze the relationship between multiple variables. Suppose provided data has age and gender categories presented in rows and columns. A two-dimensional cross-tabulation helps for seamless data analysis and research by showing the number of males and females in each age category.
- Regression analysis: For understanding the strong relationship between two variables, researchers do not look beyond the primary and commonly used regression analysis method, which is also a type of predictive analysis used. In this method, you have an essential factor called the dependent variable. You also have multiple independent variables in regression analysis. You undertake efforts to find out the impact of independent variables on the dependent variable. The values of both independent and dependent variables are assumed as being ascertained in an error-free random manner.
- Frequency tables: The statistical procedure is used for testing the degree to which two or more vary or differ in an experiment. A considerable degree of variation means research findings were significant. In many contexts, ANOVA testing and variance analysis are similar.
- Analysis of variance: The statistical procedure is used for testing the degree to which two or more vary or differ in an experiment. A considerable degree of variation means research findings were significant. In many contexts, ANOVA testing and variance analysis are similar.
- Researchers must have the necessary research skills to analyze and manipulation the data , Getting trained to demonstrate a high standard of research practice. Ideally, researchers must possess more than a basic understanding of the rationale of selecting one statistical method over the other to obtain better data insights.
- Usually, research and data analytics projects differ by scientific discipline; therefore, getting statistical advice at the beginning of analysis helps design a survey questionnaire, select data collection methods, and choose samples.
LEARN ABOUT: Best Data Collection Tools
- The primary aim of data research and analysis is to derive ultimate insights that are unbiased. Any mistake in or keeping a biased mind to collect data, selecting an analysis method, or choosing audience sample il to draw a biased inference.
- Irrelevant to the sophistication used in research data and analysis is enough to rectify the poorly defined objective outcome measurements. It does not matter if the design is at fault or intentions are not clear, but lack of clarity might mislead readers, so avoid the practice.
- The motive behind data analysis in research is to present accurate and reliable data. As far as possible, avoid statistical errors, and find a way to deal with everyday challenges like outliers, missing data, data altering, data mining , or developing graphical representation.
LEARN MORE: Descriptive Research vs Correlational Research The sheer amount of data generated daily is frightening. Especially when data analysis has taken center stage. in 2018. In last year, the total data supply amounted to 2.8 trillion gigabytes. Hence, it is clear that the enterprises willing to survive in the hypercompetitive world must possess an excellent capability to analyze complex research data, derive actionable insights, and adapt to the new market needs.
LEARN ABOUT: Average Order Value
QuestionPro is an online survey platform that empowers organizations in data analysis and research and provides them a medium to collect data by creating appealing surveys.
MORE LIKE THIS
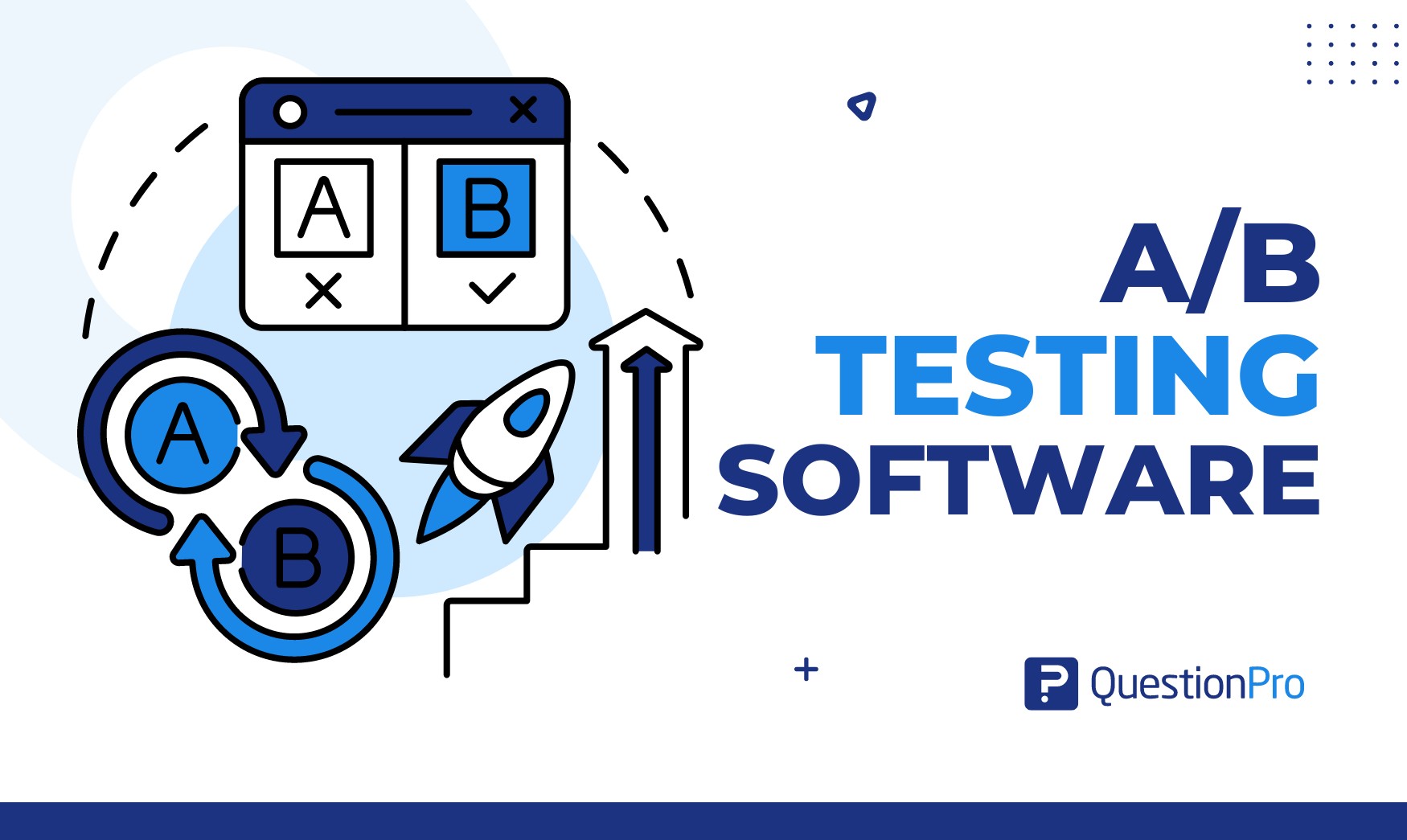
Top 13 A/B Testing Software for Optimizing Your Website
Apr 12, 2024
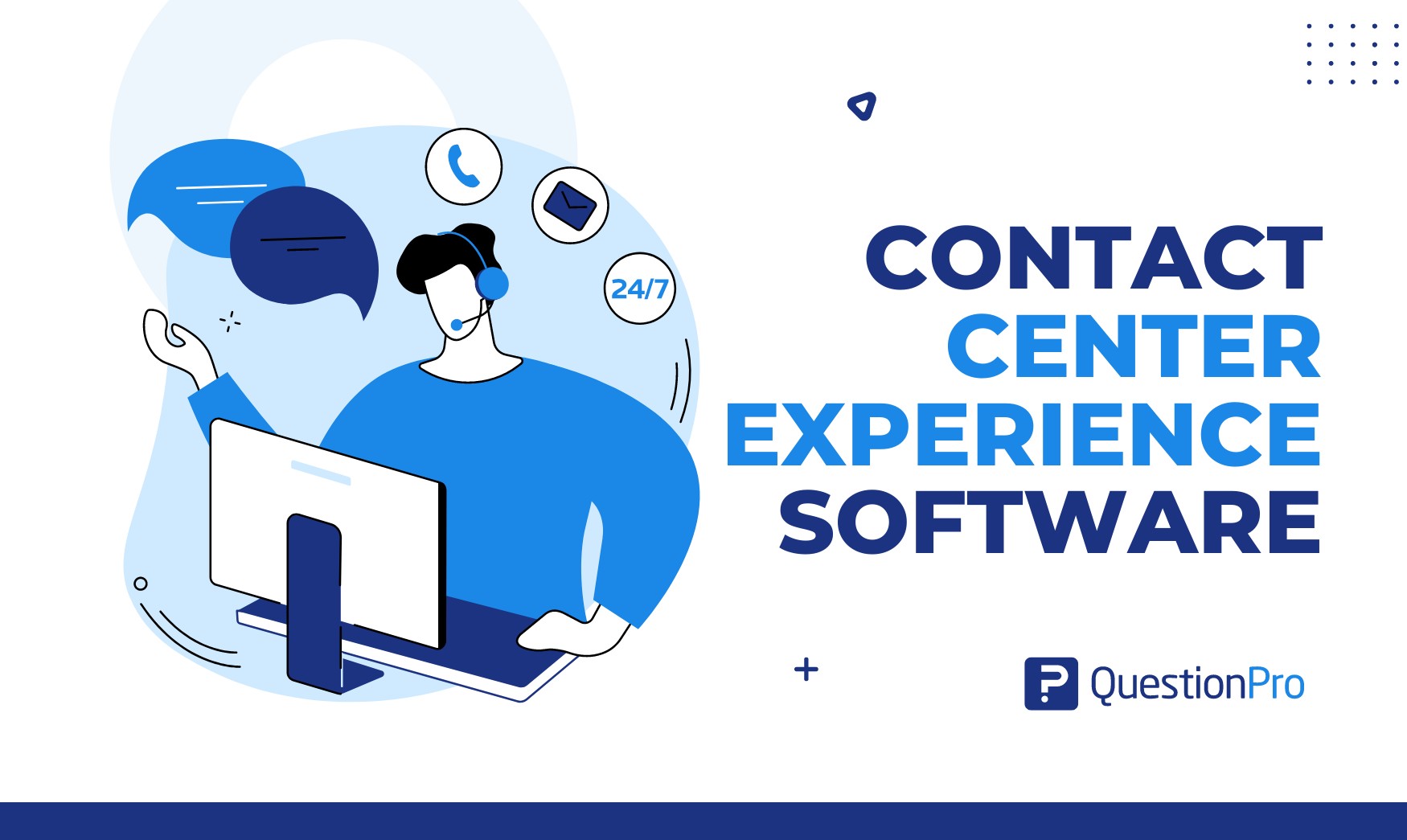
21 Best Contact Center Experience Software in 2024
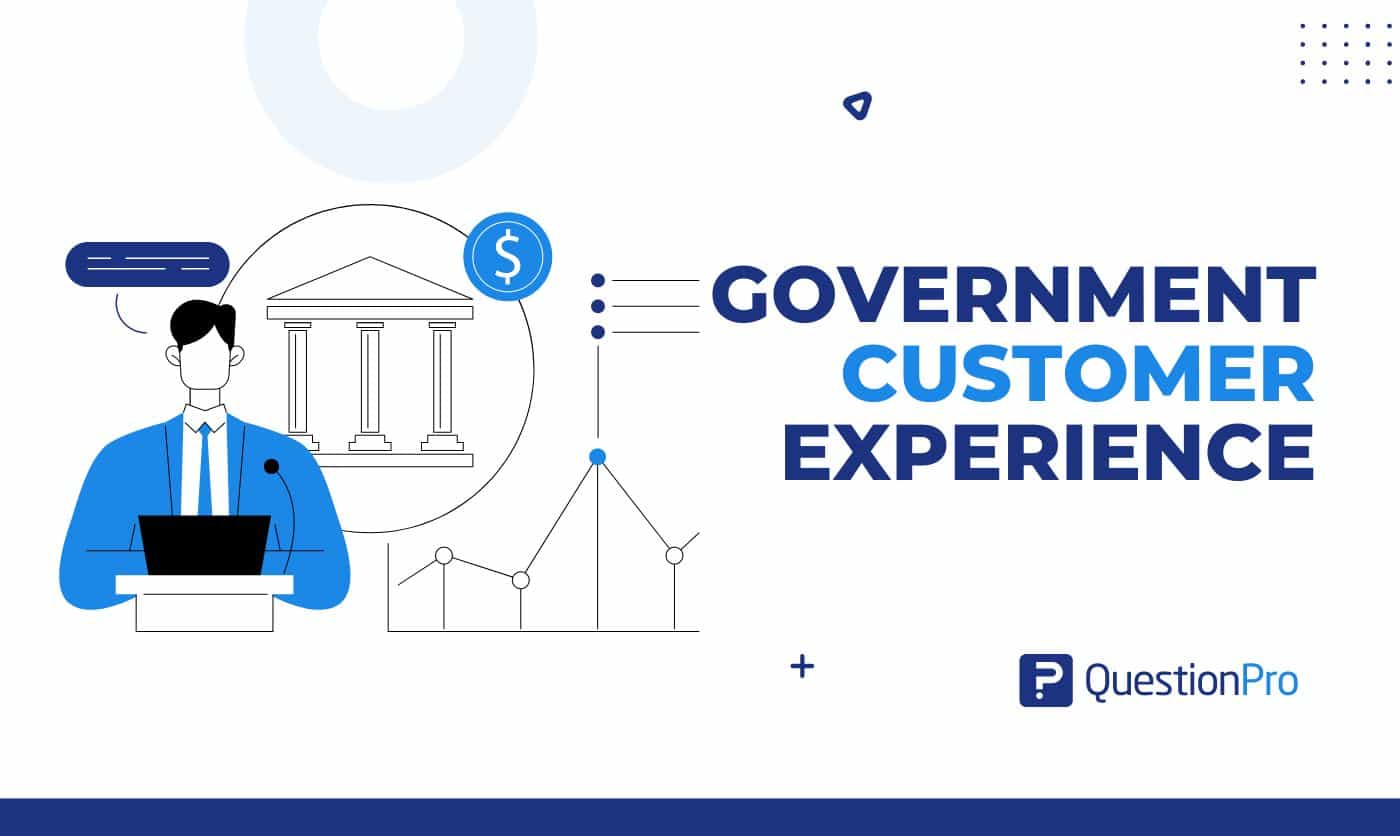
Government Customer Experience: Impact on Government Service
Apr 11, 2024
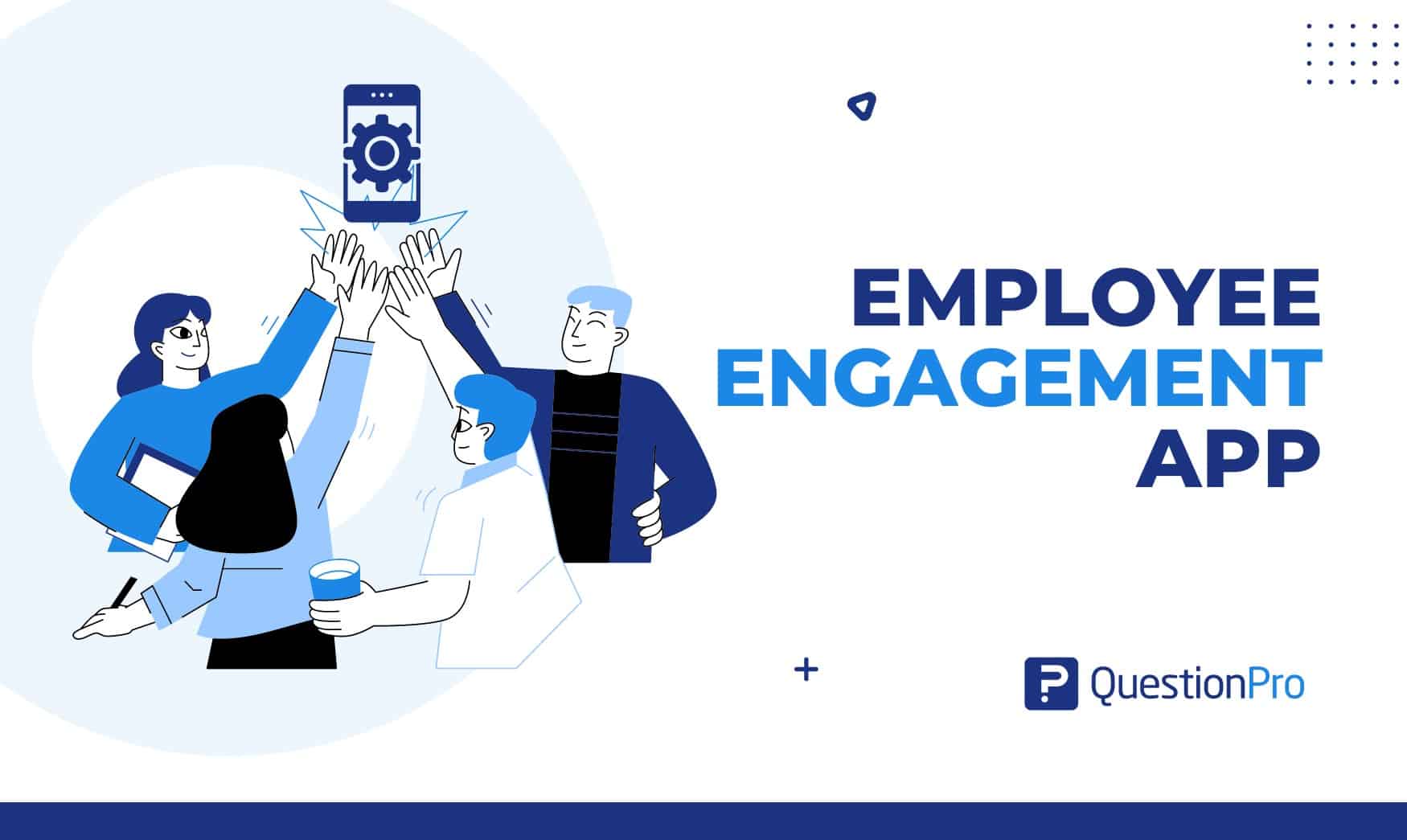
Employee Engagement App: Top 11 For Workforce Improvement
Apr 10, 2024
Other categories
- Academic Research
- Artificial Intelligence
- Assessments
- Brand Awareness
- Case Studies
- Communities
- Consumer Insights
- Customer effort score
- Customer Engagement
- Customer Experience
- Customer Loyalty
- Customer Research
- Customer Satisfaction
- Employee Benefits
- Employee Engagement
- Employee Retention
- Friday Five
- General Data Protection Regulation
- Insights Hub
- Life@QuestionPro
- Market Research
- Mobile diaries
- Mobile Surveys
- New Features
- Online Communities
- Question Types
- Questionnaire
- QuestionPro Products
- Release Notes
- Research Tools and Apps
- Revenue at Risk
- Survey Templates
- Training Tips
- Uncategorized
- Video Learning Series
- What’s Coming Up
- Workforce Intelligence
- Types of Data in Research: A Comprehensive Guide
This article explores the different types of research data, including qualitative vs quantitative, discrete vs continuous, nominal vs ordinal, and more. Learn how to combine qualitative and quantitative data in surveys for successful research projects.

When it comes to research, data is the foundation of any successful project. But what types of data are there? In this article, we'll explore the different types of data and when to use them. Qualitative and quantitative data are the two main types of data. Quantitative data is numerical and can be measured, while qualitative data is non-numerical and cannot be measured.
Quantitative data can be further divided into discrete and continuous data. Discrete data is a count that involves only whole numbers, while continuous data can be broken down into more precise levels. Nominal data is used only to label variables, without any type of quantitative value. Ordinal data shows where a number is in order.
Data can take many forms, from numerical values to images and sound recordings. Surveys remain one of the most effective types of core data that provide feedback directly from consumers. A good rule of thumb for defining whether a data is continuous or discrete is that if the measurement point can be halved and still makes sense, the data is continuous. If you are a businessman, marketer, data scientist , or other professional working with some types of data, you should be familiar with the key list of data types. Quantitative and qualitative data provide valuable information and do not conflict with each other.
The best way to combine qualitative and quantitative data in surveys is to include both multiple-choice and open-ended questions. In short, understanding the different types of data and when to use them is essential for any successful research project. Data variables can't be divided into smaller parts, so it's important to use the same type of currency for all values in the study. Working in the area of data management and having a good range of data science skills involves a deep understanding of various types of data and when to apply them.
Related Posts
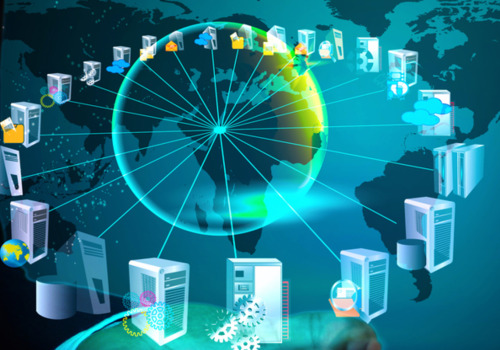
- The Ultimate Guide to Enterprise Data Management
This guide provides an overview of key elements of enterprise data management (EDM), including integration, cataloging & preparation; policies & regulations; security & governance.
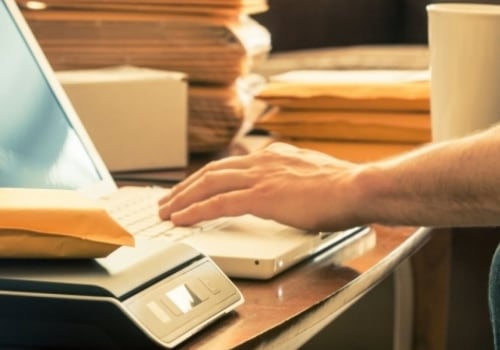
- Types of Management Tools: A Comprehensive Guide
Business management tools are essential for organizations to stay competitive in the market and improve their performance. This article provides an overview of the different types of management tools, their features, and how to choose the right one for your busines

- The Benefits of Using Management Tools for Businesses
Learn about how using management tools can help businesses increase efficiency by streamlining processes & automating tasks while saving time & money.
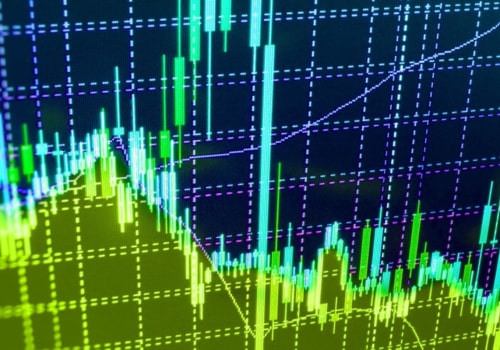
- The Significance of Data Management in Mathematics
Data management plays an essential role in today's digital economy. Learn why it's important and how it can help you get a successful job.
More articles
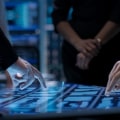
- How does data governance add value?
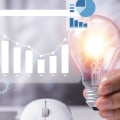
- Data Management: A Comprehensive Guide to the Process
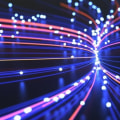
- 9 Best Practices of Master Data Management
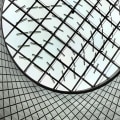
- Data Management Principles: A Comprehensive Guide
- Types of Data Management Functions Explained
- What are the 5 importance of data processing?
- Organizing IoT Device Data: A Comprehensive Guide
- Data Management: An Overview of the 5 Key Functions
The Benefits of Data Management in Biology
- 8 Core Principles of Data Management
- Data Management in Quantitative Research: An Expert's Guide
- Who will approve data management plan?
- Types of Data Management Tools: A Comprehensive Guide
- 4 Types of Data Management Explained
- Data Management: A Comprehensive Guide to Maximize Benefits
- A Comprehensive Guide to Data Management Plans
- What is the Role of Data Management in Organizations?
- Six Major Areas for Best Practices in Data Management
- The Essential Role of Data Management in Business
- What tools are used in data analysis?
- What is Data Services and How Does it Work?
- What are the 6 types of data analysis?
- The Ultimate Guide to Data Management
Data Management: A Comprehensive Guide
- The Benefits of Enterprise Data Management for Every Enterprise
- Unlocking the Secrets of Big Data Analytics: A Comprehensive Guide
- What are data management skills?
- Data Management: A Comprehensive Guide to Unlocking Data Potential
- The Benefits of Data Management: Unlocking the Potential of Data
- The Benefits of Data Management: Unlocking the Value of Data
- Data Management Standards: A Comprehensive Guide
- Data Management: A Comprehensive Guide for Researchers
- What are the 5 basic steps in data analysis?
- The Difference Between Enterprise Data Management and Data Management
- Data Management: Unlocking the Power of Data Analysis
- What are data standards and interoperability?
- Which of the following is api management tool?
- The Essential Role of Data Management in the Digital Economy
- Best Practices for Managing Big Data Analytics Programs
The Benefits of Understanding Project Data and Processes
- What should a data management plan include?
- Data Management: What is it and How to Use it?
- Data Management Plans: A Comprehensive Guide for Researchers
- A Comprehensive Guide to Database Management Systems
- What is data standard?
- Is sql a database management system?
- Data Management Tools: An Expert's Guide
- The Essential Role of Enterprise Data Management
- The Benefits of Data Management in Research
- Data Management: What is it and How to Implement it?
- Data Management Services: A Comprehensive Guide
- The Benefits of Enterprise Data Management for Businesses
- What software create and manage data base?
- What are the data management principles?
- What is Enterprise Data Management Framework?
- What is Enterprise Data Management and How to Implement It?
- The Benefits of Enterprise Data Management
- What are the best practices in big data adoption?
- What are spreadsheet data tools?
- Four Essential Best Practices for Big Data Governance
- Data Management: What You Need to Know
- What is Data Management Software and How Does it Work?
- Data Processing: Exploring the 3 Main Methods
- Do You Need Math to Become a Data Scientist?
- The Benefits of Good Data Management Explained
- What is data management framework in d365?
- The Benefits of Research Data Management
- Understanding Research Data Management Systems
- Why is data management and analysis important?
- The Advantages of Enterprise Data Management
- Data Management in Research: A Comprehensive Guide
- What is the Purpose of a Data Management Plan?
- How to Effectively Manage Big Data Analytics
- The Benefits of Data Management in Research: A Comprehensive Guide
- Data Management: Unlocking the Potential of Data
- Data Management in Research Studies: An Expert's Guide
- A Comprehensive Guide to Writing a Data Management Research Plan
- Data Management Tools: A Comprehensive Guide
- Data Management: A Comprehensive Guide to Activities Involved
- What is a data principle?
- How do you create a data standard?
- What is managing the data?
- Data Governance vs Data Management: What's the Difference?
- What is Data Management and How to Manage it Effectively?
New Articles
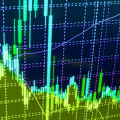
Which cookies do you want to accept?

An official website of the United States government
The .gov means it’s official. Federal government websites often end in .gov or .mil. Before sharing sensitive information, make sure you’re on a federal government site.
The site is secure. The https:// ensures that you are connecting to the official website and that any information you provide is encrypted and transmitted securely.
- Publications
- Account settings
Preview improvements coming to the PMC website in October 2024. Learn More or Try it out now .
- Advanced Search
- Journal List
- Indian J Crit Care Med
- v.23(Suppl 2); 2019 Jun
An Introduction to Statistics – Data Types, Distributions and Summarizing Data
Priya ranganathan.
1 Department of Anesthesiology, Tata Memorial Hospital, Homi Bhabha National Institute, Mumbai, Maharashtra, India
Nithya J Gogtay
2 Department of Clinical Pharmacology, King Edward Memorial Hospital, Mumbai, Maharashtra, India
The purpose of research is to gather data, which can then be used to inform decision-making. Data can be of various types and an understanding of this is crucial for its proper analysis and interpretation. In this article, we look at various types and distributions of data, and methods to summarize this data.
How to cite this article
Ranganathan P, Gogtay NJ, An Introduction to Statistics – Data Types, Distributions and Summarizing Data. Indian J Crit Care Med 2019;23(Suppl 2):S169–S170.
INTRODUCTION
In the first article of this series, we look at types of data and the methods used to describe or summarize data. Data is defined as ‘factual information (such as measurements or statistics) used as a basis for reasoning, discussion, or calculation’. 1 As statistics begins with data collection, understanding data is important, as it will help apply the right statistical tests, make the appropriate assumptions and draw meaningful and robust conclusions.
Classification of Data
At the highest level, data can be broadly classified as qualitative data (also known as categorical data ) or quantitative data (also known as numerical data ).
Qualitative or categorical data answers the question ‘What type’ or ‘Which category’. Gender (male/female), marital status (married/divorced/widowed/single), severity of pain on visual analog scale (mild/moderate/severe) are examples of qualitative or categorical data. Within categorical data, there are subtypes. Nominal data is data that does not follow any particular natural order, e.g. marital status (married / divorced/ widowed/ single). Here, there is no sequence between the various categories. In contrast, severity of pain can be ranked in ascending order as none/mild/moderate/severe/unbearable. This is known as ordinal data . Categorical data can also be classified depending on the number of categories. If only two categories are present e.g., dead/alive, married/unmarried – then the data is binary or dichotomous . If more than two categories are present e.g., married/divorced/widowed/single then this is non-binary data . In summary, qualitative data deals with characteristics, traits or descriptors or judgements.
Note: While collecting data, it is useful to collect it in as many categories as required so that information is not missed out. Subsequently, if needed, the researcher can rearrange the categories and collapse them into binary data or two categories for ease of analysis and interpretation.
Quantitative or numerical data is data that can be counted and answers the question ‘How many’ or ‘How much”. For example, age, height, weight, blood pressure, number of children, number of hospital visits. Numerical data can be further classified into discrete data and continuous data. Discrete data is data that can only be counted in whole numbers. e.g., number of hospital visits, Glasgow Coma Score, Richmond Agitation Sedation Scale, modified Rankin scale. This type of data cannot be represented in decimals – so Glasgow Coma Scale score can be 7 or 8 not 7.5. Continuous data is data that theoretically has no gap between data points can be counted in decimals (depending on the precision of the measuring instrument) e.g., blood glucose, hemoglobin, serum lipids. In summary, quantitative data deals with objective measurements.
Note: The same outcome can be measured as either numerical or categorical data depending on the measuring tool. For example, pain measured on a visual analog scale is categorical data (none/mild/moderate/severe/unbearable). The same outcome can be measured on a numerical rating scale of 0–10 where 0 represents no pain and 10 represents unbearable pain. Thus, the purpose of data is to finally answer the research question and the classification of data is not sacrosanct.
Distribution of Data
The distribution of data obtained from a sample is crucial to understand how to analyze it. There are various probability distributions for different types of data. Of these, one particularly important type used in everyday research is the distribution of continuous numerical data. This data may either be normally distributed or skewed.
Normally distributed data is data that follows a symmetric bell-shaped curve (Gaussian distribution) ( Fig. 1 ). Here, most of the data observations are centered around the middle and the curve gradually tapers on either side. Statisticians have formal methods of assessing normality of distribution. The simplest of these is to plot a histogram and visually inspect the distribution of the data. Other methods involve testing normality using a Q-Q plot or using the Kolmogorov Smirnov or the Shapiro Wilk tests.

Normal distribution of data - the mean, median and mode are fairly close to each other
As against this, skewed data does not have a symmetric distribution and may have a long tail on one side ( Fig. 2 ). Duration of surgery, duration of hospital stay or ICU stay are examples of data that is likely to be skewed.

Skewed distribution of data - the mean, median and mode differ from each other
Note: Distribution of data is important in the choice of statistical test for analysis. Tests that assume a normal or Gaussian distribution are known as parametric tests and tests that do not make assumptions about distribution are known as non-parametric or distribution-free tests. The former are in general more powerful tests.
Summarizing Data
It is often difficult to represent individual data from multiple participants in a study unless these numbers are really small. For example, if there are 100 participants in a study, we cannot enumerate the ages of each of the participants. We therefore use certain measures to summarize this data and allow us to represent it easily.
For categorical data, we use percentages and depict this using bar graphs or pie charts. Refer to Table 1 of the article by Nielsen and colleagues, in which baseline characteristics of participants are listed. 2 Gender, medical history and some of the characteristics of the cardiac arrest are examples of categorical data. These are shown as actual numbers with percentages in brackets.
For numerical data, we depict the ‘average’, which is “middle” of the data and the ‘dispersion’, which tells us how far away from the ‘average’ most of the values lie. If data is normally distributed, the average is the arithmetic mean . The spread of the data around the mean is depicted by the standard deviation . The larger the standard deviation, the more dispersed is the data (i.e., higher is the variance within the data). For example, in Table 1 of the article by Nielsen, age, body temperature, serum pH and serum lactate are all represented by the mean and standard deviation. 2 Similarly, in Table 1 of the article by Nishikimi, several baseline characteristics are represented by their mean and standard deviation. 3 For normally distributed data, the mean, median and mode are fairly close to each other.
For skewed data, the arithmetical mean does not give an idea of the true average. We therefore use another summary statistic called the median (or the 50th centile). For this, the data is arranged in ascending or descending order and divided into 100 equal parts (percentiles). The point that corresponds to the 50th percentile is the median (half of the observations are greater than and half are lesser than this value). The 25th to 75th percentiles give the inter-quartile range . In Table 1 of the article by Nielsen, time from cardiac arrest to event and Glasgow Coma scale are both examples of data that is represented by median and interquartile range. 2 Graphically, a box-and-whisker plot or a stem-and-leaf plot help good visualization of skewed data. Readers can refer to Table 2A of the article by Nishikimi, in which duration of ICU stay is depicted by a box and whiskers plot. 3 Unlike the mean, the median is not affected by extreme data points.
Rarely, one will find reference to another measure called the mode . The mode represents the observation that has the highest frequency. For example, if one was looking at age-wise distribution of incidence of a particular cancer, then the mode would tell us which age group had the highest incidence.
Care with Data
The science of statistics begins with collection of data. Apart from understanding data types, distributions and how to summarize data, we need to be meticulous in the process of collection and extremely careful in writing, entering data into software or excel sheets or transcribing data, as errors can seriously impact conclusions and the consequent decision making in patient care.
Source of support: Nil
Conflict of interest: None
- skip to Main Navigation
- skip to Main Content
- skip to Footer
- Accessibility feedback
- Data & Visualization and Research Support
- Data Management
Defining Research Data
One definition of research data is: "the recorded factual material commonly accepted in the scientific community as necessary to validate research findings." ( OMB Circular 110 ).
Research data covers a broad range of types of information (see examples below), and digital data can be structured and stored in a variety of file formats.
Note that properly managing data (and records) does not necessarily equate to sharing or publishing that data.
Examples of Research Data
Some examples of research data:
- Documents (text, Word), spreadsheets
- Laboratory notebooks, field notebooks, diaries
- Questionnaires, transcripts, codebooks
- Audiotapes, videotapes
- Photographs, films
- Protein or genetic sequences
- Test responses
- Slides, artifacts, specimens, samples
- Collection of digital objects acquired and generated during the process of research
- Database contents (video, audio, text, images)
- Models, algorithms, scripts
- Contents of an application (input, output, logfiles for analysis software, simulation software, schemas)
- Methodologies and workflows
- Standard operating procedures and protocols
Exclusions from Sharing
In addition to the other records to manage (below), some kinds of data may not be sharable due to the nature of the records themselves, or to ethical and privacy concerns. As defined by the OMB , this refers to:
- preliminary analyses,
- drafts of scientific papers,
- plans for future research,
- peer reviews, or
- communications with colleagues
Research data also do not include:
- Trade secrets, commercial information, materials necessary to be held confidential by a researcher until they are published, or similar information which is protected under law; and
- Personnel and medical information and similar information the disclosure of which would constitute a clearly unwarranted invasion of personal privacy, such as information that could be used to identify a particular person in a research study.
Some types of data, particularly software, may require special license to share. In those cases, contact the Office of Technology Transfer to review considerations for software generated in your research.
Other Records to Manage
Although they might not be addressed in an NSF data management plan, the following research records may also be important to manage during and beyond the life of a project.
- Correspondence (electronic mail and paper-based correspondence)
- Project files
- Grant applications
- Ethics applications
- Technical reports
- Research reports
- Signed consent forms
Adapted from Defining Research Data by the University of Oregon Libraries.
Opens in your default email client
URL copied to clipboard.
QR code for this page
Types of data #
In empirical research, we collect and interpret data in order to answer questions about the world. “Data” in this context usually results from some form of “measurement”. The notion of measurement here is very broad – it could include familiar acts like using a ruler to measure the length of an object, but it could also include asking a human research subject a question to “measure” their attitude about some topic.
Depending on the type of measurement that is made, the resulting data value can take different forms. Here we will discuss some of the common forms that can be taken by data.
Nominal data #
A nominal measurement is one that can take on any element in a finite set of unordered values. A commonly encountered nominal measurement that arises in research involving human subjects is biological race. There are many ways of “coding” race, but all of the common ways to do this would be considered to have a nominal form. The current United States Census Bureau approach to coding race uses five categories: Asian American, Black or African American, Native Americans and Alaska Native, Native Hawaiian and Other Pacific Islander, and White American. In some settings, subjects are given the option to respond that they have two or more races, and may be given the option to not respond. Since these race categories have no meaningful ordering, this is a nominal variable. Nominal variables are sometimes called categorical , or factor-type variables.
Nominal variables often arise in research involving human subjects, where common nominal variables include race, national origin (country of birth), biological sex, marital status, and immigration status. These may be broadly termed demographic traits .
Nominal variables arise in many other settings as well:
In medicine, we may be studying people with cancer, and there are many forms of cancer; for example, cancer can be classified based on the affected organ (lung, ovary, brain, etc.).
In market research, people have brand preferences, e.g. for toothpaste.
In astronomy, there are different types of stars (brown dwarf, red dwarf, neutron, etc.).
Many nominal variables are binary , meaning that they can only take on two possible values. These arise, for example, when the variable by definition refers to the presence of absence of some trait, e.g. responses to questions such as “do you have health insurance”, or “are you married”? These are sometimes called dichotomous traits. In some cases, the dichotomy is a simplification of a more complex reality. For example, we can ask someone whether they are currently employed, which is a binary choice, or we can give them more fine-grained options such as being employed full time, employed part time, retired, etc.
Ordinal data #
An ordinal variable is one that takes on values that can be ordered, but are not quantifiable to arbitrarily high precision. A common ordinal variable is educational attainment. This variable is often coded as (1) did not complete high school, (2) graduated from high school, (3) completed some college, (4) graduated from college, (5) completed some advanced or professional training beyond college. Note that in this setting, a person belongs to the highest level that is applicable, so that a person who completed college is in group (4) even though they also have a high school degree. Unlike a nominal variable, which cannot be ordered, there is a meaningful ordering for variables such as educational attainment.
Another common example of an ordinal variable would be a rating scale – suppose for example that patients with an injury are asked to rate their pain on a scale from 1 (least pain) to 10 (greatest pain). A very famous form of ordinal value is the five-point “Likert scale” used in questionnaires: the respondent is given a statement about a topic and can respond whether they “strongly agree”, “somewhat agree”, “neither agree nor disagree”, “somewhat disagree”, or “strongly disagree” with the statement.
A key property of an ordinal value is that the increments between adjacent categories cannot always be taken to have equal magnitudes. For example, we do not know whether the difference between “neither agree nor disagree” and “somewhat disagree” is equivalent to the difference between “somewhat disagree” and “strongly disagree”, even though these are both pairs of adjacent categories.
Quantitative data #
A “quantitative” measurement is one that can be made to very high accuracy on a numerical scale. Physical measurements such as length and weight are usually quantitative. A quantitative variable will usually have “units” associated with it, for example when measuring length the units may be centimeters. Income and age are two other measurements that would generally be considered to be quantitative, with units potentially being dollars and years, respectively.
Quantitative data are generally seen as containing more information than ordinal data, partly because they are generally measured to high precision, and also because unlike ordinal data, the presence of measurement units give a precise meaning to differences along the measurement scale. The difference between 5cm and 4cm, and the difference between 7cm and 6cm are both 1cm. On the other hand, as noted above, on a 10 point ordinal scale the difference between 5 and 4 may not convey the same meaning as the difference between 7 and 6, even though they are both one point differences.
Interval and ratio scales #
Sometimes, people refer to quantitative data as having an interval scale , and/or a ratio scale . An interval scale is one in which it makes sense to subtract two values, and a ratio scale is one in which it makes sense to divide two values. Most quantitative data have an interval scale, but it is not always the case that the scale of a quantitative variable is a ratio scale.
For example, suppose we are measuring temperature in degrees Fahrenheit, and we observe on a particular day that Cleveland and Atlanta reach high temperatures of 25 and 50 degrees, respectively. As an interval scale, it makes sense to subtract the two measurements and state that the Atlanta is 25 degrees warmer than the Cleveland. However it generally does not make sense to state that Atlanta is twice as warm as Cleveland based on Fahrenheit measurements. The origin (0 degree point) for Fahrenheit temperature is 32 degrees below the freezing point of water, which is a somewhat arbitrary value. To state that “Atlanta is twice as warm as Cleveland” would mean that Atlanta is twice as far from 0F compared to Cleveland, which is technically true but arguably not very meaningful. If we were to work on the centigrade scale, and we observe on a given day that the temperature in Atlanta is 20 degrees centigrade, and the temperature in Cleveland is 10 degrees centigrade, then we can make a stronger case for the scale having a ratio interpretation, since 0C is the freezing point of water, which has important real-world consequences.
Through this example, we can see that whether a scale is an interval and/or a ratio scale is somewhat a matter of judgment. The key thing to keep in mind at this point is that some pairs of quantitative variables can be compared through differences, some through ratios, and sometimes both type of comparisons make sense.
Practical considerations #
The typology described above is often useful, but in practice we encounter cases where it is ambiguous what type of data we have. We give examples of such ambiguous cases below. The key thing to keep in mind is that these terms are meant to help us communicate about our data. In some settings, terms such as “ordinal” or “ratio” may have unclear meanings. In that case, simply don’t use them in that setting.
For example, we might represent a person’s employment status with five levels: (i) employed full-time, (ii) employed part time, looking for full-time work, (iii) employed part time, not looking for full-time work, (iv) not employed, looking for work, (v) not employed, not looking for work. These could be treated as nominal, but alternatively, we could view this as an ordered spectrum in which (i) represents the greatest participation (desired or achieved) in the workforce, and (v) represents the least participation in the workforce. Thus, this variable could be viewed as either ordinal or nominal.
Considering educational attainment, we could code this variable as the number of years of schooling a person has completed. This could be taken to be quantitative, with units of years. However, it could also be considered to be ordinal. One reason to consider it as ordinal would be that it is much less common to have a partial year of schooling than to have a full year of schooling, and a person who completes, say, 11.5 years of schooling does not necessarily gain half of the benefit of their incomplete 12th year of schooling compared to someone with 12 full years of schooling. In other words, this scale is primarily discrete with whole-year increments, rather than being continuous with arbitrarily-fine increments.
Yet another type of ambiguity arises when we consider quantitative data. This type of data arises from measurements that are made at high precision, and are treated as real numbers. However, in practice every quantitative measurement has limited, finite precision due to the limitations of our measurement systems. For example, a digital scale may read out only to 1/100 of a gram. In some cases, a “quantitative” measurement made with very low precision might best be treated as ordinal.
When we consider the impact or consequences of changes in a variable’s value, we see that just because a variable is quantitative does not mean that every increment on its scale has the same consequences. For example, comparing temperatures of 33C to 30C, and 43C to 40C both result in a 3C difference of temperature. However, it may be argued that we care about a variable such as temperature only to the extent that it relates to something else that directly impacts us. For example, the “heat index” aims to reflect the comfort or discomfort that humans feel in environments with different temperatures. It takes account of the fact that a one degree change in temperature in a low humidity setting gives a lower perceived increase in heat than a one degree change in temperature in a high humidity setting. Thus, with respect to heat as perceived through the heat index, the temperature scale may not be an interval scale after all, since a fixed difference, say of 3 degrees C, does not always have the same impact on our preception of temperature.
Another useful example is the relationship between wealth and health. Wealth can be taken as a quantitative variable, defined to be the financial resources of an individual or family. In one sense, wealth is certainly an interval and a ratio scale. If one person has wealth of $400K, and another person has wealth of $100K, then the first person can buy $300K more stuff than the second person, suggesting that wealth is an interval scale. It is also true that the first person can buy four times more stuff than the second person, suggesting that wealth is a ratio scale. However, if we are focusing on wealth as it relates to health, then the first person may not tend to be any healthier than the second person. Both of these individuals have relatively high wealth compared to the population as a whole. It is well-established that both within and between countries, wealthier people tend to be healthier and live longer. But this effect is largely seen when contrasting the poorest individuals to individuals who are closer to the median, rather than contrasting wealthy to very wealthy individuals.
In summary, keep in mind that the purpose of the terms discussed here is to help us communicate, and we should not worry too much about the ambiguous cases. Instead, use these terms when it makes sense to do so, and avoid them in cases where it is not helpful.
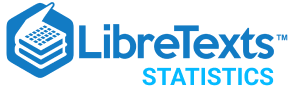
- school Campus Bookshelves
- menu_book Bookshelves
- perm_media Learning Objects
- login Login
- how_to_reg Request Instructor Account
- hub Instructor Commons
- Download Page (PDF)
- Download Full Book (PDF)
- Periodic Table
- Physics Constants
- Scientific Calculator
- Reference & Cite
- Tools expand_more
- Readability
selected template will load here
This action is not available.
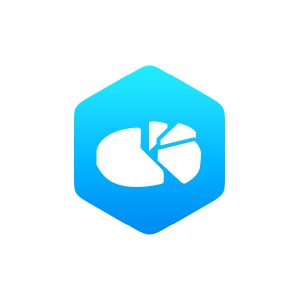
1.3: Types of Data and How to Collect Them
- Last updated
- Save as PDF
- Page ID 7081
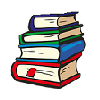
- Foster et al.
- University of Missouri-St. Louis, Rice University, & University of Houston, Downtown Campus via University of Missouri’s Affordable and Open Access Educational Resources Initiative
In order to use statistics, we need data to analyze. Data come in an amazingly diverse range of formats, and each type gives us a unique type of information. In virtually any form, data represent the measured value of variables. A variable is simply a characteristic or feature of the thing we are interested in understanding. In psychology, we are interested in people, so we might get a group of people together and measure their levels of stress (one variable), anxiety (a second variable), and their physical health (a third variable). Once we have data on these three variables, we can use statistics to understand if and how they are related. Before we do so, we need to understand the nature of our data: what they represent and where they came from.
Types of Variables
When conducting research, experimenters often manipulate variables. For example, an experimenter might compare the effectiveness of four types of antidepressants. In this case, the variable is “type of antidepressant.” When a variable is manipulated by an experimenter, it is called an independent variable. The experiment seeks to determine the effect of the independent variable on relief from depression. In this example, relief from depression is called a dependent variable. In general, the independent variable is manipulated by the experimenter and its effects on the dependent variable are measured.
Example \(\PageIndex{1}\)
Can blueberries slow down aging? A study indicates that antioxidants found in blueberries may slow down the process of aging. In this study, \(19\)-month-old rats (equivalent to \(60\)-year-old humans) were fed either their standard diet or a diet supplemented by either blueberry, strawberry, or spinach powder. After eight weeks, the rats were given memory and motor skills tests. Although all supplemented rats showed improvement, those supplemented with blueberry powder showed the most notable improvement.
- What is the independent variable? (dietary supplement: none, blueberry, strawberry, and spinach)
- What are the dependent variables? (memory test and motor skills test)
Example \(\PageIndex{2}\)
Does beta-carotene protect against cancer? Beta-carotene supplements have been thought to protect against cancer. However, a study published in the Journal of the National Cancer Institute suggests this is false. The study was conducted with 39,000 women aged 45 and up. These women were randomly assigned to receive a beta-carotene supplement or a placebo, and their health was studied over their lifetime. Cancer rates for women taking the betacarotene supplement did not differ systematically from the cancer rates of those women taking the placebo.
- What is the independent variable? (supplements: beta-carotene or placebo)
- What is the dependent variable? (occurrence of cancer)
Example \(\PageIndex{3}\)
How bright is right? An automobile manufacturer wants to know how bright brake lights should be in order to minimize the time required for the driver of a following car to realize that the car in front is stopping and to hit the brakes.
- What is the independent variable? (brightness of brake lights)
- What is the dependent variable? (time to hit brakes)
Levels of an Independent Variable
If an experiment compares an experimental treatment with a control treatment, then the independent variable (type of treatment) has two levels: experimental and control. If an experiment were comparing five types of diets, then the independent variable (type of diet) would have \(5\) levels. In general, the number of levels of an independent variable is the number of experimental conditions.
Qualitative and Quantitative Variables
An important distinction between variables is between qualitative variables and quantitative variables. Qualitative variables are those that express a qualitative attribute such as hair color, eye color, religion, favorite movie, gender, and so on. The values of a qualitative variable do not imply a numerical ordering. Values of the variable “religion” differ qualitatively; no ordering of religions is implied. Qualitative variables are sometimes referred to as categorical variables. Quantitative variables are those variables that are measured in terms of numbers. Some examples of quantitative variables are height, weight, and shoe size.
In the study on the effect of diet discussed previously, the independent variable was type of supplement: none, strawberry, blueberry, and spinach. The variable “type of supplement” is a qualitative variable; there is nothing quantitative about it. In contrast, the dependent variable “memory test” is a quantitative variable since memory performance was measured on a quantitative scale (number correct).
Discrete and Continuous Variables
Variables such as number of children in a household are called discrete variables since the possible scores are discrete points on the scale. For example, a household could have three children or six children, but not \(4.53\) children. Other variables such as “time to respond to a question” are continuous variables since the scale is continuous and not made up of discrete steps. The response time could be \(1.64\) seconds, or it could be \(1.64237123922121\) seconds. Of course, the practicalities of measurement preclude most measured variables from being truly continuous.
Levels of Measurement
Before we can conduct a statistical analysis, we need to measure our dependent variable. Exactly how the measurement is carried out depends on the type of variable involved in the analysis. Different types are measured differently. To measure the time taken to respond to a stimulus, you might use a stop watch. Stop watches are of no use, of course, when it comes to measuring someone's attitude towards a political candidate. A rating scale is more appropriate in this case (with labels like “very favorable,” “somewhat favorable,” etc.). For a dependent variable such as “favorite color,” you can simply note the color-word (like “red”) that the subject offers.
Although procedures for measurement differ in many ways, they can be classified using a few fundamental categories. In a given category, all of the procedures share some properties that are important for you to know about. The categories are called “scale types,” or just “scales,” and are described in this section.
Nominal scales
When measuring using a nominal scale, one simply names or categorizes responses. Gender, handedness, favorite color, and religion are examples of variables measured on a nominal scale. The essential point about nominal scales is that they do not imply any ordering among the responses. For example, when classifying people according to their favorite color, there is no sense in which green is placed “ahead of” blue. Responses are merely categorized. Nominal scales embody the lowest level of measurement.
Ordinal scales
A researcher wishing to measure consumers' satisfaction with their microwave ovens might ask them to specify their feelings as either “very dissatisfied,” “somewhat dissatisfied,” “somewhat satisfied,” or “very satisfied.” The items in this scale are ordered, ranging from least to most satisfied. This is what distinguishes ordinal from nominal scales. Unlike nominal scales, ordinal scales allow comparisons of the degree to which two subjects possess the dependent variable. For example, our satisfaction ordering makes it meaningful to assert that one person is more satisfied than another with their microwave ovens. Such an assertion reflects the first person's use of a verbal label that comes later in the list than the label chosen by the second person.
On the other hand, ordinal scales fail to capture important information that will be present in the other scales we examine. In particular, the difference between two levels of an ordinal scale cannot be assumed to be the same as the difference between two other levels. In our satisfaction scale, for example, the difference between the responses “very dissatisfied” and “somewhat dissatisfied” is probably not equivalent to the difference between “somewhat dissatisfied” and “somewhat satisfied.” Nothing in our measurement procedure allows us to determine whether the two differences reflect the same difference in psychological satisfaction. Statisticians express this point by saying that the differences between adjacent scale values do not necessarily represent equal intervals on the underlying scale giving rise to the measurements. (In our case, the underlying scale is the true feeling of satisfaction, which we are trying to measure.) What if the researcher had measured satisfaction by asking consumers to indicate their level of satisfaction by choosing a number from one to four? Would the difference between the responses of one and two necessarily reflect the same difference in satisfaction as the difference between the responses two and three? The answer is No. Changing the response format to numbers does not change the meaning of the scale. We still are in no position to assert that the mental step from \(1\) to \(2\) (for example) is the same as the mental step from \(3\) to \(4\).
Interval scales
Interval scales are numerical scales in which intervals have the same interpretation throughout. As an example, consider the Fahrenheit scale of temperature. The difference between \(30\) degrees and \(40\) degrees represents the same temperature difference as the difference between \(80\) degrees and \(90\) degrees. This is because each \(10\)-degree interval has the same physical meaning (in terms of the kinetic energy of molecules). Interval scales are not perfect, however. In particular, they do not have a true zero point even if one of the scaled values happens to carry the name “zero.” The Fahrenheit scale illustrates the issue. Zero degrees Fahrenheit does not represent the complete absence of temperature (the absence of any molecular kinetic energy). In reality, the label “zero” is applied to its temperature for quite accidental reasons connected to the history of temperature measurement. Since an interval scale has no true zero point, it does not make sense to compute ratios of temperatures. For example, there is no sense in which the ratio of \(40\) to \(20\) degrees Fahrenheit is the same as the ratio of \(100\) to \(50\) degrees; no interesting physical property is preserved across the two ratios. After all, if the “zero” label were applied at the temperature that Fahrenheit happens to label as \(10\) degrees, the two ratios would instead be \(30\) to \(10\) and \(90\) to \(40\), no longer the same! For this reason, it does not make sense to say that \(80\) degrees is “twice as hot” as \(40\) degrees. Such a claim would depend on an arbitrary decision about where to “start” the temperature scale, namely, what temperature to call zero (whereas the claim is intended to make a more fundamental assertion about the underlying physical reality).
Ratio scales
The ratio scale of measurement is the most informative scale. It is an interval scale with the additional property that its zero position indicates the absence of the quantity being measured. You can think of a ratio scale as the three earlier scales rolled up in one. Like a nominal scale, it provides a name or category for each object (the numbers serve as labels). Like an ordinal scale, the objects are ordered (in terms of the ordering of the numbers). Like an interval scale, the same difference at two places on the scale has the same meaning. And in addition, the same ratio at two places on the scale also carries the same meaning. The Fahrenheit scale for temperature has an arbitrary zero point and is therefore not a ratio scale. However, zero on the Kelvin scale is absolute zero. This makes the Kelvin scale a ratio scale. For example, if one temperature is twice as high as another as measured on the Kelvin scale, then it has twice the kinetic energy of the other temperature. Another example of a ratio scale is the amount of money you have in your pocket right now (25 cents, 55 cents, etc.). Money is measured on a ratio scale because, in addition to having the properties of an interval scale, it has a true zero point: if you have zero money, this implies the absence of money. Since money has a true zero point, it makes sense to say that someone with 50 cents has twice as much money as someone with 25 cents (or that Bill Gates has a million times more money than you do).
What level of measurement is used for psychological variables?
Rating scales are used frequently in psychological research. For example, experimental subjects may be asked to rate their level of pain, how much they like a consumer product, their attitudes about capital punishment, their confidence in an answer to a test question. Typically these ratings are made on a 5-point or a 7-point scale. These scales are ordinal scales since there is no assurance that a given difference represents the same thing across the range of the scale. For example, there is no way to be sure that a treatment that reduces pain from a rated pain level of 3 to a rated pain level of 2 represents the same level of relief as a treatment that reduces pain from a rated pain level of 7 to a rated pain level of 6.
In memory experiments, the dependent variable is often the number of items correctly recalled. What scale of measurement is this? You could reasonably argue that it is a ratio scale. First, there is a true zero point; some subjects may get no items correct at all. Moreover, a difference of one represents a difference of one item recalled across the entire scale. It is certainly valid to say that someone who recalled 12 items recalled twice as many items as someone who recalled only 6 items.
But number-of-items recalled is a more complicated case than it appears at first. Consider the following example in which subjects are asked to remember as many items as possible from a list of 10. Assume that (a) there are 5 easy items and 5 difficult items, (b) half of the subjects are able to recall all the easy items and different numbers of difficult items, while (c) the other half of the subjects are unable to recall any of the difficult items but they do remember different numbers of easy items. Some sample data are shown below.
Let's compare (i) the difference between Subject A's score of 2 and Subject B's score of 3 and (ii) the difference between Subject C's score of 7 and Subject D's score of 8. The former difference is a difference of one easy item; the latter difference is a difference of one difficult item. Do these two differences necessarily signify the same difference in memory? We are inclined to respond “No” to this question since only a little more memory may be needed to retain the additional easy item whereas a lot more memory may be needed to retain the additional hard itemred. The general point is that it is often inappropriate to consider psychological measurement scales as either interval or ratio.
Consequences of level of measurement Why are we so interested in the type of scale that measures a dependent variable? The crux of the matter is the relationship between the variable's level of measurement and the statistics that can be meaningfully computed with that variable. For example, consider a hypothetical study in which 5 children are asked to choose their favorite color from blue, red, yellow, green, and purple. The researcher codes the results as follows:
This means that if a child said her favorite color was “Red,” then the choice was coded as “2,” if the child said her favorite color was “Purple,” then the response was coded as 5, and so forth. Consider the following hypothetical data
Each code is a number, so nothing prevents us from computing the average code assigned to the children. The average happens to be 3, but you can see that it would be senseless to conclude that the average favorite color is yellow (the color with a code of 3). Such nonsense arises because favorite color is a nominal scale, and taking the average of its numerical labels is like counting the number of letters in the name of a snake to see how long the beast is.
Does it make sense to compute the mean of numbers measured on an ordinal scale? This is a difficult question, one that statisticians have debated for decades. The prevailing (but by no means unanimous) opinion of statisticians is that for almost all practical situations, the mean of an ordinally-measured variable is a meaningful statistic. However, there are extreme situations in which computing the mean of an ordinally-measured variable can be very misleading.

- Harvard Library
- Research Guides
- Harvard Graduate School of Education - Gutman Library
Education Data and Statistics
- Types of Data
- Data Sharing and Policies
- Data vs. Statistics
- United States Data Sources
- International Data Sources
- Research Support

Qualitative and Quantitative Data:
Education schools research and analyze two main types of data: qualitative and quantitative. Scholars typically define their methodology by either of these categories or as a mixed method combining both. Qualitative data tells a story. Such data are non-numerical in nature. They also have a low level of measurability. Qualitative data can include language or opinions. For example, in education, classroom observations or interviews with parents of students regarding family history are qualitative. Quantitative data also tells a story but in a different way. Quantitative data are expressed numerically and possess a high degree of measurability. For instance, quantitative data includes test scores, class rank, and school student populations.

Nominal and Ordinal Data:
Qualitative data can be further subdivided into nominal and ordinal categories. The nominal category labels qualitative data without any inherent rank or order. This would include categorizing students in terms of nationality or gender as well nonbinary pronouns. Ordinal data does possess an innate sense of rank but needs to be converted numerically for quantitative analysis and hence are categorized as qualitative. For example, credit/no-credit grading, class rank, and level of educational attainment are ordinal data.
Discrete and Continuous Data:
Quantitative data is composed of two categories, including discrete and continuous. Discrete data can be measured and counted as finite values assigning to whole numbers or integers that cannot be decomposed into fractions or decimals. Examples are student attendance or number of in-service professional development days for faculty. Continuous data entails information that can be subdivided and assume a value within a range. Test-taking time or GPA are examples of continuous data.
VISUALIZATION
- ATLAS.ti ATLAS.ti supports a wide range of data formats and offers functionalities like coding, annotation, and visualization, enabling researchers to uncover deep insights from textual, graphical, audio, and video data.
- Excel Microsoft software spreadsheet tool for data entry, analysis, and presentation.
- Qualtrics (Harvard Key required) Qualtrics is a web-based survey software that allows you to easily create surveys with customizable templates, integrated graphics, and statistical tools; collect, store, and download data in a variety of formats; and produce reports.
- Tableau Tableau is a data visualization tool that helps in transforming raw data into easily understandable and interactive visual formats.
COMPUTATION
- Express Scribe (Harvard Key required) Tool for transcription encouraged by the Harvard qualitative research support group.
- MATLAB Iterative analysis and design for creating scripts and combining code.
- Mplus Statistical modeling tool for data analysis.
- NVivo NVivo is a qualitative data analysis (QDA) computer software package designed for researchers working with diverse data types such as interviews, social media, surveys, and articles.
- OpenRefine OpenRefine is an open-source tool for cleaning and transforming data. It is useful in handling messy data, allowing users to standardize and clean datasets.
- Python Libraries This library reference manual describes the standard library that is distributed with Python. It also describes some of the optional components that are commonly included in Python distributions.
- R Packages R packages are extensions to the R programming language, consisting of a collection of functions, data, and compiled code, designed to add specific capabilities or perform specialized tasks. Hosted on repositories like CRAN (Comprehensive R Archive Network), these packages cover a wide range of statistical, graphical, and data manipulation functionalities.
- QDA Miner QDA Miner is designed to assist in the analysis of textual data such as interviews, open-ended responses, articles, and reports. It offers tools for coding, annotating, retrieving, and analyzing collections of documents and images.
- SAS SAS (Statistical Analysis System) is a robust, enterprise-grade software suite developed by SAS Institute for advanced analytics, multivariate analysis, business intelligence, data management, and predictive analytics.
- SPSS SPSS (Statistical Package for the Social Sciences) offers a range of statistical analysis tools, along with data management and documentation capabilities. SPSS is particularly favored for its user-friendly interface, making complex data analyses more accessible to those without extensive statistical backgrounds, and is extensively used in research, market analysis, health research, and educational sectors.
- STATA Data science software package typically used for statistics coursework.
OTHER: INSTRUCTION/ REFERENCES/ CITATIONS/ RESEARCH PROCEDURES
- APA Reference Guidelines APA guidelines on citations and references for academic writing.
- Digital Scholarship at Harvard Digital literacy, research support, and teaching support.
- Endnote Software tool for bibliography building and citation.
- IRB and Ethics (Harvard Key required) Harvard resource for IRB approval when working with human subjects in research.
- RefWorks An internet based reference manager for building bibliographies, formatting, and writing in-line citations (Write-N-Cite).
- Zotero A research organization tool for references and citations.
- << Previous: Data vs. Statistics
- Next: United States Data Sources >>
- Last Updated: Feb 8, 2024 11:06 AM
- URL: https://guides.library.harvard.edu/hgsedata
Harvard University Digital Accessibility Policy
Research Data Management
What is research data, types of data, research data life cycle, data formats, format conversion, data documentation, naming conventions, license and attributions.
- Make Data Meaningful
- Data Storage
- Ethical Considerations
- Data Sharing Policies
- Archiving & Preservation
Data is often thought of in quantitative terms. Much research data is indeed quantitative, but 'number' and 'data' are not synonymous. Data is typically considered in two broad categories: quantitative and qualitative.
Quantitative data can include experimental measurements, e.g. lab instrument data, sensor readings, survey results, and test/simulation models. Qualitative data can include text, audio, images, and video. Some definitions of data are quite broad, and include objects such as laboratory specimens.
Some types of research data and data files are fairly ubiquitous and can be found distributed across disciplines:
- Mapping/GIS data
- Numerical measurements
Research data in Social Sciences can include:
- Survey responses
- Focus group and individual interviews
- Economic indicators
- Demographics
- Opinion polling
Research data in Hard Sciences can include:
- Measurements generated by sensors/laboratory instruments
- Computer modeling
- Simulations
- Observations and/or field studies
Stages of Data Related to Research Data Life Cycle
- Raw Data: What is being measured or observed? The data being generated during the research project.
- Processed Data: Making the raw data useful/manipulable
- Analyzed Data: Manipulated/interpreted data. What does the data tell us? Is it significant? How so?
- Finalized/Published Data: How do the data support your research question?
- Existing Data across Different Sources: e.g. GIS data
These file format characteristics ensure the best chances for long-term access:
- Non-proprietary or open formats are readable by more than just the equipment and/or program that generated it. Sometimes proprietary file formats are unavoidable. However, proprietary formats can often be converted to open formats. Please see the following section for more on this topic.
- Unencrypted and uncompressed files offer the best prospects for long-term access. If files are encrypted and/or compressed, the method of encryption/compression used will need to be both discoverable and usable for file access in the future.
Here are some examples of preferred formats for various data types (from https://lib.stanford.edu/data-management-services/file-formats):
- Containers: TAR, GZIP, ZIP
- Databases: XML, CSV
- Geospatial: SHP, DBF, GeoTIFF, NetCDF
- Moving Images: MOV, MPEG, AVI, MXF
- Audio: WAVE, AIFF, MP3, MXF
- Numbers/statistics: ASCII, DTA, POR, SAS, SAV
- Images: TIFF, JPEG 2000, PDF, PNG, GIF, BMP
- Text: PDF/A, HTML, ASCII, XML, UTF-8
- Web Archive: WARC
For a list of common file formats and evaluations of format quality and long-term sustainability see http://www.digitalpreservation.gov/formats/fdd/browse_list.shtml
Some archives specify the optimal data formats they use for long-term preservation of data.
Proprietary systems and file formats can resist attempts at data integration, reuse, and sharing. These barriers can often be addressed by converting proprietary formats to open formats. The protocols and solutions for doing so can be discipline-specific (e.g. https://docs.openmicroscopy.org/bio-formats/6.9.0/supported-formats.html ), but some general guidelines apply.
Information can be lost when converting file formats. When data is converted from one format to another - through export or by using data translation software - certain changes may occur to the data:
- For data held in statistical packages, spreadsheets or databases, some data or internal metadata such as missing value definitions, decimal numbers, formulae or variable labels may be lost during conversion to another format, or data may be truncated
- For textual data, editing such as highlighting, bold text or headers/footers may be lost
After conversion data should be checked for errors or changes.
To mitigate the risk of lost information:
- Note conversion steps taken
- If possible, keep the original file as well as the converted one
Data documentation explains the who/what/where/when/why of data:
- Who collected this data? Who/what were the subjects under study?
- What was collected, and for what purpose? What is the content/structure of the data?
- Where was this data collected? What were the experimental conditions?
- When was this data collected? Is it part of a series, or ongoing experiment?
- Why was this experiment performed?
Good data documentation helps you, the researcher. Clear documentation makes it easier to interpret your findings later, helps facilitate collaboration, sharing, and reuse, and can also help ensure successful long-term preservation of your research findings.
Data documentation practices vary by discipline. These methods include lab notebooks data dictionaries and codebooks in the social sciences, and well-documented/commented code for computer science (or for really any project that uses code and/or scripting).
While formats and methods for documentation differ, the general idea is always to describe:
- What the data is
- When the data was collected
- Where it was collected
- How it was collected
- Notes about the data characteristics (including file formats/potential format conversions), and
- Any pertinent notes about experimental conditions.
Note that for collaborative research projects, it’s important to come to some agreement among members of the project team that will help ensure consistent data documentation practices by all.
No matter what, you need to have:
- File naming conventions
- Version control
Why Use File Naming Conventions?
Naming conventions make life easier.
- Help you find your data
- Help others find your data
- Help track which version of a file is most current
What File Naming Convention Should I Use?
Has your research group established a convention?
If not, general guidelines include:
- Meaningful file names that aren’t too long
- Avoid certain characters
- Dates can help with sorting and version control
Much of this guide was adapted from the New England Collaborative Data Management Curriculum .

This work is licensed under a Creative Commons Attribution-NonCommercial-ShareAlike 4.0 International License .
- << Previous: Overview
- Next: Make Data Meaningful >>
- Last Updated: Mar 18, 2024 9:59 AM
- URL: https://tnstate.libguides.com/datamanagement
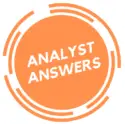
Data & Finance for Work & Life

Data Analysis: Types, Methods & Techniques (a Complete List)
( Updated Version )
While the term sounds intimidating, “data analysis” is nothing more than making sense of information in a table. It consists of filtering, sorting, grouping, and manipulating data tables with basic algebra and statistics.
In fact, you don’t need experience to understand the basics. You have already worked with data extensively in your life, and “analysis” is nothing more than a fancy word for good sense and basic logic.
Over time, people have intuitively categorized the best logical practices for treating data. These categories are what we call today types , methods , and techniques .
This article provides a comprehensive list of types, methods, and techniques, and explains the difference between them.
For a practical intro to data analysis (including types, methods, & techniques), check out our Intro to Data Analysis eBook for free.
Descriptive, Diagnostic, Predictive, & Prescriptive Analysis
If you Google “types of data analysis,” the first few results will explore descriptive , diagnostic , predictive , and prescriptive analysis. Why? Because these names are easy to understand and are used a lot in “the real world.”
Descriptive analysis is an informational method, diagnostic analysis explains “why” a phenomenon occurs, predictive analysis seeks to forecast the result of an action, and prescriptive analysis identifies solutions to a specific problem.
That said, these are only four branches of a larger analytical tree.
Good data analysts know how to position these four types within other analytical methods and tactics, allowing them to leverage strengths and weaknesses in each to uproot the most valuable insights.
Let’s explore the full analytical tree to understand how to appropriately assess and apply these four traditional types.
Tree diagram of Data Analysis Types, Methods, and Techniques
Here’s a picture to visualize the structure and hierarchy of data analysis types, methods, and techniques.
If it’s too small you can view the picture in a new tab . Open it to follow along!

Note: basic descriptive statistics such as mean , median , and mode , as well as standard deviation , are not shown because most people are already familiar with them. In the diagram, they would fall under the “descriptive” analysis type.
Tree Diagram Explained
The highest-level classification of data analysis is quantitative vs qualitative . Quantitative implies numbers while qualitative implies information other than numbers.
Quantitative data analysis then splits into mathematical analysis and artificial intelligence (AI) analysis . Mathematical types then branch into descriptive , diagnostic , predictive , and prescriptive .
Methods falling under mathematical analysis include clustering , classification , forecasting , and optimization . Qualitative data analysis methods include content analysis , narrative analysis , discourse analysis , framework analysis , and/or grounded theory .
Moreover, mathematical techniques include regression , Nïave Bayes , Simple Exponential Smoothing , cohorts , factors , linear discriminants , and more, whereas techniques falling under the AI type include artificial neural networks , decision trees , evolutionary programming , and fuzzy logic . Techniques under qualitative analysis include text analysis , coding , idea pattern analysis , and word frequency .
It’s a lot to remember! Don’t worry, once you understand the relationship and motive behind all these terms, it’ll be like riding a bike.
We’ll move down the list from top to bottom and I encourage you to open the tree diagram above in a new tab so you can follow along .
But first, let’s just address the elephant in the room: what’s the difference between methods and techniques anyway?
Difference between methods and techniques
Though often used interchangeably, methods ands techniques are not the same. By definition, methods are the process by which techniques are applied, and techniques are the practical application of those methods.
For example, consider driving. Methods include staying in your lane, stopping at a red light, and parking in a spot. Techniques include turning the steering wheel, braking, and pushing the gas pedal.
Data sets: observations and fields
It’s important to understand the basic structure of data tables to comprehend the rest of the article. A data set consists of one far-left column containing observations, then a series of columns containing the fields (aka “traits” or “characteristics”) that describe each observations. For example, imagine we want a data table for fruit. It might look like this:
Now let’s turn to types, methods, and techniques. Each heading below consists of a description, relative importance, the nature of data it explores, and the motivation for using it.
Quantitative Analysis
- It accounts for more than 50% of all data analysis and is by far the most widespread and well-known type of data analysis.
- As you have seen, it holds descriptive, diagnostic, predictive, and prescriptive methods, which in turn hold some of the most important techniques available today, such as clustering and forecasting.
- It can be broken down into mathematical and AI analysis.
- Importance : Very high . Quantitative analysis is a must for anyone interesting in becoming or improving as a data analyst.
- Nature of Data: data treated under quantitative analysis is, quite simply, quantitative. It encompasses all numeric data.
- Motive: to extract insights. (Note: we’re at the top of the pyramid, this gets more insightful as we move down.)
Qualitative Analysis
- It accounts for less than 30% of all data analysis and is common in social sciences .
- It can refer to the simple recognition of qualitative elements, which is not analytic in any way, but most often refers to methods that assign numeric values to non-numeric data for analysis.
- Because of this, some argue that it’s ultimately a quantitative type.
- Importance: Medium. In general, knowing qualitative data analysis is not common or even necessary for corporate roles. However, for researchers working in social sciences, its importance is very high .
- Nature of Data: data treated under qualitative analysis is non-numeric. However, as part of the analysis, analysts turn non-numeric data into numbers, at which point many argue it is no longer qualitative analysis.
- Motive: to extract insights. (This will be more important as we move down the pyramid.)
Mathematical Analysis
- Description: mathematical data analysis is a subtype of qualitative data analysis that designates methods and techniques based on statistics, algebra, and logical reasoning to extract insights. It stands in opposition to artificial intelligence analysis.
- Importance: Very High. The most widespread methods and techniques fall under mathematical analysis. In fact, it’s so common that many people use “quantitative” and “mathematical” analysis interchangeably.
- Nature of Data: numeric. By definition, all data under mathematical analysis are numbers.
- Motive: to extract measurable insights that can be used to act upon.
Artificial Intelligence & Machine Learning Analysis
- Description: artificial intelligence and machine learning analyses designate techniques based on the titular skills. They are not traditionally mathematical, but they are quantitative since they use numbers. Applications of AI & ML analysis techniques are developing, but they’re not yet mainstream enough to show promise across the field.
- Importance: Medium . As of today (September 2020), you don’t need to be fluent in AI & ML data analysis to be a great analyst. BUT, if it’s a field that interests you, learn it. Many believe that in 10 year’s time its importance will be very high .
- Nature of Data: numeric.
- Motive: to create calculations that build on themselves in order and extract insights without direct input from a human.
Descriptive Analysis
- Description: descriptive analysis is a subtype of mathematical data analysis that uses methods and techniques to provide information about the size, dispersion, groupings, and behavior of data sets. This may sounds complicated, but just think about mean, median, and mode: all three are types of descriptive analysis. They provide information about the data set. We’ll look at specific techniques below.
- Importance: Very high. Descriptive analysis is among the most commonly used data analyses in both corporations and research today.
- Nature of Data: the nature of data under descriptive statistics is sets. A set is simply a collection of numbers that behaves in predictable ways. Data reflects real life, and there are patterns everywhere to be found. Descriptive analysis describes those patterns.
- Motive: the motive behind descriptive analysis is to understand how numbers in a set group together, how far apart they are from each other, and how often they occur. As with most statistical analysis, the more data points there are, the easier it is to describe the set.
Diagnostic Analysis
- Description: diagnostic analysis answers the question “why did it happen?” It is an advanced type of mathematical data analysis that manipulates multiple techniques, but does not own any single one. Analysts engage in diagnostic analysis when they try to explain why.
- Importance: Very high. Diagnostics are probably the most important type of data analysis for people who don’t do analysis because they’re valuable to anyone who’s curious. They’re most common in corporations, as managers often only want to know the “why.”
- Nature of Data : data under diagnostic analysis are data sets. These sets in themselves are not enough under diagnostic analysis. Instead, the analyst must know what’s behind the numbers in order to explain “why.” That’s what makes diagnostics so challenging yet so valuable.
- Motive: the motive behind diagnostics is to diagnose — to understand why.
Predictive Analysis
- Description: predictive analysis uses past data to project future data. It’s very often one of the first kinds of analysis new researchers and corporate analysts use because it is intuitive. It is a subtype of the mathematical type of data analysis, and its three notable techniques are regression, moving average, and exponential smoothing.
- Importance: Very high. Predictive analysis is critical for any data analyst working in a corporate environment. Companies always want to know what the future will hold — especially for their revenue.
- Nature of Data: Because past and future imply time, predictive data always includes an element of time. Whether it’s minutes, hours, days, months, or years, we call this time series data . In fact, this data is so important that I’ll mention it twice so you don’t forget: predictive analysis uses time series data .
- Motive: the motive for investigating time series data with predictive analysis is to predict the future in the most analytical way possible.
Prescriptive Analysis
- Description: prescriptive analysis is a subtype of mathematical analysis that answers the question “what will happen if we do X?” It’s largely underestimated in the data analysis world because it requires diagnostic and descriptive analyses to be done before it even starts. More than simple predictive analysis, prescriptive analysis builds entire data models to show how a simple change could impact the ensemble.
- Importance: High. Prescriptive analysis is most common under the finance function in many companies. Financial analysts use it to build a financial model of the financial statements that show how that data will change given alternative inputs.
- Nature of Data: the nature of data in prescriptive analysis is data sets. These data sets contain patterns that respond differently to various inputs. Data that is useful for prescriptive analysis contains correlations between different variables. It’s through these correlations that we establish patterns and prescribe action on this basis. This analysis cannot be performed on data that exists in a vacuum — it must be viewed on the backdrop of the tangibles behind it.
- Motive: the motive for prescriptive analysis is to establish, with an acceptable degree of certainty, what results we can expect given a certain action. As you might expect, this necessitates that the analyst or researcher be aware of the world behind the data, not just the data itself.
Clustering Method
- Description: the clustering method groups data points together based on their relativeness closeness to further explore and treat them based on these groupings. There are two ways to group clusters: intuitively and statistically (or K-means).
- Importance: Very high. Though most corporate roles group clusters intuitively based on management criteria, a solid understanding of how to group them mathematically is an excellent descriptive and diagnostic approach to allow for prescriptive analysis thereafter.
- Nature of Data : the nature of data useful for clustering is sets with 1 or more data fields. While most people are used to looking at only two dimensions (x and y), clustering becomes more accurate the more fields there are.
- Motive: the motive for clustering is to understand how data sets group and to explore them further based on those groups.
- Here’s an example set:

Classification Method
- Description: the classification method aims to separate and group data points based on common characteristics . This can be done intuitively or statistically.
- Importance: High. While simple on the surface, classification can become quite complex. It’s very valuable in corporate and research environments, but can feel like its not worth the work. A good analyst can execute it quickly to deliver results.
- Nature of Data: the nature of data useful for classification is data sets. As we will see, it can be used on qualitative data as well as quantitative. This method requires knowledge of the substance behind the data, not just the numbers themselves.
- Motive: the motive for classification is group data not based on mathematical relationships (which would be clustering), but by predetermined outputs. This is why it’s less useful for diagnostic analysis, and more useful for prescriptive analysis.
Forecasting Method
- Description: the forecasting method uses time past series data to forecast the future.
- Importance: Very high. Forecasting falls under predictive analysis and is arguably the most common and most important method in the corporate world. It is less useful in research, which prefers to understand the known rather than speculate about the future.
- Nature of Data: data useful for forecasting is time series data, which, as we’ve noted, always includes a variable of time.
- Motive: the motive for the forecasting method is the same as that of prescriptive analysis: the confidently estimate future values.
Optimization Method
- Description: the optimization method maximized or minimizes values in a set given a set of criteria. It is arguably most common in prescriptive analysis. In mathematical terms, it is maximizing or minimizing a function given certain constraints.
- Importance: Very high. The idea of optimization applies to more analysis types than any other method. In fact, some argue that it is the fundamental driver behind data analysis. You would use it everywhere in research and in a corporation.
- Nature of Data: the nature of optimizable data is a data set of at least two points.
- Motive: the motive behind optimization is to achieve the best result possible given certain conditions.
Content Analysis Method
- Description: content analysis is a method of qualitative analysis that quantifies textual data to track themes across a document. It’s most common in academic fields and in social sciences, where written content is the subject of inquiry.
- Importance: High. In a corporate setting, content analysis as such is less common. If anything Nïave Bayes (a technique we’ll look at below) is the closest corporations come to text. However, it is of the utmost importance for researchers. If you’re a researcher, check out this article on content analysis .
- Nature of Data: data useful for content analysis is textual data.
- Motive: the motive behind content analysis is to understand themes expressed in a large text
Narrative Analysis Method
- Description: narrative analysis is a method of qualitative analysis that quantifies stories to trace themes in them. It’s differs from content analysis because it focuses on stories rather than research documents, and the techniques used are slightly different from those in content analysis (very nuances and outside the scope of this article).
- Importance: Low. Unless you are highly specialized in working with stories, narrative analysis rare.
- Nature of Data: the nature of the data useful for the narrative analysis method is narrative text.
- Motive: the motive for narrative analysis is to uncover hidden patterns in narrative text.
Discourse Analysis Method
- Description: the discourse analysis method falls under qualitative analysis and uses thematic coding to trace patterns in real-life discourse. That said, real-life discourse is oral, so it must first be transcribed into text.
- Importance: Low. Unless you are focused on understand real-world idea sharing in a research setting, this kind of analysis is less common than the others on this list.
- Nature of Data: the nature of data useful in discourse analysis is first audio files, then transcriptions of those audio files.
- Motive: the motive behind discourse analysis is to trace patterns of real-world discussions. (As a spooky sidenote, have you ever felt like your phone microphone was listening to you and making reading suggestions? If it was, the method was discourse analysis.)
Framework Analysis Method
- Description: the framework analysis method falls under qualitative analysis and uses similar thematic coding techniques to content analysis. However, where content analysis aims to discover themes, framework analysis starts with a framework and only considers elements that fall in its purview.
- Importance: Low. As with the other textual analysis methods, framework analysis is less common in corporate settings. Even in the world of research, only some use it. Strangely, it’s very common for legislative and political research.
- Nature of Data: the nature of data useful for framework analysis is textual.
- Motive: the motive behind framework analysis is to understand what themes and parts of a text match your search criteria.
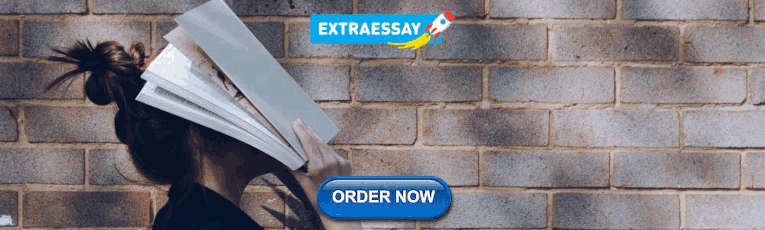
Grounded Theory Method
- Description: the grounded theory method falls under qualitative analysis and uses thematic coding to build theories around those themes.
- Importance: Low. Like other qualitative analysis techniques, grounded theory is less common in the corporate world. Even among researchers, you would be hard pressed to find many using it. Though powerful, it’s simply too rare to spend time learning.
- Nature of Data: the nature of data useful in the grounded theory method is textual.
- Motive: the motive of grounded theory method is to establish a series of theories based on themes uncovered from a text.
Clustering Technique: K-Means
- Description: k-means is a clustering technique in which data points are grouped in clusters that have the closest means. Though not considered AI or ML, it inherently requires the use of supervised learning to reevaluate clusters as data points are added. Clustering techniques can be used in diagnostic, descriptive, & prescriptive data analyses.
- Importance: Very important. If you only take 3 things from this article, k-means clustering should be part of it. It is useful in any situation where n observations have multiple characteristics and we want to put them in groups.
- Nature of Data: the nature of data is at least one characteristic per observation, but the more the merrier.
- Motive: the motive for clustering techniques such as k-means is to group observations together and either understand or react to them.
Regression Technique
- Description: simple and multivariable regressions use either one independent variable or combination of multiple independent variables to calculate a correlation to a single dependent variable using constants. Regressions are almost synonymous with correlation today.
- Importance: Very high. Along with clustering, if you only take 3 things from this article, regression techniques should be part of it. They’re everywhere in corporate and research fields alike.
- Nature of Data: the nature of data used is regressions is data sets with “n” number of observations and as many variables as are reasonable. It’s important, however, to distinguish between time series data and regression data. You cannot use regressions or time series data without accounting for time. The easier way is to use techniques under the forecasting method.
- Motive: The motive behind regression techniques is to understand correlations between independent variable(s) and a dependent one.
Nïave Bayes Technique
- Description: Nïave Bayes is a classification technique that uses simple probability to classify items based previous classifications. In plain English, the formula would be “the chance that thing with trait x belongs to class c depends on (=) the overall chance of trait x belonging to class c, multiplied by the overall chance of class c, divided by the overall chance of getting trait x.” As a formula, it’s P(c|x) = P(x|c) * P(c) / P(x).
- Importance: High. Nïave Bayes is a very common, simplistic classification techniques because it’s effective with large data sets and it can be applied to any instant in which there is a class. Google, for example, might use it to group webpages into groups for certain search engine queries.
- Nature of Data: the nature of data for Nïave Bayes is at least one class and at least two traits in a data set.
- Motive: the motive behind Nïave Bayes is to classify observations based on previous data. It’s thus considered part of predictive analysis.
Cohorts Technique
- Description: cohorts technique is a type of clustering method used in behavioral sciences to separate users by common traits. As with clustering, it can be done intuitively or mathematically, the latter of which would simply be k-means.
- Importance: Very high. With regard to resembles k-means, the cohort technique is more of a high-level counterpart. In fact, most people are familiar with it as a part of Google Analytics. It’s most common in marketing departments in corporations, rather than in research.
- Nature of Data: the nature of cohort data is data sets in which users are the observation and other fields are used as defining traits for each cohort.
- Motive: the motive for cohort analysis techniques is to group similar users and analyze how you retain them and how the churn.
Factor Technique
- Description: the factor analysis technique is a way of grouping many traits into a single factor to expedite analysis. For example, factors can be used as traits for Nïave Bayes classifications instead of more general fields.
- Importance: High. While not commonly employed in corporations, factor analysis is hugely valuable. Good data analysts use it to simplify their projects and communicate them more clearly.
- Nature of Data: the nature of data useful in factor analysis techniques is data sets with a large number of fields on its observations.
- Motive: the motive for using factor analysis techniques is to reduce the number of fields in order to more quickly analyze and communicate findings.
Linear Discriminants Technique
- Description: linear discriminant analysis techniques are similar to regressions in that they use one or more independent variable to determine a dependent variable; however, the linear discriminant technique falls under a classifier method since it uses traits as independent variables and class as a dependent variable. In this way, it becomes a classifying method AND a predictive method.
- Importance: High. Though the analyst world speaks of and uses linear discriminants less commonly, it’s a highly valuable technique to keep in mind as you progress in data analysis.
- Nature of Data: the nature of data useful for the linear discriminant technique is data sets with many fields.
- Motive: the motive for using linear discriminants is to classify observations that would be otherwise too complex for simple techniques like Nïave Bayes.
Exponential Smoothing Technique
- Description: exponential smoothing is a technique falling under the forecasting method that uses a smoothing factor on prior data in order to predict future values. It can be linear or adjusted for seasonality. The basic principle behind exponential smoothing is to use a percent weight (value between 0 and 1 called alpha) on more recent values in a series and a smaller percent weight on less recent values. The formula is f(x) = current period value * alpha + previous period value * 1-alpha.
- Importance: High. Most analysts still use the moving average technique (covered next) for forecasting, though it is less efficient than exponential moving, because it’s easy to understand. However, good analysts will have exponential smoothing techniques in their pocket to increase the value of their forecasts.
- Nature of Data: the nature of data useful for exponential smoothing is time series data . Time series data has time as part of its fields .
- Motive: the motive for exponential smoothing is to forecast future values with a smoothing variable.
Moving Average Technique
- Description: the moving average technique falls under the forecasting method and uses an average of recent values to predict future ones. For example, to predict rainfall in April, you would take the average of rainfall from January to March. It’s simple, yet highly effective.
- Importance: Very high. While I’m personally not a huge fan of moving averages due to their simplistic nature and lack of consideration for seasonality, they’re the most common forecasting technique and therefore very important.
- Nature of Data: the nature of data useful for moving averages is time series data .
- Motive: the motive for moving averages is to predict future values is a simple, easy-to-communicate way.
Neural Networks Technique
- Description: neural networks are a highly complex artificial intelligence technique that replicate a human’s neural analysis through a series of hyper-rapid computations and comparisons that evolve in real time. This technique is so complex that an analyst must use computer programs to perform it.
- Importance: Medium. While the potential for neural networks is theoretically unlimited, it’s still little understood and therefore uncommon. You do not need to know it by any means in order to be a data analyst.
- Nature of Data: the nature of data useful for neural networks is data sets of astronomical size, meaning with 100s of 1000s of fields and the same number of row at a minimum .
- Motive: the motive for neural networks is to understand wildly complex phenomenon and data to thereafter act on it.
Decision Tree Technique
- Description: the decision tree technique uses artificial intelligence algorithms to rapidly calculate possible decision pathways and their outcomes on a real-time basis. It’s so complex that computer programs are needed to perform it.
- Importance: Medium. As with neural networks, decision trees with AI are too little understood and are therefore uncommon in corporate and research settings alike.
- Nature of Data: the nature of data useful for the decision tree technique is hierarchical data sets that show multiple optional fields for each preceding field.
- Motive: the motive for decision tree techniques is to compute the optimal choices to make in order to achieve a desired result.
Evolutionary Programming Technique
- Description: the evolutionary programming technique uses a series of neural networks, sees how well each one fits a desired outcome, and selects only the best to test and retest. It’s called evolutionary because is resembles the process of natural selection by weeding out weaker options.
- Importance: Medium. As with the other AI techniques, evolutionary programming just isn’t well-understood enough to be usable in many cases. It’s complexity also makes it hard to explain in corporate settings and difficult to defend in research settings.
- Nature of Data: the nature of data in evolutionary programming is data sets of neural networks, or data sets of data sets.
- Motive: the motive for using evolutionary programming is similar to decision trees: understanding the best possible option from complex data.
- Video example :
Fuzzy Logic Technique
- Description: fuzzy logic is a type of computing based on “approximate truths” rather than simple truths such as “true” and “false.” It is essentially two tiers of classification. For example, to say whether “Apples are good,” you need to first classify that “Good is x, y, z.” Only then can you say apples are good. Another way to see it helping a computer see truth like humans do: “definitely true, probably true, maybe true, probably false, definitely false.”
- Importance: Medium. Like the other AI techniques, fuzzy logic is uncommon in both research and corporate settings, which means it’s less important in today’s world.
- Nature of Data: the nature of fuzzy logic data is huge data tables that include other huge data tables with a hierarchy including multiple subfields for each preceding field.
- Motive: the motive of fuzzy logic to replicate human truth valuations in a computer is to model human decisions based on past data. The obvious possible application is marketing.
Text Analysis Technique
- Description: text analysis techniques fall under the qualitative data analysis type and use text to extract insights.
- Importance: Medium. Text analysis techniques, like all the qualitative analysis type, are most valuable for researchers.
- Nature of Data: the nature of data useful in text analysis is words.
- Motive: the motive for text analysis is to trace themes in a text across sets of very long documents, such as books.
Coding Technique
- Description: the coding technique is used in textual analysis to turn ideas into uniform phrases and analyze the number of times and the ways in which those ideas appear. For this reason, some consider it a quantitative technique as well. You can learn more about coding and the other qualitative techniques here .
- Importance: Very high. If you’re a researcher working in social sciences, coding is THE analysis techniques, and for good reason. It’s a great way to add rigor to analysis. That said, it’s less common in corporate settings.
- Nature of Data: the nature of data useful for coding is long text documents.
- Motive: the motive for coding is to make tracing ideas on paper more than an exercise of the mind by quantifying it and understanding is through descriptive methods.
Idea Pattern Technique
- Description: the idea pattern analysis technique fits into coding as the second step of the process. Once themes and ideas are coded, simple descriptive analysis tests may be run. Some people even cluster the ideas!
- Importance: Very high. If you’re a researcher, idea pattern analysis is as important as the coding itself.
- Nature of Data: the nature of data useful for idea pattern analysis is already coded themes.
- Motive: the motive for the idea pattern technique is to trace ideas in otherwise unmanageably-large documents.
Word Frequency Technique
- Description: word frequency is a qualitative technique that stands in opposition to coding and uses an inductive approach to locate specific words in a document in order to understand its relevance. Word frequency is essentially the descriptive analysis of qualitative data because it uses stats like mean, median, and mode to gather insights.
- Importance: High. As with the other qualitative approaches, word frequency is very important in social science research, but less so in corporate settings.
- Nature of Data: the nature of data useful for word frequency is long, informative documents.
- Motive: the motive for word frequency is to locate target words to determine the relevance of a document in question.
Types of data analysis in research
Types of data analysis in research methodology include every item discussed in this article. As a list, they are:
- Quantitative
- Qualitative
- Mathematical
- Machine Learning and AI
- Descriptive
- Prescriptive
- Classification
- Forecasting
- Optimization
- Grounded theory
- Artificial Neural Networks
- Decision Trees
- Evolutionary Programming
- Fuzzy Logic
- Text analysis
- Idea Pattern Analysis
- Word Frequency Analysis
- Nïave Bayes
- Exponential smoothing
- Moving average
- Linear discriminant
Types of data analysis in qualitative research
As a list, the types of data analysis in qualitative research are the following methods:
Types of data analysis in quantitative research
As a list, the types of data analysis in quantitative research are:
Data analysis methods
As a list, data analysis methods are:
- Content (qualitative)
- Narrative (qualitative)
- Discourse (qualitative)
- Framework (qualitative)
- Grounded theory (qualitative)
Quantitative data analysis methods
As a list, quantitative data analysis methods are:
Tabular View of Data Analysis Types, Methods, and Techniques
About the author.
Noah is the founder & Editor-in-Chief at AnalystAnswers. He is a transatlantic professional and entrepreneur with 5+ years of corporate finance and data analytics experience, as well as 3+ years in consumer financial products and business software. He started AnalystAnswers to provide aspiring professionals with accessible explanations of otherwise dense finance and data concepts. Noah believes everyone can benefit from an analytical mindset in growing digital world. When he's not busy at work, Noah likes to explore new European cities, exercise, and spend time with friends and family.
File available immediately.
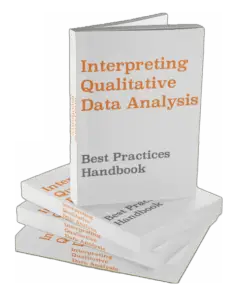
Notice: JavaScript is required for this content.

- Study and research support
- Researcher support
- Research data management
Research data management explained
What is research data.
Research data is any information that has been collected, observed, generated or created to validate original research findings.
Although usually digital, research data also includes non-digital formats such as laboratory notebooks and diaries.
Types of research data
Research data can take many forms. It might be:
- documents, spreadsheets
- laboratory notebooks, field notebooks, diaries
- questionnaires, transcripts, codebooks
- audiotapes, videotapes
- photographs, films
- test responses
- slides, artefacts, specimens, samples
- collections of digital outputs
- database contents (video, audio, text, images)
- models, algorithms, scripts
- contents of an application (input, output, logfiles for analysis software, simulation software, schemas)
- methodologies and workflows
- standard operating procedures and protocols
Non-digital data
Non-digital data such as laboratory notebooks, ice-core samples and sketchbooks is often unique. You should assess the long-term value of any non-digital data and plan how you will describe and retain them.
You could digitise the materials, but this may not be possible for all types of data.
The University of Leeds research data repository (Research Data Leeds) describes digital materials and can also be used to create records for physical artefacts.
Please contact the team if you would like to discuss requirements for non-digital data.
Sources of research data
Research data can be generated for different purposes and through different processes.
- Observational data is captured in real-time, and is usually irreplaceable, for example sensor data, survey data, sample data, and neuro-images.
- Experimental data is captured from lab equipment. It is often reproducible, but this can be expensive. Examples of experimental data are gene sequences, chromatograms, and toroid magnetic field data.
- Simulation data is generated from test models where model and metadata are more important than output data. For example, climate models and economic models.
- Derived or compiled data has been transformed from pre-existing data points. It is reproducible if lost, but this would be expensive. Examples are data mining, compiled databases, and 3D models.
- Reference or canonical data is a static or organic conglomeration or collection of smaller (peer-reviewed) datasets, most probably published and curated. For example, gene sequence databanks, chemical structures, or spatial data portals.

Data Module #1: What is Research Data?
- Defining Research Data
- Qualitative vs. Quantitative
- Types of Research Data
- Data and Statistics
- Let's Review...
Quick Navigation
Got questions contact us., review of module #1, let's review.
Congratulations! You have completed the module What is Research Data? Take a few minutes to check your understanding of the information covered in this module by answering the following questions. Once you've gone through each question, click on the "CHECK YOUR ANSWER" link to see how you did.
- << Previous: Data and Statistics
- Last Updated: Feb 2, 2024 1:41 PM
- URL: https://libguides.macalester.edu/data1

Community Blog
Keep up-to-date on postgraduate related issues with our quick reads written by students, postdocs, professors and industry leaders.
Types of Research – Explained with Examples

- By DiscoverPhDs
- October 2, 2020
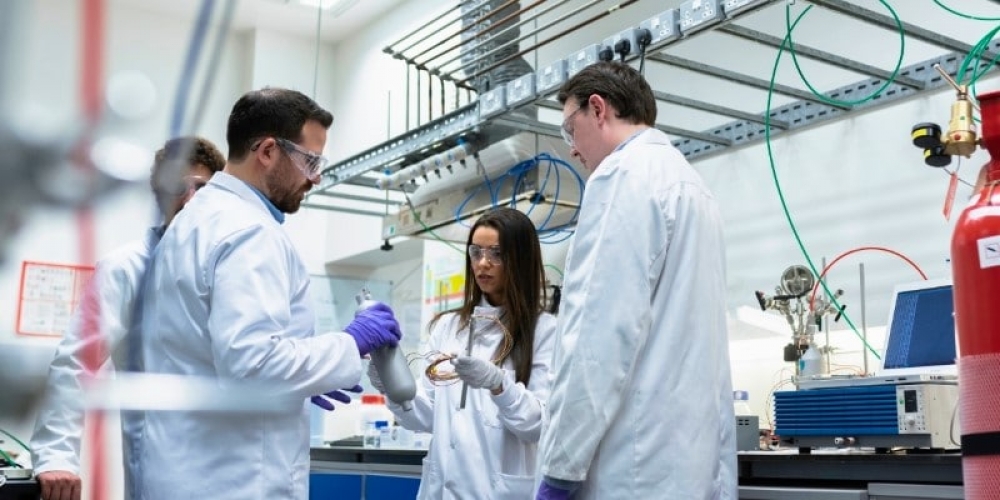
Types of Research
Research is about using established methods to investigate a problem or question in detail with the aim of generating new knowledge about it.
It is a vital tool for scientific advancement because it allows researchers to prove or refute hypotheses based on clearly defined parameters, environments and assumptions. Due to this, it enables us to confidently contribute to knowledge as it allows research to be verified and replicated.
Knowing the types of research and what each of them focuses on will allow you to better plan your project, utilises the most appropriate methodologies and techniques and better communicate your findings to other researchers and supervisors.
Classification of Types of Research
There are various types of research that are classified according to their objective, depth of study, analysed data, time required to study the phenomenon and other factors. It’s important to note that a research project will not be limited to one type of research, but will likely use several.
According to its Purpose
Theoretical research.
Theoretical research, also referred to as pure or basic research, focuses on generating knowledge , regardless of its practical application. Here, data collection is used to generate new general concepts for a better understanding of a particular field or to answer a theoretical research question.
Results of this kind are usually oriented towards the formulation of theories and are usually based on documentary analysis, the development of mathematical formulas and the reflection of high-level researchers.
Applied Research
Here, the goal is to find strategies that can be used to address a specific research problem. Applied research draws on theory to generate practical scientific knowledge, and its use is very common in STEM fields such as engineering, computer science and medicine.
This type of research is subdivided into two types:
- Technological applied research : looks towards improving efficiency in a particular productive sector through the improvement of processes or machinery related to said productive processes.
- Scientific applied research : has predictive purposes. Through this type of research design, we can measure certain variables to predict behaviours useful to the goods and services sector, such as consumption patterns and viability of commercial projects.
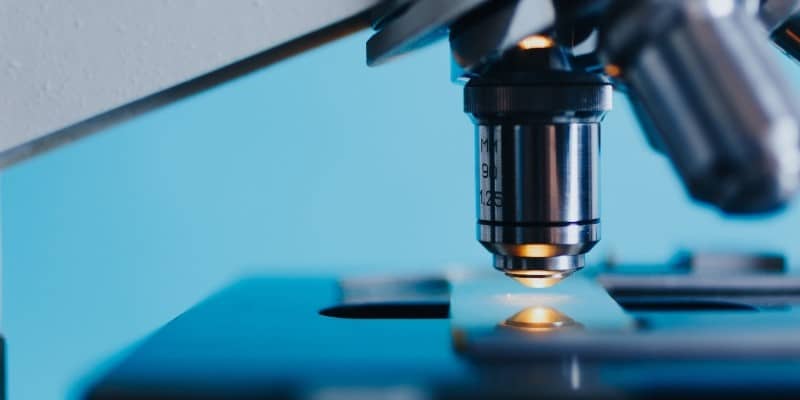
According to your Depth of Scope
Exploratory research.
Exploratory research is used for the preliminary investigation of a subject that is not yet well understood or sufficiently researched. It serves to establish a frame of reference and a hypothesis from which an in-depth study can be developed that will enable conclusive results to be generated.
Because exploratory research is based on the study of little-studied phenomena, it relies less on theory and more on the collection of data to identify patterns that explain these phenomena.
Descriptive Research
The primary objective of descriptive research is to define the characteristics of a particular phenomenon without necessarily investigating the causes that produce it.
In this type of research, the researcher must take particular care not to intervene in the observed object or phenomenon, as its behaviour may change if an external factor is involved.
Explanatory Research
Explanatory research is the most common type of research method and is responsible for establishing cause-and-effect relationships that allow generalisations to be extended to similar realities. It is closely related to descriptive research, although it provides additional information about the observed object and its interactions with the environment.
Correlational Research
The purpose of this type of scientific research is to identify the relationship between two or more variables. A correlational study aims to determine whether a variable changes, how much the other elements of the observed system change.
According to the Type of Data Used
Qualitative research.
Qualitative methods are often used in the social sciences to collect, compare and interpret information, has a linguistic-semiotic basis and is used in techniques such as discourse analysis, interviews, surveys, records and participant observations.
In order to use statistical methods to validate their results, the observations collected must be evaluated numerically. Qualitative research, however, tends to be subjective, since not all data can be fully controlled. Therefore, this type of research design is better suited to extracting meaning from an event or phenomenon (the ‘why’) than its cause (the ‘how’).
Quantitative Research
Quantitative research study delves into a phenomena through quantitative data collection and using mathematical, statistical and computer-aided tools to measure them . This allows generalised conclusions to be projected over time.

According to the Degree of Manipulation of Variables
Experimental research.
It is about designing or replicating a phenomenon whose variables are manipulated under strictly controlled conditions in order to identify or discover its effect on another independent variable or object. The phenomenon to be studied is measured through study and control groups, and according to the guidelines of the scientific method.
Non-Experimental Research
Also known as an observational study, it focuses on the analysis of a phenomenon in its natural context. As such, the researcher does not intervene directly, but limits their involvement to measuring the variables required for the study. Due to its observational nature, it is often used in descriptive research.
Quasi-Experimental Research
It controls only some variables of the phenomenon under investigation and is therefore not entirely experimental. In this case, the study and the focus group cannot be randomly selected, but are chosen from existing groups or populations . This is to ensure the collected data is relevant and that the knowledge, perspectives and opinions of the population can be incorporated into the study.
According to the Type of Inference
Deductive investigation.
In this type of research, reality is explained by general laws that point to certain conclusions; conclusions are expected to be part of the premise of the research problem and considered correct if the premise is valid and the inductive method is applied correctly.
Inductive Research
In this type of research, knowledge is generated from an observation to achieve a generalisation. It is based on the collection of specific data to develop new theories.
Hypothetical-Deductive Investigation
It is based on observing reality to make a hypothesis, then use deduction to obtain a conclusion and finally verify or reject it through experience.

According to the Time in Which it is Carried Out
Longitudinal study (also referred to as diachronic research).
It is the monitoring of the same event, individual or group over a defined period of time. It aims to track changes in a number of variables and see how they evolve over time. It is often used in medical, psychological and social areas .
Cross-Sectional Study (also referred to as Synchronous Research)
Cross-sectional research design is used to observe phenomena, an individual or a group of research subjects at a given time.
According to The Sources of Information
Primary research.
This fundamental research type is defined by the fact that the data is collected directly from the source, that is, it consists of primary, first-hand information.
Secondary research
Unlike primary research, secondary research is developed with information from secondary sources, which are generally based on scientific literature and other documents compiled by another researcher.
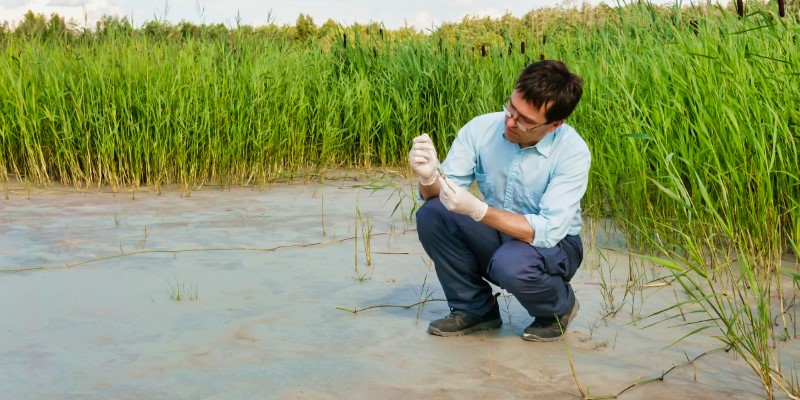
According to How the Data is Obtained
Documentary (cabinet).
Documentary research, or secondary sources, is based on a systematic review of existing sources of information on a particular subject. This type of scientific research is commonly used when undertaking literature reviews or producing a case study.
Field research study involves the direct collection of information at the location where the observed phenomenon occurs.
From Laboratory
Laboratory research is carried out in a controlled environment in order to isolate a dependent variable and establish its relationship with other variables through scientific methods.
Mixed-Method: Documentary, Field and/or Laboratory
Mixed research methodologies combine results from both secondary (documentary) sources and primary sources through field or laboratory research.

There are various types of research that are classified by objective, depth of study, analysed data and the time required to study the phenomenon etc.

Are you always finding yourself working on sections of your research tasks right up until your deadlines? Are you still finding yourself distracted the moment
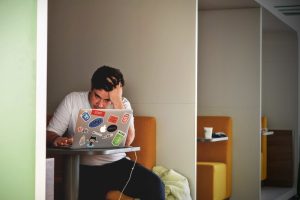
PhD stress is real. Learn how to combat it with these 5 tips.
Join thousands of other students and stay up to date with the latest PhD programmes, funding opportunities and advice.
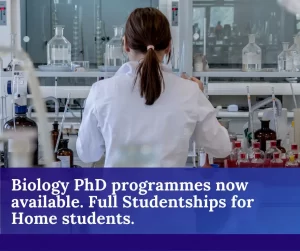
Browse PhDs Now
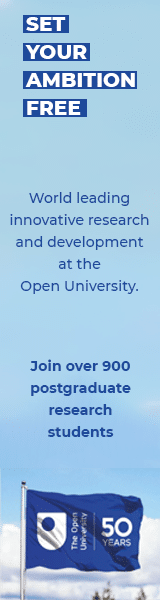
An In Press article is a paper that has been accepted for publication and is being prepared for print.
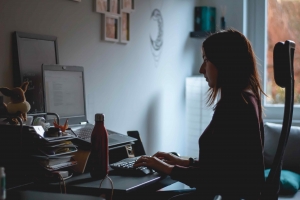
Reference management software solutions offer a powerful way for you to track and manage your academic references. Read our blog post to learn more about what they are and how to use them.

Sara is currently in the 4th year of the Physics Doctoral Program at The Graduate Center of the City University of New York. Her research investigates quantum transport properties of 2D electron systems.

Charlene is a 5th year PhD candidate at the University of Wisconsin-Madison. She studies depression and neuroticism in people with Temporal Lobe Epilepsy (TLE) using MR Imaging and behavioural tests.
Join Thousands of Students
- Data Center
- Applications
- Open Source
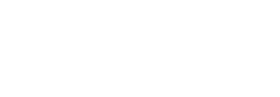
Datamation content and product recommendations are editorially independent. We may make money when you click on links to our partners. Learn More .
Raw data, often referred to as source or primary data, is data that has not yet been processed, coded, formatted, or analyzed. While raw data is a valuable resource, it can be challenging to work with or understand as it’s visually cluttered and can lack cohesion. Organizations can collect and use raw data to learn more about customers, sales, the success of marketing campaigns, and other useful targets, but first they need to structure and organize the data into a form that’s easier to read and visualize.
Enterprises that actively employ data for analysis, decision-making, or reporting must understand how raw data works and how it fits into a larger overall data strategy.
Table of Contents
How is Raw Data Used?
Raw data is data collected from one or multiple sources that remains in its unaltered initial state. At this point, the data might contain human, machine, or instrumental errors—depending on the collection method—or it could lack validation. Once the data is changed in any way to improve its quality, the data has been processed and is no longer considered raw.
Raw data has infinite potential as a resource, as it comes in a variety of forms from a wide range of sources. Collecting raw data is the first step toward gaining a more thorough understanding of a demographic, system, concept, or environment. Business intelligence analysts can extract useful and accurate information about the condition of their business from raw data—for example, audience interest, sales figures, marketing campaign performance, and overall productivity.
Raw Data Collection Steps
How raw data is collected plays a key role in its quality and future potential. Accuracy, credibility, and validity can mean the difference between a database of raw data that’s a wealth of information and insights, and unactionable data that simply takes up space. The following steps lay out a path for data collection to help ensure it meets the organization’s needs.
Defining Goals
Define the information you want to extract to lay the groundwork for your raw data-gathering goals. For example, if the desired data is user-base and customer information, online and in-person surveys focused on a specific age and geographical demographic can be used to gather it.
Other types of raw data may require advance planning. For instance, collecting data from log records would require having a monitoring system in place for anywhere from a few weeks to a year to collect data before being able to pull it.
Choosing a Collection Method
Choosing the appropriate raw data collection method can reduce the percentage of human or machine errors you’d have to scrub out when cleaning a raw database. Generally, electronic collecting methods tend to result in lower error rates—manual collection can introduce variables that leave room for interpretation, such as illegible handwriting or hard-to-understand accents in audio or video recordings.
Collecting Data
Raw data tends to be large in volume and highly complex. During the collection process, the overall volume of data is only an estimate—once you process the data by cleaning it of errors and invalid data points, you’ll have a more accurate sense of scope.
How Raw Data is Processed in 5 Steps
Analysts well-versed in data trends and patterns work with modern business intelligence tools and, on occasion, incorporate artificial intelligence (AI) applications to transform raw data into insightful knowledge using the following steps:
1. Data Preparation
Raw data, by definition, is faulty and may contain errors—it might also lack consistency in structure and format, especially when it’s been obtained from different sources. During data preparation, data is thoroughly cleaned, sorted, and filtered following predefined standards to ensure high-quality, consistent outcomes.
2. Data Input
Inputting data—sometimes referred to as data translation—involves converting raw data into a machine-readable form. The specific process will vary depending upon the tools and software that will be used for analysis.
In the case of digitally collected data, this step is minimal—some structuring and changing of file format might be needed. But for handwritten surveys, audio recordings, and video clips, data will need to be manually or digitally extracted into a form the processing software is capable of understanding.
3. Data Processing and Analysis
Raw data is processed and analyzed for insights by searching for trends, patterns, anomalies, and relationships between the various elements. This process varies depending on the source, and can be done manually or using artificial intelligence and machine learning.
4. Data Output
Once the raw data has been fully transformed into usable and insightful data, it’s translated into human-friendly form—diagrams, graphs, tables, vector files, or plain text, for example. This step makes the data usable and actionable.
5. Data Storage
Data storage is essential, as the processed data may be subjected to future analysis for additional insights—but it becomes even more critical when dealing with sensitive corporate information or user data. Storage quality should be consistent with the overall standards of the company’s data and information, while also complying with any local data privacy and security legislation, such as the European Union’s General Data Protection Regulation ( GDPR ) and the California Consumer Protection Act (CCPA) .
9 Types of Data Processing
Choosing the best approach to handle raw data is critical for effective data management . It will depend upon the type and volume of data and the pace of collection, among other concerns. Choosing the correct approach that is consistent with what the business wants to achieve not only improves workflow but also helps gain the most value from data, enabling smart choices and strategic insights.
Here are the nine most common types of data processing:
- Batch Processing— A set or batch of data is processed in bulk at regular intervals. This method is suitable for operations that do not require instant replies, such as payroll processing, when efficiency trumps real-time engagement.
- Real-Time Processing— Data is processed as soon as it is created or received, ensuring quick reactions. Commonly used in instances when rapid decision-making is essential, such as financial transactions or monitoring systems.
- Online Processing— Similar to real-time processing in that it handles data as it is entered or requested, as seen in interactive systems such as online databases. Allows for quick data retrieval and updating in response to changing user requirements.
- Distributed Processing— Spreads processing work across interconnected computers to improve overall system efficiency and performance. Frequently used in large-scale data processing applications where centralized processing is unfeasible.
- Parallel Processing— Processes many jobs or programs at the same time using multiple processors, enhancing processing speed for difficult calculations. Ideal for jobs that can be broken down into parallelizable subtasks.
- Multi-Processing— Performs many processes or applications at the same time on a computer with multiple processors. By processing several jobs concurrently, improves overall system speed and throughput.
- Transaction Processing— Individual transactions or company processes are processed in real-time. In systems such as online banking, where the rapid and correct processing of financial transactions is critical for ensuring data integrity, this is essential.
- Manual Data Processing— Involves human interaction in data processing in the absence of automated technologies. This might include procedures like manually inputting data into a system, which is inefficient yet may be required for particular jobs or data kinds.
- EDP (Electronic Data Processing)— Data processing and analysis using electronic devices and computers. Encompasses a wide range of automated operations, ranging from simple data input to complicated computations, that are routinely employed in current data processing applications across a variety of sectors.
2 Types Of Raw Data and Their Examples
Raw data includes a wide range of data types, which are typically classified as either qualitative or quantitative. The most important condition for this categorization is that the data is not cleaned or processed in any way. Raw data allows unrivaled flexibility and control over the information collected from the database.
Quantitative Raw Data
Quantitative data is raw data that consists of countable data, where each data point has a unique numerical value. This type of data is best used for mathematical calculations and technical statistical analysis. Here are some common examples:
Qualitative Raw Data
Qualitative data can be recorded and observed in a non-quantifiable and non-numerical nature. It rarely includes numbers and is usually extracted from answers that vary for each participant through audio and video recordings or one-on-one interviews. Here are some common examples:
Improving Customer Satisfaction With Insights From Raw Data
Up-to-date raw data is essential in all industries, but especially in fields where the company is capable of further optimizing operations for more profit, fewer costs, and higher levels of customer satisfaction. There are a number of ways for enterprises to use raw data to learn more about their customers and improve those relationships.
Using Customer Surveys to Collect Internal Data
Internal initiatives that actively request client input are one approach to acquiring raw data. This comprises engaging current customers to take part in quick surveys that provide important information about their contacts with the company’s services or products.
Outsourcing Data Collection
Organizations can enhance the raw data with a wider range of insights by actively outsourcing data-collecting operations to specialist organizations that target certain populations. This active approach ensures a diversified variety of ideas and opinions from an outside perspective.
Incorporating Diverse Processing and Expert Analysis
Because of the unprocessed nature of the data, a wide range of processing approaches and tools can be used. This adaptability enables businesses to react efficiently to the demands and requests of consumers and clients by actively increasing familiarity through larger data samples and expert-level analysis.
Bottom Line: Building Valuable Insights Starting With Raw Data
Raw data is data that hasn’t been cleaned, organized, or processed in any capacity. While it can’t be used directly to generate information and insights as it is, it can be processed and refined to make it actionable. Making use of accurate and up-to-date raw data can fuel data-backed decision-making and provide unique insights that can benefit organizations in many ways.
Read Data Management: Types and Challenges to learn more about how enterprise organizations work with the vast stores of data they rely upon for decision making, customer insights, and business intelligence.
This article updates an earlier article by Anina Ot.
Subscribe to Data Insider
Learn the latest news and best practices about data science, big data analytics, artificial intelligence, data security, and more.
Similar articles
Crm software examples: 10 industry use cases and tools for 2024, coursera: machine learning (ml) courses for certification in 2024, 12 top data mining certifications of 2024, get the free newsletter.
Subscribe to Data Insider for top news, trends & analysis
Latest Articles
8 best network monitoring..., what is cybersecurity definitions,..., crm software examples: 10..., coursera: machine learning (ml)....
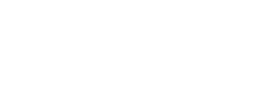
Most plug-in hybrids and all-electric vehicles use lithium-ion batteries like these.
Energy storage systems, usually batteries, are essential for all-electric vehicles, plug-in hybrid electric vehicles (PHEVs), and hybrid electric vehicles (HEVs).
Types of Energy Storage Systems
The following energy storage systems are used in all-electric vehicles, PHEVs, and HEVs.
Lithium-Ion Batteries
Lithium-ion batteries are currently used in most portable consumer electronics such as cell phones and laptops because of their high energy per unit mass and volume relative to other electrical energy storage systems. They also have a high power-to-weight ratio, high energy efficiency, good high-temperature performance, long life, and low self-discharge. Most components of lithium-ion batteries can be recycled, but the cost of material recovery remains a challenge for the industry. Most of today's all-electric vehicles and PHEVs use lithium-ion batteries, though the exact chemistry often varies from that of consumer electronics batteries. Research and development are ongoing to reduce their relatively high cost, extend their useful life, use less cobalt, and address safety concerns in regard to various fault conditions.
Nickel-Metal Hydride Batteries
Nickel-metal hydride batteries, used routinely in computer and medical equipment, offer reasonable specific energy and specific power capabilities. Nickel-metal hydride batteries have a much longer life cycle than lead-acid batteries and are safe and abuse tolerant. These batteries have been widely used in HEVs . The main challenges with nickel-metal hydride batteries are their high cost, high self-discharge rate, heat generation at high temperatures, and the need to control hydrogen loss.
Lead-Acid Batteries
Lead-acid batteries can be designed to be high power and are inexpensive, safe, recyclable, and reliable. However, low specific energy, poor cold-temperature performance, and short calendar and lifecycle impede their use. Advanced high-power lead-acid batteries are being developed, but these batteries are only used in commercially available electric-drive vehicles for ancillary loads. They are also used for stop-start functionality in internal combustion engine vehicles to eliminate idling during stops and reduce fuel consumption.
Ultracapacitors
Ultracapacitors store energy in the interface between an electrode and an electrolyte when voltage is applied. Energy storage capacity increases as the electrolyte-electrode surface area increases. Although ultracapacitors have low energy density, they have very high power density, which means they can deliver high amounts of power in a short time. Ultracapacitors can provide vehicles additional power during acceleration and hill climbing and help recover braking energy. They may also be useful as secondary energy-storage devices in electric-drive vehicles because they help electrochemical batteries level load power.
Recycling Batteries
Electric-drive vehicles are relatively new to the U.S. auto market, so only a small number of them have approached the end of their useful lives. As electric-drive vehicles become increasingly common, the battery-recycling market may expand.
Widespread battery recycling would help keep hazardous materials from entering the waste stream, both at the end of a battery's useful life and during its production. The U.S. Department of Energy is also supporting the Lithium-Ion Battery Recycling Prize to develop and demonstrate profitable solutions for collecting, sorting, storing, and transporting spent and discarded lithium-ion batteries for eventual recycling and materials recovery. After collection of spent batteries, the material recovery from recycling would also reintroduce critical materials back into the supply chain and would increase the domestic sources for such materials. Work is now underway to develop battery-recycling processes that minimize the life-cycle impacts of using lithium-ion and other kinds of batteries in vehicles. But not all recycling processes are the same and different methods of separation are required for material recovery:
- Smelting : Smelting processes recover basic elements or salts. These processes are operational now on a large scale and can accept multiple kinds of batteries, including lithium-ion and nickel-metal hydride. Smelting takes place at high temperatures where organic materials, including the electrolyte and carbon anodes, are burned as fuel or reductant. The valuable metals are recovered and sent to refining so that the product is suitable for any use. The other materials, including lithium, are contained in the slag, which is now used as an additive in concrete.
- Direct recovery : At the other extreme, some recycling processes directly recover battery-grade materials. Components are separated by a variety of physical and chemical processes, and all active materials and metals can be recovered. Direct recovery is a low-temperature process with minimal energy requirement.
- Intermediate processes : The third type of process is between the two extremes. Such processes may accept multiple kinds of batteries, unlike direct recovery, but recover materials further along the production chain than smelting does.
Separating the different kinds of battery materials is often a stumbling block in recovering high-value materials. Therefore, battery design that considers disassembly and recycling is important in order for electric-drive vehicles to succeed from a sustainability standpoint. Standardizing batteries, materials, and cell design would also make recycling easier and more cost-effective.
See the report: Technical and Economic Feasibility of Applying Used EV Batteries in Stationary Applications .
More Information
Learn more about research and development of batteries from the National Renewable Energy Laboratory's energy storage pages and the U.S. Department of Energy Vehicle Technologies Office's batteries page.
Case Studies
- Electric Vehicles Hit the Open Road
- EV Battery Recycling
- Yellowstone Park Recycles Vehicle Batteries for Solar Power
More Electricity Case Studies | All Case Studies
Publications
- Electric Vehicle Batteries and Recycling
- Lithium-Ion Battery Supply Chain for E-Drive Vehicles in the United States: 2010–2020
- Enabling Fast Charging - A Battery Technology Gap Assessment
More Electricity Publications | All Publications
- Battery Policies and Incentives

An official website of the United States government
Here’s how you know
Official websites use .gov A .gov website belongs to an official government organization in the United States.
Secure .gov websites use HTTPS A lock ( Lock A locked padlock ) or https:// means you’ve safely connected to the .gov website. Share sensitive information only on official, secure websites.
JavaScript appears to be disabled on this computer. Please click here to see any active alerts .
Global Greenhouse Gas Overview
On This Page:
Global Emissions and Removals by Gas
Global emissions by economic sector, trends in global emissions, emissions by country.
At the global scale, the key greenhouse gases emitted by human activities are:
- Carbon dioxide (CO 2 ) : Fossil fuel use is the primary source of CO 2 . CO 2 can also be emitted from the landscape through deforestation, land clearance for agriculture or development, and degradation of soils. Likewise, land management can also remove additional CO 2 from the atmosphere through reforestation, improvement of soil health, and other activities.
- Methane (CH 4 ) : Agricultural activities, waste management, energy production and use, and biomass burning all contribute to CH 4 emissions.
- Nitrous oxide (N 2 O) : Agricultural activities, such as fertilizer use, are the primary source of N 2 O emissions. Chemical production and fossil fuel combustion also generates N 2 O.
- Fluorinated gases (F-gases) : Industrial processes, refrigeration, and the use of a variety of consumer products contribute to emissions of F-gases, which include hydrofluorocarbons (HFCs), perfluorocarbons (PFCs), and sulfur hexafluoride (SF 6 ).
Additional compounds in the atmosphere including solid and liquid aerosol and other greenhouse gases, such as water vapor and ground-level ozone can also impact the climate. Learn more about these compounds and climate change on our Basics of Climate Change page .
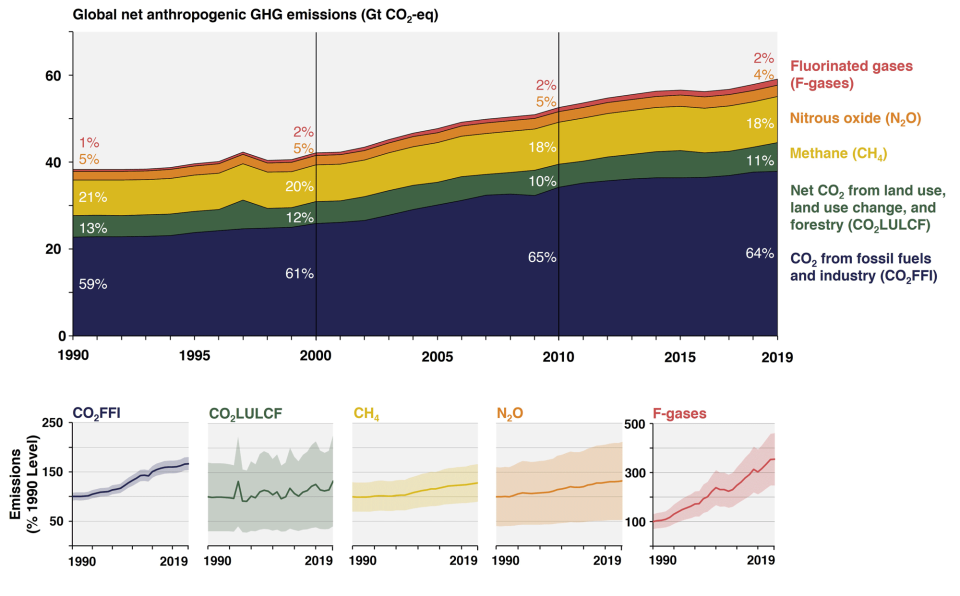
Global greenhouse gas emissions can also be broken down by the economic activities that lead to their atmospheric release. [1]
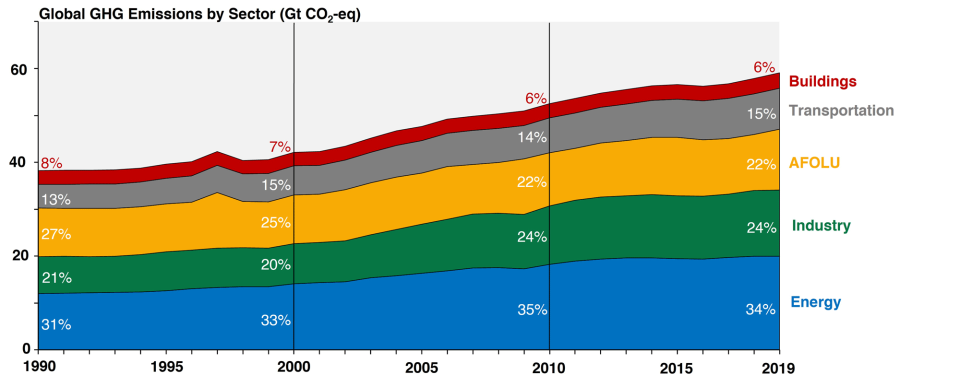
- Electricity and Heat Production (34% of 2019 global greenhouse gas emissions): The burning of coal, natural gas, and oil for electricity and heat is the largest single source of global greenhouse gas emissions.
- Industry (24% of 2019 global greenhouse gas emissions): Greenhouse gas emissions from industry primarily involve fossil fuels burned on site at facilities for energy. This sector also includes emissions from chemical, metallurgical, and mineral transformation processes not associated with energy consumption and emissions from waste management activities. (Note: Emissions from industrial electricity use are excluded and are instead covered in the Electricity and Heat Production sector.)
- Agriculture, Forestry, and Other Land Use (22% of 2019 global greenhouse gas emissions): Greenhouse gas emissions from this sector come mostly from agriculture (cultivation of crops and livestock) and deforestation. This estimate does not include the CO 2 that ecosystems remove from the atmosphere by sequestering carbon (e.g. in biomass, soils). [2]
- Transportation (15% of 2019 global greenhouse gas emissions): Greenhouse gas emissions from this sector primarily involve fossil fuels burned for road, rail, air, and marine transportation. Almost all (95%) of the world's transportation energy comes from petroleum-based fuels, largely gasoline and diesel. [3]
- Buildings (6% of 2019 global greenhouse gas emissions): Greenhouse gas emissions from this sector arise from onsite energy generation and burning fuels for heat in buildings or cooking in homes. Note: Emissions from this sector are 16% when electricity use in buildings is included in this sector instead of the Energy sector.
Note on emissions sector categories.
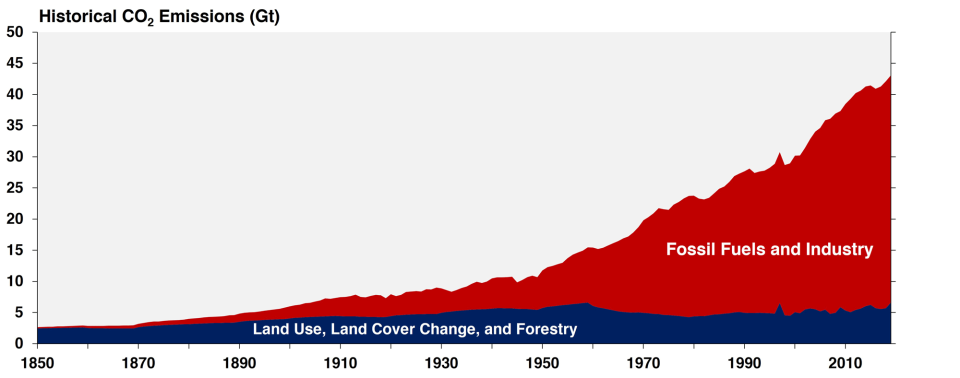
Emissions of non-CO 2 greenhouse gases (CH 4 , N 2 O, and F-gases) have also increased significantly since 1850.
- Globally, greenhouse gas emissions continued to rise across all sectors and subsectors, most rapidly in the transport and industry sectors.
- While the trend in emissions continues to rise, annual greenhouse gas growth by sector slowed in 2010 to 2019, compared to 2000 to 2009, for energy and industry, however remained roughly stable for transport.
- The trend for for AFOLU remains more uncertain, due to the multitude of drivers that affect emissions and removals for land use, land-use change and forestry.
- rising demand for construction materials and manufactured products,
- increasing floor space per capita,
- increasing building energy use,
- travel distances, and vehicle size and weight.
To learn more about past and projected global emissions of non-CO 2 gases, please see the EPA report, Global Non-CO 2 Greenhouse Gas Emission Projections & Mitigation Potential: 2015-2050 . For further insights into mitigation strategies specifically within the U.S. forestry and agriculture sectors, refer to the latest Climate Economic Analysis report on Greenhouse Gas Mitigation Potential in U.S. Forestry and Agriculture .
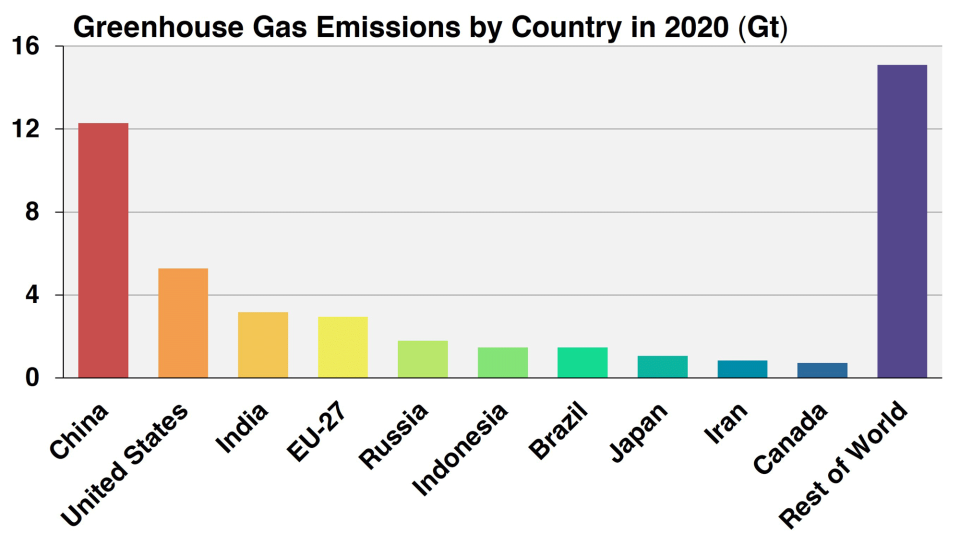
In 2020, the top ten greenhouse gas emitters were China, the United States, India, the European Union, Russia, Indonesia, Brazil, Japan, Iran, and Canada. These data include CO 2 , CH 4 , N 2 O, and fluorinated gas emissions from energy, agriculture, forestry and land use change, industry, and waste. Together, these top ten countries represent approximately 67% of total greenhouse gas emissions in 2020.
Emissions and sinks related to changes in land use are not included in these estimates. However, changes in land use can be important: estimates indicate that net global greenhouse gas emissions from agriculture, forestry, and other land use were approximately 12 billion metric tons of CO 2 equivalent, [2] or about 21% of total global greenhouse gas emissions. [3] In areas such as the United States and Europe, changes in land use associated with human activities have the net effect of absorbing CO 2 , partially offsetting the emissions from deforestation in other regions.
EPA resources
- Greenhouse Gas Emissions
- Sources of Greenhouse Gas Emissions (in the United States)
- Non-CO 2 Greenhouse Gases: Emissions and Trends
- Capacity Building for National GHG Inventories
Other resources
- UNFCCC GHG Data Interface
- European Commission Emission Database for Global Atmospheric Research
- World Development Indicators
- Climate Watch
- Carbon Dioxide and Information Analysis Center (CDIAC)
- Greenhouse Gas Emissions from Energy Data Explorer (IEA)
1. IPCC (2022), Emissions Trends and Drivers. In IPCC, 2022: Climate Change 2022: Mitigation of Climate Change. Contribution of Working Group III to the Sixth Assessment Report of the Intergovernmental Panel on Climate Change. Cambridge University Press, Cambridge, UK and New York, NY, USA. doi: 10.1017/9781009157926.004
2. Jia, G., E. Shevliakova, P. Artaxo, N. De Noblet-Ducoudré, R. Houghton, J. House, K. Kitajima, C. Lennard, A. Popp, A. Sirin, R. Sukumar, L. Verchot, 2019: Land–climate interactions . In: Climate Change and Land: an IPCC special report on climate change, desertification, land degradation, sustainable land management, food security, and greenhouse gas fluxes in terrestrial ecosystems [P.R. Shukla, J. Skea, E. Calvo Buendia, V. Masson-Delmotte, H.-O. Pörtner, D.C. Roberts, P. Zhai, R. Slade, S. Connors, R. van Diemen, M. Ferrat, E. Haughey, S. Luz, S. Neogi, M. Pathak, J. Petzold, J. Portugal Pereira, P. Vyas, E. Huntley, K. Kissick, M, Belkacemi, J. Malley, (eds.)]. https://doi.org/10.1017/9781009157988.004
3. U.S. Energy Information Administration, Annual Energy Outlook 2021 , (February 2021), www.eia.gov/aeo
Note on emissions sector categories:
The global emission estimates described on this page are from the Intergovernmental Panel (IPCC) on Climate Change's Fifth Assessment Report. In this report, some of the sector categories are defined differently from how they are defined in the Sources of Greenhouse Gas Emissions page on this website. Transportation, Industry, Agriculture, and Land Use and Forestry are four global emission sectors that roughly correspond to the U.S. sectors. Energy Supply, Commercial and Residential Buildings, and Waste and Wastewater are categorized slightly differently. For example, the IPCC's Energy Supply sector for global emissions encompasses the burning of fossil fuel for heat and energy across all sectors. In contrast, the U.S. Sources discussion tracks emissions from the electric power separately and attributes on-site emissions for heat and power to their respective sectors (i.e., emissions from gas or oil burned in furnaces for heating buildings are assigned to the residential and commercial sector). The IPCC has defined Waste and Wastewater as a separate sector, while in the Sources of Greenhouse Gas Emissions page, waste and wastewater emissions are attributed to the Commercial and Residential sector.
- GHG Emissions and Removals Home
- Overview of Greenhouse Gases
- Sources of GHG Emissions and Removals
- Global Emissions and Removals
- National Emissions and Removals
- State and Tribal GHG Data and Resources
- Facility-Level Emissions
- Gridded Methane Emissions
- Carbon Footprint Calculator
- GHG Equivalencies Calculator
- Capacity Building for GHG Inventories

- SUGGESTED TOPICS
- The Magazine
- Newsletters
- Managing Yourself
- Managing Teams
- Work-life Balance
- The Big Idea
- Data & Visuals
- Reading Lists
- Case Selections
- HBR Learning
- Topic Feeds
- Account Settings
- Email Preferences
6 Common Leadership Styles — and How to Decide Which to Use When
- Rebecca Knight

Being a great leader means recognizing that different circumstances call for different approaches.
Research suggests that the most effective leaders adapt their style to different circumstances — be it a change in setting, a shift in organizational dynamics, or a turn in the business cycle. But what if you feel like you’re not equipped to take on a new and different leadership style — let alone more than one? In this article, the author outlines the six leadership styles Daniel Goleman first introduced in his 2000 HBR article, “Leadership That Gets Results,” and explains when to use each one. The good news is that personality is not destiny. Even if you’re naturally introverted or you tend to be driven by data and analysis rather than emotion, you can still learn how to adapt different leadership styles to organize, motivate, and direct your team.
Much has been written about common leadership styles and how to identify the right style for you, whether it’s transactional or transformational, bureaucratic or laissez-faire. But according to Daniel Goleman, a psychologist best known for his work on emotional intelligence, “Being a great leader means recognizing that different circumstances may call for different approaches.”

- RK Rebecca Knight is a journalist who writes about all things related to the changing nature of careers and the workplace. Her essays and reported stories have been featured in The Boston Globe, Business Insider, The New York Times, BBC, and The Christian Science Monitor. She was shortlisted as a Reuters Institute Fellow at Oxford University in 2023. Earlier in her career, she spent a decade as an editor and reporter at the Financial Times in New York, London, and Boston.
Partner Center

Evaluating the mismatch hypothesis: An analysis of the evolutionary history of Type II Diabetes
Evolutionary mismatch is a mechanism whereby disease-associated genetic variants that experienced positive natural selection in past environments because they were beneficial to fitness, may now contribute to negative health consequences in the context of present, modern environments. This project investigates the plausibility of the mismatch hypothesis for Type II Diabetes (T2D) by integrating genome-wide association data for individuals with South Asian ancestry (n=40,850) and evolutionary genomics.
Have a language expert improve your writing
Run a free plagiarism check in 10 minutes, generate accurate citations for free.
- Knowledge Base
Methodology
- Types of Variables in Research & Statistics | Examples
Types of Variables in Research & Statistics | Examples
Published on September 19, 2022 by Rebecca Bevans . Revised on June 21, 2023.
In statistical research , a variable is defined as an attribute of an object of study. Choosing which variables to measure is central to good experimental design .
If you want to test whether some plant species are more salt-tolerant than others, some key variables you might measure include the amount of salt you add to the water, the species of plants being studied, and variables related to plant health like growth and wilting .
You need to know which types of variables you are working with in order to choose appropriate statistical tests and interpret the results of your study.
You can usually identify the type of variable by asking two questions:
- What type of data does the variable contain?
- What part of the experiment does the variable represent?
Table of contents
Types of data: quantitative vs categorical variables, parts of the experiment: independent vs dependent variables, other common types of variables, other interesting articles, frequently asked questions about variables.
Data is a specific measurement of a variable – it is the value you record in your data sheet. Data is generally divided into two categories:
- Quantitative data represents amounts
- Categorical data represents groupings
A variable that contains quantitative data is a quantitative variable ; a variable that contains categorical data is a categorical variable . Each of these types of variables can be broken down into further types.
Quantitative variables
When you collect quantitative data, the numbers you record represent real amounts that can be added, subtracted, divided, etc. There are two types of quantitative variables: discrete and continuous .
Categorical variables
Categorical variables represent groupings of some kind. They are sometimes recorded as numbers, but the numbers represent categories rather than actual amounts of things.
There are three types of categorical variables: binary , nominal , and ordinal variables .
*Note that sometimes a variable can work as more than one type! An ordinal variable can also be used as a quantitative variable if the scale is numeric and doesn’t need to be kept as discrete integers. For example, star ratings on product reviews are ordinal (1 to 5 stars), but the average star rating is quantitative.
Example data sheet
To keep track of your salt-tolerance experiment, you make a data sheet where you record information about the variables in the experiment, like salt addition and plant health.
To gather information about plant responses over time, you can fill out the same data sheet every few days until the end of the experiment. This example sheet is color-coded according to the type of variable: nominal , continuous , ordinal , and binary .
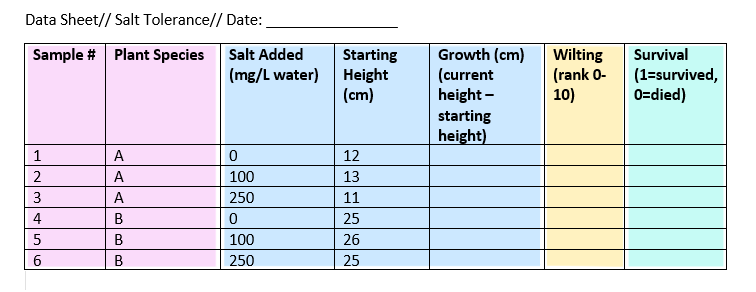
Receive feedback on language, structure, and formatting
Professional editors proofread and edit your paper by focusing on:
- Academic style
- Vague sentences
- Style consistency
See an example
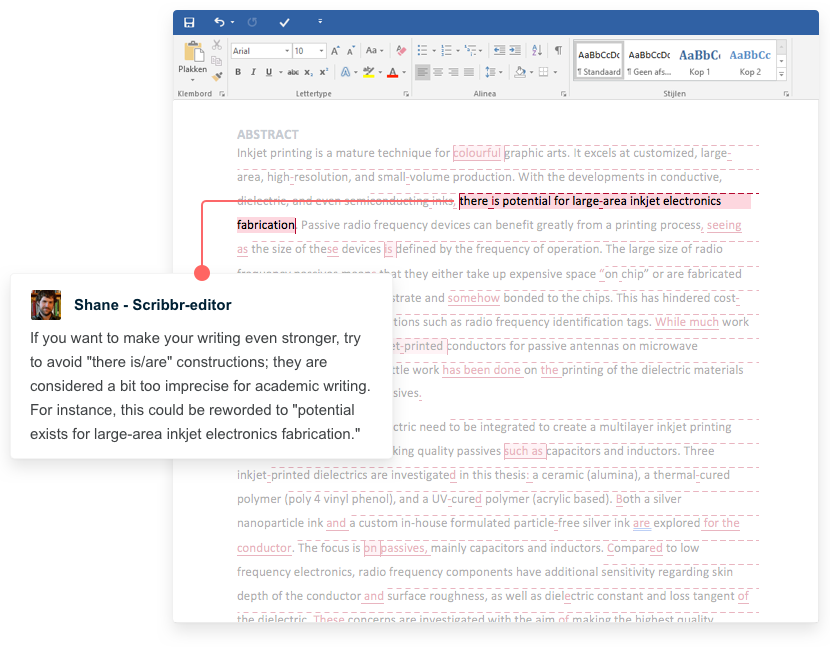
Experiments are usually designed to find out what effect one variable has on another – in our example, the effect of salt addition on plant growth.
You manipulate the independent variable (the one you think might be the cause ) and then measure the dependent variable (the one you think might be the effect ) to find out what this effect might be.
You will probably also have variables that you hold constant ( control variables ) in order to focus on your experimental treatment.
In this experiment, we have one independent and three dependent variables.
The other variables in the sheet can’t be classified as independent or dependent, but they do contain data that you will need in order to interpret your dependent and independent variables.
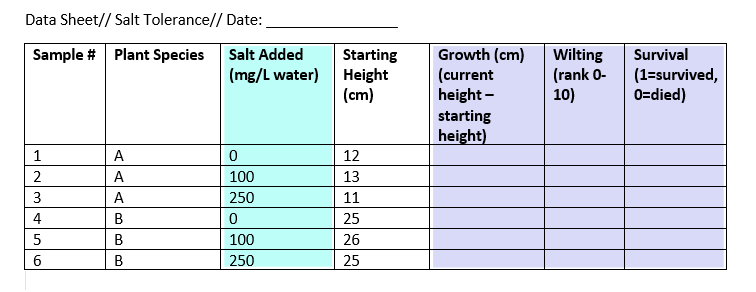
What about correlational research?
When you do correlational research , the terms “dependent” and “independent” don’t apply, because you are not trying to establish a cause and effect relationship ( causation ).
However, there might be cases where one variable clearly precedes the other (for example, rainfall leads to mud, rather than the other way around). In these cases you may call the preceding variable (i.e., the rainfall) the predictor variable and the following variable (i.e. the mud) the outcome variable .
Once you have defined your independent and dependent variables and determined whether they are categorical or quantitative, you will be able to choose the correct statistical test .
But there are many other ways of describing variables that help with interpreting your results. Some useful types of variables are listed below.
If you want to know more about statistics , methodology , or research bias , make sure to check out some of our other articles with explanations and examples.
- Student’s t -distribution
- Normal distribution
- Null and Alternative Hypotheses
- Chi square tests
- Confidence interval
- Cluster sampling
- Stratified sampling
- Data cleansing
- Reproducibility vs Replicability
- Peer review
- Likert scale
Research bias
- Implicit bias
- Framing effect
- Cognitive bias
- Placebo effect
- Hawthorne effect
- Hindsight bias
- Affect heuristic
You can think of independent and dependent variables in terms of cause and effect: an independent variable is the variable you think is the cause , while a dependent variable is the effect .
In an experiment, you manipulate the independent variable and measure the outcome in the dependent variable. For example, in an experiment about the effect of nutrients on crop growth:
- The independent variable is the amount of nutrients added to the crop field.
- The dependent variable is the biomass of the crops at harvest time.
Defining your variables, and deciding how you will manipulate and measure them, is an important part of experimental design .
A confounding variable , also called a confounder or confounding factor, is a third variable in a study examining a potential cause-and-effect relationship.
A confounding variable is related to both the supposed cause and the supposed effect of the study. It can be difficult to separate the true effect of the independent variable from the effect of the confounding variable.
In your research design , it’s important to identify potential confounding variables and plan how you will reduce their impact.
Quantitative variables are any variables where the data represent amounts (e.g. height, weight, or age).
Categorical variables are any variables where the data represent groups. This includes rankings (e.g. finishing places in a race), classifications (e.g. brands of cereal), and binary outcomes (e.g. coin flips).
You need to know what type of variables you are working with to choose the right statistical test for your data and interpret your results .
Discrete and continuous variables are two types of quantitative variables :
- Discrete variables represent counts (e.g. the number of objects in a collection).
- Continuous variables represent measurable amounts (e.g. water volume or weight).
Cite this Scribbr article
If you want to cite this source, you can copy and paste the citation or click the “Cite this Scribbr article” button to automatically add the citation to our free Citation Generator.
Bevans, R. (2023, June 21). Types of Variables in Research & Statistics | Examples. Scribbr. Retrieved April 9, 2024, from https://www.scribbr.com/methodology/types-of-variables/
Is this article helpful?
Rebecca Bevans
Other students also liked, independent vs. dependent variables | definition & examples, confounding variables | definition, examples & controls, control variables | what are they & why do they matter, "i thought ai proofreading was useless but..".
I've been using Scribbr for years now and I know it's a service that won't disappoint. It does a good job spotting mistakes”
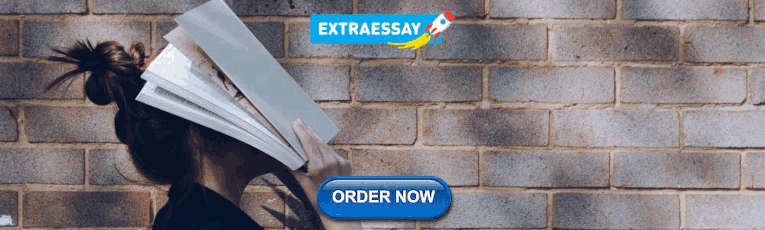
IMAGES
VIDEO
COMMENTS
Research data plays a critical role in scientific inquiry and is often subject to rigorous analysis, interpretation, and dissemination to advance knowledge and inform decision-making. Types of Research Data. There are generally four types of research data: Quantitative Data. This type of data involves the collection and analysis of numerical data.
As we mentioned above discrete and continuous data are the two key types of quantitative data. In statistics, marketing research, and data science, many decisions depend on whether the basic data is discrete or continuous. 5. Discrete data. Discrete data is a count that involves only integers.
Definition of research in data analysis: According to LeCompte and Schensul, research data analysis is a process used by researchers to reduce data to a story and interpret it to derive insights. The data analysis process helps reduce a large chunk of data into smaller fragments, which makes sense. Three essential things occur during the data ...
Qualitative and quantitative data are the two main types of data. Quantitative data is numerical and can be measured, while qualitative data is non-numerical and cannot be measured. Quantitative data can be further divided into discrete and continuous data. Discrete data is a count that involves only whole numbers, while continuous data can be ...
The distribution of data obtained from a sample is crucial to understand how to analyze it. There are various probability distributions for different types of data. Of these, one particularly important type used in everyday research is the distribution of continuous numerical data. This data may either be normally distributed or skewed.
Defining Research Data. One definition of research data is: "the recorded factual material commonly accepted in the scientific community as necessary to validate research findings." ( OMB Circular 110 ). Research data covers a broad range of types of information (see examples below), and digital data can be structured and stored in a variety of ...
This type of data allows you to perform various calculations, such as averages, correlations, and regressions, to identify patterns, trends, and relationships. Discrete data and continuous data are types of quantitative data. When analyzing quantitative data, you can use summary statistics to describe the dispersion of the data.
Research data comes in many different formats and is gathered using a wide variety of methodologies. In this module, we will provide you with a basic definition and understanding of what research data are. We'll also explore how data fits into the scholarly research process. ... "research data, unlike other types of information, ...
Types of Research Designs Compared | Guide & Examples. Published on June 20, 2019 by Shona McCombes.Revised on June 22, 2023. When you start planning a research project, developing research questions and creating a research design, you will have to make various decisions about the type of research you want to do.. There are many ways to categorize different types of research.
Data may be grouped into four main types based on methods for collection: observational, experimental, simulation, and derived. The type of research data you collect may affect the way you manage that data. For example, data that is hard or impossible to replace (e.g. the recording of an event at a specific time and place) requires extra backup ...
The research methods you use depend on the type of data you need to answer your research question. If you want to measure something or test a hypothesis, use quantitative methods. If you want to explore ideas, thoughts and meanings, use qualitative methods. If you want to analyze a large amount of readily-available data, use secondary data.
Types of data # In empirical research, we collect and interpret data in order to answer questions about the world. "Data" in this context usually results from some form of "measurement". The notion of measurement here is very broad - it could include familiar acts like using a ruler to measure the length of an object, but it could also include asking a human research subject a ...
Types of Variables. When conducting research, experimenters often manipulate variables. For example, an experimenter might compare the effectiveness of four types of antidepressants. In this case, the variable is "type of antidepressant." When a variable is manipulated by an experimenter, it is called an independent variable.
Data collection is a systematic process of gathering observations or measurements. Whether you are performing research for business, governmental or academic purposes, data collection allows you to gain first-hand knowledge and original insights into your research problem. While methods and aims may differ between fields, the overall process of ...
Qualitative and Quantitative Data: Education schools research and analyze two main types of data: qualitative and quantitative. Scholars typically define their methodology by either of these categories or as a mixed method combining both. Qualitative data tells a story. Such data are non-numerical in nature. They also have a low level of ...
Types of Data. Some types of research data and data files are fairly ubiquitous and can be found distributed across disciplines: Images. Video. Mapping/GIS data. Numerical measurements. Research data in Social Sciences can include: Survey responses. Focus group and individual interviews.
Quantitative data analysis then splits into mathematical analysis and artificial intelligence (AI) analysis. Mathematical types then branch into descriptive, diagnostic, predictive, and prescriptive. Methods falling under mathematical analysis include clustering, classification, forecasting, and optimization.
Research data is any information that has been collected, observed, generated or created to validate original research findings. Although usually digital, research data also includes non-digital formats such as laboratory notebooks and diaries. Types of research data. Research data can take many forms. It might be: documents, spreadsheets
What are the main types of qualitative approaches to research? While there are many different investigations that can be done, a study with a qualitative approach generally can be described with the characteristics of one of the following three types: Historical research describes past events, problems, issues and facts. Data are gathered from ...
Research data are often grouped into one of four major types based on the methodology used to collect it. What data type is generated by imitating the operation of a real-world process or system over time using computer test models? a - Observational data. b - Experimental data. c ...
This fundamental research type is defined by the fact that the data is collected directly from the source, that is, it consists of primary, first-hand information. Secondary research Unlike primary research, secondary research is developed with information from secondary sources, which are generally based on scientific literature and other ...
2 Types Of Raw Data and Their Examples. Raw data includes a wide range of data types, which are typically classified as either qualitative or quantitative. The most important condition for this categorization is that the data is not cleaned or processed in any way. Raw data allows unrivaled flexibility and control over the information collected ...
PURPOSE National estimates of cancer clinical trial participation are nearly two decades old and have focused solely on enrollment to treatment trials, which does not reflect the willingness of patients to contribute to other elements of clinical research. We determined inclusive, contemporary estimates of clinical trial participation for adults with cancer using a national sample of data from ...
A research design is a strategy for answering your research question using empirical data. Creating a research design means making decisions about: Your overall research objectives and approach. Whether you'll rely on primary research or secondary research. Your sampling methods or criteria for selecting subjects. Your data collection methods.
Intermediate processes: The third type of process is between the two extremes. Such processes may accept multiple kinds of batteries, unlike direct recovery, but recover materials further along the production chain than smelting does.
Global Emissions and Removals by Gas. At the global scale, the key greenhouse gases emitted by human activities are: Carbon dioxide (CO 2): Fossil fuel use is the primary source of CO 2.CO 2 can also be emitted from the landscape through deforestation, land clearance for agriculture or development, and degradation of soils. Likewise, land management can also remove additional CO 2 from the ...
Research suggests that the most effective leaders adapt their style to different circumstances — be it a change in setting, a shift in organizational dynamics, or a turn in the business cycle ...
This project investigates the plausibility of the mismatch hypothesis for Type II Diabetes (T2D) by integrating genome-wide association data for individuals with South Asian ancestry (n=40,850) and evolutionary genomics. ... Undergraduate Research and Fellowships Mentoring Office 321 Boucke Building University Park, PA 16802 814-863-8199 ...
Types of data: Quantitative vs categorical variables. Data is a specific measurement of a variable - it is the value you record in your data sheet. Data is generally divided into two categories: Quantitative data represents amounts; Categorical data represents groupings
Here, we aim to shed light on the patterns of above- and belowground nutrient dynamics mediated by mycorrhizal type and tree species richness. The research was conducted within the tree diversity experiment, MyDiv, which manipulates both tree species richness and mycorrhizal strategy (Ferlian et al., 2018b).