The Android OS stack and its vulnerabilities: an empirical study
- Published: 20 February 2019
- Volume 24 , pages 2056–2101, ( 2019 )
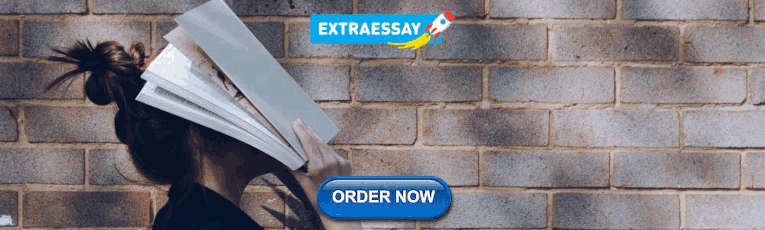
Cite this article
- Alejandro Mazuera-Rozo 1 , 2 ,
- Jairo Bautista-Mora 1 ,
- Mario Linares-Vásquez ORCID: orcid.org/0000-0003-0161-2888 1 ,
- Sandra Rueda 1 &
- Gabriele Bavota 2
1975 Accesses
15 Citations
5 Altmetric
Explore all metrics
The wide and rapid adoption of Android-based devices in the last years has motivated the usage of Android apps to support a broad range of daily activities. In that sense, being the most popular mobile platform makes it an attractive target for security attacks. In fact, 1,489 security vulnerabilities have been reported in the last three years (2015-2017) for the Android OS (which is the underlying platform for Android-based devices). While there is a plethora of approaches and tools for detecting malware and security issues in Android apps, few research has been done to identify, categorize, or detect vulnerabilities in the Android OS. In this paper we present the largest study so far aimed at analyzing software vulnerabilities in the Android OS. In particular, we analyzed a total of 1,235 vulnerabilities from four different perspectives: vulnerability types and their evolution, CVSS vectors that describe the vulnerabilities, impacted Android OS layers, and their survivability across the Android OS history. Based on our findings, we propose a list of future actions that could be performed by researchers and practitioners to reduce the number of vulnerabilities in the Android OS as well as their impact and survivability.
This is a preview of subscription content, log in via an institution to check access.
Access this article
Price includes VAT (Russian Federation)
Instant access to the full article PDF.
Rent this article via DeepDyve
Institutional subscriptions
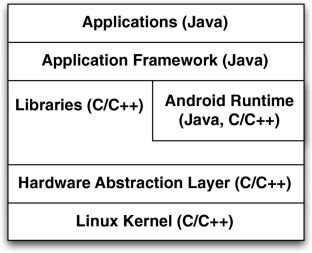
Similar content being viewed by others
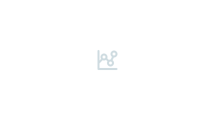
The current state and future of mobile security in the light of the recent mobile security threat reports
Ahmet Cevahir Cinar & Turkan Beyza Kara
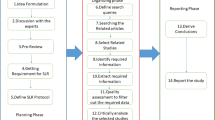
An Overview of Techniques for Obfuscated Android Malware Detection
Sidra Siddiqui & Tamim Ahmed Khan
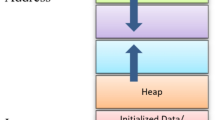
A Review on Malware Analysis for IoT and Android System
Chandra Shekhar Yadav & Sangeeta Gupta
We used the base group attributes because it is the only mandatory group.
https://tinyurl.com/yadtggsr
https://tinyurl.com/y9u5odrv
Meta-analysis is a statistical inference technique aimed at consolidating results from more than one study or experiment.
We found 129 vulnerabilities for the 2017-2 period, but we excluded this semester from the RQ 5 analysis because we do not have complete data for 2017-2.
We were not able to classify the layer affected by 5 vulnerabilities.
Note that there is also an Unclear category because in six cases we were not able to identify the type of change because of the patches complexity . For instance, in CVE-2016-3751 several changes were done over 207 files, thus it was not easy to categorize the changes.
https://tinyurl.com/y777kx6s
https://tinyurl.com/yblfv22p
https://tinyurl.com/yaehg5b2
https://tinyurl.com/ya8rbl7n
https://tinyurl.com/ychpav57
https://tinyurl.com/y6v7me9z
For the base group attributes in CVSS 2.0, there are 729 possible combinations of attribute values. Therefore, the 1,235 analyzed vulnerabilities cover 6.72% (49 out of 729) of all the CVSS 2.0 vectors for the base group attributes.
Compared to Linares-Vásquez et al. ( 2017 ), in our dataset we observed 354 new vulnerabilities in the Kernel that have been reported from November 2016 to August 2017.
Note that we only report numbers for vulnerabilities in the AOSP apps and reported as vulnerabilities in the NVD database.
Note that all p -values equal 1.0 after the holm correction procedure. Before that they were in any case all higher than 0.7.
Aosp commit cf1581c66c2ad8c5b1aaca2e43e350cf5974f46d (2017a) http://tinyurl.com/hxqdp7f
Aosp commit 8ec845c8fe0f03bc57c901bc484541bdd6a7cf80 (2017b) http://tinyurl.com/hvndh7r
Aosp commit edd4a76eb4747bd19ed122df46fa46b452c12a0d (2017c) http://tinyurl.com/hkw399d
Ahmad W, Kästner C, Sunshine J, Aldrich J (2016) Inter-app communication in android: Developer challenges. In: Proceedings of the 13th international conference on mining software repositories, MSR ’16. ACM, New York, pp 177–188. https://doi.org/10.1145/2901739.2901762
Anderson B, et al. (2016) Hpe security research. cyber risk report 2016. Tech. rep., Hewlett Packard
Armis (2017) The attack vector “blueborne” exposes almost every connected device. https://www.armis.com/blueborne/
Arzt S, Rasthofer S, Fritz C, Bodden E, Bartel A, Klein J, Le Traon Y, Octeau D, McDaniel P (2014) Flowdroid: Precise context, flow, field, object-sensitive and lifecycle-aware taint analysis for android apps. In: Proceedings of the 35th ACM SIGPLAN Conference on Programming Language Design and Implementation, PLDI ’14. ACM, New York, pp 259–269. https://doi.org/10.1145/2594291.2594299
Avdiienko V, Kuznetsov K, Gorla A, Zeller A, Arzt S, Rasthofer S, Bodden E (2015) Mining apps for abnormal usage of sensitive data. In: ICSE’15, pp 426–436. http://dl.acm.org/citation.cfm?id=2818754.2818808
Backes M, Bugiel S, Derr E (2016) Reliable third-party library detection in android and its security applications. In: Proceedings of the 2016 ACM SIGSAC conference on computer and communications security, CCS ’16. ACM, New York, pp 356–367. https://doi.org/10.1145/2976749.2978333
Bagheri H, Kang E, Malek S, Jackson D (In Press) A formal approach for detection of security flaws in the android permission system. Springer Journal on Formal Aspects of Computing
Bagheri H, Sadeghi A, Garcia J, Malek S (2015) Covert: compositional analysis of android inter-app permission leakage. IEEE Trans Softw Eng 41(9):866–886. https://doi.org/10.1109/TSE.2015.2419611
Article Google Scholar
Beres D (2015) ‘cowboy adventure’ game infects up to 1 million android users with malware. http://www.huffingtonpost.com/2015/07/10/android-security_n_7765842.html
Bhosale A (2014) Precise static analysis of taint flow for android application sets. Master’s thesis, Heinz College Carnegie Mellon University
Brady P (2008) Anatomy & physiology of an android. https://sites.google.com/site/io/anatomy--physiology-of-an-android https://sites.google.com/site/io/anatomy--physiology-of-an-android
Burgess M (2016) Millions of android devices vulnerable to new stagefright exploit. http://www.wired.co.uk/article/stagefright-android-real-world-hack
Cao C, Gao N, Liu P, Xiang J (2015) Towards analyzing the input validation vulnerabilities associated with android system services. In: Proceedings of the 31st annual computer security applications conference, ACSAC 2015. ACM, New York, pp 361–370. https://doi.org/10.1145/2818000.2818033
Castellanos JH, Wuchner T, Ochoa M, Rueda S (2016) Q-floid: Android malware detection with quantitative data flow graphs. In: Singapore cyber-security conference (SG-CRC). IOS Press, pp 13–26
Christensen R (2011) Plane Answers to Complex Questions: The Theory of Linear models, 4th edn. Springer Texts in Statistics Springer, Berlin
Book MATH Google Scholar
Conover WJ (1998) Practical Nonparametric Statistics, 3rd edn. Wiley, New York
Google Scholar
Corporation M (2017) Cve common vulnerabilities and exposures. http://cve.mitre.org
Cumming G (2011) Introduction to the new Statistics: Effect sizes, confidence intervals, and Meta-Analysis. Routledge, Evanston
Cve-2012-6636 (2017) https://www.cvedetails.com/cve/cve-2012-6636
Dimjaševic M, Atzeni S, Ugrina I, Rakamaric Z (2015) Android malware detection based on system calls
Drake JJ, Lanier Z, Mulliner C, Fora PO, Ridley SA, Wicherski G (2014) Android hacker’s handbook. Wiley, New York
Enck W, Gilbert P, Chun BG, Cox L, Jung J, McDaniel P, Sheth AN (2010) Taintdroid: an information-flow tracking system for realtime privacy monitoring on smartphones. In: Proceedings of the 9th USENIX conference on operating systems design and implementation, OSDI’10. USENIX Association, Berkeley, pp 393–407. http://dl.acm.org/citation.cfm?id=1924943.1924971
Enck W, Ongtang M, McDaniel P (2009) On lightweight mobile phone application certification. In: Proceedings of the 16th ACM conference on computer and communications security, CCS ’09. ACM, New York, pp 235–245. https://doi.org/10.1145/1653662.1653691
Fahl S, Harbach M, Muders T, Baumgärtner L., Freisleben B, Smith M (2012) Why eve and mallory love android: an analysis of android ssl (in)security. In: Proceedings of the 2012 ACM conference on computer and communications security, CCS ’12. ACM, New York, pp 50–61. https://doi.org/10.1145/2382196.2382205
Fattori A, Tam K, Khan SJ, Cavallaro L, Reina A (2014) CopperDroid: On the Reconstruction of Android Malware Behaviors. Tech. rep. Royal Holloway University of London
FIRST Organization (2019) Common vulnerability scoring system sig. https://www.first.org/cvss
for Standardization IO (2011) Iso 27005 information security risk management
Garcia J, Hammad M, Ghorbani N, Malek S (2017) Automatic generation of inter-component communication exploits for android applications. In: Proceedings of the 2017 11th joint meeting on foundations of software engineering, ESEC/FSE 2017. ACM, New York, pp 661–671. https://doi.org/10.1145/3106237.3106286
Gasior W, Yang L (2012) Exploring covert channel in android platform. In: 2012 international conference on cyber security, pp 173–177. https://doi.org/10.1109/CyberSecurity.2012.29
Ghafari M, Gadient P, Nierstrasz O (2017) Security smells in android. In: 2017 IEEE 17th international working conference on source code analysis and manipulation (SCAM), pp 121–130. https://doi.org/10.1109/SCAM.2017.24
Gilbert P, Chun BG, Cox LP, Jung J (2011) Vision: automated security validation of mobile apps at app markets. In: Proceedings of the second international workshop on mobile cloud computing and services, MCS ’11. ACM, New York, pp 21–26. https://doi.org/10.1145/1999732.1999740
Gorla A, Tavecchia I, Gross F, Zeller A (2014) Checking app behavior against app descriptions. In: ICSE’14, pp 1025–1035. https://doi.org/10.1145/2568225.2568276
Google (2016) Android security 2015 year in review. https://static.googleusercontent.com/media/source.android.com/en//security/reports/Google_Android_Security_2015_Report_Final.pdf
Google (2017a) Android security bulletins. https://source.android.com/security/bulletin/
Google (2017b) Platform architecture. https://developer.android.com/guide/platform/index.html
Graf J, Hecker MMM (2015) Jodroid: Adding android support to a static information flow control tool. In: Working conference on programming languages
Grissom RJ, Kim JJ (2005) Effect sizes for research: a broad practical approach, 2nd edn. Lawrence Earlbaum Associates, New Jersey
Hedges LV, Olkin I (1985) Statistical methods for Meta-Analysis. Academic Press, New York
MATH Google Scholar
Herzig K, Zeller A (2013) The impact of tangled code changes. In: Proceedings of the 10th Working Conference on Mining Software Repositories, MSR ’13, San Francisco, pp 121–130
Holm S (1979) A simple sequentially rejective Bonferroni test procedure. Scand J Stat 6:65–70
Huang H, Zhu S, Chen K, Liu P (2015) From system services freezing to system server shutdown in android: All you need is a loop in an app. In: Proceedings of the 22nd ACM SIGSAC conference on computer and communications security, CCS ’15. ACM, New York, pp 1236–1247. https://doi.org/10.1145/2810103.2813606
Jimenez M, Papadakis M, Bissyandé TF, Klein J (2016) Profiling android vulnerabilities. In: 2016 IEEE International conference on software quality, reliability and security (QRS), pp 222–229. https://doi.org/10.1109/QRS.2016.34 https://doi.org/10.1109/QRS.2016.34
Kantola D, Chin E, He W, Wagner D (2012) Reducing attack surfaces for intra-application communication in android. In: Proceedings of the second ACM workshop on security and privacy in smartphones and mobile devices, SPSM ’12. ACM, New York, pp 69–80. https://doi.org/10.1145/2381934.2381948
Kim S, James Whitehead Jr E, Zhang Y (2008) Classifying software changes: clean or buggy? IEEE Trans Softw Eng 34(2):181–196
Lal S, Sureka A (2012) Comparison of seven bug report types: a case-study of google chrome browser project. In: 2012 19th asia-pacific software engineering conference, vol 1, pp 517–526. https://doi.org/10.1109/APSEC.2012.54
Lee S, Hwang S, Ryu S (2017) All about activity injection: Threats, semantics, and detection. In: Proceedings of the 32nd IEEE/ACM international conference on automated software engineering, ASE 2017. IEEE Press, Piscataway, pp 252–262. http://dl.acm.org/citation.cfm?id=3155562.3155597
Li GK (2010) Computing inter-rater reliability and its variance in the presence of high agreement. Br J Math Stat Psychol 61(1):29–48. https://doi.org/10.1348/000711006X126600
MathSciNet Google Scholar
Linares-Vásquez M, Bavota G, Escobar-Velásquez C (2017) An empirical study on android-related vulnerabilities. In: Proceedings of the 14th international conference on mining software repositories, MSR ’17. IEEE Press, Piscataway, pp 2–13. https://doi.org/10.1109/MSR.2017.60
LLC PI (2014) The security impact of mobile device use by employees. Tech. rep., Ponemon Institute
Lu L, Li Z, Wu Z, Lee W, Jiang G (2012) Chex: statically vetting android apps for component hijacking vulnerabilities. In: ACM Conference on computer and communications security, pp 229–240
Mazuera-Rozo A, Bautista-Mora J, Linares-Vásquez M, Rueda S, Bavota G (2017) Replication package: “The Android OS Stack and its Vulnerabilities: An Empirical Study”. http://ml-papers.gitlab.io/android.vulnerabilities-2017/appendix/
Mell P, Scarfone K, Romanosky S (2007) A Complete Guide to the Common Vulnerability Scoring System Version 2.0, 2.0 edn
MITRE (2017a) Cwe-120: Buffer copy without checking size of input (‘classic buffer overflow’). https://cwe.mitre.org/data/definitions/120.html
MITRE (2017b) Cwe-121: Stack-based buffer overflow. https://cwe.mitre.org/data/definitions/121.html
MITRE (2017c) Cwe-122: Heap-based buffer overflow. https://cwe.mitre.org/data/definitions/122.html
MITRE (2017d) Cwe-190: Integer overflow or wraparound. https://cwe.mitre.org/data/definitions/190.html
MITRE (2017e) Cwe-201: Information exposure through sent data. https://cwe.mitre.org/data/definitions/201.html
MITRE (2017f) Cwe-275: Permission issues. https://cwe.mitre.org/data/definitions/275.html
MITRE (2017g) Cwe-296: Improper following of a certificate’s chain of trust. https://cwe.mitre.org/data/definitions/296.html
MITRE (2017h) Cwe-326: Inadequate encryption strength. https://cwe.mitre.org/data/definitions/326.html
MITRE (2017i) Cwe-327: Use of a broken or risky cryptographic algorithm. https://cwe.mitre.org/data/definitions/327.html
MITRE (2017j) Cwe-415: Double free. https://cwe.mitre.org/data/definitions/415.html
MITRE (2017k) Cwe-787: Out-of-bounds write. https://cwe.mitre.org/data/definitions/787.html
MITRE (2017l) Cwe-840: Business logic errors. https://cwe.mitre.org/data/definitions/840.html
MITRE (2017m) Cwe-862: Missing authorization. https://cwe.mitre.org/data/definitions/862.html
MITRE (2017n) Cwe-909: Missing initialization of resource. https://cwe.mitre.org/data/definitions/909.html
MITRE (2017o) Cwe-94: Improper control of generation of code (’code injection’). https://cwe.mitre.org/data/definitions/94.html
MITRE (2017p) Common weakness enumeration http://cwe.mitre.org/
MITRE (2017q) Cve details Android vulnerabilities. https://www.cvedetails.com/product/19997/Google-Android.html
MITRE (2017r) Cve details. https://www.cvedetails.com/
Morales LV, Rueda SJ (2015) Meaningful permission management in android. IEEE Lat Am Trans 13(4):1160–1166. https://doi.org/10.1109/TLA.2015.7106371
Nickinson P (2015) The ’stagefright’ exploit: what you need to know. http://www.androidcentral.com/stagefright
NIST (2015) Common vulnerability scoring system calculator version 2. https://nvd.nist.gov/vuln-metrics/cvss/v2-calculator
NIST (2017) Nvd data feeds http://nvd.nist.gov/download.cfm#{RSS}
Novak E, Tang Y, Hao Z, Li Q, Zhang Y (2015) Physical media covert channels on smart mobile devices. In: Proceedings of the 2015 ACM international joint conference on pervasive and ubiquitous computing, UbiComp ’15. ACM, New York, pp 367–378. https://doi.org/10.1145/2750858.2804253
Park Y, Reeves DS (2013) Deriving common malware behavior through graph clustering. Comput Secur 39(PART B):419–430. https://doi.org/10.1016/j.cose.2013.09.006
Ren C, Zhang Y, Xue H, Wei T, Liu P (2015) Towards discovering and understanding task hijacking in android. In: Proceedings of the 24th USENIX conference on security symposium, SEC’15. USENIX Association, Berkeley, pp 945–959. http://dl.acm.org/citation.cfm?id=2831143.2831203
Rust (2013) https://www.rust-lang.org
Sadeghi A, Bagheri H, Malek S (2015) Analysis of android inter-app security vulnerabilities using covert. In: ICSE’15, pp 725–728. http://dl.acm.org/citation.cfm?id=2819009.2819149
Sadeghi A, Bagheri H, Garcia J, Malek S (2016) A taxonomy and qualitative comparison of program analysis techniques for security assessment of android software. IEEE Trans Softw Eng PP(99):1–1. https://doi.org/10.1109/TSE.2016.2615307
Sadeghi A, Jabbarvand R, Malek S (2017) Patdroid: Permission-aware gui testing of android. In: Proceedings of the 2017 11th joint meeting on foundations of software engineering, ESEC/FSE 2017. ACM, New York, pp 220–232. https://doi.org/10.1145/3106237.3106250
Sbîrlea D, Burke MG, Guarnieri S, Pistoia M, Sarkar V (2013) Automatic detection of inter-application permission leaks in android applications. IBM J Res Dev 57(6):2:10–2:10. https://doi.org/10.1147/JRD.2013.2284403 https://doi.org/10.1147/JRD.2013.2284403
Sliwerski J, Zimmermann T, Zeller A (2005) When do changes induce fixes? In: Proceedings of the 2005 International Workshop on Mining Software Repositories
Stefanko L (2015) Aggressive android ransomware spreading in the usa. http://www.welivesecurity.com/2015/09/10/aggressive-android-ransomware-spreading-in-the-usa/
Sufatrio Tan DJJ, Chua TW, Thing VLL (2015) Securing android: a survey, taxonomy, and challenges. ACM Comput Surv 47(4):58:1–58:45. https://doi.org/10.1145/2733306
Thomas DR (2015a) The Lifetime of Android API Vulnerabilities: Case Study on the JavaScript-to-Java Interface (Transcript of Discussion). Springer International Publishing, Cham, pp 139–144. https://doi.org/10.1007/978-3-319-26096-9_14
Thomas DR, Beresford AR, Rice A (2015b) Security metrics for the android ecosystem. In: Proceedings of the 5th annual ACM CCS workshop on security and privacy in smartphones and mobile devices, SPSM ’15. ACM, New York, pp 87–98. https://doi.org/10.1145/2808117.2808118
Tufano M, Watson C, Bavota G, Di Penta M, White M, Poshyvanyk D (2018) An empirical investigation into learning bug-fixing patches in the wild via neural machine translation. In: Proceedings of the 33rd ACM/IEEE international conference on automated software engineering, ASE 2018. ACM, New York, pp 832–837. https://doi.org/10.1145/3238147.3240732
U.S. National Institute of Standards and Technology - NIST (2012) National vulnerability database. http://nvd.nist.gov
U.S. National Institute of Standards and Technology - NIST (2012) Sp 800-30 guide for conducting risk assessments
VisionMobile: Developer economics q1 2014 (2014) State of the developer nation. Tech. rep.
Wang K, Zhang Y, Liu P (2016) Call me back!: Attacks on system server and system apps in android through synchronous callback. In: Proceedings of the 2016 ACM SIGSAC conference on computer and communications security, CCS ’16. ACM, New York, pp 92–103. https://doi.org/10.1145/2976749.2978342
Weichselbaum L, Neugschwandtner M, Lindorfer M, Fratantonio Y, Veen VVD, Platzer C (2012) ANDRUBIS: Android Malware Under The Magnifying Glass. Tech. rep., Vienna University of Technology. https://www.iseclab.org/papers/andrubis_techreport.pdf
wiki. L (2015) Android kernel features. http://elinux.org/Android_Kernel_Features
Wikipedia (2017a) Android version history https://en.wikipedia.org/wiki/Android_version_history
Wikipedia (2017b) Heartbleed https://en.wikipedia.org/wiki/Heartbleed
Wikipedia (2017c) Stagefright https://en.wikipedia.org/wiki/Stagefright_(bug)
Wu L, Grace M, Zhou Y, Wu C, Jiang X (2013) The impact of vendor customizations on android security. In: Proceedings of the 2013 ACM SIGSAC conference on computer & communications security, CCS ’13. ACM, New York, pp 623–634. https://doi.org/10.1145/2508859.2516728
Xiao X, Tillman N, Fahndrich M, DeHalleux J, Moskal M (2012) User-aware privacy control via extended static-information-flow analysis. In: IEEE/ACM international conference on automated software engineering
Xu M, Song C, Ji Y, Shih MW, Lu K, Zheng C, Duan R, Jang Y, Lee B, Qian C, Lee S, Kim T (2016) Toward engineering a secure android ecosystem: a survey of existing techniques. ACM Comput Surv 49(2):38:1–38:47. https://doi.org/10.1145/2963145
You W, Liang B, Shi W, Zhu S, Wang P, Xie S, Zhang X (2016) Reference hijacking: Patching, protecting and analyzing on unmodified and non-rooted android devices. In: Proceedings of the 38th international conference on software engineering, ICSE ’16. ACM, New York, pp 959–970. https://doi.org/10.1145/2884781.2884863
Zaman S, Adams B, Hassan AE (2011) Security versus performance bugs: a case study on firefox. In: Proceedings of the 8th working conference on mining software repositories, MSR’11. ACM, New York, pp 93–102. https://doi.org/10.1145/1985441.1985457
Zhou Y, Jiang X (2012) Android malware genome project. http://www.malgenomeproject.org/
Zhou Y, Jiang X (2012) Dissecting android malware: characterization and evolution. In: 2012 IEEE Symposium on security and privacy, pp 95–109. https://doi.org/10.1109/SP.2012.16
Zuo C, Wu J, Guo S (2015) Automatically detecting ssl error-handling vulnerabilities in hybrid mobile web apps. In: Proceedings of the 10th ACM symposium on information, computer and communications security, ASIA CCS ’15. ACM, New York, pp 591–596. https://doi.org/10.1145/2714576.2714583
Download references
Author information
Authors and affiliations.
Universidad de los Andes, Bogotá, Colombia
Alejandro Mazuera-Rozo, Jairo Bautista-Mora, Mario Linares-Vásquez & Sandra Rueda
Università della Svizzera italiana, Lugano, Switzerland
Alejandro Mazuera-Rozo & Gabriele Bavota
You can also search for this author in PubMed Google Scholar
Corresponding author
Correspondence to Mario Linares-Vásquez .
Additional information
Communicated by: Lin Tan
Publisher’s note
Springer Nature remains neutral with regard to jurisdictional claims in published maps and institutional affiliations.
Rights and permissions
Reprints and permissions
About this article
Mazuera-Rozo, A., Bautista-Mora, J., Linares-Vásquez, M. et al. The Android OS stack and its vulnerabilities: an empirical study. Empir Software Eng 24 , 2056–2101 (2019). https://doi.org/10.1007/s10664-019-09689-7
Download citation
Published : 20 February 2019
Issue Date : 15 August 2019
DOI : https://doi.org/10.1007/s10664-019-09689-7
Share this article
Anyone you share the following link with will be able to read this content:
Sorry, a shareable link is not currently available for this article.
Provided by the Springer Nature SharedIt content-sharing initiative
- Vulnerabilities
- Empirical study
- Operating system
- Find a journal
- Publish with us
- Track your research
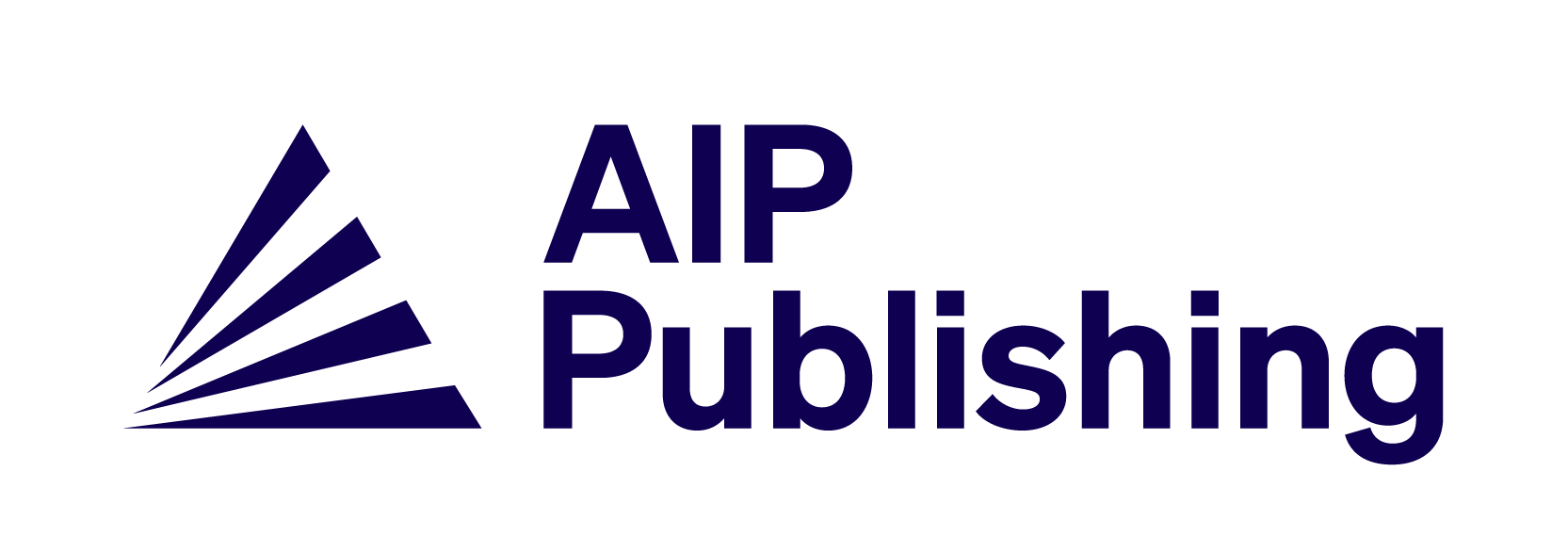
An inclusive analysis of Google’s android operating system and its security
- Article contents
- Figures & tables
- Supplementary Data
- Peer Review
- Reprints and Permissions
- Cite Icon Cite
- Search Site
Pankaj Saraswat; An inclusive analysis of Google’s android operating system and its security. AIP Conf. Proc. 27 February 2023; 2427 (1): 020097. https://doi.org/10.1063/5.0101614
Download citation file:
- Ris (Zotero)
- Reference Manager
In the era of smartphones where a kind of race for advanced development of hardware tools for mobiles setups became a significant part of the industry and to support these advanced tools and smartphones performance of prior software’s is the top priority of the software industry. As features of mobile phones completely depend on the software and to process this software a reliable operating system is need of the hour. In this context, the Android operating system (OS), the software application developed around the SDK has grown to become the most popular and used OS worldwide and considering the prominent demand of it in the industry a thorough study of this OS becomes a necessity. Despite the fact that millions of users each and every year choose mobile phones having android OS as a preference, an inclusive analysis of android OS is not yet present. In this research paper, a comprehensive analysis of this popular OS, its features, security tools etc. has been carried out and also discusses how this software application will improve and evolve in this competitive race of the advancement of the software.
Sign in via your Institution
Citing articles via, publish with us - request a quote.
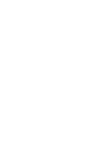
Sign up for alerts
- Online ISSN 1551-7616
- Print ISSN 0094-243X
- For Researchers
- For Librarians
- For Advertisers
- Our Publishing Partners
- Physics Today
- Conference Proceedings
- Special Topics
pubs.aip.org
- Privacy Policy
- Terms of Use
Connect with AIP Publishing
This feature is available to subscribers only.
Sign In or Create an Account
Academia.edu no longer supports Internet Explorer.
To browse Academia.edu and the wider internet faster and more securely, please take a few seconds to upgrade your browser .
Enter the email address you signed up with and we'll email you a reset link.
- We're Hiring!
- Help Center
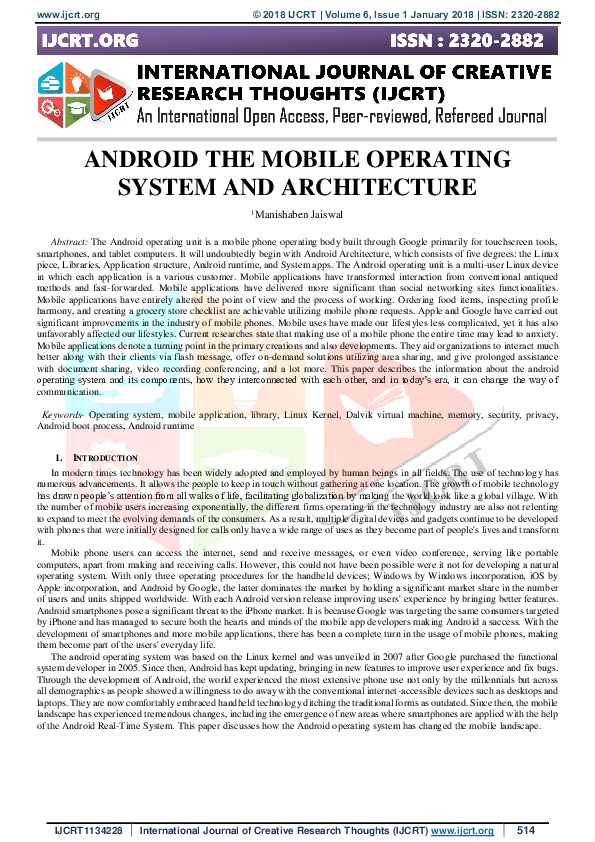
ANDROID THE MOBILE OPERATING SYSTEM AND ARCHITECTURE

2018, International Journal of Creative Research Thoughts (IJCRT), ISSN:2320-2882, Volume.6, Issue 1, pp.514-525, January 2018
The Android operating unit is a mobile phone operating body built through Google primarily for touchscreen tools, smartphones, and tablet computers. It will undoubtedly begin with Android Architecture, which consists of five degrees: the Linux piece, Libraries, Application structure, Android runtime, and System apps. The Android operating unit is a multiuser Linux device in which each application is a various customer. Mobile applications have transformed interaction from conventional antiqued methods and fast-forwarded. Mobile applications have delivered more significant than social networking sites functionalities. Mobile applications have entirely altered the point of view and the process of working. Ordering food items, inspecting profile harmony, and creating a grocery store checklist are achievable utilizing mobile phone requests. Apple and Google have carried out significant improvements in the industry of mobile phones. Mobile uses have made our lifestyles less complicated, yet it has also unfavorably affected our lifestyles. Current researches state that making use of a mobile phone the entire time may lead to anxiety. Mobile applications denote a turning point in the primary creations and also developments. They aid organizations to interact much better along with their clients via flash message, offer on-demand solutions utilizing area sharing, and give prolonged assistance with document sharing, video recording conferencing, and a lot more. This paper describes the information about the android operating system and its components, how they interconnected with each other, and in today's era, it can change the way of communication.
Related Papers
International Journal Of engineering and Computer Science (IJECS)
In the previous system, all the information has to view in a hard file, or in website [6]. At the same time while searching any information it is too difficult to access and takes a lot of time to search the particular website. Hence, in order to overcome this problem a smart phone based application using Android can be used to make this process easier, secure and less error prone. More efficient information's will be achieved through this system. Android is an open source Linux based system developed by Google, and primarily aimed at mobile handsets and other portable devices. In short, we will be using them to accomplish our daily task. One application that falls into this category is the Android Application for College. This College application provides a wide range of useful information which split into several functionalities. These include: academics, news, events, facilities and all the college details. Users can install this application in their android mobile to view all of these college details and make use it.To improve mobile experience for users[17] android project aims to create successful real world products. Android is a mobile OS but it's not limited to mobile only. It's currently used in smart phones and televisions. There are many code name of android such as Lollipop, Kitkat, Jelly Bean, Ice cream Sandwich, Froyo, Ecliar, Donut etc. In this paper we are also discussing aboutcomparison of androidLollipop version, its features, and its issues with android Marshmallow versions and also the development of Campsys mobile application.
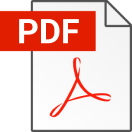
International Journal of Computer Applications
Sumit Sharma
belsti mulugeta
Journal of Computing Sciences in Colleges
Victor Manuel Fonseca Matos
2008 Third International Conference on Digital Information Management
sibte hassan
International Journal of Electronics, Mechanical and Mechatronics Engineering
Şenay Kocakoyun
International Journal of Advanced Computer Science and Applications
Cornelia Gyorodi
ht tp://developer. android. com/guide …
Awal Kharisma
Nasser Abosaq
Revolution of IT has changed the life style of people and their working habits. Also, it has totally changed the traffic patrons on the internet, due to excellent features of mobiles applications. The trend of using mobile devices has gone beyond limits and with every passing day the users are thinking to get solutions of their daily lives. That includes computing needs, liberty of communication and information sharing via Mobiles applications. This paper discusses the architecture of mobile applications, its major components and the role of mobile application in cutting edge technologies. Also, a comprehensive comparison of the performance of different platforms with respect to performance of mobile applications is presented.
Kevin Perdana
RELATED PAPERS
Gabriela Rosas Sepulveda
Gavrila Catalina
Microscopy and Microanalysis
Manuel Vieira
Kay Axhausen
Fernando Morante
Lisa van der Werff
Aini Aprilia
Dóra Révész
Scientific reports
Advances in Zoology and Botany
Swati Chaurasia
Journal of Alloys and Compounds
Professor Sadrnezhaad
Journal of Cellular and Molecular Medicine
Mustapha Najimi
Tạp chí Y học Việt Nam
Clinical Infectious Diseases
Nguyen Hoai Chinh
Antimicrobial Agents and Chemotherapy
Sanaa Botros
Marta Kotarba
Jurnal Kesehatan Kusuma Husada
Tresia Umarianti
Prof. Francesco INCHINGOLO
WSEAS transactions on signal processing
Seiichi Nakamori
Allergology International
Minako Tomiita
eric vanSonnenberg
TURKISH JOURNAL OF VETERINARY AND ANIMAL SCIENCES
Ayse Eser Elcin
Jose Coimbra
See More Documents Like This
RELATED TOPICS
- We're Hiring!
- Help Center
- Find new research papers in:
- Health Sciences
- Earth Sciences
- Cognitive Science
- Mathematics
- Computer Science
- Academia ©2024
Click through the PLOS taxonomy to find articles in your field.
For more information about PLOS Subject Areas, click here .
Loading metrics
Open Access
Peer-reviewed
Research Article
Users of the main smartphone operating systems (iOS, Android) differ only little in personality
* E-mail: [email protected]
Affiliations Department of Psychology, University of Konstanz, Konstanz, Germany, Department of Psychology, University of Cambridge, Cambridge, United Kingdom

Affiliation Department of Psychology, University of Konstanz, Konstanz, Germany
- Friedrich M. Götz,
- Stefan Stieger,
- Ulf-Dietrich Reips
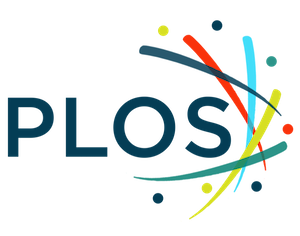
- Published: May 3, 2017
- https://doi.org/10.1371/journal.pone.0176921
- Reader Comments
The increasingly widespread use of mobile phone applications (apps) as research tools and cost-effective means of vast data collection raises new methodological challenges. In recent years, it has become a common practice for scientists to design apps that run only on a single operating system, thereby excluding large numbers of users who use a different operating system. However, empirical evidence investigating any selection biases that might result thereof is scarce. Henceforth, we conducted two studies drawing from a large multi-national (Study 1; N = 1,081) and a German-speaking sample (Study 2; N = 2,438). As such Study 1 compared iOS and Android users across an array of key personality traits (i.e., well-being, self-esteem, willingness to take risks, optimism, pessimism, Dark Triad, and the Big Five). Focusing on Big Five personality traits in a broader scope, in addition to smartphone users, Study 2 also examined users of the main computer operating systems (i.e., Mac OS, Windows). In both studies, very few significant differences were found, all of which were of small or even tiny effect size mostly disappearing after sociodemographics had been controlled for. Taken together, minor differences in personality seem to exist, but they are of small to negligible effect size (ranging from OR = 0.919 to 1.344 (Study 1), η p 2 = .005 to .036 (Study 2), respectively) and may reflect differences in sociodemographic composition, rather than operating system of smartphone users.
Citation: Götz FM, Stieger S, Reips U-D (2017) Users of the main smartphone operating systems (iOS, Android) differ only little in personality. PLoS ONE 12(5): e0176921. https://doi.org/10.1371/journal.pone.0176921
Editor: Susana Jiménez-Murcia, Hospital Universitari de Bellvitge, SPAIN
Received: October 13, 2016; Accepted: April 19, 2017; Published: May 3, 2017
Copyright: © 2017 Götz et al. This is an open access article distributed under the terms of the Creative Commons Attribution License , which permits unrestricted use, distribution, and reproduction in any medium, provided the original author and source are credited.
Data Availability: All relevant data files are available from the Open Science Framework database (URL: https://osf.io/2nmhu/ ).
Funding: The publication fee will be covered by the Open Access publication fund of the University of Konstanz ( https://www.kim.uni-konstanz.de/en/services/scholarly-publishing-and-open-access/open-access-publication-funds/ ). The funders had no role in study design, data collection and analysis, decision to publish, or preparation of the manuscript.
Competing interests: The authors declare that the research was conducted in the absence of any commercial or financial relationships that could be construed as a potential conflict of interest.
Introduction
Following the advent and proliferation of smartphones, app-based research has spread across the scientific landscape, ranging from fields as diverse as physics [ 1 ], tourism [ 2 , 3 ] and geology [ 4 , 5 ] to medicine [ 6 , 7 ]. Of note, it falls on especially fertile grounds in psychology, booming throughout the discipline, from biopsychology [ 8 ] to neuroscience [ 9 , 10 ] and personality research [ 11 , 12 , 13 ]. The reasons for this momentum are manifold:
Smartphone technology enables researchers to collect an abundance of data (high volume), that arrives as a continuous stream in real time (i.e., high velocity) from various, multifaceted sources i.e., high variety [ 14 , 15 ]. On top of experience sampling, i.e., the repeated, context-sensitive assessment of cognitive, affective, and behavioral measures across a certain period [ 16 ], modern smartphones may grant researchers access to global positioning system (GPS) based location data, communication logs, video and audio capture, motion sensing, and biosensors [ 14 , 15 , 17 ]. Linking these data to geographic information system data (e.g., climate and neighborhood characteristics) further allows scientists to paint an unprecedentedly fine-grained picture of people’s dynamic physical and social surroundings [ 18 , 19 ]. Leveraging this mechanism would facilitate to gain an in-depth understanding of the complex person × environment interactions that shape human behavior and might spur breakthroughs in personality and social psychology [ 19 ].
From a users’ perspective, science apps (i.e., mobile phone applications that serve scientific purposes as a research tool) are highly convenient as they do no longer require participants to be physically present in a lab [ 20 ] or, as in early Internet-based research, at a desktop or laptop computer. Increasingly often, both data collection and transmission happen automatically in the background, effectively reducing participant burden to a minimum [ 8 , 15 ].
Similarly, unlike their predecessors, personal digital assistants (PDAs), smartphones are already established as an integrated part of our lives [ 11 , 21 ]. Spending considerable amounts of time with smartphones has become a standout lifestyle feature [ 22 ], reflecting the contemporary philosophy of life in modern societies.
Given the remarkable growth rates of the smartphone market, the number of people carrying a smartphone is expected to skyrocket from 1 billion at the beginning of the decade [ 21 , 23 , 24 ], and 2 billion in 2016 [ 25 ] to over 5 billion by 2025 [ 17 ]. Against this backdrop, it is especially noteworthy, that smartphones have already begun to penetrate the emerging markets of developing countries and might soon become more common than computers [ 19 ]. In the absence of any available alternatives, in some regions smartphones may even constitute a monopoly-like structure, providing the only means to connect people to the Internet. In view of these tendencies, it appears encouraging, that data that has been submitted through mobile devices has been shown to be no less valid or reliable than data that was obtained from desktop users [ 26 ] or during laboratory experiments [ 23 ]. Marking the next leap towards a valid test of the universality of psychological theories [ 23 ], this trend thus has the capacity to pave the way for the inclusion of previously under-studied populations and ultimately, a wider coverage of cross-cultural research [ 20 ].
In a nutshell, smartphones are ubiquitous, fairly unobtrusive, remotely accessible, sensor-rich, and computationally powerful [ 17 , 22 ], thus setting the stage for the emerging field of Psychoinformatics at the crossroads of psychology and computer science [ 15 , 24 , 25 ]. Accordingly, smartphones may empower researchers to conduct large-scale longitudinal studies in real-world settings at low cost, featuring heterogeneous, global samples. Thanks to that, scientists may base future discoveries on an abundance of precise, and ecologically valid behavioral data above and beyond traditional self-reports [ 17 , 19 , 27 , 28 ]. Likewise, due to the remarkable computational powers, there are no apparent boundaries restricting the content of smartphone-based research and even highly complex cognitive tasks can be administered with ease [ 23 ]. Making use of these innovative possibilities would enable researchers to cross-validate, or challenge existing findings from lab settings [ 16 , 25 ] and extend the scientific body of knowledge beyond traditional Internet-based research that set out to achieve the same goals [ 29 – 31 ].
Thus, harnessing this untapped potential seems imperative. However, caution is warranted as some caveats (e.g., ethical and technical considerations with respect to data privacy and confidentiality, data transmission and storage solutions, security issues, app quality, and safety) prevail that need to be addressed for the sake of sound and adequate research practices [ 14 , 15 , 17 , 25 , 27 , 32 ]. In view of the constantly growing plethora of apps, guidance is needed to identify trustworthy, proper science apps. On a more methodological note, concerns have been voiced regarding potential technology-induced selection biases e.g., [ 33 ]. By definition, smartphone research is limited to the population of smartphone owners, creating coverage issue [ 34 ]. Yet, this might be less problematic, given the afore-mentioned rapidly expanding distribution of smartphones worldwide that will soon rise to a level of almost complete coverage.
Moreover, even though Lane and Manner [ 35 ] did find that smartphone ownership was predicted by extraversion, the authors have argued themselves that personality is, overall, a rather weak predictor of smartphone ownership. Hence, we tentatively conclude that it appears appropriate to assume that coverage issues, i.e. differences between smartphone owners and non-smartphone owners do not undermine the generalizability of app-based research to a worrying extent. Nevertheless, this does not rule out other systematic biases within the population of smartphone users.
Conducting a thorough analysis of the major smartphone operating systems (OS) in terms of their suitability as a research tool (i.e., Android, iOS, BlackBerry, Symbian, and Windows Mobile), Oliver [ 36 ] concluded that while every platform has its pros and cons, none of them is ideal or even generally superior to its competitors. As a solution, researchers could develop several native science apps in the respective programming languages or come up with web-based hybrid apps by means of cross-platform development tools (CPDTs). Alternatively, if social scientists do not see themselves fit for programming, interdisciplinary collaborations with trained computer scientists may prove effective [ 27 ].
However, while studies that accommodate both major systems (i.e., Android and iOS) do exist [ 37 , 38 ], they are much more of the exception rather than the general rule. In contrast, it is fairly common for psychological app-based studies to be run solely on either Android [ 39 – 42 ], iOS [ 43 – 46 ] or a different OS [ 13 , 47 ].
Perhaps puzzling at first, this pattern can be explained as follows: Programming multiple apps, one for each system is a tedious and time-consuming process that requires, above all, sufficient knowledge and skills in at least two programming languages, which makes it less desirable. In the meantime, CPDTs are still developing, often failing to live up to the performance of their native counterparts [ 48 , 49 ]. To make things worse, Miller [ 17 ] has pointed out that there is currently only a small minority of psychologists, sufficiently tech-savvy and advanced in computer science to program apps efficiently by themselves. While it is hence understandable, that researchers shy away from coming up with science apps that accommodate both systems, it might jeopardize the data’s generalizability if user personality is related to smartphone operating systems as much as it is related to computer operating systems [ 33 ].
In other words, if iOS and Android users were to differ systematically regarding fundamental psychological characteristics, results of smartphone app studies would be inherently biased and per se compromised in their external validity. This would be a particularly harsh setback for the burgeoning field of personality research in Psychoinformatics [ 11 , 12 , 13 ], whose results would become questionable at best. As, to our knowledge, no study has examined this possibility so far, we aim to compare iOS and Android users along an array of personality traits.
To that end, we come up with two studies that complement each other.
More precisely, Study 1 employs a holistic personality assessment to screen for potential differences in various diverse traits across a large multi-national sample. Beyond the Big Five personality traits [ 50 ] at the core, it seeks to capture other facets of user personality that tap into different aspects and may therefore add incremental value and explanatory power. As such it draws from positive psychology by collecting data on well-being [ 42 ], global self-esteem [ 51 ] and optimism [ 52 ]. In juxtaposition, it also turns to more sinister traits, namely risk proneness [ 53 ], pessimism [ 52 ] and the Dark Triad (i.e., narcissism, psychopathy, and Machiavellianism; [ 54 ]).
Building on that, Study 2 aims to consolidate those findings and further extend the scope of our research to the computer realm, drawing from an even bigger, German-speaking sample and takes not only iOS and Android, but also Windows and Mac OS users into account (throughout the remainder of this article, Mac OS refers to the computer division of Apple, i.e. Mac operating systems that run on iMacs and MacBooks.). In recognition of the pre-eminent position of the Big Five taxonomy, as the predominant personality framework in mobile phone and Internet studies [ 12 , 55 ], and in the absence of notable effects for the other personality traits in Study 1, Study 2 is deliberately restricted to the Big Five [ 50 ]. This approach limits error due to multiple testing issues [ 12 ] and together with the enhanced statistical power, arising from the large sample, allows for even more rigorous testing. Study 2 improves further on Study 1 in assessing participants’ OS non-reactively, i.e., automatically upon accessing the questionnaire, thereby avoiding self-reports, which are prone to evoke biases. Given their overlapping, yet complementary design, we believe that if the results of Study 1 and Study 2 converge, one could claim with some confidence that indeed, personality differences between users of different operating systems do–or do not exist.
Research questions and hypotheses
In marketing research and consumer psychology, brands are believed to have a personality, featuring a unique set of characteristics usually attributed to humans [ 56 ]. Henceforth, attitudes towards specific brands can be formed on the basis of these personality traits. Accordingly, these attitudes may serve the purpose of allowing consumers to express their self-concepts through the purchase, use and ownership of particular brands [ 57 ].
Reflecting its rather unique firm philosophy and marketing strategy, the Apple brand personality was built to convey qualities such as nonconformity, innovation, and creativity [ 58 ]. Unlike PC in the computer domain, or Samsung, SONY, and Nokia in the smartphone sector, Apple has successfully managed to become a lifestyle brand, echoing a modern, youthful philosophy of life that rests on the pillars of freedom, imagination, and simplicity at the heart of a seemingly truly humanistic, caring company. Lending empirical support to these observations, research has shown that whereas consumers describe Apple as exciting, SONY is rather seen as competent and sincere [ 59 ].
Moreover, the iPhone has become a status symbol for some people, inducing a feeling of belonging to a societal avant-garde in those who carry it [ 60 ]. Contrarily, consistent with its strategy to target the mass market, Samsung has cultivated a fairly different brand personality, emphasizing values such as ruggedness and functionality [ 60 ]. Summed up, on the one hand Apple stands for an outgoing, adventurous and lively brand personality, on the other hand it gives rise to an elitist self-definition of its customers, who may seek social approval and boost their self-esteem by being identified with Apple products. Meanwhile, Android brands (e.g., Samsung, SONY) appear to promote a more down-to-earth approach, grounded in a reliable, but significantly less fancy and glamorous product assortment. While we do not want to give in to mere speculations, drawing from the presented findings, we formulated the following hypotheses:
Hypothesis 1 (Study 1).
On average , iOS users will score higher on global self-esteem than Android users , reflecting the widespread belief that whereas the iPhone is a status symbol that carries prestige, fashionability, and exclusivity, all of which are suitable to make one feel valued and special, thus promoting enhanced self-esteem, Android smartphones fail to exert this same power.
Hypothesis 2 (Study 1, Study 2).
On average , iOS users will show higher Extraversion than Android users . Owing to Apple’s brand image as young, daring, outgoing and creative–an array of personality characteristics that seems to be rather closely linked to an extraverted personality, enhanced extraversion can be expected in accordance with the notion, that brand personality is supposed to mirror one’s own personality.
As neither the existing literature, nor common sense would allow similarly specific predictions, we refrained from formulating additional hypotheses for the other variables. Nonetheless, we believe that the inclusion of these constructs is conducive to the overall aim of the present research which is to detect any noteworthy personality differences as a function of users’ OS. Henceforth we tried to accomplish the most extensive coverage of user personality given existing constraints (e.g., questionnaire length) and adopted an exploratory approach in the search of potential differences. Likewise we investigated whether participants’ language (i.e., English or German) moderated the observed links, without holding any directional expectations.
Because there is very litte research on the topic of personality differences regarding used operating systems, we assumed a low effect size ( d = 0.2 and η p 2 = 0.01 according to [ 61 ]). A power analysis (α = 5%, power = 80%, two-tailed) recommends a minimal sample size of N = 788 for Study 1 and N = 1,096 for Study 2.
Materials and methods–study 1
Participants.
The sample was comprised of 1,081 participants, 624 (58%) of whom reported to be female, while 449 (41%) reported to be male, and 8 (1%) who did not disclose their sex. Reported age ranged from 18 to 94 years ( M = 24.5, SD = 8.1). Recruitment ensued online on various national and international platforms (e.g., Facebook, reddit), as well as on campus at the University of Konstanz, Germany, by word-of-mouth and custom-tailored advertisement of the study in introductory psychology lectures. Following this twofold strategy, the obtained sample comprised 507 participants (46.9%) from German-speaking countries (Germany: 44.8%, Switzerland: 1.2%, Austria: 0.9%) and 574 participants (53.1%) who were either from English-speaking countries or mastered English fluently (USA: 25.5%, Australia: 3.9%, UK: 2.5%, Canada: 2.3%).
Reported monthly budget ranged from less than 250€ to 5,001€ or more, with 76% of the sample disposing of 2,000€ or less per month, while 11% chose not to reveal their monthly budget. Regarding OS usage, 573 participants (53.0%) identified themselves as Android users, 444 participants (41.1%) indicated they use an iPhone. Meanwhile a small proportion indicated that they use either a Windows Phone (3.3%), a completely different operating system (1.1%), or no smartphone at all (1.3%). For parsimony’s sake only users of the two main OS (i.e., Android and iOS) were considered for further analysis resulting in a final sample size of 1,017.
Furthermore, the participant pool was mostly made up of college students (65.6%), active members of the workforce (31.7%), and high school students (8.8%), while others were unemployed (2.7%) or did not disclose their occupation (2.0%). (Please note that the accumulated percentages may exceed 100 percent, as participants could indicate multiple occupations, e.g. being a college student while working full-time.) The majority of the sample reported to be single (48.3%) or currently engaged in a romantic relationship (39.5%), while small fractions were married (7.3%), divorced (0.6%), or widowed (0.1%), or did not report their present marital status (2.1%).
Questionnaire length in electronically distributed online surveys deserves special attention, as the same content may appear longer on Web sites, stretching across several pages, as opposed to traditional paper-and-pencil questionnaires [ 62 ], also see [ 63 ], for the one-item-one-screen design. Furthermore, dropout decisions are based on study attributes, such as survey length [ 64 ] or incompatibilities of technology used [ 65 ]. Similarly, previous research has shown that dropout risk rose by 40% from a 10-minute questionnaire to a 30-minute questionnaire [ 66 ]. Motivated by those findings, we deliberately decided to limit the online questionnaire to a restricted number of items that would take no longer than 15 minutes to complete, in order to decrease participant burden and, in turn, foster participation. Henceforth, aside from a small battery of demographic questions, we aimed to employ short, yet effective measures that are well-suited for group-level analysis [ 67 , 68 ] and possess satisfying psychometric properties [ 67 ].
In line with this rationale, we assessed Big Five personality traits with the Mini-IPIP [ 50 ], which contains 20 items and has repeatedly been shown to have acceptable reliability estimates [ 69 , 70 ].
Moreover, we chose to gauge global self-esteem by means of the Single-Item Self-Esteem Scale (SISE) that has been successfully translated into other languages before [ 71 ] and demonstrated to be of satisfactory validity [ 51 ]. Similarly, we employed a single-item measure of well-being, which has been a robust indicator in previous research with German-speaking samples [ 42 ].
Furthermore, we chose the Dirty Dozen [ 54 ] as a representative of negative personality attributes, consisting of 12 items, which have been shown to be an efficient, psychometrically acceptable measure of the Dark Triad [ 72 ].
Apart from that, we employed some short scales, which originate from the Leibniz Institute for the Social Sciences (GESIS) and have been validated on large, stratified samples, to measure the following constructs: risk proneness (1 item), [ 53 ], optimism and pessimism (2 items), [ 52 ]. In addition, we also assessed social desirability (6 items; 2 subscales), [ 73 ].
However, both subscales yielded unacceptably low reliability estimates (NQminus α = .564, PQplus α = .495) and were henceforth not considered for any further statistical analysis. Aside from the said GESIS measures (i.e., optimism, pessimism, risk proneness), which have been validated extensively on large, stratified German samples, it was ensured that the German versions of our instruments had been translated by professionals and repeatedly used in previous studies so that their appropriateness and precision could be taken for granted (see [ 74 ] for Mini-IPIP, see [ 42 ] for well-being). The only exceptions were the Dirty Dozen and the SISE. In the absence of established German versions, the scales were translated from the original English using the parallel-blind technique [ 75 ].
The survey was designed for optimization on regular computers and smartphones, using the SoSci Survey online tool ( https://www.soscisurvey.de/ ). The questionnaire was administered online and available for a period of 3 months in English and German. As a general rule, participation was unpaid and voluntary, without further incentives, such as personalized feedback from the questionnaire.
However, psychology students, enrolled at the University of Konstanz, were offered course credit for participation. Beyond that, upon inclusion in the sample, participants automatically entered a lottery, raffling off Amazon gift card vouchers of a value of 100€ in total, unless they specifically requested otherwise.
The present study was conducted in accordance with the Ethical Guidelines of the German Psychological Society (DGPs) and the Ethical Guidelines of the Department of Psychology, University of Konstanz. Formal ethics approvals for this type of research (i.e., noninvasive, not affecting the physical or psychological integrity, the right for privacy or other personal rights of interest) are required neither by these guidelines nor by German laws.
All participants consented to the terms of the study, which were outlined in detail, preceding the actual questionnaire. As such, providing informed consent was made a prerequisite to proceed to the main part of the survey. Participants were explicitly told that they could revoke their consent and withdraw from the study at any time without any personal disadvantages arising from it. Furthermore, anonymity was ensured and no harmful procedures were applied. The same precautions and ethical standards were also upheld throughout Study 2.
Statistical analysis
Following a twofold analysis procedure, we initially checked for potential differences in demographic variables, between self-reported iOS and Android users. Hereafter, we employed inferential statistics to account for possible distinctions with respect to the available personality measurements, beyond the influences of sociodemographic variables. Thereby we conducted both, confirmatory and exploratory analyses.
Results–study 1
Demographics.
At first, we ran a series of χ 2 -tests to investigate the sample’s demographic composition. In this context, we did neither detect any significant differences for marital status (χ 2 = 4.18, df = 4, p = .38), nor for participant’s sex (χ 2 = 1.03, df = 1, p = .31). Similarly, a t -test failed to unveil any significant differences in reported age between iOS users ( M = 24.23, SD = 8.10) and Android users ( M = 24.40, SD = 7.63), t = -0.358, p = .72. However, significant differences although of very low effect size emerged in terms of the distribution of participants’ monthly budget, with iOS users tending to have access to somewhat larger financial resources (χ 2 = 22.75, df = 9, p = .007; r sp = .07).
Personality traits
Given the multitude of variables and the risk of type I error that would have resulted from multiple testing, when conducting individual ANOVAs for every trait, we decided to run a binary logistic regression model instead, whereby smartphone OS (i.e., iOS vs. Android) was predicted by well-being, SISE, risk proneness, optimism, pessimism, the Dark Triad, the Big Five as well as sex, age and monthly budget. To that end, we employed a hierarchical analysis approach, featuring three stages and thus a step-wise increase in our model’s complexity.
First, we entered the sociodemographic variables (i.e., sex, age, and monthly budget) alone to predict users’ OS. Second, we entered both the sociodemographic variables and the personality traits (i.e., well-being, SISE, risk proneness, optimism, pessimism, Dark Triad, Big Five) to see whether this would lead to a significant improvement of the model’s fit to the data above and beyond the predictive power of sociodemographic factors. Third, in order to consider moderating effects that might arise from differences grounded in language or culture, we decided to rerun the full model (step 2) independently for the English-speaking and German-speaking subsamples. Please note, that separate ANOVAs (respectively ANCOVAs when controlling for sociodemographic variables) provide a more fine-grained picture and allow to tease out personality traits’ individual contributions. However, the results remain largely unchanged and the little effects that emerge mostly disappear when controlling for age, sex, and monthly budget. Additional ANOVA-based analyses are available in an online supplement (see S1 Table ).
Overall, the data demonstrated that differences between iOS and Android users were largely absent. While Model 1 was significant and accounted for 1.6% of variance (Nagelkerke R 2 = .016) entering the personality constructs in a second step significantly improved the predictive power of the model (step: χ 2 = 23.700, df = 13, p = .034), with the proportion of explained variance rising to 5% (Nagelkerke R 2 = .050) (see Table 1 , column 1 and 2). Moreover, of all variables only two emerged as statistically significant predictors of user OS. Higher monthly budget predicted a higher likelihood of using iOS ( OR = 0.922), whereas Openness to Experience was related to an increased probability of using Android ( OR = 1.343). However, in both cases Odds Ratios gravitated towards 1.0, indicating a weak relationship and were far off common thresholds of a strong effect (e.g., 3.0 for positive associations, [ 75 ]).
- PPT PowerPoint slide
- PNG larger image
- TIFF original image
https://doi.org/10.1371/journal.pone.0176921.t001
After the language-based split of the sample was performed to unpack potential cultural differences, almost identical patterns were observed in the English subsample (see Model 2a, Table 1 , column 3). In contrast, the model for the German sample could not reliably predict smartphone OS above chance level and dropped below the threshold of statistical significance. Accordingly, no single predictor reached statistical significance. Of note, however, both Neuroticism ( b = -0.28, p = .066, OR = 0.750, 95% CI: 0.551, 1.019) and Openness to Experience ( b = 0.305, p = .069, OR = 1.357, 95% CI: 0.976, 1.885) approached statistical significance, with the latter mirroring the effect that was observed in the other models (see Model 2b, Table 1 , column 4).
In the absence of any significant differences between iOS- and Android users in self-esteem (H1) or Extraversion (H2), none of our hypotheses received empirical support, although Extraversion did approach statistical significance ( b = -0.236, p = .081, OR = 0.790, 95% CI: 0.612, 1.019) in the English subsample, showing a trend in the hypothesized direction.
In sum, our data suggest that iOS- and Android users show only minimal differences regarding psychological concepts. If anything, Android users tend to be a little more open, while iOS users may be slightly wealthier. Yet, all effect sizes were small to tiny. While Table 2 provides a summary of the measures’ descriptive statistics, detailed results of the logistic regression model are displayed in Table 1 .
https://doi.org/10.1371/journal.pone.0176921.t002
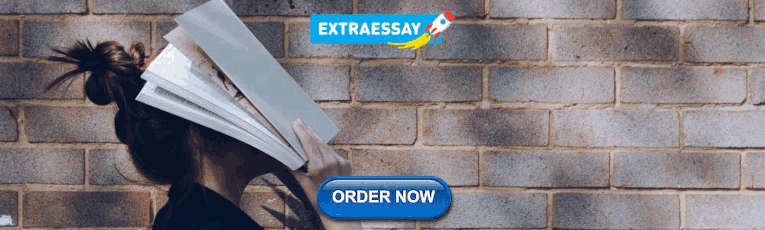
Materials and methods–study 2
Our second sample differed from the first sample insofar, as it was larger and more homogeneous with respect to its cultural composition. Several research assistants sent the link to the online questionnaire to their friends, relatives, and acquaintances by using various online channels (e.g., Facebook, Email). This snowball sampling procedure led to a community-based convenience sample of German-speaking participants, which was effectively reduced to 2,036 German-speaking participants due to the following reasons:
First, we excluded data sets from 26 participants who had exhibited suspicious responses that raised doubts about their seriousness in answering the questionnaire (always giving the same highly implausible answer to the delinquency questions, e.g., ‘99’). Second, as the study did specifically aim to compare Android-, iOS-, Windows, and Mac OS users, 145 participants relying on other operating systems were not considered for further analysis. In the final sample, 1,345 participants (66.1%) reported to be female and 685 participants (33.6%) reported to be male, 6 participants chose not to disclose their sex (0.3%). Age ranged from 18 to 78 years ( M = 25.5, SD = 11.64). Furthermore, the vast majority (79.3%) had at least graduated from high school.
In line with the approach adopted in Study 1 we chose to design the online questionnaire in a fashion that would allow participants to complete it in no more than 15 minutes for the sake of enhanced retention rates and increased data quality. Paralleling the procedure of Study 1, we relied on the Mini-IPIP [ 50 ] to measure Big Five personality traits. Due to a technical failure in the online questionnaire, one item of the Conscientiousness subscale was asked twice and one item was not asked. Therefore, the mean score of the Conscientiousness subscale is only based on three instead of four items. On top of this we also used a short battery of questions revolving around delinquency, which were part of a different research project and are henceforth not touched upon in the scope of this article.
The questionnaire was developed in accordance with standards for optimal depiction on both, regular computers and smartphones, using the SoSci Survey online tool ( https://www.soscisurvey.de ). As such, it was exclusively accessible online for a period of two months, and was in German only. In the absence of any financial or otherwise incentives, participation was per se unpaid and voluntary. The same ethical precautions and procedures were applied as in Study 1.
Results–study 2
We followed a similar analysis procedure as outlined in Study 1. However, unlike Study 1, Study 2 included smartphone and computer users alike, resulting in four groups that were compared with each other (i.e., Mac OS, Windows, iOS, Android). As this design would have required running a multinomial regression analysis, with three different models (changing the reference group to determine pairwise group differences) per column, we chose to compute ANOVAs and ANCOVAs instead, which were deemed more parsimonious and easily comprehensible in the given context. Moreover, compared to Study 1, adopting this method bore a considerably smaller risk of suffering from multiple testing issues due to the reduced set of variables.
First, we carried out χ 2 -tests to account for the distribution of primary demographic attributes across the OS groups. Results yielded significant results for sex (χ 2 = 27.44, df = 3, p < .001, φ = .116) and educational level (χ 2 = 63.54, df = 18, p < .001, φ = .177), reflecting a deviation from a balanced distribution between the respective OS groups. Distribution of participants’ sex was rather balanced among Android users (standardized residuals -0.2 for women and 0.3 for men), slightly more male-dominated among Windows users (standardized residuals -0.7 and 1.0, respectively), clearly male-dominated among Mac OS users (standardized residuals -2.0 and 2.9, respectively), and clearly female-dominated among iOS users (standardized residuals 2.1 and -3.0, respectively). With respect to educational level, computer users were more likely to have graduated from university than users using smartphones (standardized residuals: Mac OS, college degree: 2.1, Windows, college degree: 4.1, iOS, college degree: -1.8, Android, college degree: -3.1).
In line with this finding, an ANOVA yielded significant age differences ( F = 51.88, df = 3, p < .001, η p 2 = .071), with Scheffé post-hoc tests indicating that Windows users ( M = 30.1, SD = 15.4) were on average significantly older than Mac OS users ( M = 27.2, SD = 12.2) who were, in turn, on average older than Android users ( M = 23.3, SD = 8.8), and iOS users ( M = 23.0, SD = 8.5). Given this pattern we would like to suggest that the differences in educational level might actually stem from age differences, suggesting that smartphone users in our sample may not yet have graduated from college despite proactively pursuing a higher education.
In order to give a general overview, Table 3 exhibits descriptive parameters. The inferential analysis was carried out in two stages. At first, we ran ANOVAs on Big Five personality traits ( Table 4 , 1st and 2nd column). Thereafter, we conducted an ANCOVA to control for potential moderating effects of age, participant sex, and educational level ( Table 4 , 3rd and 4th column). Finally, we computed pairwise mean-differences (Bonferroni corrected) to pinpoint the concrete nature and direction of effects between the existing subgroups ( Table 4 , 5th column).
https://doi.org/10.1371/journal.pone.0176921.t003
https://doi.org/10.1371/journal.pone.0176921.t004
In resemblance with the patterns from Study 1, no significant differences between OS groups were detected, except for the Big Five personality traits Extraversion (η p 2 = .036) and Openness to Experience (η p 2 = .010), both of which were of rather small effect size (small: η p 2 = .01, medium: η p 2 = .06, large: η p 2 = .14, 76). Of note, the difference for Neuroticism also reached statistical significance, although with a tiny effect size of η p 2 = .005. However, the observed differences for Neuroticism and Openness to Experience vanished once age, sex, and educational level were controlled for ( Table 4 ). Solely the reported differences for Extraversion (η p 2 = .007) prevailed, even after accounting for the afore-mentioned moderators—although with a sharp drop in effect size.
Windows users displayed the lowest values on Extraversion, differing significantly from iOS- and Android users, both of which exhibited more extraversion. With respect to Hypothesis 2, this outcome fails to elicit significant differences between iOS- and Android users, and, like the Study 1 does not lend support to the hypothesis. However, it should be noted that the hypothesized differences were found before controlling for sociodemographic variables. All analyses are summarized in Table 4 .
In a nutshell, very much alike Study 1, we demonstrated that in spite of a few significant differences between the users of the most prominent operating systems in key psychological concepts, namely Big Five personality traits, those differences are of small to tiny effect size. In our present studies, controlling statistically for age, sex, and educational level led to an almost complete disappearance of said effects.
General discussion
Today, the rise of smartphones is already transforming our lives and will most likely continue to do so in the next years to come, as mobile technology becomes more and more ubiquitous all around the world. The new technology now impacts on various domains of our lives, yielding manifold consequences that echo throughout society. In the future this trend may be further amplified as everyday objects, e.g., fridges and cars will harbor remarkable computational powers and constant Internet connectivity, giving rise to the Internet of Things (IoT) [ 15 , 25 ].
While it is widely anticipated that social scientific research will benefit from leveraging the enormous potential of these technologies, a number of methodological, technical, ethical, and practical hurdles peculiar to smartphone-based research prevail, which need to be dealt with, first (e.g., data privacy and confidentiality, data transmission and storage solutions, security issues, app quality, and safety; [ 14 , 15 , 17 , 27 , 32 ]).
Raising and addressing another issue linked to science apps, the present studies aimed to provide a clue as to whether researchers would need to accommodate both predominant smartphone operating systems (i.e., iOS and Android), in order not to jeopardize the generalizability of their findings.
For Study 1 a step-wise analysis procedure did yield a significant impact of Openness to Experience besides differences in sociodemographic variables. At first glance, this might pose a threat to the generalizability mentioned above. However, it is important to note, that all observed effects were of small or even tiny effect size in accordance with common classifications (e.g., [ 76 ]). Likewise, both our hypotheses, assuming differences in self-esteem and Extraversion, respectively, could not be confirmed and were henceforth rejected.
Bearing this in mind, it appears legitimate to assume that in spite of minor differences between iOS and Android users, none of the found differences are sufficiently strong to be of actual practical relevance. However, this impression may be misleading. On the contrary, we would like to stress that whereas it is relatively easy to statistically eliminate the influence of sociodemographic variables, it is by far less so when it comes to gathering actual samples via certain technologies. Replicating the classic study by Buchanan and Reips [ 33 ] the present results hint that in the given context, sociodemographic factors are a force to be reckoned with that exerts a sizable impact on the studied effects. This is reflected in the fact, that the only other significant predictor of smartphone OS, aside from Openness to Experience in Study 1 was monthly budget. In a similar vein, the observation, that most effects in Study 2 vanished after sociodemographic variables were controlled for, attests to the same possibility. Unless being accounted for by matched samples, by nature, the distribution of such sociodemographic variables may vary profoundly between operating systems. In conclusion, to avoid undue biases threatening the data’s validity, great care should be taken in terms of sample composition in science app studies, especially when recruiting ad-hoc samples.
Strengths and limitations
In spite of our efforts to conduct the present research in the most beneficial and effective way, some drawbacks persisted nonetheless, which we intend to address in the following section. To start with, both studies used ad-hoc samples with very little recruitment restrictions. Although these community-based samples are more diverse in sample characteristics than common student samples and do henceforth generate a higher usability of the resulting data [ 77 ], some disadvantages need to be considered.
Notably, as a direct consequence, arising from our recruitment strategy, we faced a skewed sex distribution in the sample of Study 2, with roughly two thirds of the sample being women. This might sound worrying, because Big Five personality traits have been shown to vary as a function of sex, especially in well-developed wealthy and egalitarian societies just like Germany [ 78 ]. Both samples featured a rather wide range in terms of age, which is of interest as Big Five personality traits have also been reported to change dynamically across the lifespan [ 79 ]. This being said, one might turn this heterogeneity into an asset, as it reflects the actual age composition of the target population better than traditional psychological studies that are notoriously prone to draw from college student samples only [ 30 ]. As age was not even close to being a significant predictor of smartphone OS in Study 1, we are confident that the age distribution was fairly comparable between iOS and Android users and did not impair the results’ validity. Nonetheless, in keeping with the findings above on the link between sociodemographic variables and Big Five personality traits, we controlled statistically for sex, age, and educational level in Study 2. Of note, this had a strong influence on the obtained results that merits further attention.
From a methodological point of view, Study 2 may receive the critique that most people tend to own and use both, a smartphone and a computer system. Consequently their placement in the respective compared groups could be perceived as reflecting an arbitrary snapshot rather than a clear-cut, permanent membership in one particular user group (i.e., continuously favoring the usage of one electronic device over the other). Taking on this potential caveat, we analyzed switching patterns between smartphones and computers, drawing from a sizable longitudinal sample ( n = 204) with an average of 48 data points per person, accumulating to a total 9,745 data points that has been collected in the frame of a different research project [ 80 ]. Consistent with our claim, results indicated that 92% of all participants kept using the same device in at least 80% of all data collection waves. Against this backdrop, it appears reasonable that a pronounced preference for a single electronic device exists in most people which allows to sort participants into the user groups that we employed throughout Study 2.
Furthermore, although we have mounted our best efforts to ascertain a holistic and balanced assessment of user personality, with a strong emphasis on the Big Five taxonomy, acknowledging its role as a key concept in smartphone-based personality research [ 11 , 12 ], we cannot rule out the possibility that we have failed to detect significant differences across users of different OS along unmeasured personality dimensions. While we tried to minimize this danger by assessing a host of vastly different characteristics to cover as much of users’ personality as possible, some traits may have fallen through the cracks, such as Gray’s reward sensitivity or, similarly, social desirability, which we did measure but could not analyze due to a lack of reliability. Faced with a length-breadth tradeoff, when designing our questionnaire, we chose to pursue a holistic, yet parsimonious approach to maintain participant motivation, reduce fatigue, boredom and dropout and yield high-quality data [ 64 , 66 , 67 ]. However, future research should expand on our findings and consider other personality traits.
While it is very clear, that our research leaves some room for improvement, it benefits from an array of assets that deserve to be mentioned. To start with, we would like to stress that thanks to its design, Study 2 can be interpreted as an in-built replication of Study 1, although with a somewhat narrower focus, concentrating on Big Five personality traits in a German-speaking sample. Beyond that, it makes two valuable contributions in extension of Study 1. Notably, we assessed OS, the grouping variable in question automatically, unlike Study 1 where we relied on self-reports. Moreover, it widens the horizon of the study, by taking desktop computer OS into account as well.
Due to the novelty of smartphones in general and science apps in particular, a refined research philosophy as well as best practices to accommodate their use as data collection tools are currently still lacking. In recognition of the arising challenges, the present investigation represents an attempt to mark another step towards a robust, unified methodology for smartphone- and computer-based social science studies. Such studies provide an easy, yet cost-effective way of collecting vast amounts of ecologically valid data from diverse, geographically widely scattered samples. Events can be recorded in real time, as they occur.
Still, special care has to be taken, when employing smartphones and science apps, as an inadequate manner of using them for research purposes, may both, undermine data quality and compromise ethical standards. Against this backdrop, we aimed to shed new light on a potentially harmful selection bias that emerges following the widespread use of science apps that are compatible with one OS only. We argued, that if iOS and Android users were to differ significantly in personality, as marketing research and consumer psychology hint, the scientific community would need to introduce hybrid apps, or independently designed identical native apps for both systems, as a gold standard for app-research, for external validity’s sake. Thankfully for less tech-savvy scholars, according to our findings, this effort is not to be considered a necessity, in spite of potentially distorting differences in sociodemographic composition that researchers should be aware of. More to the point, minor differences in personality do exist, but they are of negligible effect size.
Supporting information
S1 table. pairwise comparisons of personality traits between ios and android users (study 1)..
Note . Bold values indicate significance ( p < .05). small: η p 2 = .010, medium: η p 2 = .060, large: η p 2 = .140; Cohen (1988). I…iOS, A…Android.
https://doi.org/10.1371/journal.pone.0176921.s001
Author Contributions
- Conceptualization: FMG SS.
- Data curation: FMG SS.
- Formal analysis: FMG SS.
- Funding acquisition: SS UDR.
- Investigation: FMG SS.
- Methodology: FMG SS.
- Project administration: FMG SS UDR.
- Resources: UDR.
- Software: FMG SS.
- Supervision: SS UDR.
- Validation: FMG SS UDR.
- Visualization: FMG SS.
- Writing – original draft: FMG.
- Writing – review & editing: FMG SS UDR.
- View Article
- Google Scholar
- PubMed/NCBI
- 18. Reips U-D. Schöne neue Forschungswelt: Zukunftstrends [Beautiful new world of research: Future trends]. In: König C, Stahl M, Wiegand E, editors. Nicht-reaktive Erhebungsverfahren. Bonn: GESIS Schriftenreihe, Band 1; 2009. pp. 129–138.
- 29. Birnbaum M H. Psychological experiments on the Internet. San Diego, CA: Academic Press; 2000.
- 30. Reips U-D. Das psychologische Experimentieren im Internet [Psychological experimenting on the Internet]. In: Batinic B, editor. Internet für Psychologen. Göttingen: Hogrefe; 1997. pp. 245–265.
- 31. Reips U-D. The web experiment method: Advantages, disadvantages, and solutions. In: Birnbaum M H, editor. Psychological experiments on the Internet. San Diego, CA: Academic Press; 2000. pp. 89–118.
- 33. Buchanan T, Reips, U-D. Platform-dependent biases in Online Research: Do Mac users really think different? In: Jonas KJ, Breuer P, Schauenburg B, Boos M, editors. Perspectives on Internet Research: Concepts and Methods. 2001. Available from: http://www.uni-konstanz.de/iscience/reips/pubs/papers/Buchanan_Reips2001.pdf
- 41. Staiano J, Lepri B, Aharony N, Pianesi F, Sebe N, Pentland A. Friends don’t lie: Inferring personality traits from social network structure. Proceedings of the 2012 ACM Conference on Ubiquitous Computing. 2012;321–330.
- 47. Rachuri KK, Musolesi M, Mascolo C, Rentfrow PJ, Longworth C, Aucinas A. EmotionSense: A mobile phones based adaptive platform for experimental social psychology research. Proceedings of the 12th ACM international conference on Ubiquitous computing. 2010; 281–290.
- 48. Dalmasso I, Datta SK, Bonnet C, Nikaein N. Survey comparison and evaluation of cross platform mobile application development tools. 9th International Wireless Communications and Mobile Computing Conference (IWCMC). 2013; 323–328.
- 53. Beierlein C, Kovaleva A, Kemper CJ, Rammstedt B. Eine Single-Item-Skala zur Erfassung von Risikobereitschaft: Die Kurzskala Risikobereitschaft-1 (R-1) [A single item scale for the measurement of risk proneness: The short scale Risk Proneness-1 (R-1)] (GESIS Working Papers 2014|34). Köln: GESIS; 2014. Available from: http://www.gesis.org/fileadmin/kurzskalen/working_papers/R1_WorkingPapers_2014-34.pdf
- 61. Cohen J. Statistical power analysis for the behavioral sciences. 2nd ed. Hillsdale, NJ: Erlbaum; 1988.
- 63. Reips U-D. Design and formatting in Internet-based research. In: Gosling S, Johnson J, editors. Advanced methods for conducting online behavioral research. Washington, DC: American Psychological Association; 2011. pp. 29–43.
- 65. Schwarz S, Reips U-D. CGI versus JavaScript: A Web experiment on the reversed hindsight bias. In: Reips U-D, Bosnjak M, editors. Dimensions of Internet Science. Lengerich: Pabst; 2001. pp. 75–90.
- 73. Kemper CJ, Beierlein C, Bensch D, Kovaleva A, Rammstedt B. Eine Kurzskala zur Erfassung des Gamma-Faktors sozial erwünschten Antwortverhaltens: Die Kurzskala Soziale Erwünschtheit-Gamma (KSE-G) [A short scale for assessing the gamma-factor of social desirable response behavior: The short scale Social Desirability-Gamma (KSE-G)]. (GESIS Working Papers 2012|25). Köln: GESIS; 2012. Available: http://www.gesis.org/fileadmin/kurzskalen/working_papers/KSE_G_Workingpaper.pdf
- 75. Behling O, Law KS. Translating questionnaires and other research instruments: Problems and solutions. 1st ed. Thousand Oaks, CA: Sage; 2000.
- 80. Stieger S, Götz FM, Reips, UD. Well-being during the UEFA European soccer championship. Unpublished raw data.
A memory management scheme for enhancing performance of applications on Android
Ieee account.
- Change Username/Password
- Update Address
Purchase Details
- Payment Options
- Order History
- View Purchased Documents
Profile Information
- Communications Preferences
- Profession and Education
- Technical Interests
- US & Canada: +1 800 678 4333
- Worldwide: +1 732 981 0060
- Contact & Support
- About IEEE Xplore
- Accessibility
- Terms of Use
- Nondiscrimination Policy
- Privacy & Opting Out of Cookies
A not-for-profit organization, IEEE is the world's largest technical professional organization dedicated to advancing technology for the benefit of humanity. © Copyright 2024 IEEE - All rights reserved. Use of this web site signifies your agreement to the terms and conditions.
Accessibility Links
- Skip to content
- Skip to search IOPscience
- Skip to Journals list
- Accessibility help
- Accessibility Help
Click here to close this panel.
Purpose-led Publishing is a coalition of three not-for-profit publishers in the field of physical sciences: AIP Publishing, the American Physical Society and IOP Publishing.
Together, as publishers that will always put purpose above profit, we have defined a set of industry standards that underpin high-quality, ethical scholarly communications.
We are proudly declaring that science is our only shareholder.
Design and Development of Android Application for Educational Institutes
Chukwuebuka Joseph Ejiyi 1 , Jianhua Deng 1 , Thomas Ugochukwu Ejiyi 2 , Adetunji A Salako 3 , Makuachukwu B Ejiyi 4 and Chinonso G Anomihe 5
Published under licence by IOP Publishing Ltd Journal of Physics: Conference Series , Volume 1769 , 5th International Conference on Computer Science and Information Engineering (ICCSIE 2020) 23-25 October 2020, Dalian, China Citation Chukwuebuka Joseph Ejiyi et al 2021 J. Phys.: Conf. Ser. 1769 012066 DOI 10.1088/1742-6596/1769/1/012066
Article metrics
1277 Total downloads
Share this article
Author e-mails.
Author affiliations
1 School of Information and Software Engineering, University of Electronic Science and Technology of China, Chengdu, PRC
2 Department of Pure and Industrial Chemistry, University of Nigeria Nsukka, Enugu State, Nigeria
3 School of Computer Science and Engineering, University of Electronic Science and Technology of China, Chengdu, PRC
4 Department of Agricultural Extensions, University of Nigeria Nsukka, Enugu State, Nigeria
5 Department of Biochemistry, Federal University of Technology Owerri, Imo State, Nigeria
Buy this article in print
The growth in the use android applications (Apps) has made it the most popular smart device operating system in use nowadays. Android has over 76% of the mobile operating system from December 2018–January 2020 which is quite significant. Android phones are also becoming the most used electronics globally. Students of higher institutions of learning are also becoming accustomed to the use of applications such that they want everything available for them on their mobile device if possible. This paper deals on an android application that will aid students in planning their timetable and scheduling their classes as well as having full knowledge of days according to the school calendar, get access to academic resources and information about the school right on their smart devices. From the application, one can have access to the detailed and accurate information of the school.
Export citation and abstract BibTeX RIS
Content from this work may be used under the terms of the Creative Commons Attribution 3.0 licence . Any further distribution of this work must maintain attribution to the author(s) and the title of the work, journal citation and DOI.
- Enabling Opportunity
Dig deeper with in‑depth resources, white papers, case studies, and surveys.
The android ecosystem, you say you want a mobile revolution….
Google CEO Sundar Pichai shares how Android is changing the ways people use mobile devices.
Android fuels innovation and choice
Discover how Android offers more choice and freedom to manufacturers, developers, and consumers.
Four myths about Android
ZDNet looks into common myths about Android and sets the record straight.
Economic impact
Google is a growth engine for europe.
Discover all the ways Google is helping dozens of European businesses grow online
Mobile tech drives the EU economy
See how the development of mobile devices and software contributes £73bn to the EU economy.
For the next five billion: Android One
The Android One initiative is helping people across the globe access affordable mobile devices.
Breaking the $100 barrier
See how Google teamed up with device maker Infinix to sell Android phones in Africa for less than $100.
Launching Android One in Africa
By partnering with regional players, Google has helped get low-cost phones to developing countries.
The growing mobile Internet economy
Explore the impact of mobile technology in the 13 countries that make up about 70 percent of global GDP.
Embracing innovation
How emerging markets fuel disruption.
Discover why markets like China, Vietnam and India are primed to disrupt the global mobile industry.
Keep Android harmonized
The president of the App Developers Alliance argues against further fragmentation of the Android ecosystem.
Let’s talk about Android
Discover the impact of Android’s open-source model on competition in the field of mobile operating systems.
Explore these carousel items. Use the previous and next buttons, as well as the keyboard arrows, to change the displayed item.
Help | Advanced Search
Computer Science > Computer Vision and Pattern Recognition
Title: mm1: methods, analysis & insights from multimodal llm pre-training.
Abstract: In this work, we discuss building performant Multimodal Large Language Models (MLLMs). In particular, we study the importance of various architecture components and data choices. Through careful and comprehensive ablations of the image encoder, the vision language connector, and various pre-training data choices, we identified several crucial design lessons. For example, we demonstrate that for large-scale multimodal pre-training using a careful mix of image-caption, interleaved image-text, and text-only data is crucial for achieving state-of-the-art (SOTA) few-shot results across multiple benchmarks, compared to other published pre-training results. Further, we show that the image encoder together with image resolution and the image token count has substantial impact, while the vision-language connector design is of comparatively negligible importance. By scaling up the presented recipe, we build MM1, a family of multimodal models up to 30B parameters, including both dense models and mixture-of-experts (MoE) variants, that are SOTA in pre-training metrics and achieve competitive performance after supervised fine-tuning on a range of established multimodal benchmarks. Thanks to large-scale pre-training, MM1 enjoys appealing properties such as enhanced in-context learning, and multi-image reasoning, enabling few-shot chain-of-thought prompting.
Submission history
Access paper:.
- Download PDF
- Other Formats
References & Citations
- Google Scholar
- Semantic Scholar
BibTeX formatted citation

Bibliographic and Citation Tools
Code, data and media associated with this article, recommenders and search tools.
- Institution
arXivLabs: experimental projects with community collaborators
arXivLabs is a framework that allows collaborators to develop and share new arXiv features directly on our website.
Both individuals and organizations that work with arXivLabs have embraced and accepted our values of openness, community, excellence, and user data privacy. arXiv is committed to these values and only works with partners that adhere to them.
Have an idea for a project that will add value for arXiv's community? Learn more about arXivLabs .
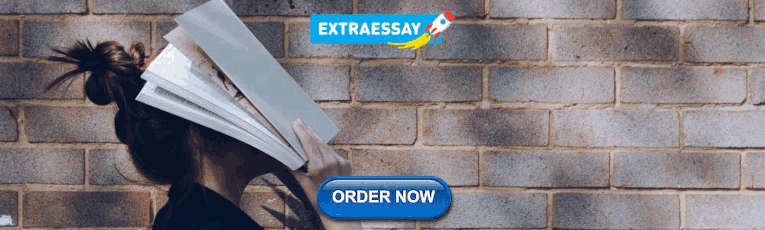
IMAGES
VIDEO
COMMENTS
Research-Paper- 1068648.html [5] ... This paper is about Android as open source operating system and why various smartphone manufactures considered it as their main operating system.
The Android operating unit is a mobile phone operating body built through Google primarily for touchscreen tools, smartphones, and tablet computers. It will undoubtedly begin with Android Architecture, which consists of five degrees: the Linux piece, Libraries, Application structure, Android runtime, and System apps.
Android is one of the mobile operating systems based on the Linux-Operating System, including a system, applications and middleware which developed by Google. Android provides platform for ...
Show abstract. ... e Android system has become one of the most popular mobile platforms for various devices. e Android system [5] is developed by Google which produces an average of two new ...
Android is a mobile operating system based on a modified version of the Linux kernel and other open source software, designed primarily for touchscreen mobile devices such as smartphones and tablets. It is an operating system for low powered devices that run on battery and are full of hardware like Global Positioning System (GPS) receivers, cameras, light and orientation sensors, Wi-Fi and LTE ...
This research paper focuses on some recent and most popular security vulnerabilities found in the android mobile operating system. The key contribution of this research includes the identification of mitigation techniques to overcome the challenges faced by its user The security of android devices can be significantly improved If mitigation ...
The wide and rapid adoption of Android-based devices in the last years has motivated the usage of Android apps to support a broad range of daily activities. In that sense, being the most popular mobile platform makes it an attractive target for security attacks. In fact, 1,489 security vulnerabilities have been reported in the last three years (2015-2017) for the Android OS (which is the ...
Although in the last few years significant research progress is seen in the field of the detection and mitigation of Android security, yet numerous challenges and gaps still exist. This paper presents a comprehensive and sound taxonomy to review the state-of-the-art approaches used in Android security.
In this paper, we perform an extensive quantitative study (Aug. 2015 to Dec. 2019) to measure the Android security updates and OS upgrades rollout process. Our study leverages multiple data sources: the Android Open Source Project (AOSP), device manufacturers, and the top four U.S. carriers (AT&T, Verizon, T-Mobile, and Sprint).
The Android platform has become one of the most popular operating system with millions of new users each year and the history including improvements involved in each release as well as the systems architecture and project structure is described. - Due to the technological development, mobile terminals have evolved into functionally sophisticated devices such as smartphones and tablets.
The paper concludes by laying down future research directions and scope of work, which can be leveraged not only by application developers, but also by researchers. This will help make Android safer for users and will further increase its demand as a mobile operating system .
With the advent of new mobile technologies, the mobile application industry is advancing rapidly. Consisting of several operating systems like symbian OS, iOS, blackberry, etc., Android OS is recognized as the most widely used, popular and user-friendly mobile platform. This open-source linux kernel-based operating system offers high flexibility due to its customization properties making it a ...
Despite the fact that millions of users each and every year choose mobile phones having android OS as a preference, an inclusive analysis of android OS is not yet present. In this research paper, a comprehensive analysis of this popular OS, its features, security tools etc. has been carried out and also discusses how this software application ...
Manishaben Jaiswal. 2018, International Journal of Creative Research Thoughts (IJCRT), ISSN:2320-2882, Volume.6, Issue 1, pp.514-525, January 2018. The Android operating unit is a mobile phone operating body built through Google primarily for touchscreen tools, smartphones, and tablet computers. It will undoubtedly begin with Android ...
Abstract. Prior to the invention of smartphones, mobile phones were simply meant for making calls. However, since then, they have developed into low-powered handheld computers. The operating system of mobile phones, which gave them intelligence and independent processing and storage, was the driving force behind this evolution.
The increasingly widespread use of mobile phone applications (apps) as research tools and cost-effective means of vast data collection raises new methodological challenges. In recent years, it has become a common practice for scientists to design apps that run only on a single operating system, thereby excluding large numbers of users who use a different operating system. However, empirical ...
Abstract. Android is a mobile operating system based on a modified version of the Linux kernel and other open source software, designed primarily for touchscreen mobile devices such as smartphones ...
Android OS is an unbridled success in the mobile market. Memory management has always been an area of concern to developers of large applications and also to consumers who want a seamless user experience. Memory leaks in applications have been talked about a lot in the past where subtle vulnerabilities often result in unwanted consequences such as application crashes. Memory is a limited ...
Abstract. The growth in the use android applications (Apps) has made it the most popular smart device operating system in use nowadays. Android has over 76% of the mobile operating system from December 2018-January 2020 which is quite significant. Android phones are also becoming the most used electronics globally.
China is currently the country with the largest number of Android smartphone users. We use a combination of static and dynamic code analysis techniques to study the data transmitted by the preinstalled system apps on Android smartphones from three of the most popular vendors in China. We find that an alarming number of preinstalled system, vendor and third-party apps are granted dangerous ...
Android is for everyone. Enabling Opportunity. Facts. Stories. Research. Dig deeper with in‑depth resources, white papers, case studies, and surveys. The Android ecosystem. MAY 2015. You say you want a mobile revolution….
Android Operating System: Architecture, Security Challenges and Solutions. March 2016; ... According to review, there is a research paper on security issues on android smart phones. Paper is
In this work, we discuss building performant Multimodal Large Language Models (MLLMs). In particular, we study the importance of various architecture components and data choices. Through careful and comprehensive ablations of the image encoder, the vision language connector, and various pre-training data choices, we identified several crucial design lessons. For example, we demonstrate that ...
Previous research has found that time-restricted eating improves several cardiometabolic health measures, such as blood pressure, blood glucose and cholesterol levels. "Restricting daily eating time to a short period, such as 8 hours per day, has gained popularity in recent years as a way to lose weight and improve heart health," said ...
Building Meta's GenAI Infrastructure. Marking a major investment in Meta's AI future, we are announcing two 24k GPU clusters. We are sharing details on the hardware, network, storage, design, performance, and software that help us extract high throughput and reliability for various AI workloads. We use this cluster design for Llama 3 training.
Android is one of the most popular operating systems for smartphones. Although Android OS includes many salient features which include its open-source nature, and its affinity to be customizable ...